Gatsby Computational Neuroscience Unit
人工智能领域中英文专有名词汇总
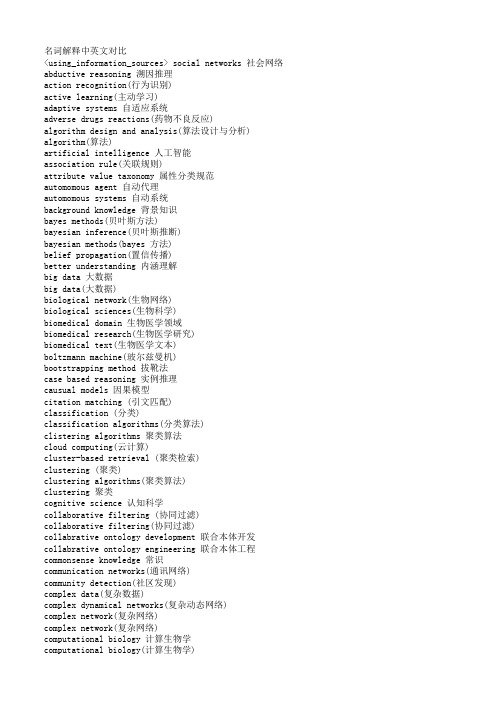
名词解释中英文对比<using_information_sources> social networks 社会网络abductive reasoning 溯因推理action recognition(行为识别)active learning(主动学习)adaptive systems 自适应系统adverse drugs reactions(药物不良反应)algorithm design and analysis(算法设计与分析) algorithm(算法)artificial intelligence 人工智能association rule(关联规则)attribute value taxonomy 属性分类规范automomous agent 自动代理automomous systems 自动系统background knowledge 背景知识bayes methods(贝叶斯方法)bayesian inference(贝叶斯推断)bayesian methods(bayes 方法)belief propagation(置信传播)better understanding 内涵理解big data 大数据big data(大数据)biological network(生物网络)biological sciences(生物科学)biomedical domain 生物医学领域biomedical research(生物医学研究)biomedical text(生物医学文本)boltzmann machine(玻尔兹曼机)bootstrapping method 拔靴法case based reasoning 实例推理causual models 因果模型citation matching (引文匹配)classification (分类)classification algorithms(分类算法)clistering algorithms 聚类算法cloud computing(云计算)cluster-based retrieval (聚类检索)clustering (聚类)clustering algorithms(聚类算法)clustering 聚类cognitive science 认知科学collaborative filtering (协同过滤)collaborative filtering(协同过滤)collabrative ontology development 联合本体开发collabrative ontology engineering 联合本体工程commonsense knowledge 常识communication networks(通讯网络)community detection(社区发现)complex data(复杂数据)complex dynamical networks(复杂动态网络)complex network(复杂网络)complex network(复杂网络)computational biology 计算生物学computational biology(计算生物学)computational complexity(计算复杂性) computational intelligence 智能计算computational modeling(计算模型)computer animation(计算机动画)computer networks(计算机网络)computer science 计算机科学concept clustering 概念聚类concept formation 概念形成concept learning 概念学习concept map 概念图concept model 概念模型concept modelling 概念模型conceptual model 概念模型conditional random field(条件随机场模型) conjunctive quries 合取查询constrained least squares (约束最小二乘) convex programming(凸规划)convolutional neural networks(卷积神经网络) customer relationship management(客户关系管理) data analysis(数据分析)data analysis(数据分析)data center(数据中心)data clustering (数据聚类)data compression(数据压缩)data envelopment analysis (数据包络分析)data fusion 数据融合data generation(数据生成)data handling(数据处理)data hierarchy (数据层次)data integration(数据整合)data integrity 数据完整性data intensive computing(数据密集型计算)data management 数据管理data management(数据管理)data management(数据管理)data miningdata mining 数据挖掘data model 数据模型data models(数据模型)data partitioning 数据划分data point(数据点)data privacy(数据隐私)data security(数据安全)data stream(数据流)data streams(数据流)data structure( 数据结构)data structure(数据结构)data visualisation(数据可视化)data visualization 数据可视化data visualization(数据可视化)data warehouse(数据仓库)data warehouses(数据仓库)data warehousing(数据仓库)database management systems(数据库管理系统)database management(数据库管理)date interlinking 日期互联date linking 日期链接Decision analysis(决策分析)decision maker 决策者decision making (决策)decision models 决策模型decision models 决策模型decision rule 决策规则decision support system 决策支持系统decision support systems (决策支持系统) decision tree(决策树)decission tree 决策树deep belief network(深度信念网络)deep learning(深度学习)defult reasoning 默认推理density estimation(密度估计)design methodology 设计方法论dimension reduction(降维) dimensionality reduction(降维)directed graph(有向图)disaster management 灾害管理disastrous event(灾难性事件)discovery(知识发现)dissimilarity (相异性)distributed databases 分布式数据库distributed databases(分布式数据库) distributed query 分布式查询document clustering (文档聚类)domain experts 领域专家domain knowledge 领域知识domain specific language 领域专用语言dynamic databases(动态数据库)dynamic logic 动态逻辑dynamic network(动态网络)dynamic system(动态系统)earth mover's distance(EMD 距离) education 教育efficient algorithm(有效算法)electric commerce 电子商务electronic health