人脸漫画 Human facial illustrations- Creation and psychophysical evaluation
夸张有趣的脸
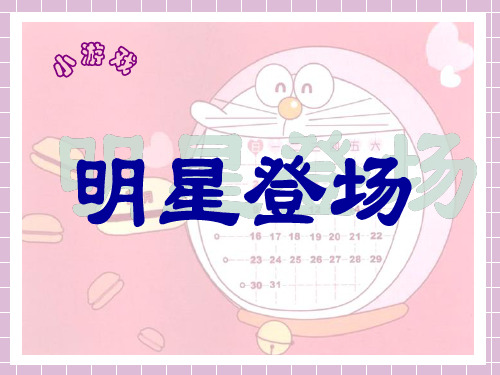
漫画
定义:用简单而夸张的手法来描绘生 活或时事的图画,一般运用变形、比 拟、象征的方法,构成幽默、诙谐的 画面,以取得讽刺或歌颂的效果。
可夸张之处:
◎脸型特点
◎五官特点 ◎外在特点
◎脸型特点
古人说“相之大概,不外八 格”
并总结以八个字形为代表
甲由申田,目国用风
国字脸: 方额头、方下巴,脸较宽。
甲字脸:脸呈倒三角形,额头显得大脸颊瘦,下巴尖
申字脸:颧骨较高,下巴尖瘦。呈枣核形。
◎五官特点
五官包括
耳、眉、眼、鼻、口
耳朵
眼睛、嘴巴
鼻子
手势
◎外在特点
一般指后天所具有的与他
人的不同之处。
眼镜
头发
帽子
发 田 型 字 脸 嘴 眉 巴 毛
画一幅
肖像小漫画
观察你周围的朋友、同 学、老师等,给他们画 一幅肖像小漫画。
人脸漫画生成技术研究进展
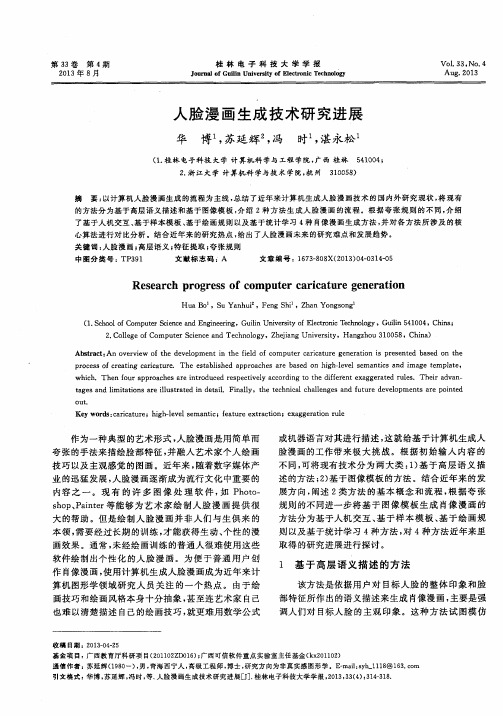
Ke y wo r d s : c a r i c a t u r e ;h i g h — l e v e l s e ma n t i c ;f e a t u r e e x t r a c t i o n ;e x a g g e r a t i o n r u l e
Ab s t r a c t : An o v e r v i e w o f t h e d e v e l o p me n t i n t h e f i e l d o f c o mp u t e r c a r i c a t u r e g e n e r a t i o n i s p r e s e n t e d b a s e d o n t h e p r o c e s s o f c r e a t i n g c a r i c a t u r e . Th e e s t a b l i s h e d a p p r o a c h e s a r e b a s e d o n h i g h — l e v e l s e ma n t i c s a n d i ma g e t e mp l a t e ,
作为一 种典 型 的艺术 形式 , 人脸 漫画是 用简 单而
夸 张的手法来 描绘 脸部特 征 , 并 融入 艺术家 个人绘 画 技 巧以及 主观感 觉 的图画 。近年来 , 随着数 字媒 体产
Hu a B o ,S u Ya n h u i 。 ,Fe n g S h i ,Z h a n Yo n g s o n g
( 1 . S c h o o l o f C o mp u t e r S c i e n c e a n d E n g i n e e r i n g ,Gu i l i n Un i v e r s i t y o f E l e c t on r i c T e c h n o l o g y,Gu i l i n 5 4 1 0 0 4 ,Ch i n a ;
Illustrator创造独特卡通人物教程
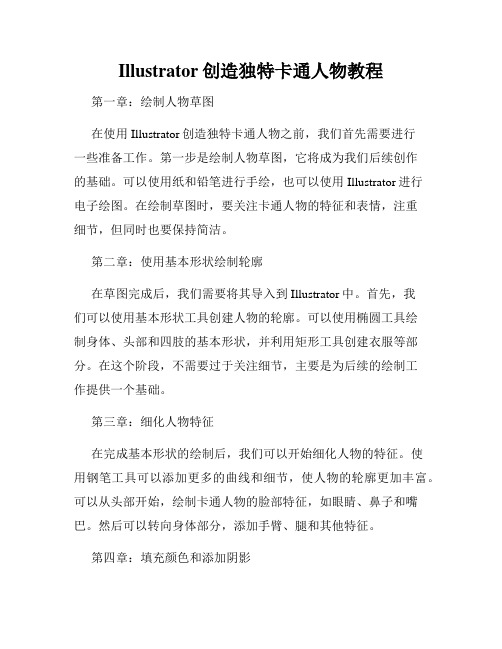
Illustrator创造独特卡通人物教程第一章:绘制人物草图在使用Illustrator创造独特卡通人物之前,我们首先需要进行一些准备工作。
第一步是绘制人物草图,它将成为我们后续创作的基础。
可以使用纸和铅笔进行手绘,也可以使用Illustrator进行电子绘图。
在绘制草图时,要关注卡通人物的特征和表情,注重细节,但同时也要保持简洁。
第二章:使用基本形状绘制轮廓在草图完成后,我们需要将其导入到Illustrator中。
首先,我们可以使用基本形状工具创建人物的轮廓。
可以使用椭圆工具绘制身体、头部和四肢的基本形状,并利用矩形工具创建衣服等部分。
在这个阶段,不需要过于关注细节,主要是为后续的绘制工作提供一个基础。
第三章:细化人物特征在完成基本形状的绘制后,我们可以开始细化人物的特征。
使用钢笔工具可以添加更多的曲线和细节,使人物的轮廓更加丰富。
可以从头部开始,绘制卡通人物的脸部特征,如眼睛、鼻子和嘴巴。
然后可以转向身体部分,添加手臂、腿和其他特征。
第四章:填充颜色和添加阴影一旦人物的轮廓完成,我们可以开始填充颜色。
选择合适的填充颜色工具,如颜色面板或取色器,为人物的各个部分选择适当的颜色。
可以使用渐变填充和图案填充等效果,使人物更加立体和有趣。
此外,还可以添加阴影效果,使人物看起来更加真实。
使用渐变工具和透明度设置可以轻松实现这一点。
第五章:绘制服装和配饰卡通人物的服装和配饰是为其增添个性和特色的重要组成部分。
在Illustrator中,可以使用形状工具和钢笔工具来绘制衣服的各个部分,如上衣、裤子和鞋子。
通过添加颜色和纹理,可以使服装更加生动。
此外,还可以使用新的图层和绘制工具,如毛刷工具,添加一些小饰品和装饰品,如帽子、眼镜和项链。
第六章:创造表情和动作卡通人物的表情和动作是为其赋予情感和生命力的关键因素。
在Illustrator中,可以使用形状工具和路径工具绘制卡通人物的眼睛、眉毛、嘴巴等部分,并通过移动和变形这些元素来创造不同的表情。
【美术教材】Q版漫画人物教程步骤-3头身练习(和服面具少女)
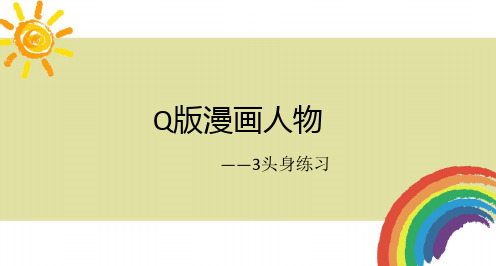
⑥后面就是选取颜色搭配上色
最后可以添加一个场景 给它。
谢谢观赏
①首先绘制三个相同的 圆。
②在下面的圆上画出表 示胸部中心,腰部和胯 部的辅助线。
③根据辅助线描绘人物 的轮廓大概画出躯干、 胯部和腿部
注意在画手和脚的时候, 可以画出细节,不过外 轮廓线要画得比写实人 物胖些,这样看起来才 够可爱。多细节。
Q版漫画人物
——3头身练习
3头身人物练习
由于3头身人物身体的平衡度较好, 头、躯干、腿的比例大约是1:1: 1,因此3头身人物在Q版中最常见。
3头身人物的躯干明显由上下两 个半身组成,有点趋向于现实 人物,但又比现实人物可爱。
今天学习的人物
3头身的Q版角色
Q版3头身人物的身体
①首先绘制三个相同的圆。 ②在下面的圆上画出表示胸 部中心,腰部和胯部的辅助 线。 ③根据刚才的辅助线,大概 画出躯干、胯部和腿部。 ④绘制3头身人物,可以想 象成在雪人身上添加手和脚, 画起来会容易一些。一般来 说,腿的长度和身体的长度 相同。
如何画出不同年龄段人物的脸(上)
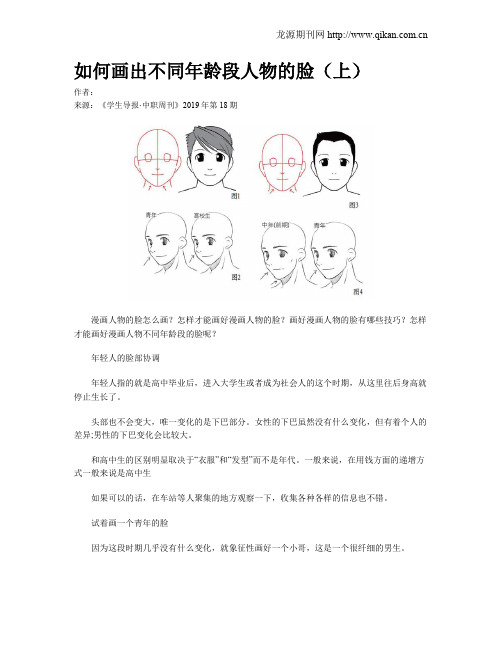
龙源期刊网
如何画出不同年龄段人物的脸(上)
作者:
来源:《学生导报·中职周刊》2019年第18期
漫画人物的脸怎么画?怎样才能画好漫画人物的脸?画好漫画人物的脸有哪些技巧?怎样才能画好漫画人物不同年龄段的脸呢?
