AUDIOVISUAL SPEECH RECOGNITION WITH ARTICULATOR POSITIONS AS HIDDEN VARIABLES
标贝科技推出声音转换解决方案 AI技术助力音色精准迁移
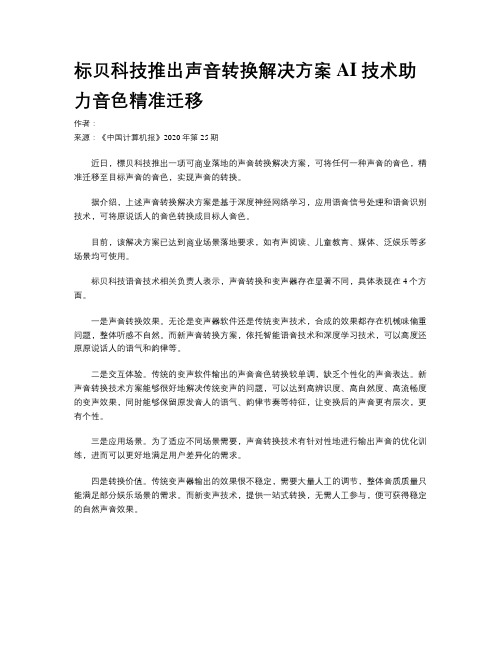
标贝科技推出声音转换解决方案 AI技术助力音色精准迁移
作者:
来源:《中国计算机报》2020年第25期
近日,標贝科技推出一项可商业落地的声音转换解决方案,可将任何一种声音的音色,精准迁移至目标声音的音色,实现声音的转换。
据介绍,上述声音转换解决方案是基于深度神经网络学习,应用语音信号处理和语音识别技术,可将原说话人的音色转换成目标人音色。
目前,该解决方案已达到商业场景落地要求,如有声阅读、儿童教育、媒体、泛娱乐等多场景均可使用。
标贝科技语音技术相关负责人表示,声音转换和变声器存在显著不同,具体表现在4个方面。
一是声音转换效果。
无论是变声器软件还是传统变声技术,合成的效果都存在机械味偏重问题,整体听感不自然。
而新声音转换方案,依托智能语音技术和深度学习技术,可以高度还原原说话人的语气和韵律等。
二是交互体验。
传统的变声软件输出的声音音色转换较单调,缺乏个性化的声音表达。
新声音转换技术方案能够很好地解决传统变声的问题,可以达到高辨识度、高自然度、高流畅度的变声效果,同时能够保留原发音人的语气、韵律节奏等特征,让变换后的声音更有层次,更有个性。
三是应用场景。
为了适应不同场景需要,声音转换技术有针对性地进行输出声音的优化训练,进而可以更好地满足用户差异化的需求。
四是转换价值。
传统变声器输出的效果很不稳定,需要大量人工的调节,整体音质质量只能满足部分娱乐场景的需求。
而新变声技术,提供一站式转换,无需人工参与,便可获得稳定的自然声音效果。
语音识别芯片有哪些
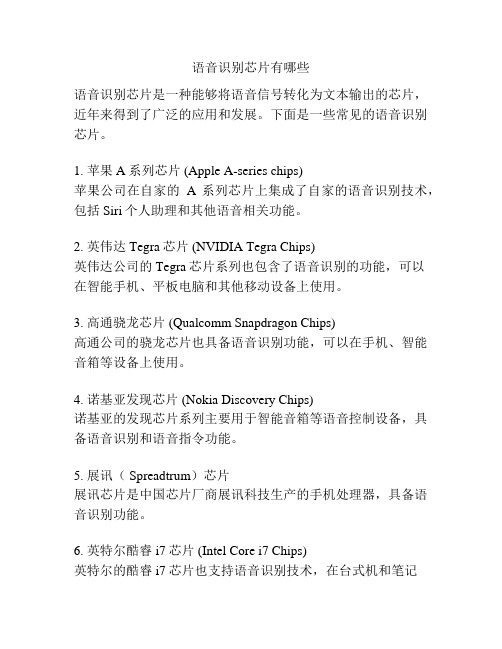
语音识别芯片有哪些语音识别芯片是一种能够将语音信号转化为文本输出的芯片,近年来得到了广泛的应用和发展。
下面是一些常见的语音识别芯片。
1. 苹果A系列芯片 (Apple A-series chips)苹果公司在自家的A系列芯片上集成了自家的语音识别技术,包括Siri个人助理和其他语音相关功能。
2. 英伟达Tegra芯片 (NVIDIA Tegra Chips)英伟达公司的Tegra芯片系列也包含了语音识别的功能,可以在智能手机、平板电脑和其他移动设备上使用。
3. 高通骁龙芯片 (Qualcomm Snapdragon Chips)高通公司的骁龙芯片也具备语音识别功能,可以在手机、智能音箱等设备上使用。
4. 诺基亚发现芯片 (Nokia Discovery Chips)诺基亚的发现芯片系列主要用于智能音箱等语音控制设备,具备语音识别和语音指令功能。
5. 展讯( Spreadtrum)芯片展讯芯片是中国芯片厂商展讯科技生产的手机处理器,具备语音识别功能。
6. 英特尔酷睿 i7芯片 (Intel Core i7 Chips)英特尔的酷睿 i7芯片也支持语音识别技术,在台式机和笔记本电脑中使用。
7. 联发科技( MediaTek)芯片联发科技是台湾的一家芯片设计公司,其芯片也支持语音识别功能,在智能手机和其他智能设备上广泛应用。
8. 德州仪器(Texas Instruments)芯片德州仪器是一家全球性的半导体设计与制造公司,其芯片也集成了语音识别技术,可应用于各种电子设备。
总结:以上是一些常见的语音识别芯片,它们都具备将语音转化为文本的能力,广泛应用于智能手机、智能音箱、智能家居等设备中。
另外,随着人工智能和语音技术的不断发展,未来还会有更多类型的语音识别芯片出现。
基于语音识别的智能音乐播放器设计与实现

基于语音识别的智能音乐播放器设计与实现音乐作为一种文化艺术形式,具有强烈的感染力和文化内涵。
然而,随着科技的发展,传统音乐播放器已经无法满足人们的需求。
因此,本文将探讨一种基于语音识别的智能音乐播放器的设计与实现,旨在提升音乐播放的体验,为人们带来更多乐趣。
一、背景分析传统的音乐播放器只能通过按钮进行操作,限制了人们使用的手势和时间。
随着人工智能技术的不断发展,语音识别技术逐渐成熟,人们可以通过语音命令来实现音乐播放。
因此,基于语音识别的智能音乐播放器成为了一个新的领域。
二、智能音乐播放器的设计1. 硬件设备智能音乐播放器需要具备麦克风,扬声器,处理器等硬件设备。
其中,麦克风用于接收用户的语音指令,扬声器用于播放音乐,处理器用于控制系统的运行。
2. 软件系统智能音乐播放器的软件系统包括语音识别引擎,自然语言处理系统,音乐播放控制系统等。
其中,语音识别引擎用于将用户的语音指令转换为文字,自然语言处理系统用于分析指令的意图和语义,音乐播放控制系统用于控制音乐的播放和停止等操作。
3. 数据库系统智能音乐播放器需要建立一个存储音乐信息的数据库系统,以便用户随时查找和播放自己喜欢的音乐。
三、智能音乐播放器的实现1. 语音识别引擎的选择目前市面上有多种语音识别引擎,如微软小冰,百度语音等。
根据对比和评估,选择一款适合自己需求的语音识别引擎。
2. 自然语言处理系统的构建自然语言处理系统需要借助机器学习和深度学习的算法,对用户的语音指令进行分析和处理,以便控制音乐播放。
通过算法,可以使系统的识别率更高,指令的执行更加精准。
3. 音乐播放控制系统的开发音乐播放控制系统需要集成语音识别引擎和自然语言处理系统,实现对音乐的控制。
例如,当用户说“播放某一首歌曲”时,系统可以通过数据库找到这首歌并播放。
4. 数据库系统的搭建为了使系统能够随时查找和播放用户想听的音乐,需要建立一个存储音乐信息的数据库系统。
数据库可以通过网络爬虫等方式进行数据的搜集和整合。
语音处理_Audio-Visual Speech Processing(视听语音处理)

Audio-Visual Speech Processing(视听语音处理)数据摘要:A human listener can use visual cues, such as lip and tongue movements, to enhance the level of speech understanding, especially in a noisy environment. The process of combining the audio modality and the visual modality is referred to as speechreading, or lipreading. Inspired by human speechreading, the goal of this project is to enable a computer to use speechreading for higher speech recognition accuracy.There are many applications in which it is desired to recognize speech under extremely adverse acoustic environments. Detecting a person's speech from a distance or through a glass window, understanding a person speaking among a very noisy crowd of people, and monitoring a speech over TV broadcast when the audio link is weak or corrupted, are some examples. In these applications, the performance of traditional speech recognition is very limited. In this project, we use a video camera to track the lip movements of the speaker to assist acoustic speech recognition. We have developed a robust lip-tracking technique, with which preliminary results have showed that speech recognition accuracy for noisy audio can be improved from less than 20% when only audio information is used, to close to 60% when lip tracking is used to assistspeech recognition. Even with the visual modality only, i.e., without listening at all, lip-tracking can achieve a recognition accuracy close to 40%.中文关键词:视听,语音处理,音频模式,视觉模式,英文关键词:Audio-Visual,Speech Processing,audio modality,visual modality,数据格式:VOICE数据用途:Data processing,Identification数据详细介绍:Audio-Visual Speech ProcessingGoalA human listener can use visual cues, such as lip and tongue movements, to enhance the level of speech understanding, especially in a noisy environment. The process of combining the audio modality and the visual modality is referred to as speechreading, or lipreading. Inspired by human speechreading, the goal of this project is to enable a computer to use speechreading for higher speech recognition accuracy.There are many applications in which it is desired to recognize speech under extremely adverse acoustic environments. Detecting a person's speech from a distance or through a glass window, understanding a person speaking among a very noisy crowd of people, and monitoring a speech over TV broadcast when the audio link is weak or corrupted, are some examples. In these applications, the