records(电子健康档案) entity disambiguation 实体消歧entity recognition 实体识别entity recognition(实体识别)entity resolution 实体解析event detection 事件检测event detection(事件检测)event extraction 事件抽取event identificaton 事件识别exhaustive indexing 完整索引expert system 专家系统expert systems(专家系统)explanation based learning 解释学习factor graph(因子图)feature extraction 特征提取feature extraction(特征提取)feature extraction(特征提取)feature selection (特征选择)feature selection 特征选择feature selection(特征选择)feature space 特征空间first order logic 一阶逻辑formal logic 形式逻辑formal meaning prepresentation 形式意义表示formal semantics 形式语义formal specification 形式描述frame based system 框为本的系统frequent itemsets(频繁项目集)frequent pattern(频繁模式)fuzzy clustering (模糊聚类)fuzzy clustering (模糊聚类)fuzzy clustering (模糊聚类)fuzzy data mining(模糊数据挖掘)fuzzy logic 模糊逻辑fuzzy set theory(模糊集合论)fuzzy set(模糊集)fuzzy sets 模糊集合fuzzy systems 模糊系统gaussian processes(高斯过程)gene expression data 基因表达数据gene expression(基因表达)generative model(生成模型)generative model(生成模型)genetic algorithm 遗传算法genome wide association study(全基因组关联分析) graph classification(图分类)graph classification(图分类)graph clustering(图聚类)graph data(图数据)graph data(图形数据)graph database 图数据库graph database(图数据库)graph mining(图挖掘)graph mining(图挖掘)graph partitioning 图划分graph query 图查询graph structure(图结构)graph theory(图论)graph theory(图论)graph theory(图论)graph theroy 图论graph visualization(图形可视化)graphical user interface 图形用户界面graphical user interfaces(图形用户界面)health care 卫生保健health care(卫生保健)heterogeneous data source 异构数据源heterogeneous data(异构数据)heterogeneous database 异构数据库heterogeneous information network(异构信息网络) heterogeneous network(异构网络)heterogenous ontology 异构本体heuristic rule 启发式规则hidden markov model(隐马尔可夫模型)hidden markov model(隐马尔可夫模型)hidden markov models(隐马尔可夫模型) hierarchical clustering (层次聚类) homogeneous network(同构网络)human centered computing 人机交互技术human computer interaction 人机交互human interaction 人机交互human robot interaction 人机交互image classification(图像分类)image clustering (图像聚类)image mining( 图像挖掘)image reconstruction(图像重建)image retrieval (图像检索)image segmentation(图像分割)inconsistent ontology 本体不一致incremental learning(增量学习)inductive learning (归纳学习)inference mechanisms 推理机制inference mechanisms(推理机制)inference rule 推理规则information cascades(信息追随)information diffusion(信息扩散)information extraction 信息提取information filtering(信息过滤)information filtering(信息过滤)information integration(信息集成)information network analysis(信息网络分析) information network mining(信息网络挖掘) information network(信息网络)information processing 信息处理information processing 信息处理information resource management (信息资源管理) information retrieval models(信息检索模型) information retrieval 信息检索information retrieval(信息检索)information retrieval(信息检索)information science 情报科学information sources 信息源information system( 信息系统)information system(信息系统)information technology(信息技术)information visualization(信息可视化)instance matching 实例匹配intelligent assistant 智能辅助intelligent systems 智能系统interaction network(交互网络)interactive visualization(交互式可视化)kernel function(核函数)kernel operator (核算子)keyword search(关键字检索)knowledege reuse 知识再利用knowledgeknowledgeknowledge acquisitionknowledge base 知识库knowledge based system 知识系统knowledge building 知识建构knowledge capture 知识获取knowledge construction 知识建构knowledge discovery(知识发现)knowledge extraction 知识提取knowledge fusion 知识融合knowledge integrationknowledge management systems 知识管理系统knowledge management 知识管理knowledge management(知识管理)knowledge model 知识模型knowledge reasoningknowledge representationknowledge representation(知识表达) knowledge sharing 知识共享knowledge storageknowledge technology 知识技术knowledge verification 知识验证language model(语言模型)language