Text Processing for Text-to-SpeechSystems in Indian Languages
声源定位技术文献综述和英文参考文献
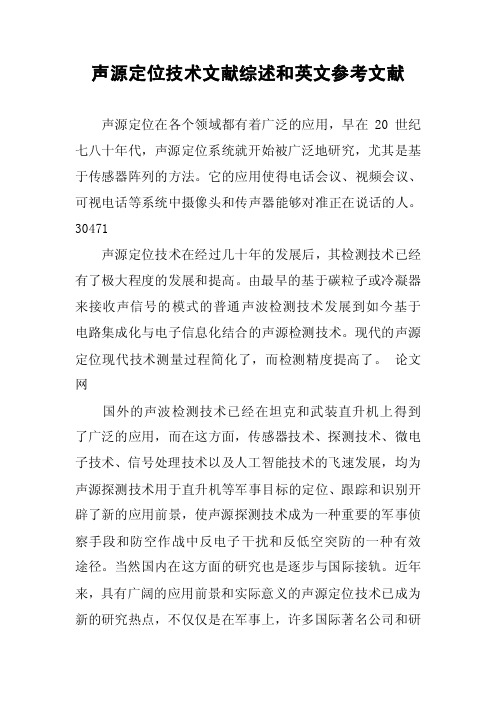
声源定位技术文献综述和英文参考文献声源定位在各个领域都有着广泛的应用,早在20世纪七八十年代,声源定位系统就开始被广泛地研究,尤其是基于传感器阵列的方法。
它的应用使得电话会议、视频会议、可视电话等系统中摄像头和传声器能够对准正在说话的人。
30471声源定位技术在经过几十年的发展后,其检测技术已经有了极大程度的发展和提高。
由最早的基于碳粒子或冷凝器来接收声信号的模式的普通声波检测技术发展到如今基于电路集成化与电子信息化结合的声源检测技术。
现代的声源定位现代技术测量过程简化了,而检测精度提高了。
论文网国外的声波检测技术已经在坦克和武装直升机上得到了广泛的应用,而在这方面,传感器技术、探测技术、微电子技术、信号处理技术以及人工智能技术的飞速发展,均为声源探测技术用于直升机等军事目标的定位、跟踪和识别开辟了新的应用前景,使声源探测技术成为一种重要的军事侦察手段和防空作战中反电子干扰和反低空突防的一种有效途径。
当然国内在这方面的研究也是逐步与国际接轨。
近年来,具有广阔的应用前景和实际意义的声源定位技术已成为新的研究热点,不仅仅是在军事上,许多国际著名公司和研究机构已经在声源定位技术研究与应用上开始了新的角力,许多产品已进入实际应用阶段。
并且已经显示出巨大的优势和市场潜力。
参考文献[1] Oyilmaz,S.Rickard. Blind Separation of Speech Mixtures via Time-Frequency Masking[J]. IEEE Transactions on Signal Processing, XX, 52(7):1830-1847.源自[2] H. Sawada, S. Araki, R. Mukai, S. Makino. Blind extraction of dominant target sources using ICA and time-frequency masking[J]. IEEE Transactions on Audio, Speech, and Language Processing , XX, 14 (6): 2165–2173.[3] M.Swartling,N.Grbic´, I.Claesson. Direction of arrival estimation for multiple speakers using time-frequency orthogonal signal separation[C]. Proceedings of IEEE International Conference on acoustic, Speech and Signal Processing, XX. 833–836.[4] M. S. Brand stein, J.E. Adcock, H.F. Silverman.A closed-form location estimator for use with room environment microphone arrays[J]. IEEE Transactions on Speech and Audio Processing, 1997, 5 (1): 45–50.[5] M. Swartling, M. Nilsson, N.Grbic. Distinguishing true and false source locations when localizing multiple concurrent speech sources[C]. Proceedings of IEEE Sensor Array and Multichannel Signal ProcessingWorkshop, XX. 361–364.[6] E. Di Claudio, R. Parisi, G. Orlandi. Multi-source localization in reverberant environments by ROOT-MUSIC and clustering[C]. Proceedings of IEEE International Conference on Acoustic, Speech and Signal Processing, XX. 921–924.[7] T. Nishiura, T. Yamada, S. Nakamura, K. Shikano. Localization of multiple sound sources based on a CSP analysis with a microphone array[C]. Proceedings of IEEE International Conference on Acoustic, Speech and Signal Processing, XX. 1053–1056.[8] R. Balan, J. Rosca, S. Rickard, J. ORuanaidh. The influence of windowing of time delay estimates[C]. Proceedings of Conference on Information Sciences and Systems, XX. 15–17.[9] S. Shifman, A. Bhomra, S. Smiley, et al. A whole genome association study of neuroticism using DNA pooling[J]. Molecular Psychiatry, XX, 13(3): 302–312.[10] S. Rickard, R. Balan, J. Rosca, Real-time time-frequency based blind source separation[C]. Proceedings of International Workshop on Independent Component Analysis and Blind Signal Separation, XX. 651–656.源自[11] K. Yiu, N. Grbic, S. Nordholm, et al. Multi-criteria design of oversampled uniform DFT filterbanks[J]. IEEE Signal Processing Letters, XX, 11(6): 541–544.[12] E. Vincent. Complex nonconvex lp nom minimization for underdetermined source separation[C]. Proc. ICA, XX. 430-437[13] C. Knapp , G. Carter. The generalized correlation method for estimation of time delay[J]. IEEE Trans. Acoust., Speech, Signal Process, 1987, 24(4): 320–327.[14] T. W. Anderson. Asymptotic theory for principal component analysis[J]. Ann. Math. Statist., XX, 34(1): 122–148.[15] D. Campbell, K. Palomäki, G. Brown. A matlab simulation of shoebox room acoustics for use inresearch and teaching[J]. Comput. Inf. Syst. J., XX, 9(3): 48–51[16] J. Huang, N. Ohnishi, N. Sugie. A biomimetic system for localization and separation of multiple sound sources[J]. IEEE Trans. Instrum.Meas., 1995(44): 733–738.[17] B. Berdugo, J. Rosenhouse, H. Azhari. Speakers’direction finding using estimated time delays in the frequency domain[J]. Signal Processing, XX, 82(1): 19–30.[18] S. T. Roweis. One microphone source separation[J]. Neural Inform.Process. Syst., 793–799.[19] J.-K. Lin, D. G. Grier, J. D. Cowan. Feature extraction approachto blind source separation[C]. Proc. IEEE Workshop Neural NetworksSignal Process, 1997. 398–405.[20] M. Van Hulle. Clustering approach to square and nonsquare blind source separation[C]. IEEE Workshop Neural Networks Signal Processing. 1999. 315–323. :。
写作业加速的英语
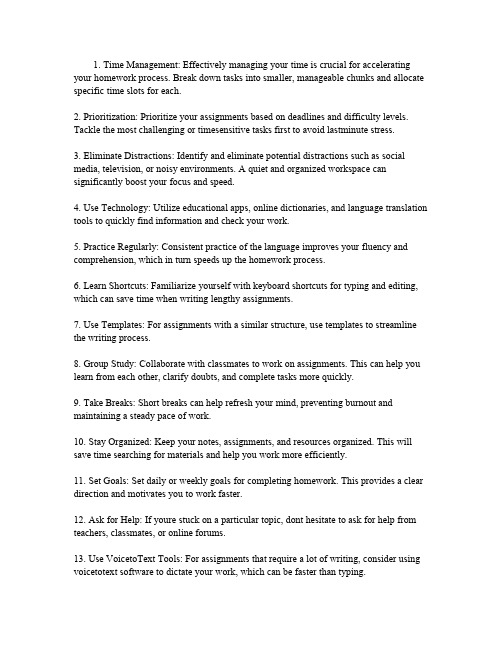
1.Time Management:Effectively managing your time is crucial for accelerating your homework process.Break down tasks into smaller,manageable chunks and allocate specific time slots for each.2.Prioritization:Prioritize your assignments based on deadlines and difficulty levels. Tackle the most challenging or timesensitive tasks first to avoid lastminute stress.3.Eliminate Distractions:Identify and eliminate potential distractions such as social media,television,or noisy environments.A quiet and organized workspace can significantly boost your focus and speed.e Technology:Utilize educational apps,online dictionaries,and language translation tools to quickly find information and check your work.5.Practice Regularly:Consistent practice of the language improves your fluency and comprehension,which in turn speeds up the homework process.6.Learn Shortcuts:Familiarize yourself with keyboard shortcuts for typing and editing, which can save time when writing lengthy assignments.e Templates:For assignments with a similar structure,use templates to streamline the writing process.8.Group Study:Collaborate with classmates to work on assignments.This can help you learn from each other,clarify doubts,and complete tasks more quickly.9.Take Breaks:Short breaks can help refresh your mind,preventing burnout and maintaining a steady pace of work.10.Stay Organized:Keep your notes,assignments,and resources organized.This will save time searching for materials and help you work more efficiently.11.Set Goals:Set daily or weekly goals for completing homework.This provides a clear direction and motivates you to work faster.12.Ask for Help:If youre stuck on a particular topic,dont hesitate to ask for help from teachers,classmates,or online forums.e VoicetoText Tools:For assignments that require a lot of writing,consider using voicetotext software to dictate your work,which can be faster than typing.14.Review and Revise:Once youve completed an assignment,review it for errors and make revisions.This ensures the quality of your work while also identifying areas for improvement.15.Develop a Routine:Establish a homework routine that works best for you.This could include specific times of day when you are most productive.16.Stay Healthy:Maintain a healthy lifestyle with proper nutrition,exercise,and sleep.A healthy body supports a more efficient mind.e Flashcards:For vocabulary or language learning,use flashcards to quickly memorize and review terms.18.Learn from Mistakes:Analyze past assignments to understand where you spent too much time and how you can improve your approach.19.Set a Timer:Use a timer to keep track of how long tasks take.This can help you gauge your speed and make adjustments as needed.20.Stay Positive:Maintain a positive attitude towards your work.A positive mindset can help you overcome challenges and work more efficiently.。
基于自然语言处理的机器翻译系统设计与实现
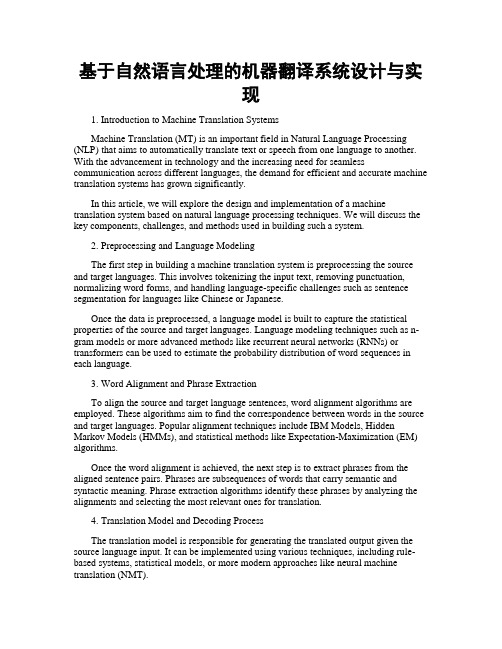
基于自然语言处理的机器翻译系统设计与实现1. Introduction to Machine Translation SystemsMachine Translation (MT) is an important field in Natural Language Processing (NLP) that aims to automatically translate text or speech from one language to another. With the advancement in technology and the increasing need for seamless communication across different languages, the demand for efficient and accurate machine translation systems has grown significantly.In this article, we will explore the design and implementation of a machine translation system based on natural language processing techniques. We will discuss the key components, challenges, and methods used in building such a system.2. Preprocessing and Language ModelingThe first step in building a machine translation system is preprocessing the source and target languages. This involves tokenizing the input text, removing punctuation, normalizing word forms, and handling language-specific challenges such as sentence segmentation for languages like Chinese or Japanese.Once the data is preprocessed, a language model is built to capture the statistical properties of the source and target languages. Language modeling techniques such as n-gram models or more advanced methods like recurrent neural networks (RNNs) or transformers can be used to estimate the probability distribution of word sequences in each language.3. Word Alignment and Phrase ExtractionTo align the source and target language sentences, word alignment algorithms are employed. These algorithms aim to find the correspondence between words in the source and target languages. Popular alignment techniques include IBM Models, Hidden Markov Models (HMMs), and statistical methods like Expectation-Maximization (EM) algorithms.Once the word alignment is achieved, the next step is to extract phrases from the aligned sentence pairs. Phrases are subsequences of words that carry semantic and syntactic meaning. Phrase extraction algorithms identify these phrases by analyzing the alignments and selecting the most relevant ones for translation.4. Translation Model and Decoding ProcessThe translation model is responsible for generating the translated output given the source language input. It can be implemented using various techniques, including rule-based systems, statistical models, or more modern approaches like neural machine translation (NMT).In statistical machine translation (SMT), the translation model estimates the probability of generating a target sentence given a source sentence and specific translation rules. NMT employs neural networks to learn the translation patterns from large amounts of parallel corpora.During the decoding process, the translation model generates the most probable translation for a given source sentence. This can be done using various search algorithms, such as beam search or dynamic programming, to find the best translation among multiple hypotheses.5. Evaluation and ImprovementEvaluating the quality of machine translation systems is crucial to measure their performance accurately. Automatic metrics like BLEU (Bilingual Evaluation Understudy) or METEOR (Metric for Evaluation of Translation with Explicit Ordering) can assess the translation quality by comparing the output with human-generated translations.Continual improvement of the translation system is achieved by analyzing errors, refining the preprocessing steps, optimizing the translation model, and incorporating feedback from human evaluators. Iterative model training and fine-tuning techniques can enhance the overall translation accuracy and fluency.6. Applications and Future ProspectsMachine translation systems find applications in various domains, including global commerce, travel, international diplomacy, and language learning. They facilitate cross-cultural communication and enable people to access information and services beyond language barriers.The future prospects of machine translation involve the integration of deep learning techniques, leveraging large-scale monolingual data, and exploring unsupervised or semi-supervised learning approaches to overcome the limitations of traditional methods. Neural machine translation has shown promising results and is likely to continue dominating the field.ConclusionIn conclusion, designing and implementing a machine translation system based on natural language processing techniques involves several key components, including preprocessing, language modeling, word alignment, phrase extraction, translation modeling, decoding, evaluation, and continuous improvement. Machine translation has made significant progress in recent years and continues to be an active area of research and development. With further advancements in artificial intelligence and deep learning, the future of machine translation looks promising for enabling seamless communication across diverse languages.。
低频活动漂浮潜水船声探测系统(LFATS)说明书

LOW-FREQUENCY ACTIVE TOWED SONAR (LFATS)LFATS is a full-feature, long-range,low-frequency variable depth sonarDeveloped for active sonar operation against modern dieselelectric submarines, LFATS has demonstrated consistent detection performance in shallow and deep water. LFATS also provides a passive mode and includes a full set of passive tools and features.COMPACT SIZELFATS is a small, lightweight, air-transportable, ruggedized system designed specifically for easy installation on small vessels. CONFIGURABLELFATS can operate in a stand-alone configuration or be easily integrated into the ship’s combat system.TACTICAL BISTATIC AND MULTISTATIC CAPABILITYA robust infrastructure permits interoperability with the HELRAS helicopter dipping sonar and all key sonobuoys.HIGHLY MANEUVERABLEOwn-ship noise reduction processing algorithms, coupled with compact twin line receivers, enable short-scope towing for efficient maneuvering, fast deployment and unencumbered operation in shallow water.COMPACT WINCH AND HANDLING SYSTEMAn ultrastable structure assures safe, reliable operation in heavy seas and permits manual or console-controlled deployment, retrieval and depth-keeping. FULL 360° COVERAGEA dual parallel array configuration and advanced signal processing achieve instantaneous, unambiguous left/right target discrimination.SPACE-SAVING TRANSMITTERTOW-BODY CONFIGURATIONInnovative technology achievesomnidirectional, large aperture acousticperformance in a compact, sleek tow-body assembly.REVERBERATION SUPRESSIONThe unique transmitter design enablesforward, aft, port and starboarddirectional transmission. This capabilitydiverts energy concentration away fromshorelines and landmasses, minimizingreverb and optimizing target detection.SONAR PERFORMANCE PREDICTIONA key ingredient to mission planning,LFATS computes and displays systemdetection capability based on modeled ormeasured environmental data.Key Features>Wide-area search>Target detection, localization andclassification>T racking and attack>Embedded trainingSonar Processing>Active processing: State-of-the-art signal processing offers acomprehensive range of single- andmulti-pulse, FM and CW processingfor detection and tracking. Targetdetection, localization andclassification>P assive processing: LFATS featuresfull 100-to-2,000 Hz continuouswideband coverage. Broadband,DEMON and narrowband analyzers,torpedo alert and extendedtracking functions constitute asuite of passive tools to track andanalyze targets.>Playback mode: Playback isseamlessly integrated intopassive and active operation,enabling postanalysis of pre-recorded mission data and is a keycomponent to operator training.>Built-in test: Power-up, continuousbackground and operator-initiatedtest modes combine to boostsystem availability and accelerateoperational readiness.UNIQUE EXTENSION/RETRACTIONMECHANISM TRANSFORMS COMPACTTOW-BODY CONFIGURATION TO ALARGE-APERTURE MULTIDIRECTIONALTRANSMITTERDISPLAYS AND OPERATOR INTERFACES>State-of-the-art workstation-based operator machineinterface: Trackball, point-and-click control, pull-down menu function and parameter selection allows easy access to key information. >Displays: A strategic balance of multifunction displays,built on a modern OpenGL framework, offer flexible search, classification and geographic formats. Ground-stabilized, high-resolution color monitors capture details in the real-time processed sonar data. > B uilt-in operator aids: To simplify operation, LFATS provides recommended mode/parameter settings, automated range-of-day estimation and data history recall. >COTS hardware: LFATS incorporates a modular, expandable open architecture to accommodate future technology.L3Harrissellsht_LFATS© 2022 L3Harris Technologies, Inc. | 09/2022NON-EXPORT CONTROLLED - These item(s)/data have been reviewed in accordance with the InternationalTraffic in Arms Regulations (ITAR), 22 CFR part 120.33, and the Export Administration Regulations (EAR), 15 CFR 734(3)(b)(3), and may be released without export restrictions.L3Harris Technologies is an agile global aerospace and defense technology innovator, delivering end-to-endsolutions that meet customers’ mission-critical needs. The company provides advanced defense and commercial technologies across air, land, sea, space and cyber domains.t 818 367 0111 | f 818 364 2491 *******************WINCH AND HANDLINGSYSTEMSHIP ELECTRONICSTOWED SUBSYSTEMSONAR OPERATORCONSOLETRANSMIT POWERAMPLIFIER 1025 W. NASA Boulevard Melbourne, FL 32919SPECIFICATIONSOperating Modes Active, passive, test, playback, multi-staticSource Level 219 dB Omnidirectional, 222 dB Sector Steered Projector Elements 16 in 4 stavesTransmission Omnidirectional or by sector Operating Depth 15-to-300 m Survival Speed 30 knotsSize Winch & Handling Subsystem:180 in. x 138 in. x 84 in.(4.5 m x 3.5 m x 2.2 m)Sonar Operator Console:60 in. x 26 in. x 68 in.(1.52 m x 0.66 m x 1.73 m)Transmit Power Amplifier:42 in. x 28 in. x 68 in.(1.07 m x 0.71 m x 1.73 m)Weight Winch & Handling: 3,954 kg (8,717 lb.)Towed Subsystem: 678 kg (1,495 lb.)Ship Electronics: 928 kg (2,045 lb.)Platforms Frigates, corvettes, small patrol boats Receive ArrayConfiguration: Twin-lineNumber of channels: 48 per lineLength: 26.5 m (86.9 ft.)Array directivity: >18 dB @ 1,380 HzLFATS PROCESSINGActiveActive Band 1,200-to-1,00 HzProcessing CW, FM, wavetrain, multi-pulse matched filtering Pulse Lengths Range-dependent, .039 to 10 sec. max.FM Bandwidth 50, 100 and 300 HzTracking 20 auto and operator-initiated Displays PPI, bearing range, Doppler range, FM A-scan, geographic overlayRange Scale5, 10, 20, 40, and 80 kyd PassivePassive Band Continuous 100-to-2,000 HzProcessing Broadband, narrowband, ALI, DEMON and tracking Displays BTR, BFI, NALI, DEMON and LOFAR Tracking 20 auto and operator-initiatedCommonOwn-ship noise reduction, doppler nullification, directional audio。
Speech-to-text and speech-to-speech summarization of spontaneous speech
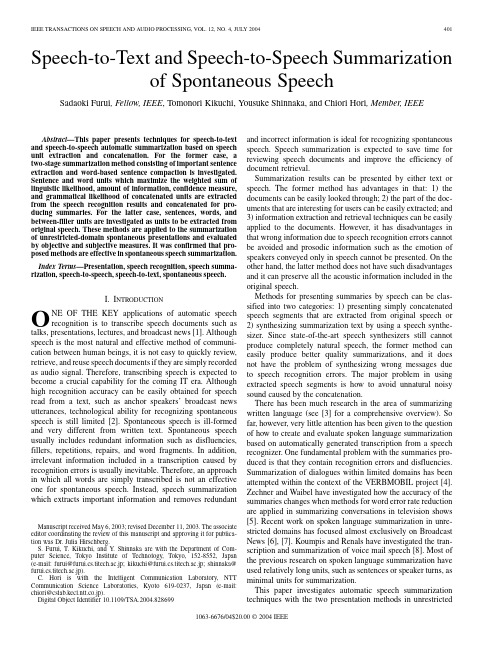
Speech-to-Text and Speech-to-Speech Summarizationof Spontaneous SpeechSadaoki Furui,Fellow,IEEE,Tomonori Kikuchi,Yousuke Shinnaka,and Chiori Hori,Member,IEEEAbstract—This paper presents techniques for speech-to-text and speech-to-speech automatic summarization based on speech unit extraction and concatenation.For the former case,a two-stage summarization method consisting of important sentence extraction and word-based sentence compaction is investigated. Sentence and word units which maximize the weighted sum of linguistic likelihood,amount of information,confidence measure, and grammatical likelihood of concatenated units are extracted from the speech recognition results and concatenated for pro-ducing summaries.For the latter case,sentences,words,and between-filler units are investigated as units to be extracted from original speech.These methods are applied to the summarization of unrestricted-domain spontaneous presentations and evaluated by objective and subjective measures.It was confirmed that pro-posed methods are effective in spontaneous speech summarization. Index Terms—Presentation,speech recognition,speech summa-rization,speech-to-speech,speech-to-text,spontaneous speech.I.I NTRODUCTIONO NE OF THE KEY applications of automatic speech recognition is to transcribe speech documents such as talks,presentations,lectures,and broadcast news[1].Although speech is the most natural and effective method of communi-cation between human beings,it is not easy to quickly review, retrieve,and reuse speech documents if they are simply recorded as audio signal.Therefore,transcribing speech is expected to become a crucial capability for the coming IT era.Although high recognition accuracy can be easily obtained for speech read from a text,such as anchor speakers’broadcast news utterances,technological ability for recognizing spontaneous speech is still limited[2].Spontaneous speech is ill-formed and very different from written text.Spontaneous speech usually includes redundant information such as disfluencies, fillers,repetitions,repairs,and word fragments.In addition, irrelevant information included in a transcription caused by recognition errors is usually inevitable.Therefore,an approach in which all words are simply transcribed is not an effective one for spontaneous speech.Instead,speech summarization which extracts important information and removes redundantManuscript received May6,2003;revised December11,2003.The associate editor coordinating the review of this manuscript and approving it for publica-tion was Dr.Julia Hirschberg.S.Furui,T.Kikuchi,and Y.Shinnaka are with the Department of Com-puter Science,Tokyo Institute of Technology,Tokyo,152-8552,Japan (e-mail:furui@furui.cs.titech.ac.jp;kikuchi@furui.cs.titech.ac.jp;shinnaka@ furui.cs.titech.ac.jp).C.Hori is with the Intelligent Communication Laboratory,NTT Communication Science Laboratories,Kyoto619-0237,Japan(e-mail: chiori@cslab.kecl.ntt.co.jp).Digital Object Identifier10.1109/TSA.2004.828699and incorrect information is ideal for recognizing spontaneous speech.Speech summarization is expected to save time for reviewing speech documents and improve the efficiency of document retrieval.Summarization results can be presented by either text or speech.The former method has advantages in that:1)the documents can be easily looked through;2)the part of the doc-uments that are interesting for users can be easily extracted;and 3)information extraction and retrieval techniques can be easily applied to the documents.However,it has disadvantages in that wrong information due to speech recognition errors cannot be avoided and prosodic information such as the emotion of speakers conveyed only in speech cannot be presented.On the other hand,the latter method does not have such disadvantages and it can preserve all the acoustic information included in the original speech.Methods for presenting summaries by speech can be clas-sified into two categories:1)presenting simply concatenated speech segments that are extracted from original speech or 2)synthesizing summarization text by using a speech synthe-sizer.Since state-of-the-art speech synthesizers still cannot produce completely natural speech,the former method can easily produce better quality summarizations,and it does not have the problem of synthesizing wrong messages due to speech recognition errors.The major problem in using extracted speech segments is how to avoid unnatural noisy sound caused by the concatenation.There has been much research in the area of summarizing written language(see[3]for a comprehensive overview).So far,however,very little attention has been given to the question of how to create and evaluate spoken language summarization based on automatically generated transcription from a speech recognizer.One fundamental problem with the summaries pro-duced is that they contain recognition errors and disfluencies. Summarization of dialogues within limited domains has been attempted within the context of the VERBMOBIL project[4]. Zechner and Waibel have investigated how the accuracy of the summaries changes when methods for word error rate reduction are applied in summarizing conversations in television shows [5].Recent work on spoken language summarization in unre-stricted domains has focused almost exclusively on Broadcast News[6],[7].Koumpis and Renals have investigated the tran-scription and summarization of voice mail speech[8].Most of the previous research on spoken language summarization have used relatively long units,such as sentences or speaker turns,as minimal units for summarization.This paper investigates automatic speech summarization techniques with the two presentation methods in unrestricted1063-6676/04$20.00©2004IEEEdomains.In both cases,the most appropriate sentences,phrases or word units/segments are automatically extracted from orig-inal speech and concatenated to produce a summary under the constraint that extracted units cannot be reordered or replaced. Only when the summary is presented by text,transcription is modified into a written editorial article style by certain rules.When the summary is presented by speech,a waveform concatenation-based method is used.Although prosodic features such as accent and intonation could be used for selection of important parts,reliable methods for automatic and correct extraction of prosodic features from spontaneous speech and for modeling them have not yet been established.Therefore,in this paper,input speech is automat-ically recognized and important segments are extracted based only on the textual information.Evaluation experiments are performed using spontaneous presentation utterances in the Corpus of Spontaneous Japanese (CSJ)made by the Spontaneous Speech Corpus and Processing Project[9].The project began in1999and is being conducted over a five-year period with the following three major targets.1)Building a large-scale spontaneous speech corpus(CSJ)consisting of roughly7M words with a total speech length of700h.This mainly records monologues such as lectures,presentations and news commentaries.The recordings with low spontaneity,such as those from read text,are excluded from the corpus.The utterances are manually transcribed orthographically and phonetically.One-tenth of