蒸发冷
七种蒸发冷却冷凝技术简介
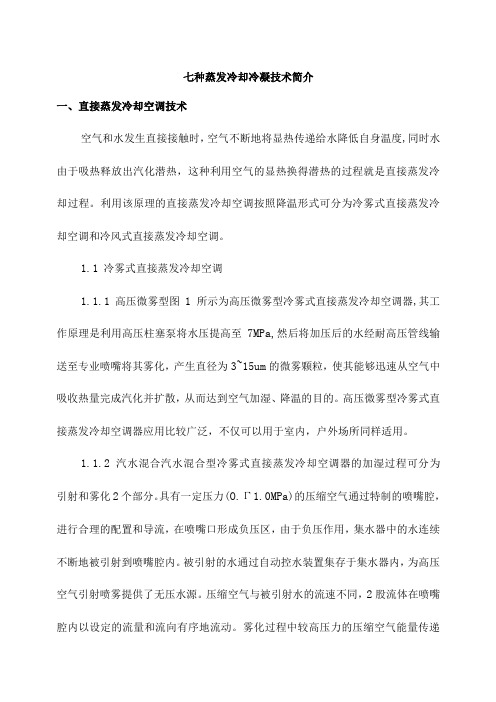
七种蒸发冷却冷凝技术简介一、直接蒸发冷却空调技术空气和水发生直接接触时,空气不断地将显热传递给水降低自身温度,同时水由于吸热释放出汽化潜热,这种利用空气的显热换得潜热的过程就是直接蒸发冷却过程。
利用该原理的直接蒸发冷却空调按照降温形式可分为冷雾式直接蒸发冷却空调和冷风式直接蒸发冷却空调。
1.1冷雾式直接蒸发冷却空调1.1.1高压微雾型图1所示为高压微雾型冷雾式直接蒸发冷却空调器,其工作原理是利用高压柱塞泵将水压提高至7MPa,然后将加压后的水经耐高压管线输送至专业喷嘴将其雾化,产生直径为3~15um的微雾颗粒,使其能够迅速从空气中吸收热量完成汽化并扩散,从而达到空气加湿、降温的目的。
高压微雾型冷雾式直接蒸发冷却空调器应用比较广泛,不仅可以用于室内,户外场所同样适用。
1.1.2汽水混合汽水混合型冷雾式直接蒸发冷却空调器的加湿过程可分为引射和雾化2个部分。
具有一定压力(O.Γ1.0MPa)的压缩空气通过特制的喷嘴腔,进行合理的配置和导流,在喷嘴口形成负压区,由于负压作用,集水器中的水连续不断地被引射到喷嘴腔内。
被引射的水通过自动控水装置集存于集水器内,为高压空气引射喷雾提供了无压水源。
压缩空气与被引射水的流速不同,2股流体在喷嘴腔内以设定的流量和流向有序地流动。
雾化过程中较高压力的压缩空气能量传递给较低压力的水,使水的能量增高。
2股流体在喷嘴出口处混合喷出,混合过程中高压空气与水流发生动量交换,与水进行剧烈摩擦与碰撞,利用空化效应将水充分雾化成细小的水珠。
当汽水混合流体从喷嘴高速喷出时,又与外界大气中的空气进行摩擦接触,从而将水滴进一步撕碎,水滴的直径可达5~10um,从而达到良好的雾化效果。
1.2冷风式直接蒸发冷却空调1.2.1蒸发式冷气机(冷风扇)蒸发式冷气机是利用淋水填料层直接与待处理的室外空气接触,由于喷淋水的温度一般都低于待处理空气(即新风)的温度,空气不断将自身显热传递给水而得以降温;与此同时,部分喷淋水(循环水)会因不断吸收空气中的热量而蒸发,蒸发后的水蒸气被气流带入室内,于是,新风既得以降温,又实现了加湿。
蒸发冷
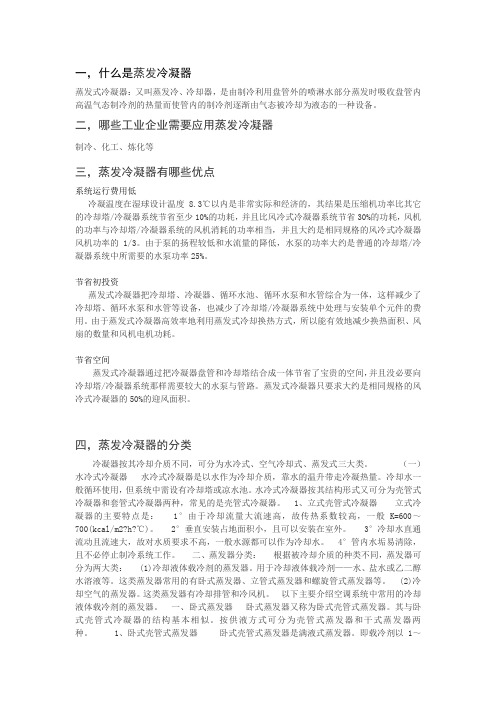
一,什么是蒸发冷凝器蒸发式冷凝器:又叫蒸发冷、冷却器,是由制冷利用盘管外的喷淋水部分蒸发时吸收盘管内高温气态制冷剂的热量而使管内的制冷剂逐渐由气态被冷却为液态的一种设备。
二,哪些工业企业需要应用蒸发冷凝器制冷、化工、炼化等三,蒸发冷凝器有哪些优点系统运行费用低冷凝温度在湿球设计温度8.3℃以内是非常实际和经济的,其结果是压缩机功率比其它的冷却塔/冷凝器系统节省至少10%的功耗,并且比风冷式冷凝器系统节省30%的功耗,风机的功率与冷却塔/冷凝器系统的风机消耗的功率相当,并且大约是相同规格的风冷式冷凝器风机功率的1/3。
由于泵的扬程较低和水流量的降低,水泵的功率大约是普通的冷却塔/冷凝器系统中所需要的水泵功率25%。
节省初投资蒸发式冷凝器把冷却塔、冷凝器、循环水池、循环水泵和水管综合为一体,这样减少了冷却塔、循环水泵和水管等设备,也减少了冷却塔/冷凝器系统中处理与安装单个元件的费用。
由于蒸发式冷凝器高效率地利用蒸发式冷却换热方式,所以能有效地减少换热面积、风扇的数量和风机电机功耗。
节省空间蒸发式冷凝器通过把冷凝器盘管和冷却塔结合成一体节省了宝贵的空间,并且没必要向冷却塔/冷凝器系统那样需要较大的水泵与管路。
蒸发式冷凝器只要求大约是相同规格的风冷式冷凝器的50%的迎风面积。
四,蒸发冷凝器的分类冷凝器按其冷却介质不同,可分为水冷式、空气冷却式、蒸发式三大类。
(一)水冷式冷凝器水冷式冷凝器是以水作为冷却介质,靠水的温升带走冷凝热量。
冷却水一般循环使用,但系统中需设有冷却塔或凉水池。
水冷式冷凝器按其结构形式又可分为壳管式冷凝器和套管式冷凝器两种,常见的是壳管式冷凝器。
1、立式壳管式冷凝器立式冷凝器的主要特点是: 1°由于冷却流量大流速高,故传热系数较高,一般K=600~700(kcal/m2?h?℃)。
2°垂直安装占地面积小,且可以安装在室外。
3°冷却水直通流动且流速大,故对水质要求不高,一般水源都可以作为冷却水。
蒸发冷却名词解释

蒸发冷却是一种利用液体蒸发过程中吸收热量而达到降温的现象和方法。
当液体蒸发时,分子从液体表面进入气相状态,这个过程需要吸收周围环境的热量来提供能量,从而使液体的温度降低。
蒸发冷却的原理基于物质的分子动能和热平衡。
在液体表面,具有较高能量的分子能够克服表面张力并转变为气体,从而带走一部分热量。
这导致剩余液体中分子的平均能量降低,从而使液体的温度下降。
蒸发冷却在自然界和人工应用中都有广泛的应用。
例如,当我们出汗时,汗水蒸发时会带走体表的热量,从而使身体感到凉爽。
在气象学中,蒸发冷却是解释湿度和温度关系的重要因素。
在工程和技术领域,蒸发冷却也被广泛应用于空调、冷却设备和热交换器等领域。
例如,蒸发冷却器利用水蒸发的热量吸收作用来冷却空气,以降低室内温度。
同样,冷却塔使用蒸发冷却原理来降低工业过程中的热量,提高能效。
总的来说,蒸发冷却是一种通过液体蒸发过程中吸收热量来降低温度的现象和方法。
它在自然界和人工应用中都发挥着重要作用,并在许多领域中得到广泛应用。
直接蒸发冷却技术

直接蒸发冷却技术
直接蒸发冷却技术是一种使用水作为制冷剂的冷却技术,这是一个显着的优势,使其环境友好。
利用空气与水的直接接触,并利用水的蒸发潜热进行制冷。
间接蒸发冷却相对于直接蒸发冷却来讲的,它是工作空气与循环水在湿通道侧直接接触等焓降温后再间接的通过换热器带走使用空气中的热量,从而达到对使用空气进行等湿降温的效果。
直接蒸发冷却技术具有以下优点:
- 环保:由于使用水作为制冷剂,因此不会对环境造成污染。
- 节能:由于利用水的蒸发潜热进行制冷,因此可以节省能源。
- 高效:由于空气与水直接接触,因此可以快速降低温度。
然而,直接蒸发冷却技术也存在一些缺点:
- 湿度控制困难:由于空气中的水蒸气含量较高,因此很难控制湿度。
- 能耗高:由于需要大量的水来进行制冷,因此能耗较高。
- 维护成本高:由于需要定期清洗和维护设备,因此维护成本较高。
蒸发冷却式冷水机组制冷原理

蒸发冷却式冷水机组制冷原理
蒸发冷却式冷水机组制冷原理
1、冷却原理:冷水机组制冷系统是利用蒸发器中由气体蒸发而释放出
来的大量热量,来把冷却水冷却下来,达到节能减温的目的。
2、运行原理:冷水机组制冷系统由压缩机、冷凝器、蒸发器、压力表
和膨胀阀组成,工作的过程可以大致分为四个部分:
(1)压缩机压缩空气,把低温、低压的冷凝空气压缩为高温、高压的
热压空气,热压空气夹带着热能,进入冷凝器;
(2)冷凝器吸收高温压空气带来的热能,所以它释出热量,冷凝空气
排出冷凝器后又变回低温、低压状态;
(3)低温、低压的冷凝空气由压力表被抽入蒸发器,在蒸发器里,把
低温空气再次加热,此时,空气回变成低温的蒸汽,同时蒸发器把低
温的冷却水冷却;
(4)低温的蒸汽从蒸发器排出,通过膨胀阀,又转变为低温低压状态,又进入压缩机,循环处理,从而实现蒸发冷却式冷水机组的制冷效果。
3、蒸发冷却式冷水机组的优点:
(1)整体高度节能:蒸发冷却式冷水机组采用空气循环变压工艺,相比水循环机组,整体高度节能,运行安全稳定;
(2)可靠性高:冷水机组是采用多联机组技术,大大提高了机组的稳定性及可靠性;
(3)结构紧凑:冷水机组不需要额外的液体冷却支架,只需要空气电机压缩机,蒸发器,冷凝器和压力表,结构紧凑,安装容易;
(4)噪音低:冷水机组的运行噪音低,更加符合人们的需求,使冷水机组发挥更好的作用。
因此,蒸发冷却式冷水机组具有高度节能、可靠性高、结构紧凑、低噪音等优势,已经成为当前常用的冷却系统。
ahu间接蒸发冷原理
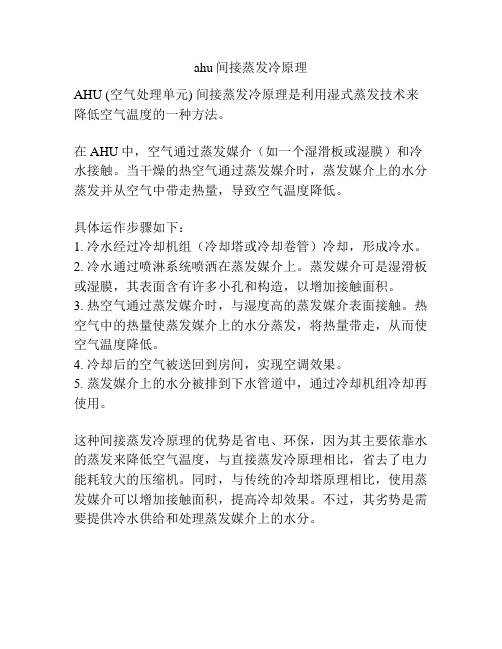
ahu间接蒸发冷原理
AHU (空气处理单元) 间接蒸发冷原理是利用湿式蒸发技术来降低空气温度的一种方法。
在AHU中,空气通过蒸发媒介(如一个湿滑板或湿膜)和冷水接触。
当干燥的热空气通过蒸发媒介时,蒸发媒介上的水分蒸发并从空气中带走热量,导致空气温度降低。
具体运作步骤如下:
1. 冷水经过冷却机组(冷却塔或冷却卷管)冷却,形成冷水。
2. 冷水通过喷淋系统喷洒在蒸发媒介上。
蒸发媒介可是湿滑板或湿膜,其表面含有许多小孔和构造,以增加接触面积。
3. 热空气通过蒸发媒介时,与湿度高的蒸发媒介表面接触。
热空气中的热量使蒸发媒介上的水分蒸发,将热量带走,从而使空气温度降低。
4. 冷却后的空气被送回到房间,实现空调效果。
5. 蒸发媒介上的水分被排到下水管道中,通过冷却机组冷却再使用。
这种间接蒸发冷原理的优势是省电、环保,因为其主要依靠水的蒸发来降低空气温度,与直接蒸发冷原理相比,省去了电力能耗较大的压缩机。
同时,与传统的冷却塔原理相比,使用蒸发媒介可以增加接触面积,提高冷却效果。
不过,其劣势是需要提供冷水供给和处理蒸发媒介上的水分。
蒸发冷空调原理
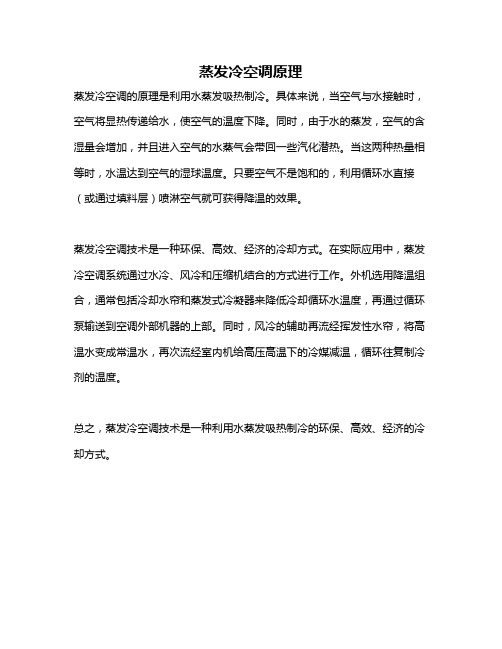
蒸发冷空调原理
蒸发冷空调的原理是利用水蒸发吸热制冷。
具体来说,当空气与水接触时,空气将显热传递给水,使空气的温度下降。
同时,由于水的蒸发,空气的含湿量会增加,并且进入空气的水蒸气会带回一些汽化潜热。
当这两种热量相等时,水温达到空气的湿球温度。
只要空气不是饱和的,利用循环水直接(或通过填料层)喷淋空气就可获得降温的效果。
蒸发冷空调技术是一种环保、高效、经济的冷却方式。
在实际应用中,蒸发冷空调系统通过水冷、风冷和压缩机结合的方式进行工作。
外机选用降温组合,通常包括冷却水帘和蒸发式冷凝器来降低冷却循环水温度,再通过循环泵输送到空调外部机器的上部。
同时,风冷的辅助再流经挥发性水帘,将高温水变成常温水,再次流经室内机给高压高温下的冷媒减温,循环往复制冷剂的温度。
总之,蒸发冷空调技术是一种利用水蒸发吸热制冷的环保、高效、经济的冷却方式。
蒸发冷却原理

蒸发冷却原理“同学们,今天我们来探讨一个很有趣的物理现象——蒸发冷却。
”我微笑着对学生们说道。
蒸发冷却,简单来说就是液体蒸发时会吸收热量,从而使周围环境温度降低。
这一原理在我们的日常生活中有很多应用。
比如说,大家在夏天的时候,是不是感觉出汗后会稍微凉快一些呢?这就是因为汗水在蒸发的过程中带走了我们体表的热量。
想象一下,炎热的夏天,我们运动过后,汗水不断地渗出,然后慢慢蒸发,这个过程就让我们的身体温度有所下降,让我们感觉没那么热了。
再比如,在一些工厂或者机房里,会有大型的冷却设备,其中就有利用蒸发冷却原理的。
这些设备会让水不断地蒸发,从而降低机器设备周围的温度,保证它们能够正常运行。
我曾经参观过一个数据中心,那里就采用了这种蒸发冷却技术。
他们有一个巨大的冷却塔,里面不断有水在循环,通过蒸发来降低整个数据中心的温度。
蒸发冷却还在农业上有重要的应用呢。
农民伯伯们在灌溉农作物的时候,水的蒸发也会起到一定的降温作用,为农作物创造一个相对适宜的生长环境。
在一些干旱地区,人们还会利用蒸发冷却的原理来建造一些特殊的房屋,让房屋内部在炎热的夏天也能保持相对凉爽。
而且啊,蒸发冷却不仅仅是降低温度这么简单。
它还可以帮助我们实现一些其他的目的。
比如在一些需要干燥的工业过程中,通过控制蒸发冷却的速度和程度,可以实现对物料的干燥处理。
同学们可能会问了,那蒸发冷却有没有什么限制呢?当然有啦。
蒸发冷却的效果会受到很多因素的影响,比如空气的湿度、温度、风速等等。
在湿度很大的情况下,蒸发冷却的效果可能就没那么明显了,因为空气中已经有很多水分了,水就不容易再蒸发了。
总之,蒸发冷却原理虽然看似简单,但是却有着广泛的应用和重要的意义。
它不仅让我们的生活更加舒适,也为很多行业的发展提供了支持。
希望同学们能够通过今天的学习,对蒸发冷却原理有更深入的理解和认识,也希望大家能够在生活中多观察、多思考,发现更多物理现象背后的奥秘。