performance of traditional speech recognition is very limited. In this project, we use a video camera to track the lip movements of the speaker to assist acoustic speech recognition. We have developed a robust lip-tracking technique, with which preliminary results have showed that speech recognition accuracy for noisy audio can be improved from less than 20% when only audio information is used, to close to 60% when lip tracking is used to assist speech recognition. Even with the visual modality only, i.e., without listening at all, lip-tracking can achieve a recognition accuracy close to 40%.System DescriptionWe explore the problem of enhancing the speech recognition in noisy environments (both Gaussian white noise and cross-talk noise cases) by using the visual information such as lip movements.We use a novel Hidden Markov Model (HMM) to model the audio-visualbi-modal signal jointly, which shows promising result for recognition. We alsoexplore the fusion of the acoustic signal and the visual information with different combinations approaches, to find the optimum method.To test the performance of this approach, we add gaussian noise to the acoustic signal with different SNR (signal-to-noise ratio). Here we plot the recognition ratio versus SNR.In the plot above, the blue curve shows the change of the recognition ratio vs. SNR when we use the audio-visual joint feature (cascade the visual parameter with the acoustic feature) as the input to the recognition system. The black curve with "o" shows the performance when we use acoustic signal as the input, just as most conventional speech recognition systems do. The flat black curve shows the performance of recognition using only the lip movement parameters.We can see that the recognition ratio of the joint HMM system drops much slower than the acoustic only system, which means the joint system is more robust to the gaussian noise corruption in the acoustic signal. We also apply this approach to cross-talk noise corrupted speech.DownloadAs the first step of this research, we collected an audio-visual data corpus, which is available to the public. In this data set, we have:10 subjects (7 males and 3 females).The vocabulary includes 78 isolated words commonly used for time, such as, "Monday", "February", "night", etc. Each word repeated 10 times.Data Collection:To collect a database with high quality, we have been very critical about the recording environment. We have set it up in a soundproof studio to collectnoise-free audio data. And we used controlled light and blue-screen background to collect the image data.We used a SONY digital camcorder with tie-clip microphone to record the data on DV tapes. The data on DV tapes is transferred to a PC by the Radius MotoDV program and stored as Quicktime files. Since the data on the DV tapes are already digital, there's no quality loss when transferred to the PC.Here are some sample Quicktime videos: ( Here the Quicktime files have been converted to streaming RealVideo sequences with lower quality, to make online viewing easier. ) Click the image to view the sample video.We have altogether 100 such Quicktime files, each one containing one subject articulates all the words in the vocabulary. Each file is about 450MBytes. Both the DV raw data and the Quicktime files are available upon request.Data Pre-processing:The raw data on the DV tapes and the Quicktimes files are big and need further processing before can be used directly in the lipreading research. We have processed the data in the following way:First of all, for the video part, we only keep the mouth area since it's the Area of Interest in the lipreading research field. We used a video editing tool (such as Adobe Premiere) to crop out the mouth area. The following figure shows how the whole face picture, size 720*480, was cut to the only picture of the mouth, size 216*264. Note that the face in the original Quicktime video frame lie horizontally due to the shooting procedure.The four offsets of the mouth picture were noted down for calculating the positions of the lip parameters later. After that, we shrank the mouth picturefrom size 216*264 to 144*176, and rotated to the final picture, size 176*144, the standardized QCIF format.And then we use a lip tracking program to extract the lip parameters from each QCIF sized file, based on deformable template and color information. The template is defined by the left and right corners of the mouth, the height of the upper lip, and the height of the lower lip. The following figure shows an example of the lip tracking result, with the template in black lines superimposed on the mouth area.Click the image to view the sample video sequence.For more details about the lip tracking and object tracking, please see our face tracking web page. The face tracking toolkit can be extended to track lip movements. The lip parameters are stored in text