美国大学生写论文
Essay Paper和Dissertation的有什么区别

方法/步骤
Paper怎么写? 这个词就太多含义了。 好的,也就是paper一般有两种(跟学术相关的): 第一种是超级学术的论文,通常是由专家写的,在书和期刊上等发表,一般叫做学术论文 Scholarly paper。 第二种是类似essay的,作为学校课程作业的文章和论文,一般叫学期论文Term paper。 学期论文Term paper是学生撰写的研究论文,占很大一部分的成绩。学期论文通常用来描述一 个事件,一个概念,或者认为一个论点。学期论文是写原创作品,详细讨论一个话题,通常都需 要几页打印纸,通常是在一个学期尾交。
Essay大家都熟悉,老师经常让我们写essay,essay就是几千字的小论文,一般来说只有文献 综述和对它的分析,没有独立的数据也没关系,本科生接触的最多的就是essay,essay也是被 包括在paper里面的。Paper一般来说就包括了所有的论文,当然,不包括毕业论文。 Dissertation相对来说字数比较多,一般来说要一万字以上,和essay不同的是,它要有独立的 研究方法和数据分析,相对essay来说,它对作者的要求更高,要完成起来也更加困难。
方法/步骤
Essay怎么写? 它几乎是留学中最常见的一个词之一。
Essay有两个特点: 1.short!短! 2.主要是指学生的课程作业(或者考核作品)啦。 3.是在某主题上写,而不是乱来的。 在国外大学,Essay一般指几千字级别的小论文/课程论文,通常只有文献综述和对文献的批判分 析,可以没有独立的数据和实证(即便有也是简化的)。可以没有完整的数据或文献,也可以只 针对一些著作或观点谈谈自己的想法和见解,可以是批判性的,也可以是赞同的。
美国大学生数学建模竞赛优秀论文
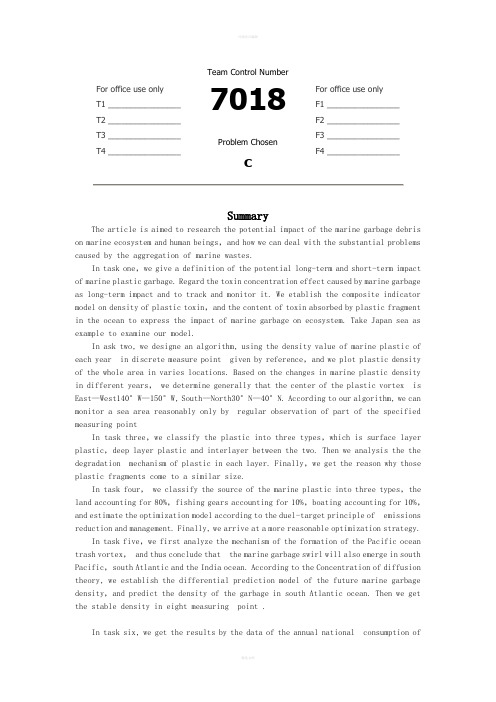
For office use onlyT1________________ T2________________ T3________________ T4________________Team Control Number7018Problem ChosencFor office use onlyF1________________F2________________F3________________F4________________ SummaryThe article is aimed to research the potential impact of the marine garbage debris on marine ecosystem and human beings,and how we can deal with the substantial problems caused by the aggregation of marine wastes.In task one,we give a definition of the potential long-term and short-term impact of marine plastic garbage. Regard the toxin concentration effect caused by marine garbage as long-term impact and to track and monitor it. We etablish the composite indicator model on density of plastic toxin,and the content of toxin absorbed by plastic fragment in the ocean to express the impact of marine garbage on ecosystem. Take Japan sea as example to examine our model.In ask two, we designe an algorithm, using the density value of marine plastic of each year in discrete measure point given by reference,and we plot plastic density of the whole area in varies locations. Based on the changes in marine plastic density in different years, we determine generally that the center of the plastic vortex is East—West140°W—150°W, South—North30°N—40°N. According to our algorithm, we can monitor a sea area reasonably only by regular observation of part of the specified measuring pointIn task three,we classify the plastic into three types,which is surface layer plastic,deep layer plastic and interlayer between the two. Then we analysis the the degradation mechanism of plastic in each layer. Finally,we get the reason why those plastic fragments come to a similar size.In task four, we classify the source of the marine plastic into three types,the land accounting for 80%,fishing gears accounting for 10%,boating accounting for 10%,and estimate the optimization model according to the duel-target principle of emissions reduction and management. Finally, we arrive at a more reasonable optimization strategy.In task five,we first analyze the mechanism of the formation of the Pacific ocean trash vortex, and thus conclude that the marine garbage swirl will also emerge in south Pacific,south Atlantic and the India ocean. According to the Concentration of diffusion theory, we establish the differential prediction model of the future marine garbage density,and predict the density of the garbage in south Atlantic ocean. Then we get the stable density in eight measuring point .In task six, we get the results by the data of the annual national consumption ofpolypropylene plastic packaging and the data fitting method, and predict the environmental benefit generated by the prohibition of polypropylene take-away food packaging in the next decade. By means of this model and our prediction,each nation will reduce releasing 1.31 million tons of plastic garbage in next decade.Finally, we submit a report to expediction leader,summarize our work and make some feasible suggestions to the policy- makers.Task 1:Definition:●Potential short-term effects of the plastic: the hazardeffects will be shown in the short term.●Potential long-term effects of the plastic: thepotential effects, of which hazards are great, willappear after a long time.The short- and long-term effects of the plastic on the ocean environment:In our definition, the short-term and long-term effects of the plastic on the ocean environment are as follows.Short-term effects:1)The plastic is eaten by marine animals or birds.2) Animals are wrapped by plastics, such as fishing nets, which hurt or even kill them.3)Deaden the way of the passing vessels.Long-term effects:1)Enrichment of toxins through the food chain: the waste plastic in the ocean has no natural degradation in theshort-term, which will first be broken down into tinyfragments through the role of light, waves,micro-organisms, while the molecular structure has notchanged. These "plastic sands", easy to be eaten byplankton, fish and other, are Seemingly very similar tomarine life’s food,causing the enrichment and delivery of toxins.2)Accelerate the greenhouse effect: after a long-term accumulation and pollution of plastics, the waterbecame turbid, which will seriously affect the marineplants (such as phytoplankton and algae) inphotosynthesis. A large number of plankton’s deathswould also lower the ability of the ocean to absorbcarbon dioxide, intensifying the greenhouse effect tosome extent.To monitor the impact of plastic rubbish on the marine ecosystem:According to the relevant literature, we know that plastic resin pellets accumulate toxic chemicals , such as PCBs、DDE , and nonylphenols , and may serve as a transport medium and soure of toxins to marine organisms that ingest them[]2. As it is difficult for the plastic garbage in the ocean to complete degradation in the short term, the plastic resin pellets in the water will increase over time and thus absorb more toxins, resulting in the enrichment of toxins and causing serious impact on the marine ecosystem.Therefore, we track the monitoring of the concentration of PCBs, DDE, and nonylphenols containing in the plastic resin pellets in the sea water, as an indicator to compare the extent of pollution in different regions of the sea, thus reflecting the impact of plastic rubbish on ecosystem.To establish pollution index evaluation model: For purposes of comparison, we unify the concentration indexes of PCBs, DDE, and nonylphenols in a comprehensive index.Preparations:1)Data Standardization2)Determination of the index weightBecause Japan has done researches on the contents of PCBs,DDE, and nonylphenols in the plastic resin pellets, we illustrate the survey conducted in Japanese waters by the University of Tokyo between 1997 and 1998.To standardize the concentration indexes of PCBs, DDE,and nonylphenols. We assume Kasai Sesside Park, KeihinCanal, Kugenuma Beach, Shioda Beach in the survey arethe first, second, third, fourth region; PCBs, DDE, andnonylphenols are the first, second, third indicators.Then to establish the standardized model:j j jij ij V V V V V min max min --= (1,2,3,4;1,2,3i j ==)wherej V max is the maximum of the measurement of j indicator in the four regions.j V min is the minimum of the measurement of j indicatorstandardized value of j indicator in i region.According to the literature [2], Japanese observationaldata is shown in Table 1.Table 1. PCBs, DDE, and, nonylphenols Contents in Marine PolypropyleneTable 1 Using the established standardized model to standardize, we have Table 2.In Table 2,the three indicators of Shioda Beach area are all 0, because the contents of PCBs, DDE, and nonylphenols in Polypropylene Plastic Resin Pellets in this area are the least, while 0 only relatively represents the smallest. Similarly, 1 indicates that in some area the value of a indicator is the largest.To determine the index weight of PCBs, DDE, and nonylphenolsWe use Analytic Hierarchy Process (AHP) to determine the weight of the three indicators in the general pollution indicator. AHP is an effective method which transforms semi-qualitative and semi-quantitative problems into quantitative calculation. It uses ideas of analysis and synthesis in decision-making, ideally suited for multi-index comprehensive evaluation.Hierarchy are shown in figure 1.Fig.1 Hierarchy of index factorsThen we determine the weight of each concentrationindicator in the generall pollution indicator, and the process are described as follows:To analyze the role of each concentration indicator, we haveestablished a matrix P to study the relative proportion.⎥⎥⎥⎦⎤⎢⎢⎢⎣⎡=111323123211312P P P P P P P Where mn P represents the relative importance of theconcentration indicators m B and n B . Usually we use 1,2,…,9 and their reciprocals to represent different importance. The greater the number is, the more important it is. Similarly, the relative importance of m B and n B is mn P /1(3,2,1,=n m ).Suppose the maximum eigenvalue of P is m ax λ, then theconsistency index is1max --=n nCI λThe average consistency index is RI , then the consistencyratio isRICI CR = For the matrix P of 3≥n , if 1.0<CR the consistency isthougt to be better, of which eigenvector can be used as the weight vector.We get the comparison matrix accoding to the harmful levelsof PCBs, DDE, and nonylphenols and the requirments ofEPA on the maximum concentration of the three toxins inseawater as follows:⎥⎥⎥⎦⎤⎢⎢⎢⎣⎡=165416131431P We get the maximum eigenvalue of P by MATLAB calculation0012.3max =λand the corresponding eigenvector of it is()2393.02975.09243.0,,=W1.0042.012.1047.0<===RI CI CR Therefore,we determine the degree of inconsistency formatrix P within the permissible range. With the eigenvectors of p as weights vector, we get thefinal weight vector by normalization ()1638.02036.06326.0',,=W . Defining the overall target of pollution for the No i oceanis i Q , among other things the standardized value of threeindicators for the No i ocean is ()321,,i i i i V V V V = and the weightvector is 'W ,Then we form the model for the overall target of marine pollution assessment, (3,2,1=i )By the model above, we obtained the Value of the totalpollution index for four regions in Japanese ocean in Table 3T B W Q '=In Table3, the value of the total pollution index is the hightest that means the concentration of toxins in Polypropylene Plastic Resin Pellets is the hightest, whereas the value of the total pollution index in Shioda Beach is the lowest(we point up 0 is only a relative value that’s not in the name of free of plastics pollution)Getting through the assessment method above, we can monitor the concentration of PCBs, DDE and nonylphenols in the plastic debris for the sake of reflecting the influence to ocean ecosystem.The highter the the concentration of toxins,the bigger influence of the marine organism which lead to the inrichment of food chain is more and more dramatic.Above all, the variation of toxins’ concentration simultaneously reflects the distribution and time-varying of marine litter. We can predict the future development of marine litter by regularly monitoring the content of these substances, to provide data for the sea expedition of the detection of marine litter and reference for government departments to make the policies for ocean governance.Task 2:In the North Pacific, the clockwise flow formed a never-ending maelstrom which rotates the plastic garbage. Over the years, the subtropical eddy current in North Pacific gathered together the garbage from the coast or the fleet, entrapped them in the whirlpool, and brought them to the center under the action of the centripetal force, forming an area of 3.43 million square kilometers (more than one-third of Europe) .As time goes by, the garbage in the whirlpool has the trend of increasing year by year in terms of breadth, density, and distribution. In order to clearly describe the variability of the increases over time and space, according to “Count Densities of Plastic Debris from Ocean Surface Samples North Pacific Gyre 1999—2008”, we analyze the data, exclude them with a great dispersion, and retain them with concentrated distribution, while the longitude values of the garbage locations in sampled regions of years serve as the x-coordinate value of a three-dimensional coordinates, latitude values as the y-coordinate value, the Plastic Count per cubic Meter of water of the position as the z-coordinate value. Further, we establish an irregular grid in the yx plane according to obtained data, and draw a grid line through all the data points. Using the inverse distance squared method with a factor, which can not only estimate the Plastic Count per cubic Meter of water of any position, but also calculate the trends of the Plastic Counts per cubic Meter of water between two original data points, we can obtain the unknown grid points approximately. When the data of all the irregular grid points are known (or approximately known, or obtained from the original data), we can draw the three-dimensional image with the Matlab software, which can fully reflect the variability of the increases in the garbage density over time and space.Preparations:First, to determine the coordinates of each year’s sampled garbage.The distribution range of garbage is about the East - West 120W-170W, South - North 18N-41N shown in the “Count Densities of Plastic Debris from Ocean Surface Samples North Pacific Gyre 1999--2008”, we divide a square in the picture into 100 grids in Figure (1) as follows:According to the position of the grid where the measuring point’s center is, we can identify the latitude and longitude for each point, which respectively serve as the x- and y- coordinate value of the three-dimensional coordinates.To determine the Plastic Count per cubic Meter of water. As the “Plastic Count per cubic Meter of water” provided by “Count Densities of P lastic Debris from Ocean Surface Samples North Pacific Gyre 1999--2008”are 5 density interval, to identify the exact values of the garbage density of one year’s different measuring points, we assume that the density is a random variable which obeys uniform distribution in each interval.Uniform distribution can be described as below:()⎪⎩⎪⎨⎧-=01a b x f ()others b a x ,∈We use the uniform function in Matlab to generatecontinuous uniformly distributed random numbers in each interval, which approximately serve as the exact values of the garbage density andz-coordinate values of the three-dimensional coordinates of the year’s measuring points.Assumptions(1)The data we get is accurate and reasonable.(2)Plastic Count per cubic Meter of waterIn the oceanarea isa continuous change.(3)Density of the plastic in the gyre is a variable by region.Density of the plastic in the gyre and its surrounding area is interdependent , However, this dependence decreases with increasing distance . For our discussion issue, Each data point influences the point of each unknown around and the point of each unknown around is influenced by a given data point. The nearer a given data point from the unknown point, the larger the role.Establishing the modelFor the method described by the previous,we serve the distributions of garbage density in the “Count Pensities of Plastic Debris from Ocean Surface Samples North Pacific Gyre 1999--2008”as coordinates ()z y,, As Table 1:x,Through analysis and comparison, We excluded a number of data which has very large dispersion and retained the data that is under the more concentrated the distribution which, can be seen on Table 2.In this way, this is conducive for us to get more accurate density distribution map.Then we have a segmentation that is according to the arrangement of the composition of X direction and Y direction from small to large by using x co-ordinate value and y co-ordinate value of known data points n, in order to form a non-equidistant Segmentation which has n nodes. For the Segmentation we get above,we only know the density of the plastic known n nodes, therefore, we must find other density of the plastic garbage of n nodes.We only do the sampling survey of garbage density of the north pacificvortex,so only understand logically each known data point has a certain extent effect on the unknown node and the close-known points of density of the plastic garbage has high-impact than distant known point.In this respect,we use the weighted average format, that means using the adverse which with distance squared to express more important effects in close known points. There're two known points Q1 and Q2 in a line ,that is to say we have already known the plastic litter density in Q1 and Q2, then speculate the plastic litter density's affects between Q1、Q2 and the point G which in the connection of Q1 and Q2. It can be shown by a weighted average algorithm22212221111121GQ GQ GQ Z GQ Z Z Q Q G +*+*=in this formula GQ expresses the distance between the pointG and Q.We know that only use a weighted average close to the unknown point can not reflect the trend of the known points, we assume that any two given point of plastic garbage between the changes in the density of plastic impact the plastic garbage density of the unknown point and reflecting the density of plastic garbage changes in linear trend. So in the weighted average formula what is in order to presume an unknown point of plastic garbage density, we introduce the trend items. And because the greater impact at close range point, and thus the density of plastic wastes trends close points stronger. For the one-dimensional case, the calculation formula G Z in the previous example modify in the following format:2212122212212122211111112121Q Q GQ GQ GQ Q Q GQ Z GQ Z GQ Z Z Q Q Q Q G ++++*+*+*=Among them, 21Q Q known as the separation distance of the known point, 21Q Q Z is the density of plastic garbage which is the plastic waste density of 1Q and 2Q for the linear trend of point G . For the two-dimensional area, point G is not on the line 21Q Q , so we make a vertical from the point G and cross the line connect the point 1Q and 2Q , and get point P , the impact of point P to 1Q and 2Q just like one-dimensional, and the one-dimensional closer of G to P , the distant of G to P become farther, the smaller of the impact, so the weighting factor should also reflect the GP in inversely proportional to a certain way, then we adopt following format:221212222122121222211111112121Q Q GQ GP GQ GQ Q Q GQ GP Z GQ Z GQ Z Z P Q Q Q Q G ++++++*+*+*=Taken together, we speculated following roles:(1) Each known point data are influence the density of plastic garbage of each unknown point in the inversely proportional to the square of the distance;(2) the change of density of plastic garbage between any two known points data, for each unknown point are affected, and the influence to each particular point of their plastic garbage diffuse the straight line along the two known particular point; (3) the change of the density of plastic garbage between any two known data points impact a specific unknown points of the density of plastic litter depends on the three distances: a. the vertical distance to a straight line which is a specific point link to a known point;b. the distance between the latest known point to a specific unknown point;c. the separation distance between two known data points.If we mark 1Q ,2Q ,…,N Q as the location of known data points,G as an unknown node, ijG P is the intersection of the connection of i Q ,j Q and the vertical line from G to i Q ,j Q()G Q Q Z j i ,,is the density trend of i Q ,j Q in the of plasticgarbage points and prescribe ()G Q Q Z j i ,,is the testing point i Q ’ s density of plastic garbage ,so there are calculation formula:()()∑∑∑∑==-==++++*=Ni N ij ji i ijGji i ijG N i Nj j i G Q Q GQ GPQ Q GQ GP G Q Q Z Z 11222222111,,Here we plug each year’s observational data in schedule 1 into our model, and draw the three-dimensional images of the spatial distribution of the marine garbage ’s density with Matlab in Figure (2) as follows:199920002002200520062007-2008(1)It’s observed and analyzed that, from 1999 to 2008, the density of plastic garbage is increasing year by year and significantly in the region of East – West 140W-150W, south - north 30N-40N. Therefore, we can make sure that this region is probably the center of the marine litter whirlpool. Gathering process should be such that the dispersed garbage floating in the ocean move with the ocean currents and gradually close to the whirlpool region. At the beginning, the area close to the vortex will have obviously increasable about plastic litter density, because of this centripetal they keeping move to the center of the vortex ,then with the time accumulates ,the garbage density in the center of the vortex become much bigger and bigger , at last it becomes the Pacific rubbish island we have seen today.It can be seen that through our algorithm, as long as the reference to be able to detect the density in an area which has a number of discrete measuring points,Through tracking these density changes ,we Will be able to value out all the waters of the density measurement through our models to determine,This will reduce the workload of the marine expedition team monitoring marine pollution significantly, and also saving costs .Task 3:The degradation mechanism of marine plasticsWe know that light, mechanical force, heat, oxygen, water, microbes, chemicals, etc. can result in the degradation of plastics . In mechanism ,Factors result in the degradation can be summarized as optical ,biological,and chemical。