蒸发冷却器工作原理

蒸发冷却器工作原理
蒸发冷却器是一种常用的空气冷却装置,其工作原理基于水的蒸发过程。
具体原理如下:
1. 蒸发水道:蒸发冷却器内部有许多细小的水道,水通过这些水道被分散喷洒在冷却器的填料层上。
2. 填料层:填料层由许多表面积大、孔隙度高的材料构成,如木脱水材料或塑料结构。
填料层的作用是增大水和空气的接触面积,以便有更多的水能够蒸发。
3. 空气循环:热空气通过蒸发冷却器时,会与填料层上的水进行热交换。
蒸发过程需要吸收热量,而这些热量来自空气本身,使空气温度下降。
4. 水循环:在蒸发过程中,水逐渐蒸发消耗,因此需要一定的水循环系统来将水重新输送到水道中。
一般情况下,蒸发冷却器的底部设有水池,通过水泵将水抽回到水道中,确保持续的冷却效果。
5. 扇风系统:蒸发冷却器通常与扇风系统结合使用。
扇风通过冷却器中的湿润填料层,带走蒸发过程中吸收的热量,然后将冷却过的空气吹出。
这样,冷却器能够迅速将周围空气的温度降低。
总的来说,蒸发冷却器的工作原理是通过水的蒸发过程将空气
冷却。
这种原理使得蒸发冷却器在炎热天气中提供有效的空气冷却,并且相对能源消耗较低。
典型蒸发冷却传热过程
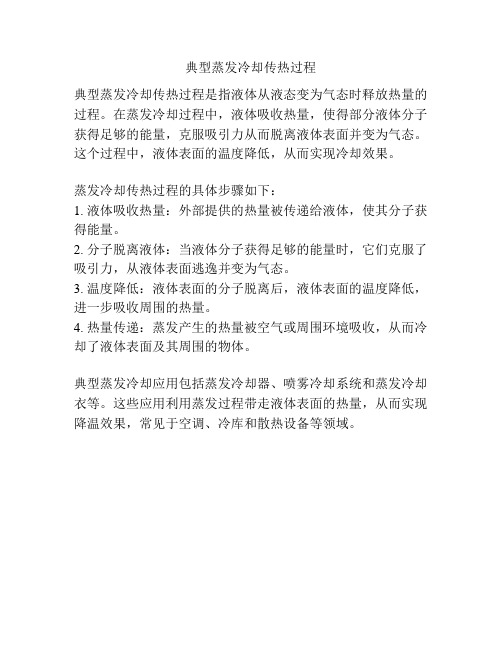
典型蒸发冷却传热过程
典型蒸发冷却传热过程是指液体从液态变为气态时释放热量的过程。
在蒸发冷却过程中,液体吸收热量,使得部分液体分子获得足够的能量,克服吸引力从而脱离液体表面并变为气态。
这个过程中,液体表面的温度降低,从而实现冷却效果。
蒸发冷却传热过程的具体步骤如下:
1. 液体吸收热量:外部提供的热量被传递给液体,使其分子获得能量。
2. 分子脱离液体:当液体分子获得足够的能量时,它们克服了吸引力,从液体表面逃逸并变为气态。
3. 温度降低:液体表面的分子脱离后,液体表面的温度降低,进一步吸收周围的热量。
4. 热量传递:蒸发产生的热量被空气或周围环境吸收,从而冷却了液体表面及其周围的物体。
典型蒸发冷却应用包括蒸发冷却器、喷雾冷却系统和蒸发冷却衣等。
这些应用利用蒸发过程带走液体表面的热量,从而实现降温效果,常见于空调、冷库和散热设备等领域。
蒸发冷工作原理

蒸发冷工作原理蒸发冷是一种利用水的蒸发吸收热量的原理来达到降温的技术。
它的工作原理是将水喷洒在空气中,通过水的蒸发吸收空气中的热量,从而达到降温的效果。
蒸发冷技术广泛应用于工业、农业、商业和家庭等领域,成为一种节能环保的降温方式。
一、蒸发冷的原理蒸发冷的原理是利用水的蒸发吸收热量的特性来达到降温的效果。
当水蒸发时,需要吸收周围环境的热量,这个过程中,水的温度会下降,周围环境的温度也会下降。
因此,将水喷洒在空气中,通过水的蒸发吸收空气中的热量,就可以达到降温的效果。
二、蒸发冷的应用1. 工业领域在工业领域,蒸发冷技术被广泛应用于冶金、化工、电力等行业。
例如,在冶金行业中,高温炉炉温过高,会影响生产效率和产品质量,使用蒸发冷技术可以有效地降低炉温,提高生产效率和产品质量。
2. 农业领域在农业领域,蒸发冷技术被广泛应用于温室种植。
温室内温度过高会影响植物的生长和产量,使用蒸发冷技术可以有效地降低温室内的温度,提高植物的生长和产量。
3. 商业领域在商业领域,蒸发冷技术被广泛应用于商场、超市、酒店等场所。
这些场所人流量大,空调系统的负荷较大,使用蒸发冷技术可以有效地降低空调系统的负荷,节约能源,降低运行成本。
4. 家庭领域在家庭领域,蒸发冷技术被广泛应用于空调、风扇等家电产品。
使用蒸发冷技术的空调和风扇可以有效地降低室内温度,提高生活舒适度。
三、蒸发冷的优点1. 节能环保蒸发冷技术不需要使用制冷剂,不会产生有害物质,对环境没有污染,是一种节能环保的降温方式。
2. 降温效果好蒸发冷技术可以有效地降低室内温度,提高生活舒适度。
3. 适用范围广蒸发冷技术适用于工业、农业、商业和家庭等领域,具有广泛的应用前景。
四、蒸发冷的缺点1. 适用范围受限蒸发冷技术适用于干燥的气候环境,湿度较高的环境下效果不佳。
2. 维护成本高蒸发冷设备需要定期清洗和维护,维护成本较高。
总之,蒸发冷技术是一种节能环保的降温方式,具有广泛的应用前景。
蒸发冷 冷却塔
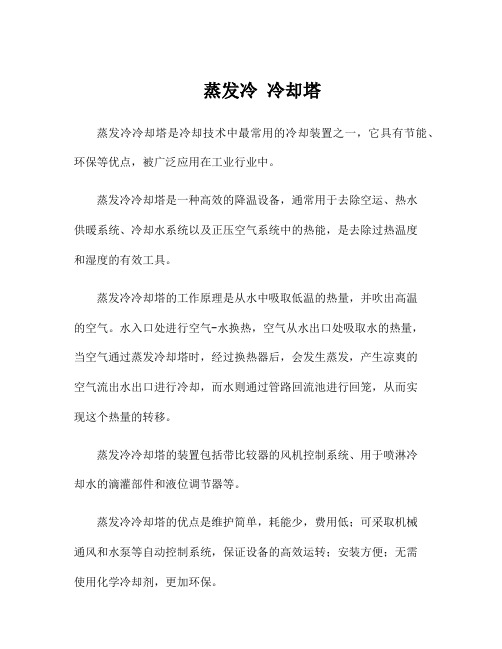
蒸发冷冷却塔
蒸发冷冷却塔是冷却技术中最常用的冷却装置之一,它具有节能、环保等优点,被广泛应用在工业行业中。
蒸发冷冷却塔是一种高效的降温设备,通常用于去除空运、热水
供暖系统、冷却水系统以及正压空气系统中的热能,是去除过热温度
和湿度的有效工具。
蒸发冷冷却塔的工作原理是从水中吸取低温的热量,并吹出高温
的空气。
水入口处进行空气-水换热,空气从水出口处吸取水的热量,
当空气通过蒸发冷却塔时,经过换热器后,会发生蒸发,产生凉爽的
空气流出水出口进行冷却,而水则通过管路回流池进行回笼,从而实
现这个热量的转移。
蒸发冷冷却塔的装置包括带比较器的风机控制系统、用于喷淋冷
却水的滴灌部件和液位调节器等。
蒸发冷冷却塔的优点是维护简单,耗能少,费用低;可采取机械
通风和水泵等自动控制系统,保证设备的高效运转;安装方便;无需
使用化学冷却剂,更加环保。
但蒸发冷冷却塔也有缺点,就是容易受恶劣环境(如腐蚀性气体、水管冻裂)影响,而且需要定期维护和清洗。
因此,在选择蒸发冷冷却塔时,应该根据各种要求,仔细考虑其
优缺点,确定合适的产品,以确保其高效运行,节约能源,保护环境。
蒸发冷原理
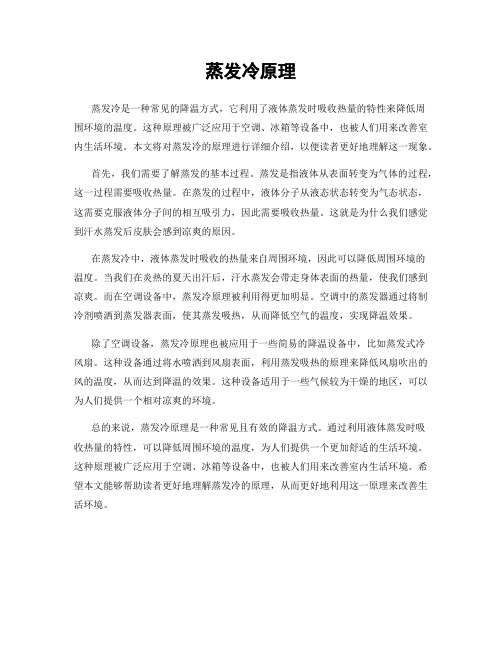
蒸发冷原理
蒸发冷是一种常见的降温方式,它利用了液体蒸发时吸收热量的特性来降低周
围环境的温度。
这种原理被广泛应用于空调、冰箱等设备中,也被人们用来改善室内生活环境。
本文将对蒸发冷的原理进行详细介绍,以便读者更好地理解这一现象。
首先,我们需要了解蒸发的基本过程。
蒸发是指液体从表面转变为气体的过程,这一过程需要吸收热量。
在蒸发的过程中,液体分子从液态状态转变为气态状态,这需要克服液体分子间的相互吸引力,因此需要吸收热量。
这就是为什么我们感觉到汗水蒸发后皮肤会感到凉爽的原因。
在蒸发冷中,液体蒸发时吸收的热量来自周围环境,因此可以降低周围环境的
温度。
当我们在炎热的夏天出汗后,汗水蒸发会带走身体表面的热量,使我们感到凉爽。
而在空调设备中,蒸发冷原理被利用得更加明显。
空调中的蒸发器通过将制冷剂喷洒到蒸发器表面,使其蒸发吸热,从而降低空气的温度,实现降温效果。
除了空调设备,蒸发冷原理也被应用于一些简易的降温设备中,比如蒸发式冷
风扇。
这种设备通过将水喷洒到风扇表面,利用蒸发吸热的原理来降低风扇吹出的风的温度,从而达到降温的效果。
这种设备适用于一些气候较为干燥的地区,可以为人们提供一个相对凉爽的环境。
总的来说,蒸发冷原理是一种常见且有效的降温方式。
通过利用液体蒸发时吸
收热量的特性,可以降低周围环境的温度,为人们提供一个更加舒适的生活环境。
这种原理被广泛应用于空调、冰箱等设备中,也被人们用来改善室内生活环境。
希望本文能够帮助读者更好地理解蒸发冷的原理,从而更好地利用这一原理来改善生活环境。
蒸发冷却的原理

蒸发冷却的原理嘿,朋友们!今天咱来聊聊蒸发冷却这个神奇的原理呀!你看,夏天的时候,我们出了一身汗,风一吹,哇,感觉一下子凉快了不少。
这其实就有点像蒸发冷却啦!水从我们的皮肤上变成水蒸气跑掉了,同时也带走了热量,可不就凉快了嘛!想象一下,蒸发冷却就像是一个魔法,能在炎热的天气里给我们带来一丝清凉的慰藉。
就好比在沙漠中行走,干渴难耐时突然发现了一汪清泉,那是多么让人惊喜呀!蒸发冷却在生活中的应用可不少呢!比如说空调,它就是利用这个原理来工作的。
空调里面有制冷剂,它会在管道里循环,一会儿变成液体,一会儿又变成气体。
当它变成气体的时候,就像水变成水蒸气一样,会吸收周围的热量,让我们的房间变凉快。
再说说我们平时用的湿毛巾。
你把湿毛巾挂在脖子上,过一会儿,你就会感觉脖子那块凉凉的。
这就是水蒸发带走了热量呀!这不就是蒸发冷却在我们身边的小例子嘛!那蒸发冷却和我们的大自然又有啥关系呢?嘿,关系可大了去了!植物的蒸腾作用也是一种蒸发冷却呀!植物通过叶子上的气孔,把水分蒸发出去,这不仅能让植物自己凉快,还能调节周围的温度呢!这不就像大自然有了自己的“空调”一样嘛!还有啊,在一些干旱的地区,水蒸发得特别快,这就会让那里的温度下降得比较快,晚上就会比较凉快。
这是不是很神奇呢?你说蒸发冷却是不是很厉害?它就像是一个默默无闻的英雄,在我们身边悄悄地发挥着作用。
它不需要我们特意去关注它,却一直在为我们的舒适生活贡献着力量。
咱再想想,如果没有蒸发冷却,这世界会变成啥样?夏天会变得更加酷热难耐,空调也没法工作了,那我们可咋办呀?所以呀,我们得好好珍惜这个神奇的原理,好好利用它带给我们的好处。
总之呢,蒸发冷却虽然看起来很简单,但它的作用可真是不容小觑啊!它让我们的生活变得更加舒适,也让大自然变得更加美好。
让我们一起为蒸发冷却点个赞吧!。
蒸发冷
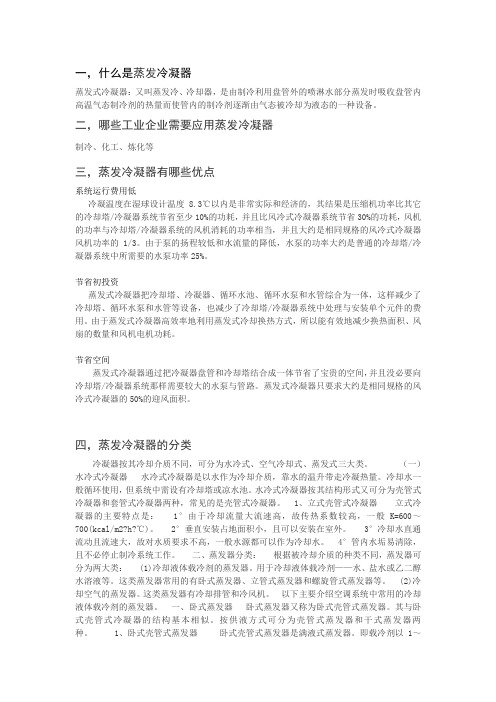
一,什么是蒸发冷凝器蒸发式冷凝器:又叫蒸发冷、冷却器,是由制冷利用盘管外的喷淋水部分蒸发时吸收盘管内高温气态制冷剂的热量而使管内的制冷剂逐渐由气态被冷却为液态的一种设备。
二,哪些工业企业需要应用蒸发冷凝器制冷、化工、炼化等三,蒸发冷凝器有哪些优点系统运行费用低冷凝温度在湿球设计温度8.3℃以内是非常实际和经济的,其结果是压缩机功率比其它的冷却塔/冷凝器系统节省至少10%的功耗,并且比风冷式冷凝器系统节省30%的功耗,风机的功率与冷却塔/冷凝器系统的风机消耗的功率相当,并且大约是相同规格的风冷式冷凝器风机功率的1/3。
由于泵的扬程较低和水流量的降低,水泵的功率大约是普通的冷却塔/冷凝器系统中所需要的水泵功率25%。
节省初投资蒸发式冷凝器把冷却塔、冷凝器、循环水池、循环水泵和水管综合为一体,这样减少了冷却塔、循环水泵和水管等设备,也减少了冷却塔/冷凝器系统中处理与安装单个元件的费用。
由于蒸发式冷凝器高效率地利用蒸发式冷却换热方式,所以能有效地减少换热面积、风扇的数量和风机电机功耗。
节省空间蒸发式冷凝器通过把冷凝器盘管和冷却塔结合成一体节省了宝贵的空间,并且没必要向冷却塔/冷凝器系统那样需要较大的水泵与管路。
蒸发式冷凝器只要求大约是相同规格的风冷式冷凝器的50%的迎风面积。
四,蒸发冷凝器的分类冷凝器按其冷却介质不同,可分为水冷式、空气冷却式、蒸发式三大类。
(一)水冷式冷凝器水冷式冷凝器是以水作为冷却介质,靠水的温升带走冷凝热量。
冷却水一般循环使用,但系统中需设有冷却塔或凉水池。
水冷式冷凝器按其结构形式又可分为壳管式冷凝器和套管式冷凝器两种,常见的是壳管式冷凝器。
1、立式壳管式冷凝器立式冷凝器的主要特点是: 1°由于冷却流量大流速高,故传热系数较高,一般K=600~700(kcal/m2?h?℃)。
2°垂直安装占地面积小,且可以安装在室外。
3°冷却水直通流动且流速大,故对水质要求不高,一般水源都可以作为冷却水。
蒸发冷填料

蒸发冷填料
蒸发冷填料是一种用于冷却系统中的填料材料。
它具有优异的热传导性能和高吸湿性。
蒸发冷填料可以通过吸湿蒸发的过程,将周围环境中的热量吸收并散发出去,从而起到降温的效果。
蒸发冷填料通常由多孔的材料制成,如聚酯纤维、聚氨酯泡沫等。
这些材料具有较大的表面积和丰富的微孔结构,能够提供更多的接触面积来促进蒸发传热。
在冷却系统中,蒸发冷填料被安装在冷却塔或空调设备的内部。
当空气通过填料时,填料表面的湿度增加,并吸收空气中的热量。
随后,这些水分通过蒸发变成水蒸气,带走热量,从而产生降温效果。
蒸发冷填料的设计和选择很关键,要考虑到填料的材质、孔径、厚度等因素。
合适的填料能够提供较大的表面积和通风性,从而提高传热效率和降温效果。
总的来说,蒸发冷填料是一种高效的实现空调和冷却系统降温的方法。
它通过蒸发传热原理,帮助将热量散发到周围环境中,起到有效冷却的作用。
