汽车数据集(cars dataset)_数据挖掘_科研数据集
uscar2解读 -回复

uscar2解读-回复UsCar2解读UsCar2是一种车辆行为数据集,该数据集记录了多辆车辆在城市环境中的行驶过程。
通过分析这些数据,我们可以深入理解车辆行为、交通流量和道路安全等方面。
本文将以UsCar2为主题,一步一步解析数据集,并探讨其应用和对交通领域的影响。
第一步:了解UsCar2数据集UsCar2数据集由德国诺伊豪斯大学的智能交通系统研究小组创建。
该数据集是通过模拟城市环境中的车辆行驶而获得的,每辆车都有自己的特定行为模式。
数据集中包含了车辆的位置、速度、加速度以及其他相关信息。
这些数据以实时序列的方式呈现,可以用来研究车辆行为和交通流量等问题。
第二步:数据集的应用与意义UsCar2数据集的应用范围广泛,主要体现在以下几个方面:1.交通规划与道路设计:通过分析UsCar2数据集,可以获得真实车辆行为的数据,为交通规划和道路设计提供参考。
例如,可以根据数据集中的交通流量和车辆速度等信息,优化道路布局、信号灯设置和车道规划,提高道路的通行效率和安全性。
2.智能驾驶技术:UsCar2数据集可以为智能驾驶技术的开发和优化提供有价值的参考。
通过分析数据集中的车辆行为模式和交通状况,可以改进自动驾驶系统的决策和控制策略,提高智能车辆的安全性和性能。
3.交通安全与风险评估:利用UsCar2数据集,可以对道路上的交通安全进行评估和风险分析。
通过分析数据集中的车辆行为数据,可以识别出潜在的交通事故风险区域,并提出相应的安全措施。
此外,还可以通过模拟不同交通场景,预测交通事故的发生概率,进一步优化交通安全管理。
4.交通行为研究与预测:UsCar2数据集为研究人员提供了研究车辆行为和交通流量的宝贵资源。
通过分析数据集中的车辆行为模式、速度分布和加速度等信息,可以深入研究车辆行为的规律和驾驶模式,为交通行为预测和交通流量管理提供理论支持和数据支持。
第三步:数据集解析与分析在对UsCar2数据集进行解析和分析时,可以采取以下步骤:1.数据清洗与格式化:首先,对数据集进行清洗和格式化,去除不完整或错误的数据,并将数据格式统一,以方便后续的分析和处理。
自动驾驶相关数据集研究综述

255中国设备工程C h i n a P l a n t E n g i n e e r i ng中国设备工程 2021.01 (上)自动驾驶是当今热门研究领域,面临许多技术挑战。
无人车在行驶时需要依赖感知识别系统对周围的环境(道路、行人、车辆等)进行感知,为接下来的基于深度学习及人工智能的驾驶决策及控制提供依据。
系统要感知检测的事物种类繁多,且容易受到天气、环境等因素的干扰。
如果自动驾驶的算法不能在大量可靠的数据上进行适量的、有效的训练,那么,当其被投入实际使用后,就可能造成不可预估的后果。
因此,为了推动这一领域的后续研究与发展,自动驾驶相关数据集应运而生,科研工作者围绕众多数据集做了很多开创性的工作。
本文在现有文献基础上,从数据集内容、采集方法、是否进行标注和标注方法等方面,针对不同的自动驾驶数据集进行总结与对比,为研究自动驾驶场景感知、行为决策及控制算法奠定基础。
1 数据集介绍从采集内容、采集设备及方法、标注及标注方法等方面对数据集进行介绍。
典型数据集包括KITTI、Apollo、BDD100K、nuScenes 、CityScapes 和HDD 等。
1.1 数据集内容KITTI 数据集包含市区、乡村和高速公路等场景采集的真实图像数据,每张图像中最多达15辆车和30个行人。
整个数据集由389对立体图像和光流图(包括194对训练图像和195对测试图像),39.2km 视觉测距序列以及超过200k 的3D 标注物体的图像组成,采样频率为10Hz,总共约3TB 。
Apollo 为百度推出的交通场景解析数据集,包括上万帧的高分辨率RGB 视频和与其对应的逐像素语义标注。
26个语义类提供了总共17062张图像和相对应的语义标注与深度信息,用于设计算法和训练模型。
BDD100K 为目前规模最大、兼具内容复杂性与多样性的公开驾驶数据集,包含了10万段高清视频,每段视频约40s 时长,分辨率为720p ,帧率为30fps 。
数据挖掘_Epinions datasets(Epinions数据集)
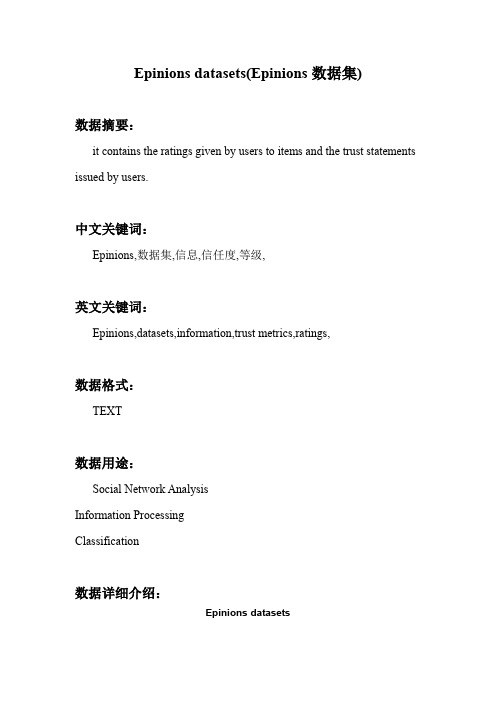
Epinions datasets(Epinions数据集)数据摘要:it contains the ratings given by users to items and the trust statements issued by users.中文关键词:Epinions,数据集,信息,信任度,等级,英文关键词:Epinions,datasets,information,trust metrics,ratings,数据格式:TEXT数据用途:Social Network AnalysisInformation ProcessingClassification数据详细介绍:Epinions datasetsThe dataset was collected by Paolo Massa in a 5-week crawl (November/December 2003) from the Web site.The dataset contains49,290 users who rated a total of139,738 different items at least once, writing664,824 reviews.487,181 issued trust statements.Users and Items are represented by anonimized numeric identifiers.The dataset consists of 2 files.Contents1 Files1.1 Ratings data1.2 Trust data1.3 Data collection procedure2 Papers analyzing Epinions datasetRatings dataratings_data.txt.bz2 (2.5 Megabytes): it contains the ratings given by users to items. Every line has the following format:user_id item_id rating_valueFor example,23 387 5represents the fact "user 23 has rated item 387 as 5"Ranges:user_id is in [1,49290]item_id is in [1,139738]rating_value is in [1,5]Trust datatrust_data.txt.bz2 (1.7 Megabytes): it contains the trust statements issued by users. Every line has the following format:source_user_id target_user_id trust_statement_valueFor example, the line22605 18420 1represents the fact "user 22605 has expressed a positive trust statement on user 18420"Ranges:source_user_id and target_user_id are in [1,49290]trust_statement_value is always 1 (since in the dataset there are only positive trust statements and not negative ones (distrust)).Note: there are no distrust statements in the dataset (block list) but only trust statements (web of trust), because the block list is kept private and not shown on the site.Data collection procedureThe data were collected using a crawler, written in Perl.It was the first program I (Paolo Massa) ever wrote in Perl (and an excuse for learning Perl) so the code is probably very ugly. Anyway I release the code under the GNU Generic Public Licence (GPL) so that other people might be use the code if they so wish.epinionsRobot_pl.txt is the version I used, this version parses the HTML and saves minimal information as perl objects. Later on, I saw this was not a wise choice (for example, I didn't save demographic information about users which might have been useful for testing, for example, is users trusted by user A comes from the same city or region). So later on I created a version that saves the original HTML pages(epinionsRobot_downloadHtml_pl.txt) but I didn't test it. Feel free to let me know if it works. Both Perl files are released under GNU Generic Public Licence (GPL), see first lines of the files. --PaoloMassaBe aware that the script was working in 2003, I didn't check but it is very likely that the format of HTML pages has changed significantly in the meantime so the script might needsome adjustments. Luckily, the code is released as open source so you can modify it. --Paolo Massa 11:34, 16 July 2010 (UTC)Papers analyzing Epinions datasetTrust-aware Recommender Systemsadd another paper!Retrieved from "/wiki/Downloaded_Epinions_dataset"数据预览:点此下载完整数据集。
数据挖掘

基于聚类分析的孤立点挖掘方法1、数据挖掘数据挖掘是应用一系列技术从大型数据库或者数据仓库的数据中提取人感兴趣的,隐含的、事先未知而潜在有用的,提取的知识表示为概念、规则、模式等形式的信息和知识。
简言之,据挖掘就是从大量的、不完全的、有噪声的、模糊的、随的数据中,提取隐含在其中的、人们事先不知道的、但又是潜在有用的信息知识的过程。
因此,数据挖掘事实上是知识发现的一个特定步骤,它是一种智能化的、综合应用各种统计分析、数据库、智能语言来分析庞大数据资料的技术,或者说是对大容量数据及数据间系进行考察和建模的方法集。
数据挖掘的目标是将大容量数据转化为有用知识和信息。
它的目的,就是拓展更加有效的利用已有数据,拓展应用。
数据开采技术的目标是从大量数据中,发现隐藏于其后的规律或数据间的关系,从而服务于决策。
因此,数据挖掘一般有以下5类主要任务:( 1 ) 数据总结:数据总结目的是对数据进行浓缩,给出它的总体综合描述。
过对数据的总结,数据挖掘能够将数据库中的有关数据从较低的个体层次抽总结到较高的总体层次上,从而实现对原始基本数据的总体把握。
( 2 ) 分类:分类即分析数据的各种属性,并找出数据的属性模型,确定哪些据属于哪些组。
这样我们就可以利用该模型来分析已有数据,并预测新数据属于哪一个组。
( 3 ) 关联分析:数据库中的数据一般都存在着关联关系,也就是说,两个或多个变量的取值之间存在某种规律性,包括关联关系有简单关联和时序关联两。
( 4 ) 聚类:聚类分析是按照某种相近程度度量方法,将用户数据分成一系列有意义的子集合。
( 5 ) 偏差的检测:对分析对象的少数的、极端的特例的描述,揭示内在的原因。
目前,研究数据挖掘的方法有很多,这些数据挖掘工具采用的主要方法包括传统统计方法,可视化技术,决策树、相关规则、神经元网络、遗传算法等。
下面分类阐述。
( 1 ) 传统统计方法:包括:抽样技术,多元统计分析,统计预测方法等。
对斯坦福汽车数据集的认识

对斯坦福汽车数据集的认识
斯坦福汽车数据集(Stanford Cars Dataset)是由斯坦福大学的计算机视觉实验室提供的一个被广泛使用的汽车图像数据集。
该数据集收集了来自不同角度和位置的16,185张汽车图像,并且涵盖了196种不同的汽车品牌。
