2016年美赛A题美国大学生获奖论文
美赛数学建模A题翻译版论文
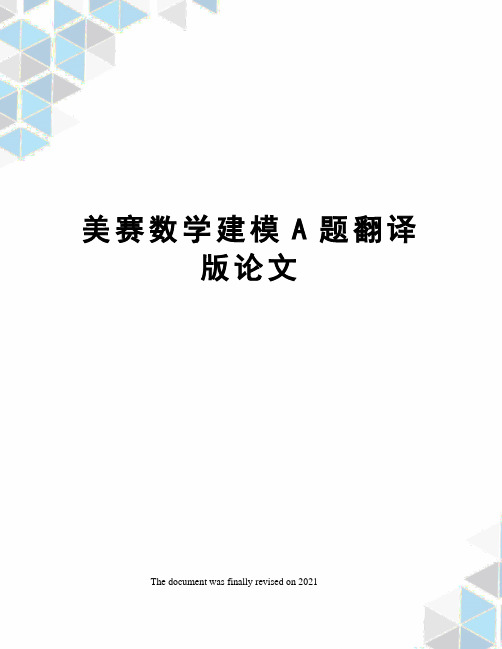
美赛数学建模A题翻译版论文The document was finally revised on 2021数学建模竞赛(MCM / ICM)汇总表基于细胞的高速公路交通模型自动机和蒙特卡罗方法总结基于元胞自动机和蒙特卡罗方法,我们建立一个模型来讨论“靠右行”规则的影响。
首先,我们打破汽车的运动过程和建立相应的子模型car-generation的流入模型,对于匀速行驶车辆,我们建立一个跟随模型,和超车模型。
然后我们设计规则来模拟车辆的运动模型。
我们进一步讨论我们的模型规则适应靠右的情况和,不受限制的情况, 和交通情况由智能控制系统的情况。
我们也设计一个道路的危险指数评价公式。
我们模拟双车道高速公路上交通(每个方向两个车道,一共四条车道),高速公路双向三车道(总共6车道)。
通过计算机和分析数据。
我们记录的平均速度,超车取代率、道路密度和危险指数和通过与不受规则限制的比较评估靠右行的性能。
我们利用不同的速度限制分析模型的敏感性和看到不同的限速的影响。
左手交通也进行了讨论。
根据我们的分析,我们提出一个新规则结合两个现有的规则(靠右的规则和无限制的规则)的智能系统来实现更好的的性能。
1介绍术语假设2模型设计的元胞自动机流入模型跟随模型超车模型超车概率超车条件危险指数两套规则CA模型靠右行无限制行驶规则3补充分析模型加速和减速概率分布的设计设计来避免碰撞4模型实现与计算机5数据分析和模型验证平均速度快车的平均速度密度超车几率危险指数6在不同速度限制下敏感性评价模型7驾驶在左边8交通智能系统智能系统的新规则模型的适应度智能系统结果9结论10优点和缺点优势弱点引用附录。
1 Introduction今天,大约65%的世界人口生活在右手交通的国家和35%在左手交通的国家交通流量。
[worldstandards。
欧盟,2013] 右手交通的国家,比如美国和中国,法规要求驾驶在靠路的右边行走。
多车道高速公路在这些国家经常使用一个规则,要求司机在最右边开车除非他们超过另一辆车,在这种情况下,他们移动到左边的车道、通过,返回到原来的车道。
2016年美赛COMAP50481作答

For office use onlyT1________________ T2________________ T3________________ T4________________Team Control Number50481Problem ChosenBFor office use onlyF1________________F2________________F3________________F4________________2016 Mathematical Contest in Modeling (MCM)Space JunkSummaryWith the rapid pace of peaceful exploitation and utilization of outer space resources and increasing frequency of activities of space launch, environment of space debris is worse and worse and amount of space debris is ever-increasing. Space debris poses great hazard to the safety of spacecraft, which arouses widespread concern, especially when the Russian satellite Kosmos-2251 and the USA satellite Iridium-33 collided on 10 February, 2009.In order to deal with the issue in a better way and search for possible business opportunity, we take into consideration of four sub problems in our paper.In the first model, we find out a program of curves concerning temporal and spatial distribution of space debris. To further understand their intrinsic link and trend, we choose the best fitting function and get distribution rule of space debris in time and space.In the second model, we consider to divide the space into several ball layers. We set probability of collision in space superposition of every ball layer in certain proportion. Then adding probability of unsuccessful launch, we can estimate the risk probability in the whole process is.In the third model, firstly we divide space debris into three categories, concerning each of which carry out revenue analysis quantitatively respectively. We can get revenue gained from three equipment disposing of debris, of which lasers satellites and water jets can achieveand $.In the fourth model, considering that investment of a firm is limited, we establish model of optimization, in which it enables the firm to achieve maximum benefit in every single day. Assume that finance of a firm is 200 billion dollars. We can program in Lingo and get the solution that the amount of each invested equipment is one and benefit of every day is dollars.Content1. Introduction (1)1.1 Background—Space debris and its urgency (1)1.2 Development of research and study (2)1.3 Qur Work (3)2. Model Analysis (3)2.1 Spatial and Temporal Distribution of Space Debris (4)2.1.1 Change of spatial density of space debris with latitude (4)2.1.2 Change of number of space debris with year (7)2.2 Estimate of risk probability (9)2.2.1 Symbol description (9)2.2.2 Assumption (10)2.2.3 Model building (10)2.2.4 Model solving (12)2.3 Number of space debris mitigation and revenue (14)2.3.1 Symbol description (14)2.3.2 Assumption (15)2.3.3 Model building (15)2.3.4 Model solving (16)2.4 Investment strategy of the private firm (18)2.4.1 Symbol description (18)2.4.2 Assumption (19)2.4.3 Model building (19)2.4.4 Model solving (19)3. Innovative Alternative (20)3.1 Ultraviolet Rays Focusing Apparatus (20)3.2 Shield of magnetic field (20)4. Assessment (21)5. Acknowledgements (21)6. Executive Summary (21)Reference (23)1. Introduction1.1Background—Space debris and its urgencySpace junk,also called orbital debris, coupled with activities of space launch, is referred collectively to artificial objects and debris with no function whatsoever. Ever since the Soviet Union launched the first artificial satellite of the world in 1957, 4300 activities of space launch have been carried out and over 5500 spacecraft have been launched into orbits by man. Space debris mainly derive from invalid spacecraft, the end of rocket body, debris left by astronauts and debris from collapse of spacecraft[1]. It’s estimated that there is over 500000 space debris. With the rapid pace of peaceful exploitation and utilization of outer space resources and increasing frequency of activities of space launch, environment of space debris is worse and worse[1]. The collision between space debris and spacecraft will pose hazards to astronautic system in many ways, many of which is fatal. The direct influence on astronautic activities made by space debris mainly aims at spacecraft. Different sizes of space debris will pose damages of different degrees to different parts of spacecraft[2]. The issue sparked widespread attention all over the world, particularly since the Russian satellite Kosmos-2251 and the USA satellite Iridium-33 collided on 10 February, 2009. How to mitigate the space debris effectively has become a problem to be solved.Figure 1.Artists impression of space debris1.2Development of research and studyMicrometeoroids and space debris (orbital debris) are collectively called as M/OD environment[3]. In 1981, American Institute Aeronautics and Astronautics(AIAA) officially proposed report about space debris for the first time. In the same year, National Aeronautics and Space Administration (NASA) started to carry out the 10-year research program of surveillance, modelling and control of M/OD environment. Besides, in 1985, ESA convened a symposium about the reentry and falling of space debris and M/OD research group was set up in the next year. In1993, launched by NASA, ESA, Russia, Japan, IADC was founded and intended to coordinate astronautic countries to act in concert, promote cooperation and communication in the field of space debris one another and address problem of space debris hand-by-hand. Chinese National Space Administration (CNSA) joined IADC officially in 1995. Under the support of national finance, Chinese National Defense and Science and Industry Council has started and carried out the special research work of Action Plan of Space Debris[1].In recent years, through ongoing research of M/OD by many astronautic countries all over the world, remarkable development has been achieved in the technical field of space debris and many approaches have been offered to mitigate space junk.It’s reported by England network section of the m agazine New Scientist that a nanoscale satellite named Cubesail uses solar sail by the aid of solar energy as a propulsion system. In addition to that, another unique function equipped by the sail is that it can help the debris to deorbit and fall into the atmosphere as a rail brake. Vauls Lappas, in charge of the project, said that if Cubesail can work well as expected, an analogous sail also can be fixed onto the future satellite and when it finishes its tasks in space and becomes space debris, the sail can bring them back and burn them down automatically. Or, Cubesails can be launched in swarm to the low earth orbit to trap those floating space debris and mitigate it in a mutually destructive way[4].What’s more, America invented a windmill type equipment for mitigation of space debris, which involves that when debris collides with blades, minute debris will be embedded into the metal fans, which achieves the goal thereby. Even if a little big debris punches through the fan, due to great decrease of the speed by collision and without adequate speed to maintain movement around the earth, it will fall into the atmosphere gradually and be burnt down[4].Another assumption is that when the satellite with a collection net linked by light-duty electronic ropes, reaches the specified location, it will loosen the rope automatically. After the collection net is loaded a certain amount of debris, they will fall into the atmosphere together under the influence of Earth’s magnetic field[4].Actually, NASA set about the tests of Laser Broom since 2000, planning to assist to mitigate the space debris in the trajectory of ISS’s moving with a diameter from 1cm to 10cm. Once certain space debris is targeted, the Laser Broom will send out a laser beam to the side of the space debris back to the earth and gasify it. And thenwith the counterforce of the gas the space debris will be forced to move to the earth and be burned down eventually. In addition, method of suicide satellite and space debris perishing together is also adopted[4].Despite floods of ways to mitigate the space debris, none has been found out to be efficient both technically and economically in the field of astronautics. When it comes to combat with space debris, it’s indispensable to cooperate with all parties, with combination of multiple means policies of prevention and control, which has hope to tackle both the cause and effect of the problem[4].1.3Qur WorkThe problem requires us to do severer jobs to search for business opportunity in the process of mitigating space debris. In order to solve it, we divided this problem into four sub-problems:1).Spatial and Temporal Distribution of Space Debris2).Risk Probability3).Mitigation of Space Debris and Revenue4).Investment Strategy of the Private FirmIn the first model, we can explicitly know about the trend of space debris with the change of time and space. Through fitting the exported data, functions of space debris in time and space can be obtained respectively, which lays solid foundation for our following work.In the second model, we take into consideration of probability of unsuccessful launch and collision with space debris. So we use risk probability to examine and weigh the whole problem and try to make it quantitative. Finally, risk probability can be obtained.In the third model, the ultimate goal of the question is to search for a business opportunity. So we need to analyze the relationship between number of mitigation of debris and revenue. Then, iscretization of the time and determination of daily revenue can get a result.In the last model, to maximize the benefit of a firm, we try to use integral optimization to finish the reasonable and economical distribution, with the assumption of limited investment.2. Model AnalysisIn order to solve it, we divided this problem into four sub-problems:1).Spatial and Temporal Distribution of Space Debris2).Risk Probability3).Mitigation of Space Debris and Revenue4).Investment Strategy of the Private Firm2.1 Spatial and Temporal Distribution of Space Debris2.1.1 Change of spatial density of space debris with latitudeAccording to the information[1], we can get a graph of change of spatial density of space debris with change of latitude. Then through Digitize of Origin software, we export the relevant detailed data shown in the Table 1.Then we fit the data through Origin and the fitting effect is shown in the below Figure 2.Figure 2. The original fitting of latitude and spatial densityIn Origin software, means significant analysis of mathematical statistics, which is an index that verifies whether the assumption is reasonable. Due to, we think the fitting effect is not pretty good. To get better effect, we modify some data (from 785.99738 to 963.50839 ) of abnormal fluctuations. The modified results are shown in the below Table 2.0.00E+0001.00E+0082.00E+0083.00E+0084.00E+0085.00E+0086.00E+008S p a c i a l D e n s i t y (n u /k m ^3)Latitude(km)After modification, we fit the data again and the fitting effect is shown as follows.Figure 3. The modified fitting of latitude and spatial densityNow, , so we can get the function of spatial density ( ) and latitude ( ) of space debris:0.00E+0001.00E+0082.00E+0083.00E+0084.00E+0085.00E+0086.00E+008S p a c i a l D e n s i t y (n u /k m ^3)Latitude(km)2.1.2 Change of number of space debris with yearBesides, we also find graph concerning change of space debris in quantity with year. Similarly, we export the relevant data through Digitize of Origin software shown in the below Table 3.We make the graph by linear fitting the data and get the following fitting effect.Figure 4. The original fitting of number of objects and yearThe fitting effect is not ideal, either, with .Figure 5. Historical growth of space debris through 2010-2000020004000600080001000012000140001600018000N u m b e r o f O b j e c t sYearAccording to the information, some severe collisions and explosions occurred from 2007, such us Fengyun-1c, Iridium-33 and so on and the number of debris soured. So, to avoid abnormal data affecting the results, we decide to analyze it piecewise. Namely, to fit the data and get the piecewise function from 1961 to 2006 and from 2007 to 2013 respectively. And the results are as below.Figure 6. Number of objects from 1961 to 2006 Figure 7. Number of objects from 2007 to 2013From Figure.6 we can get and the function ofnumber of objects with year from 1961-2006 isFrom Figure.7 we can get , and similarly the function of number of objects with year from 2007 to 2013 is2.2 Estimate of risk probability2.2.1Symbol descriptionN u m b er o f O b i e c t sYear N u m b e r o f O b j e c t sYear2.2.2Assumption●Assume that probability of successful launch of required equipment into space by the private firm mentioned in the question is unchangeable.●Assume that orbital altitude of space debris required to be mitigated has an upper bound and a lower bound.●Assume that range of possible collision with spacecraft is a ball layer and spacing between two contiguous layers is unchangeable.●Assume that space debris in every ball layer is distributed evenly.●Assume that both probability of mitigating space debris in every ball layer and probability of collision relate to spatial density.2.2.3Model buildingWhy can we assume that spacing between two contiguous ball layers is?We can consider the fact that when people wait for train pulling into the station, they are forced to st and inside the safe line so that they won’t be trapped in by airflow of running train.Based on the fact above, we can set a spacing. With it, we can describe that when the distance between spacecraft and space debris is beyond the spacing, collision will scarcely happen. So, we divide the range between low earth orbit and the upper bound of the space debris into several ranges with spacing. The schematic diagram is as below.Figure 8. Schematic diagram of space debris ball layers From the assumptions, we know that probability of collision in every ball layer is proportional to the spatial density in the ball layer. To make an estimate, we use the density of the middle of two contiguous layers. Probability of collision happening in the th ball layer isNote: in which is a very small scale coefficient.Based on conditional probability, probability of collision in the th ball layer is The risk probability of working in space born by the firm isBecause the spacing of every two ball layers is unchangeable and equals, we can identify the number of layers by dividing the spatial range. SoThe model of risk probability can be established as follows.According to the reference[7], we can get the function of probability of collision between single space debris and equipment isin which is radius of compound body; is distance on the short axis; is distance on the long axis; and is distance projection of intersection distance on the intersection plane; and is corresponding standard deviation.Figure9. Schematic diagram of parameters of intersection plane2.2.4Model solvingTo solve the model, values of some necessary parameters will be shown as below. And according to data, upper bound of orbital altitude is 2000km, lower bound orbital altitude is 100km and replaced by probability of successful launch of American satellite is 0.87.Considering that double integral is needed for probability of collision between single space debris and spacecraft and double integral is complicated, we hope to get the relationship between probability of collision and distance.Fortunately, noted in some documents[7], function of maximum probability of collision and intersection distance can bein which means intersection distance.To be more intuitive, we plot the function with MATLAB software, shown as below.Figure 10. Relationship of intersection distance and probability From the figure above, we can see that the slope of the curve is ever-decreasing with the increase of intersection distance, which means as intersection increases, the probability of collision becomes smaller.Combining the information in the figure and speed magnitude in factual orbit, we set spacing between layersProbability of collision of single space debris and equipment isDue to pretty small probability of collision, according to function of density, we setThen we substitute the data above in the expression and simplify it. Through MATLAB program we can calculate risk probability and probability of collision, as shown in the below Table 5.According to the results, probability is pretty small. But once collision occurs, it will cause enormous loss and more new debris. Without measures implemented, space in the future will be sieged by space debris.2.3 Number of space debris mitigation and revenue2.3.1Symbol description2.3.2Assumption● Assume that equipment won’t break down for no reason.● Assume that other objects apart from space debris are not taken into account.● Assume that all of the energy of the equipment used in space comes from solar energy and operating it every time may cause wastage of equipment.●Assume that maintenance cost of the equipment used and staff cost is proportional to revenue.