Lecture 1 Vector Spaces and Vector Norms
线性代数 英文讲义

}
3 Linear Independence
Consider the following vectors in R3:
1 2 1 x1 = 1, x 2 = 3 , x 3 = 3 2 8 1
Conclusion:
(1) If v1, v2, … , vn span a vector space V and one of these vectors can be written as a linear combination of the other n-1 vectors, then those n-1 vectors span V. (2) Given n vectors v1, v2, … , vn , it is possible to write one of the vectors as a linear combination of the other n-1 vectors if and only if there exist scalars c1, …, cn not all zero such that c1v1+c2v2+‥‥cnvn=0
Definition
The vectors v1, v2, … , vn in a vector space V are said to be linearly independent if c1v1+c2v2+‥‥+cnvn=0 implies that all the scalars c1, …, cn must equal 0.
The Vector Space C[a, b]
C[a,b] denote the set of all real-valued functions that are defined and continuous on the closed interval [a,b].
高等代数课件北大三版 第六章 向量空间
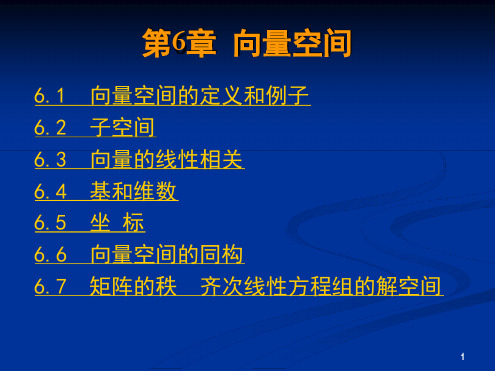
惠州学院数学系
9
(a2) [f(x)+g(x)]+h(x)= f(x)+ [g(x) +h(x) ],
任给f(x),g(x),h(x) ? F[x].
(a3) 0向量就是零多项式. (a4) f(x)的负向量为(- f(x)). (m1) (ab) f(x)= a(bf(x)).
(m2) a [f(x)+g(x)]= a f(x)+ a g(x). (m3) (a ? b) f(x)= a f(x)+ b f(x).
加法和数乘两种,并且满足(教材P183):
1. A+B=B+A 2. (A+B)+C= A+( B+C) 3. O+A=A 4. A+(-A)=O
5. a(A+B)= aA+Ab 6. (a+b)B=a B +Bb 7. (ab)A=a(b)A 还有一个显而易见的: 8. 1A =A
惠州学院数学系
5
(m4) 1 ? f(x)= f(x).
注1:刚开始,步骤要完整.
惠州学院数学系
10
例5 C[a,b] 表示区间[a,b] 上连续实函数按照通常的加法 与数乘构成实数域 R的向量空间,称为函数空间 . 证明: 比照例3,给出完整步骤. 例6 (1)数域F是F上的向量空间. (2)R是Q上的向量
空间,R是否为C上的向量空间?
惠州学数学系
12
例8 在 R2 上定义加法和数乘:
(a, b) ? (c, d) ? (a ? c, b ? d ? ac) k (a,b) ? (ka, kb? k(k ? 1) a 2 )
2
证明 R2 关于给定运算构成R上的向量空间.
北大暑期课程《回归分析报告》(Linear Regression Analysis)讲义1
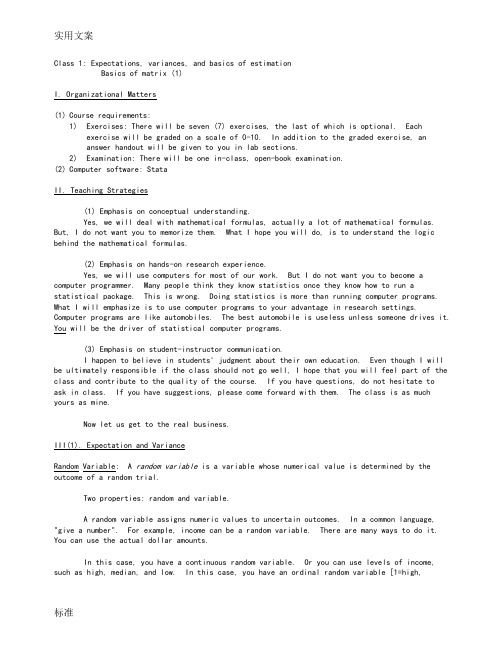
实用文案Class 1: Expectations, variances, and basics of estimationBasics of matrix (1)I. Organizational Matters(1)Course requirements:1)Exercises: There will be seven (7) exercises, the last of which is optional. Eachexercise will be graded on a scale of 0-10. In addition to the graded exercise, ananswer handout will be given to you in lab sections.2)Examination: There will be one in-class, open-book examination.(2)Computer software: StataII. Teaching Strategies(1) Emphasis on conceptual understanding.Yes, we will deal with mathematical formulas, actually a lot of mathematical formulas. But, I do not want you to memorize them. What I hope you will do, is to understand the logic behind the mathematical formulas.(2) Emphasis on hands-on research experience.Yes, we will use computers for most of our work. But I do not want you to become a computer programmer. Many people think they know statistics once they know how to run astatistical package. This is wrong. Doing statistics is more than running computer programs. What I will emphasize is to use computer programs to your advantage in research settings. Computer programs are like automobiles. The best automobile is useless unless someone drives it. You will be the driver of statistical computer programs.(3) Emphasis on student-instructor communication.I happen to believe in students' judgment about their own education. Even though I willbe ultimately responsible if the class should not go well, I hope that you will feel part of the class and contribute to the quality of the course. If you have questions, do not hesitate toask in class. If you have suggestions, please come forward with them. The class is as muchyours as mine.Now let us get to the real business.III(1). Expectation and VarianceRandom Variable: A random variable is a variable whose numerical value is determined by the outcome of a random trial.Two properties: random and variable.A random variable assigns numeric values to uncertain outcomes. In a common language, "give a number". For example, income can be a random variable. There are many ways to do it. You can use the actual dollar amounts.In this case, you have a continuous random variable. Or you can use levels of income, such as high, median, and low. In this case, you have an ordinal random variable [1=high,2=median, 3=low]. Or if you are interested in the issue of poverty, you can have a dichotomous variable: 1=in poverty, 0=not in poverty.In sum, the mapping of numeric values to outcomes of events in this way is the essenceof a random variable.Probability Distribution: The probability distribution for a discrete random variable X associates with each of the distinct outcomes x i(i = 1, 2,..., k) a probability P(X = x i). Cumulative Probability Distribution: The cumulative probability distribution for a discrete random variable X provides the cumulative probabilities P(X x) for all values x.Expected Value of Random Variable: The expected value of a discrete random variable X is denoted by E{X} and defined:E{X}= P(x i)where: P(x i) denotes P(X = x i). The notation E{ } (read “expectation of”) is called the expectation operator.In common language, expectation is the mean. But the difference is that expectation is a concept for the entire population that you never observe. It is the result of the infinite number of repetitions. For example, if you toss a coin, the proportion of tails should be .5 in the limit. Or the expectation is .5. Most of the times you do not get the exact .5, but a number close to it.Conditional ExpectationIt is the mean of a variable conditional on the value of another random variable.Note the notation: E(Y|X).In 1996, per-capita average wages in three Chinese cities were (in RMB):Shanghai: 3,778Wuhan: 1,709Xi’an: 1,155Variance of Random Variable: The variance of a discrete random variable X is denoted by V{X} and defined:V{X}=(x i - E{X})2 P(x i)where: P(x i) denotes P(X = x i). The notation V{ } (read “variance of”) is called the variance operator.Since the variance of a random variable X is a weighted average of the squared deviations, (X - E{X})2 , it may be defined equivalently as an expected value: V{X} = E{(X - E{X})2}. An algebraically identical expression is: V{X} = E{X2} - (E{X})2.Standard Deviation of Random Variable: The positive square root of the variance of X is called the standard deviation of X and is denoted by σ{X}:σ{X} =The notation σ{ } (read “standard deviation of”) is called the standard deviation operator. Standardized Random Variables: If X is a random variable with expected value E{X} and standard deviation σ{X}, then:Y=}{}{ X XEXσ-is known as the standardized form of random variable X.Covariance: The covariance of two discrete random variables X and Y is denoted by Cov{X,Y} and defined:Cov{X,Y} =where: P(x i, y j) denotes )The notation of Cov{ , } (read “covariance of”) is called the covariance operator.When X and Y are independent, Cov {X,Y} = 0.Cov {X,Y} = E{(X - E{X})(Y - E{Y})}; Cov {X,Y} = E{XY} - E{X}E{Y}(Variance is a special case of covariance.)Coefficient of Correlation: The coefficient of correlation of two random variables X and Y is denoted by ρ{X,Y} (Greek rho) and defined:where: σ{X} is the standard deviation of X; σ{Y} is the standard deviation of Y; Cov is the covariance of X and Y.Sum and Difference of Two Random Variables: If X and Y are two random variables, then the expected value and the variance of X + Y are as follows:Expected Value: E{X+Y} = E{X} + E{Y};Variance: V{X+Y} = V{X} + V{Y}+ 2 Cov(X,Y).If X and Y are two random variables, then the expected value and the variance of X - Y are as follows:Expected Value : E {X - Y } = E {X } - E {Y };Variance : V {X - Y } = V {X } + V {Y } - 2 Cov (X,Y ).Sum of More Than Two Independent Random Variables: If T = X 1 + X 2 + ... + X s is the sum of sindependent random variables, then the expected value and the variance of T are as follows:Expected Value: ; Variance:III(2). Properties of Expectations and Covariances:(1) Properties of Expectations under Simple Algebraic Operations)()(x bE a bX a E +=+This says that a linear transformation is retained after taking an expectation.bX a X +=*is called rescaling: a is the location parameter, b is the scale parameter.Special cases are:For a constant: a a E =)(For a different scale: )()(X E b bX E =, e.g., transforming the scale of dollars intothe scale of cents.(2) Properties of Variances under Simple Algebraic Operations)()(2X V b bX a V =+This says two things: (1) Adding a constant to a variable does not change the varianceof the variable; reason: the definition of variance controls for the mean of the variable[graphics]. (2) Multiplying a constant to a variable changes the variance of the variable by a factor of the constant squared; this is to easy prove, and I will leave it to you. This is the reason why we often use standard deviation instead of variance2x x σσ=is of the same scale as x.(3) Properties of Covariance under Simple Algebraic OperationsCov(a + bX, c + dY) = bd Cov(X,Y).Again, only scale matters, location does not.(4) Properties of Correlation under Simple Algebraic OperationsI will leave this as part of your first exercise:),(),(Y X dY c bX a ρρ=++That is, neither scale nor location affects correlation.IV: Basics of matrix.1. DefinitionsA. MatricesToday, I would like to introduce the basics of matrix algebra. A matrix is a rectangular array of elements arranged in rows and columns:11121211.......m n nm x x x x X x x ⎡⎤⎢⎥⎢⎥=⎢⎥⎢⎥⎣⎦Index: row index, column index.Dimension: number of rows x number of columns (n x m)Elements: are denoted in small letters with subscripts.An example is the spreadsheet that records the grades for your home work in the following way:Name 1st 2nd ....6thA 7 10 (9)B 6 5 (8)... ... ......Z 8 9 (8)This is a matrix.Notation: I will use Capital Letters for Matrices.B. VectorsVectors are special cases of matrices:If the dimension of a matrix is n x 1, it is a column vector:⎥⎥⎥⎥⎦⎤⎢⎢⎢⎢⎣⎡=n x x x x (21)If the dimension is 1 x m, it is a row vector: y' = | 1y 2y .... m y |Notation: small underlined letters for column vectors (in lecture notes)C. TransposeThe transpose of a matrix is another matrix with positions of rows and columns being exchanged symmetrically.For example: if⎥⎥⎥⎥⎦⎤⎢⎢⎢⎢⎣⎡=⨯nm n m m n x x x x x x X 12111211)( (1121112)()1....'...n m n m nm x x x x X x x ⨯⎡⎤⎢⎥⎢⎥=⎢⎥⎢⎥⎣⎦It is easy to see that a row vector and a column vector are transposes of each other. 2. Matrix Addition and SubtractionAdditions and subtraction of two matrices are possible only when the matrices have the same dimension. In this case, addition or subtraction of matrices forms another matrix whoseelements consist of the sum, or difference, of the corresponding elements of the two matrices.⎥⎥⎥⎥⎦⎤⎢⎢⎢⎢⎣⎡±±±±±=Y ±X mn nm n n m m y x y x y x y x y x (11)2121111111 Examples:⎥⎦⎤⎢⎣⎡=A ⨯4321)22(⎥⎦⎤⎢⎣⎡=B ⨯1111)22(⎥⎦⎤⎢⎣⎡=B +A =⨯5432)22(C 3. Matrix MultiplicationA. Multiplication of a scalar and a matrixMultiplying a scalar to a matrix is equivalent to multiplying the scalar to each of the elements of the matrix.11121211Χ...m n nm cx c cx cx ⎢⎥⎢⎥=⎢⎥⎢⎥⎣⎦ B. Multiplication of a Matrix by a Matrix (Inner Product)The inner product of matrix X (a x b) and matrix Y (c x d) exists if b is equal to c. The inner product is a new matrix with the dimension (a x d). The element of the new matrix Z is:c∑=kj ik ij y x zk=1Note that XY and YX are very different. Very often, only one of the inner products (XY and YX) exists.Example:⎥⎦⎤⎢⎣⎡=4321)22(x A⎥⎦⎤⎢⎣⎡=10)12(x BBA does not exist. AB has the dimension 2x1⎥⎦⎤⎢⎣⎡=42ABOther examples:If )53(x A , )35(x B , what is the dimension of AB? (3x3)If )53(x A , )35(x B , what is the dimension of BA? (5x5)If )51(x A , )15(x B , what is the dimension of AB? (1x1, scalar)If )53(x A , )15(x B , what is the dimension of BA? (nonexistent)4. Special MatricesA. Square Matrix)(n n A ⨯B. Symmetric MatrixA special case of square matrix.For )(n n A ⨯, ji ij a a =. All i, j .A' = AC. Diagonal MatrixA special case of symmetric matrix⎥⎥⎥⎥⎦⎢⎢⎢⎢⎣=X nn x x 0 (2211)D. Scalar Matrix0....0c c c c ⎡⎤⎢⎥⎢⎥=I ⎢⎥⎢⎥⎣⎦E. Identity MatrixA special case of scalar matrix⎥⎥⎥⎥⎦⎤⎢⎢⎢⎢⎣⎡=I 10 (101)Important: for r r A ⨯AI = IA = AF. Null (Zero) MatrixAnother special case of scalar matrix⎥⎥⎥⎥⎦⎤⎢⎢⎢⎢⎣⎡=O 00 (000)From A to E or F, cases are nested from being more general towards being more specific.G. Idempotent MatrixLet A be a square symmetric matrix. A is idempotent if....32=A =A =AH. Vectors and Matrices with elements being oneA column vector with all elements being 1,⎥⎥⎥⎥⎦⎤⎢⎢⎢⎢⎣⎡=⨯1......111r A matrix with all elements being 1, ⎥⎥⎥⎥⎦⎤⎢⎢⎢⎢⎣⎡=⨯1...1...111...11rr J Examples let 1 be a vector of n 1's: )1(1⨯n 1'1 = )11(⨯n11' = )(n n J ⨯I. Zero Vector A zero vector is⎥⎥⎥⎥⎦⎤⎢⎢⎢⎢⎣⎡=⨯0....001r 5. Rank of a MatrixThe maximum number of linearly independent rows is equal to the maximum number of linearly independent columns. This unique number is defined to be the rank of the matrix.For example,⎥⎥⎥⎦⎤⎢⎢⎢⎣⎡=B 542211014321 Because row 3 = row 1 + row 2, the 3rd row is linearly dependent on rows 1 and 2. The maximum number of independent rows is 2. Let us have a new matrix:⎥⎦⎤⎢⎣⎡=B 11014321* Singularity: if a square matrix A of dimension ()n n ⨯has rank n, the matrix is nonsingular. If the rank is less than n, the matrix is then singular.。
学术英语(社科)Unit2二单元原文及翻译
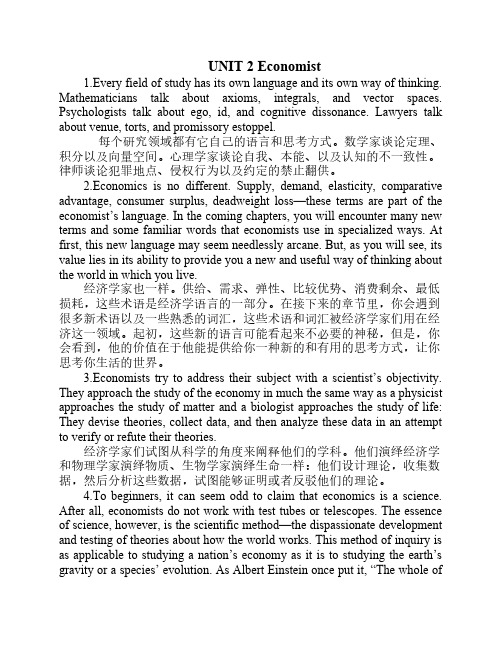
UNIT 2 Economist1.Every field of study has its own language and its own way of thinking. Mathematicians talk about axioms, integrals, and vector spaces. Psychologists talk about ego, id, and cognitive dissonance. Lawyers talk about venue, torts, and promissory estoppel.每个研究领域都有它自己的语言和思考方式。
数学家谈论定理、积分以及向量空间。
心理学家谈论自我、本能、以及认知的不一致性。
律师谈论犯罪地点、侵权行为以及约定的禁止翻供。
2.Economics is no different. Supply, demand, elasticity, comparative advantage, consumer surplus, deadweight loss—these terms are part of the economist’s language. In the coming chapters, you will encounter many new terms and some familiar words that economists use in specialized ways. At first, this new language may seem needlessly arcane. But, as you will see, its value lies in its ability to provide you a new and useful way of thinking about the world in which you live.经济学家也一样。
C++中vector使用详细说明(转)

C++中vector使⽤详细说明(转)转⾃:⼀、向量的介绍向量 vector 是⼀种对象实体, 能够容纳许多其他类型相同的元素, 因此⼜被称为容器。
与string相同, vector 同属于STL(Standard Template Library, 标准模板库)中的⼀种⾃定义的数据类型, 可以⼴义上认为是数组的增强版。
在使⽤它时, 需要包含头⽂件 vector, #include<vector>vector 容器与数组相⽐其优点在于它能够根据需要随时⾃动调整⾃⾝的⼤⼩以便容下所要放⼊的元素。
此外, vector 也提供了许多的⽅法来对⾃⾝进⾏操作。
⼆、向量的声明及初始化vector 型变量的声明以及初始化的形式也有许多, 常⽤的有以下⼏种形式:vector<int> a ; //声明⼀个int型向量avector<int> a(10) ; //声明⼀个初始⼤⼩为10的向量vector<int> a(10, 1) ; //声明⼀个初始⼤⼩为10且初始值都为1的向量vector<int> b(a) ; //声明并⽤向量a初始化向量bvector<int> b(a.begin(), a.begin()+3) ; //将a向量中从第0个到第2个(共3个)作为向量b的初始值除此之外, 还可以直接使⽤数组来初始化向量:int n[] = {1, 2, 3, 4, 5} ;vector<int> a(n, n+5) ; //将数组n的前5个元素作为向量a的初值vector<int> a(&n[1], &n[4]) ; //将n[1] - n[4]范围内的元素作为向量a的初值三、元素的输⼊及访问元素的输⼊和访问可以像操作普通的数组那样, ⽤cin>>进⾏输⼊, cout<<a[n]这样进⾏输出:⽰例:1 #include<iostream>2 #include<vector>34 using namespace std ;56 int main()7 {8 vector<int> a(10, 0) ; //⼤⼩为10初值为0的向量a910 //对其中部分元素进⾏输⼊11 cin >>a[2] ;12 cin >>a[5] ;13 cin >>a[6] ;1415 //全部输出16 int i ;17 for(i=0; i<a.size(); i++)18 cout<<a[i]<<" " ;1920 return 0 ;21 }在元素的输出上, 还可以使⽤遍历器(⼜称迭代器)进⾏输出控制。
线性代数英文ppt3

Examples
(1) V={ …, -3, -1, 1, 3, 5, 7, …} V is not closed under addition because 1+3=4 V.
