2007美国大学生数学建模竞赛A题特等奖论文翻译
美国大学生数学建模论文及其翻译31552

Page 1 of 25
Best all time college coach Summary
In order to select the “best all time college coach” in the last century fairly, We take selecting the best male basketball coach as an example, and establish the TOPSIS sort - Comprehensive Evaluation improved model based on entropy and Analytical Hierarchy Process. The model mainly analyzed such indicators as winning rate, coaching time, the time of winning the championship, the number of races and the ability to perceive .Firstly , Analytical Hierarchy Process and Entropy are integratively utilized to determine the index weights of the selecting indicators Secondly,Standardized matrix and parameter matrix are combined to construct the weighted standardized decision matrix. Finally, we can get the college men's basketball com
数学建模全国赛07年A题一等奖论文
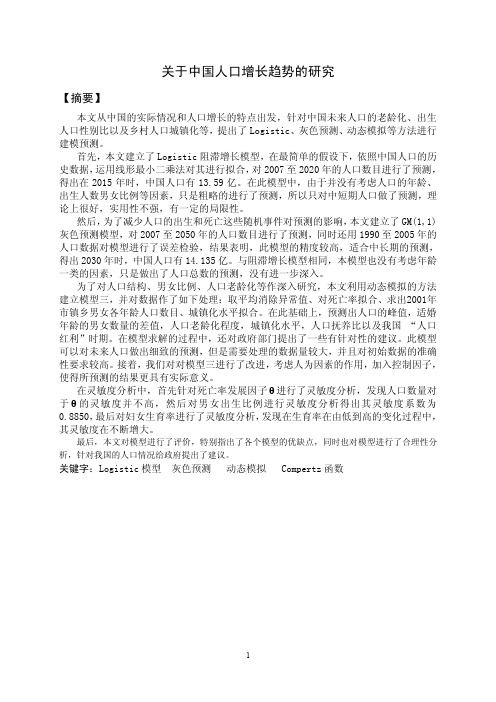
关于中国人口增长趋势的研究【摘要】本文从中国的实际情况和人口增长的特点出发,针对中国未来人口的老龄化、出生人口性别比以及乡村人口城镇化等,提出了Logistic、灰色预测、动态模拟等方法进行建模预测。
首先,本文建立了Logistic阻滞增长模型,在最简单的假设下,依照中国人口的历史数据,运用线形最小二乘法对其进行拟合,对2007至2020年的人口数目进行了预测,得出在2015年时,中国人口有13.59亿。
在此模型中,由于并没有考虑人口的年龄、出生人数男女比例等因素,只是粗略的进行了预测,所以只对中短期人口做了预测,理论上很好,实用性不强,有一定的局限性。
然后,为了减少人口的出生和死亡这些随机事件对预测的影响,本文建立了GM(1,1) 灰色预测模型,对2007至2050年的人口数目进行了预测,同时还用1990至2005年的人口数据对模型进行了误差检验,结果表明,此模型的精度较高,适合中长期的预测,得出2030年时,中国人口有14.135亿。
与阻滞增长模型相同,本模型也没有考虑年龄一类的因素,只是做出了人口总数的预测,没有进一步深入。
为了对人口结构、男女比例、人口老龄化等作深入研究,本文利用动态模拟的方法建立模型三,并对数据作了如下处理:取平均消除异常值、对死亡率拟合、求出2001年市镇乡男女各年龄人口数目、城镇化水平拟合。
在此基础上,预测出人口的峰值,适婚年龄的男女数量的差值,人口老龄化程度,城镇化水平,人口抚养比以及我国“人口红利”时期。
在模型求解的过程中,还对政府部门提出了一些有针对性的建议。
此模型可以对未来人口做出细致的预测,但是需要处理的数据量较大,并且对初始数据的准确性要求较高。
接着,我们对对模型三进行了改进,考虑人为因素的作用,加入控制因子,使得所预测的结果更具有实际意义。
在灵敏度分析中,首先针对死亡率发展因子θ进行了灵敏度分析,发现人口数量对于θ的灵敏度并不高,然后对男女出生比例进行灵敏度分析得出其灵敏度系数为0.8850,最后对妇女生育率进行了灵敏度分析,发现在生育率在由低到高的变化过程中,其灵敏度在不断增大。
数模美国赛总结部分英文

数模美国赛总结部分英文第一篇:数模美国赛总结部分英文Conclusions1、As our team set out to come up with a strategy on what would be the most efficient way to 我们提出了一种最有效的方法去解决……2、The first aspect that we took into major consideration was…….Other important findings through research made it apparent that the standard 首先我们考虑到……,其他重要的是我们通过研究使4、We have used mathematical modeling in a……to analyze some of the factors associated with such an activity。
为了分析这类问题的一些因素,我们运用数学模型……5、This “cannon problem” has been used in many forms in many differential equations courses in the Department of Mathematical Sciences for several years.这些年这些问题已经以不同的微分方程形式运用于自然科学部门。
6、In conclusion our team is very certain that the methods we came up with in 总之,我们很确定我们提出的方法7、We already know how well our results worked for…… 我们已经知道我们结果对……8、Now that the problem areas have been defined, we offer some ways to reduce the effect of these problems.既然已经定义了结果,我们提出一些方法减少对问题的影响。
美赛数学建模A题翻译版论文
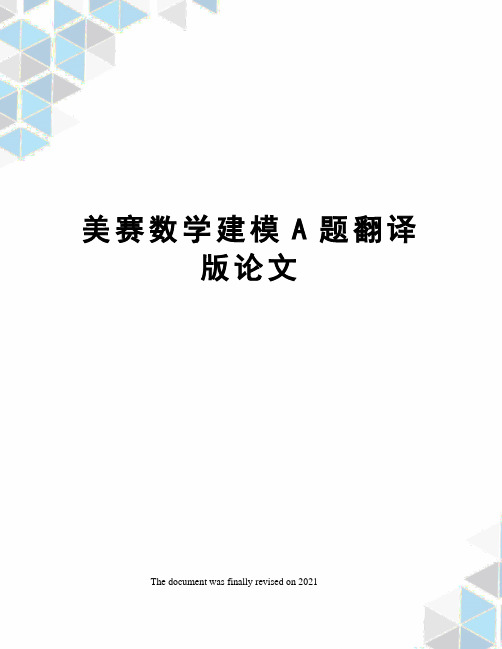
美赛数学建模A题翻译版论文The document was finally revised on 2021数学建模竞赛(MCM / ICM)汇总表基于细胞的高速公路交通模型自动机和蒙特卡罗方法总结基于元胞自动机和蒙特卡罗方法,我们建立一个模型来讨论“靠右行”规则的影响。
首先,我们打破汽车的运动过程和建立相应的子模型car-generation的流入模型,对于匀速行驶车辆,我们建立一个跟随模型,和超车模型。
然后我们设计规则来模拟车辆的运动模型。
我们进一步讨论我们的模型规则适应靠右的情况和,不受限制的情况, 和交通情况由智能控制系统的情况。
我们也设计一个道路的危险指数评价公式。
我们模拟双车道高速公路上交通(每个方向两个车道,一共四条车道),高速公路双向三车道(总共6车道)。
通过计算机和分析数据。
我们记录的平均速度,超车取代率、道路密度和危险指数和通过与不受规则限制的比较评估靠右行的性能。
我们利用不同的速度限制分析模型的敏感性和看到不同的限速的影响。
左手交通也进行了讨论。
根据我们的分析,我们提出一个新规则结合两个现有的规则(靠右的规则和无限制的规则)的智能系统来实现更好的的性能。
1介绍术语假设2模型设计的元胞自动机流入模型跟随模型超车模型超车概率超车条件危险指数两套规则CA模型靠右行无限制行驶规则3补充分析模型加速和减速概率分布的设计设计来避免碰撞4模型实现与计算机5数据分析和模型验证平均速度快车的平均速度密度超车几率危险指数6在不同速度限制下敏感性评价模型7驾驶在左边8交通智能系统智能系统的新规则模型的适应度智能系统结果9结论10优点和缺点优势弱点引用附录。
1 Introduction今天,大约65%的世界人口生活在右手交通的国家和35%在左手交通的国家交通流量。
[worldstandards。
欧盟,2013] 右手交通的国家,比如美国和中国,法规要求驾驶在靠路的右边行走。
多车道高速公路在这些国家经常使用一个规则,要求司机在最右边开车除非他们超过另一辆车,在这种情况下,他们移动到左边的车道、通过,返回到原来的车道。
05年美国大学生数学建模竞赛A题特等奖论文翻译
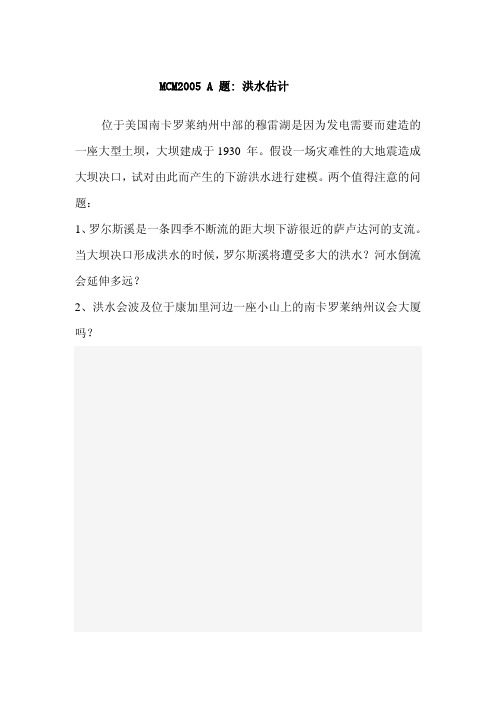
在每一时刻流出水的体积等于裂口的面积乘以水的速率乘以时间:
h h Vwater leaeing = wbreach (
−
lake
s)
dam
water
leaving ttime
step
其中:V 是体积, w 是宽度, h 是高度, s 是速度, t 是时间。
我们假设该湖是一个大的直边贮槽,所以当水的高度确定时,其面积不改变。 这意味着,湖的高度等于体积除以面积
在南卡罗莱那州的中央,一个湖被一个 75 年的土坝抑制。如果大坝被地震破坏 将会发生什么事?这个担心是基于1886年发生在查尔斯顿的一场地震,科学家们 相信它里氏7.3级[联邦能源管理委员会2002]。断层线的位置几乎直接在穆雷湖 底(SCIway 2000;1997,1998年南CarolinaGeological调查)和在这个地区小地震 的频率迫使当局考虑这样一个灾难的后果。
)
−
1 2
ห้องสมุดไป่ตู้
λ
2
(u
n j +1
−
2u j
+
n
+
u
n j
)
这里的上层指数表示时间和较低的空间, λ 是时间与空间步长的大小的比值。
(我们的模型转换以距离和时间做模型的单位,因此每个步长为 1)。第二个条 件的作用是受潮尖峰,因为它看起来不同并补偿于在每个点的任一边上的点。
我们发现该模型对粗糙度参数 n 高度敏感(注意,这是只在通道中的有效粗 糙度)。当 n 很大(即使在大河流的标准值 0.03)。对水流 和洪水堆积的倾向有 较高的抗拒,这将导致过量的陡水深资料,而且往往使模型崩溃。幸运的是,我
我们的任务是预测沿着Saluda河从湖穆雷大坝到哥伦比亚的水位变化,如果 发生了1886年相同规模的地震破坏了大坝。特别是支流罗尔斯溪会回流多远和哥 伦比亚南卡罗来纳州的州议会大厦附近的水位会多高。
2016年美国大学生数学建模大赛A题获奖论文A Hot Bath
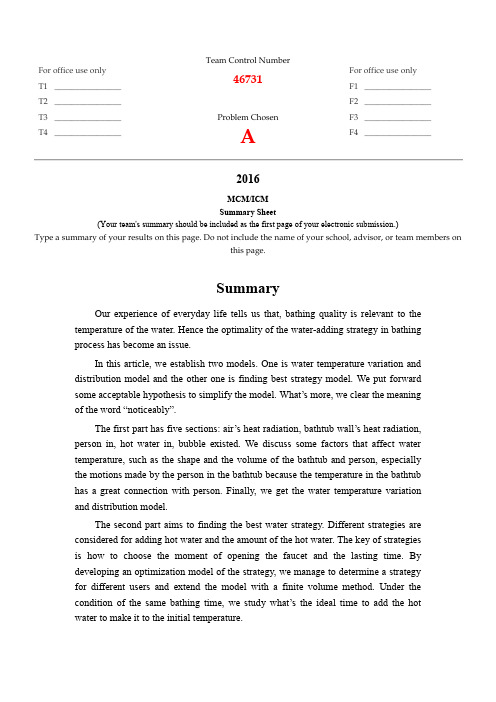
The first part has five sections: air’s heat radiation, bathtub wall’s heat radiation, person in, hot water in, bubble existed. We discuss some factors that affect water temperature, such as the shape and the volume of the bathtub and person, especially the motions made by the person in the bathtub because the temperature in the bathtub has a great connection with person. Finally, we get the water temperature variation and distribution model.