modeling approach(语言模型方法) large graph(大图)large graph(大图)learning(无监督学习)life science 生命科学linear programming(线性规划)link analysis (链接分析)link prediction(链接预测)link prediction(链接预测)link prediction(链接预测)linked data(关联数据)location based service(基于位置的服务) loclation based services(基于位置的服务) logic programming 逻辑编程logical implication 逻辑蕴涵logistic regression(logistic 回归)machine learning 机器学习machine translation(机器翻译)management system(管理系统)management( 知识管理)manifold learning(流形学习)markov chains 马尔可夫链markov processes(马尔可夫过程)matching function 匹配函数matrix decomposition(矩阵分解)matrix decomposition(矩阵分解)maximum likelihood estimation(最大似然估计)medical research(医学研究)mixture of gaussians(混合高斯模型)mobile computing(移动计算)multi agnet systems 多智能体系统multiagent systems 多智能体系统multimedia 多媒体natural language processing 自然语言处理natural language processing(自然语言处理) nearest neighbor (近邻)network analysis( 网络分析)network analysis(网络分析)network analysis(网络分析)network formation(组网)network structure(网络结构)network theory(网络理论)network topology(网络拓扑)network visualization(网络可视化)neural network(神经网络)neural networks (神经网络)neural networks(神经网络)nonlinear dynamics(非线性动力学)nonmonotonic reasoning 非单调推理nonnegative matrix factorization (非负矩阵分解) nonnegative matrix factorization(非负矩阵分解) object detection(目标检测)object oriented 面向对象object recognition(目标识别)object recognition(目标识别)online community(网络社区)online social network(在线社交网络)online social networks(在线社交网络)ontology alignment 本体映射ontology development 本体开发ontology engineering 本体工程ontology evolution 本体演化ontology extraction 本体抽取ontology interoperablity 互用性本体ontology language 本体语言ontology mapping 本体映射ontology matching 本体匹配ontology versioning 本体版本ontology 本体论open government data 政府公开数据opinion analysis(舆情分析)opinion mining(意见挖掘)opinion mining(意见挖掘)outlier detection(孤立点检测)parallel processing(并行处理)patient care(病人医疗护理)pattern classification(模式分类)pattern matching(模式匹配)pattern mining(模式挖掘)pattern recognition 模式识别pattern recognition(模式识别)pattern recognition(模式识别)personal data(个人数据)prediction algorithms(预测算法)predictive model 预测模型predictive models(预测模型)privacy preservation(隐私保护)probabilistic logic(概率逻辑)probabilistic logic(概率逻辑)probabilistic model(概率模型)probabilistic model(概率模型)probability distribution(概率分布)probability distribution(概率分布)project management(项目管理)pruning technique(修剪技术)quality management 质量管理query expansion(查询扩展)query language 查询语言query language(查询语言)query processing(查询处理)query rewrite 查询重写question answering system 问答系统random forest(随机森林)random graph(随机图)random processes(随机过程)random walk(随机游走)range query(范围查询)RDF database 资源描述框架数据库RDF query 资源描述框架查询RDF repository 资源描述框架存储库RDF storge 资源描述框架存储real time(实时)recommender system(推荐系统)recommender system(推荐系统)recommender systems 推荐系统recommender systems(推荐系统)record linkage 记录链接recurrent neural network(递归神经网络) regression(回归)reinforcement learning 强化学习reinforcement learning(强化学习)relation extraction 关系抽取relational database 关系数据库relational learning 关系学习relevance feedback (相关反馈)resource description framework 资源描述框架restricted boltzmann machines(受限玻尔兹曼机) retrieval models(检索模型)rough set theroy 粗糙集理论rough set 粗糙集rule based system 基于规则系统rule based 基于规则rule induction (规则归纳)rule learning (规则学习)rule learning 规则学习schema mapping 模式映射schema matching 模式匹配scientific domain 科学域search problems(搜索问题)semantic (web) technology 语义技术semantic analysis 语义分析semantic annotation 语义标注semantic computing 语义计算semantic integration 语义集成semantic interpretation 语义解释semantic model 语义模型semantic network 语义网络semantic relatedness 语义相关性semantic relation learning 语义关系学习semantic search 语义检索semantic similarity 语义相似度semantic similarity(语义相似度)semantic