(2)
Z={ …, -2, -1, 0, 1, 2, 3, 4, …}
Z is closed under addition because for any a, b Z, a + b Z. Z is not closed under scalar multiplication because ½ is a scalar, for any odd a Z, (½)a Z.
Ch04_9
The Complex Vector Space Cn
Let (u1 , ..., un ) be a sequence of n complexnumbers. T heset of all such sequences is denotedC n . Let operat ions of addition and scalar multiplica tion (by a complexscalar c) be defined on C n as follows:
[ f (- f )](x) f ( x) (- f )(x) f ( x) - [ f ( x)] 0 0( x)
Thus [f + (-f )] = 0, -f is the negative of f.
V={ f | f(x)=ax2+bx+c for some a,b,c R}
Ch04_11
Subspaces
In general, a subset of a vector space may or may not satisfy the closure axioms. However, any subset that is closed under both of these operations satisfies all the other vector space properties.
1.1-1.2 vector and space coordinates
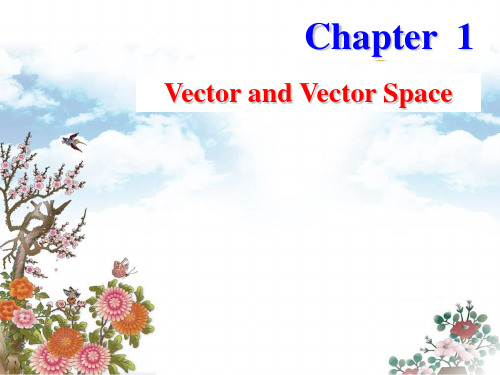
a b
ab
Suppose that a and b are any two vectors and O is any point . If we draw a vector OA from O to A and then draw the vector AB b starting from the terminal point A of a, then the vector OB is called the sum of a and b , denoted by a b , that is , a b OB, or OA AB OB
Definition 8
Put vectors 1,2 ,,k to the same initial
point. If their terminal points and their coinitial point are on the same plane we call them coplane.
a a ( 2) 0, a 0 ( 3) 0, a and a has opposite direction, a | | a a 1 2a a 2
rectangular coordinate system in space 空间直角坐标系
The right-hand rule 右手规则 octant 卦限
Coordinate decomposition of vector 向量的坐标分解式 Moduli 模 Direction cosine of vector 向量的方向余弦
M 1 :initial point,M 2 :terminal point.
vector的名词解释
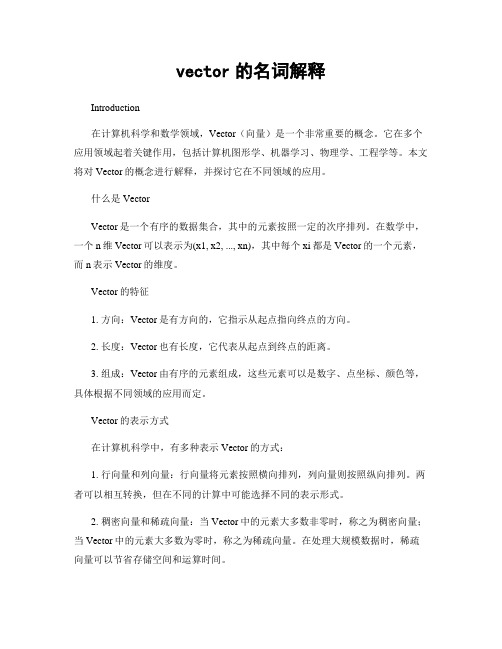
vector的名词解释Introduction在计算机科学和数学领域,Vector(向量)是一个非常重要的概念。
它在多个应用领域起着关键作用,包括计算机图形学、机器学习、物理学、工程学等。
本文将对Vector的概念进行解释,并探讨它在不同领域的应用。
什么是VectorVector是一个有序的数据集合,其中的元素按照一定的次序排列。
在数学中,一个n维Vector可以表示为(x1, x2, ..., xn),其中每个xi都是Vector的一个元素,而n表示Vector的维度。
Vector的特征1. 方向:Vector是有方向的,它指示从起点指向终点的方向。
2. 长度:Vector也有长度,它代表从起点到终点的距离。
3. 组成:Vector由有序的元素组成,这些元素可以是数字、点坐标、颜色等,具体根据不同领域的应用而定。
Vector的表示方式在计算机科学中,有多种表示Vector的方式:1. 行向量和列向量:行向量将元素按照横向排列,列向量则按照纵向排列。
两者可以相互转换,但在不同的计算中可能选择不同的表示形式。
2. 稠密向量和稀疏向量:当Vector中的元素大多数非零时,称之为稠密向量;当Vector中的元素大多数为零时,称之为稀疏向量。
在处理大规模数据时,稀疏向量可以节省存储空间和运算时间。
Vector的应用1. 计算机图形学:在计算机图形学中,Vector广泛应用于绘制图像、计算物体的位置、描述光线的传播等。
例如,通过使用2D Vector的x和y坐标,可以确定一个点的位置;而3D Vector的x、y和z坐标可以确定一个物体在3D空间中的位置。
2. 机器学习:在机器学习领域,Vector用于表示特征向量。
特征向量是将一个对象转换为一个Vector,以便计算机可以对其进行分类、识别等操作。
例如,对于图像分类任务,可以使用向量来表示图像的像素信息。
3. 物理学:在物理学中,Vector用于描述力的作用、速度、加速度等物理量的方向和大小。
大学数学专业英语教材
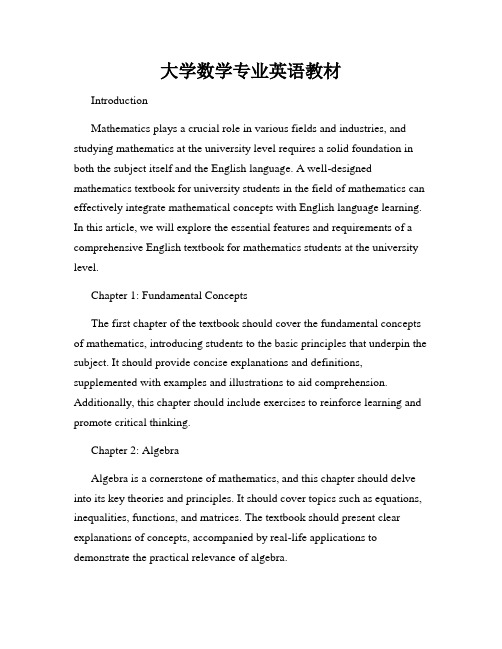
大学数学专业英语教材IntroductionMathematics plays a crucial role in various fields and industries, and studying mathematics at the university level requires a solid foundation in both the subject itself and the English language. A well-designed mathematics textbook for university students in the field of mathematics can effectively integrate mathematical concepts with English language learning. In this article, we will explore the essential features and requirements of a comprehensive English textbook for mathematics students at the university level.Chapter 1: Fundamental ConceptsThe first chapter of the textbook should cover the fundamental concepts of mathematics, introducing students to the basic principles that underpin the subject. It should provide concise explanations and definitions, supplemented with examples and illustrations to aid comprehension. Additionally, this chapter should include exercises to reinforce learning and promote critical thinking.Chapter 2: AlgebraAlgebra is a cornerstone of mathematics, and this chapter should delve into its key theories and principles. It should cover topics such as equations, inequalities, functions, and matrices. The textbook should present clear explanations of concepts, accompanied by real-life applications to demonstrate the practical relevance of algebra.Chapter 3: CalculusCalculus is essential for advanced mathematics and the study of other disciplines such as physics and engineering. The textbook should guide students through both differential and integral calculus, ensuring a thorough understanding of concepts like limits, derivatives, and integrals. Practical examples and exercises should be incorporated to enhance students' problem-solving skills.Chapter 4: Probability and StatisticsIn this chapter, the textbook should introduce students to probability theory and statistical analysis. The content should cover topics such as probability distributions, hypothesis testing, and regression analysis. The inclusion of real-world data sets and case studies can foster students' ability to apply statistical methods effectively.Chapter 5: Discrete MathematicsDiscrete mathematics is vital in areas like computer science and cryptography. This chapter should explore concepts such as set theory, logic, graph theory, and combinatorics. The textbook should present clear explanations of these topics, accompanied by relevant examples and exercises to consolidate understanding.Chapter 6: Linear AlgebraLinear algebra is widely applicable in various fields, including computer science and physics. This chapter should cover vector spaces, linear transformations, and eigenvalues. Emphasis should be placed on theconnections between linear algebra and other mathematical disciplines, demonstrating its practical significance.Chapter 7: Number TheoryNumber theory explores the properties and relationships of numbers, and it forms the basis for cryptographic algorithms and computer security systems. This chapter should introduce students to prime numbers, modular arithmetic, and cryptographic algorithms. Examples and exercises should be given to develop students' problem-solving skills in the realm of number theory.Chapter 8: Numerical AnalysisNumerical analysis involves using algorithms to solve mathematical problems on computers. This chapter should cover topics such as interpolation, numerical integration, and numerical solutions of equations. The textbook should provide step-by-step guidance on implementing numerical algorithms, allowing students to develop practical coding skills.ConclusionA comprehensive English textbook for university-level mathematics students should provide a solid foundation in mathematical concepts while simultaneously enhancing students' English language proficiency. By incorporating clear explanations, practical examples, and engaging exercises, this textbook can foster a deep understanding of mathematics within an English language learning context. Such a resource will empower students to pursue further studies in mathematics and apply their skills in various professional domains.。
工程电磁场第一章解读
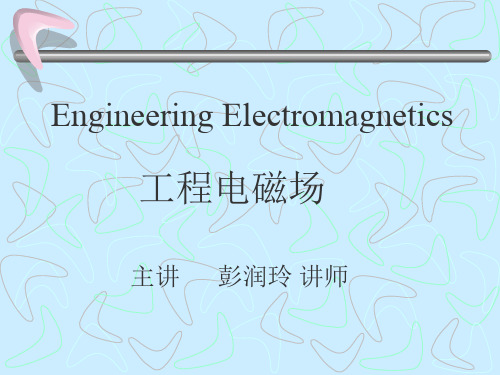
A B B A
1.2 Vector Algebra
3. Vector---two or three components
4. Vector subtraction A B A (B)
1. Three coordinate systems
Cartesian system or rectangular system Circular cylindrical system Spherical coordinate system
2. The Cartesian coordinate system has three axes
3. In other coordinate systems, points are also located at the common intersection of three surfaces, not necessarily planes, but still normal at the point of intersection. 4. Shown in Fig.1.2c, the coordinate of P is x,y,z respectively and that of Q is x+dx, y+dy, z+dz.
2. A vector:
What’s a vector?
3. Scalar and Vector fields
A field is defined as some function of that vector which connects an arbitrary origin to a general point in space.
矩阵分析英文课件1
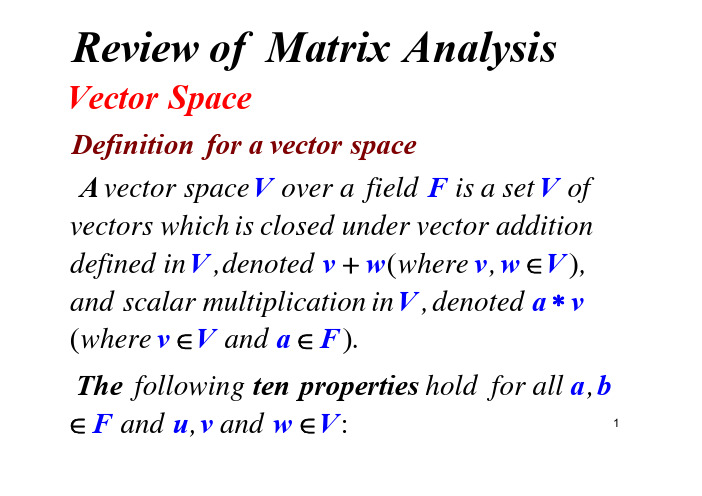
(Neutrality y of f one, , If f 1 denotes the multiplicat p ive identity of the field F ) 9. a (v w ) = a v a w (Distributivity with respect to vector addition) 10. (a b) v = a v b v (Distributivity with respect to field addition)
5
Basis
Any linearly independent set which spans a vector space V is called a basis for V . A vector t space V is i called ll d finite fi it dimensional di i l if it has h a basis. b i Basis are not unique.
