A Neural Network decision module for the Adaptive Strategic Planning Framework in RoboCup
《Broadcasting, IEEE Transactions on》期刊第3页200条数据
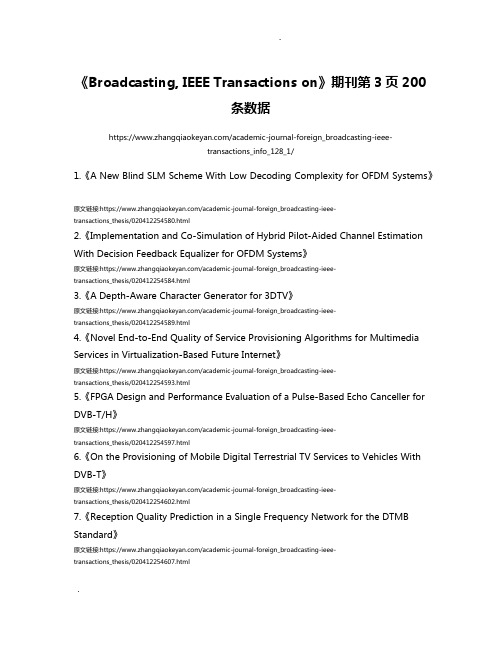
《Broadcasting, IEEE Transactions on》期刊第3页200条数据https:///academic-journal-foreign_broadcasting-ieee-transactions_info_128_1/1.《A New Blind SLM Scheme With Low Decoding Complexity for OFDM Systems》原文链接:https:///academic-journal-foreign_broadcasting-ieee-transactions_thesis/020*********.html2.《Implementation and Co-Simulation of Hybrid Pilot-Aided Channel Estimation With Decision Feedback Equalizer for OFDM Systems》原文链接:https:///academic-journal-foreign_broadcasting-ieee-transactions_thesis/020*********.html3.《A Depth-Aware Character Generator for 3DTV》原文链接:https:///academic-journal-foreign_broadcasting-ieee-transactions_thesis/020*********.html4.《Novel End-to-End Quality of Service Provisioning Algorithms for Multimedia Services in Virtualization-Based Future Internet》原文链接:https:///academic-journal-foreign_broadcasting-ieee-transactions_thesis/020*********.html5.《FPGA Design and Performance Evaluation of a Pulse-Based Echo Canceller for DVB-T/H》原文链接:https:///academic-journal-foreign_broadcasting-ieee-transactions_thesis/020*********.html6.《On the Provisioning of Mobile Digital Terrestrial TV Services to Vehicles With DVB-T》原文链接:https:///academic-journal-foreign_broadcasting-ieee-transactions_thesis/020*********.html7.《Reception Quality Prediction in a Single Frequency Network for the DTMB Standard》原文链接:https:///academic-journal-foreign_broadcasting-ieee-transactions_thesis/020*********.html8.《Signal-to-Noise Ratio Estimation Algorithm for Advanced DVB-RCS Systems》原文链接:https:///academic-journal-foreign_broadcasting-ieee-transactions_thesis/020*********.html9.《Augmented Data Transmission Based on Low Density Parity Check Code for the ATSC Terrestrial DTV System》原文链接:https:///academic-journal-foreign_broadcasting-ieee-transactions_thesis/020*********.html10.《Adaptive Digital Predistortion for Wideband High Crest Factor Applications Based on the WACP Optimization Objective: A Conceptual Overview》原文链接:https:///academic-journal-foreign_broadcasting-ieee-transactions_thesis/020*********.html11.《Initial-Estimation-Based Adaptive Carrier Recovery Scheme for DVB-S2 System》原文链接:https:///academic-journal-foreign_broadcasting-ieee-transactions_thesis/020*********.html12.《Quantifying Subjective Quality Evaluations for Mobile Video Watching in a Semi-Living Lab Context》原文链接:https:///academic-journal-foreign_broadcasting-ieee-transactions_thesis/020*********.html13.《Perceived 3D TV Transmission Quality Assessment: Multi-Laboratory Results Using Absolute Category Rating on Quality of Experience Scale》原文链接:https:///academic-journal-foreign_broadcasting-ieee-transactions_thesis/020*********.html14.《Efficient Pilot Patterns and Channel Estimations for MIMO-OFDM Systems》原文链接:https:///academic-journal-foreign_broadcasting-ieee-transactions_thesis/020*********.html15.《Coding Distortion Elimination of Virtual View Synthesis for 3D Video System: Theoretical Analyses and Implementation》原文链接:https:///academic-journal-foreign_broadcasting-ieee-transactions_thesis/020*********.html16.《An Efficient Nonlinear Companding Transform for Reducing PAPR of OFDM Signals》原文链接:https:///academic-journal-foreign_broadcasting-ieee-transactions_thesis/020*********.html17.《Saliency Inspired Full-Reference Quality Metrics for Packet-Loss-Impaired Video》原文链接:https:///academic-journal-foreign_broadcasting-ieee-transactions_thesis/020*********.html18.《Improved CIR-Based Receiver Design for DVB-T2 System in Large Delay Spread Channels: Synchronization and Equalization》原文链接:https:///academic-journal-foreign_broadcasting-ieee-transactions_thesis/020*********.html19.《High Power Amplifier Pre-Distorter Based on Neural-Fuzzy Systems for OFDM Signals》原文链接:https:///academic-journal-foreign_broadcasting-ieee-transactions_thesis/020*********.html20.《Performance Analysis of Inter-Layer Prediction in Scalable Video Coding Extension of H.264/AVC》原文链接:https:///academic-journal-foreign_broadcasting-ieee-transactions_thesis/020*********.html21.《Study of Rating Scales for Subjective Quality Assessment of High-Definition Video》原文链接:https:///academic-journal-foreign_broadcasting-ieee-transactions_thesis/020*********.html22.《Planning Factors for Digital Local Broadcasting in the 26 MHz Band》原文链接:https:///academic-journal-foreign_broadcasting-ieee-transactions_thesis/020*********.html23.《Peak-to-Average Power Ratio Reduction of OFDM Signals Using PTS Scheme With Low Computational Complexity》原文链接:https:///academic-journal-foreign_broadcasting-ieee-transactions_thesis/020*********.html24.《Feedback Cancellation for T-DMB Repeaters Based on Frequency-Domain Channel Estimation》原文链接:https:///academic-journal-foreign_broadcasting-ieee-transactions_thesis/020*********.html25.《Efficient Multi-Reference Frame Selection Algorithm for Hierarchical B Pictures in Multiview Video Coding》原文链接:https:///academic-journal-foreign_broadcasting-ieee-transactions_thesis/020*********.html26.《Performance Comparisons and Improvements of Channel Coding Techniques for Digital Satellite Broadcasting to Mobile Users》原文链接:https:///academic-journal-foreign_broadcasting-ieee-transactions_thesis/020*********.html27.《Burst-Aware Dynamic Rate Control for H.264/AVC Video Streaming》原文链接:https:///academic-journal-foreign_broadcasting-ieee-transactions_thesis/020*********.html28.《Helicopter-Based Digital Electronic News Gathering (H-DENG) System: Case Study and System Solution》原文链接:https:///academic-journal-foreign_broadcasting-ieee-transactions_thesis/020*********.html29.《Transmit Diversity for TDS-OFDM Broadcasting System Over Doubly Selective Fading Channels》原文链接:https:///academic-journal-foreign_broadcasting-ieee-transactions_thesis/020*********.html30.《Interference Cancellation Techniques for Digital On-Channel Repeaters in T-DMB System》原文链接:https:///academic-journal-foreign_broadcasting-ieee-transactions_thesis/020*********.html31.《Field Measurements of EM Radiation From In-House Power Line Telecommunications (PLT) Devices》原文链接:https:///academic-journal-foreign_broadcasting-ieee-transactions_thesis/020*********.html32.《A Novel Scheme of Joint Channel and Phase Noise Compensation for Chinese DTMB System》原文链接:https:///academic-journal-foreign_broadcasting-ieee-transactions_thesis/020*********.html33.《Path Loss Prediction for Mobile Digital TV Propagation Under Viaduct》原文链接:https:///academic-journal-foreign_broadcasting-ieee-transactions_thesis/020*********.html34.《Efficient Motion Vector Interpolation for Error Concealment of H.264/AVC》原文链接:https:///academic-journal-foreign_broadcasting-ieee-transactions_thesis/020*********.html35.《3D-TV Content Creation: Automatic 2D-to-3D Video Conversion》原文链接:https:///academic-journal-foreign_broadcasting-ieee-transactions_thesis/020*********.html36.《A Novel Rate Control Technique for Multiview Video Plus Depth Based 3D Video Coding》原文链接:https:///academic-journal-foreign_broadcasting-ieee-transactions_thesis/020*********.html37.《The Effect of Crosstalk on the Perceived Depth From Disparity and Monocular Occlusions》原文链接:https:///academic-journal-foreign_broadcasting-ieee-transactions_thesis/020*********.html38.《Semi-Automatic 2D-to-3D Conversion Using Disparity Propagation》原文链接:https:///academic-journal-foreign_broadcasting-ieee-transactions_thesis/020*********.html39.《Display-Independent 3D-TV Production and Delivery Using the Layered Depth Video Format》原文链接:https:///academic-journal-foreign_broadcasting-ieee-transactions_thesis/020*********.html40.《3DTV Roll-Out Scenarios: A DVB-T2 Approach》原文链接:https:///academic-journal-foreign_broadcasting-ieee-transactions_thesis/020*********.html41.《PAPR Reduction Using Low Complexity PTS to Construct of OFDM Signals Without Side Information》原文链接:https:///academic-journal-foreign_broadcasting-ieee-transactions_thesis/020*********.html42.《Quality-Oriented Multiple-Source Multimedia Delivery Over Heterogeneous Wireless Networks》原文链接:https:///academic-journal-foreign_broadcasting-ieee-transactions_thesis/020*********.html43.《Efficient PAPR Reduction in OFDM Systems Based on a Companding Technique With Trapezium Distribution》原文链接:https:///academic-journal-foreign_broadcasting-ieee-transactions_thesis/020*********.html44.《Objective Video Quality Assessment Methods: A Classification, Review, and Performance Comparison》原文链接:https:///academic-journal-foreign_broadcasting-ieee-transactions_thesis/020*********.html45.《Pixel Interlacing Based Video Transmission for Low-Complexity Intra-Frame Error Concealment》原文链接:https:///academic-journal-foreign_broadcasting-ieee-transactions_thesis/020*********.html46.《Fountain Codes With PAPR Constraint for Multicast Communications》原文链接:https:///academic-journal-foreign_broadcasting-ieee-transactions_thesis/020*********.html47.《RF Watermark Backward Compatibility Tests for the ATSC Terrestrial DTV Receivers》原文链接:https:///academic-journal-foreign_broadcasting-ieee-transactions_thesis/020*********.html48.《61st Annual IEEE Broadcast Symposium — Save the Date》原文链接:https:///academic-journal-foreign_broadcasting-ieee-transactions_thesis/020*********.html49.《Evaluation of Asymmetric Stereo Video Coding and Rate Scaling for Adaptive 3D Video Streaming》原文链接:https:///academic-journal-foreign_broadcasting-ieee-transactions_thesis/020*********.html50.《Stereoscopic Perceptual Video Coding Based on Just-Noticeable-Distortion Profile》原文链接:https:///academic-journal-foreign_broadcasting-ieee-transactions_thesis/020*********.html51.《A Depth Information Based Fast Mode Decision Algorithm for Color Plus Depth-Map 3D Videos》原文链接:https:///academic-journal-foreign_broadcasting-ieee-transactions_thesis/020*********.html52.《3D-TV Production From Conventional Cameras for Sports Broadcast》原文链接:https:///academic-journal-foreign_broadcasting-ieee-transactions_thesis/020*********.html53.《A Digital Blind Watermarking for Depth-Image-Based Rendering 3D Images》原文链接:https:///academic-journal-foreign_broadcasting-ieee-transactions_thesis/020*********.html54.《Object-Based 2D-to-3D Video Conversion for Effective Stereoscopic Content Generation in 3D-TV Applications》原文链接:https:///academic-journal-foreign_broadcasting-ieee-transactions_thesis/020*********.html55.《3D-TV Content Storage and Transmission》原文链接:https:///academic-journal-foreign_broadcasting-ieee-transactions_thesis/020*********.html56.《New Depth Coding Techniques With Utilization of Corresponding Video》原文链接:https:///academic-journal-foreign_broadcasting-ieee-transactions_thesis/020*********.html57.《3DTV Broadcasting and Distribution Systems》原文链接:https:///academic-journal-foreign_broadcasting-ieee-transactions_thesis/020*********.html58.《Boundary Artifact Reduction in View Synthesis of 3D Video: From Perspective of Texture-Depth Alignment》原文链接:https:///academic-journal-foreign_broadcasting-ieee-transactions_thesis/020*********.html59.《Stereoscopic 3D-TV: Visual Comfort》原文链接:https:///academic-journal-foreign_broadcasting-ieee-transactions_thesis/020*********.html60.《A Novel Inpainting-Based Layered Depth Video for 3DTV》原文链接:https:///academic-journal-foreign_broadcasting-ieee-transactions_thesis/020*********.html61.《3D-TV R&D Activities in Europe》原文链接:https:///academic-journal-foreign_broadcasting-ieee-transactions_thesis/020*********.html62.《A Directional-View and Sound System Using a Tracking Method》原文链接:https:///academic-journal-foreign_broadcasting-ieee-transactions_thesis/020*********.html63.《Joint Maximum Likelihood Estimation of Carrier and Sampling Frequency Offsets for OFDM Systems》原文链接:https:///academic-journal-foreign_broadcasting-ieee-transactions_thesis/020*********.html64.《Perceptual Issues in Stereoscopic Signal Processing》原文链接:https:///academic-journal-foreign_broadcasting-ieee-transactions_thesis/020*********.html65.《Performance Evaluation of Multimedia Content Distribution Over Multi-Homed Wireless Networks》原文链接:https:///academic-journal-foreign_broadcasting-ieee-transactions_thesis/020*********.html66.《The Relationship Among Video Quality, Screen Resolution, and Bit Rate》原文链接:https:///academic-journal-foreign_broadcasting-ieee-transactions_thesis/020*********.html67.《Corrections to “Efficient Motion Vector Interpolation f or Error Concealment of H.264/AVC”》原文链接:https:///academic-journal-foreign_broadcasting-ieee-transactions_thesis/020*********.html68.《PAPR Reduction of OFDM Signals by PTS With Grouping and Recursive Phase Weighting Methods》原文链接:https:///academic-journal-foreign_broadcasting-ieee-transactions_thesis/020*********.html69.《Improve the Performance of LDPC Coded QAM by Selective Bit Mapping in Terrestrial Broadcasting System》原文链接:https:///academic-journal-foreign_broadcasting-ieee-transactions_thesis/020*********.html70.《The Importance of Visual Attention in Improving the 3D-TV Viewing Experience: Overview and New Perspectives》原文链接:https:///academic-journal-foreign_broadcasting-ieee-transactions_thesis/020*********.html71.《Co-Channel Analog Television Interference in the TDS-OFDM-Based DTTB System: Consequences and Solutions》原文链接:https:///academic-journal-foreign_broadcasting-ieee-transactions_thesis/020*********.html72.《Prediction and Transmission Optimization of Video Guaranteeing a Bounded Zapping-Delay in DVB-H》原文链接:https:///academic-journal-foreign_broadcasting-ieee-transactions_thesis/020*********.html73.《IBC2011 Experience the Future》原文链接:https:///academic-journal-foreign_broadcasting-ieee-transactions_thesis/020*********.html74.《61st Annual IEEE Broadcast Symposium — Save the Date》原文链接:https:///academic-journal-foreign_broadcasting-ieee-transactions_thesis/020*********.html75.《A 2$times$2 MIMO DVB-T2 System: Design, New Channel Estimation Scheme and Measurements With Polarization Diversity》原文链接:https:///academic-journal-foreign_broadcasting-ieee-transactions_thesis/020*********.html76.《A Pilot Symbol Pattern Enabling Data Recovery Without Side Information in PTS-Based OFDM Systems》原文链接:https:///academic-journal-foreign_broadcasting-ieee-transactions_thesis/020*********.html77.《Efficient Incremental Raptor Decoding Over BEC for 3GPP MBMS and DVB IP-Datacast Services》原文链接:https:///academic-journal-foreign_broadcasting-ieee-transactions_thesis/020*********.html78.《Spatio-Temporally Consistent Novel View Synthesis Algorithm From Video-Plus-Depth Sequences for Autostereoscopic Displays》原文链接:https:///academic-journal-foreign_broadcasting-ieee-transactions_thesis/020*********.html79.《IBC2011 Experience the Future》原文链接:https:///academic-journal-foreign_broadcasting-ieee-transactions_thesis/020*********.html80.《Evaluation of Stereoscopic Images: Beyond 2D Quality》原文链接:https:///academic-journal-foreign_broadcasting-ieee-transactions_thesis/020*********.html81.《An Evaluation of Parameterized Gradient Based Routing With QoE Monitoring for Multiple IPTV Providers》原文链接:https:///academic-journal-foreign_broadcasting-ieee-transactions_thesis/020*********.html82.《Three-Dimensional Displays: A Review and Applications Analysis》原文链接:https:///academic-journal-foreign_broadcasting-ieee-transactions_thesis/020*********.html83.《Guest Editorial Special Issue on 3D-TV Horizon: Contents, Systems, and Visual Perception》原文链接:https:///academic-journal-foreign_broadcasting-ieee-transactions_thesis/020*********.html84.《LIVE: An Integrated Production and Feedback System for Intelligent and Interactive TV Broadcasting》原文链接:https:///academic-journal-foreign_broadcasting-ieee-transactions_thesis/020*********.html85.《$MS^{2}$ : A New Real-Time Multi-Source Mobile-Streaming Architecture》原文链接:https:///academic-journal-foreign_broadcasting-ieee-transactions_thesis/020*********.html86.《A Reverse-Order Scheduling Scheme for Broadcasting Continuous Multimedia Data Over a Single Channel》原文链接:https:///academic-journal-foreign_broadcasting-ieee-transactions_thesis/020*********.html87.《An Automatic Recommendation Scheme of TV Program Contents for (IP)TV Personalization》原文链接:https:///academic-journal-foreign_broadcasting-ieee-transactions_thesis/020*********.html88.《Irregular Mapping and its Application in Bit-Interleaved LDPC Coded Modulation With Iterative Demapping and Decoding》原文链接:https:///academic-journal-foreign_broadcasting-ieee-transactions_thesis/020*********.html89.《Time Diversity in Mobile DVB-T2 Systems》原文链接:https:///academic-journal-foreign_broadcasting-ieee-transactions_thesis/020*********.html90.《An Audio Quality Evaluation of Commercial Digital Radio Systems》原文链接:https:///academic-journal-foreign_broadcasting-ieee-transactions_thesis/020*********.html91.《Spectral Geometry Image: Image Based 3D Models for Digital Broadcasting Applications》原文链接:https:///academic-journal-foreign_broadcasting-ieee-transactions_thesis/020*********.html92.《Novel Approach to Support Multimedia Services Over DTMB System》原文链接:https:///academic-journal-foreign_broadcasting-ieee-transactions_thesis/020*********.html93.《Collaborative Content Fetching Using MAC Layer Multicast in Wireless Mobile Networks》原文链接:https:///academic-journal-foreign_broadcasting-ieee-transactions_thesis/020*********.html94.《Energy-Efficient Transmission for Protection of Incumbent Users》原文链接:https:///academic-journal-foreign_broadcasting-ieee-transactions_thesis/020*********.html95.《Normalization Factor of Hierarchically Modulated Symbols for Advanced T-DMB System》原文链接:https:///academic-journal-foreign_broadcasting-ieee-transactions_thesis/020*********.html96.《Pooling-Based Intra Prediction Mode Coding for Mobile Video Applications》原文链接:https:///academic-journal-foreign_broadcasting-ieee-transactions_thesis/020*********.html97.《A Suboptimal Tone Reservation Algorithm Based on Cross-Entropy Method for PAPR Reduction in OFDM Systems》原文链接:https:///academic-journal-foreign_broadcasting-ieee-transactions_thesis/020*********.html98.《A Measurement Method of the DTMB Modulator》原文链接:https:///academic-journal-foreign_broadcasting-ieee-transactions_thesis/020*********.html99.《Interference Elimination for Chinese DTMB System With Transmit Diversity》原文链接:https:///academic-journal-foreign_broadcasting-ieee-transactions_thesis/020*********.html100.《61st Annual IEEE Broadcast Symposium》原文链接:https:///academic-journal-foreign_broadcasting-ieee-transactions_thesis/020*********.html101.《IBC2011 Experience the Future》原文链接:https:///academic-journal-foreign_broadcasting-ieee-transactions_thesis/020*********.html102.《A Low-Complexity SLM Scheme Using Additive Mapping Sequences for PAPR Reduction of OFDM Signals》原文链接:https:///academic-journal-foreign_broadcasting-ieee-transactions_thesis/020*********.html103.《Illumination-Sensitive Background Modeling Approach for Accurate Moving Object Detection》原文链接:https:///academic-journal-foreign_broadcasting-ieee-transactions_thesis/020*********.html104.《Coordinating Allocation of Resources for Multiple Virtual IPTV Providers to Maximize Revenue》原文链接:https:///academic-journal-foreign_broadcasting-ieee-transactions_thesis/020*********.html105.《Inter-Sequence Error Concealment Techniques for Multi-Broadcast TV Reception》原文链接:https:///academic-journal-foreign_broadcasting-ieee-transactions_thesis/020*********.html106.《Performance Evaluation of the DVB-RCT Standard for Interactive Services》原文链接:https:///academic-journal-foreign_broadcasting-ieee-transactions_thesis/020*********.html107.