计量经济学(英文版)ppt课件
合集下载
计量经济学(英文PPT)Chapter 16 Panel Data Regression Models

Let the intercept vary for each company but still assume that the
slope coefficients are constant across firm.We write (16.2.1) as :
Yit =β1i +β2X2i +β3X3i +uit This is known as the fixed effects model(FEM).
• 5.Panel data enables us to study more complicated behavioral models. Such as economies of scale and technological change.
• 6.Panel data can minimize the bias. The bias might occur when we combine different types of data (such as the data of different provinces or different years).
• 2.The slope coefficients are constant but the intercept varies over individuals.
• 3.The slope coefficients are constant but the intercept varies over individual and time.
We assume that the X's are nonstochastic and
the error term uit ~ N(0, σ2).
计量经济学(英文PPT)Chapter 21 Time Series Econometrics ⅠStationarity Unit roots and Cointegration

6
k
k
0
covariance at lag k variance
obviously when k 0, 1 0
1 1 k
plot against k, the graph we obtain is known as the population correlogram k
• Returning to the example given in figure 21.8,the value of the Q statistic up to lag 25 is about 793,the LB statistic is about 891,both are highly significant, the probability of obtaining such a high
• We can rewrite the functions above as,
Yt ( 1)Yt1 ut
(21.9.1)
• or,
• Yt Yt 1 ut
(-1.96*0.1066,1.96*0.1066) or (0.2089,0.2089)
10
• In figure 21.8,the left two lines of dots represent the 95% confidence interval.
the joint hypothesis test of k H0 : all the k are simultaneo usly equal to zero.
• This can be done by using the Q statistic developed by Box and Pierce,
k
k
0
covariance at lag k variance
obviously when k 0, 1 0
1 1 k
plot against k, the graph we obtain is known as the population correlogram k
• Returning to the example given in figure 21.8,the value of the Q statistic up to lag 25 is about 793,the LB statistic is about 891,both are highly significant, the probability of obtaining such a high
• We can rewrite the functions above as,
Yt ( 1)Yt1 ut
(21.9.1)
• or,
• Yt Yt 1 ut
(-1.96*0.1066,1.96*0.1066) or (0.2089,0.2089)
10
• In figure 21.8,the left two lines of dots represent the 95% confidence interval.
the joint hypothesis test of k H0 : all the k are simultaneo usly equal to zero.
• This can be done by using the Q statistic developed by Box and Pierce,
计量经济学(英文版)精品PPT课件
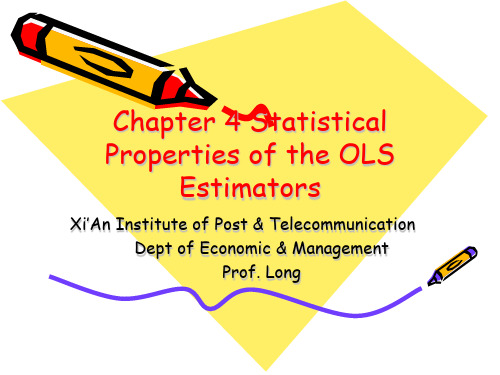
(4.3a)
Expand and multiply top and bottom by n:
b2
=
nSxiyi - Sxi Syi nSxi2-(Sxi) 2
(4.3b)
Variance of b2
4.12
Given that both yi and ei have variance s2,
the variance of the estimator b2 is:
4. cov(ei,ej) = cov(yi,yj) = 0 5. xt c for every observation
6. et~N(0,s 2) <=> yt~N(b1+ b2xt,
The population parameters b1 and b2 4.4 are unknown population constants.
b2
+
nSxiEei - Sxi SEei nSxi2-(Sxi) 2
Since Eei = 0, then Eb2 = b2 .
An Unbiased Estimator
4.8
The result Eb2 = b2 means that the distribution of b2 is centered at b2.
