model predictive control toolbox
(完整版)MATLAB中英文对照
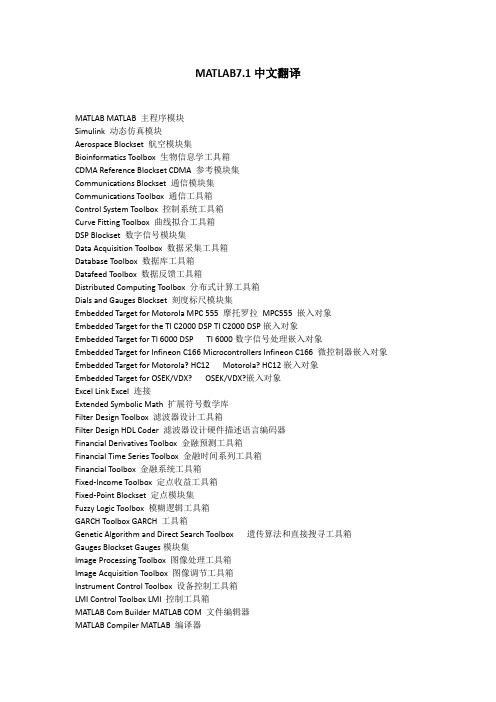
MATLAB7.1中文翻译MATLAB MATLAB 主程序模块Simulink 动态仿真模块Aerospace Blockset 航空模块集Bioinformatics Toolbox 生物信息学工具箱CDMA Reference Blockset CDMA 参考模块集Communications Blockset 通信模块集Communications Toolbox 通信工具箱Control System Toolbox 控制系统工具箱Curve Fitting Toolbox 曲线拟合工具箱DSP Blockset 数字信号模块集Data Acquisition Toolbox 数据采集工具箱Database Toolbox 数据库工具箱Datafeed Toolbox 数据反馈工具箱Distributed Computing Toolbox 分布式计算工具箱Dials and Gauges Blockset 刻度标尺模块集Embedded Target for Motorola MPC 555 摩托罗拉MPC555 嵌入对象Embedded Target for the TI C2000 DSP TI C2000 DSP嵌入对象Embedded Target for TI 6000 DSP TI 6000数字信号处理嵌入对象Embedded Target for Infineon C166 Microcontrollers Infineon C166 微控制器嵌入对象Embedded Target for Motorola? HC12 Motorola? HC12嵌入对象Embedded Target for OSEK/VDX? OSEK/VDX?嵌入对象Excel Link Excel 连接Extended Symbolic Math 扩展符号数学库Filter Design Toolbox 滤波器设计工具箱Filter Design HDL Coder 滤波器设计硬件描述语言编码器Financial Derivatives Toolbox 金融预测工具箱Financial Time Series Toolbox 金融时间系列工具箱Financial Toolbox 金融系统工具箱Fixed-Income Toolbox 定点收益工具箱Fixed-Point Blockset 定点模块集Fuzzy Logic Toolbox 模糊逻辑工具箱GARCH Toolbox GARCH 工具箱Genetic Algorithm and Direct Search Toolbox 遗传算法和直接搜寻工具箱Gauges Blockset Gauges模块集Image Processing Toolbox 图像处理工具箱Image Acquisition Toolbox 图像调节工具箱Instrument Control Toolbox 设备控制工具箱LMI Control Toolbox LMI 控制工具箱MATLAB Com Builder MATLAB COM 文件编辑器MATLAB Compiler MATLAB 编译器MATLAB Excel Builder MATLAB 外部编辑器MATLAB Link for code composer studio MATLAB 与代码设计工作室的连接MATLAB Link for ModelSim MATLAB与ModelSim的连接MATLAB Report Generator MATLAB 报告生成器MATLAB Runtime Server MATLAB 运行时间服务器MATLAB Webs Server MATLAB 支持Web 服务器MATLAB? Distributed Computing Engine MATLAB? 分布式计算引擎Mapping Toolbox 地图工具箱Model Predictive Control Toolbox 模型预测控制工具箱Model-Based Calibration Toolbox 基于模型标准工具箱Mu-Analysis and Synthesis ToolboxMU 分析与合成工具箱Neural Network Toolbox 神经网络工具箱Nonlinear Control Design Blockset 非线性控制设计模块集OPC Toolbox OPC工具箱Optimization Toolbox 优化工具箱Partial Differential Equation Toolbox 偏微分方程工具箱Real-Time Windows Target 实时视窗对象Real-Time Workshop 实时工作室Real-Time Workshop Embedded Coder 实时工作室内嵌编码器Requirements Management Interface 需求管理界面Robust Control Toolbox 强(鲁棒)控制工具箱RF Toolbox RF工具箱SB2SL(converts models to Simulink) 模型转换成Simulink工具Signal Processing Toolbox 信号处理工具箱Signal Processing Blockset 信号处理模块集Sim Driveline SIM动力传动系统Sim Mechanics SIM机械学Sim Power Systems SIM电力系统Simulink Performance ToolsSimulink 执行工具箱Simulink Report Generator Simulink 报表生成器Simulink Verification and Validation Simulink 核实与验证Simulink Response Optimization Simulink响应优化Simulink Parameter Estimation Simulink 参数估计Simulink Fixed Point Simulink不动点Simulink Control Design Simulink控制设计Simulink Accelerator Simulink加速器Spline Toolbox 样条工具箱Stateflow 状态流Stateflow Coder 状态流编码器Statistics Toolbox 统计工具箱Symbolic Math Toolbox 符号数学工具箱System Identification Toolbox 系统识别工具箱Virtual Reality Toolbox 虚拟现实工具箱Video and Image Processing Blockset 视频和图像处理模块集Wavelet Toolbox 小波分析工具箱xPC Target XPC对象xPC Target Embedded Option XPC对象内嵌属性。
matlab各种应用工具箱参考

2021/3/10
讲解:XX
11
二、通用工具箱
• Matlab主工具箱
• 前面课程所介绍的数值计算、符号运算、 绘图以及句柄绘图都是matlab主工具箱 的内容,是matlab的基本部分,也是我 们课程的重点。
• Matlab主工具箱位于:
c:\matlab\toolbox\matlab
• matlab主工具箱是任何版本的matlab都
simulink 的一般结构:
输入
系统
输出
2021/3/10
讲解:XX
27
仿真原理
• 当在框图视窗中进行仿真的同时,matlab 实际上是运行保存于simulink内存中s函数 的映象文件,而不是解释运行该m文件。
• s函数并不是标准m文件,它m文件的一种 特殊形式。
结构图创建方法
• 一个动态系统的创建过程,就是一个方框 图的绘制过程
rose - Angle histogram plot.
compass - Compass plot.
feather - Feather plot.
fplot - Plot function.
comet - Comet-like trajectory.
2021/3/10
讲解:XX
17
Graph annotation. title - Graph title. xlabel - X-axis label. ylabel - Y-axis label. text - Text annotation. gtext - Mouse placement of text. grid - Grid lines.