年轻人的脸部协调
年轻人指的就是高中毕业后,进入大学生或者成为社会人的这个时期,从这里往后身高就停止生长了。
头部也不会变大,唯一变化的是下巴部分。
女性的下巴虽然没有什么变化,但有着个人的差异;男性的下巴变化会比较大。
和高中生的区别明显取决于“衣服”和“发型”而不是年代。
一般来说,在用钱方面的递增方式一般来说是高中生
如果可以的话,在车站等人聚集的地方观察一下,收集各种各样的信息也不错。
试着画一个青年的脸
因为这段时期几乎没有什么变化,就象征性画好一个小哥,这是一个很纤细的男生。
一些简单的人物面部漫画入门篇
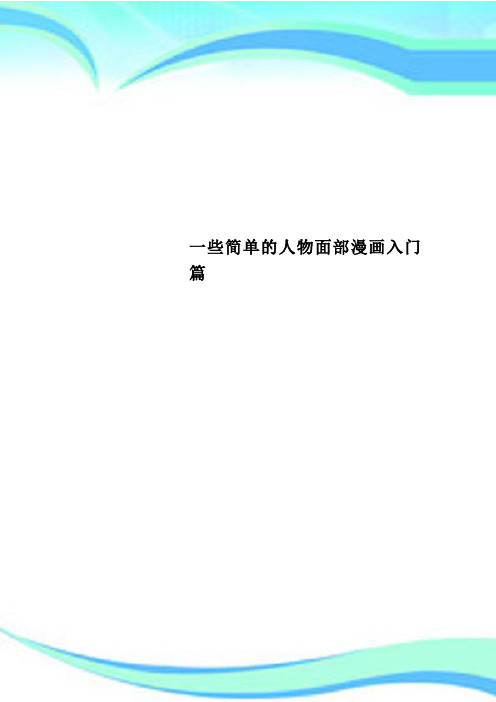
在虹膜的余下部分画好眉毛(?)及阴影。记得在高光下面画好瞳孔,并且无论你把眼珠的其它部分画得多暗,相比之下瞳孔都要稍微突出一些。
你可以使用相同的方法画出这些不同风格的女性眼睛。试着去观察每种风格的区别,其实都是大同小异的。虽然形状和比例有所改变,眼睛最高的边缘总是比较厚;眼珠上总是画出多层的阴影,等等。虽然这些有的确实是素描速写稿,看来有点散乱,不过希望这对大家仍然有所帮助。
ﻫ轻轻画两条对角辅助线,以助于定义下眼框,辅助线从上眼框的两边出发,彼此几乎成垂直。不要画得太斜或太平直了,否则眼睛的尺寸就不那么准确了。画出眼睛的下眼框线,通过辅助线帮助你确定其位置。
擦掉辅助线并画出虹膜。虹膜是一个完美的圆形, 但一部分会被眼皮遮住 。眼球别画太小,至少要能看见完整的物体。(除非要表达强烈的情绪,比如:惊奇或忿怒, 请参考教程《情绪篇》的内容)
下一步,画出眼珠高光的轮廓线。通常漫画人物的眼睛会有一些阴影。漫画中女 孩一般也都具有明显的阴影和光泽。确立你画中选择的光源,并且在整个画面中都是始终不变的。举例来说,如果在一张画中光线是从左边照射的,那么画面其余部分的高光区也必须源于左边,否则光线所产生的明暗关系就不协调了(除非你使用了多个光源,不过本教材不涉及此范围)。画两个长椭圆形: 大的椭圆形在虹膜(眼珠)的左上部(与虹膜的边缘重叠,如图所示),很小的那个在眼睛的另一边。
一些简单的人物面部漫画入门篇
———————————————————————————————— 作者:
———————————————————————————————— 日期:
ﻩ
日式漫画人物速成指南-女性的眼睛
第二课《画人像》(教学设计)人美版(2023)美术五年级上册
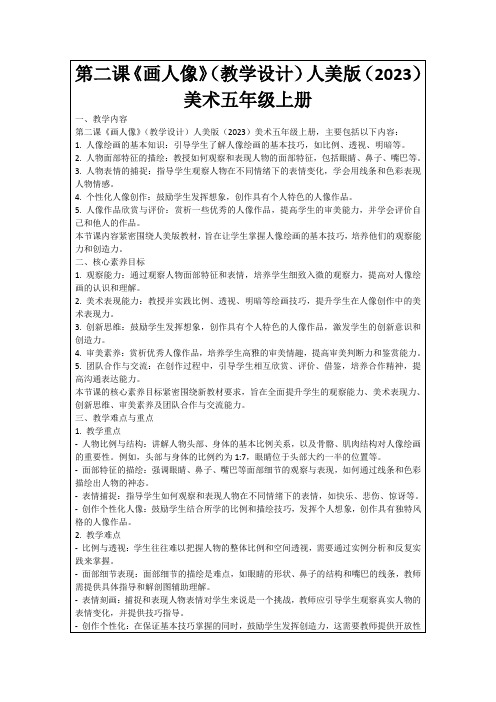
-表情捕捉:指导学生如何观察和表现人物在不同情绪下的表情,如快乐、悲伤、惊讶等。
-创作个性化人像:鼓励学生结合所学的比例和描绘技巧,发挥个人想象,创作具有独特风格的人像作品。
2.教学难点
-比例与透视:学生往往难以把握人物的整体比例和空间透视,需要通过实例分析和反复实践来掌握。
四、教学流程
(一)导入新课(用时5分钟)
同学们,今天我们将要学习的是《画人像》这一章节。在开始之前,我想先问大家一个问题:“你们在日常生活中是否注意观察过身边人的面部特征和表情?”这个问题与我们将要学习的内容密切相关。通过这个问题,我希望能够引起大家的兴趣和好奇心,让我们一同探索人像绘画的奥秘。
(二)新课讲授(用时10分钟)
3.人物表情的捕捉:指导学生观察人物在不同情绪下的表情变化,学会用线条和色彩表现人物情感。
4.个性化人像创作:鼓励学生发挥想象,创作具有个人特色的人像作品。
5.人像作品欣赏与评价:赏析一些优秀的人像作品,提高学生的审美能力,并学会评价自己和他人的作品。
本节课内容紧密围绕人美版教材,旨在让学生掌握人像绘画的基本技巧,培养他们的观察能力和创造力。
在教学过程中,教师应针对以上重点和难点内容,采用以下方法:
-示范讲解:教师现场示范人物绘画过程,强调重点和难点,让学生直观感受技巧的应用。
-互动讨论:鼓励学生在课堂上分享自己的观察和创作经验,通过讨论解决共同遇到的难题。
-个性化指导:针对不同学生的特点和问题,给予个性化指导,帮助他们突破个人难点。
-多元评价:设置多角度的评价标准,不仅关注绘画技巧,也重视学生的创意和努力程度,以激发学生的学习积极性。
漫画风格的人脸肖像生成算法

第19卷第4期2007年4月计算机辅助设计与图形学学报J OURNAL O F COM PUTER -A I DED DES I GN &COM PUTER GRA PH I CSV o l.19,N o.4A p r.,2007修回日期:2006-10-19.基金项目:国家自然科学基金(60403037).阎芳,女,1982年生,硕士研究生,主要研究方向为非真实感图形学.费广正,男,1973年生,博士,副研究员,硕士生导师,主要研究方向为计算机动画、运动编辑与合成、非真实感绘制、纹理映射与合成.柳婷婷,女,1983年生,硕士研究生,主要研究方向为非真实感图形学.马文慧,女,1982年生,硕士研究生,主要研究方向为非真实感图形学.石民勇,男,1962年生,博士,研究员,博士生导师,主要研究方向为计算机动画、图论.漫画风格的人脸肖像生成算法阎芳1)费广正2)柳婷婷1)马文慧1)石民勇2)1)(中国传媒大学计算机与软件学院北京100024)2)(中国传媒大学动画学院北京100024)(Wna g a !m )摘要为模拟艺术家画出的具有夸张效果的肖像漫画,由用户输入正面人脸照片,通过交互获取关键特征点,根据特征点计算面部特征值来判断需要夸张的部分和各自的夸张方式;然后根据特征点自动生成不同层级的网格并分层实施夸张变形;最后进行图像处理以获得不同艺术风格的图像.该算法从研究漫画家的作画风格入手,总结了肖像漫画特定的夸张规律,所生成的漫画风格人脸肖像效果较好,能够应用于非真实感图形学和数字娱乐等领域.关键词夸张;漫画风格;肖像;网格变形中图法分类号T P391A g eneration A l g orit h m of c aricat ure PortraitY an F an g 1)F eiG uan g zhen g 2)L i u T i n g ti n g 1)M a w enhui 1)S hi M i n y on g 2)1)(C o m P uter &s o f t z are s chool ,C o mm unication uniuersit y o f C hina ,B ei J in g 100024)2)(A ni m ation s chool ,C o mm unication uniuersit y o f C hina ,B ei J in g100024)AbstractT his p a p er i ntroduces a ne W f acial caricat uri n g m et hod f or 2D cartoon p ortraits W it hexa gg eration to si m ulate t he W orks o f t he artists.B y t he m et hod ,firstl y ,a p hoto o f front f ace i n p uts and its characteristic p o i nts are s p ecified b y users.A ccordi n g to t he characteristic val ues calculated based on t he characteristic p o i nts ,t he p ro p er p arts o f t he f ace de m andi n g def or m ation and t heir res p ecti ve W a y s o f exa gg eration are deci ded.T hen t he m esh m odels o f diff erent la y ers are autom