files, along with the offsets of the mouth picture.Secondly, we also have the waveform files extracted from the Quicktime video files. These waveform files contains the speech signals corresponding to the lip parameters contained in the text files mentioned above.Data FormatText files with the lip parameters. The text file contains approximately 3000-4000 lines of numbers. The first line has four numbers which are the offsets of the picture when we cropped the mouth area from the whole face picture. Those four number are the left offset, the right offset, the top offset and the bottom offset respectively, as mentioned above.For the rest of numbers, each line is consist of seven numbers. The first one is the frame number. The next four numbers are the positions of the left corner(x1,y1) and the right corner(x2,y2). The last two numbers are the the height of the upper lip(h1), and the height of the lower lip(h2). As shown in the figure above.The waveform files. These files contain audio signals which are sampled as PCM, 44.1KHz, 16 bit, mono.VocabularyFor date/time: One, two, three, four, five, six, seven, eight, nine, ten, eleven, twelve, thirteen, fourteen, fifteen, sixteen, seventeen, eighteen, nineteen, twenty,thirty, forty, fifty, sixty, seventy, eighty, ninety, hundred,thousand, million, billionFor month:January, February, March, April, May, June,July, August, September, October, November, DecemberFor day:Monday, Tuesday, Wednesday, Thursday, Friday, Saturday, SundaySome additional words:Morning, noon, afternoon, night, midnight, evening,AM, PM, now, next, last, yesterday, today, tomorrow,ago, after, before, from, for, through, until, till, that, this, day, month, week ,yearPublicationsF. J. Huang and T. Chen, "Real-Time Lip-Synch Face Animation driven by human voice", IEEE Workshop on Multimedia Signal Processing, Los Angeles, California, Dec 1998ContactAny suggestions or comments are welcome. Please send them to Wende Zhang.数据预览:点此下载完整数据集。
百度百科—语音识别
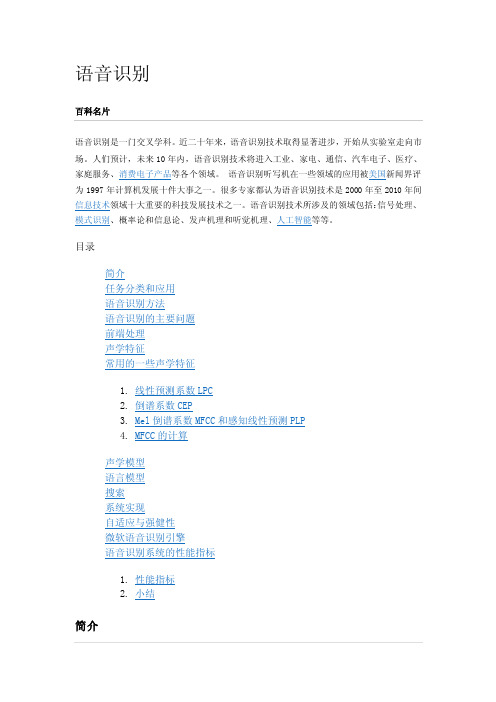
语音识别与机器进行语音交流,让机器明白你说什么,这是人们长期以来梦寐以求的事情。
语音识别技术就是让机器通过识别和理解过程把语音信号转变为相应的文本或命令的高技术。
语音识别技术主要包括特征提取技术、模式匹配准则及模型训练技术三个方面。
任务分类和应用根据识别的对象不同,语音识别任务大体可分为3类,即孤立词识别(isolated word recognition),关键词识别(或称关键词检出,keyword spotting)和连续语音识别。
其中,孤立词识别的任务是识别事先已知的孤立的词,如“开机”、“关机”等;连续语音识别的任务则是识别任意的连续语音,如一个句子或一段话;连续语音流中的关键词检测针对的是连续语音,但它并不识别全部文字,而只是检测已知的若干关键词在何处出现,如在一段话中检测“计算机”、“世界”这两个词。
根据针对的发音人,可以把语音识别技术分为特定人语音识别和非特定人语音识别,前者只能识别一个或几个人的语音,而后者则可以被任何人使用。
显然,非特定人语音识别系统更符合实际需要,但它要比针对特定人的识别困难得多。
另外,根据语音设备和通道,可以分为桌面(PC)语音识别、电话语音识别和嵌入式设备(手机、PDA等)语音识别。
不同的采集通道会使人的发音的声学特性发生变形,因此需要构造各自的识别系统。
语音识别的应用领域非常广泛,常见的应用系统有:语音输入系统,相对于键盘输入方法,它更符合人的日常习惯,也更自然、更高效;语音控制系统,即用语音来控制设备的运行,相对于手动控制来说更加快捷、方便,可以用在诸如工业控制、语音拨号系统、智能家电、声控智能玩具等许多领域;智能对话查询系统,根据客户的语音进行操作,为用户提供自然、友好的数据库检索服务,例如家庭服务、宾馆服务、旅行社服务系统、订票系统、医疗服务、银行服务、股票查询服务等等。
语音识别方法语音识别方法主要是模式匹配法。
在训练阶段,用户将词汇表中的每一词依次说一遍,并且将其特征矢量作为模板存入模板库。
使用AI技术进行声音识别与合成的实践指南
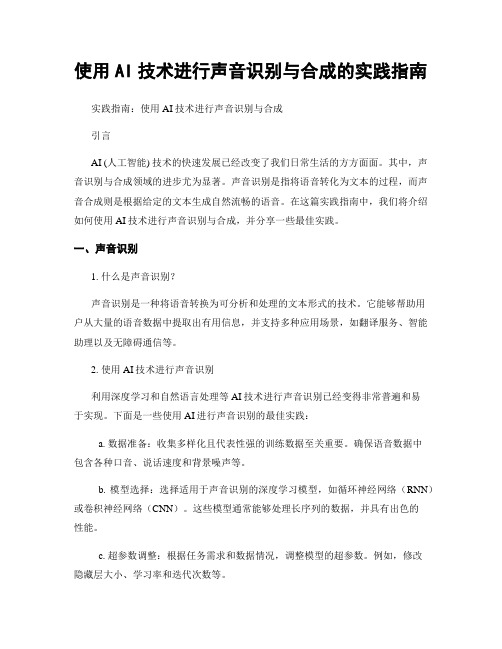
使用AI技术进行声音识别与合成的实践指南实践指南:使用AI技术进行声音识别与合成引言AI (人工智能) 技术的快速发展已经改变了我们日常生活的方方面面。
其中,声音识别与合成领域的进步尤为显著。
声音识别是指将语音转化为文本的过程,而声音合成则是根据给定的文本生成自然流畅的语音。
在这篇实践指南中,我们将介绍如何使用AI技术进行声音识别与合成,并分享一些最佳实践。
一、声音识别1. 什么是声音识别?声音识别是一种将语音转换为可分析和处理的文本形式的技术。
它能够帮助用户从大量的语音数据中提取出有用信息,并支持多种应用场景,如翻译服务、智能助理以及无障碍通信等。
2. 使用AI技术进行声音识别利用深度学习和自然语言处理等AI技术进行声音识别已经变得非常普遍和易于实现。
下面是一些使用AI进行声音识别的最佳实践:a. 数据准备:收集多样化且代表性强的训练数据至关重要。
确保语音数据中包含各种口音、说话速度和背景噪声等。
b. 模型选择:选择适用于声音识别的深度学习模型,如循环神经网络(RNN)或卷积神经网络(CNN)。
这些模型通常能够处理长序列的数据,并具有出色的性能。
c. 超参数调整:根据任务需求和数据情况,调整模型的超参数。
例如,修改隐藏层大小、学习率和迭代次数等。
d. 模型训练与优化:使用标注好的语音数据进行模型训练,并利用反向传播算法不断优化模型性能。
合理分配训练集、验证集和测试集,以避免过拟合或欠拟合。
e. 实时预测与部署:将训练好的模型部署到相应的系统中,使其能实时对输入语音进行识别。
在部署过程中需要考虑响应时间、计算资源等因素。
二、声音合成1. 什么是声音合成?声音合成是将文本转换为自然流畅的语音的过程。
它通过AI技术生成逼真且易于理解的语音输出。
在现实生活中,我们可以看到很多智能助理和语音导航设备在这方面的应用。
2. 使用AI技术进行声音合成使用AI技术进行声音合成有许多有益的应用,例如智能助手、自动问答系统和教育软件。
人工智能在音频处理中的应用
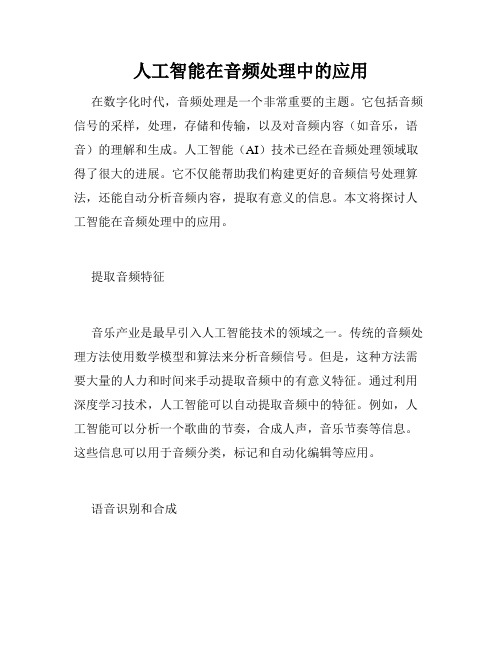
人工智能在音频处理中的应用在数字化时代,音频处理是一个非常重要的主题。
它包括音频信号的采样,处理,存储和传输,以及对音频内容(如音乐,语音)的理解和生成。
人工智能(AI)技术已经在音频处理领域取得了很大的进展。
它不仅能帮助我们构建更好的音频信号处理算法,还能自动分析音频内容,提取有意义的信息。
本文将探讨人工智能在音频处理中的应用。
提取音频特征音乐产业是最早引入人工智能技术的领域之一。
传统的音频处理方法使用数学模型和算法来分析音频信号。
但是,这种方法需要大量的人力和时间来手动提取音频中的有意义特征。
通过利用深度学习技术,人工智能可以自动提取音频中的特征。
例如,人工智能可以分析一个歌曲的节奏,合成人声,音乐节奏等信息。
这些信息可以用于音频分类,标记和自动化编辑等应用。
语音识别和合成人工智能在语音识别和合成方面也取得了显著进展。
通过利用深度学习技术,人工智能可以自动分析和转换语音信号。
例如,语音识别技术可以将语音信号转换为文本,从而实现自动化的字幕处理、语音搜索和语音控制等功能。
语音合成技术可以创建实时语音转换,包括为不同语言和方言实现翻译。
借助这些技术,人们可以实现更加智能化的音频处理系统。
音频增强人工智能技术还可以在音频增强方面发挥重要作用。
在音频信息采集的时候,由于环境噪声等因素,音频质量会存在一定程度上的下降。
通过利用深度学习技术,人工智能可以实现音频信号的去噪和降噪,因此可以从信号中获得更多的信息。
此外,人工智能还可以自动消除回声、噪音和其他干扰等因素,以使音频信号更为清晰。
智能听力助手人工智能技术还可以用于创建智能听力助手。
现代数字化时代的快节奏生活,人们的听力受到不断影响。
借助人工智能的辅助技术,我们能够实现数字化听力辅助设备。
这些设备可以自动识别音频信号的源、清晰度,以及确定最适宜的音频播放速度,以提供最佳音质。
总结在音频处理领域,人工智能技术变得越来越普及。
我们已经看到了它对识别语音、合成语音、音频增强等领域的重要影响。
语音识别中的声学模型和语言模型
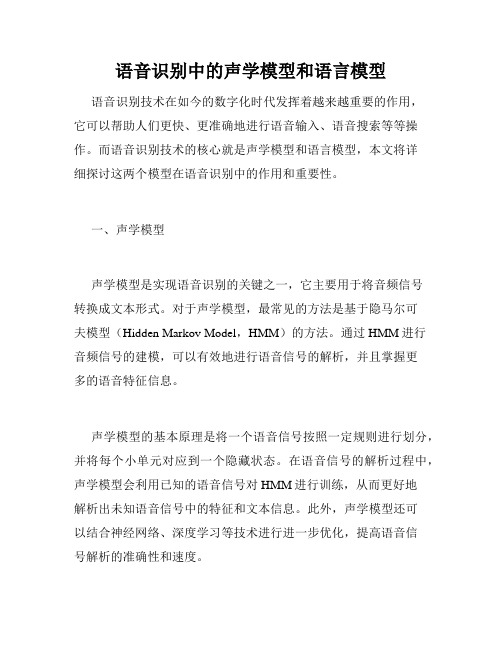
语音识别中的声学模型和语言模型语音识别技术在如今的数字化时代发挥着越来越重要的作用,它可以帮助人们更快、更准确地进行语音输入、语音搜索等等操作。
而语音识别技术的核心就是声学模型和语言模型,本文将详细探讨这两个模型在语音识别中的作用和重要性。
一、声学模型声学模型是实现语音识别的关键之一,它主要用于将音频信号转换成文本形式。
