推荐系统综述recommder
《2024年推荐系统综述》范文

《推荐系统综述》篇一一、引言随着互联网技术的飞速发展,信息过载问题日益严重,用户面临着从海量数据中筛选出有价值信息的挑战。
推荐系统作为一种解决信息过载问题的有效手段,已经广泛应用于电商、社交网络、视频网站等各个领域。
本文旨在全面综述推荐系统的研究现状、关键技术、应用领域及未来发展趋势。
二、推荐系统的研究现状推荐系统是一种利用用户的历史行为、兴趣偏好、社交关系等信息,为用户推荐可能感兴趣的内容或服务的系统。
自20世纪90年代以来,推荐系统研究取得了长足的进步。
目前,国内外学者在推荐系统的理论、算法、应用等方面进行了广泛的研究,形成了丰富的成果。
三、推荐系统的关键技术1. 协同过滤技术协同过滤是推荐系统中应用最广泛的技术之一。
它通过分析用户的历史行为、兴趣偏好等数据,找出与目标用户相似的其他用户,然后根据这些相似用户的喜好为目标用户推荐内容。
协同过滤技术包括基于用户的协同过滤和基于项目的协同过滤两种方法。
2. 内容推荐技术内容推荐技术主要依据物品的内容特征进行推荐。
它通过分析物品的文本、图片、视频等多媒体信息,提取物品的特征,然后根据用户的兴趣偏好为用户推荐与之相似的物品。
内容推荐技术的代表算法有基于文本的向量空间模型、基于深度学习的内容推荐等。
3. 混合推荐技术混合推荐技术是将协同过滤技术和内容推荐技术相结合,充分利用两者的优点进行推荐。
混合推荐技术可以提高推荐的准确性和多样性,更好地满足用户的个性化需求。
四、推荐系统的应用领域推荐系统已经广泛应用于各个领域,如电商、社交网络、视频网站、音乐平台等。
在电商领域,推荐系统可以帮助用户快速找到感兴趣的商品;在社交网络中,推荐系统可以帮助用户发现可能感兴趣的人或群组;在视频网站和音乐平台中,推荐系统可以根据用户的喜好推荐相应的视频或音乐。
此外,推荐系统还可以应用于新闻推送、广告投放等领域。
五、未来发展趋势随着人工智能、大数据等技术的发展,推荐系统将迎来新的发展机遇。
推荐系统研究综述
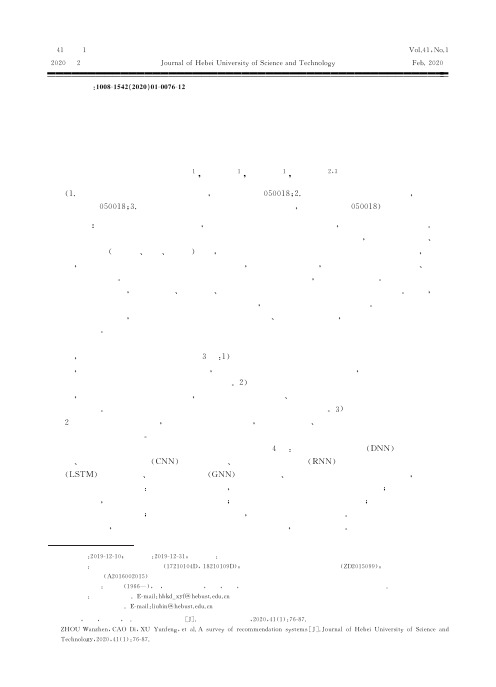
文中主要对传统的推荐方法 和 当 前 深 度 学 习 技 术 中 神 经 网 络 在 推 荐 方 法 上 的 应 用 进 行 了 归
纳,其中传统推荐方法主要分为以下 3 类:
1)基于内容推荐方法主要依据用户与项目之间的特征信
息,用户之间的联系不会影响推荐结果,所以不存在冷启动和 稀 疏 矩 阵 的 问 题,但 是 基 于 内 容 推 荐
l
ogy,
2020,
41(
1):
76
87.
第1期
77
周万珍,等:推荐系统研究综述
关键词:计算机神经网络;推荐系统;数据挖掘;深度学习;信息过载
中图分类号:
TP311.
13
文献标识码:
A
do
i:
10.
7535/hbkd.
2020yx01009
Asur
veyo
fr
e
commenda
t
i
onsys
t
ems
i
z
e
st
heapp
l
i
c
a
t
i
ono
ft
r
ad
i
t
i
ona
lr
e
c
ommenda
t
i
onme
t
hodsandt
heapp
l
i
c
a
t
i
ono
fneu
r
a
lne
two
r
k
ysumma
i
ncu
r
r
en
tde
epl
e
a
推荐系统研究综述
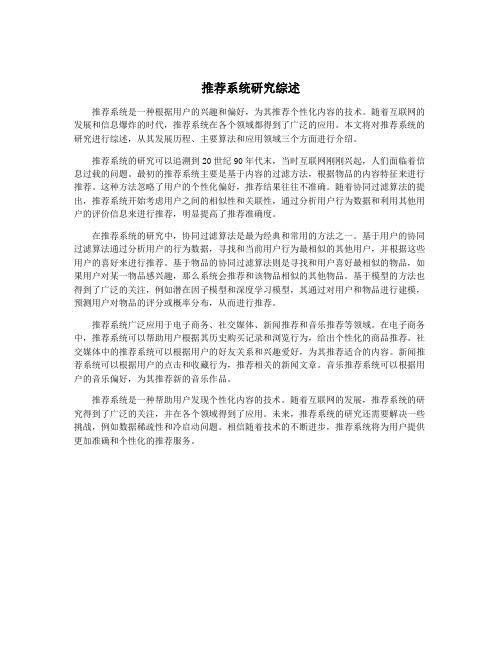
推荐系统研究综述推荐系统是一种根据用户的兴趣和偏好,为其推荐个性化内容的技术。
随着互联网的发展和信息爆炸的时代,推荐系统在各个领域都得到了广泛的应用。
本文将对推荐系统的研究进行综述,从其发展历程、主要算法和应用领域三个方面进行介绍。
推荐系统的研究可以追溯到20世纪90年代末,当时互联网刚刚兴起,人们面临着信息过载的问题。
最初的推荐系统主要是基于内容的过滤方法,根据物品的内容特征来进行推荐。
这种方法忽略了用户的个性化偏好,推荐结果往往不准确。
随着协同过滤算法的提出,推荐系统开始考虑用户之间的相似性和关联性,通过分析用户行为数据和利用其他用户的评价信息来进行推荐,明显提高了推荐准确度。
在推荐系统的研究中,协同过滤算法是最为经典和常用的方法之一。
基于用户的协同过滤算法通过分析用户的行为数据,寻找和当前用户行为最相似的其他用户,并根据这些用户的喜好来进行推荐。
基于物品的协同过滤算法则是寻找和用户喜好最相似的物品,如果用户对某一物品感兴趣,那么系统会推荐和该物品相似的其他物品。
基于模型的方法也得到了广泛的关注,例如潜在因子模型和深度学习模型,其通过对用户和物品进行建模,预测用户对物品的评分或概率分布,从而进行推荐。
推荐系统广泛应用于电子商务、社交媒体、新闻推荐和音乐推荐等领域。
在电子商务中,推荐系统可以帮助用户根据其历史购买记录和浏览行为,给出个性化的商品推荐。
社交媒体中的推荐系统可以根据用户的好友关系和兴趣爱好,为其推荐适合的内容。
新闻推荐系统可以根据用户的点击和收藏行为,推荐相关的新闻文章。
音乐推荐系统可以根据用户的音乐偏好,为其推荐新的音乐作品。
推荐系统是一种帮助用户发现个性化内容的技术。
随着互联网的发展,推荐系统的研究得到了广泛的关注,并在各个领域得到了应用。
未来,推荐系统的研究还需要解决一些挑战,例如数据稀疏性和冷启动问题。
相信随着技术的不断进步,推荐系统将为用户提供更加准确和个性化的推荐服务。
推荐系统综述

推荐系统综述推荐系统综述引言:随着互联网和电子商务的快速发展,推荐系统成为了各个行业中的重要组成部分。
推荐系统通过分析用户的行为和兴趣,为用户提供个性化的推荐,从而提高用户体验和满意度。
本文将综述推荐系统的背景、发展历程、应用领域、算法原理等方面,为读者全面介绍推荐系统的相关知识。
一、背景:随着信息爆炸和信息过载的时代到来,人们面临了获取信息的困境。
传统的信息检索方式往往无法满足用户的个性化需求。
