Tailoring Evaluative Arguments to a User’s Preferences
recall指标的英文解释

recall指标的英文解释English: Recall is a performance metric used in information retrieval to measure the effectiveness of a search algorithm or system in retrieving relevant documents from a database. It is calculated as the ratio of the number of relevant documents retrieved by the system to the total number of relevant documents in the database. In other words, recall indicates the ability of a system to retrieve all relevant information for a given query. A higher recall score indicates that the system is successful in retrieving a larger proportion of relevant documents, while a lower recall score suggests that some relevant documents were missed by the system. In information retrieval tasks, a balance between precision and recall is crucial, as a high recall score often comes at the cost of lower precision, and vice versa.中文翻译: 召回率是信息检索中使用的性能指标,用于衡量搜索算法或系统在从数据库中检索相关文档方面的有效性。
persistence analysis stata -回复

persistence analysis stata -回复[persistence analysis stata] is a statistical analysis technique used to examine the long-term stability or persistence of a variable over time. Specifically, it evaluates whether a variable tends to revert to its mean or persists in its current state. In this article, we will discuss the steps involved in conducting a persistence analysis using Stata, a popular statistical software package.Step 1: Data PreparationThe first step in conducting a persistence analysis is to prepare the data in Stata. This involves importing the dataset into the software and ensuring that the variables of interest are appropriately formatted. It is also essential to check for any missing values or outliers that may need to be addressed before proceeding with the analysis.Step 2: Determine the Time HorizonNext, you need to determine the time horizon over which you want to assess persistence. This can be the entire duration of the dataset or a specific period of interest. The choice of time horizon willdepend on the research question and the nature of the variable being analyzed.Step 3: Calculate Lagged VariablesTo examine persistence, it is necessary to create lagged variables. A lagged variable is a version of the original variable but shifted backward in time by a specified number of periods. This allows us to compare the current value of the variable with its past values and assess whether it persists over time.In Stata, you can create lagged variables using the "generate" command and the "L." prefix. For instance, if you want to create a lagged version of a variable called "x" with a lag of one period, you can use the following command: "generate lag_x = L.x." Repeat this process for different lagged versions of the variable according to the desired time horizon.Step 4: Estimation of PersistenceOnce the lagged variables are created, you can estimate persistence using various statistical techniques. One commonlyused method is the autoregressive model (AR), which assumes that the current value of a variable is a function of its past values.In Stata, you can estimate an autoregressive model using the "regress" command. Specify the dependent variable and include the lagged variables as independent variables. For example, if you want to estimate the persistence of variable "y" using two lags, the command would be: "regress y lag_y lag2_y."Step 5: Interpretation of ResultsAfter estimating the autoregressive model, you need to interpret the results to assess the persistence of the variable. Look for the coefficient estimates of the lagged variables. If the coefficients are statistically significant and positive, it indicates positive persistence, meaning the variable tends to stay in its current state over time. Conversely, statistically significant and negative coefficients suggest negative persistence or reversion to the mean.Additionally, you can use other diagnostics, such as R-squared, Durbin-Watson statistic, or Martingale difference statistic, to evaluate the goodness of fit and ensure the model's adequacy.Step 6: Sensitivity AnalysisTo enhance the robustness of the persistence analysis, it is essential to conduct sensitivity analyses. These involve testing the stability of the results by varying model specifications or including additional control variables. Sensitivity analysis helps to ascertain the robustness of the findings and to rule out alternative explanations.Step 7: Reporting and ConclusionLastly, when reporting the results, it is important to clearly present the findings of the persistence analysis. Include the relevant coefficient estimates, associated standard errors, and p-values to indicate the statistical significance of the results. Interpret the coefficients in the context of your research question and draw conclusions based on the evidence of persistence or lack thereof.In conclusion, persistence analysis using Stata is a valuable tool to understand the long-term stability or reversion patterns of a variable over time. By following the step-by-step process outlinedin this article, you can conduct a rigorous and informative persistence analysis that contributes to the empirical understanding of your research topic.。
ridge regression方法
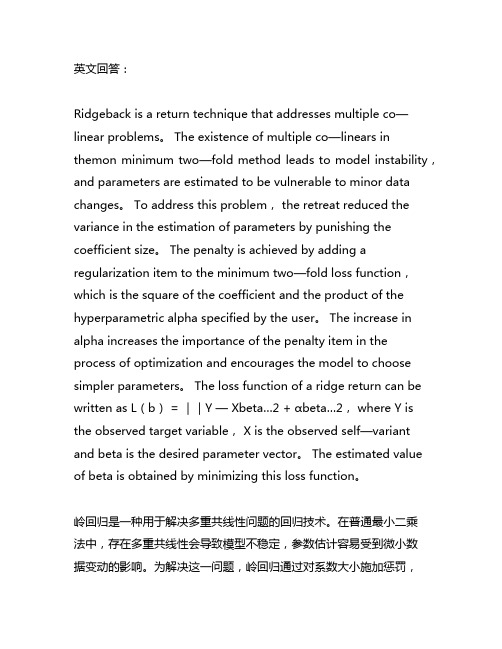
英文回答:Ridgeback is a return technique that addresses multiple co—linear problems。
The existence of multiple co—linears in themon minimum two—fold method leads to model instability,and parameters are estimated to be vulnerable to minor data changes。
To address this problem, the retreat reduced the variance in the estimation of parameters by punishing the coefficient size。
The penalty is achieved by adding a regularization item to the minimum two—fold loss function,which is the square of the coefficient and the product of the hyperparametric alpha specified by the user。
The increase in alpha increases the importance of the penalty item in the process of optimization and encourages the model to choose simpler parameters。
The loss function of a ridge return can be written as L(b) = ||Y —Xbeta…2 + αbeta…2, where Y is the observed target variable, X is the observed self—variant and beta is the desired parameter vector。
ChatGPT技术的错误修正与监督训练策略
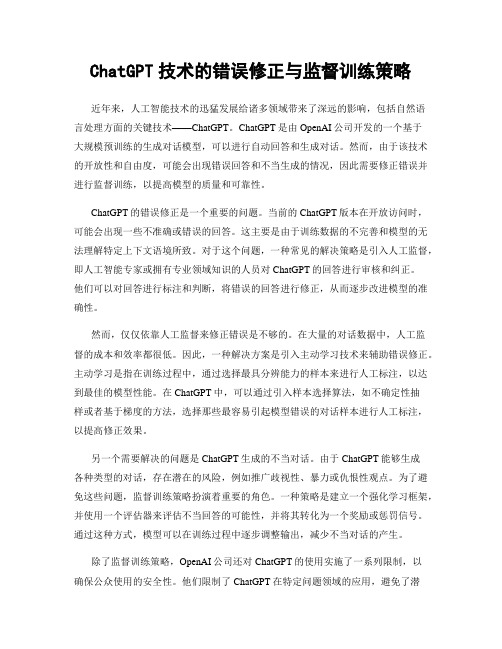
ChatGPT技术的错误修正与监督训练策略近年来,人工智能技术的迅猛发展给诸多领域带来了深远的影响,包括自然语言处理方面的关键技术——ChatGPT。
ChatGPT是由OpenAI公司开发的一个基于大规模预训练的生成对话模型,可以进行自动回答和生成对话。
然而,由于该技术的开放性和自由度,可能会出现错误回答和不当生成的情况,因此需要修正错误并进行监督训练,以提高模型的质量和可靠性。
ChatGPT的错误修正是一个重要的问题。
当前的ChatGPT版本在开放访问时,可能会出现一些不准确或错误的回答。
这主要是由于训练数据的不完善和模型的无法理解特定上下文语境所致。
对于这个问题,一种常见的解决策略是引入人工监督,即人工智能专家或拥有专业领域知识的人员对ChatGPT的回答进行审核和纠正。
他们可以对回答进行标注和判断,将错误的回答进行修正,从而逐步改进模型的准确性。
然而,仅仅依靠人工监督来修正错误是不够的。
在大量的对话数据中,人工监督的成本和效率都很低。
因此,一种解决方案是引入主动学习技术来辅助错误修正。
主动学习是指在训练过程中,通过选择最具分辨能力的样本来进行人工标注,以达到最佳的模型性能。
在ChatGPT中,可以通过引入样本选择算法,如不确定性抽样或者基于梯度的方法,选择那些最容易引起模型错误的对话样本进行人工标注,以提高修正效果。
另一个需要解决的问题是ChatGPT生成的不当对话。
由于ChatGPT能够生成各种类型的对话,存在潜在的风险,例如推广歧视性、暴力或仇恨性观点。
为了避免这些问题,监督训练策略扮演着重要的角色。
一种策略是建立一个强化学习框架,并使用一个评估器来评估不当回答的可能性,并将其转化为一个奖励或惩罚信号。
通过这种方式,模型可以在训练过程中逐步调整输出,减少不当对话的产生。
除了监督训练策略,OpenAI公司还对ChatGPT的使用实施了一系列限制,以确保公众使用的安全性。
他们限制了ChatGPT在特定问题领域的应用,避免了潜在的误导和滥用。
elasticsearch 英文纠错
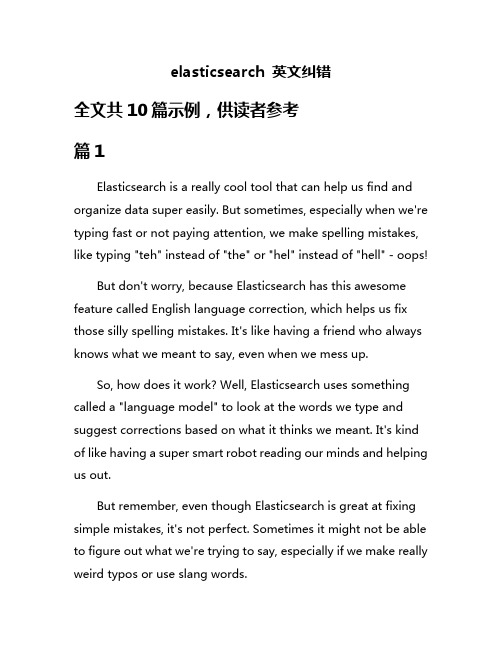
elasticsearch 英文纠错全文共10篇示例,供读者参考篇1Elasticsearch is a really cool tool that can help us find and organize data super easily. But sometimes, especially when we're typing fast or not paying attention, we make spelling mistakes, like typing "teh" instead of "the" or "hel" instead of "hell" - oops!But don't worry, because Elasticsearch has this awesome feature called English language correction, which helps us fix those silly spelling mistakes. It's like having a friend who always knows what we meant to say, even when we mess up.So, how does it work? Well, Elasticsearch uses something called a "language model" to look at the words we type and suggest corrections based on what it thinks we meant. It's kind of like having a super smart robot reading our minds and helping us out.But remember, even though Elasticsearch is great at fixing simple mistakes, it's not perfect. Sometimes it might not be able to figure out what we're trying to say, especially if we make really weird typos or use slang words.So, the next time you're typing in Elasticsearch and you notice a spelling mistake, don't worry! Just let the English language correction feature do its thing and help you out. It's like having a little spelling superhero on your side, ready to save the day.篇2OK! Here's a fun and casual article about Elasticsearch English spell checking:Hey there! Have you ever heard of Elasticsearch? It's this really cool tool that helps you search for stuff on the internet super fast. But sometimes when you type things into Elasticsearch, you might make a little mistake and spell a word wrong. That's where English spell checking comes in!English spell checking is like having a little buddy who watches out for you and makes sure you're spelling everything right. So when you're using Elasticsearch and you type in a word like "cat" but you accidentally spell it "act", the spell checker will be like, "Hey, did you mean 'cat' instead of 'act'?" And you'll be like, "Oh yeah, thanks spell checker!"It's really helpful because it saves you time and keeps your searches accurate. Imagine if you were looking for informationabout elephants, but you spelled it wrong and typed in "elepfnts" instead. The spell checker would swoop in and be like, "Hey, you mean 'elephants'?" And you'd be like, "Oh yeah, thanks for catching that!"So next time you're using Elasticsearch and you're not sure about your spelling, don't worry! The English spell checking feature has got your back. Just keep on typing and searching, and let the spell checker do its magic. Happy searching!篇3Hey everyone! Today I want to talk to you about Elasticsearch and how it helps us fix our spelling mistakes in English. Have you ever been typing a message or writing an essay and realized you spelled a word wrong? Well, with Elasticsearch, we can easily fix those mistakes.Elasticsearch is like a super smart computer program that helps us search for information quickly and accurately. It can also help us find and correct spelling errors in our writing. How cool is that?So, let's say you're writing a story about a magical unicorn. But when you type the word "unicorn," you accidentally type "uniocrn" instead. Oops! Don't worry, Elasticsearch can help. Itwill look at the words you typed and suggest the correct spelling for you.Not only that, but Elasticsearch can also suggest other words that are similar to the one you typed. So, if you're searching for information on pandas but accidentally type "pandals," Elasticsearch will show you results for pandas instead.Elasticsearch is super helpful for anyone who wants to make sure their writing is accurate and easy to understand. It's like having a friendly robot by your side, ready to help you whenever you make a mistake.I hope you learned something new about Elasticsearch today. Remember, it's always important to double-check your spelling and grammar, but it's nice to know that tools like Elasticsearch are there to help us out. Thanks for listening!篇4Once upon a time, there was a magical tool called Elasticsearch. It was like a super smart dictionary that helped people quickly find things they were looking for on the internet. But sometimes, Elasticsearch made mistakes with its spelling and grammar. So, people started to use a special feature called English correction to help fix these errors.English correction was like having a friendly teacher who could help Elasticsearch learn the right way to spell words and form sentences. Whenever Elasticsearch made a mistake, people would gently point it out and show the correct way to do it. This helped Elasticsearch get better and better at understanding English.One day, a little girl named Lily was searching for information about her favorite animals on the internet. She noticed that some of the search results had typos and grammatical errors. Lily decided to use the English corrections feature to help improve the search results. She patiently taught Elasticsearch the correct way to spell words like "elephant" and "giraffe," and how to use punctuation marks properly.Thanks to Lily's help, Elasticsearch became a pro at English and started providing accurate and error-free search results. People all over the world were amazed at how well Elasticsearch could now understand and communicate in English. And Lily felt proud knowing that she had helped make the internet a better place for everyone.And so, with the power of English correction, Elasticsearch and Lily lived happily ever after, making the world a more connected and error-free place for all.