Computer Vision计算机视觉英文ppt
合集下载
Class03computer vision 计算机视觉

(x,y,z) = k(x’,y’,z’)
2
Projective 3D Space
The projective space P3 is the set of equivalence classes of quadruplets of numbers (not all zero) where two quadruplets (x,y,z,w) and (x’,y’,z’,w’) are equivalent if and only if there is a real number k such that
(x,y,z,w) = k(x’,y’,z’,w’)
3
What happens if k=0?
k=0 can be used to represent points “at infinity”. All points at infinity in the 2D projective space lie on the line “at infinity”. Points at infinity are also called IMPROPER POINTS or IDEAL POINTS.
In projective space ALL lines intersect at a point. Some lines intersecne. We call these lines PARALLEL.
4
Duality Principle
Inverse exists
T1-1
state2
Inverse exists
T2-1
state3
state1
T1-1 * T2-1
closed under composition
计算机视觉课件2(英文版)

A 10,19,22,36,38,49,75,98,99
I0 medianA 38
18
Other nonlinear filters:
——minimum filter(remove salt noise):
I0 minA
——maximum filter (remove pepper noise)
——linear filter ——nonlinear filter
2
1. Linear system
(x x0, y y0 )
delta function
case:
Linear Space
h(x x0, y y0)
Invariant Systiemmpulse response
Continuous
——Cascading operation: x2 Let h1(x) h2(x)
e e d 222 (x22)2
e 2(
x2 2)2
If a Gaussian filter has a large parameter , it requires a large operation mask and thus, it will take long time to process.
To reduce windows boundary effect and for a smooth filterin g, filter elements are often weighted.
1 11 16 8 16
111
848
1 11
7
16 8 16
Mean Filter on Gaussian noise corrupted image
Computer-Vision计算机视觉英文ppt
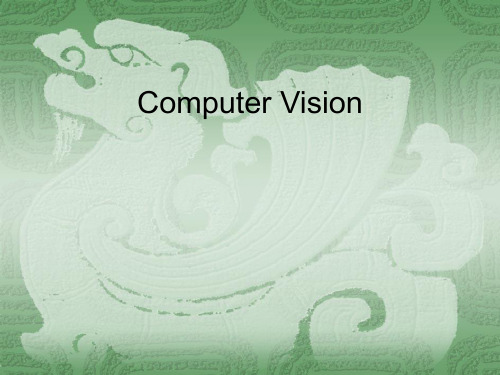
At the same time, AI MIT laboratory has attracted many famous scholars from the world to participate in the research of machine vision,which included the theory of machine vision , algorithm and design of system .
Its mainstream research is divided into three stages:
Stage 1: Research on the visual basic method ,which take the model world as the main object;
Stage 2: Research on visual model ,which is based on the computational theory;
the other is to rebuild the three dimensional object according to the two-dimensional projection images .
History of computer vision
1950s: in this period , statistical pattern recognition is most applied in computer vision , it mainly focuse on the analysis and identification of two-dimensional image,such as: optical character recognition, the surface of the workpiece, the analysis and interpretation of the aerial image.
Its mainstream research is divided into three stages:
Stage 1: Research on the visual basic method ,which take the model world as the main object;
Stage 2: Research on visual model ,which is based on the computational theory;
the other is to rebuild the three dimensional object according to the two-dimensional projection images .
History of computer vision
1950s: in this period , statistical pattern recognition is most applied in computer vision , it mainly focuse on the analysis and identification of two-dimensional image,such as: optical character recognition, the surface of the workpiece, the analysis and interpretation of the aerial image.