4.6
The Expected Values of b1 and b2
The least squares formulas (estimators) in the simple regression case:
b2 =
nSxiyi - Sxi Syi nSxi22 -(Sxi) 2
b1 = y - b2x
计量经济学(英文PPT)Chapter 11 HETEROSCEDASTICITY
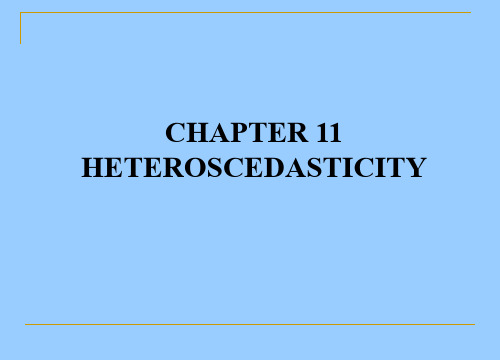
n n
X iYi
X
2 i
(
X i Yi Xi )2
(11.2.1)
under the assumption of heterscedasticity namely:
var(2 ) (
xi2
2 i
xi2 )2
(11.2.2) return
under the assumption of homoscedasticity namely::
(11.2.2)
The Method of Generalized Least
Squares(GLS)
The usual OLS method does not make use of the information, but GLS(generalized least squares) take such information into account
Consequences of Using OLS in the Presence of Heteroscedasticity
Suppose
we
use
2
,
and
use
the
variance
formula
given
in
(11.2.2),
which takes into account heteroscedasticity explicitly.
2
ui (Yi 1 2 Xi )2
(11.3.10)
But in GLS we minimize the expression(11.3.7),
which can also be written as:
计量经济学(英文PPT)Chapter 22 TIME SERIES CONOMETRICS FORECASTING
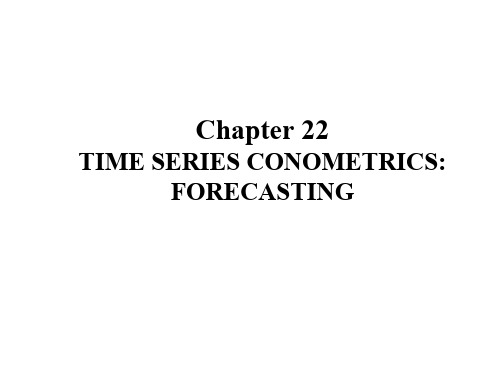
1.Single Exponential Smoothing 2.Holt’s Linear Method 3.Holt winter’s Method
and their variations.
We will not discuss them in this chapter.
§22.1 APPROACHES TO ECONOMIC
Step3.Diagnostic checking. That is, test whether the chosen model fits
the data reasonably well. Choosing the right model needs not only
the science,but also considerable skills and the art.
The prerequisite of using BJ methodology to model an ARMA process,is that we must have either a stationary time series or a time series that is stationary after one or more differencings.
In short,a moving average process is simply a linear combination of white noise error term.
Autoregressive and Moving Average (ARMA) Process
It is quite likely that Y has characteristics of both
form knowledge directly perceived through the senses
and their variations.
We will not discuss them in this chapter.
§22.1 APPROACHES TO ECONOMIC
Step3.Diagnostic checking. That is, test whether the chosen model fits
the data reasonably well. Choosing the right model needs not only
the science,but also considerable skills and the art.
The prerequisite of using BJ methodology to model an ARMA process,is that we must have either a stationary time series or a time series that is stationary after one or more differencings.
In short,a moving average process is simply a linear combination of white noise error term.
Autoregressive and Moving Average (ARMA) Process
It is quite likely that Y has characteristics of both
form knowledge directly perceived through the senses
《计量经济学英文版》课件

2 ARMA模型
介绍ARMA模型的基本原 理和参数估计方法,以及 ARMA模型在经济数据分 析中的应用。
3 GARCH模型
讲解GARCH模型的原理 和估计方法,以及 GARCH模型在金融市场 波动预测中的应用。
计量经济模型的应用
金融市场分析
应用计量经济模型进行金融市 场的预测和分析。
政策评估
利用计量经济模型评估政策的 效果和影响。
面板数据模型
1
面板数据概述
介绍面板数据的特点和应用领域,以及面板数据模型的基本概念。
2
固定效应模型
讲解固定效应模型的估计和推断方法,以及固定效应模型的优缺点。
3
随机效应模型
介绍随机效应模型的估计和推断方法,以及随机效应模型与固定效应模型的比较。
时间序列模型
1 时间序列数据基本特
征
描述时间序列数据的基本 特征,如趋势、季节性和 周期性。
《计量经济学英文版》 PPT课件
本课程介绍了计量经济学的基本概念和分析方法。涵盖了经济数据与计量分 析、经典线性回归模型、面板数据模型、时间序列模型和计量经济模型的应 用。
课程概述
本节将概述本课程的内容,包括学习目标和涵盖的主要内容。通过本课程的学习,您将掌握计量经济学的基本 理论和实际应用。
经济数据与计量分析