them,called Core,are tagged manually and used for training a morphological analysis and part-of-speech(POS)tagging program for automati-cally analyzing all of the700-h utterances.The Core is also tagged with para-linguistic information including intonation.2)Acoustic and language modeling for spontaneous speechunderstanding using linguistic,as well as para-linguistic, information in speech.3)Investigating spontaneous speech summarization tech-nology.II.S UMMARIZATION W ITH T EXT P RESENTATIONA.Two-Stage Summarization MethodFig.1shows the two-stage summarization method consisting of important sentence extraction and sentence compaction[10]. Using speech recognition results,the score for important sen-tence extraction is calculated for each sentence.After removing all the fillers,a set of relatively important sentences is extracted, and sentence compaction using our proposed method[11],[12] is applied to the set of extracted sentences.The ratio of sentence extraction and compaction is controlled according to a summa-rization ratio initially determined by the user.Speech summarization has a number of significant chal-lenges that distinguish it from general text summarization. Applying text-based technologies to speech is not always workable and often they are not equipped to capture speech specific phenomena.Speech contains a number of spontaneous effects,which are not present in written language,such as hesitations,false starts,and fillers.Speech is,to someextent,Fig. 1.A two-stage automatic speech summarization system with text presentation.always distorted by ungrammatical and various redundant expressions.Speech is also a continuous phenomenon that comes without unambiguous sentence boundaries.In addition, errors in transcriptions of automatic speech recognition engines can be quite substantial.Sentence extraction methods on which most of the text summarization methods[13]are based cannot cope with the problems of distorted information and redundant expressions in speech.Although several sentence compression methods have also been investigated in text summarization[14],[15], they rely on discourse and grammatical structures of the input text.Therefore,it is difficult to apply them to spontaneous speech with ill-formed structures.The method proposed in this paper is suitable for applying to ill-formed speech recognition results,since it simultaneously uses various statistical features, including a confidence measure of speech recognition results. The principle of the speech-to-text summarization method is also used in the speech-to-speech summarization which will be described in the next section.Speech-to-speech summarization is a comparatively much younger discipline,and has not yet been investigated in the same framework as the speech-to-text summarization.1)Important Sentence Extraction:Important sentence ex-traction is performed according to the following score for eachsentence,obtained as a result of speechrecognition(1)where is the number of words in thesentenceand, ,and are the linguistic score,the significance score,and the confidence score ofword,respectively. Although sentence boundaries can be estimated using linguistic and prosodic information[16],they are manually given in the experiments in this paper.The three scores are a subset of the scores originally used in our sentence compaction method and considered to be useful also as measures indicating theFURUI et al.:SPEECH-TO-TEXT AND SPEECH-TO-SPEECH SUMMARIZATION 403appropriateness of including the sentence in thesummary.and are weighting factors for balancing the scores.Details of the scores are as follows.Linguistic score :The linguisticscore indicates the linguistic likelihood of word strings in the sentence and is measured by n-gramprobability(2)In our experiment,trigram probability calculated using transcriptions of presentation utterances in the CSJ con-sisting of 1.5M morphemes (words)is used.This score de-weights linguistically unnatural word strings caused by recognition errors.Significance score :The significancescoreindicates the significance of eachword in the sentence and is measured by the amount of information.The amount of in-formation contained in each word is calculated for content words including nouns,verbs,adjectives and out-of-vocab-ulary (OOV)words,based on word occurrence in a corpus as shown in (3).The POS information for each word is ob-tained from the recognition result,since every word in the dictionary is accompanied with a unique POS tag.A flat score is given to other words,and(3)where is the number of occurrencesof in the recog-nizedutterances,is the number of occurrencesof ina large-scale corpus,andis the number of all content words in that corpus,thatis.For measuring the significance score,the number of occurrences of 120000kinds of words is calculated in a corpus consisting of transcribed presentations (1.5M words),proceedings of 60presentations,presentation records obtained from the World-Wide Web (WWW)(2.1M words),NHK (Japanese broadcast company)broadcast news text (22M words),Mainichi newspaper text (87M words)and text from a speech textbook “Speech Information Processing ”(51000words).Im-portant keywords are weighted and the words unrelated to the original content,such as recognition errors,are de-weighted by this score.Confidence score :The confidencescoreis incor-porated to weight acoustically as well as linguistically re-liable hypotheses.Specifically,a logarithmic value of the posterior probability for each transcribed word,which is the ratio of a word hypothesis probability to that of all other hypotheses,is calculated using a word graph obtained by a decoder and used as a confidence score.2)Sentence Compaction:After removing relatively less important sentences,the remaining transcription is auto-matically modified into a written editorial article style to calculate the score for sentence compaction.All the sentences are concatenated while preserving sentence boundaries,and a linguisticscore,,a significancescore ,and aconfidencescoreare given to each transcribed word.A word concatenationscorefor every combination of words within each transcribed sentence is also given to weighta word concatenation between words.This score is a measure of the dependency between two words and is obtained by a phrase structure grammar,stochastic dependency context-free grammar (SDCFG).A set of words that maximizes a weighted sum of these scores is selected according to a given compres-sion ratio and connected to create a summary using a two-stage dynamic programming (DP)technique.Specifically,each sentence is summarized according to all possible compression ratios,and then the best combination of summarized sentences is determined according to a target total compression ratio.Ideally,the linguistic score should be calculated using a word concatenation model based on a large-scale summary corpus.Since such a summary corpus is not yet available,the tran-scribed presentations used to calculate the word trigrams for the important sentence extraction are automatically modified into a written editorial article style and used together with the pro-ceedings of 60presentations to calculate the trigrams.The significance score is calculated using the same corpus as that used for calculating the score for important sentence extraction.The word-dependency probability is estimated by the Inside-Outside algorithm,using a manually parsed Mainichi newspaper corpus having 4M sentences with 68M words.For the details of the SDCFG and dependency scores,readers should refer to [12].B.Evaluation Experiments1)Evaluation Set:Three presentations,M74,M35,and M31,in the CSJ by male speakers were summarized at summarization ratios of 70%and 50%.The summarization ratio was defined as the ratio of the number of characters in the summaries to that in the recognition results.Table I shows features of the presentations,that is,length,mean word recognition accuracy,number of sentences,number of words,number of fillers,filler ratio,and number of disfluencies including repairs of each presentation.They were manually segmented into sentences before recognition.The table shows that the presentation M35has a significantly large number of disfluencies and a low recognition accuracy,and M31has a significantly high filler ratio.2)Summarization Accuracy:To objectively evaluate the summaries,correctly transcribed presentation speech was manually summarized by nine human subjects to create targets.Devising meaningful evaluation criteria and metrics for speech summarization is a problematic issue.Speech does not have explicit sentence boundaries in contrast with text input.There-fore,speech summarization results cannot be evaluated using the F-measure based on sentence units.In addition,since words (morphemes)within sentences are extracted and concatenated in the summarization process,variations of target summaries made by human subjects are much larger than those using the sentence level method.In almost all cases,an “ideal ”summary does not exist.For these reasons,variations of the manual summarization results were merged into a word network as shown in Fig.2,which is considered to approximately express all possible correct summaries covering subjective variations.Word accuracy of the summary is then measured in comparison with the closest word string extracted from the word network as the summarization accuracy [5].404IEEE TRANSACTIONS ON SPEECH AND AUDIO PROCESSING,VOL.12,NO.4,JULY 2004TABLE I E V ALUATION SETFig.2.Word network made by merging manual summarization results.3)Evaluation Conditions:Summarization was performed under the following nine conditions:single-stage summariza-tion without applying the important sentence extraction (NOS);two-stage summarization using seven kinds of the possible combination of scores for important sentence extraction(,,,,,,);and summarization by randomword selection.The weightingfactorsand were set at optimum values for each experimental condition.C.Evaluation Results1)Summarization Accuracy:Results of the evaluation ex-periments are shown in Figs.3and 4.In all the automatic summarization conditions,both the one-stage method without sentence extraction and the two-stage method including sen-tence extraction achieve better results than random word se-lection.In both the 70%and 50%summarization conditions,the two-stage method achieves higher summarization accuracy than the one-stage method.The two-stage method is more ef-fective in the condition of the smaller summarization ratio (50%),that is,where there is a higher compression ratio,than in the condition of the larger summarization ratio (70%).In the 50%summarization condition,the two-stage method is effective for all three presentations.The two-stage method is especially effective for avoiding one of the problems of the one-stage method,that is,the production of short unreadable and/or incomprehensible sentences.Comparing the three scores for sentence extraction,the sig-nificancescoreis more effective than the linguisticscore and the confidencescore .The summarization score can beincreased by using the combination of two scores(,,),and even more by combining all threescores.Fig. 3.Results of the summarization with text presentation at 50%summarizationratio.Fig. 4.Results of the summarization with text presentation at 70%summarization ratio.FURUI et al.:SPEECH-TO-TEXT AND SPEECH-TO-SPEECH SUMMARIZATION405The differences are,however,statistically insignificant in these experiments,due to the limited size of the data.2)Effects of the Ratio of Compression by Sentence Extrac-tion:Figs.5and6show the summarization accuracy as a function of the ratio of compression by sentence extraction for the total summarization ratios of50%or70%.The left and right ends of the figures correspond to summarizations by only sentence compaction and sentence extraction,respectively. These results indicate that although the best summarization accuracy of each presentation can be obtained at a different ratio of compression by sentence extraction,there is a general tendency where the smaller the summarization ratio becomes, the larger the optimum ratio of compression by sentence extraction becomes.That is,sentence extraction becomes more effective when the summarization ratio gets smaller. Comparing results at the left and right ends of the figures, summarization by word extraction(i.e.,sentence compaction) is more effective than sentence extraction for the M35presenta-tion.This presentation includes a relatively large amount of re-dundant information,such as disfluencies and repairs,and has a significantly low recognition accuracy.These results indicate that the optimum division of the compression ratio into the two summarization stages needs to be estimated according to the specific summarization ratio and features of the presentation in question,such as frequency of disfluencies.III.S UMMARIZATION W ITH S PEECH P RESENTATIONA.Unit Selection and Concatenation1)Units for Extraction:The following issues need to be ad-dressed in extracting and concatenating speech segments for making summaries.1)Units for extraction:sentences,phrases,or words.2)Criteria for measuring the importance of units forextraction.3)Concatenation methods for making summary speech. The following three units are investigated in this paper:sen-tences,words,and between-filler units.All the fillers automat-ically detected as the result of recognition are removed before extracting important segments.Sentence units:The method described in Section II-A.1 is applied to the recognition results to extract important sentences.Since sentences are basic linguistic as well as acoustic units,it is easy to maintain acoustical smoothness by using sentences as units,and therefore the concatenated speech sounds natural.However,since the units are rela-tively long,they tend to include unnecessary words.Since fillers are automatically removed even if they are included within sentences as described above,the sentences are cut and shortened at the position of fillers.Word units:Word sets are extracted and concatenated by applying the method described in Section II-A.2to the recognition results.Although this method has an advan-tage in that important parts can be precisely extracted in small units,it tends to cause acoustical discontinuity since many small units of speech need to be concatenated.There-fore,summarization speech made by this method some-times soundsunnatural.Fig.5.Summarization accuracy as a function of the ratio of compression by sentence extraction for the total summarization ratio of50%.Fig.6.Summarization accuracy as a function of the ratio of compression by sentence extraction for the total summarization ratio of70%.Between-filler units:Speech segments between fillers as well as sentence boundaries are extracted using speech recognition results.The same method as that used for ex-tracting sentence units is applied to evaluate these units.These units are introduced as intermediate units between sentences and words,in anticipation of both reasonably precise extraction of important parts and naturalness of speech with acoustic continuity.2)Unit Concatenation:Units for building summarization speech are extracted from original speech by using segmentation boundaries obtained from speech recognition results.When the units are concatenated at the inside of sentences,it may produce noise due to a difference of amplitudes of the speech waveforms. In order to avoid this problem,amplitudes of approximately 20-ms length at the unit boundaries are gradually attenuated before the concatenation.Since this causes an impression of406IEEE TRANSACTIONS ON SPEECH AND AUDIO PROCESSING,VOL.12,NO.4,JULY 2004TABLE IIS UMMARIZATION A CCURACY AND N UMBER OF U NITS FOR THE T HREE K INDS OF S UMMARIZATION UNITSincreasing the speaking rate and thus creates an unnatural sound,a short pause is inserted.The length of the pause is controlled between 50and 100ms empirically according to the concatenation conditions.Each summarization speech which has been made by this method is hereafter referred to as “summarization speech sentence ”and the text corresponding to its speech period is referred to as “summarization text sentence.”The summarization speech sentences are further concate-nated to create a summarized speech for the whole presentation.Speech waveforms at sentence boundaries are gradually at-tenuated and pauses are inserted between the sentences in the same way as the unit concatenation within sentences.Short and long pauses with 200-and 700-ms lengths are used as pauses between sentences.Long pauses are inserted after sentence ending expressions,otherwise short pauses are used.In the case of summarization by word-unit concatenation,long pauses are always used,since many sentences terminate with nouns and need relatively long pauses to make them sound natural.B.Evaluation Experiments1)Experimental Conditions:The three presentations,M74,M35,and M31,were automatically summarized with a summarization ratio of 50%.Summarization accuracies for the three presentations using sentence units,between-filler units,and word units,are given in Table II.Manual summaries made by nine human subjects were used for the evaluation.The table also shows the number of automatically detected units in each condition.For the case of using the between-filler units,the number of detected fillers is also shown.Using the summarization text sentences,speech segments were extracted and concatenated to build summarization speech,and subjective evaluation by 11subjects was performed in terms of ease of understanding and appropriateness as a sum-marization with five levels:1—very bad;2—bad;3—normal;4—good;and 5—very good.The subjects were instructed to read the transcriptions of the presentations and understand the contents before hearing the summarizationspeech.Fig.7.Evaluation results for the summarization with speech presentation in terms of the ease ofunderstanding.Fig.8.Evaluation results for the summarization with speech presentation in terms of the appropriateness as a summary.2)Evaluation Results and Discussion:Figs.7and 8show the evaluation results.Averaging over the three presentations,the sentence units show the best results whereas the word unitsFURUI et al.:SPEECH-TO-TEXT AND SPEECH-TO-SPEECH SUMMARIZATION407show the worst.For the two presentations,M74and M35,the between-filler units achieve almost the same results as the sen-tence units.The reason why the word units which show slightly better summarization accuracy in Table II also show the worst subjective evaluation results here is because of unnatural sound due to the concatenation of short speech units.The relatively large number of fillers included in the presentation M31pro-duced many short units when the between-filler unit method was applied.This is the reason why between-filler units show worse subjective results than the sentence units for M31.If the summarization ratio is set lower than50%,between-filler units are expected to achieve better results than sentence units,since sentence units cannot remove redundant expressions within sentences.IV.C ONCLUSIONIn this paper,we have presented techniques for com-paction-based automatic speech summarization and evaluation results for summarizing spontaneous presentations.The sum-marization results are presented by either text or speech.In the former case,the speech-to-test summarization,we proposed a two-stage automatic speech summarization method consisting of important sentence extraction and word-based sentence compaction.In this method,inadequate sentences including recognition errors and less important information are automat-ically removed before sentence compaction.It was confirmed that in spontaneous presentation speech summarization at70% and50%summarization ratios,combining sentence extraction with sentence compaction is effective;this method achieves better summarization performance than our previous one-stage method.It was also confirmed that three scores,the linguistic score,the word significance score and the word confidence score,are effective for extracting important sentences.The best division for the summarization ratio into the ratios of sentence extraction and sentence compaction depends on the summarization ratio and features of presentation utterances. For the case of presenting summaries by speech,the speech-to-speech summarization,three kinds of units—sen-tences,words,and between-filler units—were investigated as units to be extracted from original speech and concatenated to produce the summaries.A set of units is automatically extracted using the same measures used in the speech-to-text summarization,and the speech segments corresponding to the extracted units are concatenated to produce the summaries. Amplitudes of speech waveforms at the boundaries are grad-ually attenuated and pauses are inserted before concatenation to avoid acoustic discontinuity.Subjective evaluation results for the50%summarization ratio indicated that sentence units achieve the best subjective evaluation score.Between-filler units are expected to achieve good performance when the summarization ratio becomes smaller.As stated in the introduction,speech summarization tech-nology can be applied to any kind of speech document and is expected to play an important role in building various speech archives including broadcast news,lectures,presentations,and interviews.Summarization and question answering(QA)per-form a similar task,in that they both map an abundance of information to a(much)smaller piece to be presented to the user[17].Therefore,speech summarization research will help the advancement of QA systems using speech documents.By condensing important points of long presentations and lectures, speech-to-speech summarization can provide the listener with a valuable means for absorbing much information in a much shorter time.Future research includes evaluation by a large number of presentations at various summarization ratios including smaller ratios,investigation of other information/features for impor-tant unit extraction,methods for automatically segmenting a presentation into sentence units[16],those methods’effects on summarization accuracy,and automatic optimization of the division of compression ratio into the two summarization stages according to the summarization ratio and features of the presentation.A CKNOWLEDGMENTThe authors would like to thank NHK(Japan Broadcasting Corporation)for providing the broadcast news database.R EFERENCES[1]S.Furui,K.Iwano,C.Hori,T.Shinozaki,Y.Saito,and S.Tamura,“Ubiquitous speech processing,”in Proc.ICASSP2001,vol.1,Salt Lake City,UT,2001,pp.13–16.[2]S.Furui,“Recent advances in spontaneous speech recognition and un-derstanding,”in Proc.ISCA-IEEE Workshop on Spontaneous Speech Processing and Recognition,Tokyo,Japan,2003.[3]I.Mani and M.T.Maybury,Eds.,Advances in Automatic Text Summa-rization.Cambridge,MA:MIT Press,1999.[4]J.Alexandersson and P.Poller,“Toward multilingual protocol genera-tion for spontaneous dialogues,”in Proc.INLG-98,Niagara-on-the-lake, Canada,1998.[5]K.Zechner and A.Waibel,“Minimizing word error rate in textual sum-maries of spoken language,”in Proc.NAACL,Seattle,W A,2000.[6]J.S.Garofolo,E.M.V oorhees,C.G.P.Auzanne,and V.M.Stanford,“Spoken document retrieval:1998evaluation and investigation of new metrics,”in Proc.ESCA Workshop:Accessing Information in Spoken Audio,Cambridge,MA,1999,pp.1–7.[7]R.Valenza,T.Robinson,M.Hickey,and R.Tucker,“Summarization ofspoken audio through information extraction,”in Proc.ISCA Workshop on Accessing Information in Spoken Audio,Cambridge,MA,1999,pp.111–116.[8]K.Koumpis and S.Renals,“Transcription and summarization of voice-mail speech,”in Proc.ICSLP2000,2000,pp.688–691.[9]K.Maekawa,H.Koiso,S.Furui,and H.Isahara,“Spontaneous speechcorpus of Japanese,”in Proc.LREC2000,Athens,Greece,2000,pp.947–952.[10]T.Kikuchi,S.Furui,and C.Hori,“Two-stage automatic speech summa-rization by sentence extraction and compaction,”in Proc.ISCA-IEEE Workshop on Spontaneous Speech Processing and Recognition,Tokyo, Japan,2003.[11] C.Hori and S.Furui,“Advances in automatic speech summarization,”in Proc.Eurospeech2001,2001,pp.1771–1774.[12] C.Hori,S.Furui,R.Malkin,H.Yu,and A.Waibel,“A statistical ap-proach to automatic speech summarization,”EURASIP J.Appl.Signal Processing,pp.128–139,2003.[13]K.Knight and D.Marcu,“Summarization beyond sentence extraction:A probabilistic approach to sentence compression,”Artific.Intell.,vol.139,pp.91–107,2002.[14]H.Daume III and D.Marcu,“A noisy-channel model for document com-pression,”in Proc.ACL-2002,Philadelphia,PA,2002,pp.449–456.[15] C.-Y.Lin and E.Hovy,“From single to multi-document summarization:A prototype system and its evaluation,”in Proc.ACL-2002,Philadel-phia,PA,2002,pp.457–464.[16]M.Hirohata,Y.Shinnaka,and S.Furui,“A study on important sentenceextraction methods using SVD for automatic speech summarization,”in Proc.Acoustical Society of Japan Autumn Meeting,Nagoya,Japan, 2003.[17]K.Zechner,“Spoken language condensation in the21st Century,”inProc.Eurospeech,Geneva,Switzerland,2003,pp.1989–1992.。
语言学--2.Speech sounds(课堂PPT)
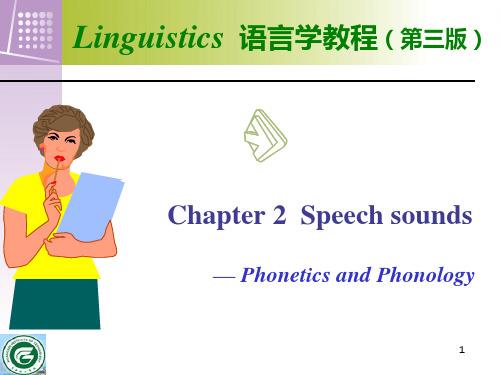
■Phonetics studies how speech sounds are produced, transmitted, and perceived.