web rule language 语义网规则语言semantic web 语义网semantic web(语义网)semantic workflow 语义工作流semi supervised learning(半监督学习)sensor data(传感器数据)sensor networks(传感器网络)sentiment analysis(情感分析)sentiment analysis(情感分析)sequential pattern(序列模式)service oriented architecture 面向服务的体系结构shortest path(最短路径)similar kernel function(相似核函数)similarity measure(相似性度量)similarity relationship (相似关系)similarity search(相似搜索)similarity(相似性)situation aware 情境感知social behavior(社交行为)social influence(社会影响)social interaction(社交互动)social interaction(社交互动)social learning(社会学习)social life networks(社交生活网络)social machine 社交机器social media(社交媒体)social media(社交媒体)social media(社交媒体)social network analysis 社会网络分析social network analysis(社交网络分析)social network(社交网络)social network(社交网络)social science(社会科学)social tagging system(社交标签系统)social tagging(社交标签)social web(社交网页)sparse coding(稀疏编码)sparse matrices(稀疏矩阵)sparse representation(稀疏表示)spatial database(空间数据库)spatial reasoning 空间推理statistical analysis(统计分析)statistical model 统计模型string matching(串匹配)structural risk minimization (结构风险最小化) structured data 结构化数据subgraph matching 子图匹配subspace clustering(子空间聚类)supervised learning( 有support vector machine 支持向量机support vector machines(支持向量机)system dynamics(系统动力学)tag recommendation(标签推荐)taxonmy induction 感应规范temporal logic 时态逻辑temporal reasoning 时序推理text analysis(文本分析)text anaylsis 文本分析text classification (文本分类)text data(文本数据)text mining technique(文本挖掘技术)text mining 文本挖掘text mining(文本挖掘)text summarization(文本摘要)thesaurus alignment 同义对齐time frequency analysis(时频分析)time series analysis( 时time series data(时间序列数据)time series data(时间序列数据)time series(时间序列)topic model(主题模型)topic modeling(主题模型)transfer learning 迁移学习triple store 三元组存储uncertainty reasoning 不精确推理undirected graph(无向图)unified modeling language 统一建模语言unsupervisedupper bound(上界)user behavior(用户行为)user generated content(用户生成内容)utility mining(效用挖掘)visual analytics(可视化分析)visual content(视觉内容)visual representation(视觉表征)visualisation(可视化)visualization technique(可视化技术) visualization tool(可视化工具)web 2.0(网络2.0)web forum(web 论坛)web mining(网络挖掘)web of data 数据网web ontology lanuage 网络本体语言web pages(web 页面)web resource 网络资源web science 万维科学web search (网络检索)web usage mining(web 使用挖掘)wireless networks 无线网络world knowledge 世界知识world wide web 万维网world wide web(万维网)xml database 可扩展标志语言数据库附录 2 Data Mining 知识图谱(共包含二级节点15 个,三级节点93 个)间序列分析)监督学习)领域 二级分类 三级分类。
计算神经科学对大脑认知过程研究
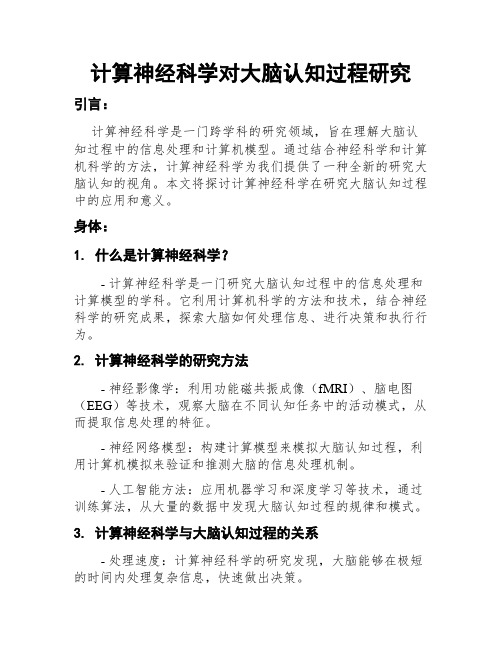
计算神经科学对大脑认知过程研究引言:计算神经科学是一门跨学科的研究领域,旨在理解大脑认知过程中的信息处理和计算机模型。
通过结合神经科学和计算机科学的方法,计算神经科学为我们提供了一种全新的研究大脑认知的视角。
本文将探讨计算神经科学在研究大脑认知过程中的应用和意义。
身体:1. 什么是计算神经科学?- 计算神经科学是一门研究大脑认知过程中的信息处理和计算模型的学科。
它利用计算机科学的方法和技术,结合神经科学的研究成果,探索大脑如何处理信息、进行决策和执行行为。
2. 计算神经科学的研究方法- 神经影像学:利用功能磁共振成像(fMRI)、脑电图(EEG)等技术,观察大脑在不同认知任务中的活动模式,从而提取信息处理的特征。
- 神经网络模型:构建计算模型来模拟大脑认知过程,利用计算机模拟来验证和推测大脑的信息处理机制。
- 人工智能方法:应用机器学习和深度学习等技术,通过训练算法,从大量的数据中发现大脑认知过程的规律和模式。
3. 计算神经科学与大脑认知过程的关系- 处理速度:计算神经科学的研究发现,大脑能够在极短的时间内处理复杂信息,快速做出决策。
- 学习和记忆:通过模型模拟,计算神经科学揭示了大脑学习和记忆的机制,进一步指导认知科学的研究。
- 决策和规划:神经网络模型的应用使我们能够更好地理解大脑在决策和规划中的运作方式,从而解析行为背后的认知过程。
4. 计算神经科学在认知障碍研究中的应用- 认知障碍是大脑认知能力受损的病理状态。
计算神经科学为我们提供了一种研究认知障碍的新视角。
- 通过将计算模型与病人的大脑影像数据结合,研究人员可以更好地理解认知障碍的机制,并提出针对性的治疗方法。
5. 可能的未来发展方向- 形态学与功能的融合:将大脑的形态学和功能信息结合起来,更全面地理解大脑的认知机制。
- 跨领域合作:加强计算神经科学与认知科学、心理学等领域的合作,形成多学科的交叉研究。
- 应用于人工智能技术:将计算神经科学的研究成果与人工智能技术相结合,制定更智能化的人工智能算法。
计算认知神经科学

阅读感受
这本书是一本非常优秀的学术著作,不仅适合于从事神经科学和认知科学研 究的人员阅读,也适合于对、机器人等领域感兴趣的人员阅读。通过阅读这本书, 我不仅了解了计算认知神经科学的基本知识和最新研究成果,还深入了解了各种 计算模型的设计和应用。我也感受到了作者对这门学科的热爱和执着,这让我更 加坚定了自己从事相关领域研究的决心。我相信,这本书对于推动计算认知神经 科学的发展和应用,将会产生重要的影响。
内容摘要