computer vision ppt
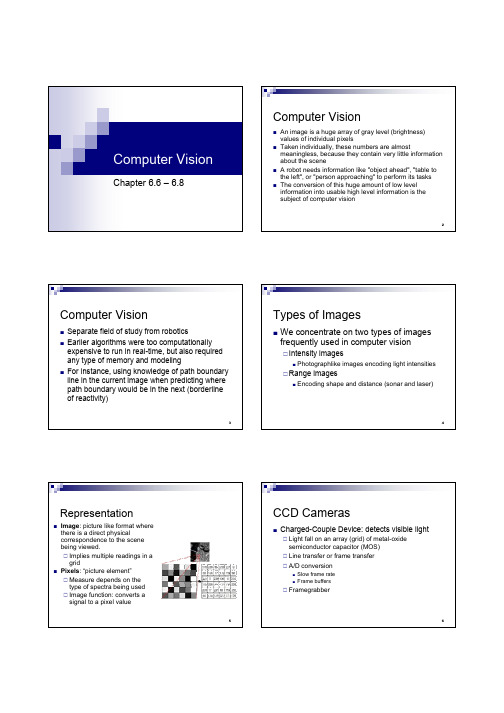
Saturation:
Challenging for robotics
11
12
SCT Representations
(Spherical Coordinate Transform)
Color Representations
RGB
Currently being explored for robotics Designed to transform RGB data to a color space more closely duplicates the response of the human eye Used in biomedical imaging
19
A bar of chart of data Size of each bar is the number of data points whose value falls into the range for the bar, called bin (buckets) Constructing histogram
3
We concentrate on two types of images frequently used in computer vision
Intensity
images
Photographlike images encoding light intensities
Range
images
Computer Vision
Computer Vision
Chapter 6.6 – 6.8
An image is a huge array of gray level (brightness) values of individual pixels Taken individually, these numbers are almost meaningless, because they contain very little information about the scene A robot needs information like "object ahead", "table to the left", or "person approaching" to perform its tasks The conversion of this huge amount of low level information into usable high level information is the subject of computer vision
Challenging for robotics
11
12
SCT Representations
(Spherical Coordinate Transform)
Color Representations
RGB
Currently being explored for robotics Designed to transform RGB data to a color space more closely duplicates the response of the human eye Used in biomedical imaging
19
A bar of chart of data Size of each bar is the number of data points whose value falls into the range for the bar, called bin (buckets) Constructing histogram
3
We concentrate on two types of images frequently used in computer vision
Intensity
images
Photographlike images encoding light intensities
Range
images
Computer Vision
Computer Vision
Chapter 6.6 – 6.8
An image is a huge array of gray level (brightness) values of individual pixels Taken individually, these numbers are almost meaningless, because they contain very little information about the scene A robot needs information like "object ahead", "table to the left", or "person approaching" to perform its tasks The conversion of this huge amount of low level information into usable high level information is the subject of computer vision
《计算机视觉》PPT课件
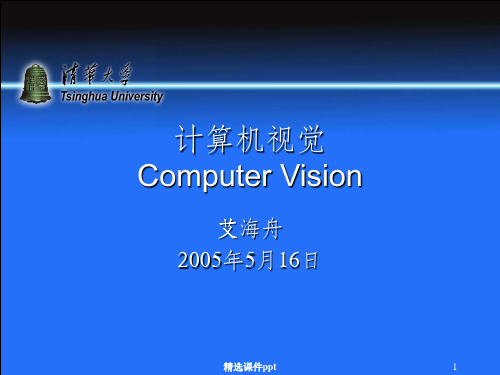
fucntion)
精选课件ppt
11
Overview (3)
计算机视觉的图像模型基础
✓ 摄像机模型及其校准
▪ 内参数、外参数
✓ 图像特征
▪ 边缘、角点、轮廓、纹理、形状…
✓ 图像序列特征 (运动)
▪ 对应点、光流
精选课件ppt
12
Overview (4)
计算机视觉的信号处理层次
低层视觉处理
✓ 单图像:滤波/边缘检测/纹理
计算机视觉的基本的分析工具和数学模型 Signal processing approach: FFT, filtering, wavelets, … Subspace approach: PCA, LDA, ICA, … Bayesian inference approach: EM, Condensation/SIS/…, MCMC, …. Machine learning approach: SVM/Kernel machine, Boosting/Adaboost, NN/Regression, … HMM, BN/DBN, … Gibbs, MRF, …
✓ 多图像:几何/立体/从运动恢复仿射或透视结构 affine/perspective structure from motion
中层视觉处理
✓ 聚类分割/拟合线条、曲线、轮廓 clustering for segmentation, fitting line…
✓ 基于概率方法的聚类分割/拟合
✓ 跟踪 tracking
精选课件ppt
6
Tools
Intel OpenCV, IPL
✓ Camera calibration (Zhang Zhengyou’s method) ✓ Face detection (a variation of Viola’s) ✓ Motion analysis and object tracking
精选课件ppt
11
Overview (3)
计算机视觉的图像模型基础
✓ 摄像机模型及其校准
▪ 内参数、外参数
✓ 图像特征
▪ 边缘、角点、轮廓、纹理、形状…
✓ 图像序列特征 (运动)
▪ 对应点、光流
精选课件ppt
12
Overview (4)
计算机视觉的信号处理层次
低层视觉处理
✓ 单图像:滤波/边缘检测/纹理
计算机视觉的基本的分析工具和数学模型 Signal processing approach: FFT, filtering, wavelets, … Subspace approach: PCA, LDA, ICA, … Bayesian inference approach: EM, Condensation/SIS/…, MCMC, …. Machine learning approach: SVM/Kernel machine, Boosting/Adaboost, NN/Regression, … HMM, BN/DBN, … Gibbs, MRF, …
✓ 多图像:几何/立体/从运动恢复仿射或透视结构 affine/perspective structure from motion
中层视觉处理
✓ 聚类分割/拟合线条、曲线、轮廓 clustering for segmentation, fitting line…
✓ 基于概率方法的聚类分割/拟合
✓ 跟踪 tracking
精选课件ppt
6
Tools
Intel OpenCV, IPL
✓ Camera calibration (Zhang Zhengyou’s method) ✓ Face detection (a variation of Viola’s) ✓ Motion analysis and object tracking
Class01 computer vision 计算机视觉
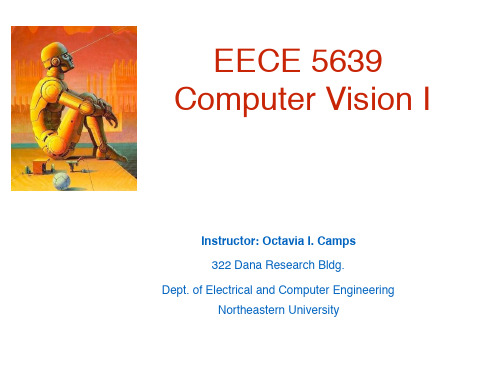
/sites/default/files/pdfs/coe/gse/Proctor_Form.pdf
If you can, I prefer that you come to school for the exams.