Extension to a basis
If {v1 , v 2 , , vk } is i a linearly li l independent i d d set of f a finite fi i dimensional vector space V , there exist additional vectors vk 1 , vk 2 , , vn V such h that h {v 1 , v 2 , , v k , v k 1 , , v n } is a basis of V . The extension of a independent set to a basis is not unique.
英文版高等数学教材推荐
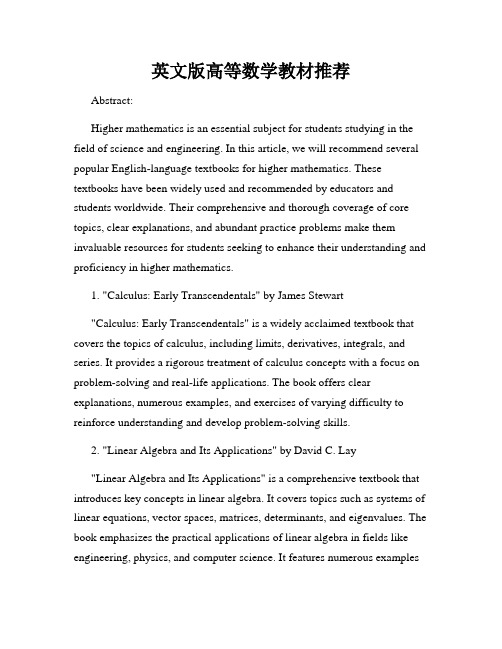
英文版高等数学教材推荐Abstract:Higher mathematics is an essential subject for students studying in the field of science and engineering. In this article, we will recommend several popular English-language textbooks for higher mathematics. These textbooks have been widely used and recommended by educators and students worldwide. Their comprehensive and thorough coverage of core topics, clear explanations, and abundant practice problems make them invaluable resources for students seeking to enhance their understanding and proficiency in higher mathematics.1. "Calculus: Early Transcendentals" by James Stewart"Calculus: Early Transcendentals" is a widely acclaimed textbook that covers the topics of calculus, including limits, derivatives, integrals, and series. It provides a rigorous treatment of calculus concepts with a focus on problem-solving and real-life applications. The book offers clear explanations, numerous examples, and exercises of varying difficulty to reinforce understanding and develop problem-solving skills.2. "Linear Algebra and Its Applications" by David C. Lay"Linear Algebra and Its Applications" is a comprehensive textbook that introduces key concepts in linear algebra. It covers topics such as systems of linear equations, vector spaces, matrices, determinants, and eigenvalues. The book emphasizes the practical applications of linear algebra in fields like engineering, physics, and computer science. It features numerous examplesand exercises to strengthen conceptual understanding and problem-solving abilities.3. "Differential Equations: An Introduction to Modern Methods and Applications" by James R. Brannan and William E. BoyceThis textbook provides a comprehensive introduction to differential equations, a fundamental topic in higher mathematics. It covers first-order, second-order, and higher-order differential equations, as well as systems of differential equations. The book presents clear explanations of various solution techniques and demonstrates their practical applications through real-world examples. It also offers a variety of exercises to reinforce understanding and develop problem-solving skills.4. "Probability and Statistics for Engineers and Scientists" by Ronald E. Walpole, Raymond H. Myers, Sharon L. Myers, and Keying Ye"Probability and Statistics for Engineers and Scientists" is a widely used textbook in the field of probability and statistics. It introduces statistical concepts and techniques essential for analyzing experimental data and making informed decisions. The book covers topics such as probability, random variables, statistical inference, regression analysis, and experimental design. It includes numerous examples, exercises, and real-life case studies to facilitate the application of statistical methods in practical situations.Conclusion:These recommended English-language textbooks provide comprehensive coverage of higher mathematics topics and offer clear explanations, examples, and exercises. They have proven to be valuable resources forstudents studying higher mathematics and have been widely used by educators worldwide. By utilizing these textbooks, students can enhance their understanding and proficiency in higher mathematics, ultimately laying a solid foundation for their academic and professional pursuits in science and engineering.。
Lecture notes

Introduction to symplectic topologyLecture notes1.Linear symplectic geometry.1.1.Let V be a vector space andωa non-degenerate skew-symmetric bilinear form on V.Suchωis called a linear symplectic structure.We writeω(u,v)for u,v∈V.The only difference with(pseudo)Euclidean structure is that the latter is symmetric.Fix a dot product in V.Then one can write:ω(u,v)=Ju·vwhere J is non-degenerate operator on V.Sinceωis skew-symmetric,J∗=−J.Taking determinants,det J∗=det J=(−1)n det Jwhere n=dim V.Thus n is even.Examples. 1.The plane with an area form(i.e.,cross-product)is a symplectic space.All2-dimensional symplectic spaces are symplectomorphic to this one.In formulas,ω=dp∧dq(using the language of linear differential forms).2.One can take direct sum of the previous example to obtain symplectic R2n with the symplectic structure dp∧dq=dp1∧dq1+...+dp n∧dq n.One has:ω(q i,q j)=ω(p i,p j)=0 andω(p i,q j)=δij.This is a symplectic basis;the respective coordinates are called Darboux coordinates.3.More conceptually,let W be a vector space.Then V=W⊕W∗is a symplectic space.The structure is as follows:ω((u1,l1),(u2,l2))=l1(u2)−l2(u1).Check that this is non-degenerate.Exercise.Let J be a skew-symmetric matrix:J∗=−J.Then det J is a polynomial in the entries of J,,and this polynomial is the square of another polynomial in the entries of J:det J=(Pf J)2.The latter is called Pfaffian.Show thatPf(A∗JA)=det A Pf J.As in Euclidean geometry,one defines(skew)orthogonal complement of a space.Unlike Euclidean geometry,one may have:U⊂U⊥.For example,this is the case when dim U=1.If U⊂U⊥then U is called isotropic.One has:dim U⊥=2n−dim U where2n is the dimension of the ambient space.Thus if U is isotropic then dim U≤n.An isotropic subspace of dimension n is called Lagrangian.Exercises. 1.Let A:W→W∗be a linear map.Then A∗=A if and only if the graph GrA⊂W⊕W∗is a Lagrangian subspace(with respect to the structure of Example 3above).2.Given two symplectic spaces(V1,ω1)and(V2,ω2)of the same dimensions and a linear map A:V1→V2,the map A is a symplectomorphism if and only if GrA⊂V1⊕V2 is a Lagrangian subspace with respect to the symplectic structureω1 ω2.1.2.Similarly to Euclidean spaces,the dimension is the only linear symplectic invari-ant.Linear Darboux Theorem.Two symplectic spaces of the same dimension are linearly symplectomorphic.Proof.Given a symplectic space V2n,pick a Lagrangian subspace W⊂V.To construct W,choose v1∈V,consider v⊥1,choose v2∈v⊥1,consider v⊥1∩v⊥2,choose v3∈v⊥1∩v⊥2,etc.,until one has v1,...,v n such thatω(v i,v j)=0.These vectors span a Lagrangian space.Claim:V is linearly symplectomorphic to the symplectic space W⊕W∗of Example 3in1.1.To see this,pick another Lagrangian subspace U,transverse to W.Then U is identified with W∗:the pairing between U and W is given byω.Since V=U⊕W,we have the desired symplectomorphism.Thus,one may choose one’s favorite model of a symplectic space.For example,one√may identify R2n with C n,and then J from1.1is the operator of multiplication byExample.Λ1is the space of lines through the origin in the plane,i.e.,RP1,topo-logically,a circle.Exercise∗.What is the topology ofΛ2?Describe a related classical construction realizingΛ2as a quadratic hypersurface of signature(+++−−)in RP4.Let the symplectic space be R4withω=p1∧q1+p2∧q2. Given a2-plane U,choose u1,u2∈U and consider the bivectorφ=u1∧u2.Thus we assignφ∈Λ2U,andφis defined up to a factor.We have constructed a map G2,2→P(Λ2U)=RP5.The bivectors inΛ2U corresponding to2-planes,satisfyφ∧φ=0(and this is sufficient too).Thus G2,2is realized a quadratic hypersurface in RP5of signature (+++−−−).If U is a Lagrangian plane thenφ∧ω=0(why?)and this is also sufficient.This is a linear condition that determines a hyperplane RP4⊂RP5.This hyperplane is transverse to the image of G2,2(why?),and the intersection is the Lagrangian Grassmanian.1.4.Given a symplectic space(V2n,ω),the group of linear symplectomorphisms is called the linear symplectic group and denoted by Sp(V)or Sp(2n,R).A symplectic space has a volume elementω∧n,therefore Sp(2n)is a subgroup of SL(2n).Let A∈Sp(2n).Thenω(Au,Av)=ω(u,v)for all u,v.Thus A∗JA=J.This is interesting to compare with the orthogonal group:A∗A=E.The relations between the classical groups are as follows.Lemma.One has:Sp(2n)∩O(2n)=Sp(2n)∩GL(n,C)=O(2n)∩GL(n,C)=U(n).Proof.One has:A∈GL(n,C)iffAJ=JA;A∈Sp(2n)iffA∗JA=J;andA∈O(2n)iffA∗A=E.Any two of these conditions imply the third.A linear map that preserves the Euclidean and the symplectic structures also preserves the Hermitian one,that is,belongs to U(n).Exercises.1.Let A∈Sp(2n)andλbe an eigenvalue of A.Prove that so are¯λand 1/λ.2.Prove that if A is symplectic then A∗is antisymplectic,that is,ω(A∗u,A∗v)=−ω(u,v)or,equivalently,AJA∗=−J.In fact,U(n)is the maximal compact subgroup of Sp(2n),and the latter is homo-topically equivalent to the former.As a consequence,π1(Sp(2n))=Z.Indeed,one has afibration det:U(n)→S1withfiber SU(n).The latter group is simply connected as follows inductively from the exact homotopy sequence of thefibration SU(n)→S2n−1 withfiber SU(n−1).1.5.Let us describe the Lie algebra sp(2n)of the Lie group Sp(2n).Let A∈Sp(2n) be close to the identity:A=E+tH+O(t2).Then the conditionω(Au,Av)=ω(u,v)for all u,v implies:ω(Hu,v)+ω(u,Hv)=0;in other words,JH+H∗J=0.Thus H is skew-symmetric with respect toω.Such H is called a Hamiltonian operator.The commutator of Hamiltonian operators is again a Hamiltonian operator.To a Hamiltonian operator there corresponds a quadratic form h(u)=ω(u,Hu)/2 called the Hamiltonian(function)of H.One can recover H from h sinceω(u,Hv)= h(u+v)−h(u)−h(v).This gives a one-one correspondence between sp(2n)and quadratic forms on V2n.Thus dim sp(2n)=n(2n+1).In terms of quadratic forms,the commutator writes as follows:{h1,h2}(u)=ω(u,(H2H1−H1H2)u)/2=ω(H1u,H2u).The operation on the LHS is called the Poisson bracket.To write formulas,it is convenient to identify linear operators with linear vectorfields: the operator H is understood as the linear differential equation u =Hu.Let(p,q)be Darboux coordinates.Lemma.The next formulas hold:H=h p∂q−h q∂p;{h1,h2}=(h1)p(h2)q−(h1)q(h2)p.Proof.To prove thefirst formula we need to show that2h=ω((p,q),(−h q,h p)).The RHS is ph p+qh q=2h,due to the Euler formula.Then the Poisson bracket is given by{h1,h2}=ω(((h2)p,−(h2)q),((h1)p,−(h1)q))=(h1)p(h2)q−(h1)q(h2)p,as claimed.More conceptually,given a quadratic form h,one considers its differential dh which is a linear differential1-form.The symplectic structure determines a linear isomorphism V→V∗which makes dh into a linear vectorfield H,that is,i Hω=−dh.1.6.One of thefirst,and most celebrated,results of symplectic topology was Gromov’s nonsqueezing theorem(1985).Let us discuss its linear version(which is infinitely simpler).Given a ball B2n(r)of radius r and a symplectic cylinder C(R)=B2(R)×R2n−2 (where the2-disc is spanned by the Darboux coordinates p1,q1),assume that there is an affine symplectic map F that takes B2n(r)inside C(R).Proposition.Then r≤R.Note that this is false for volume-preserving affine maps.Proof.The map writes F:v→Av+b where A∈Sp(2n)and b∈R2n.Assume r=1.Consider A∗and its two columnsξ1andξ2corresponding to p1,q1Darboux coordinates.Since A∗is antisymplectic(Exercise in1.4),|ω(ξ1,ξ2)|=1,and therefore |ξ1||ξ2|≥1.Assume that|ξ1|≥1,and let v=ξ1/|ξ1|.Note thatξ1andξ2are rows of A (corresponding to coordinates p1,q1).Since F(v)∈C(R),one has:(ξ1·v+b1)2+(ξ2·v+b2)2≤R2,and then|(|ξ1|+b1)|≤R.For b1≥0this implies R≥1,and for b1<0one should replace v by−v.One defines an affine symplectic invariant called linear symplectic width of a subset A⊂R2n:w(A)=max{πr2|F(B2n(r))⊂A for some affine symplectic F}. Symplectic width is monotonic:if A⊂B then w(B)≥w(A),homogeneous of degree2 with respect to dilations and nontrivial:w(B2n(r))=w(C(r))=πr2.To get a better feel of linear symplectic space,classify the ellipsoids.In Euclidean space,every ellipsoid can be written asnx2ii=1,r2iand the radii0≤r1≤...≤r n are uniquely defined.Proof.Recall how to prove the Euclidean fact.We have two Euclidean structures: u·v and Au·v.Here A is self-adjoint,and we assume,it is in general position.Consider a relative extremum problem of Au·u relative u·u.The extremum condition(Lagrange multipliers!)is that Audu=λudu,that is,Au=λu.The function Au·u is an even function on the unit sphere,that is,a function on RP n−1,and it has n critical points. Thus A has n real eigenvalues a1,...,a n,and the respective eigenspaces are orthogonal. We obtain the desired expression.A symplectic analog is as follows.We have a dot product and a symplectic structure ω(u,v)=Ju·v.Consider a relative extremum problem ofω(u,v)relative u·u and v·v. The extremum condition is:Ju dv−Jv du=λu du+µv dv,that is,Ju=µv,Jv=−λu.Thus u,v are eigenvectors of J2(with eigenvalue−λµ),a self-adjoint operator.In general position,these eigenspaces are2-dimensional and pairwise orthogonal.Thus the space is the orthogonal sum of2-dimensional subspaces.Claim:they are also symplectically orthogonal.Indeed,ω(u1,u2)=Ju1·u2=µ1v1·u2=0and,likewise,withω(u1,v2)andω(v1,v2).It remains to choose an orthogonal basis p i,q i in each2-space so that p i·p i=q i·q i andω(p i,q i)=1.The last thing to check is that the radii r i are uniquely defined.Let D(r)be the diagonal matrix with the entries1/r2i.Assume that,for a symplectic matrix A,one has:A∗D(r)A=D(r ).Since A is symplectic,A∗JA=J,or A∗=JA−1J−1.Thus A−1J−1D(r)A=J−1D(r ),that is,the eigenvalues of the matrices J−1D(r)and J−1D(r ) coincide.It follows that r=r .2.Symplectic manifolds.2.1.Let M be a smooth manifold.A symplectic structure on M is a non-degenerate closed2-formω.Since it is non-degenerate,dim M=2n.In other words,dω=0and ω∧...∧ω(n times)is a volume form.In particular,M is oriented.Also,H2(M,R)=0. Hence S2n,n≥2is not symplectic.A symplectomorphism is a diffeomorphism f:M→M such that f∗(ω)=ω.Sym-plectomorphisms form an infinite-dimensional group.2.2.Examples.(a)Linear symplectic space R2n withω=dp∧dq.(b)Any oriented surface with an area form.For example,S2,with the(standard) area formωx(u,v)=det(x,u,v).(c)(Archimedes)Consider the unit sphere and the circumscribed cylinder with its standard area form.Consider the radial projectionπfrom the sphere to the cylinder.Exercise.Prove thatπis a symplectomorphism.(d)The product of symplectic manifolds is a symplectic manifold.(e)Cotangent bundle(important for mechanics!).On T∗M one has a canonical1-formλcalled the Liouville(or action)form.Letπ:T∗M→M be the projection andξbe a tangent vector to T∗M at point(x,p).Define:λ(ξ)=p(π∗(ξ)).In coordinates,λ=pdq where q are local coordinates on M and p are the corresponding covectors(momenta).The canonical symplectic structure on T∗M isω=dλ,locally,dp∧dq.Exercise.Letαbe a1-form on M.Thenαdetermines a sectionγof the cotangent bundle.Prove thatγ∗(λ)=α.