●Assume that every equipment has a certain life span and will scrap directly till the time comes2.3.3Model buildingFrom the information[8], we can get the overview of space debris shown in the below Table 6.The amount of debris of cm dominates, about 99.67% and the mass of it only occupies 0.035%. This type of debris poses minute hazard to spacecraft and can be defensed. It’s followed by cm debris with0.031% amount and 0.035% mass , which can destroy spacecraft and is the greatest offender. The amount of debris with over 10 cm in size is the smallest with 99.93% mass however. This kind of debris poses enormous hazard and yet it can be avoided in a negative way.Clearly,total revenue from mitigating space debris in the th way in the required time isAnd total time isFrom model2, we know that the probability of equipment against debris launched successfully isAnd the probability of collision with debris in space is.The probability of unsuccessful launch of the th equipment is, and the corresponding revenue isThe probability of collision with the space debris on the first working day is, and the corresponding revenue isThe probability of collision with the space debris on the second working day is, and the corresponding revenue isBy mathematical induction, the probability of collision with the space debris on the th working day is, and the corresponding revenue isThe revenue gained in the first and second occasion is the same, so it can be induced in the first day. Detailed formula are listed in the following table.According to meaning of expectation, we can deduce that revenue gained every day is2.3.4Model solvingAssume that revenue gained from disposing of three types of debris can be shown as follows.Table8. Revenue of disposing of three types of debrisCalculation of average revenue of space-based laserWhen, it means space-based laser is the equipment of disposing of debris. According to material[9,10], we know about some parameters of the laser shown as follows.Due to lack of data, based on some characteristics, such as laser its major target for debris with 1-10cm in size, we can assume some data shown as below.By programming in MATLAB software, average revenue is. Calculation of average revenue of large satelliteWhen, it means large satellite is the equipment of disposing of debris. According to material[11], we know about some parameters of the satellite shown as follows.Due to lack of data, based on some characteristics, we can assume some data shown as below.Similarly with MATLAB, average revenue is.Calculation of average revenue of space-based water jetsWhen, it means space-based water jets is the equipment of disposing of debris. According to material[12], we know about some parameters of the satellite shown as follows.Due to lack of data, based on some characteristics, we can assume some data shown as below.Also with MATLAB, average revenue is.2.4 Investment strategy of the private firm2.4.1Symbol2.4.2Assumption●Assume that a firm sets earning profit as main goal.2.4.3Model buildingConstraint of finance isObjective function, benefit gained in time isBut the objective function has two variations, to be more convenient, to maximize the benefit, we consider to set benefit gained every day maximal.The final model of optimization is2.4.4Model solvingAssume that a firm has 200 billion dollars. According to model 3, benefit gained by lasers, large satellites, water jets in every single day equals to ratio of total benefit to days, the results of which are shown as follows.From the table above, it's apparent to see that the highest revenue gained is the second equipment----satellites, of which the benefit is 654670$, followed by which is the first method----lasers; the lowest one is water jets, 448854$.So the model can be established as follows:This is a problem of integer programming and the results are shown as below via Lingo.According to the table, ultimate investment strategy of a firm is shown that the firm spend 200 billion$ in investing water jets. Through Internet, the price of water jets is found cheap relatively and the efficiency is favorable, so the redults are credible comp aratively.3. Innovative AlternativeIf no commercial opportunity is possible, we will provide two innovative alternatives for avoiding collisions.3.1 Ultraviolet Rays Focusing ApparatusTo avoid collision in space, we take into consideration of the technology of ultraviolet rays, which are abundant in space and pose enormous harm to living beings on earth. So if we human can take fully advantage of ultraviolet and mitigate the ultraviolet rays in space in a degree, it will kill two birds with one stone.We surmise that a device with high temperature resistance, radiation resistance and heat resistance, can be fixed on the spacecraft and probes and absorbs ultraviolet rays at the time of operating in space. The device can transform the ultraviolet rays to enormous energy. Once encountering approaching space debris, the spacecraft will recognize it and release high energy to the target. The space debris will absorb energy and accelerate and transform the orbit, to avoid collision.3.2 Shield of magnetic fieldWith geomagnetic field as a protective umbrella of the earth, our home won’t be intruded upon by many kinds of radiation in outer space. Can we consider such a circumstance that a magnetic field can be artificially established inside the spacecraft according to the factual size of the spacecraft, of which applied force tends to be in a reasonable range. Neither does it have an effect on components of the spacecraft, nordoes it exert counteraction to debris outside to make it far away from the spacecraft and avoid collision accordingly. If spacecraft can absorb energy of sunlight used by created magnetic field, cost can be reduced effectively.4. AssessmentWe establish four models above and find out some advantages and disadvantages through some analysis.AdvantagesModel1:Fully fit temporal and spatial distribution of space debris.Model2:●Divide space into several ball layers to increase accuracy of probability calculation.● Use fitting functions to simplify calculating of probability of collision. Model 3: Take full consideration of space debris of different types.WeaknessModel1: Leave out individual data and decrease accuracy of trend.Model2: Ignore other factors in space and bring about errors with scale coefficient. Model3: Lack data and decrease accuracy of the final results.5. AcknowledgementsSo far, we have basically finished the paper. Recollecting the process of setting up the model, we have encountered couples of obstacles, but via constant absorption and updating pool of information and knowledge, we gradually sorted out our thoughts and tackled the problem in a better way finally. We hereby express great gratitude to the relevant authors and websites providing document and web data. It’s you that make our results more credible and rich the contents of our paper. Thank you very much.6. Executive SummaryBe a member of mitigating space debrisAs we all know, the amount of space debris in orbit around earth has been a heated issue recently. It is estimated that over 500,000 pieces of space debris, also called orbital debris, are currently being tracked as potential hazards to spacecraft. The issue itself became more widely discussed in the news media when the Russian satellite Kosmos-2251 and the USA satellite Iridium-33 collided on 10 February, 2009.Generally, space debris can be divided into three types. The first type is spacecraft debris caused by explosion with or without intention. Most of it comes from the so-called preview of space war between Union Soviet and America. The second type is inadvertent carelessness of astronauts, such as leaving bolts, spacers, pen and even life garbage. The last type is wreckage of rockets and satellites. Some invalid satellites are still floating in orbit, which will be like ghosts for possible decades of years.To address the big problem, what we should do is to be a member to devote to mitigating space debris. Saying it in a more serious way, we ought to act in concert with astronautic countries, promote cooperation and communication in the field of space debris one another and address problem of space debris hand-by-hand.Concerning the severity of the issue, we want to propose our concept to devote to dealing with space debris, which will help the private firm to gain certain profit. The detailed project is as follows.According to the results by reasonable analysis and accurate calculation, we consider that two hundred small, space-based water jets and zero high energy laser used to target specific pieces of debris and zero large satellite designed to sweep up the debris should be produced and dispatched to carry out clearing work, which will obtain over $ per day.A lot of elements can account for our idea. Some technical reasons will be left out and the following might be the critical ones.Firstly, in term of their working mechanism, a water jet loaded by a rocket into space creates a special liquid wall, which will reduce the speed of debris once it bumps into the wall. A laser can directly gasify the debris with high temperature or decelerate and deorbit the debris. Large satellite can capture the running debris and return it to the earth atmosphere.Secondly, in term of their best targeted debris in different sizes, water jets are target for space debris with below 10cm in size; Lasers are mainly tools for space debris with 1-10cm in size; Satellites are good at dealing with space debris with over 1cm in size. So, clear to see that every type of equipment possesses respective specialty and applying medicine according to indications is of great importance and necessity to proceed the severe task.Thirdly, in term of their advantages and disadvantages, water jets won’t bring about any extra pollution as other method does, but its feasibility is not favorable. Lasers will not be susceptible by atmospheric scattering and is able to deal with debris at any location with high efficiency. However, it’s too expensive to afford many of it。
2016年美赛A题O奖论文
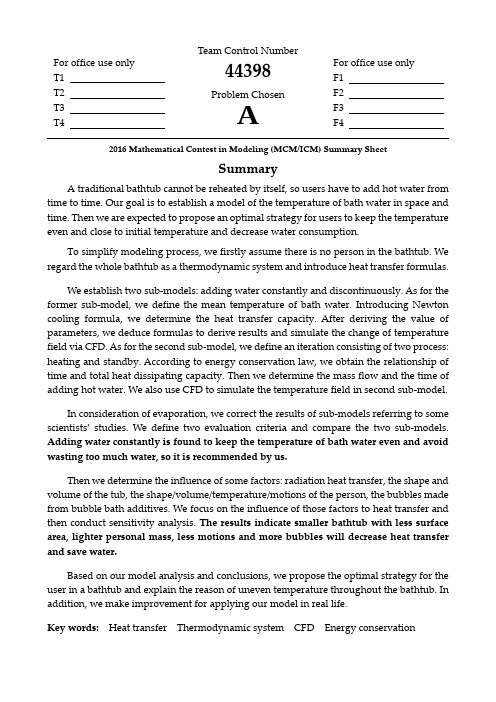
2016 Mathematical Contest in Modeling (MCM/ICM) Summary Sheet SummaryA traditional bathtub cannot be reheated by itself, so users have to add hot water from time to time. Our goal is to establish a model of the temperature of bath water in space and time. Then we are expected to propose an optimal strategy for users to keep the temperature even and close to initial temperature and decrease water consumption.To simplify modeling process, we firstly assume there is no person in the bathtub. We regard the whole bathtub as a thermodynamic system and introduce heat transfer formulas.We establish two sub-models: adding water constantly and discontinuously. As for the former sub-model, we define the mean temperature of bath water. Introducing Newton cooling formula, we determine the heat transfer capacity. After deriving the value of parameters, we deduce formulas to derive results and simulate the change of temperature field via CFD. As for the second sub-model, we define an iteration consisting of two process: heating and standby. According to energy conservation law, we obtain the relationship of time and total heat dissipating capacity. Then we determine the mass flow and the time of adding hot water. We also use CFD to simulate the temperature field in second sub-model.In consideration of evaporation, we correct the results of sub-models referring to some scientists’ studies. We define two evaluation criteria and compare the two sub-models. Adding water constantly is found to keep the temperature of bath water even and avoid wasting too much water, so it is recommended by us.Then we determine the influence of some factors: radiation heat transfer, the shape and volume of the tub, the shape/volume/temperature/motions of the person, the bubbles made from bubble bath additives. We focus on the influence of those factors to heat transfer and then conduct sensitivity analysis. The results indicate smaller bathtub with less surface area, lighter personal mass, less motions and more bubbles will decrease heat transfer and save water.Based on our model analysis and conclusions, we propose the optimal strategy for the user in a bathtub and explain the reason of uneven temperature throughout the bathtub. In addition, we make improvement for applying our model in real life.Key words: Heat transfer Thermodynamic system CFD Energy conservation For office use onlyT1 T2 T3 T4 For office use only F1 F2F3 F4 Team Control Number 44398 Problem Chosen AEnjoy a Cozy and Green BathContents1 Introduction (4)1.1 Background (4)1.2Literature Review (4)1.3Restatement of the Problem (4)2Assumptions and Justification (6)3Notations (7)4Model Overview (7)5 Sub-model I : Adding Water Continuously (8)5.1 Model Establishment (9)5.1.1 Control Equations and Boundary Conditions (9)5.1.2 Definition of the Mean Temperature (11)5.1.3 Determination of Heat Transfer Capacity (11)5.2 Results (13)5.2.1 Determination of Parameters (13)5.2.2 Calculating Results (14)5.2.3 Simulating Results (15)6 Sub-model II: Adding Water Discontinuously (18)6.1 Heating Model (18)6.1.1 Control Equations and Boundary Conditions (18)6.1.2 Determination of Inflow Time and Amount (19)6.2 Standby Model (20)6.2.1 Process Analysis (20)6.2.2 Calculation of Parameters (20)6.3 Results (21)6.3.1 Determination of Parameters (21)6.3.2 Calculating Results (23)6.3.3 Simulating Results (23)6.4 Conclusion (27)7 Correction and Contrast of Sub-Models (27)7.1 Correction with Evaporation Heat Transfer (27)7.1.1 Correction Principle (27)7.1.2 Correction Results (28)7.2 Contrast of Two Sub-Models (30)7.2.1 Evaluation Criteria (30)7.2.2 Determination of Water Consumption (30)7.2.3 Conclusion (31)8 Model Analysis and Sensitivity Analysis (31)8.1 The Influence of Different Bathtubs (32)8.1.1 Different Volumes of Bathtubs (32)8.1.2 Different Shapes of Bathtubs (34)8.2 The Influence of Person in Bathtub (36)8.2.1 When the Person Remains Static in a Bathtub (36)8.2.2 When the Person Moves in a Bathtub (37)8.2.3 Results Analysis and Sensitivity Analysis (38)8.3 The Influence of Bubble Bath Additives (42)8.4 The Influence of Radiation Heat Transfer (44)8.5 Conclusion (45)9 Further Discussion (45)9.1 Different Distribution of Inflow Faucets (45)9.2 Model Application (46)10 Strength and Weakness (47)10.1 Strength (47)10.2 Weakness (47)Report (49)Reference (50)。
美赛a题思路
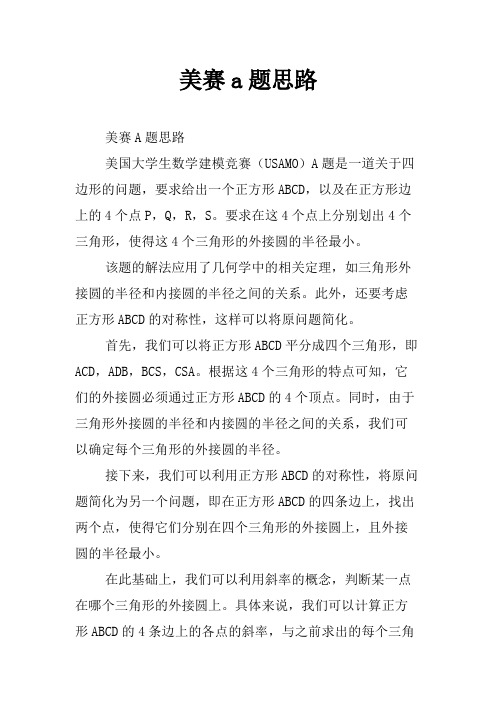
美赛a题思路
美赛A题思路
美国大学生数学建模竞赛(USAMO)A题是一道关于四边形的问题,要求给出一个正方形ABCD,以及在正方形边上的4个点P,Q,R,S。
要求在这4个点上分别划出4个三角形,使得这4个三角形的外接圆的半径最小。
该题的解法应用了几何学中的相关定理,如三角形外接圆的半径和内接圆的半径之间的关系。
此外,还要考虑正方形ABCD的对称性,这样可以将原问题简化。
首先,我们可以将正方形ABCD平分成四个三角形,即ACD,ADB,BCS,CSA。
根据这4个三角形的特点可知,它们的外接圆必须通过正方形ABCD的4个顶点。
同时,由于三角形外接圆的半径和内接圆的半径之间的关系,我们可以确定每个三角形的外接圆的半径。
接下来,我们可以利用正方形ABCD的对称性,将原问题简化为另一个问题,即在正方形ABCD的四条边上,找出两个点,使得它们分别在四个三角形的外接圆上,且外接圆的半径最小。
在此基础上,我们可以利用斜率的概念,判断某一点在哪个三角形的外接圆上。
具体来说,我们可以计算正方形ABCD的4条边上的各点的斜率,与之前求出的每个三角
形外接圆的斜率进行比较,从而判断某一点在哪个三角形的外接圆上。
最后,我们可以通过对四条边上的点的排列组合,找出最优解,即得到4个三角形的外接圆的半径最小的情况。
以上就是美赛A题的思路。
解决该题,需要综合运用几何学、代数学中的相关定理,以及斜率的概念,最终求出最优解。
美赛6种题型及通关详解
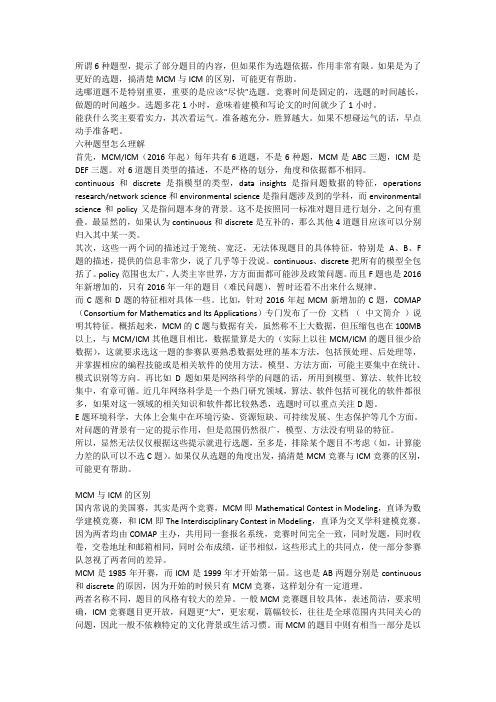
所谓6种题型,提示了部分题目的内容,但如果作为选题依据,作用非常有限。
如果是为了更好的选题,搞清楚MCM与ICM的区别,可能更有帮助。
选哪道题不是特别重要,重要的是应该“尽快”选题。
竞赛时间是固定的,选题的时间越长,做题的时间越少。
选题多花1小时,意味着建模和写论文的时间就少了1小时。
能获什么奖主要看实力,其次看运气。
准备越充分,胜算越大。
如果不想碰运气的话,早点动手准备吧。
六种题型怎么理解首先,MCM/ICM(2016年起)每年共有6道题,不是6种题,MCM是ABC三题,ICM是DEF三题。
对6道题目类型的描述,不是严格的划分,角度和依据都不相同。
continuous和discrete是指模型的类型,data insights是指问题数据的特征,operations research/network science和environmental science是指问题涉及到的学科,而environmental science和policy又是指问题本身的背景。
这不是按照同一标准对题目进行划分,之间有重叠。
最显然的,如果认为continuous和discrete是互补的,那么其他4道题目应该可以分别归入其中某一类。
其次,这些一两个词的描述过于笼统、宽泛,无法体现题目的具体特征,特别是A、B、F 题的描述,提供的信息非常少,说了几乎等于没说。
continuous、discrete把所有的模型全包括了。
policy范围也太广,人类主宰世界,方方面面都可能涉及政策问题。
而且F题也是2016年新增加的,只有2016年一年的题目(难民问题),暂时还看不出来什么规律。
而C题和D题的特征相对具体一些。
比如,针对2016年起MCM新增加的C题,COMAP (Consortium for Mathematics and Its Applications)专门发布了一份文档(中文简介)说明其特征。
概括起来,MCM的C题与数据有关,虽然称不上大数据,但压缩包也在100MB 以上,与MCM/ICM其他题目相比,数据量算是大的(实际上以往MCM/ICM的题目很少给数据),这就要求选这一题的参赛队要熟悉数据处理的基本方法,包括预处理、后处理等,并掌握相应的编程技能或是相关软件的使用方法。
美赛论文(最终版)

For office use onlyT1________________ T2________________ T3________________ T4________________Team Control Number 46639Problem ChosenCFor office use onlyF1________________F2________________F3________________F4________________2016 MCM/ICM Summary SheetAn Optimal Investment Strategy ModelSummaryWe develop an optimal investment strategy model that appears to hold promise for providing insight into not only how to sort the schools according to investment priority, but also identify optimal investment amount of a specific school. This model considers a large number of parameters thought to be important to investment in the given College Scorecard Data Set.In order to develop the required model, two sub-models are constructed as follows: 1.For Analytic Hierarchy Process (AHP) Model, we identify the prioritizedcandidate list of schools by synthesizing the elements which have an influence on investment. First we define the specific value of any two elements’ effect on investment. And then the weight of each element’s influence on investment can be identified. Ultimately, we take the relevant parameters into the calculated weight, and then we get any school’s recommended value of investment.2.For Return On Investment M odel, it’s constructed on the basis of AHP Model.Let us suppose that all the investment is used to help the students to pay tuition fee.Then we can see optimal investment as that we help more students to the universities of higher return rate. However, because of dropout rate, there will be an optimization investment amount in each university. Therefore, we can change the problem into a nonlinear programming problem. We identify the optimal investment amount by maximizing return-on-investment.Specific attention is given to the stability and error analysis of our model. The influence of the model is discussed when several fundamental parameters vary. We attempt to use our model to prioritize the schools and identify investment amount of the candidate schools, and then an optimal investment strategy is generated. Ultimately, to demonstrate how our model works, we apply it to the given College Scorecard Data Set. For various situations, we propose an optimal solution. And we also analyze the strengths and weaknesses of our model. We believe that we can make our model more precise if more information are provided.Contents1.Introduction 21.1Restatement of the Problem (2)1.2Our Approach (2)2.Assumptions 23.Notations 34.The Optimal Investment Model 44.1Analytic Hierarchy Process Model (4)4.1.1Constructing the Hierarchy (4)4.1.2Constructing the Judgement Matrix (5)4.1.3Hierarchical Ranking (7)4.2Return On Investment Model (8)4.2.1Overview of the investment strategy (8)4.2.2Analysis of net income and investment cost (9)4.2.3Calculate Return On Investment (11)4.2.4Maximize the Total Net Income (11)5.Test the Model125.1Error Analysis (12)5.2Stability Analysis (13)6.Results136.1Results of Analytic Hierarchy Process (13)6.2Results of Return On Investment Model (14)7.Strengths and Weaknesses157.1Strengths (15)7.2Weaknesses (16)References16 Appendix A Letter to the Chief Financial Officer, Mr. Alpha Chiang.171.Introduction1.1Restatement of the ProblemIn order to help improve educational performance of undergraduates attending colleges and universities in the US, the Goodgrant Foundation intends to donate a total of $100,000,000 to an appropriate group of schools per year, for five years, starting July 2016. We are to develop a model to determine an optimal investment strategy that identifies the school, the investment amount per school, the return on that investment, and the time duration that the organization’s money should be provided to have the highest likelihood of producing a strong positive effect on student performance. Considering that they don’t want to duplicate the investments and focus of other large grant organizations, we interpret optimal investment as a strategy that maximizes the ROI on the premise that we help more students attend better colleges. So the problems to be solved are as follows:1.How to prioritize the schools by optimization level.2.How to measure ROI of a school.3.How to measure investment amount of a specific school.1.2Our ApproachWe offer a model of optimal investment which takes a great many factors in the College Scorecard Data Set into account. To begin with, we make a 1 to N optimized and prioritized candidate list of school we are recommending for investment by the AHP model. For the sake that we invest more students to better school, several factors are considered in the AHP model, such as SAT score, ACT score, etc. And then, we set investment amount of each university in the order of the list according to the standard of maximized ROI. The implement details of the model will be described in section 4.2.AssumptionsWe make the following basic assumptions in order to simplify the problem. And each of our assumptions is justified.1.Investment amount is mainly used for tuition and fees. Considering that theincome of an undergraduate is usually much higher than a high school students, we believe that it’s necessary to help more poor students have a chance to go to college.2.Bank rates will not change during the investment period. The variation ofthe bank rates have a little influence on the income we consider. So we make this assumption just to simplify the model.3.The employment rates and dropout rates will not change, and they aredifferent for different schools4.For return on investment, we only consider monetary income, regardlessof the intangible income.3.NotationsWe use a list of symbols for simplification of expression.4.The Optimal Investment ModelIn this section, we first prioritize schools by the AHP model (Section 4.1), and then calculate ROI value of the schools (Section 4.2). Ultimately, we identify investment amount of every candidate schools according to ROI (Section 4.3).4.1Analytic Hierarchy Process ModelIn order to prioritize schools, we must consider each necessary factor in the College Scorecard Data Set. For each factor, we calculate its weight value. And then, we can identify the investment necessity of each school. So, the model can be developed in 3 steps as follows:4.1.1Constructing the HierarchyWe consider 19 elements to measure priority