本书介绍了计算模型在认知神经科学研究中的应用。计算模型是一种通过数学方程或计算机程序 来模拟大脑活动的工具。本书介绍了不同的计算模型,如递归神经网络、深度学习模型等,并解 释了它们如何模拟人类认知过程。本书还讨论了计算模型的应用,如模式识别、自然语言处理、 情感计算等。 《计算认知神经科学》这本书是一本非常全面的介绍认知神经科学的著作。通过阅读本书,读者 可以深入了解人脑如何处理信息以及如何通过计算模型模拟人脑的认知过程。本书不仅适合于从 事认知神经科学研究的人员阅读,也适合于对人类大脑和认知过程感兴趣的读者阅读。
目录分析
目录分析
《计算认知神经科学》是一本探讨认知神经科学的著作,其主要目的是从计 算角度揭示人类认知过程。本书的目录经过精心设计,按照主题和层次,将复杂 的认知神经科学知识进行系统的组织。
目录分析
本书的目录按照认知神经科学的主题进行划分,包括认知过程、神经机制、 信息处理、学习与记忆等方面。这种划分方式使得读者可以根据自己的兴趣和需 求选择相应的章节进行阅读。同时,每个主题下的章节又按照知识层次进行组织, 使得读者可以逐步深入了解每个主题的知识。
精彩摘录
“我们的大脑是由数十亿个神经元组成的复杂网络,每个神经元都与其他神 经元相互连接。这些神经元通过电化学信号进行通信,从而产生我们的思想和行 为。这种神经元之间的通信是通过复杂的网络结构和动态过程实现的。”
神经科学中的认知计算模型研究
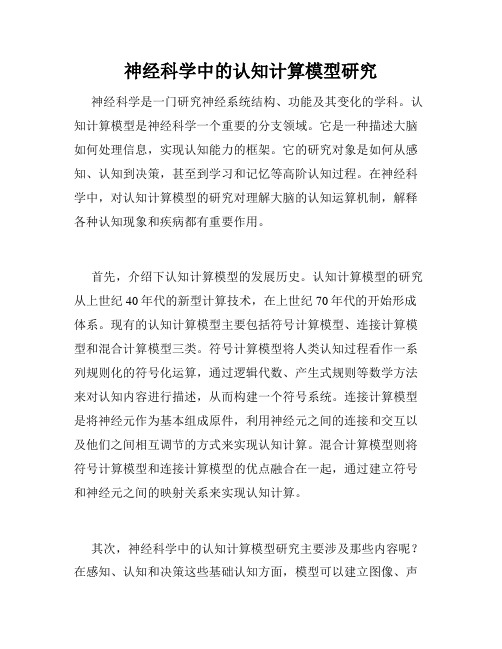
神经科学中的认知计算模型研究神经科学是一门研究神经系统结构、功能及其变化的学科。
认知计算模型是神经科学一个重要的分支领域。
它是一种描述大脑如何处理信息,实现认知能力的框架。
它的研究对象是如何从感知、认知到决策,甚至到学习和记忆等高阶认知过程。
在神经科学中,对认知计算模型的研究对理解大脑的认知运算机制,解释各种认知现象和疾病都有重要作用。
首先,介绍下认知计算模型的发展历史。
认知计算模型的研究从上世纪40年代的新型计算技术,在上世纪70年代的开始形成体系。
现有的认知计算模型主要包括符号计算模型、连接计算模型和混合计算模型三类。
符号计算模型将人类认知过程看作一系列规则化的符号化运算,通过逻辑代数、产生式规则等数学方法来对认知内容进行描述,从而构建一个符号系统。
连接计算模型是将神经元作为基本组成原件,利用神经元之间的连接和交互以及他们之间相互调节的方式来实现认知计算。
混合计算模型则将符号计算模型和连接计算模型的优点融合在一起,通过建立符号和神经元之间的映射关系来实现认知计算。
其次,神经科学中的认知计算模型研究主要涉及那些内容呢?在感知、认知和决策这些基础认知方面,模型可以建立图像、声音、语言等信息的形式化表示,探究基础认知过程如何抽象、处理信息,构建知识结构。
在学习和记忆等高阶认知方面,模型可研究人类的长期记忆、工作记忆等工作机制,阐释人类记忆被遗忘和重新学习的现象。
在疾病治疗方面,可利用认知计算模型来了解和治疗认知障碍、失语等疾病。
在人工智能领域,认知计算模型可以为智能机器人、循环神经网络等技术提供算法支持。
最后,介绍下认知计算模型研究的应用领域。
认知计算模型研究成果广泛应用于诸多领域。
在人机交互应用方面,可以通过用户行为与反馈信息对应的方式,提高人工智能的智能化水平,实现更为精准、自然、高效的人机交互。
在医疗信息学应用方面,可以扩展医疗域的知识和概念,支持临床决策和远程医疗。
在智能交通和智能制造等应用领域中,可以提高智能型设备的自主性和交互性,实现有物联网性质的智能交通和智能制造。
Semi-Supervised Learning Using Gaussian Fields and Harmonic Functions

Xiaojin Zhu ZHUXJ@ Zoubin Ghahramani ZOUBIN@ John Lafferty LAFFERTY@ School of Computer Science,Carnegie Mellon University,Pittsburgh PA15213,USAGatsby Computational Neuroscience Unit,University College London,London WC1N3AR,UKAbstractAn approach to semi-supervised learning is pro-posed that is based on a Gaussian randomfieldbeled and unlabeled data are rep-resented as vertices in a weighted graph,withedge weights encoding the similarity between in-stances.The learning problem is then formulatedin terms of a Gaussian randomfield on this graph,where the mean of thefield is characterized interms of harmonic functions,and is efficientlyobtained using matrix methods or belief propa-gation.The resulting learning algorithms haveintimate connections with random walks,elec-tric networks,and spectral graph theory.We dis-cuss methods to incorporate class priors and thepredictions of classifiers obtained by supervisedlearning.We also propose a method of parameterlearning by entropy minimization,and show thealgorithm’s ability to perform feature selection.Promising experimental results are presented forsynthetic data,digit classification,and text clas-sification tasks.1.IntroductionIn many traditional approaches to machine learning,a tar-get function is estimated using labeled data,which can be thought of as examples given by a“teacher”to a“student.”Labeled examples are often,however,very time consum-ing and expensive to obtain,as they require the efforts of human annotators,who must often be quite skilled.For in-stance,obtaining a single labeled example for protein shape classification,which is one of the grand challenges of bio-logical and computational science,requires months of ex-pensive analysis by expert crystallographers.The problem of effectively combining unlabeled data with labeled data is therefore of central importance in machine learning.The semi-supervised learning problem has attracted an in-creasing amount of interest recently,and several novel ap-proaches have been proposed;we refer to(Seeger,2001) for an