2020/4/4
李金妹制作
10
The diagram of speech organs
1. Lips 2. Teeth 3. Teeth ridge (alveolar)齿龈 4. Hard palate 硬腭 5. Soft palate (velum) 软腭 6. Uvula 小舌 7. Tip of tongue 8. Blade of tongue 舌面 9. Back of tongue 10. Vocal cords 声带 11. Pharyngeal cavity 咽腔 12. Nasal cavity 鼻腔
• Auditory phonetics----from the hearers’ point of
view, “how sounds are perceived” 听觉语音学
• Acoustic phonetics----from the physical way or
means by which sounds are transmitted from one
idea about phonetics and phonology
Teaching Focus: description of consonants and vowels;
basic knowledge about phonology
常用光学期刊缩写

光学与应用光学等领域常用期刊英文缩写Acta Optica SinicaActa Photonica SinicaAIP CONFERENCE PROCEEDINGSAIP CONF PROCAPPLIED OPTICSAPPL. OPTICSAPPLIED PHYSICS LETTERSAPPL PHYS LETTChinese Journal of LasersChinese J. LasersHigh Power Laser and Particle BeamsIEEE AEROSPACE AND ELECTRONIC SYSTEMS MAGAZINEIEEE AERO EL SYS MAGIEEE ANNALS OF THE HISTORY OF COMPUTINGIEEE ANN HIST COMPUTIEEE ANTENNAS AND PROPAGATION MAGAZINEIEEE ANTENNAS PROPAGIEEE CIRCUITS & DEVICESIEEE CIRCUITS DEVICEIEEE CIRCUITS AND DEVICES MAGAZINEIEEE CIRCUIT DEVICIEEE COMMUNICATIONS LETTERSIEEE COMMUN LETTIEEE COMMUNICATIONS MAGAZINEIEEE COMMUN MAGIEEE COMPUTATIONAL SCIENCE & ENGINEERINGIEEE COMPUT SCI ENGIEEE COMPUTER APPLICATIONS IN POWERIEEE COMPUT APPL POWIEEE COMPUTER GRAPHICS AND APPLICATIONSIEEE COMPUT GRAPHIEEE COMPUTER GROUP NEWSIEEE COMPUT GROUP NIEEE CONCURRENCYIEEE CONCURRIEEE CONTROL SYSTEMS MAGAZINEIEEE CONTR SYST MAGIEEE DESIGN & TEST OF COMPUTERSIEEE DES TEST COMPUTIEEE ELECTRICAL INSULATION MAGAZINEIEEE ELECTR INSUL MIEEE ELECTROMAGNETIC COMPATIBILITY SYMPOSIUM RECORD IEEE ELECTROMAN COMPIEEE ELECTRON DEVICE LETTERSIEEE ELECTR DEVICE LIEEE ENGINEERING IN MEDICINE AND BIOLOGY MAGAZINE IEEE ENG MED BIOLIEEE EXPERT-INTELLIGENT SYSTEMS & THEIR APPLICATIONS IEEE EXPERTIEEE INDUSTRY APPLICATIONS MAGAZINEIEEE IND APPL MAGIEEE INSTRUMENTATION & MEASUREMENT MAGAZINEIEEE INSTRU MEAS MAGIEEE INTELLIGENT SYSTEMS & THEIR APPLICATIONSIEEE INTELL SYST APPIEEE INTERNET COMPUTINGIEEE INTERNET COMPUTIEEE JOURNAL OF OCEANIC ENGINEERINGIEEE J OCEANIC ENGIEEE JOURNAL OF QUANTUM ELECTRONICSIEEE J QUANTUM ELECTIEEE JOURNAL OF ROBOTICS AND AUTOMATIONIEEE T ROBOTIC AUTOMIEEE JOURNAL OF SELECTED TOPICS IN QUANTUM ELECTRONICS IEEE J SEL TOP QUANTIEEE JOURNAL OF SOLID-STATE CIRCUITSIEEE J SOLID-ST CIRCIEEE JOURNAL ON SELECTED AREAS IN COMMUNICATIONSIEEE J SEL AREA COMMIEEE MICROIEEE MICROIEEE MICROWAVE AND GUIDED WAVE LETTERSIEEE MICROW GUIDED WIEEE MULTIMEDIAIEEE MULTIMEDIAIEEE NETWORKIEEE NETWORKIEEE PARALLEL & DISTRIBUTED TECHNOLOGYIEEE PARALL DISTRIBIEEE PERSONAL COMMUNICATIONSIEEE PERS COMMUNIEEE PHOTONICS TECHNOLOGY LETTERSIEEE PHOTONIC TECH LIEEE ROBOTICS & AUTOMATION MAGAZINEIEEE ROBOT AUTOM MAGIEEE SIGNAL PROCESSING LETTERSIEEE SIGNAL PROC LETIEEE SIGNAL PROCESSING MAGAZINEIEEE SIGNAL PROC MAGIEEE SOFTWAREIEEE SOFTWAREIEEE SPECTRUMIEEE SPECTRUMIEEE TECHNOLOGY AND SOCIETY MAGAZINEIEEE TECHNOL SOC MAGIEEE TRANSACTIONS ON ACOUSTICS SPEECH AND SIGNAL PROCESSING IEEE T ACOUST SPEECHIEEE TRANSACTIONS ON ADVANCED PACKAGINGIEEE TRANS ADV PACKIEEE TRANSACTIONS ON AEROSPACEIEEE T AEROSPIEEE TRANSACTIONS ON AEROSPACE AND ELECTRONIC SYSTEMSIEEE T AERO ELEC SYSIEEE TRANSACTIONS ON AEROSPACE AND NAVAL ELECTRONICSIEEE T AERO NAV ELECIEEE TRANSACTIONS ON AEROSPACE AND NAVIGATIONAL ELECTRONICS IEEE TRANS AEROSP NIEEE TRANSACTIONS ON ANTENNAS AND PROPAGATIONIEEE T ANTENN PROPAGIEEE TRANSACTIONS ON APPLICATIONS AND INDUSTRYIEEE T APPL INDIEEE TRANSACTIONS ON APPLIED SUPERCONDUCTIVITYIEEE T APPL SUPERCONIEEE TRANSACTIONS ON AUDIOIEEE TRANS AUDIOIEEE TRANSACTIONS ON AUDIO AND ELECTROACOUSTICSIEEE T ACOUST SPEECHIEEE TRANSACTIONS ON AUTOMATIC CONTROLIEEE T AUTOMAT CONTRIEEE TRANSACTIONS ON BIOMEDICAL ENGINEERINGIEEE T BIO-MED ENGIEEE TRANSACTIONS ON BROADCAST AND TELEVISION RECEIVERS IEEE T BROADC TELEVIEEE TRANSACTIONS ON BROADCASTINGIEEE T BROADCASTIEEE TRANSACTIONS ON CIRCUIT THEORYIEEE T CIRCUITS SYSTIEEE TRANSACTIONS ON CIRCUITS AND SYSTEMSIEEE T CIRCUITS SYSTIEEE TRANSACTIONS ON CIRCUITS AND SYSTEMS FOR VIDEO TECHNOLOGYIEEE T CIRC SYST VIDIEEE TRANSACTIONS ON CIRCUITS AND SYSTEMS I-FUNDAMENTAL THEORY AND APPLICATIONSIEEE T CIRCUITS-IIEEE TRANSACTIONS ON CIRCUITS AND SYSTEMS II-ANALOG AND DIGITAL SIGNAL PROCESSINGIEEE T CIRCUITS-IIIEEE TRANSACTIONS ON COMMUNICATION AND ELECTRONICSIEEE T COMMUN ELECTRIEEE TRANSACTIONS ON COMMUNICATION TECHNOLOGYIEEE T COMMUN TECHNIEEE TRANSACTIONS ON COMMUNICATIONSIEEE T COMMUNIEEE TRANSACTIONS ON COMMUNICATIONS SYSTEMSIEEE T COMMUN SYSTIEEE TRANSACTIONS ON COMPONENT PARTSIEEE T COMPON PARTSIEEE TRANSACTIONS ON COMPONENTS AND PACKAGING TECHNOLOGIESIEEE T COMPON PACK TIEEE TRANSACTIONS ON COMPONENTS HYBRIDS AND MANUFACTURING TECHNOLOGYIEEE T COMPON HYBRIEEE TRANSACTIONS ON COMPONENTS PACKAGING AND MANUFACTURING TECHNOLOGY PART AIEEE T COMPON PACK AIEEE TRANSACTIONS ON COMPONENTS PACKAGING AND MANUFACTURING TECHNOLOGY PART B-ADVANCED PACKAGINGIEEE T COMPON PACK BIEEE TRANSACTIONS ON COMPUTER-AIDED DESIGN OF INTEGRATED CIRCUITS AND SYSTEMSIEEE T COMPUT AID DIEEE TRANSACTIONS ON COMPUTERSIEEE T COMPUTIEEE TRANSACTIONS ON CONSUMER ELECTRONICSIEEE T CONSUM ELECTRIEEE TRANSACTIONS ON CONTROL SYSTEMS TECHNOLOGYIEEE T CONTR SYST TIEEE TRANSACTIONS ON DIELECTRICS AND ELECTRICAL INSULATIONIEEE T DIELECT EL INIEEE TRANSACTIONS ON EDUCATIONIEEE T EDUCIEEE TRANSACTIONS ON ELECTRICAL INSULATIONIEEE T ELECTR INSULIEEE TRANSACTIONS ON ELECTROMAGNETIC COMPATIBILITYIEEE T ELECTROMAGN CIEEE TRANSACTIONS ON ELECTRON DEVICESIEEE T ELECTRON DEVIEEE TRANSACTIONS ON ELECTRONIC COMPUTERSIEEE TRANS ELECTRONIEEE TRANSACTIONS ON ELECTRONICS PACKAGING MANUFACTURINGIEEE T ELECTRON PA MIEEE TRANSACTIONS ON ENERGY CONVERSIONIEEE T ENERGY CONVERIEEE TRANSACTIONS ON ENGINEERING MANAGEMENTIEEE T ENG MANAGEIEEE TRANSACTIONS ON ENGINEERING WRITING AND SPEECHIEEE T PROF COMMUNIEEE TRANSACTIONS ON EVOLUTIONARY COMPUTATIONIEEE T EVOLUT COMPUTIEEE TRANSACTIONS ON FUZZY SYSTEMSIEEE T FUZZY SYSTIEEE TRANSACTIONS ON GEOSCIENCE AND REMOTE SENSINGIEEE T GEOSCI REMOTEIEEE TRANSACTIONS ON GEOSCIENCE ELECTRONICSIEEE T GEOSCI ELECTIEEE TRANSACTIONS ON HUMAN FACTORS IN ELECTRONICSIEEE TRANS HUM FACTIEEE TRANSACTIONS ON HUMAN FACTORS IN ENGINEERINGIEEE T HUM FACT ENGIEEE TRANSACTIONS ON IMAGE PROCESSINGIEEE T IMAGE PROCESSIEEE TRANSACTIONS ON INDUSTRIAL ELECTRONICSIEEE T IND ELECTRONIEEE TRANSACTIONS ON INDUSTRIAL ELECTRONICS AND CONTROL INSTRUMENTATIONIEEE T IND EL CON INIEEE TRANSACTIONS ON INDUSTRY AND GENERAL APPLICATIONSIEEE TRANS IND GEN AIEEE TRANSACTIONS ON INDUSTRY APPLICATIONSIEEE T IND APPLIEEE TRANSACTIONS ON INFORMATION TECHNOLOGY IN BIOMEDICINEIEEE TRANSACTIONS ON INFORMATION THEORYIEEE T INFORM THEORYIEEE TRANSACTIONS ON INSTRUMENTATION AND MEASUREMENTIEEE T INSTRUM MEASIEEE TRANSACTIONS ON KNOWLEDGE AND DATA ENGINEERINGIEEE T KNOWL DATA ENIEEE TRANSACTIONS ON MAGNETICSIEEE T MAGNIEEE TRANSACTIONS ON MAN-MACHINE SYSTEMSIEEE T MAN MACHINEIEEE TRANSACTIONS ON MANUFACTURING TECHNOLOGYIEEE T MANUF TECHIEEE TRANSACTIONS ON MEDICAL IMAGINGIEEE T MED IMAGINGIEEE TRANSACTIONS ON MICROWAVE THEORY AND TECHNIQUESIEEE T MICROW THEORYIEEE TRANSACTIONS ON MILITARY ELECTRONICSIEEE T MIL ELECTRONIEEE TRANSACTIONS ON NEURAL NETWORKSIEEE T NEURAL NETWORIEEE TRANSACTIONS ON NUCLEAR SCIENCEIEEE T NUCL SCIIEEE TRANSACTIONS ON PARALLEL AND DISTRIBUTED SYSTEMSIEEE T PARALL DISTRIEEE TRANSACTIONS ON PARTS HYBRIDS AND PACKAGINGIEEE T PARTS HYB PACIEEE TRANSACTIONS ON PARTS MATERIALS AND PACKAGINGIEEE TR PARTS MATERIEEE TRANSACTIONS ON PATTERN ANALYSIS AND MACHINE INTELLIGENCE IEEE T PATTERN ANALIEEE TRANSACTIONS ON PLASMA SCIENCEIEEE T PLASMA SCIIEEE TRANSACTIONS ON POWER APPARATUS AND SYSTEMSIEEE T POWER AP SYSTIEEE TRANSACTIONS ON POWER DELIVERYIEEE T POWER DELIVERIEEE TRANSACTIONS ON POWER ELECTRONICSIEEE T POWER ELECTRIEEE TRANSACTIONS ON POWER SYSTEMSIEEE T POWER SYSTIEEE TRANSACTIONS ON PRODUCT ENGINEERING AND PRODUCTIONIEEE T PROD ENG PRODIEEE TRANSACTIONS ON PROFESSIONAL COMMUNICATIONIEEE TRANSACTIONS ON REHABILITATION ENGINEERINGIEEE T REHABIL ENGIEEE TRANSACTIONS ON RELIABILITYIEEE T RELIABIEEE TRANSACTIONS ON ROBOTICS AND AUTOMATIONIEEE T ROBOTIC AUTOMIEEE TRANSACTIONS ON SEMICONDUCTOR MANUFACTURINGIEEE T SEMICONDUCT MIEEE TRANSACTIONS ON SIGNAL PROCESSINGIEEE T SIGNAL PROCESIEEE TRANSACTIONS ON SOFTWARE ENGINEERINGIEEE T SOFTWARE ENGIEEE TRANSACTIONS ON SONICS AND ULTRASONICSIEEE T SON ULTRASONIEEE TRANSACTIONS ON SPACE ELECTRONICS AND TELEMETRYIEEE T SPACE EL TELIEEE TRANSACTIONS ON SPEECH AND AUDIO PROCESSINGIEEE T SPEECH AUDI PIEEE TRANSACTIONS ON SYSTEMS MAN AND CYBERNETICSIEEE T SYST MAN CYBIEEE TRANSACTIONS ON SYSTEMS MAN AND CYBERNETICS PART A-SYSTEMS AND HUMANSIEEE T SYST MAN CY AIEEE TRANSACTIONS ON SYSTEMS MAN AND CYBERNETICS PART B-CYBERNETICSIEEE T SYST MAN CY BIEEE TRANSACTIONS ON SYSTEMS MAN AND CYBERNETICS PART C-APPLICATIONS AND REVIEWSIEEE T SYST MAN CY CIEEE TRANSACTIONS ON SYSTEMS SCIENCE AND CYBERNETICSIEEE T SYST SCI CYBIEEE TRANSACTIONS ON ULTRASONICS FERROELECTRICS AND FREQUENCY CONTROLIEEE T ULTRASON FERRIEEE TRANSACTIONS ON VEHICULAR COMMUNICATIONSIEEE T VEH COMMUNIEEE TRANSACTIONS ON VEHICULAR TECHNOLOGYIEEE T VEH TECHNOLIEEE TRANSACTIONS ON VERY LARGE SCALE INTEGRATION VLSI SYSTEMSIEEE T VLSI SYSTIEEE TRANSACTIONS ON VISUALIZATION AND COMPUTER GRAPHICSIEEE T VIS COMPUT GRIEEE VEHICULAR TECHNOLOGY GROUP-ANNUAL CONFERENCEIEEE VEH TECHNOL GRIEEE-ACM TRANSACTIONS ON NETWORKINGIEEE ACM T NETWORKIEEE-ASME TRANSACTIONS ON MECHATRONICSIEEE-ASME T MECHJournal of Optoelectronics . LaserJ. Optoelectronics . LaserJOURNAL OF THE OPTICAL SOCIETY OF AMERICAJ OPT SOC AMJOURNAL OF THE OPTICAL SOCIETY OF AMERICA A-OPTICS IMAGE SCIENCE AND VISION J OPT SOC AM AJOURNAL OF THE OPTICAL SOCIETY OF AMERICA B-OPTICAL PHYSICSJ OPT SOC AM BOPTICAL TECHNOLOGYOPT. TECHNOL.OPTICS LETTERSOPT LETTOPTICAL TECHNICSOPT. TECH.OPTICS AND PRECISION ENGINEERINGOPT. PRECISION ENG.OPTICA ACTAOPT ACTAOPTICA APPLICATAOPT APPLOPTICAL AND QUANTUM ELECTRONICSOPT QUANT ELECTRONOPTICAL ENGINEERINGOPT ENGOPTICAL FIBER TECHNOLOGYOPT FIBER TECHNOLOPTICAL IMAGING OF BRAIN FUNCTION AND METABOLISM 2 ADV EXP MED BIOLOPTICAL INFORMATION SYSTEMSOPT INF SYSTOPTICAL MATERIALSOPT MATEROPTICAL PROPERTIES OF SEMICONDUCTOR QUANTUM DOTS SPRINGER TR MOD PHYSOPTICAL REVIEWOPT REVOPTICAL SPECTRAOPT SPECTRAOPTICS & PHOTONICS NEWSOPT PHOTONICS NEWSOPTICS AND LASER TECHNOLOGYOPT LASER TECHNOLOPTICS AND LASERS IN ENGINEERINGOPT LASER ENGOPTICS AND SPECTROSCOPYOPT SPECTROSC+OPTICS AND SPECTROSCOPY-USSROPT SPECTROSC-USSROPTICS COMMUNICATIONSOPT COMMUNOPTICS EXPRESSOPT EXPRESSOPTICS LETTERSOPT LETTOPTIKOPTIKOPTIKA I SPEKTROSKOPIYAOPT SPEKTROSK+PATTERN ANALYSIS AND APPLICATIONS PATTERN ANAL APPLPATTERN FORMATION IN GRANULAR MATERIALS SPRINGER TR MOD PHYSPATTERN RECOGNITIONPATTERN RECOGNPATTERN RECOGNITION LETTERSPATTERN RECOGN LETTPROGRESS IN OPTICSPROG OPTICSPROGRESS IN OPTICS, VOL 33PROG OPTICSPROGRESS IN OPTICS, VOL 35PROG OPTICSPROGRESS IN OPTICS, VOL 38PROG OPTICSPROGRESS IN OPTICS, VOL XLPROG OPTICSPROGRESS IN OPTICS, VOL XXXIIPROG OPTICSPROGRESS IN OPTICS, VOL XXXIXPROG OPTICSPROGRESS IN OPTICS, VOL XXXVIPROG OPTICSPROGRESS IN OPTICS, VOL. 37PROG OPTICSSpacecraft Recovery & Remote SensingSOLAR ENERGY MATERIALSSOL ENERG MATERSOLAR ENERGY MATERIALS AND SOLAR CELLS SOL ENERG MAT SOL CVISION RESEARCHVISION RESVISION TECNOLOGICAVIS TECNOL。
C语言常见基本词汇及词汇解释
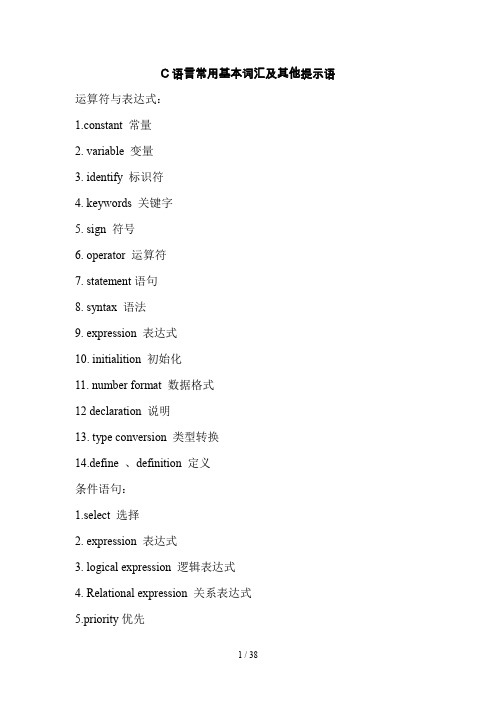
C语言常用基本词汇及其他提示语运算符与表达式:1.constant 常量2. variable 变量3. identify 标识符4. keywords 关键字5. sign 符号6. operator 运算符7. statement语句8. syntax 语法9. expression 表达式10. initialition 初始化11. number format 数据格式12 declaration 说明13. type conversion 类型转换14.define 、definition 定义条件语句:1.select 选择2. expression 表达式3. logical expression 逻辑表达式4. Relational expression 关系表达式5.priority优先6. operation运算7.structure 结构循环语句:1.circle 循环2. condition 条件3. variant 变量4. process过程5.priority优先6. operation运算数组:1. array 数组2. reference 引用3. element 元素4. address 地址5. sort 排序6. character 字符7. string 字符串8. application 应用函数:1.call 调用2.return value 返回值3.function 函数4. declare 声明5. `parameter 参数6.static 静态的7.extern 外部的指针:1. pointer 指针2. argument 参数3. array 数组4. declaration 声明5. represent 表示6. manipulate 处理结构体、共用体、链表:1 structure 结构2 member成员3 tag 标记4 function 函数5 enumerate 枚举6 union 联合(共用体)7 create 创建8 insert 插入9 delete 删除10 modify 修改文件:1、file 文件2、open 打开3、close 关闭4、read 读5、write 写6、error 错误序号主要章节常用英汉对照词汇备注1 运算符与表达式(operator and expression )汉语英语常量constant变量variable标识符identify关键字keywords符号sign运算符operator语句statement语法syntax表达式Expression初始化Initialization数据格式number format说明Declaration类型转换type conversion定义Define 、definition2 条件语句(conditionstatement) 选择select表达式expression逻辑表达式logical expression关系表达式Relational expression 优先priority运算operation结构structure3 循环语句(circle statement) 循环circle条件condition变量variant过程process优先priority运算operation4 函数(function) 调用call返回值return value函数function声明declare参数parameter静态的static外部的extern5 数组和指针(array and pointer) 数组array 引用reference元素element地址address排序sort字符character字符串string应用application指针pointer参数argument数组array声明declaration表示represent处理manipulate6 结构体、共用体(structures 、union )结构structure 成员member标记tag函数function枚举enumerate联合( 共用体) union创建create插入insert删除delete修改modify7 文件(file) 文件file打开open关闭close读read写write错误errorProgram Design 程序设计writing program 编写程序standardize vt.使标准化coding the program 编程simplify vt.单一化,简单化programming 程序revision n.校订,修正programmer n.程序员occupy vt.占领,住进logic n.逻辑,逻辑学BASIC 初学者通用符号指令代码machine code 机器代码teaching language 教学语言debug n.DOS命令,调试simplicity n.单纯,简朴compactness a.紧凑的,紧密的timesharing system 分时系统description n.描述,说明interactive language 交互式语言break n.中断manufacturer n.制造业者structure chart 结构图dialect n.方言,语调the program flow 程序流expense n.费用,代价manager module 管理模块uniformity n.同样,划一worder module 工作模块archaic a.己废的,古老的mainmodule 主模块sufficient a.充分的,足够的submodule 子模块data processing 数据处理modify v.修正,修改business application 商业应用outline n.轮廓,概要scientific application 科学应用compose分解lexical a.字典的,词汇的code 代码non-programmer n.非编程人员node vt改为密码notation n.记号法,表示法,注释pseudocode n.伪代码verbosity n.唠叨,冗长commas n.逗点逗号record n.记录documentation 文档subrecord n.子记录flowchart/flow 程表/流程data division 数据部visual a.视觉的procedure division 过程部represent vt.表现,表示,代表comprise vt.包含构成structured techniques结构化技术operator n.运算符,算子straightforward a.笔直的,率直的commercial package 商业软件包subroutine n.子程序generator n.产生器,生产者driver module 驱动模块mathematician n.专家line by line 逐行operator n.作符translate vt.翻译,解释forerunner n.先驱modular 摸块化ancestor n.祖宗cumbersome a.讨厌的,麻烦的teaching programming 编程教学lengthy a.冗长的,漫长的alter vi./vt.改变flaw n.缺点裂纹devclop vt.发达separate a.各别的recompile v.编译assist n.帮助cycle n.循环technician n.技师remove vt.移动,除去straight line 直线category n.种类,类项rectangle n.长方形,矩形P-code p代码virtrally ad.事实上symology n.象征学象征的使用register n.寄存器to summaries 总之,总而言之by convention 按照惯例cyptic n.含义模糊的,隐藏的diamond-shaped a,菱形的bracket n.括号decision n判断obviate 除去,排除terminal n. a终端机,终端的keyword n.关键字card reader 阅读器underline vt.下划线translator program 译程序monadic a. monad(单位)的Programming 程序设计dec/binary n.二进制source language 源语shift 变化,转移,移位machine language 机器overflow n.溢出machine instruction 机器指令arithmetic n.算术,算法computer language 计算机语composite symbol 复合型符号.assembly language 汇编语assignment n.赋值floating point number浮点数proliferation n.增服high-level language高级语pointer n.指针natural language 自然语言array n.数组矩阵,source text 源文本subscript n.下标intermediate language 中间语言type conversion 类型转换software development 软件开发address arithmetic 地址运算map vt.映射,计划denote vt.指示,表示maintenance cost 维护费用subprogram n.子程序legibility n.易读性,易识别separate compilation 分离式编泽amend vt.修正,改善alphabetic a.照字母次序的consumer n.消费者digit n.数字位数enormous a.巨大的,庞大的numeric expression 数值表达式reliability n.可信赖性,可信度tap n.轻打,轻敲,选择safety n.安全,安全设备print zone 打印区property n.财产,所有权column n.列correctness n.正确,functionality n.机能semicolon n.分号portable a.叮携带的,可搬运的survey n.概观.altoggle n.肘节开关task n.作,任务declaration n.宣告说明source program 源程序mufti-dimension array 多维数组object program 目标程序其他提示语:CPU(Center Processor Unit)中央处理单元mainboard主板RAM(random accessmemory)随机存储器(内存)ROM(Read Only Memory)只读存储器Floppy Disk软盘Hard Disk硬盘CD-ROM光盘驱动器(光驱)monitor监视器keyboard键盘mouse鼠标chip芯片CD-R光盘刻录机HUB集线器Modem= MOdulator-DEModulator,调制解调器P-P(Plug and Play)即插即用UPS(Uninterruptable Power Supply)不间断电源BIOS(Basic-input-OutputSystem)基本输入输出系统CMOS(Complementary Metal-Oxide-Semiconductor)互补金属氧化物半导体setup安装uninstall卸载wizzard向导OS(Operation Systrem)操作系统OA(Office AutoMation)办公自动化exit退出edit编辑copy复制cut剪切paste粘贴delete删除select选择find查找select all全选replace替换undo撤消redo重做program程序license许可(证)back前一步next下一步finish结束folder文件夹Destination Folder目的文件夹user用户click点击double click双击right click右击settings设置update更新release发布data数据data base数据库DBMS(Data Base Manege System)数据库管理系统view视图insert插入object对象configuration配置command命令document文档POST(power-on-self-test)电源自检程序cursor光标attribute属性icon图标service pack服务补丁option pack功能补丁Demo演示short cut快捷方式exception异常debug调试previous前一个column行row列restart重新启动text文本font字体size大小scale比例interface界面function函数access访问manual指南active激活computer language计算机语言menu菜单GUI(graphical user interfaces )图形用户界面template模版page setup页面设置password口令code密码print preview打印预览zoom in放大zoom out缩小pan漫游cruise漫游full screen全屏tool bar工具条status bar状态条ruler标尺table表paragraph段落symbol符号style风格execute执行graphics图形image图像Unix用于服务器的一种操作系统Mac OS苹果公司开发的操作系统OO(Object-Oriented)面向对象virus病毒file文件open打开colse关闭new新建save保存exit退出clear清除default默认LAN局域网WAN广域网Client/Server客户机/服务器ATM( AsynchronousTransfer Mode)异步传输模式Windows NT微软公司的网络操作系统Internet互联网WWW(World Wide Web)万维网protocol协议HTTP超文本传输协议FTP文件传输协议Browser浏览器homepage主页Webpage网页website网站URL在Internet的WWW服务程序上用于指定信息位置的表示方法Online在线Email电子邮件ICQ网上寻呼Firewall防火墙Gateway网关HTML超文本标识语言hypertext超文本hyperlink超级链接IP(Address)互联网协议(地址)SearchEngine搜索引擎TCP/IP用于网络的一组通讯协议Telnet远程登录IE(Internet Explorer)探索者(微软公司的网络浏览器) Navigator引航者(网景公司的浏览器)multimedia多媒体ISO国际标准化组织ANSI美国国家标准协会able 能activefile 活动文件addwatch 添加监视点allfiles 所有文件allrightsreserved 所有的权力保留altdirlst 切换目录格式andfixamuchwiderrangeofdiskproblems 并能够解决更大范围内的磁盘问题andotherinFORMation 以及其它的信息archivefileattribute 归档文件属性assignto 指定到autoanswer 自动应答autodetect 自动检测autoindent 自动缩进autosave 自动存储availableonvolume 该盘剩余空间badcommand 命令错badcommandorfilename 命令或文件名错batchparameters 批处理参数binaryfile 二进制文件binaryfiles 二进制文件borlandinternational borland国际公司bottommargin 页下空白bydate 按日期byextension 按扩展名byname 按名称bytesfree 字节空闲callstack 调用栈casesensitive 区分大小写causespromptingtoconfirmyouwanttooverwritean 要求出现确认提示,在你想覆盖一个centralpointsoftwareinc central point 软件股份公司changedirectory 更换目录changedrive 改变驱动器changename 更改名称characterset 字符集checkingfor 正在检查checksadiskanddisplaysastatusreport 检查磁盘并显示一个状态报告chgdrivepath 改变盘/路径node 节点npasswd UNIX的一种代理密码检查器,在提交给密码文件前,它将对潜在的密码进行筛选。