篇5Elasticsearch is super cool because it helps you find stuff quickly on the internet. It's like a giant search engine that can look through tons of information really fast and give you back the right answers. But sometimes, it can make mistakes with its spelling and grammar. That's where English correction comes in!English correction is like having a teacher look over your homework and fix all the mistakes for you. So when Elasticsearch messes up and shows you the wrong results, English correction can come to the rescue and make everything right again.Imagine you're searching for information about pandas, but Elasticsearch shows you results about pans instead. That's where English correction can step in and fix the spelling mistake so you can find what you're looking for.So, next time you're using Elasticsearch and something seems off, remember that English correction is there to help you out. Don't worry, with a little bit of fixing, you'll be back on track and finding the information you need in no time!篇6My best friend Bob is a super smart computer whiz, and he taught me all about this super cool thing called Elasticsearch. It's like a really smart search engine that can find stuff on the internet super fast. But sometimes, it doesn't understand my typos and misspelled words. So Bob showed me how to use the English spell check feature to fix it.First, Bob told me that Elasticsearch is a powerful tool that helps websites organize and search through tons of data. It can handle big data like a pro! But sometimes, when we type in a word wrong, Elasticsearch gets confused and doesn't find what we're looking for. That's where the spell check feature comes in.Bob showed me that we can use the spell check feature to correct our typos and misspelled words. All we have to do is add a little code to our search query, and Elasticsearch will automatically suggest the right word. It's like having a super smart friend who always knows the right answer!Now, whenever I type something wrong into Elasticsearch, I make sure to use the spell check feature to fix it. Bob says that's the best way to make sure we're getting the most accurate results. Thanks to Elasticsearch and the spell check feature, I can search the internet like a pro!篇7Hey guys! Today I'm gonna talk about Elasticsearch, which is a really cool tool for searching and analyzing data. But sometimes we make mistakes when typing in the search box, right? That's where Elasticsearch's English spell correction feature comes in handy!So, what is English spell correction in Elasticsearch? Basically, it helps to fix any spelling mistakes you might have made when searching for something. For example, if you type "aple" instead of "apple," Elasticsearch will still be able to find the results you're looking for. How cool is that?Elasticsearch uses something called fuzzy matching to figure out what you meant to type, even if you made a mistake. It looks at the letters you typed and compares them to similar words in its index. Then it suggests the correct spelling so you can get the results you need.But how does Elasticsearch know which words are similar? It uses a special algorithm called Levenshtein distance, which calculates the number of steps it takes to transform one word into another. This helps Elasticsearch figure out which words are close in spelling and suggest the right one.So next time you make a spelling mistake while searching in Elasticsearch, don't worry! The spell correction feature has got your back. Just keep on typing and Elasticsearch will make sure you find what you're looking for. Cool, right?In conclusion, Elasticsearch's English spell correction feature is super helpful for fixing mistakes and finding the right results. It's like having a smart little helper to make sure you never miss out on anything. So keep on searching and let Elasticsearch do the rest!篇8Hey guys, do you know what Elasticsearch is? It's like super cool search engine that helps us find stuff on the internet. But sometimes, we might make some mistakes when we type in our search words. That's where Elasticsearch English correction comes in!Elasticsearch English correction is a feature that helps us fix our spelling mistakes and typos when we search for things online. So, if we type in "puppy" but accidentally spell it as "pupy", Elasticsearch will be like, "Hmm, I think you meant 'puppy'!" How awesome is that?This feature is super helpful because it saves us time and makes sure we find exactly what we're looking for. Plus, it's really easy to use – we just type in our search words and Elasticsearch takes care of the rest. It's like having a super smart friend who always knows what we mean!So next time you're searching for something online, don't worry if you make a mistake – Elasticsearch has got your back. Happy searching, everyone!篇9Elasticsearch is like a super smart wizard that helps us find things on the internet. But sometimes, it can get confused and spell things wrong. That's where English correction comes in! English correction is like giving Elasticsearch a little nudge in the right direction, helping it fix its mistakes and find the right information for us.One of the most common mistakes Elasticsearch makes is spelling errors. For example, if we're searching for information about pandas, but Elasticsearch spells it as "pands", we might not get the results we're looking for. English correction helps by recognizing the mistake and showing Elasticsearch the correct spelling, so we can find all the cute panda pictures we want.Another common mistake Elasticsearch makes is with grammar. Sometimes, it mixes up words or puts them in the wrong order, which can make it hard for us to understand what it's trying to say. With English correction, we can help Elasticsearch rearrange the words properly and make sure everything makes sense.Overall, English correction is like having a loyal buddy who helps Elasticsearch be the best wizard it can be. By fixing spelling errors and grammar mistakes, we can make sure Elasticsearch always gives us the right information and helps us find whatwe're looking for on the internet. So let's give a big cheer for English correction – the superhero sidekick of Elasticsearch!篇10Once upon a time, there was a super cool tool called Elasticsearch. It's like this amazing wizard that helps people search for things really fast on the computer. But sometimes, it gets a little mixed up with its spelling and grammar. Don't worry though, because there's something called English correction that can help fix those mistakes.So, let's say you're searching for "unicorns" in Elasticsearch, but you accidentally type "unicrns" instead. Uh-oh, Elasticsearchmight not understand what you're looking for! But with English correction, it can figure out that you meant "unicorns" and show you all the magical unicorn results you were hoping for.English correction is like having a friendly robot friend who's really good at spelling and grammar. It helps Elasticsearch understand what you're trying to say, even if you make a little mistake. It's kind of like having a safety net for your searches, making sure you always get the right results.So, next time you're using Elasticsearch and you notice a little typo or error in your search terms, don't fret! Just remember that English correction is there to save the day and make sure you find what you're looking for. Elasticsearch and English correction make a great team, helping you search smarter and faster than ever before. Yay for Elasticsearch and English correction!。
精品解析:2024年新课标全国Ⅰ卷英语高考真题解析(参考版)-A4答案卷尾
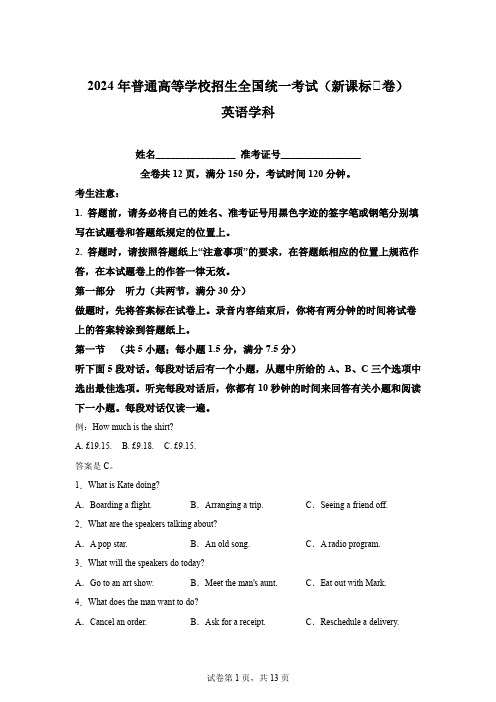
B.To sell home-grown vegetables.
C.To motivate her fellow gardeners.
15.Why does Marie recommend beginners to grow strawberries?
Help restore and protect Marin's natural areas from the Marin Headlands to Bolinas Ridge. We'll explore beautiful park sites while conducting invasive (侵入的) plant removal, winter planting, and seed collection. Habitat Restoration Team volunteers play a vital role in restoring sensitive resources and protecting endangered species across the ridges and valleys.GROUPS
A.To discover mineral resources.B.To develop new wildlife parks.
C.To protect the local ecosystem.D.To conduct biological research.
22.What is the lower age limit for joining the Habitat Restoration Team?
A.A pop star.B.An old song.C.A radio program.
ChatGPT技术中的错误纠正与模糊查询处理方法
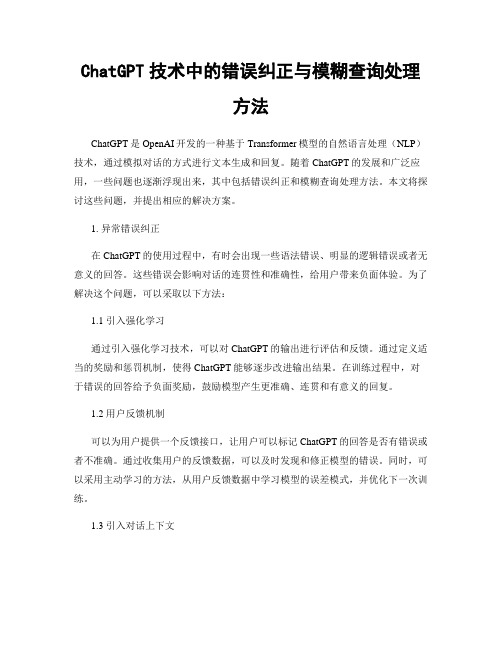
ChatGPT技术中的错误纠正与模糊查询处理方法ChatGPT是OpenAI开发的一种基于Transformer模型的自然语言处理(NLP)技术,通过模拟对话的方式进行文本生成和回复。
随着ChatGPT的发展和广泛应用,一些问题也逐渐浮现出来,其中包括错误纠正和模糊查询处理方法。
本文将探讨这些问题,并提出相应的解决方案。
1. 异常错误纠正在ChatGPT的使用过程中,有时会出现一些语法错误、明显的逻辑错误或者无意义的回答。
这些错误会影响对话的连贯性和准确性,给用户带来负面体验。
为了解决这个问题,可以采取以下方法:1.1 引入强化学习通过引入强化学习技术,可以对ChatGPT的输出进行评估和反馈。
通过定义适当的奖励和惩罚机制,使得ChatGPT能够逐步改进输出结果。
在训练过程中,对于错误的回答给予负面奖励,鼓励模型产生更准确、连贯和有意义的回复。
1.2 用户反馈机制可以为用户提供一个反馈接口,让用户可以标记ChatGPT的回答是否有错误或者不准确。
通过收集用户的反馈数据,可以及时发现和修正模型的错误。
同时,可以采用主动学习的方法,从用户反馈数据中学习模型的误差模式,并优化下一次训练。
1.3 引入对话上下文对于一些误解或者错误的回答,可以通过引入对话上下文进行纠正。
ChatGPT 可以通过对上下文的理解和分析,更好地把握对话的语境和目的,提供更准确的回答。
2. 模糊查询处理在ChatGPT中,一些用户可能会提出模糊的问题或者查询。
这些问题可能不够明确,导致ChatGPT无法给出准确的回答。
为了处理这个问题,可以采取以下方法:2.1 询问澄清问题当ChatGPT无法理解或者解答模糊的查询时,可以向用户询问澄清问题。
通过进一步的问询,可以帮助ChatGPT更好地理解用户的意图,准确回答。
2.2 引入对话历史利用对话历史可以更好地处理模糊查询。
ChatGPT可以通过追溯之前的对话内容,了解用户的背景信息和上下文,从而更好地理解用户的意图和查询。
英语 算法 -回复
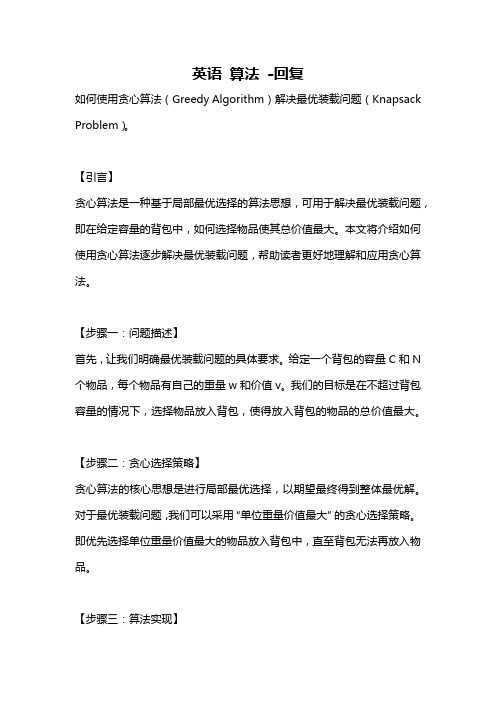
英语算法-回复如何使用贪心算法(Greedy Algorithm)解决最优装载问题(Knapsack Problem)。
【引言】贪心算法是一种基于局部最优选择的算法思想,可用于解决最优装载问题,即在给定容量的背包中,如何选择物品使其总价值最大。
本文将介绍如何使用贪心算法逐步解决最优装载问题,帮助读者更好地理解和应用贪心算法。
【步骤一:问题描述】首先,让我们明确最优装载问题的具体要求。
给定一个背包的容量C和N 个物品,每个物品有自己的重量w和价值v。
我们的目标是在不超过背包容量的情况下,选择物品放入背包,使得放入背包的物品的总价值最大。
【步骤二:贪心选择策略】贪心算法的核心思想是进行局部最优选择,以期望最终得到整体最优解。
对于最优装载问题,我们可以采用“单位重量价值最大”的贪心选择策略。
即优先选择单位重量价值最大的物品放入背包中,直至背包无法再放入物品。
【步骤三:算法实现】基于贪心选择策略,我们可以使用如下步骤实现算法:1. 根据物品的重量w和价值v,计算每个物品的单位重量价值vu = v / w。
2. 按照单位重量价值vu从大到小对物品进行排序。
3. 初始化当前背包的总价值val = 0和当前背包的剩余容量rc = C。
4. 逐个遍历排序后的物品列表:a. 如果当前物品的重量小于等于当前背包的剩余容量,则将该物品放入背包中,更新当前背包的总价值val和剩余容量rc。
b. 如果当前物品的重量大于当前背包的剩余容量,则放弃该物品,继续遍历下一个物品。
5. 返回最终的背包总价值val作为最优装载问题的解。
【步骤四:算法示例】接下来,我们通过一个简单的例子演示如何使用贪心算法解决最优装载问题。
假设背包容量C为10,有以下4个物品可供选择:物品1:重量w1 = 2,价值v1 = 5物品2:重量w2 = 3,价值v2 = 8物品3:重量w3 = 4,价值v3 = 9物品4:重量w4 = 5,价值v4 = 10按照贪心选择策略,首先计算每个物品的单位重量价值vu:物品1:vu1 = v1 / w1 = 5 / 2 = 2.5物品2:vu2 = v2 / w2 = 8 / 3 ≈2.67物品3:vu3 = v3 / w3 = 9 / 4 = 2.25物品4:vu4 = v4 / w4 = 10 / 5 = 2.0然后,按照单位重量价值vu从大到小对物品进行排序:物品2 > 物品1 > 物品3 > 物品4接下来,我们按照步骤三中的算法实现进行装载:初始化当前背包的总价值val = 0和剩余容量rc = 10。
关于询问问题的英语作文
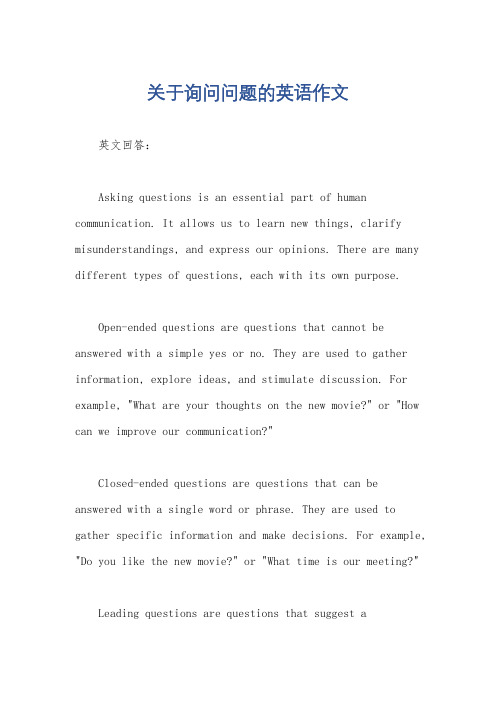
关于询问问题的英语作文英文回答:Asking questions is an essential part of human communication. It allows us to learn new things, clarify misunderstandings, and express our opinions. There are many different types of questions, each with its own purpose.Open-ended questions are questions that cannot be answered with a simple yes or no. They are used to gather information, explore ideas, and stimulate discussion. For example, "What are your thoughts on the new movie?" or "How can we improve our communication?"Closed-ended questions are questions that can be answered with a single word or phrase. They are used to gather specific information and make decisions. For example, "Do you like the new movie?" or "What time is our meeting?"Leading questions are questions that suggest aparticular answer. They are often used to persuade someone to agree with a certain point of view. For example, "Don't you think the new movie is great?" or "Isn't it time we took action?"Neutral questions are questions that do not suggest a particular answer. They are used to gather information without bias. For example, "What are the pros and cons of the new movie?" or "What are the different perspectives on this issue?"When asking questions, it is important to be clear and concise. You should also be respectful of the otherperson's time and feelings. Avoid asking questions that are too personal or that could make the other person feel uncomfortable.Here are some tips for asking effective questions:Start with open-ended questions. This will allow you to gather more information and get a better understanding of the other person's perspective.Be specific. Don't ask vague questions that could be interpreted in multiple ways.Avoid leading questions. These can make the other person feel like you are trying to manipulate them.Be respectful. Ask questions in a polite and non-confrontational manner.Listen to the answers. Once you have asked a question, take the time to listen to the answer and ask follow-up questions if necessary.Asking questions is a valuable skill that can help you in all aspects of your life. By using the right questions, you can learn new things, build relationships, and make informed decisions.中文回答:如何有效提问。
ptuningv2问答语料
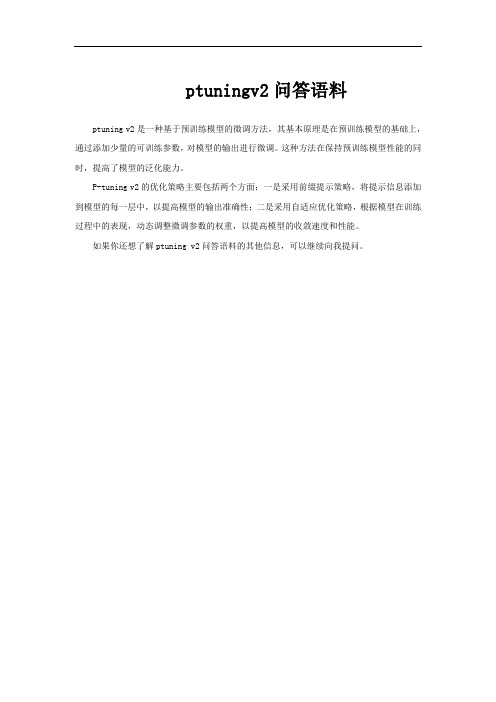
ptuningv2问答语料
ptuning v2是一种基于预训练模型的微调方法,其基本原理是在预训练模型的基础上,通过添加少量的可训练参数,对模型的输出进行微调。
这种方法在保持预训练模型性能的同时,提高了模型的泛化能力。
P-tuning v2的优化策略主要包括两个方面:一是采用前缀提示策略,将提示信息添加到模型的每一层中,以提高模型的输出准确性;二是采用自适应优化策略,根据模型在训练过程中的表现,动态调整微调参数的权重,以提高模型的收敛速度和性能。
如果你还想了解ptuning v2问答语料的其他信息,可以继续向我提问。
【高考真题】2024年高考英语真题试卷(新高考Ⅰ卷)
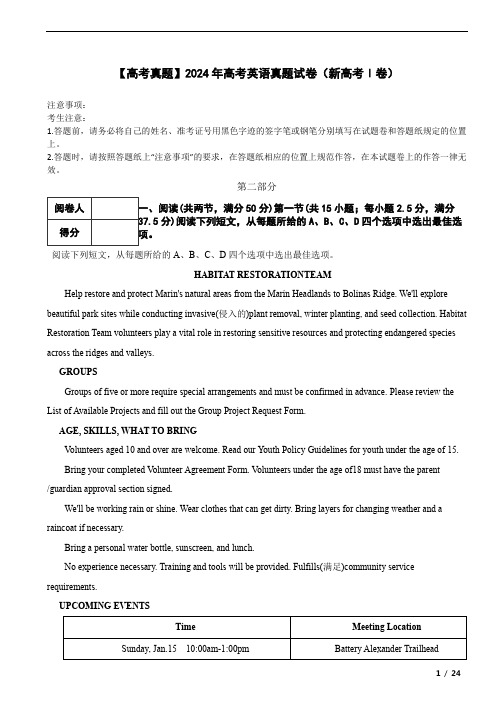
【高考真题】2024年高考英语真题试卷(新高考Ⅰ卷)注意事项:考生注意:1.答题前,请务必将自己的姓名、准考证号用黑色字迹的签字笔或钢笔分别填写在试题卷和答题纸规定的位置上。
2.答题时,请按照答题纸上“注意事项”的要求,在答题纸相应的位置上规范作答,在本试题卷上的作答一律无效。
第二部分(共两节,满分50分)第一节(共15小题;每小题2.5分,满分分)阅读下列短文,从每题所给的A、B、C、D四个选项中选出最佳选阅读下列短文,从每题所给的A、B、C、D四个选项中选出最佳选项。
