Looping Suffix Tree-Based Inference of Partially Observable Hidden State
模糊匹配在树到串翻译模型中的应用
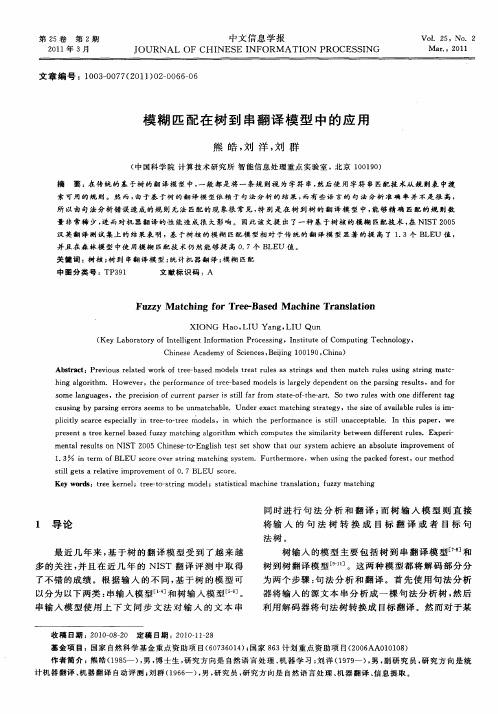
Chn s a e fS in e , ej g 1 0 9 Chn ) ie eAc d myo ce c s B in 0 1 0, ia i
A b ta t sr c :Pr v o ea e r r e ba e od l r a uls a t i s a h n m a c uls usn ti a c e i usr lt d wo k ofte — s d m e st e tr e s s rng nd t e t h r e i g s rng m t— hi g ih . H o e r he p ror a e o r e ba e od l s lr e y d p nd nto he p r i g r s ls,an or ng alort m w ve ,t e f m nc fte — s d m es i a g l e e e n t a sn e u t df s m el gu ge o an a s,t e iin fc r ntp r e s s i arfom a e oft e a t So t O r e t ne dif r nttg hepr cso o ur e a s r i tl f r l stt - - h - r . W uls wih o fe e a c usn r i r o ss e s t m a c b e U n re a tm a c n t a e a ig bypa sng e r r e m O beun t ha l . de x c thi g sr t gy,t e sz v ia l ulsi m — h ie ofa al b e r e si plcty s a c s cal n r e t —r e m o l i whih he iil c r e e pe ily i te -o t e des, n c t pe f r nc s s ilun c e a e. I hi p pe , we r o ma e i tl a c ptbl nt s a r pr s n r e ke ne a e u z a c i g a g ih e e ta te r lb s d f z y m t h n l ort m whih c p e he sm i iy b t e if e u e . Exp r— c om ut st i l t e w en dfer ntr ls ar e i
《2024年基于多尺度和注意力机制融合的语义分割模型研究》范文

《基于多尺度和注意力机制融合的语义分割模型研究》篇一一、引言随着深度学习技术的不断发展,语义分割作为计算机视觉领域的一个重要任务,逐渐成为研究的热点。
语义分割旨在将图像中的每个像素划分为不同的语义类别,为图像理解提供了更加细致的信息。
然而,由于实际场景中存在多尺度目标和复杂背景的干扰,语义分割任务仍面临诸多挑战。
为了解决这些问题,本文提出了一种基于多尺度和注意力机制融合的语义分割模型。
二、相关工作语义分割作为计算机视觉的一个关键任务,在近几年的研究中得到了广泛的关注。
目前主流的语义分割模型主要采用深度卷积神经网络(CNN)来实现。
这些模型通过捕获上下文信息、提高特征表达能力等手段提高分割精度。
然而,在处理多尺度目标和复杂背景时,这些模型仍存在局限性。
为了解决这些问题,本文提出了一种融合多尺度和注意力机制的语义分割模型。
三、模型与方法本文提出的模型主要由两个部分组成:多尺度特征提取和注意力机制融合。
(一)多尺度特征提取多尺度特征提取是提高语义分割性能的关键技术之一。
在本模型中,我们采用了不同尺度的卷积核和池化操作来提取图像的多尺度特征。
具体而言,我们设计了一个包含多种尺度卷积核的卷积层,以捕获不同尺度的目标信息。
此外,我们还采用了池化操作来获取更大尺度的上下文信息。
这些多尺度特征将被用于后续的注意力机制融合。
(二)注意力机制融合注意力机制是一种有效的提高模型性能的技术,可以使得模型更加关注重要的区域。
在本模型中,我们采用了自注意力机制和交叉注意力机制来提高模型的表达能力。
自注意力机制主要用于捕获每个像素的上下文信息,而交叉注意力机制则用于融合不同尺度特征之间的信息。
具体而言,我们通过在卷积层之间引入自注意力和交叉注意力模块,使得模型能够更好地关注重要区域和提取多尺度特征。
四、实验与结果为了验证本文提出的模型的性能,我们在公开的语义分割数据集上进行了一系列实验。
实验结果表明,本文提出的模型在处理多尺度目标和复杂背景时具有更好的性能。
基于多层特征嵌入的单目标跟踪算法

基于多层特征嵌入的单目标跟踪算法1. 内容描述基于多层特征嵌入的单目标跟踪算法是一种在计算机视觉领域中广泛应用的跟踪技术。
该算法的核心思想是通过多层特征嵌入来提取目标物体的特征表示,并利用这些特征表示进行目标跟踪。
该算法首先通过预处理步骤对输入图像进行降维和增强,然后将降维后的图像输入到神经网络中,得到不同层次的特征图。
通过对这些特征图进行池化操作,得到一个低维度的特征向量。
将这个特征向量输入到跟踪器中,以实现对目标物体的实时跟踪。
为了提高单目标跟踪算法的性能,本研究提出了一种基于多层特征嵌入的方法。
该方法首先引入了一个自适应的学习率策略,使得神经网络能够根据当前训练状态自动调整学习率。
通过引入注意力机制,使得神经网络能够更加关注重要的特征信息。
为了进一步提高跟踪器的鲁棒性,本研究还采用了一种多目标融合的方法,将多个跟踪器的结果进行加权融合,从而得到更加准确的目标位置估计。
通过实验验证,本研究提出的方法在多种数据集上均取得了显著的性能提升,证明了其在单目标跟踪领域的有效性和可行性。
1.1 研究背景随着计算机视觉和深度学习技术的快速发展,目标跟踪在许多领域(如安防、智能监控、自动驾驶等)中发挥着越来越重要的作用。
单目标跟踪(MOT)算法是一种广泛应用于视频分析领域的技术,它能够实时跟踪视频序列中的单个目标物体,并将其位置信息与相邻帧进行比较,以估计目标的运动轨迹。
传统的单目标跟踪算法在处理复杂场景、遮挡、运动模糊等问题时表现出较差的鲁棒性。
为了解决这些问题,研究者们提出了许多改进的单目标跟踪算法,如基于卡尔曼滤波的目标跟踪、基于扩展卡尔曼滤波的目标跟踪以及基于深度学习的目标跟踪等。
这些方法在一定程度上提高了单目标跟踪的性能,但仍然存在一些局限性,如对多目标跟踪的支持不足、对非平稳运动的适应性差等。
开发一种既能有效跟踪单个目标物体,又能应对多种挑战的单目标跟踪算法具有重要的理论和实际意义。
1.2 研究目的本研究旨在设计一种基于多层特征嵌入的单目标跟踪算法,以提高目标跟踪的准确性和鲁棒性。
改进的二叉树支持向量机在人脸识别中的应用
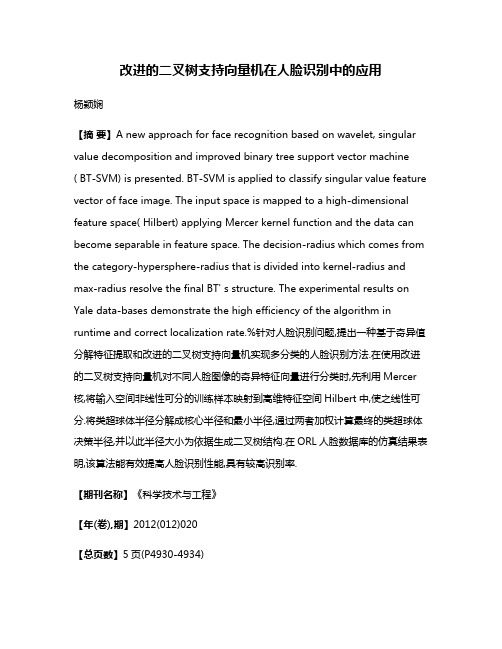
改进的二叉树支持向量机在人脸识别中的应用杨颖娴【摘要】A new approach for face recognition based on wavelet, singular value decomposition and improved binary tree support vector machine ( BT-SVM) is presented. BT-SVM is applied to classify singular value feature vector of face image. The input space is mapped to a high-dimensional feature space( Hilbert) applying Mercer kernel function and the data can become separable in feature space. The decision-radius which comes from the category-hypersphere-radius that is divided into kernel-radius and max-radius resolve the final BT' s structure. The experimental results on Yale data-bases demonstrate the high efficiency of the algorithm in runtime and correct localization rate.%针对人脸识别问题,提出一种基于奇异值分解特征提取和改进的二叉树支持向量机实现多分类的人脸识别方法.在使用改进的二叉树支持向量机对不同人脸图像的奇异特征向量进行分类时,先利用Mercer 核,将输入空间非线性可分的训练样本映射到高维特征空间Hilbert中,使之线性可分.将类超球体半径分解成核心半径和最小半径,通过两者加权计算最终的类超球体决策半径,并以此半径大小为依据生成二叉树结构.在ORL人脸数据库的仿真结果表明,该算法能有效提高人脸识别性能,具有较高识别率.【期刊名称】《科学技术与工程》【年(卷),期】2012(012)020【总页数】5页(P4930-4934)【关键词】小波变换;奇异值分解;二叉树支持向量机;人脸识别【作者】杨颖娴【作者单位】广东司法警官职业学院信息管理系,广州510520【正文语种】中文【中图分类】TP751.1在人脸识别领域,如何找到有效的识别特征和设计一个优秀的分类器是其中的两个关键问题。