高阶谱分析工具箱
2021/3/10
第6讲 matlab工具箱介绍与仿真基础
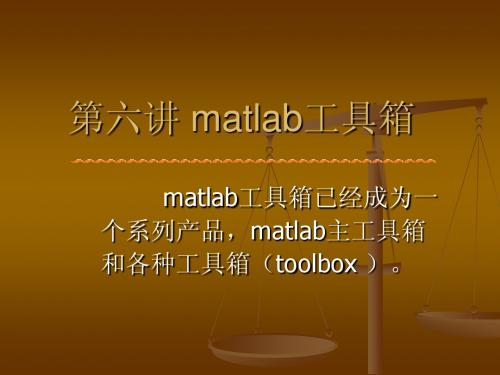
Signal Processing Toolbox——信号处理工具 箱 Spline Toolbox——样条工具箱 Statistics Toolbox——统计工具箱 Symbolic Math Toolbox——符号数学工具箱 Simulink Toolbox——动态仿真工具箱 System Identification Toolbox——系统辨识 工具箱 Wavele Toolbox——小波工具箱 等等
领域型工具箱
—— 专用型
领域型工具箱是学科专用工具 箱,其专业性很强,比如控制系统工
具箱( Control System Toolbox);信
号处理工具箱(Signal Processing
Toolbox);财政金融工具箱( Financial
Toolbox)等等。只适用于本专业。
Matlab常用工具箱
变量 f fun H A,b Aeq,beq vlb,vub X0 x1,x2 options 描 述 线性规划的目标函数f*X 或二次规划的目标函 数X’*H*X+f*X 中线性项的系数向量 非线性优化的目标函数.fun必须为行命令对象 或M文件、嵌入函数、或MEX文件的名称 二次规划的目标函数X’*H*X+f*X 中二次项的系 数矩阵 A矩阵和b向量分别为线性不等式约束: AX b 中的系数矩阵和右端向量 Aeq矩阵和beq向量分别为线性等式约束: Aeq X beq 中的系数矩阵和右端向量 X的下限和上限向量:vlb≤X≤vub 迭代初始点坐标 函数最小化的区间 优化选项参数结构,定义用于优化函数的参数 调用函数 linprog,quadprog fminbnd,fminsearch,fminunc, fmincon,lsqcurvefit,lsqnonlin, fgoalattain,fminimax quadprog linprog,quadprog,fgoalattain, fmincon, fminimax linprog,quadprog,fgoalattain, fmincon, fminimax linprog,quadprog,fgoalattain, fmincon,fminimax,lsqcurvefit, lsqnonlin 除fminbnd外所有优化函数 fminbnd 所有优化函数
Matlab工具箱中英文对照
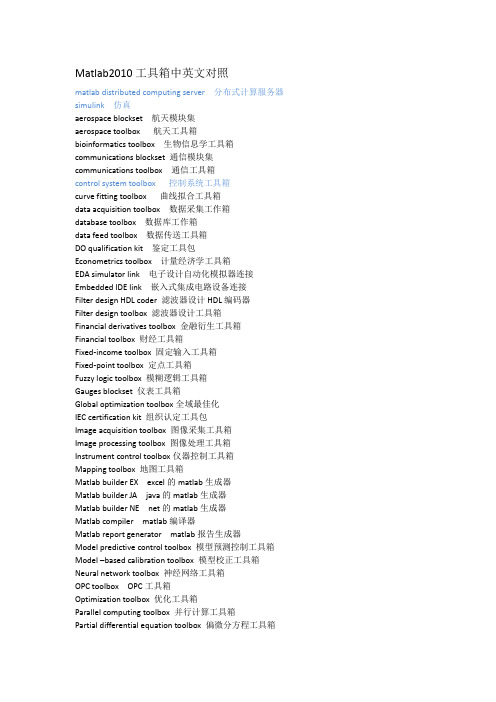
Matlab2010工具箱中英文对照matlab distributed computing server 分布式计算服务器simulink 仿真aerospace blockset 航天模块集aerospace toolbox 航天工具箱bioinformatics toolbox 生物信息学工具箱communications blockset 通信模块集communications toolbox 通信工具箱control system toolbox 控制系统工具箱curve fitting toolbox 曲线拟合工具箱data acquisition toolbox 数据采集工作箱database toolbox 数据库工作箱data feed toolbox 数据传送工具箱DO qualification kit 鉴定工具包Econometrics toolbox 计量经济学工具箱EDA simulator link 电子设计自动化模拟器连接Embedded IDE link 嵌入式集成电路设备连接Filter design HDL coder 滤波器设计HDL编码器Filter design toolbox 滤波器设计工具箱Financial derivatives toolbox 金融衍生工具箱Financial toolbox 财经工具箱Fixed-income toolbox 固定输入工具箱Fixed-point toolbox 定点工具箱Fuzzy logic toolbox 模糊逻辑工具箱Gauges blockset 仪表工具箱Global optimization toolbox全域最佳化IEC certification kit 组织认定工具包Image acquisition toolbox 图像采集工具箱Image processing toolbox 图像处理工具箱Instrument control toolbox仪器控制工具箱Mapping toolbox 地图工具箱Matlab builder EX excel的matlab生成器Matlab builder JA java的matlab生成器Matlab builder NE net的matlab生成器Matlab compiler matlab编译器Matlab report generator matlab报告生成器Model predictive control toolbox 模型预测控制工具箱Model –based calibration toolbox 模型校正工具箱Neural network toolbox 神经网络工具箱OPC toolbox OPC工具箱Optimization toolbox 优化工具箱Parallel computing toolbox 并行计算工具箱Partial differential equation toolbox 偏微分方程工具箱Real-time windows target 实时windows目标Real-time workshop embedded coder 实时工作空间内置编码器RF toolbox RF工具箱Robust control toolbox 鲁棒控制工具箱Signal processing blockset 型号处理模块集Signal processing toolbox 型号处理工具箱Simbiology 仿真生物Simdriveline 仿真驱动链接Simelectronics 仿真电子学Simevents 仿真事件Simhydraulic 仿真液压Simmechanics 仿真力学Simpowersystem 仿真动力系统SimRF 仿真RFSimscape 仿真场景Simulink 3D animation 3D动画仿真Simulink control design 控制设计仿真Simulink design optimization 设计优化仿真Simulink design verifier 设计校正仿真Simulink fixed point 定点仿真Simulink HDL coder HDL编码器仿真Simulink PLC coder PLC编码器仿真Simulink report generator 报告生成器仿真Simulink verification and validation 仿真检验与确认Spreadsheet link EX excel电子表格连接State flow 状态流State flow coder 状态流编码器Statistics toolbox 统计工具箱Symbolic math toolbox 符号数学工具箱System identification toolbox 系统识别工具箱System test 系统测试Target support package 目标支持工具包Vehicle network toolbox 运载网络工具箱Video and image processing blockset 视频和图像处理模块集Wavelet toolbox 小波工具箱xPC target xPC目标xPC target embedded option xPC目标嵌入选择。
预测控制matlab工具箱
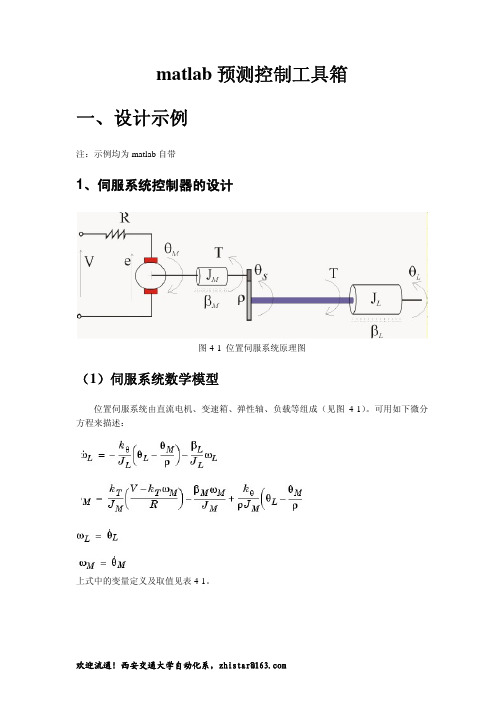
matlab预测控制工具箱一、设计示例注:示例均为matlab自带1、伺服系统控制器的设计图4-1 位置伺服系统原理图(1)伺服系统数学模型位置伺服系统由直流电机、变速箱、弹性轴、负载等组成(见图4-1)。
可用如下微分方程来描述:上式中的变量定义及取值见表4-1。
将上述微分方程写成状态方程形式,有其中,。