英语信息化处理
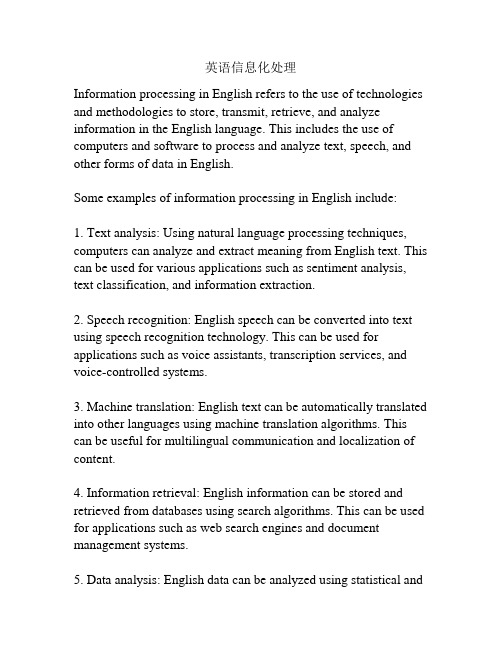
英语信息化处理Information processing in English refers to the use of technologies and methodologies to store, transmit, retrieve, and analyze information in the English language. This includes the use of computers and software to process and analyze text, speech, and other forms of data in English.Some examples of information processing in English include:1. Text analysis: Using natural language processing techniques, computers can analyze and extract meaning from English text. This can be used for various applications such as sentiment analysis, text classification, and information extraction.2. Speech recognition: English speech can be converted into text using speech recognition technology. This can be used for applications such as voice assistants, transcription services, and voice-controlled systems.3. Machine translation: English text can be automatically translated into other languages using machine translation algorithms. This can be useful for multilingual communication and localization of content.4. Information retrieval: English information can be stored and retrieved from databases using search algorithms. This can be used for applications such as web search engines and document management systems.5. Data analysis: English data can be analyzed using statistical anddata mining techniques to extract useful insights and patterns. This can be used for applications such as market research, customer segmentation, and predictive analytics.Overall, information processing in English plays a crucial role in enabling communication, analysis, and decision-making in various domains such as business, education, healthcare, and entertainment.。
计算机的常用英文单词
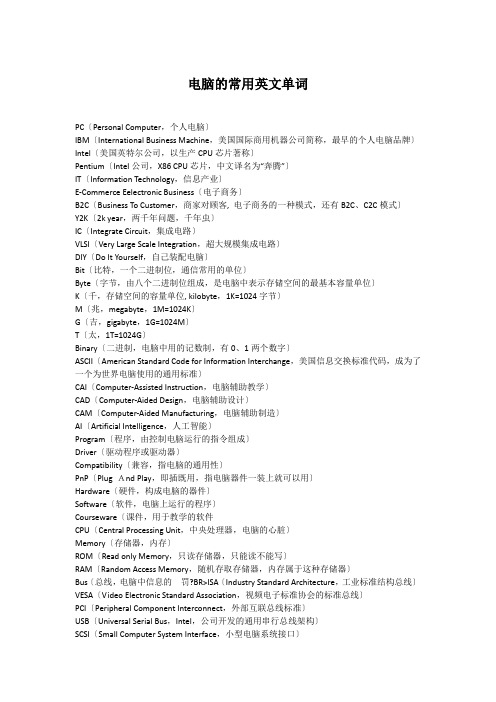
电脑的常用英文单词PC〔Personal Computer,个人电脑〕IBM〔International Business Machine,美国国际商用机器公司简称,最早的个人电脑品牌〕Intel〔美国英特尔公司,以生产CPU芯片著称〕Pentium〔Intel公司,X86 CPU芯片,中文译名为“奔腾”〕IT〔Information Technology,信息产业〕E-Commerce Eelectronic Business〔电子商务〕B2C〔Business To Customer,商家对顾客, 电子商务的一种模式,还有B2C、C2C模式〕Y2K〔2k year,两千年问题,千年虫〕IC〔Integrate Circuit,集成电路〕VLSI〔Very Large Scale Integration,超大规模集成电路〕DIY〔Do It Yourself,自己装配电脑〕Bit〔比特,一个二进制位,通信常用的单位〕Byte〔字节,由八个二进制位组成,是电脑中表示存储空间的最基本容量单位〕K〔千,存储空间的容量单位, kilobyte,1K=1024字节〕M〔兆,megabyte,1M=1024K〕G〔吉,gigabyte,1G=1024M〕T〔太,1T=1024G〕Binary〔二进制,电脑中用的记数制,有0、1两个数字〕ASCII〔American Standard Code for Information Interchange,美国信息交换标准代码,成为了一个为世界电脑使用的通用标准〕CAI〔Computer-Assisted Instruction,电脑辅助教学〕CAD〔Computer-Aided Design,电脑辅助设计〕CAM〔Computer-Aided Manufacturing,电脑辅助制造〕AI〔Artificial Intelligence,人工智能〕Program〔程序,由控制电脑运行的指令组成〕Driver〔驱动程序或驱动器〕Compatibility〔兼容,指电脑的通用性〕PnP〔Plug And Play,即插既用,指电脑器件一装上就可以用〕Hardware〔硬件,构成电脑的器件〕Software〔软件,电脑上运行的程序〕Courseware〔课件,用于教学的软件CPU〔Central Processing Unit,中央处理器,电脑的心脏〕Memory〔存储器,内存〕ROM〔Read only Memory,只读存储器,只能读不能写〕RAM〔Random Access Memory,随机存取存储器,内存属于这种存储器〕Bus〔总线,电脑中信息的罚?BR>ISA〔Industry Standard Architecture,工业标准结构总线〕VESA〔Video Electronic Standard Association,视频电子标准协会的标准总线〕PCI〔Peripheral Component Interconnect,外部互联总线标准〕USB〔Universal Serial Bus,Intel,公司开发的通用串行总线架构〕SCSI〔Small Computer System Interface,小型电脑系统接口〕AGP〔Accelerate Graphics Processor,加速图形接口〕Mouse〔鼠标,俗称“鼠”〕Keyboard〔键盘〕CRT〔Cathode Ray Tube,阴极射线管,常指显示屏〕LCD〔Liquid Crystal Display,液晶显示屏〕VGA〔Video Graphics Array,视频图形阵列,一种显示卡〕Resolution〔分辨率〕Printer〔打印机〕Scanner〔扫描仪〕Floppy Disk〔软盘〕Fixed Disk, Hard Disk〔硬盘〕CD〔Compact Disk,光盘〕Adapter〔适配器〔卡〕,俗称“卡”,如声卡、显示卡〕UPS〔Uninterruptible Power System,不间断电源〕LPT〔Line Printer,打印口,并行口〕DPI〔Dots Per Inch,每英寸点数,指打印机的分辨率〕CPS〔Characters Per Second,每秒字符数〕PPM〔Pages Per Minute,每分钟打印页数〕Multimedia〔多媒体,指电脑能综合处理声音、图像、影像、动画、文字等多种媒体〕CD〔Compact Disk,光盘,分为只读光盘和可刻录光盘〕CDR〔Compact Disk Recordable,可刻录光盘〕VCD〔Video CD,视频CD〕Audio〔音频〕Video〔视频〕MPEG〔Moving picture expert Group,运动图像专家组,一种压缩比率较大的活动图像和声音的压缩标准〕BMP〔Bitmap,位图,一种图像格式〕Image〔图像〕Pixel〔像素,图像的一个点〕WAV〔Wave,声波,一种声音格式〕MIDI〔Musical Instrument Digital Interface,乐器数字接口,声卡上有这种接口,用于与乐器相连〕Modem〔调制解调器,也称“猫”,用于把音频信号变成数字信号〕Net〔Network,网络〕WAN〔Wide area network,广域网,指地理上跨越较大范围的跨地区网〕LAN〔Local area network,局域网,地理上局限在小范围,属于一个单位组建的网〕Internet〔互联网、因特网、网际网〕Server〔服务器,网络的核心,信息的集中地〕Client〔客户,指使用电脑的用户〕C/S〔Client/Server,客户机/服务器〕B/S〔Browser/Server,浏览器/服务器,指客户通过浏览器访问服务器的信息〕Workstation〔工作站,连到服务器的单个电脑〕WWW〔World Wide Web,万维网,全球范围的节点〕BBS〔Bulletin Board System,电子布告栏系统〕FTP〔File Transfer Protocol,文件传送协议,用此协议用户通过Internet将一台电脑上的文件传送到另一台电脑上〕HTTP〔Hypertext Transfer Protocol,超文本传输协议WWW服务程序所用的协议〕HTML〔Home Page Marker Language,主页标记语言,用于浏览器浏览显示〕Hub〔网络集线器,提供许多电脑连接的端口〕Router〔路由器,互联网的标准设备,具有判断网络地址、选择路径、实现网络互联的功能〕Gateway〔网关〕TCP/IP〔Transfer Control Protocol/Internet Protocol,传输控制/互联网协议〕NDS〔Domain Name System,域名服务系统〕e-mail〔Electronic Mail,电子邮件〕〔Commerce,商业部门的域名〕.edu〔Education,教育部门的域名〕.net〔网络服务部门的域名〕.org〔Organization,非商业组织的域名〕.gov〔Government,政府部门的域名〕@〔电子邮件中用户名与域名的分隔符,读音为at〕Optics〔光的,Fiber optics 光纤〕ISDN〔Integrated Services Digital Network,综合服务数字网〕DDN〔Defense Data Service,数字数据服务〕Bandwidth〔带宽,网络线路的传输速度〕Broad〔Band 宽带,可同时在多个通道容纳数据,音像信号〕Hacker〔黑客,专门在互联网上到处从事解密、获取信息等非正规活动的不明身份的用户〕CPU(Center Processor Unit)中央处理单元mainboard主板RAM(random accessmemory)随机存储器(内存)ROM(Read Only Memory)只读存储器Floppy Disk软盘Hard Disk硬盘CD-ROM光盘驱动器(光驱)monitor监视器keyboard键盘mouse鼠标chip芯片CD-R光盘刻录机HUB集线器Modem= MOdulator-DEModulator,调制解调器P-P(Plug And Play)即插即用UPS(Uninterruptable Power Supply)不间断电源BIOS(Basic-input-OutputSystem)基本输入输出系统CMOS(Complementary Metal-Oxide-Semiconductor)互补金属氧化物半导体setup安装uninstall卸载wizzard向导OS(Operation Systrem)操作系统OA(Office AutoMation)办公自动化exit退出edit编辑copy复制cut剪切paste粘贴delete删除select选择find查找select all全选replace替换undo撤消redo重做program程序license许可(证)back前一步next下一步finish结束folder文件夹Destination Folder目的文件夹user用户click点击double click双击right click右击settings设置update更新release发布data数据data base数据库DBMS(Data Base Manege System)数据库管理系统view视图insert插入object对象configuration配置command命令document文档POST(power-on-self-test)电源自检程序cursor光标attribute属性icon图标service pack服务补丁option pack功能补丁Demo演示short cut快捷方式exception异常debug调试previous前一个column行row列restart重新启动text文本font字体size大小scale比例interface界面function函数access访问manual指南active激活computer language电脑语言menu菜单GUI(graphical userinterfaces )图形用户界面template模版page setup页面设置password口令code密码print preview打印预览zoom in放大zoom out缩小pan漫游cruise漫游full screen全屏tool bar工具条status bar状态条ruler标尺table表paragraph段落symbol符号style风格execute执行graphics图形image图像Unix用于服务器的一种操作系统Mac OS苹果公司开发的操作系统OO(Object-Oriented)面向对象virus病毒file文件open打开colse关闭new新建save保存exit退出clear清除default默认LAN局域网WAN广域网Client/Server客户机/服务器ATM( AsynchronousTransfer Mode)异步传输模式Windows NT微软公司的网络操作系统Internet互联网WWW(World Wide Web)万维网protocol协议HTTP超文本传输协议FTP文件传输协议Browser浏览器homepage主页Webpage网页website网站URL在Internet的WWW服务程序上用于指定信息位置的表示方法Online在线Email电子邮件ICQ网上寻呼Firewall防火墙Gateway网关HTML超文本标识语言hypertext超文本hyperlink超级链接IP(Address)互联网协议(地址)SearchEngine搜索引擎TCP/IP用于网络的一组通讯协议Telnet远程登录IE(Internet Explorer)探索者(微软公司的网络浏览器) Navigator引航者(网景公司的浏览器)multimedia多媒体ISO国际标准化组织ANSI美国国家标准协会Active-matrix主动距陈AActive-matrix主动距陈Adaptercards适配卡Advancedapplication高级应用Analyticalgraph分析图表Analyze分析Animations动画Applicationsoftware应用软件Arithmeticoperations算术运算Audio-outputdevice音频输出设备Accesstime存取时间access存取accuracy准确性adnetworkcookies广告网络信息记录软件Add-ons附软件Address地址Agents代理Analogsignals模拟信号Applets程序Asynchronouscommunicationsport异步通信端口Attachment附件BBarcode条形码Barcodereader条形码读卡器Basicapplication基础程序Binarycodingschemes二进制译码方案Binarysystem二进制系统Bit比特Browser浏览器Busline总线Backuptapecartridgeunits备份磁带盒单元Bandwidth带宽Bluetooth蓝牙Broadband宽带Browser浏览器Business-to-business企业对企业电子商务Business-to-consumer企业对消费者Bus总线CCables连线Cell单元箱Chainprinter链式打印机Characterandrecognitiondevice字符标识识别设备Chart图表Chassis支架Chip芯片Clarity清晰度Closedarchitecture封闭式体系结构Column列Combinationkey结合键computercompetency电脑能力connectivity连接,结点Continuous-speechrecognitionsystem连续语言识别系统Controlunit操纵单元Cordlessorwirelessmouse无线鼠标Cablemodems有线调制解调器carpaltunnelsyndrome腕骨神经综合症CD-ROM可记录光盘CD-RW可重写光盘CD-R可记录压缩光盘Channel信道Chatgroup谈话群组chlorofluorocarbons(CFCs)]氯氟甲烷Client客户端Coaxialcable同轴电缆coldsite冷战Commerceservers商业服务器Communicationchannel信道Communicationsystems信息系统CompactdiscrewritableCompactdisc光盘computerabuseamendmentsactof19941994电脑滥用法案computercrime电脑犯罪computerethics电脑道德computerfraudandabuseactof1986电脑欺诈和滥用法案computermatchingandprivacyprotectionactof1988电脑查找和隐私保护法案Computernetwork电脑网络computersupportspecialist电脑支持专家computertechnician电脑技术人员computertrainer电脑教师Connectiondevice连接设备Connectivity连接Consumer-to-consumer个人对个人cookies-cutterprograms信息记录截取程序cookies信息记录程序cracker解密高手cumulativetraumadisorder积累性损伤错乱Cybercash电子现金Cyberspace电脑空间cynic愤世嫉俗者DDatabase数据库databasefiles数据库文件Databasemanager数据库管理Databus数据总线Dataprojector数码放映机Desktopsystemunit台式电脑系统单元Destinationfile目标文件Digitalcameras数码照相机Digitalnotebooks数字笔记本Digitalbideocamera数码摄影机Discrete-speechrecognitionsystem不连续语言识别系统Document文档documentfiles文档文件Dot-matrixprinter点矩阵式打印机Dual-scanmonitor双向扫描显示器Dumbterminal非智能终端datasecurity数据安全Datatransmissionspecifications数据传输说明databaseadministrator数据库管理员Dataplay数字播放器Demodulation解调denialofserviceattack拒绝服务攻击Dial-upservice拨号服务Digitalcash数字现金Digitalsignals数字信号Digitalsubscriberline数字用户线路Digitalversatiledisc数字化通用磁盘Digitalvideodisc数字化视频光盘Directaccess直接存取Directorysearch目录搜索disasterrecoveryplan灾难恢复计划Diskcaching磁盘驱动器高速缓存Diskette磁盘Disk磁碟Distributeddataprocessingsystem分部数据处理系统Distributedprocessing分布处理Domaincode域代码Downloading下载DVD数字化通用磁盘DVD-R可写DVDDVD-RAMDVD随机存取器DVD-ROM只读DVDEe-book电子阅读器Expansioncards扩展卡enduser终端用户e-cash电子现金e-commerce电子商务electroniccash电子现金electroniccommerce电子商务electroniccommunicationsprivacyactof1986电子通信隐私法案encrypting加密术energystar能源之星Enterprisecomputing企业计算化environment环境Erasableopticaldisks可擦除式光盘ergonomics人类工程学ethics道德标准Externalmodem外置调制解调器extranet企业外部网FFaxmachine 机Field域Find搜索FireWireportport火线端口Firmware固件FlashRAM闪存Flatbedscanner台式扫描器Flat-panelmonitor纯平显示器floppydisk软盘Formattingtoolbar格式化工具条Formula公式Function函数faircreditreportingactof1970公平信用报告法案Fiber-opticcable光纤电缆Filecompression文件压缩Filedecompression文件解压缩filter过滤firewall防火墙firewall防火墙Fixeddisk固定硬盘Flashmemory闪存Flexibledisk可折叠磁盘Floppies磁盘Floppy-diskcartridge磁盘盒Formatting格式化freedomofinformationactof1970信息自由法案frustrated受挫折Full-duplexcommunication全双通通信GGeneral-purposeapplication通用运用程序Gigahertz千兆赫Graphictablet绘图板greenpc绿色个人电脑Hhandheldcomputer手提电脑Hardcopy硬拷贝harddisk硬盘hardware硬件Help帮助Hostcomputer主机Homepage主页Hyperlink超链接hacker黑客Half-duplexcommunication半双通通信Hard-diskcartridge硬盘盒Hard-diskpack硬盘组Headcrash磁头碰撞header标题helpdeskspecialist帮助办公专家helperapplications帮助软件Hierarchicalnetwork层次型网络historyfile历史文件hits匹配记录horizontalportal横向用户hotsite热战Hybridnetwork混合网络hyperlinks超连接IImagecapturingdevice图像获取设备informationtechnology信息技术Ink-jetprinter墨水喷射印刷机Integratedpackage综合性组件Intelligentterminal智能终端设备Intergratedcircuit集成电路Interfacecards接口卡Internalmodem内部调制解调器internettelephony网络internetterminal互联网终端Identification识别i-drive网络硬盘驱动器illusionofanonymity匿名梦想indexsearch索引搜索informationpushers信息推送器initializing初始化instantmessaging计时信息internalharddisk内置硬盘Internalmodem内部调制解调器Internetharddrive网络硬盘驱动器intranet企业内部网Jjoystick操纵杆Kkeywordsearch关键字搜索Llaserprinter激光打印机Layoutfiles版式文件Lightpen光笔Locate定位Logicaloperations逻辑运算Lands凸面Lineofsightcommunication视影通信Lowbandwidth低带宽lurking潜伏MMainboard主板Marksensing标志检测Mechanicalmouse机械鼠标Memory内存Menu菜单Menubar菜单条Microprocessor微处理器Microseconds微秒Modemcard调制解调器Monitor显示器Motherboard主板Mouse鼠标Multifunctionaldevice多功能设备Magnetictapereels磁带卷Magnetictapestreamers磁带条mailinglist邮件列表Mediumband媒质带宽metasearchengine整合搜索引擎Microwave微波Modem解调器Modulation解调NNetPC网络电脑Networkadaptercard网卡Networkpersonalcomputer网络个人电脑Networkterminal网络终端Notebookcomputer笔记本电脑Notebooksystemunit笔记本系统单元Numericentry数字输入naïve天真的人nationalinformationinfrastructureprotectionactof1996国际信息保护法案nationalserviceprovider全国性服务供给商Networkarchitecture网络体系结构Networkbridge网桥Networkgateway网关networkmanager网络管理员newsgroup新闻组noelectronictheftactof1997无电子盗窃法Node节点Nonvolatilestorage非易失性存储OObjectembedding对象嵌入Objectlinking目标链接Openarchitecture开放式体系结构Opticaldisk光盘Opticalmouse光电鼠标Opticalscanner光电扫描仪Outline大纲off-linebrowsers离线浏览器Onlinestorage联机存储Ppalmtopcomputer掌上电脑Parallelports并行端口Passive-matrix被动矩阵PCcard个人电脑卡Personallaserprinter个人激光打印机Personalvideorecordercard个人视频记录卡Photoprinter照片打印机Pixel像素Platformscanner平版式扫描仪Plotter绘图仪Plugandplay即插即用Plug-inboards插件卡Pointer指示器Pointingstick指示棍Port端口Portablescanner便携式扫描仪Presentationfiles演示文稿Presentationgraphics电子文稿程序Primarystorage主存Procedures规程Processor处理机Programmingcontrollanugage程序控制语言Packets数据包Paralleldatatransmission平行数据传输Peer-to-peernetworksystem得等网络系统person-personauctionsite个人对个人拍卖站点physicalsecurity物理安全Pits凹面plug-in插件程序Polling轮询privacy隐私权proactive主动地programmer程序员Protocols协议provider供给商proxyserver代理服务pullproducts推取程序pushproducts推送程序RRAMcache随机高速缓冲器Range范围Record记录Relationaldatabase关系数据库Replace替换Resolution分辨率Row行Read-only只读Reformatting重组regionalserviceprovider区域性服务供给商repetitivemotioninjury反复性动作损伤reversedirectory反向目录righttofinancialprivacyactof1979财产隐私法案Ringnetwork环形网络SScanner扫描器Search查找Secondarystoragedevice助存储设备Semiconductor半导体Serialports串行端口Server服务器Sharedlaserprinter共享激光打印机Sheet表格Siliconchip硅片Slots插槽Smartcard智能卡Softcopy软拷贝Softwaresuite软件协议Sorting排序分类Sourcefile源文件Special-purposeapplication专用文件Spreadsheet电子数据表Standardtoolbar标准工具栏Supercomputer巨型机Systemcabine系统箱Systemclock时钟Systemsoftware系统软件Satellite/airconnectionservices卫星无线连接服务searchengines搜索引擎searchproviders搜索供给者searchservices搜索服务器Sectors扇区security安全Sendingandreceivingdevices发送接收设备Sequentialaccess顺序存取Serialdatatransmission单向通信signatureline签名档snoopware监控软件softwarecopyrightactof1980软件版权法案softwarepiracy软件盗版Solid-statestorage固态存储器specializedsearchengine专用搜索引擎spiders网页爬虫spike尖峰电压Starnetwork星型网Strategy方案subject主题subscriptionaddress预定地址Superdisk超级磁盘surfing网上冲浪surgeprotector浪涌保护器systemsanalyst系统分析师TTable二维表Telephony 学Televisionboards电视扩展卡Terminal终端Template模板Textentry文本输入Thermalprinter热印刷Thinclient瘦客Togglekey触发键Toolbar工具栏Touchscreen触摸屏Trackball追踪球TVtunercard电视调谐卡Two-statesystem双状态系统technicalwriter技术协作者technostress重压技术telnet远程登录Time-sharingsystem分时系统Topology拓扑结构Tracks磁道traditionalcookies传统的信息记录程序Twistedpair双绞线UUnicode统一字符标准uploading上传usenet世界性新闻组网络VVirtualmemory虚拟内存Videodisplayscreen视频显示屏Voicerecognitionsystem声音识别系统verticalportal纵向门户videoprivacyprotectionactof1988视频隐私权保护法案viruschecker病毒检测程序virus病毒Voiceband音频带宽Volatilestorage易失性存储voltagesurge冲击性电压WWandreader条形码读入Web网络Webappliance环球网设备Webpage网页Websiteaddress网络地址Webterminal环球网终端Webcam摄像头What-ifanalysis假定分析Wirelessrevolution无线革命Word字长Wordprocessing文字处理Wordwrap自动换行Worksheetfile工作表文件webauctions网上拍卖webbroadcasters网络广播webportals门户网站websites网站webstorefrontcreationpackages网上商店创建包webstorefronts网上商店webutilities网上应用程序web-downloadingutilities网页下载应用程序webmasterweb站点管理员web万维网Wirelessmodems无线调制解调器wirelessserviceprovider无线服务供给商worldwideweb万维网worm蠕虫病毒Write-protectnotch写保护口其他缩写DVDdigitalbersatile数字化通用光盘ITingormationtechnology信息技术CDcompactdisc压缩盘PDApersonaldigitalassistant个人数字助理RAMrandomaccessmemory随机存储器WWWWorldWideWeb万维网DBMSdatabasemanagementsystem数据库管理系统HTMLHypertextMarkupLanguage超文本标示语言OLEobjectlinkingandembedding对象链接潜入SQLstructuredquerylanguage结构化查询语言URLuniformresouicelocator统一资源定位器AGPacceleratedgraphicsport加速图形接口ALUarithmetic-logicunit算术逻辑单元CPUcentralprocessingunit中央处理器CMOScomplementarymetal-oxidesemiconductor互补金属氧化物半导体CISCcomplexinstructionsetcomputer复杂指令集电脑HPSBhighperformanceserialbus高性能串行总线ISAindustrystandardarchitecture工业标准结构体系PCIperipheralcomponentinterconnect外部设备互连总线PCMCIAPersonalMemoryCardInternationalAssociation个人电脑存储卡国际协会RAMrandom-accessmemory随机存储器ROMread-onlymemory只读存储器USBuniversalserialbus通用串行总线CRTcathode-raytube阴极射线管HDTVhigh-definitiontelevision高清晰度电视LCDliquidcrystaldisplaymonitor液晶显示器MICRmagnetic-inkcharacterrecognition磁墨水字符识别器OCRoptical-characterrecognition光电字符识别器OMRoptical-markrecognition光标阅读器TFTthinfilmtransistormonitor薄膜晶体管显示器其他Zipdisk压缩磁盘Domainnamesystem〔DNS〕域名服务器filetransferprotocol(FTP)文件传送协议hypertextmarkuplanguage(HTML)超文本链接标识语言Localareanetwork〔LAN〕局域网internetrelaychat(IRC)互联网多线交谈Metropolitanareanetwork(MAN)城域网Networkoperationsystem(NOS)网络操作系统uniformresourcelocator(URL)统一资源定位器Wideareanetwork(WAN)广域网。