16
GRADING
Optional Extra Credit Project 5%
Please Read Video Streaming Policies & Procedures:
/sites/default/files/pdfs/coe/gse/policies_procedures.pdf
Make Arrangements for a proctor by submitting a Proctor Approval Form before January 22.
Henry Schneiderman
22
Face Recognition
1985
2002
23
surveillance
sports
Fire detection
medical
Challenge:
To develop human-level capabilities for computers and robots.
Tentative dates: 2/5/164 and 3/22/16
Final Exam 30%
Date TBA
Exams are closed book, closed notes 1, 2, and 3 cheat sheets allowed, respectively
15
V35 STREAMED LECTURES
18
Cameras Everywhere...
If you can, I prefer that you come to school for the exams.
16
GRADING
Optional Extra Credit Project 5%
Please Read Video Streaming Policies & Procedures:
/sites/default/files/pdfs/coe/gse/policies_procedures.pdf
Make Arrangements for a proctor by submitting a Proctor Approval Form before January 22.
Henry Schneiderman
22
Face Recognition
1985
2002
23
surveillance
sports
Fire detection
medical
Challenge:
To develop human-level capabilities for computers and robots.
Tentative dates: 2/5/164 and 3/22/16
Final Exam 30%
Date TBA
Exams are closed book, closed notes 1, 2, and 3 cheat sheets allowed, respectively
15
V35 STREAMED LECTURES
18
Cameras Everywhere...
计算机视觉课件8-PPT精品文档30页

a) Select knot position from each polyline segment.
K i (1 v i)V i v iV i 1
b)
wherevi
i
i i1
b) Form lines of guided frame:
KiVi+1: Vi+1Ki+1: KiKi+1:
discontinuous point of s plot.
3) Graph representation:
4) Establish topology relations among edge list points. 5) Each edge point is a graph node.
Struct Node {
b) Fit line segment (say, using least- square method) between the first and the last edge points of the sub list.
c) If normalized maximum error is too large, shorten the sub list at the point of maximum error. Return step b).
3. Curve fitting
Curve fitting is trying to find a smooth curve expression of mathematic form from curve’s polyline expression.
1) Conic sections:
f( x ,y ) a 2 b x c x 2 d y y e x f y 0
Advances in Computer Vision(ppt)

13
Enhanced Perception
Viewing by hearing
• The Voice
P. B. L. Meijer Future Design Technologies Philips Research Laboratories /
Advances in Computer Vision
Massimo Piccardi
Department of Computer Systems, Faculty of Information Technology, University of Technology, Sydney (UTS) e-mail: massimo@.au
• more sophisticated approaches, higher accuracy
New applications are possible
6
Advances in Computer Vision: humans as targets
In recent years, humans have become more and more the target of computer vision applications (see for instance the ICPR 2002 final program) Analysing human actions and behavior is a complex task Modern computer vision systems have provided the required computational power in a cost-effective way
Source: Face Recognition Project at VASC/CMU
Enhanced Perception
Viewing by hearing
• The Voice
P. B. L. Meijer Future Design Technologies Philips Research Laboratories /
Advances in Computer Vision
Massimo Piccardi
Department of Computer Systems, Faculty of Information Technology, University of Technology, Sydney (UTS) e-mail: massimo@.au
• more sophisticated approaches, higher accuracy
New applications are possible
6
Advances in Computer Vision: humans as targets
In recent years, humans have become more and more the target of computer vision applications (see for instance the ICPR 2002 final program) Analysing human actions and behavior is a complex task Modern computer vision systems have provided the required computational power in a cost-effective way
Source: Face Recognition Project at VASC/CMU
计算机视觉--3D Computer Vision ppt课件
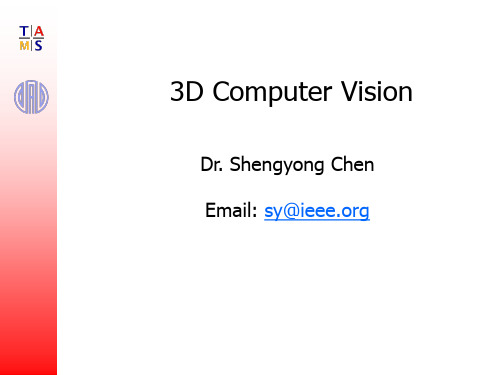
ppt课件
22
Vanishing points and lines
Parallel lines in the world intersect in the image at a “vanishing point”
ppt课件
23
Vanishing points and lines
Vanishing Line
Vanishing Pointo
oVanishing Point
ppt课件
24
Vanishing points and lines
Vanishing line
Vertical vanishing point
(at infinity)
Vanishing point
Slide from Efros, Photo from Criminisi
• Many methods have been developed using this approach. • Major advantage -- simple to use. • Low spatial resolution -- patterns become sparser with
distance. • Some close range (4cm) sensors exist with good depth
have an inadequate depth resolution (1cm at best) for most practical industrial vision purposes.