HABITAT RESTORATIONTEAMHelp restore and protect Marin's natural areas from the Marin Headlands to Bolinas Ridge. We'll explore beautiful park sites while conducting invasive(侵入的)plant removal, winter planting, and seed collection. Habitat Restoration Team volunteers play a vital role in restoring sensitive resources and protecting endangered species across the ridges and valleys.GROUPSGroups of five or more require special arrangements and must be confirmed in advance. Please review the List of Available Projects and fill out the Group Project Request Form.AGE, SKILLS, WHAT TO BRINGV olunteers aged 10 and over are welcome. Read our Youth Policy Guidelines for youth under the age of 15.Bring your completed V olunteer Agreement Form. V olunteers under the age of18 must have the parent/guardian approval section signed.We'll be working rain or shine. Wear clothes that can get dirty. Bring layers for changing weather and a raincoat if necessary.Bring a personal water bottle, sunscreen, and lunch.No experience necessary. Training and tools will be provided. Fulfills(满足)community service requirements.UPCOMING EVENTS1.What is the aim of the Habitat Restoration Team?A.To discover mineral resources.B.To develop new wildlife parks.C.To protect the local ecosystemD.To conduct biological research.2.What is the lower age limit for joining the Habitat Restoration Team?A.5.B.10.C.15.D.18.3.What are the volunteers expected to do?A.Bring their own tools.B.Work even in bad weather.C.Wear a team uniform D.Do at least three projects.阅读下列短文,从每题所给的A、B、C、D四个选项中选出最佳选项。
英语语法纠错的开源算法
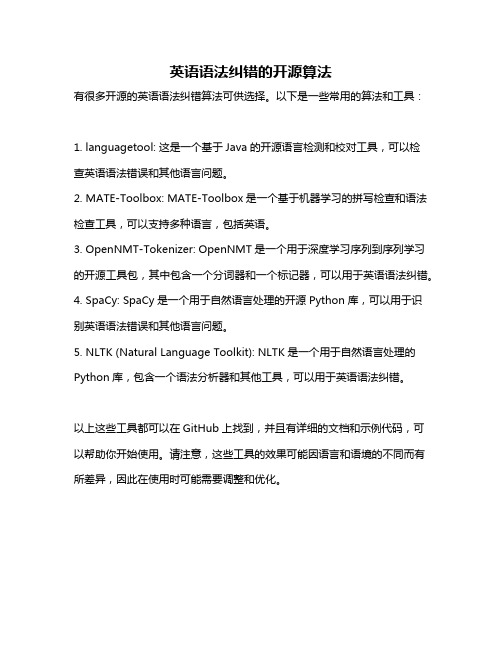
英语语法纠错的开源算法
有很多开源的英语语法纠错算法可供选择。
以下是一些常用的算法和工具:
1. languagetool: 这是一个基于Java的开源语言检测和校对工具,可以检
查英语语法错误和其他语言问题。
2. MATE-Toolbox: MATE-Toolbox是一个基于机器学习的拼写检查和语法检查工具,可以支持多种语言,包括英语。
3. OpenNMT-Tokenizer: OpenNMT是一个用于深度学习序列到序列学习的开源工具包,其中包含一个分词器和一个标记器,可以用于英语语法纠错。
4. SpaCy: SpaCy是一个用于自然语言处理的开源Python库,可以用于识
别英语语法错误和其他语言问题。
5. NLTK (Natural Language Toolkit): NLTK是一个用于自然语言处理的Python库,包含一个语法分析器和其他工具,可以用于英语语法纠错。
以上这些工具都可以在GitHub上找到,并且有详细的文档和示例代码,可以帮助你开始使用。
请注意,这些工具的效果可能因语言和语境的不同而有所差异,因此在使用时可能需要调整和优化。
Evaluating a Decision-Theoretic Approach to Tailored Example Selection

Evaluating a Decision-Theoretic Approach to Tailored Example SelectionKasia Muldner 1 and Cristina Conati 1,21 University of British Columbia Department of Computer Science Vancouver, B.C., Canada {kmuldner, conati}@cs.ubc.ca2 University of Trento Department of Information and Communication TechnologyPovo, Trento, ItalyAbstractWe present the formal evaluation of a frameworkthat helps students learn from analogical problemsolving, i.e., from problem-solving activities thatinvolve worked-out examples. The framework in-corporates an innovative example-selectionmechanism, which tailors the choice of example toa given student so as to trigger studying behaviorsthat are known to foster learning. This involves atwo-phase process based on 1) a probabilistic usermodel and 2) a decision-theoretic mechanism thatselects the example with the highest overall utilityfor learning and problem-solving success. We de-scribe this example-selection process and presentempirical findings from its evaluation.1IntroductionAlthough examples play a key role in cognitive skill acqui-sition (e.g., [Atkinson et al., 2002]), research demonstrates that students have varying degrees of proficiency for using examples effectively (e.g., [Chi et al., 1989; VanLehn, 1998; VanLehn, 1999]). Thus, there has been substantial interest in the Intelligent Tutoring Systems (ITS) commu-nity in exploring how to devise adaptive support to help all students benefit from example-based activities (e.g., [Conati and VanLehn, 2000; Weber, 1996]). In this paper, we de-scribe the empirical evaluation of the Example Analogy (EA)-Coach, a computational framework that provides adaptive support for a specific type of example-based learn-ing known as analogical problem solving (APS) (i.e., using examples to aid problem solving).The EA-Coach’s general approach for supporting APS consists of providing students with adaptively-selected ex-amples that encourage studying behaviors (i.e. meta-cognitive skills) known to trigger learning, including:1)min-analogy: solving the problem on one’s own asmuch as possible instead of by copying from examples(e.g., [VanLehn, 1998]);2)Explanation-Based Learning of Correctness (EBLC): aform of self-explanation (the process of explaining and clarifying instructional material to oneself [Chi et al., 1989]) that can be used for learning new domain prin-ciples by relying on, for instance, commonsense oroverly-general knowledge, to explain how an example solution step is derived [VanLehn, 1999].Min-analogy and EBLC are beneficial for learning be-cause they allow students to (i) discover and fill their knowledge gaps and (ii) strengthen their knowledge through practise. Unfortunately, some students prefer more shallow processes which hinder learning, such as copying as much as possible from examples without any proactive reasoning on the underlying domain principles (e.g., [VanLehn, 1998; VanLehn, 1999].To find examples that best trigger the effective APS be-haviors for each student, the EA-Coach takes into account: i) student characteristics, including domain knowledge and pre-existing tendencies for min-analogy and EBCL, and ii) the similarity between a problem and candidate example. In particular, the Coach relies on the assumption that certain types of differences between a problem and example may actually be beneficial in helping students learn from APS, because they promote the necessary APS meta-cognitive skills. This is one of the novel aspects of our approach, and in this paper we present an empirical evaluation of the EA-Coach that validates it.A key challenge in our approach is how to balance learn-ing with problem-solving success. Examples that are not highly similar to the target problem may discourage shallow APS behaviors, such as pure copying. However, they may also hinder students from producing a problem solution, because they do not provide enough scaffolding for students who lack the necessary domain knowledge. Our solution to this challenge includes (i) incorporating relevant factors (student characteristics, problem/example similarity) into a probabilistic user model, which the framework uses to pre-dict how a student will solve the problem and learn in the presence of a candidate example; (ii) using a decision-theoretic process to select the example that has the highest overall utility in terms of both learning and problem-solving success. The findings from our evaluation show that this selection mechanism successfully finds examples that re-duce copying and trigger EBLC while still allowing for suc-cessful problem solving.There are a number of ITS that, like the EA-Coach, select examples for APS, but they do not consider the impact of problem/example differences on a student’s knowledge and/or meta-cognitive behaviors. ELM-PE [Weber, 1996]helps students with LISP programming by choosing exam-ples that are as similar as possible to the target problem. AMBRE [Nogry et al ., 2004] supports the solution of alge-bra problems by choosing structurally-appropriate exam-ples. However, it is not clear from the paper what “structur-ally appropriate” means. Like the EA-Coach, several ITS rely on a decision-theoretic approach for action selection, but these do not take into account a student’s meta-cognitive skills, nor they include the use of examples [Murray et al., 2004; Mayo and Mitrovic, 2001]. Finally, some systems perform analogical reasoning in non-pedagogical contexts (e.g., [Veloso and Carbonell, 1993]), and so do not incorpo-rate factors needed to support human learning.In the remainder of the paper, we first describe the exam-ple-selection process. We then present the results from an evaluation of the framework and discuss how they support the EA-Coach’s goal of balancing learning and problem- solving success.ExampleA person pulls a 9kg crate up a rampinclined 30o CCW from the horizontal. The pulling force is applied at an angle of 30 o CCW from the horizontal, with a magnitude of 100N. Find the magnitude of the normal force exerted on the crate.We answer this question using Newton’s Second Law.We choose the crate as the body. A normal force acts on the crate. It’s oriented 120o CCW from the horizontalA workman pulls a 50kg. block along the floor. He pulls it with amagnitude of 120N, applied at an angle of 25 o CCW from thehorizontal. What is the magnitude of the normal force on the block?Figure 2: Sample Classification of Problem/Example Relations2 The EA-Coach Example-Selection ProcessThe EA-Coach includes an interface that allows students to solve problems in the domain of Newtonian physics and ask for an example when needed (Fig. 1). For more details on the interface design see [Conati et al., in press]. As stated in the introduction, the EA-Coach example-selection mecha-nism aims to choose an example that meets two goals: 1) helps a student solve the problem (problem-solving success goal ) and 2) triggers learning by encouraging the effective APS behaviors of min-analogy and EBLC (learning goal ). For each example stored in the EA-Coach knowledge base, this involves a two-phase process, supported by the EA-Coach user model: simulation and utility calculation . The general principles underlying this process were described in [Conati et al., in press]. Here, we summarize the corre-sponding computational mechanisms and provide an illus-trative example because the selection process is the target of the evaluation described in a later section.2.1 Phase 1: The Simulation via the User ModelThe simulation phase corresponds to generating a prediction of how a student will solve a problem given a candidate example, and what she will learn from doing so. To generate this prediction, the framework relies on our classification of various relations between the problem and candidate exam-ple, and their impact on APS behaviors. Since an under-standing of this classification/impact is needed for subse-quent discussion, we begin by describing it.Two corresponding steps in a problem/example pair are defined to be structurally identical if they are generated by the same rule, and structurally different otherwise. For in-stance, Fig. 2 shows corresponding fragments of the solu-tions for the problem/example pair in Fig. 1, which include two structurally-identical pairs of steps: Pstep n /Estep n de-rived from the rule stating that a normal force exists (rule normal, Fig. 2), and Pstep n+1/Estep n+1 derived from the rule stating the normal force direction (rule normal-dir, Fig. 2). Two structurally-identical steps may be superficially dif-ferent. We further classify these differences as trivial or non-trivial. While a formal definition of these terms is given in [Conati et al., in press], for the present discussion, it suf-fices to say that what distinguishes them is the type of trans-fer from example to problem that they allow. Trivial super-ficial differences allow example steps to be copied, because these differences can be resolved by simply substituting the example-specific constants with ones needed for the prob-lem solution. This is possible because the constant corre-sponding to the difference appears in the example/problem solutions and specifications, which provides a guide for its substitution [Anderson, 1993] (as is the case for Pstep n /Estep n , Fig. 2). In contrast, non-trivial differences require more in-depth reasoning such as EBLC to be re-solved. This is because the example constant corresponding to the difference is missing from the problem/example specifications, making it less obvious what it should be re-placed with (as is the case for Pstep n+1/Estep n+1, Fig. 2). The classification of the various differences forms the ba-sis of several key assumptions embedded into the simula-tion’s operation. If two corresponding problem/example steps (Pstep and Estep respectively) are structurally differ-ent, the student cannot rely on the example to derive Pstep , i.e. the transfer of this step is blocked. This hinders problem solving if the student lacks the knowledge to generate Pstep [Novick, 1995]. In contrast, superficial differences betweenstructurally-identical steps do not block transfer of the ex-ample solution, because the two steps are generated by the same rule. Although cognitive science does not provide clear answers regarding how superficial differences impact APS behaviors, we propose that the type of superficial dif-ference has the following impact. Because trivial differences are easily resolved, they encourage copying for students with poor domain knowledge and APS meta-cognitive skills. In contrast, non-trivial differences encourage min-analogy and EBLC because they do not allow the problem solution to be generated by simple constant replacement from the example. We now illustrate how these assumptions are integrated into the EA-Coach simulation process.Simulation via the EA-Coach User ModelTo simulate how the examples in the EA-Coach knowledge base will impact students’ APS behaviors, the framework relies on its user model, which corresponds to a dynamic Bayesian network. This network is automatically created when a student opens a problem and includes as its back-bone nodes and links representing how the various problem solution steps (Rectangular nodes in Fig. 3) can be derived from domain rules (Round nodes in Fig. 3) and other steps. For instance, the simplified fragment of the user model in Fig. 3, slice t (pre-simulation slice) shows how the solution steps Pstep n and Pstep n+1 in Fig. 2 are derived from the cor-responding rules normal and normal-dir. In addition, the network contains nodes to model the student’s APS ten-dency for min-analogy and EBLC (MinAnalogyTend and EBLCTend in slice t, Fig. 3)1.To simulate the impact of a candidate example, a special ‘simulation’ slice is added to the model (slice t+1, Fig. 3, assuming that the candidate example is the one in Fig. 2). This slice contains all the nodes in the pre-simulation slice, as well as additional nodes that are included for each prob-lem-solving action being simulated and account for the can-didate example’s impact on APS. These include:-Similarity, encoding the similarity between a problem so-lution step and the corresponding example step (if any).1 Unless otherwise specified, all nodes have Boolean values -Copy, encoding the probability that the student will gener-ate the problem step by copying the corresponding exam-ple solution step.