overview.Among these methods is a promising fam-ily of techniques that exploit the“manifold structure”of the data;such methods are generally based upon an assumption that similar unlabeled examples should be given the same classification.In this paper we introduce a new approach to semi-supervised learning that is based on a randomfield model defined on a weighted graph over the unlabeled and labeled data,where the weights are given in terms of a sim-ilarity function between instances.Unlike other recent work based on energy minimization and randomfields in machine learning(Blum&Chawla, 2001)and image processing(Boykov et al.,2001),we adopt Gaussianfields over a continuous state space rather than randomfields over the discrete label set.This“re-laxation”to a continuous rather than discrete sample space results in many attractive properties.In particular,the most probable configuration of thefield is unique,is character-ized in terms of harmonic functions,and has a closed form solution that can be computed using matrix methods or loopy belief propagation(Weiss et al.,2001).In contrast, for multi-label discrete randomfields,computing the low-est energy configuration is typically NP-hard,and approxi-mation algorithms or other heuristics must be used(Boykov et al.,2001).The resulting classification algorithms for Gaussianfields can be viewed as a form of nearest neigh-bor approach,where the nearest labeled examples are com-puted in terms of a random walk on the graph.The learning methods introduced here have intimate connections with random walks,electric networks,and spectral graph the-ory,in particular heat kernels and normalized cuts.In our basic approach the solution is solely based on the structure of the data manifold,which is derived from data features.In practice,however,this derived manifold struc-ture may be insufficient for accurate classification.WeProceedings of the Twentieth International Conference on Machine Learning(ICML-2003),Washington DC,2003.Figure1.The randomfields used in this work are constructed on labeled and unlabeled examples.We form a graph with weighted edges between instances(in this case scanned digits),with labeled data items appearing as special“boundary”points,and unlabeled points as“interior”points.We consider Gaussian randomfields on this graph.show how the extra evidence of class priors can help classi-fication in Section4.Alternatively,we may combine exter-nal classifiers using vertex weights or“assignment costs,”as described in Section5.Encouraging experimental re-sults for synthetic data,digit classification,and text clas-sification tasks are presented in Section7.One difficulty with the randomfield approach is that the right choice of graph is often not entirely clear,and it may be desirable to learn it from data.In Section6we propose a method for learning these weights by entropy minimization,and show the algorithm’s ability to perform feature selection to better characterize the data manifold.2.Basic FrameworkWe suppose there are labeled points, and unlabeled points;typically. Let be the total number of data points.To be-gin,we assume the labels are binary:.Consider a connected graph with nodes correspond-ing to the data points,with nodes corre-sponding to the labeled points with labels,and nodes corresponding to the unla-beled points.Our task is to assign labels to nodes.We assume an symmetric weight matrix on the edges of the graph is given.For example,when,the weight matrix can be(2)To assign a probability distribution on functions,we form the Gaussianfieldfor(3) which