(f)CP n.First,consider C n+1=R2n+2with its linear symplectic structureΩ.Con-sider the unit sphere S2n+1.The restriction ofΩon S2n+1has a1-dimensional kernel. Claim:at point x,this kernel is generated by the vector Jx.Indeed,if u⊥x then Ω(Jx,u)=J(Jx)·u=0.The vectorfield Jx generated a foliation on circles,and the space of leaves is CP n.The symplectic structureΩinduces a new symplectic structureωon CP n.The construction is called symplectic reduction.Complex projective varieties are subvarieties of CP n;they have induced symplectic structures,and this is a common source of examples.(g)Another example of symplectic reduction:the space of oriented lines in R n+1. Start with T∗R n+1with its canonical symplectic structureΩ=dp∧dq.Consider the hypersurface|p|=1.Claim:the kernel of the restriction ofΩon this hypersurface at point(p,q)is generated by the vector p∂q.Indeed,(dp∧dq)(u,p∂q)=(pdp)(u)=0.We get a foliation whose leaves are oriented lines(geodesics).We obtain a symplectic structure on the space of oriented linesω=dp∧dq where p is a unit(co)vector and q·p=0.Exercise.Prove that the above space is symplectomorphic to T∗S n.(h)Orbits of the coadjoint representation of a Lie group.Let G be a Lie group and g its Lie algebra.The action of G on itself by conjugation has e as afixed point.Since g=T e G,one obtains a representation Ad of G in g called adjoint.Likewise,one has the coadjoint representation Ad∗in g∗.One also has the respective representations of g denoted by ad and ad∗.In formulas,ad x y=[x,y],(ad∗xξ)(y)=ξ([x,y]),x,y∈g,ξ∈g∗.Theorem(Lie,Kirillov,Kostant,Souriau).An orbit of the coadjoint representation of G has a symplectic structure.Proof.Letξ∈g∗.Then the tangent space to the orbit of the coadjoint representation atξidentifies with g/gξwheregξ={x∈g|ad∗xξ=0}.On the space g/gξone has a skew-symmetric bilinear formω(x,y)=ξ([x,y])(why is it well defined?).This2-form is closed as follows from the Jacobi identity for the Lie algebra g.Exercise.Prove the last statement.Example.Let G=SO(3),then g=so(3),skew-symmetric3×3matrices.Identify them with R3.Given A∈so(3),consider T r(A2).This gives a Euclidean structure on so(3)that agrees with that in R3.We identify g and g∗.Clearly,T r(A2)is invariant under the(co)adjoint action.The orbits are level surfaces of T r(A2),that is,concentric spheres and the origin.Exercise.Similarly study another3-dimensional Lie group,SL(2).2.3.Being non-degenerate,a symplectic form defines an isomorphism between vector fields and1-forms:X→i(X)ω.Afield is called symplectic if i(X)ωis closed,in other words,if L Xω=0;the latter follows from the Cartan formula:L X=di(X)+i(X)d. Symplecticfields form the Lie algebra of the group of symplectomorphisms.Let M be a closed symplectic manifold.Lemma.Given a1-parameter family of vectorfields X t,consider the respective family of diffeomorphismsφt:dφt(x)/dt=X t(φt(x)),φ0=Id.Thenφt are symplectomorphisms for all t iffX t are symplectic for all t.Given symplectic fields X and Y,thefield[X,Y]is symplectic with i([X,Y])ω=dω(X,Y).ω),and this implies thefirst claim.As to the Proof.One has:dφ∗t(ω)/dt=φ∗t(L Xtsecond,we use the(somewhat non-traditional)definition:[X,Y]=L Y X=dψ∗t X/dt|t=0,and theni([X,Y])ω=di(ψ∗t X)ω/dt|t=0=L Y i(X)ω=di(Y)i(X)ω=dω(X,Y),as claimed.Note that with the definition of the Lie bracket above one has L[X,Y]=−[L X,L Y] (cf.McDuff-Salamon,p.82).For linear symplectomorphisms,we had a relation with quadratic functions.Like-wise,given a(Hamiltonian)function H on M,define its Hamiltonian vectorfield X H by i(X H)ω=dH.In Darboux coordinates,X H=H p∂q−H q∂p.On closed M,this gives a 1-parameter group of symplectomorphisms called the Hamiltonianflow of H.Lemma.The vector X H is tangent to a level hypersurface H=const.Proof.One has:dH(X H)=ω(X H,X H)=0.This lemma is related to the symplectic reduction construction.If S is given by H=const then X H generates the characteristic foliation on S,tangent to thefield of kernels of the restriction ofωto S.Indeed,ω(X H,v)=dH(v)=0once v is tangent to S.Define the Poisson bracket{F,G}=ω(X F,X G)=dF(X G).In Darboux coordinates, the formulas are as in1.5.This bracket satisfies the Jacobi identity;we will deduce it from Darboux theorem.Lemma.The correspondence H→X H is a Lie algebra homomorphism.Proof.We want to show that[X F,X G]=X{F,G}.One has[X F,X G]=−dφ∗t(X G)/dt|t=0=−dX G(φtF )/dt|t=0.Theni([X F,X G])ω=−d(dG(φt F))/dt|t=0=−d(dG(X F))=d({F,G}),as claimed.To summarize,here are the main formulas,in Darboux coordinates:ω=dq∧dp;X H=H p∂q−H q∂p;{H,F}=H p F q−H q F p.Note that the Hamiltonian vectorfield X H is also often called the symplectic gradient of the function H.2.4.Unlike Riemannian manifolds,symplectic manifolds do not have local invariants. Darboux Theorem.Symplectic manifolds of the same dimension are locally symplecto-morphic.Proof.Consider two symplectic manifolds withfixed points(M1,O1,ω1)and (M2,O2,ω2).We want to construct a local symplectomorphism(M1,O1,ω1)→(M2,O2,ω2).First consider a local diffeomorphism(M1,O1)→(M2,O2);now we have two(germs of)symplectic structuresω0,ω1on the same manifold(M,O),and since there is only one symplectic vector space of a given dimension(the linear Darboux theorem),we assume thatω0andω1coincide at point O.Claim:There is a local diffeomorphism f:M→M,fixing O and such that f∗(ω0)=ω1.Consider the familyωt=(1−t)ω0+tω1.This is a symplectic structure for all t∈[0,1] in a small neighborhood of the origin.We need tofind a family of diffeomorphismsφt,fixing O,such thatφ∗tωt=ω0.This is equivalent tofinding a time-dependent symplectic vectorfield X t,related toφt in the usual way,dφt(x)/dt=X t(φt(x)),and vanishing at O, such thatL Xωt+ω1−ω0=0.tChoose a1-formαsuch that dα=ω1−ω0;thisαis defined up to summation with d f. Then we have the equationi(X t)ωt+α=0.This is solvable for allαsinceωt is non-degenerate.It remains to show that X t may be taken trivial at O.For this,we need to replaceαby a1-form that vanishes at O.Every1-form can be locally written asα= x iαi+ c i dx iwhereαi are1-forms and c i are constants.Then we replaceαbyα−d( c i x i),and this 1-form vanishes at O.In fact,a similar homotopy method,due to Moser,applies to a more general situation in which the points O1,O2are replaced by germs of submanifolds N1,N2such that the pairs(N1,ω1|N1)and(N2,ω2|N2)are symplectomorphic.2.5.A Lagrangian submanifold of a symplectic manifold(M2n,ω)is a manifold L n such thatω|L=0.An informal principle is that every symplectically meaningful object is a Lagrangian manifold.Examples.(a)Every curve is Lagrangian in a symplectic surface.(b)Consider T∗M with its canonical symplectic structure,and letαbe a1-form on M.This form determines a sectionγof T∗M whose image is Lagrangian iffαis closed. Indeed,ω|γ(M)=γ∗(ω)=dγ∗(λ)=dα.(c)Let N⊂M be a submanifold.Its conormal bundle P⊂T∗M|N consists of the covectors,equal to zero on N.Then P is Lagrangian.Indeed,choose local coordinates q1,...,q n in M so that q1=...=q k=0is N.Let p,q be the respective coordinates in T∗M.Then P is given by q1=...=q k=0,p k+1=...=p n=0.If N is a point,one obtains a“delta-function”.(d)Let f:(M1,ω1)→(M2,ω2)be a symplectomorphism.Then the graph G(f)is a Lagrangian submanifold in(M1×M2,ω1 ω2).Indeed,consider u1,v1∈T M1,and let u2=Df(u1),v2=Df(v1).Then(u1,u2)and(v1,v2)are two tangent vectors to G(f), and(ω1 ω2)((u1,u2),(v1,v2))=ω1(u1,v1)−ω2(u2,v2)=(ω1−f∗(ω2))(u1,v1)=0.(e)Let N n−1⊂R n be a hypersurface.Consider the set L of oriented normal lines to N;this is a Lagrangian submanifold of the space of oriented lines in R n.To provethis,recall that the space of oriented lines in R n is symplectomorphic to T∗S n−1with its symplectic structure dp∧dq;q∈S n−1,p∈T∗q S n−1.Let n(x)be the unit normal vector to N at point x∈N.Then L is given parametrically by the equationsq=n(x),p=x−(x·n(x))n(x),x∈N.Hencepdq=xdn−(x·n)ndn=xdn=d(x·n)−ndx=d(x·n),where ndn=0since n2=1and ndx=0on N since n is a normal.Therefore dp∧dq=0 on L.The function(x·n(x))is called the support function of the hypersurface N;it plays an important role in convex geometry.Let f:S n−1→R be a support function.How can one construct the corresponding hypersurface N?Claim:N is the locus of pointsy=f(x)x+grad f(x).Indeed,we need to show that x is a normal to N at point y,i.e.,xdy=0.Note that x grad f(x)=0hence x d grad f(x)+grad f(x)dx=0.Note also that x2=1hence xdx=0.Now one has:xdy=fxdx+x2d f+x d grad f(x)=d f−grad f(x)dx=0as needed.Exercise.Let L n−1be a submanifold of the space of oriented lines in R n.When does L consist of lines,orthogonal to a hypersurface?If this is the case,how many such hypersurfaces are there?Exercise.Let f:S1→R be the support function of a closed convex plane curve. Express the following characteristics of the curve in terms of f:curvature,area,perimeter length.Exercise∗.Let L be a Lagrangian submanifold in a symplectic manifold M.Prove that a sufficiently small neighborhood of L in M is symplectomorphic to a neighborhood of the zero section in T∗L.This statement is a version of Darboux theorem,and it can be proved along similar lines.2.6.A Lagrangian foliation is an n-dimensional foliation of a symplectic manifold M2n whose leaves are Lagrangian.Similarly one defines a Lagrangianfibration.An example is given by the cotangent bundle whosefibers are Lagrangian.An affine structure on an n-dimensional manifold is given by an atlas whose transi-tion maps are affine transformations.An affine manifold is complete if every line can be extended indefinitely.Examples include R n and n-torus.Theorem.The leaves of a Lagrangian foliation have a canonical affine structure.Proof.Let M2n be a symplectic manifold,F n a Lagrangian foliation and p:M→N n=M/F the(locally defined)projection.Consider a function F on N and extend itto M as F◦p.Let u be a tangent vector to a leaf.Then dF(u)=0.Therefore X F is skew-orthogonal to the tangent space of the leaf,that is,is tangent to the leaf.Then {F,G}=ω(X F,X G)=0,so the functions,constant on the leaves,Poisson commute.Fix a point x∈N.If F is a function on N such that dF(x)=0then X F=0on the leaf F x.Choose a basis in T∗x N,choose n functions F i whose differentials at x form.These are commuting vectorfields,and we this basis and consider the vectorfields X Fiobtain a locally effective action of R n on F x.This action is well defined if the quotient space N is defined,for example,if F is a Lagrangianfibration.In general,N is defined only locally,and going from one chart to another changes the respective commuting vectorfields by affine transformations.Thus an affine structure on the leaf is well defined.Corollary.If a leaf of a Lagrangian foliation is a closed manifold then it is a torus.Proof.If a leaf is complete then it is a quotient of R n by a discrete subgroup.If the leaf is compact,the subgroup is a lattice Z n.Here is what it boils down to in dimension2.A Lagrangian foliation is given by a function f(x,y):the leaves are the level curves.The function f is defined up to composition with functions of1variable:f→¯f=φ◦f.The vectorfield X f is tangent to the leaves and,on a leaf,one can introduce a parameter t such that X f=∂t.Changing f to¯f, thefield X f multiplies by a constantφ (depending on the leaf),and the parameter t also changes to¯t=ct.This parameter,defined up to a constant,give an affine structure.2.7.A consequence is the so-called Arnold-Liouville theorem in integrable systems. Theorem.Let M2n be a symplectic manifold with n functions F1,...,F n that Poisson commute:{F i,F j}=0.Consider a non-singular level manifold M c={F i=c i,i=1,...,n} and a Hamiltonian function H=H(F1,...,F n).Then M c is a smooth manifold,invariant under the vectorfield X H.There is an affine structure on M c in which thefield X H is constant.If M c is closed and connected then it is a torus.Proof.The mapping(F1,...,F n):M→R is afibration near the value c,and itsare constant in the respective affine coordinates,and leaves are Lagrangian.Thefields X FiX H is a linear combination of these nfields with the coefficients,constant on M c(why?) There is a version of this theorem in which X H is replaced by a symplectomorphismφ: M→M such that F i◦φ=F i for all i.Thenφpreserves M c and the affine structure therein.,and thereforeφis a parallel translation x→Moreover,φpreserves each vectorfield X Fix+c.Corollary.Letφandψbe two symplectomorphisms that preserve the same Lagrangian foliation leafwise.Thenφandψcommute.Proof.Both maps are parallel translations in the same affine coordinate system,and parallel translations commute.2.8.Billiards.An example of a symplectic map is provided by billiards.Considera strictly convex domain M⊂R n with a smooth boundary N n−1.Let U be the space of oriented lines that intersect M;it has a symplectic structure discussed in2.2.Consider the billiard map T:U→U given by the familiar law of geometrical optics:the incomingand outgoing rays lie in one2-plane with the normal at the impact point and make equal angles with this normal.Theorem.The billiard transformation is a symplectic map.Proof.Consider T∗M with its canonical symplectic structureω=dp∧dq where q,p are the usual coordinates.We identify tangent and cotangent vectors by Euclidean structure.Consider two hypersurfaces in T∗M:Y={(q,p)|p2=1},Z={(q,p)|q∈N}.The characteristics of Y are oriented lines in R n(section2.2,example g),and the sym-plectic reduction yields U with its symplectic structure.What are characteristics of Z? Consider the projectionπ:Z→T∗N given by the restriction of a covector on T N.Claim:the characteristics of Z are thefibers of the projectionπ.Indeed,let n(q)be the unit normal vector to N at point q∈N.Then thefibers ofπare integral curves of the vectorfield n(q)∂p.One has:i(n(q)∂p)ω=n(q)dq=0since n is a normal vector.It follows that the symplectic reduction of Z is the space V=T∗N.Let W=Y∩Z,the set of unit vectors with foot point on N.Consider W with the symplectic structureω|W.The projections of W on U and V along the leaves of the characteristic foliations of Y and Z are double coverings.These projections are symplectic mappings(why?)One obtains two symplectic