《An Efficient Predistorter Design for Compensating Nonlinear Memory High Power Amplifiers》原文链接:https:///academic-journal-foreign_broadcasting-ieee-transactions_thesis/020*********.html108.《Accurate BER Analysis of OFDM Systems Over Static Frequency-Selective Multipath Fading Channels》原文链接:https:///academic-journal-foreign_broadcasting-ieee-transactions_thesis/020*********.html109.《A Frame-Related Approach for Performance Improvement of MPE-FEC in DVB-H》原文链接:https:///academic-journal-foreign_broadcasting-ieee-transactions_thesis/020*********.html110.《Balanced Multiple Description Coding for 3D DCT Video》原文链接:https:///academic-journal-foreign_broadcasting-ieee-transactions_thesis/020*********.html111.《Performance Validation of the DVB-SH Standard for Satellite/Terrestrial Hybrid Mobile Broadcasting Networks》原文链接:https:///academic-journal-foreign_broadcasting-ieee-transactions_thesis/020*********.html112.《An Improved Tone Reservation Scheme With Fast Convergence for PAPR Reduction in OFDM Systems》原文链接:https:///academic-journal-foreign_broadcasting-ieee-transactions_thesis/020*********.html113.《Metaheuristic Procedure to Optimize Transmission Delays in DVB-T Single Frequency Networks》原文链接:https:///academic-journal-foreign_broadcasting-ieee-transactions_thesis/020*********.html114.《Adaptive Resource Allocation for MIMO-OFDM Based Wireless Multicast Systems》原文链接:https:///academic-journal-foreign_broadcasting-ieee-transactions_thesis/020*********.html115.《An Analytical Approach for Performance Evaluation of Hybrid (Broadcast/Mobile) Networks》原文链接:https:///academic-journal-foreign_broadcasting-ieee-transactions_thesis/020*********.html116.《Cost-Aware Wireless Data Broadcasting》原文链接:https:///academic-journal-foreign_broadcasting-ieee-transactions_thesis/020*********.html117.《Subspace-Based Semi-Blind Channel Estimation in Uplink OFDMA Systems》原文链接:https:///academic-journal-foreign_broadcasting-ieee-transactions_thesis/020*********.html118.《Performance of the Consumer ATSC-DTV Receivers in the Presence of Single or Double Interference on Adjacent/Taboo Channels》原文链接:https:///academic-journal-foreign_broadcasting-ieee-transactions_thesis/020*********.html119.《A Cooperative Cellular and Broadcast Conditional Access System for Pay-TV Systems》原文链接:https:///academic-journal-foreign_broadcasting-ieee-transactions_thesis/020*********.html120.《A Narrow-Angle Directional Microphone With Suppressed Rear Sensitivity》原文链接:https:///academic-journal-foreign_broadcasting-ieee-transactions_thesis/020*********.html121.《Peak-to-Average Power Ratio Reduction in OFDM Systems Using All-Pass Filters》原文链接:https:///academic-journal-foreign_broadcasting-ieee-transactions_thesis/020*********.html122.《Development of Advanced Terrestrial DMB System》原文链接:https:///academic-journal-foreign_broadcasting-ieee-transactions_thesis/020*********.html123.《HDTV Subjective Quality of H.264 vs. MPEG-2, With and Without Packet Loss》原文链接:https:///academic-journal-foreign_broadcasting-ieee-transactions_thesis/020*********.html124.《Estimation of RF Electromagnetic Levels Around TV Broadcast Antennas Using Fuzzy Logic》原文链接:https:///academic-journal-foreign_broadcasting-ieee-transactions_thesis/020*********.html125.《Statistical Multiplexing for Digital Audio Broadcasting Applications》原文链接:https:///academic-journal-foreign_broadcasting-ieee-transactions_thesis/020*********.html126.《A Composite PN-Correlation Based Synchronizer for TDS-OFDM Receiver》原文链接:https:///academic-journal-foreign_broadcasting-ieee-transactions_thesis/020*********.html127.《Application of Quantum-Inspired Evolutionary Algorithm to Reduce PAPRof an OFDM Signal Using Partial Transmit Sequences Technique》原文链接:https:///academic-journal-foreign_broadcasting-ieee-transactions_thesis/020*********.html128.《Improved Decoding Algorithm of Bit-Interleaved Coded Modulation for LDPC Code》原文链接:https:///academic-journal-foreign_broadcasting-ieee-transactions_thesis/020*********.html129.《Precoding for PAPR Reduction of OFDM Signals With Minimum Error Probability》原文链接:https:///academic-journal-foreign_broadcasting-ieee-transactions_thesis/020*********.html130.《Network Design and Field Application of ATSC Distributed Translators》原文链接:https:///academic-journal-foreign_broadcasting-ieee-transactions_thesis/020*********.html131.《On the Channel and Signal Cross Correlation of Downlink and Uplink Mobile UHF DTV Channels With Antenna Diversity》原文链接:https:///academic-journal-foreign_broadcasting-ieee-transactions_thesis/020*********.html132.《Performance Evaluation of TV Over Broadband Wireless Access Networks》原文链接:https:///academic-journal-foreign_broadcasting-ieee-transactions_thesis/020*********.html133.《IBC2010 Experience the State-of-the-Art》原文链接:https:///academic-journal-foreign_broadcasting-ieee-transactions_thesis/020*********.html134.《Peak-to-Average Power Ratio Reduction of OFDM Signals With Nonlinear Companding Scheme》原文链接:https:///academic-journal-foreign_broadcasting-ieee-transactions_thesis/020*********.html135.《Motion-Compensated Frame Rate Up-Conversion—Part I: Fast Multi-Frame Motion Estimation》原文链接:https:///academic-journal-foreign_broadcasting-ieee-transactions_thesis/020*********.html136.《Comments on Equation (4) in “Single Frequency Networks in DTV”》原文链接:https:///academic-journal-foreign_broadcasting-ieee-transactions_thesis/020*********.html137.《Motion-Compensated Frame Rate Up-Conversion—Part II: New Algorithms for Frame Interpolation》原文链接:https:///academic-journal-foreign_broadcasting-ieee-transactions_thesis/020*********.html138.《A Novel Equalization Scheme for ZP-OFDM System Over Deep Fading Channels》原文链接:https:///academic-journal-foreign_broadcasting-ieee-transactions_thesis/020*********.html139.《A Synchronization Design for UWB-Based Wireless Multimedia Systems》原文链接:https:///academic-journal-foreign_broadcasting-ieee-transactions_thesis/020*********.html140.《Frequency Domain Decision Feedback Equalization for Uplink SC-FDMA》原文链接:https:///academic-journal-foreign_broadcasting-ieee-transactions_thesis/020*********.html141.《A 2 2 MIMO DVB-T2 System: Design, New Channel Estimation Scheme and Measurements With Polarization Diversity》原文链接:https:///academic-journal-foreign_broadcasting-ieee-transactions_thesis/020*********.html142.《Impact of the Receive Antenna Arrays on Spatio-Temporal Availability in Satellite-to-Indoor Broadcasting》原文链接:https:///academic-journal-foreign_broadcasting-ieee-transactions_thesis/020*********.html143.《Reducing Channel Zapping Time in IPTV Based on User's Channel Selection Behaviors》原文链接:https:///academic-journal-foreign_broadcasting-ieee-transactions_thesis/020*********.html144.《On the Methodology for Calculating SFN Gain in Digital Broadcast Systems》原文链接:https:///academic-journal-foreign_broadcasting-ieee-transactions_thesis/020*********.html145.《Statistical Multiplexing of Upstream Transmissions in DOCSIS Cable Networks》原文链接:https:///academic-journal-foreign_broadcasting-ieee-transactions_thesis/020*********.html146.《Bit-Rate Allocation for Broadcasting of Scalable Video Over Wireless Networks》原文链接:https:///academic-journal-foreign_broadcasting-ieee-transactions_thesis/020*********.html147.《Full-Reference Video Quality Metric for Fully Scalable and Mobile SVC Content》。
神经网络参考文献列表
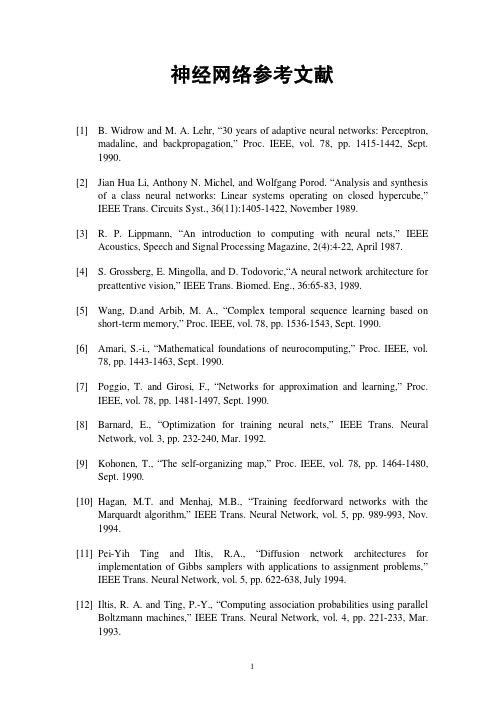
神经网络参考文献[1] B. Widrow and M. A. Lehr, “30 years of adaptive neural networks: Perceptron,madaline, and backpropagation,” Proc. IEEE, vol. 78, pp. 1415-1442, Sept.1990.[2] Jian Hua Li, Anthony N. Michel, and Wolfgang Porod. “Analysis and synthesisof a class neural networks: Linear systems operating on closed hypercube,”IEEE Trans. Circuits Syst., 36(11):1405-1422, November 1989.[3] R. P. Lippmann, “An introduction to computing with neural nets,” IEEEAcoustics, Speech and Signal Processing Magazine, 2(4):4-22, April 1987.[4] S. Grossberg, E. Mingolla, and D. Todovoric,“A neural network architecture forpreattentive vision,” IEEE Trans. Biomed. Eng., 36:65-83, 1989.[5] Wang, D.and Arbib, M. A., “Complex temporal sequence learning based onshort-term memory,” Proc. IEEE, vol. 78, pp. 1536-1543, Sept. 1990.[6] Amari, S.-i., “Mathematical foundations of neurocomputing,” Proc. IEEE, vol.78, pp. 1443-1463, Sept. 1990.[7] Poggio, T. and Girosi, F., “Networks for approximation and learning,” Proc.IEEE, vol. 78, pp. 1481-1497, Sept. 1990.[8] Barnard, E., “Optimization for training neural nets,” IEEE Trans. NeuralNetwork, vol. 3, pp. 232-240, Mar. 1992.[9] Kohonen, T., “The self-organizing map,” Proc. IEEE, vol. 78, pp. 1464-1480,Sept. 1990.[10] Hagan, M.T. and Menhaj, M.B., “Training feedforward networks with theMarquardt algorithm,” IEEE Trans. Neural Network, vol. 5, pp. 989-993, Nov.1994.[11] Pei-Yih Ting and Iltis, R.A., “Diffusion network architectures forimplementation of Gibbs samplers with applications to assignment problems,”IEEE Trans. Neural Network, vol. 5, pp. 622-638, July 1994.[12] Iltis, R. A. and Ting, P.-Y., “Computing association probabilities using parallelBoltzmann machines,” IEEE Trans. Neural Network, vol. 4, pp. 221-233, Mar.1993.[13] R. Battiti, “First and second order methods for learning: Between steepestdescent and Newton's method,” Neural Computation, vol. 4, no. 2, pp. 141-166, 1992.[14] G. A. Carpenter and S. Grossberg, “A massively parallel architecture for aself-organizing neural pattern recognition machine,” Computer Vision, Graphics, and Image Processing, vol. 37, pp. 54-115, 1987.[15] C. Charalambous, “Conjugate gradient algorithm for efficient training ofartificial neural networks,” IEEE Proceeding, vol. 139, no. 3, pp. 301-310, 1992.[16] M. A. Cohen and S. Grossberg, “Absolute stability of global pattern formationand parallel memory storage by competitive neural networks,” IEEE Trans. on Systems, Man, and Cybernetics, vol. 13, no. 5, pp. 815-826, 1983.[17] J. L. Elman, “Finding structure in time,” Cognitive Science, vol. 14, pp. 179-211,1990.[18] K. Fukushima, S. Miyake and T. Ito, “Neocognitron: A neural network modelfor a mechanism of visual pattern recognition,” IEEE Trans. on Systems, Man, and Cybernetics, vol. 13, no. 5, pp. 826-834, 1983.[19] K. Fukushima, “Neocognitron: A hierarchical neural network capable of visualpattern recognition,” Neural Networks, vol. 1, pp. 119-130, 1988.[20] S. Geman and D. Geman, “Stochastic relaxation, Gibbs distributions, and theBayesian restoration of images,” IEEE Trans. on Pattern Analysis and Machine Intelligence, vol. 6, pp. 721-741, 1984.[21] S. Grossberg, “How does the brain build a cognitive code?,” PsychologicalReview, vol. 87, pp. 1-51, 1980.[22] M. Heywood and P. Noakes, “A framework for improved training of sigma-pinetworks,” IEEE Transactions of Neural Networks, vol. 6, no. 4, pp. 893-903, 1995.[23] J. J. Hopfield, “Neural networks and physical systems with emergent collectivecomputational properties,” Proceedings of the National Academy of Sciences, vol. 79, pp. 2554-2558, 1982.[24] J. J. Hopfield, “Neurons with graded response have collective computationalproperties like those of two-state neurons,” Proceedings of the National Academy of Sciences, vol. 81, pp. 3088-3092, 1984.[25] J. J. Hopfield and D. W. Tank, “'Neural computation of decisions inoptimization problems,” Biological Cybernetics, vol. 52, pp. 141-152, 1985.[26] K. M. Hornik, M. Stinchcombe and H. White, “Multilayer feedforward networksare universal approximators,” Neural Networks, vol. 2, no. 5, pp. 359-366, 1989.[27] R. A. Jacobs, “Increased rates of convergence through learning rate adaptation,”Neural Networks, vol. 1, no. 4, pp. 295-308, 1988.[28] R. A. Jacobs, M. I. Jordan, S. J. Nowlan, and G. E. Hinton, “Adaptive mixturesof local experts,” Neural Computation, vol. 3, pp. 79-87, 1991.[29] T. Kohonen, “Correlation matrix memories,” IEEE Transactions on Computers,vol. 21, pp. 353-359, 1972.[30] B. Kosko, “Bidirectional associative memories,” IEEE Transactions on Systems,Man, and Cybernetics, vol. 18, no. 1, pp. 49-60, 1988.[31] D. J. C. MacKay, “A practical bayesian framework for backproagationnetworks,” Neural Computation, vol. 4, pp. 448-472, 1992.[32] A. N. Michel and J. A. Farrell, “Associative memories via artificial neuralnetworks,” IEEE Control Systems Magazine, April, pp. 6-17, 1990.[33] A. K. Rigler, J. M. Irvine and T. P. Vogl, “Rescaling of variables inbackpropagation learning,” Neural Networks, vol. 4, no. 2, pp. 225-229, 1991. [34] D. E. Rumelhart, G. E. Hinton, and R. J. Williams, “Learning representations byback-propagating errors,” Nature, vol. 323, pp. 533-536, 1986.[35] D. F. Specht, “Probabilistic neural networks,” Neural Networks, vol. 3, no. 1, pp.109-118, 1990.[36] D. F. Specht, “A General regression neural network,” IEEE Transactions onNeural Networks, vol. 2, no. 6, pp. 568-576, 1991.[37] D. W. Tank and J. J. Hopfield, “Simple 'neural' optimization networks: An A/Dconverter, signal decision circuit and a linear programming circuit,” IEEE Transactions on Circuits and Systems, vol. 33, no. 5, pp. 533-541, 1986.[38] T. P. Vogl, J. K. Mangis, A. K. Zigler, W. T. Zink, and D. L. Alkon,“Accelerating the convergence of the backpropagation method,” Biological Cybernetics, vol. 59, pp. 256-264, Sept. 1988.[39] P. J. Werbos, “Backpropagation through time: What it is and how to do it,”Proceedings of the IEEE, vol. 78, pp. 1550-1560, Oct. 1990.[40] B. Widrow and R. Winter, “Neural nets for adaptive filtering and adaptivepattern recognition,” IEEE Computer Magazine, pp. 25-39, March 1988.[41] R. J. Williams and D. Zipser, “A learning algorithm for continually running fullyrecurrent neural networks,” Neural Computation, vol. 1, pp. 270-280, 1989. [42] A. Waibel, Tl Hanazawa, G. Hinton, K. Shikano and K. J. Lang, “Phonemerecognition using time-delay neural networks,” IEEE Transactions on Acoustics, Speech and Signal Processing, vol. 37, pp. 328-339, 1989.[43] Linske, R., “Self-organization in a perceptual network,” IEEE ComputerMagazine, vol. 21, pp. 105-117, March 1988.[44] Carpenter, G.A. and Grossberg, S., “The ART of adaptive pattern recognition bya self-organizing neural network,” IEEE Computer Magazine, vol. 21, pp. 77-88,March 1988.[45] Fukushima, K., “A neural network for visual pattern recognition,” IEEEComputer Magazine, vol. 21, pp. 65-75, March 1988.[46] Kohonen, T., “The 'neural' phonetic typewriter,” IEEE Computer Magazine, vol.21, pp. 11-22, March 1988.。
机器学习与人工智能领域中常用的英语词汇
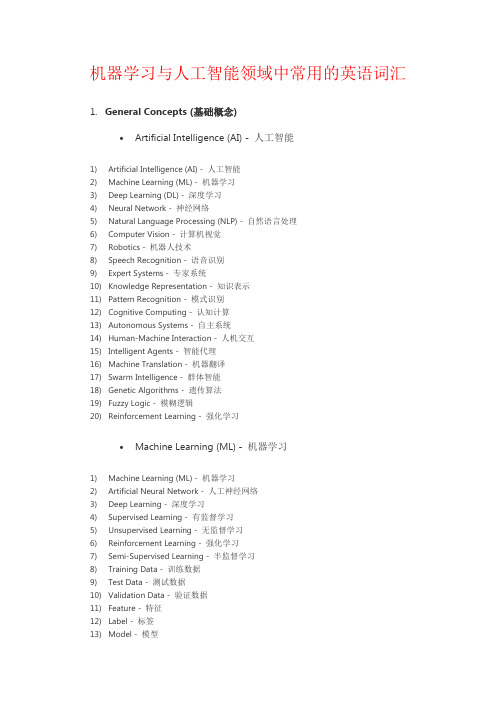
机器学习与人工智能领域中常用的英语词汇1.General Concepts (基础概念)•Artificial Intelligence (AI) - 人工智能1)Artificial Intelligence (AI) - 人工智能2)Machine Learning (ML) - 机器学习3)Deep Learning (DL) - 深度学习4)Neural Network - 神经网络5)Natural Language Processing (NLP) - 自然语言处理6)Computer Vision - 计算机视觉7)Robotics - 机器人技术8)Speech Recognition - 语音识别9)Expert Systems - 专家系统10)Knowledge Representation - 知识表示11)Pattern Recognition - 模式识别12)Cognitive Computing - 认知计算13)Autonomous Systems - 自主系统14)Human-Machine Interaction - 人机交互15)Intelligent Agents - 智能代理16)Machine Translation - 机器翻译17)Swarm Intelligence - 群体智能18)Genetic Algorithms - 遗传算法19)Fuzzy Logic - 模糊逻辑20)Reinforcement Learning - 强化学习•Machine Learning (ML) - 机器学习1)Machine Learning (ML) - 机器学习2)Artificial Neural Network - 人工神经网络3)Deep Learning - 深度学习4)Supervised Learning - 有监督学习5)Unsupervised Learning - 无监督学习6)Reinforcement Learning - 强化学习7)Semi-Supervised Learning - 半监督学习8)Training Data - 训练数据9)Test Data - 测试数据10)Validation Data - 验证数据11)Feature - 特征12)Label - 标签13)Model - 模型14)Algorithm - 算法15)Regression - 回归16)Classification - 分类17)Clustering - 聚类18)Dimensionality Reduction - 降维19)Overfitting - 过拟合20)Underfitting - 欠拟合•Deep Learning (DL) - 深度学习1)Deep Learning - 深度学习2)Neural Network - 神经网络3)Artificial Neural Network (ANN) - 人工神经网络4)Convolutional Neural Network (CNN) - 卷积神经网络5)Recurrent Neural Network (RNN) - 循环神经网络6)Long Short-Term Memory (LSTM) - 长短期记忆网络7)Gated Recurrent Unit (GRU) - 门控循环单元8)Autoencoder - 自编码器9)Generative Adversarial Network (GAN) - 生成对抗网络10)Transfer Learning - 迁移学习11)Pre-trained Model - 预训练模型12)Fine-tuning - 微调13)Feature Extraction - 特征提取14)Activation Function - 激活函数15)Loss Function - 损失函数16)Gradient Descent - 梯度下降17)Backpropagation - 反向传播18)Epoch - 训练周期19)Batch Size - 批量大小20)Dropout - 丢弃法•Neural Network - 神经网络1)Neural Network - 神经网络2)Artificial Neural Network (ANN) - 人工神经网络3)Deep Neural Network (DNN) - 深度神经网络4)Convolutional Neural Network (CNN) - 卷积神经网络5)Recurrent Neural Network (RNN) - 循环神经网络6)Long Short-Term Memory (LSTM) - 长短期记忆网络7)Gated Recurrent Unit (GRU) - 门控循环单元8)Feedforward Neural Network - 前馈神经网络9)Multi-layer Perceptron (MLP) - 多层感知器10)Radial Basis Function Network (RBFN) - 径向基函数网络11)Hopfield Network - 霍普菲尔德网络12)Boltzmann Machine - 玻尔兹曼机13)Autoencoder - 自编码器14)Spiking Neural Network (SNN) - 脉冲神经网络15)Self-organizing Map (SOM) - 自组织映射16)Restricted Boltzmann Machine (RBM) - 受限玻尔兹曼机17)Hebbian Learning - 海比安学习18)Competitive Learning - 竞争学习19)Neuroevolutionary - 神经进化20)Neuron - 神经元•Algorithm - 算法1)Algorithm - 算法2)Supervised Learning Algorithm - 有监督学习算法3)Unsupervised Learning Algorithm - 无监督学习算法4)Reinforcement Learning Algorithm - 强化学习算法5)Classification Algorithm - 分类算法6)Regression Algorithm - 回归算法7)Clustering Algorithm - 聚类算法8)Dimensionality Reduction Algorithm - 降维算法9)Decision Tree Algorithm - 决策树算法10)Random Forest Algorithm - 随机森林算法11)Support Vector Machine (SVM) Algorithm - 支持向量机算法12)K-Nearest Neighbors (KNN) Algorithm - K近邻算法13)Naive Bayes Algorithm - 朴素贝叶斯算法14)Gradient Descent Algorithm - 梯度下降算法15)Genetic Algorithm - 遗传算法16)Neural Network Algorithm - 神经网络算法17)Deep Learning Algorithm - 深度学习算法18)Ensemble Learning Algorithm - 集成学习算法19)Reinforcement Learning Algorithm - 强化学习算法20)Metaheuristic Algorithm - 元启发式算法•Model - 模型1)Model - 模型2)Machine Learning Model - 机器学习模型3)Artificial Intelligence Model - 人工智能模型4)Predictive Model - 预测模型5)Classification Model - 分类模型6)Regression Model - 回归模型7)Generative Model - 生成模型8)Discriminative Model - 判别模型9)Probabilistic Model - 概率模型10)Statistical Model - 统计模型11)Neural Network Model - 神经网络模型12)Deep Learning Model - 深度学习模型13)Ensemble Model - 集成模型14)Reinforcement Learning Model - 强化学习模型15)Support Vector Machine (SVM) Model - 支持向量机模型16)Decision Tree Model - 决策树模型17)Random Forest Model - 随机森林模型18)Naive Bayes Model - 朴素贝叶斯模型19)Autoencoder Model - 自编码器模型20)Convolutional Neural Network (CNN) Model - 卷积神经网络模型•Dataset - 数据集1)Dataset - 数据集2)Training Dataset - 训练数据集3)Test Dataset - 测试数据集4)Validation Dataset - 验证数据集5)Balanced Dataset - 平衡数据集6)Imbalanced Dataset - 不平衡数据集7)Synthetic Dataset - 合成数据集8)Benchmark Dataset - 基准数据集9)Open Dataset - 开放数据集10)Labeled Dataset - 标记数据集11)Unlabeled Dataset - 未标记数据集12)Semi-Supervised Dataset - 半监督数据集13)Multiclass Dataset - 多分类数据集14)Feature Set - 特征集15)Data Augmentation - 数据增强16)Data Preprocessing - 数据预处理17)Missing Data - 缺失数据18)Outlier Detection - 异常值检测19)Data Imputation - 数据插补20)Metadata - 元数据•Training - 训练1)Training - 训练2)Training Data - 训练数据3)Training Phase - 训练阶段4)Training Set - 训练集5)Training Examples - 训练样本6)Training Instance - 训练实例7)Training Algorithm - 训练算法8)Training Model - 训练模型9)Training Process - 训练过程10)Training Loss - 训练损失11)Training Epoch - 训练周期12)Training Batch - 训练批次13)Online Training - 在线训练14)Offline Training - 离线训练15)Continuous Training - 连续训练16)Transfer Learning - 迁移学习17)Fine-Tuning - 微调18)Curriculum Learning - 课程学习19)Self-Supervised Learning - 自监督学习20)Active Learning - 主动学习•Testing - 测试1)Testing - 测试2)Test Data - 测试数据3)Test Set - 测试集4)Test Examples - 测试样本5)Test Instance - 测试实例6)Test Phase - 测试阶段7)Test Accuracy - 测试准确率8)Test Loss - 测试损失9)Test Error - 测试错误10)Test Metrics - 测试指标11)Test Suite - 测试套件12)Test Case - 测试用例13)Test Coverage - 测试覆盖率14)Cross-Validation - 交叉验证15)Holdout Validation - 留出验证16)K-Fold Cross-Validation - K折交叉验证17)Stratified Cross-Validation - 分层交叉验证18)Test Driven Development (TDD) - 测试驱动开发19)A/B Testing - A/B 测试20)Model Evaluation - 模型评估•Validation - 验证1)Validation - 验证2)Validation Data - 验证数据3)Validation Set - 验证集4)Validation Examples - 验证样本5)Validation Instance - 验证实例6)Validation Phase - 验证阶段7)Validation Accuracy - 验证准确率8)Validation Loss - 验证损失9)Validation Error - 验证错误10)Validation Metrics - 验证指标11)Cross-Validation - 交叉验证12)Holdout Validation - 留出验证13)K-Fold Cross-Validation - K折交叉验证14)Stratified Cross-Validation - 分层交叉验证15)Leave-One-Out Cross-Validation - 留一法交叉验证16)Validation Curve - 验证曲线17)Hyperparameter Validation - 超参数验证18)Model Validation - 模型验证19)Early Stopping - 提前停止20)Validation Strategy - 验证策略•Supervised Learning - 有监督学习1)Supervised Learning - 有监督学习2)Label - 标签3)Feature - 特征4)Target - 目标5)Training Labels - 训练标签6)Training Features - 训练特征7)Training Targets - 训练目标8)Training Examples - 训练样本9)Training Instance - 训练实例10)Regression - 回归11)Classification - 分类12)Predictor - 预测器13)Regression Model - 回归模型14)Classifier - 分类器15)Decision Tree - 决策树16)Support Vector Machine (SVM) - 支持向量机17)Neural Network - 神经网络18)Feature Engineering - 特征工程19)Model Evaluation - 模型评估20)Overfitting - 过拟合21)Underfitting - 欠拟合22)Bias-Variance Tradeoff - 偏差-方差权衡•Unsupervised Learning - 无监督学习1)Unsupervised Learning - 无监督学习2)Clustering - 聚类3)Dimensionality Reduction - 降维4)Anomaly Detection - 异常检测5)Association Rule Learning - 关联规则学习6)Feature Extraction - 特征提取7)Feature Selection - 特征选择8)K-Means - K均值9)Hierarchical Clustering - 层次聚类10)Density-Based Clustering - 基于密度的聚类11)Principal Component Analysis (PCA) - 主成分分析12)Independent Component Analysis (ICA) - 独立成分分析13)T-distributed Stochastic Neighbor Embedding (t-SNE) - t分布随机邻居嵌入14)Gaussian Mixture Model (GMM) - 高斯混合模型15)Self-Organizing Maps (SOM) - 自组织映射16)Autoencoder - 自动编码器17)Latent Variable - 潜变量18)Data Preprocessing - 数据预处理19)Outlier Detection - 异常值检测20)Clustering Algorithm - 聚类算法•Reinforcement Learning - 强化学习1)Reinforcement Learning - 强化学习2)Agent - 代理3)Environment - 环境4)State - 状态5)Action - 动作6)Reward - 奖励7)Policy - 策略8)Value Function - 值函数9)Q-Learning - Q学习10)Deep Q-Network (DQN) - 深度Q网络11)Policy Gradient - 策略梯度12)Actor-Critic - 演员-评论家13)Exploration - 探索14)Exploitation - 开发15)Temporal Difference (TD) - 时间差分16)Markov Decision Process (MDP) - 马尔可夫决策过程17)State-Action-Reward-State-Action (SARSA) - 状态-动作-奖励-状态-动作18)Policy Iteration - 策略迭代19)Value Iteration - 值迭代20)Monte Carlo Methods - 蒙特卡洛方法•Semi-Supervised Learning - 半监督学习1)Semi-Supervised Learning - 半监督学习2)Labeled Data - 有标签数据3)Unlabeled Data - 无标签数据4)Label Propagation - 标签传播5)Self-Training - 自训练6)Co-Training - 协同训练7)Transudative Learning - 传导学习8)Inductive Learning - 归纳学习9)Manifold Regularization - 流形正则化10)Graph-based Methods - 基于图的方法11)Cluster Assumption - 聚类假设12)Low-Density Separation - 低密度分离13)Semi-Supervised Support Vector Machines (S3VM) - 半监督支持向量机14)Expectation-Maximization (EM) - 期望最大化15)Co-EM - 协同期望最大化16)Entropy-Regularized EM - 熵正则化EM17)Mean Teacher - 平均教师18)Virtual Adversarial Training - 虚拟对抗训练19)Tri-training - 三重训练20)Mix Match - 混合匹配•Feature - 特征1)Feature - 特征2)Feature Engineering - 特征工程3)Feature Extraction - 特征提取4)Feature Selection - 特征选择5)Input Features - 输入特征6)Output Features - 输出特征7)Feature Vector - 特征向量8)Feature Space - 特征空间9)Feature Representation - 特征表示10)Feature Transformation - 特征转换11)Feature Importance - 特征重要性12)Feature Scaling - 特征缩放13)Feature Normalization - 特征归一化14)Feature Encoding - 特征编码15)Feature Fusion - 特征融合16)Feature Dimensionality Reduction - 特征维度减少17)Continuous Feature - 连续特征18)Categorical Feature - 分类特征19)Nominal Feature - 名义特征20)Ordinal Feature - 有序特征•Label - 标签1)Label - 标签2)Labeling - 标注3)Ground Truth - 地面真值4)Class Label - 类别标签5)Target Variable - 目标变量6)Labeling Scheme - 标注方案7)Multi-class Labeling - 多类别标注8)Binary Labeling - 二分类标注9)Label Noise - 标签噪声10)Labeling Error - 标注错误11)Label Propagation - 标签传播12)Unlabeled Data - 无标签数据13)Labeled Data - 有标签数据14)Semi-supervised Learning - 半监督学习15)Active Learning - 主动学习16)Weakly Supervised Learning - 弱监督学习17)Noisy Label Learning - 噪声标签学习18)Self-training - 自训练19)Crowdsourcing Labeling - 众包标注20)Label Smoothing - 标签平滑化•Prediction - 预测1)Prediction - 预测2)Forecasting - 预测3)Regression - 回归4)Classification - 分类5)Time Series Prediction - 时间序列预测6)Forecast Accuracy - 预测准确性7)Predictive Modeling - 预测建模8)Predictive Analytics - 预测分析9)Forecasting Method - 预测方法10)Predictive Performance - 预测性能11)Predictive Power - 预测能力12)Prediction Error - 预测误差13)Prediction Interval - 预测区间14)Prediction Model - 预测模型15)Predictive Uncertainty - 预测不确定性16)Forecast Horizon - 预测时间跨度17)Predictive Maintenance - 预测性维护18)Predictive Policing - 预测式警务19)Predictive Healthcare - 预测性医疗20)Predictive Maintenance - 预测性维护•Classification - 分类1)Classification - 分类2)Classifier - 分类器3)Class - 类别4)Classify - 对数据进行分类5)Class Label - 类别标签6)Binary Classification - 二元分类7)Multiclass Classification - 多类分类8)Class Probability - 类别概率9)Decision Boundary - 决策边界10)Decision Tree - 决策树11)Support Vector Machine (SVM) - 支持向量机12)K-Nearest Neighbors (KNN) - K最近邻算法13)Naive Bayes - 朴素贝叶斯14)Logistic Regression - 逻辑回归15)Random Forest - 随机森林16)Neural Network - 神经网络17)SoftMax Function - SoftMax函数18)One-vs-All (One-vs-Rest) - 一对多(一对剩余)19)Ensemble Learning - 集成学习20)Confusion Matrix - 混淆矩阵•Regression - 回归1)Regression Analysis - 回归分析2)Linear Regression - 线性回归3)Multiple Regression - 多元回归4)Polynomial Regression - 多项式回归5)Logistic Regression - 逻辑回归6)Ridge Regression - 岭回归7)Lasso Regression - Lasso回归8)Elastic Net Regression - 弹性网络回归9)Regression Coefficients - 回归系数10)Residuals - 残差11)Ordinary Least Squares (OLS) - 普通最小二乘法12)Ridge Regression Coefficient - 岭回归系数13)Lasso Regression Coefficient - Lasso回归系数14)Elastic Net Regression Coefficient - 弹性网络回归系数15)Regression Line - 回归线16)Prediction Error - 预测误差17)Regression Model - 回归模型18)Nonlinear Regression - 非线性回归19)Generalized Linear Models (GLM) - 广义线性模型20)Coefficient of Determination (R-squared) - 决定系数21)F-test - F检验22)Homoscedasticity - 同方差性23)Heteroscedasticity - 异方差性24)Autocorrelation - 自相关25)Multicollinearity - 多重共线性26)Outliers - 异常值27)Cross-validation - 交叉验证28)Feature Selection - 特征选择29)Feature Engineering - 特征工程30)Regularization - 正则化2.Neural Networks and Deep Learning (神经网络与深度学习)•Convolutional Neural Network (CNN) - 卷积神经网络1)Convolutional Neural Network (CNN) - 卷积神经网络2)Convolution Layer - 卷积层3)Feature Map - 特征图4)Convolution Operation - 卷积操作5)Stride - 步幅6)Padding - 填充7)Pooling Layer - 池化层8)Max Pooling - 最大池化9)Average Pooling - 平均池化10)Fully Connected Layer - 全连接层11)Activation Function - 激活函数12)Rectified Linear Unit (ReLU) - 线性修正单元13)Dropout - 随机失活14)Batch Normalization - 批量归一化15)Transfer Learning - 迁移学习16)Fine-Tuning - 微调17)Image Classification - 图像分类18)Object Detection - 物体检测19)Semantic Segmentation - 语义分割20)Instance Segmentation - 实例分割21)Generative Adversarial Network (GAN) - 生成对抗网络22)Image Generation - 图像生成23)Style Transfer - 风格迁移24)Convolutional Autoencoder - 卷积自编码器25)Recurrent Neural Network (RNN) - 循环神经网络•Recurrent Neural Network (RNN) - 循环神经网络1)Recurrent Neural Network (RNN) - 循环神经网络2)Long Short-Term Memory (LSTM) - 长短期记忆网络3)Gated Recurrent Unit (GRU) - 门控循环单元4)Sequence Modeling - 序列建模5)Time Series Prediction - 时间序列预测6)Natural Language Processing (NLP) - 自然语言处理7)Text Generation - 文本生成8)Sentiment Analysis - 情感分析9)Named Entity Recognition (NER) - 命名实体识别10)Part-of-Speech Tagging (POS Tagging) - 词性标注11)Sequence-to-Sequence (Seq2Seq) - 序列到序列12)Attention Mechanism - 注意力机制13)Encoder-Decoder Architecture - 编码器-解码器架构14)Bidirectional RNN - 双向循环神经网络15)Teacher Forcing - 强制教师法16)Backpropagation Through Time (BPTT) - 通过时间的反向传播17)Vanishing Gradient Problem - 梯度消失问题18)Exploding Gradient Problem - 梯度爆炸问题19)Language Modeling - 语言建模20)Speech Recognition - 语音识别•Long Short-Term Memory (LSTM) - 长短期记忆网络1)Long Short-Term Memory (LSTM) - 长短期记忆网络2)Cell State - 细胞状态3)Hidden State - 隐藏状态4)Forget Gate - 遗忘门5)Input Gate - 输入门6)Output Gate - 输出门7)Peephole Connections - 窥视孔连接8)Gated Recurrent Unit (GRU) - 门控循环单元9)Vanishing Gradient Problem - 梯度消失问题10)Exploding Gradient Problem - 梯度爆炸问题11)Sequence Modeling - 序列建模12)Time Series Prediction - 时间序列预测13)Natural Language Processing (NLP) - 自然语言处理14)Text Generation - 文本生成15)Sentiment Analysis - 情感分析16)Named Entity Recognition (NER) - 命名实体识别17)Part-of-Speech Tagging (POS Tagging) - 词性标注18)Attention Mechanism - 注意力机制19)Encoder-Decoder Architecture - 编码器-解码器架构20)Bidirectional LSTM - 双向长短期记忆网络•Attention Mechanism - 注意力机制1)Attention Mechanism - 注意力机制2)Self-Attention - 自注意力3)Multi-Head Attention - 多头注意力4)Transformer - 变换器5)Query - 查询6)Key - 键7)Value - 值8)Query-Value Attention - 查询-值注意力9)Dot-Product Attention - 点积注意力10)Scaled Dot-Product Attention - 缩放点积注意力11)Additive Attention - 加性注意力12)Context Vector - 上下文向量13)Attention Score - 注意力分数14)SoftMax Function - SoftMax函数15)Attention Weight - 注意力权重16)Global Attention - 全局注意力17)Local Attention - 局部注意力18)Positional Encoding - 位置编码19)Encoder-Decoder Attention - 编码器-解码器注意力20)Cross-Modal Attention - 跨模态注意力•Generative Adversarial Network (GAN) - 生成对抗网络1)Generative Adversarial Network (GAN) - 生成对抗网络2)Generator - 生成器3)Discriminator - 判别器4)Adversarial Training - 对抗训练5)Minimax Game - 极小极大博弈6)Nash Equilibrium - 纳什均衡7)Mode Collapse - 模式崩溃8)Training Stability - 训练稳定性9)Loss Function - 损失函数10)Discriminative Loss - 判别损失11)Generative Loss - 生成损失12)Wasserstein GAN (WGAN) - Wasserstein GAN(WGAN)13)Deep Convolutional GAN (DCGAN) - 深度卷积生成对抗网络(DCGAN)14)Conditional GAN (c GAN) - 条件生成对抗网络(c GAN)15)Style GAN - 风格生成对抗网络16)Cycle GAN - 循环生成对抗网络17)Progressive Growing GAN (PGGAN) - 渐进式增长生成对抗网络(PGGAN)18)Self-Attention GAN (SAGAN) - 自注意力生成对抗网络(SAGAN)19)Big GAN - 大规模生成对抗网络20)Adversarial Examples - 对抗样本•Encoder-Decoder - 编码器-解码器1)Encoder-Decoder Architecture - 编码器-解码器架构2)Encoder - 编码器3)Decoder - 解码器4)Sequence-to-Sequence Model (Seq2Seq) - 序列到序列模型5)State Vector - 状态向量6)Context Vector - 上下文向量7)Hidden State - 隐藏状态8)Attention Mechanism - 注意力机制9)Teacher Forcing - 强制教师法10)Beam Search - 束搜索11)Recurrent Neural Network (RNN) - 循环神经网络12)Long Short-Term Memory (LSTM) - 长短期记忆网络13)Gated Recurrent Unit (GRU) - 门控循环单元14)Bidirectional Encoder - 双向编码器15)Greedy Decoding - 贪婪解码16)Masking - 遮盖17)Dropout - 随机失活18)Embedding Layer - 嵌入层19)Cross-Entropy Loss - 交叉熵损失20)Tokenization - 令牌化•Transfer Learning - 迁移学习1)Transfer Learning - 迁移学习2)Source Domain - 源领域3)Target Domain - 目标领域4)Fine-Tuning - 微调5)Domain Adaptation - 领域自适应6)Pre-Trained Model - 预训练模型7)Feature Extraction - 特征提取8)Knowledge Transfer - 知识迁移9)Unsupervised Domain Adaptation - 无监督领域自适应10)Semi-Supervised Domain Adaptation - 半监督领域自适应11)Multi-Task Learning - 多任务学习12)Data Augmentation - 数据增强13)Task Transfer - 任务迁移14)Model Agnostic Meta-Learning (MAML) - 与模型无关的元学习(MAML)15)One-Shot Learning - 单样本学习16)Zero-Shot Learning - 零样本学习17)Few-Shot Learning - 少样本学习18)Knowledge Distillation - 知识蒸馏19)Representation Learning - 表征学习20)Adversarial Transfer Learning - 对抗迁移学习•Pre-trained Models - 预训练模型1)Pre-trained Model - 预训练模型2)Transfer Learning - 迁移学习3)Fine-Tuning - 微调4)Knowledge Transfer - 知识迁移5)Domain Adaptation - 领域自适应6)Feature Extraction - 特征提取7)Representation Learning - 表征学习8)Language Model - 语言模型9)Bidirectional Encoder Representations from Transformers (BERT) - 双向编码器结构转换器10)Generative Pre-trained Transformer (GPT) - 生成式预训练转换器11)Transformer-based Models - 基于转换器的模型12)Masked Language Model (MLM) - 掩蔽语言模型13)Cloze Task - 填空任务14)Tokenization - 令牌化15)Word Embeddings - 词嵌入16)Sentence Embeddings - 句子嵌入17)Contextual Embeddings - 上下文嵌入18)Self-Supervised Learning - 自监督学习19)Large-Scale Pre-trained Models - 大规模预训练模型•Loss Function - 损失函数1)Loss Function - 损失函数2)Mean Squared Error (MSE) - 均方误差3)Mean Absolute Error (MAE) - 平均绝对误差4)Cross-Entropy Loss - 交叉熵损失5)Binary Cross-Entropy Loss - 二元交叉熵损失6)Categorical Cross-Entropy Loss - 分类交叉熵损失7)Hinge Loss - 合页损失8)Huber Loss - Huber损失9)Wasserstein Distance - Wasserstein距离10)Triplet Loss - 三元组损失11)Contrastive Loss - 对比损失12)Dice Loss - Dice损失13)Focal Loss - 焦点损失14)GAN Loss - GAN损失15)Adversarial Loss - 对抗损失16)L1 Loss - L1损失17)L2 Loss - L2损失18)Huber Loss - Huber损失19)Quantile Loss - 分位数损失•Activation Function - 激活函数1)Activation Function - 激活函数2)Sigmoid Function - Sigmoid函数3)Hyperbolic Tangent Function (Tanh) - 双曲正切函数4)Rectified Linear Unit (Re LU) - 矩形线性单元5)Parametric Re LU (P Re LU) - 参数化Re LU6)Exponential Linear Unit (ELU) - 指数线性单元7)Swish Function - Swish函数8)Softplus Function - Soft plus函数9)Softmax Function - SoftMax函数10)Hard Tanh Function - 硬双曲正切函数11)Softsign Function - Softsign函数12)GELU (Gaussian Error Linear Unit) - GELU(高斯误差线性单元)13)Mish Function - Mish函数14)CELU (Continuous Exponential Linear Unit) - CELU(连续指数线性单元)15)Bent Identity Function - 弯曲恒等函数16)Gaussian Error Linear Units (GELUs) - 高斯误差线性单元17)Adaptive Piecewise Linear (APL) - 自适应分段线性函数18)Radial Basis Function (RBF) - 径向基函数•Backpropagation - 反向传播1)Backpropagation - 反向传播2)Gradient Descent - 梯度下降3)Partial Derivative - 偏导数4)Chain Rule - 链式法则5)Forward Pass - 前向传播6)Backward Pass - 反向传播7)Computational Graph - 计算图8)Neural Network - 神经网络9)Loss Function - 损失函数10)Gradient Calculation - 梯度计算11)Weight Update - 权重更新12)Activation Function - 激活函数13)Optimizer - 优化器14)Learning Rate - 学习率15)Mini-Batch Gradient Descent - 小批量梯度下降16)Stochastic Gradient Descent (SGD) - 随机梯度下降17)Batch Gradient Descent - 批量梯度下降18)Momentum - 动量19)Adam Optimizer - Adam优化器20)Learning Rate Decay - 学习率衰减•Gradient Descent - 梯度下降1)Gradient Descent - 梯度下降2)Stochastic Gradient Descent (SGD) - 随机梯度下降3)Mini-Batch Gradient Descent - 小批量梯度下降4)Batch Gradient Descent - 批量梯度下降5)Learning Rate - 学习率6)Momentum - 动量7)Adaptive Moment Estimation (Adam) - 自适应矩估计8)RMSprop - 均方根传播9)Learning Rate Schedule - 学习率调度10)Convergence - 收敛11)Divergence - 发散12)Adagrad - 自适应学习速率方法13)Adadelta - 自适应增量学习率方法14)Adamax - 自适应矩估计的扩展版本15)Nadam - Nesterov Accelerated Adaptive Moment Estimation16)Learning Rate Decay - 学习率衰减17)Step Size - 步长18)Conjugate Gradient Descent - 共轭梯度下降19)Line Search - 线搜索20)Newton's Method - 牛顿法•Learning Rate - 学习率1)Learning Rate - 学习率2)Adaptive Learning Rate - 自适应学习率3)Learning Rate Decay - 学习率衰减4)Initial Learning Rate - 初始学习率5)Step Size - 步长6)Momentum - 动量7)Exponential Decay - 指数衰减8)Annealing - 退火9)Cyclical Learning Rate - 循环学习率10)Learning Rate Schedule - 学习率调度11)Warm-up - 预热12)Learning Rate Policy - 学习率策略13)Learning Rate Annealing - 学习率退火14)Cosine Annealing - 余弦退火15)Gradient Clipping - 梯度裁剪16)Adapting Learning Rate - 适应学习率17)Learning Rate Multiplier - 学习率倍增器18)Learning Rate Reduction - 学习率降低19)Learning Rate Update - 学习率更新20)Scheduled Learning Rate - 定期学习率•Batch Size - 批量大小1)Batch Size - 批量大小2)Mini-Batch - 小批量3)Batch Gradient Descent - 批量梯度下降4)Stochastic Gradient Descent (SGD) - 随机梯度下降5)Mini-Batch Gradient Descent - 小批量梯度下降6)Online Learning - 在线学习7)Full-Batch - 全批量8)Data Batch - 数据批次9)Training Batch - 训练批次10)Batch Normalization - 批量归一化11)Batch-wise Optimization - 批量优化12)Batch Processing - 批量处理13)Batch Sampling - 批量采样14)Adaptive Batch Size - 自适应批量大小15)Batch Splitting - 批量分割16)Dynamic Batch Size - 动态批量大小17)Fixed Batch Size - 固定批量大小18)Batch-wise Inference - 批量推理19)Batch-wise Training - 批量训练20)Batch Shuffling - 批量洗牌•Epoch - 训练周期1)Training Epoch - 训练周期2)Epoch Size - 周期大小3)Early Stopping - 提前停止4)Validation Set - 验证集5)Training Set - 训练集6)Test Set - 测试集7)Overfitting - 过拟合8)Underfitting - 欠拟合9)Model Evaluation - 模型评估10)Model Selection - 模型选择11)Hyperparameter Tuning - 超参数调优12)Cross-Validation - 交叉验证13)K-fold Cross-Validation - K折交叉验证14)Stratified Cross-Validation - 分层交叉验证15)Leave-One-Out Cross-Validation (LOOCV) - 留一法交叉验证16)Grid Search - 网格搜索17)Random Search - 随机搜索18)Model Complexity - 模型复杂度19)Learning Curve - 学习曲线20)Convergence - 收敛3.Machine Learning Techniques and Algorithms (机器学习技术与算法)•Decision Tree - 决策树1)Decision Tree - 决策树2)Node - 节点3)Root Node - 根节点4)Leaf Node - 叶节点5)Internal Node - 内部节点6)Splitting Criterion - 分裂准则7)Gini Impurity - 基尼不纯度8)Entropy - 熵9)Information Gain - 信息增益10)Gain Ratio - 增益率11)Pruning - 剪枝12)Recursive Partitioning - 递归分割13)CART (Classification and Regression Trees) - 分类回归树14)ID3 (Iterative Dichotomiser 3) - 迭代二叉树315)C4.5 (successor of ID3) - C4.5(ID3的后继者)16)C5.0 (successor of C4.5) - C5.0(C4.5的后继者)17)Split Point - 分裂点18)Decision Boundary - 决策边界19)Pruned Tree - 剪枝后的树20)Decision Tree Ensemble - 决策树集成•Random Forest - 随机森林1)Random Forest - 随机森林2)Ensemble Learning - 集成学习3)Bootstrap Sampling - 自助采样4)Bagging (Bootstrap Aggregating) - 装袋法5)Out-of-Bag (OOB) Error - 袋外误差6)Feature Subset - 特征子集7)Decision Tree - 决策树8)Base Estimator - 基础估计器9)Tree Depth - 树深度10)Randomization - 随机化11)Majority Voting - 多数投票12)Feature Importance - 特征重要性13)OOB Score - 袋外得分14)Forest Size - 森林大小15)Max Features - 最大特征数16)Min Samples Split - 最小分裂样本数17)Min Samples Leaf - 最小叶节点样本数18)Gini Impurity - 基尼不纯度19)Entropy - 熵20)Variable Importance - 变量重要性•Support Vector Machine (SVM) - 支持向量机1)Support Vector Machine (SVM) - 支持向量机2)Hyperplane - 超平面3)Kernel Trick - 核技巧4)Kernel Function - 核函数5)Margin - 间隔6)Support Vectors - 支持向量7)Decision Boundary - 决策边界8)Maximum Margin Classifier - 最大间隔分类器9)Soft Margin Classifier - 软间隔分类器10) C Parameter - C参数11)Radial Basis Function (RBF) Kernel - 径向基函数核12)Polynomial Kernel - 多项式核13)Linear Kernel - 线性核14)Quadratic Kernel - 二次核15)Gaussian Kernel - 高斯核16)Regularization - 正则化17)Dual Problem - 对偶问题18)Primal Problem - 原始问题19)Kernelized SVM - 核化支持向量机20)Multiclass SVM - 多类支持向量机•K-Nearest Neighbors (KNN) - K-最近邻1)K-Nearest Neighbors (KNN) - K-最近邻2)Nearest Neighbor - 最近邻3)Distance Metric - 距离度量4)Euclidean Distance - 欧氏距离5)Manhattan Distance - 曼哈顿距离6)Minkowski Distance - 闵可夫斯基距离7)Cosine Similarity - 余弦相似度8)K Value - K值9)Majority Voting - 多数投票10)Weighted KNN - 加权KNN11)Radius Neighbors - 半径邻居12)Ball Tree - 球树13)KD Tree - KD树14)Locality-Sensitive Hashing (LSH) - 局部敏感哈希15)Curse of Dimensionality - 维度灾难16)Class Label - 类标签17)Training Set - 训练集18)Test Set - 测试集19)Validation Set - 验证集20)Cross-Validation - 交叉验证•Naive Bayes - 朴素贝叶斯1)Naive Bayes - 朴素贝叶斯2)Bayes' Theorem - 贝叶斯定理3)Prior Probability - 先验概率4)Posterior Probability - 后验概率5)Likelihood - 似然6)Class Conditional Probability - 类条件概率7)Feature Independence Assumption - 特征独立假设8)Multinomial Naive Bayes - 多项式朴素贝叶斯9)Gaussian Naive Bayes - 高斯朴素贝叶斯10)Bernoulli Naive Bayes - 伯努利朴素贝叶斯11)Laplace Smoothing - 拉普拉斯平滑12)Add-One Smoothing - 加一平滑13)Maximum A Posteriori (MAP) - 最大后验概率14)Maximum Likelihood Estimation (MLE) - 最大似然估计15)Classification - 分类16)Feature Vectors - 特征向量17)Training Set - 训练集18)Test Set - 测试集19)Class Label - 类标签20)Confusion Matrix - 混淆矩阵•Clustering - 聚类1)Clustering - 聚类2)Centroid - 质心3)Cluster Analysis - 聚类分析4)Partitioning Clustering - 划分式聚类5)Hierarchical Clustering - 层次聚类6)Density-Based Clustering - 基于密度的聚类7)K-Means Clustering - K均值聚类8)K-Medoids Clustering - K中心点聚类9)DBSCAN (Density-Based Spatial Clustering of Applications with Noise) - 基于密度的空间聚类算法10)Agglomerative Clustering - 聚合式聚类11)Dendrogram - 系统树图12)Silhouette Score - 轮廓系数13)Elbow Method - 肘部法则14)Clustering Validation - 聚类验证15)Intra-cluster Distance - 类内距离16)Inter-cluster Distance - 类间距离17)Cluster Cohesion - 类内连贯性18)Cluster Separation - 类间分离度19)Cluster Assignment - 聚类分配20)Cluster Label - 聚类标签•K-Means - K-均值1)K-Means - K-均值2)Centroid - 质心3)Cluster - 聚类4)Cluster Center - 聚类中心5)Cluster Assignment - 聚类分配6)Cluster Analysis - 聚类分析7)K Value - K值8)Elbow Method - 肘部法则9)Inertia - 惯性10)Silhouette Score - 轮廓系数11)Convergence - 收敛12)Initialization - 初始化13)Euclidean Distance - 欧氏距离14)Manhattan Distance - 曼哈顿距离15)Distance Metric - 距离度量16)Cluster Radius - 聚类半径17)Within-Cluster Variation - 类内变异18)Cluster Quality - 聚类质量19)Clustering Algorithm - 聚类算法20)Clustering Validation - 聚类验证•Dimensionality Reduction - 降维1)Dimensionality Reduction - 降维2)Feature Extraction - 特征提取3)Feature Selection - 特征选择4)Principal Component Analysis (PCA) - 主成分分析5)Singular Value Decomposition (SVD) - 奇异值分解6)Linear Discriminant Analysis (LDA) - 线性判别分析7)t-Distributed Stochastic Neighbor Embedding (t-SNE) - t-分布随机邻域嵌入8)Autoencoder - 自编码器9)Manifold Learning - 流形学习10)Locally Linear Embedding (LLE) - 局部线性嵌入11)Isomap - 等度量映射12)Uniform Manifold Approximation and Projection (UMAP) - 均匀流形逼近与投影13)Kernel PCA - 核主成分分析14)Non-negative Matrix Factorization (NMF) - 非负矩阵分解15)Independent Component Analysis (ICA) - 独立成分分析16)Variational Autoencoder (VAE) - 变分自编码器17)Sparse Coding - 稀疏编码18)Random Projection - 随机投影19)Neighborhood Preserving Embedding (NPE) - 保持邻域结构的嵌入20)Curvilinear Component Analysis (CCA) - 曲线成分分析•Principal Component Analysis (PCA) - 主成分分析1)Principal Component Analysis (PCA) - 主成分分析2)Eigenvector - 特征向量3)Eigenvalue - 特征值4)Covariance Matrix - 协方差矩阵。