对于声学模型,最常见的方法是基于隐马尔可夫模型(Hidden Markov Model,HMM)的方法。
通过HMM进行音频信号的建模,可以有效地进行语音信号的解析,并且掌握更多的语音特征信息。
声学模型的基本原理是将一个语音信号按照一定规则进行划分,并将每个小单元对应到一个隐藏状态。
在语音信号的解析过程中,声学模型会利用已知的语音信号对HMM进行训练,从而更好地解析出未知语音信号中的特征和文本信息。
此外,声学模型还可以结合神经网络、深度学习等技术进行进一步优化,提高语音信号解析的准确性和速度。
总之,声学模型是语音识别技术中不可或缺的一部分,它可以为解析语音信号提供强大的能力和精确的解析结果。
二、语言模型除了声学模型外,语言模型也是语音识别技术中的重要组成部分。
与声学模型不同的是,语言模型更多的是关注文本的含义和语法规则。
语言模型主要的作用是利用已知的文本样本,掌握自然语言的规则和习惯用语,在语音识别过程中更好地解析和预测文本内容。
语言模型的核心思想是根据相关的文本语料库,对文本的结构规律进行解析和建模。
在语音识别的过程中,语言模型会根据语音信号的特征,通过已知的语法规则和单词频率等信息,预测出最可能的输入文本。
同时,语言模型也可以利用上下文信息和语言特征进行语音信号的解析,从而提高语音识别的准确性和速度。
总之,语言模型是语音识别技术中至关重要的一环,它可以为语音信号解析和文本预测提供强有力的支持和帮助。
三、声学模型和语言模型的应用声学模型和语言模型是语音识别技术中两个不可分割的组成部分,它们分别关注音频信号和文本信息,在语音识别的过程中发挥着不同的作用。
- 1、下载文档前请自行甄别文档内容的完整性,平台不提供额外的编辑、内容补充、找答案等附加服务。
- 2、"仅部分预览"的文档,不可在线预览部分如存在完整性等问题,可反馈申请退款(可完整预览的文档不适用该条件!)。
- 3、如文档侵犯您的权益,请联系客服反馈,我们会尽快为您处理(人工客服工作时间:9:00-18:30)。
AUDIOVISUAL SPEECH RECOGNITION WITH ARTICULATORPOSITIONS AS HIDDEN V ARIABLESMark Hasegawa-Johnson,Karen Livescu,Partha Lal and Kate Saenko∗University of Illinois at Urbana-Champaign,MIT,University of Edinburgh,and MIT {jhasegaw@,klivescu@,l@,saenko@}ABSTRACTSpeech recognition,by both humans and machines, benefits from visual observation of the face,espe-cially at low signal-to-noise ratios(SNRs).It has of-ten been noticed,however,that the audible and vis-ible correlates of a phoneme may be asynchronous; perhaps for this reason,automatic speech recogni-tion structures that allow asynchrony between the audible phoneme and the visible viseme outper-form recognizers that allow no such asynchrony. This paper proposes,and tests using experimen-tal speech recognition systems,a new explanation for audio-visual asynchrony.Specifically,we pro-pose that audio-visual asynchrony may be the result of asynchrony between the gestures implemented by different articulators,such that the most visibly salient articulator(e.g.,the lips)and the most au-dibly salient articulator(e.g.,the glottis)may,at any given time,be dominated by gestures associated with different phonemes.The proposed model of audio-visual asynchrony is tested by implementing an“articulatory-feature model”audiovisual speech recognizer:a system with multiple hidden state vari-ables,each representing the gestures of one articu-lator.The proposed system performs as well as a standard audiovisual recognizer on a digit recogni-tion task;the best results are achieved by combining the outputs of the two systems.Keywords:Automatic Speech Recognition(ASR), Audiovisual Speech,Articulatory Phonology,Dy-namic Bayesian Network(DBN)1.INTRODUCTIONA large number of studies have demonstrated that speech recognition,by both humans and machines, benefits from visual observation of the face,espe-cially at low signal-to-noise ratios(SNRs).For ex-ample,by integrating information from audio and video observations,it is possible to reduce the word ∗This material is based upon work supported by the National Science Foundation under grant01-21285(F.Jelinek,PI).Any opinions,findings,or recommendations expressed in this mate-rial are those of the authors and do not necessarily reflect the views of the NSF.Figure1:An example of audiovisual asynchrony.The still image shown here(one frame from avideo sequence)provides evidence that the talkerhas prepared her tongue tip and lips,respectively,for thefirst and second phonemes of the word“three.”The audio signal recorded synchronouswith this image contains onlysilence.error rate of automatic speech recognition(ASR)at low SNR[11].The simplest method for reducing word error rate is to concatenate the audio and video observations,and to use the resulting vector as the observation in a hidden Markov model(HMM).The audible and visible correlates of a phoneme,how-ever,may by asynchronous(e.g.,Fig.1).Motivated by the observed audio-visual asynchrony,several au-thors have proposed speech recognizers that use sep-arate HMMs for the audio and video observations, with some type of connection between the transition probabilities of the two HMMs[3,6].The theory of articulatory phonology[1]provides a new way of thinking about asynchrony.In the the-ory of articulatory phonology,the lexical entry for each word is composed of loosely ordered,indivis-ible mental constructs called“gestures.”In normal speech production,all of the gestures in a word are always produced;there is no such thing as gesture deletion,substitution,or insertion.The wide range of pronunciation variability observed in real-world speech is modeled in three ways:(1)the strength or duration of a gesture may be reduced,(2)gestures may be produced in non-canonical order,or(3)sev-eral different gestures may act simultaneously on the same“tract variable”[2].Any of these three causesmay result in a gesture that is not fully implemented by the articulators.In an articulatory phonology model,Fig.1does not exhibit asynchrony between the audio and video channels,but