involutionsσandτon W that interchange the preimages of a point under each projection.The billiard map T can be considered as a transformation of W equal toσ◦τ.Therefore T is a symplectomorphism.The proof shows that the billiard map can be also considered as a symplectic trans-formation of T∗N realized as the set of inward unit vectors with foot points on N.Exercise.Let n=2.Denote by t an arc length parameter along the billiard curve and byαthe angle between this curve and and the inward unit vector.The phase space of the billiard map is an annulus with coordinates(t,α).Prove that the invariant symplectic form is sinαdα∧dt.An alternative proof proceeds as follows.Let q1q2be an oriented line,q1,q2∈N.Let p1be the unit vector from q1to q2.The billiard map acts as follows:(q1,p1)→(q2,p2)N.Consider the where the covectors(q2,p1)and(q2,p2)have equal projections on T q2 generating function L(q1,q2)=|q1q2|.Then∂L/∂q1=−p1,∂L/∂q2=p1.Consider the Liouville formλ=pdq and restrict everything on T∗N.Then one has: T∗λ−λ=dL.Thereforeω=dλis T-invariant.Corollary.Billiard trajectories are extrema of the perimeter length function on polygons inscribed into N.Example.It is classically known that the billiard inside an ellipse is integrable:the invariant curves consist of the lines tangent to a confocal conic.Consider two confocal ellipses and the respective billiard transformations T1,T2.It follows from Corollary2.7that T1◦T2=T2◦T1,an interesting theorem of elementary geometry(especially its particular case,“The most elementary theorem of Euclidean geometry”)!Exercise∗.Let N be a smooth hypersurface in R n,and let X be the set of oriented lines in R n with its canonical symplectic structure.Consider the hypersurface Y⊂X that consists of the lines tangent to N.Prove that the characteristics of Y consist of the lines, tangent to a geodesic curve on N.3.Symplecticfixed points theorems and Morse theory.3.1.The next result was published by Poincar´e as a conjecture shortly before his death and proved by Birkhoffin1917.Consider the annulus A=S1×I with the standard area form and its area preserving diffeomorphism T,preserving each boundary circle and rotating them in the opposite directions.This means the a lifted diffeomorphism¯T of the strip S=R×[0,1]satisfies:¯T(x,0)=(X,0)with X>x and¯T(x,1)=(X,1)with X<x. Theorem(Poincar´e-Birkhoff).The mapping T has at least two distinctfixed points.Both conditions,that T is area preserving and that the boundary circles are rotated in the opposite sense,are necessary(why?).Proof.We prove the existence of onefixed point,the hardest part of the argument. Assume there are nofixed points.Consider the vectorfield v(x)=¯T(x)−x,x∈S.Let point x move from lower boundary to the upper one along a simple curveγ,and let r be the rotation of the vector v(x).This rotation is of the formπ+2πk,k∈Z.Note that r does not depend on the arcγ(why?).Note also that T−1has the same rotation r since the vector T−1(y)−y is opposite to T(x)−x for y=T(x).To compute r,letε>0be smaller than|T(x),x|for all x∈A;suchεexists because A is compact.Let Fεbe the vertical shift of the plane throughεand let¯Tε=Fε◦¯T. Consider the strip Sε=R×[0,ε].Its images under¯Tεare disjoint.Since¯Tεpreserves the area,the image of Sεwill intersect the upper boundary.Let k be the least number of needed iterations,and let P k be the upper most point of the upper boundary of this k-th iteration.Let P0,P1,...,P k the respective orbit,P0on the lower boundary of S.Join P0 and P1by a segment and consider its consecutive images:this is a simple arcγ.Forεsmall enough,the rotation r almost equals the winding number of the arcγ.In the limit ε→0,one has:r=π.Alternatively,we have a vectorfield v(x)=x1−x with x1=T(x)alongγ.One can homotop thisfield as follows:for1/2time freeze x at P0and let x1traverseγto P k,and for the other1/2time freeze x1at P k and let x traverseγ.Now consider the map T−1.Unlike T,it moves the lower boundary of S right and the upper one left.By the same argument,its rotation equals−π.On the other hand,by a remark above,this rotation equals that of T,a contradiction.A consequence is the existence of periodic billiard trajectories inside smooth strictly convex closed plane curves.The billiard transformation T is an area preserving map of the annulus A=S1×[0,π](we assume that the length of the curve is1).The map T。
1.1Vectors and Scalars
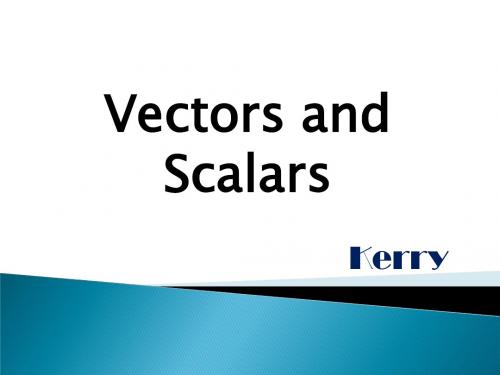
y=25 N
30º
x=43.3 N
We can see that it would be more efficient to pull the table with a horizontal force of 50 N.
If a vector of magnitude v and makes an angle θ with the horizontal then the magnitude of the components are: y=v Sin θ x = v Cos θ y θ y = v Sin θ Proof:
x Cos v x vCos y Sin v y vSin
x=v Cos θ x
A force of 15 N acts on a box as shown. What is the horizontal component of the force?
Solution:
Horizontal Component x 15Cos60 7.5 N Vertical Component
When resolving a vector into components we are doing the opposite to finding the resultant. We usually resolve a vector into components that are perpendicular to each other. Here a vector v is resolved into an x component and a y component.
A scalar quantity is a quantity that has magnitude only and has no direction in space.
线性代数 英文讲义
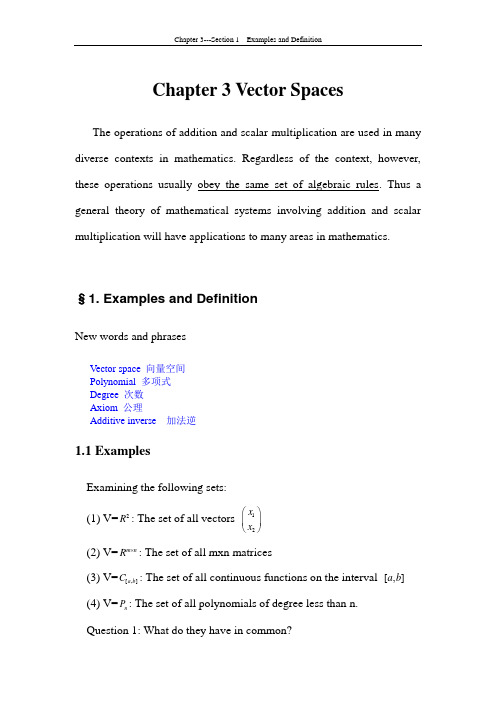
Chapter 3 Vector SpacesThe operations of addition and scalar multiplication are used in many diverse contexts in mathematics. Regardless of the context, however, these operations usually obey the same set of algebraic rules. Thus a general theory of mathematical systems involving addition and scalar multiplication will have applications to many areas in mathematics.§1. Examples and DefinitionNew words and phrasesVector space 向量空间Polynomial 多项式Degree 次数Axiom 公理Additive inverse 加法逆1.1 ExamplesExamining the following sets:(1) V=2R : The set of all vectors 12x x ⎛⎫ ⎪⎝⎭ (2) V=m n R ⨯: The set of all mxn matrices(3) V=[,]a b C : The set of all continuous functions on the interval [,]a b(4) V=n P : The set of all polynomials of degree less than n.Question 1: What do they have in common?We can see that each of the sets, there are two operations: addition and multiplication, i.e. with each pair of elements x and y in a set V, we can associate a unique element x+y that is also an element in V, and with each element x and each scalar α, we can associate a unique element αin V. And the operations satisfy some algebraic rules.xMore generally, we introduce the concept of vector space. .1.2 Vector Space Axioms★Definition Let V be a set on which the operations of addition and scalar multiplication are defined. By this we mean that, with each pair of elements x and y in a set V, we can associate a unique element x+y that is also an element in V, and with each element x and each scalar α, we can associate a unique element xαin V.The set V together with the operations of addition and scalar multiplication is said to form a vector space if the following axioms are satisfied.A1. x+y=y+x for any x and y in V.A2. (x+y)+z=x+(y+z) for any x, y, z in V.A3. There exists an element 0 in V such that x+0=x for each x in V.A4. For each x in V, there exists an element –x in V such that x+(-x)=0. A5. α(x+y)= αx+αy for each scalar αand any x and y in V.A6. (α+β)x=αx+βx for any scalars αandβand any x in V.A7. (αβ)x=α(βx) for any scalars αandβand any x in V.A8. 1x=x for all x in V.From this definition, we see that the examples in 1.1 are all vector spaces. In the definition, there is an important component, the closure properties of the two operations. These properties are summarized as follows:C1. If x is in V and αis a scalar, then αx is in VC2. If x, y are in V, then x+y is in V.An example that is not a vector space:Let {}=, on this set, the addition and W a a(,1)| is a real numbermultiplication are defined in the usually way. The operation + and scalar multiplication are not defined on W. The sum of two vector is not necessarily in W, neither is the scalar multiplication. Hence, W together with the addition and multiplication is not a vector space.In the examples in 1.1, we see that the following statements are true.Theorem 3.1.1 If V is a vector space and x is any element of V, then (i) 0x=0(ii) x+y=0 implies that y=-x (i.e. the additive inverse is unique). (iii)(-1)x=-x.But is this true for any vector space?Question: Are they obvious? Do we have to prove them?But if we look at the definition of vector space, we don’t know what the elements are, how the addition and multiplication are defined. So theorem above is not very obvious.Proof(i)x=1x=(1+0)x=1x+0x=x+0x, (A6 and A8)Thus –x+x=-x+(x+0x)=(-x+x)+0x (A2)0=0+0x=0x (A1, A3, and A4)(ii)Suppose that x+y=0. then-x=-x+0=-x+(x+y)Therefore, -x=(-x+x)+y=0+y=y(iii)0=0x=(1+(-1))x=1x+(-1)x, thusx+(-1)x=0It follows from part (ii) that (-1)x=-xAssignment for section 1, chapter 3Hand in: 9, 10, 12.§2. SubspacesNew words and phrasesSubspace 子空间Trivial subspace 平凡子空间Proper subspace 真子空间Span 生成Spanning set 生成集Nullspace 零空间2.1 DefinitionGiven a vector space V , it is often possible to form another vector space by taking a subset of V and using the operations of V . For a new subset S of V to be a vector space, the set S must be closed under the operations of addition and scalar multiplication.Examples (on page 124)The set 1212|2x S x x x ⎧⎫⎛⎫⎪⎪==⎨⎬ ⎪⎪⎪⎝⎭⎩⎭together with the usual addition and scalar multiplication is itself a vector space .The set S=| and are real numbers a a a b b ⎧⎫⎛⎫⎪⎪ ⎪⎨⎬ ⎪⎪⎪ ⎪⎝⎭⎩⎭together with the usual addition and scalar multiplication is itself a vector space.★Definition If S is a nonempty subset of a vector space V , and S satisfies the following conditions:(i) αx ∈S whenever x ∈S for any scalar α(ii) x+y ∈S whenever x ∈S and y ∈Sthen S is said to be a subspace (子空间)of V .A subspace S of V together with the operations of addition and scalar multiplication satisfies all the conditions in the definition of a vector space. Hence, every subspace of a vector space is a vector space in its own right. Trivial Subspaces and Proper SubspacesThe set containing only the zero element forms a subspace, called zero subspace, and V is also a subspace of V . Those two subspaces are called trivial subspaces of V . All other subspaces are referred to as proper subspaces.Examples of Subspaces(1) the set of all differentiable functions on [a,b] is a subspace of [,]a b C(2) the set of all polynomials of degree less than n (>1) with the property p(0) form a subspace of n P .(3) the set of matrices of the form a b b c ⎛⎫⎪-⎝⎭ forms a subspace of 22R ⨯. (4) the set of all mxm symmetric matrices forms a subspace of m m R ⨯(5) the set of all mxm skew-symmetric matrices form a subspace of m m R ⨯2.2 The Nullspace of a MatrixLet A be an mxn matrix, and{}()|,0n N A X X R AX =∈=.Then N(A) form a subspace of n R . The subspace N(A) is called the nullspace of A.The proof is a straightforward verification of the definition.2.3 The Span of a Set of VectorsIn this part, we give a method for forming a subspace of V with finite number of vectors in V .Given n vectors 12n v ,v ,,v in a vector space of V , we can form a newsubset of V as the following.{}12n 1122n n Span(v ,v ,,v )v v v |' are scalars i s αααα=+++It is easy to show that this set forms a subset of V. We call this subspace the span of 12n v ,v ,,v , or the subspace of V spanned by12n v ,v ,,v .Theorem 3.2.1 If 12n v ,v ,,v are elements of a vector space of V , then{}12n 1122n n Span(v ,v ,,v )v v v |' are scalars i s αααα=+++ is a subspace of V .For example, the subspace spanned by two vectors 100⎛⎫ ⎪ ⎪ ⎪⎝⎭and010⎛⎫ ⎪ ⎪ ⎪⎝⎭is the subspace consisting of the elements 120x x ⎛⎫ ⎪ ⎪ ⎪⎝⎭.2.4 Spanning Set for a Vector Space★Definition If 12n v ,v ,,v are vectors of V andV=12n Span(v ,v ,,v ), then the set {}12n v ,v ,,v is called a spanning set(生成集)for V .In other words, the set {}12n v ,v ,,v is a spanning set for V if andonly if every element can be written as a linear combination of 12n v ,v ,,v .The spanning sets for a vector space are not unique.Examples (Determining if a set spans for 3R )(a) (){}1231,2,3,T e e e (b) ()()(){}1,1,1,1,1,0,1,0,0T T T (c) ()(){}1,0,1,0,1,0T T (d) ()()(){}1,2,4,2,1,3,4,1,1T T T -To do this, we have to show that every vector in 3R can be written as a linear combination of the given vectors.Assignment for section 2, chapter 3 Hand in: 6, 8, 13, 16, 17, 18, 20Not required: 21Chapter 3---Section 3 Linear Independence§3. Linear IndependenceNew words and phrasesLinear independence 线性无关性Linearly independent 线性无关的Linear dependence 线性相关性Linearly dependent 线性相关的3.1 MotivationIn this section, we look more closely at the structure of vector spaces. We restrict ourselves to vector spaces that can be generated from a finite set of elements, or vector spaces that are spans of finite number of vectors. V=Span(v,v,,v)12nThe set {}v,v,,v is called a generating set or spanning set(生成集).12nIt is desirable to find a minimal spanning set. By minimal, we mean a spanning set with no unnecessary element.To see how to find a minimal spanning set, it is necessary to consider how the vectors in the collection depend on each other. Consequently we introduce the concepts of linear dependence and linear independence. These simple concepts provide the keys to understanding the structure of vector spaces.Give an example in which we can reduce the number of vectors in a spanning set.Consider the following three vectors in 3R.11x 12⎛⎫ ⎪=- ⎪ ⎪⎝⎭ 22x 31-⎛⎫ ⎪= ⎪⎪⎝⎭31x 38-⎛⎫⎪= ⎪ ⎪⎝⎭ These three vectors satisfy(1) 312x =3x +2xAny linear combination of 123x ,x ,x can be reduced to a linear combination of 12x ,x . Thus S= Span(123x ,x ,x )=Span(12x ,x ). (2) 1233x +2x +(1)x 0-= (a dependency relation)Since the three coefficients are nonzero, we could solve for any vector in terms of the other two. It follows thatSpan(123x ,x ,x )=Span(12x ,x )=Span(13x ,x )=Span(23x ,x )On the other hand, no such dependency relationship exists between12x and x . In deed, if there were scalars 1c and 2c , not both 0, such that(3) 1122c x +c x 0=then we could solve for one of the two vectors in terms of the other. However, neither of the two vectors in question is a multiple of the other. Therefore, Span(1x ) and Span(2x ) are both proper subspaces of Span(12x ,x ), and the only way that (3) can hold is if 12c =c =0.Observations: (I)If 12n v ,v ,,v span a vector space V and one of these vectors can be written as a linear combination of the other n-1 vectors, then those n-1 vectors span V .