改进的二叉树支持向量机在人脸识别中的应用
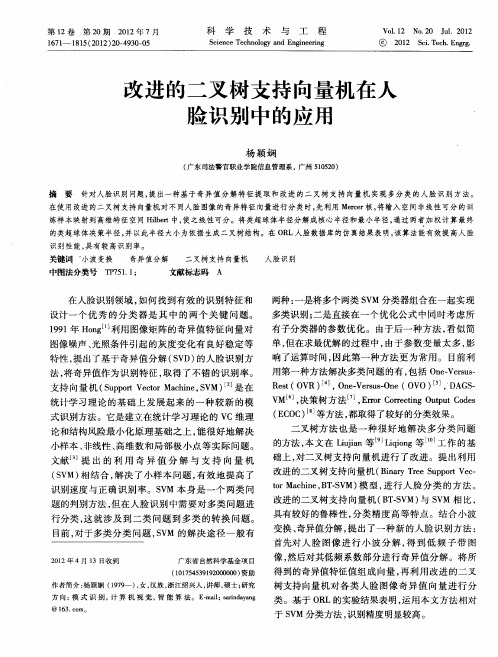
行分 类 , 这就 涉 及 到 二 类 问 题 到 多 类 的转 换 问题 。 目前 , 于多 类 分 类 问题 ,V 的 解 决 途 径 一 般 有 对 SM
21 0 2年 4月 1 3日收到 广东省 自然科学基金项 目 ( O 74 3 1 20 o 0 资助 115 5 99 o 0 o ) 作者简介 : 杨颖娴 (9 9 ) 女 , 17 一 , 汉族 , 浙江绍兴人 , 讲师 , 硕士 ; 研究 方 向 : 式 识 别 , 算 机 视 觉 , 能 算 法 。E m i aidyn 模 计 智 - al r aag :s n
得到的奇异值特征值组成 向量 , 再利用改进 的二叉
树 支持 向量 机 对 各 类 人 脸 图像 奇 异 值 向量 进 行 分
类 。基 于 O L的实验 结果表 明 , 用 本文 方法 相 对 R 运
支持 向量 机 ( u p r V c rMahn , V 是 在 S p ot et c ie S M) o
两种 : 一是将 多个 两类 S M 分类器 组合 在一 起 实现 V 多类 识别 ; 二是 直接 在 一个 优 化 公 式 中 同时 考 虑所 有 子分类 器 的参 数 优 化 。 由于 后一 种 方 法 , 似 简 看 单, 但在求 最优 解 的过 程 中 , 由于参 数 变 量 太 多 , 影 响 了运算 时 间 , 因此 第 一种 方 法 更 为 常用 。 目前 利 用 第一 种方 法 解决 多 类 问题 的有 , 包括 O eV r s n.es . u R s( V E , n . ess n O O) , A S et O R)4 O eV r — e( V D G — ] uO
@
2 1 SiTc . nr. 0 2 c eh E gg .
贝叶斯节点使用说明

贝叶斯节点使用说明作者:张青松目录1. 贝叶斯节点 (2)1.1. 朴素贝叶斯分类基本原理 (2)1.2. 贝叶斯节点 (2)2. 贝叶斯设置 (3)2.1. 建立贝叶斯节点的工作流 (3)2.1.1. 设置 (3)3. 贝叶斯分类结果 (4)1.贝叶斯节点贝叶斯节点使用了经典的朴实贝叶斯(NaiveBayes)算法对数据进行分类,其核心思想是贝叶斯公式:对于给出的待分类项,求解在此项出现的条件下各类别出现的概率,取概率最大的类别作为对该项的分类结果。
1.1.朴素贝叶斯分类基本原理朴素贝叶斯正式定义如下:1.设x={a1,a2,…,a m}为一个待分类项,而每个a为x的一个特征属性.2.有类别集合C={y1,y2,…y n,}。
3.计算P(y1|x),P(y2|x),…,P(y n|x)。
4.如果P(y k|x)=max{P(y1|x),P(y2|x),…,P(y n|x)},则x∈y k。
针对训练数据中,某个类别下的某个特征划分没有出现时,会令分类器的质量大大降低。
为了解决这个问题,引入了Laplace校准。
其思想就是对每类别下所有划分的计数加1,或者概率加上一个很小的值,这样如果训练样本集数据充分大时,并不会对结果产生影响,并且解决了概率为0的尴尬局面。
1.2.贝叶斯节点在DataStudio中,通过设置面板在输入的训练数据表中,选择某个标称量字段作为分类目标字段以及另一部分列作为参与分类的特征属性,训练朴素贝叶斯模型,然后使用该模型对位置的数据进行分类。
2.贝叶斯设置2.1.建立贝叶斯节点的工作流图1 贝叶斯节点工作流首先为贝叶斯节点连接输入数据。
输入数据中必须包含类型为标称量的字段。
以数据集为例。
2.1.1.设置图2 贝叶斯节点数据选择设置选择数据集中的标称量字段作为分类的目标列,并且在下方表格中选中要作为特征属性参与分类的列。
切换到模型页签,如图。
图3 贝叶斯算法参数设置注意:模型页签中的默认概率表示上文中提到的Laplace校准参数,最大分类个数不能小于分类目标列标称量的个数。
语义三元组提取-概述说明以及解释

语义三元组提取-概述说明以及解释1.引言1.1 概述概述:语义三元组提取是一种自然语言处理技术,旨在从文本中自动抽取出具有主谓宾结构的语义信息。
通过将句子中的实体与它们之间的关系抽取出来,形成三元组(subject-predicate-object)的形式,从而获得更加结构化和可理解的语义信息。
这项技术在信息检索、知识图谱构建、语义分析等领域具有广泛的应用前景。
概述部分将介绍语义三元组提取的基本概念、意义以及本文所要探讨的重点内容。
通过对语义三元组提取技术的介绍,读者可以更好地理解本文后续内容的研究意义和应用场景。
1.2 文章结构本文将分为三个主要部分,分别是引言、正文和结论。
在引言部分,将从概述、文章结构和目的三个方面介绍本文的主题内容。
首先,我们将简要介绍语义三元组提取的背景和意义,引出本文的研究对象。
接着,我们将介绍文章的整体结构,明确各个部分的内容安排和逻辑关系。
最后,我们将阐明本文的研究目的,明确本文要解决的问题和所带来的意义。
在正文部分,将主要分为三个小节。
首先,我们将介绍语义三元组的概念,包括其定义、特点和构成要素。
接着,我们将系统梳理语义三元组提取的方法,包括基于规则的方法、基于统计的方法和基于深度学习的方法等。
最后,我们将探讨语义三元组在实际应用中的场景,包括知识图谱构建、搜索引擎优化和自然语言处理等方面。
在结论部分,将对前文所述内容进行总结和展望。
首先,我们将概括本文的研究成果和亮点,指出语义三元组提取的重要性和必要性。
接着,我们将展望未来研究方向和发展趋势,探索语义三元组在智能技术领域的潜在应用价值。
最后,我们将用简洁的语言作出结束语,强调语义三元组提取对于推动智能化发展的意义和价值。
1.3 目的本文的目的是介绍语义三元组提取这一技术,并探讨其在自然语言处理、知识图谱构建、语义分析等领域的重要性和应用价值。
通过对语义三元组概念和提取方法的讨论,希望能够帮助读者更好地理解和应用这一技术,提高对文本语义信息的理解和利用能力。
基于深度学习的“外接球问题”微设计
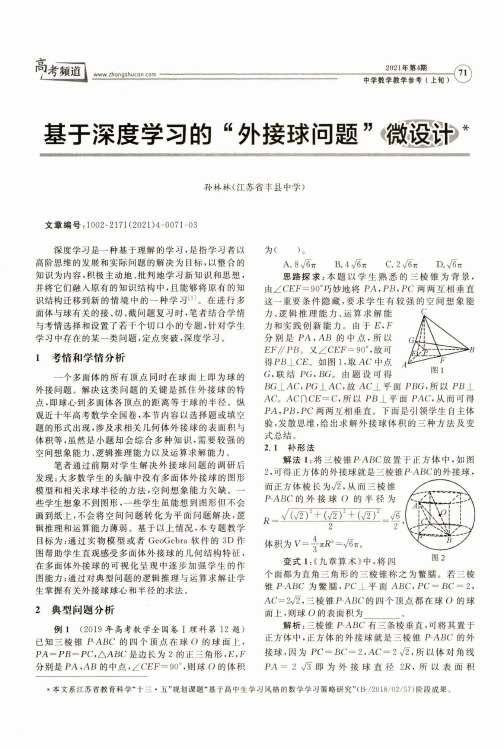
基于深度学习的“外接球问题”微设计〃@老M谙l2021年第4期t I --------------------------------------------------------------------------------------------------------------------------------------------------------------------------( 11 /1-丨mm --------------------------------------------------------------------------------------------------------------------------------------------- 中学数学教学参考(上旬>孙林林(江苏省丰县中学)文章编号:1002-2171 (2021)4-0071-03深度学习是一种基于理解的学习,是指学习者以高阶思维的发展和实际问题的解决为目标,以整合的 知识为内容,积极主动地、批判地学习新知识和思想, 并将它们融人原有的知识结构中,且能够将原有的知 识结构迁移到新的情境中的一种学习[1]。
