频谱感知技术外文翻译文献
无线电频谱感知技术研究

无线电频谱感知技术研究无线电频谱感知技术(Radio spectrum sensing technology)是一种能够对当前环境中的无线电频谱进行实时监测和感知的技术。
在无线通信系统中,频谱是一种十分重要的资源,但是由于无线电频谱的有限性和复杂性,频谱的利用效率一直是无线通信系统的瓶颈之一。
因此,频谱感知技术的使用对于提高无线通信系统的频谱利用效率和性能至关重要。
频谱感知技术的主要目标是通过对无线电频谱进行实时监测和分析,以获得当前环境下的频谱使用情况。
通过感知到的频谱信息,无线通信系统可以根据实际情况进行频谱资源的分配和管理,避免频谱的冲突和争夺,提高系统的容量和可靠性。
频谱感知技术主要包括两个关键环节:频谱检测和频谱识别。
频谱检测是指通过对频谱信号进行实时采样和处理,来检测是否存在较强的信号传输。
一般采用能量检测和周期检测等方法来实现频谱检测。
频谱识别是指通过对检测到的信号进行分析和处理,来判断信号的类型和属性。
常用的频谱识别方法包括周期识别、功率谱密度估计和模式识别等。
频谱感知技术的研究主要集中在以下几个方面:首先,频谱感知技术需要解决的一个重要问题是如何精确地感知到当前环境中的频谱信息。
由于无线电频谱是一个动态变化的环境,有时信号非常微弱,有时信号强度很大,因此如何准确、快速地感知到频谱信号是一个挑战。
目前,有很多成熟的频谱感知算法和技术被提出来,包括基于能量检测的方法、基于周期性的方法和基于功率谱密度估计的方法等。
通过采用合适的感知算法和技术,可以使系统能够实时监测并反馈当前频谱使用情况,从而合理分配频谱资源。
其次,频谱感知技术需要解决的另一个重要问题是如何准确地识别感知到的频谱信号。
不同类型的无线电信号具有不同的特点和属性,因此准确地识别信号类型对于频谱感知至关重要。
传统的频谱识别方法主要依赖于专家经验和手动设置的规则,其性能受限。
近年来,随着机器学习和模式识别等技术的发展,基于机器学习的频谱识别方法逐渐成为研究的热点。
Implementation and Evaluation of Energy-Based Spectrum Sensing Schemes in USRP Testbed
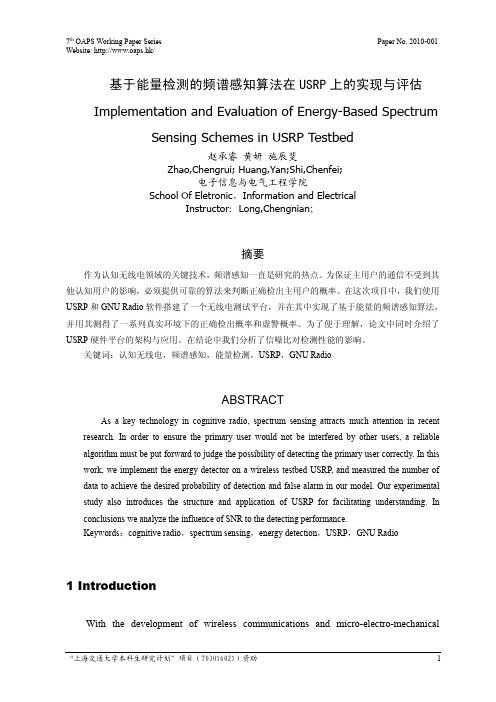
2
上海交通大学 SJTU
7th OAPS Working Paper Series
基于能量检测的频谱感知算法在 USRP 上的实现与评估
Figure 1 Spectrum Picture [2] One of the most optimal energy detection is based on matched filter which requires the knowledge of signal and noise, such as the characteristic of noise or the parameter of the transmission channel. The more it knows, the better it matches. On the other hand, if the signal is without priori knowledge, suboptimal energy detection can be used instead, which applies to the situation treated with little priori knowledge of the signal [3]. The sensing is modelled with the following two hypotheses:
信息论准则的频谱感知技术
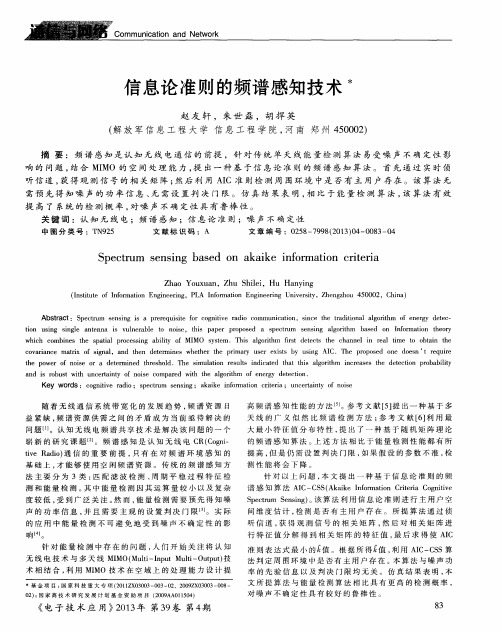
S p e c t r u m s e n s n i ‘ g b a s e d o n a k a i k ei ‘ n f t o r ma t i o n c r i ‘ t e r i 。 a
Comm u n i c a t i on a n d Ne t wor k
信 息论 准则 的频谱感知技术
赵 友 轩 ,朱 世 磊 ,胡 捍 英
( 解 放 军 信 息 工 程 大 学 信 息 工程 学 院 , 河 南 郑州 4 5 0 0 0 2 )
统 单 天 线 能量 检 测 算 法 易受噪 声 不确 定性 影
a n d i s r o bu s t wi t h u nc e r t ai n t y o f n o i s e c omp a r e d wi t h t he a l g o r i t h m o f e n e r g y d e t e c t i o n.
Ab s t r a c t :S p e c t r u m s e n s i n g i s a p r e r e q u i s i t e f o r c o g n i t i v e r a d i o c o mmu n i c a t i o n,s i n c e t h e t r a d i t i o n a l a l g o r i t h m o f e n e r g y d e t e c — t i o n u s i n g s i n g l e a n t e n n a i s v u l n e r a b l e t o n o i s e ,t h i s p a p e r p r o p o s e d a s p e c t r u m s e n s i n g a l g o r i t h m b a s e d o n I n f o r ma t i o n t h e o r y wh i c h c o mb i n e s t h e s p a t i a l p r o c e s s i n g a b i l i t y o f MI MO s y s t e m.Th i s a l g o r i t h m f i r s t d e t e c t s t h e c h a n n e l i n r e a l t i me t o o b t a i n t h e e o v a r i a n c e ma t r i x o f s i g n a l ,a n d t h e n d e t e r mi n e s wh e t h e r t h e p r i ma r y u s e r e x i s t s b y u s i n g AI C.T h e p r o p o s e d o n e d o e s n’ t r e q u i r e t h e p o we r o f n o i s e o r a d e t e r mi n e d t h r e s h o l d.T h e s i mu l a t i o n r e s u l t s i n d i c a t e d t h a t t h i s a l g o r i t h m i n c r e a s e s t h e d e t e c t i o n p r o b a b i l i t y
认知无线电中的频谱感知技术的研究
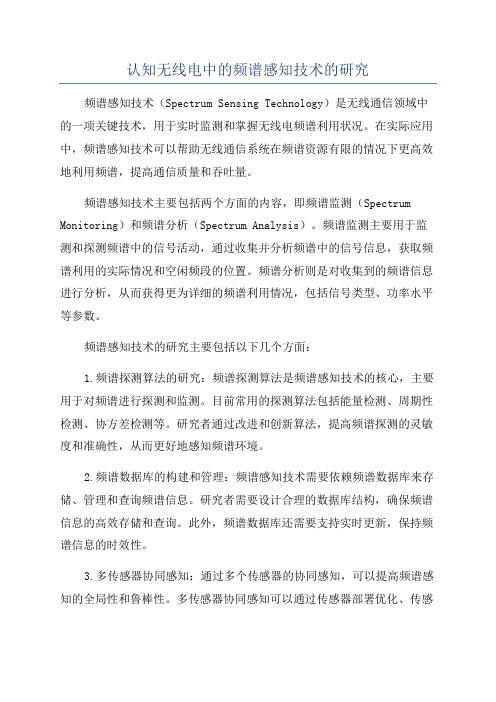
认知无线电中的频谱感知技术的研究频谱感知技术(Spectrum Sensing Technology)是无线通信领域中的一项关键技术,用于实时监测和掌握无线电频谱利用状况。
在实际应用中,频谱感知技术可以帮助无线通信系统在频谱资源有限的情况下更高效地利用频谱,提高通信质量和吞吐量。
频谱感知技术主要包括两个方面的内容,即频谱监测(Spectrum Monitoring)和频谱分析(Spectrum Analysis)。
频谱监测主要用于监测和探测频谱中的信号活动,通过收集并分析频谱中的信号信息,获取频谱利用的实际情况和空闲频段的位置。
频谱分析则是对收集到的频谱信息进行分析,从而获得更为详细的频谱利用情况,包括信号类型、功率水平等参数。
频谱感知技术的研究主要包括以下几个方面:1.频谱探测算法的研究:频谱探测算法是频谱感知技术的核心,主要用于对频谱进行探测和监测。
目前常用的探测算法包括能量检测、周期性检测、协方差检测等。
研究者通过改进和创新算法,提高频谱探测的灵敏度和准确性,从而更好地感知频谱环境。
2.频谱数据库的构建和管理:频谱感知技术需要依赖频谱数据库来存储、管理和查询频谱信息。
研究者需要设计合理的数据库结构,确保频谱信息的高效存储和查询。
此外,频谱数据库还需要支持实时更新,保持频谱信息的时效性。
3.多传感器协同感知:通过多个传感器的协同感知,可以提高频谱感知的全局性和鲁棒性。
多传感器协同感知可以通过传感器部署优化、传感器选择算法优化等方式实现,研究者需要探索合适的方法和算法,提高系统的感知性能。
4.频谱共享与动态频谱分配:频谱感知技术可以帮助实现频谱资源的共享与动态分配。
研究者需要借助频谱感知技术,实现对频谱的实时监控和调度,从而实现频谱资源的高效利用。
此外,研究者还需考虑频谱共享和动态频谱分配对无线通信系统性能的影响,并提出相应的优化策略。
5.频谱感知技术在无线电认知网络中的应用:无线电认知网络是基于频谱感知技术的一种新型无线通信网络,可以通过感知频谱,智能地分配和共享频谱资源。
a survey of spectrum sensing algorithms for cognitive radio applications
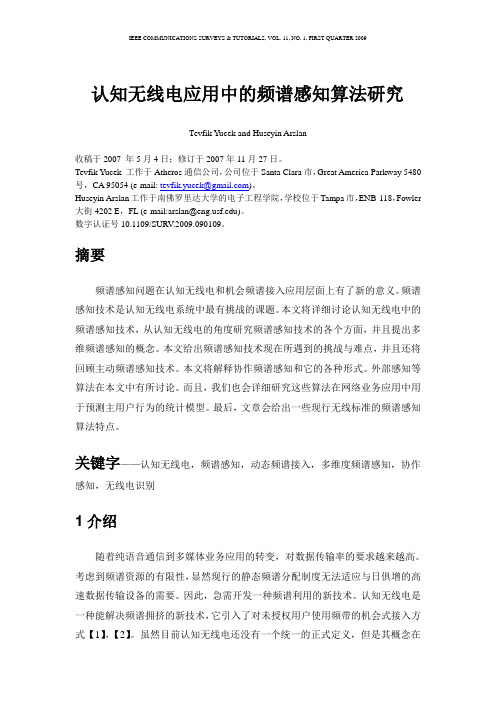
认知无线电应用中的频谱感知算法研究Tevfik Yucek and Huseyin Arslan收稿于2007 年5月4日;修订于2007年11月27日。
Tevfik Yucek 工作于Atheros通信公司,公司位于Santa Clara市,Great America Parkway 5480号,CA 95054 (e-mail: tevfik.yucek@)。
Huseyin Arslan工作于南佛罗里达大学的电子工程学院,学校位于Tampa市,ENB-118,Fowler 大街4202 E,FL (e-mail:arslan@)。
数字认证号10.1109/SURV.2009.090109。
摘要频谱感知问题在认知无线电和机会频谱接入应用层面上有了新的意义。