而推荐系统通过对用户行为和兴趣的分析,可以为用户提供个性化的推荐,从而解决了这一问题。
二、推荐系统的发展历程:推荐系统的发展经历了几个重要的阶段。
起初,推荐系统主要采用基于内容的推荐算法,即通过分析物品的内容特征来做出推荐。
然后,协同过滤成为了主流的推荐算法,它通过分析用户的历史行为和其他用户的行为来生成推荐结果。
最近几年,深度学习等技术的兴起使得推荐系统可以更好地提取和利用用户的行为特征,从而进一步提高了推荐的准确度和个性化程度。
三、推荐系统的应用领域:推荐系统广泛应用于各个行业领域。
在电子商务领域,推荐系统可以根据用户的购买历史和浏览行为,为用户推荐相关的商品,提高销售额和用户忠诚度。
在社交媒体领域,推荐系统可以根据用户的兴趣和好友关系,为用户推荐感兴趣的内容和用户。
在音乐和视频领域,推荐系统可以根据用户的偏好和历史播放记录,为用户推荐相关的音乐和视频。
四、推荐系统的算法原理:推荐系统的核心是推荐算法。
推荐算法包括基于内容的推荐算法、协同过滤算法和深度学习算法等。
基于内容的推荐算法通过分析物品的内容特征,为用户推荐与其兴趣相似的物品。
协同过滤算法通过分析用户的历史行为和与其兴趣相似的其他用户的行为,为用户生成推荐结果。
深度学习算法通过建立多层神经网络,提取和利用用户的行为特征,进一步提高了推荐的准确度和个性化程度。
五、推荐系统的挑战与展望:虽然推荐系统取得了很大的进展,但仍然面临一些挑战。
首先,数据稀疏性和冷启动问题限制了推荐系统的效果和覆盖范围。
推荐系统综述
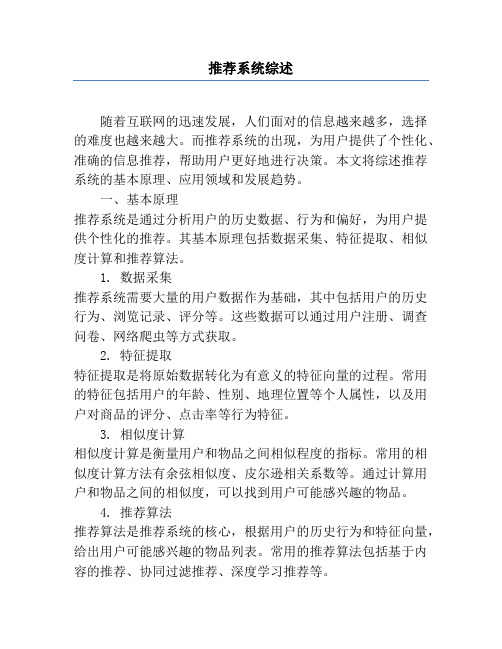
推荐系统综述随着互联网的迅速发展,人们面对的信息越来越多,选择的难度也越来越大。
而推荐系统的出现,为用户提供了个性化、准确的信息推荐,帮助用户更好地进行决策。
本文将综述推荐系统的基本原理、应用领域和发展趋势。
一、基本原理推荐系统是通过分析用户的历史数据、行为和偏好,为用户提供个性化的推荐。
其基本原理包括数据采集、特征提取、相似度计算和推荐算法。
1. 数据采集推荐系统需要大量的用户数据作为基础,其中包括用户的历史行为、浏览记录、评分等。
这些数据可以通过用户注册、调查问卷、网络爬虫等方式获取。
2. 特征提取特征提取是将原始数据转化为有意义的特征向量的过程。
常用的特征包括用户的年龄、性别、地理位置等个人属性,以及用户对商品的评分、点击率等行为特征。
3. 相似度计算相似度计算是衡量用户和物品之间相似程度的指标。
常用的相似度计算方法有余弦相似度、皮尔逊相关系数等。
通过计算用户和物品之间的相似度,可以找到用户可能感兴趣的物品。
4. 推荐算法推荐算法是推荐系统的核心,根据用户的历史行为和特征向量,给出用户可能感兴趣的物品列表。
常用的推荐算法包括基于内容的推荐、协同过滤推荐、深度学习推荐等。
二、应用领域推荐系统已广泛应用于电子商务、社交网络、个性化新闻推荐等领域。
1. 电子商务电子商务是推荐系统最早应用的领域之一。
通过分析用户的购买记录、浏览历史等信息,推荐系统可以为用户提供个性化的商品推荐,增加用户购买的可能性。
2. 社交网络社交网络中存在大量用户生成的内容,推荐系统可以通过分析用户的社交关系、兴趣爱好等信息,为用户推荐感兴趣的文章、照片、视频等。
3. 个性化新闻推荐随着新闻来源和内容的爆炸式增长,用户往往面临信息过载的问题。
推荐系统可以根据用户的阅读历史、偏好等,过滤和推荐用户可能感兴趣的新闻内容,提高用户的阅读体验。
三、发展趋势随着互联网和人工智能的发展,推荐系统正呈现出以下几个发展趋势。
1. 深度学习在推荐系统中的应用深度学习技术具有强大的模式识别和特征提取能力,可以更精确地挖掘用户的兴趣和推荐物品。
推荐系统的发展综述

推荐系统的发展综述推荐系统发展至今,其背后的技术大致可以划分为三类:基于内容的模型,基于协同过滤的模型,以及混合模型。
基于内容的推荐模型主要在于分别建立用户和物品的档案资料,从而计算用户和物品之间的相似度。
物品的档案通常由它的各种属性资料构成,以服装领域为例,可以为价格、品牌、类别、颜色、风格、款式、尺寸等等。
用户的档案可以包括她们的人口统计学资料,也可以是从她们的历史交互过的物品档案中构建,例如,用户经常购买杰克琼斯的服装,说明她比较喜欢这个服装品牌。
建立了用户和物品的档案之后,可以直接计算相似度,也可以把它们当做特征放入机器学习的模型。
基于内容的推荐模型的优点是,只要得到了物品或者用户的档案,就可以处理冷启动的问题:其次,因为档案都是显式的特征,最终的模型有比较好的可解释性。
基于协同过滤的推荐模型是目前学术界研究得最广泛的模型。
它不需要使用用户或者物品的档案资料,只需要收集用户历史的行为记录,发掘其中用户和用户、物品与物品之间潜在的相似性,并基于这种群组的相似性完成推荐。
协同过滤模型可以分为两类:基于邻居的方法和基于模型的方法。
基于邻居的方法的核心在于根据历史的行为记录,构建user一user,或者item一item的相似度矩阵。
常用的相似度计算方法有余弦相似度、皮尔逊相似度等。
基于樸型的推荐中最常用的方法是隐因子樸型。
是这一技术发展的重要里程碑。
在这类模型中,用户和物品都被嵌入到一个低维的向量表示,用户和物品的相关性则体现在它们对应的隐向量的点积关系。
这种方法的优点在于效率高,一旦训练出了模型,用户和物品的关系就能很方便地通过点积计算出来;同时准确度也相比于邻居模型要好。
其缺点也很明显,不能解决冷启动的问题,同时学习出的隐向量不方便解释。
混合模型则是指将多种推荐模型相结合,取长补短,从而得到更好的推荐效果。
工业界常用的模型往往是混合的模型。
这类模型可以是通过各种集成学习的方式组合而来,也可以是一种端到端的樸型,Wide&Deep 等樸型。
推荐系统研究综述
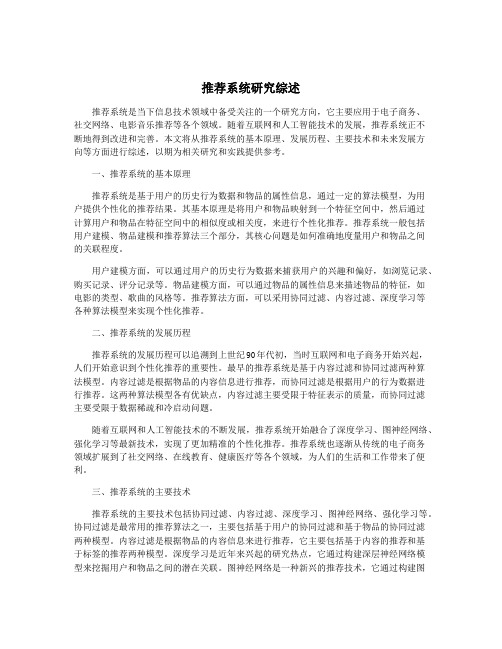