of candidate schools, which can be seen in Fig 1. The hierarchy could be diagrammed as follows:Fig.1AHP for the investment decisionThe goal is red, the criteria are green and the alternatives are blue. All the alternatives are shown below the lowest level of each criterion. Later in the process, each alternatives will be rated with respect to the criterion directly above it.As they build their hierarchy, we should investigate the values or measurements of the different elements that make it up. If there are published fiscal policy, for example, or school policy, they should be gathered as part of the process. This information will be needed later, when the criteria and alternatives are evaluated.Note that the structure of the investment hierarchy might be different for other foundations. It would definitely be different for a foundation who doesn't care how much his score is, knows he will never dropout, and is intensely interested in math, history, and the numerous aspects of study[1].4.1.2Constructing the Judgement MatrixHierarchy reflects the relationship among elements to consider, but elements in the Criteria Layer don’t always weigh equal during aim measure. In deciders’ mind, each element accounts for a particular proportion.To incorporate their judgments about the various elements in the hierarchy, decision makers compare the elements “two by two”. The fundamental scale for pairwise comparison are shown in Fig 2.Fig 2Right now, let's see which items are compared. Our example will begin with the six criteria in the second row of the hierarchy in Fig 1, though we could begin elsewhere if we want. The criteria will be compared as to how important they are to the decisionmakers, with respect to the goal. Each pair of items in this row will be compared.Fig 3 Investment Judgement MatrixIn the next row, there is a group of 19 alternatives under the criterion. In the subgroup, each pair of alternatives will be compared regarding their importance with respect to the criterion. (As always, their importance is judged by the decision makers.) In the subgroup, there is only one pair of alternatives. They are compared as to how important they are with respect to the criterion.Things change a bit when we get to the alternatives row. Here, the factor in each group of alternatives are compared pair-by-pair with respect to the covering criterion of the group, which is the node directly above them in the hierarchy. What we are doing here is evaluating the models under consideration with respect to score, then with respect to Income, then expenditure, dropout rate, debt and graduation rate.The foundation can evaluate alternatives against their covering criteria in any order they choose. In this case, they choose the order of decreasing priority of the covering criteria.Fig 4 Score Judgement MatrixFig 5 Expenditure Judgement MatrixFig 6 Income Judgement MatrixFig 7 Dropout Judgement MatrixFig 8 Debt Judgement MatrixFig 9 Graduation Matrix4.1.3 Hierarchical RankingWhen the pairwise comparisons are as numerous as those in our example, specialized AHP software can help in making them quickly and efficiently. We will assume that the foundation has access to such software, and that it allows the opinions of various foundations to be combined into an overall opinion for the group.The AHP software uses mathematical calculations to convert these judgments to priorities for each of the six criteria. The details of the calculations are beyond the scope of this article, but are readily available elsewhere[2][3][4][5]. The software also calculates a consistency ratio that expresses the internal consistency of the judgments that have been entered. In this case the judgments showed acceptable consistency, and the software used the foundation’s inputs to assign these new priorities to the criteria:Fig 10.AHP hierarchy for the foundation investing decision.In the end, the AHP software arranges and totals the global priorities for each of the alternatives. Their grand total is 1.000, which is identical to the priority of the goal. Each alternative has a global priority corresponding to its "fit" to all the foundation's judgments about all those aspects of factor. Here is a summary of the global priorities of the alternatives:Fig 114.2 ROI Model4.2.1 Overview of the investment strategyConsider a foundation making investment on a set of N geographically dispersed colleges and university in the United States, D = {1, 2, 3……N }. Then we can select top N schools from the candidate list which has been sorted through analytic hierarchy process. The total investment amount is M per year which is donated by the Goodgrant Foundation. The investment amount is j m for each school j D ∈, satisfying the following balance constraint:j j D mM ∈=∑ (1)W e can’t invest too much or too little money to one school because we want to help more students go to college, and the student should have more choices. Then the investment amount for each school must have a lower limit lu and upper limit bu as follows:j lu m bu ≤≤ (2)The tuition and fees is j p , and the time duration is {1,2,3,4}j t ∈. To simplify ourmodel, we assume that our investment amount is only used for freshmen every year. Because a freshmen oriented investment can get more benefits compared with others. For each school j D ∈, the number of the undergraduate students who will be invested is j n , which can be calculated by the following formula :,jj j j m n j D p t =∈⨯ (3)Figure12The foundation can use the ROI model to identify j m and j t so that it canmaximize the total net income. Figure1 has shown the overview of our investment model. We will then illustrate the principle and solution of this model by a kind of nonlinear programming method.4.2.2 Analysis of net income and investment costIn our return on investment model, we first focus on analysis of net income and investment cost. Obviously, the future earnings of undergraduate students are not only due to the investment itself. There are many meaning factors such as the effort, the money from their parents, the training from their companies. In order to simplify the model, we assume that the investment cost is the most important element and we don’t consider other possible influence factors. Then we can conclude that the total cost of the investment is j m for each school j D ∈.Figure 13For a single student, the meaning of the investment benefits is the expected earnings in the future. Assuming that the student is not going to college or university after graduating from high school and is directly going to work. Then his wage base is 0b as a high school graduate. If he works as a college graduate, then his wage base is 0a . Then we can give the future proceeds of life which is represented symbolically by T and we use r to represent the bank rates which will change over time. We assume that the bank rates will not change during the investment period. Here, we use bank rates in 2016 to represent the r . The future proceeds of life of a single undergraduate student will be different due to individual differences such as age, physical condition environment, etc. If we consider these differences, the calculation process will be complicated. For simplicity’s sake, we uniform the future proceeds of life T for 20 years. Then we will give two economics formulas to calculate the total expected income in the next T years for graduates and high school graduates:40(1)Tk k a u r +==+∑(4) 40(1)T kk b h r +==+∑(5) The total expected income of a graduate is u , and the total expected income of a highschool graduate is h .Then, we continue to analyze the net income. The net income can be calculated by the following formula:os NetIncome TotalIncome C t =- (6) For each school j D ∈, the net income is j P , the total income is j Q , and the cost is j m . Then we will get the following equation through formula (6):j j j P Q m =- (7)Therefore, the key of the problem is how to calculate j Q . In order to calculate j Q, weneed to estimate the number of future employment j ne . The total number of the invested is j n , which has been calculated above. Considering the dropout rates j α and the employment rates j β for each school j , we can calculate the number of future employment j ne through the following formula:(4)(1)jt j j j j n e n βα-=⨯⨯- (8)That way, we can calculate j Q by the following formula:()j j Q ne u h =⨯- (9)Finally, we take Eq. (2) (3) (4) (7) (8) into Eq. (6), and we will obtain Eq. (9) as follows:4(4)00400(1)()(1)(1)j TT t j j j j j k kk k j jm a b P m p t r r βα+-+===⨯⨯-⨯--⨯++∑∑ (10) We next reformulate the above equation of j P for concise presentation:(4)(1)j t j jj j j jc m P m t λα-⨯⨯=⨯-- (11)where jj j p βλ= and 400400(1)(1)TT k kk k a b c r r ++===-++∑∑ .4.2.3 Calculate Return On InvestmentROI is short of return on investment which can be determined by net income andinvestment cost [7]. It conveys the meaning of the financial assessment. For each schoolj D ∈ , the net income is j P , and the investment cost equals to j m . Then the j ROIcan be calculated by the following formula:100%j j jP ROI m =⨯ (12)We substitute Eq. (10) into Eq. (11), and we will get a new formula as follows:(4)((1)1)100%j t j j j jc ROI t λα-⨯=⨯--⨯ (13)4.2.4 Maximize the Total Net IncomeGiven the net income of each school, we formulate the portfolio problem that maximize the total net income, S=Max(4)((1))j t j jj j j j Dj Djc m P m t λα-∈∈⨯⨯=⨯--∑∑ (14)S. T.jj DmM ∈=∑,{1,2,3,4}t = ,j lu m bu ≤≤ ,Considering the constraint jj DmM ∈=∑, we can further simplify the model,S is equivalent to S’=Max(4)((1))j t j jj j j Dj Djc m P t λα-∈∈⨯⨯=⨯-∑∑ (15)S. T.jj DmM ∈=∑,{1,2,3,4t = ,j l u m b u ≤≤. By solving the nonlinear programming problem S’, we can get the sameanswer as problem S.5. Testing the Model 5.1 Error AnalysisSince the advent of analytic hierarchy process, people pay more attention to it due to the specific applicability, convenience, practicability and systematization of the method. Analytic hierarchy process has not reached the ideal situation whether in theory or application level because the results depend largely on the preference and subjective judgment. In this part, we will analyze the human error problem in analytic hierarchy process.Human error is mainly caused by human factors. The human error mainly reflects on the structure of the judgment matrix. The causes of the error are the following points:1. The number of times that human judge the factors’ importance is excessive.2. The calibration method is not perfect.Then we will give some methods to reduce errors:1. Reduce times of human judgment. One person repeatedly gave the samejudgment between two factors. Or many persons gave the same judgment between two factors one time. Finally, we take the average as result.2. Break the original calibration method. If we have defined the ranking vector111121(,...)n a a a a =between the factor 1A with others. Then we can get all theother ranking vector. For example : 12122111(,1...)na a a a a =.5.2 Stability AnalysisIt is necessary to analyze the stability of ranking result [6], because the strong subjectivefactors. If the ranking result changed a little while the judgment changed a lot, we can conclude that the method is effective and the result is acceptable, and vice versa. We assume that the weight of other factors will change if the weight of one factor changed from i ξ to i η:[8](1)(,1,2...,)(1)i j j i i j n i j ηξηξ-⨯==≠- (16)And it is simple to verify the equation:11nii η==∑ (17)And the new ranking vector ω will be:A ωη=⨯ (18)By this method, the Relative importance between other factors remain the same while one of the factor has changed.6. Results6.1 Results of Analytic Hierarchy ProcessWe can ranking colleges through the analytic hierarchy process, and we can get the top N = 20 schools as follows6.2 Results of Return On Investment ModelBased on the results above, we next use ROI model to distribute investment amountj m and time duration j t for each school j D ∈ by solving the following problem:Max (4)((1))j t j jj j j Dj Djc m P t λα-∈∈⨯⨯=⨯-∑∑S. T.jj DmM ∈=∑,{1,2,3,4t = , j l u m b u≤≤ . In order to solve the problem above, we collected the data from different sources. Inthe end, we solve the model with Lingo software. The program code is as follows:model: sets:roi/1..20/:a,b,p,m,t;endsets data:a = 0.9642 0.9250 0.9484 0.9422 0.9402 0.9498 0.90490.9263 0.9769 0.9553 0.9351 0.9123 0.9410 0.98610.9790 0.9640 0.8644 0.9598 0.9659 0.9720;b = 0.8024 0.7339 0.8737 0.8308 0.8681 0.7998 0.74920.6050 0.8342 0.8217 0.8940 0.8873 0.8495 0.87520.8333 0.8604 0.8176 0.8916 0.7527 0.8659;p = 3.3484 3.7971 3.3070 3.3386 3.3371 3.4956 3.22204.0306 2.8544 3.1503 3.2986 3.3087 3.3419 2.78452.9597 2.92713.3742 2.7801 2.5667 2.8058;c = 49.5528;enddatamax=@sum(roi(I):m(I)/t(I)/p(I)*((1-b(I))^4)*c*(1-a(I)+0.05)^(4-t(I)));@for(roi:@gin(t));@for(roi(I):@bnd(1,t(I),4));@for(roi(I):@bnd(0,m(I),100));@sum(roi(I):m(I))=1000;ENDFinally, we can get the investment amount and time duration distribution as follows:7.Strengths and Weaknesses7.1Strengths1.Fixing the bank rates during the investment period may run out, but it will haveonly marginal influences.2.For return on investment, we only consider monetary income, regardless of the3.intangible income. But the quantization of these intangible income is very importantand difficult. It needs to do too much complicated technical analysis and to quantify 4.too many variables. Considering that the investment persists for a short time, thiskind of random error is acceptable.5.Due to our investment which is freshmen oriented, other students may feel unfair.It is likely to produce adverse reaction to our investment strategy.6.The cost estimation is not impeccable. We only consider the investment amount andignore other non-monetary investment.5. AHP needs higher requirements for personnel quality.7.2Weaknesses1.Our investment strategy is distinct and clear, and it is convenient to implement.2.Our model not only identifies the investment amount for each school, but alsoidentifies the time duration that the organization’s money should be provide d.3.Data processing is convenient, because the most data we use is constant, average ormedian.4.Data sources are reliable. Our investment strategy is based on some meaningful anddefendable subset of two data sets.5.AHP is more simple, effective and universal.References[1] Saaty, Thomas L. (2008). Decision Making for Leaders: The Analytic Hierarchy Process for Decisions in a Complex World. Pittsburgh, Pennsylvania: RWS Publications. ISBN 0-9620317-8-X.[2] Bhushan, Navneet, Kanwal Rai (January 2004). Strategic Decision Making: Applying the Analytic Hierarchy Process. London: Springer-Verlag. ISBN 1-8523375-6-7.[3] Saaty, Thomas L. (2001). Fundamentals of Decision Making and Priority Theory. Pittsburgh, Pennsylvania: RWS Publications. ISBN 0-9620317-6-3.[4] Trick, Michael A. (1996-11-23). "Analytic Hierarchy Process". Class Notes. Carnegie Mellon University Tepper School of Business. Retrieved 2008-03-02.[5] Meixner, Oliver; Reiner Haas (2002). Computergestützte Entscheidungs-findung: Expert Choice und AHP – innovative Werkzeuge zur Lösung komplexer Probleme (in German). Frankfurt/Wien: Redline Wirtschaft bei Ueberreuter. ISBN 3-8323-0909-8.[6] Hazelkorn, E. The Impact of League Tables and Ranking System on Higher Education Decision Making [J]. Higher Education Management and Policy, 2007, 19(2), 87-110.[7] Leslie: Trainer Assessment: A Guide to Measuring the Performance of Trainers and Facilitors, Second Edition, Gower Publishing Limited, 2002.[8] Aguaron J, Moreno-Jimenea J M. Local stability intervals in the analytic hierarchy process. European Journal of Operational Research. 2000Letter to the Chief Financial Officer, Mr. Alpha Chiang. February 1th, 2016.I am writing this letter to introduce our optimal investment strategy. Before I describe our model, I want to discuss our proposed concept of a return-on-investment (ROI). And then I will describe the optimal investment model by construct two sub-model, namely AHP model and ROI model. Finally, the major results of the model simulation will be showed up to you.Considering that the Goodgrant Foundation aims to help improve educational performance of undergraduates attending colleges and universities in the US, we interpret return-on-investment as the increased income of undergraduates. Because the income of an undergraduate is generally much higher than a high school graduate, we suggest all the investment be used to pay for the tuition and fees. In that case, if we take both the income of undergraduates’ income and dropout rate into account, we can get the return-in-investment value.Our model begins with the production of an optimized and prioritized candidate list of schools you are recommending for investment. This sorted list of school is constructed through the use of specification that you would be fully qualified to provided, such as the score of school, the income of graduate student, the dropout rate, etc. With this information, a precise investment list of schools will be produced for donation select.Furthermore, we developed the second sub-model, ROI model, which identifies the investment amount of each school per year. If we invest more money in a school, more students will have a chance to go to college. However, there is an optimal investment amount of specific school because of the existence of dropout. So, we can identify every candidate school’s in vestment amount by solve a nonlinear programming problem. Ultimately, the result of the model simulation show that Washington University, New York University and Boston College are three schools that worth investing most. And detailed simulation can be seen in our MCM Contest article.We hope that this model is sufficient in meeting your needs in any further donation and future philanthropic educational investments within the United States.。
2016 美国大学生数学竞赛优秀论文AB
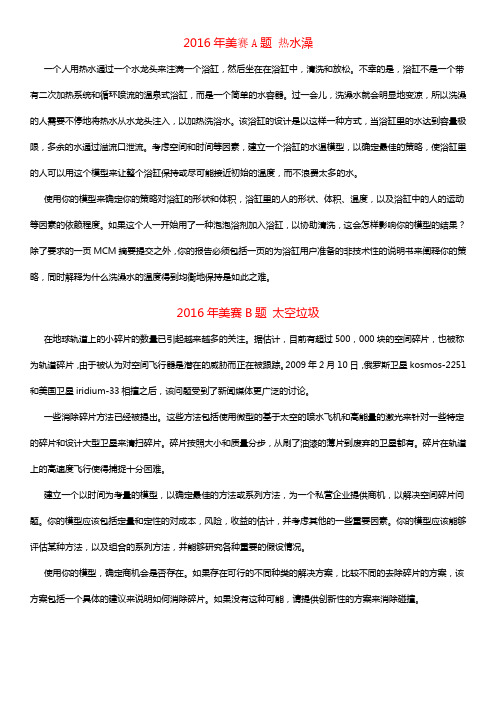
2016年美赛A题热水澡一个人用热水通过一个水龙头来注满一个浴缸,然后坐在在浴缸中,清洗和放松。
不幸的是,浴缸不是一个带有二次加热系统和循环喷流的温泉式浴缸,而是一个简单的水容器。
过一会儿,洗澡水就会明显地变凉,所以洗澡的人需要不停地将热水从水龙头注入,以加热洗浴水。
该浴缸的设计是以这样一种方式,当浴缸里的水达到容量极限,多余的水通过溢流口泄流。
考虑空间和时间等因素,建立一个浴缸的水温模型,以确定最佳的策略,使浴缸里的人可以用这个模型来让整个浴缸保持或尽可能接近初始的温度,而不浪费太多的水。
使用你的模型来确定你的策略对浴缸的形状和体积,浴缸里的人的形状、体积、温度,以及浴缸中的人的运动等因素的依赖程度。
如果这个人一开始用了一种泡泡浴剂加入浴缸,以协助清洗,这会怎样影响你的模型的结果?除了要求的一页MCM摘要提交之外,你的报告必须包括一页的为浴缸用户准备的非技术性的说明书来阐释你的策略,同时解释为什么洗澡水的温度得到均衡地保持是如此之难。
2016年美赛B题太空垃圾在地球轨道上的小碎片的数量已引起越来越多的关注。
据估计,目前有超过500,000块的空间碎片,也被称为轨道碎片,由于被认为对空间飞行器是潜在的威胁而正在被跟踪。
2009年2月10日,俄罗斯卫星kosmos-2251和美国卫星iridium-33相撞之后,该问题受到了新闻媒体更广泛的讨论。
一些消除碎片方法已经被提出。
这些方法包括使用微型的基于太空的喷水飞机和高能量的激光来针对一些特定的碎片和设计大型卫星来清扫碎片。
碎片按照大小和质量分步,从刷了油漆的薄片到废弃的卫星都有。
碎片在轨道上的高速度飞行使得捕捉十分困难。
建立一个以时间为考量的模型,以确定最佳的方法或系列方法,为一个私营企业提供商机,以解决空间碎片问题。
你的模型应该包括定量和定性的对成本,风险,收益的估计,并考虑其他的一些重要因素。
你的模型应该能够评估某种方法,以及组合的系列方法,并能够研究各种重要的假设情况。
美赛一等奖论文-中文翻译版

目录问题回顾 (3)问题分析: (4)模型假设: (6)符号定义 (7)4.1---------- (8)4.2 有热水输入的温度变化模型 (17)4.2.1模型假设与定义 (17)4.2.2 模型的建立The establishment of the model (18)4.2.3 模型求解 (19)4.3 有人存在的温度变化模型Temperature model of human presence (21)4.3.1 模型影响因素的讨论Discussion influencing factors of the model (21)4.3.2模型的建立 (25)4.3.3 Solving model (29)5.1 优化目标的确定 (29)5.2 约束条件的确定 (31)5.3模型的求解 (32)5.4 泡泡剂的影响 (35)5.5 灵敏度的分析 (35)8 non-technical explanation of the bathtub (37)Summary人们经常在充满热水的浴缸里得到清洁和放松。
本文针对只有一个简单的热水龙头的浴缸,建立一个多目标优化模型,通过调整水龙头流量大小和流入水的温度来使整个泡澡过程浴缸内水温维持基本恒定且不会浪费太多水。
首先分析浴缸中水温度变化的具体情况。
根据能量转移的特点将浴缸中的热量损失分为两类情况:沿浴缸四壁和底面向空气中丧失的热量根据傅里叶导热定律求出;沿水面丧失的热量根据水由液态变为气态的焓变求出。
因涉及的参数过多,将系数进行回归分析的得到一个一元二次函数。
结合两类热量建立了温度关于时间的微分方程。
加入阻滞因子考虑环境温湿度升高对水温的影响,最后得到水温度随时间的变化规律(见图**)。
优化模型考虑保持水龙头匀速流入热水的情况。
将过程分为浴缸未加满和浴缸加满而水从排水口溢出的两种情况,根据能量守恒定律优化上述微分方程,建立一个有热源的情况下水的温度随时间变化的分段模型,(见图**)接下来考虑人在浴缸中对水温的影响。
2016年美国大学生数学建模大赛A题获奖论文A Hot Bath
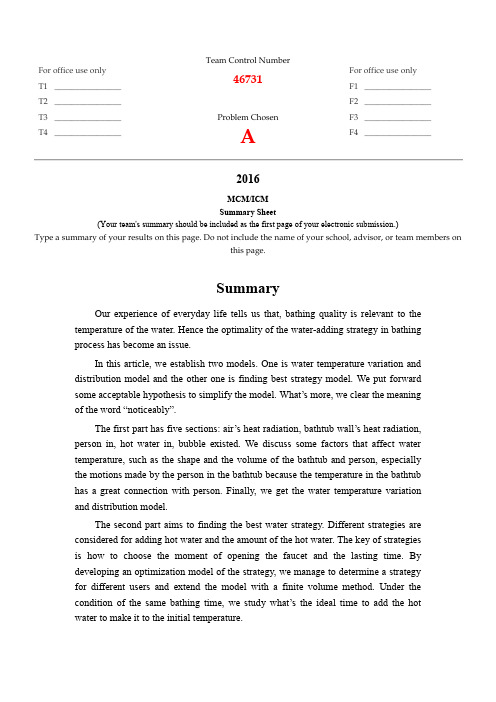
The first part has five sections: air’s heat radiation, bathtub wall’s heat radiation, person in, hot water in, bubble existed. We discuss some factors that affect water temperature, such as the shape and the volume of the bathtub and person, especially the motions made by the person in the bathtub because the temperature in the bathtub has a great connection with person. Finally, we get the water temperature variation and distribution model.