企业ቤተ መጻሕፍቲ ባይዱ策
运用计量经济模型辅助企业的 决策制定。
总结
通过本课程的学习,您将获得计量经济学分析的基本知识和技能,能够应用计量经济模型进行实证研究,并在 实际问题中运用计量经济学的方法和工具。
• 经济数据的概念与特点 • 统计概率基础 • 经济计量分析的目的与意义
经典线性回归模型
普通最小二乘法
计量经济学英文课件共35页

8
One-Sided Alternatives (cont)
Having picked a significance level, a, we look up the (1 – a)th percentile in a t distribution with n – k – 1 df and call this c, the critical value We can reject the null hypothesis if the t statistic is greater than the critical value If the t statistic is less than the critical value then we fail to reject the null
Under the CLM assumptions, conditional on the sample values of the independent variable s
bˆ j ~ Normal b j ,Var bˆ j , so that
bˆ j b j sd bˆ j ~ Normal 0,1
7
t Test: One-Sided Alternatives
Besides our null, H0, we need an alternative hypothesis, H1, and a significance level H1 may be one-sided, or two-sided
because we have to estimate s 2by sˆ 2
Note the degrees of freedom : n k 1
5
The t Test (cont)
One-Sided Alternatives (cont)
Having picked a significance level, a, we look up the (1 – a)th percentile in a t distribution with n – k – 1 df and call this c, the critical value We can reject the null hypothesis if the t statistic is greater than the critical value If the t statistic is less than the critical value then we fail to reject the null
Under the CLM assumptions, conditional on the sample values of the independent variable s
bˆ j ~ Normal b j ,Var bˆ j , so that
bˆ j b j sd bˆ j ~ Normal 0,1
7
t Test: One-Sided Alternatives
Besides our null, H0, we need an alternative hypothesis, H1, and a significance level H1 may be one-sided, or two-sided
because we have to estimate s 2by sˆ 2
Note the degrees of freedom : n k 1
5
The t Test (cont)
计量经济学(英文PPT)Chapter 15 Qualitative Response Regression Model
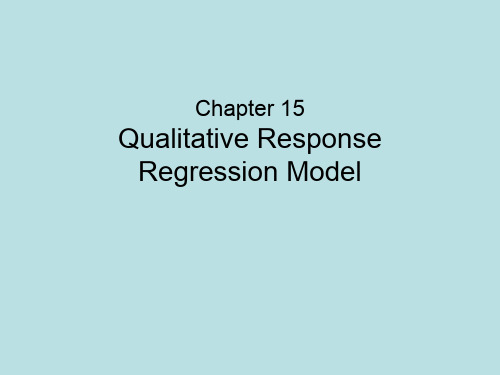
The probability distribution of ui is :
Obviously, they follow the Bernoulli distribution. The OLS point estimates still remain unbiased. As the sample size increases indefinitely, the OLS estimators tend to be normally distributed generally.
Ask: Eui ? var(ui) ?
2. Heteroscedastic Variances of the Disturbances.
Answer : Eui Pi(1 1 2 Xi) (1 Pi)(1 1 2 Xi)
Pi 1 2 Xi
• Step 1: Run the OLS regression (15.2.1) despite the
heteroscedasticity problem and obtain Yi = estimate of the true
E
(Yi
|
Xi).
Then
obtain
Wi
Yi(1 Yi)
• The qualitative variable can be divided into three kind.
1. Dichotomous Variable
for example:
if male, Y=1 if female, Y=0
if vote for the Democratic Party, Y=1 if vote for the Republican Party, Y=0
Obviously, they follow the Bernoulli distribution. The OLS point estimates still remain unbiased. As the sample size increases indefinitely, the OLS estimators tend to be normally distributed generally.
Ask: Eui ? var(ui) ?
2. Heteroscedastic Variances of the Disturbances.
Answer : Eui Pi(1 1 2 Xi) (1 Pi)(1 1 2 Xi)
Pi 1 2 Xi
• Step 1: Run the OLS regression (15.2.1) despite the
heteroscedasticity problem and obtain Yi = estimate of the true
E
(Yi
|
Xi).
Then
obtain
Wi
Yi(1 Yi)
• The qualitative variable can be divided into three kind.