2014年美国大学生数学建模MCM-B题O奖论文
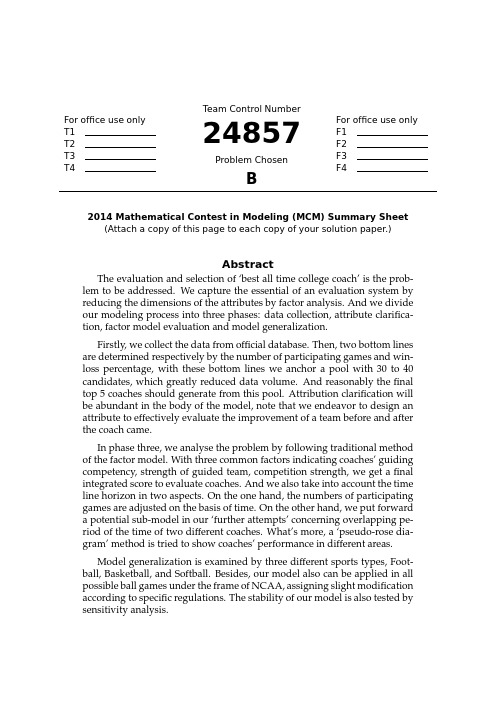
For office use only T1T2T3T4T eam Control Number24857Problem ChosenBFor office use onlyF1F2F3F42014Mathematical Contest in Modeling(MCM)Summary Sheet (Attach a copy of this page to each copy of your solution paper.)AbstractThe evaluation and selection of‘best all time college coach’is the prob-lem to be addressed.We capture the essential of an evaluation system by reducing the dimensions of the attributes by factor analysis.And we divide our modeling process into three phases:data collection,attribute clarifica-tion,factor model evaluation and model generalization.Firstly,we collect the data from official database.Then,two bottom lines are determined respectively by the number of participating games and win-loss percentage,with these bottom lines we anchor a pool with30to40 candidates,which greatly reduced data volume.And reasonably thefinal top5coaches should generate from this pool.Attribution clarification will be abundant in the body of the model,note that we endeavor to design an attribute to effectively evaluate the improvement of a team before and after the coach came.In phase three,we analyse the problem by following traditional method of the factor model.With three common factors indicating coaches’guiding competency,strength of guided team,competition strength,we get afinal integrated score to evaluate coaches.And we also take into account the time line horizon in two aspects.On the one hand,the numbers of participating games are adjusted on the basis of time.On the other hand,we put forward a potential sub-model in our‘further attempts’concerning overlapping pe-riod of the time of two different coaches.What’s more,a‘pseudo-rose dia-gram’method is tried to show coaches’performance in different areas.Model generalization is examined by three different sports types,Foot-ball,Basketball,and Softball.Besides,our model also can be applied in all possible ball games under the frame of NCAA,assigning slight modification according to specific regulations.The stability of our model is also tested by sensitivity analysis.Who’s who in College Coaching Legends—–A generalized Factor Analysis approach2Contents1Introduction41.1Restatement of the problem (4)1.2NCAA Background and its coaches (4)1.3Previous models (4)2Assumptions5 3Analysis of the Problem5 4Thefirst round of sample selection6 5Attributes for evaluating coaches86Factor analysis model106.1A brief introduction to factor analysis (10)6.2Steps of Factor analysis by SPSS (12)6.3Result of the model (14)7Model generalization15 8Sensitivity analysis189Strength and Weaknesses199.1Strengths (19)9.2Weaknesses (19)10Further attempts20 Appendices22 Appendix A An article for Sports Illustrated221Introduction1.1Restatement of the problemThe‘best all time college coach’is to be selected by Sports Illustrated,a magazine for sports enthusiasts.This is an open-ended problem—-no limitation in method of performance appraisal,gender,or sports types.The following research points should be noted:•whether the time line horizon that we use in our analysis make a difference;•the metrics for assessment are to be articulated;•discuss how the model can be applied in general across both genders and all possible sports;•we need to present our model’s Top5coaches in each of3different sports.1.2NCAA Background and its coachesNational Collegiate Athletic Association(NCAA),an association of1281institution-s,conferences,organizations,and individuals that organizes the athletic programs of many colleges and universities in the United States and Canada.1In our model,only coaches in NCAA are considered and ranked.So,why evaluate the Coaching performance?As the identity of a college football program is shaped by its head coach.Given their impacts,it’s no wonder high profile athletic departments are shelling out millions of dollars per season for the services of coaches.Nick Saban’s2013total pay was$5,395,852and in the same year Coach K earned$7,233,976in total23.Indeed,every athletic director wants to hire the next legendary coach.1.3Previous modelsTraditionally,evaluation in athletics has been based on the single criterion of wins and losses.Years later,in order to reasonably evaluate coaches,many reseachers have implemented the coaching evaluation model.Such as7criteria proposed by Adams:[1] (1)the coach in the profession,(2)knowledge of and practice of medical aspects of coaching,(3)the coach as a person,(4)the coach as an organizer and administrator,(5) knowledge of the sport,(6)public relations,and(7)application of kinesiological and physiological principles.1Wikipedia:/wiki/National_Collegiate_Athletic_ Association#NCAA_sponsored_sports2USAToday:/sports/college/salaries/ncaaf/coach/ 3USAToday:/sports/college/salaries/ncaab/coach/Such models relatively focused more on some subjective and difficult-to-quantify attributes to evaluate coaches,which is quite hard for sports fans to judge coaches. Therefore,we established an objective and quantified model to make a list of‘best all time college coach’.2Assumptions•The sample for our model is restricted within the scale of NCAA sports.That is to say,the coaches we discuss refers to those service for NCAA alone;•We do not take into account the talent born varying from one player to another, in this case,we mean the teams’wins or losses purely associate with the coach;•The difference of games between different Divisions in NCAA is ignored;•Take no account of the errors/amendments of the NCAA game records.3Analysis of the ProblemOur main goal is to build and analyze a mathematical model to choose the‘best all time college coach’for the previous century,i.e.from1913to2013.Objectively,it requires numerous attributes to judge and specify whether a coach is‘the best’,while many of the indicators are deemed hard to quantify.However,to put it in thefirst place, we consider a‘best coach’is,and supposed to be in line with several basic condition-s,which are the prerequisites.Those prerequisites incorporate attributes such as the number of games the coach has participated ever and the win-loss percentage of the total.For instance,under the conditions that either the number of participating games is below100,or the win-loss percentage is less than0.5,we assume this coach cannot be credited as the‘best’,ignoring his/her other facets.Therefore,an attempt was made to screen out the coaches we want,thus to narrow the range in ourfirst stage.At the very beginning,we ignore those whose guiding ses-sions or win-loss percentage is less than a certain level,and then we determine a can-didate pool for‘the best coach’of30-40in scale,according to merely two indicators—-participating games and win-loss percentage.It should be reasonably reliable to draw the top5best coaches from this candidate pool,regardless of any other aspects.One point worth mentioning is that,we take time line horizon as one of the inputs because the number of participating games is changing all the time in the previous century.Hence,it would be unfair to treat this problem by using absolute values, especially for those coaches who lived in the earlier ages when sports were less popular and games were sparse comparatively.4Thefirst round of sample selectionCollege Football is thefirst item in our research.We obtain data concerning all possible coaches since it was initiated,of which the coaches’tenures,participating games and win-loss percentage etc.are included.As a result,we get a sample of2053in scale.Thefirst10candidates’respective information is as below:Table1:Thefirst10candidates’information,here Pct means win-loss percentageCoach From To Years Games Wins Losses Ties PctEli Abbott19021902184400.5Earl Abell19281930328141220.536Earl Able1923192421810620.611 George Adams1890189233634200.944Hobbs Adams1940194632742120.185Steve Addazio20112013337201700.541Alex Agase1964197613135508320.378Phil Ahwesh19491949193600.333Jim Aiken19461950550282200.56Fred Akers19751990161861087530.589 ...........................Firstly,we employ Excel to rule out those who begun their coaching career earlier than1913.Next,considering the impact of time line horizon mentioned in the problem statement,we import our raw data into MATLAB,with an attempt to calculate the coaches’average games every year versus time,as delineated in the Figure1below.Figure1:Diagram of the coaches’average sessions every year versus time It can be drawn from thefigure above,clearly,that the number of each coach’s average games is related with the participating time.With the passing of time and the increasing popularity of sports,coaches’participating games yearly ascends from8to 12or so,that is,the maximum exceed the minimum for50%around.To further refinethe evaluation method,we make the following adjustment for coaches’participating games,and we define it as each coach’s adjusted participating games.Gi =max(G i)G mi×G iWhere•G i is each coach’s participating games;•G im is the average participating games yearly in his/her career;and•max(G i)is the max value in previous century as coaches’average participating games yearlySubsequently,we output the adjusted data,and return it to the Excel table.Obviously,directly using all this data would cause our research a mass,and also the economy of description is hard to achieved.Logically,we propose to employ the following method to narrow the sample range.In general,the most essential attributes to evaluate a coach are his/her guiding ex-perience(which can be shown by participating games)and guiding results(shown by win-loss percentage).Fortunately,these two factors are the ones that can be quantified thus provide feasibility for our modeling.Based on our common sense and select-ed information from sports magazines and associated programs,wefind the winning coaches almost all bear the same characteristics—-at high level in both the partici-pating games and the win-loss percentage.Thus we may arbitrarily enact two bottom line for these two essential attributes,so as to nail down a pool of30to40candidates. Those who do not meet our prerequisites should not be credited as the best in any case.Logically,we expect the model to yield insight into how bottom lines are deter-mined.The matter is,sports types are varying thus the corresponding features are dif-ferent.However,it should be reasonably reliable to the sports fans and commentators’perceptual intuition.Take football as an example,win-loss percentage that exceeds0.75 should be viewed as rather high,and college football coaches of all time who meet this standard are specifically listed in Wikipedia.4Consequently,we are able tofix upon a rational pool of candidate according to those enacted bottom lines and meanwhile, may tender the conditions according to the total scale of the coaches.Still we use Football to further articulate,to determine a pool of candidates for the best coaches,wefirst plot thefigure below to present the distributions of all the coaches.From thefigure2,wefind that once the games number exceeds200or win-loss percentage exceeds0.7,the distribution of the coaches drops significantly.We can thus view this group of coaches as outstanding comparatively,meeting the prerequisites to be the best coaches.4Wikipedia:/wiki/List_of_college_football_coaches_ with_a_.750_winning_percentageFigure2:Hist of the football coaches’number of games versus and average games every year versus games and win-loss percentageHence,we nail down the bottom lines for both the games number and the win-loss percentage,that is,0.7for the former and200for the latter.And these two bottom lines are used as the measure for ourfirst round selection.After round one,merely35 coaches are qualified to remain in the pool of candidates.Since it’s thefirst round sifting,rather than direct and ultimate determination,we hence believe the subjectivity to some extent in the opt of bottom lines will not cloud thefinal results of the best coaches.5Attributes for evaluating coachesThen anchored upon the35candidate selected,we will elaborate our coach evaluation system based on8attributes.In the indicator-select process,we endeavor to examine tradeoffs among the availability for data and difficulty for data quantification.Coaches’pay,for example,though serves as the measure for coaching evaluation,the corre-sponding data are limited.Situations are similar for attributes such as the number of sportsmen the coach ever cultivated for the higher-level tournaments.Ultimately,we determine the8attributes shown in the table below:Further explanation:•Yrs:guiding years of a coach in his/her whole career•G’:Gi =max(G i)G mi×G i see it at last section•Pct:pct=wins+ties/2wins+losses+ties•SRS:a rating that takes into account average point differential and strength of schedule.The rating is denominated in points above/below average,where zeroTable2:symbols and attributessymbol attributeYrs yearsG’adjusted overall gamesPct win-lose percentageP’Adjusted percentage ratioSRS Simple Rating SystemSOS Strength of ScheduleBlp’adjusted Bowls participatedBlw’adjusted Bowls wonis the average.Note that,the bigger for this value,the stronger for the team performance.•SOS:a rating of strength of schedule.The rating is denominated in points above/below average,where zero is the average.Noted that the bigger for this value,the more powerful for the team’s rival,namely the competition is more fierce.Sports-reference provides official statistics for SRS and SOS.5•P’is a new attribute designed in our model.It is the result of Win-loss in the coach’s whole career divided by the average of win-loss percentage(weighted by the number of games in different colleges the coach ever in).We bear in mind that the function of a great coach is not merely manifested in the pure win-loss percentage of the team,it is even more crucial to consider the improvement of the team’s win-loss record with the coach’s participation,or say,the gap between‘af-ter’and‘before’period of this team.(between‘after’and‘before’the dividing line is the day the coach take office)It is because a coach who build a comparative-ly weak team into a much more competitive team would definitely receive more respect and honor from sports fans.To measure and specify this attribute,we col-lect the key official data from sports-reference,which included the independent win-loss percentage for each candidate and each college time when he/she was in the team and,the weighted average of all time win-loss percentage of all the college teams the coach ever in—-regardless of whether the coach is in the team or not.To articulate this attribute,here goes a simple physical example.Ike Armstrong (placedfirst when sorted by alphabetical order),of which the data can be ob-tained from website of sports-reference6.We can easily get the records we need, namely141wins,55losses,15ties,and0.704for win-losses percentage.Fur-ther,specific wins,losses,ties for the team he ever in(Utab college)can also be gained,respectively they are602,419,30,0.587.Consequently,the P’value of Ike Armstrong should be0.704/0.587=1.199,according to our definition.