蒸发冷却原理
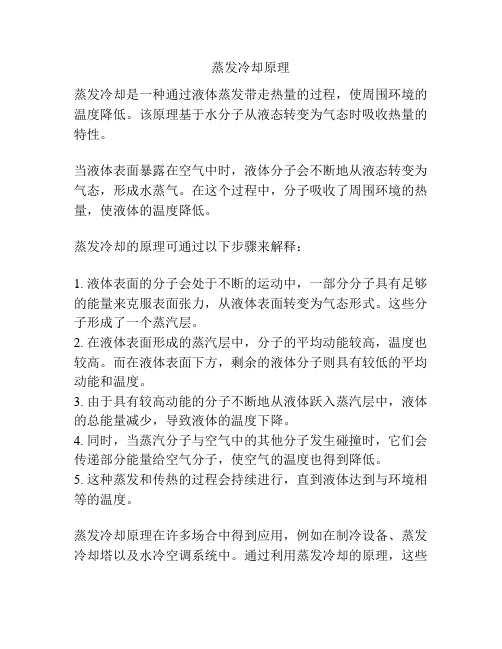
蒸发冷却原理
蒸发冷却是一种通过液体蒸发带走热量的过程,使周围环境的温度降低。
该原理基于水分子从液态转变为气态时吸收热量的特性。
当液体表面暴露在空气中时,液体分子会不断地从液态转变为气态,形成水蒸气。
在这个过程中,分子吸收了周围环境的热量,使液体的温度降低。
蒸发冷却的原理可通过以下步骤来解释:
1. 液体表面的分子会处于不断的运动中,一部分分子具有足够的能量来克服表面张力,从液体表面转变为气态形式。
这些分子形成了一个蒸汽层。
2. 在液体表面形成的蒸汽层中,分子的平均动能较高,温度也较高。
而在液体表面下方,剩余的液体分子则具有较低的平均动能和温度。
3. 由于具有较高动能的分子不断地从液体跃入蒸汽层中,液体的总能量减少,导致液体的温度下降。
4. 同时,当蒸汽分子与空气中的其他分子发生碰撞时,它们会传递部分能量给空气分子,使空气的温度也得到降低。
5. 这种蒸发和传热的过程会持续进行,直到液体达到与环境相等的温度。
蒸发冷却原理在许多场合中得到应用,例如在制冷设备、蒸发冷却塔以及水冷空调系统中。
通过利用蒸发冷却的原理,这些
设备能够降低空气温度,提供舒适的环境,并实现能源的高效利用。
蒸发冷却技术原理
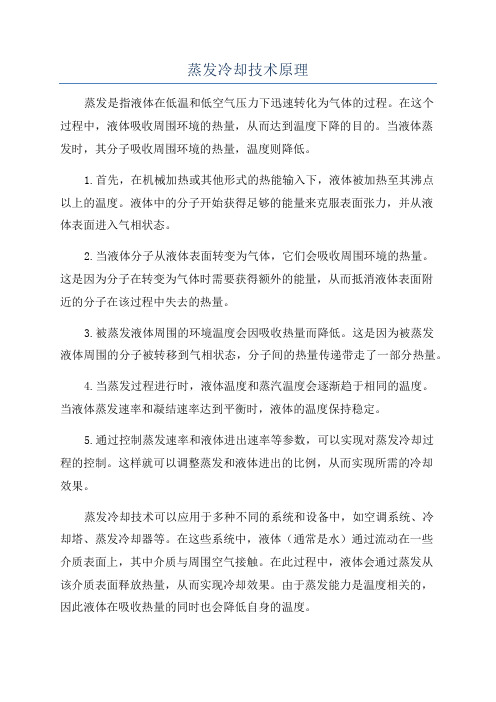
蒸发冷却技术原理蒸发是指液体在低温和低空气压力下迅速转化为气体的过程。
在这个过程中,液体吸收周围环境的热量,从而达到温度下降的目的。
当液体蒸发时,其分子吸收周围环境的热量,温度则降低。
1.首先,在机械加热或其他形式的热能输入下,液体被加热至其沸点以上的温度。
液体中的分子开始获得足够的能量来克服表面张力,并从液体表面进入气相状态。
2.当液体分子从液体表面转变为气体,它们会吸收周围环境的热量。
这是因为分子在转变为气体时需要获得额外的能量,从而抵消液体表面附近的分子在该过程中失去的热量。
3.被蒸发液体周围的环境温度会因吸收热量而降低。
这是因为被蒸发液体周围的分子被转移到气相状态,分子间的热量传递带走了一部分热量。
4.当蒸发过程进行时,液体温度和蒸汽温度会逐渐趋于相同的温度。
当液体蒸发速率和凝结速率达到平衡时,液体的温度保持稳定。
5.通过控制蒸发速率和液体进出速率等参数,可以实现对蒸发冷却过程的控制。
这样就可以调整蒸发和液体进出的比例,从而实现所需的冷却效果。
蒸发冷却技术可以应用于多种不同的系统和设备中,如空调系统、冷却塔、蒸发冷却器等。
在这些系统中,液体(通常是水)通过流动在一些介质表面上,其中介质与周围空气接触。
在此过程中,液体会通过蒸发从该介质表面释放热量,从而实现冷却效果。
由于蒸发能力是温度相关的,因此液体在吸收热量的同时也会降低自身的温度。
除了蒸发原理以外,蒸发冷却技术还需要考虑一些其他因素,如湿度、通风等。
湿度对蒸发冷却过程起到重要的影响,因为当空气湿度较高时,空气中已经含有大量水分,从而降低了液体蒸发的速率。
通风可以帮助移走蒸发所释放的湿气,以保持蒸发效果。
综上所述,蒸发冷却技术利用液体蒸发时吸收热量的特性,通过将热量从液体中吸收并释放到周围环境中,从而实现降温的目的。
这种技术在许多应用领域都得到了广泛应用,能够有效提供舒适和节能的冷却效果。
- 1、下载文档前请自行甄别文档内容的完整性,平台不提供额外的编辑、内容补充、找答案等附加服务。
- 2、"仅部分预览"的文档,不可在线预览部分如存在完整性等问题,可反馈申请退款(可完整预览的文档不适用该条件!)。
- 3、如文档侵犯您的权益,请联系客服反馈,我们会尽快为您处理(人工客服工作时间:9:00-18:30)。
Artificial neural network analysis of a refrigeration systemwith an evaporative condenserH.M.Ertunc a ,M.Hosozb,*aDepartment of Mechatronics Engineering,Kocaeli University,41040Kocaeli,Turkey bDepartment of Mechanical Education,Kocaeli University,41380Kocaeli,TurkeyReceived 14December 2004;accepted 7June 2005Available online 3August 2005AbstractThis paper describes an application of artificial neural networks (ANNs)to predict the performance of a refrigeration system with an evaporative condenser.In order to gather data for training and testing the proposed ANN,an experimental refrigeration system with an evaporative condenser was set up.Then,steady-state test runs were conducted varying the evaporator load,air and water flow rates passing through the condenser and both dry and wet bulb temperatures of the air stream entering the condenser.Utilizing some of the experimental data,an ANN model for the system based on standard backpropagation algorithm was devel-oped.The ANN was used for predicting various performance parameters of the system,namely the condenser heat rejection rate,refrigerant mass flow rate,compressor power,electric power input to the compressor motor and the coefficient of performance.The ANN predictions usually agree well with the experimental values with correlation coefficients in the range of 0.933–1.000,mean rel-ative errors in the range of 1.90–4.18%and very low root mean square errors.Results show that refrigeration systems,even complex ones involving concurrent heat and mass transfer such as systems with an evaporative condenser,can alternatively be modelled using ANNs within a high degree of accuracy.Ó2005Elsevier Ltd.All rights reserved.Keywords:Artificial neural network;Refrigeration;Evaporative condenser1.IntroductionThe vapour-compression refrigeration system em-ploys a condenser to reject the heat absorbed by the refrigerant in the evaporator.Depending on the type of the cooling medium,condensers can be classified as air-cooled,water-cooled and evaporative condensers.The evaporative condenser is a compact heat exchanger combining the functions of air and water-cooled con-densers and a cooling tower.In this condenser,thesuperheated vapour pumped by the compressor flows through a bank of tubes.The external surface of the tubes is continually kept wet by a water-distributing system.On the other hand,air is drawn through the condenser casing and sent upwards over the tubes.Absorbing heat from the refrigerant,some of the water on the tubes evaporates into the air.Consequently,the refrigerant vapour gives up its heat and condenses.Because the evaporative condenser offers condensing temperatures limited by ambient wet bulb temperature,which is almost always lower than ambient dry bulb temperature,refrigeration systems employing it can work with lower condensing temperatures.Therefore,these systems are usually more energy-efficient com-pared to ones using air-cooled condensers.Furthermore,initial cost of the evaporative condenser is lower than1359-4311/$-see front matter Ó2005Elsevier Ltd.All rights reserved.doi:10.1016/j.applthermaleng.2005.06.002*Corresponding author.Tel.:+902623032279;fax:+902623032203.E-mail addresses:mhosoz@.tr ,mhosoz@ (M.Hosoz)./locate/apthermengApplied Thermal Engineering 26(2006)627–635that of the water-cooled condenser due to the reduced space and number of the components.While it is relatively simple to accurately model the heat transfer in air and water-cooled condensers,the evaporative condenser presents some difficulties owing to water evaporation into the air stream involved.Based on the early evaporative condenser models[1,2],which usually yielded incorrect results,Peterson et al.devel-oped and tested an analytical condenser model[3].They found that their model underpredicted the heat load by 30%.Goswami et al.