频谱感知技术是认知无线电系统中最有挑战的课题。
本文将详细讨论认知无线电中的频谱感知技术,从认知无线电的角度研究频谱感知技术的各个方面,并且提出多维频谱感知的概念。
本文给出频谱感知技术现在所遇到的挑战与难点,并且还将回顾主动频谱感知技术。
本文将解释协作频谱感知和它的各种形式。
外部感知等算法在本文中有所讨论。
而且,我们也会详细研究这些算法在网络业务应用中用于预测主用户行为的统计模型。
最后,文章会给出一些现行无线标准的频谱感知算法特点。
关键字——认知无线电,频谱感知,动态频谱接入,多维度频谱感知,协作感知,无线电识别1介绍随着纯语音通信到多媒体业务应用的转变,对数据传输率的要求越来越高。
考虑到频谱资源的有限性,显然现行的静态频谱分配制度无法适应与日俱增的高速数据传输设备的需要。
因此,急需开发一种频谱利用的新技术。
认知无线电是一种能解决频谱拥挤的新技术,它引入了对未授权用户使用频带的机会式接入方式【1】,【2】。
虽然目前认知无线电还没有一个统一的正式定义,但是其概念在不同环境下具有不同的含义【3】。
在本文中,我们采用联邦委员会(Federal Communications Commission,FCC)的定义:“认知无线电:一种具有感知其运行电磁环境,独立并且动态地改变其无线电运行参数以适应系统运行的无线电或无线电系统,系统运行包括增大吞吐量,减小干扰,易于互操作,接入次级用户。
认知无线电中频谱感知技术研究
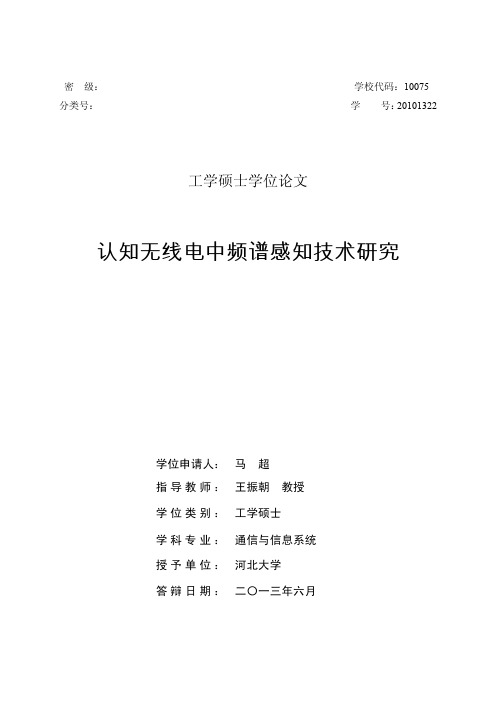
密级:学校代码:10075 分类号:学号:********工学硕士学位论文认知无线电中频谱感知技术研究学位申请人:马超指导教师:王振朝教授学位类别:工学硕士学科专业:通信与信息系统授予单位:河北大学答辩日期:二〇一三年六月Classified Index: CODE: 10075 U.D.C: NO:20101322A Dissertation for the Degree of M. EngineeringResearch of Spectrum SensingTechnique in Cognitive RadioCandidate: Ma chaoSupervisor: Prof. Wang zhen-chaoAcademic Degree Applied for: Master of EngineeringSpecialty: Comm. &Info. SystemUniversity: Hebei UniversityDate of Oral Examination: June, 2013摘要当前社会科学技术日新月异,使得无线移动通信技术同样呈现出了蓬勃发展的趋势。
与之相对的,人们对无线通信业务的需求也在自然而然地不断增长,进而形成了无线频谱资源越来越稀缺这样一个事实。
而这本就有限的频谱资源加之当前所采用的固定的频谱分配策略,就使得频谱的使用情况很不理想,这些因素无疑限制了无线通信业务进一步地发展。
近年来所兴起的认知无线电(Cognitive Radio,CR)技术可以有效地解决这个问题,从而越来越受到学术界和IEEE标准化组织的重视,为合理地利用空闲频谱资源开辟了一条新的途径。
认知无线电技术通过对外界无线通信环境不断的进行检测与感知,再根据一定的算法,每时每刻地改变自身的某些参数,动态地感知授权用户暂时闲置的频谱空穴,并在不妨碍授权用户使用该频段的前提下实现频谱的再次利用,从而达到对频谱资源充分、有效的利用这一目的。
基于深度学习的无线电频谱感知技术研究
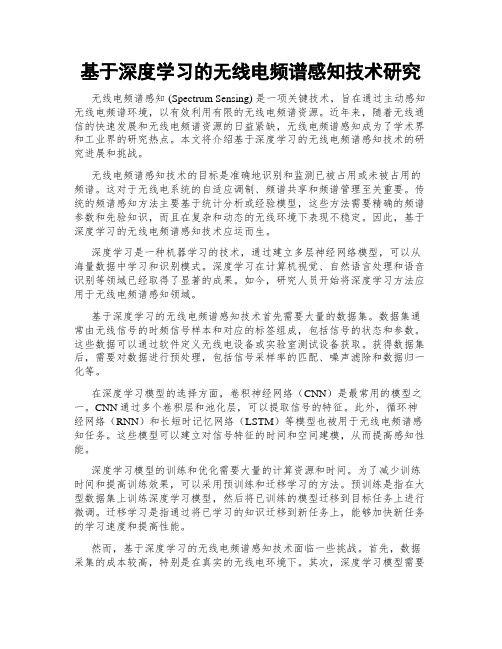
基于深度学习的无线电频谱感知技术研究无线电频谱感知 (Spectrum Sensing) 是一项关键技术,旨在通过主动感知无线电频谱环境,以有效利用有限的无线电频谱资源。
近年来,随着无线通信的快速发展和无线电频谱资源的日益紧缺,无线电频谱感知成为了学术界和工业界的研究热点。
本文将介绍基于深度学习的无线电频谱感知技术的研究进展和挑战。
无线电频谱感知技术的目标是准确地识别和监测已被占用或未被占用的频谱。
这对于无线电系统的自适应调制、频谱共享和频谱管理至关重要。
传统的频谱感知方法主要基于统计分析或经验模型,这些方法需要精确的频谱参数和先验知识,而且在复杂和动态的无线环境下表现不稳定。
因此,基于深度学习的无线电频谱感知技术应运而生。
深度学习是一种机器学习的技术,通过建立多层神经网络模型,可以从海量数据中学习和识别模式。
深度学习在计算机视觉、自然语言处理和语音识别等领域已经取得了显著的成果。
如今,研究人员开始将深度学习方法应用于无线电频谱感知领域。
基于深度学习的无线电频谱感知技术首先需要大量的数据集。
数据集通常由无线信号的时频信号样本和对应的标签组成,包括信号的状态和参数。
这些数据可以通过软件定义无线电设备或实验室测试设备获取。
获得数据集后,需要对数据进行预处理,包括信号采样率的匹配、噪声滤除和数据归一化等。
在深度学习模型的选择方面,卷积神经网络(CNN)是最常用的模型之一。
CNN通过多个卷积层和池化层,可以提取信号的特征。
此外,循环神经网络(RNN)和长短时记忆网络(LSTM)等模型也被用于无线电频谱感知任务。
这些模型可以建立对信号特征的时间和空间建模,从而提高感知性能。
深度学习模型的训练和优化需要大量的计算资源和时间。
为了减少训练时间和提高训练效果,可以采用预训练和迁移学习的方法。
预训练是指在大型数据集上训练深度学习模型,然后将已训练的模型迁移到目标任务上进行微调。
迁移学习是指通过将已学习的知识迁移到新任务上,能够加快新任务的学习速度和提高性能。
浅析认知无线电的频谱感知技术
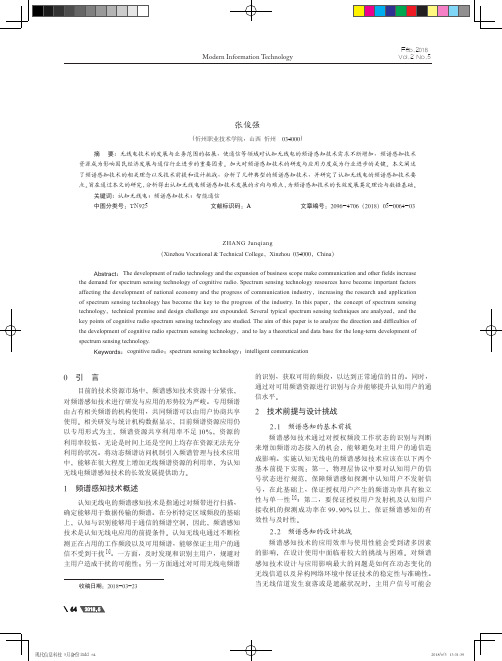
现代信息科技 Modern Information Technology
Feb.2018 Vol.2 No.5
浅析认知无线电的频谱感知技术
张俊强
(忻州职业技术学院,山西 忻州 034000)
摘 要:无线电技术的发展与业务范围的拓展,使通信等领域对认知无线电的频谱感知技术需求不断增加,频谱感知技术
收稿日期:2018-03-23
的识别,获取可用的频段,以达到正常通信的目的。同时, 通过对可用频谱资源进行识别与合并能够提升认知用本前提 频谱感知技术通过对授权频段工作状态的识别与判断 来增加频谱动态接入的机会,能够避免对主用户的通信造 成影响。实施认知无线电的频谱感知技术应该在以下两个 基本前提下实现:第一,物理层协议中要对认知用户的信 号状态进行规范,保障频谱感知探测中认知用户不发射信 号,在此基础上,保证授权用户产生的频谱功率具有独立 性与单一性 [2];第二,要保证授权用户发射机及认知用户 接收机的探测成功率在 99.90%以上,保证频谱感知的有 效性与及时性。 2.2 频谱感知的设计挑战 频谱感知技术的应用效率与使用性能会受到诸多因素 的影响,在设计使用中面临着较大的挑战与困难。对频谱 感知技术设计与应用影响最大的问题是如何在动态变化的 无线信道以及异构网络环境中保证技术的稳定性与准确性。 当无线信道发生衰落或是遮蔽状况时,主用户信号可能会
1 频谱感知技术概述
认知无线电的频谱感知技术是指通过对频带进行扫描, 确定能够用于数据传输的频谱。在分析特定区域频段的基础 上,认知与识别能够用于通信的频谱空洞,因此,频谱感知 技术是认知无线电应用的前提条件。认知无线电通过不断检 测正在占用的工作频段以及可用频谱,能够保证主用户的通 信不受到干扰 [1]。一方面,及时发现和识别主用户,规避对 主用户造成干扰的可能性;另一方面通过对可用无线电频谱
认知无线电中频谱感知算法的研究的开题报告
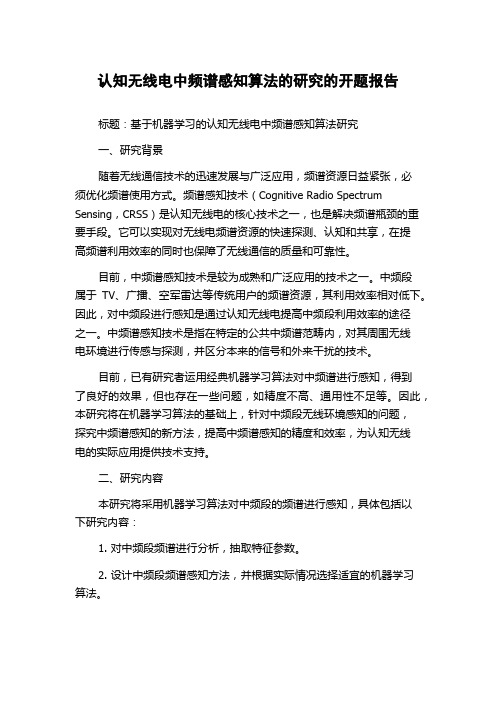
认知无线电中频谱感知算法的研究的开题报告标题:基于机器学习的认知无线电中频谱感知算法研究一、研究背景随着无线通信技术的迅速发展与广泛应用,频谱资源日益紧张,必须优化频谱使用方式。
频谱感知技术(Cognitive Radio Spectrum Sensing,CRSS)是认知无线电的核心技术之一,也是解决频谱瓶颈的重要手段。
它可以实现对无线电频谱资源的快速探测、认知和共享,在提高频谱利用效率的同时也保障了无线通信的质量和可靠性。
目前,中频谱感知技术是较为成熟和广泛应用的技术之一。
中频段属于TV、广播、空军雷达等传统用户的频谱资源,其利用效率相对低下。
因此,对中频段进行感知是通过认知无线电提高中频段利用效率的途径之一。
中频谱感知技术是指在特定的公共中频谱范畴内,对其周围无线电环境进行传感与探测,并区分本来的信号和外来干扰的技术。
目前,已有研究者运用经典机器学习算法对中频谱进行感知,得到了良好的效果,但也存在一些问题,如精度不高、通用性不足等。
因此,本研究将在机器学习算法的基础上,针对中频段无线环境感知的问题,探究中频谱感知的新方法,提高中频谱感知的精度和效率,为认知无线电的实际应用提供技术支持。
二、研究内容本研究将采用机器学习算法对中频段的频谱进行感知,具体包括以下研究内容:1. 对中频段频谱进行分析,抽取特征参数。
2. 设计中频段频谱感知方法,并根据实际情况选择适宜的机器学习算法。
3. 采集中频段频谱样本并对其进行处理,构建适合机器学习算法的数据集。
4. 使用所选机器学习算法进行模型训练,优化模型并测试其准确度。
5. 针对采集到的数据集进行可视化处理,直观展现感知结果。
三、研究意义通过研究中频谱感知算法,本研究将推动认知无线电技术的发展,解决无线频谱资源不足的问题,提高频谱的利用效率。
具体来说,本研究的研究意义包括:1. 提高中频段频谱感知的精度和效率,使得中频段的使用更加合理和充分,提高无线通信的性能和可靠性。
移动通信网络中的频谱感知技术研究
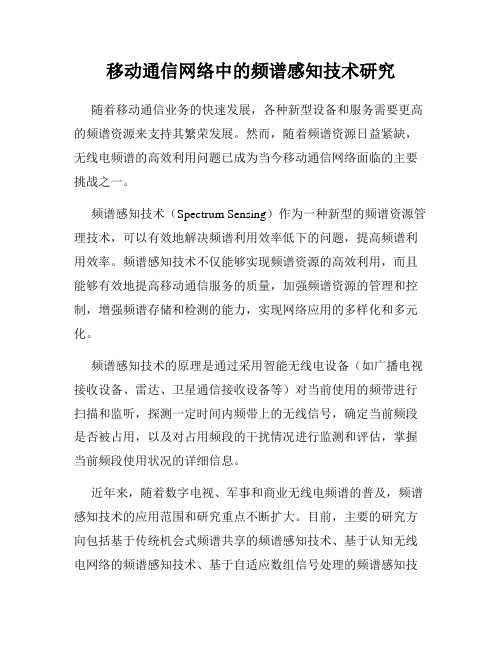
移动通信网络中的频谱感知技术研究随着移动通信业务的快速发展,各种新型设备和服务需要更高的频谱资源来支持其繁荣发展。
然而,随着频谱资源日益紧缺,无线电频谱的高效利用问题已成为当今移动通信网络面临的主要挑战之一。
频谱感知技术(Spectrum Sensing)作为一种新型的频谱资源管理技术,可以有效地解决频谱利用效率低下的问题,提高频谱利用效率。
频谱感知技术不仅能够实现频谱资源的高效利用,而且能够有效地提高移动通信服务的质量,加强频谱资源的管理和控制,增强频谱存储和检测的能力,实现网络应用的多样化和多元化。