A Cognitive Approach to the Teaching of College En

Sino-US English Teaching, February 2018, Vol. 15, No. 2, 92-96doi:10.17265/1539-8072/2018.02.005 A Cognitive Approach to the Teaching ofCollege English Writing *SHAN Xiao-mingChina University of Petroleum-Beijing (CUPB), Beijing, ChinaOne major objective in the teaching of college English writing is to help students master basic language skills, inthe hope that students will finally learn to write passably well in English. In keeping with the concept that alanguage is a huge system, a cognitive approach to college English writing aims at the link and association ofvarious language elements to help to inspire the formation of the linguistic instinct on the part of the students.Based on the practical teaching practice, ways are recommended to coordinate various elements of a sentence,including “vowel spectrum”, “sentence analyzing spectrum”, and “focus in context”.Keywords: college English writing, a cognitive approach, coordination of “vowel sound spectrum”, “sentenceanalysis spectrum” and “spectrum of focus in context”IntroductionIn order to learn to write in English, students need to have basic language skills in the first place. However,generally speaking, Chinese college students have not mastered satisfactorily basic language skills when they begin to learn to write in English. An official from Ministry of Education has made the remark that “there is still a part of the students who do not understand, can not say and read in English.” (LIU, 2012, p. 44). The fundamental difficulty in learning to write in English lays in the fact that a language is a huge system that comprises of many fields of study. A simplistic or sole approach will never yield the desirous result. A cognitive approach to the teaching of college English writing will be more effective and efficient because it makes use of various fields of study while focusing on the links and association of various language elements. In this way, students will be inspired to form their linguistic instincts to help them learn to write. However, in the practice of a cognitive approach to the teaching of college English writing, most efforts are tentative and exploratory in nature.The present study of a cognitive approach to college English writing starts from the basic cognitive practice and suggests that the most basic elements of the written language are like the musical notation and atomic weight, which can be utilized for the teaching of college English writing. It then moves on to provide a more systematic cognition and study of college English writing, consisting of mainly three parts, “the vowel spectrum ”, “sentence analyzing spectrum”, and “the focus in context”.*Acknowledgements: This paper is sponsored by China University of Petroleum-Beijing (CUPB).SHAN Xiao-ming, associated professor, master, Schools of Foreign Language Studies, China University of Petroleum-Beijing (CUPB), Beijing, China.All Rights Reserved.A COGNITIVE APPROACH TO THE TEACHING OF COLLEGE ENGLISH WRITING93DiscussionBasic language skills are closely related with meta cognition as far as college English writing is concerned.The general metacognition refers to the cognition of the cognitive subject to its own cognitive phenomenon andthe knowledge of cognition, such as the individual factors that affect cognitive processes and outcomes, the waythese factors work. In college English writing, the way to use the basic language skills is a metacognitive strategy.It includes conscious planning, monitoring, and evaluation. In the course of metacognition, the followingquestions are frequently asked: What kind of linguistic basis should English writing have? What language skillshould be mastered to avoid primary mistakes? Is writing purely a matter of language, or depended on interactingwith the reader via language? Which is of greater priority, to develop the ability to read, dictate, speak, andtranslate in a comprehensive way, or to highlight the main text? Should one be guided entirely in writing by his orher sense of language, or should one parse sentences word by word to learn to write? All these questions peopleencounter in writing should be answered with metacognitive knowledge and the answers to these questions areconducive to the development and formation of the linguistic instinct and English writing ability.From “Word Core” to “Five Basic Sentence Patterns”The cognitive process starts from the very basics. Writing involves word, sentence, and text. Each word, sentence, and text has a core. The basis of writing can then be summed up as the coordination of “three cores”.The “word core” includes the “core of meaning ” (word root) and “the core of the sound” (the vowel of stressed syllable). The consciousness of word root is strongly beneficial to the expansion of vocabulary. Theconfusion in spelling out the 20 vowel phonemes from the five vowel letters affects seriously readingcomprehension and ultimately writing.All Rights Reserved.The “Five basic sentence patterns”, a combination of “vowel spectrum”, and “sentence analyzing spectrum ” are suggested in the light of cognition.Firstly, the “Five basic sentence patterns” perform the function of sound spectrum. Each of them representsa vowel letter (including a letter cluster) that can spell out all the different vowels and every phonetic codes. Allthe vowel spectra make use of only 10 symbols: a short line (-) signaling “long open vowel”(ā[ei]ō[əu]ē[I:]ū[ju:][u:] Ī(ӯ)[ai]); a positive hook (ˇ) signaling “short shut vowel” (ǎ[æ]ǒ[ɒ]ě[e]ǔ[ʌ]ǐ(Ў)[i]); ainverted hook (ˆ) and two points (¨) signaling two R tones (â[ɑ:]ô[ɔ:]ô[ɜ:]ê[ɜ:]ê[ɑ:]û[ɜ:]î[ɜ:] orä[eə][ɛə]ö[uə]ë[iə]ë[eə]ü[uə]ï(ÿ)[ai-ə]); left skimming (`), right skimming (´), and middle point (.) are used tosignal three special spelling vowels of each letter, with the exception of letter “o” which is signaled by a wave line(~) (à[ɔ:]á[ɔ]ȧ[e]ó[u:]ò[u]ȯ[ʌ]Õ[au]ǒ[ɔi]è[ju:]é[ei]ė[əu]ù[u]ú[e]ủ[w]ì[i:]í[iə]). If the same letters in differentsyllables spell the same vowel, their symbols are the same. For example, in both the two words “car” and “father”,the letter “a” is considered as the same symbols as [â].Secondly, the “Five basic sentence patterns” reflect the function of sentence element spectrum. All the elements of a sentence are represented differently. This way, we can determine the basic sentence pattern,centering around the predicate (the sentence core) of the sentence.The different elements of a sentence are represented as follows:subject, predicate, object, object clause^|, predicative, predicative clause^\, {attribute}, [adverbial], *attributive clause, # adverbial clause, <object supplement>, appositive= \appositive clause=/, non-predicateA COGNITIVE APPROACH TO THE TEACHING OF COLLEGE ENGLISH WRITING94 verb, \parentheses/ (function words are not marked)By coordinating “vowel spectrum” and “sentence spectrum”, five basic sentence patterns are suggested as follows:(1) Sentence pattern one with “a”: A sentence made of words with letter of “a”. {Some} sn ākes, r ăts and wásps {in mány glâss boxes}[àll] âre put [ăt a .corner] {of the village squäre}—(A sentence pattern of subject+predicate+object in passive voice) (2) Sentence pattern two with “o”: A sentence made of words with letter of “o”. The wôrds \=/ that The n ōse {of a f ŏx}, the tòoth {of a wólf}, the j ŏint {of a m ȯnkey}, the hôrns {of a cÕw} are all impôrtant.{sóme pöor women]{living by a reservöir} said [c ŏmmo .nly]—(A sentence pattern of complex sentence with an appositive clause )(3) Sentence pattern three with “e”: A sentence made of words with letter of “e”. A woman ėntrepre’nêur s ėwed hêr nephèw [hêartily] a sh ēet {with pictures} {of éight diff e re .nt bëars} {nëar an ĕlephant}—(A sentence pattern of subject+predicate+double object)(4) Sentence pattern four with “u”: A sentence made of words with letter of “u”. They have been q ủickly busy [digging something] [in a building of the zoo] [u .ntil now] for some r ūbies {pùt in a pûrse} are [sürely] búried [ŭnder it]—(A sentence pattern of coordinate sentence)(5) Sentence pattern five with “i”: A sentence made of words with letter of “i” . Some Ind ỉan polìcemen {with blue t īes} {in a taxi} carried materíals {for w ĭnder} [in holi .days] [to a fìeld][fri ĕndly] [to make bîrds and lïons] {there} <l ĭve [quïetly]>—(A sentence pattern of subject+predicate+compound object sentence) Contextual Cryptography and Unique Core of WritingAfter the repeated practice and analysis of the five sentence patterns suggested above, in terms of cognition, students will be greatly helped in college English writing. For example, a detailed analysis of a short poem by Keats, with the application of the five sentence patterns, will help them better understand the different sentences and their elements and after sufficient practice, students tend to write in the similar fashion, inspired by the linguistic instinct developed and formed in the course of time. There are only two sentences in the eight lines of the poem (the main clause is in bold).This living hand , \ {now warm and capable}[Of earnest grasping]/, would , \ if it were coldAnd in the Īcy, s Īlence, {of the tomb}/, So hàunt th ӯ days and chill th ӯ dreaming nights #[That thÕu wouldst wish th Īne own heart <dry of blood>]#[So] [in my veins] red life might stream [again], And thÕu be conscience-calmed——see here it is I hold it <towards you>. (DING & ZHU, 1994, p. 13)Commentary:(1) The first line to the seventh line is one sentence. The subject of the sentence is “this living hand”. The predicate is juxtaposed “haunt thy days ”and “chill thy dreaming nights”.All Rights Reserved.A COGNITIVE APPROACH TO THE TEACHING OF COLLEGE ENGLISH WRITING95(2) Before “would” in the second line, there is an adjective phrase to modify “hand”. Behind it is the “ofstructure” as an adverbial modifier, modifying “capable” and meaning “this hand” can grasp one’s feelings.(3) The fifth line is an adverbial clause of purpose to modify the main predicate, meaning “you will expectthe blood of your heart to become thirsty”.(4) The sixth line is an adverbial clause of the result, meaning “I will save your feelings”.(5) The seventh line is the coordinate part of the adverbial clause of the result from the above line, meaning“I am waiting for you here”.(6) The eighth line is the “poetic eyes” (it is equal to the “core of prose”), meaning “Grasp my hand soon,please”.From these sentences, students can go on to coordinate “focus in context”, as can be explained by the following:(a) The nouns and subject, containing five focuses which coordinate: (1) number, (2) article , (3) pronoun, (4)adjective, (5) preposition.For example, in “This living hand”, The noun “hand” is a core of the subject. Using the pronoun “this”instead of article “the” means this hand is a specific hand; it refers to the poet himself. From this we know that thepoet is “capable and warm” to be worthy of love.There are also several attributive nouns in the poem, such as “in the icy silence of the tomb”. Nouns like “tomb” have the connotation of horrifying environment (silence, cold) on the part of the poet’s girlfriend.(b) Verb and predicate, including 10 focuses to coordinate (6) morphology, (7) tense, (8) voice, (9) modality,(10) adverbial, (11) predicative, (12) object, (13) subjunctive mood, (14) non-finite verb, (15) verb phrase.All Rights Reserved.In the poem, the “would” is changed to “wouldst”. It shows that the poet is anxious to face his girlfriend. He hopes she will love him.(c) Clause, with 5 focuses to coordinate: (16) coordinate clauses; (17) noun clause; (18) attributive clauses;(19) adverbial clauses; (20) special clauses.There are more than four clauses in the first sentence of the poem. In accordance with the principles of English writing, the logic focus is in the last clause of the poem. When the poet says, “see here it is”, it means ifnot, his red vein would not stream again. So, he would hold his living hand to his lovely girl as soon as possible.ConclusionThe main function of language is expression and communication. The purpose of writing is to enable the reader to empathize with the work. Otherwise, it can only be parole, not real language. Dukas, an Americanscholar, said:The use of words to express personal intention is to convey it to others, and, if possible, use it to influence the behavior of others. However, this performance may not achieve the purpose of communication, so it still stays at the levelof parole. (Dukas, 1988, p. 27)A cognitive approach to college English writing is to coordinate all aspects of language to test the author’sprecise and accurate cognition of complex things. It is very much in keeping with the concept that language is ahuge system. As far as its signifier series is concerned, it can include pronunciation, vocabulary, grammar, text,and so on; As far as its signified series are concerned, they involve a wider range. The cognitive linguistic schools96A COGNITIVE APPROACH TO THE TEACHING OF COLLEGE ENGLISH WRITINGin recent years mainly study the relationship among language, communication, and cognition. In language,cognition, consciousness, experience, embodiment, the brain, the individual, the human, the society, the culture,and the history are all blended in a rich, complex, and dynamic way. Therefore, language learning involves allaspects of cognition (GUI, 2010, pp. 275-281).Stephen Pinker, a famous cognitive psychologist, affirmed the role of linguistic forms. In his book Language Instinct, he advocated the acceptance of cognitive linguistics on the basis of scientific understanding ofChomsky’s transformational generative grammar. He said, “if I were to comprehensive opposing views on bothsides of the debate, such as formalism and functionalism, syntax, semantics and pragmatics, got inclusiveness forall, this may be because there are no differences between them.” (Pinker, 2015, p. 93). He went on to say:Language instinct is both genetic and inseparable from the environment…… It is a natural learning ability of human being to get induction according to “similarity”. Although we speak different languages, we have the same mentalstructure. Language is a window on human nature. (Pinker, 2015, p. 203)In sum, a cognitive approach to the teaching of college English writing is to enforce the basic language skills by coordinating the various elements of a sentence, in the hope of inspiring the students’ mental structure oflanguage instinct.ReferencesDING, W. D., & ZHU, Q. (Eds.).(1994). Poetry introduction (p. 13). Shanghai Translation Publishing House.Dukas, D. (1988). A new theory of art philosophy (p. 27). Beijing: Guangming Daily Press.GUI, S. C. (2010). Thinking about some problems of foreign language teaching in China. Foreign Language Teaching and Research, 4, 275-281.All Rights Reserved.LIU, G. Q. (2012). Attach great importance to the reform of college English teaching and strive to improve the quality of college English teaching. Foreign Language Teaching and Research,24, 44.Pinker, S. (2015). The evolution of human language instinct: The mystery of language (p. 93; p. 203). China: Zhejiang People’s Press.。
chatgpt for dummies的译文书
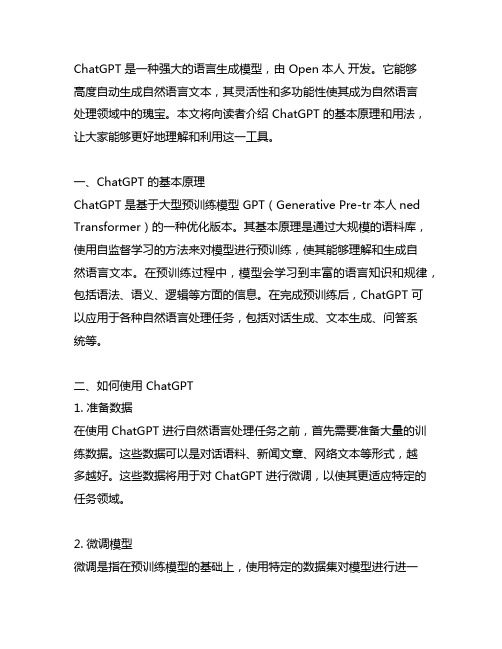
ChatGPT 是一种强大的语言生成模型,由 Open本人开发。
它能够高度自动生成自然语言文本,其灵活性和多功能性使其成为自然语言处理领域中的瑰宝。
本文将向读者介绍 ChatGPT 的基本原理和用法,让大家能够更好地理解和利用这一工具。
一、ChatGPT 的基本原理ChatGPT 是基于大型预训练模型 GPT(Generative Pre-tr本人ned Transformer)的一种优化版本。
其基本原理是通过大规模的语料库,使用自监督学习的方法来对模型进行预训练,使其能够理解和生成自然语言文本。
在预训练过程中,模型会学习到丰富的语言知识和规律,包括语法、语义、逻辑等方面的信息。
在完成预训练后,ChatGPT 可以应用于各种自然语言处理任务,包括对话生成、文本生成、问答系统等。
二、如何使用 ChatGPT1. 准备数据在使用 ChatGPT 进行自然语言处理任务之前,首先需要准备大量的训练数据。
这些数据可以是对话语料、新闻文章、网络文本等形式,越多越好。
这些数据将用于对 ChatGPT 进行微调,以使其更适应特定的任务领域。
2. 微调模型微调是指在预训练模型的基础上,使用特定的数据集对模型进行进一步训练,以适应特定的任务需求。
对 ChatGPT 进行微调可以通过调整模型的参数和超参数来完成,一般需要使用较大规模的计算资源和时间。
微调的目的是让模型学会更好地适应特定的任务,并提高模型在特定任务上的表现。
3. 应用到实际任务中当 ChatGPT 完成微调后,就可以将其应用于实际的自然语言处理任务中。
比如可以用它来生成对话内容、完成文本摘要、进行问答系统等。
在应用过程中,需要根据具体的任务需求来调整模型的参数和输入数据,以获得最佳的效果。
三、ChatGPT 的优势和局限1. 优势ChatGPT 具有以下几个主要优势:- 强大的生成能力。
ChatGPT 在生成自然语言文本方面有着卓越的表现,能够生成通顺、连贯的文本,并且语义准确。
英文文献翻译
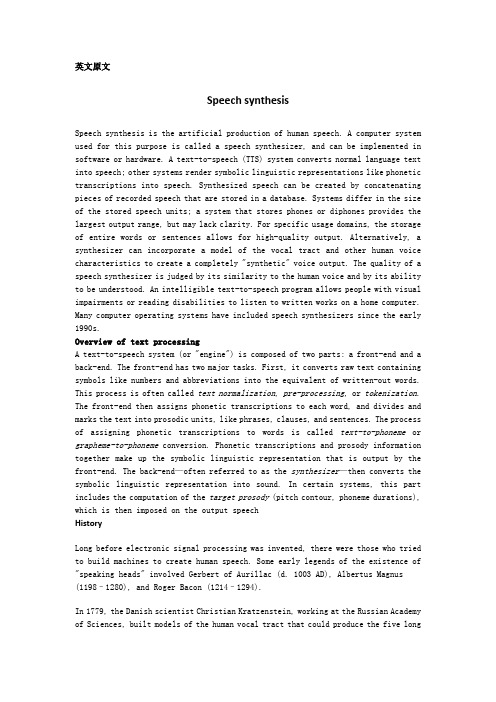