29
Structured Light Methods
• Project patterns of light (grids, stripes, elliptical patterns
最新Computer Vision Multiview Stereo计算机视觉的多视点立体幻灯片课件
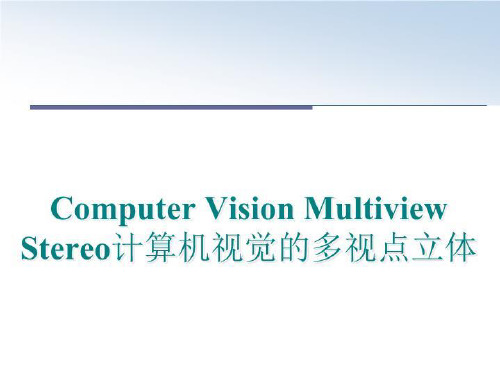
Stereo image rectification
computervisionmultiviewstereo计算机视觉的多视点立体论文总结英语资料ppt文档免费阅读免费分享如需请下载
3D Computer Vision
The main goal here is to reconstruct geometry of 3D worlds.
How can we estimate the camera parameters?
Nonlinear camera calibration
Perspective projection:
ui fx
vi
0
1 0
fy 0
uv00•rr12TT 1 r3T
ttt132•xzy1iii
Nonlinear camera calibration
Perspective projection:
- known 3D points on calibration targets
- find corresponding 2D points in image using feature detection algorithm
Camera parameters
Known 3D coords and 2D coords
• D. Scharstein and R. Szeliski. A taxonomy and evaluation of dense two-frame stereo correspondence algorithms. International Journal of Computer Vision, 47(1/2/3):742, April-June 2002.
《计算机视觉》课件 (2)

《计算机视觉》PPT课件 (2)
计算机视觉是指计算机通过模拟人类视觉系统的方式,利用摄像机和计算机 算法来实现对图像和视频的理解与处理。
计算机视觉的概述
定义与发展
探索计算机和图像处理技术的交叉领域,起 源于20世纪60年代。
技术基础
图像处理、模式识别、机器学习等。
关键任务
图像识别、目标检测、运动跟踪、立体重建 等。
虚拟现实
计算机视觉技术为虚拟现实提 供更真实和沉浸式的体验。
总结和重点
计算机视觉的定义与发展历程。 计算机视觉面临的挑战和限制。
计算机视觉的核心技术和应用领域。 计算机视觉的未来发展趋势。
安防
人脸识别、行为检测等。
工业
质检、自动化生产等。
计算机视觉的挑战和限制
1 复杂场景
2 准确性
光照变化、遮挡等导致图像处理的困难。
目标识别和跟踪的精确度有待提高。
3 隐私问题
人脸识别等技术引发的隐私争议。
4 算力需求
大规模图像和视频分析对计算资源的需求 高。
计算机视觉在实际生活中的应用
1
人脸识别技术
应用广泛
医学影像分析、智能监控、自动驾驶等。
计算机视觉中的核心技术
1 图像预处理
去噪、增强、图像配准等。
3 目标检测与识别
基于机器学习的特征分类和模式匹配。
2 特征提取和描述