•Bowl games is a special event in thefield of Football games.In North America,a bowl game is one of a number of post-season college football games that are5sports-reference:/cfb/coaches/6sports-reference:/cfb/coaches/ike-armstrong-1.htmlprimarily played by teams from the Division I Football Bowl Subdivision.The times for one coach to eparticipate Bowl games are important indicators to eval-uate a coach.However,noted that the total number of Bowl games held each year is changing from year to year,which should be taken into consideration in the model.Other sports events such as NCAA basketball tournament is also ex-panding.For this reason,it is irrational to use the absolute value of the times for entering the Bowl games (or NCAA basketball tournament etc.)and the times for winning as the evaluation measurement.Whereas the development history and regulations for different sports items vary from one to another (actually the differentiation can be fairly large),we here are incapable to find a generalized method to eliminate this discrepancy ,instead,in-dependent method for each item provide a way out.Due to the time limitation for our research and the need of model generalization,we here only do root extract of blp and blw to debilitate the differentiation,i.e.Blp =√Blp Blw =√Blw For different sports items,we use the same attributes,except Blp’and Blw’,we may change it according to specific sports.For instance,we can use CREG (Number of regular season conference championship won)and FF (Number of NCAA Final Four appearance)to replace Blp and Blw in basketball games.With all the attributes determined,we organized data and show them in the table 3:In addition,before forward analysis there is a need to preprocess the data,owing to the diverse dimensions between these indicators.Methods for data preprocessing are a lot,here we adopt standard score (Z score)method.In statistics,the standard score is the (signed)number of standard deviations an observation or datum is above the mean.Thus,a positive standard score represents a datum above the mean,while a negative standard score represents a datum below the mean.It is a dimensionless quantity obtained by subtracting the population mean from an individual raw score and then dividing the difference by the population standard deviation.7The standard score of a raw score x is:z =x −µσIt is easy to complete this process by statistical software SPSS.6Factor analysis model 6.1A brief introduction to factor analysisFactor analysis is a statistical method used to describe variability among observed,correlated variables in terms of a potentially lower number of unobserved variables called factors.For example,it is possible that variations in four observed variables mainly reflect the variations in two unobserved variables.Factor analysis searches for 7Wikipedia:/wiki/Standard_scoreTable3:summarized data for best college football coaches’candidatesCoach From To Yrs G’Pct Blp’Blw’P’SRS SOS Ike Armstrong19251949252810.70411 1.199 4.15-4.18 Dana Bible19151946313860.7152 1.73 1.0789.88 1.48 Bernie Bierman19251950242780.71110 1.29514.36 6.29 Red Blaik19341958252940.75900 1.28213.57 2.34 Bobby Bowden19702009405230.74 5.74 4.69 1.10314.25 4.62 Frank Broyles19571976202570.7 3.162 1.18813.29 5.59 Bear Bryant19451982385080.78 5.39 3.87 1.1816.77 6.12 Fritz Crisler19301947182080.76811 1.08317.15 6.67 Bob Devaney19571972162080.806 3.16 2.65 1.25513.13 2.28 Dan Devine19551980222800.742 3.16 2.65 1.22613.61 4.69 Gilmour Dobie19161938222370.70900 1.27.66-2.09 Bobby Dodd19451966222960.713 3.613 1.18414.25 6.6 Vince Dooley19641988253250.715 4.47 2.83 1.09714.537.12 Gus Dorais19221942192320.71910 1.2296-3.21 Pat Dye19741992192400.707 3.16 2.65 1.1929.68 1.51 LaVell Edwards19722000293920.716 4.69 2.65 1.2437.66-0.66 Phillip Fulmer19922008172150.743 3.87 2.83 1.08313.42 4.95 Woody Hayes19511978283290.761 3.32 2.24 1.03117.418.09 Frank Kush19581979222710.764 2.65 2.45 1.238.21-2.07 John McKay19601975162070.7493 2.45 1.05817.298.59 Bob Neyland19261952212860.829 2.65 1.41 1.20815.53 3.17 Tom Osborne19731997253340.8365 3.46 1.18119.7 5.49 Ara Parseghian19561974192250.71 2.24 1.73 1.15317.228.86 Joe Paterno19662011465950.749 6.08 4.9 1.08914.01 5.01 Darrell Royal19541976232970.7494 2.83 1.08916.457.09 Nick Saban19902013182390.748 3.74 2.83 1.12313.41 3.86 Bo Schembechler19631989273460.775 4.12 2.24 1.10414.86 3.37 Francis Schmidt19221942212670.70800 1.1928.490.16 Steve Spurrier19872013243160.733 4.363 1.29313.53 4.64 Bob Stoops19992013152070.804 3.74 2.65 1.11716.66 4.74 Jock Sutherland19191938202550.81221 1.37613.88 1.68 Barry Switzer19731988162090.837 3.61 2.83 1.16320.08 6.63 John Vaught19471973253210.745 4.24 3.16 1.33814.7 5.26 Wallace Wade19231950243070.765 2.24 1.41 1.34913.53 3.15 Bud Wilkinson19471963172220.826 2.83 2.45 1.14717.54 4.94 such joint variations in response to unobserved latent variables.The observed vari-ables are modelled as linear combinations of the potential factors,plus‘error’terms. The information gained about the interdependencies between observed variables can be used later to reduce the set of variables in a putationally this technique is equivalent to low rank approximation of the matrix of observed variables.8 Why carry out factor analyses?If we can summarise a multitude of measure-8Wikipedia:/wiki/Factor_analysisments with a smaller number of factors without losing too much information,we have achieved some economy of description,which is one of the goals of scientific investi-gation.It is also possible that factor analysis will allow us to test theories involving variables which are hard to measure directly.Finally,at a more prosaic level,factor analysis can help us establish that sets of questionnaire items(observed variables)are in fact all measuring the same underlying factor(perhaps with varying reliability)and so can be combined to form a more reliable measure of that factor.6.2Steps of Factor analysis by SPSSFirst we import the decided datasets of8attributes into SPSS,and the results can be obtained below after the software processing.[2-3]Figure3:Table of total variance explainedFigure4:Scree PlotThefirst table and scree plot shows the eigenvalues and the amount of variance explained by each successive factor.The remaining5factors have small eigenvalues value.Once the top3factors are extracted,it adds up to84.3%,meaning a great as the explanatory ability for the original information.To reflect the quantitative analysis of the model,we obtain the following factor loading matrix,actually the loadings are in corresponding to the weight(α1,α2 (i)the set ofx i=αi1f1+αi2f2+...+αim f j+εiAnd the relative strength of the common factors and the original attribute can also be manifested.Figure5:Rotated Component MatrixThen,with Rotated Component Matrix above,wefind the common factor F1main-ly expresses four attributes they are:G,Yrs,P,SRS,and logically,we define the com-mon factor generated from those four attributes as the guiding competency of the coach;similarly,the common factor F2mainly expresses two attributes,and they are: Pct and Blp,which can be de defined as the integrated strength of the guided team; while the common factor F3,mainly expresses two attributes:SOS and Blw,which can be summarized into a‘latent attribute’named competition strength.In order to obtain the quantitative relation,we get the following Component Score Coefficient Matrix processed by SPSS.Further,the function of common factors and the original attributes is listed as bel-low:F1=0.300x1+0.312x2+0.023x3+0.256x4+0.251x5+0.060x6−0.035x7−0.053x8F2=−0.107x1−0,054x2+0.572x3+0.103x4+0.081x5+0.280x6+0.372x7+0.142x8 F3=−0.076x1−0,098x2−0.349x3+0.004x4+0.027x5−0.656x6+0.160x7+0.400x8 Finally we calculate out the integrated factor scores,which should be the average score weighted by the corresponding proportion of variance contribution of each com-mon factor in the total variance contribution.And the function set should be:F=0.477F1+0.284F2+0.239F3Figure6:Component Score Coefficient Matrix6.3Result of the modelwe rank all the coaches in the candidate pool by integrated score represented by F.Seetable4:Table4:Integrated scores for best college football coach(show15data due to the limi-tation of space)Rank coaches F1F2F3Integrated factor1Joe Paterno 3.178-0.3150.421 1.3622Bobby Bowden 2.51-0.2810.502 1.1113Bear Bryant 2.1420.718-0.142 1.0994Tom Osborne0.623 1.969-0.2390.8205Woody Hayes0.140.009 1.6130.4846Barry Switzer-0.705 2.0360.2470.4037Darrell Royal0.0460.161 1.2680.4018Vince Dooley0.361-0.442 1.3730.3749Bo Schembechler0.4810.1430.3040.32910John Vaught0.6060.748-0.870.26511Steve Spurrier0.5180.326-0.5380.18212Bob Stoops-0.718 1.0850.5230.17113Bud Wilkinson-0.718 1.4130.1050.16514Bobby Dodd0.08-0.2080.7390.16215John McKay-0.9620.228 1.870.151Based on this model,we can make a scientific rank list for US college football coach-es,the Top5coaches of our model is Joe Paterno,Bobby Bowden,Bear Bryant,TomOsborne,Woody Hayes.In order to confirm our result,we get a official list of bestcollege football coaches from Bleacherreport99Bleacherreport:/articles/890705-college-football-the-top-50-coTable5:The result of our model in football,the last column is official college basketball ranking from bleacherreportRank Our model Integrated scores bleacherreport1Joe Paterno 1.362Bear Bryant2Bobby Bowden 1.111Knute Rockne3Bear Bryant 1.099Tom Osborne4Tom Osborne0.820Joe Paterno5Woody Hayes0.484Bobby Bowden By comparing thoes two ranking list,wefind that four of our Top5coaches ap-peared in the offical Top5list,which shows that our model is reasonable and effective.7Model generalizationOur coach evaluation system model,of which the feasibility of generalization is sat-isfying,can be accommodated to any possible NCAA sports concourses by assigning slight modification concerning specific regulations.Besides,this method has nothing to do with the coach’s gender,or say,both male and female coaches can be rationally evaluated by this system.And therefore we would like to generalize this model into softball.Further,we take into account the time line horizon,making corresponding adjust-ment for the indicator of number of participating games so as to stipulate that the evaluation measure for1913and2013would be the same.To further generalize the model,first let’s have a test in basketball,of which the data available is adequate enough as football.And the specific steps are as following:1.Obtain data from sports-reference10and rule out the coaches who begun theircoaching career earlier than1913.2.Calculate each coach’s adjusted number of participating games,and adjust theattribute—-FF(Number of NCAA Final Four appearance).3.Determine the bottom lines for thefirst round selection to get a pool of candidatesaccording to the coaches’participating games and win-loss percentage,and the ideal volumn of the pool should be from30to40.Hist diagrams are as below: We determine800as the bottom line for the adjusted participating games and0.7 for the win-loss percentage.Coincidently,we get a candidate pool of35in scale.4.Next,we collect the corresponding data of candidate coaches(P’,SRS,SOS etc.),as presented in the table6:5.Processed by z score method and factor analysis based on the8attributes anddata above,we get three common factors andfinal integrated scores.And among 10sports-reference:/cbb/coaches/Figure7:Hist of the basketball coaches’number of games versus and average gamesevery year versus games and win-loss percentagethe top5candidates,Mike Krzyzewski,Adolph Rupp,Dean SmithˇcˇnBob Knightare the same with the official statistics from bleacherreport.11We can say theeffectiveness of the model is pretty good.See table5.We also apply similar approach into college softball.Maybe it is because the popularity of the softball is not that high,the data avail-able is not adequate to employ ourfirst model.How can our model function in suchsituation?First and foremost,specialized magazines like Sports Illustrated,its com-mentators there would have more internal and confidential databases,which are notexposed publicly.On the one hand,as long as the data is adequate enough,we can saythe original model is completely feasible.While under the situation that there is datadeficit,we can reasonably simplify the model.The derivation of the softball data is NCAA’s official websites,here we only extractdata from All-Division part.12Softball is a comparatively young sports,hence we may arbitrarily neglect the re-stricted condition of‘100years’.Subsequently,because of the data deficit it is hard toadjust the number of participating games.We may as well determine10as the bottomline for participating games and0.74for win-loss percentage,producing a candidatepool of33in scaleAttributed to the inadequacy of the data for attributes,it is not convenient to furtheruse the factor analysis similarly as the assessment model.Therefore,here we employsolely two of the most important attributes to evaluate a coach and they are:partic-ipating games and win-loss percentage in the coach’s whole career.Specifically,wefirst adopt z score to normalize all the data because of the differentiation of various dimensions,and then the integrated score of the coach can be reached by the weighted11bleacherreport:/articles/1341064-10-greatest-coaches-in-ncaa-b 12NCAA softball Coaching Record:/Docs/stats/SB_Records/2012/coaches.pdf。
美本主文书例文
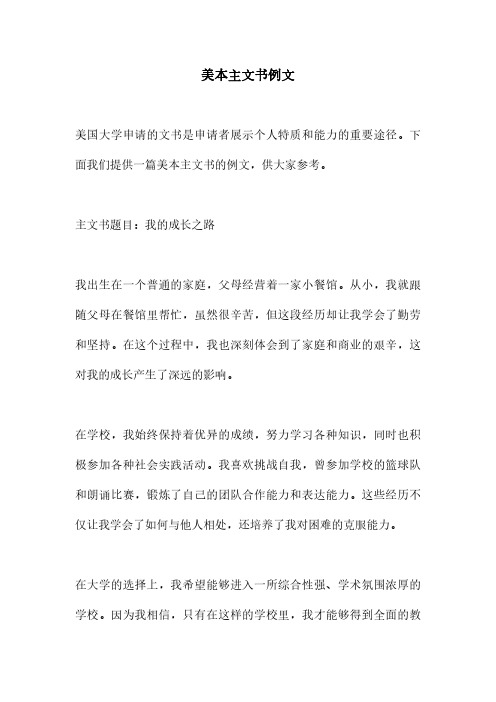
美本主文书例文
美国大学申请的文书是申请者展示个人特质和能力的重要途径。
下面我们提供一篇美本主文书的例文,供大家参考。
主文书题目:我的成长之路
我出生在一个普通的家庭,父母经营着一家小餐馆。
从小,我就跟随父母在餐馆里帮忙,虽然很辛苦,但这段经历却让我学会了勤劳和坚持。
在这个过程中,我也深刻体会到了家庭和商业的艰辛,这对我的成长产生了深远的影响。
在学校,我始终保持着优异的成绩,努力学习各种知识,同时也积极参加各种社会实践活动。
我喜欢挑战自我,曾参加学校的篮球队和朗诵比赛,锻炼了自己的团队合作能力和表达能力。
这些经历不仅让我学会了如何与他人相处,还培养了我对困难的克服能力。
在大学的选择上,我希望能够进入一所综合性强、学术氛围浓厚的学校。
因为我相信,只有在这样的学校里,我才能够得到全面的教
育和培养。
我渴望接受挑战,拓展自己的视野,了解更多不同领域的知识。
未来,我希望能够成为一名成功的商业人士,为社会做出自己的贡献。
在我看来,大学不仅是知识的传授和学业的追求,更是一个人品格的塑造和成长的舞台。
我相信,通过自己的努力和不懈的追求,我一定能够在大学里取得优异的成绩,成为一名受人尊敬的人才。
以上就是我个人的主文书,希望能够得到您的认可。
我相信,在您的学校里,我一定能够找到适合我的发展和成长之路。
谢谢!。
2013美国大学生数学建模竞赛论文
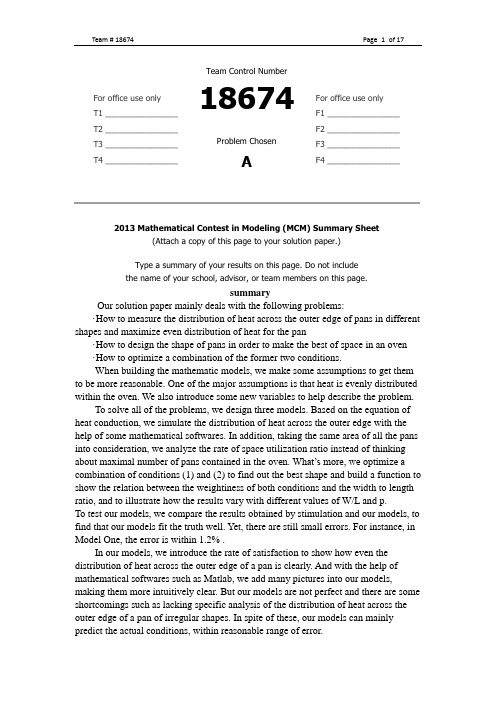
summaryOur solution paper mainly deals with the following problems:·How to measure the distribution of heat across the outer edge of pans in differentshapes and maximize even distribution of heat for the pan·How to design the shape of pans in order to make the best of space in an oven·How to optimize a combination of the former two conditions.When building the mathematic models, we make some assumptions to get themto be more reasonable. One of the major assumptions is that heat is evenly distributedwithin the oven. We also introduce some new variables to help describe the problem.To solve all of the problems, we design three models. Based on the equation ofheat conduction, we simulate the distribution of heat across the outer edge with thehelp of some mathematical softwares. In addition, taking the same area of all the pansinto consideration, we analyze the rate of space utilization ratio instead of thinkingabout maximal number of pans contained in the oven. What’s more, we optimize acombination of conditions (1) and (2) to find out the best shape and build a function toshow the relation between the weightiness of both conditions and the width to lengthratio, and to illustrate how the results vary with different values of W/L and p.To test our models, we compare the results obtained by stimulation and our models, tofind that our models fit the truth well. Yet, there are still small errors. For instance, inModel One, the error is within 1.2% .In our models, we introduce the rate of satisfaction to show how even thedistribution of heat across the outer edge of a pan is clearly. And with the help ofmathematical softwares such as Matlab, we add many pictures into our models,making them more intuitively clear. But our models are not perfect and there are someshortcomings such as lacking specific analysis of the distribution of heat across theouter edge of a pan of irregular shapes. In spite of these, our models can mainlypredict the actual conditions, within reasonable range of error.For office use onlyT1 ________________T2 ________________T3 ________________T4 ________________ Team Control Number18674 Problem Chosen AFor office use only F1 ________________ F2 ________________ F3 ________________ F4 ________________2013 Mathematical Contest in Modeling (MCM) Summary Sheet(Attach a copy of this page to your solution paper.)Type a summary of your results on this page. Do not includethe name of your school, advisor, or team members on this page.The Ultimate Brownie PanAbstractWe introduce three models in the paper in order to find out the best shape for the Brownie Pan, which is beneficial to both heat conduction and space utility.The major assumption is that heat is evenly distributed within the oven. On the basis of this, we introduce three models to solve the problem.The first model deals with heat distribution. After simulative experiments and data processing, we achieve the connection between the outer shape of pans and heat distribution.The second model is mainly on the maximal number of pans contained in an oven. During the course, we use utility rate of space to describe the number. Finally, we find out the functional relation.Having combined both of the conditions, we find an equation relation. Through mathematical operation, we attain the final conclusion.IntroductionHeat usage has always been one of the most challenging issues in modern world. Not only does it has physic significance, but also it can influence each bit of our daily life. Likewise,space utilization, beyond any doubt, also contains its own strategic importance. We build three mathematic models based on underlying theory of thermal conduction and tip thermal effects.The first model describes the process and consequence of heat conduction, thus representing the temperature distribution. Given the condition that regular polygons gets overcooked at the corners, we introduced the concept of tip thermal effects into our prediction scheme. Besides, simulation technique is applied to both models for error correction to predict the final heat distribution.Assumption• Heat is distributed evenly in the oven.Obviously, an oven has its normal operating temperature, which is gradually reached actually. We neglect the distinction of temperature in the oven and the heating process, only to focus on the heat distribution of pans on the basis of their construction.Furthermore, this assumption guarantees the equivalency of the two racks.• Thermal conductivity is temperature-invariant.Thermal conductivity is a physical quantity, symbolizing the capacity of materials. Always, the thermal conductivity of metal material usually varies with different temperatures, in spite of tiny change in value. Simply, we suppose the value to be a constant.• Heat flux of boundaries keeps steady.Heat flux is among the important indexes of heat dispersion. In this transference, we give it a constant value.• Heat conduction dom inates the variation of temperature, while the effects ofheat radiation and heat convection can be neglected.Actually, the course of heat conduction, heat radiation and heat convectiondecide the variation of temperature collectively. Due to the tiny influence of other twofactors, we pay closer attention to heat conduction.• The area of ovens is a constant.I ntroduction of mathematic modelsModel 1: Heat conduction• Introduction of physical quantities:q: heat fluxλ: Thermal conductivityρ: densityc: specific heat capacityt: temperature τ: timeV q : inner heat sourceW q : thermal fluxn: the number of edges of the original polygonsM t : maximum temperaturem t : minimum temperatureΔt: change quantity of temperatureL: side length of regular polygon• Analysis:Firstly, we start with The Fourier Law:2(/)q gradt W m λ=- . (1) According to The Fourier Law, along the direction of heat conduction, positionsof a larger cross-sectional area are lower in temperature. Therefore, corners of panshave higher temperatures.Secondly, let’s analyze the course of heat conduction quantitatively.To achieve this, we need to figure out exact temperatures of each point across theouter edge of a pan and the variation law.Based on the two-dimension differential equation of heat conduction:()()V t t t c q x x y yρλλτ∂∂∂∂∂=++∂∂∂∂∂. (2) Under the assumption that heat distribution is time-independent, we get0t τ∂=∂. (3)And then the heat conduction equation (with no inner heat source)comes to:20t ∇=. (4)under the Neumann boundary condition: |W s q t n λ∂-=∂. (5)Then we get the heat conduction status of regular polygons and circles as follows:Fig 1In consideration of the actual circumstances that temperature is higher at cornersthan on edges, we simulate the temperature distribution in an oven and get resultsabove. Apparently, there is always higher temperature at corners than on edges.Comparatively speaking, temperature is quite more evenly distributed around circles.This can prove the validity of our model rudimentarily.From the figure above, we can get extreme values along edges, which we callM t and m t . Here, we introduce a new physical quantity k , describing the unevennessof heat distribution. For all the figures are the same in area, we suppose the area to be1. Obviously, we have22sin 2sin L n n n ππ= (6) Then we figure out the following results.n t M t m t ∆ L ksquare 4 214.6 203.3 11.3 1.0000 11.30pentagon 5 202.1 195.7 6.4 0.7624 8.395hexagon 6 195.7 191.3 4.4 0.6204 7.092heptagon 7 193.1 190.1 3.0 0.5246 5.719octagon 8 191.1 188.9 2.2 0.4551 4.834nonagon 9 188.9 187.1 1.8 0.4022 4.475decagon 10 189.0 187.4 1.6 0.3605 4.438Table 1It ’s obvious that there is negative correlation between the value of k and thenumber of edges of the original polygons. Therefore, we can use k to describe theunevenness of temperature distribution along the outer edge of a pan. That is to say, thesmaller k is, the more homogeneous the temperature distribution is.