频谱感知技术的原理是通过采用智能无线电设备(如广播电视接收设备、雷达、卫星通信接收设备等)对当前使用的频带进行扫描和监听,探测一定时间内频带上的无线信号,确定当前频段是否被占用,以及对占用频段的干扰情况进行监测和评估,掌握当前频段使用状况的详细信息。
近年来,随着数字电视、军事和商业无线电频谱的普及,频谱感知技术的应用范围和研究重点不断扩大。
目前,主要的研究方向包括基于传统机会式频谱共享的频谱感知技术、基于认知无线电网络的频谱感知技术、基于自适应数组信号处理的频谱感知技术、大规模天线阵列网络中的频谱感知技术、多媒体通信系统中的频谱感知技术、无线通信中基于智慧城市的频谱感知技术等。
这些研究方向都在探讨更多的应用场景,从而进一步提高频谱资源的利用效率。
事实上,频谱感知技术本身就是一种多学科交叉的研究领域。
它需要涉及到实际信号处理技术、统计电子学、通信理论等多个方面的知识。
同时还需要结合运筹学模型和算法来进行频谱分析和统计推断,保证检测结果的准确性和可靠性。
在移动通信网络中,频谱感知技术的研究和应用已经开始逐渐成熟,但是在实际应用中仍面临一些问题,如信道质量实时监测、通信设备功率控制等。
因此,开展更深入和广泛的研究,不仅可以解决上述问题,还可以实现网络的智能化调度和管理,提高用户体验,推动移动通信网络的创新和升级。
频谱感知技术matlab
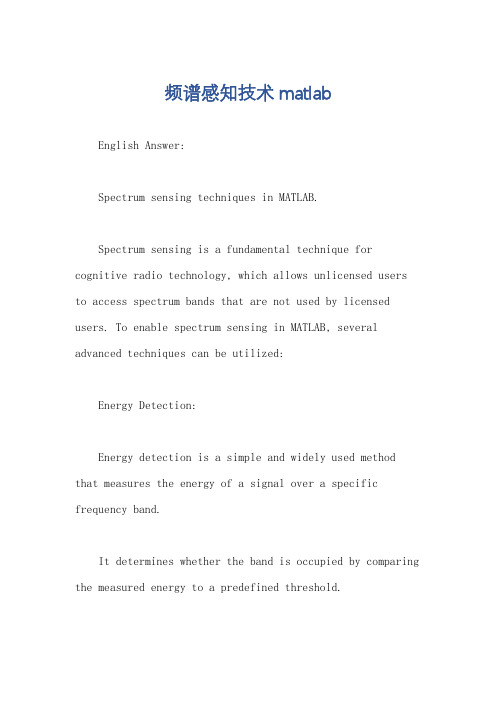
频谱感知技术matlabEnglish Answer:Spectrum sensing techniques in MATLAB.Spectrum sensing is a fundamental technique for cognitive radio technology, which allows unlicensed users to access spectrum bands that are not used by licensed users. To enable spectrum sensing in MATLAB, several advanced techniques can be utilized:Energy Detection:Energy detection is a simple and widely used methodthat measures the energy of a signal over a specific frequency band.It determines whether the band is occupied by comparing the measured energy to a predefined threshold.In MATLAB, you can implement energy detection using the `energyDetector` function.Matched Filtering:Matched filtering correlates the received signal with a known waveform representing the expected signal in the desired band.If the correlation coefficient exceeds a threshold, the band is considered occupied.MATLAB provides the `matchedFilter` function for this technique.Cyclostationary Feature Detection:Cyclostationary features refer to statistical properties of a signal that exhibit periodic variations over time.This technique exploits these features to distinguishbetween occupied and unoccupied bands.In MATLAB, you can use the `cyclostationaryDetector` function.Wavelet-Based Detection:Wavelet-based detection decomposes the signal into different scales and analyzes the energy distribution.It identifies suspicious bands based on specific wavelet coefficients.MATLAB offers the `waveletDetector` function for this approach.Cooperative Sensing:Cooperative sensing involves multiple nodes collaborating to enhance the accuracy of spectrum sensing.Each node collects its own measurements and shares themwith others for decision-making.MATLAB supports cooperative sensing through the`cooperativeDetector` function.Implementation in MATLAB.To implement spectrum sensing in MATLAB, you can follow these steps:1. Load the signal to be analyzed and define the frequency band of interest.2. Select the desired spectrum sensing technique from the functions mentioned above.3. Set the necessary parameters, such as the threshold for occupancy determination.4. Run the spectrum sensing function on the signal to obtain the occupancy information.5. Display the results and analyze the performance of the chosen technique.By utilizing these techniques and following the outlined implementation steps, you can effectively perform spectrum sensing in MATLAB for your cognitive radio applications.Chinese Answer:频谱感知技术在 MATLAB 中的实现。
一种低速采样的协同宽带频谱感知方法

一种低速采样的协同宽带频谱感知方法吴迪【摘要】Spectrum sensing is a key capability to enhance the anti-jamming and intelligence level of a communication system.The narrowband spectrum sensing performance of cognitive radio system is restricted by the technological progress of the analog digital converter,so it is difficult to realize the high real-time performance and wideband detection in system.In response to the problem,a method is proposed based on cooperative wideband spectrum sensing between multi-cognitive nodes in the network.In order to achieve high real-time performance and wideband detection of cognitive network system,the method reduces the sampling rate by making cognitive nodes perform sub-Nyquist sampling on the sensing frequency band,and realizes the positioning and judgment of jamming signal in the wide range of frequency through the centralized fusion and decision mechanism based on energy detection.The simulation and experimental results show that the proposed method can achieve 90%detection probability under the condition that compression ratio is0.025.So the method is correct and reliable.%频谱感知是通信系统抗干扰和智能化的关键能力.针对认知无线电系统窄带频谱感知技术受制于数模转换器件的发展水平,难以解决认知无线电系统宽带、实时频谱感知的问题,提出一种多节点协作的认知无线电系统宽带频谱感知方法.该方法设计由多个认知节点对目标频段执行次奈奎斯特采样来降低采样速率,采用能量检测方式对采样矢量进行集中式融合判决,实现宽频段范围内干扰信号的谱定位和判断,降低各个感知节点的采样速率,支撑认知网络系统构建高实时、宽频带频谱感知的能力.计算机仿真试验结果表明,所提方法达到90%检测概率时压缩比要求为0.025,具有可靠性与有效性.【期刊名称】《电讯技术》【年(卷),期】2017(057)006【总页数】6页(P629-634)【关键词】认知无线电;宽带频谱感知;协作频谱感知;次奈奎斯特采样;能量检测【作者】吴迪【作者单位】中国西南电子技术研究所,成都610036【正文语种】中文【中图分类】TN911.7通信需求的增长、电磁环境的恶化和频谱资源的匮乏是无线通信系统当前面临的典型问题[1]。