In this article, we establish two models. One is water temperature variation and distr one is finding best strategy model. We put forward some acceptable hypothesis to simplify the model. What’s more, we clear the meaning of the word “noticeably”.
2016美国大学生数学建模大赛C题特等奖(原版论文)C42939Tsinghua University, China
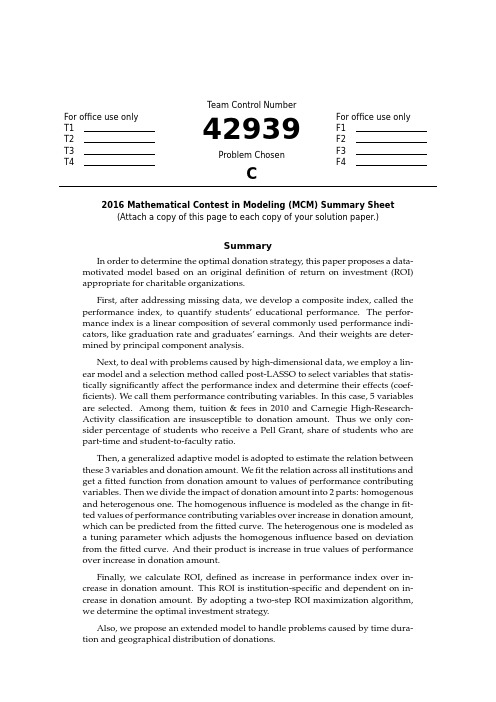
For office use only T1T2T3T4T eam Control Number42939Problem ChosenCFor office use onlyF1F2F3F42016Mathematical Contest in Modeling(MCM)Summary Sheet (Attach a copy of this page to each copy of your solution paper.)SummaryIn order to determine the optimal donation strategy,this paper proposes a data-motivated model based on an original definition of return on investment(ROI) appropriate for charitable organizations.First,after addressing missing data,we develop a composite index,called the performance index,to quantify students’educational performance.The perfor-mance index is a linear composition of several commonly used performance indi-cators,like graduation rate and graduates’earnings.And their weights are deter-mined by principal component analysis.Next,to deal with problems caused by high-dimensional data,we employ a lin-ear model and a selection method called post-LASSO to select variables that statis-tically significantly affect the performance index and determine their effects(coef-ficients).We call them performance contributing variables.In this case,5variables are selected.Among them,tuition&fees in2010and Carnegie High-Research-Activity classification are insusceptible to donation amount.Thus we only con-sider percentage of students who receive a Pell Grant,share of students who are part-time and student-to-faculty ratio.Then,a generalized adaptive model is adopted to estimate the relation between these3variables and donation amount.Wefit the relation across all institutions and get afitted function from donation amount to values of performance contributing variables.Then we divide the impact of donation amount into2parts:homogenous and heterogenous one.The homogenous influence is modeled as the change infit-ted values of performance contributing variables over increase in donation amount, which can be predicted from thefitted curve.The heterogenous one is modeled as a tuning parameter which adjusts the homogenous influence based on deviation from thefitted curve.And their product is increase in true values of performance over increase in donation amount.Finally,we calculate ROI,defined as increase in performance index over in-crease in donation amount.This ROI is institution-specific and dependent on in-crease in donation amount.By adopting a two-step ROI maximization algorithm, we determine the optimal investment strategy.Also,we propose an extended model to handle problems caused by time dura-tion and geographical distribution of donations.A Letter to the CFO of the Goodgrant FoundationDear Chiang,Our team has proposed a performance index quantifying the students’educational per-formance of each institution and defined the return of investment(ROI)appropriately for a charitable organization like Goodgrant Foundation.A mathematical model is built to help predict the return of investment after identifying the mechanism through which the donation generates its impact on the performance.The optimal investment strategy is determined by maximizing the estimated return of investment.More specifically,the composite performance index is developed after taking all the pos-sible performance indicators into consideration,like graduation rate and graduates’earnings. The performance index is constructed to represents the performance of the school as well as the positive effect that a college brings to students and the community.From this point of view, our definition manages to capture social benefits of donation.And then we adopt a variable selection method tofind out performance contributing vari-ables,which are variables that strongly affect the performance index.Among all the perfor-mance contributing variables we select,three variables which can be directly affected by your generous donation are kept to predict ROI:percentage of students who receive a Pell Grant, share of students who are part-time and student-to-faculty ratio.Wefitted a relation between these three variables and the donation amount to predict change in value of each performance contributing variable over your donation amount.And we calculate ROI,defined as increase in the performance index over your donation amount, by multiplying change in value of each performance contributing variable over your donation amount and each performance contributing variable’s effect on performance index,and then summing up the products of all performance contributing variables.The optimal investment strategy is decided after maximizing the return of investment according to an algorithm for selection.In conclusion,our model successfully produced an investment strategy including a list of target institutions and investment amount for each institution.(The list of year1is attached at the end of the letter).The time duration for the investment could also be determined based on our model.Since the model as well as the evaluation approach is fully data-motivated with no arbitrary criterion included,it is rather adaptable for solving future philanthropic educational investment problems.We have a strong belief that our model can effectively enhance the efficiency of philan-thropic educational investment and provides an appropriate as well as feasible way to best improve the educational performance of students.UNITID names ROI donation 197027United States Merchant Marine Academy21.85%2500000 102711AVTEC-Alaska’s Institute of Technology21.26%7500000 187745Institute of American Indian and Alaska Native Culture20.99%2000000 262129New College of Florida20.69%6500000 216296Thaddeus Stevens College of Technology20.66%3000000 229832Western Texas College20.26%10000000 196158SUNY at Fredonia20.24%5500000 234155Virginia State University20.04%10000000 196200SUNY College at Potsdam19.75%5000000 178615Truman State University19.60%3000000 199120University of North Carolina at Chapel Hill19.51%3000000 101648Marion Military Institute19.48%2500000187912New Mexico Military Institute19.31%500000 227386Panola College19.28%10000000 434584Ilisagvik College19.19%4500000 199184University of North Carolina School of the Arts19.15%500000 413802East San Gabriel Valley Regional Occupational Program19.09%6000000 174251University of Minnesota-Morris19.09%8000000 159391Louisiana State University and Agricultural&Mechanical Col-19.07%8500000lege403487Wabash Valley College19.05%1500000 Yours Sincerely,Team#42939An Optimal Strategy of Donation for Educational PurposeControl Number:#42939February,2016Contents1Introduction51.1Statement of the Problem (5)1.2Baseline Model (5)1.3Detailed Definitions&Assumptions (8)1.3.1Detailed Definitions: (8)1.3.2Assumptions: (9)1.4The Advantages of Our Model (9)2Addressing the Missing Values93Determining the Performance Index103.1Performance Indicators (10)3.2Performance Index via Principal-Component Factors (10)4Identifying Performance Contributing Variables via post-LASSO115Determining Investment Strategy based on ROI135.1Fitted Curve between Performance Contributing Variables and Donation Amount145.2ROI(Return on Investment) (15)5.2.1Model of Fitted ROIs of Performance Contributing Variables fROI i (15)5.2.2Model of the tuning parameter P i (16)5.2.3Calculation of ROI (17)5.3School Selection&Investment Strategy (18)6Extended Model186.1Time Duration (18)6.2Geographical Distribution (22)7Conclusions and Discussion22 8Reference23 9Appendix241Introduction1.1Statement of the ProblemThere exists no doubt in the significance of postsecondary education to the development of society,especially with the ascending need for skilled employees capable of complex work. Nevertheless,U.S.ranks only11th in the higher education attachment worldwide,which makes thefinancial support from large charitable organizations necessary.As it’s essential for charitable organizations to maximize the effectiveness of donations,an objective and systematic assessment model is in demand to develop appropriate investment strategies.To achieve this goal,several large foundations like Gates Foundation and Lumina Foundation have developed different evaluation approaches,where they mainly focus on spe-cific indexes like attendance and graduation rate.In other empirical literature,a Forbes ap-proach(Shifrin and Chen,2015)proposes a new indicator called the Grateful Graduates Index, using the median amount of private donations per student over a10-year period to measure the return on investment.Also,performance funding indicators(Burke,2002,Cave,1997,Ser-ban and Burke,1998,Banta et al,1996),which include but are not limited to external indicators like graduates’employment rate and internal indicators like teaching quality,are one of the most prevailing methods to evaluate effectiveness of educational donations.However,those methods also arise with widely acknowledged concerns(Burke,1998).Most of them require subjective choice of indexes and are rather arbitrary than data-based.And they perform badly in a data environment where there is miscellaneous cross-section data but scarce time-series data.Besides,they lack quantified analysis in precisely predicting or measuring the social benefits and the positive effect that the investment can generate,which serves as one of the targets for the Goodgrant Foundation.In accordance with Goodgrant Foundation’s request,this paper provides a prudent def-inition of return on investment(ROI)for charitable organizations,and develops an original data-motivated model,which is feasible even faced with tangled cross-section data and absent time-series data,to determine the optimal strategy for funding.The strategy contains selection of institutions and distribution of investment across institutions,time and regions.1.2Baseline ModelOur definition of ROI is similar to its usual meaning,which is the increase in students’educational performance over the amount Goodgrant Foundation donates(assuming other donationsfixed,it’s also the increase in total donation amount).First we cope with data missingness.Then,to quantify students’educational performance, we develop an index called performance index,which is a linear composition of commonly used performance indicators.Our major task is to build a model to predict the change of this index given a distribution of Goodgrant Foundation$100m donation.However,donation does not directly affect the performance index and we would encounter endogeneity problem or neglect effects of other variables if we solely focus on the relation between performance index and donation amount. Instead,we select several variables that are pivotal in predicting the performance index from many potential candidates,and determine their coefficients/effects on the performance index. We call these variables performance contributing variables.Due to absence of time-series data,it becomes difficult tofigure out how performance con-tributing variables are affected by donation amount for each institution respectively.Instead, wefit the relation between performance contributing variables and donation amount across all institutions and get afitted function from donation amount to values of performance contribut-ing variables.Then we divide the impact of donation amount into2parts:homogenous and heteroge-nous one.The homogenous influence is modeled as the change infitted values of performance contributing variables over increase in donation amount(We call these quotientsfitted ROI of performance contributing variable).The heterogenous one is modeled as a tuning parameter, which adjusts the homogenous influence based on deviation from thefitted function.And their product is the institution-specific increase in true values of performance contributing variables over increase in donation amount(We call these values ROI of performance contributing vari-able).The next step is to calculate the ROI of the performance index by adding the products of ROIs of performance contributing variables and their coefficients on the performance index. This ROI is institution-specific and dependent on increase in donation amount.By adopting a two-step ROI maximization algorithm,we determine the optimal investment strategy.Also,we propose an extended model to handle problems caused by time duration and geographical distribution of donations.Note:we only use data from the provided excel table and that mentioned in the pdffile.Table1:Data SourceVariable DatasetPerformance index Excel tablePerformance contributing variables Excel table and pdffileDonation amount PdffileTheflow chart of the whole model is presented below in Fig1:Figure1:Flow Chart Demonstration of the Model1.3Detailed Definitions&Assumptions 1.3.1Detailed Definitions:1.3.2Assumptions:A1.Stability.We assume data of any institution should be stable without the impact from outside.To be specific,the key factors like the donation amount and the performance index should remain unchanged if the college does not receive new donations.A2.Goodgrant Foundation’s donation(Increase in donation amount)is discrete rather than continuous.This is reasonable because each donation is usually an integer multiple of a minimum amount,like$1m.After referring to the data of other foundations like Lumina Foundation,we recommend donation amount should be one value in the set below:{500000,1000000,1500000, (10000000)A3.The performance index is a linear composition of all given performance indicators.A4.Performance contributing variables linearly affect the performance index.A5.Increase in donation amount affects the performance index through performance con-tributing variables.A6.The impact of increase in donation amount on performance contributing variables con-tains2parts:homogenous one and heterogenous one.The homogenous influence is repre-sented by a smooth function from donation amount to performance contributing variables.And the heterogenous one is represented by deviation from the function.1.4The Advantages of Our ModelOur model exhibits many advantages in application:•The evaluation model is fully data based with few subjective or arbitrary decision rules.•Our model successfully identifies the underlying mechanism instead of merely focusing on the relation between donation amount and the performance index.•Our model takes both homogeneity and heterogeneity into consideration.•Our model makes full use of the cross-section data and does not need time-series data to produce reasonable outcomes.2Addressing the Missing ValuesThe provided datasets suffer from severe data missing,which could undermine the reliabil-ity and interpretability of any results.To cope with this problem,we adopt several different methods for data with varied missing rate.For data with missing rate over50%,any current prevailing method would fall victim to under-or over-randomization.As a result,we omit this kind of data for simplicity’s sake.For variables with missing rate between10%-50%,we use imputation techniques(Little and Rubin,2014)where a missing value was imputed from a randomly selected similar record,and model-based analysis where missing values are substituted with distribution diagrams.For variables with missing rate under10%,we address missingness by simply replace miss-ing value with mean of existing values.3Determining the Performance IndexIn this section,we derive a composite index,called the performance index,to evaluate the educational performance of students at every institution.3.1Performance IndicatorsFirst,we need to determine which variables from various institutional performance data are direct indicators of Goodgrant Foundation’s major concern–to enhance students’educational performance.In practice,other charitable foundations such as Gates Foundation place their focus on core indexes like attendance and graduation rate.Logically,we select performance indicators on the basis of its correlation with these core indexes.With this method,miscellaneous performance data from the excel table boils down to4crucial variables.C150_4_P OOLED_SUP P and C200_L4_P OOLED_SUP P,as completion rates for different types of institutions,are directly correlated with graduation rate.We combine them into one variable.Md_earn_wne_p10and gt_25k_p6,as different measures of graduates’earnings,are proved in empirical studies(Ehren-berg,2004)to be highly dependent on educational performance.And RP Y_3Y R_RT_SUP P, as repayment rate,is also considered valid in the same sense.Let them be Y1,Y2,Y3and Y4.For easy calculation and interpretation of the performance index,we apply uniformization to all4variables,as to make sure they’re on the same scale(from0to100).3.2Performance Index via Principal-Component FactorsAs the model assumes the performance index is a linear composition of all performance indicators,all we need to do is determine the weights of these variables.Here we apply the method of Customer Satisfaction Index model(Rogg et al,2001),where principal-component factors(pcf)are employed to determine weights of all aspects.The pcf procedure uses an orthogonal transformation to convert a set of observations of pos-sibly correlated variables into a set of values of linearly uncorrelated variables called principal-component factors,each of which carries part of the total variance.If the cumulative proportion of the variance exceeds80%,it’s viable to use corresponding pcfs(usually thefirst two pcfs)to determine weights of original variables.In this case,we’ll get4pcfs(named P CF1,P CF2,P CF3and P CF4).First,the procedure provides the linear coefficients of Y m in the expression of P CF1and P CF2.We getP CF1=a11Y1+a12Y2+a13Y3+a14Y4P CF2=a21Y1+a22Y2+a23Y3+a24Y4(a km calculated as corresponding factor loadings over square root of factor k’s eigenvalue) Then,we calculate the rough weights c m for Y m.Let the variance proportions P CF1and P CF2 represent be N1and N2.We get c m=(a1m