• Usability testing:We use regular hendecagon to test the availability of the model.Based on the existing figures, we get a fitting function to analyze the trend of thevalue of k. Again, we introduce a parameter to measure the value of k.Simply, we assume203v k =, (7) so that100v ≤. (8)n k v square 4 11.30 75.33pentagon 5 8.39 55.96hexagon 6 7.09 47.28heptagon 7 5.72 38.12octagon 8 4.83 32.23nonagon9 4.47 29.84 decagon 10 4.44 29.59Table 2Then, we get the functional image with two independent variables v and n.Fig 2According to the functional image above, we get the fitting function0.4631289.024.46n v e -=+.(9) When it comes to hendecagons, n=11. Then, v=26.85.As shown in the figure below, the heat conduction is within our easy access.Fig 3So, we can figure out the following result.vnActually,2026.523tvL∆==.n ∆t L k vhendecagons 11 187.1 185.8 1.3 0.3268 3.978 26.52Table 3Easily , the relative error is 1.24%.So, our model is quite well.• ConclusionHeat distribution varies with the shape of pans. To put it succinctly, heat is more evenly distributed along more edges of a single pan. That is to say, pans with more number of peripheries or more smooth peripheries are beneficial to even distribution of heat. And the difference in temperature contributes to overcooking. Through calculation, the value of k decreases with the increase of edges. With the help of the value of k, we can have a precise prediction of heat contribution.Model 2: The maximum number• Introduction of physical quantities:n: the number of edges of the original polygonsα: utility rate of space• Analysis:Due to the fact that the area of ovens and pans are constant, we can use the area occupied by pans to describe the number of pans. Further, the utility rate of space can be used to describe the number. In the following analysis, we will make use of the utility rate of space to pick out the best shape of pans. We begin with the best permutation devise of regular polygon. Having calculated each utility rate of space, we get the variation tendency.• Model Design:W e begin with the scheme which makes the best of space. Based on this knowledge, we get the following inlay scheme.Fig 4Fig 5According to the schemes, we get each utility rate of space which is showed below.n=4 n=5 n=6 n=7 n=8 n=9 n=10 n=11 shape square pentagon hexagon heptagon octagon nonagon decagon hendecagon utility rate(%)100.00 85.41 100.00 84.22 82.84 80.11 84.25 86.21Table 4Using the ratio above, we get the variation tendency.Fig 6 nutility rate of space• I nstructions:·The interior angle degrees of triangles, squares, and regular hexagon can be divided by 360, so that they all can completely fill a plane. Here, we exclude them in the graph of function.·When n is no more than 9, there is obvious negative correlation between utility rate of space and the value of n. Otherwise, there is positive correlation.·The extremum value of utility rate of space is 90.69%,which is the value for circles.• Usability testing:We pick regular dodecagon for usability testing. Below is the inlay scheme.Fig 7The space utility for dodecagon is 89.88%, which is around the predicted value. So, we’ve got a rather ideal model.• Conclusion:n≥), the When the number of edges of the original polygons is more than 9(9 space utility is gradually increasing. Circles have the extreme value of the space utility. In other words, circles waste the least area. Besides, the rate of increase is in decrease. The situation of regular polygon with many sides tends to be that of circles. In a word, circles have the highest space utility.Model 3: Rounded rectangle• Introduction of physical quantities:A: the area of the rounded rectanglel: the length of the rounded rectangleα: space utilityβ: the width to length ratio• Analysis:Based on the combination of consideration on the highest space utility of quadrangle and the even heat distribution of circles, we invent a model using rounded rectangle device for pans. It can both optimize the cooking effect and minimize the waste of space.However, rounded rectangles are exactly not the same. Firstly, we give our rounded rectangle the same width to length ratio (W/L) as that of the oven, so that least area will be wasted. Secondly, the corner radius can not be neglected as well. It’ll give the distribution of heat across the outer edge a vital influence. In order to get the best pan in shape, we must balance how much the two of the conditions weigh in the scheme.• Model Design:To begin with, we investigate regular rounded rectangle.The area224r ar a A π++= (10) S imilarly , we suppose the value of A to be 1. Then we have a function between a and r :21(4)2a r r π=+--(11) Then, the space utility is()212a r α=+ (12) And, we obtain()2114rαπ=+- (13)N ext, we investigate the relation between k and r, referring to the method in the first model. Such are the simulative result.Fig 8Specific experimental results arer a ∆t L k 0.05 0.90 209.2 199.9 9.3 0.98 9.49 0.10 0.80 203.8 196.4 7.4 0.96 7.70 0.15 0.71 199.6 193.4 6.2 0.95 6.56 0.20 0.62 195.8 190.5 5.3 0.93 5.69 0.25 0.53 193.2 189.1 4.1 0.92 4.46Table 5According to the table above, we get the relation between k and r.Fig 9So, we get the function relation3.66511.190.1013r k e -=+. (14) After this, we continue with the connection between the width to length ratioW Lβ=and heat distribution. We get the following results.krFig 10From the condition of heat distribution, we get the relation between k and βFig 11And the function relation is4.248 2.463k β=+ (15)Now we have to combine the two patterns together:3.6654.248 2.463(11.190.1013)4.248 2.463r k e β-+=++ (16)Finally, we need to take the weightiness (p) into account,(,,)()(,)(1)f r p r p k r p βαβ=⋅+⋅- (17)To standard the assessment level, we take squares as criterion.()(,)(1)(,,)111.30r p k r p f r p αββ⋅⋅-=+ (18) Then, we get the final function3.6652(,,)(1)(0.37590.2180)(1.6670.0151)1(4)r p f r p p e rββπ-=+-⋅+⋅++- (19) So we get()()3.6652224(p 1)(2.259β 1.310)14r p f e r r ππ--∂=-+-+∂⎡⎤+-⎣⎦ (20) Let 0f r∂=∂,we can get the function (,)r p β. Easily,0r p∂<∂ and 0r β∂>∂ (21) So we can come to the conclusion that the value of r decreases with the increase of p. Similarly, the value of r increases with the increase of β.• Conclusion:Model 3 combines all of our former analysis, and gives the final result. According to the weightiness of either of the two conditions, we can confirm the final best shape for a pan.• References:[1] Xingming Qi. Matlab 7.0. Beijing: Posts & Telecom Press, 2009: 27-32[2] Jiancheng Chen, Xinsheng Pang. Statistical data analysis theory and method. Beijing: China's Forestry Press, 2006: 34-67[3] Zhengshen Fan. Mathematical modeling technology. Beijing: China Water Conservancy Press, 2003: 44-54Own It NowYahoo! Ladies and gentlemen, please just have a look at what a pan we have created-the Ultimate Brownie Pan.Can you imagine that just by means of this small invention, you can get away of annoying overcookedchocolate Brownie Cake? Pardon me, I don’t want to surprise you, but I must tell you , our potential customers, that we’ve made it! Believing that it’s nothing more than a common pan, some people may think that it’s not so difficult to create such a pan. To be honest, it’s not just a simple pan as usual, and it takes a lot of work. Now let me show you how great it is. Here we go!Believing that it’s nothing more than a common pan, some people may think that it’s not so difficult to create such a pan. To be honest, it’s not just a simple pan as usual, and it takes a lot of work. Now let me show you how great it is. Here we go!Maybe nobody will deny this: when baked in arectangular pan, cakes get easily overcooked at thecorners (and to a lesser extent at the edges).But neverwill this happen in a round pan. However, round pansare not the best in respects of saving finite space in anoven. How to solve this problem? This is the key pointthat our work focuses on.Up to now, as you know, there have been two factors determining the quality of apan -- the distribution of heat across the outer edge of and thespace occupied in an oven. Unfortunately, they cannot beachieved at the same time. Time calls for a perfect pan, andthen our Ultimate Brownie Pan comes into existence. TheUltimate Brownie Pan has an outstandingadvantage--optimizing a combination of the two conditions. As you can see, it’s so cute. And when you really begin to use it, you’ll find yourself really enjoy being with it. By using this kind of pan, you can use four pans in the meanwhile. That is to say you can bake more cakes at one time.So you can see that our Ultimate Brownie Pan will certainly be able to solve the two big problems disturbing so many people. And so it will! Feel good? So what are you waiting for? Own it now!。
2016年美国大学生数学建模E题英文版论文正稿
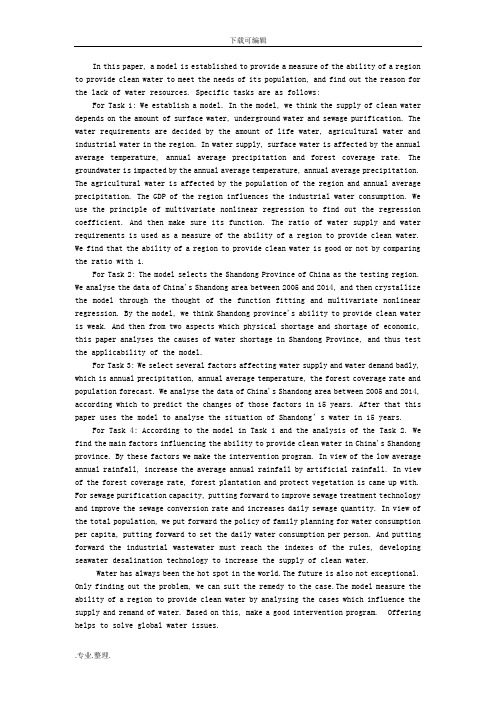
In this paper, a model is established to provide a measure of the ability of a region to provide clean water to meet the needs of its population, and find out the reason for the lack of water resources. Specific tasks are as follows:For Task 1: We establish a model. In the model, we think the supply of clean water depends on the amount of surface water, underground water and sewage purification. The water requirements are decided by the amount of life water, agricultural water and industrial water in the region. In water supply, surface water is affected by the annual average temperature, annual average precipitation and forest coverage rate. The groundwater is impacted by the annual average temperature, annual average precipitation. The agricultural water is affected by the population of the region and annual average precipitation. The GDP of the region influences the industrial water consumption. We use the principle of multivariate nonlinear regression to find out the regression coefficient. And then make sure its function. The ratio of water supply and water requirements is used as a measure of the ability of a region to provide clean water. We find that the ability of a region to provide clean water is good or not by comparing the ratio with 1.For Task 2: The model selects the Shandong Province of China as the testing region. We analyse the data of China's Shandong area between 2005 and 2014, and then crystallize the model through the thought of the function fitting and multivariate nonlinear regression. By the model, we think Shandong province's ability to provide clean water is weak. And then from two aspects which physical shortage and shortage of economic, this paper analyses the causes of water shortage in Shandong Province, and thus test the applicability of the model.For Task 3: We select several factors affecting water supply and water demand badly, which is annual precipitation, annual average temperature, the forest coverage rate and population forecast. We analyse the data of China's Shandong area between 2005 and 2014, according which to predict the changes of those factors in 15 years. After that this paper uses the model to analyse the situation of Shandong’s water in 15 years.For Task 4: According to the model in Task 1 and the analysis of the Task 2. We find the main factors influencing the ability to provide clean water in China's Shandong province. By these factors we make the intervention program. In view of the low average annual rainfall, increase the average annual rainfall by artificial rainfall. In view of the forest coverage rate, forest plantation and protect vegetation is came up with. For sewage purification capacity, putting forward to improve sewage treatment technology and improve the sewage conversion rate and increases daily sewage quantity. In view of the total population, we put forward the policy of family planning for water consumption per capita, putting forward to set the daily water consumption per person. And putting forward the industrial wastewater must reach the indexes of the rules, developing seawater desalination technology to increase the supply of clean water.Water has always been the hot spot in the world.The future is also not exceptional. Only finding out the problem, we can suit the remedy to the case.The model measure the ability of a region to provide clean water by analysing the cases which influence the supply and remand of water. Based on this, make a good intervention program. Offering helps to solve global water issues.1 Introduction (4)1.1 Problem Statement (4)1.2Problem Analysis (4)1.2.1Task 1 Analysis (4)1.2.2Task 2 Analysis (4)1.2.3Task 3 Analysis (5)1.2.4Task 4 Analysis (5)1.2.5Task 4 and 5 Analysis (5)2 Assumptions and Notations (6)2.1 Assumptions (6)2.2 Notations (6)3 Model Establishment and Solution (7)3.1 The effect of single factor on the water supply in a certain area (7)3.1.1Effects of annual average temperature, annual average precipitation andforest coverage on surface water resources in a certain area (7)3.1.2 Effects of annual average temperature and annual precipitation ongroundwater resources in a certain area (8)3.1.3 Influence of total population and per capita water consumption on dailywater consumption in a certain area (8)3.1.4 The influence of average annual rainfall and total population onagricultural water consumption in a certain area (9)3.1.5 Effect of average annual rainfall and population in an area of agriculturalwater use (9)3.2 Function Arrangement (9)3.2.1 Water supply function (9)3.2.2 Water demand function (10)3.2.3 The ability of a region to provide clean water (10)3.3 In order to test the accuracy and usability of the model, this model is selectedas a test area in Shandong Province, China. (10)3.3.1 Total surface water resources (11)3.3.2 Total groundwater resources (14)3.3.3 Total industrial water consumption function (16)3.3.4 Total agricultural water consumption function (17)3.3.5 Assessment of water supply capacity (18)3.4.2 Remediation Measures (19)3.5 Forecast for the next 15 years (20)3.5.1 Forecast of average annual rainfall (20)3.5.2 Prediction of annual temperature (21)3.5.3 Prediction of forest cover (22)3.5.4 Prediction of population (23)3.6 Intervention Program (24)3.6.1 Present ofthe Intervention Program (24)3.6.2 Implement ofthe Intervention Program (25)4 Advantages and Shortcoming of the model (26)4.1Advantages: (26)4.2 Shortcoming (26)5 Improvement of model (26)6 Reference (27)7 Appendices (28)7.1 Data used in task 2 (28)7.2 Matlab Source Code (30)1 Introduction1.1 Problem StatementOn the earth, the water that human beings can use the water directly or indirectly, is an important part of natural resources. At present, the total amount of the earth's water is about billion cubic meters, of which the ocean water is billion cubic meters, accounting for about 96.5% of the total global water. In the remaining water, the surface water accounts for 1.78%, 1.69% of the groundwater. The fresh water that human mainly use of is about billion cubic meters, accounting for only 2.53% in the global total water storage. Few of them is distributed in lakes, rivers, soil and underground water, and most of them are stored in the form of glaciers, permafrost and permafrost, The glacier water storage of about billion cubic meters, accounting for 69% of the world's total water, mostly stored in the Antarctic and Greenland, the available clean water in the dwindlingwith time going by.In order to assess the ability to provide clean water of an area, we set up an assessment model.1.2Problem Analysis1.2.1Task 1 AnalysisTask 1 requires establishing a model to measure the ability of a region to provide clean water. At the same time,we also need to provide a measure standard.This paper make the ratio of water supply and water requirements of a region as the measure standard, by which to measure the ability of a region to provide clean water.A region's main source of water is groundwater, surface water and sewagepurification.The model assumes the volume of groundwater in a region is mainly affected by average annual temperature, annual precipitation;Thevolume of surface water is mainly affected by the average annual temperature, annual precipitation, the forest coverage rate.These factors decide water supply of an area.The waterdemand of an area mainly includes living water, agriculture water and industrial water. We assume living water is affected by the population and per capita consumption decision;Agricultural waterdepends an annual precipitation and population decision;Industrial water is mainly decided by a gross regional product.The above factors decide the water demand in a certain area.1.2.2Task 2 AnalysisAccording to the information provided in the map, in Asia, China's Shandong Province is the region meeting the requirements.Through the data collection of Shandong Province, we can find the annual temperature, annual precipitation, the forest coverage rate, groundwater, surface water, sewage treatment capacity, water, agricultural water, industrial water, population,per capita consumption and GDP data.And then according to the model of Task 1,we analyze the ratio by using multivariate nonlinear regression to make sure that Shandong province is a water-deficient area.After proving that Shandon province is short of water through the model, we analyze the reasons for lack of water from two aspects: physical shortage and economic shortage.1.2.3Task 3 AnalysisBecause we already have the relevant data, we can function to fit the relationship between the variables and the year.Thus it is possible to predict in Shandong Provinc e’s data in the 15 years, then input the data into the model to achieve the purpose of prediction.In addition, it can be combined with the actual situation and the selected areas of the corresponding policy to analysis which factors will have a great change in the15 years.We still can analyze from two aspects of society and the environment.Socialaspects includes the promotion of water conservation, population growth;Environmental aspects includes policy changes to the environment, sewage purification capacity enhancement and so on.1.2.4Task 4 AnalysisFormulating plans for intervention mainly start from the perspective of the main model. According to the content of the model, we can still divide all of factors into two types: the social and environmental factors. The intervention programs can be developed based on two types of factors that affect the supply of water, reducing as much as possible the negative impact of the factors that control factors and intensifying the development of a positive impact. In addition, because Shandong Province is beside the sea, desalination and other measures can be developed to increase clean water supply sources.1.2.5Task 4 and 5 AnalysisTask 4 intervention programs indirectly impact the water supply and demand water through a direct impact on GDP model of forest cover, annual precipitation, annual temperature, water emissions, sewage treatment capacity, population growth and the region.2 Assumptions and Notations2.1 Assumptions●The water resources in a region are derived from the purification of surfacewater, groundwater and sewage, and the demand of water resources comes from domestic water, industrial water and agricultural water.●The surface water supply in a certain area is affected only by the average annualtemperature, annual precipitation and forest coverage. The groundwater supply is affected by the annual average temperature and annual precipitation.