1. Dichotomous Variable
for example:
if male, Y=1 if female, Y=0
if vote for the Democratic Party, Y=1 if vote for the Republican Party, Y=0
计量经济学英文课件 (22)
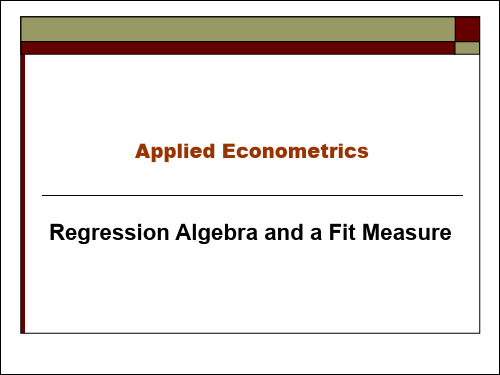
Comparing fits of regressions
Make sure the denominator in R2 is the same - i.e., same left hand side variable. Example, linear vs. loglinear. Loglinear will almost always appear to fit better because taking logs reduces variation.
o What are the practical implications of this result?
n Transformation does not affect the fit of a model to a body of
data.
n Transformation does affect the “estimates.” If b is an estimate
Dropping a variable(s) cannot improve the fit - that is, reduce the sum of squares.
Adding a variable(s) cannot degrade the fit - that is, increase the sum of squares.
Then, uu = (y - Xd)(y-Xd) = [y - Xb - X(d - b)][y - Xb - X(d - b)] = [e - X(d - b)] [e - X(d - b)]
Expand to find uu = ee + (d-b)XX(d-b) > ee
Dropping a Variable
计量经济学(英文PPT)Chapter 10 Multicollinearity
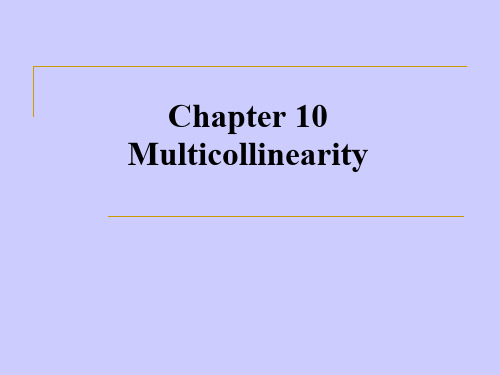
errors are infinite。To a three-variable regression model:
yi 2 x2i 3 x3i uˆi
(10.2.1)
From chapter 7 we obtain:
( 2
yi x2i )( x32i ) ( yi x3i )( x2i x3i ) ( x22i )( x32i ) ( x2i x3i )2
Estimation in the presence of perfect multicollinearity
In the case of perfect muiticollinearity the regression
coefficients remain indeterminate and their standard
Chapter 10 Multicollinearity
The Nature of Multicollinearity
Mean: originally it meant the existence of a perfect ,or exact, linear relationship among some or all explanatory
Theoretical consequences of multicollinearity
It is true that even in the case of near multicollinearity the OLS estimators are unbiased. But unbiasedness is a multisample or repeated sampling property Collinearity does not destroy the property of minimum variance. But this does not mean that the variance of an OLS estimator will necessarily be small in any given sample
计量经济学(英文PPT)Chapter 5 Interval Estimation and Hypothesis Testing

likely to differ from the true value, although E(ˆ2 ) 2 . Therefore, instead of
relying on the point estimate alone, we may construct a interval around the point estimate, such that this interval has a certain probability of the true parameter value. This is the idea behind the interval estimation.
ˆ2 t / 2se(ˆ2 )
Example: P123
Second , confidence interval for 1
By the virtue of E(ˆ1 ) 1 and
2 ˆ1 n
Xi2 xi 2
2
,we
can
get
the
equations
as
Equation(5.2.1)shows that the interval has a probability 1 of including the
true 2. The interval estimator thus give a ranger of values within which the true
§5.3confidence intervals for regression
Coefficients 1 and 2
First, confidence interval for 2
It is shown before that the OLS estimator ˆ1 and ˆ2 are themselves normally
relying on the point estimate alone, we may construct a interval around the point estimate, such that this interval has a certain probability of the true parameter value. This is the idea behind the interval estimation.