(II) Given n vectors 12n v ,v ,,v , it is possible to write one of thevectors as a linear combination of the other n-1 vectors if and only if there exist scalars 12n c ,c ,,c not all zero such that1122n n v v v 0c c c +++=Proof of I: Suppose that n v can be written as a linear combination of the vectors 12n-1v ,v ,,v .Proof of II: The key point here is that there at least one nonzero coefficient.3.2 Definitions★Definition The vectors 12n v ,v ,,v in a vector space V are said to be linearly independent (线性独立的) if1122n n v v v 0c c c +++=implies that all the scalars 12n c ,c ,,c must equal zero. Example: 12n e ,e ,,e are linearly independent.Definition The vectors 12n v ,v ,,v in a vector space V are said to be linearly dependent (线性相关的)if there exist scalars 12n c ,c ,,c not all zero such that1122n n v v v 0c c c +++=.Let 12n e ,e ,,e ,x be vector in n R . Then 12n e ,e ,,e ,x are linearlydependent.If there are nontrivial choices of scalars for which the linear combination 1122n n v v v c c c +++ equals the zero vector, then 12n v ,v ,,vare linearly dependent. If the only way the linear combination1122n n v v v c c c +++ can equal the zero vector is for all scalars 12n c ,c ,,cto be 0, then 12n v ,v ,,v are linearly independent.3.3 Geometric InterpretationThe linear dependence and independence in 2R and 3R .Each vector in 2R or 3R represents a directed line segment originated at the origin.Two vector are linearly dependent in 2R or 3R if and only if two vectors are collinear. Three or more vector in 2R must be linearly dependent.Three vectors in 3R are linearly dependent if and only if three vectors are coplanar. Four or more vectors in 3R must be linearly dependent.3.4 Theorems and ExamplesIn this part, we learn some theorems that tell whether a set of vectors is linearly independent.Example: (Example 3 on page 138) Which of the following collections of vectors are linearly independent?(a) (){}1231,2,3,Te e e(b) ()()(){}1,1,1,1,1,0,1,0,0TTT(c) ()(){}1,0,1,0,1,0T T(d) ()()(){}1,2,4,2,1,3,4,1,1T TT-The problem of determining the linear dependency of a collection of vectors in m R can be reduced to a problem of solving a linear homogeneous system.If the system has only the trivial solution, then the vectors are linearly independent, otherwise, they are linearly dependent, We summarize the this method in the following theorem:Theorem n vectors 12n x ,x ,,x in m R are linearly dependent if the linear system Xc=0 has a nontrivial solution, where 12n X=(x ,x ,,x ). Proof: 1122n n c x +c x +c x 0+= ⇔ Xc=0.Theorem 3.3.1 Let 12n x ,x ,,x be n vectors in n R and let12n X=(x ,x ,,x ). The vectors 12n x ,x ,,x will be linearly dependent if andonly if X is singular. (the determinant of X is zero)Proof: Xc=0 has a nontrivial solution if and only X is singular.Theorem 3.3.2 Let 12n v ,v ,,v be vectors in a vector space V. A vector v in Span(12n v ,v ,,v ) can be written uniquely as a linear combination of12n v ,v ,,v if and only if 12n v ,v ,,v are linearly independent.(A vector v in Span(12n v ,v ,,v ) can be written as two different linear combinations of 12n v ,v ,,v if and only if 12n v ,v ,,v are linearly dependent.)(Note: If---sufficient condition ; Only if--- necessary condition ) Proof: Let v ∈ Span(12n v ,v ,,v ), then 1122n n v v v v ααα=+++Necessity: (contrapositive law for propositions)Suppose that vector v in Span(12n v ,v ,,v ) can be written as two different linear combination of 12n v ,v ,,v , then prove that 12n v ,v ,,v are linearly dependent. The difference of two different linear combinations gives a dependency relation of 12n v ,v ,,vSuppose that 12n v ,v ,,v are linearly dependent, then there exist twodifferent representations. The sum of the original relation plus the dependency relation gives a new representation.Assignment for section 3, chapter 3Hand in : 5, 11, 13, 14, 15, ; Not required: 6, 7, 8, 9, 10,§4. Basis and DimensionNew words and phrasesBasis 基Dimension 维数Minimal spanning set 最小生成集Standard Basis 标准基4.1 Definitions and TheoremsA minimal spanning set for a vector space V is a spanning set with no unnecessary elements (i.e., all the elements in the set are needed in order to span the vector space). If a spanning set is minimal, then its elements are linearly independent. This is because if they were linearly dependent, then we could eliminate a vector from the spanning set, the remaining elements still span the vector space, this would contradicts the assumption of minimality. The minimal spanning set forms the basic building blocks for the whole vector space and, consequently, we say that they form a basis for the vector space(向量空间的基).★Definition The vectorsv,v,,v form a basis for a vector space V12nif and only if(i)v,v,,v are linearly independent12n(ii)v,v,,v span V.12nA basis of V actually is a minimal spanning set(最小张成集)for V.We know that spanning sets for a vector space are not unique. Minimal spanning sets for a vector space are also not unique. Even though, minimal spanning sets have something in common. That is, the number of elements in minimal spanning sets.We will see that all minimal spanning sets for a vector space have the same number of elements.Theorem 3.4.1 If {}12n v ,v ,,v is a spanning set for a vector space V , then any collection of m vectors in V , where m>n, is linearly dependent.Proof Let {}12m u ,u ,,u be a collection of m vectors in V . Then each u i can be written as a linear combination of 12n v ,v ,,v .i 1122n u =v +v ++v i i in a a aA linear combination 1122m u + u u m c c c ++can be written in the formnnn11j 22j j j=1j=1j=1v + v v j j m nj c a c a c a ++∑∑∑Rearranging the terms, we see that 1122m j 11u + u u ()v nmm ij i j i c c c a c ==++=∑∑Then we consider the equation 1122m m c u + c u c u 0++= to see if we canfind a nontrivial solution (12n c ,c ,,c ). The left-hand side of the equation can be written as a linear combination of 12n v ,v ,,v . We show that thereare scalars 12n c ,c ,,c , not all zero, such that 1122m m c u + c u c u 0++=.Here, we have to use a theorem: A homogeneous linear system must have a nontrivial solution if it has more unknowns than equations. Corollary 3.4.2 If {}12n v ,v ,,v and {}12m u ,u ,,u are both bases for a vector space V , then n=m. (all the bases must have the same number of vectors.)Proof Since 12n v ,v ,,v span V , if m>n, then {}12m u ,u ,,u must be linearly dependent. This contradicts the hypothesis that {}12m u ,u ,,u is linearly independent. Hence m n ≤. By the same reasoning, n m ≤. So m=n.From the corollary above, all the bases for a vector space have the same number of elements (if it is finite). This number is called the dimension of the vector space.★Definition Let V be a vector space. If V has a basis consisting of n vectors, we say that V has dimension n (the dimension of a vector space of V is the number of elements in a basis.) The subspace {0} of V is said to have dimension 0. V is said to be finite-dimensional if there is a finite set of vectors that spans V; otherwise, we say that V is infinite-dimensional.Recall that a set of n vector is a basis for a vector space if two conditions are satisfied. If we know that the dimension of the vector space is n, then we just need to verify one condition.Theorem 3.4.3 If V is a vector space of dimension n>0:I.Any set of n linearly independent vectors spans V (so this setforms a basis for the vector space).II.Any n vectors that span V are linearly independent (so this set forms a basis for the vector space).ProofProof of I: Suppose thatv,v,,v are linearly independent and v is12nany vector in V. Since V has dimension n, the collection of vectorsv,v,,v,v must be linearly dependent. Then we show that v can be 12nexpressed in terms ofv,v,,v.12nProof of II: Ifv,v,,v are linearly dependent, then one of v’s can12nbe written as a linear combination of the other n-1 vectors. It follows that those n-1 vectors still span V. Thus, we will obtain a spanning set with k<n vectors. This contradicts dimV=n (having a basis consisting of n vectors).Theorem 3.4.4 If V is a vector space of dimension n>0:(i) No set of less than n vectors can span V .(ii)Any subset of less than n linearly independent vectors can be extended to form a basis for V .(iii) Any spanning set containing more than n vectors can be pareddown (to reduce or remove by or as by cutting) to form a basis for V . Proof(i): If there are m (<n) vectors that can span V , then we can argue that dimV<n. this contradicts the assumption.(ii) We assume that 12k v ,v ,,v are linearly independent ( k<n). Then Span(12k v ,v ,,v ) is a proper subspace of V . There exists a vector1v k + that is in V but not in Span(12k v ,v ,,v ). We can show that12k v ,v ,,v ,1v k + must be linearly independent. Continue this extensionprocess until n linearly independent vectors are obtained.(iii) The set must be linearly independent. Remove (eliminate) one vector from the set, the remaining vectors still span V . If m-1>n, we can continue to eliminate vectors in this manner until we arrive at a spanning set containing n vectors.4.2 Standard BasesThe standard bases(标准基)for n R, m nR .Although the standard bases appear to be the simplest and most natural to use, they are not the most appropriate bases for many applied problems. Once the application is solved in terms of the new basis, it is a simple matter to switch back and represent the solution in terms of the standard basis.Assignment for section 4, chapter 3Hand in : 4, 7, 9,10,12,16,17,18Not required: 11,13,14, 15,§5. Change of BasisNew words and phrasesTransition matrix 过渡矩阵5.1 MotivationMany applied problems can be simplified by changing from one coordinate system to another. Changing coordinate systems in a vector space is essentially the same as changing from one basis to another. For example, in describing the motion of a particle in the plane at a particular time, it is often convenient to use a basis for 2R consisting of a unit tangent vector t and a unit normal vector n instead of the standard basis. In this section we discuss the problem of switching from one coordinate system to another. We will show that this can be accomplished by multiplying a given coordinate vector x by a nonsingular matrix S.5.2 Changing Coordinates in 2RThe standard basis for 2R is 12{e ,e }. Any vector in 2R can be written as a linear combination 12{e ,e }1122x=e +e x x .The scalars 12 and x x can be thought of as the coordinates (坐标) of x with respect to the standard basis. Actually, for any basis 12{u ,u } for 2R , a given vector x can be represented uniquely as a linear combination1122x=u +u c cThe scalars 12 and c c are the coordinates of x with respect to the basis12{u ,u }. Let us denote the ordered bases by [12e ,e ] and [12u ,u ]. 12(,)T x x iscalled the coordinate vector of x with respect to [12e ,e ],12(,)T c c the coordinate vector of x with respect to [12u ,u ].We wish to find the relationship between the coordinate vectors x and c.11122122x=e +e (e ,e )x x x x ⎛⎫= ⎪⎝⎭11122122x=u +u (u ,u )c c c c ⎛⎫= ⎪⎝⎭11121222(e ,e )(u ,u )x y x y ⎛⎫⎛⎫= ⎪ ⎪⎝⎭⎝⎭111222(u ,u )x c x c ⎛⎫⎛⎫= ⎪ ⎪⎝⎭⎝⎭Or simply, x=UcThe matrix U is called the transition matrix (过渡矩阵)from the ordered basis [12u ,u ] to [12e ,e ].The matrix U is nonsingular since 12u ,u are linearly independent. By the formula x=Uc, we see that if given a vector 1122u +u c c , its coordinate vector with respect to [12e ,e ] is given by Uc.Conversely if given a vector 12(,)T x x , then its coordinate vector with respect to [12u ,u ] is given by -1U xNow let us consider the general problem of changing from one basis[12v ,v ] to another basis [12u ,u ]. In this case, we assume that 112212x v +v (v ,v )c c c == and 112212x u +u (u ,u )d d d == ThenVc=UdIt follows that1d U Vc -=.Thus, given a vector x in 2R and its coordinate vector c with respect to the ordered basis [12v ,v ], to find the coordinate vector of x with respect to the new basis [12u ,u ], we simply multiply c by the transition matrix1S U V -=.where 12V=(v ,v ) and 12U=(u ,u ) Example (example 4 on page 156) Given two bases15v 2⎛⎫= ⎪⎝⎭, 27v 3⎛⎫= ⎪⎝⎭and 13u 2⎛⎫= ⎪⎝⎭, 21u 1⎛⎫= ⎪⎝⎭(1) Find the coordinate vectors c and d of the vector ()x=12,5Twith respect to the bases [12v ,v ] and [12u ,u ], respectively.12[e ,e ]12[v ,v ]12[u ,u ]1U -UV1U V -(2) And find the transition matrix S corresponding to the change of basis from [12v ,v ] to [12u ,u ]. (3) Check that d=Sc.Solution: The coordinate vector with respect to the basis [12v ,v ] is15712371212352551--⎛⎫⎛⎫⎛⎫⎛⎫⎛⎫== ⎪ ⎪ ⎪⎪ ⎪-⎝⎭⎝⎭⎝⎭⎝⎭⎝⎭The coordinate vector with respect to the basis [12u ,u ] is13112111272152359--⎛⎫⎛⎫⎛⎫⎛⎫⎛⎫== ⎪ ⎪ ⎪⎪ ⎪--⎝⎭⎝⎭⎝⎭⎝⎭⎝⎭The transition matrix corresponding to the change of the basis from [12v ,v ] to [12u ,u ] isS=131571157342123232345--⎛⎫⎛⎫⎛⎫⎛⎫⎛⎫== ⎪ ⎪ ⎪⎪ ⎪---⎝⎭⎝⎭⎝⎭⎝⎭⎝⎭Check that73419451⎛⎫⎛⎫⎛⎫= ⎪ ⎪⎪---⎝⎭⎝⎭⎝⎭.The discussion of the coordinate changes in 2R can be easily generalized to that in n R . We summarize it as follows.12n [v ,v ,,v ]1U -UV1U V -12n [e ,e ,,e ]12n [u ,u ,,u ]where 12V=(v ,v ,,v )n and 12U=(u ,u ,,u )nInterpretation: if x=12(,,,)T n x x x is a vector in n R , then the coordinate vector c of x with respect to 12[v ,v ,,v ]n is given by x=Vc, (c=-1V x ), the coordinate vector d of x with respect to 12[u ,u ,,u ]n is given by x=Ud, (d=-1U x ). The transition matrix from 12[v ,v ,,v ]n to 12[u ,u ,,u ]n is given by S=1U V -.5.3 Change of Basis for a General Vector Space★Definition (coordinate) Let V be a vector space and let E=[12n v ,v ,,v ] be an ordered basis for V . If v is any element of V , then v can be written in the form121122n n 12n n v v v v [v ,v ,,v ]c c c c c c ⎛⎫ ⎪ ⎪=+++= ⎪ ⎪⎝⎭(this is a formal multiplication since vectors here are not necessarily column vectors in n R ) where 12n c ,c ,,c are scalars. Thus we can associate with each vector v a unique vector c=12n (c ,c ,,c )T in n R . The vector c defined in this way is called theE [v]. The i c ’s are called coordinates of v relative to E . Transition MatrixLet E=[12n w ,w ,,w ], F=[12n v ,v ,,v ] be two ordered bases for V .Then11112121212122221122w v v v w v v v w v v v n n n n n n n nn ns s s s s s s s s =+++=+++=+++Formally, this change of bases can be written as111212122212n 