●The region's water consumption of a certain region depends on the populationand per capita water consumption; Agricultural water consumption is affected by the average annual precipitation and the numberof people. Industrial water is mainly determined by a regional GDP.● A certain region will not suddenly increase or decrease the population largely.●There will not be a serious natural disasters in a region in the next periodof time.2.2 Notations3 Model Establishment and SolutionThe model established here is a use of a region's water supply and water demand ratio to determine whether the water shortage in the region, the main variables involved.3.1 The effect of single factor on the water supply in a certain area3.1.1Effects of annual average temperature, annual average precipitation and forest coverage on surface water resources in a certain areaDue to the average annual temperature, annual precipitation, the forest coverage rate and surface water of linear or nonlinear relationship exists, so first in order to determine the average annual temperature, annual precipitation, the forest coverage rate and surface water, and then the nonlinear multiple regression analysis method to determine the functional relationship between the three factors and surface water.The surface water content is 1y , Average annual precipitation, annual average temperature and forest coverage rate are 1x ,2x ,3x , Using nonlinear regression statistical methods, the use of MATLAB fitting toolbox were identified 1x ,2x ,3x of the highest regression power(MATLAB fitting toolbox of the highest fitting function is the 9 power, greater than the 9 power function is too complex, not much research value), According to the decision coefficient R 2of the regression equation, the corresponding probability value of the statistic P, the regression coefficients β,0β,1n β,2n β, get the regression equation:35612412312345699999910123123111 (1)n n n n n n n n n n n n n n n y x x x x x x βββββ====++++∑∑∑∑∑∑3.1.2 Effects of annual average temperature and annual precipitation on groundwater resources in a certain areaThere is a linear or nonlinear relationship between the average annual temperature, average annual rainfall and the supply of groundwater, according to the idea of 5.1.1, the relationship between the average annual amount of groundwater supply, the average annual precipitation and the supply of groundwater is calculated. And the regression coefficient is determined, and the function relationship between the average annual temperature, average annual precipitation and the supply of groundwater is based on the regression coefficient:Design of underground water for 2y , with an average annual precipitation, annual average air temperature respectively 1x ,2x , using nonlinear regression statistical methods, according to the regression equation with coefficient of determination R2, F statistic corresponds to the probability value p, to determine the regression coefficients β,0β,1n β,2n β, got the regression equation:312412123499992012121111n n n n n n n n n n y x x x x ββββ=====+++∑∑∑∑ (2)3.1.3 Influence of total population and per capita water consumption on daily water consumption in a certain areaThe total population of a region and the amount of water consumption per capita and the daily use of the product of the relationship between the amount of water = total amount *Water usage per person consumption.Set daily water consumption is 5y , the total population, per capita water consumption were 5x , Q , 5y ,Q ,5x , the function of the relationship between the:55y Qx =(3)3.1.4 The influence of average annual rainfall and total population on agricultural water consumption in a certain areaDue to the annual precipitation and the total population and the area of agriculture of area of a water there is a linear or nonlinear relationship, according to the thought of multivariate nonlinear regression can be calculated average annual precipitation and the total population and the area of agriculture with the function relationship between water and to determine the regression coefficient and regression coefficient write GDP and industrial functional relationship between Gross domestic product GDP and industrial water consumption.Let industrial water consumption of 3y , gross production set 4x , using statistical nonlinear regression, regression equation based on the coefficient of determination 2R , F statistical probability value p corresponding to the amount determined regression coefficients 0β,1n β, the regression equation:11193011n n n y x ββ==+∑(4)3.1.5 Effect of average annual rainfall and population in an area of agricultural water useAgricultural water consumption of 4y , design with an average annual rainfall of 1x , 5x , using statistical nonlinear regression, regression equation based on the coefficient of determination 2R , F statistical probability value p corresponding to the amount determined regression coefficients 0β,1n β, the regression equation:3124121234999940151511n n n n n n n n n n y x x x x ββββ===+++∑∑∑∑(5) 3.2 Function Arrangement3.2.1 Water supply functionThe model takes into account a region's water supply from three aspects: surfacewater resources, groundwater resources and the amount of sewage treatment. The function relation between surface water resources, groundwater resources, sewage treatment and water supply is the function, that is, the amount of water supply = surface water resources + groundwater resources.The amount of water supply isX , and the sewage treatment capacity is *Q , by(2)(1): *12X y y Q =++(6)3.2.2 Water demand functionThe model takes into account the need for a region from three aspects: daily water consumption, industrial water consumption and agricultural water consumption. Daily water consumption, industrial water and agricultural water consumption and water demand is a function of the relationship between the function and the function, that is: water demand = daily water consumption + industrial water + agricultural water consumption.A demand for Y , by (3) (4) (5) to:345Y y y y =++(7)3.2.3 The ability of a region to provide clean waterA region to provide clean water and the area of water supply and water demand about, if water supply is greater than demand, the region provide clean water ability strong; on the contrary, the region provide clean water ability is weak. This model provides that a region to provide clean water capacity by the area of water supply and water demand ratio λ determined by (6) (7) available:(1) 1λ>: the region's ability to provide clean water;(2) 1λ=: the area provides a warning of the ability to provide clean water;(3) 1λ<: the ability of the region to provide clean water is weak;3.3 In order to test the accuracy and usability of the model, this model is selected as a test area in Shandong Province, China.Provide the capacity of water resources in China's Shandong Province, we collected in 2005 to 2014 this decade, Shandong Province, the total water supply, surface water resources amount, quantity of groundwater resources, sewage treatment capacity, agricultural water consumption, industrial water, living water, sewage emissions, forest coverage, total population, per capita water use, annual precipitation, GDP (see Appendix for the specific data).3.3.1 Total surface water resourcesSurface water resources amount 1y , groundwater resources quantity is 2y , industrial water use 3y , agricultural water use for 4y , with an average annual precipitation 1x , with an average annual temperature 2x , the forest coverage rate 3x , GDP for 4x , with a total population of 5x .The factors 1y that is affected by 1x , 2x , 3x , in order to determine the relationship between the 1y , and 1x , 2x , 3x ,first use the data in the appendix table to make 1y with 1x , 2x , 3x scatter plots, such as the figure:Figure 1 Surface water resources and average annual rainfallFigure 2 Surface water resources and annual temperatureFigure 3 Surface water resources and forest coverFigure 1 is obtained by MATLAB fitting curve, the fitting found that 1x and 1y is the 6 power function model (εfor random error).(9)Figure 2 is obtained by MATLAB fitting curve, the fitting found that 2x and 1y isthe 61011n n n y x ββε==++∑8 power function model(10)Figure 3 is obtained by MATLAB fitting curve, the fitting found that 3x and 1y is the 8 power function model(11)Combined with the above analysis, the model (9) (10) (11) established the following regression model(12)Directly using the MATLAB statistics toolbox in the command regress solution, the format is[b,bint,r,rint,stats]=regress(x,y,alpha)Output value of b for the estimation of the regression coefficient β, bint is the confidence intervals for b and r is the residual vector, rint is the confidence interval of r , stats is regression model test statistics, in the first number is a regression equation with coefficient of determination 2R ;The regression coefficients of the model (12) are estimated and their confidence intervals (confidence level α=0.05), test statistic 2R , F , ρ, and the results are shown in table.Table 1 Surface water regression coefficientCan get the regression coefficient from the figure, the estimated value of the regression coefficient into the model (12) forecast equation81021n n n y x ββε==++∑31031n n n y x ββε==++∑33121212312456683999103331231131111n n n n n n n n n n n n n n n y x x x x x x βββββε=======+++++∑∑∑∑∑∑(13)3.3.2 Total groundwater resourcesFactors that affect the 2y include 1x ,2x , in order to determine the relationship between 2y and 1x ,2x , first use the data in the appendix table to make the A3 and A4 and A5 of the scatter diagram, as shown in figure:Figure 4 the amount of groundwater resources Figure 5 the amount of groundwater resources and annual average temperatureand the average annual rainfallFigure 4 is obtained by MATLAB fitting curve, the fitting found that 1x and 2y is the 6 power function model (ε for random error),(14)Figure 5 is obtained by MATLAB fitting curve, the fitting found that 2x and 2y is the 8 power function model.(15)Combined with the above analysis, the model (9) (10) (11) established the following regression model.(16) Directly using the MATLAB statistics toolbox in the command regress solution, the format ^9665444111138273322223724 1.7610 1.0610 1.8910 1.18103.8410 2.610 3.2910 1.0110y x x x x x x x x x --------=+⨯-⨯+⨯+⨯-⨯+⨯-⨯+⨯62011n n n y x ββε==++∑82021n n n y x ββε==++∑121212123468992013121111n n n n n n n n n n y x x x x ββββε=====++++∑∑∑∑is[b,bint,r,rint,stats]=regress(x,y,alpha)Output value of b for the estimation of the regression coefficient β, bint is the confidence intervals for b ,and 2R is the residual vector, rint is the confidence interval of r , stats is regression model test statistics, in the first number is a regression equation with coefficient of determination 2R ;The regression coefficients of the model (12) are estimated and their confidence intervals (confidence level α=0.05), test statistic 2R , F , ρ, and the results are shown in table.Table 2 Regression coefficients of groundwater resourcesCan get the regression coefficient from the figure, the estimated value of the regression coefficient into the model (16) forecast equation(17)Its image is shown in Figure 6^9665445111123821000 1.8210 1.3410 3.37108.49101.3610y x x x x x -----=+⨯-⨯+⨯+⨯-⨯Figure 6 groundwater resources3.3.3 Total industrial water consumption functionFactors that affect 3y is 4x , in order to determine the relationship between 3y and 4x , the first use of the data in the appendix table to make the X and the scatter diagram, as shown in figure:Figure 7 industrial water consumption and GDPFigure 8 industrial water useFigure 7 is obtained by MATLAB fitting curve, the fitting found that 4x and 3y is a function model (εfor random errors),(18)The regression coefficient can be got from the following chartTable 3 Regression coefficient of industrial water consumptionAccording to the above analysis, combined with the model to establish the following regression model, regression coefficient estimation values are substituted into the model (18) to forecast equation.(19)Image as figure 8 3014y x ββε=++^443105.2888410y x -=+⨯3.3.4 Total agricultural water consumption functionFactors that affect the 4y are 1x , 5x , in order to determine the relationship between 4y and 1x , 5x , first using the data in the appendix table to make the 4y and 1x , 5x scatter diagram, as shown in figure:Figure 9 total agricultural water consumption Figure 10 the amount of agricultural water and the average annual rainfalland populationFigure 9 is obtained by MATLAB fitting curve, the fitting found that 4x and 4y is a function model (εfor random errors),(20)Figure 10 is obtained by MATLAB fitting curve, the fitting found that 5x and 4y is a function model (εfor random errors),(21)Combined with the above analysis, the model (20) (21) established the following regression model.(22)Directly using the MATLAB statistics toolbox in the command regress solution, the format is[b,bint,r,rint,stats]=regress(x,y,alpha)Output value of b for the estimation of the regression coefficient β, bint is the confidence intervals for b ,and 2Ris the residual vector, rint is the confidence 3014y x ββε=++74051n n n y x ββε==++∑121212123468994013121111n n n n n n n n n n y x x x x ββββε=====++++∑∑∑∑interval of r , stats is regression model test statistics, in the first number is a regression equation with coefficient of determination 2R ;The regression coefficients of the model (12) are estimated and their confidence intervals (confidence level α=0.05), test statistic 2R , F , ρ, and the results are shown in table.Table 4 regression coefficients of agricultural water useAccording to the above analysis, combined with the model to establish the following regression model, regression coefficient estimation values are substituted into the model (22) to forecast equation.(22)Its image is shown in Figure 11Figure 11 function of agricultural water3.3.5 Assessment of water supply capacityAccording to the data model obtained in 3.2.3, Shandong Province in China, therelevant ^737424155514 1.010 2.110510910 2.56810y x x x x ----=⨯-⨯+⨯-⨯+⨯data and the above function is brought into the model and calculated results:By the conclusion of the model, 1λ< shows that the ability to provide clean water in Shandong province is weak.3.4 Cause Analysis and Treatment Measures Water Shortage.3.4.1 the causes of water shortage in Shandong.(1) Water and soil erosion in hilly areas is serious, and water cannot be brought together into a river(2) Shandong is a temperate monsoon climate. Instability is one of the characters of the monsoon climate. Shandong is located in a part a Plain, and it is short of water. It is a big agricultural province. The water used in industry and agriculture is a lot.(3) Water shortage is the basic situation in the province of Shandong, the contradiction between water supply and demand have become increasingly prominent.(4) Total water resources shortage, average, low mu water resources, less water and more and more people, water resources and population, cultivated land resources serious imbalance, which is the main reason caused by a very prominent contradiction between water supply and demand in Shandong.(5) Have a great relationship with the natural geographical location. Shandong is located at the junction of the north and the south, which is a warm temperate monsoon climate. From the rainfall, the first is the uneven distribution of rainfall during the year.(6) As to rainfall distribution, in the southeast of Shandong Province annual rainfall average is up to 8.5 mm, and northwest region's annual average rainfall is only 550 millimeters, basically showing decreases from the southeast Shandong Province to the northwest of successive trend.(7) East Province is a coastal province, but the sea is not the water for drinking.A lot of rain in the coastal areas is typhoon. The available water in these areas is actually very little.(8) Groundwater levels continue to decline due to over exploitation of underground water in many places. The eastern provinces have formed a number of super mining areas.A series of environmental geological problems, such as groundwater pollution, are formed by the formation of the super mining area.(9)Water must not lack of water in the Yellow River in Shandong province. However, the amount of water in the Yellow River is declining year by year, and the available amount is decreasing.(10) Water conservancy project aging, degradation, water supply reduction3.4.2 Remediation Measures(1)With more rain and floods, water conservation, improvement of water cycle, reserve of groundwater resources, to achieve the use of abundant dry.(2)In strict accordance with the requirements of the state on the implementation of 216.030.741289.69X Y λ===<。
2007美国大学生数学建模竞赛B题特等奖论文