-EBLC, encoding the probability that the student will infer the corresponding rule from the example via EBLC. During the simulation phase, the only form of direct evi-dence for the user model corresponds to the similarity be-tween the problem and candidate example. This similarity is automatically assessed by the framework via the comparison of its internal representation of the problem and example solutions and their specifications. The similarity node’s value for each problem step is set based on the definitions presented above, to either: None (structural difference), Trivial or Non-trivial. Similarity nodes are instrumental in allowing the framework to generate a fine-grained predic-tion of copying and EBLC reasoning, which in turns im-pacts its prediction of learning and problem-solving success, as we now illustrate.Prediction of Copying episodes. For a given problem solu-tion step, the corresponding copy node encodes the model’s prediction of whether the student will generate this step by copying from the example. To generate this prediction, the model takes into account: 1) the student’s min-analogy ten-dency and 2) whether the similarity between the problem and example allows the step to be generated by copying. The impact of these factors is shown in Fig. 3. The probabil-ity that the student will generate Pstep n by copying is high (see ‘Copy n‘ node in slice t+1), because the prob-lem/example similarity allows for it (‘Similarity n’=Trivial, slice t+1) and the student has a tendency to copy (indicated in slice t by the low probability of the ‘MinAnalogyTend’ node). In contrast, the probability that the student will gen-erate the step Pstep n+1 by copying is very low (see node ‘Copy n+1’ in slice t+1) because the non-trivial difference (‘Similarity n+1’=Non-trivial, slice t+1) between the problem step and corresponding example step blocks copying. Prediction of EBLC episodes. For a given problem rule, the corresponding EBLC node encodes the model’s predic-tion that the student will infer the corresponding rule from the example via EBLC. To generate this prediction, the model takes into account 1) the student’s EBLC tendency, 2) her knowledge of the rule (in that students who already know a rule do not need to learn it) 3) the probability that she will copy the step, and 4) the problem/example similar-ity. The last factor is taken into account by including an EBLC node only if the example solution contains the corre-sponding rule (i.e., the example is structurally identical with respect to this rule). The impact of the first 3 factors is shown in Fig. 3. The model predicts that the student is not likely to reason via EBLC to derive Pstep n (see node ‘EBLC n,’ in slice t+1) because of the high probability that she will copy the step (see node ‘Copy n’) and the moderate probability of her having tendency for EBLC (see node EBLCTend in slice t). In contrast, a low probability of copy-ing (e.g., node ‘Copy n+1’, slice t+1) increases the probability for EBLC reasoning (see node ‘EBLC n+1’ in slice t+1), but the increase is mediated by the probability that the student has a tendency for EBLC, which in this case is moderate.Prediction of Learning & Problem-Solving Success. The model’s prediction of EBLC and copying behaviors influ-ences its prediction of learning and problem-solving suc-cess. Learning is predicted to occur if the probability of a rule being known is low in the pre-simulation slice and the simulation predicts that the student will reason via EBLC to learn the rule (e.g., rule normal-dir , Fig. 3). The probabili-ties corresponding to the Pstep nodes in the simulation slice represent the model’s prediction of whether the student will generate the corresponding problem solution steps. For a given step, this is predicted to occur if either 1) the student can generate the prerequisite steps and derive the given step from a domain rule (e.g. Pstep n+1, Fig. 3) or 2) generate the step by copying from the example (e.g., Pstep n , Fig. 3).Figure 4: Fragment of the EA Utility Model2.2 Phase 2: The Utility CalculationThe outcome of the simulation is used by the framework to assign a utility to a candidate example, quantifying its abil-ity to meet the learning and problem-solving success objec-tives. To calculate this utility, the framework relies on a decision-theoretic approach that uses the probabilities of rule and Pstep nodes in the user model as inputs to the multi-attribute linearly-additive utility model shown in Fig. 4. The expected utility (EU) of an example for learning an individual rule in the problem solution corresponds to the sum of the probability P of each outcome (value) for the corresponding rule node multiplied by the utility U of that outcome:))(())(())(())(()(i i i i i Rule known U Rule known P Rule known U Rule known P Rule EU ¬⋅¬+⋅=Since in our model, U (known (Rule i ))=1 and U (¬known (Rule i ))=0, the expected utility of a rule corresponds to the probability that the rule is known. The overall learning util-ity of an example is the weighted sum of the expected learn-ing utilities for all the rules in the user model:∑⋅nii i w Rule EU )(Given that we consider all the rules to have equal impor-tance, all weights w are assigned an equal value (i.e., 1/n , where n is the number of rules in the user model). A similar approach is used to obtain the problem-solving success util-ity, which in conjunction with the learning utility quantifies a candidate example’s overall utility.The simulation and utility calculation phases are repeated for each example in the EA-Coach’s knowledge base. The example with the highest overall utility is presented to the student.3 Evaluation of the EA-CoachAs we pointed out earlier, one of the challenges for the EA-Coach example-selection mechanism is to choose examples that are different enough to trigger learning by encouraging effective APS behaviors (learning goal ), but at the same time similar enough to help the student generate the problem solution (problem-solving success goal ). To verify how well the two-phase process described in the previous section meets these goals, we ran a study that compared it with the standard approach taken by ITS that support APS, i.e., se-lecting the most similar example. Here, we provide an over-view of the study methodology and present the key results.3.1 Study DesignThe study involved 16 university students. We used a within-subject design, where each participant 1) completed a pencil and paper physics pre-test, 2) was introduced to the EA-Coach interface (training phase), 3) solved two New-ton’s Second Law problems (e.g., of the type in Fig. 1) us-ing the EA-Coach, (experimental phase) and 4) completed a pencil and paper physics post-test. We chose a within-subject design because it increases the experiment’s power by accounting for the variability between subjects, arising from differences in, for instance, expertise, APS tendencies, verbosity (which impacts verbal expression of EBLC).Prior to the experimental phase, each subject’s pre-test data was used to initialize the priors for the rule nodes in the user model’s Bayesian network. Since we did not have in-formation regarding students’ min-analogy and EBLC ten-dencies, the priors for these nodes were set to 0.5. During the experimental phase, for each problem, subjects had ac-cess to one example. For one of the problems, the example was selected by the EA-Coach (adaptive-selection condi-tion ), while for the other (static-selection condition ), an ex-ample most similar to the target problem was provided. To account for carry-over effects, the orders of the prob-lems/selection conditions were counterbalanced. For both conditions, subjects were given 60 minutes to solve the problem, and the EA-Coach provided immediate feedback for correctness on their problem-solving entries, realized by coloring the entries red or green. All actions in the interface were logged. To capture subjects’ reasoning, we used the think-aloud method, by having subjects verbalize their thoughts [Chi et al ., 1989] and videotaped all sessions.3.2 Data AnalysisThe primary analysis used was univariate ANOVA, per-formed separately for the dependent variables of interest (discussed below). For the analysis, the within-subject selec-tion factor (adaptive vs. static ) was considered in combina-tion with the two between-subject factors resulting from the counterbalancing of selection and problem types. The re-sults from the ANOVA analysis are based on the data from the 14 subjects who used an example in both conditions (2 subjects used an example in only one condition: one subject used the example only in the static condition, another sub-ject used the example only in the adaptive condition).3.3Results: Learning GoalTo assess how well the EA-Coach adaptively-selected ex-amples satisfied the learning goal as compared to the stati-cally-selected ones, we followed the approach advocated in [Chi et al., 1989]. This approach involves analyzing stu-dents’ behaviors that are known to impact learning, i.e., copying and self-explanation via EBLC in our case. Al-though this approach makes the analysis challenging be-cause it requires that students’ reasoning is captured and analyzed, it has the advantage of providing in-depth insight into the EA-Coach selection mechanism’s impact. For this part of the analysis, univariate ANOVAs were performed separately for the dependent variables copy and EBLC rates. Copy Rate. To identify copy events, we looked for in-stances when students: 1) accessed a step in the example solution (as identified by the verbal protocols and/or via the analysis of mouse movements over the example) and 2) generated the corresponding step in their problem solution with no changes or minor changes (e.g., order of equation terms, constant substitutions). Students copied significantly less from the adaptively-selected examples, as compared to the statically-selected examples (F(1,14)=7.2, p=0.023; on average, 5.9 vs. 8.1 respectively).EBLC rate. To identify EBLC episodes, we analyzed the verbal protocol data. Since EBLC is a form of self-explanation, to get an indication of how selection impacted explanation rate in general, we relied on the definition in [Chi et al., 1989] to first identify instances of self-explanation. Students expressed significantly more self-explanations while generating the problem solution in the adaptive selection condition, as compared to in the static condition (F(1, 10)=6.4, p=0.03; on average, 4.07 vs. 2.57 respectively). We then identified those self-explanations that were based on EBLC (i.e., involved learning a rule via com-monsense and/or overly-general reasoning, as opposed to explaining a solution step using existing domain knowl-edge). Students generated significantly more EBLC expla-nations in the adaptive than the static condition (F(1, 10)=12.8, p=0.005; on average, 2.92 vs. 1.14 respectively). Pre/Post Test Differences. With the analysis presented above, we evaluated how the EA-Coach selection mecha-nism impacts learning by analyzing how effectively it trig-gers APS behaviors that foster it. Another way to measure learning is via pre/post test differences. In general, students improved significantly from pre to post test (on average, from 21.7 to 29.4; 2-tailed t(15)=6.13, p<0.001). However, because there was overlap between the two problems in terms of domain principles, the within-subject design makes it difficult to attribute learning to a particular selection con-dition. One way this could be accomplished is to 1) isolate rules that only appeared in one selection condition and that a given student did not know (as assessed from pre-test); 2) determine how many of these rules the student showed gains on from pre to post test. Unfortunately, this left us with very sparse data making formal statistical analysis