In this article, we establish two models. One is water temperature variation and distr one is finding best strategy model. We put forward some acceptable hypothesis to simplify the model. What’s more, we clear the meaning of the word “noticeably”.
数学建模美赛07年A题 中文翻译
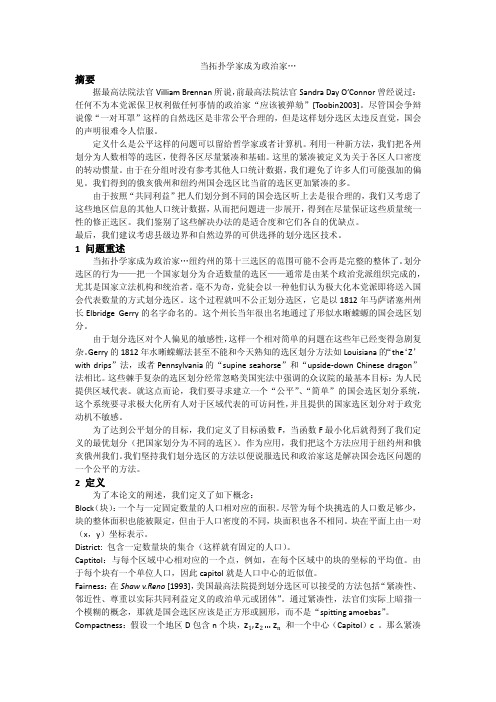
当拓扑学家成为政治家…摘要据最高法院法官Villiam Brennan所说,前最高法院法官Sandra Day O’Connor曾经说过:任何不为本党派保卫权利做任何事情的政治家“应该被弹劾”[Toobin2003]。
尽管国会争辩说像“一对耳罩”这样的自然选区是非常公平合理的,但是这样划分选区太违反直觉,国会的声明很难令人信服。
定义什么是公平这样的问题可以留给哲学家或者计算机。
利用一种新方法,我们把各州划分为人数相等的选区,使得各区尽量紧凑和基础。
这里的紧凑被定义为关于各区人口密度的转动惯量。
由于在分组时没有参考其他人口统计数据,我们避免了许多人们可能强加的偏见。
我们得到的俄亥俄州和纽约州国会选区比当前的选区更加紧凑的多。
由于按照“共同利益”把人们划分到不同的国会选区听上去是很合理的,我们又考虑了这些地区信息的其他人口统计数据,从而把问题进一步展开,得到在尽量保证这些质量统一性的修正选区。
我们鉴别了这些解决办法的是适合度和它们各自的优缺点。
最后,我们建议考虑县级边界和自然边界的可供选择的划分选区技术。
1 问题重述当拓扑学家成为政治家…纽约州的第十三选区的范围可能不会再是完整的整体了。
划分选区的行为——把一个国家划分为合适数量的选区——通常是由某个政治党派组织完成的,尤其是国家立法机构和统治者。
毫不为奇,党徒会以一种他们认为极大化本党派即将送入国会代表数量的方式划分选区。
这个过程就叫不公正划分选区,它是以1812年马萨诸塞州州长Elbridge Gerry的名字命名的。
这个州长当年很出名地通过了形似水晰蝾螈的国会选区划分。
由于划分选区对个人偏见的敏感性,这样一个相对简单的问题在这些年已经变得急剧复杂。
Gerry的1812年水晰蝾螈法甚至不能和今天熟知的选区划分方法如Louisiana的“the‘Z’with drips”法,或者Pennsylvania的“supine seahorse”和“upside-down Chinese dragon”法相比。
历年美国大学生数学建模竞赛试题MCM.(翻译版)doc
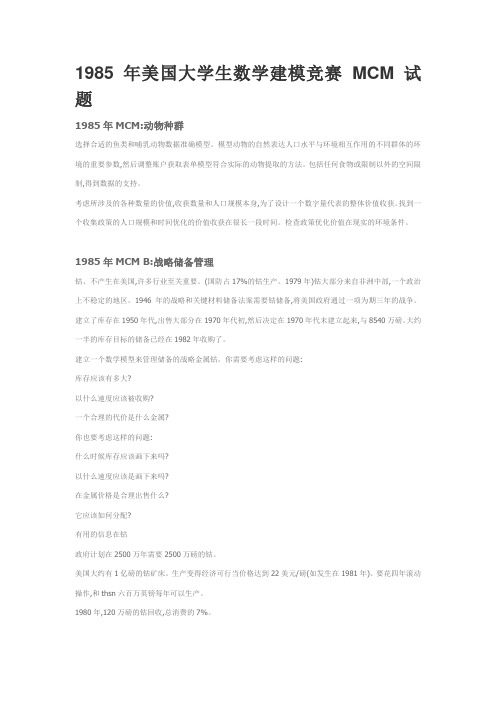
1985 年美国大学生数学建模竞赛MCM 试题1985年MCM:动物种群选择合适的鱼类和哺乳动物数据准确模型。
模型动物的自然表达人口水平与环境相互作用的不同群体的环境的重要参数,然后调整账户获取表单模型符合实际的动物提取的方法。
包括任何食物或限制以外的空间限制,得到数据的支持。
考虑所涉及的各种数量的价值,收获数量和人口规模本身,为了设计一个数字量代表的整体价值收获。
找到一个收集政策的人口规模和时间优化的价值收获在很长一段时间。
检查政策优化价值在现实的环境条件。
1985年MCM B:战略储备管理钴、不产生在美国,许多行业至关重要。
(国防占17%的钴生产。
1979年)钴大部分来自非洲中部,一个政治上不稳定的地区。
1946年的战略和关键材料储备法案需要钴储备,将美国政府通过一项为期三年的战争。
建立了库存在1950年代,出售大部分在1970年代初,然后决定在1970年代末建立起来,与8540万磅。
大约一半的库存目标的储备已经在1982年收购了。
建立一个数学模型来管理储备的战略金属钴。
你需要考虑这样的问题:库存应该有多大?以什么速度应该被收购?一个合理的代价是什么金属?你也要考虑这样的问题:什么时候库存应该画下来吗?以什么速度应该是画下来吗?在金属价格是合理出售什么?它应该如何分配?有用的信息在钴政府计划在2500万年需要2500万磅的钴。
美国大约有1亿磅的钴矿床。
生产变得经济可行当价格达到22美元/磅(如发生在1981年)。
要花四年滚动操作,和thsn六百万英镑每年可以生产。
1980年,120万磅的钴回收,总消费的7%。
1986 年美国大学生数学建模竞赛MCM 试题1986年MCM A:水文数据下表给出了Z的水深度尺表面点的直角坐标X,Y在码(14数据点表省略)。
深度测量在退潮。
你的船有一个五英尺的草案。
你应该避免什么地区内的矩形(75200)X(-50、150)?1986年MCM B:Emergency-Facilities位置迄今为止,力拓的乡牧场没有自己的应急设施。
2007年美国数学建模题论文-基于模式识别的选区划分

基于模式识别的选区划分1.摘要本文针对研究众议员的选区重新划分问题。
运用方法简洁有效、充分公平且可行性强的“简单”原则,引入类基尼系数,并通过0-1矩阵确定合并方案。
为确保类似于少数族裔群体的利益,运用建设性杰利蝾螈模型,将某些少数群体合并。
最后,扩展到多个城市同时合并的模型,以便提高运算速度。
经过理论分析和数值计算结果验证表明模型设计合理,实用性强。
关键词:选区划分类基尼系数 0-1矩阵建设性杰利蝾螈2.问题的提出美国宪法规定众议院由一定数目的众议员组成,目前是435人,他们是由各州按照该州人口占全国总人口的百分比选出来的。
尽管这种规定提供了确定每个州有多少众议员的方法,但是一点也没有说及有关一个特定的众议员所代表的选区应该怎样按地区决定的问题。
这种疏忽已经导致了按某种标准看来是违反常情的很不好的选区安排,至少某些人认为通常是不必这样做的。
因此提出以下问题:假设有机会去制定一个州的众议院的选区,如何把它作为一种纯“基础性”的练习来创建一个州的所有选区的“最简单”的划分。
这些划分规则中至少要包含一条:该州的每个选区必须有同样的人口。
确定“简单”的定义;并就解决方法公正的做出一个能够使该州选民信服的论证。
作为方法的应用,试创建纽约州的按地域来说是简单的选区划分。
3.问题的分析所谓选举,其实质就是在评选人对候选人先后(优劣)次序排队的基础上,根据某一事先规定的选举规则决定出候选人的一个先后次序,即得出选举结果。
为了便于管理,举办选举一般都需要按人口大致平均地划分选区,不仅如此,每隔一段时间,选区往往有必要重新划分或作出调整,因为每个选区里面能选出来的人是固定的,但是里面的人口是变化的;当选区内人口出现大幅波动时,会导致被选出来的人和选区人口不成比例的现象,“一人一票”的原则就会被破坏,这就有必要重新划分选区。
纽约州按地域的简单的划分选区是一个纯基础性研究。
基础研究是指为获得关于现象和可观察事实的基本原理及新知识而进行的实验性和理论性工作,它不以任何专门或特定的应用或使用为目的。
美赛一等奖论文中文翻译版
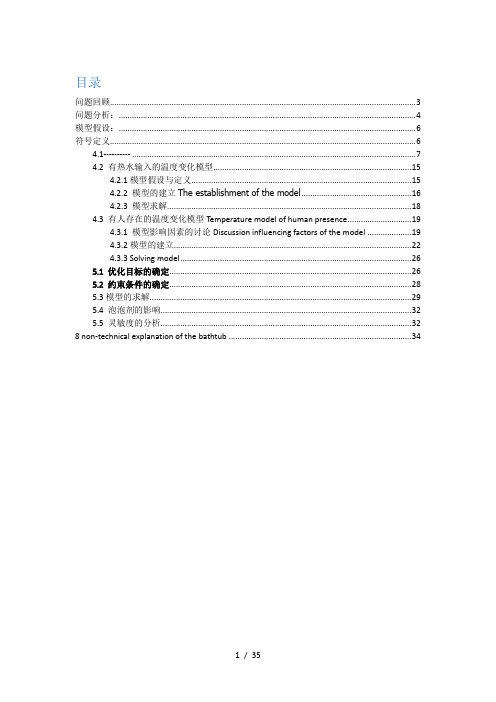
目录问题回顾 (3)问题分析: (4)模型假设: (6)符号定义 (6)4.1---------- (7)4.2 有热水输入的温度变化模型 (15)4.2.1模型假设与定义 (15)4.2.2 模型的建立The establishment of the model (16)4.2.3 模型求解 (18)4.3 有人存在的温度变化模型Temperature model of human presence (19)4.3.1 模型影响因素的讨论Discussion influencing factors of the model (19)4.3.2模型的建立 (22)4.3.3 Solving model (26)5.1 优化目标的确定 (26)5.2 约束条件的确定 (28)5.3模型的求解 (29)5.4 泡泡剂的影响 (32)5.5 灵敏度的分析 (32)8 non-technical explanation of the bathtub (34)Summary人们经常在充满热水的浴缸里得到清洁和放松。
本文针对只有一个简单的热水龙头的浴缸,建立一个多目标优化模型,通过调整水龙头流量大小和流入水的温度来使整个泡澡过程浴缸内水温维持基本恒定且不会浪费太多水。
首先分析浴缸中水温度变化的具体情况。
根据能量转移的特点将浴缸中的热量损失分为两类情况:沿浴缸四壁和底面向空气中丧失的热量根据傅里叶导热定律求出;沿水面丧失的热量根据水由液态变为气态的焓变求出。
因涉及的参数过多,将系数进行回归分析的得到一个一元二次函数。
结合两类热量建立了温度关于时间的微分方程。
加入阻滞因子考虑环境温湿度升高对水温的影响,最后得到水温度随时间的变化规律(见图**)。
优化模型考虑保持水龙头匀速流入热水的情况。
将过程分为浴缸未加满和浴缸加满而水从排水口溢出的两种情况,根据能量守恒定律优化上述微分方程,建立一个有热源的情况下水的温度随时间变化的分段模型,(见图**)接下来考虑人在浴缸中对水温的影响。
2007美国大学生数学建模竞赛B题特等奖论文_免费下载
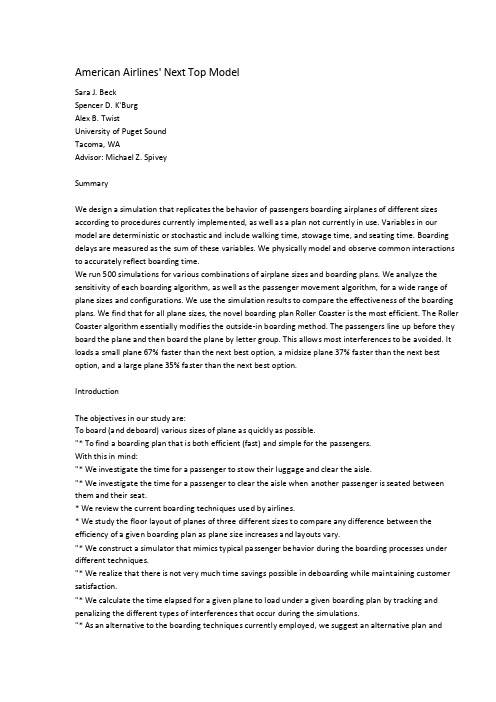
American Airlines' Next Top ModelSara J. BeckSpencer D. K'BurgAlex B. TwistUniversity of Puget SoundTacoma, WAAdvisor: Michael Z. SpiveySummaryWe design a simulation that replicates the behavior of passengers boarding airplanes of different sizes according to procedures currently implemented, as well as a plan not currently in use. Variables in our model are deterministic or stochastic and include walking time, stowage time, and seating time. Boarding delays are measured as the sum of these variables. We physically model and observe common interactions to accurately reflect boarding time.We run 500 simulations for various combinations of airplane sizes and boarding plans. We analyze the sensitivity of each boarding algorithm, as well as the passenger movement algorithm, for a wide range of plane sizes and configurations. We use the simulation results to compare the effectiveness of the boarding plans. We find that for all plane sizes, the novel boarding plan Roller Coaster is the most efficient. The Roller Coaster algorithm essentially modifies the outside-in boarding method. The passengers line up before they board the plane and then board the plane by letter group. This allows most interferences to be avoided. It loads a small plane 67% faster than the next best option, a midsize plane 37% faster than the next best option, and a large plane 35% faster than the next best option.IntroductionThe objectives in our study are:To board (and deboard) various sizes of plane as quickly as possible."* To find a boarding plan that is both efficient (fast) and simple for the passengers.With this in mind:"* We investigate the time for a passenger to stow their luggage and clear the aisle."* We investigate the time for a passenger to clear the aisle when another passenger is seated between them and their seat.* We review the current boarding techniques used by airlines.* We study the floor layout of planes of three different sizes to compare any difference between the efficiency of a given boarding plan as plane size increases and layouts vary."* We construct a simulator that mimics typical passenger behavior during the boarding processes under different techniques."* We realize that there is not very much time savings possible in deboarding while maintaining customer satisfaction."* We calculate the time elapsed for a given plane to load under a given boarding plan by tracking and penalizing the different types of interferences that occur during the simulations."* As an alternative to the boarding techniques currently employed, we suggest an alternative plan andassess it using our simulator."* We make recommendations regarding the algorithms that proved most efficient for small, midsize, and large planes.Interferences and Delays for BoardingThere are two basic causes for interference-someone blocking a passenger,in an aisle and someone blocking a passenger in a row. Aisle interference is caused when the passenger ahead of you has stopped moving and is preventing you from continuing down the aisle towards the row with your seat. Row interference is caused when you have reached the correct row but already-seated passengers between the aisle and your seat are preventing you from immediately taking your seat. A major cause of aisle interference is a passenger experiencing rowinterference.We conducted experiments, using lined-up rows of chairs to simulate rows in an airplane and a team member with outstretched arms to act as an overhead compartment, to estimate parameters for the delays cause by these actions. The times that we found through our experimentation are given in Table 1.We use these times in our simulation to model the speed at which a plane can be boarded. We model separately the delays caused by aisle interference and row interference. Both are simulated using a mixed distribution definedas follows:Y = min{2, X},where X is a normally distributed random variable whose mean and standard deviation are fixed in our experiments. We opt for the distribution being partially normal with a minimum of 2 after reasoning that other