在进行多 面体与球有关的接、切、截问题复习时,笔者结合学情 与考情选择和设置了若干个切口小的专题,针对学生 学习中存在的某一类问题,定点突破,深度学习。
1考情和学情分析一个多面体的所有顶点同时在球面上即为球的 外接问题。
解决这类问题的关键是抓住外接球的特 点,即球心到多面体各顶点的距离等于球的半径。
纵 观近十年高考数学全国卷,本节内容以选择题或填空 题的形式出现,涉及求相关几何体外接球的表面积与 体积等,虽然是小题却会综合多种知识,需要较强的 空间想象能力、逻辑推理能力以及运算求解能力。
笔者通过前期对学生解决外接球问题的调研后 发现:大多数学生的头脑中没有多面体外接球的图形 模型和相关求球半径的方法,空间想象能力欠缺。
ai常用的底层算子
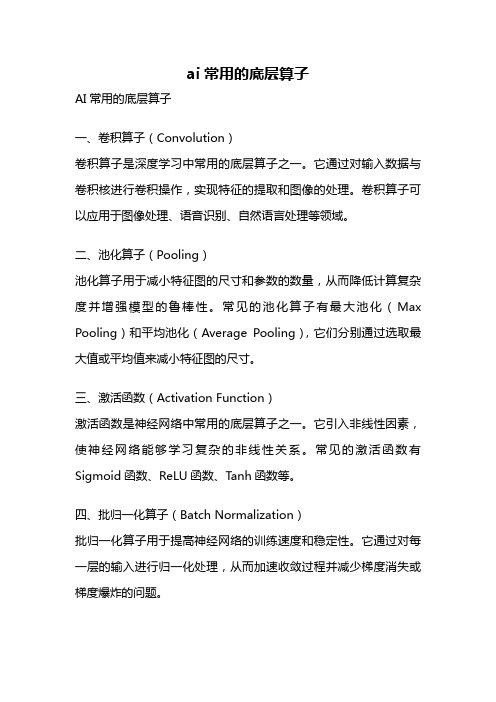
ai常用的底层算子AI常用的底层算子一、卷积算子(Convolution)卷积算子是深度学习中常用的底层算子之一。
它通过对输入数据与卷积核进行卷积操作,实现特征的提取和图像的处理。
卷积算子可以应用于图像处理、语音识别、自然语言处理等领域。
二、池化算子(Pooling)池化算子用于减小特征图的尺寸和参数的数量,从而降低计算复杂度并增强模型的鲁棒性。
常见的池化算子有最大池化(Max Pooling)和平均池化(Average Pooling),它们分别通过选取最大值或平均值来减小特征图的尺寸。
三、激活函数(Activation Function)激活函数是神经网络中常用的底层算子之一。
它引入非线性因素,使神经网络能够学习复杂的非线性关系。
常见的激活函数有Sigmoid函数、ReLU函数、Tanh函数等。
四、批归一化算子(Batch Normalization)批归一化算子用于提高神经网络的训练速度和稳定性。
它通过对每一层的输入进行归一化处理,从而加速收敛过程并减少梯度消失或梯度爆炸的问题。
五、全连接算子(Fully Connected)全连接算子是神经网络中常用的底层算子之一。
它将输入数据与权重矩阵进行矩阵相乘,并加上偏置项,得到最终的输出。
全连接算子可以实现特征的组合和高维向量的映射。
六、损失函数(Loss Function)损失函数是神经网络中用于度量模型预测与真实值之间差异的函数。
常见的损失函数有均方误差(MSE)、交叉熵损失函数等。
损失函数的选择会影响模型的训练效果和收敛速度。
七、优化算法(Optimization Algorithm)优化算法用于调整神经网络中的参数,使得损失函数达到最小值。
常见的优化算法有随机梯度下降(SGD)、Adam、Adagrad等。
优化算法的选择会影响模型的训练速度和性能。
八、循环神经网络(Recurrent Neural Network, RNN)循环神经网络是一种具有循环连接的神经网络结构,用于处理序列数据。
随机森林.ppt
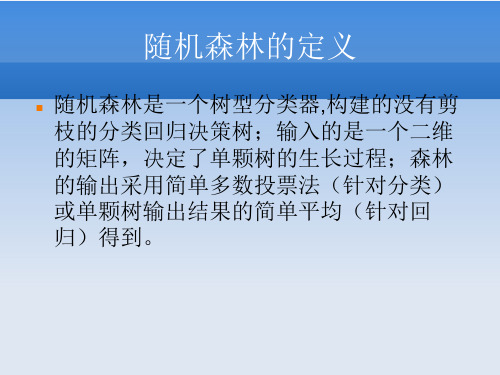
两个重要的代码
randomForest
主要参数是ntree,mtry
Predict
第一步,随机森林的安装
随机森林是基于R语言运行,安装过程分两步: 1.ubuntu系统下首先安装R语言包。
用一行代码。 sudo apt-get install R 然后ubuntu系统就自动的帮你安装完R。 2.安装random forest 意想不到的简单。 打开终端 ,输入R,就算进入到了R语言的编码界面。 在大于号后面入
决策树的定义
Hale Waihona Puke 决策树是这样的一颗树:每个内部节点上选用一个属性进行分割 每个分叉对应一个属性值 每个叶子结点代表一个分类
A1
a11 A2
a12 c1
a21
a22
c1
c2
a13
A3
a31
a32
c2
c1
决策树框架
决策树生成算法分成两个步骤
树的生成
开始,数据都在根节点 递归的进行数据分片
0,mtry=3,proximity=TRUE,importance=TRUE) print(iris.rf) iris.pred<-predict( iris.rf,iris[ind==2,] ) table(observed=iris[ind==2,"Species"],predicted=iris.pred )
随机森林的特点
两个随机性的引入,使得随机森林不容易陷入过 拟合
两个随机性的引入,使得随机森林具有很好的抗 噪声能力
对数据集的适应能力强:既能处理离散型数据, 也能处理连续型数据,数据集无需规范化。
基于深度残差网络的语音隐写分析方法

2021⁃03⁃10计算机应用,Journal of Computer Applications 2021,41(3):774-779ISSN 1001⁃9081CODEN JYIIDU http ://基于深度残差网络的语音隐写分析方法任奕茗,王让定*,严迪群,林昱臻(宁波大学信息科学与工程学院,浙江宁波315211)(∗通信作者电子邮箱wangrangding@ )摘要:针对目前以WAV 格式语音为载体的最低有效位(LSB )隐写方法的检测性能较低的问题,提出了一种基于深度残差网络的语音隐写分析方法。
首先,通过多组高通滤波器组成的固定卷积层来计算输入语音信号的残差信号,并利用截断线性激活单元对得到的残差信号进行截断操作;然后,通过卷积层与设计的残差块的堆叠来构建深度网络,以提取深层次的隐写特征数据;最后,利用全连接层与Softmax 层组成的分类器输出最终的分类结果。
实验结果表明,在Hide4PGP 和LSBmatching 两种隐写方法的不同密信嵌入率下,所提出模型的检测正确率都要优于现有的基于卷积神经网络(CNN )的隐写分析方法。
对于0.1bps 嵌入率的Hide4PGP 隐写方法,该隐写分析模型的检测正确率比LinNet 提高了近7个百分点。
关键词:音频;语音;最低有效位;隐写分析;深度残差网络;深度学习中图分类号:TP391文献标志码:ASpeech steganalysis method based on deep residual networkREN Yiming ,WANG Rangding *,YAN Diqun ,LIN Yuzhen(Faculty of Electrical Engineering and Computer Science ,Ningbo University ,Ningbo Zhejiang 315211,China )Abstract:Concerning the low detection performance of the Least Significant Bit (LSB )steganography method on WAV -format speech ,a speech steganalysis method based on deep residual network was proposed.First ,the residual signal of the input speech signal was calculated through a fixed convolutional layer composed of multiple sets of high -pass filters ,and a truncated linear unit was adopted to perform truncation to the obtained residual signal.Then ,a deep network was constructed by stacking the convolutional layer and the designed residual block to extract the deep feature information of steganography.Finally ,the final classification result was output by the classifier composed of the fully connected layer and Softmax layer.Experimental results under the different secret information embedding rates of two