[4]investigated the performance of air conditioners with air-cooled condensers using evapo-ratively-cooled ambient air.Ettouney et al.[5]developed correlations for the external heat transfer coefficient of an evaporative condenser.Hwang et al.[6]determined experimental performance of a novel evaporative condenser relying on rotating wet disks to reject heat. Hosoz and Kilicarslan[7]compared performance characteristics of refrigeration systems using evapora-tive,water-cooled and air-cooled condensers.It is seen from the review of the literature that it is too hard to accurately determine the performance of an evaporative condenser and consequently the perfor-mance of a refrigeration system with this condenser using classical modelling techniques.As an alternative, a refrigeration system employing an evaporative con-denser can be modelled using ANNs.This new model-ling technique is based on imitating the structure and mechanisms of the human brain,and being used in more and more engineering applications where classical ap-proaches fail or they are too complicated to be used. ANNs are applied to estimate desired output parameters when enough experimental data is provided.Therefore, ANNs allow the modelling of physical phenomena in complex systems without requiring explicit mathemati-cal representations.ANN modelling of energy systems has been recently studied by numerous investigators,as reviewed by Kal-ogirou[8].Pacheco-Vega et al.[9]applied the ANN to model the heat transfer of afin-tube heat exchanger used for refrigeration applications.Bechtler et al.[10]pre-sented an ANN model for predicting the steady-state performance of a vapour-compression heat pump with various refrigerants.They also modelled dynamic pro-cesses of vapour-compression liquid chillers to predict the coefficient of performance(COP)and compressorNomenclaturea actual outputA0orifice cross-section area(m2)b biasCOP coefficient of performanceCov covariancef activation functionh enthalpy of the refrigerant(kJ kgÀ1)h a enthalpy of the air(kJ kgÀ1)h m orifice differential(mm H2O)I currentflow through the heaters(A)K0flow coefficient_m massflow rate(g sÀ1)MRE mean relative errorn sum of the weighted inputsN number of the points in the data setp predicted outputP number of elements in the input vectorQ cond heat rejection rate in the condenser(W)Q evap refrigeration capacity(W)R correlation coefficientRMSE root mean square errorT a,i dry bulb temperature of the air at the inlet of the condenser(°C)T a,i,w wet bulb temperature of the air at the inlet of the condenser(°C)V voltage across the heaters(V)v a,e specific volume of the air at the exit of the condenser(m3kgÀ1)w interconnection weightW c compressor power(W)W el electric power input to the compressor motor (W)j W p j power absorbed by water in the pump(W) x input of a neuronY expansion factorGreek symbolsg mech mechanical efficiency of the compressorg motor efficiency of the compressor motorq a,e density of the air at the exit of the condenser (kg mÀ3)D P orifice pressure drop(Pa)Subscriptsa airc compressorcond condensere exitel electricevap evaporatori inletr refrigerantw water or wet bulb628H.M.Ertunc,M.Hosoz/Applied Thermal Engineering26(2006)627–635work input[11].Prieto et al.[12]presented an ANN model for predicting the performance of a power plant condenser.Chouai et al.[13]worked on the ANN modelling of the thermodynamic properties of several refrigerants.Swider[14]compared empirically based steady-state models including ANNs for modelling vapour-compression liquid chillers.Sozen et al.[15] compared the results of an ANN model for the analysis of ejector-absorption refrigeration systems with those of analytic functions.Arcaklioglu[16]developed an ANN model for predicting the COP and total irreversibility of a vapour-compression refrigeration system.Arcaklioglu et al.[17]determined the performance of a vapour-com-pression heat pump with different ratios of R12/R22 refrigerant mixtures using ANNs.Islamoglu[18]devel-oped an ANN model for predicting the suction line outlet temperature and massflow rate of a capillary tube suction line heat exchanger used in household refrigerators.In this study,the ANN approach has been applied to a vapour-compression refrigeration system employing an evaporative condenser.Utilizing data obtained from steady-state test runs of an experimental refrigeration system,an ANN model for the system has been devel-oped.The ANN model has been used for predicting var-ious performance parameters of the refrigeration system,namely the condenser heat rejection rate,refrig-erant massflow rate,compressor power,electric power input to the compressor motor and COP.2.Artificial neural networksAn ANN tries to mirror the brain functions in a computerized way by resorting to the learning mecha-nism as the basis of human behaviour.Utilizing the samples from the experiments,ANNs can be applied to the problems with no algorithmic solutions or with too complex algorithmic solutions to be found.Their ability of learning by examples makes the ANNs more flexible and powerful than the parametric approaches [19].An ANN consists of massively interconnected pro-cessing nodes known as neurons.Each neuron accepts a weighted set of inputs and responds with an output. Such a neuronfirst forms the sum of the weighted inputs given byn¼X Pi¼1w i x i!