每张图像都配有对应的汽车品牌和型号的标注。
这个数据集在计算机视觉领域的研究中经常被用于目标检测、图像分类和图像识别等任务。
研究人员可以使用这个数据集训练和测试他们的算法和模型,并评估它们在汽车图像处理方面的性能。
斯坦福汽车数据集的特点包括:
1. 大规模数据集:数据集包含了数万张汽车图像,可以提供充足的样本用于训练和测试。
2. 多样性:数据集包含了不同角度和位置拍摄的汽车图像,涵盖了各种汽车品牌和型号,具有较好的代表性。
3. 标注信息:每张图像都有对应的汽车品牌和型号的标注,这对于进行有监督学习非常有用。
斯坦福汽车数据集的使用使得研究者能够开展汽车图像相关的机器学习和深度学习研究,推动了计算机视觉领域在汽车识别和相关应用方向的发展。
巴文彤(汽车市场信息数据挖掘探索与研究)
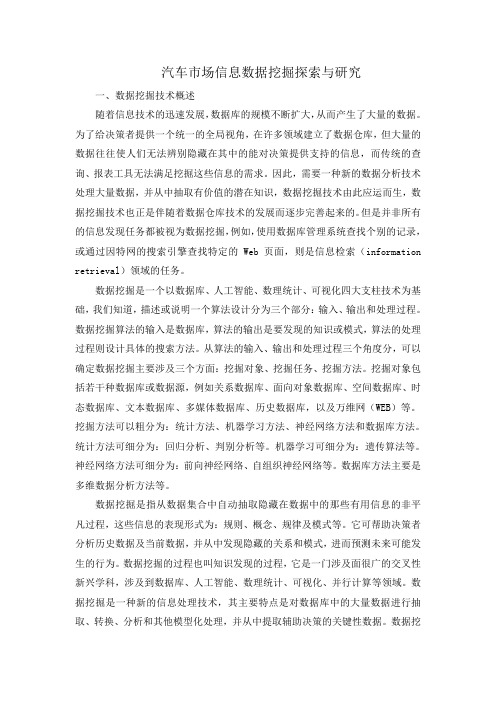
汽车市场信息数据挖掘探索与研究一、数据挖掘技术概述随着信息技术的迅速发展,数据库的规模不断扩大,从而产生了大量的数据。
为了给决策者提供一个统一的全局视角,在许多领域建立了数据仓库,但大量的数据往往使人们无法辨别隐藏在其中的能对决策提供支持的信息,而传统的查询、报表工具无法满足挖掘这些信息的需求。
因此,需要一种新的数据分析技术处理大量数据,并从中抽取有价值的潜在知识,数据挖掘技术由此应运而生,数据挖掘技术也正是伴随着数据仓库技术的发展而逐步完善起来的。
但是并非所有的信息发现任务都被视为数据挖掘,例如,使用数据库管理系统查找个别的记录,或通过因特网的搜索引擎查找特定的Web页面,则是信息检索(information retrieval)领域的任务。
数据挖掘是一个以数据库、人工智能、数理统计、可视化四大支柱技术为基础,我们知道,描述或说明一个算法设计分为三个部分:输入、输出和处理过程。
数据挖掘算法的输入是数据库,算法的输出是要发现的知识或模式,算法的处理过程则设计具体的搜索方法。
从算法的输入、输出和处理过程三个角度分,可以确定数据挖掘主要涉及三个方面:挖掘对象、挖掘任务、挖掘方法。
挖掘对象包括若干种数据库或数据源,例如关系数据库、面向对象数据库、空间数据库、时态数据库、文本数据库、多媒体数据库、历史数据库,以及万维网(WEB)等。
挖掘方法可以粗分为:统计方法、机器学习方法、神经网络方法和数据库方法。
统计方法可细分为:回归分析、判别分析等。
机器学习可细分为:遗传算法等。
神经网络方法可细分为:前向神经网络、自组织神经网络等。
数据库方法主要是多维数据分析方法等。
数据挖掘是指从数据集合中自动抽取隐藏在数据中的那些有用信息的非平凡过程,这些信息的表现形式为:规则、概念、规律及模式等。
它可帮助决策者分析历史数据及当前数据,并从中发现隐藏的关系和模式,进而预测未来可能发生的行为。
数据挖掘的过程也叫知识发现的过程,它是一门涉及面很广的交叉性新兴学科,涉及到数据库、人工智能、数理统计、可视化、并行计算等领域。
汽车CPS大数据若干关键技术

汽车cps大数据若干关键技术
contents
目录
• CPS数据采集与预处理 • CPS数据存储与管理 • CPS数据挖掘与分析 • CPS数据可视化与交互式探索 • CPS大数据安全与隐私保护 • CPS大数据应用案例分享
01
CPS数据采集与预处理
数据采集技术
传感器数据采集
哈希索引
利用哈希函数将关键字映射到索引中,支持快速查找。
数据查询优化
查询优化算法
利用统计信息和其他优化方法,选择最优的查询 执行计划,提高查询效率。
索引优化
根据查询需求选择合适的索引类型,优化索引结 构,提高查询速度。
数据压缩
对数据进行压缩,减少存储空间占用和网络传输 量,提高数据处理效率。
03
基于图标的可视化技术
使用图形元素(如柱状图、折线图、饼图等 )展示数据,便于用户直观理解数据。
基于3D技术的可视化技术
利用3D建模和渲染技术,创建逼真的数据场景和模 型,提供沉浸式的数据体验。
交互式可视化技术
允许用户通过鼠标、键盘或其他交互设备与 数据展示进行交互,实现数据的探索和分析 。
可视化交互设计
数据归一化
将数据的尺度进行统一,以避免因数据尺度不同而导 致的分析偏差。
数据离散化
将连续型数据进行离散化处理,以方便后续的分类与 聚类分析。
02
CPS数据存储与管理
分布式存储系统ຫໍສະໝຸດ 010203分布式文件系统
利用网络资源,将数据分 散存储在多台独立的设备 上,实现数据的冗余备份 和负载均衡。
分布式数据库
通过对车辆行驶数据和驾驶员操作数据的分析, 对驾驶行为进行评估和优化,帮助驾驶员提高驾 驶技能和安全意识。
KDD CUP 2004_数据挖掘_科研数据集
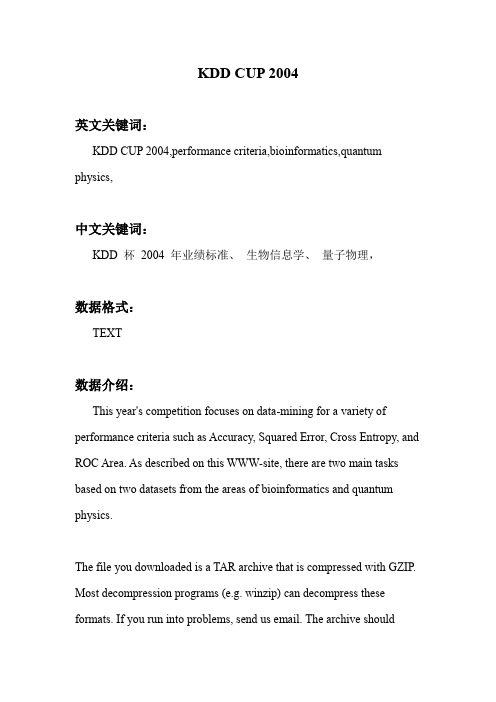
KDD CUP 2004英文关键词:KDD CUP 2004,performance criteria,bioinformatics,quantum physics,中文关键词:KDD 杯2004 年业绩标准、生物信息学、量子物理,数据格式:TEXT数据介绍:This year's competition focuses on data-mining for a variety of performance criteria such as Accuracy, Squared Error, Cross Entropy, and ROC Area. As described on this WWW-site, there are two main tasks based on two datasets from the areas of bioinformatics and quantum physics.The file you downloaded is a TAR archive that is compressed with GZIP. Most decompression programs (e.g. winzip) can decompress these formats. If you run into problems, send us email. The archive shouldcontain four files:phy_train.dat: Training data for the quantum physics task (50,000 train cases)phy_test.dat: Test data for the quantum physics task (100,000 test cases) bio_train.dat: Training data for the protein homology task (145,751 lines) bio_test.dat: Test data for the protein homology task (139,658 lines)The file formats for the two tasks are as follows.Format of the Quantum Physics DatasetEach line in the training and the test file describes one example. The structure of each line is as follows:The first element of each line is an EXAMPLE ID that uniquely describes the example. You will need this EXAMPLE ID when you submit results. The second element is the class of the example. Positive examples are denoted by 1, negative examples by 0. Test examples have a "?" in this position. This is a balanced problem so the target values are roughly half 0's and 1's.All following elements are feature values. There are 78 feature values in each line.Missing values: columns 22,23,24 and 46,47,48 use a value of "999" to denote "not available", and columns 31 and 57 use "9999" to denote "not available". These are the column numbers in the data tables starting with 1 for the first column (the case ID numbers). If you remove the first twocolumns (the case ID numbers and the targets), and start numbering the columns at the first attribute, these are attributes 20,21,22, and 44,45,46, and 29 and 55, respectively. You may treat missing values any way you want, including coding them as a unique value, imputing missing values, using learning methods that can handle missing values, ignoring these attributes, etc.The elements in each line are separated by whitespace.Format of the Protein Homology DatasetEach line in the training and the test file describes one example. The structure of each line is as follows:The first element of each line is a BLOCK ID that denotes to which native sequence this example belongs. There is a unique BLOCK ID for each native sequence. BLOCK IDs are integers running from 1 to 303 (one for each native sequence, i.e. for each query). BLOCK IDs were assigned before the blocks were split into the train and test sets, so they do not run consecutively in either file.The second element of each line is an EXAMPLE ID that uniquely describes the example. You will need this EXAMPLE ID and the BLOCK ID when you submit results.The third element is the class of the example. Proteins that are homologous to the native sequence are denoted by 1, non-homologous proteins (i.e. decoys) by 0. Test examples have a "?" in this position.All following elements are feature values. There are 74 feature values in each line. The features describe the match (e.g. the score of a sequence alignment) between the native protein sequence and the sequence that is tested for homology.There are no missing values (that we know of) in the protein data.To give an example, the first line in bio_train.dat looks like this:279 261532 0 52.00 32.69 ... -0.350 0.26 0.76279 is the BLOCK ID. 261532 is the EXAMPLE ID. The "0" in the third column is the target value. This indicates that this protein is not homologous to the native sequence (it is a decoy). If this protein was homologous the target would be "1". Columns 4-77 are the input attributes.The elements in each line are separated by whitespace.[以下内容来由机器自动翻译]今年的比赛重点的性能标准精度、平方错误、交叉熵和中华民国区等各种数据挖掘。