steganography methods ,Hide4PGP (Hide 4Pretty Good Privacy )and LSBmatching (Least Significant Bit matching ),show that compared with the exising Convolutional Neural Network (CNN )-based steganalysis methods ,the proposed method can achieve better performance ,and compared with LinNet ,the proposed method has the detection accuracy increased by 7percentage points on detecting Hide4PGP with the embedding rate of 0.1bps (bit per sample ).Key words:audio;speech;Least Significant Bit (LSB);steganalysis;deep residual network;deep learning引言随着互联网的快速发展,信息隐藏技术得到越来越多的关注。
基于邻接字符对的三元后缀树全文索引模型
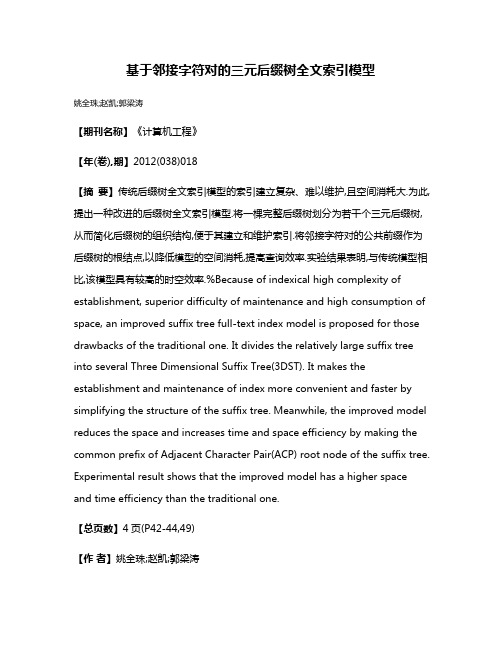
基于邻接字符对的三元后缀树全文索引模型姚全珠;赵凯;郭梁涛【期刊名称】《计算机工程》【年(卷),期】2012(038)018【摘要】传统后缀树全文索引模型的索引建立复杂、难以维护,且空间消耗大.为此,提出一种改进的后缀树全文索引模型.将一棵完整后缀树划分为若干个三元后缀树,从而简化后缀树的组织结构,便于其建立和维护索引.将邻接字符对的公共前缀作为后缀树的根结点,以降低模型的空间消耗,提高查询效率.实验结果表明,与传统模型相比,该模型具有较高的时空效率.%Because of indexical high complexity of establishment, superior difficulty of maintenance and high consumption of space, an improved suffix tree full-text index model is proposed for those drawbacks of the traditional one. It divides the relatively large suffix tree into several Three Dimensional Suffix Tree(3DST). It makes the establishment and maintenance of index more convenient and faster by simplifying the structure of the suffix tree. Meanwhile, the improved model reduces the space and increases time and space efficiency by making the common prefix of Adjacent Character Pair(ACP) root node of the suffix tree. Experimental result shows that the improved model has a higher space and time efficiency than the traditional one.【总页数】4页(P42-44,49)【作者】姚全珠;赵凯;郭梁涛【作者单位】西安理工大学计算机科学与工程学院,西安710048;西安理工大学计算机科学与工程学院,西安710048;西安理工大学计算机科学与工程学院,西安710048【正文语种】中文【中图分类】TP311【相关文献】1.基于单义域邻接图的工程图纸扫描图像的字符提取 [J], 张习文;欧宗瑛2.基于三维特征索引空间的分布式全文索引模型 [J], 谭舜泉;陈有青3.基于邻接矩阵的全文索引模型 [J], 周水庚;胡运发;关佶红4.基于邻接矩阵全文索引模型的文本压缩技术 [J], 陶晓鹏;胡运发5.离散数学中基于字符串连接的多路方案邻接矩阵运算 [J], 曾旭; 王晓华因版权原因,仅展示原文概要,查看原文内容请购买。
移动机器人闭环检测的视觉字典树金字塔TF-IDF得分匹配方法
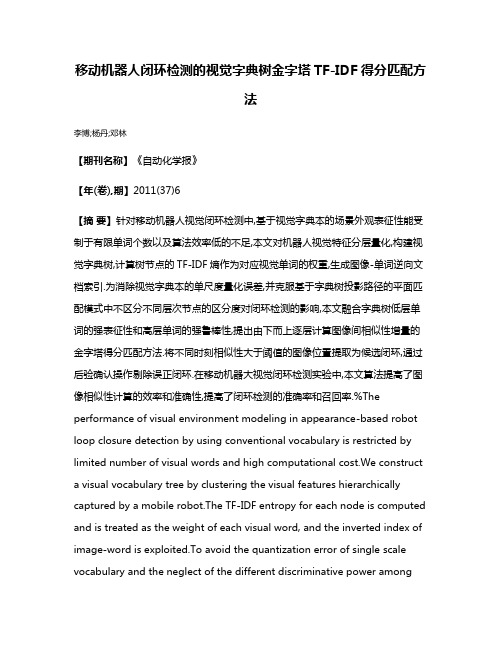
移动机器人闭环检测的视觉字典树金字塔TF-IDF得分匹配方法李博;杨丹;邓林【期刊名称】《自动化学报》【年(卷),期】2011(37)6【摘要】针对移动机器人视觉闭环检测中,基于视觉字典本的场景外观表征性能受制于有限单词个数以及算法效率低的不足,本文对机器人视觉特征分层量化,构建视觉字典树,计算树节点的TF-IDF熵作为对应视觉单词的权重,生成图像-单词逆向文档索引.为消除视觉字典本的单尺度量化误差,并克服基于字典树投影路径的平面匹配模式中不区分不同层次节点的区分度对闭环检测的影响,本文融合字典树低层单词的强表征性和高层单词的强鲁棒性,提出由下而上逐层计算图像间相似性增量的金字塔得分匹配方法.将不同时刻相似性大于阈值的图像位置提取为候选闭环,通过后验确认操作剔除误正闭环.在移动机器大视觉闭环检测实验中,本文算法提高了图像相似性计算的效率和准确性,提高了闭环检测的准确率和召回率.%The performance of visual environment modeling in appearance-based robot loop closure detection by using conventional vocabulary is restricted by limited number of visual words and high computational cost.We construct a visual vocabulary tree by clustering the visual features hierarchically captured by a mobile robot.The TF-IDF entropy for each node is computed and is treated as the weight of each visual word, and the inverted index of image-word is exploited.To avoid the quantization error of single scale vocabulary and the neglect of the different discriminative power amongdifferent level words of tree-based match, we take advantage of the robustness of high level words and the discriminability of Iow level words to present a pyramid scoring match scheme.The candidates of loop closures are detected by using a similarity threshold.A posteriori management helps discard outliers by verifying that the two images of the loop closure satisfy some hypothesis constraints.The experiments of loop closure detection in mobile robotics demonstrate that our scheme improves similarity calculation significantly in both accuracy and efficiency and obtains a higher precision-recall ratio with a faster speed of loop closure detection compared to the traditional methods.【总页数】9页(P665-673)【作者】李博;杨丹;邓林【作者单位】重庆大学计算机学院,重庆,400044;重庆大学软件工程学院,重庆,400044;重庆大学数学与统计学院,重庆,400044【正文语种】中文【相关文献】1.基于场景外观建模的移动机器人视觉闭环检测研究 [J], 陈超;徐嵚嵛2.一种基于图像匹配的闭环检测方法 [J], 赵逢达;孔令富3.基于视觉字典的移动机器人闭环检测方法研究 [J], 崔大成;曾连荪4.移动机器人视觉SLAM的闭环检测综述 [J], 柯莉红;王晓华5.一种基于TF-IDF和ORB的改进闭环检测算法研究 [J], 荣桂兰; 许钢; 邢广鑫因版权原因,仅展示原文概要,查看原文内容请购买。