12n 12[w ,w ,,w ][v ,v ,,v ]n n n n nn s s s s s s s s s ⎛⎫ ⎪ ⎪= ⎪ ⎪⎝⎭(The multiplication is formal matrix multiplication. If the vector space is the Euclidean space, then the multiplication becomes the actual multiplication.)This is called the change of basis from E=[12n w ,w ,,w ] to F =[12n v ,v ,,v ].A vector v has different coordinate vectors in different bases. Let x=E [v], i.e. 1122n v w +w ++w n x x x = and y=F [v], 1122n v v +v ++v n y y y =, then 1122n 111v ()v +()v ++()v nnnj j j j nj j j j j s x s x s x ====∑∑∑1ni ij j j y s x ==∑In matrix notation, we have y=Sx, whereS=111212122212n n n n nn s s s ss s s s s ⎛⎫ ⎪ ⎪⎪ ⎪⎝⎭This matrix is referred to as the transition matrix corresponding to the change of basis from E=[12n w ,w ,,w ] to F =[12n v ,v ,,v ] S is nonsingular, since Sx=y if and only if1122n n 1122n n w +w ++w v +v ++v x x x y y y =Sx=0 implies that 1122n n w +w ++w 0x x x =. Hence x must be zero. 1S y x -=1S - is the transition matrix corresponding to the change of base from F=[12n v ,v ,,v ] to E=[12n w ,w ,,w ]Any nonsingular matrix can be thought of as a transition matrix. If S is an nxn nonsingular matrix and [12n v ,v ,,v ] is an ordered basis for V , then define [12n w ,w ,,w ] by111212122212n 12n 12[w ,w ,,w ][v ,v ,,v ]n n n n nn s s s s s s s s s ⎛⎫ ⎪ ⎪= ⎪ ⎪⎝⎭Then12nw ,w ,,w arelinearly independent. Suppose that1122n n w +w ++w 0x x x =Then1122n 111()v +()v ++()v 0nnnj j j j nj j j j j s x s x s x ====∑∑∑By the linear independence of 12n v ,v ,,v , it follows that10nij jj s x==∑or , equivalentlySx=0Since S is nonsingular, x must equal zero. Therefore, 12n w ,w ,,w are linearly independent and hence they form a basis for V . The matrix S is the transition matrix corresponding to the change from the ordered basis [12n w ,w ,,w ] to [12n v ,v ,,v ]. Example Let 110u 01⎛⎫= ⎪⎝⎭ 210u 01⎛⎫= ⎪-⎝⎭ 301u 10⎛⎫= ⎪⎝⎭401u 10⎛⎫= ⎪-⎝⎭; 110v 00⎛⎫=⎪⎝⎭ 201v 00⎛⎫= ⎪⎝⎭ 301v 10⎛⎫= ⎪⎝⎭ 410v 01⎛⎫= ⎪-⎝⎭.Find the transition matrix corresponding to the change of base from E=[1234u ,u ,u ,u ] to F =[1234v ,v ,v ,v ]In many applied problems it is important to use the right type of basis for the particular application. In chapter 5 we will see that the key to solving least squared problems is to switch to a special type of basis called an orthonormal basis. In chapter 6 we will consider a number of applications involving the eigenvalues and eigenvectors associated with an nxn matrix A. The key to solving these types of problems is to switch to a basis for n R consisting of eigenvectors of A.Chapter 3---Section 5 Change of Basis Assignment for section 5, chapter 3 Hand in: 6, 7, 8, 11 ,Not required; 9, 10,§6. Row Space and Column SpaceNew words and phrasesRow space 行空间Column space 列空间Rank 秩6.1 DefinitionsWith an mxn matrix A, we can associate two subspaces.Definition If A is an mxn matrix, the subspace of 1n R ⨯ spanned by the row vectors of A is called the row space of A, the subspace of m R spanned by the column vectors of A is called the column space of A.Theorem 3.6.1 Two row equivalent matrices have the same row space. Proof 21kE E E A B =The row vectors of B must be a linear combination of the row vectors of A. Consequently, the row space of B must be a subspace of the row space of A. By the same reasoning, the row space of A is a subspace of the row space of B. So, they are the same.★Definition The rank (秩)of a matrix of A is the dimension of the row space of A.。
高等数学教材英文版

高等数学教材英文版Higher Mathematics Textbook (English Edition)Introduction:In the realm of mathematics, higher mathematics plays a pivotal role in shaping our understanding of complex mathematical concepts and their applications. As an essential component of advanced education, the Higher Mathematics Textbook in its English edition provides a comprehensive resource for students seeking to master this subject. This article highlights the importance and key features of the Higher Mathematics Textbook in its English edition.Chapter 1: Functions and GraphsThis chapter delves into the fundamental concept of functions and their graphical representation. It explores various types of functions, such as linear, quadratic, exponential, and logarithmic functions, along with their properties and behaviors. The chapter also introduces the notion of limits and continuity, laying the groundwork for future mathematical analysis.Chapter 2: Differentiation and IntegrationBuilding upon the foundation of functions, Chapter 2 delves into the concepts of differentiation and integration. It covers the principles of finding derivatives and calculating integrals, as well as their respective applications in various fields, including physics, economics, and engineering. Through carefully curated examples and exercises, students gain a solid understanding of these essential calculus techniques.Chapter 3: Sequences and SeriesThis chapter emphasizes the study of sequences and series, encompassing arithmetic, geometric, and power series. It examines convergence and divergence criteria and explores the concepts of convergence tests, including the ratio test, root test, and integral test. By mastering these concepts, students develop a deep comprehension of the behavior of sequences and series.Chapter 4: Differential EquationsDifferential equations play an integral role in modeling various natural phenomena and engineering systems. This chapter introduces students to ordinary differential equations and their applications. It covers topics such as first-order equations, linear differential equations, and higher-order differential equations. By comprehending the principles governing differential equations, students become equipped to tackle real-world problem-solving scenarios.Chapter 5: Multivariable CalculusMultivariable calculus extends the principles of differentiation and integration to functions of multiple variables. This chapter explores partial derivatives, multiple integrals, and vector calculus. By understanding the intricacies of multivariable calculus, students gain the ability to tackle complex mathematical problems involving multiple variables.Chapter 6: Linear AlgebraLinear algebra provides a powerful framework for solving systems of linear equations and studying vector spaces. This chapter introduces studentsto matrices, determinants, vector spaces, and linear transformations. It explores topics such as eigenvalues and eigenvectors, diagonalization, and the applications of linear algebra in diverse fields, including computer graphics and network analysis.Conclusion:The Higher Mathematics Textbook in its English edition serves as an indispensable resource for students pursuing a deeper understanding of higher mathematics. By covering a wide range of topics and providing clear explanations, examples, and exercises, this textbook equips students with the necessary skills to tackle complex mathematical problems. With its well-organized content and comprehensive approach, the Higher Mathematics Textbook facilitates effective learning and fosters a solid foundation in advanced mathematics.。
线性代数英语短句带翻译

Translation: 两个垂直的向量的点积为零。
The cross product of two vectors is a vector that is perpendicular to both vectors and has a magnitude equal to the product of their magnitudes and the sine of the angle between them.
Translation: 一个向量是具有大小和方向的量。在数学中,向量通常用在字母上方的箭头表示,如 → 。
Example Sentence: The displacement of an object can be represented by a vector.
Translation: 物体的位移可以用一个向量来表示。
Translation: 一个矩阵是以行和列排列的数字或符号的矩形阵列。矩阵通常用大写字母来表示,如 。
Example Sentence: Matrix multiplication is not commutative.
Translation: 矩阵乘法不满足交换律。
5. Eigenvalues and Eigenvectors
2. Vector Addition and Subtraction
To add or subtract two vectors, we can combine their corresponding components.
Translation: 要对两个向量进行加法或减法运算,我们可以对它们的对应分量进行组合。
向量的单词
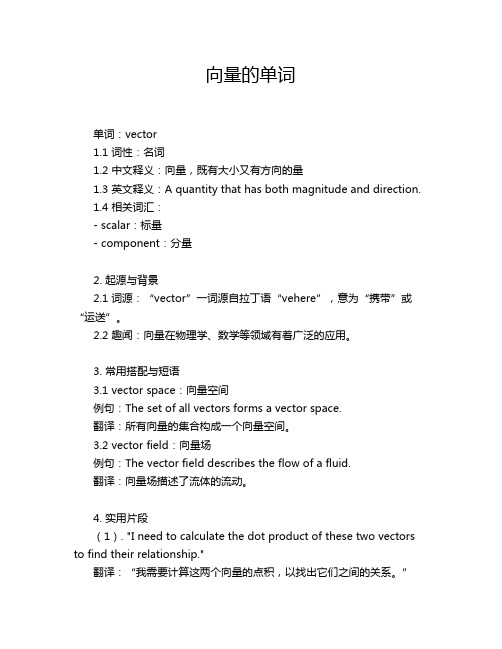
向量的单词单词:vector1.1 词性:名词1.2 中文释义:向量,既有大小又有方向的量1.3 英文释义:A quantity that has both magnitude and direction.1.4 相关词汇:- scalar:标量- component:分量2. 起源与背景2.1 词源:“vector”一词源自拉丁语“vehere”,意为“携带”或“运送”。
2.2 趣闻:向量在物理学、数学等领域有着广泛的应用。
3. 常用搭配与短语3.1 vector space:向量空间例句:The set of all vectors forms a vector space.翻译:所有向量的集合构成一个向量空间。
3.2 vector field:向量场例句:The vector field describes the flow of a fluid.翻译:向量场描述了流体的流动。
4. 实用片段(1). "I need to calculate the dot product of these two vectors to find their relationship."翻译:“我需要计算这两个向量的点积,以找出它们之间的关系。
”(2). "The vector represents the force acting on the object."翻译:“这个向量代表作用在物体上的力。
”(3). "She is studying the properties of vector spaces in her math class."翻译:“她在数学课上研究向量空间的性质。
”(4). "The engineer is analyzing the vector field to understand the behavior of the system."翻译:“工程师正在分析向量场,以了解系统的行为。
THEDIMENSIONOFAVECTORSPACE一个向量空间的维数
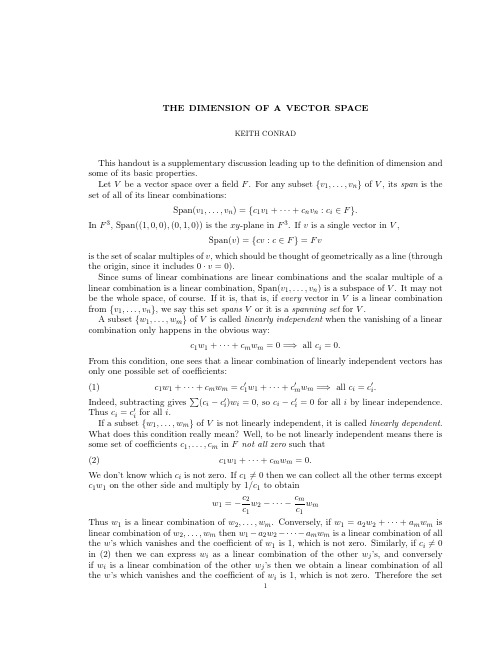
THE DIMENSION OF A VECTOR SPACEKEITH CONRADThis handout is a supplementary discussion leading up to the definition of dimension and some of its basic properties.Let V be a vector space over a field F .For any subset {v 1,...,v n }of V ,its span is the set of all of its linear combinations:Span(v 1,...,v n )={c 1v 1+···+c n v n :c i ∈F }.In F 3,Span((1,0,0),(0,1,0))is the xy -plane in F 3.If v is a single vector in V ,Span(v )={cv :c ∈F }=F vis the set of scalar multiples of v ,which should be thought of geometrically as a line (through the origin,since it includes 0·v =0).Since sums of linear combinations are linear combinations and the scalar multiple of a linear combination is a linear combination,Span(v 1,...,v n )is a subspace of V .It may not be the whole space,of course.If it is,that is,if every vector in V is a linear combination from {v 1,...,v n },we say this set spans V or it is a spanning set for V .A subset {w 1,...,w m }of V is called linearly independent when the vanishing of a linear combination only happens in the obvious way:c 1w 1+···+c m w m =0=⇒all c i =0.From this condition,one sees that a linear combination of linearly independent vectors has only one possible set of coefficients:(1)c 1w 1+···+c m w m =c 1w 1+···+c m w m =⇒all c i =c i .Indeed,subtracting gives (c i −c i )w i =0,so c i −c i =0for all i by linear independence.Thus c i =c i for all i .If a subset {w 1,...,w m }of V is not linearly independent,it is called linearly dependent .What does this condition really mean?Well,to be not linearly independent means there is some set of coefficients c 1,...,c m in F not all zero such that (2)c 1w 1+···+c m w m =0.We don’t know which c i is not zero.If c 1=0then we can collect all the other terms except c 1w 1on the other side and multiply by 1/c 1to obtainw 1=−c 2c 1w 2−···−c m c 1w m Thus w 1is a linear combination of w 2,...,w m .Conversely,if w 1=a 2w 2+···+a m w m is linear combination of w 2,...,w m then w 1−a 2w 2−···−a m w m is a linear combination of all the w ’s which vanishes and the coefficient of w 1is 1,which is not zero.Similarly,if c i =0in (2)then we can express w i as a linear combination of the other w j ’s,and conversely if w i is a linear combination of the other w j ’s then we obtain a linear combination of all the w ’s which vanishes and the coefficient of w i is 1,which is not zero.Therefore the set12KEITH CONRAD{w1,...,w m}is linearly dependent precisely when at least one of these vectors(we are not saying which,and it could be true for more than one)is a linear combination of the rest.Spanning sets for V and linearly independent subsets of V are in some sense opposite concepts.Any subset of a linearly independent subset is still linearly independent,but this need not be true of spanning sets.Any superset of a spanning set for V is still a spanning set for V,but this need not be true of linearly independent subsets.A subset of V which has both of the above properties is called a basis.That is,a basis of V is a linearly independent subset of V which also spans V.For most vector spaces, there is no God-given basis,so what matters conceptually is the size of a basis rather than a particular choice of basis(compare with the notion of a cyclic group and a choice of generator for it).The following theorem is afirst result which links spanning sets in V with linearly inde-pendent subsets.Theorem1.Suppose V={0}and it admits afinite spanning set v1,...,v n.Some subset of this spanning set is a linearly independent spanning set.The theorem says that once there is afinite spanning set,which could have lots of linear dependence relations,there is a basis for the space.Moreover,the theorem tells us a basis can be found within any spanning set at all.Proof.While{v1,...,v n}may not be linearly independent,it contains linearly independent subsets,such as any one single nonzero v i.Of course,such small linearly independent subsets can hardly be expected to span V.But consider linearly independent subsets of{v1,...,v n} which are as large as possible.Reindexing,without loss of generality,we can write such a subset as{v1,...,v k}.For i=k+1,...,n,the set{v1,...,v k,v i}is not linearly independent(otherwise {v1,...,v k}is not a maximal linearly independent subset).Thus there is some linear relationc1v1+···+c k v k+c i v i=0,where the c’s are in F are not all of them are0.The coefficient c i cannot be zero,since otherwise we would be left with a linear dependence relation on v1,...,v k,which does not happen due to their linear independence.Since c i=0,we see that v i is in the span of v1,...,v k.This holds for i=k+1,...,n,so any linear combination of v1,...,v n is also a linear combination of just v1,...,v k.As every element of V is a linear combination of v1,...,v n,we conclude that v1,...,v k spans V.By its construction,this is a linearly independent subset of V as well.Notice the non-constructive character of the proof.If we somehow can check that a (finite)subset of V spans the whole space,Theorem1says a subset of this is a linearly independent spanning set,but the proof is not constructively telling us which subset of {v1,...,v n}this might be.Theorem1is a“top-down”theorem.It says any(finite)spanning set has a linearly independent spanning set inside of it.It is natural to ask if we can go“bottom-up,”and show any linearly independent subset can be enlarged to a linearly independent spanning set.Something along these lines will be proved in Theorem3.Lemma1.Suppose{v1,...,v n}spans V,where n≥2.Pick any v∈V.If some v i is a linear combination of the other v j’s