alternative and common distributions (such as the exponential) are too prone to throw a small value, which is unrealistic. We find that the average row interference time is approximately 4 s with a standard deviation of 2 s, while the average aisle interference time is approximately 7 s with a standard deviation of 4 s. These values are slightly adjusted based on our team's cumulative experience on airplanes.Typical Plane ConfigurationsEssential to our model are industry standards regarding common layouts of passenger aircraft of varied sizes. We use an Airbus 320 plane to model a small plane (85-210 passengers) and the Boeing 747 for a midsize plane (210-330 passengers). Because of the lack of large planes available on the market, we modify the Boeing 747 by eliminating the first-class section and extending the coach section to fill the entire plane. This puts the Boeing 747 close to its maximum capacity. This modified Boeing 747 has 55 rows, all with the same dimensions as the coach section in the standard Boeing 747. Airbus is in the process of designing planes that can hold up to 800 passengers. The Airbus A380 is a double-decker with occupancy of 555 people in three different classes; but we exclude double-decker models from our simulation because it is the larger, bottom deck that is the limiting factor, not the smaller upper deck.Current Boarding TechniquesWe examine the following industry boarding procedures:* random-order* outside-in* back-to-front (for several group sizes)Additionally, we explore this innovative technique not currently used by airlines:* "Roller Coaster" boarding: Passengers are put in order before they board the plane in a style much like those used by theme parks in filling roller coasters.Passengers are ordered from back of the plane to front, and they board in seatletter groups. This is a modified outside-in technique, the difference being that passengers in the same group are ordered before boarding. Figure 1 shows how this orderi ng could take place. By doing this, most interferencesare avoided.Current Deboarding TechniquesPlanes are currently deboarded in an aisle-to-window and front-to-back order. This deboarding method comes out of the passengers' desire to be off the plane as quickly as possible. Any modification of this technique could leadto customer dissatisfaction, since passengers may be forced to wait while others seated behind them on theplane are deboarding.Boarding SimulationWe search for the optimal boarding technique by designing a simulation that models the boarding process and running the simulation under different plane configurations and sizes along with different boarding algorithms. We then compare which algorithms yielded the most efficient boarding process.AssumptionsThe environment within a plane during the boarding process is far too unpredictable to be modeled accurately. To make our model more tractable,we make the following simplifying assumptions:"* There is no first-class or special-needs seating. Because the standard industry practice is to board these passengers first, and because they generally make up a small portion of the overall plane capacity, any changes in the overall boarding technique will not apply to these passengers."* All passengers board when their boarding group is called. No passengers arrive late or try to board the plane early."* Passengers do not pass each other in the aisles; the aisles are too narrow."* There are no gaps between boarding groups. Airline staff call a new boarding group before the previous boarding group has finished boarding the plane."* Passengers do not travel in groups. Often, airlines allow passengers boarding with groups, especially with younger children, to board in a manner convenient for them rather than in accordance with the boarding plan. These events are too unpredictable to model precisely."* The plane is full. A full plane would typically cause the most passenger interferences, allowing us to view the worst-case scenario in our model."* Every row contains the same number of seats. In reality, the number of seats in a row varies due to engineering reasons or to accommodate luxury-class passengers.ImplementationWe formulate the boarding process as follows:"* The layout of a plane is represented by a matrix, with the rows representing rows of seats, and each column describing whether a row is next to the window, aisle, etc. The specific dimensions vary with each plane type. Integer parameters track which columns are aisles."* The line of passengers waiting to board is represented by an ordered array of integers that shrinks appropriately as they board the plane."* The boarding technique is modeled in a matrix identical in size to the matrix representing the layout of the plane. This matrix is full of positive integers, one for each passenger, assigned to a specific submatrix, representing each passenger's boarding group location. Within each of these submatrices, seating is assigned randomly torepresent the random order in which passengers line up when their boarding groups are called."* Interferences are counted in every location where they occur within the matrix representing the plane layout. These interferences are then cast into our probability distribution defined above, which gives ameasurement of time delay."* Passengers wait for interferences around them before moving closer to their assigned seats; if an interference is found, the passenger will wait until the time delay has finished counting down to 0."* The simulation ends when all delays caused by interferences have counted down to 0 and all passengers have taken their assigned seats.Strengths and Weaknesses of the ModelStrengths"* It is robust for all plane configurations and sizes. The boarding algorithms that we design can be implemented on a wide variety of planes with minimal effort. Furthermore, the model yields reasonable results as we adjust theparameters of the plane; for example, larger planes require more time to board, while planes with more aisles can load more quickly than similarlysized planes with fewer aisles."* It allows for reasonable amounts of variance in passenger behavior. While with more thorough experimentation a superior stochastic distribution describing the delays associated with interferences could be found, our simulationcan be readily altered to incorporate such advances."* It is simple. We made an effort to minimize the complexity of our simulation, allowing us to run more simulations during a greater time period and mini mizing the risk of exceptions and errors occurring."* It is fairly realistic. Watching the model execute, we can observe passengers boarding the plane, bumping into each other, taking time to load their baggage, and waiting around as passengers in front of them move out of theway. Its ability to incorporate such complex behavior and reduce it are key to completing our objective. Weaknesses"* It does not account for passengers other than economy-class passengers."* It cannot simulate structural differences in the boarding gates which couldpossibly speed up the boarding process. For instance, some airlines in Europeboard planes from two different entrances at once."* It cannot account for people being late to the boarding gate."