有关spectrumhandoff研究
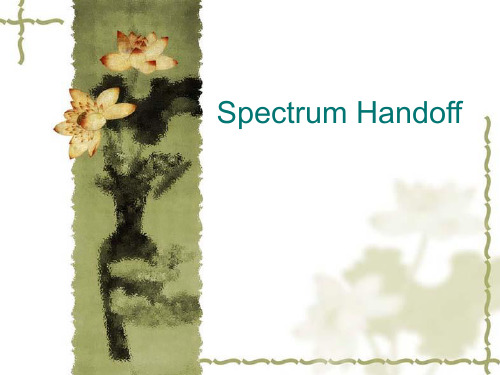
空闲频谱的空时变化特性以及各种业务的不同QoS要 求是CRN目前面临的两个主要问题,而动态频谱管 理是解决这些问题最核心的技术。
目前关于动态频谱管理的研究主要分为频谱检测、频 谱判决、频谱共享和频谱移动性四个方面
而频谱移动性即频谱切换可使用户通过更新工作频谱 保持与CRN的持续良好连接。
按照之前决策的接入顺序,在每一次频谱切换时刻依次排队方式接 入相应信道。
对reactive没有查到具体文献,从“?”文献中可看出,应该多出了对 每个信道进行感知选择的过程。
排队接入模型:
高优先级队列存放 主用户,低优先级 队列存放次级用户, 相互不能串入。
发生频谱切换的用户 可以在当前信道排队, 即等待,此种用户在 认知队列中优先级最
关注的方面
❖ 频谱切换时刻 ❖ 频谱切换目的 ❖ 频谱切换方式 ❖ 频谱切换指标 ❖ 切换信道选择 ❖ 频谱切换前景
主用户到来
信对道主质用量户不的能保满护足CR业务需求 Proactive 保证认知用切户换通时信延的和持准续确性性和的业tr务adQeooSff Reactive 链路维W护a概it 率、切换延时、切换频数等等
3、由于现实中对主用户的状态、参数、位置等的观测不准确,加之阴影、 衰落、干扰、噪声更大的不确定度,使得fuzzy理论很有应用价值。
论域中元素:x, 依某隶属函数Uf,属于linguistic variable:T(x)
CR接 受到的 主用户 发射功
率
主用户 发射信 噪比
HO:是否切换
MOD:功率是 否调整
本文工作:推导了在虚警影响下,reactive方式下的感知时间的表 达式。 1、认为频谱切换可能是由虚警引起的 2、寻找目标频谱过程中,频谱感知也存在由虚警引起的检测错误。 3、对目标信道逐一感知,直到找到最佳信道,模式:
TCCSS:宽带认知无线电网络频谱感知算法
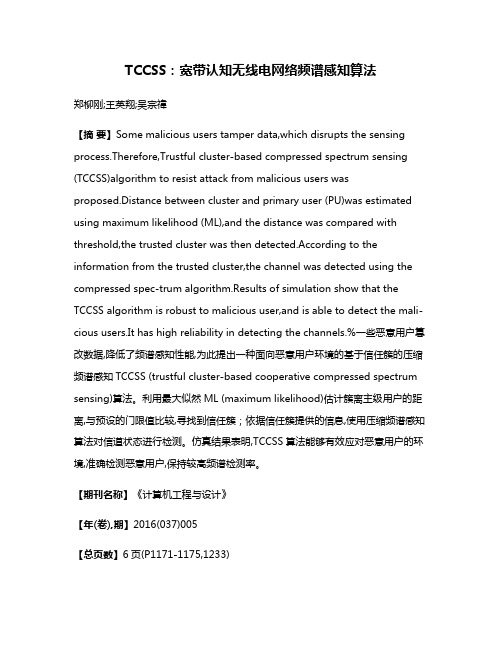
TCCSS:宽带认知无线电网络频谱感知算法郑柳刚;王英翔;吴宗禕【摘要】Some malicious users tamper data,which disrupts the sensing process.Therefore,Trustful cluster-based compressed spectrum sensing (TCCSS)algorithm to resist attack from malicious users was proposed.Distance between cluster and primary user (PU)was estimated using maximum likelihood (ML),and the distance was compared with threshold,the trusted cluster was then detected.According to the information from the trusted cluster,the channel was detected using the compressed spec-trum algorithm.Results of simulation show that the TCCSS algorithm is robust to malicious user,and is able to detect the mali-cious users.It has high reliability in detecting the channels.%一些恶意用户篡改数据,降低了频谱感知性能,为此提出一种面向恶意用户环境的基于信任簇的压缩频谱感知TCCSS (trustful cluster-based cooperative compressed spectrum sensing)算法。
浅析感知无线电技术中的频谱感测技术
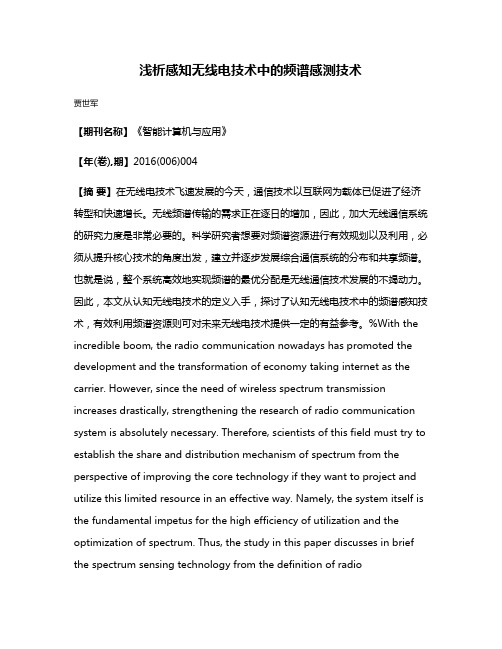
浅析感知无线电技术中的频谱感测技术贾世军【期刊名称】《智能计算机与应用》【年(卷),期】2016(006)004【摘要】在无线电技术飞速发展的今天,通信技术以互联网为载体已促进了经济转型和快速增长。
无线频谱传输的需求正在逐日的增加,因此,加大无线通信系统的研究力度是非常必要的。
科学研究者想要对频谱资源进行有效规划以及利用,必须从提升核心技术的角度出发,建立并逐步发展综合通信系统的分布和共享频谱。
也就是说,整个系统高效地实现频谱的最优分配是无线通信技术发展的不竭动力。
因此,本文从认知无线电技术的定义入手,探讨了认知无线电技术中的频谱感知技术,有效利用频谱资源则可对未来无线电技术提供一定的有益参考。
%With the incredible boom, the radio communication nowadays has promoted the development and the transformation of economy taking internet as the carrier. However, since the need of wireless spectrum transmission increases drastically, strengthening the research of radio communication system is absolutely necessary. Therefore, scientists of this field must try to establish the share and distribution mechanism of spectrum from the perspective of improving the core technology if they want to project and utilize this limited resource in an effective way. Namely, the system itself is the fundamental impetus for the high efficiency of utilization and the optimization of spectrum. Thus, the study in this paper discusses in brief the spectrum sensing technology from the definition of radiocommunication, so as to provide certain reference for the development of radio communication, in the sense of effective utilization of spectrum resources.【总页数】3页(P116-118)【作者】贾世军【作者单位】武汉贝斯特通信集团股份有限公司,武汉430022【正文语种】中文【中图分类】TP391【相关文献】1.感知无线电技术中的动态功率分配* [J], 王晶晶;陈媛媛2.自动驾驶技术中的感测器件分析 [J], 蒋以兆3.感知无线电中频谱共享技术的研究 [J], 陈晓娜4.浅析感知无线电技术中的频谱感测技术 [J], 贾世军;5.感知无线电中基于 SNR 的频谱检测技术 [J], 宫海梅因版权原因,仅展示原文概要,查看原文内容请购买。
- 1、下载文档前请自行甄别文档内容的完整性,平台不提供额外的编辑、内容补充、找答案等附加服务。
- 2、"仅部分预览"的文档,不可在线预览部分如存在完整性等问题,可反馈申请退款(可完整预览的文档不适用该条件!)。
- 3、如文档侵犯您的权益,请联系客服反馈,我们会尽快为您处理(人工客服工作时间:9:00-18:30)。
频谱感知技术外文翻译文献(文档含中英文对照即英文原文和中文翻译)译文:一种新的协作频谱感知算法摘要该文提出了一种在认知无线网络控制信道带宽受限条件下基于信任度的双门限协同频谱感知算法。
首先每个认知用户基于双检测门限独立进行频谱感知,但只有部分可靠的认知用户通过控制信道向认知无线网络基站发送本地感知结果。
当所有的用户都不可靠时,选取信任度最高的认知用户发送本地感知结果进行判决。
理论分析和仿真表明,同常规能量检测算法相比较,该算法能够在控制信道带宽受限条件下,以较少的网络开销获得更好的频谱感知性能。
关键词:认知无线电;频谱感知;信任度;双门限1引言随着无线通信技术的飞速发展,有限的频谱资源与不断增长的无线通信需求的矛盾越来越突出。
然而根据现有的固定分配频谱资源策略,绝大多数频谱资源得不到有效利用。
据FCC 的调查统计,70%的已分配频谱资源没有得到有效利用]1[。
为了提高频谱资源的利用率,认知无线电技术由Joseph Mitola Ⅲ提出并得到了广泛的关注]5[]2[ 。
频谱感知技术是认知无线电网络的支撑技术之一。
通常它又可以分为能量检测法、匹配滤波器法和循环平稳特征法[4]。
能量检测算法因为应用简单且无需知道任何授权用户信号的先验知识成为研究热点。
认知用户在接入授权频带之前,必须首先感知该频带空闲即授权用户没有工作,否则会对授权用户造成干扰。
一旦授权用户重新工作,认知用户必须退避,实现在不对授权用户产生干扰的情况下对频谱资源的共享。
由于实际信道中的多径和阴影效应,单个认知用户频谱感知的性能并不乐观,针对这个问题D. Cabric 等人提出了协同频谱感知算法[5]-[6]。
协同频谱感知算法性能较好,但是当认知用户数量很大的时候,控制信道的带宽将不够用。
文献[7]中提出了一种在控制信道带宽受限条件下的基于双检测门限的频谱感知算法,该算法能够以较小的网络开销,获得接近普通单门限频谱检测算法的性能。
针对认知无线电频谱感知的需要,本文提出了认知无线电环境下一种基于信任度的双门限协同频谱感知算法。
该算法中每个认知用户基于双检测门限独立进行频谱感知,但只有部分可靠的认知用户通过控制信道向认知无线网络基站发射感知报告。