rather,among the articulatory positions of the lips,tongue,and glot-tis/lungs.Articulatory phonology has inspired several re-cent automatic speech recognition experiments [8,9,5].The goal of this paper is to test an audiovi-sual speech recognizer based on the “articulatory-feature based recognizer”of Livescu and Glass [5].A system based on Livescu’s model has been previ-ously demonstrated for recognition of speech from video [10],but we believe that the experiments re-ported in this article are the first to demonstrate au-diovisual fusion using such a system.2.MODELSThree classes of automatic speech recognition mod-els are tested in this paper:hidden Markov models (HMMs;Fig.2),coupled hidden Markov models (CHMMs;Fig.3),and articulatory-feature models (AFMs;Fig.4).All of these systems are imple-mented in the graphical modeling toolkit (GMTK)using the notation of a dynamic Bayesian network (DBN).The goal of this section is to describe DBN notation,and to define the models used in this paper.Figure 2:DBN representation of a phone-based HMM speechrecognizerA DBN is a graph in which nodes represent random variables,and edges represent stochas-tic dependence of one random variable upon an-other.Fig.2,for example,shows two frames from the DBN representation of a standard phone-based HMM speech recognizer.Fig.2specifies the follow-ing assumption about the random variables shown,where p (Λt |Λt −1)is a short-hand notation for the probability of all variables at time t ,given all vari-ables at time t −1:(1)p (Λt |Λt −1)=p (w t |w t −1,winc t −1)·p (φt |φt −1,qinc t −1,winc t −1)·p (q t |φt ,w t )·p (qinc t |q t )p (winc t |qinc t ,φt ,w t )·p (x t |q t )p (y t |q t )This paper follows several notational conventions exemplified in Fig.2,and described in the next five paragraphs.Shaded circles in a DBN represent observable vectors.In Fig.2,the observable vectors are x t and y t .Subscript represents frame number;all of the ex-periments reported in this paper use 10ms frames.In all of the experiments in this paper,x t is an acous-tic observation vector composed of perceptual LPC coefficients,energy,and their first and second tem-poral derivatives.The video observation vector y t includes the first 35coefficients from a discrete co-sine transform of the grayscale pixel values in a rect-angle including the lips,upsampled from 30to 100frames per second,and their first temporal deriva-tives.The probability density functions p (x t |q t )and p (y t |q t )are mixture Gaussian PDFs,trained using expectation maximization.Unshaded circles and rectangles represent hidden variables.There are three types of hidden variables:categorical variables,counters,and indicators.Categorical variables are represented using Ro-man letters.In Fig.2,there are two categorical vari-ables:w t and q t .w t is the word label at time t ,w t ∈W ,where W is the vocabulary.In all experi-ments reported in this paper,the vocabulary contains eleven “words:”the ten English digits,and silence.q t ∈Q is the phonestate label at time t .“Phones-tates”are subsegments of a phone.In the experi-ments in this paper,every phone has three subseg-ments (beginning,middle,and end).The cardinal-ity of set Q is therefore three times the number of phones:in our experiments,|Q|=3·42=126.Counters,represented using Greek letters,de-scribe the ordinal position of a phone within a word.In Fig.2,for example,φt is the position of phon-estate q t in word w t .The probability distribution p (φt +1|φt ,qinc t ,winc t )has an extremely limited form.φt +1is always equal to φt unless there is a phonestate transition or a word transition.If there is a word transition,φt +1is reset to φt +1=1.If there is a phonestate transition but no word transition,then φt +1=φt +1.Indicators are binary random variables:an indi-cator variable set to 1indicates that some event oc-curs in frame t ,otherwise the indicator variable is 0.An indicator variable has a two-letter name,and its node in the DBN is drawn as a rectangle.The indica-tor variables in Fig.3are winc t (“word increment”)and qinc t (“phonestate increment”).Indicator vari-ables are generally used to gather information from other variables,make a binary decision,and then distribute that information to variables in the next frame.For example,a phonestate increment occurswith some probability p (qinc t =1|q t )that depends on the current phonestate label q t .If qinc t =1,and if φt is equal to the number of phonestates in the dictionary entry for word w t ,then a word transition also occurs;otherwise,winc t =0.Figure 3:DBN representation of a coupled HMM (CHMM)audiovisual speechrecognizerMany experiments have demonstrated that the word error rate of an audiovisual speech recognizer is reduced if the audible phonestate and the visible visemestate are allowed to be asynchronous [6,3].For example,a “coupled hidden Markov model”(CHMM)is a pair of hidden Markov