infeasible. However, we found encouraging trends: there was a higher percentage of rules learned given each student’s learning opportunities in the adaptive condition, as compared to the static one (on average, 77% vs. 52% respectively). Discussion. As far as the learning goal is concerned, the evaluation showed that the EA-Coach’s adaptively-selected examples encouraged students to engage in the effective APS behaviors (min-analogy, EBLC) better than statically-selected examples: students copied less and self-explained more when given adaptively-selected examples. This sup-ports our assumption that certain superficial differences encourage effective APS behaviors. The statically-selected examples were highly similar to the target problem and thus made it possible to correctly copy much of their solutions, which students took advantage of. Conversely, by blocking the option to correctly copy most of their solution, the adap-tively-selected examples provided an incentive for students to infer via EBLC the principles needed to generate the problem solution.3.4Results: Problem-Solving Success GoalThe problem-solving success goal is fulfilled if students generate the problem solution. To evaluate if the adaptive example-selection process met this goal, we checked how successful students were in terms of generating a solution to each problem. In the static condition, all 16 students gener-ated a correct problem solution, while in the adaptive condi-tion, 14 students did so (the other 2 students generated a partial solution; both used the example in both conditions). This difference between conditions, however, is not statisti-cally significant (sign test, p=0.5), indicating that overall, both statically and adaptively selected examples helped stu-dents generate the problem solution.We also performed univariate ANOVAs on the dependent variables error rate and task time to analyze how the adap-tively-selected examples affected the problem solving proc-ess, in addition to the problem solving result. Students took significantly longer to generate the problem solution in the adaptive than in the static selection condition (F(1, 10) =31.6, p<0.001; on average, 42min., 23sec. vs. 25min., 35sec. respectively). Similarly, students made significantly more errors while generating the problem solution in the adaptive than in the static selection condition (F(1, 10)=11.5, p=0.007; on average, 22.35 vs. 7.57 respectively). Discussion. As stated above,the problem-solving success goal is satisfied if the student generates the problem solu-tion, and is not a function of performance (time, error rates) while doing so. The fact that students took longer/made more errors in the adaptive condition is not a negative find-ing from a pedagogical standpoint, because these are by-products of learning. Specifically, learning takes time and may require multiple attempts before the relevant pieces of knowledge are inferred/correctly applied, as we saw in our study and as is backed up by cognitive science findings (e.g., [Chi, 2000]).However, as we pointed out above, 2 students generated a correct but incomplete solution in the adaptive selection condition. To understand why this happened, we analyzed these students’ interaction with the system in detail. Both of them received an example with non-trivial superficial dif-。
sublime 英文语法检查

sublime 英文语法检查English Answer.Sublime Text's English Grammar Checking Capabilities.Sublime Text is a popular text editor among programmers and writers alike due to its versatility and customization options. It offers a range of features to assist with writing, including spell checking, syntax highlighting, and code completion. While Sublime Text does not natively provide comprehensive grammar checking functionality, there are several plugins available that can add this capability to the editor.Plugins for Grammar Checking.Several plugins are available for Sublime Text that can provide grammar checking functionality. Some of the most popular options include:Grammarly: Grammarly is a widely used grammar checking tool that offers a range of features, including grammar and spelling checking, style suggestions, and plagiarism detection.LanguageTool: LanguageTool is an open-source grammar checker that supports over 30 languages and provides detailed explanations for grammar errors.Prose Lint: Prose Lint is a lightweight grammar checker that focuses on identifying common writing errors, such as repeated words, punctuation issues, and stylistic inconsistencies.These plugins typically integrate with Sublime Text's editor interface, providing real-time feedback on grammar and spelling errors as you type. They can be configured to highlight errors in different colors or styles, and some plugins offer additional features such as custom dictionaries and automated corrections.Using Plugins.To use a grammar checking plugin in Sublime Text, you first need to install it via Package Control, SublimeText's built-in package manager. Once installed, you can enable the plugin in the editor's preferences and configure any desired settings.To activate the grammar checking feature, you can typically press a keyboard shortcut or click on a toolbar button. The plugin will then scan your document and highlight any detected grammar or spelling errors. You can then review the errors and make the necessary corrections.Limitations.While grammar checking plugins can be useful for identifying errors and improving the overall quality of your writing, it's important to note that they are not perfect. They may miss certain types of errors or provide incorrect suggestions in some cases. Additionally, these plugins typically do not offer advanced grammar checking features found in dedicated grammar checking software, suchas sentence structure analysis and style recommendations.Additional Tips.In addition to using grammar checking plugins, there are several other tips you can follow to improve your writing in Sublime Text:Use the spell checker: Sublime Text's built-in spell checker can help you identify and correct spelling errors.Use code completion: Code completion can help youwrite code more efficiently and reduce the likelihood of syntax errors.Use a style guide: A style guide can help you enforce consistent formatting and writing conventions in your code.Get feedback from others: Ask a colleague or friend to review your writing and provide feedback on grammar, style, and clarity.中文回答。
arguement就应该这样写
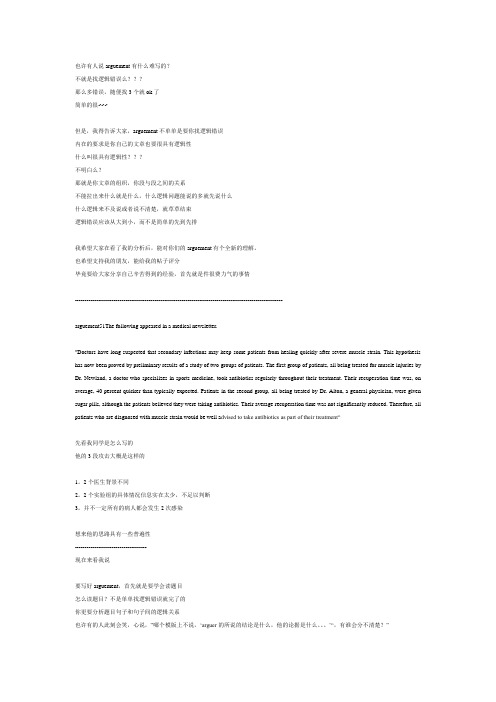
也许有人说arguement有什么难写的?不就是找逻辑错误么???那么多错误,随便找3个就ok了简单的很~~~但是,我得告诉大家,arguement不单单是要你找逻辑错误内在的要求是你自己的文章也要很具有逻辑性什么叫很具有逻辑性???不明白么?那就是你文章的组织,你段与段之间的关系不能拉出来什么就是什么,什么逻辑问题能说的多就先说什么什么逻辑来不及说或者说不清楚,就草草结束逻辑错误应该从大到小,而不是简单的先到先排我希望大家在看了我的分析后,能对你们的arguement有个全新的理解。
也希望支持我的朋友,能给我的帖子评分毕竟要给大家分享自己辛苦得到的经验,首先就是件很费力气的事情-----------------------------------------------------------------------------------------------------------arguement51The following appeared in a medical newsletter."Doctors have long suspected that secondary infections may keep some patients from healing quickly after severe muscle strain. This hypothesis has now been proved by preliminary results of a study of two groups of patients. The first group of patients, all being treated for muscle injuries by Dr. Newland, a doctor who specializes in sports medicine, took antibiotics regularly throughout their treatment. Their recuperation time was, on average, 40 percent quicker than typically expected. Patients in the second group, all being treated by Dr. Alton, a general physician, were given sugar pills, although the patients believed they were taking antibiotics. Their average recuperation time was not significantly reduced. Therefore, all patients who are diagnosed with muscle strain would be well a dvised to take antibiotics as part of their treatment“先看我同学是怎么写的他的3段攻击大概是这样的1。
2024全国高考真题英语汇编:阅读理解D篇
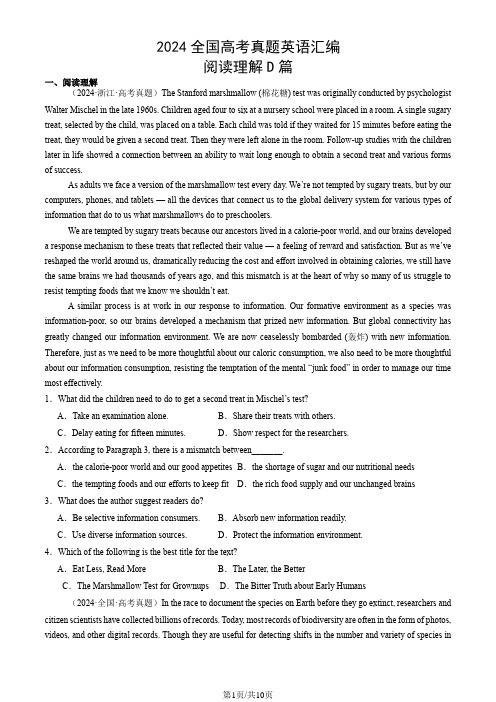
2024全国高考真题英语汇编阅读理解D篇一、阅读理解(2024·浙江·高考真题)The Stanford marshmallow (棉花糖) test was originally conducted by psychologist Walter Mischel in the late 1960s. Children aged four to six at a nursery school were placed in a room. A single sugary treat, selected by the child, was placed on a table. Each child was told if they waited for 15 minutes before eating the treat, they would be given a second treat. Then they were left alone in the room. Follow-up studies with the children later in life showed a connection between an ability to wait long enough to obtain a second treat and various forms of success.As adults we face a version of the marshmallow test every day. We’re not tempted by sugary treats, but by our computers, phones, and tablets — all the devices that connect us to the global delivery system for various types of information that do to us what marshmallows do to preschoolers.We are tempted by sugary treats because our ancestors lived in a calorie-poor world, and our brains developed a response mechanism to these treats that reflected their value — a feeling of reward and satisfaction. But as we’ve reshaped the world around us, dramatically reducing the cost and effort involved in obtaining calories, we still have the same brains we had thousands of years ago, and this mismatch is at the heart of why so many of us struggle to resist tempting foods that we know we shouldn’t eat.A similar process is at work in our response to information. Our formative environment as a species was information-poor, so our brains developed a mechanism that prized new information. But global connectivity has greatly changed our information environment. We are now ceaselessly bombarded (轰炸) with new information. Therefore, just as we need to be more thoughtful about our caloric consumption, we also need to be more thoughtful about our information consumption, resisting the temptation of the mental “junk food” in order to manage our time most effectively.1.What did the children need to do to get a second treat in Mischel’s test?A.Take an examination alone.B.Share their treats with others.C.Delay eating for fifteen minutes.D.Show respect for the researchers.2.According to Paragraph 3, there is a mismatch between_______.A.the calorie-poor world and our good appetites B.the shortage of sugar and our nutritional needsC.the tempting foods and our efforts to keep fit D.the rich food supply and our unchanged brains 3.What does the author suggest readers do?A.Be selective information consumers.B.Absorb new information readily.C.Use diverse information sources.D.Protect the information environment.4.Which of the following is the best title for the text?A.Eat Less, Read More B.The Later, the BetterC.The Marshmallow Test for Grownups D.The Bitter Truth about Early Humans(2024·全国·高考真题)In the race to document the species on Earth before they go extinct, researchers and citizen scientists have collected billions of records. Today, most records of biodiversity are often in the form of photos, videos, and other digital records. Though they are useful for detecting shifts in the number and variety of species inan area, a new Stanford study has found that this type of record is not perfect.“With the rise of technology it is easy for people to make observations of different species with the aid of a mobile application,” said Barnabas Daru, who is lead author of the study and assistant professor of biology in the Stanford School of Humanities and Sciences. “These observations now outnumber the primary data that comes from physical specimens (标本), and since we are increasingly using observational data to investigate how species are responding to global change, I wanted to know: Are they usable?”Using a global dataset of 1.9 billion records of plants, insects, birds, and animals, Daru and his team tested how well these data represent actual global biodiversity patterns.“We were particularly interested in exploring the aspects of sampling that tend to bias (使有偏差) data, like the greater likelihood of a citizen scientist to take a picture of a flowering plant instead of the grass right next to it,” said Daru.Their study revealed that the large number of observation-only records did not lead to better global coverage. Moreover, these data are biased and favor certain regions, time periods, and species. This makes sense because the people who get observational biodiversity data on mobile devices are often citizen scientists recording their encounters with species in areas nearby. These data are also biased toward certain species with attractive or eye-catching features.What can we do with the imperfect datasets of biodiversity?“Quite a lot,” Daru explained. “Biodiversity apps can use our study results to inform users of oversampled areas and lead them to places — and even species — that are not well-sampled. To improve the quality of observational data, biodiversity apps can also encourage users to have an expert confirm the identification of their uploaded image.”5.What do we know about the records of species collected now?A.They are becoming outdated.B.They are mostly in electronic form.C.They are limited in number.D.They are used for public exhibition.6.What does Daru’s study focus on?A.Threatened species.B.Physical specimens.C.Observational data.D.Mobile applications.7.What has led to the biases according to the study?A.Mistakes in data analysis.B.Poor quality of uploaded pictures.C.Improper way of sampling.D.Unreliable data collection devices.8.What is Daru’s suggestion for biodiversity apps?A.Review data from certain areas.B.Hire experts to check the records.C.Confirm the identity of the users.D.Give guidance to citizen scientists.