þbð1Þwhere P and w i are the number of elements and the interconnection weight of the input vector x i,respec-tively,and b is the bias for the neuron[20].Note that the knowledge is stored as a set of connection weights and biases.The sum of the weighted inputs with a bias is processed through an activation function,represented by f,and the output that it computes isfðnÞ¼fX Pi¼1w i x i!þb"#ð2ÞBasically,the neuron model represents the biological neuron thatfires when its inputs are significantly excited,i.e.,n is big enough.There are many ways to de-fine the activation function such as threshold function, sigmoid function,and hyperbolic tangent function.ANNs can be trained to perform a particular func-tion by adjusting the values of connections,i.e.,weight-ing coefficients,between the processing nodes.In other words,ANNs are trained to reach from a particular in-put to a specific target output using a suitable learning method until the network output matches the target. The error between the output of the network and the de-sired output is minimized by modifying the weights and biases.When the error falls below a certain value or the maximum number of epochs is exceeded,training pro-cess is ceased.Then,this trained network can be used for simulating the system outputs for the inputs which have not been introduced before.The architecture of an ANN is usually divided into three parts:an input layer,a hidden layer(s)and an output layer.The information contained in the input layer is mapped to the output layer through the hidden layer(s).Each unit can send its output to the units only on the higher layer and receive its input from the lower layer.For a given modelling problem,the numbers of nodes in the input and output layers are determined from the physics of the problem,and equal to the numbers of input and output parameters,respectively.The performance of the ANN-based prediction is evaluated by a regression analysis between the network outputs,i.e.,predicted parameters,and the correspond-ing targets,i.e.,experimental(actual)values.The corre-lation coefficient,mean relative error and root mean square error are the three criterions that can be used in this evaluation.The correlation coefficient is a mea-sure of how well the variation in the output is explained by the targets.This coefficient between the actual versus predicted outputs is defined as follows[21]:Rða;pÞ¼Covða;pÞffiffiffiffiffiffiffiffiffiffiffiffiffiffiffiffiffiffiffiffiffiffiffiffiffiffiffiffiffiffiffiffiffiffiffiffiffiffiffiCovða;aÞCovðp;pÞpð3Þwhere Cov(a,p)is covariance between a and p sets that refer to the actual output and predicted output sets, respectively.Likewise,Cov(a,a)and Cov(p,p)are the auto covariances of a and p sets,correspondingly.The correlation coefficient ranges betweenÀ1and+1.R val-ues closer to+1indicate a stronger positive linear rela-tionship while R values closer toÀ1indicate a stronger negative relationship.H.M.Ertunc,M.Hosoz/Applied Thermal Engineering26(2006)627–635629The mean relative error,which shows the mean ratio between the errors and experimental values,is evaluated byMREð%Þ¼1NX Ni¼1100ða iÀp iÞa ið4Þwhere N is the number of the points in the data set.Finally,the root mean square error is defined asRMSE¼ffiffiffiffiffiffiffiffiffiffiffiffiffiffiffiffiffiffiffiffiffiffiffiffiffiffiffiffiffiffiffi1NX Ni¼1ða iÀp iÞ2v uu tð5Þ3.Description of the experimental setup and testing procedureThe ANN modelling has been applied to an experi-mental R134a vapour-compression refrigeration unit consisting of a reciprocating compressor,an evaporative condenser,a liquid receiver,afilter–drier,a thermostatic expansion valve and an electrically heated evaporator, as shown in Fig.1.The twin-cylinder open-type com-pressor is driven by a single-phase electric motor.The evaporator consists of copper tube and electric heaters rolled inside the tube.The refrigeration load is supplied to the evaporator by varying the voltage across the elec-tric heaters between0and220V.The evaporative condenser consists of air and water circuit elements along with a bare copper coil having three refrigerant circuits connected in parallel by head-ers.The tube outside diameter and total external surface area of the coil are6.35mm and0.100m2,respectively. The air,water and refrigerant streams exchange heat in a column of150mm·150mm·600mm high.Ambient air is pulled into the column by means of a centrifugal fan at a rate determined by the