基于python的汽车行业大数据分析系统的设计与实现

基于python的汽车行业大数据分析系统的设计与实现
设计思路:
1. 数据采集:使用爬虫技术从汽车网站或其他渠道爬取相关数据。
(注意遵守爬虫规范和法律法规)
2. 数据清洗:对采集到的数据进行清洗和去重处理,确保数据的质量和可靠性。
3. 数据存储:将清洗后的数据存储至数据库中(MySQL、MongoDB等),方便后续对数据进行分析和处理。
4. 数据分析:使用Python数据分析相关库(如pandas、numpy、matplotlib等)对数据进行分析,提取有用信息,进行数据建模和预测等。
5. 可视化呈现:使用Python可视化相关库(如seaborn、plotly等)对分析结果进行可视化呈现,方便用户理解和使用。
具体实现步骤:
1. 首先根据需求确定所需要采集的数据,设计爬虫程序,在不影响网站正常运营的情况下爬取到所需数据。
2. 对采集到的数据进行清洗,按照具体业务需求进行处理,如去除重复数据、空值处理等。
3. 将清洗后的数据存储至数据库中,以便后续进行分析处理。
4. 根据所需业务需求,使用Python数据分析相关库对数据进行分析处理,如特征提取、分类、聚类等。
5. 将分析结果使用Python可视化相关库进行呈现,如生成折线图、散点图、热力图等。
需要注意的事项:
1. 遵循爬虫规范和法律法规,不违反相关法律规定;
2. 数据隐私和信息安全保护,确保用户隐私安全;
3. 代码的可读性和可扩展性,方便后续的维护和迭代;
4. 根据业务需求和用户反馈,不断进行优化和改进。
深度学习与自动驾驶领域的数据集(KITTI,Oxford,Cityscape,Comma.。。。

深度学习与⾃动驾驶领域的数据集(KITTI,Oxford,Cityscape,Comma.。
TorontocityHCImiddleburycaltech ⾏⼈检测数据集ISPRS航拍数据集mot challenge跟踪数据集数据集名称KITTI很知名的数据集Oxford RobotCar对⽜津的⼀部分连续的道路进⾏了上百次数据采集,收集到了多种天⽓、⾏⼈和交通情况下的数据,也有建筑和道路施⼯时的数据。
1000⼩时以上。
Cityscape⼀个⾯向城市道路街景语义理解的数据集Comma.aigeohot创办的comma.ai的数据集,80G左右Udacity也有模拟器BDDVBerkeley的⼤规模⾃动驾驶视频数据集GTAgrand theft auto游戏TORCSThe Open Racing Car SimulatorCARLAIntel和丰⽥共同推出的⼀个开源的模拟器KITTI论⽂链接Oxford RobotCar论⽂链接Over the period of May 2014 to December 2015 we traversed a route through central Oxford twice a week on average using the Oxford RobotCar platform, an autonomous Nissan LEAF. This resulted in over 1000km of recorded driving with almost 20 million images collected from 6 cameras mounted to the vehicle, along with LIDAR, GPS and INS ground truth.数据集采集时⾛过的路线:Data was collected in all weather conditions, including heavy rain, night, direct sunlight and snow. Road and building works over the period of a year significantly changed sections of the route from the beginning to the end of data collection.By frequently traversing the same route over the period of a year we enable research investigating long-term localisation and mapping for autonomous vehicles in real-world, dynamic urban environments.在不同天⽓、光线情况和交通状况下的数据集中的⽰例图:Cityscape论⽂链接Comma.ai论⽂链接论⽂中对于数据集的描述如下UdacityUdacity为其⾃动驾驶算法⽐赛专门准备的数据集The dataset includes driving in Mountain View California and neighboring cities during daylight conditions. It contains over 65,000 labels across 9,423 frames collected from a Point Grey research cameras running at full resolution of 1920x1200 at 2hz. The dataset was annotated by CrowdAI using a combination of machine learning and humans. LabelsCarTruckPedestrianThis dataset is similar to dataset 1 but contains additional fields for occlusion and an additional label for traffic lights. The dataset was annotated entirely by humans using Autti and is slightly larger with 15,000 frames.LabelsCarTruckPedestrianStreet LightsBDDV论⽂链接Berkeley的deepdrive研究组的⽤于⾃动驾驶的⼤规模数据集。
汽车研究数据分析报告(3篇)
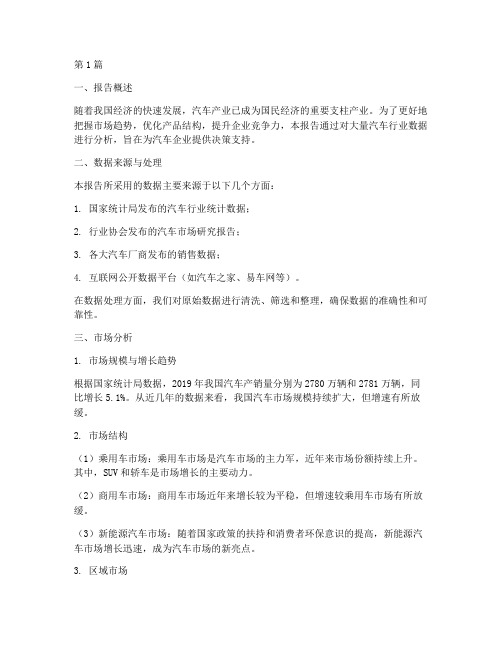
第1篇一、报告概述随着我国经济的快速发展,汽车产业已成为国民经济的重要支柱产业。
为了更好地把握市场趋势,优化产品结构,提升企业竞争力,本报告通过对大量汽车行业数据进行分析,旨在为汽车企业提供决策支持。
二、数据来源与处理本报告所采用的数据主要来源于以下几个方面:1. 国家统计局发布的汽车行业统计数据;2. 行业协会发布的汽车市场研究报告;3. 各大汽车厂商发布的销售数据;4. 互联网公开数据平台(如汽车之家、易车网等)。
在数据处理方面,我们对原始数据进行清洗、筛选和整理,确保数据的准确性和可靠性。
三、市场分析1. 市场规模与增长趋势根据国家统计局数据,2019年我国汽车产销量分别为2780万辆和2781万辆,同比增长5.1%。
从近几年的数据来看,我国汽车市场规模持续扩大,但增速有所放缓。
2. 市场结构(1)乘用车市场:乘用车市场是汽车市场的主力军,近年来市场份额持续上升。
其中,SUV和轿车是市场增长的主要动力。
(2)商用车市场:商用车市场近年来增长较为平稳,但增速较乘用车市场有所放缓。
(3)新能源汽车市场:随着国家政策的扶持和消费者环保意识的提高,新能源汽车市场增长迅速,成为汽车市场的新亮点。
3. 区域市场(1)东部地区:东部地区经济发达,汽车消费需求旺盛,市场份额较大。
(2)中部地区:中部地区汽车市场发展迅速,增速较快。
(3)西部地区:西部地区汽车市场发展潜力巨大,但市场占有率相对较低。
四、竞争格局1. 企业集中度近年来,我国汽车行业集中度有所提高,主要表现为大型企业市场份额扩大。
如上汽、一汽、东风等企业市场份额持续上升。
2. 产品竞争(1)技术竞争:汽车企业纷纷加大研发投入,提高产品技术水平,以提升市场竞争力。
(2)品牌竞争:汽车品牌竞争激烈,企业通过品牌建设、营销推广等方式争夺市场份额。
(3)价格竞争:在市场竞争加剧的背景下,部分企业通过降价促销来提高市场份额。
五、政策环境1. 产业政策近年来,国家出台了一系列产业政策,支持汽车产业发展。
机器学习_Automobile Data Set(汽车数据集)
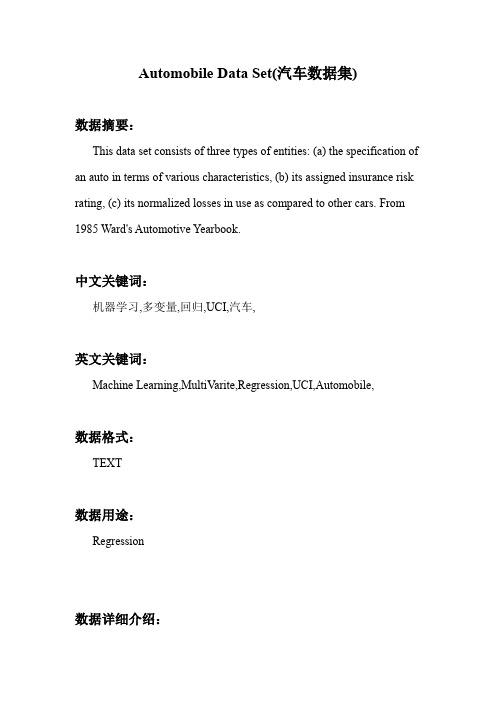
Automobile Data Set(汽车数据集)数据摘要:This data set consists of three types of entities: (a) the specification of an auto in terms of various characteristics, (b) its assigned insurance risk rating, (c) its normalized losses in use as compared to other cars. From 1985 Ward's Automotive Yearbook.中文关键词:机器学习,多变量,回归,UCI,汽车,英文关键词:Machine Learning,MultiVarite,Regression,UCI,Automobile,数据格式:TEXT数据用途:Regression数据详细介绍:Automobile Data SetAbstract: From 1985 Ward's Automotive YearbookSource:Creator/Donor:Jeffrey C. Schlimmer (Jeffrey.Schlimmer '@' )Sources:1) 1985 Model Import Car and Truck Specifications, 1985 Ward's Automotive Yearbook.2) Personal Auto Manuals, Insurance Services Office, 160 Water Street, New York, NY 100383) Insurance Collision Report, Insurance Institute for Highway Safety, Watergate 600, Washington, DC 20037Data Set Information:This data set consists of three types of entities: (a) the specification of an auto in terms of various characteristics, (b) its assigned insurance risk rating, (c) its normalized losses in use as compared to other cars. The second rating corresponds to the degree to which the auto is more risky than its price indicates. Cars are initially assigned a risk factor symbol associated with its price. Then, if it is more risky (or less), this symbol is adjusted by moving it up (or down) the scale. Actuarians call this process "symboling". A value of +3 indicates that the auto is risky, -3 that it is probably pretty safe.The third factor is the relative average loss payment per insured vehicle year. This value is normalized for all autos within a particular size classification (two-door small, station wagons, sports/speciality, etc...), and represents the average loss per car per year.Note: Several of the attributes in the database could be used as a "class" attribute.Attribute Information:Attribute: Attribute Range1. symboling: -3, -2, -1, 0, 1, 2, 3.2. normalized-losses: continuous from 65 to 256.3. make:alfa-romero, audi, bmw, chevrolet, dodge, honda,isuzu, jaguar, mazda, mercedes-benz, mercury,mitsubishi, nissan, peugot, plymouth, porsche,renault, saab, subaru, toyota, volkswagen, volvo4. fuel-type: diesel, gas.5. aspiration: std, turbo.6. num-of-doors: four, two.7. body-style: hardtop, wagon, sedan, hatchback, convertible.8. drive-wheels: 4wd, fwd, rwd.9. engine-location: front, rear.10. wheel-base: continuous from 86.6 120.9.11. length: continuous from 141.1 to 208.1.12. width: continuous from 60.3 to 72.3.13. height: continuous from 47.8 to 59.8.14. curb-weight: continuous from 1488 to 4066.15. engine-type: dohc, dohcv, l, ohc, ohcf, ohcv, rotor.16. num-of-cylinders: eight, five, four, six, three, twelve, two.17. engine-size: continuous from 61 to 326.18. fuel-system: 1bbl, 2bbl, 4bbl, idi, mfi, mpfi, spdi, spfi.19. bore: continuous from 2.54 to 3.94.20. stroke: continuous from 2.07 to 4.17.21. compression-ratio: continuous from 7 to 23.22. horsepower: continuous from 48 to 288.23. peak-rpm: continuous from 4150 to 6600.24. city-mpg: continuous from 13 to 49.25. highway-mpg: continuous from 16 to 54.26. price: continuous from 5118 to 45400.Relevant Papers:Kibler, D., Aha, D.W., & Albert,M. (1989). Instance-based prediction of real-valued attributes. Computational Intelligence, Vol 5, 51--57.[Web Link]数据预览:3,?,alfa-romero,gas,std,two,convertible,rwd,front,88.60,168.80,64.10, 48.80,2548,dohc,four,130,mpfi,3.47,2.68,9.00,111,5000,21,27,13495 3,?,alfa-romero,gas,std,two,convertible,rwd,front,88.60,168.80,64.10, 48.80,2548,dohc,four,130,mpfi,3.47,2.68,9.00,111,5000,21,27,16500 1,?,alfa-romero,gas,std,two,hatchback,rwd,front,94.50,171.20,65.50,52 .40,2823,ohcv,six,152,mpfi,2.68,3.47,9.00,154,5000,19,26,165002,164,audi,gas,std,four,sedan,fwd,front,99.80,176.60,66.20,54.30,2337 ,ohc,four,109,mpfi,3.19,3.40,10.00,102,5500,24,30,139502,164,audi,gas,std,four,sedan,4wd,front,99.40,176.60,66.40,54.30,2824 ,ohc,five,136,mpfi,3.19,3.40,8.00,115,5500,18,22,174502,?,audi,gas,std,two,sedan,fwd,front,99.80,177.30,66.30,53.10,2507,oh c,five,136,mpfi,3.19,3.40,8.50,110,5500,19,25,152501,158,audi,gas,std,four,sedan,fwd,front,105.80,192.70,71.40,55.70,284 4,ohc,five,136,mpfi,3.19,3.40,8.50,110,5500,19,25,177101,?,audi,gas,std,four,wagon,fwd,front,105.80,192.70,71.40,55.70,2954, ohc,five,136,mpfi,3.19,3.40,8.50,110,5500,19,25,189201,158,audi,gas,turbo,four,sedan,fwd,front,105.80,192.70,71.40,55.90,3 086,ohc,five,131,mpfi,3.13,3.40,8.30,140,5500,17,20,238750,?,audi,gas,turbo,two,hatchback,4wd,front,99.50,178.20,67.90,52.00,3 053,ohc,five,131,mpfi,3.13,3.40,7.00,160,5500,16,22,?2,192,bmw,gas,std,two,sedan,rwd,front,101.20,176.80,64.80,54.30,2395, ohc,four,108,mpfi,3.50,2.80,8.80,101,5800,23,29,164300,192,bmw,gas,std,four,sedan,rwd,front,101.20,176.80,64.80,54.30,2395 ,ohc,four,108,mpfi,3.50,2.80,8.80,101,5800,23,29,169250,188,bmw,gas,std,two,sedan,rwd,front,101.20,176.80,64.80,54.30,2710, ohc,six,164,mpfi,3.31,3.19,9.00,121,4250,21,28,209700,188,bmw,gas,std,four,sedan,rwd,front,101.20,176.80,64.80,54.30,2765 ,ohc,six,164,mpfi,3.31,3.19,9.00,121,4250,21,28,211051,?,bmw,gas,std,four,sedan,rwd,front,103.50,189.00,66.90,55.70,3055,o hc,six,164,mpfi,3.31,3.19,9.00,121,4250,20,25,245650,?,bmw,gas,std,four,sedan,rwd,front,103.50,189.00,66.90,55.70,3230,o hc,six,209,mpfi,3.62,3.39,8.00,182,5400,16,22,307600,?,bmw,gas,std,two,sedan,rwd,front,103.50,193.80,67.90,53.70,3380,oh c,six,209,mpfi,3.62,3.39,8.00,182,5400,16,22,41315hc,six,209,mpfi,3.62,3.39,8.00,182,5400,15,20,368802,121,chevrolet,gas,std,two,hatchback,fwd,front,88.40,141.10,60.30,53 .20,1488,l,three,61,2bbl,2.91,3.03,9.50,48,5100,47,53,51511,98,chevrolet,gas,std,two,hatchback,fwd,front,94.50,155.90,63.60,52. 00,1874,ohc,four,90,2bbl,3.03,3.11,9.60,70,5400,38,43,62950,81,chevrolet,gas,std,four,sedan,fwd,front,94.50,158.80,63.60,52.00, 1909,ohc,four,90,2bbl,3.03,3.11,9.60,70,5400,38,43,65751,118,dodge,gas,std,two,hatchback,fwd,front,93.70,157.30,63.80,50.80, 1876,ohc,four,90,2bbl,2.97,3.23,9.41,68,5500,37,41,55721,118,dodge,gas,std,two,hatchback,fwd,front,93.70,157.30,63.80,50.80, 1876,ohc,four,90,2bbl,2.97,3.23,9.40,68,5500,31,38,63771,118,dodge,gas,turbo,two,hatchback,fwd,front,93.70,157.30,63.80,50.8 0,2128,ohc,four,98,mpfi,3.03,3.39,7.60,102,5500,24,30,79571,148,dodge,gas,std,four,hatchback,fwd,front,93.70,157.30,63.80,50.60 ,1967,ohc,four,90,2bbl,2.97,3.23,9.40,68,5500,31,38,62291,148,dodge,gas,std,four,sedan,fwd,front,93.70,157.30,63.80,50.60,198 9,ohc,four,90,2bbl,2.97,3.23,9.40,68,5500,31,38,66921,148,dodge,gas,std,four,sedan,fwd,front,93.70,157.30,63.80,50.60,198 9,ohc,four,90,2bbl,2.97,3.23,9.40,68,5500,31,38,76091,148,dodge,gas,turbo,?,sedan,fwd,front,93.70,157.30,63.80,50.60,2191 ,ohc,four,98,mpfi,3.03,3.39,7.60,102,5500,24,30,8558-1,110,dodge,gas,std,four,wagon,fwd,front,103.30,174.60,64.60,59.80,2 535,ohc,four,122,2bbl,3.34,3.46,8.50,88,5000,24,30,89213,145,dodge,gas,turbo,two,hatchback,fwd,front,95.90,173.20,66.30,50.2 0,2811,ohc,four,156,mfi,3.60,3.90,7.00,145,5000,19,24,129642,137,honda,gas,std,two,hatchback,fwd,front,86.60,144.60,63.90,50.80, 1713,ohc,four,92,1bbl,2.91,3.41,9.60,58,4800,49,54,64792,137,honda,gas,std,two,hatchback,fwd,front,86.60,144.60,63.90,50.80, 1819,ohc,four,92,1bbl,2.91,3.41,9.20,76,6000,31,38,68551,101,honda,gas,std,two,hatchback,fwd,front,93.70,150.00,64.00,52.60, 1837,ohc,four,79,1bbl,2.91,3.07,10.10,60,5500,38,42,53991,101,honda,gas,std,two,hatchback,fwd,front,93.70,150.00,64.00,52.60, 1940,ohc,four,92,1bbl,2.91,3.41,9.20,76,6000,30,34,65291,101,honda,gas,std,two,hatchback,fwd,front,93.70,150.00,64.00,52.60, 1956,ohc,four,92,1bbl,2.91,3.41,9.20,76,6000,30,34,71290,110,honda,gas,std,four,sedan,fwd,front,96.50,163.40,64.00,54.50,201 0,ohc,four,92,1bbl,2.91,3.41,9.20,76,6000,30,34,72950,78,honda,gas,std,four,wagon,fwd,front,96.50,157.10,63.90,58.30,2024 ,ohc,four,92,1bbl,2.92,3.41,9.20,76,6000,30,34,72950,106,honda,gas,std,two,hatchback,fwd,front,96.50,167.50,65.20,53.30, 2236,ohc,four,110,1bbl,3.15,3.58,9.00,86,5800,27,33,78950,106,honda,gas,std,two,hatchback,fwd,front,96.50,167.50,65.20,53.30, 2289,ohc,four,110,1bbl,3.15,3.58,9.00,86,5800,27,33,9095,ohc,four,110,1bbl,3.15,3.58,9.00,86,5800,27,33,88450,85,honda,gas,std,four,sedan,fwd,front,96.50,175.40,62.50,54.10,2372 ,ohc,four,110,1bbl,3.15,3.58,9.00,86,5800,27,33,102950,85,honda,gas,std,four,sedan,fwd,front,96.50,175.40,65.20,54.10,2465 ,ohc,four,110,mpfi,3.15,3.58,9.00,101,5800,24,28,129451,107,honda,gas,std,two,sedan,fwd,front,96.50,169.10,66.00,51.00,2293 ,ohc,four,110,2bbl,3.15,3.58,9.10,100,5500,25,31,103450,?,isuzu,gas,std,four,sedan,rwd,front,94.30,170.70,61.80,53.50,2337, ohc,four,111,2bbl,3.31,3.23,8.50,78,4800,24,29,67851,?,isuzu,gas,std,two,sedan,fwd,front,94.50,155.90,63.60,52.00,1874,o hc,four,90,2bbl,3.03,3.11,9.60,70,5400,38,43,?0,?,isuzu,gas,std,four,sedan,fwd,front,94.50,155.90,63.60,52.00,1909, ohc,four,90,2bbl,3.03,3.11,9.60,70,5400,38,43,?2,?,isuzu,gas,std,two,hatchback,rwd,front,96.00,172.60,65.20,51.40,27 34,ohc,four,119,spfi,3.43,3.23,9.20,90,5000,24,29,110480,145,jaguar,gas,std,four,sedan,rwd,front,113.00,199.60,69.60,52.80,4 066,dohc,six,258,mpfi,3.63,4.17,8.10,176,4750,15,19,322500,?,jaguar,gas,std,four,sedan,rwd,front,113.00,199.60,69.60,52.80,406 6,dohc,six,258,mpfi,3.63,4.17,8.10,176,4750,15,19,355500,?,jaguar,gas,std,two,sedan,rwd,front,102.00,191.70,70.60,47.80,3950 ,ohcv,twelve,326,mpfi,3.54,2.76,11.50,262,5000,13,17,360001,104,mazda,gas,std,two,hatchback,fwd,front,93.10,159.10,64.20,54.10, 1890,ohc,four,91,2bbl,3.03,3.15,9.00,68,5000,30,31,51951,104,mazda,gas,std,two,hatchback,fwd,front,93.10,159.10,64.20,54.10, 1900,ohc,four,91,2bbl,3.03,3.15,9.00,68,5000,31,38,60951,104,mazda,gas,std,two,hatchback,fwd,front,93.10,159.10,64.20,54.10, 1905,ohc,four,91,2bbl,3.03,3.15,9.00,68,5000,31,38,67951,113,mazda,gas,std,four,sedan,fwd,front,93.10,166.80,64.20,54.10,194 5,ohc,four,91,2bbl,3.03,3.15,9.00,68,5000,31,38,66951,113,mazda,gas,std,four,sedan,fwd,front,93.10,166.80,64.20,54.10,195 0,ohc,four,91,2bbl,3.08,3.15,9.00,68,5000,31,38,73953,150,mazda,gas,std,two,hatchback,rwd,front,95.30,169.00,65.70,49.60, 2380,rotor,two,70,4bbl,?,?,9.40,101,6000,17,23,109453,150,mazda,gas,std,two,hatchback,rwd,front,95.30,169.00,65.70,49.60, 2380,rotor,two,70,4bbl,?,?,9.40,101,6000,17,23,118453,150,mazda,gas,std,two,hatchback,rwd,front,95.30,169.00,65.70,49.60, 2385,rotor,two,70,4bbl,?,?,9.40,101,6000,17,23,136453,150,mazda,gas,std,two,hatchback,rwd,front,95.30,169.00,65.70,49.60, 2500,rotor,two,80,mpfi,?,?,9.40,135,6000,16,23,156451,129,mazda,gas,std,two,hatchback,fwd,front,98.80,177.80,66.50,53.70, 2385,ohc,four,122,2bbl,3.39,3.39,8.60,84,4800,26,32,88450,115,mazda,gas,std,four,sedan,fwd,front,98.80,177.80,66.50,55.50,241 0,ohc,four,122,2bbl,3.39,3.39,8.60,84,4800,26,32,84951,129,mazda,gas,std,two,hatchback,fwd,front,98.80,177.80,66.50,53.70, 2385,ohc,four,122,2bbl,3.39,3.39,8.60,84,4800,26,32,105950,115,mazda,gas,std,four,sedan,fwd,front,98.80,177.80,66.50,55.50,241 0,ohc,four,122,2bbl,3.39,3.39,8.60,84,4800,26,32,102450,?,mazda,diesel,std,?,sedan,fwd,front,98.80,177.80,66.50,55.50,2443, ohc,four,122,idi,3.39,3.39,22.70,64,4650,36,42,107950,115,mazda,gas,std,four,hatchback,fwd,front,98.80,177.80,66.50,55.50 ,2425,ohc,four,122,2bbl,3.39,3.39,8.60,84,4800,26,32,112450,118,mazda,gas,std,four,sedan,rwd,front,104.90,175.00,66.10,54.40,26 70,ohc,four,140,mpfi,3.76,3.16,8.00,120,5000,19,27,182800,?,mazda,diesel,std,four,sedan,rwd,front,104.90,175.00,66.10,54.40,2 700,ohc,four,134,idi,3.43,3.64,22.00,72,4200,31,39,18344-1,93,mercedes-benz,diesel,turbo,four,sedan,rwd,front,110.00,190.90,7 0.30,56.50,3515,ohc,five,183,idi,3.58,3.64,21.50,123,4350,22,25,25552 -1,93,mercedes-benz,diesel,turbo,four,wagon,rwd,front,110.00,190.90,7 0.30,58.70,3750,ohc,five,183,idi,3.58,3.64,21.50,123,4350,22,25,28248 0,93,mercedes-benz,diesel,turbo,two,hardtop,rwd,front,106.70,187.50,7 0.30,54.90,3495,ohc,five,183,idi,3.58,3.64,21.50,123,4350,22,25,28176 -1,93,mercedes-benz,diesel,turbo,four,sedan,rwd,front,115.60,202.60,7 1.70,56.30,3770,ohc,five,183,idi,3.58,3.64,21.50,123,4350,22,25,31600 -1,?,mercedes-benz,gas,std,four,sedan,rwd,front,115.60,202.60,71.70,5 6.50,3740,ohcv,eight,234,mpfi,3.46,3.10,8.30,155,4750,16,18,341843,142,mercedes-benz,gas,std,two,convertible,rwd,front,96.60,180.30,70 .50,50.80,3685,ohcv,eight,234,mpfi,3.46,3.10,8.30,155,4750,16,18,3505 60,?,mercedes-benz,gas,std,four,sedan,rwd,front,120.90,208.10,71.70,56 .70,3900,ohcv,eight,308,mpfi,3.80,3.35,8.00,184,4500,14,16,40960 1,?,mercedes-benz,gas,std,two,hardtop,rwd,front,112.00,199.20,72.00,5 5.40,3715,ohcv,eight,304,mpfi,3.80,3.35,8.00,184,4500,14,16,45400 1,?,mercury,gas,turbo,two,hatchback,rwd,front,102.70,178.40,68.00,54. 80,2910,ohc,four,140,mpfi,3.78,3.12,8.00,175,5000,19,24,165032,161,mitsubishi,gas,std,two,hatchback,fwd,front,93.70,157.30,64.40,5 0.80,1918,ohc,four,92,2bbl,2.97,3.23,9.40,68,5500,37,41,53892,161,mitsubishi,gas,std,two,hatchback,fwd,front,93.70,157.30,64.40,5 0.80,1944,ohc,four,92,2bbl,2.97,3.23,9.40,68,5500,31,38,61892,161,mitsubishi,gas,std,two,hatchback,fwd,front,93.70,157.30,64.40,5 0.80,2004,ohc,four,92,2bbl,2.97,3.23,9.40,68,5500,31,38,66691,161,mitsubishi,gas,turbo,two,hatchback,fwd,front,93,157.30,63.80,50 .80,2145,ohc,four,98,spdi,3.03,3.39,7.60,102,5500,24,30,76893,153,mitsubishi,gas,turbo,two,hatchback,fwd,front,96.30,173.00,65.40 ,49.40,2370,ohc,four,110,spdi,3.17,3.46,7.50,116,5500,23,30,99593,153,mitsubishi,gas,std,two,hatchback,fwd,front,96.30,173.00,65.40,4 9.40,2328,ohc,four,122,2bbl,3.35,3.46,8.50,88,5000,25,32,84993,?,mitsubishi,gas,turbo,two,hatchback,fwd,front,95.90,173.20,66.30,5 0.20,2833,ohc,four,156,spdi,3.58,3.86,7.00,145,5000,19,24,12629 3,?,mitsubishi,gas,turbo,two,hatchback,fwd,front,95.90,173.20,66.30,5 0.20,2921,ohc,four,156,spdi,3.59,3.86,7.00,145,5000,19,24,14869 3,?,mitsubishi,gas,turbo,two,hatchback,fwd,front,95.90,173.20,66.30,5 0.20,2926,ohc,four,156,spdi,3.59,3.86,7.00,145,5000,19,24,144891,125,mitsubishi,gas,std,four,sedan,fwd,front,96.30,172.40,65.40,51.6 0,2365,ohc,four,122,2bbl,3.35,3.46,8.50,88,5000,25,32,69891,125,mitsubishi,gas,std,four,sedan,fwd,front,96.30,172.40,65.40,51.6 0,2405,ohc,four,122,2bbl,3.35,3.46,8.50,88,5000,25,32,81891,125,mitsubishi,gas,turbo,four,sedan,fwd,front,96.30,172.40,65.40,51 .60,2403,ohc,four,110,spdi,3.17,3.46,7.50,116,5500,23,30,9279-1,137,mitsubishi,gas,std,four,sedan,fwd,front,96.30,172.40,65.40,51. 