ˆ2 t / 2se(ˆ2 )
Example: P123
Second , confidence interval for 1
By the virtue of E(ˆ1 ) 1 and
2 ˆ1 n
Xi2 xi 2
2
,we
can
get
the
equations
as
Equation(5.2.1)shows that the interval has a probability 1 of including the
true 2. The interval estimator thus give a ranger of values within which the true
§5.3confidence intervals for regression
Coefficients 1 and 2
First, confidence interval for 2
It is shown before that the OLS estimator ˆ1 and ˆ2 are themselves normally
计量经济学(英文PPT)Chapter 2 TWO-VARIABLE REGRESSION ANALYSIS SOME BASIC IDEAS

As the X value increases, the conditional mean value of Y increases, too. The dark circled points in Figure 2.1 show the conditional mean values of Y against the various X values.
is a linear function of X i ,say, of the type
E(Y | X i ) 1 2 X i (2.2.2)
1 and 2 are known as the regression coefficients. Equation(2.2.2)itself is known as the linear population regression function or simply linear population regression.
If we want to get the relationship between weekly family consumption expenditure (Y) and weekly family income (X).
In the hypothetical community, there is a total population of 60 families. The 60 families are divided into 10 income groups (from $80 to $260) . And assume that we get the observations given in Table 2.1.
Therefore, we can express the deviation of an individual Yi around its expected value as follows:
is a linear function of X i ,say, of the type
E(Y | X i ) 1 2 X i (2.2.2)
1 and 2 are known as the regression coefficients. Equation(2.2.2)itself is known as the linear population regression function or simply linear population regression.
If we want to get the relationship between weekly family consumption expenditure (Y) and weekly family income (X).
In the hypothetical community, there is a total population of 60 families. The 60 families are divided into 10 income groups (from $80 to $260) . And assume that we get the observations given in Table 2.1.
Therefore, we can express the deviation of an individual Yi around its expected value as follows:
计量经济学(英文PPT)Chapter 12 Autocorrelation

The interpolation of data The extrapolation of data
OLS ESTMATION IN THE PRESENCE OF AUTOCORELATION
For two-variable regression model:
Yt 1 2 X t ut
(12.1.1)
In our book, autocorrelation and serial correlation shares the same meaning.
The hypothesis harms: the wrong
function form
If in the research of cost----out put ,the real model is:
t is stochastic disturbance term satisfied the following standard OLS assumption,
E(t) 0
var(t ) 2
(12.2.2)
cov(t ,ts ) 0 s 0
The scheme (12.2.1) is known as Markov first-order autoregressive scheme, or a first-order autoregressive scheme, denoted as AR(1)
Under the AR(1) scheme, it can be shown that the variance of estimator is:
(12.2.5)
var(2 )AR1
2
xt2
2 2
OLS ESTMATION IN THE PRESENCE OF AUTOCORELATION
For two-variable regression model:
Yt 1 2 X t ut
(12.1.1)
In our book, autocorrelation and serial correlation shares the same meaning.
The hypothesis harms: the wrong
function form
If in the research of cost----out put ,the real model is:
t is stochastic disturbance term satisfied the following standard OLS assumption,
E(t) 0
var(t ) 2
(12.2.2)
cov(t ,ts ) 0 s 0
The scheme (12.2.1) is known as Markov first-order autoregressive scheme, or a first-order autoregressive scheme, denoted as AR(1)
Under the AR(1) scheme, it can be shown that the variance of estimator is:
(12.2.5)
var(2 )AR1
2
xt2
2 2
计量经济学(英文PPT)Chapter 0 Introduction

consumption
is:2
,
namely,
-231.8
and
0.7194.
Thus,
the
estimated
Yˆ 231.8 0.7194 X
We see that,for the period 1980—1992, MPC≈0.72 in America, suggesting that for the sample period an increase in real income of 1 dollar led, on average, to an increase of about 72 cents in real
However, the establishment of World Econometric Society in December 29th, 1930 and the publication of its academic journal Econometrics in 1933 are generally acknowledged as a landmark of econometrics as a separate discipline.
Why a separate discipline?
Economic theory makes statements or hypotheses that are mostly qualitative in nature; It is the job of the econometrician to provide such numerical estimates.
Example: Keynesian theory of consumption
is:2
,
namely,
-231.8
and
0.7194.
Thus,
the
estimated
Yˆ 231.8 0.7194 X
We see that,for the period 1980—1992, MPC≈0.72 in America, suggesting that for the sample period an increase in real income of 1 dollar led, on average, to an increase of about 72 cents in real
However, the establishment of World Econometric Society in December 29th, 1930 and the publication of its academic journal Econometrics in 1933 are generally acknowledged as a landmark of econometrics as a separate discipline.