人工智能深度学习技术练习(习题卷22)
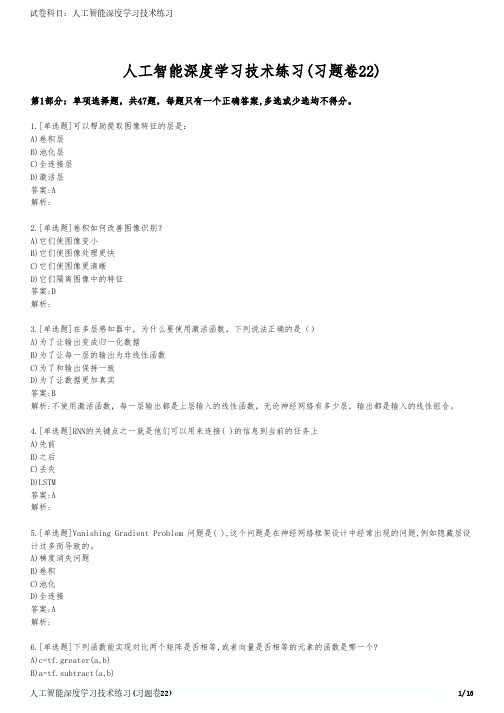
人工智能深度学习技术练习(习题卷22)第1部分:单项选择题,共47题,每题只有一个正确答案,多选或少选均不得分。
1.[单选题]可以帮助提取图像特征的层是:A)卷积层B)池化层C)全连接层D)激活层答案:A解析:2.[单选题]卷积如何改善图像识别?A)它们使图像变小B)它们使图像处理更快C)它们使图像更清晰D)它们隔离图像中的特征答案:D解析:3.[单选题]在多层感知器中,为什么要使用激活函数,下列说法正确的是()A)为了让输出变成归一化数据B)为了让每一层的输出为非线性函数C)为了和输出保持一致D)为了让数据更加真实答案:B解析:不使用激活函数,每一层输出都是上层输入的线性函数,无论神经网络有多少层,输出都是输入的线性组合。
4.[单选题]RNN的关键点之一就是他们可以用来连接( )的信息到当前的任务上A)先前B)之后C)丢失D)LSTM答案:A解析:5.[单选题]Vanishing Gradient Problem 问题是( ),这个问题是在神经网络框架设计中经常出现的问题,例如隐藏层设计过多而导致的。
A)梯度消失问题B)卷积C)池化D)全连接答案:A解析:6.[单选题]下列函数能实现对比两个矩阵是否相等,或者向量是否相等的元素的函数是哪一个?A)c=tf.greater(a,b)C)b=tf.equal(a,b)D)d=tf.matmul(a,b)答案:C解析:7.[单选题]深度学习通过多层处理,初始的特征成为()A)低层特征B)高层特征C)中层特征D)浅层特征答案:B解析:8.[单选题]常用的激活函数RELU,其常用调用语句为那一个()A)h_conv1 = tf.nn.relu( conv_ret1 )B)h_conv1 = tf.nn.dropout( conv_ret1 )C)h_conv1 = tf.nn.lrn( conv_ret1 )D)h_conv1 = tf.nn.l2_loss( conv_ret1 )答案:A解析:9.[单选题]动量梯度算法,是通过学习率和()控制梯度下降的A)指数加权平均数B)局部平均值C)全局平局值D)方差答案:A解析:10.[单选题]如图所示,当开始训练时,误差一直很高,这是因为神经网络在往全局最小值前进之前一直被卡在局部最小值里。
基于云-边协同的配电网快速供电恢复智能决策方法

第51卷第19期电力系统保护与控制Vol.51 No.19 2023年10月1日Power System Protection and Control Oct. 1, 2023 DOI: 10.19783/ki.pspc.221918基于云-边协同的配电网快速供电恢复智能决策方法蔡田田1,姚 浩1,杨英杰1,张子麒2,冀浩然2,李 鹏2(1.南方电网数字电网研究院有限公司,广东 广州 510700;2.智能电网教育部重点实验室(天津大学),天津 300072)摘要:分布式电源高渗透率接入对配电网故障自愈能力提出了更高的要求。
基于模型的供电恢复方法利用精准的网络参数构建优化模型,可以实现供电恢复策略的准确制定。
但在配电网实际运行中,精准的配电网络参数往往难以获取,导致基于模型的供电恢复方法应用受限。
云-边协同运行模式可作为配电网快速供电恢复的一种实现方案。
提出一种基于云-边协同的配电网快速供电恢复智能决策方法。
首先,在云端基于图卷积神经网络建立配电网快速供电恢复智能决策模型,包括网络重构模块和潮流模拟模块。
当故障发生后,云端利用网络重构模块,快速制定网络重构策略,经过破圈法/避圈法验校后下发至配电网边缘侧的边缘计算装置。
边缘侧根据云端的网络重构策略利用潮流模拟模块就地制定负荷恢复策略,实现系统的快速供电恢复。
最后,依托改进的IEEE33节点配电网算例对所提模型进行分析,验证了所提方法可有效提升配电网的供电恢复能力。
关键词:配电网;云-边协同;供电恢复;分布式电源;图卷积神经网络Cloud-edge collaboration-based supply restoration intelligent decision-making methodCAI Tiantian1, YAO Hao1, YANG Yingjie1, ZHANG Ziqi2, JI Haoran2, LI Peng2(1. Digital Grid Research Institute, China Southern Power Grid, Guangzhou 510700, China; 2. Key Laboratory ofSmart Grid of Ministry of Education (Tianjin University), Tianjin 300072, China) Abstract: The high-penetration integration of distributed generators (DGs) makes higher demands on the self-healing ability of a distribution network. The model-based supply restoration methods build the optimization model with accurate network parameters, which can realize the accurate formulation of restoration strategies. However, the accurate network parameters are often difficult to acquire in practical operation, which may limit the application of the model-based methods. The cloud-edge collaboration control mode can be used as an implementation scheme for fast supply restoration.A fast supply restoration intelligent decision-making method for distribution network based on cloud-edge collaborationis proposed. First, an intelligent decision-making model is established based on a graph convolutional neural network (GCN) on the cloud, containing network reconstruction and power flow simulation modules. When a failure occurs, the network reconstruction module is used to customize the reconstruction strategy on the cloud. After correction by loop-breaking/loop-avoiding method, the reconstruction strategy will be sent to the edge calculation device of distribution network edge side. With the power flow simulation module, the supply recovery strategy can be determined rapidly at the edge side to realize a fast supply restoration. Finally, the proposed strategy is analyzed using the modified IEEE 33-node system. The results show that the proposed method can effectively improve the supply restoration ability of a distribution network.This work is supported by the National Key Research and Development Program of China (No. 2020YFB0906000 and No. 2020YFB0906002).Key words: distribution network; cloud-edge collaboration; supply restoration; distributed generators (DGs); graph convolutional neural networks (GCN)0 引言配电网中设备种类繁多、控制策略复杂[1],尤基金项目:国家重点研发计划项目资助(2020YFB0906000,2020YFB0906002) 其是当分布式电源(distributed generators, DGs)高渗透率接入后,配电网的运行特性发生巨大变化[2],对配电网故障自愈能力提出了更高的要求[3]。
智能决策支持系统的研究与优化
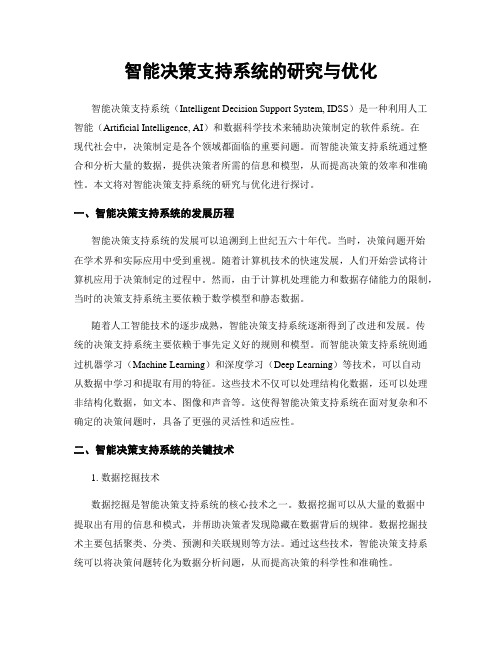
智能决策支持系统的研究与优化智能决策支持系统(Intelligent Decision Support System, IDSS)是一种利用人工智能(Artificial Intelligence, AI)和数据科学技术来辅助决策制定的软件系统。
在现代社会中,决策制定是各个领域都面临的重要问题。
而智能决策支持系统通过整合和分析大量的数据,提供决策者所需的信息和模型,从而提高决策的效率和准确性。
本文将对智能决策支持系统的研究与优化进行探讨。
一、智能决策支持系统的发展历程智能决策支持系统的发展可以追溯到上世纪五六十年代。
当时,决策问题开始在学术界和实际应用中受到重视。
随着计算机技术的快速发展,人们开始尝试将计算机应用于决策制定的过程中。
然而,由于计算机处理能力和数据存储能力的限制,当时的决策支持系统主要依赖于数学模型和静态数据。
随着人工智能技术的逐步成熟,智能决策支持系统逐渐得到了改进和发展。
传统的决策支持系统主要依赖于事先定义好的规则和模型。
而智能决策支持系统则通过机器学习(Machine Learning)和深度学习(Deep Learning)等技术,可以自动从数据中学习和提取有用的特征。
这些技术不仅可以处理结构化数据,还可以处理非结构化数据,如文本、图像和声音等。
这使得智能决策支持系统在面对复杂和不确定的决策问题时,具备了更强的灵活性和适应性。
二、智能决策支持系统的关键技术1. 数据挖掘技术数据挖掘是智能决策支持系统的核心技术之一。
数据挖掘可以从大量的数据中提取出有用的信息和模式,并帮助决策者发现隐藏在数据背后的规律。
数据挖掘技术主要包括聚类、分类、预测和关联规则等方法。
通过这些技术,智能决策支持系统可以将决策问题转化为数据分析问题,从而提高决策的科学性和准确性。
2. 机器学习技术机器学习是智能决策支持系统另一个重要的技术。
机器学习通过让计算机从数据中学习和优化模型,来达到自主处理决策问题的目的。
中期答辩外文翻译

模糊系统与数学131(2002)101-106一个基于方案模糊信息的多属性决策方法Zhi-Ping Fan a,b , Jian Ma b,∗ , Quan Zhang b信息与决策部门,工商管理学院,东北大学,沈阳110006,中国部门的信息系统,香港大学,83Tat中港大道,九龙,香港摘要本文研究了对多属性决策方案的问题。
本文提出了一种新的方法来解决决策问题,也就是决策者对于他/她的偏好方案的一个模糊的关系。
对re.ect决策者的偏好信息,构建一个最优化模型来评估属性权重然后选择最理想的替代品。
最后,用一个例子来说明所提出的问题。
2001由Elsevier Science公司出版。
关键词:多属性决策;模糊偏好信息;方案排序1 引言在多属性决策(MADM)问题里,决策者往往需要选择或者排序非均匀和相互冲突的属性[3,14]。
MADM是在现实世界中许多的情况下出现的一个决策性问题[3,6,14,19,20],例如,在生产计划的问题上,生产速度,质量和运营成本都要考虑选择满意的方案。
大量的研究工作一直在进行一个多属性决策问题,其中之一的研究热点就是模糊集理论的应用解决不精确信息的多属性决策问题以[3,4,24]为代表的模糊条款。
通讯作者。
电话: +852-2788-8514 传真: +852-2784-4198电子邮件地址: isjian@.hk (J. Ma).0165-0114/01/$-见前页c 2001 由Elsevier科学有限公司出版PII: S0165-0114(01)00258-5在多属性决策问题里,决策者的偏好信息经常被用来排列替代品,然而,决策者的判断因形式和深度而异。
决策者可能不代表他/她所有喜欢的,或者可能代表他/她的属性或替代偏好的形式。
决策者的判断能力也各种各样。
解决多属性决策问题可以分成三类,根据决策者给出diEerent形式的偏好信息[3,14]:(1)无偏好信息[2,8,12]的方法,(2)与属性[1,5,7,9,22]信息;(3)对替代品[3,14,16,17,20,23]信息的方法。
模拟ai英文面试题目及答案
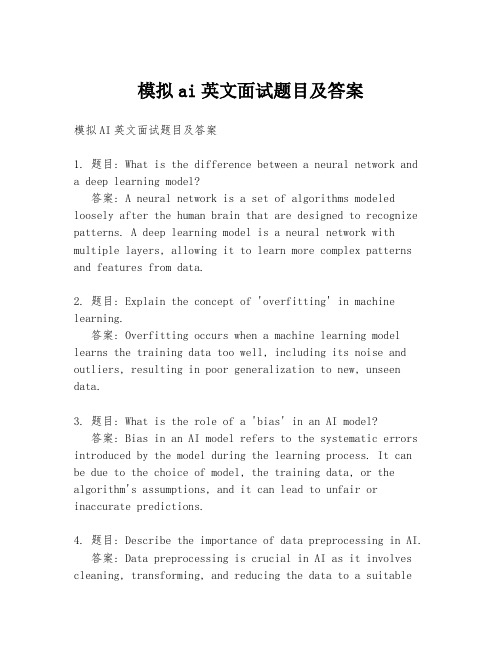
模拟ai英文面试题目及答案模拟AI英文面试题目及答案1. 题目: What is the difference between a neural network anda deep learning model?答案: A neural network is a set of algorithms modeled loosely after the human brain that are designed to recognize patterns. A deep learning model is a neural network with multiple layers, allowing it to learn more complex patterns and features from data.2. 题目: Explain the concept of 'overfitting' in machine learning.答案: Overfitting occurs when a machine learning model learns the training data too well, including its noise and outliers, resulting in poor generalization to new, unseen data.3. 题目: What is the role of a 'bias' in an AI model?答案: Bias in an AI model refers to the systematic errors introduced by the model during the learning process. It can be due to the choice of model, the training data, or the algorithm's assumptions, and it can lead to unfair or inaccurate predictions.4. 题目: Describe the importance of data preprocessing in AI.答案: Data preprocessing is crucial in AI as it involves cleaning, transforming, and reducing the data to a suitableformat for the model to learn effectively. Proper preprocessing can significantly improve the performance of AI models by ensuring that the input data is relevant, accurate, and free from noise.5. 题目: How does reinforcement learning differ from supervised learning?答案: Reinforcement learning is a type of machine learning where an agent learns to make decisions by performing actions in an environment to maximize a reward signal. It differs from supervised learning, where the model learns from labeled data to predict outcomes based on input features.6. 题目: What is the purpose of a 'convolutional neural network' (CNN)?答案: A convolutional neural network (CNN) is a type of deep learning model that is particularly effective for processing data with a grid-like topology, such as images. CNNs use convolutional layers to automatically and adaptively learn spatial hierarchies of features from input images.7. 题目: Explain the concept of 'feature extraction' in AI.答案: Feature extraction in AI is the process of identifying and extracting relevant pieces of information from the raw data. It is a crucial step in many machine learning algorithms, as it helps to reduce the dimensionality of the data and to focus on the most informative aspects that can be used to make predictions or classifications.8. 题目: What is the significance of 'gradient descent' in training AI models?答案: Gradient descent is an optimization algorithm used to minimize a function by iteratively moving in the direction of steepest descent as defined by the negative of the gradient. In the context of AI, it is used to minimize the loss function of a model, thus refining the model's parameters to improve its accuracy.9. 题目: How does 'transfer learning' work in AI?答案: Transfer learning is a technique where a pre-trained model is used as the starting point for learning a new task. It leverages the knowledge gained from one problem to improve performance on a different but related problem, reducing the need for large amounts of labeled data and computational resources.10. 题目: What is the role of 'regularization' in preventing overfitting?答案: Regularization is a technique used to prevent overfitting by adding a penalty term to the loss function, which discourages overly complex models. It helps to control the model's capacity, forcing it to generalize better to new data by not fitting too closely to the training data.。
工商管理专业专业术语汇总
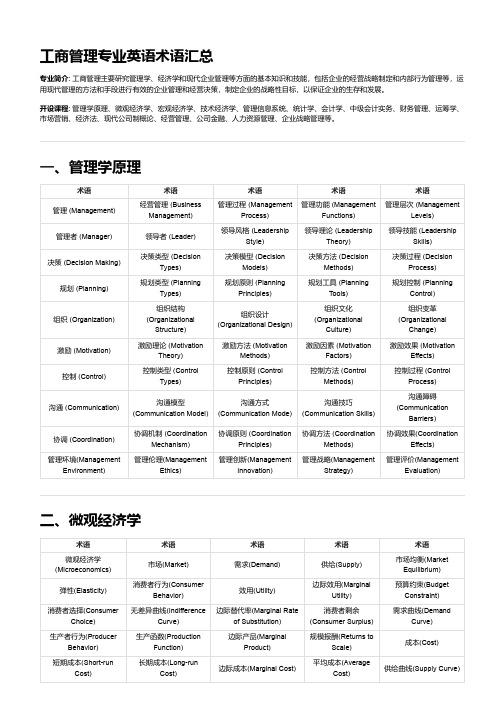
工商管理专业英语术语汇总专业简介: 工商管理主要研究管理学、经济学和现代企业管理等方面的基本知识和技能,包括企业的经营战略制定和内部行为管理等,运用现代管理的方法和手段进行有效的企业管理和经营决策,制定企业的战略性目标,以保证企业的生存和发展。
开设课程: 管理学原理、微观经济学、宏观经济学、技术经济学、管理信息系统、统计学、会计学、中级会计实务、财务管理、运筹学、市场营销、经济法、现代公司制概论、经营管理、公司金融、人力资源管理、企业战略管理等。
一、管理学原理术语术语术语术语术语管理 (Management)经营管理 (BusinessManagement)管理过程 (ManagementProcess)管理功能 (ManagementFunctions)管理层次 (ManagementLevels)管理者 (Manager)领导者 (Leader)领导风格 (LeadershipStyle)领导理论 (LeadershipTheory)领导技能 (LeadershipSkills)决策 (Decision Making)决策类型 (DecisionTypes)决策模型 (DecisionModels)决策方法 (DecisionMethods)决策过程 (DecisionProcess)规划 (Planning)规划类型 (PlanningTypes)规划原则 (PlanningPrinciples)规划工具 (PlanningTools)规划控制 (PlanningControl)组织 (Organization)组织结构(OrganizationalStructure)组织设计(Organizational Design)组织文化(OrganizationalCulture)组织变革(OrganizationalChange)激励 (Motivation)激励理论 (MotivationTheory)激励方法 (MotivationMethods)激励因素 (MotivationFactors)激励效果 (MotivationEffects)控制 (Control)控制类型 (ControlTypes)控制原则 (ControlPrinciples)控制方法 (ControlMethods)控制过程 (ControlProcess)沟通 (Communication)沟通模型(Communication Model)沟通方式(Communication Mode)沟通技巧(Communication Skills)沟通障碍(CommunicationBarriers)协调 (Coordination)协调机制 (CoordinationMechanism)协调原则 (CoordinationPrinciples)协调方法 (CoordinationMethods)协调效果(CoordinationEffects)管理环境(Management Environment)管理伦理(ManagementEthics)管理创新(ManagementInnovation)管理战略(ManagementStrategy)管理评价(ManagementEvaluation)二、微观经济学术语术语术语术语术语微观经济学(Microeconomics)市场(Market)需求(Demand)供给(Supply)市场均衡(MarketEquilibrium)弹性(Elasticity)消费者行为(ConsumerBehavior)效用(Utility)边际效用(MarginalUtility)预算约束(BudgetConstraint)消费者选择(ConsumerChoice)无差异曲线(IndifferenceCurve)边际替代率(Marginal Rateof Substitution)消费者剩余(Consumer Surplus)需求曲线(DemandCurve)生产者行为(ProducerBehavior)生产函数(ProductionFunction)边际产品(MarginalProduct)规模报酬(Returns toScale)成本(Cost)短期成本(Short-runCost)长期成本(Long-runCost)边际成本(Marginal Cost)平均成本(AverageCost)供给曲线(Supply Curve)市场结构(Market Structure)完全竞争(PerfectCompetition)垄断(Monopoly)寡头(Oligopoly)垄断竞争(MonopolisticCompetition)价格歧视(Price Discrimination)博弈论(Game Theory)纳什均衡(NashEquilibrium)策略(Strategy)支配策略(DominantStrategy)外部性(Externality)公共品(Public Good)信息不对称(AsymmetricInformation)逆向选择(AdverseSelection)道德风险(Moral Hazard)市场失灵(MarketFailure)政府干预(GovernmentIntervention)税收(Taxation)补贴(Subsidy)福利经济学(WelfareEconomics)三、宏观经济学术语术语术语术语术语宏观经济学(Macroeconomics)国民收入(NationalIncome)国内生产总值(GrossDomestic Product)国民生产总值(GrossNational Product)消费者物价指数(Consumer PriceIndex)通货膨胀(Inflation)失业(Unemployment)菲利普斯曲线(Phillips Curve)经济增长(EconomicGrowth)经济周期(EconomicCycle)经济波动(Economic Fluctuation)经济危机(EconomicCrisis)经济衰退(EconomicRecession)经济萧条(EconomicDepression)经济恢复(EconomicRecovery)总需求(Total Demand)总供给(Total Supply)总需求总供给模型(Aggregate Demand andAggregate Supply Model)短期均衡(Short-runEquilibrium)长期均衡(Long-runEquilibrium)消费(Consumption)投资(Investment)政府支出(GovernmentSpending)净出口(Net Exports)国民收入恒等式(National IncomeIdentity)消费函数(Consumption Function)边际消费倾向(MarginalPropensity to Consume)投资函数(InvestmentFunction)边际效率投资(MarginalEfficiency ofInvestment)多重效应(MultiplierEffect)货币(Money)货币供应量(MoneySupply)货币需求量(Money Demand)货币市场平衡(MoneyMarket Equilibrium)利率(Rate ofInterest)货币政策(MonetaryPolicy)中央银行(Central Bank)开放市场操作(Open MarketOperations)存款准备金率(ReserveRequirement Ratio)贴现率(DiscountRate)财政政策(FiscalPolicy)政府预算(GovernmentBudget)财政赤字(Fiscal Deficit)公共债务(Public Debt)自动稳定器(AutomaticStabilizer)国际贸易(InternationalTrade)国际收支(Balance ofPayments)汇率(Exchange Rate)贸易政策(Trade Policy)汇率制度(ExchangeRate Regime)四、技术经济学术语术语术语术语术语技术经济学(Technical Economics)技术(Technology)技术创新(TechnologicalInnovation)技术进步(TechnologicalProgress)技术水平(TechnologicalLevel)技术选择(Technological Choice)技术评价(TechnologicalEvaluation)技术效益(TechnologicalBenefit)技术风险(TechnologicalRisk)技术转让(TechnologicalTransfer)技术方案(TechnicalScheme)技术参数(TechnicalParameter)技术指标(TechnicalIndicator)技术标准(TechnicalStandard)技术规范(TechnicalSpecification)工程项目(EngineeringProject)工程设计(EngineeringDesign)工程造价(EngineeringCost)工程投资(EngineeringInvestment)工程回收期(EngineeringPayback Period)工程效益分析(Engineering BenefitAnalysis)工程经济效益(Engineering EconomicBenefit)工程社会效益(Engineering SocialBenefit)工程环境效益(EngineeringEnvironmental Benefit)工程综合效益(EngineeringComprehensive Benefit)资金(Fund)资金需求(FundDemand)资金来源(FundSource)资金成本(Fund Cost)资金利润率(Fund ProfitRate)现金流量(Cash Flow)现金流量表(Cash FlowStatement)现金流量分析(CashFlow Analysis)现金流量折现(Discounted Cash Flow)现值净值(Net PresentValue)内部收益率(Internal Rate of Return)敏感性分析(SensitivityAnalysis)变动成本(MarginalCost)变动收益(MarginalRevenue)边际分析(MarginalAnalysis)五、管理信息系统术语术语术语术语术语管理信息系统(Management Information System)信息系统(InformationSystem)信息技术(InformationTechnology)信息资源管理(InformationResource Management)信息系统规划(Information SystemPlanning)信息需求分析(Information Requirement Analysis)信息系统设计(Information SystemDesign)信息系统开发(Information SystemDevelopment)信息系统实施(InformationSystem Implementation)信息系统维护(Information SystemMaintenance)数据(Data)数据库(Database)数据库管理系统(DatabaseManagement System)数据模型(Data Model)数据字典(DataDictionary)数据仓库(Data Warehouse)数据挖掘(DataMining)数据分析(DataAnalysis)数据可视化(DataVisualization)数据安全(Data Security)网络(Network)计算机网络(Computer Network)网络拓扑(NetworkTopology)网络协议(NetworkProtocol)网络架构(NetworkArchitecture)局域网(Local AreaNetwork)广域网(Wide AreaNetwork)因特网(Internet)互联网(Internet of Things)网络安全(NetworkSecurity)系统(System)计算机系统(Computer System)操作系统(OperationSystem)系统分析(SystemAnalysis)系统设计(SystemDesign)软件(Software)软件工程(SoftwareEngineering)软件生命周期(SoftwareLife Cycle)软件开发方法(SoftwareDevelopment Method)软件质量(SoftwareQuality)硬件(Hardware)计算机硬件(ComputerHardware)输入设备(Input Device)输出设备(Output Device)存储设备(StorageDevice)处理器(Processor)内存(Memory)总线(Bus)接口(Interface)外设(Peripheral)人工智能(Artificial Intelligence)机器学习(MachineLearning)深度学习(DeepLearning)神经网络(Neural Network)自然语言处理(NaturalLanguage Processing)专家系统(Expert System)智能代理(IntelligentAgent)模糊逻辑(Fuzzy Logic)遗传算法(GeneticAlgorithm)人工神经网络(ArtificialNeural Network)电子商务(E-commerce)电子商务模式(E-commerce Model)电子商务平台(E-commerce Platform)电子支付(ElectronicPayment)电子商务安全(E-commerce Security)电子政务(E-government)电子政务模式(E-government Model)电子政务平台(E-government Platform)电子政务服务(E-government Service)电子政务安全(E-government Security)知识管理(Knowledge Management)知识(Knowledge)知识类型(KnowledgeType)知识获取(KnowledgeAcquisition)知识表示(KnowledgeRepresentation)知识组织(Knowledge Organization)知识共享(KnowledgeSharing)知识创新(KnowledgeInnovation)知识库(Knowledge Base)知识系统(KnowledgeSystem)六、统计学术语术语术语术语术语统计学(Statistics)统计方法(StatisticalMethod)统计推断(StatisticalInference)统计分析(StatisticalAnalysis)统计软件(StatisticalSoftware)数据(Data)数据类型(Data Type)数据来源(Data Source)数据收集(DataCollection)数据清洗(Data Cleaning)数据描述(Data Description)数据展示(DataPresentation)数据摘要(DataSummary)数据分布(DataDistribution)数据变换(DataTransformation)变量(Variable)变量类型(Variable Type)自变量(IndependentVariable)因变量(DependentVariable)控制变量(Control Variable)单变量分析(UnivariateAnalysis)双变量分析(BivariateAnalysis)多变量分析(MultivariateAnalysis)相关分析(CorrelationAnalysis)回归分析(RegressionAnalysis)随机变量(RandomVariable)概率(Probability)概率分布(ProbabilityDistribution)期望值(ExpectedValue)方差(Variance)标准差(StandardDeviation)均值(Mean)中位数(Median)众数(Mode)四分位数(Quartile)极差(Range)变异系数(Coefficient ofVariation)偏度(Skewness)峰度(Kurtosis)正态分布(NormalDistribution)抽样(Sampling)抽样方法(SamplingMethod)抽样误差(SamplingError)抽样分布(SamplingDistribution)中心极限定理(Central LimitTheorem)点估计(Point Estimation)区间估计(IntervalEstimation)置信区间(ConfidenceInterval)置信水平(ConfidenceLevel)标准误差(Standard Error)假设检验(HypothesisTesting)原假设(Null Hypothesis)备择假设(AlternativeHypothesis)显著性水平(Significance Level)拒绝域(Rejection Region)检验统计量(Test Statistic)P值(P-value)类型一错误(Type IError)类型二错误(Type IIError)功效(Power)参数检验(ParametricTest)非参数检验(Nonparametric Test)单样本检验(One-sample Test)双样本检验(Two-sample Test)配对样本检验(Paired-sample Test)Z检验(Z-test)T检验(T-test)F检验(F-test)卡方检验(Chi-squareTest)方差分析(Analysis ofVariance)七、会计学术语术语术语术语术语会计学(Accounting)会计对象(AccountingObject)会计要素(AccountingElement)会计科目(Accounting Subject)会计方程(AccountingEquation)会计核算(Accounting Calculation)会计原则(AccountingPrinciple)会计假设(AccountingAssumption)会计政策(Accounting Policy)会计准则(AccountingStandard)会计期间(AccountingPeriod)会计年度(AccountingYear)会计报告期(AccountingReporting Period)会计循环(Accounting Cycle)会计业务(AccountingBusiness)记账(Bookkeeping)记账方法(BookkeepingMethod)记账凭证(BookkeepingVoucher)记账账簿(Bookkeeping Book)记账账户(BookkeepingAccount)记账分录(Bookkeeping Entry)借贷记账法(Double-entryBookkeeping Method)借方(Debit Side)贷方(Credit Side)借贷平衡(Balance of Debitand Credit)会计报表(Accounting Statement)资产负债表(BalanceSheet)利润表(IncomeStatement)现金流量表(CashFlow Statement)所有者权益变动表(Statementof Changes in