N1+a2m N2)/(N1+N2)(This formulation is justifiedbecause the variance proportions can be viewed as the significance of pcfs).If we let perfor-mance index=(P CF 1N 1+P CF 2N 2)/(N 1+N 2),c m is indeed the rough weight of Y m in terms of variance)Next,we get the weights by adjusting the sum of rough weights to 1:c m =c m /(c 1+c 2+c 3+c 4)Finally,we get the performance index,which is the weighted sum of the 4performance indicator.Performance index= m (c m Y m )Table 2presents the 10institutions with largest values of the performance index.This rank-ing is highly consistent with widely acknowledged rankings,like QS ranking,which indicates the validity of the performance index.Table 2:The Top 10Institutions in Terms of Performance IndexInstitutionPerformance index Los Angeles County College of Nursing and Allied Health79.60372162Massachusetts Institute of Technology79.06066895University of Pennsylvania79.05044556Babson College78.99269867Georgetown University78.90468597Stanford University78.70586395Duke University78.27719116University of Notre Dame78.15843964Weill Cornell Medical College 78.143341064Identifying Performance Contributing Variables via post-LASSO The next step of our model requires identifying the factors that may exert an influence on the students’educational performance from a variety of variables mentioned in the excel table and the pdf file (108in total,some of which are dummy variables converted from categorical variables).To achieve this purpose,we used a model called LASSO.A linear model is adopted to describe the relationship between the endogenous variable –performance index –and all variables that are potentially influential to it.We assign appropriate coefficient to each variable to minimize the square error between our model prediction and the actual value when fitting the data.min β1J J j =1(y j −x T j β)2where J =2881,x j =(1,x 1j ,x 2j ,...,x pj )THowever,as the amount of the variables included in the model is increasing,the cost func-tion will naturally decrease.So the problem of over fitting the data will arise,which make the model we come up with hard to predict the future performance of the students.Also,since there are hundreds of potential variables as candidates.We need a method to identify the variables that truly matter and have a strong effect on the performance index.Here we take the advantage of a method named post-LASSO (Tibshirani,1996).LASSO,also known as the least absolute shrinkage and selection operator,is a method used for variableselection and shrinkage in medium-or high-dimensional environment.And post-LASSO is to apply ordinary least squares(OLS)to the model selected byfirst-step LASSO procedure.In LASSO procedure,instead of using the cost function that merely focusing on the square error between the prediction and the actual value,a penalty term is also included into the objective function.We wish to minimize:min β1JJj=1(y j−x T jβ)2+λ||β||1whereλ||β||1is the penalty term.The penalty term takes the number of variables into con-sideration by penalizing on the absolute value of the coefficients and forcing the coefficients of many variables shrink to zero if this variable is of less importance.The penalty coefficient lambda determines the degree of penalty for including variables into the model.After min-imizing the cost function plus the penalty term,we couldfigure out the variables of larger essence to include in the model.We utilize the LARS algorithm to implement the LASSO procedure and cross-validation MSE minimization(Usai et al,2009)to determine the optimal penalty coefficient(represented by shrinkage factor in LARS algorithm).And then OLS is employed to complete the post-LASSO method.Figure2:LASSO path-coefficients as a function of shrinkage factor sFigure3:Cross-validated MSEFig2.displays the results of LASSO procedure and Fig3displays the cross-validated MSE for different shrinkage factors.As specified above,the cross-validated MSE reaches minimum with shrinkage factor between0.4-0.8.We choose0.6andfind in Fig2that6variables have nonzero coefficients via the LASSO procedure,thus being selected as the performance con-tributing variables.Table3is a demonstration of these6variables and corresponding post-LASSO results.Table3:Post-LASSO resultsDependent variable:performance_indexPCTPELL−26.453∗∗∗(0.872)PPTUG_EF−14.819∗∗∗(0.781)StudentToFaculty_ratio−0.231∗∗∗(0.025)Tuition&Fees20100.0003∗∗∗(0.00002)Carnegie_HighResearchActivity 5.667∗∗∗(0.775)Constant61.326∗∗∗(0.783)Observations2,880R20.610Adjusted R20.609Note:PCTPELL is percentage of students who receive aPell Grant;PPTUG_EF is share of students who are part-time;Carnegie_HighResearchActivity is Carnegie classifica-tion basic:High Research ActivityThe results presented in Table3are consistent with common sense.For instance,the pos-itive coefficient of High Research Activity Carnegie classification implies that active research activity helps student’s educational performance;and the negative coefficient of Student-to-Faculty ratio suggests that decrease in faculty quantity undermines students’educational per-formance.Along with the large R square value and small p-value for each coefficient,the post-LASSO procedure proves to select a valid set of performance contributing variables and describe well their contribution to the performance index.5Determining Investment Strategy based on ROIWe’ve identified5performance contributing variables via post-LASSO.Among them,tu-ition&fees in2010and Carnegie High-Research-Activity classification are quite insusceptible to donation amount.So we only consider the effects of increase in donation amount on per-centage of students who receive a Pell Grant,share of students who are part-time and student-to-faculty ratio.We denote them with F1,F2and F3,their post-LASSO coefficients withβ1,β2andβ3.In this section,wefirst introduce the procedure used tofit the relation between performance contributing variables and donation amount.Then we provide the model employed to calcu-latefitted ROIs of performance contributing variables(the homogenous influence of increase in donation amount)and the tuning parameter(the heterogenous influence of increase in dona-tion amount).Next,we introduce how to determine stly,we show how the maximiza-tion determines the investment strategy,including selection of institutions and distribution of investments.5.1Fitted Curve between Performance Contributing Variables and Donation AmountSince we have already approximated the linear relation between the performance index with the3performance contributing variables,we want to know how increase in donation changes them.In this paper,we use Generalized Adaptive Model(GAM)to smoothlyfit the relations. Generalized Adaptive Model is a generalized linear model in which the dependent variable depends linearly on unknown smooth functions of independent variables.Thefitted curve of percentage of students who receive a Pell Grant is depicted below in Fig4(see the other two fitted curves in Appendix):Figure4:GAM ApproximationA Pell Grant is money the U.S.federal government provides directly for students who needit to pay for college.Intuitively,if the amount of donation an institution receives from other sources such as private donation increases,the institution is likely to use these donations to alleviate students’financial stress,resulting in percentage of students who receive a Pell Grant. Thus it is reasonable to see afitted curve downward sloping at most part.Also,in commonsense,an increase in donation amount would lead to increase in the performance index.This downward sloping curve is consistent with the negative post-LASSO coefficient of percentage of students who receive a Pell Grant(as two negatives make a positive).5.2ROI(Return on Investment)5.2.1Model of Fitted ROIs of Performance Contributing Variables fROI iFigure5:Demonstration of fROI1Again,we usefitted curve of percentage of students who receive a Pell Grant as an example. We modeled the bluefitted curve to represent the homogeneous relation between percentage of students who receive a Pell Grant and donation amount.Recallfitted ROI of percentage of students who receive a Pell Grant(fROI1)is change in fitted values(∆f)over increase in donation amount(∆X).SofROI1=∆f/∆XAccording to assumption A2,the amount of each Goodgrant Foundation’s donation falls into a pre-specified set,namely,{500000,1000000,1500000,...,10000000}.So we get a set of possible fitted ROI of percentage of students who receive a Pell Grant(fROI1).Clearly,fROI1is de-pendent on both donation amount(X)and increase in donation amount(∆X).Calculation of fitted ROIs of other performance contributing variables is similar.5.2.2Model of the tuning parameter P iAlthough we’ve identified the homogenous influence of increase in donation amount,we shall not neglect the fact that institutions utilize donations differently.A proportion of do-nations might be appropriated by the university’s administration and different institutions allocate the donation differently.For example,university with a more convenient and well-maintained system of identifying students who needfinancial aid might be willing to use a larger portion of donations to directly aid students,resulting in a lower percentage of under-graduate students receiving Pell grant.Also,university facing lower cost of identifying and hiring suitable faculty members might be inclined to use a larger portion of donations in this direction,resulting in a lower student-to-faculty ratio.These above mentioned reasons make institutions deviate from the homogenousfitted func-tion and presents heterogeneous influence of increase in donation amount.Thus,while the homogenous influence only depends on donation amount and increase in donation amount, the heterogeneous influence is institution-specific.To account for this heterogeneous influence,we utilize a tuning parameter P i to adjust the homogenous influence.By multiplying the tuning parameter,fitted ROIs of performance con-tributing variables(fitted value changes)convert into ROI of performance contributing variable (true value changes).ROI i=fROI i·P iWe then argue that P i can be summarized by a function of deviation from thefitted curve (∆h),and the function has the shape shown in Fig6.The value of P i ranges from0to2,because P i can be viewed as an amplification or shrinkage of the homogenous influence.For example,P i=2means that the homogeneous influence is amplified greatly.P i=0means that this homogeneous influence would be entirely wiped out. The shape of the function is as shown in Fig6because of the following reasons.Intuitively,if one institution locates above thefitted line,when deviation is small,the larger it is,the larger P i is.This is because the institution might be more inclined to utilize donations to change that factor.However,when deviation becomes even larger,the institution grows less willing to invest on this factor.This is because marginal utility decreases.The discussion is similar if one institution initially lies under thefitted line.Thus,we assume the function mapping deviation to P i is similar to Fig6.deviation is on the x-axis while P i is on the y-axis.Figure6:Function from Deviation to P iIn order to simplify calculation and without loss of generality,we approximate the function。
美国 数学 竞赛 2016A 试题

What is the value of?SolutionFor what value ofdoes?SolutionFor every dollar Ben spent on bagels, David spent cents less. Ben paidmore than David. How much did theyspend in the bagel store together?SolutionThe remainder can be defined for all real numbersandwithbywhere denotes the greatest integer less than or equal to . What is the value of ?SolutionA rectangular box has integer side lengths in the ratio. Which of the following could be the volume of the box?SolutionXimena lists the whole numbers through once. Emilio copies Ximena's numbers, replacing each occurrence of the digit by the digit . Ximena adds her numbers and Emilio adds his numbers. How much larger is Ximena's sum than Emilio's?2016 AMC 10A ProblemsProblem 1Problem 2Problem 3Problem 4Problem 5Problem 6SolutionThe mean, median, and mode of the data valuesare all equal to. What is thevalue of ?SolutionTrickster Rabbit agrees with Foolish Fox to double Fox's money every time Fox crosses the bridge by Rabbit's house, as long as Fox pays coins in toll to Rabbit after each crossing. The payment is made after the doubling, Fox is excited about his good fortune until he discovers that all his money is gone after crossing the bridge three times. How many coins did Fox have at the beginning?SolutionA triangular array of coins has coin in the first row, coins in the second row, coins in the third row, and so onup to coins in theth row. What is the sum of the digits of ?SolutionA rug is made with three different colors as shown. The areas of the three differently colored regions form an arithmeticprogression. The inner rectangle is one foot wide, and each of the two shaded regions is foot wide on all four sides.What is the length in feet of the inner rectangle?SolutionProblem 7Problem 8Problem 9Problem 10What is the area of the shaded region of the givenrectangle?SolutionThree distinct integers are selected at random between and , inclusive. Which of the following is a correct statement about the probability that the product of the three integers is odd?SolutionFive friends sat in a movie theater in a row containing seats, numbered to from left to right. (The directions "left" and "right" are from the point of view of the people as they sit in the seats.) During the movie Ada went to the lobby to get some popcorn. When she returned, she found that Bea had moved two seats to the right, Ceci had moved one seat to the left,and Dee and Edie had switched seats, leaving an end seat for Ada. In which seat had Ada been sitting before she got up?SolutionHow many ways are there to writeas the sum of twos and threes, ignoring order? (For example,andare two such ways.)SolutionSeven cookies of radius inch are cut from a circle of cookie dough, as shown. Neighboring cookies are tangent, and all except the center cookie are tangent to the edge of the dough. The leftover scrap is reshaped to form another cookie of the same thickness. What is the radius in inches of the scrap cookie?Problem 11Problem 12Problem 13Problem 14Problem 15SolutionA triangle with vertices ,, andis reflected about the-axis, then the imageis rotated counterclockwise about the origin byto produce. Which of the followingtransformations will return to ?counterclockwise rotation about the origin by .clockwise rotation about the origin by .reflection about the-axisreflection about the line reflection about the -axis.SolutionLetbe a positive multiple of . One red ball and green balls are arranged in a line in random order. Letbethe probability that at leastof the green balls are on the same side of the red ball. Observe that and thatapproachesasgrows large. What is the sum of the digits of the least value ofsuch that?SolutionEach vertex of a cube is to be labeled with an integer through , with each integer being used once, in such a way that the sum of the four numbers on the vertices of a face is the same for each face. Arrangements that can be obtained from each other through rotations of the cube are considered to be the same. How many different arrangements are possible?Problem 16Problem 17Problem 18SolutionIn rectangle and. Pointbetweenand, and pointbetweenandaresuch that. Segmentsand intersect at and , respectively. The ratio can be written aswhere the greatest common factor ofand is 1. What is?SolutionFor some particular value of , when is expanded and like terms are combined, theresulting expression contains exactly terms that include all four variables and , each to some positivepower. What is ?SolutionCircles with centers and , having radii and , respectively, lie on the same side of line and are tangent toat and , respectively, with between and . The circle with center is externally tangent to each of the other two circles. What is the area of triangle ?SolutionFor some positive integer, the numberhaspositive integer divisors, including and the number .How many positive integer divisors does the numberhave?SolutionA binary operation has the properties that and that for all nonzero realnumbers and . (Here represents multiplication). The solution to the equation canbe written as , where and are relatively prime positive integers. What isSolutionProblem 19Problem 20Problem 21Problem 22Problem 23Copyright © 2016 Art of Problem SolvingA quadrilateral is inscribed in a circle of radius . Three of the sides of this quadrilateral have length. What isthe length of the fourth side?SolutionHow many ordered triplesof positive integers satisfyand?Solution2 3 4 5 6 7 8 9 10 11 12 13 14 15 16 17 18 19 20 21 22 23 24 25AMC 10AMC 10 Problems and Solutions 2016 AMC 10AMathematics competition resourcesThe problems on this page are copyrighted by the Mathematical Association of America's American MathematicsCompetitions.Retrieved from "/wiki/index.php?title=2016_AMC_10A_Problems&oldid=75390"Problem 24Problem 25See also。
2016美国大学生数学建模竞赛F题M奖
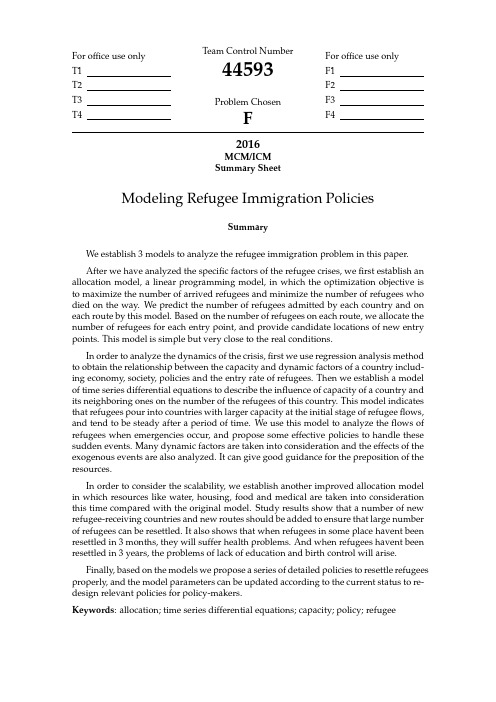
Improve Model 1 with consideration of dynamic capacity . . . . . . . . . . 5.2.1 5.2.2 Terminology and definitions . . . . . . . . . . . . . . . . . . . . . . Improvement of model . . . . . . . . . . . . . . . . . . . . . . . . . .
5.3 5.4 5.5
Deal with the influence of dynamic capacity . . . . . . . . . . . . . . . . . . The applicability for other countries . . . . . . . . . . . . . . . . . . . . . . Strengths and weaknesses of the model . . . . . . . . . . . . . . . . . . . .
5 Model 2: Dynamic model for refugee immigration 5.1 Dynamic factors . . . . . . . . . . . . . . . . . . . . . . . . . . . . . . . . . . 5.1.1 5.1.2 5.1.3 5.2 Dynamic factors that influence Total Capacity(t) . . . . . . . . . . . Dynamic factors that influence Accepted Refugees Number . . . . Further discussion . . . . . . . . . . . . . . . . . . . . . . . . . . . .