Why a separate discipline?
Economic theory makes statements or hypotheses that are mostly qualitative in nature; It is the job of the econometrician to provide such numerical estimates.
Example: Keynesian theory of consumption
计量经济学(英文PPT)Chapter 3 TWO-VARIABLE REGRESSION MODEL-THE PROBLEM OF ESTIMATION
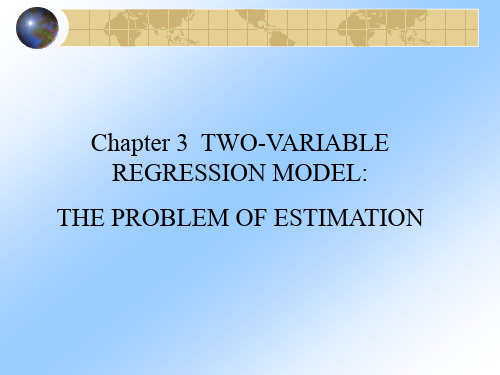
Chapter 3 TWO-VARIABLE REGRESSION MODEL:
THE PROBLEM OF ESTIMATION
§3.1 THE METHOD OF ORDINARY LEAST SQUARES
The Method of ordinary least squares is attributed to Carl Friedrich Gauss, a German mathematician.
Ⅱ.The estimators which are point estimators are different from interval estimators.
Ⅲ.Once the OLS estimators are obtained from the sample data, the sample regression line can be easily obtained. The regression line thus obtained has the following properties:
Yˆi ˆ1 ˆ2 X i (Y ˆ2 X ) ˆ2 X i Y ˆ2 ( X i X )
while ∵ (Xi X ) 0
∴sum the equation above for the sample value on both sides and divide the result through by n( sum for i,then dived by n),
least-squares estimators, for they are derived from the least-
squares principle.
note:
THE PROBLEM OF ESTIMATION
§3.1 THE METHOD OF ORDINARY LEAST SQUARES
The Method of ordinary least squares is attributed to Carl Friedrich Gauss, a German mathematician.
Ⅱ.The estimators which are point estimators are different from interval estimators.
Ⅲ.Once the OLS estimators are obtained from the sample data, the sample regression line can be easily obtained. The regression line thus obtained has the following properties:
Yˆi ˆ1 ˆ2 X i (Y ˆ2 X ) ˆ2 X i Y ˆ2 ( X i X )
while ∵ (Xi X ) 0
∴sum the equation above for the sample value on both sides and divide the result through by n( sum for i,then dived by n),
least-squares estimators, for they are derived from the least-
squares principle.
note:
计量经济学英文课件 (14)
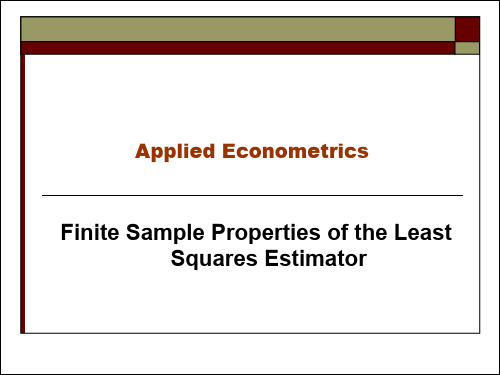
Nonnormal disturbances - Least Absolute Deviations and other nonparametric approache
Fixed X or Conditioned on X?
The role of the assumption of nonstochastic regressors
Finite sample results: Conditional vs. unconditional results
Its importance in the asymptotic results.
Specification Errors-1
Omitting relevant variables: Suppose the correct model is y = X11 + X22 + . I.e., two sets of variables. Compute least squares omitting X2. Some easily proved results:
MVLUE
1. Linear estimator =
n i1
vii
2. Unbiased: E[b|X] = β
Comparing positive definite matrices:
Var[c|X] – Var[b|X] is nonnegative definite for any other linear and unbiased estimator. What are the implications?
Other estimators
Biased estimation – a minimum mean squared error estimator. Is there a biased estimator with a smaller ‘dispersion’?
Fixed X or Conditioned on X?
The role of the assumption of nonstochastic regressors
Finite sample results: Conditional vs. unconditional results
Its importance in the asymptotic results.