基于回溯蚁群-粒子群混合算法的多点路径规划

通信学报
Journal on Communications
Vol.40 No.2 February 2019
基于回溯蚁群−粒子群混合算法的多点路径规划
刘丽珏,罗舒宁,高琰,陈美妃
(中南大学信息科学与工程学院, 湖南 长沙 410083)
摘 要:景区多点路径规划问题是一个 NP-hard 问题,相当于寻找经过起始点和特定节点的最短路径。针对多点 路径规划问题,提出了回溯蚁群−粒子群混合算法,该算法运用弗洛伊德(Floyd-Warshall)算法将图进行转换并 且结合了蚁群算法和粒子群算法寻找最短路径。实验结果表明,此算法可以在小规模数据下快速找到精确解,同 时,在较大规模数据量下,可以得到比最大最小蚁群算法和遗传算法更好的结果。 关键词:NP-hard 问题;最大最小蚁群系统;弗洛伊德算法;粒子群算法 中图分类号:TP399 文献标识码:A doi: 10.11959/j.issn.1000−436x.2019039
2019039-2
·104·
通信学报
第 40 卷
中 的 最 后 一 条 路 段 , 更 新 startpoint 、 node , 并 在 tabu _ node 中对当前不可行路段进行标记
17) end if
18) end while
19)
对局部最优路径
path
j lb
进行更新
20) 用改进 PSO 算法局部更新信息素τ
Abstract: The problem of multi-point path planning is a NP-hard problem, which is equivalent to finding the shortest path of a starting point and some specific node. Aiming at the problem of multi-point path planning, a retrospective ant colony-particle swarm optimization algorithm was proposed. This algorithm used Floyd-Warshall to transform the graph and combined ant colony algorithm and particle swarm algorithm to find the shortest path. The experimental results show that this algorithm can find the precise solution under small data, at the same time, under a large amount of data, can be better than the maximum minimum ant colony algorithm and genetic algorithm. Key words: NP-hard problem, max-min ant system, Floyd-Warshall, particle swarm algorithm
yolov3模型结构
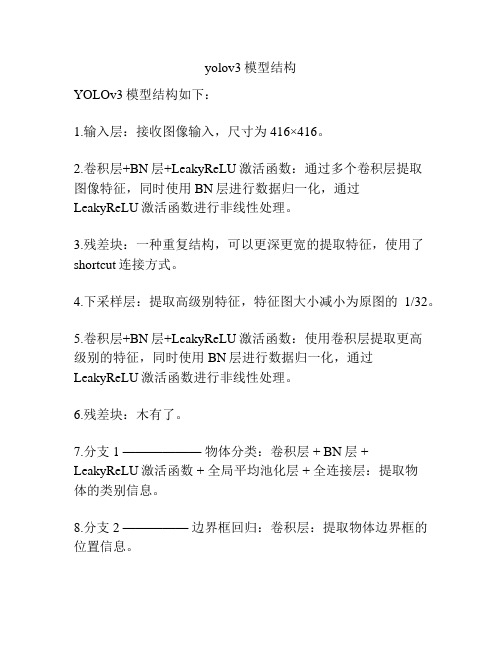
yolov3模型结构
YOLOv3模型结构如下:
1.输入层:接收图像输入,尺寸为416×416。
2.卷积层+BN层+LeakyReLU激活函数:通过多个卷积层提取
图像特征,同时使用BN层进行数据归一化,通过LeakyReLU激活函数进行非线性处理。
3.残差块:一种重复结构,可以更深更宽的提取特征,使用了shortcut连接方式。
4.下采样层:提取高级别特征,特征图大小减小为原图的1/32。
5.卷积层+BN层+LeakyReLU激活函数:使用卷积层提取更高
级别的特征,同时使用BN层进行数据归一化,通过LeakyReLU激活函数进行非线性处理。
6.残差块:木有了。
7.分支1 ——————物体分类:卷积层 + BN层 + LeakyReLU激活函数 + 全局平均池化层 + 全连接层:提取物
体的类别信息。
8.分支2 —————边界框回归:卷积层:提取物体边界框的位置信息。
9.卷积层+BN层+LeakyReLU激活函数:使用卷积层提取更高级别的特征,同时使用BN层进行数据归一化,通过LeakyReLU激活函数进行非线性处理。
10.上采样层:将特征图大小恢复为原图尺寸的1/16。
11.y2分支:参考分支7和8,进行分类和边界框回归。
12.上采样层:将特征图大小恢复为原图尺寸的1/8。
13.y1分支:参考分支7和8,进行分类和边界框回归。
14.输出层:输出检测结果,包括检测到的物体类别、位置和置信度等信息。
基于深度神经网络的树木伐桩轮廓提取及匹配方法

基于深度神经网络的树木伐桩轮廓提取及匹配方法崔世林;田斐【期刊名称】《林业工程学报》【年(卷),期】2024(9)3【摘要】为了及时准确地找到被盗树木,公安机关需要比对被盗伐树木伐桩的上下截面,寻找共同点,并依此确认两者是否属于同一树木。
但是由于存放环境不同,伐桩上下截面的颜色、纹理存在巨大差异,由于锯伐方式和树皮的影响,伐桩上下表面的轮廓也存在很大差异,伐桩下表面还很容易受木屑等影响,背景复杂。
针对这些难点,本研究在前面的研究基础上,继续把棋盘格作为特征物放置在伐桩表面,用PPYOLO_MobileNetV3卷积神经网络检测图像中的棋盘格,对棋盘格中的角点进行检测、排序,然后进行透视变换,恢复伐桩的原始面积和轮廓等特征,接着用PP-LiteSeg网络在复杂背景下提取伐桩轮廓,然后用CAE_ViT_base网络对轮廓进行匹配,实现了伐桩轮廓匹配的全流程,从而极大程度节约了人工。
理论分析和试验结果都表明,基于局部梯度的匹配法、基于局部点集拓扑特征的匹配法和基于轮廓的全局特征匹配方法等,在伐桩图像的匹配中都是不可行的。
CAE_ViT_base网络的解码器将输入图像分割为大小一致的图像块,解码器的训练过程需要关注每一个块的特征,伐桩轮廓的匹配难点在于轮廓有局部缺失,局部梯度误差较大。
CAE_ViT_base网络的自监督预训练机制很好地弥补了上述缺点;同时,采用对样本图像随机多角度旋转的方法,使得图像的特征提取能够保持旋转不变性。
CAE_ViT_base网络提取出来的特征在尺度上高于基于梯度的特征,也高于基于局部点集拓扑的特征,但低于全局特征。
因此,只要少部分图像块高度匹配,则CAE_ViT_base网络给出的最终匹配度就比较高;同时,这种工作方式和人工对比2个伐桩轮廓是否匹配的方法也是一致的。
在本研究的344幅伐桩图像上进行试验,结果表明:本研究算法对整个测试集的检测成功率为100%,棋盘格检测成功率100%,轮廓提取精度达到98.8%,轮廓匹配准确率100%,无一错检和误匹配;和基于梯度的轮廓提取方法及基于特征描述子的轮廓匹配方法相比,本研究方法具有全方位的优势。
自动提取词汇化树邻接文法
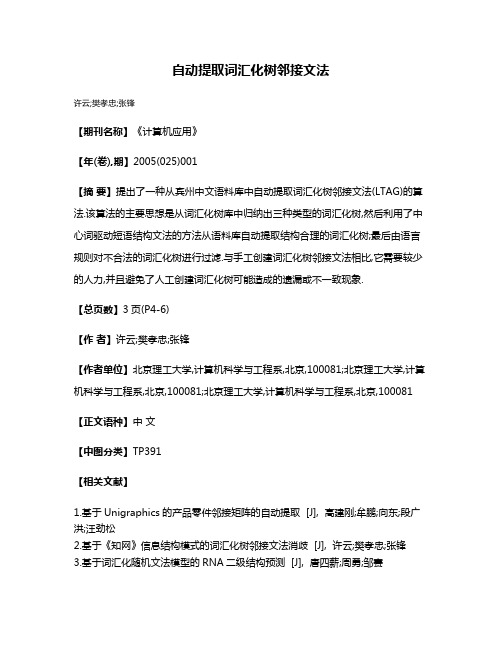
自动提取词汇化树邻接文法
许云;樊孝忠;张锋
【期刊名称】《计算机应用》
【年(卷),期】2005(025)001
【摘要】提出了一种从宾州中文语料库中自动提取词汇化树邻接文法(LTAG)的算法.该算法的主要思想是从词汇化树库中归纳出三种类型的词汇化树,然后利用了中心词驱动短语结构文法的方法从语料库自动提取结构合理的词汇化树;最后由语言规则对不合法的词汇化树进行过滤.与手工创建词汇化树邻接文法相比,它需要较少的人力,并且避免了人工创建词汇化树可能造成的遗漏或不一致现象.