美国大学生数学建模大赛优秀论文一等奖摘要
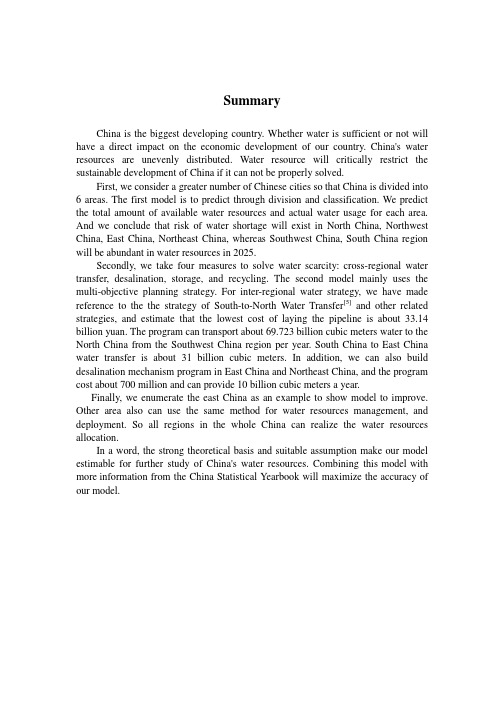
SummaryChina is the biggest developing country. Whether water is sufficient or not will have a direct impact on the economic development of our country. China's water resources are unevenly distributed. Water resource will critically restrict the sustainable development of China if it can not be properly solved.First, we consider a greater number of Chinese cities so that China is divided into 6 areas. The first model is to predict through division and classification. We predict the total amount of available water resources and actual water usage for each area. And we conclude that risk of water shortage will exist in North China, Northwest China, East China, Northeast China, whereas Southwest China, South China region will be abundant in water resources in 2025.Secondly, we take four measures to solve water scarcity: cross-regional water transfer, desalination, storage, and recycling. The second model mainly uses the multi-objective planning strategy. For inter-regional water strategy, we have made reference to the the strategy of South-to-North Water Transfer[5]and other related strategies, and estimate that the lowest cost of laying the pipeline is about 33.14 billion yuan. The program can transport about 69.723 billion cubic meters water to the North China from the Southwest China region per year. South China to East China water transfer is about 31 billion cubic meters. In addition, we can also build desalination mechanism program in East China and Northeast China, and the program cost about 700 million and can provide 10 billion cubic meters a year.Finally, we enumerate the east China as an example to show model to improve. Other area also can use the same method for water resources management, and deployment. So all regions in the whole China can realize the water resources allocation.In a word, the strong theoretical basis and suitable assumption make our model estimable for further study of China's water resources. Combining this model with more information from the China Statistical Yearbook will maximize the accuracy of our model.。
2016美赛E题,H奖渣渣
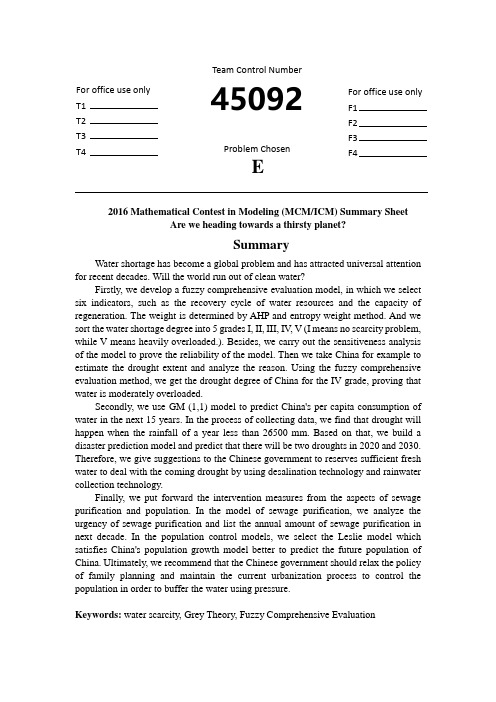
2016 Mathematical Contest in Modeling (MCM/ICM) Summary SheetAre we heading towards a thirsty planet?SummaryWater shortage has become a global problem and has attracted universal attention for recent decades. Will the world run out of clean water?Firstly, we develop a fuzzy comprehensive evaluation model, in which we select six indicators, such as the recovery cycle of water resources and the capacity of regeneration. The weight is determined by AHP and entropy weight method. And we sort the water shortage degree into 5 grades I, II, III, IV , V (I means no scarcity problem, while V means heavily overloaded.). Besides, we carry out the sensitiveness analysis of the model to prove the reliability of the model. Then we take China for example to estimate the drought extent and analyze the reason. Using the fuzzy comprehensive evaluation method, we get the drought degree of China for the IV grade, proving that water is moderately overloaded.Secondly, we use GM (1,1) model to predict China's per capita consumption of water in the next 15 years. In the process of collecting data, we find that drought will happen when the rainfall of a year less than 26500 mm. Based on that, we build a disaster prediction model and predict that there will be two droughts in 2020 and 2030. Therefore, we give suggestions to the Chinese government to reserves sufficient fresh water to deal with the coming drought by using desalination technology and rainwater collection technology.Finally, we put forward the intervention measures from the aspects of sewage purification and population. In the model of sewage purification, we analyze the urgency of sewage purification and list the annual amount of sewage purification in next decade. In the population control models, we select the Leslie model which satisfies China's population growth model better to predict the future population of China. Ultimately, we recommend that the Chinese government should relax the policy of family planning and maintain the current urbanization process to control the population in order to buffer the water using pressure.Keywords: water scarcity, Grey Theory, Fuzzy Comprehensive Evaluation45092Team Control NumberProblem ChosenEFor office use only T1 T2 T3 T4For office use only F1 F2 F3 F4Team #45092 Page 1 of 24 1Introduction1.1 Problem BackgroundWe cannot live without water. However, water problems are becoming increasingly serious in recent years, especially water scarcity. Water use has been growing at twice the rate of population over the last century. For the fact that there are many areas of severe water shortage on the earth, effective measures should be taken to prevent water resources from drying up.1.2 Our WorkFirstly, we are asked to build a model that provides a measure of the ability of a region to provide clean water to meet the demands of its population, of which the dynamic nature of the factors is taken into consideration. Secondly, we take China for example to explain why and how water is scarce. We then use our model to predict what the water situation will be in 15 years and analyze how this situation impact the lives of citizens in China, in which the environmental drivers’ effects on the model components is incorporated. Next, an intervention plan taking all the drivers of water scarcity into account is built. Finally, we use the intervention and model designed to project water availability into the future.2 Model One: Water demand and supply analysis2.1 IntroductionThe water supply ability is determined by many factors and differs from region to region. To describe the ability of a region to provide clean water to meet the demand of its residents,In view of the fuzziness and uncertainty of the indexes of water shortage risk evaluation, we build up a water resources comprehensive evaluation model based on entropy weight, with entropy theory applied into it.2.2 Fuzzy Synthetic Evaluation Model2.2.1 The analytic hierarchy processWe select five indicators[1,2] to evaluate the quality of water. According to paired comparisons, we can get a pairwise comparison matrix A, which is shown following table.Table 1. Pairwise comparison matrixRisk Ratio Vulnerability RecoverabilityRecurrencePeriodRiskDegreeRisk Ratio 1.0000 1.1445 0.9066 0.8461 1.1153 Vulnerability0.8738 1.0000 0.7921 0.7393 0.9745 Recoverability1.1338 1.2976 1.0000 0.9593 1.2645 RecurrencePeriod1.1484 1.3143 1.0411 1.0000 1.2808RiskDegree0.8966 1.0261 0.8129 0.7587 1.0000A=1.0000 1.14450.90660.8461 1.11530.8738 1.00000.79210.73930.97451.1338 1.2976 1.00000.9593 1.2645 1.1484 1.3143 1.0411 1.0000 1.2808 0.8966 1.02610.81290.7587 1.0000It is obviously that this matrix is a consistent matrix, so there is no need for consistency check. After that, we used the method as follows to calculate weight:a.normalize Each column vectorww�ii ii=aa ii ii∑aa ii ii nn ii=1 ;b.sum according to row for ww�ii iiww�ii=�ww�ii ii nn ii=1;c.normalize ww�iiww ii=ww�ii∑ww�ii nn ii=1 ,ww=(ww1,ww2,⋯,ww nn)TT is used to approximate the feature vector;d.calculate λ=1nn∑(AAAA)ii AA ii nn ii=1as the approximate value of maximum eigenvalue.The weight of each evaluation index schemed out is#(0.198,0.173,0.224,0.227,0.177)w=.2.3 Entropy Method2.3.1Modeling ProcessThe steps of comprehensive evaluation are to establish factor set U={uu 1,uu 2,⋯,uu nn } for evaluation, to establish evaluation set V ={VV 1,VV 2,⋯,VV nn } and set a fuzzy relationship matrix to represent the relationship between evaluation set and factor set. The matrix isR =�rr 11rr 12⋯rr 1mmrr 21⋯rr22⋯⋯⋯rr 2mm ⋯rr nn1rr nn2⋯rr nnmm �.The key of the fuzzy comprehensive evaluation model is to get a result set, where we can choose the optimal one as the final result. The result set isB =�bb ii �1xxmm =ww ∙RR ,where:1) W is the weight of each factors on the water shortage risk indicators ,W =(ww 1,ww 2,⋯,ww nn )and the elements satisfy�ww ii =1nnii=1;2) "∙" is the fuzzy synthetic operator, of which we choose the weighted average operator for comprehensive evaluation;3) B is the result sets of water shortage risk evaluation,bb ii =�ww ii rr ii ii (jj =1,2,⋯,mm )nnii=1,and the maximum of bb ii was selected as the final evaluation results.2.3.2 The determination of relative membership degreeThe value to assess the risk of water scarcity has no obvious boundaries, thus, we can use fuzzy set theory to describe the continuous changes of the indicators. According to the theory of fuzzy mathematics, the evaluation indexes can be divided into several levels. We sorted the evaluations into five levels, corresponding to the five standard values respectively, namely lower, low, medium, high and higher.The water shortage risk evaluation indexes can be described by the following function:rr ii ii (xx )=�1−mmmmxx {mm ii 1−xx ,xx−mm ii 2}mmmmxx {mm ii 1−mmii nn xx ,mmmmxx−mm ii 2}xx∉[aa ii 1,aa ii 2]1 xx ϵϵ [aa ii 1,aa ii 2]ii =1,2,⋯,nn ;jj =1,2,⋯,mm(3.1)Table 2. The evaluation index and classificationRisk level uu 1uu 2uu 3uu 4uu 5vv 1(ll ll wwllrr ) ≤0.200 ≤0.200 ≥0.800 ≥0.900 ≤0.200vv 2(ll ll ww )0.200~0.400 0.200~0.400 0.600~0.800 6.000~9.000 0.200~0.600vv 3(mmllmmii uu mm ) 0.400~0.600 0.400~0.600 0.400~0.600 3.000~6.000 0.200~0.600 vv 4(ℎii ii ℎ)0.600~0.800 0.600~0.800 0.200~0.400 1.000~3.000 1.000~1.200 vv 5(ℎii ii ℎllrr ) ≥0.800≥0.800≤0.200≤1.000≥2.0002.3.3 The entropy value method to determine the weight coefficientIn information theory, the weight of index is determined by the judgment matrix made up of evaluation index, instead of subjective factors, which makes the evaluation more approximate to physical truth. The process is demonstrated below:(1) build a judgement matrix of several evaluation objects, the number is m, and several judgment matrixes of evaluation indexes, the number is n: R =�rr ii ii �mm∙nn (ii =1,2,⋯,mm )(2) normalize the judgment matrix, then a normalized matrix B was got. The elements of B is defined by following equation:bb ii ii =rr ii ii −rr mm ii nnrr mm mmxx −rr mm ii nnwhere:rr mmmmxx is the optimal value of different objects under the same indicators; rr mm ii nn is the worst value of different objects under the same indicators; (3) The entropy of evaluation indexes can be written asHH ii =−1ln mm�ff ii ii mmii=1ll nn ff ii ii(3.2)where ,ff ii ii =bb ii ii∑bb ii iimm ii=1 ii =1,2,⋯,mm ;jj =1,2,⋯,mm0≤HH ii ≤1obviously, ln ff ii ii makes no sense when ff ii ii =0, thus, ff ii ii need to be redefine asff ii ii =1+bb ii ii∑�1+bb ii ii �mm ii=1(4) Calculate the entropy weight of each evaluation index by using the entropy;ww ∗=ww ii ∗=1−HH iinn −∑HH iinn ii−1(3.3)where, i =1,2,⋯,n , also, ∑ww ii ∗=1nn ii=1;It can be seen from equations above that the smaller the entropy value is, the bigger the entropy weight will be.(5) Analyze the comprehensive weights of evaluation indexes. The comprehensive weight of an evaluation index can be written as,ww ii =ww ii ∗ww ii #∑ww ii ∗ww ii #nn ii=1(3.4)where, ww ii # is weight determined by the AHP method.2.4 Model calculationFigures about the national water consumption we getting from China Statistical Yearbook in 2014[3] are listed in Table 3.Table 3. Figures on the national water consumptionYearWaterconsumptionAgriculture water consumption Industrial water consumption Domesticwater consumption20005497.6 3783.5 1139.1 574.9 20015567.4 3825.7 1141.8 599.9 20025497.3 3736.2 1142.4 618.7 20035320.4 3432.8 1177.2 630.9 20045547.8 3585.7 1228.9 651.2 20055633.0 3580.0 1285.2 675.1 20065795.0 3664.4 1343.8 693.8 20075818.7 3599.5 1403.0 710.4 20085910.0 3663.5 1397.1 729.3 20095965.2 3723.1 1390.9 748.2 20106022.0 3689.1 1447.3 765.8 20116107.2 3743.6 1461.8 789.9 20126141.8 3880.3 1423.9 728.8 20136183.4 3921.5 1406.4 750.1Then we can get another table .Table 4.Water shortage risk evaluation index value of some provinces in China . Province Risk RatioVulnerability RecoverabilityRecurrence PeriodRisk DegreeHebei 0.734 0.384 0.384 3.624 0.297 Shandong 0.984 0.403 0.000 3.104 0.457 Sichuan 0.615 0.295 0.451 3.721 0.235 Zhejiang 0.428 0.127 0.507 3.887 0.196 Xinjiang 0.892 0.315 0.106 3.419 0.425 Shanxi 0.402 0.113 0.619 4.079 0.127 Fujian 0.547 0.287 0.473 4.000 0.224 Jilin0.7820.2940.3383.5420.315After normalization, we can get a matrix F:F =⎣⎢⎢⎢⎢⎢⎢⎡0.534440.81030.62040.53220.48551.00001.00000.00000.00000.94830.36000.04470.84190.00000.24910.65290.62760.04830.69660.00000.60000.62410.72860.81910.17121.00000.76410.54600.63150.80140.32240.99800.68680.44830.31030.19830.85630.00000.27870.5042⎦⎥⎥⎥⎥⎥⎥⎤ After normalize the Equation (3.1), we can get the entropy of each evaluation index,H =(0.9870 0.9886 0.9899 0.9915 0.9896).Besides, according to Equation (3.2), we are able to figure out the weight of each evaluation index,ww ∗=(0.2428 0.2138 0.1896 0.1590 0.1949)The weights calculated by the AHP method are#(0.198,0.173,0.224,0.227,0.177)w =Finally, according to Equation (3.3), the combination weight vector is figured out as w =(0.181 0.183 0.233 0.237 0.165 )Based on the data of Table 3 and Founction (3.1), we can schemed out the relative membership degree and estabilish the fuzzy relation matrix R,R=0.34570.46401.00000.70570.78140.2708 1.00000.48250.81880.34120.38400.4088 1.00000.64000.37960.00000.08820.1478 1.00000.11150.62261.00000.19470.35940.0908,and the final comprehensive evaluation results are:B=(0.317 0.587 0.562 0.713 0.335 ).For the reason that the maximum is 0.731, so the degree of drought in China is grade IV, namely overload. For limits of economic scarcity, China is still moderately loaded though has abundant water resources,2.5 Sensitivity analysisThe fuzzy membership matrix is related to people's subjectivity for the fact that it is voted by experts. Therefore, sensitivity analysis should be taken with the fuzzy membership matrix.Based on data we have got, we can draw a table as shown below:After calculation, we were able to get a weight vectorB=(0.317 0.587 0.562 0.713 0.335 ).As can be seen from the result that, water shortage degree in China belongs to the fourth grade, namely overloaded.Assume that analysis of the risk ratio is far from sufficient for some reason, if the risk ratio of sensitivity matrix R reduced by 10%, the new weight vector will beB=(0.340 0.601 0.605 0.690 0.327).There is no obvious change in value of the matrix B and China is still in the high degree. Then we analyze the sensitivity of the other four indicators in the same way, therefore, we can come to the conclusion that some small fluctuations in a single index will not produce significant effect on the results of fuzzy comprehensive evaluation, suggesting the validity of our model.3 Model Two: Forecasting3.1 Assumptions(1) Assume that China’s population and related policies have not changed dramatically.(2) Assume that China’s economy is growing steadily.3.2 DGM(2,1) Model[4]Defining XX0as the original data sequence of water consumption per capita:xx(0)=(xx(0)(1),xx(0)(2),⋯,xx(0)(nn))where:xx(1)is 1-AGO data sequence;αα(1)xx(0)is 1-IAGO data sequence.Then we define αα(1)xx(0)(kk)+aaxx(0)(kk)=bb as DGM(2,1) model and dd2xx(1)dddd+addxx (1)dddd=b is the albinism differential equation of DGM(2,1) model.The least squares criterion of the parameters in the DGM(2,1) model satisfyuu �=�aa � ,bb��TT =(BB TT BB )−1BB TT YY . The solution to the albinism differential equationdd 2xx (1)dddd +addxx (1)dddd=b isxx �(1)(tt )=�bb �aa �2−xx (0)(1)aa ��ll −mm�dd +bb �aa �tt +1+aa �aa �xx (0)(1)−bb �aa �2 .And the reduced value is xx �(0)(kk +1)=αα(1)xx �(1)(kk +1)=xx �(1)(kk +1)−xx �(1)(kk ) .Table 5. Annual water consumption per capita in China Year 2000 2001 2002 2003 2004 2005 2006 Waterconsumption435.4 437.7 429.3 412.9 428.0 432.1 442.0 Year 2007 2008 2009 2010 2011 2012 2013 Water consumption 441.5446.2448.0450.2454.4454.7455.5Based on figures in the table, the original data sequence can be written as^(0)(443.4,437.5,437.8,438.2,438.6,439.2,439.9,440.9,442,443.5,445.4,447.8,450.9,454.8)x=The respective time response sequence of DGM model is^(1)0.238146k(1)436.469*k+3.90908*+439.491k xe+=therefore,^(1)(443.4,880.9,1318.7,1756.9,2195.5,2634.7,3074.6,3515.5,3957.5,4401,4846.5,5294.3,5745.2,6200)x=And the reduced can be obtained by repeated decreasing:^^^(0)(1)(1)()()(1)k k k xxx =−−By applying the figures into equation above, we can get annual water consumption per capita:^(0)(443.4,437.5,437.8,438.2,438.6,439.2,439.9,440.9,442,443.5,445.4,447.8,450.9,454.8)x=Graph 1. Predicted water consumption per capital by DGM(2,1).3.3 GM(1,1) ModelThe reference sequence has been known asxx (0)=�xx (0)(1),xx (0)(2),⋯,xx (0)(nn )� .Besides, 1-AGO is a once accumulation generation sequence, in which zz (1)=0.5xx (1)(kk )+0.5xx (1)(kk −1),kk =2,3,⋯,nn .So the estimated value can be gotddxx (1)dddd+a xx (1)(t)=b . Let Y =�xx (0)(2),xx (0)(3),⋯,xx (0)(nn )�TT , B =⎣⎢⎢⎡−zz (1)(2)1−zz (1)(3)⋮1⋮−zz (1)(nn )1⎦⎥⎥⎤,we can get an estimated value of u to obtain the minimum of J(u) through the least square method, J (u )=(Y −Bu )TT (YY −BBuu ). At last, the estimated value of u is uu �=�aa �,bb��TT =(BB TT BB )−1BB TT YY . Then solve the albinism differential equation ddxx (1)dddd+a xx(1)(t)=b , the result is xx �(1)(kk +1)=�xx(0)(1)−bb�mm ��ll −mm�dd +bb�mm �tt ,kk =0,1,⋯,nn −1,⋯. And we can get the reducedxx �(0) by repeated decreasing:^^^(0)(1)(1)(1)(1)()1,2,,1,k k k k n xxx +=+−=− ,.Time sequence is as followsxx (0)=�xx (0)(1),xx (0)(2),⋯,xx (0)(7)�=(435.4,437.7,429.3,412.9,428.0,432.1,442.0,441.5,446.2,448.0,450.2,454.4,454.7,455.5).By applying data into the albinism differential equation,xx�(1)(kk+1)=�xx(0)(1)−bb�aa��ll−mm�dd+bb�aa�tt=67459.3×ll0.00627374×kk−67023.9 Then, we can get annual water consumption per capita:xx(0)=�xx(0)(1),xx(0)(2),⋯,xx(0)(7)�=(435.4,424.5,427.2,429.9,432.6,435.3,438.0,440.8,443.6,449.2,452.0,Graph 2. Water consumption per capita predicted by GM(1,1)It can be seen from the graph 1 and graph 2 that the data predicted by DGM(2,1) agree with the actual data well at the beginning, but the growth trend changes too dramatic and it is far from actual situation. While, the GM(1,1) model fit the actual situation well.Then we analyze the Residual Error, Relative Error of GM(1,1) prediction, and the following table shows the results.Table 6. Analysis outcomeyearActualconsumptionper capitalPredictedConsumptionper capitalResidualErrorrelativeError2000 435.4027 435.4000 0.0000 0.000%2001 437.7427 424.5525 -13.1902 3.013%2002 429.3408 427.2244 -2.1164 0.493%2003 412.9463 429.9131 16.9668 4.109%2004 428.0000 432.6187 4.61874 1.079%2005 432.0698 435.3414 3.2716 0.757%2006 442.0199 438.0812 -3.9386 0.891%2007 441.5157 440.8383 -0.6774 0.153%2008 446.1501 443.6127 -2.5374 0.569%2009 448.0430 446.4045 -1.6384 0.366%2010 450.1736 449.214 -0.9596 0.213%2011 454.4000 452.0411 -2.3589 0.519%2012 454.7142 454.8860 0.1717 0.038%2013 455.5430 457.7488 2.2057 0.484%As we can see from table 6, we can prove the consistency between GM(1,1) prediction and the actual water consumption per capital.Table 7. Water consumption per capital predictionyear 2015 2016 2017 2018 2019 2020 2021 2022water consumptionper capital463.5 466.4 469.3 472.3 475.3 478.2 481.3 484.3 year 2023 2024 2025 2026 2027 2028 2029 2030 water consumptionper capital487.3 490.4 493.5 496.6 499.7 502.9 506.0 509.23.4 Disaster Prediction modelIn order to predict the time of the next drought, we have gathered a lot of information about annual rainfall in each province in China from 2003 to 2013. The national annual rainfall can be revealed by the sum of annual rainfall of each province. (unit: mm)Table 8. Annual rainfallYear 2003 2004 2005 2006 2007 2008Annual27345.3 28149.8 24421.9 29706.9 27445.2 30162.5 rainfallYear 2009 2010 2011 2012 2013Annual28613 24395.8 30345.4 28981 26443.4rainfallAccording to the situation in China, we found that drought will happen when the rainfall of a year less than 26500 mm. In order to forecast the drought years,Rainfall sequence is written as follows:xx(0)=(27345,28149,24421,29706,27445,30162,28613,24395,30345,28981,26443).If annual rainfall satisfies xx(0)(ii)<26500, we can regard the year as a drought year. With 2003 marked as the first year, it is not difficult to get the dry year sequencet=(3,8,11).we developed a GM(1,1) model to predict the drought years. The result is showed in the following table:Table 9. The result of disaster prediction model3.0003 7.921962 10.86366 14.89778 20.42994 28.01641From the figures in Table 8, we are easy to draw a conclution that the next two droughts will take place in 20 years and 28 years later after 2003. In other words, the next two troughts will occur in 2022 and 2030 in the next 15 years. While, if annual rainfall is over than 29000mm, we will say that this year's rainfall is abundant.After taking all these into account, we suggest the Chinese government to collect rainwater in 2021 by the rainwater collecting technology. Then desalination technology should be adopted to get enough fresh water to provent China from the drought in 2030.The results show that the per capita water consumption will increase and drought year and flood year will occur frequently, which will make some consquences.4. Model Three: Intervention Plan4.1 Pollution intervention