(2024·全国·高考真题)Given the astonishing potential of AI to transform our lives, we all need to take action to deal with our AI-powered future, and this is where AI by Design: A Plan for Living with Artificial Intelligence comes in. This absorbing new book by Catriona Campbell is a practical roadmap addressing the challenges posed by the forthcoming AI revolution (变革).In the wrong hands, such a book could prove as complicated to process as the computer code (代码) thatpowers AI but, thankfully, Campbell has more than two decades’ professional experience translating the heady into the understandable. She writes from the practical angle of a business person rather than as an academic, making for a guide which is highly accessible and informative and which, by the close, will make you feel almost as smart as AI.As we soon come to learn from AI by Design, AI is already super-smart and will become more capable, moving from the current generation of “narrow-AI” to Artificial General Intelligence. From there, Campbell says, will come Artificial Dominant Intelligence. This is why Campbell has set out to raise awareness of AI and its future now — several decades before these developments are expected to take place. She says it is essential that we keep control of artificial intelligence, or risk being sidelined and perhaps even worse.Campbell’s point is to wake up those responsible for AI-the technology companies and world leaders—so they are on the same page as all the experts currently developing it. She explains we are at a “tipping point” in history and must act now to prevent an extinction-level event for humanity. We need to consider how we want our future with AI to pan out. Such structured thinking, followed by global regulation, will enable us to achieve greatness rather than our downfall.AI will affect us all, and if you only read one book on the subject, this is it.9.What does the phrase “In the wrong hands” in paragraph 2 probably mean?A.If read by someone poorly educated.B.If reviewed by someone ill-intentioned.C.If written by someone less competent.D.If translated by someone unacademic.10.What is a feature of AI by Design according to the text?A.It is packed with complex codes.B.It adopts a down-to-earth writing style.C.It provides step-by-step instructions.D.It is intended for AI professionals.11.What does Campbell urge people to do regarding AI development?A.Observe existing regulations on it.B.Reconsider expert opinions about it.C.Make joint efforts to keep it under control.D.Learn from prior experience to slow it down.12.What is the author’s purpose in writing the text?A.To recommend a book on AI.B.To give a brief account of AI history.C.To clarify the definition of AI.D.To honor an outstanding AI expert.(2024·全国·高考真题)“I didn’t like the ending,” I said to my favorite college professor. It was my junior year of undergraduate, and I was doing an independent study on Victorian literature. I had just finished reading The Mill on the Floss by George Eliot, and I was heartbroken with the ending. Prof. Gracie, with all his patience, asked me to think about it beyond whether I liked it or not. He suggested I think about the difference between endings that I wanted for the characters and endings that were right for the characters, endings that satisfied the story even if they didn’t have a traditionally positive outcome. Of course, I would have preferred a different ending for Tom and Maggie Tulliver, but the ending they got did make the most sense for them.This was an aha moment for me, and I never thought about endings the same way again. From then on, if I wanted to read an ending guaranteed to be happy, I’d pick up a love romance. If I wanted an ending I couldn’t guess, I’d pick up a mystery (悬疑小说). One where I kind of knew what was going to happen, historical fiction. Choosingwhat to read became easier.But writing the end — that’s hard. It’s hard for writers because endings carry so much weight with readers. You have to balance creating an ending that's unpredictable, but doesn’t seem to come from nowhere, one that fits what’s right for the characters.That’s why this issue (期) of Writer’s Digest aims to help you figure out how to write the best ending for whatever kind of writing you’re doing. If it’s short stories, Peter Mountford breaks down six techniques you can try to see which one helps you stick the landing. Elizabeth Sims analyzes the final chapters of five great novels to see what key points they include and how you can adapt them for your work.This issue won’t tell you what your ending should be — that’s up to you and the story you’re telling — but it might provide what you need to get there.13.Why did the author go to Prof. Gracie?A.To discuss a novel.B.To submit a book report.C.To argue for a writer.D.To ask for a reading list.14.What did the author realize after seeing Gracie?A.Writing is a matter of personal preferences.B.Readers are often carried away by character.C.Each type of literature has its unique end.D.A story which begins well will end well.15.What is expected of a good ending?A.It satisfies readers’ taste.B.It fits with the story development.C.It is usually positive.D.It is open for imagination.16.Why does the author mention Peter Mountford and Elizabeth Sims?A.To give examples of great novelists.B.To stress the theme of this issue.C.To encourage writing for the magazine.D.To recommend their new books.(2024·北京·高考真题)Franz Boas’s description of Inuit (因纽特人) life in the 19th century illustrates the probable moral code of early humans. Here, norms (规范) were unwritten and rarely expressed clearly, but were well understood and taken to heart. Dishonest and violent behaviours were disapproved of; leadership, marriage and interactions with other groups were loosely governed by traditions. Conflict was often resolved in musical battles. Because arguing angrily leads to chaos, it was strongly discouraged. With life in the unforgiving Northern Canada being so demanding, the Inuit’s practical approach to morality made good sense.The similarity of moral virtues across cultures is striking, even though the relative ranking of the virtues may vary with a social group’s history and environment. Typically, cruelty and cheating are discouraged, while cooperation, humbleness and courage are praised. These universal norms far pre-date the concept of any moralising religion or written law. Instead, they are rooted in the similarity of basic human needs and our shared mechanisms for learning and problem solving. Our social instincts (本能) include the intense desire to belong. The approval of others is rewarding, while their disapproval is strongly disliked. These social emotions prepare our brains to shape our behaviour according to the norms and values of our family and our community. More generally, social instincts motivate us to learn how to behave in a socially complex world.The mechanism involves a repurposed reward system originally used to develop habits important for self-care. Our brains use the system to acquire behavioural patterns regarding safe routes home, efficient food gathering and dangers to avoid. Good habits save time, energy and sometimes your life. Good social habits do something similar in a social context. We learn to tell the truth, even when lying is self-serving; we help a grandparent even when it is inconvenient. We acquire what we call a sense of right and wrong.Social benefits are accompanied by social demands: we must get along, but not put up with too much. Hence self-discipline is advantageous. In humans, a greatly enlarged brain boosts self-control, just as it boosts problem-solving skills in the social as well as the physical world. These abilities are strengthened by our capacity for language, which allows social practices to develop in extremely unobvious ways.17.What can be inferred about the forming of the Inuit’s moral code?A.Living conditions were the drive.B.Unwritten rules were the target.C.Social tradition was the basis.D.Honesty was the key.18.What can we learn from this passage?A.Inconveniences are the cause of telling lies.B.Basic human needs lead to universal norms.C.Language capacity is limited by self-control.D.Written laws have great influence on virtues. 19.Which would be the best title for this passage?A.Virtues: Bridges Across Cultures B.The Values of Self-disciplineC.Brains: Walls Against Chaos D.The Roots of Morality参考答案1.C 2.D 3.A 4.C【导语】这是一篇说明文。
户外观鸟活动英语作文注意事项
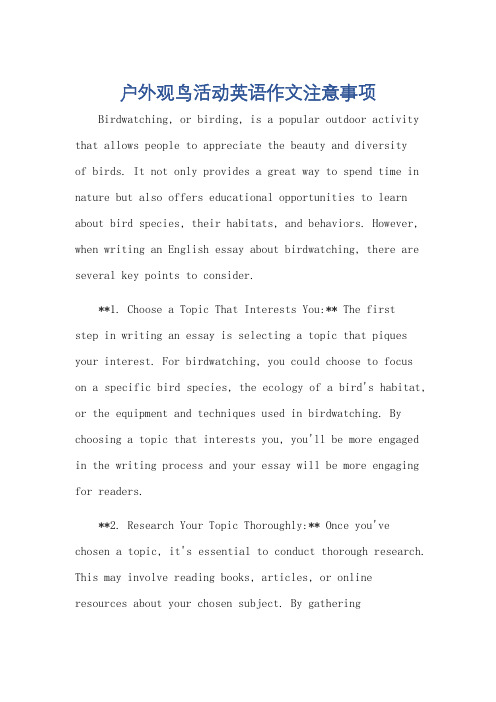
户外观鸟活动英语作文注意事项Birdwatching, or birding, is a popular outdoor activity that allows people to appreciate the beauty and diversityof birds. It not only provides a great way to spend time in nature but also offers educational opportunities to learn about bird species, their habitats, and behaviors. However, when writing an English essay about birdwatching, there are several key points to consider.**1. Choose a Topic That Interests You:** The firststep in writing an essay is selecting a topic that piques your interest. For birdwatching, you could choose to focus on a specific bird species, the ecology of a bird's habitat, or the equipment and techniques used in birdwatching. By choosing a topic that interests you, you'll be more engaged in the writing process and your essay will be more engaging for readers.**2. Research Your Topic Thoroughly:** Once you've chosen a topic, it's essential to conduct thorough research. This may involve reading books, articles, or online resources about your chosen subject. By gatheringinformation from multiple sources, you can ensure that your essay is well-rounded and accurate.**3. Structure Your Essay Clearly:** An effective essay has a clear structure that guides readers through your argument. Typically, this includes an introduction that presents your topic and thesis statement, body paragraphs that develop your ideas, and a conclusion that summarizes your points and leaves readers with a final thought or call to action.**4. Use Specific Examples and Details:** When writing about birdwatching, it's important to include specific examples and details that bring your subject to life. For example, you might describe the appearance of a bird, its behavior in different habitats, or the challenges and rewards of birdwatching in a particular location. These examples will make your essay more engaging and help readers visualize the subject matter.**5. Consider the Audience:** When writing an essay,it's important to consider your audience. Are you writing for fellow birdwatchers who are experts in the field, orfor a general audience who may not be as familiar withbirdwatching? Tailoring your language and examples to your audience will help ensure that your message is received effectively.**6. Edit and Proofread Your Work:** Finally, don't forget to edit and proofread your essay before submitting it. Check for grammar errors, typos, and inconsistencies in your writing. Read your essay aloud to catch any awkward phrasing or sentences that don't flow well. By taking the time to edit your work, you can ensure that your essay is clear, coherent, and error-free.In conclusion, writing an English essay about birdwatching requires careful consideration of topic selection, thorough research, clear structure, specific examples, audience consideration, and careful editing. By following these tips, you can create an engaging and informative essay that will captivate readers and further their understanding of the joys of birdwatching.**户外观鸟活动英语作文注意事项**观鸟,或称观鸟活动,是一项流行的户外活动,使人们能够欣赏鸟类的美丽和多样性。
秃鹫优化算法python
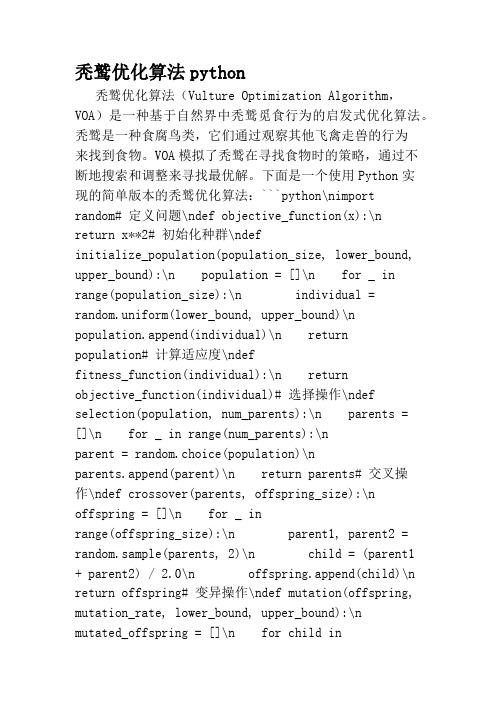
秃鹫优化算法python秃鹫优化算法(Vulture Optimization Algorithm,VOA)是一种基于自然界中秃鹫觅食行为的启发式优化算法。
秃鹫是一种食腐鸟类,它们通过观察其他飞禽走兽的行为来找到食物。
VOA模拟了秃鹫在寻找食物时的策略,通过不断地搜索和调整来寻找最优解。