* It does not account for passenger preferences or satisfaction.Results and Data AnalysisFor each plane layout and boarding algorithm, we ran 500 boarding simulations,calculating mean time and standard deviation. The latter is important because the reliability of plane loading is important for scheduling flights.We simulated the back-to-front method for several possible group sizes.Because of the difference in thenumber of rows in the planes, not all group size possibilities could be implemented on all planes.Small PlaneFor the small plane, Figure 2 shows that all boarding techniques except for the Roller Coaster slowed the boarding process compared to the random boarding process. As more and more structure is added to the boarding process, while passenger seat assignments continue to be random within each of the boarding groups, passenger interference backs up more and more. When passengers board randomly, gaps are created between passengers as some move to the back while others seat themselves immediately upon entering the plane, preventing any more from stepping off of the gate and onto the plane. These gaps prevent passengers who board early and must travel to the back of the plane from causing interference with many passengers behind them. However, when we implement the Roller Coaster algorithm, seat interference is eliminated, with the only passenger causing aisle interference being the very last one to boardfrom each group.Interestingly, the small plane's boarding times for all algorithms are greater than their respective boarding time for the midsize plane! This is because the number of seats per row per aisle is greater in the small plane than in the midsize plane.Midsize PlaneThe results experienced from the simulations of the mid-sized plane areshown in Figure 3 and are comparable to those experienced by the small plane.Again, the Roller Coaster method proved the most effective.Large PlaneFigure 4 shows that the boarding time for a large aircraft, unlike the other plane configurations, drops off when moving from the random boarding algorithm to the outside-in boarding algorithm. Observing the movements by the passengers in the simulation, it is clear that because of the greater number of passengers in this plane, gaps are more likely to form between passengers in the aisles, allowing passengers to move unimpeded by those already on board.However, both instances of back-to-front boarding created too much structure to allow these gaps to form again. Again, because of the elimination of row interference it provides for, Roller Coaster proved to be the most effective boarding method.OverallThe Roller Coaster boarding algorithm is the fastest algorithm for any plane pared to the next fastest boarding procedure, it is 35% faster for a large plane, 37% faster for a midsize plane, and 67% faster for a small plane. The Roller Coaster boarding procedure also has the added benefit of very low standard deviation, thus allowing airlines a more reliable boarding time. The boarding time for the back-to-front algorithms increases with the number of boarding groups and is always slower than a random boarding procedure.The idea behind a back-to-front boarding algorithm is that interference at the front of the plane is avoided until passengers in the back sections are already on the plane. A flaw in this procedure is that having everyone line up in the plane can cause a bottleneck that actually increases the loading time. The outside-in ("Wilma," or window, middle, aisle) algorithm performs better than the random boarding procedure only for the large plane. The benefit of the random procedure is that it evenly distributes interferences throughout theplane, so that they are less likely to impact very many passengers.Validation and Sensitivity AnalysisWe developed a test plane configuration with the sole purpose of implementing our boarding algorithms on planes of all sizes, varying from 24 to 600 passengers with both one or two aisles.We also examined capacities as low as 70%; the trends that we see at full capacity are reflected at these lower capacities. The back-to-front and outside-in algorithms do start to perform better; but this increase in performance is relatively small, and the Roller Coaster algorithm still substantially outperforms them. Underall circumstances, the algorithms we test are robust. That is, they assign passenger to seats in accordance with the intention of the boarding plans used by airlines and move passengers in a realistic manner.RecommendationsWe recommend that the Roller Coaster boarding plan be implemented for planes of all sizes and configurations for boarding non-luxury-class and nonspecial needs passengers. As planes increase in size, its margin of success in comparison to the next best method decreases; but we are confident that the Roller Coaster method will prove robust. We recommend boarding groups that are traveling together before boarding the rest of the plane, as such groups would cause interferences that slow the boarding. Ideally, such groups would be ordered before boarding.Future WorkIt is inevitable that some passengers will arrive late and not board the plane at their scheduled time. Additionally, we believe that the amount of carry-on baggage permitted would have a larger effect on the boarding time than the specific boarding plan implemented-modeling this would prove insightful.We also recommend modifying the simulation to reflect groups of people traveling (and boarding) together; this is especially important to the Roller Coaster boarding procedure, and why we recommend boarding groups before boarding the rest of the plane.。
美国大学生数学建模大赛优秀论文一等奖摘要
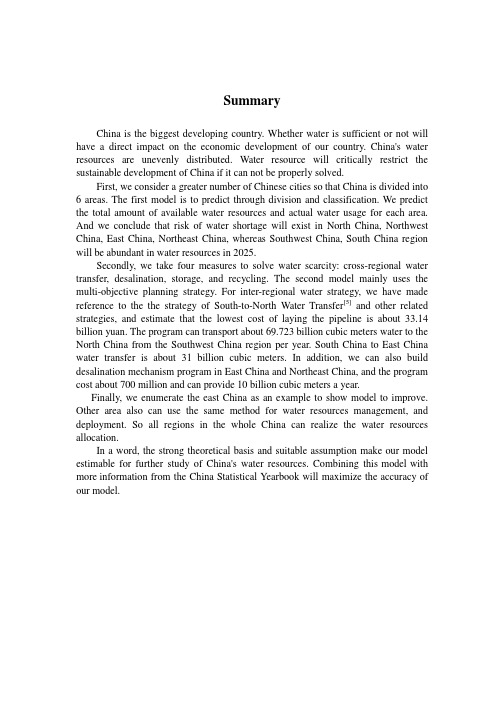
SummaryChina is the biggest developing country. Whether water is sufficient or not will have a direct impact on the economic development of our country. China's water resources are unevenly distributed. Water resource will critically restrict the sustainable development of China if it can not be properly solved.First, we consider a greater number of Chinese cities so that China is divided into 6 areas. The first model is to predict through division and classification. We predict the total amount of available water resources and actual water usage for each area. And we conclude that risk of water shortage will exist in North China, Northwest China, East China, Northeast China, whereas Southwest China, South China region will be abundant in water resources in 2025.Secondly, we take four measures to solve water scarcity: cross-regional water transfer, desalination, storage, and recycling. The second model mainly uses the multi-objective planning strategy. For inter-regional water strategy, we have made reference to the the strategy of South-to-North Water Transfer[5]and other related strategies, and estimate that the lowest cost of laying the pipeline is about 33.14 billion yuan. The program can transport about 69.723 billion cubic meters water to the North China from the Southwest China region per year. South China to East China water transfer is about 31 billion cubic meters. In addition, we can also build desalination mechanism program in East China and Northeast China, and the program cost about 700 million and can provide 10 billion cubic meters a year.Finally, we enumerate the east China as an example to show model to improve. Other area also can use the same method for water resources management, and deployment. So all regions in the whole China can realize the water resources allocation.In a word, the strong theoretical basis and suitable assumption make our model estimable for further study of China's water resources. Combining this model with more information from the China Statistical Yearbook will maximize the accuracy of our model.。
数模美赛2007年B题Airline Boarding
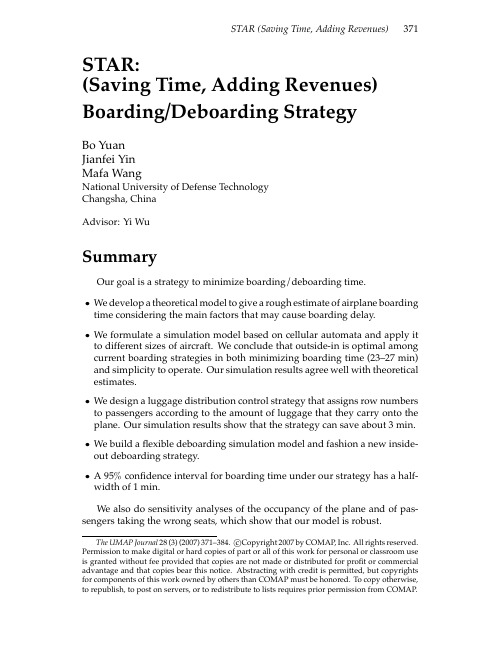