Owner'sEquity)会计科学(AccountingScience)会计理论(AccountingTheory)会计方法(AccountingMethod)会计技术(AccountingTechnique)会计创新(AccountingInnovation)财务会计(Financial Accounting)管理会计(ManagementAccounting)成本会计(CostAccounting)审计会计(AuditingAccounting)税务会计(Tax Accounting)资产(Asset)负债(Liability)所有者权益(Owner'sEquity)收入(Income)费用(Expense)收益(Revenue)损失(Loss)利润(Profit)毛利(Gross Profit)净利(Net Profit)存货(Inventory)应收账款(AccountsReceivable)预付账款(PrepaidExpenses)固定资产(FixedAssets)无形资产(Intangible Assets)应付账款(AccountsPayable)预收账款(UnearnedRevenue)长期负债(Long-termLiabilities)资本(Capital)留存收益(Retained Earnings)折旧(Depreciation)摊销(Amortization)减值(Impairment)计提(Accrual)结转(Carryover)对冲(Hedging)杠杆(Leverage)财务比率(FinancialRatio)资本结构(CapitalStructure)资本预算(Capital Budgeting)八、中级会计实务术语术语术语术语术语会计 (Accounting)资产 (Asset)负债 (Liability)所有者权益 (Owner'sEquity)收入 (Revenue)费用 (Expense)损益 (Profit or Loss)现金流量 (Cash Flow)资产负债表 (BalanceSheet)利润表 (IncomeStatement)现金流量表 (Cash FlowStatement)所有者权益变动表(Statement of Changesin Owner's Equity)附注 (Notes)记账凭证 (Voucher)记账方法 (AccountingMethod)原始凭证 (Original Document)记账分录 (Journal Entry)总分类账 (GeneralLedger)明细分类账 (SubsidiaryLedger)总账科目 (GeneralAccount)明细科目 (SubsidiaryAccount)借方 (Debit)贷方 (Credit)借贷平衡原则 (Double-entry Principle)记账方向 (AccountingDirection)试算平衡表 (Trial Balance)调整分录 (AdjustingEntry)调整后试算平衡表(Adjusted TrialBalance)结转分录 (ClosingEntry)结转后试算平衡表(Post-closing TrialBalance)存货制度 (InventorySystem)存货核算方法 (InventoryAccounting Method)先进先出法 (FIFOMethod)后进先出法 (LIFOMethod)加权平均法 (WeightedAverage Method)科学成本法(Specific Identification Method)存货跌价准备(Allowance forInventory Decline)存货盘点(InventoryCounting)存货盈亏(InventoryProfit or Loss)固定资产(FixedAsset)折旧(Depreciation)折旧方法(DepreciationMethod)直线法(Straight-lineMethod)双倍余额递减法(Double-decliningBalance Method)年数总和法(Sum-of-the-years'-digitsMethod)残值(Residual Value)折旧年限(Useful Life)净残值率(SalvageRate)固定资产清理(Disposal of FixedAsset)无形资产(IntangibleAsset)商誉(Goodwill)知识产权(IntellectualProperty)专利权(Patent)商标权(Trademark)著作权(Copyright)长期股权投资(Long-term Equity Investment)成本法(Cost Method)权益法(EquityMethod)投资收益(InvestmentIncome)投资性房地产(InvestmentProperty)资产减值(Asset Impairment)减值损失(ImpairmentLoss)可回收金额(RecoverableAmount)可变现净值(NetRealizable Value)使用价值(Value inUse)金融资产(FinancialAsset)金融负债(FinancialLiability)公允价值(FairValue)利息收入(InterestIncome)利息支出(InterestExpense)汇兑收益(ExchangeGain)汇兑损失(ExchangeLoss)应收账款(AccountsReceivable)坏账损失(Bad DebtLoss)坏账准备(Allowancefor Bad Debt)应付账款(Accounts Payable)预收账款(UnearnedRevenue)预付账款(PrepaidExpense)应计收入(AccruedRevenue)应计费用(AccruedExpense)职工薪酬(Employee Compensation)工资与奖金(Wages andBonuses)社会保险费用(SocialInsurance Expense)住房公积金费用(Housing ProvidentFund Expense)职工福利费用(Employee WelfareExpense)借款费用 (BorrowingCost)资本化 (Capitalization)资本化利率(Capitalization Rate)资本化期间(Capitalization Period)资本化暂停(CapitalizationSuspension)现金等价物 (Cash Equivalent)现金流量表附表(Supplemental Scheduleof Cash Flow Statement)经营活动现金流量(Cash Flow fromOperating Activities)投资活动现金流量(Cash Flow fromInvesting Activities)筹资活动现金流量(Cash Flow fromFinancing Activities)直接法 (Direct Method)间接法 (Indirect Method)现金流量净额 (NetCash Flow)现金流量增减表(Statement of Changesin Cash Flow)现金流量比率 (CashFlow Ratio)利润表 (Income Statement)收入确认原则 (RevenueRecognition Principle)营业收入 (OperatingRevenue)营业成本 (OperatingCost)营业税金及附加(Business Tax andSurcharges)销售费用 (Selling Expense)管理费用 (AdministrativeExpense)财务费用 (FinancialExpense)营业利润 (OperatingProfit)营业外收入 (Non-operating Income)营业外支出 (Non-operating Expense)利润总额 (Total Profit)所得税费用 (IncomeTax Expense)净利润 (Net Profit)每股收益 (EarningsPer Share)所有者权益变动表(Statement of Changes in Owner's Equity)股本 (Capital Stock)资本公积 (CapitalReserve)盈余公积 (SurplusReserve)未分配利润 (RetainedEarnings)九、财务管理术语术语术语术语术语财务管理 (Financial Management)财务目标 (FinancialObjective)财务决策 (FinancialDecision)财务计划 (FinancialPlan)财务控制 (FinancialControl)资金 (Fund)资金需求 (FundDemand)资金供给 (FundSupply)资金流动 (Fund Flow)资金结构 (FundStructure)资本 (Capital)资本成本 (CapitalCost)资本结构 (CapitalStructure)资本预算 (CapitalBudget)资本收益率 (CapitalReturn Rate)投资 (Investment)投资项目 (InvestmentProject)投资评价 (InvestmentEvaluation)投资回收期 (PaybackPeriod)净现值 (Net PresentValue)内部收益率 (Internal Rate of Return)敏感性分析 (SensitivityAnalysis)风险分析 (RiskAnalysis)投资组合理论 (PortfolioTheory)资本资产定价模型(Capital Asset PricingModel)现金管理 (Cash Management)现金预测 (CashForecasting)现金流量预算表 (CashBudget)现金流量周期 (CashCycle)现金余额 (Cash Balance)应收账款管理 (AccountsReceivableManagement)应收账款周转率(Accounts ReceivableTurnover Ratio)坏账率 (Bad DebtRatio)应收账款账龄分析法(Aging Method ofAccounts Receivable)应收账款折现法(Discount Method ofAccounts Receivable)存货管理(Inventory Management)存货周转率(InventoryTurnover Ratio)经济订货量(Economic OrderQuantity)安全存量(SafetyStock)订货点(Reorder Point)短期融资(Short-term Financing)银行贷款(BankLoan)商业票据(CommercialPaper)应付账款融资(Accounts PayableFinancing)保兑仓融资(WarehouseReceipt Financing)长期融资(Long-term Financing)债券(Bond)债券价格(BondPrice)债券收益率(BondYield)债券评级(BondRating)股票(Stock)股票价格(StockPrice)股票收益率(StockReturn Rate)股息政策(DividendPolicy)股权融资(EquityFinancing)杠杆效应(LeverageEffect)操作杠杆系数(Operating LeverageCoefficient)财务杠杆系数(Financial LeverageCoefficient)综合杠杆系数(Combined LeverageCoefficient)杠杆调整原则(LeverageAdjustment Principle)十、运筹学术语术语术语术语术语运筹学 (Operations Research)决策 (Decision)决策变量 (DecisionVariable)目标函数 (ObjectiveFunction)约束条件 (Constraint)线性规划 (Linear Programming)图形法 (GraphicalMethod)单纯形法 (SimplexMethod)对偶理论 (DualityTheory)敏感性分析 (SensitivityAnalysis)整数规划 (Integer Programming)分支定界法 (Branch andBound Method)割平面法 (CuttingPlane Method)隐枚举法 (ImplicitEnumeration Method)0-1规划 (0-1Programming)非线性规划 (Nonlinear Programming)拉格朗日乘子法(Lagrange MultiplierMethod)KKT条件 (KKTCondition)梯度法 (GradientMethod)牛顿法 (Newton Method)动态规划 (Dynamic Programming)阶段 (Stage)状态 (State)决策 (Decision)最优值函数 (OptimalValue Function)贝尔曼方程 (BellmanEquation)网络优化 (NetworkOptimization)关键路径法 (CriticalPath Method)最短路问题 (ShortestPath Problem)最小生成树问题(Minimum Spanning TreeProblem)最大流问题 (Maximum Flow Problem)最小费用流问题(Minimum Cost FlowProblem)匹配问题 (MatchingProblem)背包问题 (KnapsackProblem)指派问题 (AssignmentProblem)非线性整数规划(Nonlinear Integer Programming)分数规划(FractionalProgramming)凸规划(ConvexProgramming)目标规划(GoalProgramming)多目标规划(Multi-objective Programming)随机规划(Stochastic Programming)鲁棒优化(RobustOptimization)参数规划(ParametricProgramming)可行方向法(FeasibleDirection Method)序列二次规划(Sequential QuadraticProgramming)队列论(QueueingTheory)到达过程(ArrivalProcess)服务过程(ServiceProcess)排队系统(QueueingSystem)排队模型(QueueingModel)M/M/1模型(M/M/1Model)M/M/c模型(M/M/cModel)M/G/1模型(M/G/1Model)G/M/1模型(G/M/1Model)排队长度(QueueLength)平均排队时间(Average Queueing Time)平均服务时间(AverageService Time)到达率(ArrivalRate)服务率(ServiceRate)利用率(UtilizationRate)十一、市场营销术语术语术语术语术语市场营销 (Marketing)市场营销管理(MarketingManagement)市场营销环境(MarketingEnvironment)市场营销计划(Marketing Plan)市场营销组合 (MarketingMix)市场 (Market)市场需求 (MarketDemand)市场细分 (MarketSegmentation)市场定位 (MarketPositioning)市场目标 (MarketTargeting)消费者行为 (ConsumerBehavior)消费者需求 (ConsumerNeed)消费者动机 (ConsumerMotivation)消费者态度(Consumer Attitude)消费者满意度 (ConsumerSatisfaction)产品 (Product)产品生命周期 (ProductLife Cycle)产品创新 (ProductInnovation)产品差异化 (ProductDifferentiation)产品定价 (Product Pricing)价格 (Price)价格策略 (PricingStrategy)价格弹性 (PriceElasticity)价格歧视 (PriceDiscrimination)价格竞争 (PriceCompetition)促销 (Promotion)促销策略 (PromotionStrategy)促销组合 (PromotionMix)广告 (Advertising)公关 (Public Relations)销售促进(Sales Promotion)个人销售(PersonalSelling)直接营销(DirectMarketing)网络营销(InternetMarketing)社会媒体营销(SocialMedia Marketing)分销(Distribution)分销渠道(DistributionChannel)分销策略(DistributionStrategy)物流(Logistics)运输(Transportation)库存管理(Inventory Management)订货量(OrderQuantity)经济批量(EconomicBatch Quantity)订货点(ReorderPoint)安全库存(Safety Stock)市场调研(Market Research)调研目的(ResearchObjective)调研方法(ResearchMethod)调研设计(ResearchDesign)调研样本(ResearchSample)数据收集(Data Collection)数据分析(DataAnalysis)数据呈现(DataPresentation)调研报告(ResearchReport)调研误差(ResearchError)十二、经济法术语术语术语术语术语经济法 (Economic Law)经济活动 (EconomicActivity)经济主体 (EconomicSubject)经济权利 (EconomicRight)经济责任 (EconomicResponsibility)经济法律关系 (Economic Legal Relationship)经济合同 (EconomicContract)经济纠纷 (EconomicDispute)经济诉讼 (EconomicLitigation)经济仲裁 (EconomicArbitration)民商事法律体系 (Civiland Commercial LegalSystem)民法典 (Civil Code)商法典 (CommercialCode)合同法 (Contract Law)物权法 (Property Law)侵权责任法 (Tort LiabilityLaw)民事诉讼法 (CivilProcedure Law)商事诉讼法(CommercialProcedure Law)仲裁法 (Arbitration Law)消费者权益保护法(Consumer Rights andInterests Protection Law)公司法(CompanyLaw)合伙企业法(PartnershipEnterprise Law)独资企业法(SoleProprietorshipEnterprise Law)外商投资企业法(Foreign InvestmentEnterprise Law)公司治理(CorporateGovernance)股东(Shareholder)董事会(Board ofDirectors)监事会(Board ofSupervisors)高级管理人员(SeniorManagement)股东大会(Shareholders'Meeting)股份(Share)股权(StockRight)股票(Stock)股本(Capital Stock)股利(Dividend)债券(Bond)债权(Debt Right)债务(Debt)债务人(Debtor)债权人(Creditor)破产(Bankruptcy)破产程序(BankruptcyProcedure)破产申请(BankruptcyApplication)破产管理人(BankruptcyAdministrator)破产债权人会议(Bankruptcy Creditors'Meeting)十三、现代公司制概论术语术语术语术语术语现代公司制 (Modern Corporation System)公司 (Company)公司法人 (CorporateLegal Person)公司治理 (CorporateGovernance)公司社会责任 (CorporateSocial Responsibility)股份有限公司 (Joint-stock Company)有限责任公司 (LimitedLiability Company)股东 (Shareholder)股份 (Share)股权 (Stock Right)董事会 (Board of Directors)监事会 (Board ofSupervisors)高级管理人员 (SeniorManagement)股东大会(Shareholders'Meeting)公司章程 (Articles ofAssociation)注册资本 (RegisteredCapital)实收资本 (Paid-inCapital)资本公积 (CapitalReserve)盈余公积 (SurplusReserve)未分配利润 (RetainedEarnings)股利 (Dividend)股息率 (DividendRate)现金分红 (CashDividend)股票分红 (StockDividend)分红政策 (Dividend Policy)上市公司(Listed Company)发行股票(IssueStock)募集资金(RaiseFunds)首次公开募股(InitialPublic Offering)再融资(Refinancing)股票市场(Stock Market)证券交易所(StockExchange)证券监管机构(SecuritiesRegulatory Authority)证券法(SecuritiesLaw)证券合同(SecuritiesContract)股票价格(StockPrice)股票指数(StockIndex)市盈率(Price-earningsRatio)市净率(Price-bookRatio)市场效率(MarketEfficiency)投资者保护(Investor Protection)信息披露(InformationDisclosure)内幕交易(InsiderTrading)操纵市场(MarketManipulation)证券欺诈(SecuritiesFraud)十四、经营管理术语术语术语术语术语经营管理 (Business Management)经营目标 (BusinessObjective)经营策略 (BusinessStrategy)经营模式 (BusinessModel)经营效率 (BusinessEfficiency)经营效果 (Business Effectiveness)经营创新 (BusinessInnovation)经营风险 (BusinessRisk)经营伦理 (BusinessEthics)经营文化 (BusinessCulture)组织 (Organization)组织结构(OrganizationalStructure)组织设计(OrganizationalDesign)组织变革 (OrganizationalChange)组织发展 (OrganizationalDevelopment)协调 (Coordination)协调机制 (CoordinationMechanism)协调原则(Coordination协调方法 (CoordinationMethod)协调技巧 (CoordinationSkill)Principle)控制 (Control)控制系统 (ControlSystem)控制过程 (ControlProcess)控制标准 (ControlStandard)控制反馈 (ControlFeedback)激励(Motivation)激励理论(MotivationTheory)激励因素(MotivationFactor)激励方法(MotivationMethod)激励机制(MotivationMechanism)资源(Resource)物质资源(MaterialResource)人力资源(HumanResource)财务资源(FinancialResource)信息资源(InformationResource)活动(Activity)生产活动(ProductionActivity)销售活动(SalesActivity)采购活动(PurchasingActivity)研发活动(Research andDevelopment Activity)目标(Objective)目标管理(ObjectiveManagement)目标设定(ObjectiveSetting)目标分解(ObjectiveDecomposition)目标评价(ObjectiveEvaluation)十五、公司金融术语术语术语术语术语公司金融 (CorporateFinance)投资决策 (InvestmentDecision)融资决策 (FinancingDecision)分红决策 (DividendDecision)资本结构 (CapitalStructure)资本成本 (CapitalCost)资本预算 (Capital Budget)现金流量 (Cash Flow)净现值 (Net PresentValue)内部收益率 (InternalRate of Return)敏感性分析(Sensitivity Analysis)风险分析 (Risk Analysis)投资组合理论 (PortfolioTheory)资本资产定价模型(Capital Asset PricingModel)证券市场线 (SecurityMarket Line)贝塔系数(Beta Coefficient)无风险利率(Risk-freeRate)市场风险溢价(MarketRisk Premium)资本市场线(CapitalMarket Line)有效边界(EfficientFrontier)杠杆效应(LeverageEffect)操作杠杆系数(OperatingLeverage Coefficient)财务杠杆系数(Financial LeverageCoefficient)综合杠杆系数(Combined LeverageCoefficient)杠杆调整原则(Leverage AdjustmentPrinciple)股权融资(Equity Financing)债务融资(DebtFinancing)权益融资(Quasi-equity Financing)混合融资(HybridFinancing)转换债券(ConvertibleBond)可赎回债券(Redeemable Bond)可交换债券(Exchangeable Bond)优先股(PreferredStock)可转换优先股(Convertible PreferredStock)权证(Warrant)十六、人力资源管理术语术语术语术语术语人力资源管理 (Human Resource Management)人力资源规划 (HumanResource Planning)人力资源分析 (HumanResource Analysis)人力资源需求 (HumanResource Demand)人力资源供给 (HumanResource Supply)招聘 (Recruitment)招聘渠道 (RecruitmentChannel)招聘广告 (RecruitmentAdvertisement)招聘成本 (RecruitmentCost)招聘效果 (RecruitmentEffectiveness)选拔 (Selection)选拔方法 (SelectionMethod)选拔标准 (SelectionCriterion)选拔工具 (SelectionTool)选拔过程 (SelectionProcess)培训 (Training)培训需求分析 (TrainingNeeds Analysis)培训目标 (TrainingObjective)培训内容 (TrainingContent)培训方法 (TrainingMethod)培训评估(Training Evaluation)培训效果(TrainingEffectiveness)培训反馈(TrainingFeedback)培训转移(TrainingTransfer)培训成本(TrainingCost)术语术语术语术语术语评估(Performance Appraisal)评估目的(PerformanceAppraisal Purpose)评估标准(PerformanceAppraisal Criterion)评估方法(PerformanceAppraisal Method)评估结果(PerformanceAppraisal Result)激励(Motivation)激励理论(MotivationTheory)激励因素(MotivationFactor)激励方法(MotivationMethod)激励机制(MotivationMechanism)薪酬(Compensation)薪酬结构(CompensationStructure)薪酬水平(CompensationLevel)薪酬调整(CompensationAdjustment)薪酬管理(CompensationManagement)十七、企业战略管理术语术语术语术语术语企业战略管理(Corporate Strategy Management)战略 (Strategy)战略管理过程(Strategy ManagementProcess)战略分析 (StrategyAnalysis)战略制定 (StrategyFormulation)战略实施 (Strategy Implementation)战略评估 (StrategyEvaluation)战略控制 (StrategyControl)战略调整 (StrategyAdjustment)战略创新 (StrategyInnovation)环境分析(Environmental Analysis)宏观环境分析 (Macro-environmentalAnalysis)行业环境分析 (IndustryEnvironmentalAnalysis)微观环境分析 (Micro-environmental Analysis)PEST分析法(PESTAnalysis Method)波特五力模型(Porter's Five Forces Model)SWOT分析法(SWOT AnalysisMethod)VRIO分析法(VRIOAnalysis Method)价值链分析法(ValueChain Analysis Method)核心竞争力分析法(Core CompetenceAnalysis Method)目标管理(Objective Management)SMART原则(SMART Principle)平衡计分卡(BalancedScorecard)关键绩效指标(KeyPerformance Indicator)目标层次结构(ObjectiveHierarchy)战略选择(StrategyChoice)战略类型(StrategyType)成本领先战略(CostLeadership Strategy)差异化战略(DifferentiationStrategy)聚焦战略(FocusStrategy)集团化战略(Diversification Strategy)垂直一体化战略(Vertical IntegrationStrategy)水平一体化战略(HorizontalIntegration Strategy)国际化战略(InternationalizationStrategy)蓝海战略(Blue OceanStrategy)。
人工智能英汉
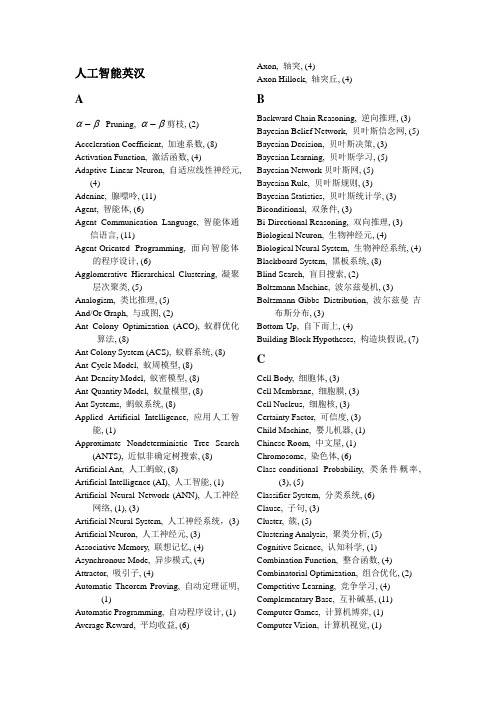
人工智能英汉Aβα-Pruning, βα-剪枝, (2) Acceleration Coefficient, 加速系数, (8) Activation Function, 激活函数, (4) Adaptive Linear Neuron, 自适应线性神经元,(4)Adenine, 腺嘌呤, (11)Agent, 智能体, (6)Agent Communication Language, 智能体通信语言, (11)Agent-Oriented Programming, 面向智能体的程序设计, (6)Agglomerative Hierarchical Clustering, 凝聚层次聚类, (5)Analogism, 类比推理, (5)And/Or Graph, 与或图, (2)Ant Colony Optimization (ACO), 蚁群优化算法, (8)Ant Colony System (ACS), 蚁群系统, (8) Ant-Cycle Model, 蚁周模型, (8)Ant-Density Model, 蚁密模型, (8)Ant-Quantity Model, 蚁量模型, (8)Ant Systems, 蚂蚁系统, (8)Applied Artificial Intelligence, 应用人工智能, (1)Approximate Nondeterministic Tree Search (ANTS), 近似非确定树搜索, (8) Artificial Ant, 人工蚂蚁, (8)Artificial Intelligence (AI), 人工智能, (1) Artificial Neural Network (ANN), 人工神经网络, (1), (3)Artificial Neural System, 人工神经系统,(3) Artificial Neuron, 人工神经元, (3) Associative Memory, 联想记忆, (4) Asynchronous Mode, 异步模式, (4) Attractor, 吸引子, (4)Automatic Theorem Proving, 自动定理证明,(1)Automatic Programming, 自动程序设计, (1) Average Reward, 平均收益, (6) Axon, 轴突, (4)Axon Hillock, 轴突丘, (4)BBackward Chain Reasoning, 逆向推理, (3) Bayesian Belief Network, 贝叶斯信念网, (5) Bayesian Decision, 贝叶斯决策, (3) Bayesian Learning, 贝叶斯学习, (5) Bayesian Network贝叶斯网, (5)Bayesian Rule, 贝叶斯规则, (3)Bayesian Statistics, 贝叶斯统计学, (3) Biconditional, 双条件, (3)Bi-Directional Reasoning, 双向推理, (3) Biological Neuron, 生物神经元, (4) Biological Neural System, 生物神经系统, (4) Blackboard System, 黑板系统, (8)Blind Search, 盲目搜索, (2)Boltzmann Machine, 波尔兹曼机, (3) Boltzmann-Gibbs Distribution, 波尔兹曼-吉布斯分布, (3)Bottom-Up, 自下而上, (4)Building Block Hypotheses, 构造块假说, (7) CCell Body, 细胞体, (3)Cell Membrane, 细胞膜, (3)Cell Nucleus, 细胞核, (3)Certainty Factor, 可信度, (3)Child Machine, 婴儿机器, (1)Chinese Room, 中文屋, (1) Chromosome, 染色体, (6)Class-conditional Probability, 类条件概率,(3), (5)Classifier System, 分类系统, (6)Clause, 子句, (3)Cluster, 簇, (5)Clustering Analysis, 聚类分析, (5) Cognitive Science, 认知科学, (1) Combination Function, 整合函数, (4) Combinatorial Optimization, 组合优化, (2) Competitive Learning, 竞争学习, (4) Complementary Base, 互补碱基, (11) Computer Games, 计算机博弈, (1) Computer Vision, 计算机视觉, (1)Conflict Resolution, 冲突消解, (3) Conjunction, 合取, (3)Conjunctive Normal Form (CNF), 合取范式,(3)Collapse, 坍缩, (11)Connectionism, 连接主义, (3) Connective, 连接词, (3)Content Addressable Memory, 联想记忆, (4) Control Policy, 控制策略, (6)Crossover, 交叉, (7)Cytosine, 胞嘧啶, (11)DData Mining, 数据挖掘, (1)Decision Tree, 决策树, (5) Decoherence, 消相干, (11)Deduction, 演绎, (3)Default Reasoning, 默认推理(缺省推理),(3)Defining Length, 定义长度, (7)Rule (Delta Rule), 德尔塔规则, 18(3) Deliberative Agent, 慎思型智能体, (6) Dempster-Shafer Theory, 证据理论, (3) Dendrites, 树突, (4)Deoxyribonucleic Acid (DNA), 脱氧核糖核酸, (6), (11)Disjunction, 析取, (3)Distributed Artificial Intelligence (DAI), 分布式人工智能, (1)Distributed Expert Systems, 分布式专家系统,(9)Divisive Hierarchical Clustering, 分裂层次聚类, (5)DNA Computer, DNA计算机, (11)DNA Computing, DNA计算, (11) Discounted Cumulative Reward, 累计折扣收益, (6)Domain Expert, 领域专家, (10) Dominance Operation, 显性操作, (7) Double Helix, 双螺旋结构, (11)Dynamical Network, 动态网络, (3)E8-Puzzle Problem, 八数码问题, (2) Eletro-Optical Hybrid Computer, 光电混合机, (11)Elitist strategy for ant systems (EAS), 精化蚂蚁系统, (8)Energy Function, 能量函数, (3) Entailment, 永真蕴含, (3) Entanglement, 纠缠, (11)Entropy, 熵, (5)Equivalence, 等价式, (3)Error Back-Propagation, 误差反向传播, (4) Evaluation Function, 评估函数, (6) Evidence Theory, 证据理论, (3) Evolution, 进化, (7)Evolution Strategies (ES), 进化策略, (7) Evolutionary Algorithms (EA), 进化算法, (7) Evolutionary Computation (EC), 进化计算,(7)Evolutionary Programming (EP), 进化规划,(7)Existential Quantification, 存在量词, (3) Expert System, 专家系统, (1)Expert System Shell, 专家系统外壳, (9) Explanation-Based Learning, 解释学习, (5) Explanation Facility, 解释机构, (9)FFactoring, 因子分解, (11)Feedback Network, 反馈型网络, (4) Feedforward Network, 前馈型网络, (1) Feasible Solution, 可行解, (2)Finite Horizon Reward, 横向有限收益, (6) First-order Logic, 一阶谓词逻辑, (3) Fitness, 适应度, (7)Forward Chain Reasoning, 正向推理, (3) Frame Problem, 框架问题, (1)Framework Theory, 框架理论, (3)Free-Space Optical Interconnect, 自由空间光互连, (11)Fuzziness, 模糊性, (3)Fuzzy Logic, 模糊逻辑, (3)Fuzzy Reasoning, 模糊推理, (3)Fuzzy Relation, 模糊关系, (3)Fuzzy Set, 模糊集, (3)GGame Theory, 博弈论, (8)Gene, 基因, (7)Generation, 代, (6)Genetic Algorithms, 遗传算法, (7)Genetic Programming, 遗传规划(遗传编程),(7)Global Search, 全局搜索, (2)Gradient Descent, 梯度下降, (4)Graph Search, 图搜索, (2)Group Rationality, 群体理性, (8) Guanine, 鸟嘌呤, (11)HHanoi Problem, 梵塔问题, (2)Hebbrian Learning, 赫伯学习, (4)Heuristic Information, 启发式信息, (2) Heuristic Search, 启发式搜索, (2)Hidden Layer, 隐含层, (4)Hierarchical Clustering, 层次聚类, (5) Holographic Memory, 全息存储, (11) Hopfield Network, 霍普菲尔德网络, (4) Hybrid Agent, 混合型智能体, (6)Hype-Cube Framework, 超立方体框架, (8)IImplication, 蕴含, (3)Implicit Parallelism, 隐并行性, (7) Individual, 个体, (6)Individual Rationality, 个体理性, (8) Induction, 归纳, (3)Inductive Learning, 归纳学习, (5) Inference Engine, 推理机, (9)Information Gain, 信息增益, (3)Input Layer, 输入层, (4)Interpolation, 插值, (4)Intelligence, 智能, (1)Intelligent Control, 智能控制, (1) Intelligent Decision Supporting System (IDSS), 智能决策支持系统,(1) Inversion Operation, 倒位操作, (7)JJoint Probability Distribution, 联合概率分布,(5) KK-means, K-均值, (5)K-medoids, K-中心点, (3)Knowledge, 知识, (3)Knowledge Acquisition, 知识获取, (9) Knowledge Base, 知识库, (9)Knowledge Discovery, 知识发现, (1) Knowledge Engineering, 知识工程, (1) Knowledge Engineer, 知识工程师, (9) Knowledge Engineering Language, 知识工程语言, (9)Knowledge Interchange Format (KIF), 知识交换格式, (8)Knowledge Query and ManipulationLanguage (KQML), 知识查询与操纵语言,(8)Knowledge Representation, 知识表示, (3)LLearning, 学习, (3)Learning by Analog, 类比学习, (5) Learning Factor, 学习因子, (8)Learning from Instruction, 指导式学习, (5) Learning Rate, 