models:one linked to the acoustic observation vector x t ,and one linked to the visual observation vector y t .State vari-ables in the two HMMs depend on one another;thus the two HMMs are allowed to be asynchronous,but not by very much.Fig.3shows our implementation of a CHMM;to our knowledge,this is the first time a CHMM has been implemented using phone-like subword units.In our implementation,the acoustic observation vec-tor x t depends on the phonestate label q t ,which de-pends,in turn,on the phonestate counter φt ;all of these variables are defined exactly as they were in Fig.2.The visual observation vector y t depends on the visemestate label v t ,which depends,in turn,on the visemestate counter βt .The phonestate and the visemestate are allowed to be asynchronous,but not by very much.The degree of asynchrony between the phonestate and the visemestate is measured by the asynchrony variable,whose value is always δt =φt −βt .If δt is greater than or equal to some pre-set limit δmax ,then the probability of a phonestate transition is p (qinc t =1|δt ≥δmax )=0.If δt is less than or equal to −δmax ,then the probabil-ity of a visemestate transition is p (vinc t =1|δt ≤−δmax )=0.Transition probabilities under other circumstances are trained using expectation maxi-mization.The third speech recognition algorithm tested in our work is an articulatory-feature model (AFM),Figure 4:DBN representation of an articula-tory feature model (AFM)automatic speech rec-ognizerbased on the model of Livescu and Glass [5].Fig.4shows our implementation of an AFM;it differs from our CHMM in three ways.First,there are three phone-like hidden variables,instead of two.In Fig.4,l t ,the lipstate,specifies the current lip configuration,and depends on the lipstate counter λt .t t ,the tonguestate,specifies the current tongue configuration,and depends on the tongue-state counter τt .g t ,the glotstate,specifies the cur-rent state of the glottis,velum,and lungs,and de-pends on the glotstate counter γt .Asynchrony be-tween the counters is measured using three asyn-chrony variables,of which two are shown in the fig-ure:δt =λt −τt ,and t =τt −γt .Second,the AFM differs from the CHMM be-cause x t and y t depend on all three hidden state vari-ables.Fig.4does not show x t and y t because space is limited,but the forms of their probability density functions are as follows.x t depends on all three ar-ticulators:p (x t |l t ,t t ,g t )is modeled using a mixture Gaussian PDF.y t depends on the states of the lips and tongue,and p (y t |l t ,t t )is modeled using a mix-ture Gaussian;the glottis/velum state variable g t is assumed to have no visual correlates.Third,and most important,the cardinality of the hidden state variables is considerably reduced.Car-dinality of the hidden variables affects speech recog-nition accuracy and computational complexity.In general,system precision improves with greater car-dinality,because the recognizer is able to represent a greater number of acoustic distinctions.General-ization error (error caused by differences between the training and test corpus)also increases with the cardinality of the hidden variables.The optimum balance between precision and generalization error is achieved when the hidden variables represent all,and only,the acoustic distinctions that can be gen-eralized from training data to test data.In prac-tice,achieving a reasonable balance between pre-cision and generalization requires experimentation. In our CHMM,for example,the variables q t and v t are each drawn from set Q,the set of all phonestates known to the speech recognizer,whose cardinality is 3·42=126.The variable l t∈L,on the other hand, represents only the set of English phoneme distinc-tions that are implemented using labial gestures; likewise t t∈T represents phoneme distinctions im-plemented using the tongue,while g t∈G represents distinctions implemented by the glottis,lungs,and soft palate.The sets L,T,and G are specified in Table1.These sets were designed based on con-siderations of articulatory specificity(gestures must be local to the named articulator)and phone dis-tinctiveness(each vector[l t,t t,g t]may correspond to no more than one value of the HMM phonestate label q t),and were refined using a small number of preliminary experiments.In addition to the set of articulator-specific gestures,each of the three artic-ulators was allowed to take the value“Silent,”be-cause during silence,the setting of the articulator is unspecified by phonetic requirements.Table1:State variables of the articulatory featuremodel are drawn from the sets shown:l t∈L,t t∈T,g t∈G.The operation“×{1,2,3}”per-forms temporal segmentation,dividing each ges-ture into initial,medial,andfinal subgestures,thusthe cardinalities of the three sets are|L|=18,|T|=63,and|G|=12.3.EXPERIMENTAL METHODS Experiments reported in this paper used the CUA VE database[7].CUA VE is a database of