(2)控制目标及约束控制目标:在电压V的控制下,使过载角位置跟踪指定值。
输出量仅有可观测。
弹性轴承受的强度有一定的限制,因此对输出力矩T的赋值作一定约束:对输入电压的约束:该系统有一个输入V,两个输出:(可测量)和T(不可测量)。
(3)在matlab中定义该系统的状态空间模型首先需要在matlab中对系统的数学模型进行定义。
可以直接在命令行输入mpcmotormodel(建议做法),也可以在命令行中输入下列命令:% DC-motor with elastic shaft%%Parameters (MKS)%-----------------------------------------------------------Lshaft=1.0; %Shaft lengthdshaft=0.02; %Shaft diametershaftrho=7850; %Shaft specific weight (Carbon steel)G=81500*1e6; %Modulus of rigiditytauam=50*1e6; %Shear strengthMmotor=100; %Rotor massRmotor=.1; %Rotor radiusJmotor=.5*Mmotor*Rmotor^2; %Rotor axial moment of inertia Bmotor=0.1; %Rotor viscous friction coefficient (A CASO)R=20; %Resistance of armatureKt=10; %Motor constantgear=20; %Gear ratioJload=50*Jmotor; %Load inertiaBload=25; %Load viscous friction coefficientIp=pi/32*dshaft^4; %Polar momentum of shaft(circular) sectionKth=G*Ip/Lshaft; %Torsional rigidity(Torque/angle)Vshaft=pi*(dshaft^2)/4*Lshaft; %Shaft volumeMshaft=shaftrho*Vshaft; %Shaft massJshaft=Mshaft*.5*(dshaft^2/4); %Shaft moment of inertiaJM=Jmotor;JL=Jload+Jshaft;Vmax=tauam*pi*dshaft^3/16; %Maximum admissible torqueVmin=-Vmax;%Input/State/Output continuous time form%----------------------------------------------------------AA=[0 1 0 0;-Kth/JL -Bload/JL Kth/(gear*JL) 0;0 0 0 1;Kth/(JM*gear) 0 -Kth/(JM*gear^2)-(Bmotor+Kt^2/R)/JM];BB=[0;0;0;Kt/(R*JM)];Hyd=[1 0 0 0];Hvd=[Kth 0 -Kth/gear 0];Dyd=0;Dvd=0;% Define the LTI state-space modelsys=ss(AA,BB,[Hyd;Hvd],[Dyd;Dvd]);(4)利用MPCTOOL界面设计控制器第一步:导入模型在命令行窗口中输入mpctool,工具箱界面出来后点击Imort Plant…,此时界面如4-2所示。
MATLAB中的模型预测控制算法实现方法
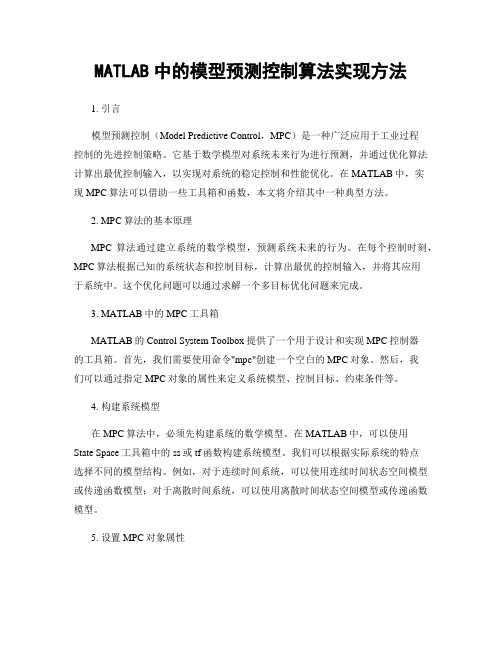
MATLAB中的模型预测控制算法实现方法1. 引言模型预测控制(Model Predictive Control,MPC)是一种广泛应用于工业过程控制的先进控制策略。
它基于数学模型对系统未来行为进行预测,并通过优化算法计算出最优控制输入,以实现对系统的稳定控制和性能优化。
在MATLAB中,实现MPC算法可以借助一些工具箱和函数,本文将介绍其中一种典型方法。
2. MPC算法的基本原理MPC算法通过建立系统的数学模型,预测系统未来的行为。
在每个控制时刻,MPC算法根据已知的系统状态和控制目标,计算出最优的控制输入,并将其应用于系统中。
这个优化问题可以通过求解一个多目标优化问题来完成。
3. MATLAB中的MPC工具箱MATLAB的Control System Toolbox提供了一个用于设计和实现MPC控制器的工具箱。
首先,我们需要使用命令"mpc"创建一个空白的MPC对象。
然后,我们可以通过指定MPC对象的属性来定义系统模型、控制目标、约束条件等。
4. 构建系统模型在MPC算法中,必须先构建系统的数学模型。
在MATLAB中,可以使用State Space工具箱中的ss或tf函数构建系统模型。
我们可以根据实际系统的特点选择不同的模型结构。
例如,对于连续时间系统,可以使用连续时间状态空间模型或传递函数模型;对于离散时间系统,可以使用离散时间状态空间模型或传递函数模型。
5. 设置MPC对象属性创建MPC对象后,我们需要设置一些重要的属性。
其中,PredictionHorizon属性定义了预测时间窗口的长度,即MPC算法根据模型预测未来的时长;ControlHorizon属性定义了控制时间窗口的长度,即MPC算法计算最优控制输入的时间长度。
一般来说,预测时间窗口应大于控制时间窗口。
6. 设定控制目标与约束条件MPC算法的目标是使系统的输出尽可能地接近控制目标,并同时满足一定的约束条件。
MATLAB环境下的模型预测控制理论的应用
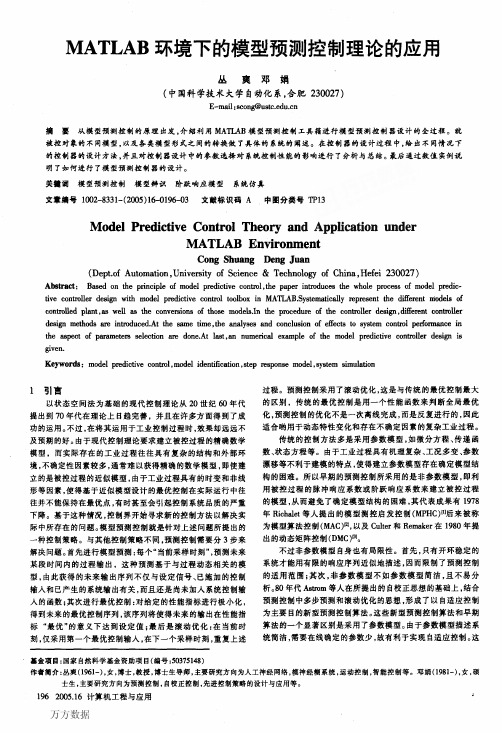
MATLAB环境下的模型预测控制理论的应用丛爽邓娟(中国科学技术大学自动化系,合肥230027)E-mail:scong@ustc.edu.cn摘要从模型预测控制的原理出发。
介绍利用MA7ⅡAB模型预测控制工具箱进行模型预测控制器设计的全过程。
就被控对象的不同模型。
以及备类模型形式之间的转换做了具体的系统的阐述。
在控刺器的设计过程中,给出不同情况下的控制器的设计方法,并且对控制器设计申的参数选择对系统控制性能的影响进行了分析与总结。
最后通过数值实例说明了如何进行了模型预测控制器的设计。
关键词模型预测控制模型辨识阶跃响应模型系统仿真文章编号l002—8331一(2005)16-0196—03文献标识码A中圈分类号TPl3ModelPredictiveControlTheoryandApplicationunderMATLABEnvironment(Dept.ofAutomation,UniversityofScience&TechnologyofChina,Hefei230027)Abstract:Basedtheprincipleofmodelpredictivecontrol,thepaperintroducesthewholeprocessofmodelpredic—fivecontrollerdesignwithmodelpredictivecontroltoolboxinMATLAB.Systematicallyrepresentthedifferentmodelsofcontrolledplant,aswellastheconversionsofthosemodels.Intheprocedureofthecontrollerdesign,differentcontrollerdesignmethodsintroduced.Atthe8alTletime,theanalysesandconclusionofeffectstosystemcontrolperformanceintheaspectofparametersselection啪done.Atlast.annumericalexampleofthemodelpredictivecontrollerdesignisgiven.Keywords:modelpredictivecontrol,modelidentification,stepresponsemodel,systemsimulation1引言以状态空间法为基础的现代控制理论从20世纪60年代提出到70年代在理论上日趋完善,并且在许多方面得到了成功的运用。