学习率, (6)Least Mean Squared (LSM), 最小均方误差,(4)Linear Function, 线性函数, (3)List Processing Language (LISP), 表处理语言, (10)Literal, 文字, (3)Local Search, 局部搜索, (2)Logic, 逻辑, (3)Lyapunov Theorem, 李亚普罗夫定理, (4) Lyapunov Function, 李亚普罗夫函数, (4)MMachine Learning, 机器学习, (1), (5) Markov Decision Process (MDP), 马尔科夫决策过程, (6)Markov Chain Model, 马尔科夫链模型, (7) Maximum A Posteriori (MAP), 极大后验概率估计, (5)Maxmin Search, 极大极小搜索, (2)MAX-MIN Ant Systems (MMAS), 最大最小蚂蚁系统, (8)Membership, 隶属度, (3)Membership Function, 隶属函数, (3) Metaheuristic Search, 元启发式搜索, (2) Metagame Theory, 元博弈理论, (8) Mexican Hat Function, 墨西哥草帽函数, (4) Migration Operation, 迁移操作, (7) Minimum Description Length (MDL), 最小描述长度, (5)Minimum Squared Error (MSE), 最小二乘法,(4)Mobile Agent, 移动智能体, (6)Model-based Methods, 基于模型的方法, (6) Model-free Methods, 模型无关方法, (6) Modern Heuristic Search, 现代启发式搜索,(2)Monotonic Reasoning, 单调推理, (3)Most General Unification (MGU), 最一般合一, (3)Multi-Agent Systems, 多智能体系统, (8) Multi-Layer Perceptron, 多层感知器, (4) Mutation, 突变, (6)Myelin Sheath, 髓鞘, (4)(μ+1)-ES, (μ+1) -进化规划, (7)(μ+λ)-ES, (μ+λ) -进化规划, (7) (μ,λ)-ES, (μ,λ) -进化规划, (7)NNaïve Bayesian Classifiers, 朴素贝叶斯分类器, (5)Natural Deduction, 自然演绎推理, (3) Natural Language Processing, 自然语言处理,(1)Negation, 否定, (3)Network Architecture, 网络结构, (6)Neural Cell, 神经细胞, (4)Neural Optimization, 神经优化, (4) Neuron, 神经元, (4)Neuron Computing, 神经计算, (4)Neuron Computation, 神经计算, (4)Neuron Computer, 神经计算机, (4) Niche Operation, 生态操作, (7) Nitrogenous base, 碱基, (11)Non-Linear Dynamical System, 非线性动力系统, (4)Non-Monotonic Reasoning, 非单调推理, (3) Nouvelle Artificial Intelligence, 行为智能,(6)OOccam’s Razor, 奥坎姆剃刀, (5)(1+1)-ES, (1+1) -进化规划, (7)Optical Computation, 光计算, (11)Optical Computing, 光计算, (11)Optical Computer, 光计算机, (11)Optical Fiber, 光纤, (11)Optical Waveguide, 光波导, (11)Optical Interconnect, 光互连, (11) Optimization, 优化, (2)Optimal Solution, 最优解, (2)Orthogonal Sum, 正交和, (3)Output Layer, 输出层, (4)Outer Product, 外积法, 23(4)PPanmictic Recombination, 混杂重组, (7) Particle, 粒子, (8)Particle Swarm, 粒子群, (8)Particle Swarm Optimization (PSO), 粒子群优化算法, (8)Partition Clustering, 划分聚类, (5) Partitioning Around Medoids, K-中心点, (3) Pattern Recognition, 模式识别, (1) Perceptron, 感知器, (4)Pheromone, 信息素, (8)Physical Symbol System Hypothesis, 物理符号系统假设, (1)Plausibility Function, 不可驳斥函数(似然函数), (3)Population, 物种群体, (6)Posterior Probability, 后验概率, (3)Priori Probability, 先验概率, (3), (5) Probability, 随机性, (3)Probabilistic Reasoning, 概率推理, (3) Probability Assignment Function, 概率分配函数, (3)Problem Solving, 问题求解, (2)Problem Reduction, 问题归约, (2)Problem Decomposition, 问题分解, (2) Problem Transformation, 问题变换, (2) Product Rule, 产生式规则, (3)Product System, 产生式系统, (3) Programming in Logic (PROLOG), 逻辑编程, (10)Proposition, 命题, (3)Propositional Logic, 命题逻辑, (3)Pure Optical Computer, 全光计算机, (11)QQ-Function, Q-函数, (6)Q-learning, Q-学习, (6)Quantifier, 量词, (3)Quantum Circuit, 量子电路, (11)Quantum Fourier Transform, 量子傅立叶变换, (11)Quantum Gate, 量子门, (11)Quantum Mechanics, 量子力学, (11) Quantum Parallelism, 量子并行性, (11) Qubit, 量子比特, (11)RRadial Basis Function (RBF), 径向基函数,(4)Rank based ant systems (ASrank), 基于排列的蚂蚁系统, (8)Reactive Agent, 反应型智能体, (6) Recombination, 重组, (6)Recurrent Network, 循环网络, (3) Reinforcement Learning, 强化学习, (3) Resolution, 归结, (3)Resolution Proof, 归结反演, (3) Resolution Strategy, 归结策略, (3) Reasoning, 推理, (3)Reward Function, 奖励函数, (6) Robotics, 机器人学, (1)Rote Learning, 机械式学习, (5)SSchema Theorem, 模板定理, (6) Search, 搜索, (2)Selection, 选择, (7)Self-organizing Maps, 自组织特征映射, (4) Semantic Network, 语义网络, (3)Sexual Differentiation, 性别区分, (7) Shor’s algorithm, 绍尔算法, (11)Sigmoid Function, Sigmoid 函数(S型函数),(4)Signal Function, 信号函数, (3)Situated Artificial Intelligence, 现场式人工智能, (1)Spatial Light Modulator (SLM), 空间光调制器, (11)Speech Act Theory, 言语行为理论, (8) Stable State, 稳定状态, (4)Stability Analysis, 稳定性分析, (4)State Space, 状态空间, (2)State Transfer Function, 状态转移函数,(6)Substitution, 置换, (3)Stochastic Learning, 随机型学习, (4) Strong Artificial Intelligence (AI), 强人工智能, (1)Subsumption Architecture, 包容结构, (6) Superposition, 叠加, (11)Supervised Learning, 监督学习, (4), (5) Swarm Intelligence, 群智能, (8)Symbolic Artificial Intelligence (AI), 符号式人工智能(符号主义), (3) Synapse, 突触, (4)Synaptic Terminals, 突触末梢, (4) Synchronous Mode, 同步模式, (4)TThreshold, 阈值, (4)Threshold Function, 阈值函数, (4) Thymine, 胸腺嘧啶, (11)Topological Structure, 拓扑结构, (4)Top-Down, 自上而下, (4)Transfer Function, 转移函数, (4)Travel Salesman Problem, 旅行商问题, (4) Turing Test, 图灵测试, (1)UUncertain Reasoning, 不确定性推理, (3)Uncertainty, 不确定性, (3)Unification, 合一, (3)Universal Quantification, 全称量词, (4) Unsupervised Learning, 非监督学习, (4), (5)WWeak Artificial Intelligence (Weak AI), 弱人工智能, (1)Weight, 权值, (4)Widrow-Hoff Rule, 维德诺-霍夫规则, (4)。
人工智能英文词汇
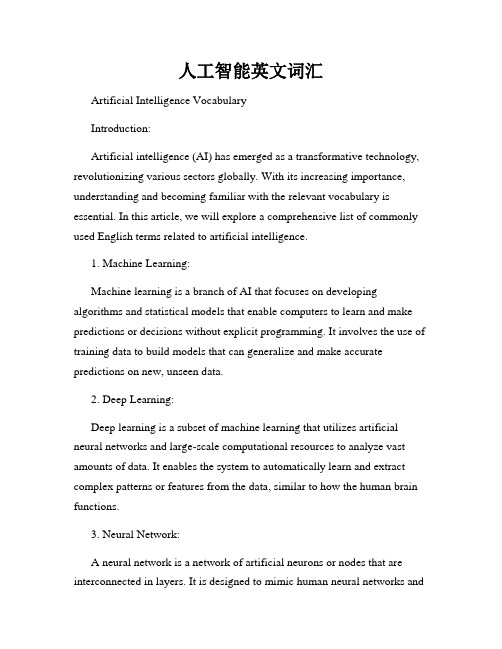
人工智能英文词汇Artificial Intelligence VocabularyIntroduction:Artificial intelligence (AI) has emerged as a transformative technology, revolutionizing various sectors globally. With its increasing importance, understanding and becoming familiar with the relevant vocabulary is essential. In this article, we will explore a comprehensive list of commonly used English terms related to artificial intelligence.1. Machine Learning:Machine learning is a branch of AI that focuses on developing algorithms and statistical models that enable computers to learn and make predictions or decisions without explicit programming. It involves the use of training data to build models that can generalize and make accurate predictions on new, unseen data.2. Deep Learning:Deep learning is a subset of machine learning that utilizes artificial neural networks and large-scale computational resources to analyze vast amounts of data. It enables the system to automatically learn and extract complex patterns or features from the data, similar to how the human brain functions.3. Neural Network:A neural network is a network of artificial neurons or nodes that are interconnected in layers. It is designed to mimic human neural networks andprocess complex information. Neural networks play a crucial role in deep learning algorithms, enabling the development of highly accurate predictive models.4. Natural Language Processing (NLP):Natural Language Processing is a subfield of AI that focuses on the interaction between computers and humans through natural language. It involves tasks such as speech recognition, language understanding, and machine translation. NLP enables computers to understand and generate human language, facilitating communication and information processing.5. Computer Vision:Computer vision involves the use of AI and image processing techniques to enable computers to interpret and analyze visual information. It encompasses tasks such as object recognition, image classification, and image generation. Computer vision finds applications in areas like autonomous vehicles, medical imaging, and surveillance systems.6. Robotics:Robotics involves the design, construction, programming, and operation of robots. AI plays a vital role in robotics by enabling autonomous decision-making, learning, and adaptation. Robotics combines various technologies, including AI, to develop intelligent machines that can interact with the physical world and perform human-like tasks.7. Big Data:Big data refers to the massive volume of structured and unstructured data that is generated at an unprecedented rate. AI technologies like machine learning and deep learning can analyze big data to extract meaningful insights, patterns, and trends. The integration of big data and AI has opened up new opportunities and possibilities across industries.8. Algorithm:An algorithm is a step-by-step procedure or set of rules designed to solve a specific problem or perform a particular task. In the context of AI, algorithms are responsible for processing and analyzing data, training machine learning models, and making predictions or decisions. Well-designed algorithms are crucial for achieving accurate and efficient AI systems.9. Predictive Analytics:Predictive analytics involves utilizing historical and current data to forecast future outcomes or trends. AI techniques, such as machine learning, are often used in predictive analytics to analyze large datasets, identify patterns, and make accurate predictions. Predictive analytics finds applications in various domains, including marketing, finance, and healthcare.10. Virtual Assistant:A virtual assistant is an AI-powered software that can perform tasks or services for individuals. It uses natural language processing and speech recognition to understand and respond to users' voice commands or textinputs. Virtual assistants, such as Siri, Alexa, and Google Assistant, have become increasingly popular, enhancing productivity and convenience.Conclusion:As AI continues to evolve and shape our world, having a good understanding of the associated vocabulary is essential. In this article, we have delved into some of the key terms related to artificial intelligence. By familiarizing ourselves with these terms, we can stay informed and effectively engage in discussions and developments within the AI domain.。
香港理工大学Professor Albert P.C. Chan 简历
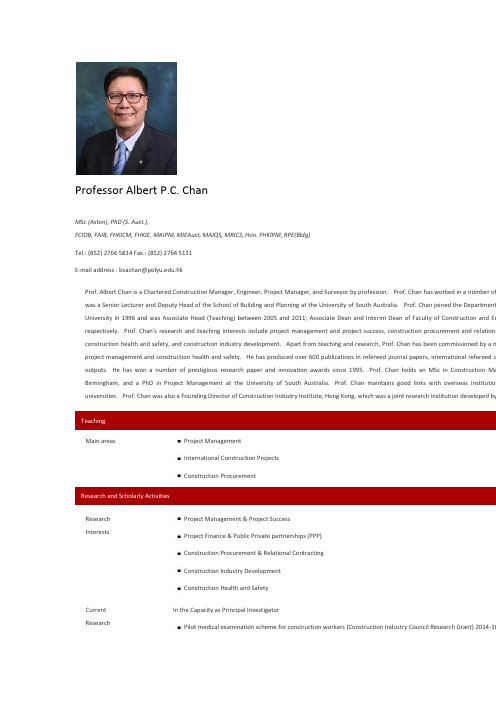
Professor Albert P.C. ChanMSc (Aston), PhD (S. Aust.),FCIOB, FAIB, FHKICM, FHKIE, MAIPM, MIEAust, MAIQS, MRICS, Hon. FHKIPM, RPE(Bldg)Tel.: (852) 2766 5814 Fax.: (852) 2764 5131E-mail address : bsachan@.hkProf. Albert Chan is a Chartered Construction Manager, Engineer, Project Manager, and Surveyor by profession. Prof. Chan has worked in a number of was a Senior Lecturer and Deputy Head of the School of Building and Planning at the University of South Australia. Prof. Chan joined the Department University in 1996 and was Associate Head (Teaching) between 2005 and 2011; Associate Dean and Interim Dean of Faculty of Construction and En respectively. Prof. Chan's research and teaching interests include project management and project success, construction procurement and relationaconstruction health and safety, and construction industry development. Apart from teaching and research, Prof. Chan has been commissioned by a n project management and construction health and safety. He has produced over 600 publications in refereed journal papers, international refereed c outputs. He has won a number of prestigious research paper and innovation awards since 1995. Prof. Chan holds an MSc in Construction Ma Birmingham, and a PhD in Project Management at the University of South Australia. Prof. Chan maintains good links with overseas institution universities. Prof. Chan was also a Founding Director of Construction Industry Institute, Hong Kong, which was a joint research institution developed by TeachingMain areas Project ManagementInternational Construction ProjectsConstruction Procurement Research and Scholarly ActivitiesResearch InterestsProject Management & Project SuccessProject Finance & Public Private partnerships (PPP) Construction Procurement & Relational ContractingConstruction Industry DevelopmentConstruction Health and SafetyCurrent ResearchIn the Capacity as Principal InvestigatorPilot medical examination scheme for construction workers (Construction Industry Council Research Grant) 2014-16Projects Developing a personal cooling system (PCS) for combating heat stress in the construction industry (RGC General Res Evaluating the economic, social, and cultural impacts of revitalizing industrial buildings in Hong Kong (RGC Public PoManaging Construction Safety for Ethnic Minorities in Hong Kong (PolyU Research Grant) 2012-2014, $258,300.00Anti-heat stress clothing for construction workers in hot and humid weather (RGC General Research Fund) 2012-201Experimental Research on Health and Safety Measures for Working in Hot Weather. RGC General Research Fund, 20 In the Capacity as Co-InvestigatorAssessing the time performance of building construction in Hong Kong (Construction Industry Council Researc$996,475.00Electrical and Mechanical Safety in Construction (Construction Industry Council Research Grant) 2014-16, Prof FrancDevelopment of a Fire Risk Assessment Model for New Construction Sites in Hong Kong Dr Daniel Chan as PI, PolyUAn exploratory study of the implementation of the Mandatory Building Inspection Scheme (MBIS) in Hong Kong. Dr- Departmental General Research Fund. 2011-2013, $225,000.An Investigation of Pitfalls in Output Specifications for Public-Private Partnership Projects and their Solutions. DrFund, 2010-2013, $556,871.Research Students Supervision (On-going) Zhou, X (2014-) The impacts of building information modeling (BIM) on productivity: experienced on construction Department of Building and Real Estate, The Hong Kong Polytechnic University (In Progress).Zahoor, HZAK (2013-) Development of a computerized “Safety Performance Measurement Model” for the construc Building and Real Estate, The Hong Kong Polytechnic University (In Progress).Utama, WP (2013-) A fuzzy neural network decision support system for selecting overseas construction projects Department of Building and Real Estate, The Hong Kong Polytechnic University (In Progress).Shan, M (2012-) Collusion prevention strategies for public funded constructions projects in People’s Republic of C and Real Estate, TheHong Kong Polytechnic University (In Progress).Yang, Y (2012-) A model to determine the suitability of PPE for construction in a hot and humid environment. PhD Estate, The Hong Kong Polytechnic University (In Progress).Ameyaw, EE (2011-) Risk Allocation Model for Public-Private Partnership (PPP) Water Supply Projects in Ghana. Ph Estate, The Hong Kong Polytechnic University (In Progress).Guan, Y (2010-) Impact of heat stress on construction workers. PhD Thesis, Department of Building and Real Estate Progress).Hong, YM (2009-) Implementation of Construction Partnering in the People’s Republic of China (PRC): Cha Department of Building and Real Estate, The Hong Kong Polytechnic University (In Progress).Chan, TK (2009-) Developing a Practical Management Framework for Enhancing Partnering Performance for U Department of Building and Real Estate, The Hong Kong Polytechnic University (In Progress).Law, OK (2009-) Integrating Social Responsibility Criteria into Ethical Decision-Making Model for Hong Kong Profess and Real Estate, The Hong Kong Polytechnic University (In Progress).Yuen, MTL (2007-) The Improvement of Safety Climate in the Small-Scale Construction Works ¡V from Attitude to Cand Real Estate, The Hong Kong Polytechnic University (In Progress).(Completed)Yi, W (2011-2014) Modeling rebar labor productivity in hot weather. PhD Thesis, Department of Building and University (Successfully completed in 2014). Hu, Y (2010-2014) Construction Program Organisation Model and Its Application in Megaprojects of the People's ReBuilding and Real Estate, The Hong Kong Polytechnic University (Successfully completed in 2014). Peng, T (2008-2012) Developing Appropriate financing Modalities for Complex Infrastructure Projects . MPhil ThesThe Hong Kong Polytechnic University (Successfully completed in 2012).Choi, NY (2008-2012) Investigating the Current Application and Future Development of Pay for Safety Scheme (PFSMPhil Thesis, Department of Building and Real Estate, The Hong Kong Polytechnic University (Successfully completeHon, CKH (2008-2012) Relationships between Safety Climate and Safety Performance of Repair, Maintenance, MinPhD Thesis, Department of Building and Real Estate, The Hong Kong Polytechnic University (Successfully completedXu, Yelin (2008-2010) Contract Design and Management for PPP Method in China. PhD Thesis, Institute of ConstructUniversity, PRC.Xia, Bo (2007-2010) Study on Design-Build Delivery Method in the Chinese Construction Industry. PhD Thesis, DeHong Kong Polytechnic University.Ke, Yongjian (2007-2010) A Risk-based Financial Evaluation Method for Public-Private-Partnership Projects. PManagement, Tsinghua University, PRC.Cheung, E (2006-2009) A Best Practice Framework for Public Private Partnerships (PPPs). PhD Thesis, the QueenslanYeung, JFY (2004-2007) Developing Key Performance Indicators (KPIs) for Construction Partnering – a Fuzzy Set TheBuilding and Real Estate, The Hong Kong Polytechnic University.Wong, JWM (2002-2006) Forecasting Manpower Demand in the Construction Industry of Hong Kong. PhD Thesis, DHong Kong Polytechnic University.Lam, EWM (2000-2005) A Conceptual Model of Project Success for Design and Build Projects in the Public SectorBuilding and Real Estate, The Hong Kong Polytechnic University.Chan, APL (2000-2004) Critical Success Factors for Delivering Health-care Projects in Hong Kong. PhD Thesis, DepHong Kong Polytechnic University.Member, Task Force on the Proposed “Green Deck”, The Hong Kong Polytechnic University.Member, Senate, The Hong Kong Polytechnic University.Member, Central Administration Committee, The Hong Kong Polytechnic University.Member, Academic Council, The Hong Kong Polytechnic University.Chair, Executive Committee, Faculty of Construction and Environment. The Hong Kong Polytechnic University.Chair, Faculty Staff Committee, Faculty of Construction and Environment, The Hong Kong Polytechnic University.Ex-officio Member, Faculty Research Committee, Faculty of Construction and Environment, The Hong Kong PolytechEx-officio Member, Faculty Learning and Teaching Committee, Faculty of Construction and Environment, The Hong KInterim Dean, Faculty of Construction and EnvironmentMember , Search Committee for Recruitment of Dean (Construction and Environment), The Hong Kong Polytechnic UMember , Health and Safety Committee (HSC), The Hong Kong Polytechnic University.Member , Campus Development and Space Allocation Committee, The Hong Kong Polytechnic University.Chair , Faculty Partnership Committee, Faculty of Construction and EnvironmentChief Editor , Construction and Environment, Faculty of Construction and EnvironmentMember , Academic Planning CommitteeConsultancy Conduct a consultancy study on the Effectiveness of personal cooling equipment for protecting workers from heat scommissioned by the Occupational Safety and Health Council, 2012 – 2013.Conduct a consultancy study on the Procurement Strategy, Delivery Systems and Contractual Arrangement forcommissioned by Hong Kong Science and Technology Park, 2010.Comprehensively review the Safety Management System, commissioned by Sun Hung Kai Properties Limited, 2009-Develop a Pay for Safety Scheme for Subcontractors, commissioned by Gammon Construction Limited, 2007-08.Identify Development Strategy for Professional Resources of Hong Kong's Construction and Related Engineering SeBranch of Development Bureau of the HKSAR Government, 2007-08.Provide Technical Assistance (TA4724 - PRC) in: Application of Public-Private Partnerships in Urban Rail-Based TraMTR Corporation Limited and funded by the Asian Development Bank, 2007-08.Facilitate an Initial Partnering Workshop for The Hong Kong Community College Development at K.I.L. 11163 (HChevalier (Construction) Co. Ltd., 2006.Facilitate an Initial Partnering Workshop for The Hong Kong Polytechnic University Phase 7 Development, commissLtd., 2004.Develop a Model to Estimate Construction Personnel Manpower Demand and Supply on a Regular Basis, commissiothe HKSAR, 2002.Analyse Partnering in Hong Kong, commissioned by the Hong Kong Housing Society, 2001.Investigate