60,2403,ohc,four,110,spdi,3.17,3.46,7.50,116,5500,23,30,92791,128,nissan,gas,std,two,sedan,fwd,front,94.50,165.30,63.80,54.50,188 9,ohc,four,97,2bbl,3.15,3.29,9.40,69,5200,31,37,54991,128,nissan,diesel,std,two,sedan,fwd,front,94.50,165.30,63.80,54.50, 2017,ohc,four,103,idi,2.99,3.47,21.90,55,4800,45,50,70991,128,nissan,gas,std,two,sedan,fwd,front,94.50,165.30,63.80,54.50,191 8,ohc,four,97,2bbl,3.15,3.29,9.40,69,5200,31,37,66491,122,nissan,gas,std,four,sedan,fwd,front,94.50,165.30,63.80,54.50,19 38,ohc,four,97,2bbl,3.15,3.29,9.40,69,5200,31,37,68491,103,nissan,gas,std,four,wagon,fwd,front,94.50,170.20,63.80,53.50,20 24,ohc,four,97,2bbl,3.15,3.29,9.40,69,5200,31,37,73491,128,nissan,gas,std,two,sedan,fwd,front,94.50,165.30,63.80,54.50,195 1,ohc,four,97,2bbl,3.15,3.29,9.40,69,5200,31,37,72991,128,nissan,gas,std,two,hatchback,fwd,front,94.50,165.60,63.80,53.30 ,2028,ohc,four,97,2bbl,3.15,3.29,9.40,69,5200,31,37,77991,122,nissan,gas,std,four,sedan,fwd,front,94.50,165.30,63.80,54.50,19 71,ohc,four,97,2bbl,3.15,3.29,9.40,69,5200,31,37,74991,103,nissan,gas,std,four,wagon,fwd,front,94.50,170.20,63.80,53.50,20 37,ohc,four,97,2bbl,3.15,3.29,9.40,69,5200,31,37,79992,168,nissan,gas,std,two,hardtop,fwd,front,95.10,162.40,63.80,53.30,2 008,ohc,four,97,2bbl,3.15,3.29,9.40,69,5200,31,37,82490,106,nissan,gas,std,four,hatchback,fwd,front,97.20,173.40,65.20,54.7 0,2324,ohc,four,120,2bbl,3.33,3.47,8.50,97,5200,27,34,89490,106,nissan,gas,std,four,sedan,fwd,front,97.20,173.40,65.20,54.70,23 02,ohc,four,120,2bbl,3.33,3.47,8.50,97,5200,27,34,95490,128,nissan,gas,std,four,sedan,fwd,front,100.40,181.70,66.50,55.10,3 095,ohcv,six,181,mpfi,3.43,3.27,9.00,152,5200,17,22,134990,108,nissan,gas,std,four,wagon,fwd,front,100.40,184.60,66.50,56.10,3 296,ohcv,six,181,mpfi,3.43,3.27,9.00,152,5200,17,22,143990,108,nissan,gas,std,four,sedan,fwd,front,100.40,184.60,66.50,55.10,3 060,ohcv,six,181,mpfi,3.43,3.27,9.00,152,5200,19,25,134993,194,nissan,gas,std,two,hatchback,rwd,front,91.30,170.70,67.90,49.70 ,3071,ohcv,six,181,mpfi,3.43,3.27,9.00,160,5200,19,25,171993,194,nissan,gas,turbo,two,hatchback,rwd,front,91.30,170.70,67.90,49. 70,3139,ohcv,six,181,mpfi,3.43,3.27,7.80,200,5200,17,23,196991,231,nissan,gas,std,two,hatchback,rwd,front,99.20,178.50,67.90,49.70 ,3139,ohcv,six,181,mpfi,3.43,3.27,9.00,160,5200,19,25,183990,161,peugot,gas,std,four,sedan,rwd,front,107.90,186.70,68.40,56.70,3 020,l,four,120,mpfi,3.46,3.19,8.40,97,5000,19,24,119000,161,peugot,diesel,turbo,four,sedan,rwd,front,107.90,186.70,68.40,56 .70,3197,l,four,152,idi,3.70,3.52,21.00,95,4150,28,33,13200点此下载完整数据集。
nuscenes数据解析
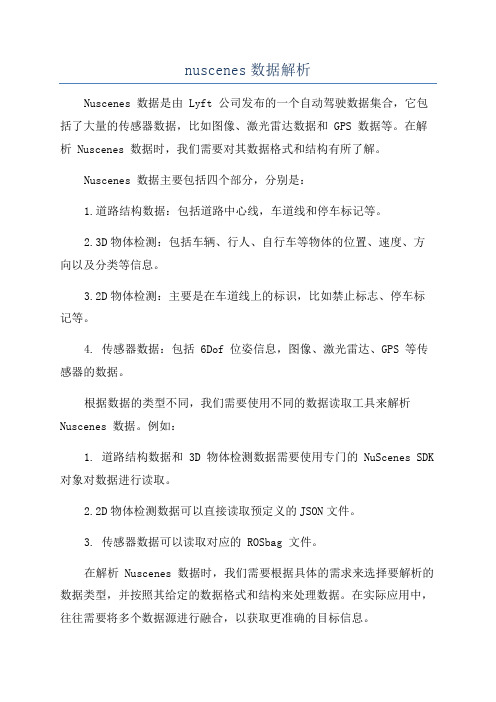
nuscenes数据解析
Nuscenes 数据是由 Lyft 公司发布的一个自动驾驶数据集合,它包括了大量的传感器数据,比如图像、激光雷达数据和 GPS 数据等。
在解析 Nuscenes 数据时,我们需要对其数据格式和结构有所了解。
Nuscenes 数据主要包括四个部分,分别是:
1.道路结构数据:包括道路中心线,车道线和停车标记等。
2.3D物体检测:包括车辆、行人、自行车等物体的位置、速度、方
向以及分类等信息。
3.2D物体检测:主要是在车道线上的标识,比如禁止标志、停车标
记等。
4. 传感器数据:包括 6Dof 位姿信息,图像、激光雷达、GPS 等传感器的数据。
根据数据的类型不同,我们需要使用不同的数据读取工具来解析Nuscenes 数据。
例如:
1. 道路结构数据和 3D 物体检测数据需要使用专门的 NuScenes SDK 对象对数据进行读取。
2.2D物体检测数据可以直接读取预定义的JSON文件。
3. 传感器数据可以读取对应的 ROSbag 文件。
在解析 Nuscenes 数据时,我们需要根据具体的需求来选择要解析的数据类型,并按照其给定的数据格式和结构来处理数据。
在实际应用中,往往需要将多个数据源进行融合,以获取更准确的目标信息。
基于机器学习的车辆大数据分析研究
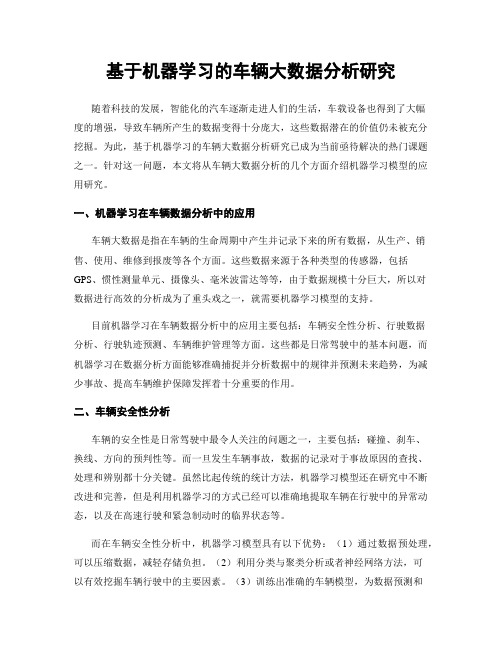
基于机器学习的车辆大数据分析研究随着科技的发展,智能化的汽车逐渐走进人们的生活,车载设备也得到了大幅度的增强,导致车辆所产生的数据变得十分庞大,这些数据潜在的价值仍未被充分挖掘。
为此,基于机器学习的车辆大数据分析研究已成为当前亟待解决的热门课题之一。
针对这一问题,本文将从车辆大数据分析的几个方面介绍机器学习模型的应用研究。
一、机器学习在车辆数据分析中的应用车辆大数据是指在车辆的生命周期中产生并记录下来的所有数据,从生产、销售、使用、维修到报废等各个方面。
这些数据来源于各种类型的传感器,包括GPS、惯性测量单元、摄像头、毫米波雷达等等,由于数据规模十分巨大,所以对数据进行高效的分析成为了重头戏之一,就需要机器学习模型的支持。
目前机器学习在车辆数据分析中的应用主要包括:车辆安全性分析、行驶数据分析、行驶轨迹预测、车辆维护管理等方面。
这些都是日常驾驶中的基本问题,而机器学习在数据分析方面能够准确捕捉并分析数据中的规律并预测未来趋势,为减少事故、提高车辆维护保障发挥着十分重要的作用。
二、车辆安全性分析车辆的安全性是日常驾驶中最令人关注的问题之一,主要包括:碰撞、刹车、换线、方向的预判性等。
而一旦发生车辆事故,数据的记录对于事故原因的查找、处理和辨别都十分关键。
虽然比起传统的统计方法,机器学习模型还在研究中不断改进和完善,但是利用机器学习的方式已经可以准确地提取车辆在行驶中的异常动态,以及在高速行驶和紧急制动时的临界状态等。
而在车辆安全性分析中,机器学习模型具有以下优势:(1)通过数据预处理,可以压缩数据,减轻存储负担。
(2)利用分类与聚类分析或者神经网络方法,可以有效挖掘车辆行驶中的主要因素。
(3)训练出准确的车辆模型,为数据预测和事故预警提供保障。
(4)通过捕捉不同车辆动态,为实现自动化驾驶系统与云处理技术的协同提供数据支撑。
三、行驶数据分析行驶数据是车辆大数据的核心,包括车辆加速度、姿态角、速度、行驶轨迹等信息,更能直观地反映出车辆行驶中的细节和特点。
大数据汽车实验报告

大数据汽车实验报告引言随着科技的不断进步,大数据在各个领域得到了广泛的应用,其中,汽车行业也开始积极探索大数据的应用。
在此次实验中,我们重点关注了大数据在汽车行业中的应用场景和优势,并通过实地调研和数据分析,对相关实验结果进行了总结。
实验目的本次实验的目的是了解大数据在汽车行业中的应用现状和发展趋势,并通过对大数据的采集和分析,探索大数据在汽车企业运营和用户体验方面的优势。
为了达到以上目的,我们进行了如下实验操作:1. 调研现有的大数据应用车型和设备2. 采集汽车行驶过程中的实时数据3. 分析数据,并提取出其中的有用信息4. 根据分析结果,评估大数据在汽车行业的应用优势和潜在问题5. 提出相关建议,用以指导汽车企业进一步利用大数据优化运营和提升用户体验实验过程调研现有的大数据应用车型和设备我们首先调研了当前市场上已经应用大数据的汽车车型和相关设备,如特斯拉的自动驾驶车型、宝马的智能座舱系统等。
通过调研,我们对大数据在汽车行业的应用场景和技术特点有了初步的了解。
采集汽车行驶过程中的实时数据借助现有的汽车电子设备和传感器技术,我们利用预装的数据采集器和GPS设备,实时采集了一辆汽车行驶过程中的相关数据,包括车速、油耗、车辆维护情况等。
分析数据,并提取出其中的有用信息通过对采集到的数据进行处理和分析,我们提取出了一些有用的信息。
例如,通过分析车速和油耗的关系,我们可以评估一个车型的燃油经济性能;通过分析车辆维护数据,可以判断车辆是否需要维修等。
评估大数据在汽车行业的应用优势和潜在问题基于对数据的分析结果,我们评估了大数据在汽车行业中的应用优势和潜在问题。
例如,大数据可以帮助汽车企业更精确地制定营销策略和车辆定价,提高运营效率;但同时也会给个人隐私带来一定的风险和挑战。
提出相关建议最后,我们根据实验结果向汽车企业提出了一些相关的建议。
例如,建议汽车企业进一步整合车辆数据和用户需求,提供个性化的服务和产品;同时,也建议加强数据安全保护工作,保护用户隐私。
汽车数据集(cars dataset)_数据挖掘_科研数据集
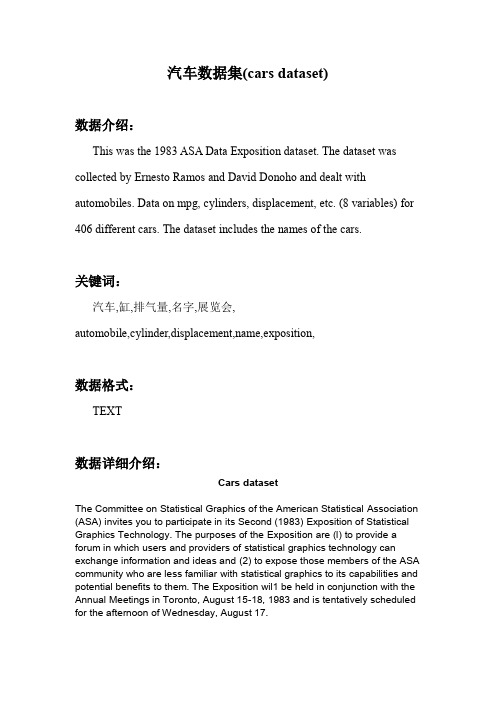