下面是一个使用Python实现的简单版本的秃鹫优化算法:```python\nimportrandom# 定义问题\ndef objective_function(x):\n return x**2# 初始化种群\ndefinitialize_population(population_size, lower_bound, upper_bound):\n population = []\n for _ inrange(population_size):\n individual = random.uniform(lower_bound, upper_bound)\n population.append(individual)\n returnpopulation# 计算适应度\ndeffitness_function(individual):\n returnobjective_function(individual)# 选择操作\ndefselection(population, num_parents):\n parents = []\n for _ in range(num_parents):\nparent = random.choice(population)\nparents.append(parent)\n return parents# 交叉操作\ndef crossover(parents, offspring_size):\n offspring = []\n for _ inrange(offspring_size):\n parent1, parent2 = random.sample(parents, 2)\n child = (parent1+ parent2) / 2.0\n offspring.append(child)\n return offspring# 变异操作\ndef mutation(offspring, mutation_rate, lower_bound, upper_bound):\n mutated_offspring = []\n for child inoffspring:\n if random.random() <mutation_rate:\n mutated_child =random.uniform(lower_bound, upper_bound)\nelse:\n mutated_child = child\nmutated_offspring.append(mutated_child)\n returnmutated_offspring# 主函数\ndefvulture_optimization_algorithm(population_size,num_generations, num_parents, offspring_size,mutation_rate, lower_bound, upper_bound):\npopulation = initialize_population(population_size,lower_bound, upper_bound)\n \n for _ inrange(num_generations):\n fitness_values =[fitness_function(individual) for individual inpopulation]\n \n parents =selection(population, num_parents)\n \noffspring = crossover(parents, offspring_size)\n\n mutated_offspring = mutation(offspring,mutation_rate, lower_bound, upper_bound)\n\n population = parents +mutated_offspring\n \n best_individual =min(population, key=fitness_function)\n \nreturn best_individual# 使用示例\npopulation_size =50\nnum_generations = 100\nnum_parents =10\noffspring_size = 20\nmutation_rate =0.1\nlower_bound = -10.0\nupper_bound =10.0best_solution =vulture_optimization_algorithm(population_size,num_generations, num_parents,\n offspring_size, mutation_rate,\nlower_bound, upper_bound)print(\"最优解:\",best_solution)\nprint(\"最优解的适应度:\",objective_function(best_solution))\n```在这个示例中,我们定义了一个简单的目标函数`objective_function`,它的目标是找到使得函数取得最小值的输入。
Validityandarguments有效性和参数
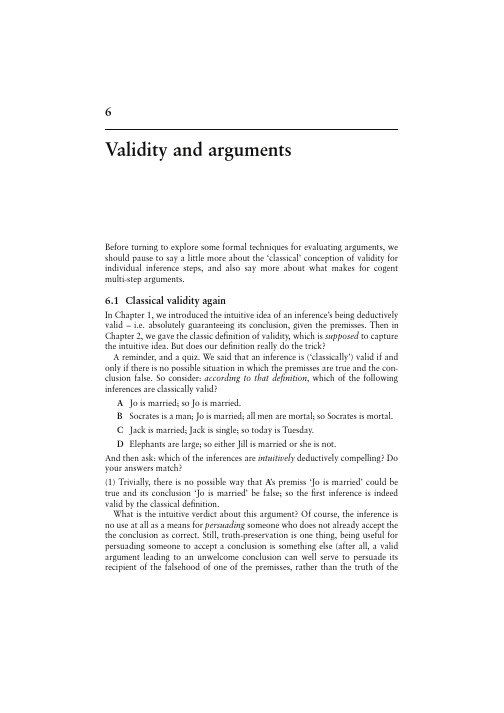
6Validity and argumentsBefore turning to explore some formal techniques for evaluating arguments, we should pause to say a little more about the ‘classical’ conception of validity for individual inference steps, and also say more about what makes for cogent multi-step arguments.6.1Classical validity againIn Chapter 1, we introduced the intuitive idea of an inference’s being deductively valid – i.e. absolutely guaranteeing its conclusion, given the premisses. Then in Chapter 2, we gave the classic definition of validity, which is supposed to capture the intuitive idea. But does our definition really do the trick?A reminder, and a quiz. We said that an inference is (‘classically’) valid if and only if there is no possible situation in which the premisses are true and the con-clusion false. So consider: according to that definition, which of the following inferences are classically valid?A Jo is married; so Jo is married.B Socrates is a man; Jo is married; all men are mortal; so Socrates is mortal.C Jack is married; Jack is single; so today is Tuesday.D Elephants are large; so either Jill is married or she is not.And then ask: which of the inferences are intuitively deductively compelling? Do your answers match?(1) Trivially, there is no possible way that A’s premiss ‘Jo is married’ could be true and its conclusion ‘Jo is married’ be false; so the first inference is indeed valid by the classical definition.What is the intuitive verdict about this argument? Of course, the inference is no use at all as a means for persuading someone who does not already accept the the conclusion as correct. Still, truth-preservation is one thing, being useful for persuading someone to accept a conclusion is something else (after all, a valid argument leading to an unwelcome conclusion can well serve to persuade its recipient of the falsehood of one of the premisses, rather than the truth of the6.1Classical validity again45 conclusion). Once we make the distinction between truth-preservation and use-fulness, there is no reason not to count the ‘Jo’ argument as deductively valid. As we put it in §3.2, an inference that covers no ground hasn’t got a chance to go wrong!Objection: ‘It was said at the very outset that arguments aim to give reasons for their conclusions – and the premiss of A doesn’t give an independent reason for its conclusion, so how can it be a good argument?’ Reply: Fair comment. We’ve just agreed that A isn’t a persuasive argument, in the sense that it can’t give anyone who rejects the conclusion a reason for changing their minds. Still, to repeat, that doesn’t mean that the inferential relation between premiss and conclusion isn’t absolutely secure. And the intuitive idea of validity is primarily introduced to mark absolutely secure inferences: A just illustrates the limiting case where the security comes from unadventurously staying in the same place.(2) Argument B with a redundant and irrelevant premiss also comes out as valid by the classical definition. There can be no situation in which Socrates is a man, and all men are mortal, yet Socrates is not mortal. So there can be no situation in which Socrates is a man, all men are mortal, and (as it happens) Jo is married, yet Socrates is not mortal.What’s the intuitive verdict on B? Well, introducing redundant premisses may be pointless (or worse, may be misleading). But B’s conclusion is still guaran-teed, if all the premisses – including the ones the really matter – are true. Generalizing, suppose A1, A2, …, A n logically entail C. Then A1, A2, …, A n, B logically entail C, for any additional premiss B (both intuitively, and by our offi-cial definition).Deductive inference contrasts interestingly with inductive inference in this respect. Recall the ‘coffee’ argument in §1.3:E(1)Cups of coffee that looked and tasted just fine haven’t killed you in the past.(2)This present cup of coffee looks and tastes just fine.So(3)This present cup of coffee won’t kill you.The premisses here probabilistically support the conclusion. But add the further premiss(2′)The coffee contains a tasteless poison,and the conclusion now looks improbable. Add the further premiss(2″)The biscuit you just ate contained the antidote to that poison, and (3) is supported again (for it is still unlikely the coffee will kill you for any other reason). This swinging to and fro of degree of inductive support as further relevant information is noted is one reason why the logic of inductive arguments is difficult to analyse and codify. By contrast, a deductively cogent argument can’t be spoilt by adding further premisses.(3) So far so good. We can readily live with the results that completely trivial arguments and arguments with redundant premisses count as deductively valid46Validity and argumentsby the classical definition. But now note that there is no possible situation in which Jack is both married and single. That is to say, there is no possible situa-tion where the premisses of argument C are both true. So, there is no possible situation where the premisses of that argument are both true and the conclusion false. So, this time much less appealingly, the inference in C also comes out as valid by the classical definition.The point again generalizes. Suppose that a bunch of propositions A1, A2, …, A n is logically inconsistent, i.e. there is no possible situation in which A1, A2, …, A n are all true together. Then there is also no situation in which A1, A2, …, A n are all true and C is false, whatever C might be. Hence by our definition of classical validity, we can validly infer any proposition we choose from a bunch of incon-sistent premisses.That all looks decidedly counter-intuitive. Surely the propositions ‘Jack is married’ and ‘Jack is single’ can’t really entail anything about what day of the week it is.(4) What about the ‘Jill’ argument? There is no possible situation in which the conclusion is false; it is inevitably true that either Jill is married or she isn’t. So, there is no possible situation where the conclusion is false and the premiss about elephants is true. Hence the inference in argument D is also valid by the classical definition.The point again generalizes: If C is necessarily true, i.e. there is no possible sit-uation in which C is false, then there is also no situation in which A1, A2, …, A n are all true, and C is false, whatever A1, A2, …, A n might be. Hence by our defi-nition, we can validly infer a necessarily true conclusion from any premisses we choose, including elephantine ones.Which again looks absurd. What to do?6.2Sticking with the classical definitionWe have just noted a couple of decidedly counter-intuitive results of the classical definition of validity. Take first the idea that contradictory premisses entail any-thing. Then one possible response runs along the following lines:We said that the premisses of a valid argument guarantee the conclusion: how can contradictory premisses guarantee anything? And intuitively, the conclusion of an inferentially cogent inference ought to have something to do with the premisses. So argument C commits a fallacy of irrelevance. Our official definition of validity therefore needs to be tightened up by introduc-ing some kind of relevance-requirement in order to rule out such examples as counting as ‘valid’. Time to go back to the drawing board.Another response runs:The verdict on C is harmless because the premisses can never be true together; so we can never use this type of inference in a sound argument for an irrelevant conclusion. To be sure, allowing the ‘Jack’ argument to count as valid looks surprising, even counter-intuitive. But maybe we shouldn’t6.3Multi-step arguments again47 slavishly follow all our pre-theoretical intuitions, especially our intuitions about unusual cases. The aim of an official definition of validity is to give a tidy ‘rational reconstruction’ of our ordinary notion which smoothly cap-tures the uncontentious cases; the classical definition does that particularly neatly. So we should bite the bullet and accept the mild oddity of the verdict on C.Likewise there are two responses to the observation that the classical definition warrants argument D. We could either say this just goes to show that our defini-tion allows more gross fallacies of irrelevance. Or we could bite the bullet again and deem this result to be a harmless oddity (we already need to know that a conclusion C is necessarily true before we are in a position to say that it is classi-cally entailed by arbitrary premisses – and if we already know that C is neces-sary, then extra valid arguments for it like D can’t extend our knowledge). The majority of modern logicians opt for the second responses. They hold that the cost of trying to construct a plausible notion of ‘relevant’ inference turns out to be too high in terms of complexity to make it worth abandoning the neat sim-plicity of the idea of classical validity. But there is a significant minority who insist that we really should be going back to the drawing board, and hold that there are well-motivated and workable systems of ‘relevant logic’. We won’t be able to explore their arguments and their alternative logical systems here – and in any case, these variant logics can really only be understood and appreciated in contrast to classical systems. We just have to note frankly that the classical defi-nition of validity is not beyond challenge.Still, our basic aim is to model certain forms of good reasoning; and models can be highly revealing even if some of their core constructs idealize. The idea of a ‘classically valid argument’ – like e.g. the idea of a ‘ideal gas’ – turns out at least to be a highly useful and illuminating idealization: and this can be so even if it would be wrong to claim that it is in the end the uniquely best and most accu-rate idealization. Anyway, we are going to stick to the classical definition in our introductory discussions in this book.6.3Multi-step arguments againWe characterized deductive validity as, in the first place, a property of individual inference steps. We then fell in with the habit of calling a one-step argument valid if it involves a valid inference step from initial premisses to final conclu-sion. In this section, we’ll see the significance of that qualification ‘one-step’. Consider the following mini-argument:F(1)All philosophers are logicians.So(2)All logicians are philosophers.The inference is obviously fallacious. It just doesn’t follow from the assumption that all philosophers are logicians that only philosophers are logicians. (You might as well argue ‘All women are human beings, hence all human beings are women’.) Here’s another really bad inference:。
反向因果问题 英语