推荐系统研究综述推荐系统是当下信息技术领域中备受关注的一个研究方向,它主要应用于电子商务、社交网络、电影音乐推荐等各个领域。
随着互联网和人工智能技术的发展,推荐系统正不断地得到改进和完善。
本文将从推荐系统的基本原理、发展历程、主要技术和未来发展方向等方面进行综述,以期为相关研究和实践提供参考。
一、推荐系统的基本原理推荐系统是基于用户的历史行为数据和物品的属性信息,通过一定的算法模型,为用户提供个性化的推荐结果。
其基本原理是将用户和物品映射到一个特征空间中,然后通过计算用户和物品在特征空间中的相似度或相关度,来进行个性化推荐。
推荐系统一般包括用户建模、物品建模和推荐算法三个部分,其核心问题是如何准确地度量用户和物品之间的关联程度。
用户建模方面,可以通过用户的历史行为数据来捕获用户的兴趣和偏好,如浏览记录、购买记录、评分记录等。
物品建模方面,可以通过物品的属性信息来描述物品的特征,如电影的类型、歌曲的风格等。
推荐算法方面,可以采用协同过滤、内容过滤、深度学习等各种算法模型来实现个性化推荐。
二、推荐系统的发展历程推荐系统的发展历程可以追溯到上世纪90年代初,当时互联网和电子商务开始兴起,人们开始意识到个性化推荐的重要性。
最早的推荐系统是基于内容过滤和协同过滤两种算法模型。
内容过滤是根据物品的内容信息进行推荐,而协同过滤是根据用户的行为数据进行推荐。
这两种算法模型各有优缺点,内容过滤主要受限于特征表示的质量,而协同过滤主要受限于数据稀疏和冷启动问题。
随着互联网和人工智能技术的不断发展,推荐系统开始融合了深度学习、图神经网络、强化学习等最新技术,实现了更加精准的个性化推荐。
推荐系统也逐渐从传统的电子商务领域扩展到了社交网络、在线教育、健康医疗等各个领域,为人们的生活和工作带来了便利。
三、推荐系统的主要技术推荐系统的主要技术包括协同过滤、内容过滤、深度学习、图神经网络、强化学习等。
协同过滤是最常用的推荐算法之一,主要包括基于用户的协同过滤和基于物品的协同过滤两种模型。
推荐系统研究综述
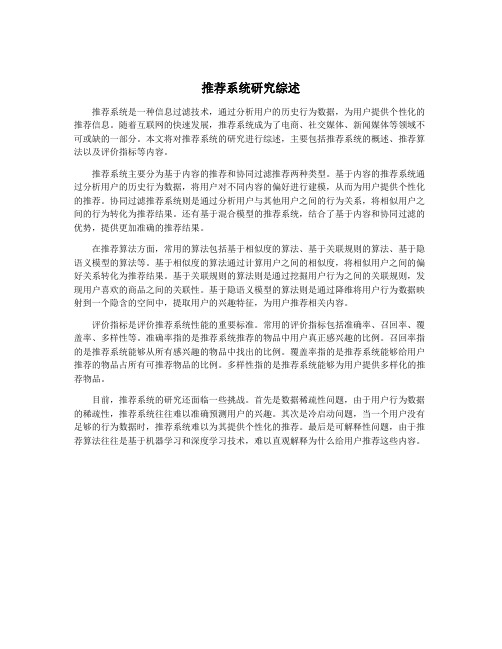
推荐系统研究综述推荐系统是一种信息过滤技术,通过分析用户的历史行为数据,为用户提供个性化的推荐信息。
随着互联网的快速发展,推荐系统成为了电商、社交媒体、新闻媒体等领域不可或缺的一部分。
本文将对推荐系统的研究进行综述,主要包括推荐系统的概述、推荐算法以及评价指标等内容。
推荐系统主要分为基于内容的推荐和协同过滤推荐两种类型。
基于内容的推荐系统通过分析用户的历史行为数据,将用户对不同内容的偏好进行建模,从而为用户提供个性化的推荐。
协同过滤推荐系统则是通过分析用户与其他用户之间的行为关系,将相似用户之间的行为转化为推荐结果。
还有基于混合模型的推荐系统,结合了基于内容和协同过滤的优势,提供更加准确的推荐结果。
在推荐算法方面,常用的算法包括基于相似度的算法、基于关联规则的算法、基于隐语义模型的算法等。
基于相似度的算法通过计算用户之间的相似度,将相似用户之间的偏好关系转化为推荐结果。
基于关联规则的算法则是通过挖掘用户行为之间的关联规则,发现用户喜欢的商品之间的关联性。
基于隐语义模型的算法则是通过降维将用户行为数据映射到一个隐含的空间中,提取用户的兴趣特征,为用户推荐相关内容。
评价指标是评价推荐系统性能的重要标准。
常用的评价指标包括准确率、召回率、覆盖率、多样性等。
准确率指的是推荐系统推荐的物品中用户真正感兴趣的比例。
召回率指的是推荐系统能够从所有感兴趣的物品中找出的比例。
覆盖率指的是推荐系统能够给用户推荐的物品占所有可推荐物品的比例。
多样性指的是推荐系统能够为用户提供多样化的推荐物品。
目前,推荐系统的研究还面临一些挑战。
首先是数据稀疏性问题,由于用户行为数据的稀疏性,推荐系统往往难以准确预测用户的兴趣。
其次是冷启动问题,当一个用户没有足够的行为数据时,推荐系统难以为其提供个性化的推荐。
最后是可解释性问题,由于推荐算法往往是基于机器学习和深度学习技术,难以直观解释为什么给用户推荐这些内容。
推荐系统研究综述
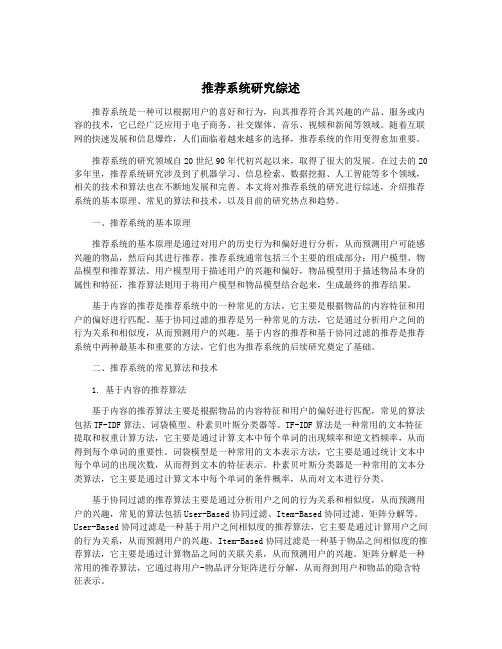
推荐系统研究综述推荐系统是一种可以根据用户的喜好和行为,向其推荐符合其兴趣的产品、服务或内容的技术,它已经广泛应用于电子商务、社交媒体、音乐、视频和新闻等领域。
随着互联网的快速发展和信息爆炸,人们面临着越来越多的选择,推荐系统的作用变得愈加重要。
推荐系统的研究领域自20世纪90年代初兴起以来,取得了很大的发展。
在过去的20多年里,推荐系统研究涉及到了机器学习、信息检索、数据挖掘、人工智能等多个领域,相关的技术和算法也在不断地发展和完善。
本文将对推荐系统的研究进行综述,介绍推荐系统的基本原理、常见的算法和技术,以及目前的研究热点和趋势。
一、推荐系统的基本原理推荐系统的基本原理是通过对用户的历史行为和偏好进行分析,从而预测用户可能感兴趣的物品,然后向其进行推荐。
推荐系统通常包括三个主要的组成部分:用户模型、物品模型和推荐算法。
用户模型用于描述用户的兴趣和偏好,物品模型用于描述物品本身的属性和特征,推荐算法则用于将用户模型和物品模型结合起来,生成最终的推荐结果。
基于内容的推荐是推荐系统中的一种常见的方法,它主要是根据物品的内容特征和用户的偏好进行匹配。
基于协同过滤的推荐是另一种常见的方法,它是通过分析用户之间的行为关系和相似度,从而预测用户的兴趣。
基于内容的推荐和基于协同过滤的推荐是推荐系统中两种最基本和重要的方法,它们也为推荐系统的后续研究奠定了基础。
二、推荐系统的常见算法和技术1. 基于内容的推荐算法基于内容的推荐算法主要是根据物品的内容特征和用户的偏好进行匹配,常见的算法包括TF-IDF算法、词袋模型、朴素贝叶斯分类器等。
TF-IDF算法是一种常用的文本特征提取和权重计算方法,它主要是通过计算文本中每个单词的出现频率和逆文档频率,从而得到每个单词的重要性。