Specification Errors-1
Omitting relevant variables: Suppose the correct model is y = X11 + X22 + . I.e., two sets of variables. Compute least squares omitting X2. Some easily proved results:
MVLUE
1. Linear estimator =
n i1
vii
2. Unbiased: E[b|X] = β
Comparing positive definite matrices:
Var[c|X] – Var[b|X] is nonnegative definite for any other linear and unbiased estimator. What are the implications?
Other estimators
Biased estimation – a minimum mean squared error estimator. Is there a biased estimator with a smaller ‘dispersion’?
计量经济学英文课件 (3)
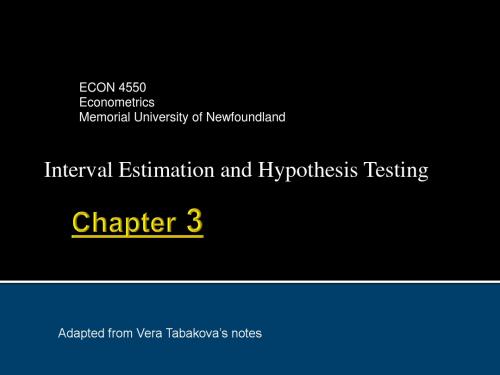
Z
b2 2
2
xi x
2
~ N 0,1
(3.1)
The standardized random variable Z is normally distributed with mean 0 and variance 1.
Slide 3-4
Principles of Econometrics, 3rd Edition
and its standard error
se(b2 ) var(b2 ) 4.38 2.09
Principles of Econometrics, 3rd Edition
Slide 3-12
A “95% confidence interval estimate” for 2:
The critical value tc for degrees of freedom m is the percentile value t1 2,m .
Principles of Econometrics, 3rd Edition
Slide 3-9
Figure 3.1 Critical Values from a t-distribution
Principles of Econometrics, 3rd Edition
Slide 3-16
The Null Hypothesis
parameter.
The null hypothesis, which is denoted H0 (H-naught), specifies a value for a regression The null hypothesis is stated H 0 : k c, where c is a constant, and is an important value in the context of a specific regression model.
Stock 计量经济学ppt一到三章
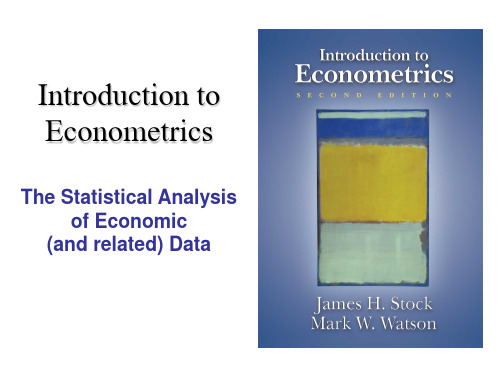
Introduction to Econometrics
The Statistical Analysis of Economic (and related) Data
Brief Overview of the Course
Economics suggests important relationships, often with policy implications, but virtually never suggests quantitative magnitudes of causal effects. What is the quantitative effect of reducing class size on student achievement? How does another year of education change earnings? What is the price elasticity of cigarettes? What is the effect on output growth of a 1 percentage point increase in interest rates by the Fed? What is the effect on housing prices of environmental improvements?
9
Initial data analysis: Compare districts with “small” (STR < 20) and “large” (STR ≥ 20) class sizes:
Class Size Average score (Y ) Small 657.4 Large 650.0 Standard deviation (sBYB) 19.4 17.9 n 238 182
The Statistical Analysis of Economic (and related) Data
Brief Overview of the Course
Economics suggests important relationships, often with policy implications, but virtually never suggests quantitative magnitudes of causal effects. What is the quantitative effect of reducing class size on student achievement? How does another year of education change earnings? What is the price elasticity of cigarettes? What is the effect on output growth of a 1 percentage point increase in interest rates by the Fed? What is the effect on housing prices of environmental improvements?