STAR:(Saving Time,Adding Revenues) Boarding/Deboarding StrategyBo YuanJianfei YinMafa WangNational University of Defense TechnologyChangsha,ChinaAdvisor:Yi WuSummaryOur goal is a strategy to minimize boarding/deboarding time.•We develop a theoretical model to give a rough estimate of airplane boarding time considering the main factors that may cause boarding delay.•We formulate a simulation model based on cellular automata and apply it to different sizes of aircraft.We conclude that outside-in is optimal among current boarding strategies in both minimizing boarding time(23–27min) and simplicity to operate.Our simulation results agree well with theoretical estimates.•We design a luggage distribution control strategy that assigns row numbers to passengers according to the amount of luggage that they carry onto the plane.Our simulation results show that the strategy can save about3min.•We build aÁexible deboarding simulation model and fashion a new inside-out deboarding strategy.•A95%conÀdence interval for boarding time under our strategy has a half-width of1min.We also do sensitivity analyses of the occupancy of the plane and of pas-sengers taking the wrong seats,which show that our model is robust.The UMAP Journal28(3)(2007)371–384.c Copyright2007by COMAP,Inc.All rights reserved. Permission to make digital or hard copies of part or all of this work for personal or classroom use is granted without fee provided that copies are not made or distributed for proÀt or commercial advantage and that copies bear this notice.Abstracting with credit is permitted,but copyrights for components of this work owned by others than COMAP must be honored.To copy otherwise, to republish,to post on servers,or to redistribute to lists requires prior permission from COMAP.IntroductionAirline boarding and deboarding has been studied extensively in operations research literature.U.S.domestic carriers lose$220million per year in revenue for take-off delays[Funk2003].We examine strategies for boarding and deboarding planes with varying numbers of passengers,trying to minimize the boarding and deboarding time. Literature ReviewMarelli et al.[1998]designed a computer program called PEDS(Passen-ger Enplaning/Deplaning Simulation)that used a probabilistic discrete-event simulation to simulate boarding methods.PEDS predicted that it would take 22min to board a Boeing747-200.However,the paper did not lay out the boarding procedure.Van Landeghem[2000]stated that the fastest boarding strategy is individu-ally boarding by seat and row number,and the second fastest is a back-to-front “alternate half-row”boarding system,which was cited to take15.8min.He also proposed strategies with small numbers of boarding groups that are both faster and more robust against disturbances.A problem with the data is that onlyÀve replications were done for each boarding procedure tested[Pan2006].Later,van den Briel et al.[2003]showed that a reverse-pyramid boarding strategy could reduce airplane’s turn time by3-5min compared to a traditional back-to-front boarding approach.The boarding time depends on events called “interferences.”Unfortunately,all of these researchers used simulation based on small or mid-size airplanes that do not extend to the much larger aircraft under devel-opment today.Our approach and results can be applied in all sizes of airplanes. Basic Assumptions•First-class passengers boardÀrst.Hence,our simulation considers only economy-class passengers.•Passengers do not try to pass other passengers in the aisle.The aisles are narrow,so passengers have to wait to move until there are no“obstacles”in front of them.•A“call-off”system is used.Passengers board in ordered groups;gate agents announce which group is to board.•A passenger does not take the wrong seat and does not walk past the row of the right seat.Such mistakes inevitably delay boarding.Reasons for Boarding DelayNormal Delay“Interference”is the main reason for boarding delay.Van den Briel et al. [2003;2005]divide boarding interferences into two types:•Aisle interference:Since the aisle is narrow enough to allow only one pas-senger to proceed forward,aisle interference occurs when a passenger stows luggage.To do this,the passenger must stand in the aisle for a moment, thereby acting as an“obstacle”for passengers behind.•Seat interference:This kind of interference occur when a passenger is stalled by another one or two passengers sitting in the same half-row.Because of the limited space between contiguous rows,this passenger must ask these passenger already sitting in their seats to stand up and move into the aisle.Abnormal DelayPassengers take the wrong seats,or are late.These behaviors can hardly be avoided.Because of their complexity and variety,we don’t take them into consideration.Our main objective is to reduce seat and aisle interference.Theoretical Estimate ModelWe consider boarding time as made up of two parts:•Free boarding time t free,the total time if all passengers board without any interference or delay.•Interference time t inter,the total interference time including aisle interference and seat interference.So the total boarding time isT tota l=t free+t inter,(1)Free Boarding TimeWe consider the passengers as a steadyÁow that pours into the plane at a rate of vÁow passengers per minute.So the free boarding time ist free=nvÁow,(2)where n is the number of passengers.Interference TimeSeat InterferenceWe assume that the times to get from the seat to the aisle and get back are the same,both denoted by t S.Suppose that three passengers on the same side of a row are assigned to the same boarding group,passengers sitting in positions A (window),B(middle),and C(aisle).There are six equally likely kinds of seat interferences,corresponding to the boarding orders ABC,ACB,BAC,BCA, CAB,CBA.We calculate the interference time for each case.Take ACB as an example:The window-seat passenger boardsÀrst,followed by the aisle seat passenger;then the middle-seat passenger needs the aisle-seat passenger to get up and move to the aisle,the middle-seat passenger moves from the aisle to the seat,and the aisle-seat passenger and sits back down again.So the interference time is3t S.The results are shown in Table1.Table1.Seat interference time by boarding order.Boarding order ABC ACB BAC BCA CAB CBASeat interference time03t S3t S5t S6t S8t S The average seat interference time for3passengers in the same half-row is¯t S=256t S.With n passengers boarding,the total seat interference time ist S:inter=¯t S·n3=256t Sn3.(3)Aisle InterferenceLet P1,...,P n be the passengers in order in the queue,with corresponding row numbers r1,...,r n.We say P i blocks P j if r i<r j.We use the number of blocking times as the number of aisle interference times,that is,when we calculate total interference times,we don’t consider the situation that two or more blockings happen together.For example,for passengers P1,...,P5in rows1,4,5,2and3,P1blocks P2,P2blocks P3,and P4blocks P5.But actually, after P1is seated,P2and P4can stow luggage simultaneously,and only P3and P5need to wait(two intervals of interference)to stow luggage.To simplify the calculations,we think of this as a total of three intervals of interference.As a result,to calculate the aisle interference times,we need calculate only the number of instances of r i<r i+1.Since the order of passengers is random, the number i of aisle interference times is a random variable.We assume that every permutation is equally likely,so the average aisle interference time isI=1n!i(r1,...,r n),where we sum over all permutations.The permutations can be divided into n!/2pairs,each of which is the reverse of the other;together,each pair will have(n−1)instances of r i<r i+1.HenceI=n−1 2.With t L for the average time to stow luggage,the total aisle interference time ist A:inter=n−12·t L.(4)From(1)–(4),we get the total boarding time asT=nvÁow+256t sn3+n−12t L.Data CollectionAircraft of Different SizesWe base our computer simulations on three types of airplane of different sizes:Airbus A320(small—124seats),Airbus A300(midsize—266seats),Air-bus A380(large—555seats).Experimental DataWe could not collect the needed by experimenting or by interviewing airline executives.Fortunately,this work has already been done by van den Briel et al.[2003]as cited by Pan[2006].They found the following average times:•Get-on time(time between gate agent and gate—assuming one gate agent):9.0s.•To advance one row:0.95s.•Stowage:7.1s.