当所有的用户都不可靠时,选取信任度最高的认知用户发射感知报告进行判决。
本文对该算法进行了性能分析并通过仿真表明,本文方法比较常规能量检测算法,在减小网络开销的同时提高了检测性能。
2系统模型假设一个认知无线电网络有N 个认知用户和一个认知无线网络基站,如图1 所示。
认知无线网络基站负责管理和联系N 个认知用户,在收到认知用户的检测报告后做出最终判决。
图1. 认知无线电网络示意图频谱感知的实质是一个二元假设问题,即01(),,()()()(),n t H x t h t s t n t H ⎧=⎨⋅+⎩ (1)其中x (t )代表认知用户接收到的信号,s (t )表示授权用户的发送信号,h (t )代表授权用户与认知用户之间信道的衰落因子。
0H 代表授权用户没有工作,1H 代表授权用户正在工作。
设θ是认知用户接收信号的能量,根据能量检测理论[8],θ服从以下分布:⎪⎩⎪⎨⎧122022),(,~H X H X m m γθ (2) 其中γ表示瞬时信噪比,并且其服从均值为_γ的指数分布,22m X 表自由度为2m 的中心卡方分布,)(22γm X 代表自由度为m 2非中心参数为γ的卡方分布,m 表示时间带宽积。
在能量检测算法本地判决中,每个认知用户把接收到的能量θ跟预设的门限λ进行比较,如图2(a )所示。
当λθ>时,本地能量检测器做出本地判决1=D ,表示授权用户在工作,否则判决 D 为 0。
而双门限能量检测算法本地判决如图3(b)所示,本地能量检测器判决规则如下:(3)其中ND 表示认知用户接受到的能量值不可靠,认知用户不作出任何判决,发送感知报告给认知无线电网络基站。
如果出现所有认知用户都不作出判决的情况,则选择信用度最高的认知用户依据单门限能量检测算法作出本地判决。
并发送感知报告给认知无线电网络基站。
⎪⎩⎪⎨⎧<<<<<<=21211,1,0,0λθλλθλλθND D图2.(a )一般能量检测算法本地判决示意图(b )双门限能量检测算法本地判决示意图信用度获取方法采取文献[9]的方法:在最开始阶段,认知无线电网络基站把每个认知用户数目的可信度设为0,当某认知用户本地判决结果与认知无线电网络基站的最终判决结果一致时,该认知用户可信度加1,否则减1。
假设认知用户i 的可信度是i γ,则其更新过程如(4):u u i i i +-+←)1(γγ (4) 其中u 是认知用户传送给认知无线电网络基站的判决结果,1u 是认知无线电网络基站的最终判决结果。
据文献[8]可知,认知用户在高斯信道下的平均检测概率、平均漏检概率和平均虚警概率如下所示: ),2()|(1λγλθm d Q H P P =>= (5) d m p H p P -=>=1)|(1λθ (6))()2/,()|(0m m H p P f ΓΓ=>=λλθ (7) 出于对授权用户的保护,认知无线电网络基站最终采用OR 准则作出判决。
3频谱感知性能分析3.1网络开销在1bit 量化条件下,avg K 代表归一化平均感知位数,K T 和K N T -分别代表K 个已向认知无线电网络基站发送数据和N-K 个未向认知无线电网络基站发送报告。
则:K i K p T P )](1[}{21λθλ<<-=,K N i K N P T P --<<=)]([}{21`λθλ。
设}{11H P P=和}{00H P P =,则划归一划平均感知位数acg K 如式8所示:∑∑==-=⎪⎪⎭⎫ ⎝⎛+⎪⎪⎭⎫ ⎝⎛=N K N K k N k K N k avgH T P H T P K N K P H T P H T P K N K P K 111110'00}|{}|{}|{}|{ (8)定义:)|(02110H P λθλ<<=∆,)|(02110H P λθλ<<=∆ 则:11001∆-∆-=P P K a v g (9) 由9式可得:1<avg K可知:基于双门限的协同频谱检测算法的网络开销始终小于常规的能量检测算法。
3.2检测性能分析设)(λF 和)(λG 别表示 θ在假设0H 和1H 下的概率分布,则根据文献[10]可知:⎰ΓΓ-==λλθθλ00)()2/,(1)|()(m m d H F F (10) ⎰=λθθλ01)|()(d H f G =⎥⎥⎥⎦⎤⎢⎢⎢⎣⎡⎪⎪⎪⎭⎫ ⎝⎛+-⨯⎪⎪⎪⎭⎫ ⎝⎛++⎪⎭⎫ ⎝⎛⎩⎨⎧-∑∑==+-==--202__2)1(2201_22)1(2!11211m n m n m n e e n eγγλγγλλγλλ (11) 显然)()(210λλF F -=∆,)()(121λλG G -=∆。
假设0β,1β分别代表在授权用户在工作和授权用户未工作情况下没有认知用户发送感知报告,即当K=0时,则:NN F F H K P 01200))()((}|0{∆=-===∆λλβ (12)NN G G H K P 11211))()((}|0{∆=-===∆λλβ (13)基于双门限的频谱感知算法在瑞利信道下的虚警概率f Q ,漏检概率m Q 和检测概率d Q 分别为:)1)(1(}1,|1{}|1{}|0,1{0000A f P K H u P H K P H K u P Q --=≥=≥=≥==β (14)m Q =d Q H K u P -=≥=1}|0,0{1(15) )1)(1(}1,|1{}|1{}|0,1{1111βP a K H u P H K P H K u P Q d --=≥=≥=≥==(16)其中:d A Q H K u P P -=≥==1}|0,0{1=∑=--⎪⎪⎭⎫ ⎝⎛NK K N k F F F K N 1121))()(()(λλλ =02)(βλ-N F (17) 12)(βλ-=G P B (18) 则:))(1)(1(020βλβ+--=N f F Q (19)))(1)(1(121βλβ+--=N f G Q (20) 由上式可知当0β=0时,此算法与常规算法相同。
当参与协同的认知用户数目N 较大时,00→β,则基于双门限的频谱检测算法的检测性能与常规能量算法的检测性能近似,可知在控制信道带宽受限制的情况下以较小的性能损失大大降低了网络开销。
4 仿真及分析本节通过计算机仿真来评估所提出的基于信任度的双门限协同频谱感知算法的性能。
仿真参数设置如表1 所示。
表1 仿真参数设置图3 给出了在1.00=∆的情况下算法的检测性能。
可以看出同常规能量检测算法相比较,本文所提出算法的检测性能得到了明显的改善。
例如当001.0=f Q 时,基于信任度的双门限协同频谱感知算法的检测概率d Q 比常规能量检测算法高出0.019。
图3检测性能示意图图4 描述了在不同0β的条件下,基于信任度的双门限协同频谱感知算法对网络开销的影响。
同常规能量检测算法即0β=0时相比较,本文所提出算法的归一化平均感知位数avg K 急剧下降,控制信道带宽与认知用户数量之间的矛盾得到了缓解。
例如当01.0=f Q ,0β= 0.01 时,基于信任度的双门限协同频谱感知算法的归一化平均感知位数avg K 下降了38%。
当01.0=f Q ,0β=0.001时,归一化平均感知位数avg K 则下降了44%图4 不同0β条件下算法对网络开销的影响5结束语频谱感知技术是认知无线电网络的支撑技术之一。
当认知用户数量很大的时候,控制信道的带宽将不够用。
本文提出了认知无线电环境下一种基于信任度的双门限协同频谱感知算法。
每个认知用户基于双检测门限独立进行频谱感知,但只有部分可靠的认知用户通过控制信道向认知无线网络基站发射感知报告。
当所有的用户都不可靠时,选取信任度最高的认知用户发射感知报告进行判决。
本文对该算法进行了性能分析并通过仿真表明,本文方法比较常规能量检测算法,在减小网络开销的同时提高了检测性能。
参考文献[1] Federal Communications Commission. Spectrum Policy Task Force, Rep. ET Docket no. 02-135[R]. Nov. 2002.[2] J. Mitola and G. Q. Maguire. Cognitive radio: Making software radios more personal[C],IEEEPersonal Communication. vol. 6, pp. 13–18, Aug. 1999.[3] S. Haykin. Cognitive radio: brain-empowered wireless communications [J]. IEEE J. Sel. AreasCommunication. vol. 23, pp. 201–220, Feb. 2005.[4] AKYLDIZ IF. Next generation/dynamic spectrum access/cognitive radio wireless networks: ASurvey [J]. ELSEVIER Computer Networks, 2006(50):2127-2159.[5] D. Cabric, S. M. Mishra, and R. W. Brodersen. Implementation issues in spectrum sensing forcognitive radios[C]// in Proc. Of A silomar Conf. on Signals, Systems, and Computers, Pacific Grove,CA, USA, Nov. 7-10, 2004, pp. 772 - 776.[6] A.Ghasemi and E. S. Sousa. Collaborative spectrum sensing for opportunistic access in fadingenvironments[C]// in Proc. 1st IEEES ymp. New Frontiers in Dynamic Spectrum Access Networks, Baltimore, USA, Nov. 8–11, 2005, pp. 131–136.[7] Chunhua Sun, Wei Zhang, Letaief K.B. Cooperative spectrum sensing for cognitive radios underbandwidth constraints[C]// in Proc. IEEE WCNC, March 11-15, 2007, pp. 1-5.