adjustment of the dam-per setting.Having absorbed heat,the air stream passes through a droplet arrester,and then discharged to the atmosphere via an orifice used for measuring the airflow rate.The water stream is let to fall down the column spreading over the coil surface.Afterwards,the water passes into a tank from where the circulation pump draws it and sends to the top of the column via a control valve determining the waterflow rate in the system.The evaporator,condenser,liquid receiver,expansion valve and pipeline were insulated with either elastomeric insu-lator or polyurethane foam.During tests,the evapora-tive condenser was located inside a special room where dry and wet bulb temperatures could be kept at required values.The locations where mechanical and electrical mea-surements were conducted are also shown in Fig.1. All temperature measurements were performed using K-type thermocouples.Refrigerant thermocouples were located at the inlet and outlet of each component.Both dry and wet bulb temperatures of the air stream entering and leaving the evaporative condenser were measured. Refrigerant pressures were detected at the inlet and out-let of the compressor.Since the length of the pipeline is relatively short,the evaporator and condenser pressures were assumed to be equal to the measured ones.The refrigerant and water massflow rates were measured by variable-areaflow meters.The air massflow rate was determined by measuring orifice differential with an inclined manometer,finding specific volume of the air leaving the condenser with the help of dry and wet bulb temperature measurements,and evaluating them in the followingequation:Fig.1.Schematic diagram of the experimental refrigeration system. 630H.M.Ertunc,M.Hosoz/Applied Thermal Engineering26(2006)627–635_m a¼q a;e K0A0Yffiffiffiffiffiffiffiffiffi2D Pqa;esffi0.0137ffiffiffiffiffiffiffih mv a;esð6ÞThe heat input to the evaporator was found by mea-suring the voltage across the electric heaters and the cur-rent passing through them.The electric power input to the compressor motor was measured by an analogue wattmeter.The evaporator load,i.e.,the refrigeration capacity of the system,can be evaluated for both the heaters side and the refrigerant side.Assuming that the evaporator is insulated perfectly,the evaporator load can be written asQevap¼VIffi_m rðh evap;eÀh evap;iÞð7ÞAs seen in Eq.(7),the evaporator load for the heaters side utilizes voltage and current measurements while that for the refrigerant side uses measurements of the refrigerant massflow rate along with pressures and tem-peratures of the refrigerant at the exit and inlet of the evaporator.The capacity deviation between two sides was usually within±5%,and only the heaters side re-sults were used as the evaporator load due to their hav-ing lower uncertainities.Then,the refrigerant massflow rate in the system based on evaporator load for the heat-ers side was determined from:_m r¼VIh evap;eÀh evap;ið8ÞThe accuracy for the refrigerant massflow rate mea-surements conducted by the variable-areaflow meter, which is equal to±5%,is poorer than the uncertainity for theflow rates determined from Eq.(8).Therefore, only the results of this equation were used as the refrig-erant massflow rate while the results of the direct mea-surements were used for checking purposes.The heat rejection rate in the evaporative condenser for the refrigerant side can be evaluated byQcond¼_m rðh cond;iÀh cond;eÞð9ÞAssuming that the evaporative condenser is perfectly insulated and applying the principle of energy conserva-tion to the condenser,in steady state operation the heat rejected by the refrigerant can be related to the heat absorbed by the air stream as follows:_m rðh cond;iÀh cond;eÞþj W p jffi_m aðh a;eÀh a;iÞð10Þwhere j W p j is the power absorbed by water in the circu-lation pump.As seen in Eq.(10),the heat rejected by the refrigerant is affected by the enthalpy of the air stream entering the condenser.Since it is hard to accurately determine the power absorbed by the water in the pump, the condenser heat rejection rate was determined only from the refrigerant side.However,the air dry and wet bulb temperatures at the inlet of the condenser were measured for developing the ANN because the con-denser heat rejection rate and consequently the perfor-mance of the refrigeration system are considerably influenced by these temperatures.Assuming that the compression process is adiabatic, the compressor power absorbed by the refrigerant can be expressed asW c¼_m rðh comp;eÀh comp;iÞð11ÞThe electric power input to the compressor motor is linked to the compressor power by[22]W el¼W cg mech g motorð12Þwhere g mech is the mechanical efficiency of the compres-sor and g motor is the efficiency of the electric motor, which is a function of the load and speed of the motor.The ratio of the refrigeration capacity to the com-pressor power gives the energetic performance of the system,i.e.,COP¼Qevapcð13ÞIn the experimental study,totally60different steady-state test runs have been conducted to gather data for training