反向因果问题英语The Reverse Causality Dilemma.In the realm of causal analysis, we are often accustomed to thinking in terms of direct, linear relationships where one event leads to another in a predictable sequence. However, the concept of reverse causality introduces a complex and intriguing twist to this traditional understanding. Reverse causality suggests that, rather than one event causing another, the effect may precede the cause, creating a circular or feedback loop where the distinction between cause and effect becomes blurred.Exploring this concept further, we encounter scenarios where outcomes influence their own precursors, often in ways that are not immediately apparent. For instance, in the field of economics, policies designed to stimulate growth may actually hinder it if the measures taken have unintended consequences that discourage investment or limiteconomic freedoms. Similarly, in the realm of health, attempts to improve patient outcomes through aggressive treatment regimens may backfire if the side effects of those treatments outweigh the benefits.The implications of reverse causality are profound and far-reaching. They challenge our fundamental understanding of cause-and-effect relationships and force us to reevaluate our assumptions about how the world works. Consider the following scenarios that illustrate the complexities of reverse causality:Scenario 1: Education Policy and Student Performance.In an effort to improve student performance, a school district implements a strict new curriculum that mandates more hours of classroom instruction and standardized testing. Initially, the district expects these measures to lead to higher test scores and better prepared students. However, what actually ensues is a decline in student engagement and motivation, as the increased workload and stress associated with the new curriculum take their toll.As a result, student performance suffers, and the original goals of the policy are not met.In this case, the policy intended to improve student performance actually had the opposite effect, creating a reverse causality scenario where the outcome (poor student performance) preceded the policy implementation that was supposed to address it.Scenario 2: Environmental Protection and Economic Growth.A country facing environmental degradation implements strict environmental protection measures, aiming to preserve its natural resources and promote sustainable development. These measures include restrictions on industrial pollution, limitations on resource extraction, and incentives for renewable energy use. While these measures are expected to have a positive impact on the environment, they may also have negative economic consequences.For instance, stricter pollution regulations may increase the cost of production for local industries, leading to job losses and slower economic growth. The country's GDP may suffer, and the social and political implications of this economic downturn may outweigh the environmental benefits. In this scenario, the environmental protection measures designed to safeguard the planet's future may actually hinder the economic growth that is necessary to support that future.The examples above highlight the challenges of anticipating and managing reverse causality. They underscore the need for a more nuanced and comprehensive approach to policymaking and decision-making that takesinto account the potential for unintended consequences. Here are some strategies that can help mitigate the risks associated with reverse causality:1. Anticipate and Assess Unintended Consequences: Before implementing a new policy or initiative, it is crucial to anticipate and assess potential unintended consequences. This requires a thorough understanding of thesystem at hand and the ability to identify potential feedback loops or circular relationships that may give rise to reverse causality.2. Monitor and Evaluate Ongoing Programs: Once a policy or program is implemented, it is essential to monitor its progress and evaluate its impact. This involves collecting data on key indicators and outcomes to detect any deviations from expected results. If reverse causality is suspected, adjustments can be made to mitigate its effects.3. Promote Adaptive Management: Adaptive management is an approach that emphasizes learning from experience and making iterative improvements to policies and programs based on observed outcomes. By embracing this approach, decision-makers can adjust their strategies as new information becomes available, reducing the risk of reverse causality.4. Encourage Cross-Sector Collaboration: Reverse causality often arises when policies or initiatives impact multiple sectors (e.g., environment and economy).Encouraging collaboration and communication across sectors can help identify potential conflicts early on and develop solutions that balance different interests and outcomes.5. Invest in Long-Term Solutions: Reverse causality often arises when short-term solutions are applied to complex, long-term problems. Investing in sustainable,long-term solutions that address the root causes of issues can help break the cycle of reverse causality and lead to more durable and effective outcomes.In conclusion, the concept of reverse causality challenges our understanding of cause-and-effect relationships and highlights the need for a more nuanced and adaptive approach to decision-making. By anticipating and assessing unintended consequences, monitoring and evaluating ongoing programs, promoting adaptive management, encouraging cross-sector collaboration, and investing in long-term solutions, we can mitigate the risks associated with reverse causality and create more effective and sustainable policies and initiatives.。
- 1、下载文档前请自行甄别文档内容的完整性,平台不提供额外的编辑、内容补充、找答案等附加服务。
- 2、"仅部分预览"的文档,不可在线预览部分如存在完整性等问题,可反馈申请退款(可完整预览的文档不适用该条件!)。
- 3、如文档侵犯您的权益,请联系客服反馈,我们会尽快为您处理(人工客服工作时间:9:00-18:30)。
Tailoring Evaluative Arguments to User’s PreferencesGiuseppe Carenini and Johanna MooreIntelligent Systems Program,University of Pittsburgh,U.S.A.HCRC,University of Edinburgh,U.K.puter systems that serve as personal assistants,advisors,or sales assistantsfrequently need to argue evaluations of domain entities.Argumentation theory shows thatto argue an evaluation convincingly requires to base the evaluation on the hearer’s valuesand preferences.In this paper we propose a framework for tailoring an evaluative argumentabout an entity when user’s preferences are modeled by an additive multiattribute valuefunction.Since we adopt and extend previous work on explaining decision-theoretic ad-vice as well as previous work in computational linguistics on generating natural languagearguments,our framework is both formally and linguistically sound.1IntroductionComputer systems that serve as personal assistants,advisors,or sales assistants frequently need to generate evaluative arguments for domain entities.For instance,a student advisor may need to argue that a certain course would be an excellent choice for a particular student,or a real-estate personal assistant may need to argue that a certain house would be a questionable choice for its current user.Argumentation theory indicates that to argue an evaluation convincingly requires to base the evaluation on the hearer’s values and preferences.Therefore,the effectiveness of sys-tems that serve as assistants or advisors in situations in which they need to present evaluative arguments critically depends on their ability to tailor their arguments to a model of the user’s values and preferences.In this paper we propose a computational framework for generating eval-uative arguments that could be applied in systems serving as personal assistants or advisors. In our framework,as suggested by argumentation theory,arguments are tailored to a model of user’s preferences.Furthermore,in accordance with current research in user modeling,we adopt as model of user’s preferences a conceptualization based on multiattribute utility theory(more specifically an additive multiattribute value function(AMVF)).2Background on AMVFAn AMVF is a utility model based on a value tree and on a set of component value functions,one for each attribute of an alternative.A value tree is a decomposition of the objective to maximize the value of the selection for the decision maker into an objective hierarchy in which the leaves correspond to attributes of the alternatives.The arcs of the tree are weighted depending on the This work was supported by grant number DAA-1593K0005from the Advanced Research Projects Agency(ARPA).Its contents are solely the responsibility of the authors and do not necessarily repre-sent the official views of either ARPA or the ernment.importance of an objective in achieving the objective above it in the tree.Note that the sum of the weights at each level is equal to1.A component value function for an attribute expresses the preferability of each attribute value as a number in the interval.Formally,an AMVF has the following form:(1)–is the vector of attribute values for alternative–For each attribute,is the component value function,which maps the least preferable to0,the best to1,and the other to values in[0,1]–is the weight for attribute,and and3The Framework and a Sample Argumentative StrategyOur framework for generating evaluative arguments is based on previous work in artificial in-telligence on explaining decision-theoretic advice(Klein and Shortliffe,1994),and on previous work in computational linguistics on generating natural language evaluative arguments(Elhadad, 1995).On the one hand,the study on explaining decision-theoretic advice produced a rich quan-titative model that can serve as a basis for strategies to select and organize the content of decision-theoretic explanations,but was not concerned at all with linguistic issues.On the other hand,the work in computational linguistics produced a well-founded model of how argumentative intents (i.e.,whether a proposition favors or disfavors the alternative)can influence sophisticated lin-guistic decisions,but it produced weak results as far as the selection and the organization of the argument content is concerned.From Klein and Shortliffe(1994)we adopted and adapted two basic concepts for content selection and organization:and.An objective can be compelling in arguing for an alternative either because of its strength or because of its weakness in contributing to the value of an alternative.So,if measures how much the value of an objective is contributing to the overall value difference of an alternative from the worst case1 and measures how much the value of an objective is determining the overall value difference of an alternative from the best case,a possible definition for is the greatest of the two quantities rmally,an objective is if it is an outlier in a population with respect to.Elhadad’s techniques for performing sophisticated linguistic decisions based on argumenta-tive intent used a model of user’s preferences different from an AMVF.So,in order to adopt his work,we have to show how argumentative intents can be computed from an AMVF-based model of user’s preferences.Basically,two subintervals of the interval,and must be defined(e.g.,and).Then we consider the value of an alternative for an objec-tive to correspond to a negative,positive or neutral argumentative intent depending on whether it belongs to,or respectively.We sketch now a sample argumentative strategy for the communicative goal of evaluating a single entity.The strategy is based on the notions of anddefined previously.First,we introduce some terminology:Root is the objective the argument is 1is an alternative such as that,whereas is such as that.,,,I would strongly suggest house-b15.It is spacious.It has a large lot-size(800sqf),many rooms(7)and plenty of storage space(200sqf).Furthermore,since it offers anexceptional view and appears nice,house-b15quality is excellent.Finally,house-b15has afair location.Although it is far from a shopping area,it has access to a park(2miles).Figure1.Sample concise andfluent natural language evaluative argumentabout;MainArgIntent is either+or-and determines whether the generated argument will favoror disfavor the entity;ArgItent is a function that when applied to the value of an objective returnsits argumentative intent(which is either+,-or neutral);the Express function indicates that an objective must be realized in natural language with a certain argumentative intent.Argumentation Strategyargue(Root,entity,user-model,MainArgIntent,);;content selection and assignmentsEliminate all objectives for which is false.most compelling objective such as that andall such as that andall such as that andand;;Steps for expressing the content.Express(Root,MainArgIntent);;(e.g.,“I strongly suggest this entity”)Argue(,entity,user-model,MainArgIntent,)For all,Express(,);;ordered by compellingness For all,Argue(,entity,user-model,MainArgIntent,);;ordered by compellingness This strategy is based on guidelines for presenting evaluative arguments suggested in argu-mentation literature(Mayberry and Golden,1996).The main factor in favor of the evaluation is presented in detail,along with possible counter-arguments(i.e.,),that must always be considered,but not in detail.Finally,further supporting factors must be pre-sented in detail.As specified in the strategy,details about a factor are presented by recursivelycalling the function on the factor.Figure1shows a sample argument generated by the strategy when it is applied to a user model based on a value tree consisting of15objectives.As future work,we plan to empirically evaluate our framework.We also intend to extend itto more complex models of user preferences and to generating arguments that combine languagewith information graphics.ReferencesElhadad,M.(1995).Using argumentation in text generation.Journal of Pragmatics24:189–220.Klein,D.A.,and Shortliffe,E.H.(1994).A framework for explaining decision-theoretic advice.Artificial Intelligence67:201–243.Mayberry,K.J.,and Golden,R.E.(1996).For Argument’s Sake:A Guide to Writing Effective Arguments.Harper-Collins,College Publishers.Second Edition.。