American Airlines' Next Top ModelSara J. BeckSpencer D. K'BurgAlex B. TwistUniversity of Puget SoundTacoma, WAAdvisor: Michael Z. SpiveySummaryWe design a simulation that replicates the behavior of passengers boarding airplanes of different sizes according to procedures currently implemented, as well as a plan not currently in use. Variables in our model are deterministic or stochastic and include walking time, stowage time, and seating time. Boarding delays are measured as the sum of these variables. We physically model and observe common interactions to accurately reflect boarding time.We run 500 simulations for various combinations of airplane sizes and boarding plans. We analyze the sensitivity of each boarding algorithm, as well as the passenger movement algorithm, for a wide range of plane sizes and configurations. We use the simulation results to compare the effectiveness of the boarding plans. We find that for all plane sizes, the novel boarding plan Roller Coaster is the most efficient. The Roller Coaster algorithm essentially modifies the outside-in boarding method. The passengers line up before they board the plane and then board the plane by letter group. This allows most interferences to be avoided. It loads a small plane 67% faster than the next best option, a midsize plane 37% faster than the next best option, and a large plane 35% faster than the next best option.IntroductionThe objectives in our study are:To board (and deboard) various sizes of plane as quickly as possible."* To find a boarding plan that is both efficient (fast) and simple for the passengers.With this in mind:"* We investigate the time for a passenger to stow their luggage and clear the aisle."* We investigate the time for a passenger to clear the aisle when another passenger is seated between them and their seat.* We review the current boarding techniques used by airlines.* We study the floor layout of planes of three different sizes to compare any difference between the efficiency of a given boarding plan as plane size increases and layouts vary."* We construct a simulator that mimics typical passenger behavior during the boarding processes under different techniques."* We realize that there is not very much time savings possible in deboarding while maintaining customer satisfaction."* We calculate the time elapsed for a given plane to load under a given boarding plan by tracking and penalizing the different types of interferences that occur during the simulations."* As an alternative to the boarding techniques currently employed, we suggest an alternative plan andassess it using our simulator."* We make recommendations regarding the algorithms that proved most efficient for small, midsize, and large planes.Interferences and Delays for BoardingThere are two basic causes for interference-someone blocking a passenger,in an aisle and someone blocking a passenger in a row. Aisle interference is caused when the passenger ahead of you has stopped moving and is preventing you from continuing down the aisle towards the row with your seat. Row interference is caused when you have reached the correct row but already-seated passengers between the aisle and your seat are preventing you from immediately taking your seat. A major cause of aisle interference is a passenger experiencing rowinterference.We conducted experiments, using lined-up rows of chairs to simulate rows in an airplane and a team member with outstretched arms to act as an overhead compartment, to estimate parameters for the delays cause by these actions. The times that we found through our experimentation are given in Table 1.We use these times in our simulation to model the speed at which a plane can be boarded. We model separately the delays caused by aisle interference and row interference. Both are simulated using a mixed distribution definedas follows:Y = min{2, X},where X is a normally distributed random variable whose mean and standard deviation are fixed in our experiments. We opt for the distribution being partially normal with a minimum of 2 after reasoning that other alternative and common distributions (such as the exponential) are too prone to throw a small value, which is unrealistic. We find that the average row interference time is approximately 4 s with a standard deviation of 2 s, while the average aisle interference time is approximately 7 s with a standard deviation of 4 s. These values are slightly adjusted based on our team's cumulative experience on airplanes.Typical Plane ConfigurationsEssential to our model are industry standards regarding common layouts of passenger aircraft of varied sizes. We use an Airbus 320 plane to model a small plane (85-210 passengers) and the Boeing 747 for a midsize plane (210-330 passengers). Because of the lack of large planes available on the market, we modify the Boeing 747 by eliminating the first-class section and extending the coach section to fill the entire plane. This puts the Boeing 747 close to its maximum capacity. This modified Boeing 747 has 55 rows, all with the same dimensions as the coach section in the standard Boeing 747. Airbus is in the process of designing planes that can hold up to 800 passengers. The Airbus A380 is a double-decker with occupancy of 555 people in three different classes; but we exclude double-decker models from our simulation because it is the larger, bottom deck that is the limiting factor, not the smaller upper deck.Current Boarding TechniquesWe examine the following industry boarding procedures:* random-order* outside-in* back-to-front (for several group sizes)Additionally, we explore this innovative technique not currently used by airlines:* "Roller Coaster" boarding: Passengers are put in order before they board the plane in a style much like those used by theme parks in filling roller coasters.Passengers are ordered from back of the plane to front, and they board in seatletter groups. This is a modified outside-in technique, the difference being that passengers in the same group are ordered before boarding. Figure 1 shows how this ordering could take place. By doing this, most interferencesare avoided.Current Deboarding TechniquesPlanes are currently deboarded in an aisle-to-window and front-to-back order. This deboarding method comes out of the passengers' desire to be off the plane as quickly as possible. Any modification of this technique could leadto customer dissatisfaction, since passengers may be forced to wait while others seated behind them on theplane are deboarding.Boarding SimulationWe search for the optimal boarding technique by designing a simulation that models the boarding process and running the simulation under different plane configurations and sizes along with different boarding algorithms. We then compare which algorithms yielded the most efficient boarding process.AssumptionsThe environment within a plane during the boarding process is far too unpredictable to be modeled accurately. To make our model more tractable,we make the following simplifying assumptions:"* There is no first-class or special-needs seating. Because the standard industry practice is to board these passengers first, and because they generally make up a small portion of the overall plane capacity, any changes in the overall boarding technique will not apply to these passengers."* All passengers board when their boarding group is called. No passengers arrive late or try to board the plane early."* Passengers do not pass each other in the aisles; the aisles are too narrow."* There are no gaps between boarding groups. Airline staff call a new boarding group before the previous boarding group has finished boarding the plane."* Passengers do not travel in groups. Often, airlines allow passengers boarding with groups, especially with younger children, to board in a manner convenient for them rather than in accordance with the boarding plan. These events are too unpredictable to model precisely."* The plane is full. A full plane would typically cause the most passenger interferences, allowing us to view the worst-case scenario in our model."* Every row contains the same number of seats. In reality, the number of seats in a row varies due to engineering reasons or to accommodate luxury-class passengers.ImplementationWe formulate the boarding process as follows:"* The layout of a plane is represented by a matrix, with the rows representing rows of seats, and each column describing whether a row is next to the window, aisle, etc. The specific dimensions vary with each plane type. Integer parameters track which columns are aisles."* The line of passengers waiting to board is represented by an ordered array of integers that shrinks appropriately as they board the plane."* The boarding technique is modeled in a matrix identical in size to the matrix representing the layout of the plane. This matrix is full of positive integers, one for each passenger, assigned to a specific submatrix, representing each passenger's boarding group location. Within each of these submatrices, seating is assigned randomly torepresent the random order in which passengers line up when their boarding groups are called."* Interferences are counted in every location where they occur within the matrix representing the plane layout. These interferences are then cast into our probability distribution defined above, which gives ameasurement of time delay."* Passengers wait for interferences around them before moving closer to their assigned seats; if an interference is found, the passenger will wait until the time delay has finished counting down to 0."* The simulation ends when all delays caused by interferences have counted down to 0 and all passengers have taken their assigned seats.Strengths and Weaknesses of the ModelStrengths"* It is robust for all plane configurations and sizes. The boarding algorithms that we design can be implemented on a wide variety of planes with minimal effort. Furthermore, the model yields reasonable results as we adjust theparameters of the plane; for example, larger planes require more time to board, while planes with more aisles can load more quickly than similarlysized planes with fewer aisles."* It allows for reasonable amounts of variance in passenger behavior. While with more thorough experimentation a superior stochastic distribution describing the delays associated with interferences could be found, our simulationcan be readily altered to incorporate such advances."* It is simple. We made an effort to minimize the complexity of our simulation, allowing us to run more simulations during a greater time period and mini mizing the risk of exceptions and errors occurring."* It is fairly realistic. Watching the model execute, we can observe passengers boarding the plane, bumping into each other, taking time to load their baggage, and waiting around as passengers in front of them move out of theway. Its ability to incorporate such complex behavior and reduce it are key to completing our objective. Weaknesses"* It does not account for passengers other than economy-class passengers."* It cannot simulate structural differences in the boarding gates which couldpossibly speed up the boarding process. For instance, some airlines in Europeboard planes from two different entrances at once."* It cannot account for people being late to the boarding gate."* It does not account for passenger preferences or satisfaction.Results and Data AnalysisFor each plane layout and boarding algorithm, we ran 500 boarding simulations,calculating mean time and standard deviation. The latter is important because the reliability of plane loading is important for scheduling flights.We simulated the back-to-front method for several possible group sizes.Because of the difference in thenumber of rows in the planes, not all group size possibilities could be implemented on all planes.Small PlaneFor the small plane, Figure 2 shows that all boarding techniques except for the Roller Coaster slowed the boarding process compared to the random boarding process. As more and more structure is added to the boarding process, while passenger seat assignments continue to be random within each of the boarding groups, passenger interference backs up more and more. When passengers board randomly, gaps are created between passengers as some move to the back while others seat themselves immediately upon entering the plane, preventing any more from stepping off of the gate and onto the plane. These gaps prevent passengers who board early and must travel to the back of the plane from causing interference with many passengers behind them. However, when we implement the Roller Coaster algorithm, seat interference is eliminated, with the only passenger causing aisle interference being the very last one to boardfrom each group.Interestingly, the small plane's boarding times for all algorithms are greater than their respective boarding time for the midsize plane! This is because the number of seats per row per aisle is greater in the small plane than in the midsize plane.Midsize PlaneThe results experienced from the simulations of the mid-sized plane areshown in Figure 3 and are comparable to those experienced by the small plane.Again, the Roller Coaster method proved the most effective.Large PlaneFigure 4 shows that the boarding time for a large aircraft, unlike the other plane configurations, drops off when moving from the random boarding algorithm to the outside-in boarding algorithm. Observing the movements by the passengers in the simulation, it is clear that because of the greater number of passengers in this plane, gaps are more likely to form between passengers in the aisles, allowing passengers to move unimpeded by those already on board.However, both instances of back-to-front boarding created too much structure to allow these gaps to form again. Again, because of the elimination of row interference it provides for, Roller Coaster proved to be the most effective boarding method.OverallThe Roller Coaster boarding algorithm is the fastest algorithm for any plane pared to the next fastest boarding procedure, it is 35% faster for a large plane, 37% faster for a midsize plane, and 67% faster for a small plane. The Roller Coaster boarding procedure also has the added benefit of very low standard deviation, thus allowing airlines a more reliable boarding time. The boarding time for the back-to-front algorithms increases with the number of boarding groups and is always slower than a random boarding procedure.The idea behind a back-to-front boarding algorithm is that interference at the front of the plane is avoided until passengers in the back sections are already on the plane. A flaw in this procedure is that having everyone line up in the plane can cause a bottleneck that actually increases the loading time. The outside-in ("Wilma," or window, middle, aisle) algorithm performs better than the random boarding procedure only for the large plane. The benefit of the random procedure is that it evenly distributes interferences throughout theplane, so that they are less likely to impact very many passengers.Validation and Sensitivity AnalysisWe developed a test plane configuration with the sole purpose of implementing our boarding algorithms on planes of all sizes, varying from 24 to 600 passengers with both one or two aisles.We also examined capacities as low as 70%; the trends that we see at full capacity are reflected at these lower capacities. The back-to-front and outside-in algorithms do start to perform better; but this increase inperformance is relatively small, and the Roller Coaster algorithm still substantially outperforms them. Underall circumstances, the algorithms we test are robust. That is, they assign passenger to seats in accordance with the intention of the boarding plans used by airlines and move passengers in a realistic manner.RecommendationsWe recommend that the Roller Coaster boarding plan be implemented for planes of all sizes and configurations for boarding non-luxury-class and nonspecial needs passengers. As planes increase in size, its margin of success in comparison to the next best method decreases; but we are confident that the Roller Coaster method will prove robust. We recommend boarding groups that are traveling together before boarding the rest of the plane, as such groups would cause interferences that slow the boarding. Ideally, such groups would be ordered before boarding.Future WorkIt is inevitable that some passengers will arrive late and not board the plane at their scheduled time. Additionally, we believe that the amount of carry-on baggage permitted would have a larger effect on the boarding time than the specific boarding plan implemented-modeling this would prove insightful.We also recommend modifying the simulation to reflect groups of people traveling (and boarding) together; this is especially important to the Roller Coaster boarding procedure, and why we recommend boarding groups before boarding the rest of the plane.。
2015美国大学生数学建模竞赛一等奖论文

2015 Mathematical Contest in Modeling (MCM) Summary Sheet
Summary
In this paper ,we not only analyze the spread of Ebola, the quantity of the medicine needed, the speed of manufacturing of the vaccine or drug, but also the possible feasible delivery systems and the optimal locations of delivery. Firstly, we analyze the spread of Ebola by using the linear fitting model, and obtain that the trend of development of Ebola increases rapidly before the medicine is used. And then, we build susceptible-infective-removal (SIR) model to predict the trend after the medicine is used, and find that the ratio of patients will decrease. Secondly, we investigate that the quantity of patients equals the quantity of the medicine needed. Via SIR model, the demand of medicine can be calculated and the speed of manufacturing of the vaccine or drug can be gotten by using Calculus (Newton.1671). Thirdly, as for the study of locations of delivery and delivery system, in Guinea, Liberia, and Sierra Leone, we establish the Network graph model and design a kind of arithmetic. Through attaching weights to different points, solving the problem of shortest distance, and taking the optimization mathematical model into consideration, we acquire four optimal locations and the feasible delivery systems on the map. Finally, we consider the other critical factors which may affect the spread of Ebola, such as production capacity, climate, vehicle and terrain, and analyze the extent of every factor. We also analyze the sensitivity of model and give the method that using negative feedback system to improve the accuracy of our models. In addition, we explore our models to apply to other fields such as the H1N1 and the earthquake of Sichuan in China. Via previous analysis, we can predict spread of Ebola and demand of medicine, get the optimal locations. Besides, our model can be applied to many fields.
大学申请essay范文
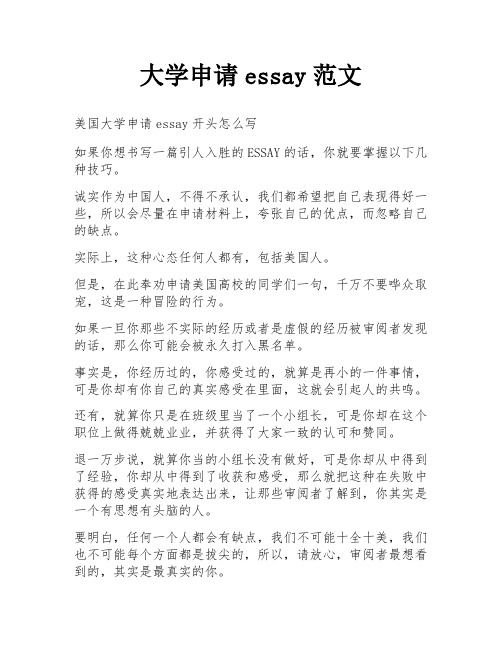
大学申请essay范文美国大学申请essay开头怎么写如果你想书写一篇引人入胜的ESSAY的话,你就要掌握以下几种技巧。
诚实作为中国人,不得不承认,我们都希望把自己表现得好一些,所以会尽量在申请材料上,夸张自己的优点,而忽略自己的缺点。
实际上,这种心态任何人都有,包括美国人。
但是,在此奉劝申请美国高校的同学们一句,千万不要哗众取宠,这是一种冒险的行为。
如果一旦你那些不实际的经历或者是虚假的经历被审阅者发现的话,那么你可能会被永久打入黑名单。
事实是,你经历过的,你感受过的,就算是再小的一件事情,可是你却有你自己的真实感受在里面,这就会引起人的共鸣。
还有,就算你只是在班级里当了一个小组长,可是你却在这个职位上做得兢兢业业,并获得了大家一致的认可和赞同。
退一万步说,就算你当的小组长没有做好,可是你却从中得到了经验,你却从中得到了收获和感受,那么就把这种在失败中获得的感受真实地表达出来,让那些审阅者了解到,你其实是一个有思想有头脑的人。
要明白,任何一个人都会有缺点,我们不可能十全十美,我们也不可能每个方面都是拔尖的,所以,请放心,审阅者最想看到的,其实是最真实的你。
简捷好吧,我们会觉得,天呢,我要在一篇ESSAY里把我所有的优点都要表达出来,我要向审阅者展示一个完美的我,展示一个全面的我,所以我要有大量的材料,大量的文字去描述。
好,不过值得提醒的是,请把这些东西都统统放在你的准备材料之前吧,千万不要出现在你的ESSAY里。
准备的时候,你可以大量准备材料,然后从中选择一个最最有意义,而能引起区鸣的事件来写。
要知道,提交ESSAY的学生并不是只有你一个人,而审阅的老师不能一一逐字逐句地往下念。
所以,在规定的最少字数以上,保持700字是最好的。
这样,也不会给审阅者带来阅读上的压力,也会让你的文章变得简捷而方便阅读。
注意,准确地表达你自己,要运用最精确的字眼。
尤其是事件带来的感受部分或者是领悟部分,一定要写得能深入人心。
美国大学生数学建模大赛英文写作
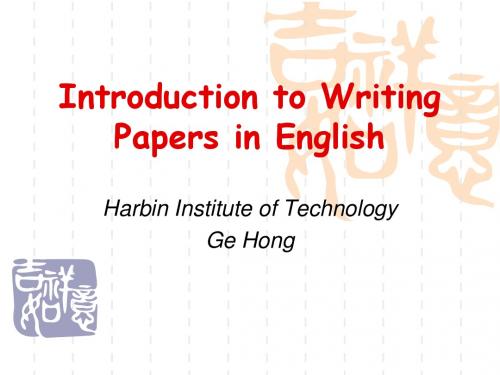
写作要求 : 1. 简短 论文标题一般在10个字内,最多不超 过15个词。
多用复合词
如:self-design, cross-sectional, dust-free, water-proof, input-orientation, piece-wiselinear 利用缩略词 如:e.g., i.e., vs.(与…相对), ibid.(出处相同), etc., cit.(在上述引文中), et al.(等人), viz.(即,就是), DEA (data envelopment analysis), OLS(Ordinary least-squares)
“Investigation on …”, “Observation on …”, “The Method of …”, “Some thought on…”, “A research on…”等冗余套语 。
4. 少用问题性标题 5. 避免名词与动名词混杂使用 如:标题是 “The Treatment of Heating and Eutechticum of Steel” 宜改为 “Heating and Eutechticuming of Steel” 6. 避免使用非标准化的缩略语 论文标题要 求简洁,但一般不使用缩略语 ,更不能使用 非标准化的缩略语 。
关键词(Keywords)
基本功能:顾名思义;便于检索 语言特点:多用名词;字数有限(4-6); 出处明确 写作要求 :论文的关键字一般列在作者与单 位之下,论文摘要之上。也有列在论文摘 要之下的。关键词除第一个字母大写外, 一般不要求大写。关键词间用逗号、分号 或大间隔隔开。最末一个关键词一般不加 用逗号、分号或句号。
美国大学文书题目整理及写作指导申请季巨献Word版
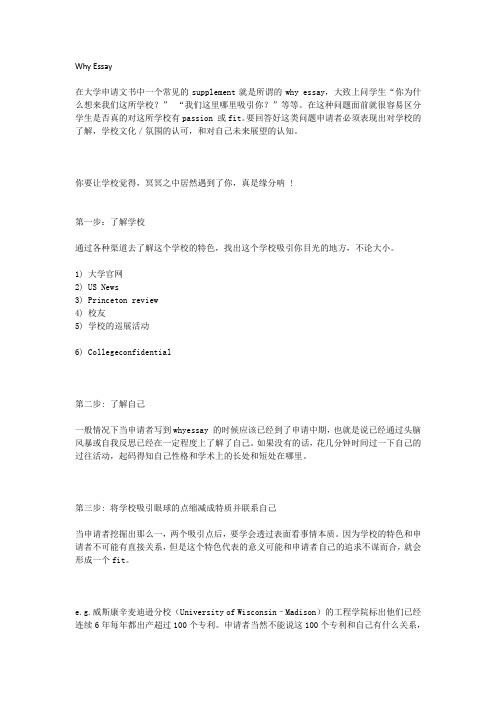
Why Essay在大学申请文书中一个常见的supplement就是所谓的why essay,大致上问学生“你为什么想来我们这所学校?”“我们这里哪里吸引你?”等等。
在这种问题面前就很容易区分学生是否真的对这所学校有passion 或fit。
要回答好这类问题申请者必须表现出对学校的了解,学校文化/氛围的认可,和对自己未来展望的认知。
你要让学校觉得,冥冥之中居然遇到了你,真是缘分呐 !第一步:了解学校通过各种渠道去了解这个学校的特色,找出这个学校吸引你目光的地方,不论大小。
1) 大学官网2) US News3) Princeton review4) 校友5) 学校的巡展活动6) Collegeconfidential第二步: 了解自己一般情况下当申请者写到whyessay 的时候应该已经到了申请中期,也就是说已经通过头脑风暴或自我反思已经在一定程度上了解了自己。
如果没有的话,花几分钟时间过一下自己的过往活动,起码得知自己性格和学术上的长处和短处在哪里。
第三步: 将学校吸引眼球的点缩减成特质并联系自己当申请者挖掘出那么一,两个吸引点后,要学会透过表面看事情本质。
因为学校的特色和申请者不可能有直接关系,但是这个特色代表的意义可能和申请者自己的追求不谋而合,就会形成一个fit。
e.g.威斯康辛麦迪逊分校(University of Wisconsin–Madison)的工程学院标出他们已经连续6年每年都出产超过100个专利。
申请者当然不能说这100个专利和自己有什么关系,大部分的申请者不可能有自己的专利,而且就算有也和威斯康辛的100个专利无关。
但是透过威斯康辛骄傲地标出自己100个专利出产可以总结出什么这个学校特别专注实验和实践。
相信那实验和实践这两个特色是很容易和学生自己产生共鸣的吧。
同时,当申请者已经把本质挖掘到这个地步,一般情况下写出来的答案也不会特别空泛。
所以,在很久前就知道自己的兴趣所在,有目的地开展课外活动,写Essay的时候就倍感轻松呢 !第四步;融合和证明证明不能只靠说的。
2016年美国大学生数学建模大赛A题获奖论文A Hot Bath
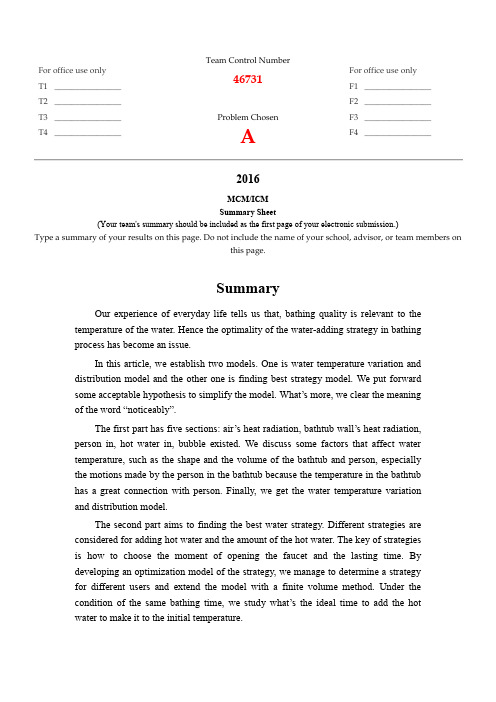
The first part has five sections: air’s heat radiation, bathtub wall’s heat radiation, person in, hot water in, bubble existed. We discuss some factors that affect water temperature, such as the shape and the volume of the bathtub and person, especially the motions made by the person in the bathtub because the temperature in the bathtub has a great connection with person. Finally, we get the water temperature variation and distribution model.