词袋模型是一种常用的文本表示方法,它主要是通过统计文本中每个单词的出现次数,从而得到文本的特征表示。
朴素贝叶斯分类器是一种常用的文本分类算法,它主要是通过计算文本中每个单词的条件概率,从而对文本进行分类。
《2024年推荐系统综述》范文
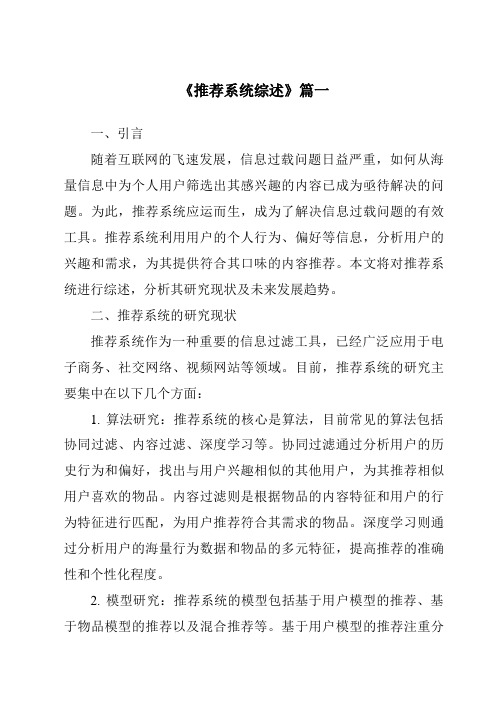
《推荐系统综述》篇一一、引言随着互联网的飞速发展,信息过载问题日益严重,如何从海量信息中为个人用户筛选出其感兴趣的内容已成为亟待解决的问题。
为此,推荐系统应运而生,成为了解决信息过载问题的有效工具。
推荐系统利用用户的个人行为、偏好等信息,分析用户的兴趣和需求,为其提供符合其口味的内容推荐。
本文将对推荐系统进行综述,分析其研究现状及未来发展趋势。
二、推荐系统的研究现状推荐系统作为一种重要的信息过滤工具,已经广泛应用于电子商务、社交网络、视频网站等领域。
目前,推荐系统的研究主要集中在以下几个方面:1. 算法研究:推荐系统的核心是算法,目前常见的算法包括协同过滤、内容过滤、深度学习等。
协同过滤通过分析用户的历史行为和偏好,找出与用户兴趣相似的其他用户,为其推荐相似用户喜欢的物品。
内容过滤则是根据物品的内容特征和用户的行为特征进行匹配,为用户推荐符合其需求的物品。
深度学习则通过分析用户的海量行为数据和物品的多元特征,提高推荐的准确性和个性化程度。
2. 模型研究:推荐系统的模型包括基于用户模型的推荐、基于物品模型的推荐以及混合推荐等。
基于用户模型的推荐注重分析用户的历史行为和偏好,从而为用户提供个性化的推荐;基于物品模型的推荐则更注重物品的内容特征和与其他物品的关联性;混合推荐则综合了这两种模型的特点,以提高推荐的准确性和个性化程度。
3. 用户行为分析:为了更好地为用户提供符合其需求和偏好的推荐,研究者在不断探索用户的行为模式和兴趣偏好。
通过对用户的历史行为数据进行分析,了解用户的兴趣、需求、喜好等信息,为推荐系统提供更加精准的推荐依据。
三、推荐系统的技术挑战尽管推荐系统在许多领域取得了显著的成果,但仍面临一些技术挑战:1. 数据稀疏性:对于新用户或新物品,由于缺乏历史数据,推荐系统的准确性会受到影响。
如何解决数据稀疏性问题,提高新用户和新物品的推荐准确性是当前研究的重点。
2. 冷启动问题:对于新加入的物品或服务,由于缺乏用户反馈和行为数据,难以进行有效的推荐。
推荐系统研究综述
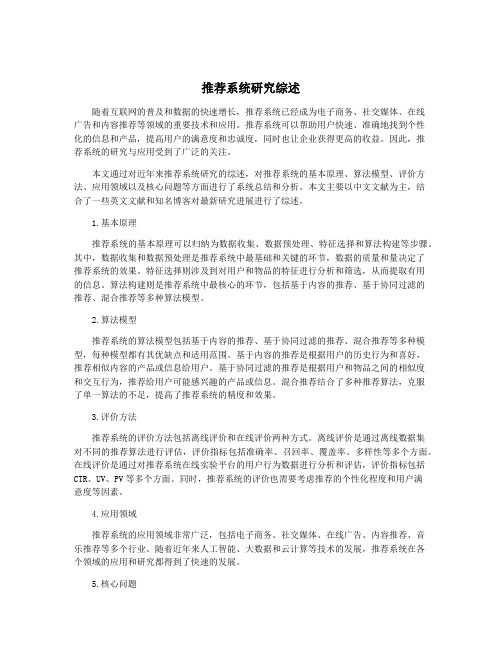
推荐系统研究综述随着互联网的普及和数据的快速增长,推荐系统已经成为电子商务、社交媒体、在线广告和内容推荐等领域的重要技术和应用。
推荐系统可以帮助用户快速、准确地找到个性化的信息和产品,提高用户的满意度和忠诚度,同时也让企业获得更高的收益。
因此,推荐系统的研究与应用受到了广泛的关注。
本文通过对近年来推荐系统研究的综述,对推荐系统的基本原理、算法模型、评价方法、应用领域以及核心问题等方面进行了系统总结和分析。
本文主要以中文文献为主,结合了一些英文文献和知名博客对最新研究进展进行了综述。
1.基本原理推荐系统的基本原理可以归纳为数据收集、数据预处理、特征选择和算法构建等步骤。
其中,数据收集和数据预处理是推荐系统中最基础和关键的环节,数据的质量和量决定了推荐系统的效果。
特征选择则涉及到对用户和物品的特征进行分析和筛选,从而提取有用的信息。
算法构建则是推荐系统中最核心的环节,包括基于内容的推荐、基于协同过滤的推荐、混合推荐等多种算法模型。
2.算法模型推荐系统的算法模型包括基于内容的推荐、基于协同过滤的推荐、混合推荐等多种模型,每种模型都有其优缺点和适用范围。
基于内容的推荐是根据用户的历史行为和喜好,推荐相似内容的产品或信息给用户。
基于协同过滤的推荐是根据用户和物品之间的相似度和交互行为,推荐给用户可能感兴趣的产品或信息。
混合推荐结合了多种推荐算法,克服了单一算法的不足,提高了推荐系统的精度和效果。
3.评价方法推荐系统的评价方法包括离线评价和在线评价两种方式。
离线评价是通过离线数据集对不同的推荐算法进行评估,评价指标包括准确率、召回率、覆盖率、多样性等多个方面。
在线评价是通过对推荐系统在线实验平台的用户行为数据进行分析和评估,评价指标包括CTR、UV、PV等多个方面。
同时,推荐系统的评价也需要考虑推荐的个性化程度和用户满意度等因素。
4.应用领域推荐系统的应用领域非常广泛,包括电子商务、社交媒体、在线广告、内容推荐、音乐推荐等多个行业。
《2024年推荐系统综述》范文

《推荐系统综述》篇一一、引言随着互联网技术的迅猛发展,信息过载问题日益突出,使得用户难以从海量数据中筛选出自己感兴趣的信息。
为了解决这一问题,推荐系统应运而生。
推荐系统通过分析用户的行为数据、兴趣偏好等信息,为用户提供个性化的信息推荐服务,有效提高了用户的信息获取效率和满意度。
本文将对推荐系统进行综述,包括其基本原理、主要方法、应用领域及未来发展趋势。
二、推荐系统基本原理推荐系统主要基于以下原理:利用用户的行为数据、兴趣偏好等信息,分析用户的兴趣特点,然后根据这些特点为用户推荐其可能感兴趣的内容。
推荐系统通常包括以下几个核心模块:数据收集模块、用户建模模块、推荐算法模块和结果评估模块。
1. 数据收集模块:收集用户的行为数据、兴趣偏好等信息,为后续的推荐提供数据支持。
2. 用户建模模块:根据收集到的数据,分析用户的兴趣特点,建立用户模型。
3. 推荐算法模块:根据用户模型和推荐算法,为用户推荐其可能感兴趣的内容。
4. 结果评估模块:对推荐结果进行评估,不断优化推荐算法和模型。
三、推荐系统主要方法推荐系统的主要方法包括协同过滤、内容过滤、混合推荐等。
1. 协同过滤:协同过滤是推荐系统中应用最广泛的方法之一。
它通过分析用户的行为数据,找出与目标用户兴趣相似的其他用户,然后根据这些相似用户的行为数据为目标用户推荐内容。
协同过滤又可分为基于用户的协同过滤和基于项目的协同过滤。