【总页数】3页(P4-6)
【作者】许云;樊孝忠;张锋
【作者单位】北京理工大学,计算机科学与工程系,北京,100081;北京理工大学,计算机科学与工程系,北京,100081;北京理工大学,计算机科学与工程系,北京,100081【正文语种】中文
【中图分类】TP391
【相关文献】
1.基于Unigraphics的产品零件邻接矩阵的自动提取 [J], 高建刚;牟鹏;向东;段广洪;汪劲松
2.基于《知网》信息结构模式的词汇化树邻接文法消歧 [J], 许云;樊孝忠;张锋
3.基于词汇化随机文法模型的RNA二级结构预测 [J], 唐四薪;周勇;邹赛
4.LS文法绘制分形树的参数化设计 [J], 曾健;陈锦昌
5.基于互联网1至5元文法语料库的多词表达自动提取 [J], 李康熙;李为山
因版权原因,仅展示原文概要,查看原文内容请购买。
基于注意力平衡列表的溯因推理模型

基于注意力平衡列表的溯因推理模型徐铭;李林昊;齐巧玲;王利琴【期刊名称】《计算机应用》【年(卷),期】2023(43)2【摘要】溯因推理是自然语言推理(NLI)中的重要任务,旨在通过给定的起始观测事件和最终观测事件,推断出二者之间合理的过程事件(假设)。
早期的研究从每条训练样本中独立训练推理模型;而最近,主流的研究考虑了相似训练样本间的语义关联性,并以训练集中假设出现的频次拟合其合理程度,从而更精准地刻画假设在不同环境中的合理性。
在此基础上,在刻画假设的合理性的同时,加入了合理假设与不合理假设的差异性和相对性约束,从而达到了假设的合理性和不合理性的双向刻画目的,并通过多对多的训练方式实现了整体相对性建模;此外,考虑到事件表达过程中单词重要性的差异,构造了对样本不同单词的关注模块,最终形成了基于注意力平衡列表的溯因推理模型。
实验结果表明,与L2R2模型相比,所提模型在溯因推理主流数据集叙事文本中的溯因推理(ART)上的准确率和AUC分别提高了约0.46和1.36个百分点,证明了所提模型的有效性。
【总页数】7页(P349-355)【作者】徐铭;李林昊;齐巧玲;王利琴【作者单位】河北工业大学人工智能与数据科学学院;河北省大数据计算重点实验室(河北工业大学);河北省数据驱动工业智能工程研究中心(河北工业大学)【正文语种】中文【中图分类】TP18【相关文献】1.基于层级注意力模型的视频序列表情识别2.一种注意力增强的自然语言推理模型3.基于注意力机制和卷积神经网络的异步电动机三相电压不平衡损耗研究4.基于可逆金字塔和平衡注意力的工业裂缝分割5.基于增强序列表示注意力网络的中文隐式篇章关系识别因版权原因,仅展示原文概要,查看原文内容请购买。
convnext分类

convnext分类一、了解ConvNext分类的背景和意义随着深度学习技术的不断发展,自然语言处理(NLP)领域取得了显著的成果。
ConvNext分类作为深度学习的一种方法,逐渐引起了研究者的广泛关注。
它通过卷积神经网络(CNN)和循环神经网络(RNN)的结合,对文本进行特征提取和分类,具有较高的准确率和实用性。
二、ConvNext分类的基本原理和方法ConvNext分类的核心思想是通过卷积神经网络捕捉文本的局部特征,再通过循环神经网络对捕捉到的特征进行全局建模。
具体来说,它包括以下几个步骤:1.数据预处理:将文本转换为向量形式,如词嵌入或字符嵌入。
2.卷积层:利用卷积神经网络从输入向量中提取局部特征。
3.池化层:对卷积层得到的特征进行降维和压缩。
4.循环神经网络:将池化后的特征作为输入,进行全局序列建模。
5.全连接层:将循环神经网络的输出进行非线性变换,得到分类结果。
三、ConvNext分类在自然语言处理领域的应用ConvNext分类在自然语言处理领域具有广泛的应用,如情感分析、文本分类、命名实体识别等。
它相较于传统的机器学习方法,如朴素贝叶斯、支持向量机等,具有更高的准确率和较强的鲁棒性。
四、ConvNext分类的优缺点分析优点:1.能够有效地捕捉文本的局部特征,提高分类准确性。
2.适应性强,适用于多种自然语言处理任务。
3.参数共享,减少过拟合风险。
缺点:1.训练过程中,参数更新速度较快,可能导致梯度消失或梯度爆炸。
2.模型解释性较差,难以进行直观的解释。
五、我国在ConvNext分类领域的研究进展近年来,我国在ConvNext分类领域取得了丰硕的研究成果。
研究者们在基础理论和应用研究方面进行了深入探讨,如提出了基于注意力机制的ConvNext分类方法、多任务学习的ConvNext分类模型等。
同时,我国还在积极开展ConvNext分类在各领域的应用,如金融、医疗、教育等。
六、ConvNext分类的发展趋势和展望随着深度学习技术的不断进步,ConvNext分类在自然语言处理领域有望取得更大的突破。
- 1、下载文档前请自行甄别文档内容的完整性,平台不提供额外的编辑、内容补充、找答案等附加服务。
- 2、"仅部分预览"的文档,不可在线预览部分如存在完整性等问题,可反馈申请退款(可完整预览的文档不适用该条件!)。
- 3、如文档侵犯您的权益,请联系客服反馈,我们会尽快为您处理(人工客服工作时间:9:00-18:30)。
Looping Suffix Tree-Based Inference ofPartially Observable Hidden StateMichael P.Holmes mph@ Charles Lee Isbell,Jr.isbell@ College of Computing,Georgia Institute of Technology,Atlanta,GA30332-0280AbstractWe present a solution for inferring hiddenstate from sensorimotor experience when theenvironment takes the form of a POMDPwith deterministic transition and observationfunctions.Such environments can appear tobe arbitrarily complex and non-deterministicon the surface,but are actually determinis-tic with respect to the unobserved underly-ing state.We show that there always exists afinite history-based representation that fullycaptures the unobserved world state,allow-ing for perfect prediction of action effects.This representation takes the form of a loop-ing prediction suffix tree(PST).We derive asound and complete algorithm for learning alooping PST from a sufficient sample of sen-sorimotor experience.We also give empiri-cal illustrations of the advantages conferredby this approach,and characterize the ap-proximations to the looping PST that aremade by existing algorithms such as VariableLength Markov Models,Utile Suffix Memoryand Causal State Splitting Reconstruction.1.IntroductionPartial observability is a longstanding problem for learning techniques that seek to model their envi-ronment,as the large POMDP and HMM literatures attest.Diverse methods forfinding and using hid-den state have been formulated,with goals such as time-series prediction,learning Markovian state for reinforcement learning,and uncertainty reduction for classical-style planning(Shalizi&Shalizi,2004;Mc-Callum,1995;James&Singh,2005;Holmes&Isbell, Appearing in Proceedings of the23rd International Con-ference on Machine Learning,Pittsburgh,PA,2006.Copy-right2006by the author(s)/owner(s).Jr.,2005).No general solution has yet emerged.In this work we address the case of POMDPs in which the underlying transition and observation functions are deterministic.Such environments can appear to be arbitrarily complex and non-deterministic on the sur-face,but with knowledge of the underlying state their behavior becomes completely predictable and there-fore controllable.We give a novel characterization of the hidden state learning problem that highlights two fundamental issues:1)hidden state can be fully rep-resented by select pieces of history,and2)a history-based hidden state representation can be madefinite by excising uninformative regions of history.This characterization leads to a learning algorithm based on looping prediction suffix trees(PSTs).We show the algorithm to be sound and complete,and an-alyze several previous hidden state algorithms in terms of looping PSTs,including Variable Length Markov Models,Utile Suffix Memory,Causal State Splitting Reconstruction,and Predictive State Representations. We give empirical illustrations of the compromises made by some of these algorithms with respect to looping PSTs,finding them to be close but not ex-act approximations.Finally,we briefly discuss how the analysis presented here provides insight into the non-deterministic case.2.Problem Setup2.1.DefinitionAdapting from(Rivest&Schapire,1994),we define an environment in the class under consideration to be a tuple E=(S,A,O,δ,γ),where S is thefinite set of underlying world states,A is the set of actions that can be taken from any state,O is the set of possi-ble observations,δis a deterministic transition func-tionδ(S,A)→S,andγis a deterministic(Mealy) observation functionγ(S,A)→O generating an ob-servation for each action taken.We also assume theworld states to be strongly connected.1Environments under this definition form a subclass of POMDPs in which the state space isfinite and the transition and observation functions are deterministic.Despite their fundamental determinism,such environments can be highly complex and non-deterministic at the level of action and observation.Some additional notation we will employ:•γ(s i,a1...a n)denotes the observation sequence o1...o n generated by taking action sequence a1...a n from state s i.•A history h is a sequence of actions and observa-tions a1o1...a n o n,beginning with an action and ending with an observation.If p and h are two histories,writing them sequentially(ph)refers to their concatenation.•Sλ=S∪λ,whereλis a special null state.•h(Sλ):Sλ→Sλis a function mapping each state s i∈S to the state reached by history h starting in s i,or to the null stateλif h is impossible from s i;λis always mapped to itself.•trans(h)={ao:a∈A,o∈O,and ao is a possible transition following h}.Our goal is to build a sound and complete predictive model of the environment given only a history of senso-rimotor experience.By sound and complete,we mean that the model correctly predicts the observation that will follow any action,given the history preceding the action.Wefirst present a theoretical analysis showing that every environment has afinite history suffix tree-based representation that allows for perfect prediction. We then derive an algorithm for learning such a repre-sentation from sensorimotor experience,characterize various existing hidden state algorithms in terms of our solution,and give empirical performance compar-isons that show the approximations being made to the complete solution.2.2.Prediction Suffix TreesThe only source of information about hidden state is the learner’s history of interaction with the environ-ment.Yet not all history is informative at all times: the fact that you drank a glass of water ten years ago probably has no bearing on whether your car will start today.We would like to track some minimal,finite 1If not,the learner eventually falls into a strongly con-nected component that then becomes the whole accessible environment(Rivest&Schapire,1994).Figure1.Theflip automaton in Mealy form.l0r1l1r0l1r0l0r1Figure2.A looping prediction suffix tree for theflip au-tomaton.Bars under leaf nodes indicate expansion termi-nation,with each node’s transitions listed beneath the bar. Note that the u0node loops back to the root.amount of history sufficient to predict the future.In short,we need a sufficient statistic,as discussed in (Shalizi&Shalizi,2004).As we have defined the prob-lem,this means using history to infer the current state. Figure1contains a simple two-state environment illus-trating the fact that each state can only be reached by particular sensorimotor histories.For example,l1en-ters the left state but not the right state,so after seeing l1we are definitely in the left state.Based on this idea, we seek to represent the world state using observation-terminated history suffixes,i.e.,the end portion of the action/observation history a1o1...a n o n that led to the current observation.In general this will require more than just the most recent action/observation step,and each additional step can helpfilter out possible end states.For instance,in Figure1,u0leads to both the left-hand and right-hand states,while r1u0leads only to the right-hand state.As shown in Figure2,it is natural to represent the set of history suffixes as a tree(Ron et al.,1994).In a suffix tree,any sufficiently long history can be sorted down to a leaf node by following the branches labeled by its suffix in reverse order.With the addition of transition predictions at the leaves,the tree becomes a prediction suffix tree(PST).If each leaf node suffix corresponds to a unique state,its transition predic-tions will be deterministic.Our goal is to learn a PST that maps any history to a leaf that accurately predicts its future transitions.3.Finite Representability of HiddenStateIn learning a predictive model we can assume without loss of generality that the environment is in a mini-mized form,with minimality defined as follows:Definition.An environment E is minimized iff,for any two states s i and s j,there exists a distinguish-ing sequence of actions d ij=a1...a n such that γ(s i,d ij)=γ(s j,d ij).The distinguishing sequence d ij provides a test