In this article, we establish two models. One is water temperature variation and distr one is finding best strategy model. We put forward some acceptable hypothesis to simplify the model. What’s more, we clear the meaning of the word “noticeably”.
美国学术类论文、科学研究类网站推荐
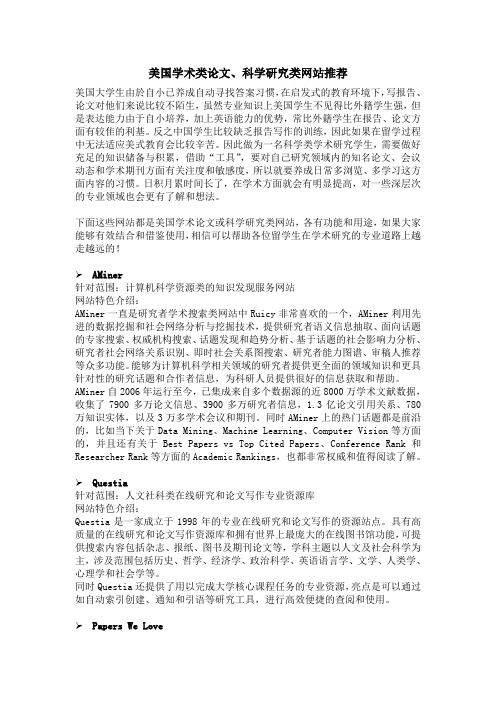
美国学术类论文、科学研究类网站推荐美国大学生由於自小已养成自动寻找答案习惯,在启发式的教育环境下,写报告、论文对他们来说比较不陌生,虽然专业知识上美国学生不见得比外籍学生强,但是表达能力由于自小培养,加上英语能力的优势,常比外籍学生在报告、论文方面有较隹的利基。
反之中国学生比较缺乏报告写作的训练,因此如果在留学过程中无法适应美式教育会比较辛苦。
因此做为一名科学类学术研究学生,需要做好充足的知识储备与积累,借助“工具”,要对自己研究领域内的知名论文、会议动态和学术期刊方面有关注度和敏感度,所以就要养成日常多浏览、多学习这方面内容的习惯。
日积月累时间长了,在学术方面就会有明显提高,对一些深层次的专业领域也会更有了解和想法。
下面这些网站都是美国学术论文或科学研究类网站,各有功能和用途,如果大家能够有效结合和借鉴使用,相信可以帮助各位留学生在学术研究的专业道路上越走越远的!AMiner针对范围:计算机科学资源类的知识发现服务网站网站特色介绍:AMiner一直是研究者学术搜索类网站中Ruicy非常喜欢的一个,AMiner利用先进的数据挖掘和社会网络分析与挖掘技术,提供研究者语义信息抽取、面向话题的专家搜索、权威机构搜索、话题发现和趋势分析、基于话题的社会影响力分析、研究者社会网络关系识别、即时社会关系图搜索、研究者能力图谱、审稿人推荐等众多功能。
能够为计算机科学相关领域的研究者提供更全面的领域知识和更具针对性的研究话题和合作者信息,为科研人员提供很好的信息获取和帮助。
AMiner自2006年运行至今,已集成来自多个数据源的近8000万学术文献数据,收集了7900多万论文信息、3900多万研究者信息,1.3亿论文引用关系、780万知识实体,以及3万多学术会议和期刊。
同时AMiner上的热门话题都是前沿的,比如当下关于Data Mining、Machine Learning、Computer Vision等方面的,并且还有关于Best Papers vs Top Cited Papers、Conference Rank和Researcher Rank等方面的Academic Rankings,也都非常权威和值得阅读了解。
经济学博士生论文写作指南-芝加哥大学

经济资料译丛2013年第2期 经济学博士生论文写作指南①约翰•H•科克伦②, ③芝加哥大学布斯商学院伊利诺伊州芝加哥市伍德朗街区南5807号邮编:60637电话:(773)7023059电子邮件:john.cochrane@/fac/john.cochrane/research/Papers/2013年3月8日① 作者系美国芝加哥大学布斯商学院教授。
作者电子邮箱:john.cochrane@。
(本刊编辑部注:此页为原文单独的标题页,包括作者信息、联系方式、论文完成时间等信息。
考虑到经济学论文一般须有单独的标题页,中文版仍然保留了此页单列。
此外,正文中的黑体系英文原文中的斜体,表示强调。
)本文经作者同意授权本刊于2013年第2期发表。
中文版由厦门大学王亚南经济研究院的袁诚博士研究生翻译,傅十和校对。
英文原文可在此网站下载:/ fac/ john. cochrane/research/Papers/)②一定记得将你自己的联系方式放在论文首页,以便读者能够找到你的论文并给你提出意见!现在已经是21世纪了——建个个人主页吧。
如果论文已经成型,可供教授们阅读,就应该将它放到你的主页上。
论文要注明日期,这样读者一眼便知这是否是最新版本。
③谨对Toby Moskowitz的评论表示感谢。
·69·Journal of Translation from Foreign Literature of Economics1论文结构首先要找出论文的一个核心的、有创意的贡献,用一段话表述这个贡献。
这一段和整个文章一样,都必须具体。
不要写出这样的抽象句子“我分析了企业经理薪酬数据,发现了许多有趣的结果”。
你要详细解释这些主要结论具体是什么。
例如,Fama和French (1992)的摘要是这样开头的:“股票平均收益率往往与市场β、公司规模、杠杆率、账面市值比和收益价格比等多个因素相关,但公司规模和账面市值比这两个容易度量的变量一起就可以解释股票平均收益率的截面差异。
美国大学生数学建模竞赛二等奖论文

美国⼤学⽣数学建模竞赛⼆等奖论⽂The P roblem of R epeater C oordination SummaryThis paper mainly focuses on exploring an optimization scheme to serve all the users in a certain area with the least repeaters.The model is optimized better through changing the power of a repeater and distributing PL tones,frequency pairs /doc/d7df31738e9951e79b8927b4.html ing symmetry principle of Graph Theory and maximum coverage principle,we get the most reasonable scheme.This scheme can help us solve the problem that where we should put the repeaters in general cases.It can be suitable for the problem of irrigation,the location of lights in a square and so on.We construct two mathematical models(a basic model and an improve model)to get the scheme based on the relationship between variables.In the basic model,we set a function model to solve the problem under a condition that assumed.There are two variables:‘p’(standing for the power of the signals that a repeater transmits)and‘µ’(standing for the density of users of the area)in the function model.Assume‘p’fixed in the basic one.And in this situation,we change the function model to a geometric one to solve this problem.Based on the basic model,considering the two variables in the improve model is more reasonable to most situations.Then the conclusion can be drawn through calculation and MATLAB programming.We analysis and discuss what we can do if we build repeaters in mountainous areas further.Finally,we discuss strengths and weaknesses of our models and make necessary recommendations.Key words:repeater maximum coverage density PL tones MATLABContents1.Introduction (3)2.The Description of the Problem (3)2.1What problems we are confronting (3)2.2What we do to solve these problems (3)3.Models (4)3.1Basic model (4)3.1.1Terms,Definitions,and Symbols (4)3.1.2Assumptions (4)3.1.3The Foundation of Model (4)3.1.4Solution and Result (5)3.1.5Analysis of the Result (8)3.1.6Strength and Weakness (8)3.1.7Some Improvement (9)3.2Improve Model (9)3.2.1Extra Symbols (10)Assumptions (10)3.2.2AdditionalAdditionalAssumptions3.2.3The Foundation of Model (10)3.2.4Solution and Result (10)3.2.5Analysis of the Result (13)3.2.6Strength and Weakness (14)4.Conclusions (14)4.1Conclusions of the problem (14)4.2Methods used in our models (14)4.3Application of our models (14)5.Future Work (14)6.References (17)7.Appendix (17)Ⅰ.IntroductionIn order to indicate the origin of the repeater coordination problem,the following background is worth mentioning.With the development of technology and society,communications technology has become much more important,more and more people are involved in this.In order to ensure the quality of the signals of communication,we need to build repeaters which pick up weak signals,amplify them,and retransmit them on a different frequency.But the price of a repeater is very high.And the unnecessary repeaters will cause not only the waste of money and resources,but also the difficulty of maintenance.So there comes a problem that how to reduce the number of unnecessary repeaters in a region.We try to explore an optimized model in this paper.Ⅱ.The Description of the Problem2.1What problems we are confrontingThe signals transmit in the way of line-of-sight as a result of reducing the loss of the energy. As a result of the obstacles they meet and the natural attenuation itself,the signals will become unavailable.So a repeater which just picks up weak signals,amplifies them,and retransmits them on a different frequency is needed.However,repeaters can interfere with one another unless they are far enough apart or transmit on sufficiently separated frequencies.In addition to geographical separation,the“continuous tone-coded squelch system”(CTCSS),sometimes nicknamed“private line”(PL),technology can be used to mitigate interference.This system associates to each repeater a separate PL tone that is transmitted by all users who wish to communicate through that repeater. The PL tone is like a kind of password.Then determine a user according to the so called password and the specific frequency,in other words a user corresponds a PL tone(password)and a specific frequency.Defects in line-of-sight propagation caused by mountainous areas can also influence the radius.2.2What we do to solve these problemsConsidering the problem we are confronting,the spectrum available is145to148MHz,the transmitter frequency in a repeater is either600kHz above or600kHz below the receiver frequency.That is only5users can communicate with others without interferences when there’s noPL.The situation will be much better once we have PL.However the number of users that a repeater can serve is limited.In addition,in a flat area ,the obstacles such as mountains ,buildings don’t need to be taken into account.Taking the natural attenuation itself is reasonable.Now the most important is the radius that the signals transmit.Reducing the radius is a good way once there are more users.With MATLAB and the method of the coverage in Graph Theory,we solve this problem as follows in this paper.Ⅲ.Models3.1Basic model3.1.1Terms,Definitions,and Symbols3.1.2Assumptions●A user corresponds a PLz tone (password)and a specific frequency.●The users in the area are fixed and they are uniform distribution.●The area that a repeater covers is a regular hexagon.The repeater is in the center of the regular hexagon.●In a flat area ,the obstacles such as mountains ,buildings don’t need to be taken into account.We just take the natural attenuation itself into account.●The power of a repeater is fixed.3.1.3The Foundation of ModelAs the number of PLz tones (password)and frequencies is fixed,and a user corresponds a PLz tone (password)and a specific frequency,we can draw the conclusion that a repeater can serve the limited number of users.Thus it is clear that the number of repeaters we need relates to the density symboldescriptionLfsdfminrpµloss of transmission the distance of transmission operating frequency the number of repeaters that we need the power of the signals that a repeater transmits the density of users of the areaof users of the area.The radius of the area that a repeater covers is also related to the ratio of d and the radius of the circular area.And d is related to the power of a repeater.So we get the model of function()min ,r f p µ=If we ignore the density of users,we can get a Geometric model as follows:In a plane which is extended by regular hexagons whose side length are determined,we move a circle until it covers the least regular hexagons.3.1.4Solution and ResultCalculating the relationship between the radius of the circle and the side length of the regular hexagon.[]()()32.4420lg ()20lg Lfs dB d km f MHz =++In the above formula the unit of ’’is .Lfs dB The unit of ’’is .d Km The unit of ‘‘is .f MHz We can conclude that the loss of transmission of radio is decided by operating frequency and the distance of transmission.When or is as times as its former data,will increase f d 2[]Lfs .6dB Then we will solve the problem by using the formula mentioned above.We have already known the operating frequency is to .According to the 145MHz 148MHz actual situation and some authority material ,we assume a system whose transmit power is and receiver sensitivity is .Thus we can conclude that ()1010dBm mW +106.85dBm ?=.Substituting and to the above formula,we can get the Lfs 106.85dBm ?145MHz 148MHz average distance of transmission .()6.4d km =4mile We can learn the radius of the circle is 40mile .So we can conclude the relationship between the circle and the side length of regular hexagon isR=10d.1)The solution of the modelIn order to cover a certain plane with the least regular hexagons,we connect each regular hexagon as the honeycomb.We use A(standing for a figure)covers B(standing for another figure), only when As don’t overlap each other,the number of As we use is the smallest.Figure1According to the Principle of maximum flow of Graph Theory,the better of the symmetry ofthe honeycomb,the bigger area that it covers(Fig1).When the geometric centers of the circle andthe honeycomb which can extend are at one point,extend the honeycomb.Then we can get Fig2,Fig4:Figure2Fig3demos the evenly distribution of users.Figure4Now prove the circle covers the least regular hexagons.Look at Fig5.If we move the circle slightly as the picture,you can see three more regular hexagons are needed.Figure 52)ResultsThe average distance of transmission of the signals that a repeater transmit is 4miles.1000users can be satisfied with 37repeaters founded.3.1.5Analysis of the Result1)The largest number of users that a repeater can serveA user corresponds a PL and a specific frequency.There are 5wave bands and 54different PL tones available.If we call a code include a PL and a specific frequency,there are 54*5=270codes.However each code in two adjacent regular hexagons shouldn’t be the same in case of interfering with each other.In order to have more code available ,we can distribute every3adjacent regular hexagons 90codes each.And that’s the most optimized,because once any of the three regular hexagons have more codes,it will interfere another one in other regular hexagon.2)Identify the rationality of the basic modelNow we considering the influence of the density of users,according to 1),90*37=3330>1000,so here the number of users have no influence on our model.Our model is rationality.3.1.6Strength and Weakness●Strength:In this paper,we use the model of honeycomb-hexagon structure can maximize the use of resources,avoiding some unnecessary interference effectively.It is much more intuitive once we change the function model to the geometric model.●Weakness:Since each hexagon get too close to another one.Once there are somebuildingsor terrain fluctuations between two repeaters,it can lead to the phenomenon that certain areas will have no signals.In addition,users are distributed evenly is not reasonable.The users are moving,for example some people may get a party.3.1.7Some ImprovementAs we all know,the absolute evenly distribution is not exist.So it is necessary to say something about the normal distribution model.The maximum accommodate number of a repeater is 5*54=270.As for the first model,it is impossible that 270users are communicating in a same repeater.Look at Fig 6.If there are N people in the area 1,the maximum number of the area 2to area 7is 3*(270-N).As 37*90=3330is much larger than 1000,our solution is still reasonable to this model.Figure 63.2Improve Model3.2.1Extra SymbolsSigns and definitions indicated above are still valid.Here are some extra signs and definitions.symboldescription Ra the radius of the circular flat area the side length of a regular hexagon3.2.2Additional AdditionalAssumptionsAssumptions ●The radius that of a repeater covers is adjustable here.●In some limited situations,curved shape is equal to straight line.