model predictive control 参考课程
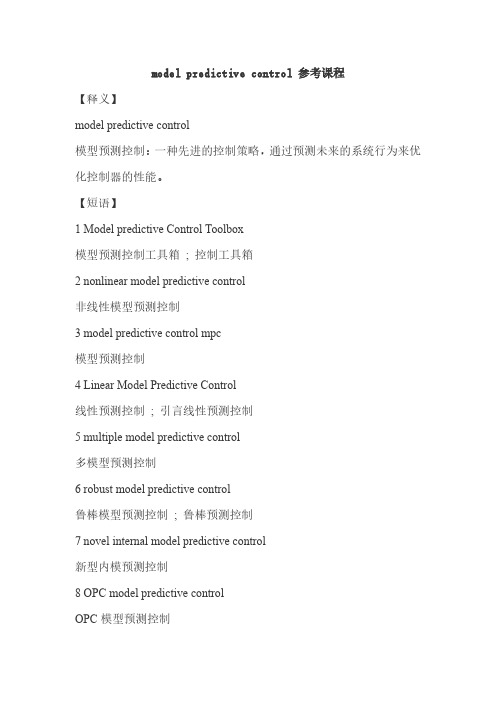
model predictive control参考课程【释义】model predictive control模型预测控制:一种先进的控制策略,通过预测未来的系统行为来优化控制器的性能。
【短语】1Model predictive Control Toolbox模型预测控制工具箱;控制工具箱2nonlinear model predictive control非线性模型预测控制3model predictive control mpc模型预测控制4Linear Model Predictive Control线性预测控制;引言线性预测控制5multiple model predictive control多模型预测控制6robust model predictive control鲁棒模型预测控制;鲁棒预测控制7novel internal model predictive control新型内模预测控制8OPC model predictive controlOPC模型预测控制【例句】1The application of Model Predictive Control to PTA equipment is presented.介绍了模型预测控制在PTA装置中的应用。
2Firstly,it considers the simple linear model predictive control algorithms.首先考虑简单的线性预测控制。
3A nonlinear model predictive control(NMPC)strategy based on T_S fuzzy model is proposed.提出了一种新的基于T_S模糊模型的非线性预测控制策略。
4Model predictive control based on the local linearization state-space model is introduced in detail.详细的介绍了基于局部线性化状态空间模型的预测控制算法。
matlab各工具箱功能简介(部分)

Toolbox工具箱序号工具箱备注一、数学、统计与优化1Symbolic Math Toolbox符号数学工具箱Symbolic Math Toolbox™提供用于求解和推演符号运算表达式以及执行可变精度算术的函数。
您可以通过分析执行微分、积分、化简、转换以及方程求解。
另外,还可以利用符号运算表达式为MATLAB®、Simulink®和Simscape™生成代码。
Symbolic Math Toolbox 包含MuPAD®语言,并已针对符号运算表达式的处理和执行进行优化。
该工具箱备有MuPAD 函数库,其中包括普通数学领域的微积分和线性代数,以及专业领域的数论和组合论。
此外,还可以使用MuPAD 语言编写自定义的符号函数和符号库。
MuPAD 记事本支持使用嵌入式文本、图形和数学排版格式来记录符号运算推导。
您可以采用HTML 或PDF 的格式分享带注释的推导。
2Partial Differential Euqation Toolbox偏微分方程工具箱偏微分方程工具箱™提供了用于在2D,3D求解偏微分方程(PDE)以及一次使用有限元分析。
它可以让你指定和网格二维和三维几何形状和制定边界条件和公式。
你能解决静态,时域,频域和特征值问题在几何领域。
功能进行后处理和绘图效果使您能够直观地探索解决方案。
你可以用偏微分方程工具箱,以解决从标准问题,如扩散,传热学,结构力学,静电,静磁学,和AC电源电磁学,以及自定义,偏微分方程的耦合系统偏微分方程。
3Statistics Toolbox统计学工具箱Statistics and Machine Learning Toolbox 提供运用统计与机器学习来描述、分析数据和对数据建模的函数和应用程序。
您可以使用用于探查数据分析的描述性统计和绘图,使用概率分布拟合数据,生成用于Monte Carlo 仿真的随机数,以及执行假设检验。
回归和分类算法用于依据数据执行推理并构建预测模型。
matlab在控制方面的示例
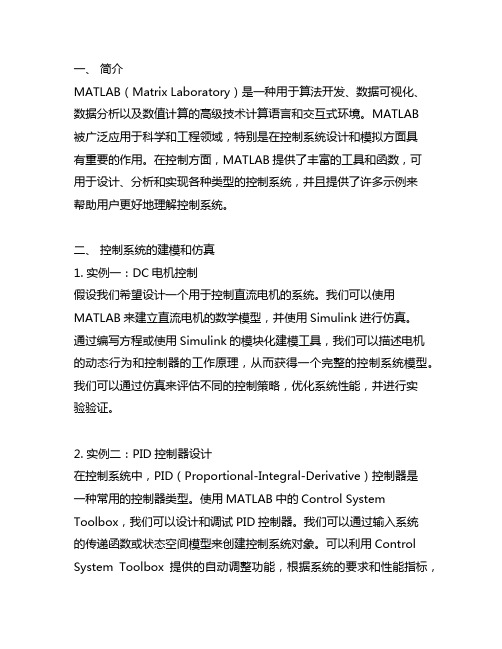
一、简介MATLAB(Matrix Laboratory)是一种用于算法开发、数据可视化、数据分析以及数值计算的高级技术计算语言和交互式环境。
MATLAB被广泛应用于科学和工程领域,特别是在控制系统设计和模拟方面具有重要的作用。
在控制方面,MATLAB提供了丰富的工具和函数,可用于设计、分析和实现各种类型的控制系统,并且提供了许多示例来帮助用户更好地理解控制系统。
二、控制系统的建模和仿真1. 实例一:DC电机控制假设我们希望设计一个用于控制直流电机的系统。
我们可以使用MATLAB来建立直流电机的数学模型,并使用Simulink进行仿真。
通过编写方程或使用Simulink的模块化建模工具,我们可以描述电机的动态行为和控制器的工作原理,从而获得一个完整的控制系统模型。
我们可以通过仿真来评估不同的控制策略,优化系统性能,并进行实验验证。
2. 实例二:PID控制器设计在控制系统中,PID(Proportional-Integral-Derivative)控制器是一种常用的控制器类型。
使用MATLAB中的Control System Toolbox,我们可以设计和调试PID控制器。
我们可以通过输入系统的传递函数或状态空间模型来创建控制系统对象。
可以利用Control System Toolbox提供的自动调整功能,根据系统的要求和性能指标,自动调整PID控制器的参数来实现系统稳定和性能优化。
三、控制系统分析和优化1. 实例三:系统频域分析在设计控制系统时,频域分析是一种重要的方法。
MATLAB提供了许多函数和工具,可用于进行频域分析。
我们可以使用bode函数来绘制系统的频率响应曲线,了解系统的增益和相位裕度,并进行稳定性分析。
MATLAB还提供了工具来进行奈奎斯特图和极点分析等分析方法,帮助用户更好地理解系统的动态特性。
2. 实例四:多目标优化在实际控制系统设计中,通常需要同时满足多个设计指标,例如稳定性、快速响应和抑制干扰等。
matlab电机算法
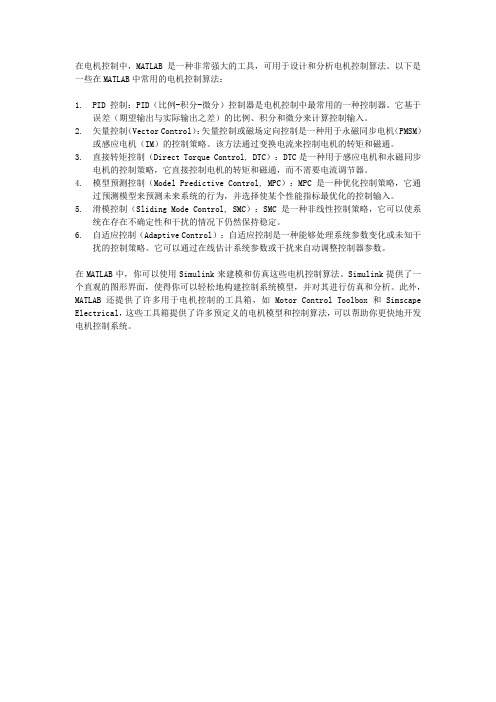
在电机控制中,MATLAB是一种非常强大的工具,可用于设计和分析电机控制算法。
以下是一些在MATLAB中常用的电机控制算法:1.PID控制:PID(比例-积分-微分)控制器是电机控制中最常用的一种控制器。
它基于误差(期望输出与实际输出之差)的比例、积分和微分来计算控制输入。
2.矢量控制(Vector Control):矢量控制或磁场定向控制是一种用于永磁同步电机(PMSM)或感应电机(IM)的控制策略。
该方法通过变换电流来控制电机的转矩和磁通。
3.直接转矩控制(Direct Torque Control, DTC):DTC是一种用于感应电机和永磁同步电机的控制策略,它直接控制电机的转矩和磁通,而不需要电流调节器。
4.模型预测控制(Model Predictive Control, MPC):MPC是一种优化控制策略,它通过预测模型来预测未来系统的行为,并选择使某个性能指标最优化的控制输入。
5.滑模控制(Sliding Mode Control, SMC):SMC是一种非线性控制策略,它可以使系统在存在不确定性和干扰的情况下仍然保持稳定。
6.自适应控制(Adaptive Control):自适应控制是一种能够处理系统参数变化或未知干扰的控制策略。
它可以通过在线估计系统参数或干扰来自动调整控制器参数。
在MATLAB中,你可以使用Simulink来建模和仿真这些电机控制算法。
Simulink提供了一个直观的图形界面,使得你可以轻松地构建控制系统模型,并对其进行仿真和分析。
此外,MATLAB还提供了许多用于电机控制的工具箱,如Motor Control Toolbox和Simscape Electrical,这些工具箱提供了许多预定义的电机模型和控制算法,可以帮助你更快地开发电机控制系统。