aticall y g enerated based on t he characteristic p o i nts and exa gg erati ve def or m ations are carried out la y er b y la y er.A t last ,t he i m a g e is m ani p ulated to g et diff erent st y les.T he st ud y o f t he arit h m etic starts fromt he research on t he st y les o f t he artist W orks ,and su mm arizes t he exa gg eration rules o f cartoon p ortraits.T his a pp roach can hel p g enerate relati vel y g ood cartoon p ortraits and can be used i n unrealistic com p uter g ra p hics and di g ital entertai n m ent.K e y words exa gg eration ;cartoon st y le ;p ortraits ;m esh m eta m or p hosis 近年来,在艺术工作程序自动化方面已有许多研究,其中包括以图像为基础的处理方法:由计算机模拟画具(如铅笔、油画笔等)的笔触,从而生成铅笔画、油画等.但这些方法没有夸张,图像趋于真实.还有以特征为基础的影像处理方法[1].B rennan 在1982年提出了一套以互动方式产生夸张肖像画的系统[2].T om i na g a 等提出以模板为基础的夸张肖像画系统P I CA SSO ,但该方法的笔调生硬不自然[3].陈洪等提出基于学习样本的人脸线条画生成系统,让计算机从大量的训练资料中学习画家的风格及夸张技巧,通过采用非参数化采样方法和灵活的线条画模板,生成效果较好的人脸线条画[4]!!!!!!!!!!!!!!!!!!!!!!!!!!!!!!!!!!!!!!!!!!!!!!!!!!!!!!!!!!!!!!!;但是其夸张效果有限,过于写实,而且每学习一种画家风格就需要由一位画家绘制大量的训练资料,费工费时.江佩颖等提出了以脸部特征为基础的肖像漫画产生系统,只需要少量的计算时间和单张的画家作品就可绘制出具有该画家风格的夸张肖像漫画[5];但由于其夸张方式不合适,效果差强人意.本文通过研究漫画家夸张的作画风格,分析出肖像漫画的夸张规律:漫画家夸张的仅仅是人脸的少数显著特征,并且使用了不同的夸张手法.人的面部特征主要包括脸型、五官尺寸等.将照片输入后,面部特征数值可通过交互拾取的少数关键特征点的坐标进行计算.本文算法将人脸正面照片经过艺术化的夸张和各种风格的图像处理后,绘制结果接近漫画家手绘风格,用户交互仅包括拾取关键特征点和选择绘制风格.!特征数值计算和显著特征的判断文献[5]中定出了119个脸部特征点以及由所有特征点构成的8个群组,并以群组作为分析对比的单位,逐个特征点与平均脸相应特征点比较,找出各项差异值.在差异值超过正常范围时加以夸张,夸张的程度与差异度成正比.但是,平均人脸的计算需要大量的资料,计算复杂,而且特征点的各项差异值不一定能反映人脸的视觉特征.该方法对超过差异值正常范围的部分都进行夸张变形,容易改变人脸的所有特征而失去面部整体的个性特征.经过观察和学习漫画家的作画过程[6],对比肖像漫画和照片,本文分析出其中特定的夸张规律和手法.针对亚洲人面部的特点并结合美术学常识,本文采用中国美学的标准人脸作为对比标准,以特征比例和标准人脸相应比例的差异值作为判断是否夸张变形的标准.漫画家对面部的变形夸张主要分为2个方面:整体效果的变形(如脸型、三庭等)和局部特征的变形(如眼睛、鼻子、嘴巴).变形的判断依据是特定人脸与标准人脸相应的特征比例的差异值.假如对全部特征都进行不同程度的夸张变形,将会完全改变一个人的所有特征而失去其原貌和神韵,所以漫画家一般针对其中显著的特征进行夸大.面部的特征主要包括脸型、三庭的长度、五官的位置和尺寸,而不同人脸视觉特征的区别主要取决于各个特征数值间的比例关系,而非数值本身.首先定义面部特征,计算特征数值及其比例,然后把所得特征比例与标准人脸的相应比例进行比较.脸型取决于脸的长宽比,决定了面部的整体视觉效果.三庭是中国画肖像的标准,指从前额中央发际线开始到下巴尖之间的距离,共分为3等分:从发际线到眉毛的距离为第一等分,即上庭;从眉毛到鼻端的距离为第二等分,即中庭;从鼻端到下巴的距离为第三等分,即下庭.在标准人脸中,三庭的比例为11111.一般人脸往往不符合标准比例.五官的位置基本取决于三庭的位置.本文算法首先考虑五官的整体区域占脸部面积的大小,这影响五官整体与脸部轮廓的关系.对面部特征有较大影响的器官是眼睛、鼻子、嘴巴,三者尺寸数值的比例关系比三者的数值本身更能反映一个人的特点.眼睛、鼻子、嘴巴的宽度在标准人脸中的比例为2121 3.标准人脸各项特征比例如表1所示.将特定人脸的相应比例与之比较,即可判断出五官中的一个或两个显著特征,从而对其进行夸张变形.表!标准人脸特征比例脸长1脸宽上庭1中庭1下庭人中1下庭眼宽1鼻宽1嘴宽5141111121311111.5图1算法流程图"算法流程本文算法的流程如图1所示.首先由用户选择绘制风格和交互标定关键特征点,进一步计算特征数值和特征比例,判断出需要夸张的显著特征,确定变形方式.根据变形方式不同,分2步变形:第一步是对脸型、三庭等整体特征的变形;第二步是对眼睛、鼻子、嘴巴等局部特征的变形.变形过程由程序自动进行,然后实施用户选择的绘制风格,进行图像处理后就得到具有夸张效果的人脸肖像漫画.3444期阎芳等:漫画风格的人脸肖像生成算法!算法步骤!"#图像预处理获取图像后进行图像预处理.首先对图像进行光线补偿,以抵消整个图像中存在的色彩偏差;然后增强图像的对比度,突出图像特征;最后把经过预处理的图像存储为纹理.!"$交互拾取关键特征点计算特征数值和特征比例在已有的卡通人脸生成系统中,大部分都需要大量的特征点.在基于样本的线条画生成系统[4]中,83个特征点自动定位,用于在不同人脸之间建立几何对应、几何结构变换和线条画模板.在以脸部特征为基础的肖像漫画产生系统[5]中,119个特征点用于构建群组化的脸部网格,并以群组作为分析对比以及夸张化的基本单位对网格进行变形[5].在S ato 等提出的算法中,60个特征点用于生成轮廓线[7].特征点越多,脸部和五官细节的轮廓线越能准确地表达一个人的外貌特征,但是大量的特征点影响了计算的效率.部分方法中特征点的交互标定给用户带来大量的工作;而部分使用A SM (acti vesha p e m odel )等算法对特征点自动定位的方法,事先需要经过大量的训练,费时费力.图2关键特征点在本文算法中,为了避免轮廓线不准确而影响面部特征,取消了轮廓线的绘制,所以不需要大量的特征点;又因为分层变形简化了网格,所以特征点仅用于特征比例的计算以及形成简单的面部网格.本系统仅需14个关键特征点,便于交互获取,而且这些关键特征点也可以由方向积分投影、边缘检测、角点提取等方法相结合自动生成,有利于系统以后的改进.特征数值通过关键特征点之间的坐标运算得到.用户通过简单的交互,在指定位置标记关键特征点.关键特征点的定义和交互过程如图2所示,步骤如下:S te p 1.选择发际线中点、眉心中点、鼻端中点和下巴中点,定出三庭的大体位置,计算三庭的长度和脸长.a 三庭特征点b 眼睛特征点c 鼻子和嘴巴特征点S te p 2.选择左眼外部眼角点、左眼内部眼角点、右眼内部眼角点、右眼外部眼角点、两眼中心点连线与脸部轮廓左边和右边的交点,计算出眼宽和脸宽.S te p 3.选择鼻翼最宽处左边和右边的端点、左嘴角点和右嘴角点,计算出鼻宽和嘴宽.