the proposed ANN and testing its performance. The inputs varied in the tests were the evaporator load, water and airflow rates,and air dry and wet bulb tem-peratures at the inlet of the condenser.The ranges of these inputs are shown in Table1.Based on the experi-mental results,various performance parameters of the system were determined from the above equations,and used for developing and testing the ANN model.4.Modelling with the ANNIn order to develop the ANN for the experimental refrigeration system,the available data set from the experimental work was divided into training and valida-tion sets.The data set consists of60input–output pairs. While70%of the data set was randomly assigned as the training set,the remaining30%was employed for test-ing/validation of the network.The architecture of the ANN for the refrigeration sys-tem with the names of input and output parameters is schematically illustrated in Fig.2.The inputs to the ANN are the evaporator load(Q evap),air massflow rate (_m a),water massflow rate(_m w)along with air dry bulb Table1Range of the inputs in the experimentsEvaporator load55–486WWater massflow rate 5.5–44.0g sÀ1Air massflow rate45.4–59.3g sÀ1Air dry bulb temperature at the condenser inlet20.5–24.7°CAir wet bulb temperature at the condenser inlet15.3–19.2°CH.M.Ertunc,M.Hosoz/Applied Thermal Engineering26(2006)627–635631(T a,i)and wet bulb(T a,i,w)temperatures at the condenser inlet.The outputs from the ANN are the condenser heat rejection rate(Q cond),refrigerant massflow rate(_m r), compressor power absorbed by the refrigerant(W c), electric power consumed by the compressor motor (W el)and the coefficient of performance(COP).The performance of an ANN is affected by the char-acteristics of the network such as the number of hidden layers and the number of nodes in each hidden layer.By trial and error with different ANN configurations,the network was decided to consist of one hidden layer with four neurons along with input and output layers each withfive neurons.The activation function in the hidden layer was chosen as tangent sigmoid function.Conse-quently,the prediction of thefive output parameters was performed using a three-layer feed forward ANN. Utilizing standard backpropagation algorithm,the most popular algorithm in engineering applications,the input vectors withfive variables and the corresponding target vectors withfive variables from the training set were used for training the network.The training procedure adjusted the weighting coefficients using Levenberg–Marquardt algorithm.The output of the network was compared to the desired output at each presentation, and an error was computed.This error was then back-propagated to the ANN and used for adjusting the weights such that the error decreases with each iteration and the neural model gets closer and closer to producing the desired output.Finally,the training procedure approximates a function between the input and output parameters.Then,the input vectors from the validation data set were presented to the trained network.The responses of the network,i.e.,the predicted output parameters,were compared with the experimental ones for the performance measurement.The computer code used for solving the backpropagation algorithm and measuring the network performance was developed using MATLABÒ7.5.Results and discussionThe predictions of the trained ANN for the perfor-mance parameters of the considered refrigeration system as a function of the experimental values are shown in Figs.3–8.Note that in all graphics the comparisons were made using only values from the test data set, which was not introduced to the network during the training process.In allfigures except the last one,the accuracy of the ANN predictions was evaluated withthe help of a straight line indicating perfect predictionand a ±10%error band.A plot of the predicted versus experimental values for the condenser heat rejection rate is shown in Fig.3.The ANN predictions for this parameter result in a mean rel-ative error (MRE)of 1.90%,a root mean square error (RMSE)of 4.12W and a correlation coefficient of 1.000with the experimental data.These results demon-strate that the ANN excellently predicts the heat rejec-tion rate from the refrigeration system despite wide ranges of operating conditions.A plot of the predicted versus experimental values for the refrigerant mass flow rate is reported in Fig.4.For this parameter,the ANN yields a correlation coefficient of 0.999,a MRE of 2.55%and a RMSE of 0.04g s À1,which are slightly poorer than the previous predictions.It is clear that if a higher number of test runs had beenperformed to provide a larger amount of experimental data for training the ANN,the network predictions would have been better.The ANN predictions versus experimental values for the compressor power absorbed by the refrigerant are indicated in Fig.5.For this parameter,the ANN yields a correlation coefficient of0.998,a MRE of4.18%and a RMSE of2.41W.Although the compressor power in a refrigeration system is a performance parameter of ther-modynamic