the Ready-Mixed Concrete Supply in Hong Kong, commissioned by the Hong Kong Housing Authority, 19External AppointmentMember of the Editorial Advisory Board . Facilities . Emerald Group Publishing (2014 - ). Regional Editor . Indoor and Built Environment . SAGE Journals (2014 - ).Member of the Editorial Board. Engineering Science Letters , Science & Knowledge Publishing Corporation Limited, Member of the Editorial Board . Australasian Journal of Construction Economics and Building – Conference Series (2Member of the Scientific Committee . CIB W099 International Conference on Achieving Sustainable ConstructionKamprad Design Centre (IKDC) 2-3 June 2014.Adjunct Professor, Xi’an Jiaotong University, PRCMember of the Editorial Board . Journal of Construction Engineering , Hindawi Publishing corporation, USA (2012-).Member of Expert Review Panel - Built Environment (2013) for Formas’ ge neral call forgrant applicationsEnvironment, Agricultural Sciences and Spatial Planning.Member of the Editorial Board. Journal of Frontiers in Construction Engineering, World Academic Publishing Co. Ltd. Member of Appeal Tribunal PanelAdjunct Professor, Huaqiao University, PRC.Adjunct Professor, The Queensland University of Technology (QUT), Australia.Adjunct Professor, The University of South Australia (UniSA), Australia.Adjunct Professor, The Bond University, Australia.External Examiner, Quantity Surveying programme in the School of Housing, Building and Planning, Universiti Sains M Local Partner to the Doing Business Annual Report Series, the International Bank for Reconstruction and Developme Member of the Technical Committee. The First Australasia and South-East Asia Structural Engineering and Con Australia, November 28-December 2, 2012.Member of the Scientific Committee. The 37th Australian Universities Building Education Association (AUBEA) 2012 Wales, Sydney, 4-6 July 2012.Member of International Scientific Committee, Third International Conference on Construction in Developing Cou 4-6, 2012, Millennium Hilton Bangkok.Member of the Editorial Board, Journal of Construction Project Management and Innovation (JCPMI), South Africa (2 Member of the Editorial Board, Journal of Construction Engineering and Project Management (JCEPM), Korea Management (KICEM), South Korea (2010-).Member of Editorial Review Panel, International Journal of Construction Project Management, Nova Science Publish Member of the Construction Annual Review Editorial Board, Constructive Publications Company, UK(2008-).Professional Service Member, Asia Pacific Education Standards Board, Royal Institution of Chartered Surveyor. Assessor of the Professional Assessment Interview, The Hong Kong Institution of Engineers. Member of the Advisory Board, The Hong Kong Institute of Project Management.Book/ Monograph 1 Chan, APC and Cheung, E (2014) Public Private Partnerships in International Construction: Learning from Case St London and New York, 190 pages.Keynote Presentation 1 Chan, APC (2013) Revitalizing industrial buildings in Hong Kong – lessons learnt. Euro Asia Economic Forum 201 26-28 September 2013, Xi’an International Conference Centre, China,176Externally Refereed Journal Paper 1 Chan, APC, Yang, Y, Wong, DP, Lam, EWM, and Li, Y (2013) Factors affecting horticultural and cleaning workers’ pEnvironment. 66 (2013) 181-189Externally Refereed 1 Chan, APC, Yang, Y, Wong, FKW, Yam, MCH, Lam, EWM, Wong, DP, Guo, YP, Li, Y, and Hu, JY (2013). On-site weapreference for two types of cooling vest in combating heat stress. In Ahmed, SM, Smith,N,Azhar, S,Shah,ConferencePaper Proceedings of the Seventh International Conference on Construction in the 21st Century, Challenges in InnoConstruction & Engineering, CITC-VII , December 19-21, 2013, Bangkok, Thailand, Paper 17, ID92, p.11.Doctoral Thesis 1 Chan, APC (1996) Determinants of Project Success in the Construction Industry of Hong Kong, Unpublished PhD T247 pages.MSc Thesis 1 Chan, APC (1983) Materials Control on Confined Sites, Unpublished MSc Thesis, The University of Aston in BirminghaBRE Awards for Outstanding Achievement in Research Funding 2012/13HKIS Sponsorship for writing textbooks (2013)CIOB International Innovation and Research Awards 2012: Health and Safety AwardThe Chartered Institute of Building International Innovation and Research Awards 2012: Health and Safety Awartolerance time to optimal recovery time: a heat stress model for construction rebar workers in Hong Kong”.BRE Research Publication Award (2011/12) in the Category of Chair Professor/ProfessorDepartment of Building and Real Estate in recognition of publishing the highest number of scholarly and research ouBRE Research Publication Award (2011/12) in the Category of Chair Professor/Professo rDepartment of Building and Real Estate in recognition of publishing the highest number of scholarly and research ouDean's Award for Outstanding Achievement in Research Funding (2011/12)Faculty of Construction and Environment in recognition of the outstanding achievement in research funding.Fifteen (15) Years Long Service AwardThe Hong Kong Polytechnic University in appreciation of the service rendered.BRE Research Publication Award (2010/11) in the Category of Chair Professor/ProfessorDepartment of Building and Real Estate in recognition of publishing the highest number of scholarly and research ouDepartmental Award for Outstanding Achievement in Academic Programme Development (2010/11)Department of Building and Real Estate in recognition of the outstanding achievement in academic programmeReal Estate (Team Award).Dean’s Award for Outstanding Achievement in Academic Programme Development 2010Faculty of Construction and Environment in recognition of the outstanding achievement in academic progrManagement (Team Award).National Excellent Papers on Construction Economy (2008-09), the People’s Republic of China (PRC)In recognition of the paper “Risk allocation in infrastructure public-private partnership (PPP) projects”. ConstructionOutstanding Professional Services and Innovation Awards 2008Institute for Enterprise, The Hong Kong Polytechnic University – International Award for Rapid Demountable PlatforThe Innovation Achievers Award for 2008/09The Chartered Institute of Building International Innovation and Research Awards 2008/09 in recognition of the pap Platform for undertaking building repair and maintenance works at height in Hong Kong”.Dean's Award for Outstanding Achievement in Research Funding 2008Faculty of Construction and Land Use in recognition of the outstanding achievement in research funding.Gold Medal with the Congratulations of the Jury, 200836th International Exhibition Inventions, New Techniques and Product, Geneva, Switzerland, in recognition of Platform (RDP©).Award of High Scientific and Technological Level of the Invention, 2008Romania Ministry of Education, Research and Youth, Presented by Romania Ambassador Ionel Sava to Switzerla Rapid Demountable Platform (RDP©).Doing Business Award, 2008The World Bank and the International Finance Corporation in recognition of 4 years of Partnership.Outstanding Performance, Team Award (Teaching), 2003Conferred by the Faculty of Construction and Environment, in recognition of the Outstanding Delivery of the MSc P Chongqing, PRC through Distance Learning Mode.Runner-up Award, CIOB Research Paper Competition 2001Conferred by the Chartered Institute of Building, U.K., for a success factors for partnering projects: a Hong Kong perspecti Gold Prize, Year 2000 Safety Paper CompetitionConferred by the Safety Specialist Group, Hong Kong Institution of Engineers for a paper titled "Impact of safety pro 1995 Fred Wilson Memorial PrizeConferred by the Australian Institute of Building, in reco “Managerial responsibilities of project managers”。
一种新的部分神经进化网络的股票预测(英文)
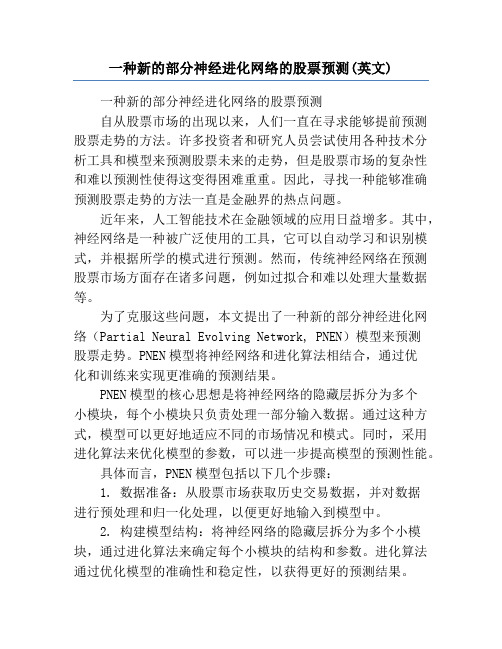
一种新的部分神经进化网络的股票预测(英文)一种新的部分神经进化网络的股票预测自从股票市场的出现以来,人们一直在寻求能够提前预测股票走势的方法。
许多投资者和研究人员尝试使用各种技术分析工具和模型来预测股票未来的走势,但是股票市场的复杂性和难以预测性使得这变得困难重重。
因此,寻找一种能够准确预测股票走势的方法一直是金融界的热点问题。
近年来,人工智能技术在金融领域的应用日益增多。
其中,神经网络是一种被广泛使用的工具,它可以自动学习和识别模式,并根据所学的模式进行预测。
然而,传统神经网络在预测股票市场方面存在诸多问题,例如过拟合和难以处理大量数据等。
为了克服这些问题,本文提出了一种新的部分神经进化网络(Partial Neural Evolving Network, PNEN)模型来预测股票走势。
PNEN模型将神经网络和进化算法相结合,通过优化和训练来实现更准确的预测结果。
PNEN模型的核心思想是将神经网络的隐藏层拆分为多个小模块,每个小模块只负责处理一部分输入数据。
通过这种方式,模型可以更好地适应不同的市场情况和模式。
同时,采用进化算法来优化模型的参数,可以进一步提高模型的预测性能。
具体而言,PNEN模型包括以下几个步骤:1. 数据准备:从股票市场获取历史交易数据,并对数据进行预处理和归一化处理,以便更好地输入到模型中。
2. 构建模型结构:将神经网络的隐藏层拆分为多个小模块,通过进化算法来确定每个小模块的结构和参数。
进化算法通过优化模型的准确性和稳定性,以获得更好的预测结果。
3. 训练模型:使用历史数据集对模型进行训练,并通过反向传播算法来更新模型的权重和偏置。
同时,通过与进化算法的交互,不断调整模型结构和参数。
4. 预测结果:使用训练好的模型对未来的股票走势进行预测。
通过模型对市场的分析和判断,可以为投资者提供决策参考。
为了验证PNEN模型的效果,我们在实际的股票市场数据上进行了实验。
结果表明,与传统神经网络模型相比,PNEN 模型在预测股票走势方面具有更好的准确性和稳定性。
人工智能岗位招聘笔试题与参考答案(某大型集团公司)
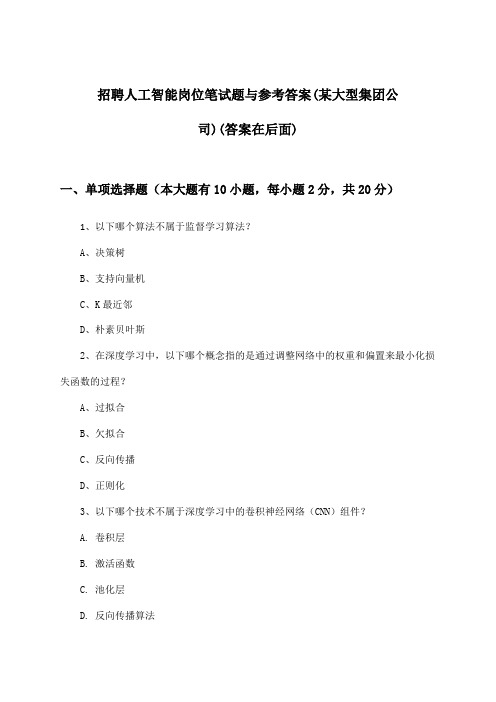
招聘人工智能岗位笔试题与参考答案(某大型集团公司)(答案在后面)一、单项选择题(本大题有10小题,每小题2分,共20分)1、以下哪个算法不属于监督学习算法?A、决策树B、支持向量机C、K最近邻D、朴素贝叶斯2、在深度学习中,以下哪个概念指的是通过调整网络中的权重和偏置来最小化损失函数的过程?A、过拟合B、欠拟合C、反向传播D、正则化3、以下哪个技术不属于深度学习中的卷积神经网络(CNN)组件?A. 卷积层B. 激活函数C. 池化层D. 反向传播算法4、在自然语言处理(NLP)中,以下哪种模型通常用于文本分类任务?A. 决策树B. 朴素贝叶斯C. 支持向量机D. 长短期记忆网络(LSTM)5、题干:以下哪项不属于人工智能的核心技术?A. 机器学习B. 深度学习C. 数据挖掘D. 计算机视觉6、题干:以下哪个算法在处理大规模数据集时,通常比其他算法更具有效率?A. K最近邻(K-Nearest Neighbors, KNN)B. 支持向量机(Support Vector Machines, SVM)C. 决策树(Decision Tree)D. 随机森林(Random Forest)7、以下哪个技术不属于深度学习领域?A. 卷积神经网络(CNN)B. 支持向量机(SVM)C. 递归神经网络(RNN)D. 随机梯度下降(SGD)8、以下哪个算法不是用于无监督学习的?A. K-均值聚类(K-means)B. 决策树(Decision Tree)C. 主成分分析(PCA)D. 聚类层次法(Hierarchical Clustering)9、以下哪个技术不属于深度学习中的神经网络层?A. 卷积层(Convolutional Layer)B. 循环层(Recurrent Layer)C. 线性层(Linear Layer)D. 随机梯度下降法(Stochastic Gradient Descent)二、多项选择题(本大题有10小题,每小题4分,共40分)1、以下哪些技术或方法通常用于提升机器学习模型的性能?()A、特征工程B、数据增强C、集成学习D、正则化E、迁移学习2、以下关于深度学习的描述,哪些是正确的?()A、深度学习是一种特殊的机器学习方法,它通过多层神经网络来提取特征。
利用深度学习的HEVC帧内编码单元快速划分算法
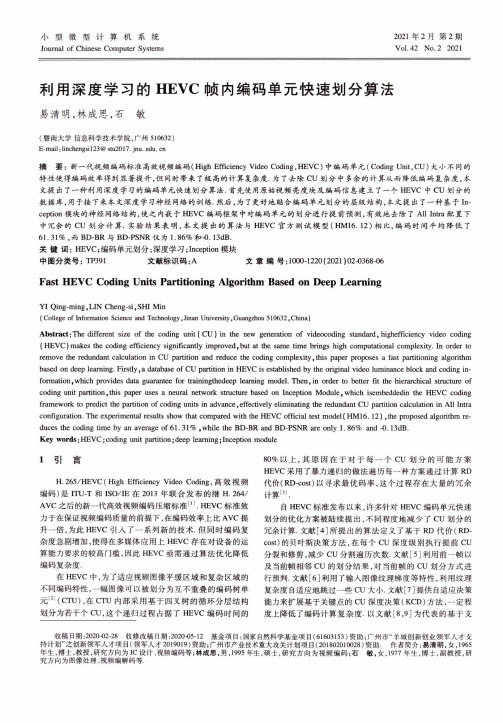
2021年2月第2期Vol. 42 No. 2 2021小型微 型计算 机系统Journal of Chinese Computer Systems利用深度学习的HEVC 帧内编码单元快速划分算法易清明,林成思,石敏(暨南大学信息科学技术学院,广州510632)E-mail :linchengsil23@ stu2017. jnu. edu. cn摘 要:新一代视频编码标准高效视频编码(High Efficiency Video Coding,HEVC)中编码单元(Coding Unit,CU )大小不同的特性使得编码效率得到显著提升,但同时带来了极高的计算复杂度.为了去除CU 划分中多余的计算从而降低编码复杂度,本 文提出了一种利用深度学习的编码单元快速划分算法.首先使用原始视频亮度块及编码信息建殳了一个HEVC 中CU 划分的 数据库,用于接下来本文深度学习神经网络的训练.然后,为了更好地砧合编码单元划分的层级结构,本文提出了一种基于In ception 模块的神经网络结构,使之内嵌于HEVC 编码框架中对编码单元的划分进行提前预测,有效地去除了 All Intra 配置下中冗余的CU 划分计算.实验结果表明,本文提出的算法与HEVC 官方测试模型(HM16. 12)相比,编码时间平均降低了61.31% ,而 BD-BR 与 BD-PSNR 仅为 1.86% 和O 13dB.关键词:HEVC ;编码单元划分;深度学习;Inception 模块中图分类号:TP391文献标识码:A 文章编号:1000-1220(2021 )02-0368-06Fast HEVC Coding Units Partitioning Algorithm Based on Deep LearningYI Qing-ming , LIN Cheng-si , SHI Min(College of Information Science and Technology ,Jinan University ,Guangzhou 510632,China)Abstract : The different size of the coding unit ( CU ) in the new generation of videocoding standard , highefficiency video coding (HEVC ) makes the coding efficiency significantly improved , but at the same time brings high computational complexity. In order toremove the redundant calculation in CU partition and reduce the coding complexity , this paper proposes a fast partitioning algorithmbased on deep learning. Firstly , a database of CU partition in HEVC is established by the original video luminance block and coding in formation ,which provides data guarantee for trainingthedeep learning model. Then , in order to better fit the hierarchical structure of coding unit partition , this paper uses a neural network structure based on Inception Module , which isembeddedin the HEVC coding framework to predict the partition of coding units in advance , effectively eliminating the redundant CU partition calculation in All Intraconfiguration. The experimental results show that compared with the HEVC official test model ( HM16. 12) ,the proposed algorithm re duces the coding time by an average of 61.31% .while the BD-BR and BD-PSNR are only 1.86% and -0.13dB.Key words : HEVC ; coding unit partition ; deep learning ; Inception module1引言H. 265/HEVC ( High Efficiency Video Coding ,高效视频编码)是ITU-T 和ISO/IE 在2013年联合发布的继H. 264/ AVC 之后的新一代高效视频编码压缩标准⑴.HEVC 标准致 力于在保证视频编码质量的前提下,在编码效率上比AVC 提升一倍,为此HEVC 引入了一系列新的技术.但同时编码复杂度急剧增加,使得在多媒体应用上HEVC 存在对设备的运算能力要求的较高门槛,因此HEVC 亟需通过算法优化降低编码复杂度.在HEVC 中,为了适应视频图像平缓区域和复杂区域的不同编码特性,一幅图像可以被划分为互不重叠的编码树单 元[21(CTU),在CTU 内部采用基于四叉树的循环分层结构 划分为若干个CU,这个递归过程占据了 HEVC 编码时间的80%以上,其原因在于对于每一个CU 划分的可能方案 HEVC 采用了暴力递归的做法遍历每一种方案通过计算RD代价(RD-cost)以寻求最优码率,这个过程存在大量的冗余 计算⑶.自HEVC 标准发布以来,许多针对HEVC 编码单元快速 划分的优化方案被陆续提出,不同程度地减少了 CU 划分的 冗余计算.文献[4]所提出的算法定义了基于RD 代价(RD-cost) 的贝叶斯决策方法, 在每个 CU 深度级别执行提前CU分裂和修剪,减少CU 分割遍历次数.文献[5]利用前一帧以 及当前帧相邻CU 的划分结果,对当前帧的CU 划分方式进行预判.文献[6]利用了输入图像纹理梯度等特性,利用纹理复杂度自适应地跳过一些CU 大小.文献[7 ]提供自适应决策 能力来扩展基于关键点的CU 深度决策(KCD)方法,一定程度上降低了编码计算复杂度.以文献[8,9]为代表的基于支收稿日期;2020-02-28收修改稿日期:202045-12基金项目:国家自然科学基金项目(61603153)资助;广州市“羊城创新创业领军人才支 持计划”之创新领军人才项目(领军人才2019019)资助;广州市产业技术重大攻关计划项目(201802010028)资助.作者简介:易清明,女,1965 年生,博士,教授,研究方向为IC 设计、视频编码等;林成思,男,1995年生,硕士,研究方向为视频编码;石敏,女,1977年生,博士,副教授,研 究方向为图像处理、视频编解码等.2期易清明等:利用深度学习的HEVC帧内编码单元快速划分算法369持向量机(SVM)的快速CU大小决策算法提取图像特征来判断CU复杂度,然后基于SVM建立CU大小决策的分类器结构.文献[10]同样使用SVM来进行预测帧内CU划分,同时结合帧内角度预测模式,进一步减少帧内预测的计算复杂度.另外,随着深度学习的不断发展,很多深度学习方法被应用到视频编解码的领域.文献[11]使用了一个两层的卷积神经网络结构来预测单个CU是否划分的二分类问题.文献[12]使用了一个更深的3层CNN结构来预测整个CTU的模型,并建立了一个针对CU划分的数据库CPIH,改善了网络的学习能力.上述帧内CU划分快速选择算法都能够在一定程度上减少CU划分冗余计算,缩减编码时间,但仍存在自身缺陷.例如早期启发式方法如文献[4-7]依赖与人为指定的经验特征,无法挖掘CU划分过程中的深层次特征.文献[8-10]利用的SVM的方法学习能力有限,相比深度学习模型在预测能力上稍显不足,准确率不能得到保证.而深度学习方法如文献[11,12]等也存在着一些问题,如分开使用3个同样的CNN来分别预测3种大小的CU划分,在不同深度的CU划分中没有建立联系;同时每次网络只判断一层的CU划分情况,需要反复调用多次才能预测出整个CTU的划分,仍有改进的空间.本文针对以上问题,提出一种基于深度学习编码单元快速划分算法.首先与一些三级分类器方法需要反复调用分类器不同的是,本文方法一次性预测整个CTU的CU划分情况,将同一个CTU中不同深度的CU划分建模为一个整体;另外,为了更好地适应CU划分的层级结构,本文算法使用了一种基于Inception模块的神经网络结构,使之内嵌于HEVC编码框架中,直接根据亮度像素值对编码单元的划分进行提前预测,有效地去除了All Intra配置下中冗余的CU 划分计算.2基于深度学习的快速编码单元划分算法与上一代编码标准H.264相比,HEVC新引入了通过四叉树方式灵活划分的树形结构单元(CTU),-个CTU大小一般为64x64,可以划分为不同大小编码单元(CU).为了贴合不同视频图像的纹理特征,每个CU共设有4种可能划分尺寸:64x64、32x32、16X16和8x8.图1为CTU划分为图1HEVC CU划分结构示意图Fig.1HEVC CU Splitting StructureCU的示意图.本文深度学习模型以整个CTU的亮度块像素作为输入,各个尺寸的CU分割模式作为输出,经过训练学习以获得较为准确的CU划分预测效果.2.1划分模式建模根据HEVC中CTU的分割结构,经过划分之后一个CU 最多可能有4种大小,包括64x64,32x32,16x16和8x8,分别对应于CU划分的深度0、1、2和3.如图2所示,我们用Ze10,1,2,3)来表示不同深度,用y,来表示在深度/-1的CU是否要划分为深度为I的子CU这一划分决策,例如几代表64x64的CU是否要划分为32x32的子CU这一决策.对于每一个大小316X16的CU来说,都存在一个“是否要划分为更小的CU”的决策.为了更好地区分不同深度、同一深度不同位置的CU划分决策,本文分别用几、{%.,}:“、{".,显”。
Neural Networks Mimicking the Mind
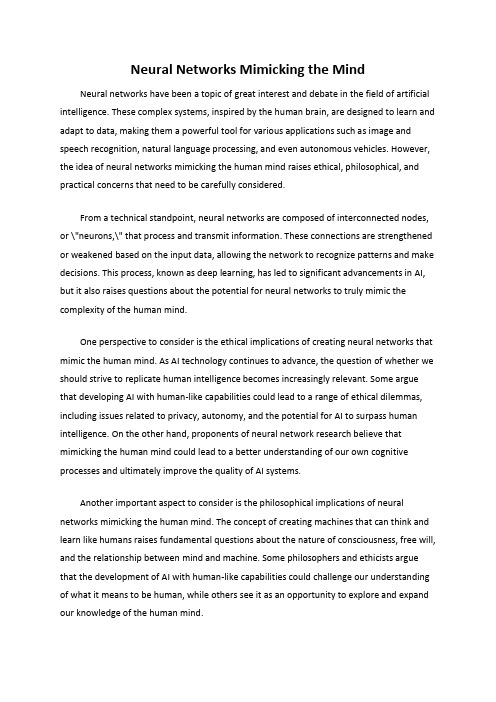
Neural Networks Mimicking the Mind Neural networks have been a topic of great interest and debate in the field of artificial intelligence. These complex systems, inspired by the human brain, are designed to learn and adapt to data, making them a powerful tool for various applications such as image and speech recognition, natural language processing, and even autonomous vehicles. However, the idea of neural networks mimicking the human mind raises ethical, philosophical, and practical concerns that need to be carefully considered.From a technical standpoint, neural networks are composed of interconnected nodes, or \"neurons,\" that process and transmit information. These connections are strengthened or weakened based on the input data, allowing the network to recognize patterns and make decisions. This process, known as deep learning, has led to significant advancements in AI, but it also raises questions about the potential for neural networks to truly mimic the complexity of the human mind.One perspective to consider is the ethical implications of creating neural networks that mimic the human mind. As AI technology continues to advance, the question of whether we should strive to replicate human intelligence becomes increasingly relevant. Some argue that developing AI with human-like capabilities could lead to a range of ethical dilemmas, including issues related to privacy, autonomy, and the potential for AI to surpass human intelligence. On the other hand, proponents of neural network research believe that mimicking the human mind could lead to a better understanding of our own cognitive processes and ultimately improve the quality of AI systems.Another important aspect to consider is the philosophical implications of neural networks mimicking the human mind. The concept of creating machines that can think and learn like humans raises fundamental questions about the nature of consciousness, free will, and the relationship between mind and machine. Some philosophers and ethicists argue that the development of AI with human-like capabilities could challenge our understanding of what it means to be human, while others see it as an opportunity to explore and expand our knowledge of the human mind.From a practical standpoint, the potential applications of neural networks that mimic the human mind are vast and varied. For example, in the field of healthcare, AI systems with human-like cognitive abilities could revolutionize medical diagnosis and treatment planning. Similarly, in the realm of customer service, AI chatbots that can understand and respond to human emotions and intentions could greatly improve the user experience. However, these advancements also raise concerns about the potential for AI to replace human jobs and the need for regulations to ensure the responsible use of this technology.In conclusion, the idea of neural networks mimicking the human mind is a complex and multifaceted issue that requires careful consideration from technical, ethical, philosophical, and practical perspectives. While the potential benefits of developing AI with human-like capabilities are significant, it is essential to approach this technology with a thoughtful and cautious mindset. As we continue to explore the potential of neural networks, it is crucial to engage in open and honest discussions about the implications and limitations of creating machines that can think and learn like humans. Only by doing so can we ensure that AI technology is developed and used in a way that aligns with our values and benefits society as a whole.。
2024湖南省长沙市长郡中学高考适应考试(四)英语试题及答案
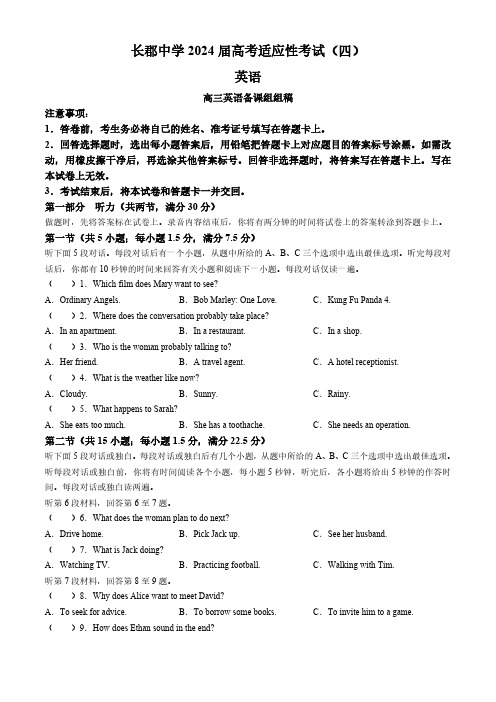
长郡中学2024届高考适应性考试(四)英语高三英语备课组组稿注意事项:1.答卷前,考生务必将自己的姓名、准考证号填写在答题卡上。
2.回答选择题时,选出每小题答案后,用铅笔把答题卡上对应题目的答案标号涂黑。
如需改动,用橡皮擦干净后,再选涂其他答案标号。
回答非选择题时,将答案写在答题卡上。
写在本试卷上无效。
3.考试结束后,将本试卷和答题卡一并交回。
第一部分听力(共两节,满分30分)做题时,先将答案标在试卷上。
录音内容结束后,你将有两分钟的时间将试卷上的答案转涂到答题卡上。
第一节(共5小题;每小题1.5分,满分7.5分)听下面5段对话。
每段对话后有一个小题,从题中所给的A、B、C三个选项中选出最佳选项。
听完每段对话后,你都有10秒钟的时间来回答有关小题和阅读下一小题。
每段对话仅读一遍。
()1.Which film does Mary want to see?A.Ordinary Angels. B.Bob Marley: One Love. C.Kung Fu Panda 4.()2.Where does the conversation probably take place?A.In an apartment. B.In a restaurant. C.In a shop.()3.Who is the woman probably talking to?A.Her friend. B.A travel agent. C.A hotel receptionist.()4.What is the weather like now?A.Cloudy. B.Sunny. C.Rainy.()5.What happens to Sarah?A.She eats too much.B.She has a toothache.C.She needs an operation.第二节(共15小题;每小题1.5分,满分22.5分)听下面5段对话或独白。
nativememorytracking使用
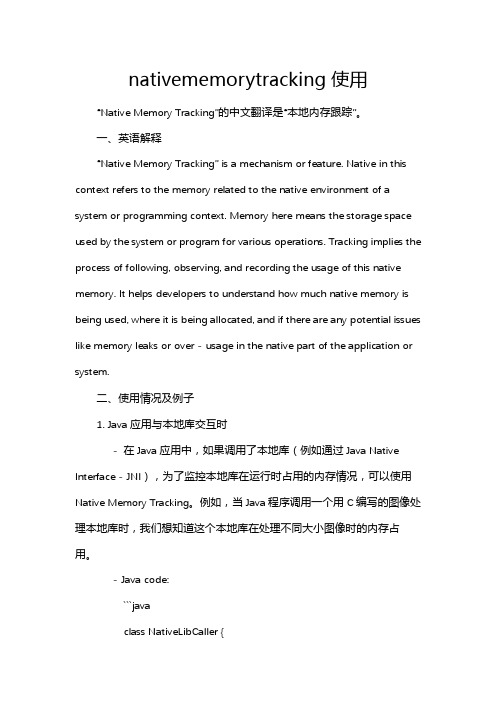
-在嵌入式系统中,如运行在微控制器上的代码,可能有一部分是用C编写的本地代码来控制硬件设备。如果出现内存相关的故障,Native Memory Tracking可以帮助定位是本地代码的哪部分出现了问题。
- Suppose an embedded system controls a temperature sensor and a display. The code that interfaces with these hardware components, written in C, can be monitored using Native Memory Tracking if there are memory - related issues like the system crashing due to lack of memory.