通过面部各特征数值的计算,即可得出特征比例值,将其与标准人脸的相应比例进行比较,可对显著特征做出判断.但是,因为手工标记特征点并不准确,所以相应的特征值和比例值也不能精确地反映面部特征.我们在前期通过对同一幅照片的特征点多次标记,取得多个样本值.设置信水平为!,计算置信区间并划定置信上下限,从而可以得到特征数值和特征比例数值的取值范围,作为标准人脸各项比例的正常差异范围.!"!变形夸张3.3.1变形方式已有的夸张变形算法往往忽略了三庭的长度和脸型,这也是漫画家进行夸张的重要因素.文献[5]方法是在面部网格群组化后,改变了大量特征点的坐标.特征点被分为主导点、校正点和从随点3种.3种类型的点之间相互影响,定位过程比较复杂.为了简化变形的过程,本文把变形方式进行分类,并依据变形方式分步变形,以达到简化网格模型的目的.网格根据关键特征点自动生成,分层自动夸张变形,并考虑了三庭、脸型等的艺术化夸张.夸张变形的主要方式是拉伸压缩和鱼眼放大挤压.直观而言,面部整体特征(脸型、三庭、人中)的变形是通过拉伸或压缩的方式进行的,而局部特征(五官的整体分布、眼睛、鼻子、嘴巴)的变形是使用鱼眼放大或挤压的方式.根据夸张方式的不同,变形采用2种网格,分为2步进行.图3网格模型13.3.2第一步变形———脸型、三庭、人中的夸张为了便于脸部轮廓、三庭、人中的拉伸和压缩,网格模型1定义如图3所示.网格将根据关键特征点的坐标自动生成.444计算机辅助设计与图形学学报2007年通过计算人脸的长宽比可判断脸型.脸型主要分为长脸、宽脸和标准脸型.如果特定人脸长宽比在标准人脸长宽比的正常差异值范围内,则认为是标准脸型不予夸张;否则,根据长宽比判断为长脸或者宽脸.对于不同类型的脸型,可结合三庭的比例,采用不同方式的变形,再根据人中长度与下庭长度的比例判断是否夸张人中长度.对于脸部的长宽比在标准脸型比例的正常差异范围内的,面部长、宽不变,三庭根据长度比例夸张,即改变相应的网格点坐标.脸型为宽脸,则对脸部整体进行压扁,即纵向缩小.长脸的情况比较复杂,如果三庭符合标准比例,则对脸部纵向长方形均匀拉伸;如果上庭相对较长,则对面部纵向梯形(上底长)拉伸;如果下庭相对较长,则对面部纵向梯形(下底长)拉伸;如果中庭相对较长,则对面部“申”字形变形,即上庭纵向梯形(下底长)拉伸,中庭纵向长方形拉伸,下庭纵向梯形(上底长)拉伸.三庭拉伸的比例依据特定人脸的三庭比例.长脸的变型算法如下:float scale U P,scale m id,scale d o z n;!!上庭、中庭、下庭比例m eta P hoseL on g Face(){if三庭等长rect c han g e(scale U P,scale m id,scale d o z n);!!按三庭比例,纵向长方形拉伸e lse if上庭较长tra P eziac han g e1(scale U P,scale m id,scale d o z n);!!按三庭比例,纵向梯形(上底长)拉伸e lse if下庭较长tra P eziac han g e2(scale U P,scale m id,scale d o z n);!!按三庭比例,纵向梯形(下底长)拉伸e lse if中庭较长shenc han g e(scale U P,scale m id,scale d o z n);!!申字变形.上庭纵向梯形(上底长)拉伸,中庭纵向长方形拉伸,下庭纵向梯形(下底长)拉伸if人中长度需要调整chan g ePhiltru m();!!调整人中长度}三庭及人中的变形涉及的网格点如表2所示.表!图"变形涉及的网格点特征影响的网格点上庭2,3行网格点的间距中庭4,5行网格点的间距下庭7,8行网格点的间距人中6,7行网格点的间距网格点坐标的调整量为特定人脸与标准人脸的比例值之差与某随机值b的乘积,b的取值范围位于三庭平均值与脸长之间,使效果具有某种程度的随机性.第一步变形的部分示例如图4!8所示.图4宽脸及其变形图5标准脸型(下庭长)及其变形图6长脸(上庭长)及其变形图7长脸(中庭长)及其变形图8长脸(下庭长)及其变形3.3.3第二步变形———五官的夸张本文中用到的变形方法为鱼眼放大和挤压.根据对漫画家作品的观察,鱼眼放大的效果比以往采用的线性放大的效果更加理想.5444期阎芳等:漫画风格的人脸肖像生成算法首先考虑五官的整体效果,需要计算从眉心点到嘴巴中心点的距离与脸长的比例,即中庭长度与人中长度之和与脸长的比例.中国美学的标准人脸的相应比例为7i10,如果特定人脸的这项比例超过标准人脸相应比例的正常差异范围,则需要对五官整体进行鱼眼放大或缩小.然后考虑眼睛、鼻子和嘴巴的大小.之前已经通过对三者比例与标准比例的比较判断出显著特征,这里仅需夸大显著特征.s te p1.生成如图5所示的细密的方形网格.s te p2.然后对需要夸张的特征周围的网格点位置进行鱼眼变形.图9网格模型2肖像漫画一般会缩小脖子以下的部分,本文对嘴巴与下巴中心以下的特定区域的网格点进行挤压变形.鱼眼和挤压算法是对特定区域的网格点进行几何变形.首先预设影响五官整体,眼睛、鼻子和嘴巴各自的矩形区域,变形区域为矩形区域内的最大圆形区域,把网格点坐标由笛卡儿坐标系转换到极坐标系.设R是最大圆的半径;w是曲率,它取决于特征比例计算的差异度.鱼眼算法如下:ne w rad ius=s lO g(1+w(ol d rad ius));s=R!lO g(w!R+1);R=(m i n(w idt h,hei g ht))!2;!!w idt h,hei g ht为预设矩形的宽和高G et w;s=R!lO g(w!R+1);f Or ever y p O i nt i n t he m esh dO{P olar P oint=current P oint i n p O lar cOOrd i nates(rad ius,an g le);if(P olar P oint.r"R){P olar P oint.r=s!lO g(1+w!r);f ilter P oint=P olar P oint i n c artes ian cOOrd i nates;}e lsef ilter P oint=current P oint;!!圆形以外的点不需要变化}挤压算法类似,只是ne w rad ius=(e^(ol d rad ius!s)-1)!w);图10所示为将挤压算法作用于网格模型产生的五官整体挤压的变形效果.a第一步变形后b第二步变形:挤压图10五官整体所占比例小对网格模型使用挤压算法图11漫画风格肖像的生成结果经过对整体特征和局部特征的分步变形,绘制结果就是基本的夸张漫画效果.!"#影像绘制风格化经过夸张变形的人脸照片已经极具漫画效果,依据用户选择的绘制风格对图像进行处理,可实现油画笔、卡通着色等效果,从而得到不同绘制风格的漫画肖像.部分效果如图11所示,从左至右依次为照片、变形后的照片、某种绘制风格的漫画肖像.644计算机辅助设计与图形学学报2007年!结论本文通过分析夸张肖像漫画的作画规律,提出了基于分阶段变形的漫画风格的人脸肖像生成算法,可以根据用户给定的人脸照片,通过对关键特征点的交互标定自动生成具有夸张效果的正面人脸肖像漫画.本文算法仅需用户交互实现14个特征点的标定和绘制风格的选择,易于操作,并且生成效果具有一定的随机性,计算简单、效率较高.在深刻理解漫画作画规律的基础上,采用了合理的变形方式,分阶段变形夸张,取得了接近手绘的良好效果.下一步的工作包括用人脸识别的相应研究成果来自动生成关键特征点代替用户的交互;实现更多的图像绘制风格,以满足用户的个性化需求;研究如何生成任意姿态和角度的肖像漫画,以及结合侧面照片进一步改进夸张效果等.