•Seat interference time:9.7s.Cellular Automata Simulation AlgorithmIn the cellular automata model of boarding analysis,each cell is designated as a passenger,a barrier,a road or a seat.The model restricts individual move-ments on the plane and computes total boarding time.Time,position,and passenger behavior are each discrete quantities.The passenger compartmentis speciÀed as a grid of rectangular cells,while time is incremented using a con-venient time step.During one simulation time step(STS),a passenger can move only one cell/row,and all cells representing passengers are processed once and in random order.The simulate iterates time steps and update passengers’state and position until all passengers sit down.Call-off FunctionBefore passengers board the plane,they are usually divided by a gate agent into groups,often by consecutive rows,for boarding efÀciency.We develop our call-off function with three steps:1.Divide different seats into groups according to a speciÀc strategy.For exam-ple,in implementing outside-in,we put seats in one column into a group.2.Generate a random order number in each group.3.Queue the groups consecutively.Enplane Simulation FunctionSimulation of the Next Passenger BoardingThe get-on time has an exponential distribution with mean that we estimate to be10STS.Individual Behavior JudgmentsWhat do passengers do in each time step?•Stand still when there is an obstacle.•Move forward by one cell toward the seat when there is free space in front.•Stow luggage.This behavior needs a counter to record its STS because it requires more than one step.•Seat interference when the passenger already seated must stand up and let other passengers move in.It also needs a counter.Simulation Results and AnalysisWe simulate common boarding strategies,including random,back-to-front, rotating-zone,outside-in,and reverse-pyramid[Finney2006].Back-to-front and rotating-zone allow us to choose the number of rows per group;we try4, 6,and8to see how variation affects the strategies.Similarly,reverse-pyramid can also vary in layers,and we choose2,3,and4layers to analyze.Simulation ResultsWe simulate each boarding strategy100times;the results are in Table3.Table3.Simulation results for strategies.Strategy Rows Average Seat Aisle(or layers)interference interferenceRandom247252Rows327255Back-to-front#14257251Back-to-front#26257252Back-to-Front#38257353Rotating#14257253Rotating#26257354Rotating#38257254Outside-in23042Reverse-pyramid#1223043Reverse-pyramid#2323042Reverse-pyramid#3423042 Analysis of the Simulation Results•The more rows in a group,the shorter the boarding time.This is really unexpected!Usually,we think that if we divide the passengers into more groups before boarding in accordance with a boarding strategy,the passen-gers will be better organized and board the plane more efÀciently.But to our surprise,our simulations run in the opposite direction.Take back-to-front as an example.When a group contains8rows,the boarding time is24.6min;but when there are4rows per group,the boarding time increases to25.0min.With the two extremes(i.e.,one row per group vs.all the passengeras a group),the contrast is even more obvious:32min vs.24min.How could this happen?Through analysis of the simulation processes,we Ànd that two or more interferences can happen at the same moment(Figure1) without inÁuencing the boarding process adversely.With more rows in a group,multi-interferences increase but boarding time decreases.•Dividing passenger groups according to their columns such as outside-in way and reverse-pyramid way avoids seat interference and reduces aisle interference.This is easy to understand.If we divide the groups by rows, passengers in the same row get on the plane together,and try to stow their luggage at the same time.However,dividing the group by columns staggers the time when passengers stow luggage into the same overhead bin,which lead to a reduced number of aisle interference.Optimal StrategyBased on the above analysis,we draw the conclusion that dividing pas-senger groups by columns is more efÀcient than by rows.The two optimal strategies are outside-in(23.0min)and reverse-pyramid(22.7min).Although R-P takes a little less time,outside-in is easier to operate both for gate agents and also passengers.Considering this,we choose outside-in as our boarding strategy. Cross-Validation between Theoretical and Simulation Models We compare the results from the simulation with the results of our analytical mode,where we had total boarding time asT=nvÁow+256t sn3+n−12t L.Using parameter value estimates from van den Briel et al.[2003],we have¯t S=256t S=9.7s,t L=7.1s.We also estimate1vÁow=4.5s−1.For the A320,we have n=126,for which we calculate the total boarding time to be23.2min,a value that agrees closely with our simulation time.Mid-size PlanesWe extend our simulation model and boarding strategies to midsize aircraft such as the A300;outside-in takes24.6min,reverse-pyramid takes24.4min.The A300has two aisles in economy class,with most(although not all) rows in a2–4–2seat conÀguration.Correspondingly,we adjust our simulation algorithm.Since there are two aisles but only one boarding gate,we divide the passengers into two lines and assume that they don’t get into the wrong aisle.The two strategies are again comparable in average boarding time;again, considering simplicity,we recommend outside-in.Large PlanesWe extend our simulation model and boarding strategies to large aircraft such as the Airbus A380,with two decks and555seats in three classes.Usually,the A380opens two gates in front of the plane to let passengers board,one of which leads directly to the upper deck(where all business seats are located and a small portion of the economy seats)and the other goes to the main deck(where most economy seats are located).Since seats in the upper deck are more spread out,it takes less time to board than the main deck.So we consider only the boarding process on the main deck, which is similar to that of a midsize plane,with two aisles and most rows with a3–4–3seat conÀguration.Both outside-in and reverse-pyramid take26.8min. We still recommend outside-in.Luggage Distribution Control(LDC)A Creative New Boarding StrategyWe offer a brand-new idea to reduce boarding time.During ticket-check time,the passengers are assigned numbers according to how many pieces of luggage they will take onto the plane.Although we do not completely con-trol the order in which passengers board,we can control the distribution of passengers with different amounts of luggage.A passenger in the last row of the plane blocks nobody when stowing lug-gage;a passenger in the front row blocks all other passengers behind.Let P(r) denote the probability that a passenger in row r blocks other passengers be-hind;P(r)is a decreasing function of r.The expected aisle interference time that this passenger causes ist A:I=P(r)t L,where t l is the time to stow the luggage..As for seat interference,it has no direct connection with the row number.We simply deÀne the average seat interference time as t S:I.So the total expected interference time isT total:I=nr=1(t A:I+t S:I)=nr=1P(r)t L+T S:I,where T S:I= nr=1t S:I is a constant.A passenger with more luggage increases the total.To reduce the effect on interference time,we want to put this passenger as far back as possible. Simulation Results of LDCThrough simulation,we compare outside-in and reverse-pyramid strategies with our LDC strategy.With our LDC strategy,boarding times for all sizes of aircraft can be reduced by2–3min.That is because we send passengers with much luggage to the back of the plane,which reduces the number of interference times.How to Implement LDC?Before passengers board,they exchange their ticket for a boarding card with their seat number.Our strategy is to assign seat numbers according to the amount of carry-on luggage.For the distribution of number of pieces of luggage,we use60%have one piece,30%have two pieces,and10%have three.We divide the seats from back to front in these proportions.We assign to passengers a seat in the group according to number of pieces of luggage;if seats in that group are exhausted,we still follow our basic principle:the more luggage a passenger takes,the farther back the seat.Orderly DeboardingDeboarding StrategiesMost airlines conduct deboarding without any organization.As a result, passengers in the front rows can easily get offÀrst,stalling those behind,much like an inverse back-to-front procedure.This process is still faster than board-ing.However,if we could adopt a strategy like outside-in,that is,let aisle passengers all get their luggage and get off the plane,then the middle passen-gers,andÀnally window passengers,we could fully use the aisle space without interference,leading to higher efÀciency.We put forward the deboarding strategies reversed from boarding strate-gies:random,front-to-back,inside-out,and V(the strategy derived from the reverse-pyramid boarding strategy).Deboarding Simulation ModelWe develop a simulation model to compare deboarding strategies.Differing from the boarding process,deboarding has its own characteristics,as follows:•All passengers start in different positions(“their own seat”)and go to thesame destination(“outside”).