9
Initial data analysis: Compare districts with “small” (STR < 20) and “large” (STR ≥ 20) class sizes:
Class Size Average score (Y ) Small 657.4 Large 650.0 Standard deviation (sBYB) 19.4 17.9 n 238 182
计量经济学英文课件 (1)

– The owner of a local Pizza Hut must decide how much advertising space to purchase in the local newspaper, and thus must estimate the relationship between advertising and sales
1.2 What is Econometrics About
1.2.1 Some Examples
•
Every day, decision-makers face ‘‘how much’’ questions
: (Continued)
– A real estate developer must predict by how much population and income will increase to the south of Baton Rouge, Louisiana, over the next few years, and whether it will be profitable to begin construction of a gambling casino and golf course
– You must decide how much of your savings will go into a stock fund, and how much into the money market. This requires you to make predictions of the level of economic activity, the rate of inflation, and interest rates over your planning horizon
1.2 What is Econometrics About
1.2.1 Some Examples
•
Every day, decision-makers face ‘‘how much’’ questions
: (Continued)
– A real estate developer must predict by how much population and income will increase to the south of Baton Rouge, Louisiana, over the next few years, and whether it will be profitable to begin construction of a gambling casino and golf course
– You must decide how much of your savings will go into a stock fund, and how much into the money market. This requires you to make predictions of the level of economic activity, the rate of inflation, and interest rates over your planning horizon
- 1、下载文档前请自行甄别文档内容的完整性,平台不提供额外的编辑、内容补充、找答案等附加服务。
- 2、"仅部分预览"的文档,不可在线预览部分如存在完整性等问题,可反馈申请退款(可完整预览的文档不适用该条件!)。
- 3、如文档侵犯您的权益,请联系客服反馈,我们会尽快为您处理(人工客服工作时间:9:00-18:30)。
(4.1a) (4.1b)
where y = Syi / n and. x = Sxi / n 6
Substitute in yi = b1 + b2xi + ei
4.7
to get:
b2 =
b2
+
nSxiei - Sxi Sei nSxi2-(Sxi) 2
The mean of b2 is:
Eb2 =
the variance of the estimator b2 is:
var(b2) =
s2
S(xi - x)2
b2 is a function of the yi values but
they are called estimators of b1 and b2
which are random variables because they are different fr.om sample to 4
4.5
Estimators are Random Variables ( estimates are not )
• If the least squares estimators b0 and b1 are random variables, then what are their means, variances, covariances and probability distributions?
• Compare the properties of alternative estimators to the prop. erties of the 5
Since the distribution of b2 is centered at b2 ,we say that b2 is an unbiased e. stimator of b2. 8
Wrong Model Specification 4.9
The unbiasedness result on the previous slide assumes that we are using the correct model.
4.6
The Expected Values of b1 and b2
The least squares formulas (estimators) in the simple regression nSxi22 -(Sxi) 2
b1 = y - b2x
If the model is of the wrong form
or is missing important variables,
then Eei = 0, then Eb2 = b2 .
.
9
4.10
Unbiased Estimator of the Intercept
In a similar manner, the estimator b1 of the intercept or constant term can be
b2
+
nSxiEei - Sxi SEei nSxi2-(Sxi) 2
Since Eei = 0, then Eb2 = b2 .
.
7
An Unbiased Estimator
4.8
The result Eb2 = b2 means that the distribution of b2 is centered at b2.
Chapter 4 Statistical Properties of the OLS
Estimators
Xi’An Institute of Post & Telecommunication Dept of Economic & Management Prof. Long
.
1
Simple Linear Regression Model 4.2
shown to be an unbiased estimator of b1
when the model is correctly specified.
Eb1 = b1
.
10
4.11
Equivalent expressions for b2:
b2
=
S(xi - x )(yi S(xi - x )2
yt = b1 + b2 xt + et
yt = household weekly food expenditures xt = household weekly income
For a given level of xt, the expected
level of food expenditures will be:
The formulas that produce the
sample estimates b1 and called the estimators of
b2 are
b1 and
b2.
When b0 and b1 are used to represent the formulas rather than specific values,
y
)
(4.3a)
Expand and multiply top and bottom by n:
b2 =
nSxiyi - Sxi Syi nSxi2-(S.xi) 2
(4.3b)
11
Variance of b2
4.12
Given that both yi and ei have variance s2,
E(yt|xt) =
b1
+
.
b2
xt
2
Assumptions of the Simple 4.3 Linear Regression Model
1. yt = b1 + b2xt + et 2. E(et) = 0 <=> E(yt) = b1 +
b2x t
3. var(et) = s 2 = var(yt)
4. cov(ei,ej) = cov(yi,yj) = 0 5. xt c for every observation
6. et~N(0,s 2) <=.> yt~N(b1+ b2x3 t,
The population parameters b1 and b2 4.4 are unknown population constants.