[8] H. Urkowitz. Energy detection of unknown deterministic signals [C]. Proceedings of IEEE,vol.55, pp. 523-531, April 1967.[9] Ruiliang Chen, Jung-Min Park, Kaigui Bian. Robust Distributed Spectrum Sensing in CognitiveRadio Networks[C]. in Proc. IEEEINFOCOM, April 2008, pp. 1876-1884.[10] F. F. Digham, M. -S. Alouini, and M. K. Simon. On the energy detection of unknown signalsover fading channels[C]. in Proc. IEEE ICC, Anchorage, AK, USA, May 11-15, 2003, pp.3575–3579.原文:A New Cooperative Spectrum Sensing Algorithmfor Cognitive Radio Networks Abstract—spectrum sensing is a critical phase in building a cognitive radio network. However, the bandwidth for reporting secondary users’ sensing results will be insufficient, when the number of secondary user is very large. In this paper, we propose a new cooperative spectrum sensing algorithm to alleviate the bandwidth problem of reporting channel. Compared with conventional method, only the secondary users with reliable information are allowed to report their sensing results. When no user with reliable information, only the secondary user with highest reputation will report its sensing result. Simulation results show that our algorithm achieves better sensing performance and the average number of sensing bits decrease greatly. Keywords—cognitive radio; cooperative spectrum sensing; double threshold; reputationⅠ. INTRODUCTIONDue to the increasingly development of wireless applications, more and more spectrum resources are needed to support numerous emerging wireless service. However, recent measurements by Federal Communication Commission (FCC) have shown that 70% of the allocated spectrum in US is not utilized [1]. In order to increase the efficiency of spectrum utilization, cognitive radio technology was recently proposed [2],[3].A requirement of cognitive radios is that their transmission should not cause harmful interference to primary users. Namely, the secondary users can use the licensed spectrum as long as the primary user is absent. However, when the primary user comes back into operation, the secondary users should vacate the spectrum instantly to avoid interference with the primary user. Accordingly, spectrum sensing is a crucial phase in building a cognitive radio system.One of the great challenges of implementing spectrum sensing is the hidden terminal problem which caused by the fading of the channels and the shadowing effects. In order to deal with the hidden terminal problem, cooperative spectrum sensing hasbeen studied to improve the spectrum sensing performance [4], [5].In[6], due to control channel for each cognitive radio to report its sensing result is usually bandwidth limited, a censoring method which has two thresholds is given to decrease the average number of sensing bits to the common receiver. By censoring the collected local observations, only the secondary users with enough information will send their local decisions to the common receiver.In this paper, we present a new double threshold cooperative spectrum sensing method with reputation. In our system, every cognitive user will firstly obtain an observation independently and only the users with reliable information send their local decisions to the common receiver based on double thresholds. If no user is reliable, only the cognitive user with the highest reputation is selected to sense the spectrum. Simulation results show that the spectrum sensing performance under AWGN channels is improved and the communication traffic is also reduced as opposed to the conventional method.The rest of the paper is organized as follows. In section Ⅱ, system model is briefly introduced. Sensing performance is analyzed in Section Ⅲ. In Section Ⅳ, we present the simulation results of our cooperative spectrum sensing method. Finally, we draw our conclusions in Section Ⅴ.II. SYSTEM MODELIn cognitive radio systems, spectrum sensing is a critical element as it should be firstly performed before allowing secondary users to access a vacant licensed channel. Cooperative spectrum sensing has been widely used to detect the primary user with a high agility and accuracy. The essence of spectrum sensing is a binary hypothesis-testing problem:H:primary user is absent;H:primary user is present.1For implementation simplicity, we restrict ourselves to energy detection in the spectrum sensing. The local spectrum sensing is to decide between the following two hypotheses:01(),,()()()(),n t H x t h t s t n t H ⎧=⎨⋅+⎩ (1) Where )(t x is the signal received by