边缘检测、角点检测、特征描述子等。
4 立体视觉
利用多个图像重建场景的三维模型。
计算机视觉应用领域
医疗
病变检测、肿瘤分析等。
人脸解锁、相册自动分类等智能手机
增强现实
2
应用。
通过摄像头将虚拟物体叠加到真实世
计算机视觉是指计算机通过模拟人类视觉系统的方式,利用摄像机和计算机 算法来实现对图像和视频的理解与处理。
计算机视觉的概述
定义与发展
探索计算机和图像处理技术的交叉领域,起 源于20世纪60年代。
技术基础
图像处理、模式识别、机器学习等。
关键任务
图像识别、目标检测、运动跟踪、立体重建 等。
虚拟现实
计算机视觉技术为虚拟现实提 供更真实和沉浸式的体验。
总结和重点
计算机视觉的定义与发展历程。 计算机视觉面临的挑战和限制。
计算机视觉的核心技术和应用领域。 计算机视觉的未来发展趋势。
安防
人脸识别、行为检测等。
工业
质检、自动化生产等。
计算机视觉的挑战和限制
1 复杂场景
2 准确性
光照变化、遮挡等导致图像处理的困难。
目标识别和跟踪的精确度有待提高。
3 隐私问题
人脸识别等技术引发的隐私争议。
4 算力需求
大规模图像和视频分析对计算资源的需求 高。
计算机视觉在实际生活中的应用
1
人脸识别技术
应用广泛
医学影像分析、智能监控、自动驾驶等。
计算机视觉中的核心技术
1 图像预处理
去噪、增强、图像配准等。
3 目标检测与识别
基于机器学习的特征分类和模式匹配。
2 特征提取和描述
边缘检测、角点检测、特征描述子等。
4 立体视觉
利用多个图像重建场景的三维模型。
计算机视觉应用领域
医疗
病变检测、肿瘤分析等。
人脸解锁、相册自动分类等智能手机
增强现实
2
应用。
通过摄像头将虚拟物体叠加到真实世
计算机视觉技术 ppt课件
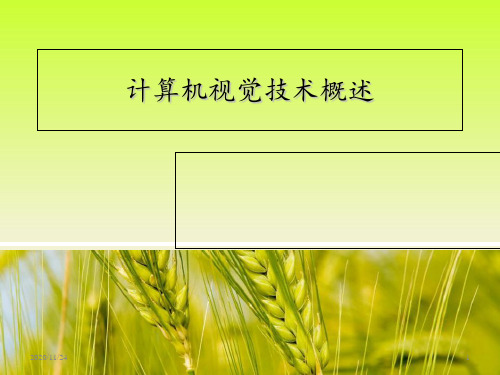
2020/11/24
13
计算机视觉的发展趋势
目前,过去由于CPU处理能力强大,可以进行较为 复杂的图像处理,并且一个Pc可支持多个相机进行多 方位的检测,因此PC Based方案受到了广大厂商的青 睐。目前国内多数厂商对计算机视觉的认识,已不仅 仅停留在PC Based方案层面。嵌入式方案越来越引起 厂商们的重视,其具有更大的灵活性,成本又低于PC Based方案,就抗干扰能力来说,嵌入式方案也更能适 应工业环境的电子干扰、温度变化、供电电压波动等 多种干扰,因此,目前计算机视觉正在向嵌入式的方 向发展。
2020/11/24
12
5. 交通: 汽车车牌识别、高速公路收费、违章闯红灯检 测、交通管制系统等。采用智能交通管理系统,通过在 交通要道放置摄像头,当有违章车辆(如闯红灯) 时,摄像 头将车辆的牌照拍摄下来,传输给中央管理系统,系统利 用图像处理技术,对拍摄的图片进行分析,提取出车牌号, 存储在数据库中,可以供管理人员进行检索。 6. 商标管理:可以建立商标图像库,利用图像检索技术, 对新申请的商标与图像库里的注册商标进行分析,检查 是否设计相似或雷同。
计算机视觉技术概述
2020/11/24
1
学习内容:
★计算机视觉技术的定义 ★计算机视觉技术的发展 ★计算机视觉技术的应用 ★计算机视觉技术的图像处理方法 ★计算机视觉技术的发展趋势
2020/11/24
2
精品资料
• 你怎么称呼老师?
• 如果老师最后没有总结一节课的重点的难点,你 是否会认为老师的教学方法需要改进?