and v,then V is spanned by the other v j’s and v.THE DIMENSION OF A VECTOR SPACE3 For example,if V is spanned by v1,v2,and v3,and v1is a linear combination of v,v2, and v3,where v is another vector in V,then V is spanned by v,v2,and v3.Lemma1should be geometrically reasonable.See if you can prove it before reading the proof below.Proof.Reindexing if necessary,we can suppose it is v1which is a linear combination of v,v2,...,v n.We will show every vector in V is a linear combination of v,v2,...,v n,so these vectors span V.Pick any w∈V.By hypothesis,w=c1v1+c2v2+···+c n v nfor some c i∈F.Since v1is a linear combination of v,v2,...,v n,we feed this linear combination into the above equation to see w is a linear combination of v,v2,...,v n.As w was arbitrary in V,we have shown V is spanned by v,v2,...,v m.The following important technical result relates spanning sets for V and linearly inde-pendent subsets of V.It is called the exchange theorem.The name arises from the process in its proof,which relies on repeated applications of Lemma1.Theorem2(Exchange Theorem).Suppose V is spanned by n vectors,where n≥1.Any linearly independent subset of V has at most n vectors.If you think about linear independence as“degrees of freedom,”the exchange theorem makes sense.What makes the theorem somewhat subtle to prove is that the theorem bounds the size of any linearly independent subset once we know the size of one spanning set.Most linearly independent subsets of V are not directly related to the original choice of spanning set,so linking the two sets of vectors is tricky.The proof will show how to link linearly independent sets and spanning sets by an exchange process,one vector at a time. Proof.First,let’s check the result when n=1.In this case,V=F v for some v(that is,V is spanned by one vector).Two different scalar multiples of v are linearly dependent,so a linearly independent subset of V can have size at most1.Now we take n≥2.We give a proof by contradiction.If the theorem is false,then V contains a set of n+1linearly independent vectors,say w1,...,w n+1.Step1:We are told that V can be spanned by n vectors.Let’s call such a spanning set v1,...,v n.We also have the n+1linearly independent vectors w1,...,w n+1in V.Write thefirst vector from our linearly independent set in terms of our spanning set:w1=c1v1+c2v2+···+c n v nfor some c i∈F.Since w1=0(a linearly independent set never contains the vector0), some coefficient c j is nonzero.Without loss of generality,we can reindex the v’s so that c1is nonzero.Then the above equation can be solved for v1as a linear combination of w1,v2,...,v n.By Lemma1,(3)V=Span(w1,v2,...,v n).Notice that we have taken one element from the initial spanning set out and inserted an element from the linearly independent set in its place,retaining the spanning property.Step2:Let’s repeat the procedure,this time using our new spanning set(3).Write w2 in terms of this new spanning set:(4)w2=c 1w1+c 2v2+···+c n v n4KEITH CONRADfor some c i in F.We want to use this equation to show w2can be inserted into(3)and one of the original vectors can be taken out,without destroying the spanning property.Some care is needed,because we want to keep w1in the spanning set rather than accidentally swap it out.(This is an issue that we did not meet in thefirst step,where no new vectors had yet been placed in the spanning set.)Certainly one of c 1,c 2,...,c n is nonzero,since w2is nonzero.But in fact we can say something a bit sharper:regardless of the value of c 1,one of c 2,...,c n is nonzero.Indeed, if c 2,...,c m are all zero,then w2=c 1w1is a scalar multiple of w1,and that violates linear independence(as{w1,...,w m}is linearly independent,so is the subset{w1,w2}).Without loss of generality,we can reindex v2,...,v n so it is c 2which is nonzero.Then we can use(4)to express v2as a linear combination of w1,w2,v3,...,v n.By another application of Lemma1,using our new spanning set in(3)and the auxiliary vector w2,it follows thatV=Span(w1,w2,v3,...,v n).Step3:Now that we see how things work,we argue inductively.Suppose for some k between1and n−1that we have shownV=Span(w1,...,w k,v k+1,...,v n).(This has already been checked for k=1in Step1,and k=2in Step2,although Step2is not logically necessary for what we do;it was just included to see concretely the inductive step we now carry out for any k.)Using this spanning set for V,write(5)w k+1=a1w1+···+a k w k+a k+1v k+1+···+a n v nwith a i∈F.One of a k+1,...,a n is nonzero,since otherwise this equation expresses w k+1 as a linear combination of w1,...,w k,and that violates linear independence of the w’s.Reindexing v k+1,...,v n if necessary,we can suppose it is a k+1which is nonzero.Then (5)can be solved for v k+1as a linear combination of w1,...,w k,w k+1,v k+2,...,v n.By Lemma1,using the spanning set{w1,...,w k,v k+1,...,v n}and the auxiliary vector w k+1, we can swap w k+1into the spanning set in exchange for v k+1without losing the spanning property:V=Span(w1,...,w k,w k+1,v k+2,...,v n).We have added an new vector to the spanning set and taken one of the original vectors out. Now by induction(or,more loosely,“repeating this step n−k−1more times”),we arrive at the conclusion that(6)V=Span(w1,...,w n).However,we were starting with n+1linearly independent vectors w1,...,w n+1,so w n+1is not in the span of w1,...,w n.That contradicts the meaning of(6),which says every vector in V is a linear combination of w1,...,w n.We have reached a contradiction,so no linearly independent subset of V contains more than n vectors,where n is the size of a spanning set for V. Example1.Consider M3(R),the3×3real matrices.It is a vector space over R under matrix addition and the usual multiplication of a matrix by a real number.This vector space has a9-element spanning set,namely the9matrices with a1in one component and 0elsewhere.Therefore any linearly independent subset of M3(R)has at most9elements in it.THE DIMENSION OF A VECTOR SPACE5 Corollary1.Suppose V={0}and V admits afinite basis.Any two bases for V have the same size.Proof.Let{v1,...,v n}and{v 1,...,v m}be bases for V.Treating thefirst set as a spanning set for V and the second set as a linearly independent subset of V,the exchange theorem tells us that m≤n.Reversing these roles(which we can do since bases are both linearly independent and span the whole space),we get n≤m.Thus m=n.The(common)size of any basis of V is called the dimension of V(over F).Example2.From the known bases of R n and M n(R),these spaces have dimension n and n2over R,respectively.Example3.The dimension of C as a real vector space is2,with one basis being{1,i}.Example4.The vector space{0}has no basis,or you might want to say its basis is the empty set.In any event,it is natural to declare the zero vector space to have dimension0.Theorem3.Let V be a vector space with dimension n≥1.Any spanning set has at least n elements,and contains a basis inside of it.Any linearly independent subset has at most n elements,and can be extended to a basis of V.Finally,an n-element subset of V is a spanning set if and only if it is a linearly independent set.Proof.Since V has a basis of n vectors,let’s pick such a basis,say v1,...,v n.We will compare this basis to the spanning sets and the linearly independent sets in V to draw our conclusions,taking advantage of the dual nature of a basis as both a linearly independent subset of V and as a spanning set for V.If{u1,...,u k}is a spanning set for V,then a comparison with{v1,...,v n}(interpreted as a linearly independent subset of V)shows n≤k by the exchange theorem.Equivalently, k≥n.Moreover,Theorem1says that{u1,...,u k}contains a basis for V.This settles the first part of the theorem.For the next part,suppose{w1,...,w m}is a linearly independent subset of V.A compar-ison with{v1,...,v n}(interpreted as a spanning set for V)shows m≤n by the exchange theorem.To see that the w’s can be extended to a basis of V,apply the exchange process from the proof of the exchange theorem,but only m times since we have only m linearly independent w’s.Wefind at the end thatV=Span(w1,...,w m,v m+1,...,v n),which shows the w’s can be extended to a spanning set for V.This spanning set contains a basis for V,by Theorem1.Since all bases of V have n elements,this n-element spanning set must be a basis itself.Taking m=n in the previous paragraph shows any n-element linearly independent subset is a basis(and thus spans V).Conversely,any n-element spanning set is linearly independent,since any linear dependence relation would let us cut down to a spanning set of fewer than n elements,but that violates thefirst result in this proof:a spanning set for an n-dimensional vector space has at least n elements. Theorem4.If V is an n-dimensional vector space,any subspace W isfinite-dimensional, with dimension at most n.Proof.This theorem is trivial for the zero vector space,so we may assume V={0},i.e., n≥1.6KEITH CONRADAny linearly independent subset of W is also a linearly independent subset of V,and thus has size at most n by Theorem3.Choose a linearly independent subset{w1,...,w m} of W where m is maximal.Then m≤n.We will show Span(w1,...,w m)=W.For any w∈W,the set{w,w1,...,w m}has more than m elements,so it can’t be linearly independent.Therefore there is some vanishing linear combinationaw+a1w1+···+a m w m=0where a,a1,...,a m are in F and are not all0.If a=0then the a i’s all vanish since w1,...,w m are linearly independent.Therefore a=0,so we can solve for w:w=−a1aw1−···−a maw m.Thus w is a linear combination of w1,...,w m.Since w was arbitrary in W,this shows the w i’s span W.So{w1,...,w m}is a spanning set for W which is linearly independent by construction.This proves W isfinite-dimensional with dimension m≤n.Theorem5.If V has dimension n and W is a subspace with dimension n,then W=V.Proof.When W has dimension n,any basis for W is a linearly independent subset of V with n elements,so it spans V by Theorem3.The span is also W(by definition of a basis for W),so W=V.It is really important that throughout our calculations(expressing one vector as a linear combination of others when we have a nontrivial linear combination of vectors equal to0) we can scale a nonzero coefficient of a vector to make the coefficient equal to1.For example, suppose we tried to do linear algebra over the integers Z instead of over afield.Then we can’t scale a coefficient in Z to be1without possibly needing rational coefficients for other vectors in a linear combination.That suggests results like the ones we have established for vector spaces overfields might not hold for“vector spaces over Z.”And it’s true: linear algebra over Z is more subtle than overfields.For example,Theorem5is false if we work with“vector spaces”over Z.Consider the integers Z and the even integers2Z. By any reasonable definitions,both Z and2Z should be considered“one-dimensional”over the integers,where Z has basis{1}and2Z has basis{2}(since every integer is a unique integral multiple of2).But2Z⊂Z,so a“one-dimensional vector space over Z”can lie inside another without them being equal.This is a pretty clear failure of Theorem5when we use scalars from Z instead of from afield.。
高级工程数学(1)
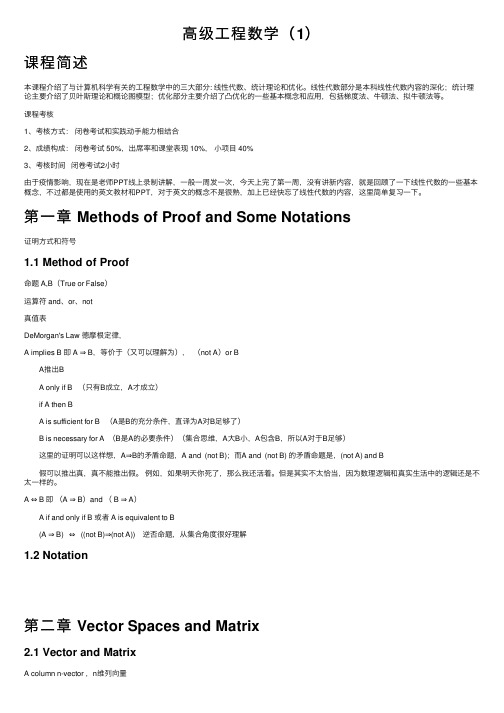
⾼级⼯程数学(1)课程简述本课程介绍了与计算机科学有关的⼯程数学中的三⼤部分: 线性代数、统计理论和优化。
线性代数部分是本科线性代数内容的深化;统计理论主要介绍了贝叶斯理论和概论图模型;优化部分主要介绍了凸优化的⼀些基本概念和应⽤,包括梯度法、⽜顿法、拟⽜顿法等。
课程考核1、考核⽅式:闭卷考试和实践动⼿能⼒相结合2、成绩构成:闭卷考试 50%,出席率和课堂表现 10%,⼩项⽬ 40%3、考核时间闭卷考试2⼩时由于疫情影响,现在是⽼师PPT线上录制讲解,⼀般⼀周发⼀次,今天上完了第⼀周,没有讲新内容,就是回顾了⼀下线性代数的⼀些基本概念,不过都是使⽤的英⽂教材和PPT,对于英⽂的概念不是很熟,加上已经快忘了线性代数的内容,这⾥简单复习⼀下。
第⼀章 Methods of Proof and Some Notations证明⽅式和符号1.1 Method of Proof命题 A,B(True or False)运算符 and、or、not真值表DeMorgan's Law 德摩根定律,A impliesB 即 A ⇒ B,等价于(⼜可以理解为),(not A)or B A推出BA only ifB (只有B成⽴,A才成⽴) if A then BA is sufficient forB (A是B的充分条件,直译为A对B⾜够了) B is necessary for A (B是A的必要条件)(集合思维,A⼤B⼩,A包含B,所以A对于B⾜够) 这⾥的证明可以这样想,A⇒B的⽭盾命题,A and (not B);⽽A and (not B) 的⽭盾命题是,(not A) and B 假可以推出真,真不能推出假。
例如,如果明天你死了,那么我还活着。
但是其实不太恰当,因为数理逻辑和真实⽣活中的逻辑还是不太⼀样的。
A ⇔B 即(A ⇒ B)and ( B ⇒ A)A if and only ifB 或者 A is equivalent to B (A ⇒ B) ⇔ ((not B)⇒(not A)) 逆否命题,从集合⾓度很好理解1.2 Notation第⼆章 Vector Spaces and Matrix2.1 Vector and MatrixA column n-vector ,n维列向量A row n-vector,n维列向量转置 T ,向量加(减)法、结合律数乘和分配律 multiplication of a vector by a real scalar、distributive向量的线性相关、线性⽆关、线性组合的概念 linearly independent, coefficients(系数) 定理1. 推论: linear combination,线性组合 线性相关定理 subspace⼦空间,封闭空间概念 span⽣成 basis 基,可由基(线性⽆关的向量组)来张成这个空间。
- 1、下载文档前请自行甄别文档内容的完整性,平台不提供额外的编辑、内容补充、找答案等附加服务。
- 2、"仅部分预览"的文档,不可在线预览部分如存在完整性等问题,可反馈申请退款(可完整预览的文档不适用该条件!)。
- 3、如文档侵犯您的权益,请联系客服反馈,我们会尽快为您处理(人工客服工作时间:9:00-18:30)。
•
HOMEWORK
7,8. Section 5.1: (4),(5). 9. Section 5.4: (7).
38
• Section 5.1, 5.2, 5.4.1-5.4.7
1
2
3
4
5
6
7
8
9
(f)
(a b)
10
11
1
2
k
12
13
14
15
16
17
V
1
18
V
19
One of the most remarkable features of vector spaces is the notion of dimension. We need one simple result that makes this happen, the basis theorem.
24
Norms derived from inner product
1.:Biblioteka 2.:25
If
, we can proof that it is a vector norm.
Firstly, we will proof the
26
Then, we proof the
With the triangle inequality, we can easily proof that is a vector norm. we say it is derived from the inner product.
*Every inner product has the Cauchy-Schwarz inequality. * Every inner product can be used to define a vector norm; * Every vector norm is a continuous function; *All vector norms are equivalent; 37
20
21
22
23
Norms are a way of putting a measure of distance on vector spaces. The purpose is for the refined analysis of vector spaces from the viewpoint of many applications. It is also to all the comparison of various vectors on the basis of their length.
Important principles:
• *A span is a subspace.
•
*Zero vector is l.d. to all vectors; *Subset of a l.i. set is l.i.; *L.i. vectors can be added to form a basis; *Every basis has the same number of vectors; *Each vector has a unique basis-representation;
MATRIX ANALYSIS @ HITSZ
TIME: Autumn 2011 INSTRUCTOR: You-Hua Fan
Lecture 1: Vector Spaces and Vector Norms Reading assignment on the textbook
• Section 0.1
27
In fact, (i) is a norm derived from the standard inner product:
28
29
=
30
31
32
33
34
35
36
CONCLUSION
Basic concepts:
• vector space, subspace, span, • linear combination, linearly independent, linear dependent, basis, dimension, • vector norm, inner product.