参考文献[1]B e ier T,N ee l y S,F eature-based i m a g e m eta m or p hos is[C]!!C om p uter G ra p h ics P roceed i n g s,A nnual C onf erence S eries,ACM S I GGRAPH,Ch ica g o,1992:35-42[2]B rennan S E.C aricature g enerator[D].C a m bri d g e,M A:M I T,1982[3]T om i na g a M,Fukuoka S,M uraka m i K,!"#$.F acial caricaturi n g W it h m o tion caricaturi n g i n P I CA S SO s y ste m[C]!!P roceed i n g s of t he I EEE!A SM E I nternational C onf erence onA dvanced I nte lli g ent M echatron ics’97,T ok y o,1997:30-37[4]Chen H on g,Zhen g N ann i n g,Xu Y i n gg i n g,!"#$.A n exa m p le-based f acial sketch g eneration s y ste m[J].Journal o f S o ft W are,2003,14(3):202-208(i n Ch i nese)(陈洪,郑南宁,徐迎庆,等.基于样本学习的人像线条画生成系统[J].软件学报,2003,14(3):202-208)[5]Jian g Pe i y i n g,L iao W enhon g,L i C ai y an.A utom atic caricatureg eneration b y anal y zi n g f acial f eatures[C]!!P roceed i n g s o f2004A s ia C onf erence on C om p uter V is ion,Je j u Is land,2004:89-94(i n Ch i nese)(江佩颖,廖文宏,李蔡彦.以脸部特征为基础的肖像画产生系统[C]!!亚洲计算机视觉会议论文集,Je j u Is land,2004:89-94)[6]R ed m an L enn.H oW to dra W caricatures[M].Ch ica g o:M c G ra W-H ill,1984[7]S ato M,S ai g o Y,H ash i m a K azuo,!"#$.A n autom atic f acial caricaturi n g m et hod f or2D realistic p ortraits us i n g characteristicp o i nts[OL].[2006-06-12].htt p:!!WWW.i de m p lo y ee.i d.tue.n l!g.W.m.rauterber g!conf erences!CD doN o t O p en!ADC!fi nalp a p er!309.p df7444期阎芳等:漫画风格的人脸肖像生成算法漫画风格的人脸肖像生成算法作者:阎芳, 费广正, 柳婷婷, 马文慧, 石民勇, Yan Fang, Fei Guangzheng, LiuTingting, Ma Wenhui, Shi Minyong作者单位:阎芳,柳婷婷,马文慧,Yan Fang,Liu Tingting,Ma Wenhui(中国传媒大学计算机与软件学院,北京,100024), 费广正,石民勇,Fei Guangzheng,Shi Minyong(中国传媒大学动画学院,北京,100024)刊名:计算机辅助设计与图形学学报英文刊名:JOURNAL OF COMPUTER-AIDED DESIGN & COMPUTER GRAPHICS年,卷(期):2007,19(4)被引用次数:3次1.Redman Lenn How to draw caricatures 19842.江佩颖;廖文宏;李蔡彦以脸部特征为基础的肖像画产生系统 20043.陈洪;郑南宁;徐迎庆基于样本学习的人像线条画生成系统[期刊论文]-软件学报 2003(03)4.Sato M;Saigo Y;Hashima Kazuo An automatic facial caricaturing method for 2D realistic portraits using characteristic points 20065.Tominaga M;Fukuoka S;Murakami K Facial caricaturing with motion caricaturing in PICASSO system 19976.Brennan S E Caricature generator 19827.Beier T;Neely S Feature-based image metamorphosis 19921.陈文娟.石民勇.孙庆杰利用人脸特征及其关系的漫画夸张与合成[期刊论文]-计算机辅助设计与图形学学报2010(1)2.陈文娟.石民勇.孙庆杰人脸漫画特征关系夸张及相像度优化[期刊论文]-计算机应用 2009(z2)3.陈文娟.石民勇.孙国玉.孙庆杰计算机肖像漫画方法综述[期刊论文]-计算机应用 2009(8)本文链接:/Periodical_jsjfzsjytxxxb200704007.aspx。
肖像漫画知识点总结

肖像漫画知识点总结一、肖像漫画的历史肖像漫画的历史可以追溯到古代的壁画和雕塑,人们通过绘画和雕刻来描绘和表现个人形象。
随着时间的推移,肖像艺术逐渐演变为肖像画和肖像雕塑,成为了艺术史上的重要分支。
在18世纪,随着印刷技术的发展,漫画作品开始出现在意大利、法国等国家,成为了一种新型的艺术表现形式。
在19世纪,随着漫画杂志和报纸的出现,肖像漫画开始进入大众视野,成为了一种受欢迎的艺术媒介。
目前,随着数字技术的发展,肖像漫画已经成为了一种新兴的艺术形式,受到了越来越多人的喜爱和欢迎。
二、肖像漫画的特点肖像漫画具有丰富的表现形式和独特的艺术特点,主要包括以下几个方面:1. 生动有趣:肖像漫画注重通过夸张、变形等手法来表现人物的个性和特征,使得人物形象更加生动有趣。
2. 夸张变形:肖像漫画常常通过夸张和变形的表现形式来强调人物的特征和个性,营造出夸张、搞笑的效果。
3. 表情夸张:肖像漫画通过捕捉人物的表情和情感,表现出人物的内心世界和个性特征,从而增强作品的趣味性和可读性。
4. 故事性强:肖像漫画通常通过情节和故事情节来展现人物的形象和特征,使得作品更加丰富和有趣。
三、肖像漫画的技巧肖像漫画的创作需要掌握一定的技巧和方法,包括以下几个方面:1. 观察人物:肖像漫画的创作需要通过对人物的观察,捕捉人物的特征和表情,从而使得作品更加生动和真实。
2. 线条和构图:肖像漫画的创作需要通过线条和构图的设计,来表现人物的特征和个性,使得作品更加传神和有趣。
3. 色彩和光影:肖像漫画的创作需要通过色彩和光影的运用,来表现人物的情感和内心世界,使得作品更加丰富和有趣。
4. 情节和故事:肖像漫画的创作需要通过情节和故事情节的设计,来展现人物的形象和特征,使得作品更加生动和有趣。
四、肖像漫画的表现形式肖像漫画的表现形式多种多样,包括以下几种常见的形式:1. 个人肖像:肖像漫画可以通过对个人形象和特征的表现,来展现人物的个性和魅力。
人美版七年级上册美术第8课《漫画》参考课件(共16张)

夸张法
比拟法
2021/10/10
10
漫画的主要特点
误会法
• 漫画具有多种表 现方法:对比手 法、夸张手法、 比喻法、推理表 现法、比拟手法 等等。
2021/10/10
比喻法
11
2021/10/10
12
漫画的绘制方法
• 同学们,如果让你绘制一幅漫画你会选用 那种绘制方法来绘制呢?
2021/10/10
漫画
caricature
1
什么是漫画?