is consistent with our prior notion of smoothness of with respect to the graph.Expressed slightly differently, ,where.Because of the maximum principle of harmonic functions(Doyle&Snell,1984),is unique and is either a constant or it satisfiesfor.To compute the harmonic solution explicitly in terms of matrix operations,we split the weight matrix(and sim-ilarly)into4blocks after the th row and column:(4) Letting where denotes the values on the un-labeled data points,the harmonic solution subject to is given by(5)Figure2.Demonstration of harmonic energy minimization on twosynthetic rge symbols indicate labeled data,otherpoints are unlabeled.In this paper we focus on the above harmonic function as abasis for semi-supervised classification.However,we em-phasize that the Gaussian randomfield model from which this function is derived provides the learning frameworkwith a consistent probabilistic semantics.In the following,we refer to the procedure described aboveas harmonic energy minimization,to underscore the har-monic property(3)as well as the objective function being minimized.Figure2demonstrates the use of harmonic en-ergy minimization on two synthetic datasets.The leftfigure shows that the data has three bands,with,, and;the rightfigure shows two spirals,with,,and.Here we see harmonic energy minimization clearly follows the structure of data, while obviously methods such as kNN would fail to do so.3.Interpretation and ConnectionsAs outlined briefly in this section,the basic framework pre-sented in the previous section can be viewed in several fun-damentally different ways,and these different viewpoints provide a rich and complementary set of techniques for rea-soning about this approach to the semi-supervised learning problem.3.1.Random Walks and Electric NetworksImagine a particle walking along the graph.Starting from an unlabeled node,it moves to a node with proba-bility after one step.The walk continues until the par-ticle hits a labeled node.Then is the probability that the particle,starting from node,hits a labeled node with label1.Here the labeled data is viewed as an“absorbing boundary”for the random walk.This view of the harmonic solution indicates that it is closely related to the random walk approach of Szummer and Jaakkola(2001),however there are two major differ-ences.First,wefix the value of on the labeled points, and second,our solution is an equilibrium state,expressed in terms of a hitting time,while in(Szummer&Jaakkola,2001)the walk crucially depends on the time parameter. We will return to this point when discussing heat kernels. An electrical network interpretation is given in(Doyle& Snell,1984).Imagine the edges of to be resistors with conductance.We connect nodes labeled to a positive voltage source,and points labeled to ground.Thenis the voltage in the resulting electric network on each of the unlabeled nodes.Furthermore minimizes the energy dissipation of the electric network for the given.The harmonic property here follows from Kirchoff’s and Ohm’s laws,and the maximum principle then shows that this is precisely the same solution obtained in(5).3.2.Graph KernelsThe solution can be viewed from the viewpoint of spec-tral graph theory.The heat kernel with time parameter on the graph is defined as.Here is the solution to the heat equation on the graph with initial conditions being a point source at at time.Kondor and Lafferty(2002)propose this as an appropriate kernel for machine learning with categorical data.When used in a kernel method such as a support vector machine,the kernel