MATLAB常用工具箱及常用函数
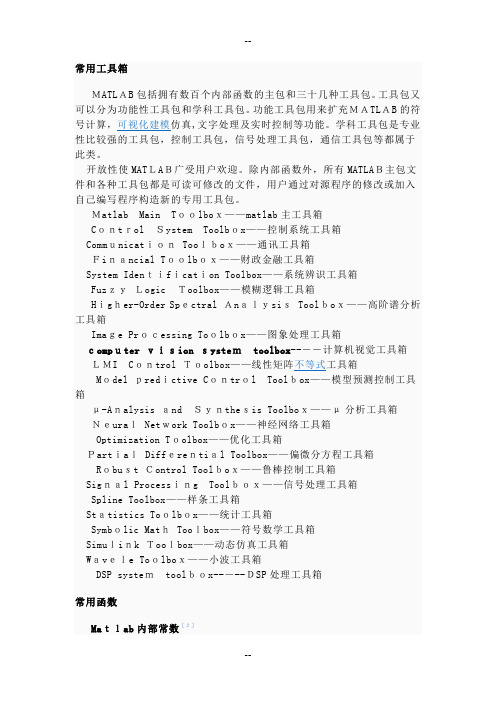
常用工具箱MATLAB包括拥有数百个内部函数的主包和三十几种工具包。
工具包又可以分为功能性工具包和学科工具包。
功能工具包用来扩充MATLAB的符号计算,可视化建模仿真,文字处理及实时控制等功能。
学科工具包是专业性比较强的工具包,控制工具包,信号处理工具包,通信工具包等都属于此类。
开放性使MATLAB广受用户欢迎。
除内部函数外,所有MATLAB主包文件和各种工具包都是可读可修改的文件,用户通过对源程序的修改或加入自己编写程序构造新的专用工具包。
Matlab Main Toolbox——matlab主工具箱Control System Toolbox——控制系统工具箱Communication Toolbox——通讯工具箱Financial Toolbox——财政金融工具箱System Identification Toolbox——系统辨识工具箱FuzzyLogic Toolbox——模糊逻辑工具箱Higher-Order Spectral Analysis Toolbox——高阶谱分析工具箱Image Processing Toolbox——图象处理工具箱computer vision systemtoolbox----计算机视觉工具箱LMI Control Toolbox——线性矩阵不等式工具箱Model predictive Control Toolbox——模型预测控制工具箱μ-Analysis and Synthesis Toolbox——μ分析工具箱Neural Network Toolbox——神经网络工具箱Optimization Toolbox——优化工具箱Partial Differential Toolbox——偏微分方程工具箱Robust Control Toolbox——鲁棒控制工具箱Signal Processing Toolbox——信号处理工具箱 Spline Toolbox——样条工具箱Statistics Toolbox——统计工具箱Symbolic Math Toolbox——符号数学工具箱Simulink Toolbox——动态仿真工具箱Wavele Toolbox——小波工具箱DSP systemtoolbox-----DSP处理工具箱常用函数Matlab内部常数[2]eps:浮点相对精度exp:自然对数的底数ei 或j:基本虚数单位inf 或Inf:无限大, 例如1/0nan或NaN:非数值(Not a number),例如0/0 pi:圆周率p(= 3.1415926...)realmax:系统所能表示的最大数值realmin:系统所能表示的最小数值nargin: 函数的输入引数个数nargout: 函数的输出引数个数lasterr:存放最新的错误信息lastwarn:存放最新的警告信息MATLAB常用基本数学函数abs(x):纯量的绝对值或向量的长度angle(z):复数z的相角(Phase angle)sqrt(x):开平方real(z):复数z的实部imag(z):复数z的虚部conj(z):复数z的共轭复数round(x):四舍五入至最近整数fix(x):无论正负,舍去小数至最近整数floor(x):下取整,即舍去正小数至最近整数ceil(x):上取整,即加入正小数至最近整数rat(x):将实数x化为多项分数展开rats(x):将实数x化为分数表示sign(x):符号函数(Signum function)。
matlab工具箱介绍
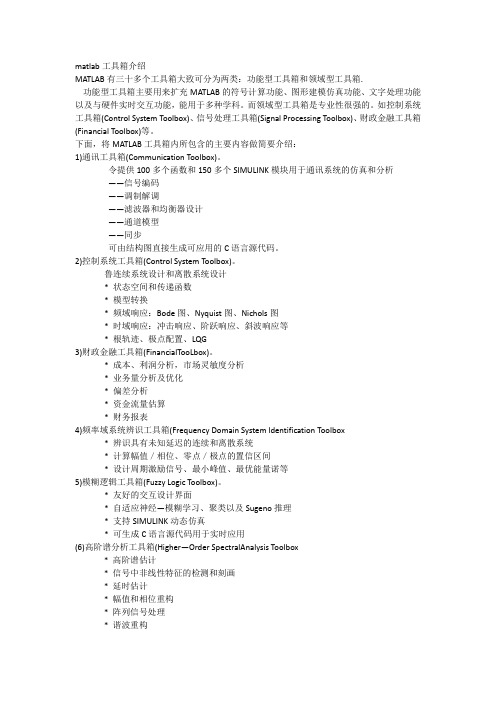
matlab工具箱介绍MATLAB有三十多个工具箱大致可分为两类:功能型工具箱和领域型工具箱.功能型工具箱主要用来扩充MATLAB的符号计算功能、图形建模仿真功能、文字处理功能以及与硬件实时交互功能,能用于多种学科。
而领域型工具箱是专业性很强的。
如控制系统工具箱(Control System Toolbox)、信号处理工具箱(Signal Processing Toolbox)、财政金融工具箱(Financial Toolbox)等。
下面,将MATLAB工具箱内所包含的主要内容做简要介绍:1)通讯工具箱(Communication Toolbox)。
令提供100多个函数和150多个SIMULINK模块用于通讯系统的仿真和分析——信号编码——调制解调——滤波器和均衡器设计——通道模型——同步可由结构图直接生成可应用的C语言源代码。
2)控制系统工具箱(Control System Toolbox)。
鲁连续系统设计和离散系统设计* 状态空间和传递函数* 模型转换* 频域响应:Bode图、Nyquist图、Nichols图* 时域响应:冲击响应、阶跃响应、斜波响应等* 根轨迹、极点配置、LQG3)财政金融工具箱(FinancialTooLbox)。
* 成本、利润分析,市场灵敏度分析* 业务量分析及优化* 偏差分析* 资金流量估算* 财务报表4)频率域系统辨识工具箱(Frequency Domain System ldentification Toolbox* 辨识具有未知延迟的连续和离散系统* 计算幅值/相位、零点/极点的置信区间* 设计周期激励信号、最小峰值、最优能量诺等5)模糊逻辑工具箱(Fuzzy Logic Toolbox)。
* 友好的交互设计界面* 自适应神经—模糊学习、聚类以及Sugeno推理* 支持SIMULINK动态仿真* 可生成C语言源代码用于实时应用(6)高阶谱分析工具箱(Higher—Order SpectralAnalysis Toolbox* 高阶谱估计* 信号中非线性特征的检测和刻画* 延时估计* 幅值和相位重构* 阵列信号处理* 谐波重构(7)图像处理工具箱(Image Processing Toolbox)。
model-based control 和 model predictive control -回复

model-based control 和model predictivecontrol -回复Model-based control和Model Predictive Control(MPC)是现代控制理论中两个重要的概念。
它们都基于系统的模型来实现对系统的控制,并在工业应用中得到广泛应用。
本文将逐步介绍Model-based control 和MPC的概念、原理、优缺点以及其在不同领域的应用。
一、Model-based control(基于模型的控制)Model-based control是一种基于系统模型来进行控制的方法。
它的基本思想是通过建立系统的数学模型,预测系统的响应,并根据预测结果实现控制。
Model-based control可以分为开环控制和闭环控制两种方式。
1. 开环控制:开环控制是指控制器输出信号仅根据输入信号进行决策,不考虑系统的状态或输出。
在开环控制中,系统的模型被用来预测系统的输出,并根据预测结果调整控制器的输出信号。
开环控制的一个典型应用是步进电机的控制,其中控制器根据输入信号控制电机的步进角度。
2. 闭环控制:闭环控制是指控制器输出信号不仅依赖于输入信号,还依赖于当前系统的状态或输出。
在闭环控制中,系统的模型被用来预测系统的响应,并根据响应调整控制器的输出信号。
闭环控制的一个典型应用是温度控制,其中控制器根据当前温度和设定值之间的误差来调整加热器的输出功率。
Model-based control的优点是可以提供对系统行为的准确预测,并且可以根据预测结果进行优化控制。
然而,它的缺点是对系统模型的准确性要求较高,且对于复杂系统的模型建立较为困难。
此外,Model-based control往往是一种离线计算的方法,所以在实时控制中可能需要进行一定的近似或简化。
二、Model Predictive Control(模型预测控制)Model Predictive Control是一种基于系统模型的高级控制方法。
model-based control 和 model predictive control -回复

model-based control 和model predictivecontrol -回复model-based control和model predictive control是两种常见的控制方法。