secondary user, )(t s is primary user’s transmitted signal,)(t n is AWGN, and )(t h is the temporary amplitude gain of the channel.According to energy detection theory [7], we have the following distribution:⎪⎩⎪⎨⎧122022),(,~H X H X m m γθ (2) Where θ is the energy value collected by secondary user,γ is instantaneous SNR and follows exponentially distribution with the mean value _γ, m is the timebandwidth product of the energy detector,22m χrepresents a central chi-square distributionwith 2m degrees of freedom and. )(22γχm represents a non-central chi-square distribution with m 2degrees of freedom and a non-centrality parameter γ2.In conventional energy detection method, the local decision is made by comparing the observation with a pre-fixed threshold as Fig.1 (a). When the collected energy θ exceeds the threshold λ, decision 0H will be made. Otherwise decision 1H will be made. In contrast, the system model which has two thresholds of our interest is shown inFig.1 (b). Where ― Decision 0H ‖ and ―Decision 1H ‖ represent the absence and the presence of licensed user, respectively.“No decision‖ means that the observation is not reliable enough and the i th cognitive user will send nothing to the common receiver. But when all the secondary users don’t send their local decisions, only the cognitive user with the highest reputation is selected to sense spectrum based on conventional energy detection method, and send its local decision to the common receiver.Reputation is obtained based on the accuracy of cognitive us er’s sensing results. The reputation value is set to zero at the beginning. Whenever its local spectrum sensing report is consistent with the final sensing decision, its reputation is incremented by one; otherwise it is decremented by one. Under this rule, assuming the i th cognitive user’s reputation value is 1, the last sensing report of cognitive user i send to common receiver is u , and the final decision is i u ,then i γ is updated according to thefollowing relation: u u i i i +-+←)1(γγFor the cognitive radio users with the energy detector, the average probabilities of detection, the average probabilities of missed detection, and the average probabilities of false alarm over AWGN channels are given, respectively, by [7]:),2()|(1λγλθm d Q H P P =>= (3)d m p H p P -=>=1)|(1λθ (4))()2/,()|(0m m H p P f ΓΓ=>=λλθ (5) Where )(a Γ, ),(b a Γare complete and incomplete gamma function respectively, and ),(b a Q m is the generalized Marcum function.In this paper, we consider cooperative spectrum sensing with 1bit quantization. Let avg K represent the normalizedFig1. (a)Conventional detection method(b)Double threshold energy detection methodaverage number of sensing bit. Let K T and 'K N T -represent he event that there are K unlicensed users reporting 1-bit decision and N-K users not reporting to the commonreceiver, respectively. The K i K p T P )](1[}{21λθλ<<-=, K N i K N P T P --<<=)]([}{21`λθλ.andthen the average number of sensing bits for our method can be derived as:∑∑==-=⎪⎪⎭⎫ ⎝⎛+⎪⎪⎭⎫ ⎝⎛=NK N K k N k K N k avg H T P H T P K N K P H T P H T P K N K P K 111110'00}|{}|{}|{}|{ (6)For simplicity, we define:)|(02110H P λθλ<<=∆ ,)|(02110H P λθλ<<=∆ (7)Let navg K denote the normalized average number of sensing bits, then, we obtain navg K as follows :11001∆-∆-=P P K a v g (8) From (8), It can be seen that, the normalized average number of sensing bits navg K is always smaller than 1. the communication traffic of our method is are deduced as opposed to the conventional energy detection method.III. THE PERFORMANCE ANALYSIS OF SPECTRUMSENSINGIn this section, the spectrum sensing performance of the proposed method will be analyzed. Assume the control channel between the unlicensed users and the common receiver is perfect, the local decisions are reported without any error. Let )(λF and )(λG denote the cumulative distribution function (CDF) of the local test statistic θ under the hypothesis 0H and 1H , respectively. Then, we have [10]:⎰ΓΓ-==λλθθλ00)()2/,(1)|()(m m d H F F (9) ⎰=λθθλ01)|()(d H f G (10)Obviously,)()(210λλF F -=∆,)()(121λλG G -=∆.If no any local decision is reported to the common receiver, i.e., K=0 , we call that fail sensing. For this case, the common receiver will request the user which has the highest reputation to send its local decision based on conventional energy detection method. Let 0βand 1βdenote the probability of fail sensing under hypothesis 0H and 1H , respectively. Here we have:NN F F H K P 