plan4.1.1 IntroductionThe Yangtze river is the longest river in China and third longest in the world, which has been seriously polluted. After consideration, we take the Yangtze river for example.4.1.2 One-dimensional water quality mathematical modelThe differential equation of one dimensional steady decline rule was used to describe the concentration of river pollution. The equation is shown as follows:dc u Kc dx =− in which the discrete function is ignored., after integration, /0Kx uC C e−= .where:u is the average flow velocity distribution in the cross section of the river ( the unit is meters per second );x is the distance along the river ( the unit is km );K is the comprehensive degradation coefficient ( the unit is 1/d ); C is the pollutants concentration along the river ( the unit is mg/L ); cc 0is the concentration of the pollutants after a node (the unit is mg/L ).4.1.3: The river diluted mixture model under the condition of constant designIn terms of point source, the dilution equation of the mixture of water and wastewater isPEPEPEC Q Q C C Q Q+=+ ,where:C is the concentration of completely mixed water ( the unit is mg/L );QQ pp ,CC pp are the water designed from upstream and water quality concentration designed respectively. ( The units are cubic meters per second and milligrams per liter.)QQ EE ,CC EE are sewage flow designed and wastewater discharge concentration designed respectively. ( The units are cubic meters per second and milligrams per liter.) For the fact that the pollution source function fit linear superposition well, we set Single point source discharge formula as;where:ww cc is water load ( the unit is g/L );S is water quality standards of the control section ( the unit is mg/L ). While multipoint sources discharge formula is shown as follows:where:pp E p C C Q Q Q S W ⋅−+⋅=)(pp Ei ni p C C Q Q Q S W ⋅−+⋅=∑=)(1QQ EEii is the sewage flow designed from sewage outlet i; n is the number of sewage outlet.4.1.4 Water environment capacity(1) overview of the river(2) Environmental capacity calculationBy applying into the model, we can get a formula of one-dimensional water environmental capacity:where:ww ii is the emissions from sewage outlet which is number i. ( t/a ) cc ii is background concentration around node i in the river. ( mg/L ) C is the concentration along the river. ( mg/L ) QQ ii is flow after the node. (Cubic meters per second)QQ ii is the quantity of waste water into the river around node i. (Cubic meters per second)u is flow velocity of river i. (meters per second)x is the distance between calculated point and node i.The water environment capacity value of environment function is.According to the analysis above, measures should be taken to prevent the Yangtze river from being polluted more seriously.4.1.5 Water category proportion in the total basin analysisji i Q Q Wi C C +=54.31/+)(*)*(*54.31*4.86j i i uKx Q Q C eC Wi +−=∑=ni WiWGraph 3. Variation of water quality in the Yangtze River BasinIf no effective measures is taken, water in level I would tend to scarcity. While, water in level IV would fluctuate to 14%. At the same time water in level V would get further increased. Therefore the treatment of Yangtze river is of great urgency.4.1.6 Analysis of the changes of total sewage[5]Table 8. The changes of total sewageYear 2004 2005 2006 2007 2008Total discharge of174 179 183 189 207 waste water(Onehundred million tons)Year 2009 2010 2011 2012 2013 Total discharge of234 220.5 256 270 285 waste water(Onehundred million tons)The simulation is accomplished by the least square method,QQ FF=167.375+3.68×tt+0.835×tt2in which, t is increase in the number of years.Wastewater emissions in the next 10 years are listed in the follow table:Year 2004 2005 2006 2007 2008Total discharge of308.9 331.8 356.3 382.5 410.4 waste water(Onehundred million tons)Year 2009 2010 2011 2012 2013 Total discharge of440.0 471.3 504.2 538.7 575.0 waste water(Onehundred million tons)By far, we have gathered all the information that we need to predict the quantity of sewage should be treated every year, with the proportion of water belonging to level IV and V stay below 20%.We simulate the percentage of water of IV, V and VI respectively, and get three prediction model.II VV FF=4.3−2.18×tt+0.06×tt2+10.5×ln ttVV FF=2.15+0.23×tt+0.001×tt2−0.3×ln ttVVII FF=2.3+0.5×tt−0.005×tt2−1.14×ln ttin which, t is the year.The final predicted results are listed in the following table:Year 2004 2005 2006 2007 2008308.9 331.8 356.3 381.5 410.4 Total discharge of wastewater(One hundredmillion tons)Year 2009 2010 2011 2012 2013 Total discharge of waste440.0 471.3 504.2 538.7 575.0 water(One hundredmillion tons)The extra sewage need to treat every year is showed in the following table:Year2004 2005 2006 2007 2008Extra sewage need to treat(One hundred million tons)24 47 71 98 125Year2009 2010 2011 2012 2013Extra sewage need to treat(One hundred million tons) 155 186 219 254 1954.2 Population intervention plan4.2.1 Logistic modelAssuming that the population in a particular time t is x(t) and environment capacities of population is xx mm , the net population growth rate can be written as)1.()(mx xr t r −=Establish the differential equation of the block type population:0)0(,).1(x x x x xr dt dx m =−= Therefore,t r mme x x x t x .0.11)(−−+=,which is regarded as Logistic model.The result is obtained by simulating the data,e ty .0342.05656.212939.18−+=. The root-mean-square-error 1346.0=RMSE .4.2.2 Leslie modelThe outcome we get by estimating the parameters in the Leslie model are shown as follows:1212001000(1)0100()10n n X t X t λλλµµµ −+=−−Graph 4. The comparison of Logistic and Leslie model.As showed in graph 4, the Leslie model match China's population growth model well, so we select the Leslie model to predict the future population of China. Prediction of population growth in ChinaThe long term forecast of population growth must take the impact of policy into consideration. Policies that can be taken containing Family Planning and Urbanization.Next we analyze the impact on population of five policies. Policy one: keep the existing policy not change;Policy two: Strengthen the work of family planning and limit the process of Urbanization;Policy three: unwind the work of family planning and limit the process of Urbanization;Policy four: unwind the work of family planning and keep the process of Urbanization;Policy five: unwind the work of family planning and limit the process of Urbanization strictly.In this paper, we carry on predict and estimate aiming at the five policies, in order to provide useful information to the government of China.。
2016年美赛模拟
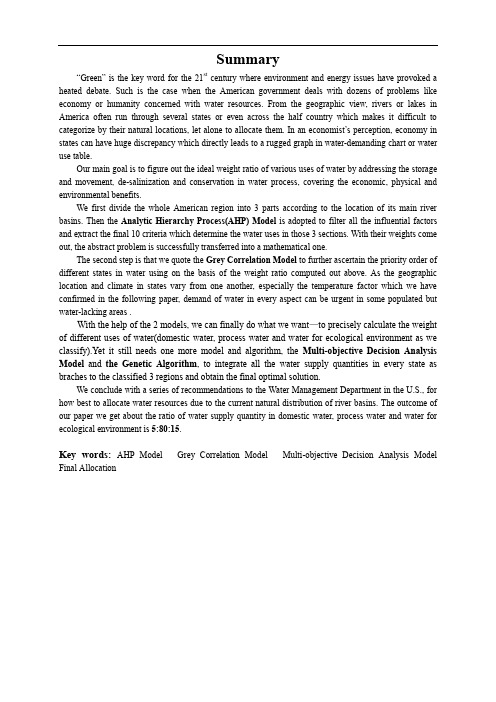
“Green” is the key word for the 21st century where environment and energy issues have provoked a heated debate. Such is the case when the American government deals with dozens of problems like economy or humanity concerned with water resources. From the geographic view, rivers or lakes in America often run through several states or even across the half country which makes it difficult to categorize by their natural locations, let alone to allocate them. In an economist‟s perception, economy in states can have huge discrepancy which directly leads to a rugged graph in water-demanding chart or water use table. Our main goal is to figure out the ideal weight ratio of various uses of water by addressing the storage and movement, de-salinization and conservation in water process, covering the economic, physical and environmental benefits. We first divide the whole American region into 3 parts according to the location of its main river basins. Then the Analytic Hierarchy Process(AHP) Model is adopted to filter all the influential factors and extract the final 10 criteria which determine the water uses in those 3 sections. With their weights come out, the abstract problem is successfully transferred into a mathematical one. The second step is that we quote the Grey Correlation Model to further ascertain the priority order of different states in water using on the basis of the weight ratio computed out above. As the geographic location and climate in states vary from one another, especially the temperature factor which we have confirmed in the following paper, demand of water in every aspect can be urgent in some populated but water-lacking areas .
2016美国大学生数学建模竞赛 F题
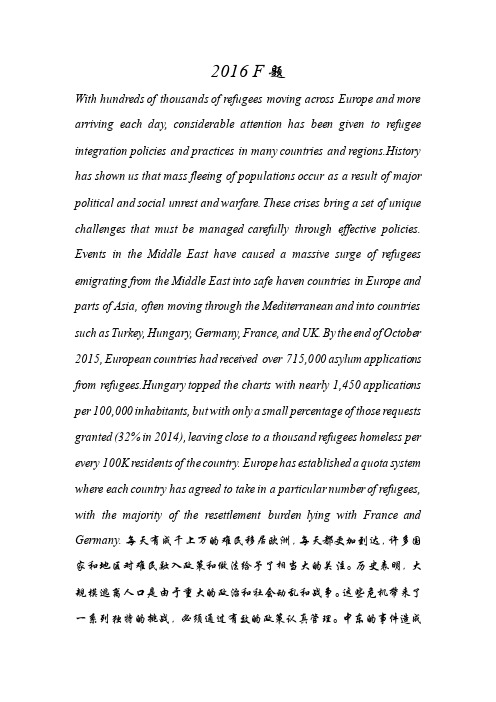
2016 F题With hundreds of thousands of refugees moving across Europe and more arriving each day, considerable attention has been given to refugee integration policies and practices in many countries and regions.History has shown us that mass fleeing of populations occur as a result of major political and social unrest and warfare. These crises bring a set of unique challenges that must be managed carefully through effective policies. Events in the Middle East have caused a massive surge of refugees emigrating from the Middle East into safe haven countries in Europe and parts of Asia, often moving through the Mediterranean and into countries such as Turkey, Hungary, Germany, France, and UK. By the end of October 2015, European countries had received over 715,000 asylum applications from refugees.Hungary topped the charts with nearly 1,450 applications per 100,000 inhabitants, but with only a small percentage of those requests granted (32% in 2014), leaving close to a thousand refugees homeless per every 100K residents of the country. Europe has established a quota system where each country has agreed to take in a particular number of refugees, with the majority of the resettlement burden lying with France and Germany.每天有成千上万的难民移居欧洲,每天都更加到达,许多国家和地区对难民融入政策和做法给予了相当大的关注。
2016美赛数学建模A题题目

2016 MCMProblem AA Hot Bathand relax.Unfortunately, the bathtub is not a spa-style tub with a secondary heating system and circulating jets, but rather a simple water containment vessel. After a while, the bath gets noticeably cooler, so the person adds a constant trickle of hot water from the faucet to reheat the bathing water. The bathtub is designed in such a way that when the tub reaches its capacity, excess water escapes through an overflow drain.Develop a model of the temperature of the bathtub water in space and time to determine the best strategy the person in the bathtub can adopt to keep the temperature even throughout the bathtub and as close as possible to the initial temperature without wasting too much water.Use your model to determine the extent to which your strategy depends upon the shape and volume of the tub, the shape/volume/temperature of the person in the bathtub, and the motions made by the person in the bathtub. If the person used a bubble bath additive while initially filling the bathtub to assist in cleansing, how would this affect your model’s results?In addition to the required one-page summary for your MCM submission, your report must include a one-page non-technical explanation for users of the bathtub that describes your strategy while explaining why it is so difficult to get an evenly maintained temperature throughout the bath water.。
美赛金奖论文
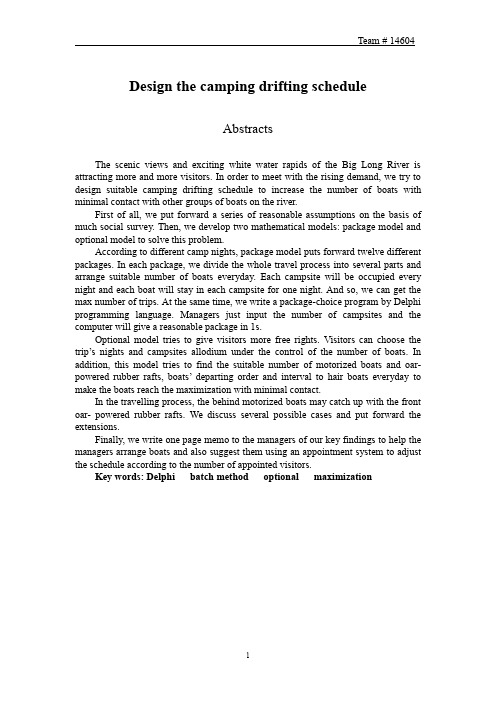
1
Team # 14604
Catalogue
Abstracts ........................................................................................................................................... 1 Contents ............................................................................................................................................ 3 1. Introduction ................................................................................................................................... 3 1.1 Restatement of the Problem ................................................................................................ 3 1.2 Survey of the Previous Research......................................................................................... 3 2. Assumptions .................................................................................................................................. 4 3. Parameters ..................................................................................................................................... 4 4. Model A ----------Package model .................................................................................................. 6 4.1 Motivation ........................................................................................................................... 6 4.2 Development ....................................................................................................................... 6 4.2.1 Module 1: Introduce of model A .............................................................................. 6 4.2.2 Module 2: Solution of model A .............................................................................. 10 4.3 Conclusion ........................................................................................................................ 11 5. Model B----------Optional model ................................................................................................ 12 5.1 Motivation ......................................................................................................................... 12 5.2 Development ..................................................................................................................... 12 5.2.1 Module B: Choose oar- powered rubber rafts or motorized boats either ............... 12 5.2.2 Module 2: Choose mix of oar- powered rubber rafts and motorized boats ............ 14 5.3 Initial arrangement ............................................................................................................ 17 5.4. Deepened model B ........................................................................................................... 18 5.4.1 Choose the campsites allodium .............................................................................. 18 5.4.2 Choose the oar- powered rubber rafts or motorized boats allodium ...................... 19 5.5 An example of reasonable arrangement ............................................................................ 19 5.6 The strengths and weakness .............................................................................................. 20 6. Extensions ................................................................................................................................... 21 7. Memo .......................................................................................................................................... 25 8. References ................................................................................................................................... 26 9. Appendices .................................................................................................................................. 27 9.1 Appendix I .................................................................................................. 27 9.2 Appendix II ....................................................................................................................... 29
我校学生在美国大学生数学建模竞赛中获佳绩

我校学生在美国大学生数学建模竞赛中获佳绩
佚名
【期刊名称】《长江大学学报(自然版)理工卷》
【年(卷),期】2016(013)013
【摘要】近日,从美国大学生数学建模大赛官网获悉:我校2016年在竞赛中取得
了1个一等奖、1个二等奖的好成绩。
美国大学生数学建模竞赛(MCM/ICM)是一项国际级的竞赛项目,为现今各类数学建模竞赛之鼻祖。
MCM始于1985
年,ICM始于2000年,由美国数学及其应用联合会COMAP主办,得到SIAM、NSA、INFORMS等多个组织的赞助。
MCM/ICM着重强调研究问题、解决方案的原创性、团队合作、交流以及结果的合理性。
竞赛以3人(本科生)为一组,在4天时
间内,
【总页数】1页(P15-15)
【正文语种】中文
【相关文献】
1.我校学生在2007年全国大学生数学建模竞赛中获佳绩 [J],
2.我校学生在2016年全国大学生数学建模竞赛陕西赛区中荣获佳绩 [J],
3.我校学生在2008年全国大学生数学建模竞赛中再获佳绩 [J],
4.我校学生在全国大学生数学建模竞赛中获佳绩 [J],
5.我校参加竞赛所获奖励我校学生在数学建模竞赛中再获佳绩 [J],
因版权原因,仅展示原文概要,查看原文内容请购买。
- 1、下载文档前请自行甄别文档内容的完整性,平台不提供额外的编辑、内容补充、找答案等附加服务。
- 2、"仅部分预览"的文档,不可在线预览部分如存在完整性等问题,可反馈申请退款(可完整预览的文档不适用该条件!)。
- 3、如文档侵犯您的权益,请联系客服反馈,我们会尽快为您处理(人工客服工作时间:9:00-18:30)。
For office use onlyT1________________ T2________________ T3________________ T4________________ Team Control Number54164Problem ChosenAFor office use onlyF1________________F2________________F3________________F4________________ 2016MCM/ICMSummary Sheet(Your team's summary should be included as the first page of your electronic submission.)Type a summary of your results on this page. Do not include the name of your school, advisor, or team members on this page.AbstractWe present a user-friendly strategy for keeping the water in a bathtub as close aspossible to a certain preferred temperature. This strategy consists in two mainconstituents: adjusting the hot water flow from the faucet to keep the bathtubtemperature suitably high, and using hand motions to distribute the heat evenlythroughout the bath water.As for determining the necessary water flow, we first create a model that determinesthe temperature of the bath water at any point in time. We posit that the temperature ofthe water in the bath is dependent on the cooling due to the ambient temperature of theroom, which obeys the proportionality given by Newton’s Law of Cooling; and theheating due to the added hot water from the faucet. Our model combines thetemperature changes over time from these two entities into one consummatedifferential equation.We determine the experimental constant of proportionality for Newton’s Law of Coolingby proposing that this constant itself is dependent on a combination of volume of thewater and surface area exposed to the air. Taking experimental data from variedsources, including an experiment we conducted and documented over the time frame ofthe MCM competition, we were able to extrapolate values for the constant attributableto any bathtub given its volume and surface area exposed to air.We then run a computer simulation to model the temperature changes over time in astandard bathtub with our differential equation, determining how much hot waterneeds to flow in to keep the total deviance over time of the temperature of the bathfrom a desired temperature at a minimum. We found the optimal flow rate for astandard bathtub at standard conditions to be 1.81 gallons/minute, or about 11% of themaximum flow rate.Our model was able to predict optimal flow rates for many different combinations ofvolume, surface area, tub material, and additives. We found that more effectiveinsulating materials led to lower optimal flow rates (less hot water needed), as did theaddition of additives. Volume and surface area, surprisingly, showed no clear trend.As for keeping the temperature roughly even in the bathtub, we suggest that the userswirl his hands counter-clockwise with respect to the faucet as shown in Figure 1. Thisis a simple yet effective way to distribute the heat acceptably evenly throughout the tubwater.As alternatives for those who do not wish to make their bath into an exercise session,we invented an original product (Home Jacuzzi) that is low-cost and aids in heating thewater evenly.The Math Behind the Bath:A”Cool”Investigation onCoolingTeam#54164February20161AbstractWe present a user-friendly strategy for keeping the water in a bathtub as close as possible to a certain preferred temperature.This strategy consists in two main constituents:adjusting the hot waterflow from the faucet to keep the bathtub temperature suitably high,and using hand motions to distribute the heat evenly throughout the bath water.As for determining the necessary waterflow,wefirst create a model that determines the tem-perature of the bath water at any point in time.We posit that the temperature of the water in the bath is dependent on the cooling due to the ambient temperature of the room,which obeys the proportionality given by Newton’s Law of Cooling;and the heating due to the added hot water from the faucet.Our model combines the temperature changes over time from these two entities into one consummate differential equation.We determine the experimental constant of proportionality for Newton’s Law of Cooling by proposing that this constant itself is dependent on a combination of volume of the water and surface area exposed to the air.Taking experimental data from varied sources,including an experiment we conducted and documented over the time frame of the MCM competition,we were able to extrapolate values for the constant attributable to any bathtub given its volume and surface area exposed to air.We then run a computer simulation to model the temperature changes over time in a standard bathtub with our differential equation,determining how much hot water needs toflow in to keep the total deviance over time of the temperature of the bath from a desired temperature at a minimum.We found the optimalflow rate for a standard bathtub at standard conditions to be1.81 gallons/minute,or about11%of the maximumflow rate.Our model was able to predict optimalflow rates for many different combinations of volume, surface area,tub material,and additives.We found that more effective insulating materials led to lower optimalflow rates(less hot water needed),as did the addition of additives.Volume and surface area,surprisingly,showed no clear trend.As for keeping the temperature roughly even in the bathtub,we suggest that the user swirl his hands counter-clockwise with respect to the faucet as shown in Figure1.This is a simple yet effective way to distribute the heat acceptably evenly throughout the tub water.As alternatives for those who do not wish to make their bath into an