2. 内容过滤:内容过滤主要是通过分析内容的特征和用户的兴趣偏好,为用户推荐与其兴趣相符的内容。
它主要包括基于关键词的内容过滤和基于机器学习的内容过滤等方法。
3. 混合推荐:混合推荐是结合协同过滤和内容过滤的优点,根据具体需求和场景,将多种推荐方法进行融合,以提高推荐的准确性和满意度。
四、推荐系统应用领域推荐系统在各个领域都有广泛的应用,如电商、音乐、视频、社交网络等。
1. 电商领域:推荐系统可以根据用户的购买记录、浏览记录等信息,为用户推荐其可能感兴趣的商品,提高购买转化率和用户满意度。
推荐系统调研报告及综述
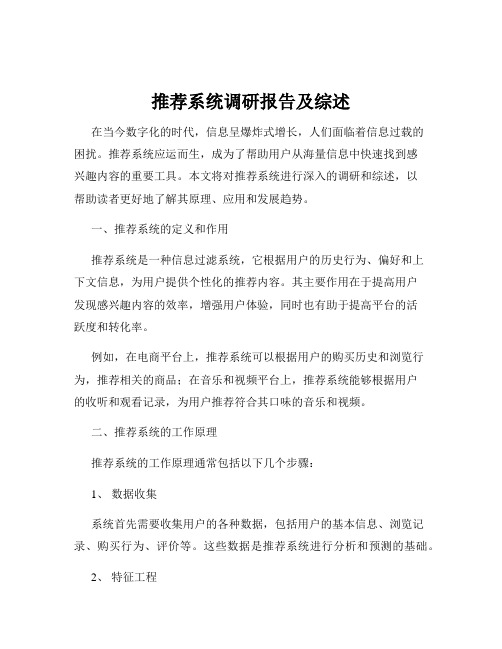
推荐系统调研报告及综述在当今数字化的时代,信息呈爆炸式增长,人们面临着信息过载的困扰。
推荐系统应运而生,成为了帮助用户从海量信息中快速找到感兴趣内容的重要工具。
本文将对推荐系统进行深入的调研和综述,以帮助读者更好地了解其原理、应用和发展趋势。
一、推荐系统的定义和作用推荐系统是一种信息过滤系统,它根据用户的历史行为、偏好和上下文信息,为用户提供个性化的推荐内容。
其主要作用在于提高用户发现感兴趣内容的效率,增强用户体验,同时也有助于提高平台的活跃度和转化率。
例如,在电商平台上,推荐系统可以根据用户的购买历史和浏览行为,推荐相关的商品;在音乐和视频平台上,推荐系统能够根据用户的收听和观看记录,为用户推荐符合其口味的音乐和视频。
二、推荐系统的工作原理推荐系统的工作原理通常包括以下几个步骤:1、数据收集系统首先需要收集用户的各种数据,包括用户的基本信息、浏览记录、购买行为、评价等。
这些数据是推荐系统进行分析和预测的基础。
2、特征工程对收集到的数据进行处理和提取特征。
例如,将商品的类别、价格、品牌等作为特征,将用户的年龄、性别、地域等作为用户特征。
3、模型训练使用机器学习或深度学习算法,基于提取的特征训练推荐模型。
常见的算法包括协同过滤、基于内容的推荐、基于矩阵分解的推荐等。
4、推荐生成根据训练好的模型,为用户生成推荐列表。
推荐列表的生成可以基于用户的相似性、商品的相似性或者两者的结合。
三、推荐系统的常用算法1、协同过滤协同过滤是推荐系统中最经典和常用的算法之一。
它分为基于用户的协同过滤和基于物品的协同过滤。
基于用户的协同过滤是找到与目标用户相似的其他用户,然后将这些相似用户喜欢的物品推荐给目标用户;基于物品的协同过滤则是根据用户过去喜欢的物品,推荐与之相似的其他物品。
2、基于内容的推荐基于内容的推荐是根据物品的内容特征(如文本描述、标签等)和用户的偏好特征来进行推荐。
它需要对物品和用户的特征进行准确的建模和匹配。
推荐系统概述范文
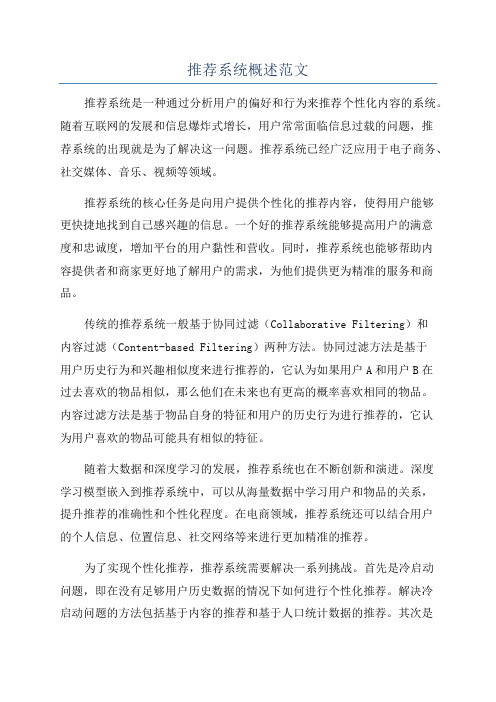
推荐系统概述范文推荐系统是一种通过分析用户的偏好和行为来推荐个性化内容的系统。
随着互联网的发展和信息爆炸式增长,用户常常面临信息过载的问题,推荐系统的出现就是为了解决这一问题。
推荐系统已经广泛应用于电子商务、社交媒体、音乐、视频等领域。
推荐系统的核心任务是向用户提供个性化的推荐内容,使得用户能够更快捷地找到自己感兴趣的信息。
一个好的推荐系统能够提高用户的满意度和忠诚度,增加平台的用户黏性和营收。
同时,推荐系统也能够帮助内容提供者和商家更好地了解用户的需求,为他们提供更为精准的服务和商品。
传统的推荐系统一般基于协同过滤(Collaborative Filtering)和内容过滤(Content-based Filtering)两种方法。
协同过滤方法是基于用户历史行为和兴趣相似度来进行推荐的,它认为如果用户A和用户B在过去喜欢的物品相似,那么他们在未来也有更高的概率喜欢相同的物品。
内容过滤方法是基于物品自身的特征和用户的历史行为进行推荐的,它认为用户喜欢的物品可能具有相似的特征。
随着大数据和深度学习的发展,推荐系统也在不断创新和演进。
深度学习模型嵌入到推荐系统中,可以从海量数据中学习用户和物品的关系,提升推荐的准确性和个性化程度。
在电商领域,推荐系统还可以结合用户的个人信息、位置信息、社交网络等来进行更加精准的推荐。
为了实现个性化推荐,推荐系统需要解决一系列挑战。
首先是冷启动问题,即在没有足够用户历史数据的情况下如何进行个性化推荐。
解决冷启动问题的方法包括基于内容的推荐和基于人口统计数据的推荐。
其次是数据稀疏性问题,即用户历史数据通常是稀疏的,这就导致了推荐系统在预测用户兴趣时的不确定性。
解决数据稀疏性问题的方法包括协同过滤算法的改进和引入辅助信息。
还有一个重要的问题是推荐结果的可解释性,即用户为什么会对一些物品感兴趣,为什么会被推荐给他们。
解决可解释性问题的方法包括利用用户和物品的特征向量进行解释和设计可解释的推荐模型。
《2024年推荐系统综述》范文

《推荐系统综述》篇一一、引言随着互联网的飞速发展,信息过载问题日益严重,如何从海量信息中为用户筛选出符合其兴趣和需求的内容,成为了互联网行业的重要问题。
推荐系统作为解决这一问题的有效手段,得到了广泛的应用和关注。
本文将对推荐系统的研究进行综述,分析其发展历程、基本原理、主要方法以及应用领域,旨在为后续研究者提供一定的参考。
二、推荐系统的发展历程推荐系统的发展历程大致可划分为三个阶段:基于内容的推荐系统、协同过滤推荐系统和混合推荐系统。
1. 基于内容的推荐系统:早期的推荐系统主要基于内容,通过分析用户的历史行为和偏好,以及物品的属性特征,为用户推荐与其历史兴趣相似的物品。
这种方法具有解释性强、冷启动问题相对较少的优点,但需要大量的人工干预来维护物品的属性信息。
2. 协同过滤推荐系统:随着大数据和机器学习技术的发展,协同过滤成为了推荐系统的主流方法。
协同过滤通过分析用户的行为数据,找出与目标用户兴趣相似的其他用户,然后根据这些相似用户的喜好为目标用户推荐物品。