本文将分析并比较这两种方法的原理、优缺点以及适用范围,以帮助读者更好地理解它们。
一、Model-based Control(基于模型的控制)1. 基本原理:基于模型的控制是一种控制方法,通过建立系统模型来描述物理过程,并利用模型进行控制。
首先,需要获得对被控制过程的准确数学描述,然后基于该模型设计控制器。
控制器根据当前的状态变量和预期的控制目标,计算出控制指令,从而实现对系统的控制。
2. 优点:a) 可以得到系统的全局最优解。
由于基于数学模型进行设计和仿真,理论上可以找到系统的最佳控制策略。
b) 可以处理复杂的系统。
通过合适的模型和控制算法,可以控制具有多个变量和非线性动态的系统。
c)可以进行系统故障检测和故障排除。
通过模型预测,可以提前检测系统故障并采取相应措施。
3. 缺点:a) 对系统模型要求高。
需要对被控制的系统进行准确地数学建模,如果模型不准确,可能导致控制器性能下降甚至系统不稳定。
b) 难以处理系统模型的不确定性。
当系统模型存在未知的不确定性时,基于模型的控制方法可能无法很好地适应。
c) 需要较多的计算资源。
为了进行系统建模和控制计算,通常需要较大的计算能力。
二、Model Predictive Control(模型预测控制)1. 基本原理:模型预测控制是一种基于动态系统模型的控制方法,它在每个时间步长上通过对未来状态的预测来计算控制指令。
首先,需要建立系统的数学模型,并使用该模型预测未来一段时间内的系统行为。
然后,通过求解一个优化问题,选择最优的控制指令,最小化控制误差和一些性能指标。
2. 优点:a) 可以处理约束条件。
通过在优化问题中加入约束条件,模型预测控制可以有效地处理系统输入和状态的限制。
预测控制
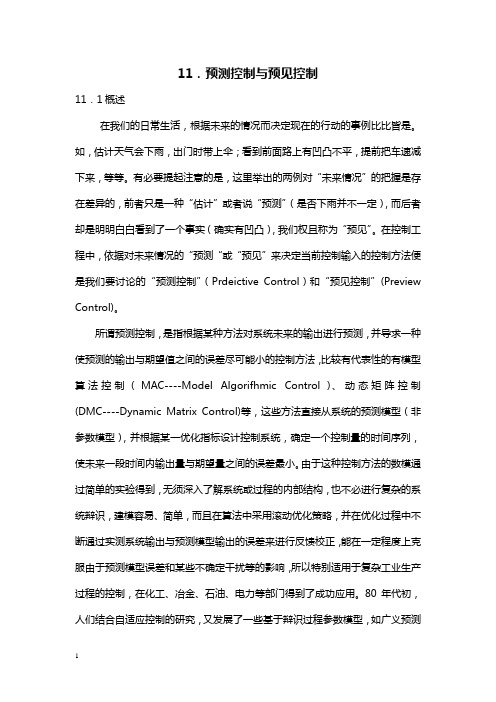
11.预测控制与预见控制11.1概述在我们的日常生活,根据未来的情况而决定现在的行动的事例比比皆是。
如,估计天气会下雨,出门时带上伞;看到前面路上有凹凸不平,提前把车速减下来,等等。
有必要提起注意的是,这里举出的两例对“未来情况”的把握是存在差异的,前者只是一种“估计”或者说“预测”(是否下雨并不一定),而后者却是明明白白看到了一个事实(确实有凹凸),我们权且称为“预见”。
在控制工程中,依据对未来情况的“预测“或“预见”来决定当前控制输入的控制方法便是我们要讨论的“预测控制”(Prdeictive Control)和“预见控制”(Preview Control)。
所谓预测控制,是指根据某种方法对系统未来的输出进行预测,并导求一种使预测的输出与期望值之间的误差尽可能小的控制方法,比较有代表性的有模型算法控制(MAC----Model Algorifhmic Control)、动态矩阵控制(DMC----Dynamic Matrix Control)等,这些方法直接从系统的预测模型(非参数模型),并根据某一优化指标设计控制系统,确定一个控制量的时间序列,使未来一段时间内输出量与期望量之间的误差最小。
由于这种控制方法的数模通过简单的实验得到,无须深入了解系统或过程的内部结构,也不必进行复杂的系统辩识,建模容易、简单,而且在算法中采用滚动优化策略,并在优化过程中不断通过实测系统输出与预测模型输出的误差来进行反馈校正,能在一定程度上克服由于预测模型误差和某些不确定干扰等的影响,所以特别适用于复杂工业生产过程的控制,在化工、冶金、石油、电力等部门得到了成功应用。
80年代初,人们结合自适应控制的研究,又发展了一些基于辩识过程参数模型,如广义预测控制(GPC Generalized Predictive Control)等。
与预测控制不同的是,预见控制对未来情报的了解和掌握不是基于某种推测或判断,预见控制是基于一个控制对象的模型是已知的,系统中未来的目标值或外扰是可以通过某种手段预先明确地得知和确定的假设,也就是说,预见控制是根据已经确认的系统未来应该满足什么要求,系统未来的目标信号或外部干扰将会有怎样的变化等信息来做出当前时刻的控制决策。
MATLAB 和COMSOL 介绍

Matlab的程序设计
利用MATLAB的命令窗口,可以完成比较简单的运算,但当遇到较为复 杂的问题时,就需要借助程序设计。 一、程序设计的基本原则 1、设置完整的路径,最好把当前的处理位置定为Current Directory。 2、参数值要集中放在程序的开始部分,便于程序维护。 3、MATLAB的每条命令后,若为逗号或无标点符号,则显示命令的结 果;若命令后为分号,则禁止显示结果。 4、“%” 后面所有文字为注释,“…”表示续行。 5、程序尽量模块化,也就是采用主程序调用子程序的方法,将所有子 程序合并在一起来执行全部的操作。
构造矩阵的 3、矩阵函数 矩阵计算的 四、算术运算符
zeros, ones, eye, rand, diag, triu, tril, … size, det, rank, inv, eig, trace, norm, …
矩阵的输入和运算
一、矩阵的输入 1、一维矩阵的输入 (1)逐个输入法:如果数据元素之间均用空格(或逗号)隔开,该向量称 为行向量;如果数据元素之间均用分号隔开,该向量称为列向量。 例:x=[2 3 sqrt(3) 5] (2)冒号生成法:x=first:increment:last,表示包含由first开始,步长 为increment,到last结束的整数组成的行向量。 例:x=0:pi/4:pi (3)利用内部函数:x=linspace(first,last,n),创建从first开始,到 last结束,有n个元素的均匀间隔的行向量。 例:x=linspace(0,pi,5)
2、多维矩阵的输入 多维矩阵必须有以下三个要素:整个输入矩阵必须以“[ ]”为其首尾; 矩阵的行与行之间必须用分号“;”或回车键(Enter)隔离;矩阵元 素必须由逗号“,”或空格分隔。 例:输入A=[1,2,3;4,5,6;7,8,9] 3、特殊矩阵的函数生成 zeros(m,n) ~ m×n全0阵 ones(m,n) ~ m×n全1阵 二、矩阵元素的操作 1、取矩阵中的一个元素:x(a,b)其中a,b分别代表元素的行和列。 2、取矩阵中的一行或一列:x(a,:),x(:,b)。 3、取矩阵A的第i1~i2行、第j1~j2列构成新矩阵:A(i1:i2,j1:j2)。 eye(m,n) ~ m×n对角线1矩阵 rand(m,n) ~ m×n (0,1) 随机阵
MATLAB中常用英文词汇解释

bold 粗体italic 斜体comment 注释评论意见decrease indent 减少缩进increase indent 增加缩进smart indent 敏捷缩进巧妙缩进reverse 颠倒case (语法)格preformat 预先格式cursor 指针alphabetize 依字母顺序排列以字母表示cascade 层叠patent 专利wrap 覆盖遮蔽隐藏previous 在…以前stack 堆叠toolboxs 工具箱plot tools 画图工具箱time series tools 时间序列工具箱phylogenetic tree 系统发生树,动植物种类史的树curve fitting toolbox 曲线拟合工具箱dfittool 拟合工具箱data acquisition toolbox 数据获得工具箱distributed computing toolbox 分布式计算工具箱fuzzy logic toolbox 模糊逻辑工具箱image acquisition toolbox 图像获得工具箱image processing toolbox 图像处理工具箱mapping toolbox 地图工具箱model predictive control toolbox 模型预测控制工具箱model-based calibration toolbox 基于模型校准工具箱neural network toolbox 神经网络工具箱optimization toolbox 最优化工具箱partial differential equation toolbox 偏最小二乘法工具箱RF Toolbox 射频(无线电频率)工具箱(radio freqency) robust control toolbox 强控制工具箱signal processing toolbox 信号处理工具箱pline toolbox 样条工具箱statistics toolbox 统计工具箱symbolic math toolbox 符号数学工具箱system identification toolbox 系统辨识工具箱virtual reality toolbox 虚拟现实工具箱wavelet toolbox 小波工具箱algorithm 算法statistics toolbox中的distribution fitting tool (dfittool)分布拟合工具箱polynomial fitting tool (polytool)多元拟合工具箱nonlinear fitting tool (nlintool)非线性拟合工具箱analysis of covariance tool (aoctool)协方差分析工具箱。