01200))()((}|0{∆=-===∆λλβ (11)NN G G H K P 11211))()((}|0{∆=-===∆λλβ (12)Apparently,N00∆=β and N 11∆=β.In our scheme, the false alarm probabilityf Q ,the detection probability d Q ,and the missing probability m Q :)1)(1(}1,|1{}|1{}|0,1{0000A f P K H u P H K P H K u P Q --=≥=≥=≥==β (13)m Q =d Q H K u P -=≥=1}|0,0{1 (14))1)(1(}1,|1{}|1{}|0,1{1111βP a K H u P H K P H K u P Q d --=≥=≥=≥== (15)For simplicity, we assume the channel between the unlicensed users and the base station are ideal, the local decision will be reported without any error. So A P stand for the probability of the event that under hypothesis 0H , all the K users claim 0H and other N-K users make no local decisions.d A Q H K u P P -=≥==1}|0,0{1=∑=--⎪⎪⎭⎫ ⎝⎛NK K N k F F F K N 1121))()(()(λλλ =02)(βλ-N F (16) 12)(βλ-=G P B (17) ))(_1)(1(020βλβ+-=N f F Q (18) ))(_1)(1(121βλβ+-=N f G Q (19)IV. SIMULATION RESULTSIn this section, some simulation results are presented to illustrate the system performance of our cooperative spectrum sensing algorithm based on reputation. The results of the conventional one threshold energy detection method are also shown for a comparison. In our simulation, the common simulation parameters are given as follows:Fig.2 depicts the performance of cooperative spectrum sensing d Q and f Q .1.00=∆.It can be observed that, compared it with the conventional method, the detection performance has improved significantly. For example, while f Q = 0.001, our method achieves extra 0.019 detection probability. Fig.3 shows the decrease of the normalized transmission bits for different values of fail sensing, i.e. 0β= 0, 0.001, 0.01, 0.1. Compared with conventional method, i.e., when 0β = 0, the normalized average number of sensing bits is dramatically decreased and bandwidth limited problem of the reporting channel is relieved. For example, when f Q = 0.01, almost 44% and 38% reduction of the normalized average number of sensing bits can be obtained for 0β= 0.001 and 0β = 0.01, respectively. In our algorithm, f Q is upper bounded and lower bounded because of the probability of fail sensing 0βand the false alarm probability are based on (7), (13).Fig 2.d Q vs.f Q , 1.00=∆Fig 3.navg K vs.f Q ,0 =00,0.001,0.01,0.1V. CONCLUSIONIn this paper, a new scheme in cooperative spectrum sensing for cognitive radio networks under bandwidth constraints was proposed. In our method, only the secondary users with reliable information are allowed to report their sensing results. When no user has reliable information, only he secondary user with highest reputation will report its sensing result. We analyzed the closed expression for the probability of the detection and the false-alarm. From the preliminary simulation results, we demonstrated the average number of sensing bits decrease greatly and the sensing performance is also improved.REFERENCES[1] Federal Communications Commission. Spectrum Policy Task Force, Rep. ET Docket no. 02-135[R]. Nov. 2002.[2] J. Mitola and G. Q. Maguire. Cognitive radio: Making software radios more personal[C],IEEE Personal Communication. vol. 6, pp. 13–18, Aug. 1999.[3] S. Haykin. Cognitive radio: brain-empowered wireless communications [J]. IEEE J. Sel. Areas Communication. vol. 23, pp. 201–220, Feb. 2005.[4] AKYLDIZ IF. Next generation/dynamic spectrum access/cognitive radio wireless networks: A Survey [J]. ELSEVIER Computer Networks, 2006(50):2127-2159.[5] D. Cabric, S. M. Mishra, and R. W. Brodersen. Implementation issues in spectrum sensing forcognitive radios[C]// in Proc. Of A silomar Conf. on Signals, Systems, and Computers, Pacific Grove,CA, USA, Nov. 7-10, 2004, pp. 772 - 776.[6] A.Ghasemi and E. S. Sousa. Collaborative spectrum sensing for opportunistic access in fadingenvironments[C]// in Proc. 1st IEEES ymp. New Frontiers in Dynamic Spectrum Access Networks, Baltimore, USA, Nov. 8–11, 2005, pp. 131–136.[7] Chunhua Sun, Wei Zhang, Letaief K.B. Cooperative spectrum sensing for cognitive radios underbandwidth constraints[C]// in Proc. IEEE WCNC, March 11-15, 2007, pp. 1-5.[8] H. Urkowitz. Energy detection of unknown deterministic signals [C]. Proceedings of IEEE,vol.55, pp. 523-531, April 1967.[9] Ruiliang Chen, Jung-Min Park, Kaigui Bian. Robust Distributed Spectrum Sensing in CognitiveRadio Networks[C]. in Proc. IEEEINFOCOM, April 2008, pp. 1876-1884.[10] F. F. Digham, M. -S. Alouini, and M. K. Simon. On the energy detection of unknown signalsover fading channels[C]. in Proc. IEEE ICC, Anchorage, AK, USA, May 11-15, 2003, pp.3575–3579.。