英文原文Speech synthesisSpeech synthesis is the artificial production of human speech. A computer system used for this purpose is called a speech synthesizer, and can be implemented in software or hardware. A text-to-speech (TTS) system converts normal language text into speech; other systems render symbolic linguistic representations like phonetic transcriptions into speech. Synthesized speech can be created by concatenating pieces of recorded speech that are stored in a database. Systems differ in the size of the stored speech units; a system that stores phones or diphones provides the largest output range, but may lack clarity. For specific usage domains, the storage of entire words or sentences allows for high-quality output. Alternatively, a synthesizer can incorporate a model of the vocal tract and other human voice characteristics to create a completely "synthetic" voice output. The quality of a speech synthesizer is judged by its similarity to the human voice and by its ability to be understood. An intelligible text-to-speech program allows people with visual impairments or reading disabilities to listen to written works on a home computer. Many computer operating systems have included speech synthesizers since the early 1990s.Overview of text processingA text-to-speech system (or "engine") is composed of two parts: a front-end and a back-end. The front-end has two major tasks. First, it converts raw text containing symbols like numbers and abbreviations into the equivalent of written-out words. This process is often called text normalization, pre-processing, or tokenization. The front-end then assigns phonetic transcriptions to each word, and divides and marks the text into prosodic units, like phrases, clauses, and sentences. The process of assigning phonetic transcriptions to words is called text-to-phoneme or grapheme-to-phoneme conversion. Phonetic transcriptions and prosody information together make up the symbolic linguistic representation that is output by the front-end. The back-end—often referred to as the synthesizer—then converts the symbolic linguistic representation into sound. In certain systems, this part includes the computation of the target prosody(pitch contour, phoneme durations), which is then imposed on the output speechHistoryLong before electronic signal processing was invented, there were those who tried to build machines to create human speech. Some early legends of the existence of "speaking heads" involved Gerbert of Aurillac (d. 1003 AD), Albertus Magnus (1198–1280), and Roger Bacon (1214–1294).In 1779, the Danish scientist Christian Kratzenstein, working at the Russian Academy of Sciences, built models of the human vocal tract that could produce the five longvowel sounds (in International Phonetic Alphabet notation, they are [aː], [eː], [iː], [oː] and [uː]).[5] This was followed by the bellows-operated"acoustic-mechanical speech machine" by Wolfgang von Kempelen of Pressburg, Hungary, described in a 1791 paper.[6] This machine added models of the tongue and lips, enabling it to produce consonants as well as vowels. In 1837, Charles Wheatstone produced a "speaking machine" based on von Kempelen's design, and in 1857, M. Faber built the "Euphonia". Wheatstone's design was resurrected in 1923 by Paget.In the 1930s, Bell Labs developed the vocoder, which automatically analyzed speech into its fundamental tone and resonances. From his work on the vocoder, Homer Dudley developed a manually keyboard-operated voice synthesizer called The Voder (Voice Demonstrator), which he exhibited at the 1939 New York World's Fair.The Pattern playback was built by Dr. Franklin S. Cooper and his colleagues at Haskins Laboratories in the late 1940s and completed in 1950. There were several different versions of this hardware device but only one currently survives. The machine converts pictures of the acoustic patterns of speech in the form of a spectrogram back into sound. Using this device, Alvin Liberman and colleagues were able to discover acoustic cues for the perception of phonetic segments (consonants and vowels).Dominant systems in the 1980s and 1990s were the MITalk system, based largely on the work of Dennis Klatt at MIT, and the Bell Labs system;[8] the latter was one of the first multilingual language-independent systems, making extensive use of natural language processing methods.Early electronic speech synthesizers sounded robotic and were often barely intelligible. The quality of synthesized speech has steadily improved, but output from contemporary speech synthesis systems is still clearly distinguishable from actual human speech.As the cost-performance ratio causes speech synthesizers to become cheaper and more accessible to the people, more people will benefit from the use of text-to-speech programs.Electronic devicesThe first computer-based speech synthesis systems were created in the late 1950s. The first general English text-to-speech system was developed by Noriko Umeda et al. in 1968 at the Electrotechnical Laboratory, Japan.[10] In 1961, physicist John Larry Kelly, Jr and colleague Louis Gerstman[11] used an IBM 704 computer to synthesize speech, an event among the most prominent in the history of Bell Labs. Kelly's voice recorder synthesizer (vocoder) recreated the song "Daisy Bell", with musical accompaniment from Max Mathews. Coincidentally, Arthur C. Clarke was visiting his friend and colleague John Pierce at the Bell Labs Murray Hill facility. Clarke was so impressed by the demonstration that he used it in the climactic sceneof his screenplay for his novel 2001: A Space Odyssey,Arthur C. Clarke Biography at the Wayback Machine (archived December 11, 1997) where the HAL 9000 computer sings the same song as it is being put to sleep by astronaut Dave Bowman."Where "HAL"First Spoke (Bell Labs Speech Synthesis website)". Bell Labs. /news/1997/march/5/2.html. Retrieved 2010-02-17. Despite the success of purely electronic speech synthesis, research is still being conducted into mechanical speech synthesizers.Anthropomorphic Talking Robot Waseda-Talker SeriesHandheld electronics featuring speech synthesis began emerging in the 1970s. One of the first was the Telesensory Systems Inc. (TSI) Speech+ portable calculator for the blind in 1976.TSI Speech+ & other speaking calculators Gevaryahu, Jonathan, "TSI S14001A Speech Synthesizer LSI Integrated Circuit Guide"[dead link] Other devices were produced primarily for educational purposes, such as Speak & Spell, produced by Texas InstrumentsBreslow, et al. United States Patent 4326710: "Talking electronic game" April 27, 1982 in 1978. Fidelity released a speaking version of its electronic chess computer in 1979.Voice Chess Challenger The first video game to feature speech synthesis was the 1980 shoot 'em up arcade game, Stratovox, from Sun Electronics.Gaming's Most Important Evolutions, GamesRadar Another early example was the arcade version of Bezerk, released that same year. The first multi-player electronic game using voice synthesis was Milton from Milton Bradley Company, which produced the device in 1980.Synthesizer technologiesThe most important qualities of a speech synthesis system are naturalness and intelligibility.[citation needed]Naturalness describes how closely the output sounds like human speech, while intelligibility is the ease with which the output is understood. The ideal speech synthesizer is both natural and intelligible. Speech synthesis systems usually try to maximize both characteristics.The two primary technologies for generating synthetic speech waveforms are concatenative synthesis and formant synthesis. Each technology has strengths and weaknesses, and the intended uses of a synthesis system will typically determine which approach is used.Concatenative synthesisConcatenative synthesis is based on the concatenation (or stringing together) of segments of recorded speech. Generally, concatenative synthesis produces the most natural-sounding synthesized speech. However, differences between natural variations in speech and the nature of the automated techniques for segmenting the waveforms sometimes result in audible glitches in the output. There are three main sub-types of concatenative synthesis.Unit selection synthesisUnit selection synthesis uses large databases of recorded speech. During database creation, each recorded utterance is segmented into some or all of the following: individual phones, diphones, half-phones, syllables, morphemes, words, phrases, and sentences. Typically, the division into segments is done using a specially modifiedspeech recognizer set to a "forced alignment" mode with some manual correction afterward, using visual representations such as the waveform and spectrogram.[12]An index of the units in the speech database is then created based on the segmentation and acoustic parameters like the fundamental frequency (pitch), duration, position in the syllable, and neighboring phones. At run time, the desired target utterance is created by determining the best chain of candidate units from the database (unit selection). This process is typically achieved using a specially weighted decision tree.Unit selection provides the greatest naturalness, because it applies only a small amount of digital signal processing (DSP) to the recorded speech. DSP often makes recorded speech sound less natural, although some systems use a small amount of signal processing at the point of concatenation to smooth the waveform. The output from the best unit-selection systems is often indistinguishable from real human voices, especially in contexts for which the TTS system has been tuned. However, maximum naturalness typically require unit-selection speech databases to be very large, in some systems ranging into the gigabytes of recorded data, representing dozens of hours of speech.[13] Also, unit selection algorithms have been known to select segments from a place that results in less than ideal synthesis (e.g. minor words become unclear) even when a better choice exists in the database.[14]Recently, researchers have proposed various automated methods to detect unnatural segments in unit-selection speech synthesis systems.Diphone synthesisDiphone synthesis uses a minimal speech database containing all the diphones (sound-to-sound transitions) occurring in a language. The number of diphones depends on the phonotactics of the language: for example, Spanish has about 800 diphones, and German about 2500. In diphone synthesis, only one example of each diphone is contained in the speech database. At runtime, the target prosody of a sentence is superimposed on these minimal units by means of digital signal processing techniques such as linear predictive coding, PSOLA[16] or MBROLA.[17] Diphone synthesis suffers from the sonic glitches of concatenative synthesis and the robotic-sounding nature of formant synthesis, and has few of the advantages of either approach other than small size. As such, its use in commercial applications is declining,[citation needed] although it continues to be used in research because there are a number of freely available software implementationsDomain-specific synthesisDomain-specific synthesis concatenates prerecorded words and phrases to create complete utterances. It is used in applications where the variety of texts the system will output is limited to a particular domain, like transit schedule announcements or weather reports.[18] The technology is very simple to implement, and has been in commercial use for a long time, in devices like talking clocks and calculators. The level of naturalness of these systems can be very high because the variety of sentence types is limited, and they closely match the prosody and intonation of the original recordings.Because these systems are limited by the words and phrases in their databases, they are not general-purpose and can only synthesize the combinations of words and phrases with which they have been preprogrammed. The blending of words within naturally spoken language however can still cause problems unless the many variations are taken into account. For example, in non-rhotic dialects of English the "r" in words like "clear" /ˈklɪə/ is usually only pronounced when the following word has a vowel as its first letter (e.g. "clear out" is realized as /ˈklɪəɾˈʌʊt/). Likewise in French, many final consonants become no longer silent if followed by a word that begins with a vowel, an effect called liaison. This alternation cannot be reproduced by a simple word-concatenation system, which would require additional complexity to be context-sensitive.Formant synthesisFormant synthesis does not use human speech samples at runtime. Instead, the synthesized speech output is created using additive synthesis and an acoustic model (physical modelling synthesis).[19]Parameters such as fundamental frequency, voicing, and noise levels are varied over time to create a waveform of artificial speech. This method is sometimes called rules-based synthesis; however, many concatenative systems also have rules-based components. Many systems based on formant synthesis technology generate artificial, robotic-sounding speech that would never be mistaken for human speech. However, maximum naturalness is not always the goal of a speech synthesis system, and formant synthesis systems have advantages over concatenative systems. Formant-synthesized speech can be reliably intelligible, even at very high speeds, avoiding the acoustic glitches that commonly plague concatenative systems. High-speed synthesized speech is used by the visually impaired to quickly navigate computers using a screen reader. Formant synthesizers are usually smaller programs than concatenative systems because they do not have a database of speech samples. They can therefore be used in embedded systems, where memory and microprocessor power are especially limited. Because formant-based systems have complete control of all aspects of the output speech, a wide variety of prosodies and intonations can be output, conveying not just questions and statements, but a variety of emotions and tones of voice.Examples of non-real-time but highly accurate intonation control in formant synthesis include the work done in the late 1970s for the Texas Instruments toy Speak & Spell, and in the early 1980s Sega arcade machines[20]and in many Atari, Inc. arcade games[21]using the TMS5220 LPC Chips. Creating proper intonation for these projects was painstaking, and the results have yet to be matched by real-time text-to-speech interface.Articulatory synthesisArticulatory synthesis refers to computational techniques for synthesizing speech based on models of the human vocal tract and the articulation processes occurringthere. The first articulatory synthesizer regularly used for laboratory experiments was developed at Haskins Laboratories in the mid-1970s by Philip Rubin, Tom Baer, and Paul Mermelstein. This synthesizer, known as ASY, was based on vocal tract models developed at Bell Laboratories in the 1960s and 1970s by Paul Mermelstein, Cecil Coker, and colleagues.Until recently, articulatory synthesis models have not been incorporated into commercial speech synthesis systems. A notable exception is the NeXT-based system originally developed and marketed by Trillium Sound Research, a spin-off company of the University of Calgary, where much of the original research was conducted. Following the demise of the various incarnations of NeXT (started by Steve Jobs in the late 1980s and merged with Apple Computer in 1997), the Trillium software was published under the GNU General Public License, with work continuing as gnuspeech. The system, first marketed in 1994, provides full articulatory-based text-to-speech conversion using a waveguide or transmission-line analog of the human oral and nasal tracts controlled by Carré's "distinctive region model".HMM-based synthesisHMM-based synthesis is a synthesis method based on hidden Markov models, also called Statistical Parametric Synthesis. In this system, the frequency spectrum (vocal tract), fundamental frequency (vocal source), and duration (prosody) of speech are modeled simultaneously by HMMs. Speech waveforms are generated from HMMs themselves based on the maximum likelihood criterionSinewave synthesisSinewave synthesis is a technique for synthesizing speech by replacing the formants (main bands of energy) with pure tone whistlesChallengesText normalization challengesThe process of normalizing text is rarely straightforward. Texts are full of heteronyms, numbers, and abbreviations that all require expansion into a phonetic representation. There are many spellings in English which are pronounced differently based on context. For example, "My latest project is to learn how to better project my voice" contains two pronunciations of "project".Most text-to-speech (TTS) systems do not generate semantic representations of their input texts, as processes for doing so are not reliable, well understood, or computationally effective. As a result, various heuristic techniques are used to guess the proper way to disambiguate homographs, like examining neighboring words and using statistics about frequency of occurrence.Recently TTS systems have begun to use HMMs (discussed above) to generate "parts of speech" to aid in disambiguating homographs. This technique is quite successful for many cases such as whether "read" should be pronounced as "red" implying past tense, or as "reed" implying present tense. Typical error rates when using HMMs in this fashion are usually below five percent. These techniques also work well for most European languages, although access to required training corpora is frequently difficult in these languages.Deciding how to convert numbers is another problem that TTS systems have to address. It is a simple programming challenge to convert a number into words (at least in English), like "1325" becoming "one thousand three hundred twenty-five." However, numbers occur in many different contexts; "1325" may also be read as "one three two five", "thirteen twenty-five" or "thirteen hundred and twenty five". A TTS system can often infer how to expand a number based on surrounding words, numbers, and punctuation, and sometimes the system provides a way to specify the context if it is ambiguous.Roman numerals can also be read differently depending on context. For example "Henry VIII" reads as "Henry the Eighth", while "Chapter VIII" reads as "Chapter Eight".Similarly, abbreviations can be ambiguous. For example, the abbreviation "in" for "inches" must be differentiated from the word "in", and the address "12 St John St." uses the same abbreviation for both "Saint" and "Street". TTS systems with intelligent front ends can make educated guesses about ambiguous abbreviations, while others provide the same result in all cases, resulting in nonsensical (and sometimes comical) outputs, such as "co-operation" being rendered as "company operation".Text-to-phoneme challengesSpeech synthesis systems use two basic approaches to determine the pronunciation of a word based on its spelling, a process which is often called text-to-phoneme or grapheme-to-phoneme conversion (phoneme is the term used by linguists to describe distinctive sounds in a language). The simplest approach to text-to-phoneme conversion is the dictionary-based approach, where a large dictionary containing all the words of a language and their correct pronunciations is stored by the program. Determining the correct pronunciation of each word is a matter of looking up each word in the dictionary and replacing the spelling with the pronunciation specified in the dictionary. The other approach is rule-based, in which pronunciation rules are applied to words to determine their pronunciations based on their spellings. This is similar to the "sounding out", or synthetic phonics, approach to learning reading.Each approach has advantages and drawbacks. The dictionary-based approach is quick and accurate, but completely fails if it is given a word which is not in its dictionary.