exercise session,we invented an original product(Home Jacuzzi)that is low-cost and aids in heating the water evenly.1.1Non-Technical ExplanationWhy is it so difficult to keep the temperature of the water even throughout when taking a bath?In a standard single-faucet bathtub,hot water comes out of the faucet on only one side of the bath, leaving the other side of the bath colder until enough time passes for the temperature to even out. When left running,this might take more time than a user wants to wait for.To further complicate things,hot water is also slightly less dense than cold water,and will thus be more concentrated on the surface of the bathtub than the bottom of the tub.To make the temperature consistent,the easiest solution is to cup your hands and circulate the hot water from the side with the faucet to the other side of the tub,and also circulate the hot water from the water surface to the bottom of the top.Another option,however,for those of us who don’t want to spend the duration of our bath flailing our arms,is to use our invention,”Home Jacuzzi.”Our invention spans the length andPage1width of the bathtub,and attaches onto the faucet.The hot water from the faucet is then funneled through the device towards the bottom of the tub,creating a Jacuzzi-like effect.The hot water then rises to the surface,mixing with the water above it and creating an evenly distributed,uniform temperature throughout the tub.Now that we can keep the temperature consistent throughout the tub,we’re still left tofigure out how high we should turn our faucet to keep the water from going cold.Of course,turning it too high is a waste of water and,on top of that,can increase the temperature to uncomfortably high levels.Too low,and we get to wade around in lukewarm to cold waters that make us not want to take a bath at all.So we want tofind the optimalflow rate on our faucet that gets our temperature to where we want it to be.Not all bathtubs were created equal.The optimalflow rate isn’t a set rate for all bathtubs.For the standard60”x30”x14”bathtub,we suggest turning the faucet up to about a ninth of its max.To conserve even more hot water,you even have the option of purchasing products like the ”Water Blanket”(”Water Blanket”)to keep the temperature of the bath warmer.Interestingly enough,even bubble bath can keep your bath water hotter for longer.And no,it’s not”just for kids.”Contents1Abstract11.1Non-Technical Explanation (1)2Introduction32.1Background (3)2.2Restatement of Problem (3)3The Strategy43.1Distribution:A Simple Solution (4)3.2Global Assumptions (4)4Simulating Heat Transfer54.1Local Assumptions (5)4.1.1Variable Nomenclature (6)4.2Creation of the Model (6)4.2.1Cooling (6)4.2.2Experimental Data (7)4.2.3Heating (12)4.3Simulation Design (13)4.3.1Selecting Optimal Flow Rate (14)4.3.2Validation of Model (16)5Sensitivity Analysis:Varying Bathtub Characteristics165.1Varying Surface Area Under Constant Volume (16)5.2Varying Volume Under Constant Surface Area (24)5.3Observations (32)Page25.4Material of Bathtub (34)5.5Additives(bubble bath) (35)6Conclusion366.1Results (36)6.2Strengths of Model (36)6.3Limitations of Model (36)6.4Future Work/Discussion (37)6.5Home Jacuzzi (37)Appendices39A Experimental Design for Real-World Bathtub Data39B Simulation Code39 2Introduction2.1BackgroundBaths,the best way we had to cleanse ourselves–until we became teens and discovered showers,at which point baths became”uncool.”Yet,whenever we want to slow life down and relax a little, we can rest assured that the bath will always be there to ease our nerves.The health benefits of taking a bath are plentiful(”5Reasons You Need To Take A Bath”),but no one wants to hop into a steaming hot tub only tofind him or herself in cold or lukewarm water a half-hour later.Then, how can one keep a bath warm long enough to last a preferred duration?Most people own simple bathtubs,unequipped with secondary heating systems or circulating jets.Several factors can affect the temperature change of bath water,including:•the surface area of water exposed to air•the volume of water that is being cooled•the surrounding air temperature•the rate of hot waterflow into the bathtub•the material the bathtub is made out of(and how well it is able to provide insulation)•whether any additives are introduced to the water,such as a bubble bath solution2.2Restatement of ProblemA person has settled into a bathtub for a long,relaxing bath,only tofind that after some time,the water has become noticeably cooler.In an attempt to salvage his satisfaction,he elects to turn on the faucet and let in some hot water to bring the temperature back up.Excess tub water leaves the tub through an overflow drain.Of course,he runs into a set of issues:Page3•The water in the tub is of inconsistent temperature,being hotter in some areas and cooler in others.•Turning the hot waterflow too high is a waste of water(and money).3The StrategyOur”strategy”presented to the person in the tub will be a combination of the solutions to queries 1and2—the person will have to take action to evenly distribute the heat in the bathtub water, and the person will have to set the hot waterflow in at a certainflow rate to keep the bathtub water hot.3.1Distribution:A Simple SolutionWe begin by addressing query number”1”.In a standard single-faucet bathtub,waterflows from above on one side of the bathtub.Since hot water rises,more heat will be concentrated at the top of the bathtub.Because the faucet is only on one side of the tub,more heat will be concentrated on the side of the tub closest to the faucet.Therefore,we advise the person in the tub to cup his hands and make circles in the water as illustrated below:Figure1:Proposed strategy for equalizing the temperature of the water in any single-faucet bathtub.3.2Global Assumptions•The standard bathtub is open and exposed to the air on the top surface,and built with acrylic material that is heavily insulated(”Bathtub Sizes”).•Heat loss through heavily insulated surfaces is negligible.•Heat loss through surfaces of poor insulation(thin glass,thinfiberglass)behaves as if that surface was directly exposed to air.•The person in the bathtub follows the suggestion in Figure1,allowing us to assume that water temperature is consistent throughout the tub at any point in time.Page4•The temperature sensitivity of the human body is such that we begin noticing appreciable differences in temperature at±1.4◦C from the original temperature(Davies et al.171)•The target temperature for the bath water is human body temperature,which is approxi-mately37◦C.•Since the person inside the tub is at thermal equilibrium with the water in the tub,we can treat the person’s body as an extension of the bathtub water.•Room temperature remains constant throughout the duration of the bath,at21◦C,the ac-cepted value for ambient room temperature.•The faucet from which the hot waterflows has a maximumflow rate of16gallons/min (”Bathtub Faucets”),and the person is able to toggle the faucet’sflow rate to any rate between0gallons/min and16gallons/min.•The hottest water we can release from the faucet has a temperature of48◦C to prevent injury from scalding(A.O.Smith Corporation1).This will be the assumed temperature of the additional water from the faucet.•All bathtubs used in this paper for simulation and data arefilled to the brim with the com-bination of water and person.4Simulating Heat TransferTo address our second query,we consider a thermodynamic system offive constituents:the water in the tub,the water entering and leaving the tub,the walls of the tub,the human in the tub,and the air outside the tub.Heat transfer with the human and the walls is negligible as justified in our global assumptions.Thus,we need only consider the cooling of the tub water due to the air outside,and the heating of the tub water due to the incoming hot water.4.1Local Assumptions•The industry standard bathtub in America is60”×30”×14”,which results in a volume of 60×30×14=25,200in3and surface area exposed to the air of60×30=1,800in2(”Bathtub Sizes”).•Since the majority of bathtubs in America are insulated,we assume that the bathtub that we are simulating is well insulated on all sides except for the top.Therefore,heat can only escape from the bathtub from the top surface.•Water cooling obeys Newton’s Law of Cooling closely enough to approximate a model for temperature over time.•The ambient temperature is21◦C.•The temperature of the hot water from the faucet is48◦C.Page54.1.1Variable NomenclatureBelow are the variables used throughout our model:•k=cooling constant•T=temperature of the water minus ambient room temperature•T0=initial temperature of the bathtub•t=time in minutes•SA E=surface area exposed to air in square meters•V=volume in liters•Q=change in heat energy,in joules•m=mass of the water in the tub,in grams•C p=specific heat of the water,in Joules per gram-degree◦C4.2Creation of the ModelSince the temperature of the water is constantly being altered by the cooling due to the surrounding air temperature and the heating due to the incoming waterflow,we created an equation to model the changes in temperature,based on Newton’s Law of Cooling and the specific heat capacity formula.4.2.1CoolingThefirst part of the model accounts for the cooling of the water due to the temperature of the environment.We chose to model this cooling with Newton’s Law of Cooling,which states that the difference in temperature is directly proportional to the negative of the current temperature,ordTdt=−kT(1) where T is the temperature,and k is the cooling constant that is derived from experimentation. To derive the form we want our regression equation for our experimental data to be in,we solve our differential equation by integration with separation of variables:1 T dT=−kdt(2)1T dT=−kdt(3)ln(T)=−kt+C(4) where C is a constant.Solving for T,T=e−kt+C=Ce−kt(5)Page6To solve for this constant of integration,C,we substitute in the initial temperature T0of the water at time t=0.T0=Ce−k×0=C×1(6) giving us the regression equation formT=T0e−kt(7) Since k is dependent on the sum of physical conditions in each specific scenario,it can only be derived from experimental data.Then,to predict the value of k for our industry standard bathtub, we take sets of experimental data and analyze trends between them to extrapolate a value of k for our purposes.4.2.2Experimental DataWe created a graph to represent the data we found for temperature over time in100mL,300mL, and800mL volumes of water(Gieseking).The ambient room temperature in this setting was23◦C. We realized that it is wrong to model these data with exponential regressions that have horizontal asymptotes at0◦C.Instead,since logically the temperature of the water should approach—butPage7never dip below—the room temperature,we create exponential regressions with horizontal asymp-totes at23◦C instead.The easiest way to do this is to subtract23◦C from all points for the purpose of regression,and then modifying the regression equation to account for the difference.The results are as follows:The resulting equations are:y=77e−0.029x+23(8)y=77e−0.036x+23(9)y=77e−0.052x+23(10) for the100mL beaker,300mL beaker,and the800mL beaker,respectively.Note that23◦C has been added to each equation to account for the shift.It’s unreasonable to hope that data from small beakers alone can accurately predict the tem-perature changes in a large bathtub.However,we could notfind any accessible experimental data for large volumes of water.This is likely due to the fact that when studying water cooling,most scientists will choose to use small quantities of water for convenience.Since it was difficult to obtain large-scale water cooling data,we performed our own experiment with a bathtub to obtain a k-value that pertains to our model.The procedure of our experiment can be found in Appendix A.The results of our experiment are as follows:Page8The regression equation for our bathtub temperature versus time,then,iswhich means our experimental k per minute is 0.0044860=0.000075.However,this k was only relevant to our specific bathtub.In order to find k -values for bathtubs of varied volume and surface area,we needed to create a model for k .We consider that there is a relationship between k and exposed surface area (SA E )and volume (V ).Reasoning through the fact that k is to be a constant,we assumed that k should be unitless.Thus,when relating k to V and SA E ,k should be dependent on a power of V 2SA 3E to cancel the units of meters in the numerator and denominator.The calculations for SA E must take into account the fact that bathtubs are heavily insulated on five of six faces,but beakers are not.The effective SA E of the bathtub,then,is its top surface’s area,or 55×23=1,265in 2.The effective SA E of the beakers are their total surface area,because of our assumption that thin glass provides virtually no insulation.Then,their SA E is given by:Page 9SA cylinder=2πr2+πrh(11) where r is the radius of the beaker and h is the height of the water in the beaker.Because the beakers were notfilled in the experiments,we recalculate the effective heights with the following relationship:h=h beaker×V waterV beaker(12)giving us a surface area equation for the beakers ofSA cylinder=2πr2+rh beaker×V waterV beaker(13)Below is a table of V,SA E,V2SA3E ,and k values for our four data sets.SA E(m)V(L)k0.0521504380.80.0262593850.0307876080.30.0303166420.0157079630.10.0405089080.8161274269.485270.000075Proceeding,we test an exponential regression on the four sets of data relating k to V2SA3E.Firstwe tested V2SA3E,which gave us an R2value of0.99764.We then test(V2SA3E)2Page10which gave us an R 2value of 0.996529.Seeing as this is worse,we test V 2SA 3E ,which gave us an R 2value of 0.999294.Noticing improvement,we test 3 V 2SA 3E ,noting a possible connection between the three dimensions our heat transfer acts in,and the third-degree root wetake on our V 2SA 3E ,Page 11which gives us the regression equation:k=0.385387190197681e −0.16708193785762233V2SA3E(14)This modelfit the data with an accuracy of R2=0.99929854436231100000,which was even bet-ter than the previous ing this model for k,we predict a k-value of0.385387190197681 for our standard60”×30”×14”bathtub.Thus,ourfinal equation for the change in temperature due to the environment isdTdt=−0.385387190197681T(15) 4.2.3HeatingThe second part of the model calculates the amount of heat added by the person as he turned on the water faucet to a certainflow rate.To achieve this,we used the specific heat capacity formula, which is∆Q=mC p T(16) where∆Q is the change in heat,m is mass,C p is the specific heat capacity of water,and T is the change in temperature.There are two instances of heat change resulting from the addition of hot water:heat is being added through the addition of hot water from the faucet,and heat is being removed through the drainage of excess water.The heat contributed by the waterflowing in is given by the following equation:Q1=dmdtC p T hot(17)where Q1is the heat from the waterflowing in,dmdt is the massflow rate of the waterflowingin,and T is the absolute temperature of the waterflowing in(Kelvins).Page12The heat lost from excess water drainage is given by the following equation:Q2=dm lostdtC p T lost(18)where Q2is the heat from the water draining out,dm lostdt is the massflow rate of the waterdraining out,and T lost is the absolute temperature of the water draining out(Kelvins).By our global assumption that temperature is consistent throughout the tub,we have that T lost is simply the temperature of the tub,T.Because the volume of waterflowing in at any point in time is equivalent to the volume of waterflowing out due to overflow,the massflow rate out is equal to the massflow rate in,ordm lostdt =dmdt.Then,the heat change of the water in the tub can be obtained by subtracting theabove two equations:∆Q=dmdtC p(T hot−T)(19)We also have that∆Q=m tub C p T tub(20)Since∆Q is common to both equations,we can substitute the expression dmdt C p(T hot−T)for∆Q in the second equation and solve for T tub,which becomes:4.3Simulation DesignAll that is left now is to combine the cooling and heating of the water to simulate the overall temperature change.In order to simulate the cooling of the water when the person has not turned on the faucet,we use the Newton’s law differential obtained earlier:dTdt=−0.385387190197681T(21) And to simulate the change in temperature due to the addition of hot water,we add the equation obtained in the previous section to the above:dT dt =−0.385387190197681T+dmdt(T hot−T)m tub(22)We used a computer program written in Python(code included in Appendix B)to simulate the change in temperature of the bathtub according to the differential equation over a period of40 minutes.All units are converted to standard SI units in the simulation.The simulation accounts for the loss of heat to the environment,as well as the heating of the water due to the person operating the faucet.In order to achieve this,we set up a loop to run the simulation between time t=0minutes to t=40minutes,what we assumed to be the end of the bath.During each one-minute”frame”in this interval,we calculated the new temperature of the water using the previous temperature with the two equations for change in temperature per minute calculated in the previous section.The simulation will begin by applying thefirst equation to the temperature of the water every frame,since the hot water faucet is initially off.When the temperature of the water reaches aPage13noticeably cooler temperature(of1.4◦C below its initial temperature),the person will turn on the hot water,and the simulation will no longer apply thefirst equation,but instead the second equation,in order to account for the temperature gained from the hot water.4.3.1Selecting Optimal Flow RateIn order to evaluate the results for the variousflow rates,we used the deviance value,obtained by finding the sum of the squares of the differences between the”current”temperature and the initial temperature every frame:∆total=Nn(x n−¯x)2(23)Comparing the deviance of everyflow rate produces the following:Now,selecting the optimalflow rate is trivial.Since the optimalflow rate is characterized by the least difference from the original temperature,it is represented by the point indicated at the bottom of the graph,at(1.81,10.179381314125882).This means that our optimalflow rate to be recommended to the person is1.81gallons/minute,which results in a total deviance of10.179 from the original temperature of his bath.The following is a temperature vs.time graph of the simulation using the optimalflow rate of1.81gallons per minute.Note that the curve initially decreases,as the temperature of the water is only affected by the cooler temperature of the room. However,once the temperature of the water has dropped below1.4◦C from the initial temperature of37◦C,the person turns on the hot water faucet which begins to increase the temperature of the water.Page14Page154.3.2Validation of ModelTo confirm that our cooling simulation accurately models what occurs in the real world,we ran the simulation on the circumstances of our real-world experiment in Appendix B.Fitting the temperatures predicted by the simulation to the actual experimentally obtained data,wefind that the simulation is able to very accurately predict the temperatures at given points in time retroactively.As a matter of fact,this differential simulation is even more accurate than the previous regression model we had obtained for the data:y=16e−0.00448x+21(24) as that regression led to an R-squared value of0.97326,and the simulation gave an R-squared value of0.999836.While the relationship between temperature and time in this graph appears to be linear,it is still exponential–the very small k-value is what makes it appear linear.Indeed,there is a very slight,hardly noticeable curvature to the above graph.5Sensitivity Analysis:Varying Bathtub CharacteristicsWe now address query number”3”by creating various bathtub designs,varying the surface areas exposed and volumes,to observe the effect of the surface area to volume ratio on the rate of cooling. The changes in surface area and volume change the k value,so we recalculate k for each case and test each bathtub design with our simulation.5.1Varying Surface Area Under Constant VolumeThe standard bathtub model has a volume of25,200in3(”Bathtub Sizes”),so we keep that volume constant while creating three other common bathtub ing our model,we predict thePage16optimalflow rate for following designs created using Autodesk Inventor,choosingflow rates with the lowest total deviance from initial temperature.Page17Standard bathtub modelDimensions(length x width x height):60”×30”×14”Surface area:1,800in2Volume:25,200in3The standard bathtub model has a predicted optimalflow rate of1.81gallons/minute and a deviance of10.18◦C2.Page18Page19Circular bathtub modelDimensions(radius x height):23.14”×15”Surface area:1,680in2Volume:25,200in3The circular bathtub model has a predicted optimalflow rate of1.61gallons/minute and a deviance of7.86◦C2.Page20Page21Triangular bathtub modelDimensions(side1x side2x side3x height):57.02”×57.02”×80.64”×15.5”Surface area:1,625.81in2Volume:25,200in3The triangular bathtub model has a predicted optimalflow rate of1.95gallons/minute and a deviance of5.16◦C2.Page22。