•There is no seat interference,since in most cases passengers in the same row will leave from aisle seat to window seat.•In the boarding simulation model,passengers enter the plane one by one, forming a queue.During deboarding,the passengers are a crowd and ev-eryone tries to get out of the planeÀrst.Rush to One Goal:Object PositionDuring deboarding,passengers occupy the aisle.We cannot move the pas-sengers according to a certain order,as in the boarding process,but have toconsider the conÁict that one position is wanted by several passengers.There-fore,we deÀne the concept of object position,the position that a passenger wants to get into in the next time step.Our simulation program allows passengers to move forward by one cell in one time step;it canÀnd out passengers’object po-sitions before moving them,determine which passengers want to move to the same object position,determine which passengers cannot move because of ob-stacles,and then conÀrm which passengers can move forward and which must stay still.If an object position is wanted by several passengers,we randomly choose one to move and the others have to wait.ApplicabilityOur deboarding strategies are to divide passengers into several groups,and then let the groups deboard in order.We deÀne a P AD(Passengers Allowed to Deboard)set,a set of passengers allowed to deboard together. Simulation Results and Analysis for Small Planes We simulate each proposed deboarding strategy100times.Inside-out took 9.9min,V10.25,random12.6,and front-to-back14.0.Compared with random and front-to-back,inside-out is better because it makes full use of the whole aisle,while the other two strategies only partly use the aisle.The main reason that we think the V-strategy is no better is that it needs to have more groups and it doesn’t make full use of the aisle at the beginning and end of deboarding.Is there any better strategy?Can inside-out be improved?During deboard-ing,passengers in the plane can still get their luggage as long as the aisle near their seats is empty.But during boarding,passengers who haven’t boarded can do nothing but wait.Considering this,weÀnd that there is no need to let the next group of passengers wait to deboard until the previous group is completely off the plane.We modify our model by changing it to when propor-tionαof the previous group still remains on board,we allow the next group to start to deboard—our advanced inside-out strategy.WeÀnd thatα=15%to20% yields best results,a deboarding time of about9.4min instead of9.9.There is no need to get an exact optimal value ofα,since it will be almost impossible for theÁight crew to implement an optimal strategy exactly.Deboarding with Luggage Distribution ControlIf the airline is using our LDC boarding strategy,we already know the distribution of luggage.In this case,our simulation program does not need to judge if a passenger has to get luggage and how long it takes.We simulate the inside-out strategy with different values ofαunder the luggage distribution given by our LDC boarding strategy.Again,α=15%to20%gives best results. The deboarding time too is reduced by2–3min;our LDC strategy can reducenot only boarding time but also deboarding time,because we put the passengers who need less time to get their luggage in the front of the plane.(The optimal value ofαis not sensitive to the distribution of luggage.)Results for Midsize and Large PlanesWhen we apply the advanced inside-out strategy in midsize and large planes:•The optimal value ofαincreases to20–30%.The reason for this is possibly the increased number of rows in the deck.Testing of Simulation ModelsAre our simulation results reliable?We apply probability theory.We ran each simulation model100times.The times are independent trials from the same distribution.According to the Central Limit Theorem,the sam-ple mean has approximately a normal distribution.As a result,we can make an interval estimate[Rozanov1969]:T=X±s√ntα/2,n−1,where s is the sample standard deviation and n=100.We choose95%conÀ-dence.WeÀnd for each boarding strategy an interval of±1min,meaning that our simulation results are reliably consistent.Sensitivity AnalysesIn reality,the boarding and deboarding times are inÁuenced by various random events.Will these factors inÁuence our simulation results?•Occupancy level below100%,that is,there will be empty seats.To showhow occupancy affects our simulation result,we resimulate the strategies under occupancies from20%to90%.Result:If occupancy is more than90%, there are no distinguishable changes in results with variation in time step size.If it is below90%,the boarding time will be quite short and therefore affect boarding strategies very little.•Passengers(especially thoseÁying for theÀrst time)may get into the wrong aisle in a midsize or large plane,which has more than one aisle.So we test strategies under a wrong-aisle possibility of5%.Result:The boarding time increases by an average of3min.That is a long time!Proper guidance from the cabin crew is essential on midsize and large planes.•Our boarding strategies can be implemented on all kinds of aircraft,because the outside-in strategy divides passengers by columns,so small variability in seat numbers won’t affect our boarding strategy much.Further DiscussionPassingOur simulation models assume that passengers do not try to pass other passengers in the aisle.But in reality,research indicates that on average,one person in10does this.Boarding StairsWe assume a boarding bridge,but in reality a boarding stairs may be used (e.g.,on the Airbus A380).The difference is that the airport must send a bus to take the passengers from the waiting room to the boarding stairs.Airports want to make full use of the bus and take as many passengers as possible.As a result,boarding in groups according to our strategy is hard to implement.But if the number of passengers in the bus equals the number in each group,we can still adopt our boarding strategy.When they are not equal,we adopt the following boarding strategy:Let R be the number of rows in the deck,with R=pm+q,where m is the half-capacity of the bus,p and q are integers,and q<m.We implement outside-in for pm rows in front;the other passengers are in one group and get on the plane randomly.Disobedient DeboardersSome passengers do not follow directions.We introduce an obedience fac-torβ,the proportion of obedient passengers,picked at random.Disobedient passengers get off the plane if they get the chance,regardless of whether it is their turn.When obedient passengers are less than40%,any strategy is useless.Strengths and WeaknessesStrengths•We develop a simple theoretical model that gives a rough estimate of airplane boarding time,considering the main factors that may cause boarding delay.•We design a new boarding strategy that assigns seats according to amount of luggage,which could save about3min in boarding.•With95%conÀdence,our simulation resultsÁuctuate by only1min.Weaknesses•We don’t consider the weight balance of a ually,the passenger and luggage distribution on the plane should be as uniform as possible.•There are differences in seat conÀguration between our model and some actual planes.ReferencesCAAC Resource.2006.FirstÁight of Airbus A380./ css/carnoc2.Finney,Paul Burnham.2006.Loading an airliner is rocket science.New York Times(14November2006)./2006/11/14/ business/14boarding.html.Funk,M.2003.The visualization of the quantiÀcation of the commodiÀcation of air travel or:WhyÁying makes you feel like a rat in a lab cage.Popular Science263(5)(November2003):67–74.Marelli,Scott,Gregory Mattocks,and Remick Merry.1998.The role of com-puter simulation in reducing airplane turn time.Aero Magazine(4th Quar-ter1998)./commercial/aeromagazine/aero_01/ textonly/t01txt.html.Pan,Matthew.2006.[No title.]/~dbvan1/papers/ MatthewPanEssay.pdf.Rozanov,Y.A.1969.Probability Theory.New York:Dover.van dan Briel,Menkes H.L.,J.Ren´e Villalobos,and Gary L.Hogg.2003.The aircraft boarding problem.Proceedings of the12th Industrial Engineering Re-search Conference(IERC),article number2153.http://www.public.asu.edu/~dbvan1/papers/IERC2003MvandenBriel.pdf.,Tim Lindemann,and Anthony V.Mul´e.2005.America West Airlines develops efÀcient boarding strategies.Interfaces35(3)(May-June2005): 191–200.Van Landeghem,H.2000.A simulation study of passenger boarding times in airplanes./535105.html.。