[citation needed] As dictionary size grows, so too does the memory spacerequirements of the synthesis system. On the other hand, the rule-based approach works on any input, but the complexity of the rules grows substantially as the system takes into account irregular spellings or pronunciations. (Consider that the word "of" is very common in English, yet is the only word in which the letter "f" is pronounced [v].) As a result, nearly all speech synthesis systems use a combination of these approaches.Languages with a phonemic orthography have a very regular writing system, and the prediction of the pronunciation of words based on their spellings is quite successful. Speech synthesis systems for such languages often use the rule-based method extensively, resorting to dictionaries only for those few words, like foreign names and borrowings, whose pronunciations are not obvious from their spellings. On the other hand, speech synthesis systems for languages like English, which have extremely irregular spelling systems, are more likely to rely on dictionaries, and to use rule-based methods only for unusual words, or words that aren't in their dictionaries.Evaluation challengesThe consistent evaluation of speech synthesis systems may be difficult because of a lack of universally agreed objective evaluation criteria. Different organizations often use different speech data. The quality of speech synthesis systems also depends to a large degree on the quality of the production technique (which may involve analogue or digital recording) and on the facilities used to replay the speech. Evaluating speech synthesis systems has therefore often been compromised by differences between production techniques and replay facilities.Recently, however, some researchers have started to evaluate speech synthesis systems using a common speech dataset.Prosodics and emotional contentA study in the journal "Speech Communication" by Amy Drahota and colleagues at the University of Portsmouth, UK, reported that listeners to voice recordings could determine, at better than chance levels, whether or not the speaker was smiling. It was suggested that identification of the vocal features that signal emotional content may be used to help make synthesized speech sound more natural.Computer operating systems or outlets with speech synthesisAtariArguably, the first speech system integrated into an operating system was the 1400XL/1450XL personal computers designed by Atari, Inc. using the Votrax SC01 chip in 1983. The 1400XL/1450XL computers used a Finite State Machine to enable WorldEnglish Spelling text-to-speech synthesis.[31] Unfortunately, the 1400XL/1450XL personal computers never shipped in quantity.The Atari ST computers were sold with "stspeech.tos" on floppy disk.AppleThe first speech system integrated into an operating system that shipped in quantity was Apple Computer's MacInTalk in 1984. The software was licensed from 3rd party developers Joseph Katz and Mark Barton (later, SoftVoice, Inc.) and was featured during the 1984 introduction of the Macintosh computer. Since the 1980s Macintosh Computers offered text to speech capabilities through The MacinTalk software. In the early 1990s Apple expanded its capabilities offering system wide text-to-speech support. With the introduction of faster PowerPC-based computers they included higher quality voice sampling. Apple also introduced speech recognition into its systems which provided a fluid command set. More recently, Apple has added sample-based voices. Starting as a curiosity, the speech system of Apple Macintosh has evolved into a fully supported program, PlainTalk, for people with vision problems. VoiceOver was for the first time featured in Mac OS X Tiger (10.4). During 10.4 (Tiger) & first releases of 10.5 (Leopard) there was only one standard voice shipping with Mac OS X. Starting with 10.6 (Snow Leopard), the user can choose out of a wide range list of multiple voices. VoiceOver voices feature the taking of realistic-sounding breaths between sentences, as well as improved clarity at high read rates over PlainTalk. Mac OS X also includes say, a command-line based application that converts text to audible speech. The AppleScript Standard Additions includes a say verb that allows a script to use any of the installed voices and to control the pitch, speaking rate and modulation of the spoken text.The Apple iOS operating system used on the iPhone, iPad and iPod Touch uses VoiceOver speech synthesis for accessibility. Some third party applications also provide speech synthesis to facilitate navigating, reading web pages or translating text.AmigaOSThe second operating system with advanced speech synthesis capabilities was AmigaOS, introduced in 1985. The voice synthesis was licensed by Commodore International from SoftVoice, Inc., who also developed the original MacinTalk text-to-speech system. It featured a complete system of voice emulation, with both male and female voices and "stress" indicator markers, made possible by advanced features of the Amiga hardware audio chipset.[33] It was divided into a narrator device and a translator library. Amiga Speak Handler featured a text-to-speech translator. AmigaOS considered speech synthesis a virtual hardware device, so the user could even redirect console output to it. Some Amiga programs, such as word processors, made extensive use of the speech system.Microsoft WindowsSee also: Microsoft AgentModern Windows desktop systems can use SAPI 4 and SAPI 5 components to support speech synthesis and speech recognition. SAPI 4.0 was available as an optional add-on for Windows 95 and Windows 98. Windows 2000 added Narrator, a text–to–speech utility for people who have visual handicaps. Third-party programs such as CoolSpeech, Textaloud and Ultra Hal can perform various text-to-speech tasks such as reading text aloud from a specified website, email account, text document, the Windows clipboard, the user's keyboard typing, etc. Not all programs can use speech synthesis directly.[34] Some programs can use plug-ins, extensions or add-ons to read text aloud. Third-party programs are available that can read text from the system clipboard.Microsoft Speech Server is a server-based package for voice synthesis and recognition. It is designed for network use with web applications and call centers. Text-to-Speech (TTS) refers to the ability of computers to read text aloud. A TTS Engine converts written text to a phonemic representation, then converts the phonemic representation to waveforms that can be output as sound. TTS engines with different languages, dialects and specialized vocabularies are available through third-party publishers.AndroidVersion 1.6 of Android added support for speech synthesis (TTS).InternetCurrently, there are a number of applications, plugins and gadgets that can read messages directly from an e-mail client and web pages from a web browser or Google Toolbar such as Text-to-voice which is an add-on to Firefox. Some specialized software can narrate RSS-feeds. On one hand, online RSS-narrators simplify information delivery by allowing users to listen to their favourite news sources and to convert them to podcasts. On the other hand, on-line RSS-readers are available on almost any PC connected to the Internet. Users can download generated audio files to portable devices, e.g. with a help of podcast receiver, and listen to them while walking, jogging or commuting to work.A growing field in Internet based TTS is web-based assistive technology, e.g. 'Browsealoud' from a UK company and Readspeaker. It can deliver TTS functionality to anyone (for reasons of accessibility, convenience, entertainment or information) with access to a web browser. The non-profit project Pediaphon was created in 2006 to provide a similar web-based TTS interface to the Wikipedia.Other work is being done in the context of the W3C through the W3C Audio Incubator Group with the involvement of The BBC and Google Inc.。
英语专业(语言学)历年真题试卷汇编25

英语专业(语言学)历年真题试卷汇编25(总分:72.00,做题时间:90分钟)一、填空题(总题数:5,分数:10.00)1.There has been a maxim in 1which claims that "You are what you say". (中山大学2008研)(分数:2.00)填空项1:__________________ (正确答案:正确答案:quantity)解析:解析:格莱斯的数量准则指1.使你的话语如(交谈的当前目的)所要求的那样信息充分;2.不要使你的话语比要求的信息更充分。
即说你该说的。
2.The theory of conversational implicature was proposed by 1. (中山大学2008研)(分数:2.00)填空项1:__________________ (正确答案:正确答案:Grice)解析:解析:格赖斯认为一定存在一些管理话语产生和理解的机制。
他把这种机制称为合作原则,在这个大原则下有四条准则,它们分别为数量、质量、关系和方式准则。
3. 1were sentences that did not state a fact or describe a state, and were not verifiable. (分数:2.00)填空项1:__________________ (正确答案:正确答案:Performatives)解析:解析:施为句是用来做事的,既不陈述事实,也不描述情况,且不能验证其真假。
4.In making conversation, the general principle that all participants are expected to observe is called the 1principle proposed by J. Grice.(分数:2.00)填空项1:__________________ (正确答案:正确答案:Cooperative)解析:解析:通常在对话中,所有的参与者都被希望能够遵守由格莱斯提出的合作原则,这样就不会有会话含义的产生。
黑龙江省哈尔滨市第三中学校2023-2024学年高三上学期开学第二次验收考试英语试题
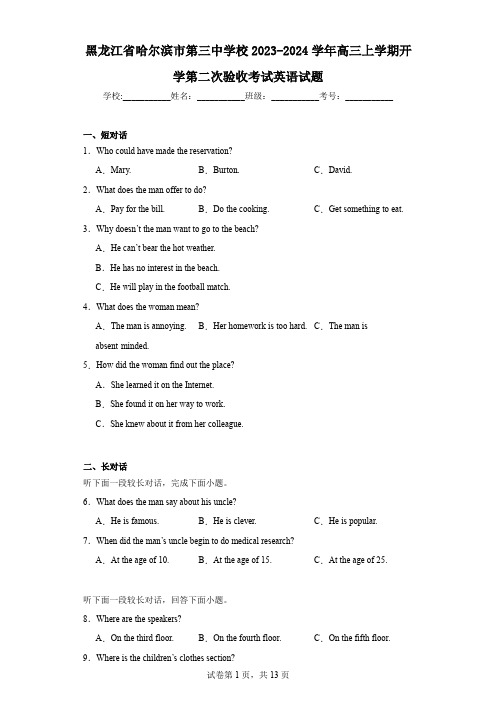
黑龙江省哈尔滨市第三中学校2023-2024学年高三上学期开学第二次验收考试英语试题学校:___________姓名:___________班级:___________考号:___________一、短对话1.Who could have made the reservation?A.Mary.B.Burton.C.David.2.What does the man offer to do?A.Pay for the bill.B.Do the cooking.C.Get something to eat. 3.Why doesn’t the man want to go to the beach?A.He can’t bear the hot weather.B.He has no interest in the beach.C.He will play in the football match.4.What does the woman mean?A.The man is annoying.B.Her homework is too hard.C.The man isabsent-minded.5.How did the woman find out the place?A.She learned it on the Internet.B.She found it on her way to work.C.She knew about it from her colleague.二、长对话听下面一段较长对话,完成下面小题。
6.What does the man say about his uncle?A.He is famous.B.He is clever.C.He is popular. 7.When did the man’s uncle begin to do medical research?A.At the age of 10.B.At the age of 15.C.At the age of 25.听下面一段较长对话,回答下面小题。
英语论文参考文献(全英文版)

英语论文参考文献(全英文版)英语论文参考文献(全英文版)关键词:英文版,参考文献,英语论文英语论文参考文献(全英文版)简介:参考文献是英文类学术论文、研究报告中不可缺少的一部分,不可随意“从略”,更不可马虎了事或错误百出,很多作者在引用英文参考文献时,会出现引用不当、格式错误等问题,为大家分享正确的英语论文参考文献格式及范例。
一、英文论文参考文献格式要求英文参考文献与中文参考文献的格式英语论文参考文献(全英文版)内容:参考文献是英文类学术论文、研究报告中不可缺少的一部分,不可随意“从略”,更不可马虎了事或错误百出,很多作者在引用英文参考文献时,会出现引用不当、格式错误等问题,为大家分享正确的英语论文参考文献格式及范例。
一、英文论文参考文献格式要求英文参考文献与中文参考文献的格式要求基本相同,但写英文参考文献要注意一点,外文作者姓名的着录格式采用姓在前(全拼,首字母大写),名在后(缩写为首字母),中间用空格;着作类文献题名的实词首字母大写,期刊文献题名的首词首字母大写,期刊名称请用全称,勿用缩写。
具体如下:1、单一作者着作的书籍姓,名字首字母.(年). 书名(斜体). 出版社所在城市:出版社.如:Sheril, R. D.(1956). The terrifying future: Contemplating color television. San Diego:Halstead.2、两位作者以上合着的书籍姓,名字首字母., 姓,名字首字母.(年). 书名(斜体). 出版社所在城市:出版社.如:Smith, J., Peter, Q. (1992).Hairball: An intensive peek behind the surface of an enigma. Hamilton, ON:McMaster University Press.3、文集中的如:Mcdonalds, A.(1993). Practical methods for the apprehension and sustained containment ofsupernatural entities. In G. L. Yeager (Ed.), Paranormal and occult studies:Case studies in application (pp. 42–64). London: OtherWorld Books.4、期刊中的(非连续页码)如:Crackton, P.(1987). The Loonie: God's long-awaited gift to colourful pocket change?Canadian Change, 64(7), 34–37.5、期刊中的(连续页码):姓,名字首字母.(年). 题目. 期刊名(斜体). 第几期,页码.如:Rottweiler, F. T., Beauchemin, J. L. (1987). Detroit and Narnia: Two foes on the brink ofdestruction. Canadian/American Studies Journal, 54, 66–146.6、月刊杂志中的如:Henry, W. A., III.(1990, April 9). Making the grade in today's schools. Time, 135, 28-31.二、英文论文参考文献范例。
Intonation adjustment in text-to-speech systems
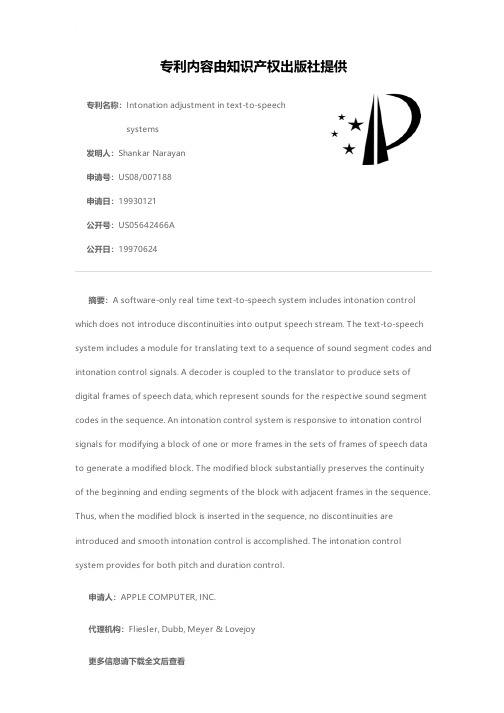
专利名称:Intonation adjustment in text-to-speech systems发明人:Shankar Narayan申请号:US08/007188申请日:19930121公开号:US05642466A公开日:19970624专利内容由知识产权出版社提供摘要:A software-only real time text-to-speech system includes intonation control which does not introduce discontinuities into output speech stream. The text-to-speech system includes a module for translating text to a sequence of sound segment codes and intonation control signals. A decoder is coupled to the translator to produce sets of digital frames of speech data, which represent sounds for the respective sound segment codes in the sequence. An intonation control system is responsive to intonation control signals for modifying a block of one or more frames in the sets of frames of speech data to generate a modified block. The modified block substantially preserves the continuity of the beginning and ending segments of the block with adjacent frames in the sequence. Thus, when the modified block is inserted in the sequence, no discontinuities are introduced and smooth intonation control is accomplished. The intonation control system provides for both pitch and duration control.申请人:APPLE COMPUTER, INC.代理机构:Fliesler, Dubb, Meyer & Lovejoy更多信息请下载全文后查看。
自然语言理解与生成技术:Text-to-speech和Speech-to-text

自然语言理解与生成技术:Text-to-speech和Speech-to-text自然语言理解与生成技术:Text-to-speech和Speech-to-text 随着科技的快速发展,自然语言理解与生成技术得到了越来越多的关注。
其中,Text-to-speech (TTS)和Speech-to-text (STT)技术被广泛应用于日常生活中,它们的应用范围涉及到电子商务、智能家居、语音识别、自动化售货等多个领域。
本文将从两个方面介绍TTS 和STT技术,分别从原理、技术发展、应用场景、发展前景等角度展开讲解。
一、Text-to-speech1.原理Text-to-speech是将文本转换为语音的技术。
其基本原理是通过语音合成技术,将文字转换为声音。
传统的语音合成技术是通过将已有的语音样本组成音素库,然后根据待合成的文本,选取相应的音素并拼接成语音。
这种方法由于采用的是固定的音素库,因此,合成出的语音比较生硬,没有很好的感观效果。
为此,近年来,人们开发了多种新的文本转语音技术,如HMM、DNN、TTS和Tacotron等。
2.技术发展TTS技术的发展历程可追溯到二十世纪五十年代。
1950年,贝尔实验室开始研究语音合成技术,并于1957年推出了第一款语音合成器。
此后,一系列语音合成器相继问世,包括基于规则的语音合成技术、基于聚类的语音合成技术、基于统计的语音合成技术等。
到了21世纪,随着深度学习技术的发展,TTS技术得到了快速发展。
2017年,Google推出了Tacotron2模型,该模型能够将文本转换为自然语言的语音。
3.应用场景TTS技术的应用场景非常广泛。
其可以用于语音提醒、新闻播报、语音导航、交互式语音应答系统等。
目前,TTS技术在智能助手、语音合成考试、虚拟主播等领域已经得到了广泛应用。
例如,Siri和小度在语音合成方面的表现就是典型的TTS技术应用案例。
4.发展前景从历史上看,TTS技术对于人工智能行业的长期发展势必产生深远的影响。
口述作文软件整理
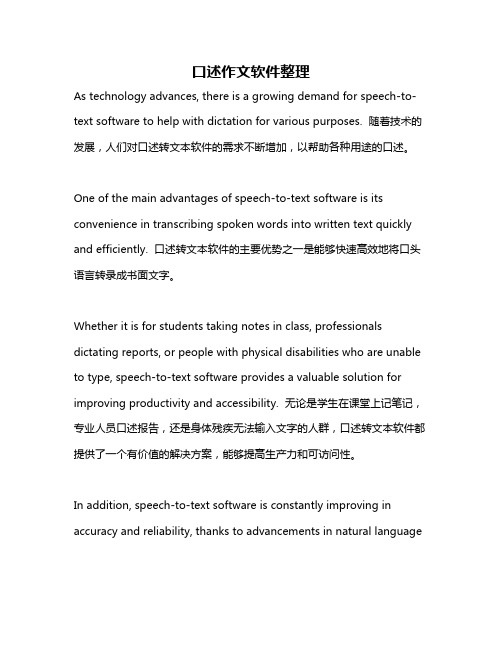
口述作文软件整理As technology advances, there is a growing demand for speech-to-text software to help with dictation for various purposes. 随着技术的发展,人们对口述转文本软件的需求不断增加,以帮助各种用途的口述。
One of the main advantages of speech-to-text software is its convenience in transcribing spoken words into written text quickly and efficiently. 口述转文本软件的主要优势之一是能够快速高效地将口头语言转录成书面文字。
Whether it is for students taking notes in class, professionals dictating reports, or people with physical disabilities who are unable to type, speech-to-text software provides a valuable solution for improving productivity and accessibility. 无论是学生在课堂上记笔记,专业人员口述报告,还是身体残疾无法输入文字的人群,口述转文本软件都提供了一个有价值的解决方案,能够提高生产力和可访问性。
In addition, speech-to-text software is constantly improving in accuracy and reliability, thanks to advancements in natural languageprocessing and machine learning algorithms. 此外,由于自然语言处理和机器学习算法的进步,口述转文本软件在准确性和可靠性方面不断提高。
危!我用python克隆了女朋友的声音!