• “漫”就是对漫画艺术最好 解释。“漫”使得漫画艺术 有比其它艺术更多的表现天 地,无论古今中外,无论社 会科学、自然科学、文化哲 理、风俗人情,各种题材皆 可入画,而且不受时间和空 间的限制,这就是漫画之所 以有深邃内涵之本源。
2021/10/10
2
漫画的类别
• 按用途可分为:讽刺漫画、幽默漫画、肖 像漫画、公益漫画。
• 讽刺漫画:讽刺社会生活存在的一些现象, 讽刺的内容大到国际问题,小到生活琐事; 幽默漫画:能给读者带来一种健康的快乐 的漫画;肖像漫画:用简约的线条概括出 对象的特点,利用夸张的手法,描绘人物 形象。
2021/10/10
3
讽刺漫画
2021/10/10
4
肖像漫画
2021/10/10
5
幽默漫画
2021/10/10
6
2021/10/10
漫画的类别
• 按篇幅来分类,可 分为:单幅漫画、 多幅漫画(四格漫 画)、系列漫画、 长篇故事漫画。
四格漫画
7
2021/10/10
系列漫画
8
2021/10/10
长篇故事漫画
一些简单的人物面部漫画入门篇
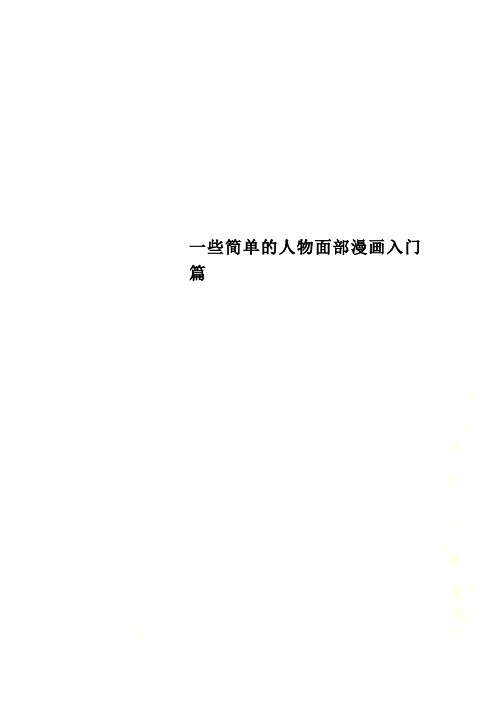
一些简单的人物面部漫画入门篇日式漫画人物速成指南-女性的眼睛眼睛是体现漫画式人物个性的最重要特征之一,双眼是面部最具表现力的部份, 也是区分不同人物使其鲜明可辨的部份。
所以画好眼睛是相当重要的。
在“常规面部绘画自学指南”的这部分内容中,大家将会知道该如何绘画多种漫画风格的眼睛。
一些常见的在线教程往往只告诉大家怎样画美女们的大眼睛,但没有仔细区分不同风格眼睛的明显变化。
本教程包括漫画中男性、女性不同类型眼睛的绘画方法,并附带一些其他风格的范例,希望可以帮你画出自己的原创漫画人物, 或许能让你的人物画得更精美。
(这篇教程部分内容基于图形处理软件--如ps而言的,但似乎更适合于手绘学习)女性的眼睛现在就让我们从最常见的漫画式眼睛--“美女型大眼”的画法开始啦。
先画一条向上弯的曲线,曲线最高处的线条要画得略微粗些。
这里我们画的是脸部右边的眼睛(也就是人物的左眼),因此曲线左端要比右端高(日式的大眼睛眼角有些下垂,楚楚可怜的样子,不过垂得太厉害了,小龙女就会成老龙婆)。
其实这个还没成型眼睛的顶端并非完美的曲线,而是稍带些棱角。
也有些类型的眼睛几乎完全在正顶端弯曲。
接下来,我们来画眼睛的下半部分。
为了确定其位置,我们从上眼线的边缘开始向下轻轻画两条斜线作为辅助线。
辅助线的倾斜程度决定眼睛会有多大多宽。
你可看看本页后面的例子,来体会线条倾斜度所产生的变化效果。
通过辅助线画出下眼线,眼角稍微向下往右倾斜一些,右下角的线条要画得略粗些。
擦掉辅助线,在眼睛里面画一个长的椭圆形。
也有些漫画人物的虹膜(就是眼珠子)是个大的圆形, 不过这里我们把它画成细长的椭圆形,当然你可以根据自己的喜好把形状调宽些。
椭圆形上部将被上眼线所遮盖--其实不论哪种风格的眼睛,我们都无法完全看到整个眼珠,上部几乎都要被眼框边缘掩盖(当然惊恐、愤怒时例外)。
下一步,画出眼珠高光的轮廓线。
通常漫画人物的眼睛会有一些阴影。
漫画中女孩一般也都具有明显的阴影和光泽。
- 1、下载文档前请自行甄别文档内容的完整性,平台不提供额外的编辑、内容补充、找答案等附加服务。
- 2、"仅部分预览"的文档,不可在线预览部分如存在完整性等问题,可反馈申请退款(可完整预览的文档不适用该条件!)。
- 3、如文档侵犯您的权益,请联系客服反馈,我们会尽快为您处理(人工客服工作时间:9:00-18:30)。
Human Facial Illustrations:Creation and Psychophysical EvaluationBRUCE GOOCHNorthwestern UniversityERIK REINHARDUniversity of Central FloridaandAMY GOOCHNorthwestern University We present a method for creating black-and-white illustrations from photographs of human faces.In addition an interactive technique is demonstrated for deforming these black-and-white facial illustrations to create caricatures which highlight and exaggerate representative facial features.We evaluate the effectiveness of the resulting images through psychophysical studies to assess accuracy and speed in both recognition and learning tasks.These studies show that the facial illustrations and caricatures generated using our techniques are as effective as photographs in recognition tasks.For the learning task we find that illustrations are learned two times faster than photographs and caricatures are learned one and a half times faster than photographs.Because our techniques produce images that are effective at communicating complex information,they are useful in a number of potential applications,ranging from entertainment and education to low bandwidth telecommunications and psychology research.Categories and Subject Descriptors:I.3.3[Computer Graphics ]:Picture/image Generation—bitmap and framebuffer operations ;I.3.8[Computer Graphics ]:Applications;I.4.3[Image Processing and Computer Vision ]:Enhancement—filtering General Terms:Algorithms,Human factorsAdditional Key Words and Phrases:Caricatures,Super-portraits,Validation1.INTRODUCTIONIn many applications a non-photorealistic rendering (NPR)has advantages over a photorealistic im-age.NPR images may convey information better by:omitting extraneous detail;focusing attention on relevant features;and by clarifying,simplifying,and disambiguating shape.The control of detail in an image for purposes of communication is becoming the hallmark of NPR images.This control of image detail is often combined with stylization to evoke the impression of complexity in an image without explicit representation.This work was carried out under NSF grants NSF/STC for computer graphics EIA 8920219,NSF 99-77218,NSF 99-78099,NSF/ACR,NSF/MRI,and by the DOE AVTC/VIEWS.Authors’addresses:B.Gooch and A.Gooch,Department of Computer Science,Northwestern University ,1890Maple,Suite 300,Evanston,IL 60201;email:{bgooch,amygooch }@;E.Reinhard,School of Computer Science,University of Central Florida,Orlando,FL 32816-2362;email:reinhard@.Permission to make digital or hard copies of part or all of this work for personal or classroom use is granted without fee provided that copies are not made or distributed for profit or direct commercial advantage and that copies show this notice on the first page or initial screen of a display along with the full citation.Copyrights for components of this work owned by others than ACM must be honored.Abstracting with credit is permitted.To copy otherwise,to republish,to post on servers,to redistribute to lists,or to use any component of this work in other works requires prior specific permission and/or a fee.Permissions may be requested from Publications Dept.,ACM,Inc.,1515Broadway ,New York,NY 10036USA,fax:+1(212)869-0481,or permissions@.c2004ACM 0730-0301/04/0100-0027$5.00ACM Transactions on Graphics,Vol.23,No.1,January 2004,Pages27–44.28• B.Gooch et al.Fig.1.Left Pair:Examples of illustrations generated using our software.Right Pair:Caricatures created by exaggerating the portraits’representative facial features.This technique is based on the methods of caricature artist Lenn Redman. Following this trend,we present two techniques that may be applied in sequence to transform a photograph of a human face into a caricature.First,we remove extraneous information from the pho-tograph while leaving intact the lines and shapes that would be drawn by cartoonists.The output of thefirst step is a two-tone image that we call an illustration.Second,this facial illustration may then be warped into a caricature using a practical interactive technique developed from observations of a method used to train caricature artists.Examples of images produced with our algorithms are shown in pared to previous caricature generation algorithms this method requires only a small amount of untrained user intervention.As with all non-photorealistic renderings,our images are intended to be used in specific tasks.While we provide ample examples of our results throughout the paper,relying on visual inspection to deter-mine that one method out-performs another,is a doubtful practice.Instead,we show in this paper that our algorithms produce images that allow observers to perform certain tasks better with our illustra-tions and caricatures than with the photographs they were derived from.Measuring the ability and effectiveness of an image to communicate the intent of its creator can only be achieved in an indirect way.In general,a user study is conducted whereby participants perform specific tasks on sets of visual stimuli.Relative task performance is then related to the images’effec-tiveness.If participants are statistically better at performing such tasks for certain types of images, these image types are said to be better at communicating their intent for the given task.For the algorithms presented in this article,we are interested in the speed and accuracy with which human portraits can be recognized.In addition,we are interested in the speed and accuracy with which human portraits may be learned.If it can be shown that our illustrations afford equal or better performance in these tasks over photographs,then the validity of our algorithms is demonstrated and many interesting applications are opened up.We conducted such a validation experiment,and our results show that recognition speed and accuracy as well as learning accuracy are unaffected by our algorithms,and that learning speed is in fact improved.This makes our techniques suitable for a wide variety of applications.We provide three examples of potential applications:First,the resulting images are suitable for rapid transmission over low band-width networks.Two-tone images may be stored with only one bit per pixel and image compression may further reduce memory requirements.As such,we foresee applications in telecommunications where users may transmit illustrated signature images of themselves in lieu of a caller I.D.number when making phone calls or when using their PDA’s.While wireless hand-held digital devices are already quite advanced,bandwidth for rapidly transmitting images is still a bottleneck.Second,because learning tasks can be sped up by using our images,visual learning applications may benefit from the use of images created using our process.We envision lightweight everyday applications ACM Transactions on Graphics,Vol.23,No.1,January2004.Human Facial Illustrations•29 that could,for example,allow a guest instructor to lean the names of all the students in a class,or allow firemen to learn the names and faces of all the residents while en route.Third,research in face recognition may benefit from using our techniques.The learning speedup and the recognition invariance demonstrated in our user study suggests that different brain structures or processing strategies may be involved in the perception of artistic images than in the perception of photographs.In the remainder of this paper we show how illustrations are derived from photographs(Section2) and how caricatures are created from illustrations(Section3).These two steps are then subjected to a user study(Section4),leading to our conclusions(Section5).2.ILLUSTRA TIONSPrevious research has shown that black-and-white imagery is useful for communicating complex infor-mation in a comprehensible and effective manner while consuming less storage[Ostromoukhov1999; Salisbury et al.1997,1996,1994;Winkenbach and Salesin1994;Tanaka and Ohnishi1997].With this idea in mind,we would like to produce easily recognizable black-and-white illustrations of faces. Some parts of the image may befilled in if this increases recognizability.However,shading effects that occur as a result of the lighting conditions under which the photograph was taken should be removed because they are not an intrinsic aspect of the face.In addition,we would like to be able to derive such illustrations from photographs without skilled user input.Creating a black-and-white illustration from a photograph can be done in many ways.A number of proposed methods are stroke-based and rely heavily on user input[Durand et al.2001;Ostromoukhov 1999;Sousa and Buchanan1999;Wong1999].In addition,stroke-based methods are mainly concerned with determining stroke placement in order to maintain tonal values across an object’s surface.For our application,we prefer a method that could largely be automated and does away with tonal information. One second possible method for creating facial