这种方法无需手动维护物品的属性信息,具有自适应性强的优点,但面临着数据稀疏性和冷启动等问题。
3. 混合推荐系统:为了弥补基于内容和协同过滤推荐系统的不足,研究者们提出了混合推荐系统。
混合推荐系统结合了基于内容和协同过滤的优点,通过将两种方法进行融合,以提高推荐的准确性和用户满意度。
三、推荐系统的主要方法1. 协同过滤:协同过滤是推荐系统的核心方法之一,主要包括基于用户的协同过滤和基于物品的协同过滤。
前者通过寻找与目标用户兴趣相似的其他用户来推荐物品,后者则通过分析物品之间的相似性来为用户推荐物品。
2. 基于内容的推荐:该方法主要通过分析物品的属性信息和用户的历史行为数据来为用户推荐相似的物品。
常用的技术包括文本挖掘、图像识别等。
3. 混合推荐:混合推荐结合了协同过滤和基于内容的推荐的优点,通过将两种方法进行融合,以提高推荐的准确性和用户满意度。
推荐系统综述
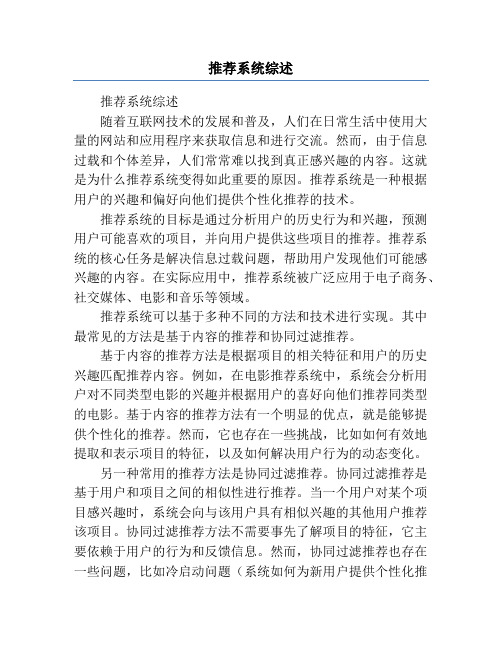
推荐系统综述推荐系统综述随着互联网技术的发展和普及,人们在日常生活中使用大量的网站和应用程序来获取信息和进行交流。
然而,由于信息过载和个体差异,人们常常难以找到真正感兴趣的内容。
这就是为什么推荐系统变得如此重要的原因。
推荐系统是一种根据用户的兴趣和偏好向他们提供个性化推荐的技术。
推荐系统的目标是通过分析用户的历史行为和兴趣,预测用户可能喜欢的项目,并向用户提供这些项目的推荐。
推荐系统的核心任务是解决信息过载问题,帮助用户发现他们可能感兴趣的内容。
在实际应用中,推荐系统被广泛应用于电子商务、社交媒体、电影和音乐等领域。
推荐系统可以基于多种不同的方法和技术进行实现。
其中最常见的方法是基于内容的推荐和协同过滤推荐。
基于内容的推荐方法是根据项目的相关特征和用户的历史兴趣匹配推荐内容。
例如,在电影推荐系统中,系统会分析用户对不同类型电影的兴趣并根据用户的喜好向他们推荐同类型的电影。
基于内容的推荐方法有一个明显的优点,就是能够提供个性化的推荐。
然而,它也存在一些挑战,比如如何有效地提取和表示项目的特征,以及如何解决用户行为的动态变化。
另一种常用的推荐方法是协同过滤推荐。
协同过滤推荐是基于用户和项目之间的相似性进行推荐。
当一个用户对某个项目感兴趣时,系统会向与该用户具有相似兴趣的其他用户推荐该项目。
协同过滤推荐方法不需要事先了解项目的特征,它主要依赖于用户的行为和反馈信息。
然而,协同过滤推荐也存在一些问题,比如冷启动问题(系统如何为新用户提供个性化推荐)和稀疏性问题(用户-项目矩阵中的大部分值为缺失值)。
除了基于内容的推荐和协同过滤推荐,还有一些其他的推荐方法,比如混合推荐、基于社交网络的推荐和基于领域的推荐等。
混合推荐是将多种不同的推荐方法结合起来,以提供更准确和可靠的推荐结果。
基于社交网络的推荐利用用户在社交网络中的关系和行为信息来进行个性化推荐。
基于领域的推荐是根据用户在特定领域的兴趣和偏好进行推荐。
这些方法提供了不同的视角和策略来解决推荐系统中的问题。
推荐系统综述recommder
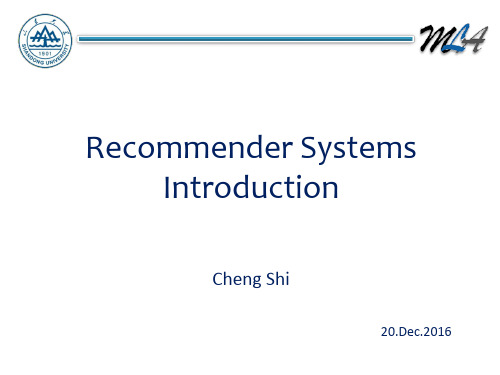
简单的算法+海量数据应该是能符合实际生产环境
Thanks !
history
After 2006 —Interpretability of the recommendation results —Join the sentiment analysis to the Matrix Factorization
—Recommender Systems with Deep Learning
2006年,NETFLIX宣布,设立一项大赛,公 开Байду номын сангаас集电影推荐系统的最佳电脑算法,第一 个能把现有推荐系统的准确率提高10%的参赛 者将获得一百万美元的奖金。2009 年 9 月 21 日,来自全世界 186 个国家的四万多个 参赛团队经过近三年的较量,终于有了结果。 一个由工程师和统计学家组成的七人团队夺 得了大奖,拿到了那张百万美元的超大支票。
Matrix factorization
Matrix factorization
Biases:
Temporal dynamics: Confidence levels:
Browsing Additional input sources : history,gender,age group
Zip code,income level
Matrix factorization
LFM(Latent factor model)
Matrix factorization
Probabilistic MF
Salakhutdinov, Ruslan, and Andriy Mnih. "Probabilistic matrix factorization."NIPS. Vol. 20. 2011.
推荐系统研究综述

推荐系统研究综述推荐系统是指通过利用大数据、机器学习等技术对用户兴趣偏好进行分析,向用户推荐与其兴趣相关的内容或信息的系统。
随着互联网普及,推荐系统开始在电子商务、社交网络、新闻资讯等领域中被广泛应用,为用户提供更加个性化的服务和体验。
推荐系统研究综述主要涉及推荐系统的发展历程、实现方式、评估指标和研究热点等方面。
本篇综述将从以下几个方面进行介绍。
一、推荐系统的发展历程推荐系统的发展始于上世纪90年代,当时主要应用于电子邮件和新闻推荐。
随着互联网的发展,推荐系统逐渐地被应用到在线商店、音乐、电影、图书等各个领域中。
传统的推荐系统主要基于协同过滤(Collaborative Filtering)算法,该算法通过分析用户历史行为和偏好,寻找相似的用户群体,然后根据这些用户的行为和偏好,向目标用户推荐相关内容。
近年来,基于内容的推荐系统(Content-based Recommendation)和混合推荐系统(Hybrid Recommendation)等新型推荐算法相继被提出,进一步丰富了推荐系统的技术层面。