importance,the user also wants to know the total energy consumed by the system.Therefore,the electric power input to the compressor motor,a major portion of the total energy input to the considered sys-tem,was also predicted by the ANN.As seen in Fig.6,the ANN predictions for the electric power con-sumed by the compressor yield a MRE of2.17%,a RMSE of11.67W and a correlation coefficient of 0.991with the experimental data.The ANN predictions versus experimental values for the COP are shown in Fig.7.Results show that although the ANN yields a MRE of3.03%and a RMSE of0.18,it results in a relatively poor correlation coeffi-cient of0.933.This is probably due to the fact that both the evaporator load and compressor power are required to determine the COP.The performances of the ANN predictions for W el, _m r,Q cond,W c and COP are alternatively presented in Fig.8.It is seen that the tests patterns consist of 18tests,corresponding to30%of totally60tests. Although data from these tests has not been introduced to the ANN before,the ANN remarkably predicts thefive output parameters for the whole range of the experiments.6.ConclusionsIn order to show the applicability of the ANN mod-elling to refrigeration systems,especially to those involv-ing concurrent heat and mass transfer processes,an ANN based on backpropagation algorithm has been developed to predict various output parameters of a refrigeration system with an evaporative condenser.In order to acquire data for training and testing the pro-posed ANN,an experimental system was set up and steady-state test runs were conducted.Based onfive in-put parameters,the trained ANN was used for predict-ing the performance of the refrigeration system in terms of the condenser heat rejection rate,refrigerant mass flow rate,compressor power,electric power input to the compressor motor and the COP.The performance of the ANN predictions was measured using the correla-tion coefficient,mean relative error and root mean square error.The ANN modelling for the refrigeration system usually demonstrated a good statistical perfor-mance with the correlation coefficients in the range of 0.933–1.000and MREs in the range of 1.90–4.18%. Moreover,the RMSE values for the predicted parame-ters were very low compared to the ranges of the experiments.This study reveals that a refrigeration system with an evaporative condenser,probably the hardest one to model using classical techniques,can alternatively be modelled using ANNs within a high degree of accuracy. This not only help application engineers but also manu-facturers of these systems determine their performance easily without requiring exhaustive experiments,thus saving both funds and time.References[1]W.Goodman,The evaporative condenser,Heating Piping and AirConditioning10(1938)165–328.[2]R.O.Parker,R.E.Treybal,The heat,mass transfer characteristicsof evaporative coolers,Chemical Engineering Progress Sympo-sium Series57(1961)138–149.[3]D.Peterson,D.Glasser,R.Ramsden,Predicting the performanceof an evaporative condenser,ASME Transactions—Journal of Heat Transfer110(3)(1988)748–753.[4]D.Y.Goswami,G.D.Mathur,S.M.Kulkarni,Experimentalinvestigation of performance of a residential air conditioning system with an evaporatively cooled condenser,Journal of Solar Energy Engineering115(4)(1993)206–211.[5]H.M.Ettouney,H.T.El-Dessouky,W.Bouhamra,B.Al-Azmi,Performance of evaporative condensers,Heat Transfer Engineer-ing22(2001)41–55.[6]Y.Hwang,R.Radermacher,W.Kopko,An experimentalevaluation of a residential-sized evaporatively cooled con-denser,International Journal of Refrigeration24(2001)238–249.[7]M.Hosoz,A.Kilicarslan,Performance evaluations of refrigera-tion systems with air-cooled,water-cooled and evaporative condensers,International Journal of Energy Research28(2004) 683–696.[8]S.A.Kalogirou,Application of artificial neural-networks forenergy systems,Applied Energy67(2000)17–35.[9]A.Pacheco-Vega,M.Sen,K.T.Yang,R.L.McClain,Neuralnetwork analysis offin-tube refrigerating heat exchanger with limited experimental data,International Journal of Heat and Mass Transfer44(2001)763–770.[10]H.Bechtler,M.W.Browne,P.K.Bansal,V.Kecman,Neuralnetworks—a new approach to model vapour-compression heat pumps,International Journal of Energy Research25(2001)591–599.[11]H.Bechtler,M.W.Browne,P.K.Bansal,V.Kecman,Newapproach to dynamic modelling of vapour-compression liquid chillers:artificial neural networks,Applied Thermal Engineering 21(2001)941–953.[12]M.M.Prieto,E.Montanes,O.Menendez,Power plant condenserperformance forecasting using a non-fully connected ANN, Energy26(2001)65–79.[13]A.Chouai,ugeier,D.Richon,Modelling of thermodynamicproperties using neural networks—application to refrigerants, Fluid Phase Equilibria199(2002)53–62.[14]D.J.Swider,A comparison of empirically based steady-statemodels for vapour-compression liquid chillers,Applied Thermal Engineering23(2003)539–556.[15]A.Sozen, E.Arcaklioglu,M.Ozalp,A new approach tothermodynamic analysis of ejector-absorbtion cycle:artificial634H.M.Ertunc,M.Hosoz/Applied Thermal Engineering26(2006)627–635。