illustrations is to only draw pixels in the image with a high intensity gradient[Pearson and Robinson1985;Pearson et al.1990].These pixels can be identified using a valleyfilter and then thresholded by computing the average brightness and setting all pixels that are above average brightness to white and all other pixels to black.However,this approach fails to preserve important high luminance details,and thus only captures some facial features.While the resulting image can be interpreted as a black-and-white drawing,wefind that it leaves“holes”in areas that should be all dark and thatfilling in the dark parts produces images that are more suitable.This filling in can be accomplished by thresholding the input luminances separately and multiplying the result of this operation with the thresholded brightness image[Pearson and Robinson1985].A third approach could be the use of edge detection algorithms to remove redundant data.These algorithms are often used in machine vision applications.Most of these edge detection algorithms produce thin lines that are connected.While connectedness is a basic requirement in machine vision, it is specifically not needed for portraits of faces and may reduce recognizability[Davies et al.1978]. To comply with our requirements that the method needs minimal trained user input and produces easily recognizable images,we choose to base our algorithm on a model of human brightness perception. Such models are good candidates for further exploration because theyflag areas of the image where interesting transitions occur,while removing regions of constant gradient.How this is achieved is explained next.In addition,we preserve a sense of absolute luminance levels by thresholding the input image and adding this to the result.The general approach of our method is outlined in Figure2.2.1Contrast and Brightness PerceptionBefore introducing our algorithm for computing illustrations,in this subsection,we briefly summa-rize models of brightness perception.It should be noted that there are many reasonable brightnessACM Transactions on Graphics,Vol.23,No.1,January2004.30• B.Gooch etal.Fig.2.Brightness is computed from a photograph,then thresholded and multiplied with thresholded luminance to create a line art portrait.models available and that this is still an active area of research,which is much more fully described in excellent authoritative texts such as Boff et al.[1986],Gilchrist [1994],Wandell [1995],and Palmer[1999].While light is necessary to convey information from objects to the retina,the human visual system attempts to discard certain properties of light [Atick and Redlich 1992;Blommaert and Martens 1990].An example is brightness constancy ,where brightness is de fined as a measure of how humans perceive luminance [Palmer 1999].Brightness perception can be modeled using operators such as differentiation,integration and thresh-olding [Arend and Goldstein 1987;Land and McCann 1971].These methods model lateral inhibition which is one of the most pervasive structures in the visual nervous system [Palmer 1999].Lateral inhibition is implemented by a cell ’s receptive field having a center-surround organization.Thus cells in the earliest stages of human vision respond most vigorously to a pattern of light which is bright in the center of the cell ’s receptive field and dark in the surround,or vice-versa.Such antagonistic center-surround behavior can be modeled using neural networks,or by computational models such as Difference of Gaussians [Blommaert and Martens 1990;Cohen and Grossberg 1984;Gove et al.1995;Hansen et al.2000;Pessoa et al.1995],Gaussian smoothed Laplacians [Marr and Hildreth 1980;Marr 1982]and Gabor filters [Jernigan and McLean 1992].Closely related to brightness models are edge detection algorithms that are based on the physiology of the mammalian visual system.An example is Marr and Hildreth ’s zero-crossing algorithm [Marr and Hildreth 1980].This algorithm computes the Laplacian of a Gaussian blurred image (LoG)and detects zero crossings in the result.The LoG is a two dimensional isotropic measure of the second spatial derivative of an image.It highlights regions of rapid intensity change and can therefore be used for edge detection.Note that the Laplacian of Gaussian can be closely approximated by com-puting the difference of two Gaussian blurred images,provided the Gaussians are scaled by a fac-tor of 1.6with respect to each other [Marrmodel.ACM Transactions on Graphics,Vol.23,No.1,January 2004.Human Facial Illustrations •312.2Computing IllustrationsTo create illustrations from photographs we adapt Blommaert and Martens ’[1990]model of human brightness perception,which was recently shown to be effective in a different type of application (tone reproduction,see Reinhard et al.[2002]).The aim of the Blommaert model is to understand brightness perception in terms of cell properties and neural structures.For example,the scale invariance property of the human visual system can be modeled by assuming that the outside world is interpreted at different levels of resolution,controlled by varying receptive field sizes.Blommaert and Martens [1990]demonstrate that,to a first approximation,the receptive fields of the human visual system are isotropic with respect to brightness perception,and can be modeled by circularly symmetric Gaussian pro files R i :R i (x ,y ,s )=1i s )2exp −x 2+y 2(αi s )2.(1)These Gaussian pro files operate at different scales s and at different image positions (x ,y ).We use R 1for the center and R 2to model the surround and let α1=1/(2√2).The latter ensures that two standard deviations of the Gaussian overlap with the number of pixels speci fied by s .For the surround we specify α2=1.6α1.A neural response V i as function of image location,scale and luminance distribution L can be computed by convolution:V i (x ,y ,s )=L (x ,y )⊗R i (x ,y ,s ).(2)The firing frequency evoked across scales by a luminance distribution L is modeled by a center-surround mechanism:V (x ,y ,s )=V 1(x ,y ,s )−V 2(x ,y ,s )2φ/s 2+V 1(x ,y ,s ),(3)where center V 1and surround V 2responses are derived from Eqs.(1)and (2).Subtracting V 1and V 2leads to a Mexican hat shape,which is normalized by V 1.The term 2φ/s 2is introduced to avoid the singularity that occurs when V 1approaches zero and models the (scale-dependent)rest activity associated with the center of the receptive field [Blommaert and Martens 1990].The value 2φis the transition flux at which a cell starts to be photopically adapted.In the Blommaert model,the parameter 2φis set to 100cd arcmin 2m −2.Because in our application we deal with low dynamic range images,as well as an uncontrolled display environment (see below),we adapt the model heuristically by setting φ=1.We have found that this parameter can be varied to manipulate the amount of fine detail present in the illustration.An expression for brightness B is now derived by summing V over all scales:B (x ,y )=s maxs =s 0V (x ,y ,s ).(4)The Blommaert model,in line with other models of brightness perception,speci fies these boundaries in visual angles,ranging from 2arcmin to 50degrees.In a practical application,the size of each pixel as it is displayed on a monitor,is generally unknown.In addition,the distance of the viewer can not be accurately controlled.For these reasons,we translate these angles into image sizes under the reasonable assumption that the smallest size is 1pixel (s 0=1).The number of discrete scales is chosen to be 8,which provides a good trade-off between speed of computation and accuracy of the result.These two parameters fix the upper boundary s max to be 1.68≈43pixels.For computational convenience,the scales s are spaced by a factor of 1.6with respect to each other.This allows us to reuse the surround computation at scale s i for the center at scale s i +1.ACM Transactions on Graphics,Vol.23,No.1,January 2004.32• B.Gooch et al.Fig.3.Thresholded brightness(left),high-passfiltered image followed by thresholding(middle)and Canny’s edge detector (right).The result of these computations is an image which could be seen as an interpretation of human brightness perception.One of the effects of this computation is that constant regions in the input image remain constant in the brightness image.Also,areas with a constant gradient are removed and become areas of constant intensity.In practice,this has the effect of removing shading from an image.Removing shading is an advantage for computing illustrations because shading information that is typically not shown in illustrations.Brightness images are typically grey with lighter and darker areas near regions with nonzero second derivatives.In illustrations,these regions are usually indicated with lines.There is therefore a direct relation between the information present in a brightness image and the lines drawn by illustrators.As such,thefinal step is converting our brightness representation into a two-tone image that re-sembles an illustration.We do this by computing the average intensity of the brightness image and setting all pixels that are above this threshold to white and all other pixels to black.Dependent on the composition of the photograph,this threshold may be manually increased or decreased.However,it is our experience that the average brightness is always a good initial estimate and that deviations in choice of threshold tend to be small.All other constants in the brightness model arefixed as indicated above and therefore do not require further human intervention.Figure3shows the result of thresholding our brightness representation and compares it to thresh-olding a high passfiltered image(middle)and an edge detected image(right).Thresholding a high-pass filtered image and edge detecting are perhaps more obvious strategies that could potentially lead to similar illustrations.Figure3shows that this is not necessarily the case.The high-passfiltered im-age was obtained by applying a5×5Gaussian kernel to the input image and subtracting the result from the input image.The threshold-level was chosen to maximize detail while at the same time mini-mizing salt-and-pepper noise.Note that it is difficult to simultaneously achieve both goals within this scheme.The comparison with Canny’s edge detector[Canny1986]is provided to illustrate the fact that connected thin lines are less useful for this particular application.The thresholded brightness image can be interpreted as a black-and-white illustration,although we find thatfilling in the dark parts produces images that are easier to recognize.Filling in is accomplished by thresholding the luminance of the input image separately and multiplying the result of this operation with the thresholded brightness image[Pearson and Robinson1985].The threshold value is chosen manually according to taste,but often falls in the range from about3to5%grey.The process of computing a portrait is illustrated in Figure2.ACM Transactions on Graphics,Vol.23,No.1,January2004.Human Facial Illustrations•33Fig.4.Source images(top)and results of our perception based portrait algorithm(bottom).Table I.Storage Space(in Bits Per Pixel)for Photographsand Facial Illustrations Computed Using Our MethodPhotograph 1.210.96 1.20Illustration0.100.110.19Our facial illustrations are based on photographs but contain much less information,as shown in Figure4.For example,shading is removed from the image,which is a property of Difference of Gaussians approaches.As such,the storage space required for these illustrations is decreased from the space needed to store photographs.See Table I.On a400-MHz R12k processor,the computation time for a10242image is28.5s,while a5122can be computed in6.0s.These timings are largely due to the FFT computation used to compute the convolu-tion of Eq.