汽车数据集(cars dataset)数据介绍:This was the 1983 ASA Data Exposition dataset. The dataset was collected by Ernesto Ramos and David Donoho and dealt with automobiles. Data on mpg, cylinders, displacement, etc. (8 variables) for 406 different cars. The dataset includes the names of the cars.关键词:汽车,缸,排气量,名字,展览会,automobile,cylinder,displacement,name,exposition,数据格式:TEXT数据详细介绍:Cars datasetThe Committee on Statistical Graphics of the American Statistical Association (ASA) invites you to participate in its Second (1983) Exposition of Statistical Graphics Technology. The purposes of the Exposition are (l) to provide a forum in which users and providers of statistical graphics technology can exchange information and ideas and (2) to expose those members of the ASA community who are less familiar with statistical graphics to its capabilities and potential benefits to them. The Exposition wil1 be held in conjunction with the Annual Meetings in Toronto, August 15-18, 1983 and is tentatively scheduled for the afternoon of Wednesday, August 17.Seven providers of statistical graphics technology participated in the l982 Exposition. By all accounts, the Exposition was well received by the ASA community and was a worthwhile experience for the participants. We hope to have those seven involved again this year, along with as many new participants as we can muster. The 1982 Exposition was summarized in a paper to appear in the Proceeding of the Statistical Computing Section. A copy of that paper is enclosed for your information.The basic format of the 1983 Exposition will be similar to that of 1982. However, based upon comments received and experience gained, there are some changes. The basic structure, intended to be simpler and more flexible than last year, is as follows:A fixed data set is to be analyzed. This data set is a version of the CRCARS data set ofDonoho, David and Ramos, Ernesto (1982), ``PRIMDATA: ata Sets for Use With PRIM-H'' (DRAFT).Because of the Committee's limited (zero) budget for the Exposition, we are forced to provide the data in hardcopy form only (enclosed). (Sorry!)There are 406 observations on the following 8 variables: MPG (miles per gallon), # cylinders, engine displacement (cu. inches), horsepower, vehicle weight (lbs.), time to accelerate from O to 60 mph (sec.), model year (modulo 100), and origin of car (1. American, 2. European,3. Japanese). These data appear on seven pages. Also provided are the car labels (types) in the same order as the 8 variables on seven separate pages. Missing data values are marked by series of question marks.You are asked to analyze these data using your statistical graphics software. Your objective should be to achieve graphical displays which will be meaningful to the viewers and highlight relevant aspects of the data. If you can best achieve this using simple graphical formats,fine. If you choose to illustrate some of the more sophisticated capabilities of your software and can do so without losing relevancy to the data, that is fine, too. This year, there will be no Committee commentary on the individual presentations, so you are not competingwith other presenters. The role of each presenter is to do his/her best job of presenting their statistical graphics technology to the viewers.Each participant will be provided with a 6'(long) by 4'(tall) posterboard on which to display the results of their analyses. This is the same format as last year. You are encouraged to remain by your presentation during the Exposition to answer viewers' questions. Threecopies of your presentation must be submitted to me by July 1. Movie or slide show presentations cannot be accommodated (sorry). The Committee will prepare its own poster presentation which will orient the viewers to the data and the purposes of the Exposition.The ASA has asked us to remind all participants that the Exposition is intended for educational and scientific purposes and is not a marketing activity. Even though last year's participants did an excellent job of maintaining that distinction, a cautionary note at this point is appropriate.Those of us who were involved with the 1982 Exposition found it worthwhile and fun to do. We would very much like to have you participate this year. For planning purposes, please RSVP (to me, in writing please) by April 15 as to whether you plan to accept the Committee's invitation.If you have any questions about the Exposition, please call me on(301/763-5350). If you have specific questions about the data, or the analysis, please call Karen Kafadar on (301/921-3651). If you cannot participate but know of another person or group in your organization who can, please pass this invitation along to them.Sincerely,LAWRENCE H. COXStatistical Research DivisionBureau of the CensusRoom 3524-3Washington, DC 20233数据预览:点此下载完整数据集。
nuTonomy发布自动驾驶数据集nuScenes
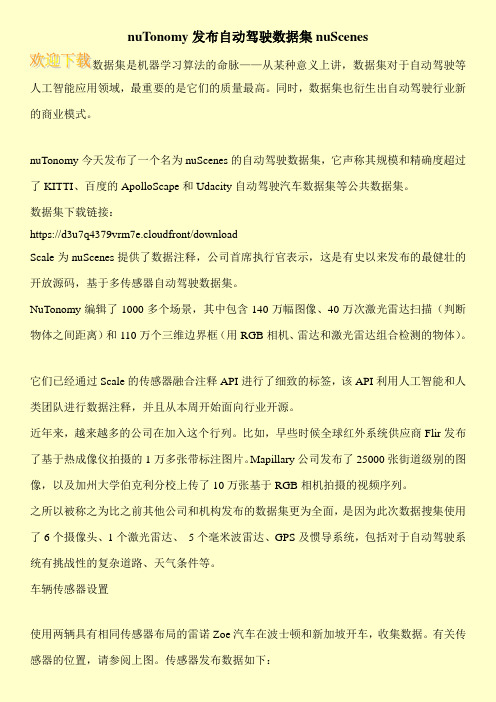
nuTonomy发布自动驾驶数据集nuScenes数据集是机器学习算法的命脉——从某种意义上讲,数据集对于自动驾驶等人工智能应用领域,最重要的是它们的质量最高。
同时,数据集也衍生出自动驾驶行业新的商业模式。
nuTonomy今天发布了一个名为nuScenes的自动驾驶数据集,它声称其规模和精确度超过了KITTI、百度的ApolloScape和Udacity自动驾驶汽车数据集等公共数据集。
数据集下载链接:https://d3u7q4379vrm7e.cloudfront/downloadScale为nuScenes提供了数据注释,公司首席执行官表示,这是有史以来发布的最健壮的开放源码,基于多传感器自动驾驶数据集。
NuTonomy编辑了1000多个场景,其中包含140万幅图像、40万次激光雷达扫描(判断物体之间距离)和110万个三维边界框(用RGB相机、雷达和激光雷达组合检测的物体)。
它们已经通过Scale的传感器融合注释API进行了细致的标签,该API利用人工智能和人类团队进行数据注释,并且从本周开始面向行业开源。
近年来,越来越多的公司在加入这个行列。
比如,早些时候全球红外系统供应商Flir发布了基于热成像仪拍摄的1万多张带标注图片。
Mapillary公司发布了25000张街道级别的图像,以及加州大学伯克利分校上传了10万张基于RGB相机拍摄的视频序列。
之所以被称之为比之前其他公司和机构发布的数据集更为全面,是因为此次数据搜集使用了6个摄像头、1个激光雷达、5个毫米波雷达、GPS及惯导系统,包括对于自动驾驶系统有挑战性的复杂道路、天气条件等。
车辆传感器设置使用两辆具有相同传感器布局的雷诺Zoe汽车在波士顿和新加坡开车,收集数据。
有关传感器的位置,请参阅上图。
传感器发布数据如下:1x旋转激光雷达:5倍长距离雷达传感器:相机方向和重叠请参阅下图:20Hz捕获频率32个频道360°水平视场,+ 10°至-30°垂直视场80m-100m范围,可用返回70米,精度±2厘米每秒高达~139百万点用于77GHz13Hz捕获频率使用调频连续波在一个周期内独立测量距离和速度距离最远250米速度精度为±0.1 km / hsan6x相机:12Hz捕获频率1 / 1.8英寸CMOS传感器,1600x1200分辨率Bayer8格式,每像素1字节编码1600x900 ROI从原始分辨率中裁剪,以减少处理和传输带宽曝光时间限制为最大20 ms的自动曝光图像被解压缩为BGR格式并压缩为JPEG传感器校准要获得高质量的多传感器数据集,必须校准每个传感器的外在和内在因素。
nuscenes数据集解析

nuscenes数据集解析nuscenes数据集解析:从数据集到自动驾驶的未来引言自动驾驶技术一直是科技领域的热门话题,而要实现自动驾驶,就需要大量的高质量数据集来训练和测试算法。
nuscenes数据集就是其中之一,它是用于自动驾驶感知和决策的公开数据集之一。
本文将介绍nuscenes数据集的特点、用途以及对自动驾驶研究的意义。
一、nuscenes数据集的特点1. 数据集规模庞大:nuscenes数据集包含超过140万个带有多传感器数据的样本,其中包括雷达、相机和激光雷达等多种传感器数据。
2. 多样性的场景和环境:数据集涵盖了城市、高速公路、乡村等不同的行驶场景和复杂的交通环境,使得算法可以在各种情况下进行训练和测试。
3. 高质量的标注数据:nuscenes数据集提供了精确的标注信息,包括车辆、行人、自行车等多种道路参与者的位置、速度、方向等信息。
二、nuscenes数据集的用途1. 自动驾驶感知算法的训练和评估:通过nuscenes数据集,研究人员可以训练和评估自动驾驶感知算法,例如目标检测、目标跟踪和语义分割等算法。
2. 自动驾驶决策算法的训练和评估:nuscenes数据集不仅提供了传感器数据,还提供了高质量的标注信息,使得研究人员可以训练和评估自动驾驶决策算法,例如路径规划和行为预测等算法。
3. 自动驾驶系统的性能评估:研究人员可以使用nuscenes数据集来评估自动驾驶系统的性能,包括感知准确度、决策准确度和系统鲁棒性等指标。
三、nuscenes数据集对自动驾驶研究的意义1. 推动自动驾驶技术的发展:nuscenes数据集提供了一个标准的评估平台,可以促进自动驾驶技术的发展和创新。
2. 提供丰富的数据源:通过nuscenes数据集,研究人员可以获取丰富的真实场景数据,从而更好地理解和解决自动驾驶中的各种挑战。