• 你所经历的课堂,是讲座式还是讨论式? • 教师的教鞭
• “不怕太阳晒,也不怕那风雨狂,只怕先生骂我 笨,没有学问无颜见爹娘 ……”
《计算机视觉》PPT课件

实例:雷达测距系统
computer vision
3
7.1 立体视觉基础
被动测距方法
双目视觉系统:使用两个相隔一定距离的 摄像机同时获取场景图像来生成深度图。
单目运动视觉:一个摄像机在不同空间位 置上获取两幅或两幅以上图像,通过多幅 图像的灰度信息和成像几何来生成深度图
特征深度测量:使用灰度图象的明暗特征、 纹理特征、运动特征间接的估算深度信息。
14
7.2 立体成像
依据双目立体视觉几何关系的深度计算
结合以下公式:
x
x
l
zF
x B x r zF
可以得到: z B F x l x r
其中F是焦距,B是基线距离, xl 是xr 视差。
各种场景中的点的深度就可以通过计算视差来实 现。视差一般是整数。
对于一组给定的摄像机参数,提高场景点深度计
即使两个摄像机处于一般的位置和方向时, 对应场景点的两个图像点仍然位于图像平 面和外极平面的交线(外极线)上。
computer vision
20
7.3 立体成像
从原理上讲根据“立体图象对”抽取深度信息的 处理应包括以下四部分:
在图象中寻找在两幅图象中都便于区分的特征或 用于匹配的基元(primitive)。
立体匹配的匹配规则约束
立体匹配:立体成像的深度信息测量的一个重要 步骤就是寻找立体成像对中的共轭对,即求解对 应问题。
问题:实际中求解对应问题是非常困难的,一是 计算量大,二是匹配的准确度要求高。
解决:为了求解对应,建立了许多约束来减少对 应点误匹配,并最终得到正确的匹配特征点的对 应。
computer vision
computer vision
4
《计算机视觉》课件

特征提取方法
学习常用的特征提取方法, 如边缘检测、角点检测和 纹理描述子等。
分类器的选择与训练
了解不同的分类器及其应 用,学会使用机器学习算 法对图像进行分类。
第三部分:视觉任务
图像分类
学习图像分类的基本概念、传 统方法和深度学习方法,以及 其在各个应用领域中的应用。
对象检测
掌握对象检测的基本概念、传 统方法和深度学习方法,了解 对象检测在不同领域的应用。
《计算机视觉》PPT课件
计算机视觉是研究如何使机器“看”的技术。本课程将帮助您全面了解计算机 视觉的定义、应用领域以及其历史和发展。
第一部分:简介
什么是计算机视觉?计算机视觉的应用领域及其历史和发展。
第二部分:基础知识
图像处理基础
掌握图像处理的基本概念 和常用技术,包括滤波、 增强、几何变换等。
3
计算机视觉技术的发展趋势
分析计算机视觉技术的发展趋势,包括硬件设备的进步、深度学习的发展和数据 集的丰富等。
结束语
总结课程内容并鼓励学生主动探索计算机视觉领域的未来发展方向,以提升技术水平和应用能力。
语义分割
了解语义分割的基本概念、传 统方法和深度学习方法,探索 语义分割在各个应用领域中的 意义。
第四部分:计算机视觉技术的未来
1
计算机视觉技术的未来发展方向
展望计算机视觉技术的未来发展趋势,如智能交通、医疗诊断和智能安防等领域。
2
可能的应用பைடு நூலகம்域与场景
探讨计算机视觉技术在各个行业中的可能应用,如无人驾驶、人脸识别和机器人 导航等。
winter1516_lecture1 computer vision class 机器视觉 课件

Fei-Fei Li & Andrej Karpathy & Justin Johnson
Lecture 1 -
25
4-Jan-16
CS231n focuses on one of the most important problems of visual recognition – image classification
20
4-Jan-16
PASCAL Visual Object Challenge (20 object categories)
[Everingham et al. 2006-2012]
1
all aeroplane bicycle bird boat bottle bus car cat chair cow diningtable dog horse motorbike person pottedplant sheep sofa train tvmonitor
0.9
Average Precision
0.8
0.7
0.6
0.5
0.4
2009
2010
2011
2012
Challenge Year
Fei-Fei Li & Andrej Karpathy & Justin Johnson
Lecture 1 -
21
4-Jan-16
22K categories and 14M images
Fei-Fei Li & Andrej Karpathy & Justin Johnson
Lecture 1 -
26
4-Jan-16
Fei-Fei Li & Andrej Karpathy & Justin Johnson
计算机视觉第一章 绪论 PPT
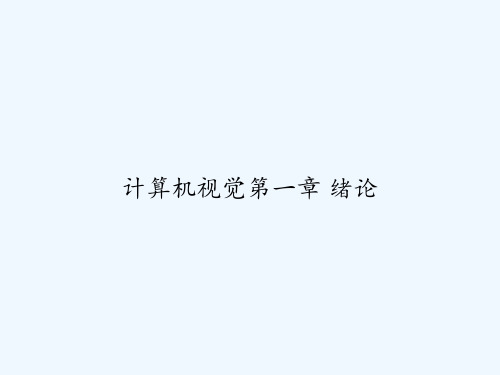
特征提取I
(3.20,3.23) 点特征
(5 lectures) 特征提取II 边缘及线特征
Week 5
图像分割I
(3.27,3.30) 主动轮廓线
(5 lectures) 图像分割II Mean shift
Week 6
图像对准
(4.3,4.8)
(5 lectures) 摄像机标定
Week 7
单幅图像深度估
知识导引: 同样的图像在不同的知识导引下,将会产生 不同的识别结果.