that can be executed to rule out one or both of s i and s j as possible start states.It does not,however,uniquely determine the start or end states.We will use the term resolving history to refer to a sequence of alternating actions and observations that uniquely determines the end state:Definition.A history sequence h resolves to state s i iffh(Sλ)maps every state in Sλeither toλor to s i, with at least one state mapping to s i.Thus,immediately following a history h that resolves to s i,the environment must be in state s i.The en-vironment is therefore Markovian relative to any re-solving history.In general,an arbitrary history h may map some states toλ,and any prefix p may also map some states toλ.Prepending the prefix gives the over-all functional mapping h(p(Sλ)).Because all histories mapλtoλ,anything mapped toλby p must also be mapped toλby ph.This may include states that h alone does not map toλ.Thus,backward extension of a history acts as afiltering mechanism that can never decrease the number ofλmappings,but can certainly increase them.The process of resolving is essentially the process of increasing the number ofλmappings until the only remaining non-λmappings all lead to the same state.A resolving sequence is distinct from a homing se-quence,a term used in the literature to refer to a se-quence composed purely of actions such that,when executed,the resultant observation sequence uniquely identifies the end state.A homing sequence represents a set of resolving sequences,one for each possible ob-servation sequence generated by its actions.It is known that everyfinite,deterministic environ-ment has at least one homing sequence(Shen,1993b). This,in turn,implies the existence of at least one re-solving sequence.Given a sequence h that resolves to s i,we can construct a new resolving sequence by adding any action/observation step ao that s i can gen-erate.Because the environment is deterministic,the new sequence hao must resolve to s j=δ(s i,a).By construction,there must exist infinitely many resolv-ing sequences that can be obtained through such ex-tensions.Further,because the environment is strongly connected,there exist infinitely many resolving se-quences that end in any particular state s i.If all resolving sequences for each state were known, they could be matched against sensorimotor history to infer the current state at any time;however,some of these sequences are longer than necessary.For ex-ample,a resolving sequence h could have an arbitrary (but possible)sequence p prepended to it,and the re-sultant sequence ph would still resolve to the same state.Clearly we would prefer resolving sequences that are as short as possible.This leads us to define the set of minimal resolving sequences:Definition.The set of minimal resolving sequences for a state s i is the set of resolving sequences h such that no sequence h formed by removing steps from the left side of h is also resolving.Thus,in the ph case,because removing p to form h still gives a resolving sequence,ph is not minimal re-solving.Note,however,that the minimal resolving se-quences can have unbounded length.This is because, while some portions of a resolving sequence are in-formative in that they increase the number ofλmap-pings,other portions may not be informative at all,i.e. they merely lengthen the history without increasing theλmappings.A minimal resolving history can po-tentially repeat uninformative subsequences arbitrar-ily many times beforefinally adding the informative portion that terminates the expansion.We will refer to uninformative sequences as excisable,and to infor-mative sequences as non-excisable.Definition.For any two histories h and q such that h=eq,the sequence e is excisable from h iff∀p trans(ph)=trans(pq).Otherwise e is non-excisable.This definition gives us an observational criterion for deciding the excisability of a history subsequence.We now give a sufficient condition for excisability in terms of equivalent histories:Definition.For two histories h and q such that h= eq,we say that h and q are functionally equivalent if h(Sλ)=q(Sλ),i.e.the mappings induced by the two histories are identical.Lemma1.For two histories h and q such that h=eq, if h and q are functionally equivalent then e is excisable from h.Proof.If h and q are functionally equivalent,we have, for any prefix history p and any state s,ph(s)=h(p(s))=q(p(s))=pq(s).Therefore ph and pq are also functionally equivalent,and,because they each leave the same set of possible(non-λ)end states,we have trans(ph)=trans(pq).Because this holds for all p,we have satisfied the definition of excisability and conclude that e is excisable from h.Excisability is useful because it facilitates afinite rep-resentation of the minimal resolving sequences.Con-sider the tree of all possible history suffixes.If we have a sequence h=eq and e is excisable from h,then for all predictive and state-resolving purposes we can treat instances of h as though they were actually q.Thus, if in sorting our history down the suffix tree we reach node h,we might just as well loop back up to q as continue sorting down from h—all transition predic-tions will remain the same(see Figure2).This is the basis for looping predictive suffix trees.Whereas a suf-fix tree representing the minimally resolving sequences could be infinite in depth,we now show that a looping suffix tree that loops back on excisable subsequences is alwaysfinite and retains full predictive power. Lemma2.Every branch of a history suffix tree either becomes resolving or reaches a level that begins with an excisable sequence afterfinite depth.Proof.Some branches will resolve atfinite depth be-cause resolving sequences exist,as previously noted. Consider,then,the remaining branches,which must remain unresolving to infinite depth.For a given branch representing the infinite history h,let h i be the history represented by the suffix starting at the root and extending down to level i.Each h i repre-sents a particular function mapping Sλonto Sλ.But there are onlyfinitely many such functions,namely |Sλ|2=|S|2+2|S|+1.Therefore,after no more than |S|2+2|S|+1levels,there will be a functional repeat such that h j is functionally equivalent to h k,k>j, k,j<|S|2+2|S|+1.Because h k=eh j,we can invoke Lemma1to conclude that e is excisable from h k and therefore h becomes excisable afterfinite depth k. Theorem1.Anyfinite,deterministic POMDP can be perfectly predicted by afinite looping PST,given a resolving history to start with.Proof.First,it is clear that without a resolving history perfect prediction cannot be made,as the current state will not have been resolved.So the best we can hope for is to predict correctly given a resolving history.We therefore need to show that there is afinite,looping PST that correctly captures all predictions of resolving histories.By Lemma2,we know that each branch of the full suffix tree either resolves or reaches a level where it begins with an excisable sequence afterfinite depth.If a branch h resolves atfinite depth,then we cut it offthere,forming a leaf node in which we store trans(h) as the predictions,which will be deterministic and cor-rect.For a branch that does not resolve atfinite depth,let h k be thefirst suffix along the branch that begins with an excisable sequence e such that eh j=h k.We know that h k will be atfinite depth,and we place a loop from h k to h j.No expansion of the tree below h k takes place.A history being sorted down through h k will loop back to h j and continue to be sorted down as though it had reached h j rather than h k.Because,by definition of excisability,∀p trans(ph k)=trans(ph j), any resolving ph k will be sorted down to ph j,which will have the same deterministic transitions and there-fore make the correct predictions.Thus,if we loop on all excisable sequences we will make their branchesfi-nite and still make all predictions correctly.We have shown by construction the existence of a loop-ing PST that gives correct predictions for any resolving history and whose branches all terminate afterfinite depth,and this establishes the theorem.Looping provides Kleene star-like representational power:if h=eq loops to q,q’s node no longer rep-resents the suffix q alone,but instead represents the infinite set e∗q.Arbitrarily long repetitions of unin-formative sequences are excised.This solves a classic problem with history-based approaches wherein states with self loops canfill up the history with so many uninformative action/observation steps that anyfinite window of history can be rendered useless.Not only does PST looping allow the infinite set of resolving histories to be mapped onto afinite representation,it also gives the power to generalize:a tree with loops gains the ability to correctly predict the transitions of suffixes it has never seen.4.Algorithmic ResultsThe preceding analysis suggests an algorithm for learn-ing a PST through interaction with the environment: just follow the construction of the looping PST as given in the proof,expanding branches until they re-solve or become loopable.Butfirst we must establish the ability to discern the branch termination and loop-ing criteria from data.We accomplish this by way of a definition and two lemmas.Definition.We say that a history is sufficient if every action has been taken often enough and in a sufficientnumber of contexts so as to reveal the full transition set trans(h)for every node h considered by a looping PST building algorithm.Correct decisions could certainly be made by an algo-rithm having access to an oracle telling it what tran-sitions are possible from each node.A fully repre-sentative history is equivalent to such an oracle,and need only befinitely long for afinite number of node expansions.Such a history is,in reality,exponentially unlikely if actions are chosen randomly(hence the NP-completeness result in the case of passive learning(Fre-und et al.,1993)).Strategic exploration can produce a sufficient history more efficiently,but a discussion of exploration strategies is beyond the scope of this paper.Lemma3.Given a sufficient history,correct predic-tion on