- 1、下载文档前请自行甄别文档内容的完整性,平台不提供额外的编辑、内容补充、找答案等附加服务。
- 2、"仅部分预览"的文档,不可在线预览部分如存在完整性等问题,可反馈申请退款(可完整预览的文档不适用该条件!)。
- 3、如文档侵犯您的权益,请联系客服反馈,我们会尽快为您处理(人工客服工作时间:9:00-18:30)。
Using one of the two blocks available in Model Predictive Control Toolbox to design and simulate a controller directly in Simulink.Working with Model Predictive Control ToolboxModel Predictive Control Toolbox uses the Control and Estimation Tools Manager, a GUI that organizes your controller development into projects, enabling you to manage the design and evaluation of multiple controllers.The Control and Estimation Tools Manager simplifies the tasks of importing plant models and previously designed controllers and defining plant inputs and outputs, their units, and their nominal values. It shows your controller structure in one view by indicating the number of set points, manipulated variables, disturbances, and measured and unmeasured outputs.With the Control and Estimation Tools Manager, you can:▪Define internal plant models used in calculating future control actions▪Design a model predictive controller▪Simulate the closed-loop behavior of the controller with linear modelsGetting Started with Model Predictive Control Toolbox9:59Defining Internal Plant ModelsModel predictive controllers base their control actions on an internal plant model of the process. The internal model lets the controller forecast future process behavior and respect output constraints. The ability to model process interactions makes model predictive control easier to maintain and often better performing than multiple proportional-integral-derivative (PID) control loops, which require individual tuning and other techniques to reduce loop coupling.Model Predictive Control Toolbox uses linear time invariant (LTI) models, enabling you to use transfer function model structures common to all MathWorks control system design products. You can import multiple LTI models into the toolbox from the MATLAB workspace or a MAT-file. The toolbox also lets you directly import multiple models estimated in System Identification Toolbox.Using Simulink Control Design™ and Simulink, you can extract a linearized form of the Simulink model that is automatically imported as the internal plant model of the controller.Plant Model Importer for bringing a model into the toolbox either from the MATLAB workspace or a MAT-file.Designing ControllersThe toolbox lets you design controllers in MATLAB or in Simulink.Designing Controllers in MATLABYou can design multiple controllers and use simulation to determine the optimal design. For each controller design, you can select a plant model and specify the following controller parameters:Prediction and control horizons▪Constraints on the manipulated and output variables▪Weighting factors on input and output variables▪Models for measurement noise and for unmeasured input and output disturbancesThe toolbox supports time-varying constraints and weights, off-diagonal weights, and custom unmeasured disturbance models.Designing Controllers in SimulinkModel Predictive Control Toolbox, when used with Simulink Control Design, can generate a