大量数据: 灰度图像,彩色图像,深度图像的信息量十 分巨大,巨大的数据量需要很大的存贮空间,同时不易实 现快速处理.
上世纪50年代,从统计模式识别开始,主要集中 在二维图像分析与识别,主要应用包括字符识别、 工件表面检测等等。
(5(3.1l5e,ctu5r.1es8) ) 点特征
(5 le54c.1tu02res) 稠布特密置征运作提动业取估II 计 ((22 lleeccttuurreess)) 光边流缘及线特征
考 阅读报告:两人一组,从30篇计算机视 核 觉的经典文献中挑选一篇阅读,并提交 方 一份阅读报告及PPT。 式 项目报告:2-4人一组,从五个候选项目
(5 le43c.12tu92res) 图测视像距觉分成基割像本系I特I 统性I ((22 lleeccttuurreess)) M生e物an特s性hift
We43e.2k4710 图劳视像动觉对节基准放本假特性II (((353.l1leecc,ttuu5rr.ee4ss))) 物理特性
(5 le43c.2tu69res) 摄三视像维觉机重基标建本定I特性III ((22 lleeccttuurreess)) 几何特性
硬件实现,就是具体的计算装置和一些细节配置
计算机视觉课件14
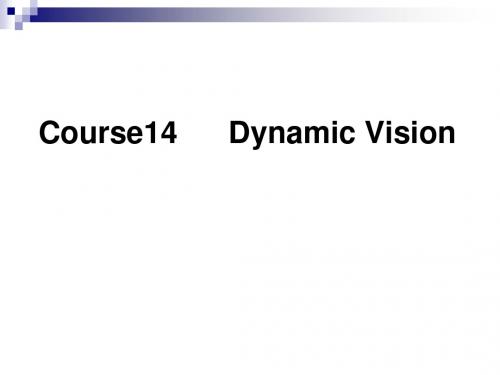
1) Point Matching (relaxation labeling) Given: a set of feature points in image frame 1 and another set of feature point in image 2. Find: a unique correspondences of points between the two sets of points.
image sequence, moving points are not so. Thus, moving
points and stationary points can be separated.
2. 3D structure from motion
3D structure from images means to find the depth of scene of the corresponding feature points in image.
Computer vision does in the similar ways. But we have only focus on: ----- Stationary camera, stationary objects ----- Stationary camera, moving objects ----- Moving camera, stationary objects ----- Moving camera, moving objects
d tan1 z
dv d
x z
a
dz dz
dz
y b
d z
1 y
b
image sequence, moving points are not so. Thus, moving
points and stationary points can be separated.
2. 3D structure from motion
3D structure from images means to find the depth of scene of the corresponding feature points in image.
Computer vision does in the similar ways. But we have only focus on: ----- Stationary camera, stationary objects ----- Stationary camera, moving objects ----- Moving camera, stationary objects ----- Moving camera, moving objects
d tan1 z
dv d
x z
a
dz dz
dz
y b
d z
1 y
b
- 1、下载文档前请自行甄别文档内容的完整性,平台不提供额外的编辑、内容补充、找答案等附加服务。
- 2、"仅部分预览"的文档,不可在线预览部分如存在完整性等问题,可反馈申请退款(可完整预览的文档不适用该条件!)。
- 3、如文档侵犯您的权益,请联系客服反馈,我们会尽快为您处理(人工客服工作时间:9:00-18:30)。
At the same time, AI MIT laboratory has attracted many famous scholars from the world to participate in the research of machine vision,which included the theory of machine vision , algorithm and design of system . Professor David Marr in 1973 was invited to lead a research team which is consisted of doctoral students in the MIT AI Lab .in 1977,David Marr put forward a new computer vision theory which is different from the past theory which is build on the analysis method of "blocks world".in the 1980s,his theory had become a very important theoretical framework in the field of machine vision .
Later, people began to makes a deep study of block world and establish a variety of data structures and inference rules.The
scope of the study is abroad,which include the feature extraction
Stage 1: Research on the visual basic method ,which take the model world as the main object; Stage 2: Research on computational theory; visual model ,which is based on the
the research scope of the block world is extensive. it includes the feature extraction of edge and corner,the geometric factor analysis of line and plane.It also build a variety of data structures and inference rules. In the middle of 70's,artificial intelligence (AI) laboratory of Massachusetts Institute of Technology (MIT) officially opened the "machine vision" course.
In the work of Roberts, the relationship between 3D objects and 2D imaging is introduced, and some simple methods of feature extraction are introduced.