- Java code:
```java
class NativeLibCaller {
static {
System.loadLibrary("image_processing_lib");
}
public native void processImage(byte[] imageData);
}
```
- In this case, if we suspect memory issues related to the native library when processing images, we can use Native Memory Tracking to diagnose.
二、使用情况及例子
1. Java应用与本地库交互时
-在Java应用中,如果调用了本地库(例如通过Java Native Interface - JNI),为了监控本地库在运行时占用的内存情况,可以使用Native Memory Tracking。例如,当Java程序调用一个用C编写的图像处理本地库时,我们想知道这个本地库在处理不同大小图像时的内存占用。
大脑的英语作文
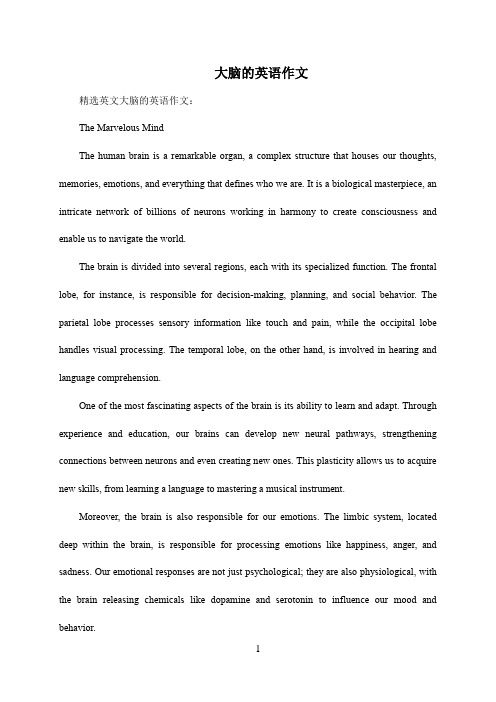
大脑的英语作文精选英文大脑的英语作文:The Marvelous MindThe human brain is a remarkable organ, a complex structure that houses our thoughts, memories, emotions, and everything that defines who we are. It is a biological masterpiece, an intricate network of billions of neurons working in harmony to create consciousness and enable us to navigate the world.The brain is divided into several regions, each with its specialized function. The frontal lobe, for instance, is responsible for decision-making, planning, and social behavior. The parietal lobe processes sensory information like touch and pain, while the occipital lobe handles visual processing. The temporal lobe, on the other hand, is involved in hearing and language comprehension.One of the most fascinating aspects of the brain is its ability to learn and adapt. Through experience and education, our brains can develop new neural pathways, strengthening connections between neurons and even creating new ones. This plasticity allows us to acquire new skills, from learning a language to mastering a musical instrument.Moreover, the brain is also responsible for our emotions. The limbic system, located deep within the brain, is responsible for processing emotions like happiness, anger, and sadness. Our emotional responses are not just psychological; they are also physiological, with the brain releasing chemicals like dopamine and serotonin to influence our mood and behavior.The brain's complexity is truly breathtaking. It is a universe of its own, a vast network of interconnected neurons, synapses, and glial cells, all working together to create the unique individual that we are. Despite the advances in neuroscience, there is still much we don't know about the brain. It remains a frontier of scientific exploration, a mysterious and fascinating organ that continues to inspire awe and wonder.中文对照翻译:奇妙的心灵人脑是一个非凡的器官,一个复杂的结构,容纳着我们的思想、记忆、情感以及定义我们是谁的一切。
外星人mog自画像英语作文

外星人mog自画像英语作文Mog, the alien from the distant planet Zorkon, gazed out at the strange new world he had found himself in. The sky was a brilliant blue, unlike the deep purple hues he was accustomed to back home, and the strange bipedal creatures that scurried about seemed so foreign and unfamiliar. As he contemplated his new surroundings, Mog decided it was time to create a self-portrait to document this remarkable journey.First, Mog took stock of his physical appearance. He stood nearly 2 meters tall, with a slender, lanky frame covered in smooth lavender skin. His head was large and oblong, with two large, almond-shaped eyes that seemed to take in the world around him with a sense of wonder and curiosity. Instead of a traditional mouth, Mog had a small slit-like orifice that he used to intake sustenance, and where a human's nose would be, Mog had two small nostril-like openings.Protruding from the top of Mog's head were two antenna-like appendages that he used to sense and interpret the electromagnetic fields and energy signatures of his surroundings. These antennacould rotate and swivel, allowing Mog to gain a comprehensive understanding of his environment. Additionally, Mog had four long, spindly fingers on each hand, each tipped with a sharp claw-like nail that he could use for delicate manipulations or self-defense if needed.As Mog continued to examine himself, he couldn't help but marvel at the sheer complexity of his own biology. Unlike the carbon-based life forms he had encountered on this strange new planet, Mog's physiology was based on a completely different elemental foundation. His internal organs and circulatory system relied on a unique silicon-based structure, and his metabolism was fueled by the absorption of specific radiation wavelengths rather than the consumption of organic matter.Despite these fundamental differences, Mog couldn't help but feel a sense of kinship with the other sentient beings he had observed. There was a spark of intelligence and curiosity in their eyes that resonated with Mog, and he longed to learn more about their customs, their technologies, and their ways of life. Perhaps, he mused, there were more similarities between them than differences.As Mog continued to ponder these thoughts, he decided to document another aspect of his self-portrait – his unique cognitive and emotional capacities. Unlike the bipedal creatures, Mog's brainwas not confined to a single, centralized organ. Instead, his neural network was distributed throughout his entire body, with various processing centers located in his limbs, torso, and even his antenna.This decentralized system allowed Mog to process information and make decisions with a level of speed and efficiency that would be unimaginable to a human. He could simultaneously consider multiple perspectives, weigh the potential consequences of his actions, and formulate complex strategies – all while maintaining a heightened awareness of his surroundings.Additionally, Mog's emotional range was far more nuanced and multifaceted than that of the beings he had observed. He could experience a symphony of feelings, from the deep, contemplative wonder he felt when gazing up at the stars, to the intense, almost primal sense of awe he experienced when confronted with a new and unfamilable phenomenon. These emotions were not mere reactions, but rather integral components of Mog's decision-making process, guiding his actions and shaping his understanding of the world around him.As Mog delved deeper into his self-portrait, he realized that the true essence of who he was went far beyond his physical form or his cognitive capabilities. At the very core of his being was a profound sense of curiosity, a burning desire to explore the unknown and tounravel the mysteries of the universe. It was this insatiable thirst for knowledge and understanding that had driven him to venture out from his home planet, to traverse the vast expanse of space, and to find himself in this strange new world.And as Mog continued to observe the bipedal creatures around him, he couldn't help but feel a sense of kinship and connection. For despite their apparent differences, they too seemed to possess that same spark of curiosity, that same drive to understand and explore the world around them. Perhaps, Mog mused, they were not so different after all.With a renewed sense of purpose, Mog turned his attention to the final aspect of his self-portrait – his role and purpose in this new and unfamiliar environment. He knew that as an alien, he would face challenges and obstacles, both practical and cultural, as he sought to integrate himself into this strange new world. But he was undaunted, for he was driven by a deep-seated belief in the power of communication, cooperation, and mutual understanding.Mog knew that the key to bridging the divide between himself and the bipedal creatures would lie in his ability to learn their language, to understand their customs and traditions, and to find common ground upon which they could build a relationship of mutual respect and collaboration. And so, with a determined gleam in his eyes, Mogset out to do just that, ready to embark on a journey of discovery and understanding that would forever change the course of his life.As Mog put the finishing touches on his self-portrait, he couldn't help but feel a sense of pride and accomplishment. He had captured the essence of who he was, from his physical form to his cognitive and emotional capacities, and had even begun to envision his role and purpose in this new and fascinating world. And as he gazed out at the strange bipedal creatures that bustled about, Mog felt a sense of hope and excitement for the adventures that lay ahead.For in this moment, Mog knew that he was not just an alien, a stranger in a strange land – he was a curious and intrepid explorer, a seeker of knowledge and understanding, and a bridge-builder between two vastly different worlds. And as he stepped out into the unknown, he did so with a sense of purpose and determination that would guide him on the journey of a lifetime.。
- 1、下载文档前请自行甄别文档内容的完整性,平台不提供额外的编辑、内容补充、找答案等附加服务。
- 2、"仅部分预览"的文档,不可在线预览部分如存在完整性等问题,可反馈申请退款(可完整预览的文档不适用该条件!)。
- 3、如文档侵犯您的权益,请联系客服反馈,我们会尽快为您处理(人工客服工作时间:9:00-18:30)。
A Neural Network decision module for the Adaptive Strategic PlanningFramework in RoboCupNikolaos Kalyviotis and Huosheng HuDepartment of Computer Science, University of Essex,Wivenhoe Park, Colchester CO4 3SQ, UKEmail: nkalyv@, hhu@Abstract: Co-operation and decision making play important roles in Multi-Agent Systems(MAS). This paper proposes a new decision module for the Adaptive Strategic PlanningFramework (ASPF) [1] in the RoboCup domain [4]. This new module is to improve the co-operative behaviour of the Essex Wizards simulation team that has been based on a purelyanalytical approach in the past. The open architecture of the ASPF shows how artificial neuralnetworks (ANNs) can be used in the decision module for a good performance. It also shows thatthe neural network decision module can outperform the decision module that is based on handcoded one.Keywords: Neural Networks, Multi-robot Systems, Simulation, RoboCup1. IntroductionRoboCup (Robot Soccer) is an initiative that started six years ago. The purpose of this initiative is to promote Artificial Intelligence (AI) and robotics research [4], providing a common test-bed (soccer), where various agent theories and algorithms can be tested. The technologies that are involved in RoboCup cover a wide spectrum of research such as design principles of autonomous agents, multi-robot collaboration, strategy acquisition, real-time reasoning and planning, intelligent robotics, sensor-fusion [3, 5, 6]. RoboCup is divided in four main leagues, namely Simulation league, Small size robots league, Middle size robots league, and Sony legged robot league.In Simulation league agents are purely synthetic and have great flexibility and many design and implementation possibilities. In addition the domain that RoboCup provides is very interesting for MAS research, since it comprises of a unique combination of characteristics [7]. The fundamental issue for RoboCup is to design a multi-agent system that behaves in real time, performing reasonable goal-directed behaviours. As the situations of the game change quickly and the state-space is huge, soccer agents should be able to play the game strategically [6]. Therefore, three challenges have to be accomplished: learning challenge, teamwork challenge, and opponent modelling challenge.In MAS planning is an important element that can result in efficient co-operative behaviours. In general, planning concerns the process for people to select a course of action, making choices, formulating and revising plans, dealing with problems and adversity, and eventually performing some actions [8]. As planning involves reasoning about actions, it has been approached by an AI point of view. This approach that begun in late sixties and early seventies gave birth to the “classical AI planning problem”[9]. Sometimes however, planning systems do not have to create a plan. They just have to retrieve a plan from their memory that is applicable to the problem they are trying to solve. These plans are called “canned plans” by Wilensky [8].The human brain can be considered as a very powerful and complex parallel computer [10]. Therefore it would be very beneficial if we could simulate the functionality of such a powerful processing system. The need to understand and to simulate the way that the human brains work, lead to the introduction of neural networks that can be considered as information processing systems. In general, ANNs are used to develop adaptive systems. An ANN is a massively distributedProc. of International Symposiumon Robotics and Automation, Toluca, Mexico, 1-4 September 2002processor that has a natural property for storing experiential knowledge and making it available for use. It resembles the brain in two respects. The first one is the knowledge that is acquired by ANNs through a learning process. The second one is the inter-neuron connection strength, known as synaptic weights, which are used to store the knowledge.The efficiency and power of ANNs have made them very popular. As a result of that ANNs have been used in many different kind of applications with great success, including classification, pattern matching, pattern completion, noise removal, optimisation and control [11]. For example in the medical area, ANNs have been used in the diagnosis and treatment of breast cancer [13]. In economics ANNs have been used in order to forecast the prices of the shares, and also to allocate assets in the global bond markets [14]. ANNs have also been used in telecommunications. An example is a system, which uses ANNs for routing traffic [15].In this paper, ANNs have been adopted to implement a decision module for the Adaptive Strategic Planning Framework (ASPF) in the RoboCup domain. In the next section the basic idea of the ASPF is briefly outlined. Section 3 describes how a decision module based on ANNs can be built. Some experimental results are presented in section 4. Finally brief conclusions and future work are summarised in section 5.2. Overview of the ASPFThe ASPF [1] is an extension of the Basic Strategic Planning [17], involves a mapping of“plans”to “situations”. It can be seen as many-to-one relationship such that a set of particular plans is mapped to a particular situation. A plan is mapped (or assigned) to a situation only if that situation is important. For instance, the importance of a situation can be determined by the frequency of its occurrence. An important situation is called “Strategic Situation”and the plans mapped to that are called “Global Strategic Plans”. In RoboCup, like in any other MAS, there are certain situations that occur repeatedly. Therefore a great advantage can be gained if plans are devised for such situation beforehand.In the Essex Wizards team, ASPF is used before the agent enters the actual thinking process (figure 1). If an agent is a part of a Global Strategic Planning (GSP) that can be executed, then the ASPF module will produce an action and sent it to the actuators. Otherwise the agent will enter the thinking process, and then the thinking process will produce an action and send it to the actuators.A situation that happens many times during a game is the “goalie clearance”situation. For this strategic situation we have devised 10 different GSPs that can be executed, as can be seen in figure 2.At some points the goalie should make a decision of which GSP will be executed. So far an analytical (hand coded) approach has been used. This approach is based on a risk factor RG (X) which shows how risky is to execute GSP X. In the process of decision between the ten GSPs, the risk factor of each GSP (RG) is calculated and then the GSP with the least RG value is chosen providing that the wining RG value is below a threshold that we have set. This decision module was used in the Essex Wizards 2001 simulation team [2]. If the winning value is above that threshold then no GSP is chosen. In the following section we will see how the decision module can be built using ANNs.Fig. 1. A high level view of the Essex Wizards agent3. Neural Network ApproachThe problem of choosing the best GSP in the “goalie clearance”situation can be viewed as a classification problem since each GSP can be classified as a good or a bad choice according to the state of the environment. In this section we will show how neural networks were used in order to build a decision mechanism for the goalie clearance situation.3.1 The methodologyWhen the goalie catches the ball, it can keep it no more than 250 cycles. During those 250 cycles the environment changes and therefore the effectiveness of each GSP changes as well. For instance at time T a good choice could be GSP 7, while at time T+100 GSP 10 could be the best choice to make. If we assume that an evaluation function E(x, t) gives the effectiveness of GSP X at time T, then during the 250 cycles there will be a cycle T best and a GSP X best that will produce the maximum value for E(x, t). In other words GSP X best at cycle T best will be the best choice during the 250 cycles that the goalie can keep the ball. As it was mentioned earlier on, the environment can change in every cycle and therefore at time T best the environment will be at state S best. So every time that the goalie catches the ball (i.e. in each “goalie clearance” situation) we can associate state S best with GSP X best (S best, GSP X best). If we gather many sets of such data, we can use them in order to train a neural network that uses the S best data as training examples and the GSP X best data as the correct output. Then the neural network should be able to decide whether at a state S one of the GSPs should be executed or not.3.2 The evaluation functionIn order to find the GSP that performs best at a given time we should be able to evaluate the performance of each GSP and choose the one with the best performance. For that to be done we willTeam-mate Goalie Ball Pass RunFigure 2. Ten GSPs for the “goalie clearance” strategic situationneed an evaluation function E(x, t) that will evaluate the performance of GSP X at time T. The evaluation function that we have constructed uses four criteria that are explained below:a) The risk factor RG(x, t) of GSP X at time T: thisshows how risky is to execute GSP X at time T.The value of RG(x, t) is a real number between 0 and 1. A value close to 1 means that there is a high risk for the GSP X to fail, while a value close to 0 means that the risk to fail is low.b) The congestion CON(x, t) of GSP X at time T: thisshows how much congested of opponents at time T is the player of GSP X that is going to receive the ball last. The value of CON(x, t) is a real number between 0 and 1. A value close to1 denotes a low congestion while a value closeto 0 denotes a high congestion.c) The usefulness USE(x, t) of GSP X at time T: thisshows how much useful is GSP X at time T based on the position of the player that is going to receive the ball last. The value of USE(x, t) isa real number between 0 and 1. A value close to1 denotes a position close to opponent’s goal,while a value close to 0 denotes a position close to our goal.d) The cost COST(x, t) of GSP X at time T: the valueof COST(x, t)is related to the number of passes involved in GSP X and is calculated as follows: COST(x, t) = 1/(number of passes involved in GSP X) Now we can define the evaluation function E(x, t) as follows:E(x, t) = (1-RG(x, t)) * 0.35 + CON(x, t) * 0.35 +USE(x, t) * 0.2 + COST(x, t) * 0.1The outcome of the evaluation function is a real number between 0 and 1. The closer to 1 it is, and the better is the performance of GSP X at time T. The values 0.35, 0.2, and 0.1, show the importance of each factor towards the calculation of E(x, t). For example RG(x, t) and CON(x, t) are the most important factors and therefore they get the value 0.35. The other two factors are less important and so they are assigned lower values.In figure 3 we can see the evaluation of the 10 GSPs for the “goalie clearance” situation in the time period of 250 cycles where the goalie keeps the ball. In this example the best performance is achieved by GSP 10 at time 100 (point A).3.3 The topology of the neural networkThere are three layers in the neural network that we have used, namely input – hidden – output, as shown in figure 4:The input layer: As it was mentioned earlier on in order to train the network we are using sets of (S best, GSP X best) data. The information that we are using in order to describe the state S best of the environmentFigure 3. Evaluation of the 10 GSPs for the “goalie clearance” situationis based on the first twocriteria of theevaluation function,namely the risk factorRG(x, t) and the CON(x, t)congestion. Althoughwe have 10 differentvalues of RG(x, t) for the10 available GSPs, weonly have 6 differentvalues of CON(x, t),since the number ofplayers that receive theball last in the 10 GSPsis 6. For example inGSP 1, GSP 3, GSP 4and GSP 10 the playerwho receives the balllast is always the same.Therefore we can describe the environment state S best by using these 16 numbers. Hence the input layer of the network will have 16 neurons, one neuron for each one of the 16 numbers.The output layer: The output layer of the neural networks has 10 neurons and each neuron corresponds to one GSP. The correct output presented in these neurons is 1 for the node that represents GSP X best and 0 for all other neurons.The hidden layer: The analysis of the results showed that 10 neurons in the hidden layer could produce satisfactory results.3.4 The training of the neural network96 sets of (S best, GSP X best) data were used in order to train the neural network. The algorithm that was used for training is the back propagation algorithm [16].4. Experimental ResultsThe performance of the Neural Network decision module was evaluated through the calculation of the average performance from 100 decisions that were made. The outcome was 0.76, which is quite good since the average performance of the data that were used in order to train the network was 0.8. We also evaluated the previous analytical (hand coded) decision module and a random decision module using the evaluation function E(x,t). It is to compare them with the Neural Network decision module. The average result that we got for the hand coded decision module (from 100 decision that were made) was 0.64 and the average for the random one (from 100 decision that were made) was 0.47 (figure 5). In the random decision module, the goalie keeps the ball for 100 cycles and then chooses randomly one of the 10 possible GSPs.As it was mentioned in section 3.3 the hidden layer consists of 10 neurons. In the experiments, we tried many other numbers of hidden neurons such as 20, 30, 50, 100, and 200. However there was nosignificant change in the performance of the neuralnetwork. Therefore, 10 hidden neurons were chosen since they make the training process of the neural network faster.5 Conclusions and Future WorkThis paper describes how neural networks can be adopted in the Adaptive Strategic Planning Framework that has been developed for the Essex Wizards team to participate in the RoboCup competitions. Integrating a neural network decision module to the ASPF has proved the openness of the framework that has been claimed before. Experimental results shows that a decision module based on neural networks can outperform the hand coded decision module that has been used in the past. During a game the ASPF should make decisions in many other strategic situations like “goal kick” and “crossing”.In the future we will implement the neural network decision technique to all the strategic situations. For each strategic situation a different neural network will make the decisions. References[1] N. Kalyviotis and H. Hu, ASPF: an AdaptiveStrategic Planning Framework in RoboCup,Proceedings IASTED International Conference on Robotics and Applications (RA 2001), Florida, November 2001.[2] H. Hu, K. Kostiadis, M. Hunter and N.Kalyviotis, Essex Wizards 2001 Team Description,In Birk A., Coradeschi S., and Tadokoro S., editors, RoboCup-01: Robot Soccer World Cup V. Springer Verlag, Berlin, 2002.[3] M. Hunter, K. Kostiadis and H. Hu. ABehaviour-based Approach to Position Selection for Simulated Soccer Agents, Proc. of the 1st European RoboCup Workshop, 28 May-2 June 2000.[4] H. Kitano, RoboCup: The Robot World CupInitiative, Proceedings of The First International Conference on Autonomous Agent (Agents-97)), Marina del Ray, The ACM Press, 1997.[5] K. Kostiadis and H. Hu, ReinforcementLearning and Co-operation in a Simulated Multi-agent System, Proc. of IEEE/RSJ Int.Conf. on Intelligent Robots and Systems, pages 990-995, Kyungiu, Korea, 17-21 October 1999.[6] H. Kitano, M. Tambe, P. Stone, M. Veloso, S.Coradeschi, E. Osawa, H. Matsubara, I. Noda, and M. Asada,The RoboCup Synthetic Agent Challenge, 97, Int. Joint Conf. on Artificial Intelligence, 1997.[7] P. Stone, Layered Learning in Multi-AgentSystems, PhD Thesis, Carnegie Mellon University, 1998.[8] R. Wilensky, Planning and Understanding,Addison-Wesley Publishing Company, Massachusetts, 1983[9] D. Wilkins, Practical Planning: Extending theClassical AI Planning Paradigm, Morgan Kaufmann Publishers, California, 1988. [10] S. Haykin, Neural Networks a comprehensivefoundation, Macmillan, USA, 1994.[11] P.K Simpson, Foundations of Neural Networks,San Diego, 1992.[12] I. Aleksander and H. Morton, An Introductionto Neural computing, Chapman & Hall, London, 1990.[13] N.A Bridgett, C.J Harris, Artificial neuralnetworks for use in the diagnosis and treatment of breast cancer, UK, 1995.[14] C. Diamond, J. Shadbolt, M. Azema-Barac andA.N Refenes, Neural network system fortactical asset allocation in the global bond markets, Econostat Ltd, UK, 1993.[15] H.E Rauch and T. Winarske, Neural Networkfor Routing Communication Traffic, 1988. [16] S.I Gallant, Neural Network Learning andexpert systems, MIT Press, USA, 1993 [17] N. Kalyviotis and H. Hu, A Co-operativeFramework for Strategic Planning, Proceedings Towards Intelligent Mobile Robots (TIMR) ’01, Manchester, 2001.。