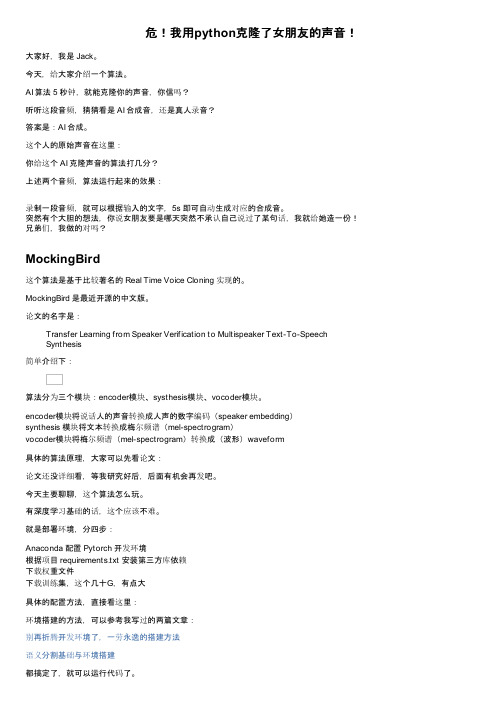
危!我用python克隆了女朋友的声音!大家好,我是 Jack。
今天,给大家介绍一个算法。
AI 算法 5 秒钟,就能克隆你的声音,你信吗?听听这段音频,猜猜看是 AI 合成音,还是真人录音?答案是:AI 合成。
这个人的原始声音在这里:你给这个 AI 克隆声音的算法打几分?上述两个音频,算法运行起来的效果:录制一段音频,就可以根据输入的文字,5s 即可自动生成对应的合成音。
突然有个大胆的想法,你说女朋友要是哪天突然不承认自己说过了某句话,我就给她造一份!兄弟们,我做的对吗?MockingBird这个算法是基于比较著名的 Real Time Voice Cloning 实现的。
MockingBird 是最近开源的中文版。
论文的名字是:Transfer Learning from Speaker Verification to Multispeaker Text-To-SpeechSynthesis简单介绍下:算法分为三个模块:encoder模块、systhesis模块、vocoder模块。
encoder模块将说话人的声音转换成人声的数字编码(speaker embedding)synthesis 模块将文本转换成梅尔频谱(mel-spectrogram)vocoder模块将梅尔频谱(mel-spectrogram)转换成(波形)waveform具体的算法原理,大家可以先看论文:论文还没详细看,等我研究好后,后面有机会再发吧。
今天主要聊聊,这个算法怎么玩。
有深度学习基础的话,这个应该不难。
就是部署环境,分四步:Anaconda 配置 Pytorch 开发环境根据项目 requirements.txt 安装第三方库依赖下载权重文件下载训练集,这个几十G,有点大具体的配置方法,直接看这里:环境搭建的方法,可以参考我写过的两篇文章:别再折腾开发环境了,一劳永逸的搭建方法语义分割基础与环境搭建都搞定了,就可以运行代码了。
- 1、下载文档前请自行甄别文档内容的完整性,平台不提供额外的编辑、内容补充、找答案等附加服务。
- 2、"仅部分预览"的文档,不可在线预览部分如存在完整性等问题,可反馈申请退款(可完整预览的文档不适用该条件!)。
- 3、如文档侵犯您的权益,请联系客服反馈,我们会尽快为您处理(人工客服工作时间:9:00-18:30)。
Text Processing for Text-to-SpeechSystems in Indian Languages Anand Arokia Raj1,Tanuja Sarkar1,Satish Chandra Pammi1, Santhosh Yuvaraj1,Mohit Bansal2,Kishore Prahallad13,Alan W Black31InternationalInstitute of InformationTechnology,Hyderabad,India.2Indian Institute of Technology,Kanpur,India.3Language TechnologiesInstitute,Carnegie Mellon University,Pittsburgh,USA.skishore@,awb@AbstractTo build a natural soundingspeech synthesissystem,it is essen-tial that the text processing component produce an appropriate sequence of phonemic units correspondingto an arbitrary input text.In this paper we discuss our efforts in addressingthe issues of Font-to-Aksharamapping,pronunciationrules for Aksharas, text normalizationin the context of building text-to-speechsys-tems in Indian languages.1.IntroductionThe objective of a text to speechsystemis to convert an arbitrary given text into a correspondingspoken waveform.Text process-ing and speech generationare two main componentsof a text to speech system.The objective of the text processing component is to process the given input text and produce appropriate se-quence of phonemic units.These phonemic units are realized by the speech generation component either by synthesis from parametersor by selection of a unit from a large speech corpus. For natural sounding speech synthesis,it is essential that the text processing component produce an appropriate sequence of phonemic units correspondingto an arbitrary input text.One of the question often asked by end-users is why we don’t have TTS systems for all or many of the23official Indian languages.What are the complexities:Is it because the syn-thesis technology isn’t matured enough to be able to build for any language or is it because of the non-existence of speech databases in Indian languages?.Unfortunately,for a decade the core speech generationtechnologyi.e.,generationof speech from a phonemic sequence has largely been automated due to unit selection techniques[1].With the introduction of statisti-cal parametric speech synthesis techniques,it is much easier to build a voice in a language with fewer sentences and a smaller speech corpus[2][3].It is difficult to convince an end-user that the input to a TTS system is not a phonemic sequence but rather the raw text as available in news websites,blogs,documents etc which contain the required text in font-encodings,native scripts and non-standard words such as addresses,numbers,currency etc. The majority of the issues are associated in building a TTS for a new language is associated with handling of real-world text[4].Current state-of-art TTS system in English and other well-researched languages use such rich set of linguistic re-sources such as word-sense disambiguation,morphologic al an-alyzer,Part-of-Speech tagging,letter-to-sound rules,syllabifi-cation,stress-patterns in one form or the other to build a text processing component of a TTS system.However for minority languages(which are not well researchedor do not have enough linguistic resources),it involves several complexities starting from accumulation of text corpora in digital and processable format.Linguistic components are not available in such rich fashion for all languages of the world.In practical world,mi-nority languages including some of the Indian languages do not have that luxury of assuming some or any of the linguisticcom-ponents.The purpose of this paper is to describe our efforts at IIIT Hyderabad to build a generic framework for build text process-ing modules and linguistic resources which could be extended to all of the Indian languages with minimal efforts and time. Our approach is to make use of minimal language informa-tion(i.e.,informationavailable with an average educated native speakers),take the aid of acoustic data and machine learning techniques[5].In this paper we summarize some of our ef-forts in this directionbut mainly for font identification,Font-to-Akshara conversion,pronunciation rules for Aksharas and text normalization.2.Nature of Indian Language Scripts The scripts in Indian languageshave originatedfrom the ancient Brahmi script.The basic units of the writing system are referred to as Aksharas.The properties of Aksharas are as follows:(1) An Akshara is an orthographicrepresentationof a speech sound in an Indian language;(2)Aksharas are syllabic in nature;(3) The typical forms of Akshara are V,CV,CCV and CCCV,thus have a generalizedform of C*V.The shape of an Akshara depends on its composition of consonants and the vowel,and sequence of the consonants.In defining the shape of an Akshara,one of the consonantsymbols acts as pivotal symbol(referred to as semi-full form).Depend-ing on the context,an Akshara can have a complex shape with other consonant and vowel symbols being placed on top,be-low,before,after or sometimes surrounding the pivotal symbol (referred to as half-form).Thus to render an Akshara,a set of semi-full or half-forms have to be rendered,which in turn are rendered using a set of basic shapes referred to as glyphs.Often a semi-full form or half-formis rendered using two or more glyphs,thus there is no one-to-one correspondencebetween glyphs of a font and semi-full or half-forms[6].2.1.Convergence and DivergenceThere are23official languages of India,and all of them ex-cept English and Urdu share a common phonetic base,i.e.,they share a common set of speech sounds.While all of these lan-guages share a common phonetic base,some of thelanguagessuch as Hindi,Marathi and Nepali also share a common script known as Devanagari.But languages such as Telugu,Kannada and Tamil have their own scripts.The property that makes these languages separate can be attributed to the phonotactics in each of these languages rather than the scripts and speech sounds.phonotactics is the permis-sible combinationsof phones that can co-occur in a language.2.2.Digital Storage of Indian Language ScriptsThere is a chaos as far as the text in Indian languages in elec-tronic form is concerned.Neither can one exchange the notes in Indian languages as conveniently as in English language,nor can one performsearcheasily on texts in Indianlanguagesavail-able over the web.This is because the texts are being stored in ASCII font dependent glyph codes as opposed to Unicode.The glyph coding schemes are typicallydifferent for differ-ent languages and within a language there could exists several font-typeswith their own glyph codes(as many as major news-portals in a language).To view the websites hosting the content in a particular font-type,these fonts have to be installedon local machine.As this was the technology existed before the era of Unicode and hence a lot of electronic data in Indian languages were made and available in that form[7].2.3.Need for Handling Font-DataThe text available in a font-encoding(or font-type)is referred to as font-data.While Unicode based news-portals and web-pages are increasing,there are two main reasons to deal with ASCII based font-data:1)Given that there are23official In-dian languages,and the amountof data availablein ASCII based font-encodingsis much larger than the text content available in Unicode format,2)If a TTS system has to read the text from a ASCII font based website then the TTS system should au-tomatically identify the font-type and process the font-data to generate speech.2.4.A Phonetic TransliterationScheme for Digital storage of Indian Language ScriptsTo handle diversified storage formats of scripts of Indian lan-guages such as ASCII based fonts,ISCII(Indian Standard code for Information Interchange)and Unicode etc,it is useful and becomes necessary to use a meta-storageformat.A transliterationscheme maps the Aksharas of Indian lan-guages onto English alphabets and it could serve as meta-storage format for text-data.Since Aksharas in Indian lan-guages are orthographic represent of speech sound,and they have a common phonetic base,it is suggested to have a pho-netic transliterationscheme such as IT3[8][6].Thus when the font-data is converted into IT3,it essentially turns the whole effort into font-to-Aksharaconversion.3.Identificationof Font-T ypeGiven a document we often need to identify the font-type,and sometimes a document can contain the data encoded in differ-ent font-types.Then the task would boil down to identifyingthe font-type for each line or for each word.In this paper,we pro-pose the use of TF-IDF approach for identificationof font-type. The term frequency-inverse document frequency(TF-IDF)ap-proach is used to weigh each glyph-sequence in the font-data according to how unique it is.In other words,the TF-IDF ap-proach captures the relevancy among glyph-sequenceand font-type.In this approach,the term refers to a’glyph’and the docu-ment refers to the font-data of a particular’font-type’.Here the glyph-sequencecould mean a single glyph or’current and next’glyph or’previous,current and next’glyph etc.To build a document for each font-type,a web-site for each font-type was manually identified and around0.12million unique words were crawled for each of the font-type.The set of unique words for each font-type are referred to as a document representing the particular font-type.Thus given N documents (each representing a font-type),we considered three different terms namely,a single glyph or current and next glyph or pre-vious,current and next glyph.For each term a TF-IDF weight was obtained as follows:(i)Calculate the term frequency for the glyph-sequence:The number of times that glyph-sequence occurred divided by the total number of glyph-sequences in that specific document.(ii)Calculate document frequency:In how many different documents(font-types)that specific glyph-sequence has occurred.(iii)Calculate inverse document fre-quency of the term and take logarithm of inverse document fre-quency.To identify the font-type of a given test font-data,the steps involved are as follows:1)Generate the terms(glyph-sequences)of the test font-data2)Computethe relevancy scores of the terms and for each of the document(font-type)using the correspondi ng TF-IDF weights of the terms3)The test font-databelongs to the document(font-type)which produces a maximum relevancy score.The performance of TF-IDF approach for identification of font-type was evaluated on1000unique sentences and words per font-type.We have added English data as also one of the testing set,and is referred to as English-te xt.The perfor-mance of font-type identification system using different terms single glyph,current and next glyphs,previous,current and next glyphs are shown in Table1,Table2and Table3respec-tively and it could be observed that the use of previous,current and next glyphs as a term provided an accuracy of100%in iden-tification of font-type even at the word level.Table1:Performance of Single glyph based font models Font Name Sentence-Le vel Word-Level Amarujala(Hindi)100%100%Jagran(Hindi)100%100%Webdunia(Hindi)100%0.1% SHREE-TEL(Telugu)100%7.3%Eenadu(Telugu)0%0.2%Vaarttha(Telugu)100%29.1% Elango Panchali(Tamil)100%93%Amudham(Tamil)100%100% SHREE-T AM(Tamil)100% 3.7% English-te xt0%0%4.Font-to-AksharaMappingFont-data conversion can be defined as converting the font en-coded data into Aksharasrepresentedusing phonetictranslitera-tion scheme such as IT3.As we already mentioned that Aksha-ras are split into glyphs of a font,and hence a conversion from font-data has essentially to deal with glyphs and model how a sequenceof glyphs are merged to form an Akshara.As there ex-ist many fonts in Indian languages,we have designed a generic framework has been designed for the conversion of font-data.ItTable2:Performanceof current and next glyph based font mod-elsFont Name Sentence-Le vel Word-Level Amarujala(Hindi)100%100%Jagran(Hindi)100%100%Webdunia(Hindi)100%100% SHREE-TEL(Telugu)100%100%Eenadu(Telugu)100%100%Vaarttha(Telugu)100%100% Elango Panchali(Tamil)100%100%Amudham(Tamil)100%100% SHREE-T AM(Tamil)100%100% English-te xt100%96.3%Table3:Performance of previous,current and next based font modelsFont Name Sentence-Le vel Word-Level Amarujala(Hindi)100%100%Jagran(Hindi)100%100%Webdunia(Hindi)100%100% SHREE-TEL(Telugu)100%100%Eenadu(Telugu)100%100%Vaarttha(Telugu)100%100% Elango Panchali(Tamil)100%100%Amudham(Tamil)100%100% SHREE-T AM(Tamil)100%100% English-te xt100%100%has two phases,in thefirst phase we are building the base-map table for a given font-type and in the second phase forming and ordering the assimilationrules for a specific language.4.1.Building a Base-Map Table for a Font-typeThe base-map table provides the mapping basic between the glyphs of the font-type to the Aksharas represented in IT3 transliteration scheme.The novelty in our mapping was that the shape of a glyph was also included in building this mapping table.The shape of a glyph is dictated by whether it is rendered as pivotal consonant,or on top,bottom,left or right of the piv-otal consonant.Thus the pivotal glyphs were appended with0 (for full characters such as e,ka)or1(for half consonants such as k1,p1),’2’for glyphs occur at left hand side of a basic char-acter(ex:i2,r2),’3’for glyphs occur at right hand side of a basic character(ex:au3,y3),’4’for glyphs occur at top of a basic character(ex:ai4,r4)and’5’for glyphs occur at bottom of a basic character(ex:u5,t5).4.2.Forming AssimilationRulesIn the conversion process the above explained basic-mapping table will be used as the seed.A well defined and ordered set of assimilation rules have to be formed for each and every language.Assimilation is the process of merging two or more glyphs and generating a valid single character.This assimilation happens at different levels and our observation across many lan-guages was that thefiring of following assimilation rules were universally applicable.The rules are:(i)Modifier Modification, (ii)Language Preprocessing,(iii)Consonant Assimilation,(iv) Maatra Assimilation,(v)Consonant-V owel Assimilation,(vi) V owel-Maatra Assimilation,(vii)Consonants Clustering and (viii)Schwa Deletion.The Modifier Modificationis the process where the charac-ters get modified because of the language modifiers like virama and nukta(ka+virama=k1).The Language Preprocessing step deals with some language specific processing like(aa3+ i3=ri in Tamil)and(r4moves in front of the previousfirst full consonant in Hindi).The Consonant Assimilation is known as getting merged two or more consonantglyphs and forms a valid single consonant like(d1+h5=dh1in Telugu).The Maatra Assimilation is known as getting merged two or more maatra glyphs and forms a valid single maatra like(aa3+e4=o3in Hindi).The Consonant-V owel Assimilationis known as getting merged two or more consonant and vowel glyphs and forms a valid single consonant like(e+a4+u5=pu in Telugu).The V owel-Maatra Assimilation is known as getting merged two or more vowel and maatra glyphs and forms a valid single vowel like(a+aa3=aa in Hindi).The ConsonantClusteringin known as merging the half consonant which usually occurs at the bot-tom of a full consonant to that full consonant like(la+l5=lla in Hindi).The Schwa Deletion is deleting the inherent vowel ’a’from a full consonant in necessary places like(ka+ii3= kii).4.3.Testing and EvaluationThe evaluation on these font converters is carried out in two phases.We picked up three different font-types for training or forming the assimilationrules and one new font-typefor testing per language.In thefirst phase for the selected three font-types the assimilation rules are formed and refined.In the second phase we chose a new font-type and built the base-map table only and used the existing converter without any modifications. We have taken500unique words per font-type and generated the conversion output.The evaluation results in Table4show that the font converter performs consistent ly even for a new font-type.So it is only sufficient to provide the base-map table for a new font-type to get a good conversion results.The issue of Font-to-Aksharamapping has been attempted in[7]and[9] but we believe that our framework is a generic one which could easily be extended to a new font-type with>99%conversion accuracy.5.Building PronunciationModels ForAksharasHaving converted the font-data into Aksharas,the next step is to obtain appropriate pronunciation for each of the Aksharas. As noted earlier,Aksharas are orthographic representation of speech sounds and it is commonly believed or quoted that there is direct correspondence between what is written and what is spoken in Indian languages,however,there is no one-to-one correspondence between what is written and what is spoken. Often some of the sounds are deleted such as Schwa deletion in Hindi.Schwa is the default short vowel/a/which is associ-ated with a consonant,and often it is deleted to aid in faster pronunciation of a word.Similarly there exists exceptions for Bengali and Tamil.There are attempts to model these excep-tions in the form of the rules,however,they are often met with limited success or they use linguistic resources such as Morph analyzer.Such linguisticresourcesmay not always be available for minority languages.Thus we had built a framework based on machine learning techniqueswhere pronunciationof Aksha-ras could be modeled using machine learning techniques and using a small set of supervised training data.Table4:Performance results for font conversion in Indian lan-guagesLanguage Font Name Training/T esting Accuracy Hindi Amarujala Training99.2%Jagran Training99.4%Naidunia Training98.8%Webdunia Training99.4%Chanakya Testing99.8%Marathi Shree Pudhari Training100%Shree Dev Training99.8%TTY ogesh Training99.6%Shusha Testing99.6%Telugu Eenadu Training93%Vaartha Training92%Hemalatha Training93%TeluguFont Testing94% Tamil Elango Valluvan Training100%Shree Tam Training99.6%Elango Panchali Training99.8%Tboomis Testing100%Kannada Shree Kan Training99.8%TTNandi Training99.4%BRH Kannada Training99.6%BRH Vijay Testing99.6% Malayalam Revathi Training100%Karthika Training99.4%Thoolika Training99.8%ShreeMal Testing99.6%Gujarati Krishna Training99.6%Krishnaweb Training99.4%Gopika Training99.2%Divya Testing99.4%5.1.Creation of Data-setGiven the input word list with the correspondingpronunciations in terms of phones,feature vectors were extracted for training the pronunciation model at the phone level.About12200sen-tences in IT3format were used to collect the training data,for buildingthe pronunciationmodel in Hindi.These sentenceshad about26000unique words,which were used to extract around 32800feature vectors.Different sets of feature vectors to ex-periment on the selectionof features.As for Bengali and Tamil, 5000words with corresponding pronunciations were used for obtaining about9000feature vectors.e of Contextual FeaturesContextual features refers to the neighbor phones in a definite window-size/le ing the contextual features,experiments were performedfor various Contextual Levels(CL).A decision forest was built for each phone to model its pronunciation.A decision forest is a set of decision trees built using overlapping but different sub-sets of the training data and it employs a ma-jority voting scheme on individual prediction of different trees to predict the pronunciation of a phone.Table5shows the re-sults of pronunciationmodel for Hindi,Bengali and Tamil using various level of contextual features.We found that that a con-text level of4(i.e.,4phones to the left and4phones to the right) was sufficient to model the pronunciation and moving beyond the level of4,the performancewas degraded.Table5:PronunciationModel with Contextual featuresLanguages Context Level2346 Hindi90.24%91.44%91.78%91.61%Bengali82.77%84.48%84.56%83.56%Tamil98.16%98.24%98.10%98.05%5.3.Acoustic-Phoneticand Syllabic Features Acousticphoneticfeatureslists the articulatorypropertiesof the consonants and the vowels.Typically vowels are characterized by the front,back,mid position of the tongue while consonants are characterizedby manner and place of articulationand voic-ing and nasalization features.Syllabic features indicate where a particular syllable is of type CV or CCV,or CVC etc.The performance of the pronunciation model for Hindi,Tamil and Bengali using syllabicand acoustic-phoneticfeaturesof the cur-rent and neighboring phones are shown in Table6and Table 7respecti vely.We found that the use of syllabic or acoustic-phonetic features didn’t show any significantimprovement than that of contextual features for Hindi,Tamil and Bengali.A rule based algorithm for Hindi LTS is given in[10].To compareour resultswith the rule-basedalgorithm,we have used the same algorithm with out morphologicalanalyzer on our test data set.We found that the performanceof pronunciationmodel using rule-basedtechniquewas88.17%.while the decision for-est model in Table6was providing an accuracy of92.29%.Table6:PronunciationModel with Syllabic featuresFeature Sets LanguagesHindi Bengali Tamil Syl Struct.of Cur.Phone92.29%82.41%98.31% Syl Struct.of all Phones91.61%67.56%98.27%Table7:PronunciationModel with Acoustic-Phoneticfeatures Feature Sets LanguagesHindi Bengali Tamil Acoustic Phonetic89.73%84.78%98.18% +Syl Struct.of Curr.Phone89.73%81.21%98.17% +Syl Struct.of all Phones91.09%69.33%98.13%6.Normalizingof Non-StandardWords Unrestrictedtexts include Standard Words(common words and Proper Names)and Non-Standar d Words(NSWs).Standard Words have a specific pronunciation that can be phonetically described either in a lexicon,using a disambiguation process-ing to some extent,or by letter-to-sound rules.In the context of TTS the problem is to decide how an automatic system should pronounce a token;even before the pronunciationof a token,itTable8:Taxonomy of NSWs with examples Category Description ExamplesAddr Address12/451(house/streetno.)Janapath Road Curr Currency Rs.7635.42Count Count of items10computers,500people Date Date(to be expanded)1/1/05,1997-99PhoneNo As sequence of digits0402300675Pin As sequence of digits208023Score Cricket,tennis scores India123/4,sets3-53-45-6 Time Time(to be expanded) 1.30,10:45-12:30,11.12.05,1930hrsUnits As decimal or number10.5kms,98%,13.67acresNUM Default categoryis importantto identify the NSW-Category of a token.A typical set of NSW-category and their examples are shown in Table8.6.1.Creation of Supervised Training DataTo build a training dataset,it typically requires a large man-ual effort to annotate an example with the appropriate NSW-category.For example,given a word corpus>3M words in Telugu,Tamil and Hindi,we extracted150-500K sentences containing an NSW.Annotating such huge set of examples needs lots of time and effort.To minimize such effort,we used a novel frequency based approach to create a representati v e ex-ample set.NSW techniques uses context information for disambigua-tion with various window sizes,context information contains a set of word like units which occurs in left and right side of a NSW,and this information is to be considered as a features characterizing a NSW.However,not of all context would be useful,so we used a window size of2(left and right)as a default and given to the pattern generator module.The pattern gener-ator takes the four tokens(two to left and two to the right of a NSW)and generates15patterns using all possible combina-tions of4(like examples,0001,0010,0011,0100,.,111)where 1represent presence of a token and0represent deletion of the token.Given such15patterns for each example,these patterns were sorted in the descendingorder of their frequency and based on a threshold a set of patterns were choosen and given to a na-tive speaker to annotate the NSW category.The user interface was built such that if the native speaker couldn’t annotate the NSW with the given pattern,then an extended context was pre-sented to him at varying ing the frequency based ap-proach,we could reduce the training examples to around1000-1500which a native could annotate within a couple of hours. Having got the annotation done,we looked at level of context the native speaker has used to annotate a NSW.We found less than10%of time the user has looked into a context information more than a window size of two.6.2.Performance of Base-line SystemUsing word level units and decision tree,we built a base-line system to predict the category of a NSW.We have tested the performanceof the system on a separatemanually prepareddata obtained from a different source(web)referred to as Test-Set-1Table9:Performance of prediction of NSW-category Using Word Level FeaturesLanguage%accuracy on%accuracy onTraining set TS1Telugu99.57%63.52%Hindi99.80%66.99%Tamil99.01%55.42%Table10:Performance of prediction of NSW-category Using Syllable level FeaturesLanguage%accuracy on%accuracy on Diff withTraining set TS1base-line Telugu99.57%91.00%27.48% Hindi99.80%82.80%15.81% Tamil99.01%87.20%31.78%(TS1).The results of prediction of NSW category on TS1is shown in Table9.The performance of the base-line system on TS1is around 60%.After analyzing the errors made by the system,we found that the errors are primarily due to new words found in the con-text of NSW,and Indian languagesbeing rich in inflectionaland derivative morphology,the roots of many of these words were present in the training data.It suggests that we should use roots of the context as the features to predict NSW-category,how-ever,such approach needs morphological analyzers.Many of the Indian languages fall into category of minority languages where linguisticresourcesare scarce.Thus we wanted to inves-tigate sub-word units such as syllables and their combinations as features for prediction of NSW-category.Our experiments on POS-tagging on Hindi,Bengali and Telugu using syllable-le vel units further provided evidence that syllable level features could be used as alternati ve and afirst-order approximationof root of a word[11].After initial set of experiments to explore different possibilitiesof using syllable-level features,we confined to a set of following three syllable level features.They are:1)F1:previous ten and next ten syl-lables of a NSW,2)F2:previous ten and next ten syllables and onset of each syllables and3)F3:Onset,vowel and coda of previous ten and next ten syllables.Using decision forest,thefinal predictionof NSW-category is chosen based on voting on the outputs of the three decision trees built using F1,F2and F3.This strategy gets the results of each decision tree and performs a majority voting to predict the NSW-category.The performance of the decision forest based system using syllable level features is shown in Table10.We found that the results of using syllable-le vel features for text normalization performed significantly better than that of using word-level features.This significantimprovement in the perfor-mance is primarily due to syllables acting afirst-order approx-imation of roots of the context words and thus minimizing the problem of unseen context.Thefinal performance of the text normalization system is further improved after using expander module from91.00%,82.80%and87.20%to96.60%,96.65% and93.38%for languagesTelugu,Hindi and Tamil respecti vely.7.ConclusionsThis paper explained the nature and difficulties associated with building text processing components of TTS systems in In-dian languages.We have discussed the relevancy of font-identification and font-to-Akshara conversion and proposed a TF-IDF based approach for font-identification.A novel ap-proach of conversion from font-to-Akshara using the shapes of the glyphs and the assimilation rules was explained.We have also studied the performance of pronunciationmodels for different features including contextual,syllabic and acoustic-phonetic features.Finally we have shown that syllable-le vel features could be used to build a text normalization system whose performance is significantly better than the word-level features.8.References[1]Hunt A.J.and Black A.W.,“Unit selectionin a concatena-tive speech synthesis system for a large speech database,”in Proceedings of IEEE Int.Conf.Acoust.,Speech,and Signal Processing,1996,pp.373–376.[2]Black A.W.,Zen H.,and Tokuda K.,“Statisticalparamet-ric speech synthesis,”in Proceedings of IEEE Int.Conf.Acoust.,Speech,and Signal Processing,Honolulu,USA, 2007.[3]Zen H.,Nose T.,Yamagishi J.,Sako S.,Masuko T.,BlackA.W.,and Tokuda K.,“The hmm-based speech synthe-sis system version2.0,”in Proc.of ISCA SSW6,Bonn, Germany,2007.[4]Sproat R.,Black A.W.,Chen S.,Kumar S.,Ostendorf M.,and Richards C.,“Normalizationof non-standardwords,”Computer Speech and Language,pp.287–333,2001. [5]HaileMaria m S.and Prahallad K.,“Extraction of lin-guistic information with the aid of acoustic data to build speech systems,”in Proceedings of IEEE Int.Conf.Acoust.,Speech,and Signal Processing,Honolulu,USA, 2007.[6]PrahalladL.,PrahalladK.,and GanapathirajuM.,“A sim-ple approach for building transliterationeditors for indian languages,”Journal of Zhejiang University Science,vol.6A,no.11,pp.1354–1361,2005.[7]Garg H.,Overcoming the Font and Script Barriers AmongIndian Languages,MS dissertation,InternationalInstitute of InformationTechnology,Hyderabad,India,2004. [8]GanapathirajuM.,BalakrishnanM.,BalakrishnanN.,andReddy R.,“Om:One tool for many(Indian)languages,”Journal of Zhejiang University Science,vol.6A,no.11, pp.1348–1353,2005.[9]Khudanpur S.and Schafer C.,“/cschafer/jhu devanagari cvt ver2.tar.gz,”2003. [10]Choudhury M.,“Rule-based grapheme to phoneme map-ping for hindi speech synthesis,”in90th Indian Science Congress of the InternationalSpeech CommunicationAs-sociation(ISCA),Bangalore,India,2003.[11]S.Chandra Pammi and Prahallad K.,“POS tagging andchunking using decision forests,”in Proceedingsof Work-shop on Shallow Parsing in South Asian Languages,IJ-CAI,Hyderabad,India,2007.。