全国大学生数学建模竞赛A题“特等奖”译文

全国大学生数学建模竞赛A题“特等奖”译文Abstract(摘要)这是一篇关于全国大学生数学建模竞赛A题“特等奖”的译文。
本文通过对该题目的深入分析和理解,将其精确地翻译成英文,以满足相关需要。
1. Introduction(引言)The National College Student Mathematical Modeling Competition (NCSMMC) is held annually in China, aiming to promote the mathematical modeling abilities of college students. The "Special Prize" in the competition represents the highest level of achievement. This article provides a translation of the problem statement for the "Special Prize" question in the NCSMMC A problem.2. Problem Statement(问题描述)Consider a rectangular grid with n rows and m columns. Each cell in the grid can be either black or white. The task is to find the largest possible connected black region in the grid.To represent the grid, we use a two-dimensional array of size n×m, denoted as G[n][m]. The value G[i][j] is 1 if the cell at row i and column j is black, and 0 if it is white.A region is defined as a set of black cells that are connected either horizontally or vertically but not diagonally. Two cells are considered connected if they share a common side.The objective is to determine the size of the largest connected black region in the grid.3. Translation(译文)Consider a rectangular grid with n rows and m columns. Each cell in the grid can be either black or white. The task is to find the largest possible connected black region in the grid.考虑一个有n行m列的矩形网格。
07年全国大学生数学建模一等奖获奖论文
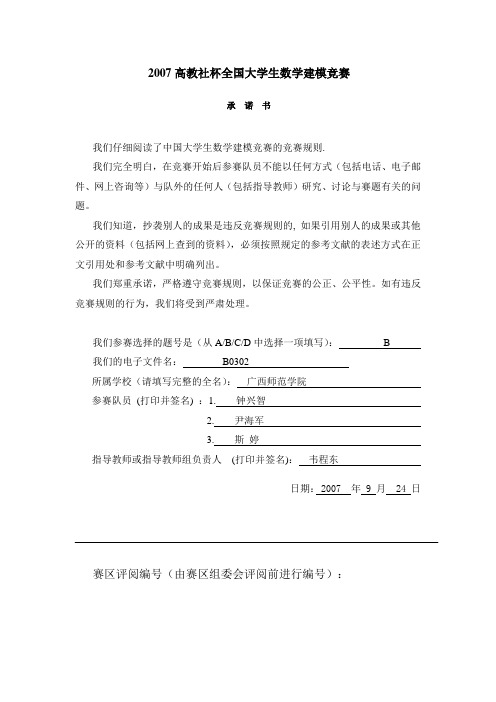
承诺书我们仔细阅读了中国大学生数学建模竞赛的竞赛规则.我们完全明白,在竞赛开始后参赛队员不能以任何方式(包括电话、电子邮件、网上咨询等)与队外的任何人(包括指导教师)研究、讨论与赛题有关的问题。
我们知道,抄袭别人的成果是违反竞赛规则的, 如果引用别人的成果或其他公开的资料(包括网上查到的资料),必须按照规定的参考文献的表述方式在正文引用处和参考文献中明确列出。
我们郑重承诺,严格遵守竞赛规则,以保证竞赛的公正、公平性。
如有违反竞赛规则的行为,我们将受到严肃处理。
我们参赛选择的题号是(从A/B/C/D中选择一项填写): B我们的电子文件名:B0302所属学校(请填写完整的全名):广西师范学院参赛队员(打印并签名) :1. 钟兴智2. 尹海军3. 斯婷指导教师或指导教师组负责人(打印并签名):韦程东日期: 2007 年 9 月 24 日赛区评阅编号(由赛区组委会评阅前进行编号):编号专用页赛区评阅编号(由赛区组委会评阅前进行编号):全国统一编号(由赛区组委会送交全国前编号):全国评阅编号(由全国组委会评阅前进行编号):乘公交,看奥运摘要我们基于最小换乘次数算法,设计了公交查询系统,能够分别从时间和花费出发考虑,选择最优路径,以满足查询者的各种不同需求。
问题一:采用最小换乘次数算法,求出任意两站的最小换乘次数,在次数一定的情况下,分别选取花费最少和时间最少作为优化目标,建立两种模型:最少时间模型:∑∑==+-+⨯=31315)))1(((3),(min i i i i i i i x q x n x B A f ;最少花费模型:))1((),(min '''31i i i y x x B A g -+=∑;利用两种模型求出6组数局的最佳路线如下(两地铁的线路转化成公交的问题,改进问题一中的模型求出此问题的最少时间模型++-+⨯=∑∑∑===)))5)))1(((3((),(min 313131i i i i i i i i i x q x n x y B A f++-+⨯-∑∑∑===)4))))1(((5.2)(1((3131'31i i i i i i i i i x q x n x y ∑=-31i )z 1(7i i y +∑=31i z 6i i y最小换乘算法进行了改进。
- 1、下载文档前请自行甄别文档内容的完整性,平台不提供额外的编辑、内容补充、找答案等附加服务。
- 2、"仅部分预览"的文档,不可在线预览部分如存在完整性等问题,可反馈申请退款(可完整预览的文档不适用该条件!)。
- 3、如文档侵犯您的权益,请联系客服反馈,我们会尽快为您处理(人工客服工作时间:9:00-18:30)。
1.序言定义的国会选区在美国长期以来一直是争论的来源。
由于district-drawers都是由当前的执政者选择,边界被用来将不支持的少数人口和支持的大多数分成一组来影响未来的选举,这一过程称为徇私。
各选区普遍呈现出奇特的形状,以一种随意的方式跨多城市和农村的纤细部门,唯一合法的立法边界的限制规定选区必须含有相同的人群,但各区的构造是完全留给district-drawers。
在英国和加拿大,各地区都更加紧凑和直观的。
他们在缓解徇私上的成功归因于将边界划分任务交给无党派顾问小组。
然而,这些独立的委员会可以采取2-3年才能最终确定一个新的划分方案,要求其有效性问题。
它似乎很清楚,美国应该建立类似的公正委员会,但应做出一些努力,以提高这些群体的效率。
因此,我们的目标是过程中,开发有助于选区重划得一个小的工具箱。
具体来说,我们将创建一个模型,使用简单的几何结构划定合理的边界。
1.1当前模型大多数用于创建选区的方法可分为两类:一是依赖于当前分界线布局(最常用县)和另一是那些不依赖于当前的分界线。
多数属于前一类。
通过使用当前的选区分界,问题归结为通过使用多种数学程序将选区分界以一个理想的方式分组。
特拉等人使用图形分割理论来聚集总人口的变化在2%左右的平均选区规模。
赫斯和韦弗使用一个迭代的过程来定义人口的重心,使用整数规划将各县分组成相等人口的选区,然后重复上述过程,直到的质心达到一个极限。
Garfinkel和Nemhauser的使用迭代矩阵操作搜索的选区组合,是连续和紧凑。
凯撒开始系统地用当前选区和相邻地区进行人口交换。
所有这些方法都使用县为他们的分界,因为它们将国家分割成数量相对较少的部分。
这是必要的,因为当使用更多分界时,大多数的他们使用的数学工具变得缓慢,不精确。
(这就像是说,当国家被划分为更多的连续部门,在极限状态下他们变得不可用。
)因此,使用小的部门,如邮政编码,平均比纽约的一个县小5倍,变得不切实际。
其他类别的方法是不常见的。
出于我们所有的研究论文和文档,只有两个方法不依赖于当前状态分界线。
福雷斯特的方法不断地将国家分成两部分,同时保持人口平等,直到所需数量满足。
硬朗,赎金和拉姆齐创建人口中心的扇形图。
这将创建同质化行政区,它包含了部分大的城市,郊区和人口较少的地区。
这些方法因较小偏见而出名,因为他们唯一的考虑是人口平等和不使用预先存在的分界。
此外,他们是直接应用的。
然而,他们不考虑任何其他可能重要的考虑因素区,如:地理freaures的状态以及他们如何围绕城市。
1.2发展我们的方法由于我们的目标是创造新的方法,增加多种型号可供给一个委员会,我们应该专注有关创建的独立于当前分界的选区分界。
这种方法不仅没有被最充分的开发,同时为什么县是一个很好模型的起点也不是很明显:正如行政区用随意的方式创建,县也是一样,由于县通常不比行政区更小,因此它们也包含偏见。
许多依赖模型的分界,为了保持相等的人群,最终以放松县际分界线结束。
这使得初始假设使用县级分界变得没用,也考虑到徇私,如果这种宽松的方法没有良好的监管。
把国家看成是连续的(即没有预先存在的分界)不会招致任何特定类型的方法。
它给了我们很大的自由,但同时,我们可以处理更多的情况。
如果阿甘和海尔等人方法有任何迹象显示,我们应着眼于保持选区内的城市,引入地域因素。
(需要注意的是,这些条件不必须考虑到,如果我们具体对待这些问题,因为目前的分界,像县一样,很可能是依赖于突出的地理特征。
)目标:通过把国家当作连续地来创建重新规划选区的方法。
我们要求的最后选区含有相同的人口和连续的。
此外,各区应尽可能简单(见§2简单的定义)和最佳考虑到国家重要的地理特征。
2,符号和定义连续的:集合R是连续的,如果它是连接路径。
紧凑:我们想定义紧凑直观。
一种方式是将紧凑看成的有界区域的面积周长的平方之比。
换句话说其中,CR是区域R的致密性,AR是面积, PR是周长,Q是等参商。
我们没有明确的使用这个公式,但当我们评估我们的模型,我们做记着这个想法。
简单:简单的地区是紧凑型和凸。
请注意,此描述了一种相对质量,所以我们可以比较该地区的简单。
Voronoi图:RESP等平面分区在平面上n个节点,如果它们更接近,使得在平面上的点是在同一个区域的没有,没有比任何其他的点到点(详细说明,请参阅§4.1)初始点:一个节点Voronoi图简:一个初始点所代表地区Voronoiesque图:基于平等的集体Voronoi图的变化区域(见§4.2)人口中心:人口密度高地区。
3我们的模型理论评价我们如何分析我们的模型的结果是一个棘手的事情,因为在重新划分选区的文献关键问题上有分歧。
人口平等是最好的定义。
根据法律规定,选区内的人口与各区平均人口的百分之几是相同的。
没有给出具体的百分比,但被认为是5%左右。
创建一个成功的选区重划模型也需要连续性。
依据国家法律规定,各区需要路径明智连接,以至于不能是,选区的一部分是国家的一端,而另一部分在国家另一端,此要求是为了维持地区内的地区和社区。
但是,它并没有限制离岛区包括岛屿,岛上的人口是否低于所需的人口水平。
最后,有一个对地区的愿望,一个字,简单。
这里有一特点没有争议,最常见的术语是紧凑。
泰勒将简单作为衡量紧凑的分歧,并给出了一个有关的地区的边界非自反和自反内角的方程。
Young提供了另七种紧凑措施。
该·勒克测试是地区最大可刻圆的面积和另一个地区的面积的比例。
施瓦茨贝里测试将区域可调的周长和区域相同的面积的圆的周长之比作为区域的面积。
通过比较不同地区布局的瞬时惯性,瞬间惯性测试测量相紧凑。
博伊斯 - 克拉克测试比较区的边界上的点与点之间和质量的中心区的差异,这些差异的偏差为零,是最可取的。
外围的测试通过不同计算每个区总周长来比较不同的选区布局。
最后,还有就是视觉测试。
该测试决定简单地基于直觉。
Young指出:“衡量[紧凑]时,只表示计划比另一个“紧凑。
因此,不仅是有没有共识,如何分析重新分区建议,也难以对它们进行比较。
最后,我们要注意的,上述名单只限制产生的区的形状。
我们没有提及任何其他潜在的相关功能。
例如,它不考虑如何以及人口分布或新建小区边界符合其他的界限,如县或邮政编码。
即使它是个短名单。
图1:矢量Voronoi图与欧氏度量。
注意各区域的紧凑性和简洁性。
明确指出,我们不是在一个位置定义一个严格的定义简单。
然而,我们可以做是识别我们所提出的简单和不简单的地区的特征。
这是符合被限制在1.2秒我们的目标。
因为此列表可以提供到选区划分委员会决定有关这些简单的功能如何。
我们这样做没有明确的定义简单,我们松散评估基于整体简单,连续性,紧凑性,凸性和直观模型的地区。
4方法说明我们的做法在很大程度上取决于使用Voronoi图。
我们以一个定义,其功能,并推动其应用到选区重划。
4.1Voronoi图Voronoi图是一组称为Voronoi多边形,多边形,形成包含在平面内n个不同的点。
每个生成的Pi被包含在一个绘制Voronoi多边形v(Pi),有以下属性:V ( pi )= {q |d( pi,q ) ≤ d( pj,q ), i 6= j }其中,d(X,Y)是从点X到点y的距离。
即,集合中所有这样的q为到pi的距离比任何其他PĴ的点近的集合。
然后由下式给出的关系图(见图1)V = {V ( p1) ,...,V ( pn) },需要注意的是没有我们使用假设的公制。
除了许多可能的选择,我们使用最常见的三种:1 欧式度量: d( p, q)=q( xp − xq)2+(yp − yq)22曼哈顿度量:d( p, q)= |xp − xq| + |yp − yq|3统一度量:d( p, q) = max {| xp − xq|, |yp − yq|}4.1.1 Voronoi 图的实用功能下面是相关属性的摘要:•对于一个给定的发生器点的Voronoi 图是唯一的,并产生多边形的路径连接。
•最近的发电机点到pi 确定V (圆周率)的边缘• Voronoi 多边形的多边形线不相交发电机点。
•当工作在欧氏度量,凹凸有致的所有地区。
这些属性对我们的模型是非常重要的。
第一个属性告诉我们如何选择我们的发起点这方面来产生独特的地区。
这是好当的,考虑到徇私政治。
第二个属性意味着每个地区被限制在周边发起点,而反过来,每个区域是相对紧凑。
Voronoi 图,有效地满足这些三个标准分割区域的两个的功能:连续性和简单。
4.2 Voronoiesque 图我们用它来创建区域的第二种方法是Voronoi 图的直观的结构的调整。
该方法不属于Voronoi 图的定义,但由于是类似于Voronoi 图,我们称之为他们Voronoiesque 图。
一种可视化Voronoi 图的结构是,想象形状(由度量),以恒定的速率从每个发生器点径向向外生长。
在欧氏度量这些形状是圆。
在曼哈顿度量他们的架构。
在统一的度量标准中,它们是正方形。
这些形状的内部形成的区域图。
作为区域相交时,它们形成区域的边界线。
脑中有这么张图,我们定义Voronoiesque 的图是所定义十字路口这些日益增长的形状的边界。
Voronoi 图和Voronoiesque 图的根本区别是,Voronoiesque 图以恒定的速率沿径向向外生长的形状,这和Voronoi 图一样。
他们的径向生长被定义一些相对的实函数的一个子集R2(代表的空间,在其上图是产生)。
见图2。
图2:一个人口密度图来说明相对于日益增长的Voronoiesque 图的进程。
只有三个发起点。
图从左至右代表迭代时间。
所有VI ,VJ 代表不同的地区。
()t i v “的种植方式沿径向从一次迭代到下一个判定所使用的度量值。
Voronoiesque 图有用之处是,在每一次迭代中,为每个区域下的面积的函数f ,他们的生长是可以控制的。
在我们的模型中,我们采取的f 到国家的人口分布。
因此,上述方程是一个人口平等的状态。
此外,当f 是恒定的,地区的增长以恒定的速度,因此产生的Voronoi 图。
最终代价使用Voronoiesque 图确定的位置发起点。
4.3确定发电机 更严格的是,我们定义了一个Voronoi 图的交点的()t i v ,其中 ()t iv 是Voronoiesque 区域,或者仅“区域”,所产生的发起点pi ,在 迭代 t 。
随着每一个迭代,()(1)t t i i v v +⊂ 和 (,)(,)vi vj f x y dA f x y dA =⎰⎰人口密度分布点 现在,我们已经定义了如何生成给定的一组发起点区域。
这里我们考虑如何定义发起点来创建的VoronoiVornoiesque 图。
Voronoi 图的情况下,这是我们唯一的自由度,因为根算符点产生独特的Voronoi 区域。