isolated dig-its,telephone numbers,and read sentences recorded in high resolution video,with good lighting,in a quiet recording studio;acoustic noise is electroni-cally added.Experiments in this paper used discrete speech:ten-digit sequences spoken with silence af-ter each word.The corpus was divided arbitrarily into a training subset(60%of the talkers),a valida-tion subset(20%of the talkers),and an evaluation subset(20%of the talkers);each subset was evenly divided between male and female talkers.Error rates using the evaluation subset were not computed for lack of time;results reported here are from the vali-dation subset.All recognition models used a uniform grammar, constrained to produce exactly ten words per utter-ance.All recognizers were trained using an SNR of ∞(no added noise),and were tested at six different SNRs:∞,12dB,10dB,6dB,4dB,and-4dB.Rec-ognizer training consisted of three stages.First,the observation PDFs each recognizer was trained using noise-free training data,with a Gaussian represent-ing each of the PDFs p(x t|l t,t t,g t)and p(y t|l t,t t). Second,the number of Gaussians per PDF was dou-bled,the recognizer was re-trained using noise-free data,and the recognizer was tested using noise-free validation data.Minimum validation error was achieved with32Gaussians per PDF for the audio-only HMM,16Gaussians per PDF for the video and audiovisual HMMs,2Gaussians per PDF for the articulatory feature model,and4-16Gaussians per PDF for the CHMMs,as specified in Sec.4.Third, the recognizer was tested using validation data rep-resenting each of the six SNRs(∞,12,10,6,4,and -4dB),and for each SNR,a video stream weight,ρ,was selected to minimize word error rate.ρis used to determine the extent to which the observa-tion probability depends on video vs.audio obser-vations:the model used here is p(x t,y t|l t,t t,g t)= p(y t|l t,t t)ρp(x t|l t,t t,g t)1−ρ.Experimental results(reported in Sec.4.)demon-strated that the articulatory feature model(AFM) performs comparably to the CHMM.Analysis re-vealed,however,that the two systems make different specific errors.When speech recognition systems have similar word error rate but different specific er-rors,it is common to allow the systems to correct each others’mistakes,using a system combination strategy called ROVER[4],in which the word tran-scriptions generated by the systems are aligned with one another,and majority voting determines thefinal ROVER output.If the specific error patterns of the systems are sufficiently complementary,the com-bined system will have lower word error rate than any component system.We used the NIST ROVERimplementation [4]to test the hypothesis that AFM and CHMM are complementary in this way.4.RESULTSFigures 5through 8describe the word error rates achieved,on validation data,under the experimen-tal conditions described in Sec.3.Figure 5:Word error rates of video-only,audio-only,and audiovisual HMM recognizers in six dif-ferentSNRs.Fig.5plots the word error rate of HMM systems using audio-only,video-only,or audiovisual obser-vations,as a function of signal to noise ratio.Word error rate of the video-only recognizer is about 60%,independent of acoustic SNR.Word error rate of the audiovisual recognizer is lower than that of the audio-only recognizer under every condition with acoustic noise.The difference is statistically signifi-cant (MAPSSWE test,p <0.05).Figure 6:Word error rates of CHMM recogniz-ers with maximum allowed asynchrony (δmax =max |φt −βt |)of 0,1,2,or an unlimited number ofphonestates.Fig.6plots word error rate as a function of signal to noise ratio for systems with maximum allowed asynchrony (δmax =max |φt −βt |)of 0,1,2,or an unlimited number of phonestates (using 16,16,8,and 4Gaussians per PDF,respectively).The sys-tem with no allowed asynchrony (δmax =0)is an HMM,and its error rates are also plotted in Fig.5.The system with unlimited asynchrony has no rep-resentation of dependence between the two modali-ties:both qinc t and vinc t are independent of δt .The system with δmax =2produces the lowest average word error rate (averaged across six SNRs).The dif-ference between δmax =2and other conditions is statistically significant (MAPSSWE test,p <0.05).Figure 7:Word error rate of CHMM and articu-latory feature model speech recognizers as a func-tion of signal to noiseratio.Fig.7plots word error rate versus signal to noise ratio of an articulatory feature model (AFM)speech recognizer with a maximum inter-articulator asyn-chrony of δmax = max =2.For comparison,word error rate of the best CHMM from Fig.6(δmax =2)is re-plotted on the same axes.The CHMM has a lower word error rate for some signal to noise ratios,and tends to have a lower word error rate on average,but the difference is not statistically significant.Figure 8:Word error rates of three original systems (CHMM systems with δmax =1and δmax =2,and AFM with δmax =2)and two ROVER