二、推荐系统的实现方式推荐系统的实现方式主要包括基于服务器的推荐、基于浏览器的推荐和移动推荐。
基于服务器的推荐是通过向用户提供推荐产品或内容的服务来实现,如购物网站或音乐网站提供的“猜你喜欢”服务。
基于浏览器的推荐则是通过在用户浏览器中展示相关推荐内容来实现,如浏览器插件或应用程序等。
移动推荐则是通过基于用户地理位置等信息来推荐相关内容或服务,如附近的餐厅、景点等信息。
三、推荐系统评估指标评估指标是评估推荐系统性能的重要标准,主要包括准确性、覆盖率、新颖性、多样性等。
其中,准确性是指推荐系统所推荐物品与用户真实兴趣的匹配度,覆盖率是指推荐系统所推荐物品的覆盖度,新颖性是指推荐系统所推荐物品的新颖度,多样性是指推荐系统所推荐物品的多样性。
目前,推荐系统研究的热点主要集中在以下几个方面:1.基于深度学习的推荐算法;2.社交推荐;3.增量学习和在线算法;4.多任务学习和多目标优化;5.可解释性推荐和隐私保护;6.面向长尾问题的推荐算法。
restormer解析
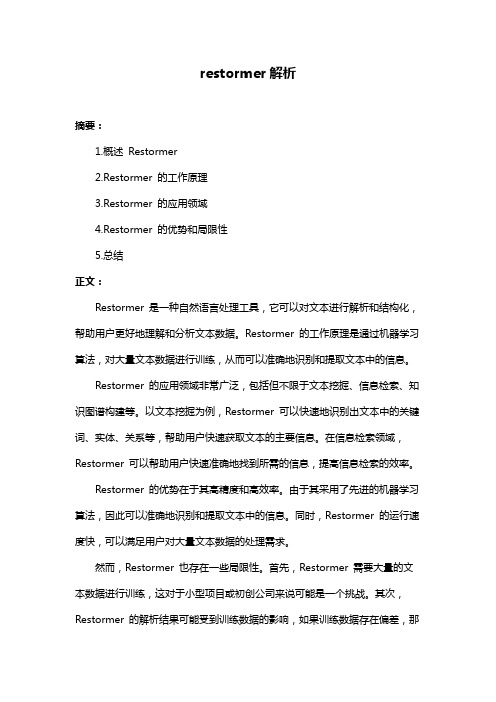
restormer解析
摘要:
1.概述Restormer
2.Restormer 的工作原理
3.Restormer 的应用领域
4.Restormer 的优势和局限性
5.总结
正文:
Restormer 是一种自然语言处理工具,它可以对文本进行解析和结构化,帮助用户更好地理解和分析文本数据。
Restormer 的工作原理是通过机器学习算法,对大量文本数据进行训练,从而可以准确地识别和提取文本中的信息。
Restormer 的应用领域非常广泛,包括但不限于文本挖掘、信息检索、知识图谱构建等。
以文本挖掘为例,Restormer 可以快速地识别出文本中的关键词、实体、关系等,帮助用户快速获取文本的主要信息。
在信息检索领域,Restormer 可以帮助用户快速准确地找到所需的信息,提高信息检索的效率。
Restormer 的优势在于其高精度和高效率。
由于其采用了先进的机器学习算法,因此可以准确地识别和提取文本中的信息。
同时,Restormer 的运行速度快,可以满足用户对大量文本数据的处理需求。
然而,Restormer 也存在一些局限性。
首先,Restormer 需要大量的文本数据进行训练,这对于小型项目或初创公司来说可能是一个挑战。
其次,Restormer 的解析结果可能受到训练数据的影响,如果训练数据存在偏差,那
么Restormer 的解析结果也可能存在偏差。
总的来说,Restormer 是一种强大的自然语言处理工具,它可以帮助用户更好地理解和分析文本数据。
- 1、下载文档前请自行甄别文档内容的完整性,平台不提供额外的编辑、内容补充、找答案等附加服务。
- 2、"仅部分预览"的文档,不可在线预览部分如存在完整性等问题,可反馈申请退款(可完整预览的文档不适用该条件!)。
- 3、如文档侵犯您的权益,请联系客服反馈,我们会尽快为您处理(人工客服工作时间:9:00-18:30)。
A Comparison
Scalability Diversity&
Precision
Netflix Prize
Netflix是一家美国在线视频网站,公司一 开始的主要业务是提供DVD和Blu-ray光盘的出 租服务。现在的主要业务是原创内容的网络流 媒体服务。2013年凭借高端自制美剧《纸牌屋》 和随后的多部剧集的超高质量引起全球瞩目。
2006年,NETFLIX宣布,设立一项大赛,公 开征集电影推荐系统的最佳电脑算法,第一 个能把现有推荐系统的准确率提高10%的参赛 者将获得一百万美元的奖金。2009 年 9 月 21 日,来自全世界 186 个国家的四万多个 参赛团队经过近三年的较量,终于有了结果。 一个由工程师和统计学家组成的七人团队夺 得了大奖,拿到了那张百万美元的超大支票。
Probabilistic MF
Probabilistic MF
Automatic Complexity Control
Constrained PMF
Experimental Results
Experimental Results
Problem
评价一个推荐系统标准
算法效率、可解释性
collaborative filtering
The Long Tail
User-based CF
Konstan, Joseph A., et al. "GroupLens: applying collaborative filtering to Usenet news." Communications of the ACM 40.3 (1997): 77-87
Linden, Greg, Brent Smith, and Jeremy York. "Amazon. com recommendations: Item-to-item collaborative filtering." IEEE Internet computing 7.1 (2003): 76-80.
Cosine Similarity
Jaccard Coefficient
Neighborhoods
K-neighborhoods or Threshold-based neighborhoods
Similarity Computing
Similarity Computing
Item-based CF
Item-based CF
-Few details
-basi
M users, N items
O( N M )
2
O( NM )
Item-based CF sample
Scalability