(2).We anticipate that these images could be computed faster with approximate methods, although this could be at the cost of some quality.In particular,we believe that a multiresolution spline method may yield satisfactory results[Burt and Adelson1983].On the other hand,it should be pointed out that the brightness computation involves a number of FFT computations to facilitate the convolution operations.This makes the algorithm relatively expen-sive.Also,the brightness threshold and the threshold on luminance of the source image are currently specified by hand.While a reasonable initial value may be specified based on the average intensity found in the brightness images,a small deviation from this initial threshold almost always improves the result.Further research may automate this process and so make this method better suitable for producing animated illustrations from video sequences.Should better models of brightness perception become available,then these may improve our results.In particular,because human visual perception is to a lesser extent sensitive to absolute intensity levels,a brightness model that incorporates both relative as well as absolute light levels may further improve our results.Although Blommaert and Martens discuss a notion of absolute light levels[Blommaert and Martens1990],for our application their model requires the additional application of thresholded absolute luminance levels.It would be better if this could be incorporated directly into the brightness model.ACM Transactions on Graphics,Vol.23,No.1,January2004.34• B.Gooch et al.Fig.5.Left Trio:Photographic example.Right Trio:Facial illustration example.In both examples thefirst images are of50% anti-caricatures,the second images are the source images,and the third images are50%super portraits.While Figure4allows the reader to subjectively assess the performance of our algorithm,its real merit lies in the fact that specific tasks can be performed quicker using facial illustration images than when using photographs.This remarkablefinding is presented in Section4.Finally,some of the facial features that a cartoonist would draw,are absent from our illustrations while some noise is present.Parameters in our model may be adjusted to add more lines,but this also increases the amount of noise in the illustrations.While our algorithm produces plausible results with the parameters outlined above,future research into alternative algorithms may well lead to improved quality.2.3Creating a Super-PortraitSuper-portraits are closely related to the peak shift effect,which is a well-known principle in animal learning[Hansen1959;Ramachandran and Hirstein1999].It is best explained by an example.Suppose a laboratory rat is taught to discriminate a square from a rectangle by being rewarded for choosing the rectangle,it will soon learn to respond more frequently to the rectangle.Moreover,if a prototype rectangle of aspect ratio3:2is used to train the rat,it will respond even more positively to a longer and thinner rectangle with an aspect ratio of4:1.This result implies the rat is not learning to value a particular rectangle but a rule,in this case that rectangles are better than squares.So the more oblong the rectangle,the better the rectangle appears to the rat.Super-portraits of human faces are a well studied example of the peak shift effect in human visual perception[Benson and Perret1994;Rhodes et al.1987;Rhodes and Tremewan1994,1996;Stevenage 1995].It has been postulated that humans recognize faces based on the amount that facial features deviate from an average face[Tversky and Baratz1985;Valentine1991].Thus,to produce a super portrait,features are exaggerated based on how far the face’s features deviate from an average or norm face[Brennan1985].Figure5shows examples of super-portraits.Two paradigms exist that explain how humans perform face recognition.In the average-based coding theory,a“feature space distance”from an average face to a given face is encoded by the brain[Valentine 1991].An alternative model of face recognition is based on exemplars[Lewis and Johnston1998],where face representations are stored in memory as absolutes.Both models equally account for the effects observed in face recognition tasks,but the average-based coding paradigm lets itself be cast more easily into a computational model.In our approach,super-portraits may be created in a semiautomatic way.A face isfirst framed with four lines that comprise a rectangle,as shown in Figure7.Four vertical lines are then introduced marking the inner and outer corners of the eyes,respectively.Next,three additional horizontal lines mark the position of the eyes,the tip of the nose,and the mouth.We call this set of horizontal and vertical lines a facial feature grid(FFG).ACM Transactions on Graphics,Vol.23,No.1,January2004.Human Facial Illustrations•35 To generate a FFG for a norm face,we apply a previously defined metric[Redman1984].The vertical lines are set to be equidistant,while the horizontal eye,nose and mouth lines are assigned distance values4/9,6/9and7/9respectively,from the top of the frame.The norm FFG is automatically computed when the face is framed by the user.Gridding rules can also be specified for profile views[Redman1984], but for the purpose of this work,we have constrained our input to frontal views.When a feature grid is specified for a given photograph or portrait,it is unlikely to coincide with the norm FFG.The difference between the norm face grid and the user-set grid can be exaggerated by computing the vectors between corresponding vertices in both grids.Then,these vectors are scaled by a given percentage and the source image is warped correspondingly.When this percentage is positive, the result is called a super portrait,whereas negative percentages give rise to anticaricatures,images that are closer to the norm face than the input image(Figure5).3.CARICA TURESThe documented ability of caricatures to augment the communication content of images of human faces motivated the investigation of computer generated caricatures[Brennan1985;Benson and Perret1991; Rhodes et al.1987;Stevenage1995].To create a caricature,the difference between an average face and a particular face can be computed for various facial features,which is then exaggerated by a specified amount.We describe methods for automatically deviating from an average face,as well as techniques that allow meaningful warping to perform more extreme caricaturing.Traditionally caricatures have been created by skilled artists who use lines to represent facial fea-tures.The skill of the artist lies in knowing which particular facial features are essential and which are incidental.For facial caricatures both artists and psychologists agree that the feature shift for a particular face should exaggerate the differences from an average face[Brennan1985;Redman1984; Rhodes et al.1987].Automatically creating such drawings has been an elusive goal,and attempts to automate this process are sparse.The most well-known attempt is the“Caricature Generator”[Brennan1985],which is based on the notion of an average face.The positions of165feature points are indicated by a knowledgeable user marking points on a scanned photograph with mouse clicks.The points for the given face are then compared with the positions of similar points on an average face.By moving the user defined points away from the average,an exaggerated effect can be created.A line drawing is created by connecting the feature points with lines.A caricature is created by translating the feature points over some distance and then connecting them with lines.This method was later extended to allow the feature translation to be applied to the input image in order to produce a photographic caricature[Benson and Perret 1991].The Caricature Generator is used in many psychophysical experiments and has become a de facto standard for conducting research in face recognition[Benson and Perret1994;Rhodes et al.1987; Rhodes and Tremewan1994,1996;Stevenage1995](see also Section4).Examples of facial illustrations and caricatures created using an implementation of the“Caricature Generator”software are shown in Figure6.A second semi-automated caricature generator is based on simplicial complexes[Akleman et al.2000]. The deformations applied to a photograph of a face are defined by pairs of simplices(triangles in this case).Each pair of triangles specifies a deformation,and deformations can be blended for more general warps.This system is capable of interactively producing extreme exaggerations of facial features,but requires experience to meaningfully specify source and target simplices.Both previous methods require expert knowledge or skilled user input,which limits their applicability for every-day use.We propose semi-automatic methods to produce super-portraits and caricatures which rely much less on the presence of well-trained users.ACM Transactions on Graphics,Vol.23,No.1,January2004.。