system combinations,averaged across all test-setSNRs.Despite the similarity in their word error rates,the articulatory feature model and the CHMM are not identical.Fig.8shows word error rate of three recognizers,and of two different ROVER system combinations,averaged across all signal to noise ratios.The leftmost bar shows the word error rate achieved by a system combination using two CHMM systems (δmax =1and δmax =2)andone articulatory-feature model(AFM).The second bar shows the result of system combination in which the AFM has been replaced by another CHMM (the CHMM with unlimitedδmax).The word er-ror rates achieved using system combination are lower than those achieved without system combina-tion(MAPSSWE test,p<0.05).The system com-bination that includes an articulatory feature model tends to have a lower word error rate,on average, than the system that includes only CHMMs,but the difference is not statistically significant.5.CONCLUSIONSThis paper proposes a new paradigm for articulatory-feature based audiovisual speech recognition.Specifically,we propose that the apparent asynchrony between acoustic and visual modalities(noted in many previous A VSR papers) may be effectively modeled as asynchrony among the articulatory gestures implemented by the lips, tongue,and glottis/velum.Experimental tests using the CUA VE corpus provide tentative support for four conclusions.First,the combination of audio and visual features can reduce word error rate at low SNR.Second,word error rate of an audiovisual speech recognizer may be further reduced using a coupled hidden Markov model,and by allowing up to2states of asynchrony(about2/3of a phoneme) between the audio and video HMMs.Third,the benefits of the CHMM are also achieved by an articulatory feature model,in which asynchrony between the lips,tongue,and glottis/velum replaces the asynchrony between audio and video modalities. Fourth,the best results are achieved by combining the outputs of multiple systems,and there is a tendency for the combination of CHMM and AFM systems to outperform a system combination using only CHMMs.The articulatory feature model described in this paper includes target specifications for every articu-lator,during every phoneme.One goal of future re-search will be the development of a model in which the lexical entries better approximate the minimally specified lexical entries used in theoretical studies of articulatory phonology[1].The lexicon,in most theoretical studies,specifies only a small number of mandatory gestures,and each gesture may be asso-ciated with multiple phonemes(e.g.,an Aspiration gesture may cover the entire onset of a syllable,as in the word“speech”).Our current model is also unsatisfactory because it contains no representation of prosody.The acous-tic and articulatory correlates of a gesture are influ-enced by many prosodic context variables,includ-ing syllable structure,lexical stress,phrasal promi-nence,and prosodic phrase boundaries.Thefirst two levels are necessary in order to distinguish the phones used in most HMM-based ASR:e.g.,most systems differentiate nasal consonants in the nucleus vs.onset of a syllable,and most systems distinguish reduced vs.unreduced vowels.It might be possible to develop a comprehensive model,covering all of these levels,using theπ-gesture theory of Byrd and Saltzman[2].6.REFERENCES[1]Browman,C.P.,Goldstein,L.1992.Articulatoryphonology:An overview.Phonetica49,155–180.[2]Byrd, D.,Saltzman, E.2003.The elasticphrase:modeling the dynamics of boundary-adjacent lengthening.J.Phonetics31,149–180. [3]Chu,S.,Huang,T.S.2000.Bimodal speech recog-nition using coupled hidden Markov models.Proc.Interspeech Beijing.[4]Fiscus,J.1997.A post-processing system to yieldreduced word error rates:Recognizer output vot-ing error reduction(ROVER).IEEE Workshop onASRU Santa Barbara.[5]Livescu,K.,Glass,J.2004.Feature-based pro-nunciation modeling for speech recognition.Proc.HLT/NAACL Boston.[6]Neti,C.,Luettin,G.P.J.,Matthews,I.,Glotin,H.,Vergyri,D.,Sison,J.,Mashari,A.,Zhou,J.2000.Audio-visual speech recognition:Final re-port.Technical Report WS00Johns Hopkins Uni-versity Center for Language and Speech Process-ing.[7]Patterson,E.,Gurbuz,S.,Tufecki,Z.,Gowdy,J.2002.CUA VE:A new audio-visual database formultimodal human-computer interface research.Proc.ICASSP Orlando.[8]Richardson,M.,Bilmes,J.,Diorio, C.2000.Hidden-articulator Markov models:performanceimprovements and robustness to noise.Proc.In-terspeech Beijing.[9]Richmond,K.,King,S.,Taylor,P.2003.Model-ing the uncertainty in recovering articulation fromputer Speech and Language17(2-3),153–172.[10]Saenko,K.,K,L.,Siracusa,M.,Wilson,K.,Glass,J.,Darrell,T.2005.Visual speech recognition withloosely synchronized feature streams.Proc.ICCVBeijing.[11]Silsbee,P.L.,Bovik, puterlipreading for improved accuracy in automaticspeech recognition.IEEE Trans.Speech and Au-dio Processing4,337–351.。