has more than 29 million customers and several million catalog items. For large retailers like , a good recommendation algorithm is scalable over very large customer bases and product catalogs, requires only subsecond processing time to generate online recommendations.
history
After 2006 —Interpretability of the recommendation results —Join the sentiment analysis to the Matrix Factorization
—Recommender Systems with Deep Learning
Matrix factorization
LFM(Latent factor model)
Matrix factorization
Probabilistic MF
Salakhutdinov, Ruslan, and Andriy Mnih. "Probabilistic matrix factorization."NIPS. Vol. 20. 2011.
Recommender Systems Introduction
Cheng Shi
20.Dec.2016
Outline
Backgrounds
& history
Algorithms
Content-based Recommendation Collaborative Filtering-based Recommendation • User-based Recommendation • Item-based Recommendation • Model-based Recommendation Problem
Backgrounds
Information overload is one of the most critical problems, and personalized recommendation system is a powerful tool to solve this problem.
Matrix factorization
Yehuda, Robert Bell, and Chris Volinsky. "Matrix factorization techniques for recommender systems." Computer 42.8 (2009): 30-37.
Matrix factorization
history
Content Filtering [Before 1992] Grouplens [1994] —Frist recommender system using rating data Movielens[1997] —Frist movie recommender system —Provide well-known dataset for researchers Amazon —proposed item-based collaborative filtering (Patent is filed in 1998 and issued in 2001) Netflix Prize[2006] —Latent Factor Model(SVD,RSVD,NSVD,SVD++) —Yehuda Koren’s team get prize
User-based CF
Grouplens
—The rating servers predict scores based on the heuristic that people who agreed in the past will probably agree again.
—Basic idea: recommend items similar to users favorite items. —GroupLens Architecture Overview —A Dynamic and Fast-Paced Information System —Ratings Sparsity —Performance Challenges
A basic matrix factorization model
Matrix factorization
ˆ R P QT = R
Matrix factorization
K ˆij piT q j k r 1 pik qkj
2 K 2 ˆij ) 2 (rij k eij (rij r p q ) 1 ik kj
Content-based
example (movie)
Item Profiles - its genre - the participating actors - Its box office popularity - so forth User Profiles - movies and score list
简单的算法+海量数据应该是能符合实际生产环境
Thanks !
Matrix factorization
Matrix factorization
Biases:
Temporal dynamics: Confidence levels:
Browsing Additional input sources : history,gender,age group
Zip code,income level
User-based CF
Establishment of user model
Similarity Computing
Find similar users set
Euclidean Distance Similarity
Similarity Computing
Pearson Correlation Similarity