2The second purpose is to calculate the Motion parameters from the observation point to the object according to one or more 2D images; 3 T h e third purpose is to c a l c u l a t e t h e p h y s i c a l characteristics from the observation point to the object according to one or more 2D images; There are two ultimate goal that computer vision will realize, they can be simply described as follow:
the simulation of human visual perception generation of computer vision.
Specifically, purpose. computer vision will
leads to the
achieve three basic
1The first purpose is to calculate the distance from the observation point to the object according to one or more 2D images;
Computer Vision
Computer vision research
At the end of the 1950s, computers had became an important tool for human beings to realize the human intelligence and human perception, with the help of computer, For the first time, human beings can realize the extension of mental and perceptual ability as well as the extension of physical strength with the aid of the machine.
In 1991, the publication is divided into two versions, which are CVGIPGMIP (graphics model and image processing) and CVGIP-IU (image underuter vision research
After nearly 50 years, research in computer vision has made considerable achievements. This makes the field to become the most active part of the AI research in the past 40 years. Its mainstream research is divided into three stages:
In 1995, the publication become two magazine . the former named GMIP (graphics model and image processing), the latter named CVIU (computer vision and image understanding). The upsurge of research on computer vision began in 1980s, and in the middle of 80's, the computer vision has developed rapidly, new concepts, new methods and new theories have emerged. So far, computer vision is still a very active research area.
Changes in the name of a publication can reflect the progress of computer vision: 1972, CGIP (computer graphics and image processing) publication; In 1983, its name is processing); CVGIP (computer vision, graphics and image
1960s: Roberts (1965) use a computer program to extracte the threedimensional structure of a polyhedron from the digital images .he also describe the shape and the spatial relationship of objects.
Roberts created a new situation for the research of computer vision . his purpose of research began to become the understanding the threedimensional scene.
History of computer vision
1950s: in this period , statistical pattern recognition is most applied in computer vision , it mainly focuse on the analysis and identification of two-dimensional image,such as: optical character recognition, the surface of the workpiece, the analysis and interpretation of the aerial image.
Later, people began to makes a deep study of block world and establish a variety of data structures and inference rules.The
scope of the study is abroad,which include the feature extraction
Stage 1: Research on the visual basic method ,which take the model world as the main object; Stage 2: Research on computational theory; visual model ,which is based on the
the research scope of the block world is extensive. it includes the feature extraction of edge and corner,the geometric factor analysis of line and plane.It also build a variety of data structures and inference rules. In the middle of 70's,artificial intelligence (AI) laboratory of Massachusetts Institute of Technology (MIT) officially opened the "machine vision" course.
In the work of Roberts, the relationship between 3D objects and 2D imaging is introduced, and some simple methods of feature extraction are introduced.
2The second purpose is to calculate the Motion parameters from the observation point to the object according to one or more 2D images; 3 T h e third purpose is to c a l c u l a t e t h e p h y s i c a l characteristics from the observation point to the object according to one or more 2D images; There are two ultimate goal that computer vision will realize, they can be simply described as follow:
the simulation of human visual perception generation of computer vision.
Specifically, purpose. computer vision will
leads to the
achieve three basic
1The first purpose is to calculate the distance from the observation point to the object according to one or more 2D images;
Computer Vision
Computer vision research
At the end of the 1950s, computers had became an important tool for human beings to realize the human intelligence and human perception, with the help of computer, For the first time, human beings can realize the extension of mental and perceptual ability as well as the extension of physical strength with the aid of the machine.
In 1991, the publication is divided into two versions, which are CVGIPGMIP (graphics model and image processing) and CVGIP-IU (image underuter vision research
After nearly 50 years, research in computer vision has made considerable achievements. This makes the field to become the most active part of the AI research in the past 40 years. Its mainstream research is divided into three stages:
In 1995, the publication become two magazine . the former named GMIP (graphics model and image processing), the latter named CVIU (computer vision and image understanding). The upsurge of research on computer vision began in 1980s, and in the middle of 80's, the computer vision has developed rapidly, new concepts, new methods and new theories have emerged. So far, computer vision is still a very active research area.
Changes in the name of a publication can reflect the progress of computer vision: 1972, CGIP (computer graphics and image processing) publication; In 1983, its name is processing); CVGIP (computer vision, graphics and image
1960s: Roberts (1965) use a computer program to extracte the threedimensional structure of a polyhedron from the digital images .he also describe the shape and the spatial relationship of objects.
Roberts created a new situation for the research of computer vision . his purpose of research began to become the understanding the threedimensional scene.
History of computer vision
1950s: in this period , statistical pattern recognition is most applied in computer vision , it mainly focuse on the analysis and identification of two-dimensional image,such as: optical character recognition, the surface of the workpiece, the analysis and interpretation of the aerial image.