●Assumptions concerning the anterior process are the same as the Basic Model3.2.3The Foundation of ModelThe same as the Basic Model except that:We only consider one variable(p)in the function model of the basic model ;In this model,we consider two varibles(p and µ)of the function model.3.2.4Solution and Result1)SolutionIf there are 10,000users,the number of regular hexagons that we need is at least ,thus according to the the Principle of maximum flow of Graph Theory,the 10000111.1190=result that we draw needed to be extended further.When the side length of the figure is equal to 7Figure 7regular hexagons,there are 127regular hexagons (Fig 7).Assuming the side length of a regular hexagon is ,then the area of a regular hexagon is a .The area of regular hexagons is equal to a circlewhose radiusis 22a =1000090R.Then according to the formula below:.221000090a R π=We can get.9.5858R a =Mapping with MATLAB as below (Fig 8):Figure 82)Improve the model appropriatelyEnlarge two part of the figure above,we can get two figures below (Fig 9and Fig 10):Figure 9AREAFigure 10Look at the figure above,approximatingAREA a rectangle,then obtaining its area to getthe number of users..The length of the rectangle is approximately equal to the side length of the regular hexagon ,athe width of the rectangle is ,thus the area of AREA is ,then R ?*R awe can get the number of users in AREA is(),2**10000 2.06R a R π=????????9.5858R a =As 2.06<<10,000,2.06can be ignored ,so there is no need to set up a repeater in.There are 6suchareas(92,98,104,110,116,122)that can be ignored.At last,the number of repeaters we should set up is,1276121?=2)Get the side length of the regular hexagon of the improved modelThus we can getmile=km 40 4.1729.5858a == 1.6* 6.675a =3)Calculate the power of a repeaterAccording to the formula[]()()32.4420lg ()20lg Lfs dB d km f MHz =++We get32.4420lg 6.67520lg14592.156Los =++=32.4420lg 6.67520lg14892.334Los =++=So we get106.85-92.156=14.694106.85-92.334=14.516As the result in the basic model,we can get the conclusion the power of a repeater is from 14.694mW to 14.516mW.3.2.5Analysis of the ResultAs 10,000users are much more than 1000users,the distribution of the users is more close toevenly distribution.Thus the model is more reasonable than the basic one.More repeaters are built,the utilization of the outside regular hexagon are higher than the former one.3.2.6Strength and Weakness●Strength:The model is more reasonable than the basic one.●Weakness:Repeaters don’t cover all the area,some places may not receive signals.And thefoundation of this model is based on the evenly distribution of the users in the area,if the situation couldn’t be satisfied,the interference of signals will come out.Ⅳ.Conclusions4.1Conclusions of the problem●Generally speaking,the radius of the area that a repeater covers is4miles in our basic model.●Using the model of honeycomb-hexagon structure can maximize the use of resources,avoiding some unnecessary interference effectively.●The minimum number of repeaters necessary to accommodate1,000simultaneous users is37.The minimum number of repeaters necessary to accommodate10,000simultaneoususers is121.●A repeater's coverage radius relates to external environment such as the density of users andobstacles,and it is also determined by the power of the repeater.4.2Methods used in our models●Analysis the problem with MATLAB●the method of the coverage in Graph Theory4.3Application of our models●Choose the ideal address where we set repeater of the mobile phones.●How to irrigate reasonably in agriculture.●How to distribute the lights and the speakers in squares more reasonably.Ⅴ.Future WorkHow we will do if the area is mountainous?5.1The best position of a repeater is the top of the mountain.As the signals are line-of-sight transmission and reception.We must find a place where the signals can transmit from the repeater to users directly.So the top of the mountain is a good place.5.2In mountainous areas,we must increase the number of repeaters.There are three reasons for this problem.One reason is that there will be more obstacles in the mountainous areas. The signals will be attenuated much more quickly than they transmit in flat area.Another reason is that the signals are line-of-sight transmission and reception,we need more repeaters to satisfy this condition.Then look at Fig11and Fig12,and you will know the third reason.It can be clearly seen that hypotenuse is larger than right-angleFig11edge(R>r).Thus the radius will become smaller.In this case more repeaters are needed.Fig125.3In mountainous areas,people may mainly settle in the flat area,so the distribution of users isn’t uniform.5.4There are different altitudes in the mountainous areas.So in order to increase the rate of resources utilization,we can set up the repeaters in different altitudes.5.5However,if there are more repeaters,and some of them are on mountains,more money will be/doc/d7df31738e9951e79b8927b4.html munication companies will need a lot of money to build them,repair them when they don’t work well and so on.As a result,the communication costs will be high.What’s worse,there are places where there are many mountains but few persons. Communication companies reluctant to build repeaters there.But unexpected things often happen in these places.When people are in trouble,they couldn’t communicate well with the outside.So in my opinion,the government should take some measures to solve this problem.5.6Another new method is described as follows(Fig13):since the repeater on high mountains can beFig13Seen easily by people,so the tower which used to transmit and receive signals can be shorter.That is to say,the tower on flat areas can be a little taller..Ⅵ.References[1]YU Fei,YANG Lv-xi,"Effective cooperative scheme based on relay selection",SoutheastUniversity,Nanjing,210096,China[2]YANG Ming,ZHAO Xiao-bo,DI Wei-guo,NAN Bing-xin,"Call Admission Control Policy based on Microcellular",College of Electical and Electronic Engineering,Shijiazhuang Railway Institute,Shijiazhuang Heibei050043,China[3]TIAN Zhisheng,"Analysis of Mechanism of CTCSS Modulation",Shenzhen HYT Co,Shenzhen,518057,China[4]SHANGGUAN Shi-qing,XIN Hao-ran,"Mathematical Modeling in Bass Station Site Selectionwith Lingo Software",China University of Mining And Technology SRES,Xuzhou;Shandong Finance Institute,Jinan Shandon,250014[5]Leif J.Harcke,Kenneth S.Dueker,and David B.Leeson,"Frequency Coordination in the AmateurRadio Emergency ServiceⅦ.AppendixWe use MATLAB to get these pictures,the code is as follows:1-clc;clear all;2-r=1;3-rc=0.7;4-figure;5-axis square6-hold on;7-A=pi/3*[0:6];8-aa=linspace(0,pi*2,80);9-plot(r*exp(i*A),'k','linewidth',2);10-g1=fill(real(r*exp(i*A)),imag(r*exp(i*A)),'k');11-set(g1,'FaceColor',[1,0.5,0])12-g2=fill(real(rc*exp(i*aa)),imag(rc*exp(i*aa)),'k');13-set(g2,'FaceColor',[1,0.5,0],'edgecolor',[1,0.5,0],'EraseMode','x0r')14-text(0,0,'1','fontsize',10);15-Z=0;16-At=pi/6;17-RA=-pi/2;18-N=1;At=-pi/2-pi/3*[0:6];19-for k=1:2;20-Z=Z+sqrt(3)*r*exp(i*pi/6);21-for pp=1:6;22-for p=1:k;23-N=N+1;24-zp=Z+r*exp(i*A);25-zr=Z+rc*exp(i*aa);26-g1=fill(real(zp),imag(zp),'k');27-set(g1,'FaceColor',[1,0.5,0],'edgecolor',[1,0,0]);28-g2=fill(real(zr),imag(zr),'k');29-set(g2,'FaceColor',[1,0.5,0],'edgecolor',[1,0.5,0],'EraseMode',xor';30-text(real(Z),imag(Z),num2str(N),'fontsize',10);31-Z=Z+sqrt(3)*r*exp(i*At(pp));32-end33-end34-end35-ezplot('x^2+y^2=25',[-5,5]);%This is the circular flat area of radius40miles radius 36-xlim([-6,6]*r) 37-ylim([-6.1,6.1]*r)38-axis off;Then change number19”for k=1:2;”to“for k=1:3;”,then we get another picture:Change the original programme number19“for k=1:2;”to“for k=1:4;”,then we get another picture:。
正确写作美国大学生数学建模竞赛论文

1)、鉴别阶段: (10分钟)
所有论文在此阶段按其质量分别归入一下三类:第一类 是可以进入下一评审阶段的论文(略少于二分之一);第二类 是满足竞赛要求,但不足以进入下一评审阶段的论文(这一类 就被定为合格论文);第三类是不符合竞赛要求的论文(不合 格论文)。 由于在第一阶段中,评委只有10分钟左右的时间评审一 篇论文,因此评委常常只能通过阅读摘要来判断论文水平的高 低。
例如,2010年MCM竞赛中有一道赛题,要求参赛小 组根据以往的作案地点预测连环犯罪的位置。
3.1)、假设条件和解释 解答这道赛题的重点是犯罪活动方式。在一篇题为 “Centroids, Clusters, and Crime: Anchoring the Geographic Profiles of Serial Criminals”的论文中,有一条假设是“罪犯 的活动不受限制”,但罪犯是在市区的活动,实际上会受 到街道的布局及街道两旁建筑物的限制。由于街道布局通 常类似于网格,所以参赛小组对这个假设做了如下解释: Criminal’s movement is unconstrained. Because of the difficulty of finding real-world distance data, we invoke the „Manhattan assumption‟: There are enough streets and sidewalks in a sufficiently grid-like pattern that movements along real-world movement routes is the same as „straight-line‟ movement in a space discretized into city blocks…
3分钟搞懂美国作业论文格式要求

3分钟搞懂美国作业论文格式要求对于所有初来美国的留学小白,需要适应的有太多太多了。
比如,半小时一趟的公交车,没有蛋清的炒鸡蛋,没有关东煮的711,没有大米饭的肯德基...等等等等。
不过,对于热爱学习的我们来说,这些都是次要的,我们的重点是学习!学习的重点是做作业!第一次用英语写作业,有没有一点小激动呢?不过,很多新生都会面临一个问题:呃...该用什么格式呢?字号多大?行间距多少?尤其是到了期末,各种Final Essay扑面而来,更是应接不暇,好不容易凑够了字数和页数,还需要改引用格式,没有比这更心累的了。
今天小编就给大家分享一下在美国写论文的格式,还有平时作业需要注意的小问题。
首先,美国大学论文的学术性格式主要有三种Style:APA MLA Chicago。
APAAmerican Psychological Association is used by Education, Psychology, and Sciences.MLAModern Language Associationstyle is used by the Humanities.ChicagoChicago/Turabian style is generally used by Business, History, and the Fine Arts.翻译一下:APA多用于教育,心理学和科学的专业;MLA风格由人文学科使用;芝加哥/ Turabian风格通常被商业,历史和美术所用。
其实,真正决定你用什么格式的就是你的教授了,不同教授会有不同要求。
根据小编的切身体验,芝加哥格式是最简单的,MLA是最麻烦的,APA是正常的。
小编曾经遇到一个非常Nice的教授,他的期末论文是不要求格式的,他说,你喜欢什么格式就用什么格式,根据你的个人习惯就好。
然后,小编也不幸的遇到一个特别严格的老师,还是在研究生的第一学期,然后就写下了唯一一篇用MLA格式的20页论文。
2014美国大学生数学建模特等奖优秀论文
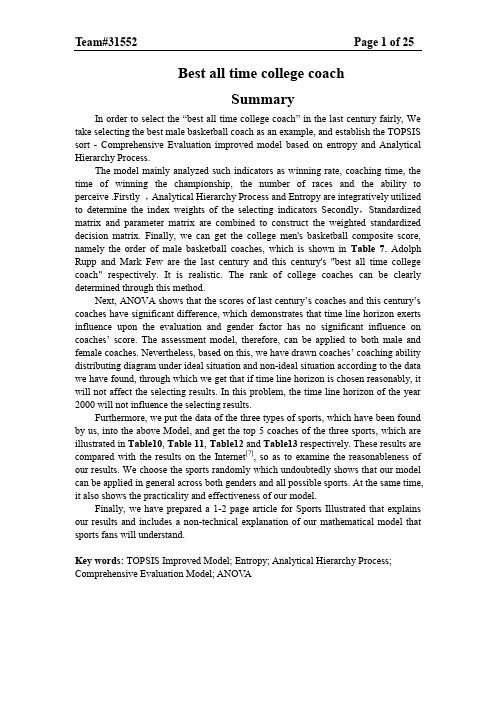
Page 1 of 25
Best all time college coach Summary
In order to select the “best all time college coach” in the last century fairly, We take selecting the best male basketball coach as an example, and establish the TOPSIS sort - Comprehensive Evaluation improved model based on entropy and Analytical Hierarchy Process. The model mainly analyzed such indicators as winning rate, coaching time, the time of winning the championship, the number of races and the ability to perceive .Firstly , Analytical Hierarchy Process and Entropy are integratively utilized to determine the index weights of the selecting indicators Secondly,Standardized matrix and parameter matrix are combined to construct the weighted standardized decision matrix. Finally, we can get the college men's basketball composite score, namely the order of male basketball coaches, which is shown in Table 7. Adolph Rupp and Mark Few are the last century and this century's "best all time college coach" respectively. It is realistic. The rank of college coaches can be clearly determined through this method. Next, ANOVA shows that the scores of last century’s coaches and this century’s coaches have significant difference, which demonstrates that time line horizon exerts influence upon the evaluation and gender factor has no significant influence on coaches’ score. The assessment model, therefore, can be applied to both male and female coaches. Nevertheless, based on this, we have drawn coaches’ coaching ability distributing diagram under ideal situation and non-ideal situation according to the data we have found, through which we get that if time line horizon is chosen reasonably, it will not affect the selecting results. In this problem, the time line horizon of the year 2000 will not influence the selecting results. Furthermore, we put the data of the three types of sports, which have been found by us, into the above Model, and get the top 5 coaches of the three sports, which are illustrated in Table10, Table 11, Table12 and Table13 respectively. These results are compared with the results on the Internet[7], so as to examine the reasonableness of our results. We choose the sports randomly which undoubtedly shows that our model can be applied in general across both genders and all possible sports. At the same time, it also shows the practicality and effectiveness of our model. Finally, we have prepared a 1-2 page article for Sports Illustrated that explains our results and includes a non-technical explanation of our mathematical model that sports fans will understand. Key words: TOPSIS Improved Model; Entropy; Analytical Hierarchy Process; Comprehensive Evaluation Model; ANOVA
2011美国大学生数学建模论文

1 / 14
A DYNAMIC MODEL FOR OPTIMUM DESIGN OF SNOWBOARD HALF-Fra bibliotekIPE SHAPE
Abstract- Firstly the types of skiing about how a skier uses the snowboard are being discussed and we conclude that a skilled skier can perform carved turns to minimize the speed loss while turning. We assume that a skilled snowboarder can always choose the best path in a “half-pipe” game and can turn without energy reduction. Then a dynamic model studying the process of a snowboarder moving from the bottom to the air is set up, aiming at investigating the relationship between “speed loss” and “path choice” in a “half-pipe”. Applying the functional analysis technique we work out a solution to the simplified model. With this solution a new type “half-pipes” that can minimize the resistance effect are designed. When analyzing the maximum twists in the air, we recognize that the kinetics just before the snowboarder taking off is the key. We do the analysis and conclude that a steep end of the course can help to fly high. At last we simply research the “practical” snow course and remark the strengths and weaknesses of our model.
- 1、下载文档前请自行甄别文档内容的完整性,平台不提供额外的编辑、内容补充、找答案等附加服务。
- 2、"仅部分预览"的文档,不可在线预览部分如存在完整性等问题,可反馈申请退款(可完整预览的文档不适用该条件!)。
- 3、如文档侵犯您的权益,请联系客服反馈,我们会尽快为您处理(人工客服工作时间:9:00-18:30)。
在美国,每门课一个学期一般要写3~4篇论文。
为了完成论文,学生们就得大量读书、上网。
虽然书籍和网络上各种相关资料应有尽有,但是信手拈来恐怕不行。
每门课开课的第一天老师就会告诫大家,不许抄袭。
引用别人的话,就要注明出处。
即便不照抄人家的原话,而是用自己的话表述,也是剽窃了人家的观点。
凡是抄袭,一旦发现,轻则警告,重则开除。
因此,美国大学生写论文很少有东拼西凑、蒙混过关的,他们必须好好动脑筋,展示出自己的观点和论据。
出勤率、作业、发言、期中考试、期末考试、论文,这些都要算在最后的总评成绩里,一样都不能疏忽,所以大多数美国学生都认为大学学习十分辛苦。
至于毕业论文,绝大多数美国大学的本科生是不用写的,只有普林斯顿等很少的几所大学要求本科生写毕业论文。
即便如此,美国还是有不少大学生不能按时修完所有的学分,推迟毕业的现象非常普遍。