controller directly in a Simulink model. Using an MPC block and the appropriately connected block inputs and outputs, Simulink Control Design can extract a linearized plant model and generate a controller. Model Predictive Control Toolbox uses the same GUI to specify the controller parameters in Simulink as to design a controller in MATLAB.Using Tuning Adviser for Designing Model Predictive Controllers7:23You can use the Multiple MPC Controllers block for controlling a nonlinear Simulink model over a wide range of operating conditions. With this block you can design a model predictive controller for each operating point and switch between model predictive controllers at run time. The Multiple MPC Controllers block ensures bumpless control transfer from one model predictive controller to another. You can create linear plant models for controller design at each operating point either by linearizing a Simulink model with Simulink Control Design or by specifying the plant model directly.Multiple MPC Controllers block (red) for controlling nonlinear models over a wide operating range using multiple model predictive controllers with bumpless control transfer. With this block you can design a model predictive controller for each operating point and switch between model predictive controllers at run time.Simulating Closed-Loop BehaviorYou can simulate your controller in MATLAB or Simulink to evaluate its performance.Simulating in MATLABYou can use MATLAB functions or the Control and Estimation Tools Manager to run closed-loop simulations of your model predictive controller against linear plant models. The Control and Estimation Tools Manager lets you set up multiple simulation scenarios. For each scenario you can input controller set points and unmeasured disturbances from the following signal profiles:▪Constant▪Step▪Pulse▪Ramp▪Sine▪GaussianConfiguring and running a simulation to test a controller using the Control and Estimation Tools Manager.You can compare controller and plant model configurations to judge the effects of model mismatch and different weighting factors on constraints and variables. Constraints can be disabled to evaluate the characteristics of the closed-loop dynamics, such as stability and damping.Simulating in SimulinkYou can use Simulink blocks provided with Model Predictive Control Toolbox to run closed-loop simulation ofyour model predictive controller against a nonlinear Simulink model.Setting constraints on manipulated and output variables with the Control and Estimation Tools Manager.Deploying Model Predictive ControllersOnline System Identification and Control Using OPC Communication18:06The toolbox provides two ways to deploy a controller in an application. You can use Simulink Coder to generate C code from Simulink blocks provided with Model Predictive Control Toolbox and deploy the code to a target system for implementation or rapid prototyping. (For a list of supported targets, see the section “Using Model Predictive Control Toolbox with Simulink Coder” in the product documentation.) Using OPC Toolbox™, you can connect a controller operating in MATLAB directly to an OPC-compliant system.Product Details, Demos, and System Requirements/products/mpcTrial Software/trialrequestSales/contactsalesTechnical Support/support Rapid prototyping of a model predictive controller on PC-compatible hardware using Simulink Coder and xPC Target.ResourcesOnline User Community /matlabcentral Training Services /training Third-Party Products and Services /connections Worldwide Contacts /contact。