3. 促进算法的迭代和改进:nuscenes数据集可以用于训练和测试算法,并提供了准确的标注信息,使得研究人员可以迭代和改进他们的算法,从而提升自动驾驶系统的性能。
apolloscape trajectory dataset -回复

apolloscape trajectory dataset -回复什么是ApolloScape轨迹数据集?ApolloScape轨迹数据集是一个由中国的阿波罗无人车开发团队发布的数据集,旨在为自动驾驶技术和相关研究提供高质量的视觉和定位数据。
该数据集包含丰富多样的实际驾驶场景,涵盖城市、高速公路和乡村等不同类型道路的驾驶情况。
通过使用ApolloScape轨迹数据集,研究人员可以训练和评估自动驾驶系统,以改进其感知、规划和决策能力。
ApolloScape轨迹数据集的收集是通过无人驾驶汽车在真实环境中进行行驶任务而完成的。
虽然这些任务是由无人驾驶汽车完成的,但数据集中还包括了准确的参考GPS轨迹。
这些GPS轨迹提供了准确的位置和方向信息,使研究人员能够在无人驾驶汽车实际行驶的同时了解其准确位置和姿态。
ApolloScape轨迹数据集的内容非常丰富。
首先,它包含了各种各样的驾驶环境,从城市道路到乡村道路,再到高速公路。
这些不同类型的道路提供了不同的挑战和场景,使研究人员能够更好地评估自动驾驶系统在各种复杂驾驶情况下的表现。
其次,ApolloScape轨迹数据集还提供了多个感知传感器的数据,包括摄像头、激光雷达和毫米波雷达。
这些传感器提供了丰富的环境信息,使自动驾驶系统能够感知其周围环境并做出准确的决策。
除了环境感知,ApolloScape轨迹数据集还提供了高精度地图和相机位姿数据。
这些数据使自动驾驶系统能够更准确地定位自己在地图中的位置,并进行路径规划和决策。
ApolloScape轨迹数据集还包含了大量的标注数据。
这些标注数据包括物体检测、语义分割和实例分割等任务的标注,以及车道线和交通标记的标注。
这些标注数据非常有用,帮助研究人员训练和评估自动驾驶系统在各种任务中的性能。
使用ApolloScape轨迹数据集进行研究和开发有很多优势。
首先,该数据集提供了真实场景中的驾驶数据,使研究人员能够更准确地评估和改进自动驾驶系统的性能。
- 1、下载文档前请自行甄别文档内容的完整性,平台不提供额外的编辑、内容补充、找答案等附加服务。
- 2、"仅部分预览"的文档,不可在线预览部分如存在完整性等问题,可反馈申请退款(可完整预览的文档不适用该条件!)。
- 3、如文档侵犯您的权益,请联系客服反馈,我们会尽快为您处理(人工客服工作时间:9:00-18:30)。
汽车数据集(cars dataset)数据介绍:This was the 1983 ASA Data Exposition dataset. The dataset was collected by Ernesto Ramos and David Donoho and dealt with automobiles. Data on mpg, cylinders, displacement, etc. (8 variables) for 406 different cars. The dataset includes the names of the cars.关键词:汽车,缸,排气量,名字,展览会,automobile,cylinder,displacement,name,exposition,数据格式:TEXT数据详细介绍:Cars datasetThe Committee on Statistical Graphics of the American Statistical Association (ASA) invites you to participate in its Second (1983) Exposition of Statistical Graphics Technology. The purposes of the Exposition are (l) to provide a forum in which users and providers of statistical graphics technology can exchange information and ideas and (2) to expose those members of the ASA community who are less familiar with statistical graphics to its capabilities and potential benefits to them. The Exposition wil1 be held in conjunction with the Annual Meetings in Toronto, August 15-18, 1983 and is tentatively scheduled for the afternoon of Wednesday, August 17.Seven providers of statistical graphics technology participated in the l982 Exposition. By all accounts, the Exposition was well received by the ASA community and was a worthwhile experience for the participants. We hope to have those seven involved again this year, along with as many new participants as we can muster. The 1982 Exposition was summarized in a paper to appear in the Proceeding of the Statistical Computing Section. A copy of that paper is enclosed for your information.The basic format of the 1983 Exposition will be similar to that of 1982. However, based upon comments received and experience gained, there are some changes. The basic structure, intended to be simpler and more flexible than last year, is as follows:A fixed data set is to be analyzed. This data set is a version of the CRCARS data set ofDonoho, David and Ramos, Ernesto (1982), ``PRIMDATA: ata Sets for Use With PRIM-H'' (DRAFT).Because of the Committee's limited (zero) budget for the Exposition, we are forced to provide the data in hardcopy form only (enclosed). (Sorry!)There are 406 observations on the following 8 variables: MPG (miles per gallon), # cylinders, engine displacement (cu. inches), horsepower, vehicle weight (lbs.), time to accelerate from O to 60 mph (sec.), model year (modulo 100), and origin of car (1. American, 2. European,3. Japanese). These data appear on seven pages. Also provided are the car labels (types) in the same order as the 8 variables on seven separate pages. Missing data values are marked by series of question marks.You are asked to analyze these data using your statistical graphics software. Your objective should be to achieve graphical displays which will be meaningful to the viewers and highlight relevant aspects of the data. If you can best achieve this using simple graphical formats,fine. If you choose to illustrate some of the more sophisticated capabilities of your software and can do so without losing relevancy to the data, that is fine, too. This year, there will be no Committee commentary on the individual presentations, so you are not competingwith other presenters. The role of each presenter is to do his/her best job of presenting their statistical graphics technology to the viewers.Each participant will be provided with a 6'(long) by 4'(tall) posterboard on which to display the results of their analyses. This is the same format as last year. You are encouraged to remain by your presentation during the Exposition to answer viewers' questions. Threecopies of your presentation must be submitted to me by July 1. Movie or slide show presentations cannot be accommodated (sorry). The Committee will prepare its own poster presentation which will orient the viewers to the data and the purposes of the Exposition.The ASA has asked us to remind all participants that the Exposition is intended for educational and scientific purposes and is not a marketing activity. Even though last year's participants did an excellent job of maintaining that distinction, a cautionary note at this point is appropriate.Those of us who were involved with the 1982 Exposition found it worthwhile and fun to do. We would very much like to have you participate this year. For planning purposes, please RSVP (to me, in writing please) by April 15 as to whether you plan to accept the Committee's invitation.If you have any questions about the Exposition, please call me on(301/763-5350). If you have specific questions about the data, or the analysis, please call Karen Kafadar on (301/921-3651). If you cannot participate but know of another person or group in your organization who can, please pass this invitation along to them.Sincerely,LAWRENCE H. COXStatistical Research DivisionBureau of the CensusRoom 3524-3Washington, DC 20233数据预览:点此下载完整数据集。