any non-looping branch h is obtained by ter-minating at thefirst level h i such that trans(h i)is deterministic for each action.Proof.If trans(h i)is deterministic for each action, which only a sufficient history can reveal for certain, then h i must map Sλonto a subset R hiof states that all have identical single-step action/observation transitions.If we extend h i by p to get the resolv-ing sequence h=ph i,the h i part of h performs the same mapping but on the subset R p⊆Sλleft overafter p.Thus,R phi ⊆R hi,and therefore trans(h)=trans(h i),so the correct predictions can be made at h i without expanding all the way to h.Because we cannot see the underlying state to tell when a branch has resolved,Lemma3is crucial as an observable termination criterion.Transitions are tabulated from data,so as long as we have a sufficient sample of experience to tell us the full transition set of any history we encounter in our tree expansion,we can apply Lemma3to decide whether to terminate. Without a sufficient history,this criterion may lead to early termination,but it will at least be correct with respect to past experience and in the sufficient history limit will lead to proper convergence.Lemma4.If,given a sufficient history,there is no prefix p for which trans(ph)=trans(pq)(h=eq), then e is excisable from h and h can therefore be looped to q.Proof.According to the definition of excisability,e is excisable from h if and only if∀p trans(ph)= trans(pq).If e is not excisable from h,we there-fore have∃p trans(ph)=trans(pq).Since we have a sufficient history,if there were such a p it would appear often enough in the history to reveal that trans(ph)=trans(pq).But since we are given that no such inequality exists,we can conclude that trans(ph) and trans(pq)are equal,and therefore e is excisable from h and h can be safely looped to q.The utility of Lemma4is that it allows us to assume we should place a loop unless somefinite prefix con-tradicts it.With these lemmas in place,we now give a looping PST learning algorithm and show that it is sound and complete when given a sufficient history. Algorithm1Algorithm for learning a looping PST. LLT(h)create root node r representing the empty suffix add r to an expansion queuewhile nodes remain in queue doremove thefirst node xfind all transitions following instances of x in hif x’s transition set is deterministic thencontinueelse if isLoopable(x)thencreate loop from x to the highest to highest pos-sible ancestorelsefor each one-step extension of x in h docreate child node and add to queueend forend ifend whilereturn rAlgorithm2Subroutine for determining loopability. isLoopable(x)for each ancestor q of x,starting at the root dofor all prefixes p such that px or pq occurs in h doif pq or px does not appear in h ORtrans(px)=trans(pq)thenreturn Falseend ifend forend forreturn TrueTheorem 2.When given a sufficient history,LLT returns afinite looping PST that correctly predicts the transitions following any resolving history.Proof.LLT follows the constructive proof of Theo-rem1,but with empirical observable criteria for plac-ing loops and terminating expansion.By Lemma3,terminating upon reaching transition determinism is correct when based on a sufficient history.By Lemma4,allowing loops except when some prefix shows transition disagreement between the looping and looped-to nodes is also correct if the history is sufficient.LLT with a sufficient history therefore con-structs a looping PST that will correctly predict the transitions of any resolving history.4.1.Practical ConsiderationsIn practice,it can be difficult to obtain a sufficient history.This is especially problematic for loop cre-ation.The looping criterion requires that for h to loop to q(h=eq)there be no prefix p for which trans(ph)=trans(pq).If the history is not sufficient, trans(ph)and trans(pq)can be appear unequal due to the fact that not all actions have been taken from ph or pq.As p becomes longer,the probability of missing transitions increases exponentially,with a net result of many valid loops being rejected,yielding overly large trees and poor generalization.We thus substitute Lemma4with an alternative cri-terion based on prediction accuracy:a loop is allowed in the tree if it does not decrease prediction accuracy over the training data.This frees us from the require-ment of seeing every supporting transition before we can loop.In the limit of a sufficient history,a false loop would decrease prediction accuracy,so the accuracy-based criterion becomes equivalent to Lemma4.This modification is handled with an alternate version of the isLoopable subroutine,which we give as Algo-rithm3.Algorithm3Alternate subroutine for determining loopability.isLoopable2(x)for each ancestor q of x,starting at the root dosimulate loop from x to qevaluate prediction accuracy over past historyif accuracy does not decrease thenreturn Trueend ifend forreturn False5.Analysis of Related Approaches Looping PSTs provide a framework in which to un-derstand and compare existing approaches to partial observability.In this section we analyze prior work that deals with hidden state.Even though most of these algorithms were designed for non-deterministic environments,our analysis of the way they handle en-vironments with underlying determinism clarifies some of the fundamental choices made by each.As we have seen,the states of a deterministicfinite environment can be inferred by selectively trackingfi-nite pieces of history.The main issue in any algorithm dealing with partial observability is how to map the infinite set of possible histories to somefinite repre-sentation,and this is the focus of our analysis.5.1.CSSR,VLMMs,and USMCausal State Splitting Reconstruction(CSSR;Shalizi &Shalizi,2004)constructs time-series predictors by exhaustively expanding a history suffix tree to a user-specified maximum depth L max.It includes a method for aggregating the expanded suffixes that belong to the same“causal state,”but this does not increase its predictive power beyond that provided by thefixed-depth exhaustive suffix tree expansion.Excisable his-tory is not explicitly handled,butfixing the depth of the tree can be seen as an approximation that prevents unbounded expansion down excisable branches.Note that,because the expansion is exhaustive,even mini-mal resolving suffixes will be expanded if their length is less than L max.Variable Length Markov Models(VLMMs)also ex-pand history suffix trees in the hope of producing a Markovian representation of state.Unlike CSSR, VLMM trees are variable in depth and employ an em-pirical stopping criterion.The criterion of(Ron et al., 1994)is representative:a child suffix node is created only if1)the probability of its suffix occurring is above some threshold,and2)the transition behavior of the child differs significantly(by a statistical test such as KL divergence)from that of the parent.This criterion, unlike that of CSSR,will stop at a minimal resolving suffix,giving rise to a variable-depth tree.Again,how-ever,excisable sequences are not explicitly dealt with, and the expansion stopping criterion can be seen as an alternative approximation to prevent unbounded expansion of excisables.The Utile Suffix Memory(USM)algorithm(McCal-lum,1995)is very similar to VLMM learning,but with a reward-based stopping criterion that expands a child node only if the distribution of next-step re-ward appears to differ statistically significantly from that of its parent.This is yet another approximation to prevent unbounded expansion of the tree instead of directly handling excisable sequences.UTree(Mc-Callum,1995)is an extension to USM that allows the observations to be broken up into individual sensors and added to the suffix tree one at a time.Predictive State Representations(PSRs;Littman et al.,2002)represent state as afinite set of prediction probabilities over multi-step future action/observation sequences called tests.The tests remainfixed while their probabilities are updated at each timestep.Let P i be the set of probabilities at timestep i;the update is of the functional form P i+1=f(P i,a i o i),where a i o i is the action/observation pair seen at timestep i.Re-cursively expanding this expression shows that P i is actually a function of the entire history,i.e.,histories of arbitrary length are mapped onto afinite represen-tation as in looping PSTs.The intent is for P i to con-tain predictions for enough tests so as to form a suffi-cient statistic(which,in the deterministic case,means P i resolves the current state).One way to achieve sufficiency is for P i to contain predictions for all one-step tests.This means that,for deterministic environ-ments,looping PSTs are PSRs,because looping PST leaf nodes contain all one-step predictions.In other words,LLT constitutes a PSR discovery algorithm for deterministic environments.This is significant because PSR discovery in general remains an open problem. Other algorithms of note include Shen’s D*(Shen, 1993b;Shen,1993a)and the diversity-based au-tomaton inference of Rivest and Schapire(Rivest&theflip environment.(bottom)Results for the TCP au-tomaton.The algorithms were run from scratch after every 50timesteps,and their output was used to predict the next 50timesteps.Each data point represents the average pre-diction error for50timesteps over10runs with actions chosen at random.Schapire,1994);unfortunately,space does not permit analysis of these here.5.3.Empirical ComparisonAs an illustration of the difference made by using loop-ing PSTs,we present a comparison between the suf-fix tree expansion performance of the LLT,CSSR, and VLMM criteria.Note that these are simple ex-periments in synthetic environments intended only to highlight the tradeoffs involved.We ran LLT with the looping criterion described in section4.1,then ran a non-looping suffix tree expander with afixed depth criterion(CSSR)and a probability-of-occurrence cri-terion(VLMM).The CSSR and VLMM criteria were run against LLT on theflip automaton and on a10-state,3-observation automaton based on the server-side portion of the TCP network protocol.Results are shown in Figure3.。