classifier can be viewed as a solution to the heat equation with initial heat sourceson the labeled data.The time parameter must,however, be chosen using an auxiliary technique,for example cross-validation.Our algorithm uses a different approach which is indepen-dent of,the diffusion time.Let be the lower right submatrix of.Since,it is the Laplacian restricted to the unlabeled nodes in.Consider the heat kernel on this submatrix:.Then describes heat diffusion on the unlabeled subgraph with Dirichlet boundary conditions on the labeled nodes.The Green’s function is the inverse operator of the restricted Laplacian,,which can be expressed in terms of the integral over time of the heat kernel:(6) The harmonic solution(5)can then be written asor(7)Expression(7)shows that this approach can be viewed as a kernel classifier with the kernel and a specific form of kernel machine.(See also(Chung&Yau,2000),where a normalized Laplacian is used instead of the combinatorial Laplacian.)From(6)we also see that the spectrum of is ,where is the spectrum of.This indicates a connection to the work of Chapelle et al.(2002),who ma-nipulate the eigenvalues of the Laplacian to create variouskernels.A related approach is given by Belkin and Niyogi (2002),who propose to regularize functions on by select-ing the top normalized eigenvectors of corresponding to the smallest eigenvalues,thus obtaining the bestfit toin the least squares sense.We remark that ourfits the labeled data exactly,while the order approximation may not.3.3.Spectral Clustering and Graph MincutsThe normalized cut approach of Shi and Malik(2000)has as its objective function the minimization of the Raleigh quotient(8)subject to the constraint.The solution is the second smallest eigenvector of the generalized eigenvalue problem .Yu and Shi(2001)add a grouping bias to the normalized cut to specify which points should be in the same group.Since labeled data can be encoded into such pairwise grouping constraints,this technique can be applied to semi-supervised learning as well.In general, when is close to block diagonal,it can be shown that data points are tightly clustered in the eigenspace spanned by thefirst few eigenvectors of(Ng et al.,2001a;Meila &Shi,2001),leading to various spectral clustering algo-rithms.Perhaps the most interesting and substantial connection to the methods we propose here is the graph mincut approach proposed by Blum and Chawla(2001).The starting point for this work is also a weighted graph,but the semi-supervised learning problem is cast as one offinding a minimum-cut,where negative labeled data is connected (with large weight)to a special source node,and positive labeled data is connected to a special sink node.A mini-mum-cut,which is not necessarily unique,minimizes the objective function,and label0other-wise.We call this rule the harmonic threshold(abbreviated “thresh”below).In terms of the random walk interpreta-tion,ifmakes sense.If there is reason to doubt this assumption,it would be reasonable to attach dongles to labeled nodes as well,and to move the labels to these new nodes.6.Learning the Weight MatrixPreviously we assumed that the weight matrix is given andfixed.In this section,we investigate learning weight functions of the form given by equation(1).We will learn the’s from both labeled and unlabeled data;this will be shown to be useful as a feature selection mechanism which better aligns the graph structure with the data.The usual parameter learning criterion is to maximize the likelihood of labeled data.However,the likelihood crite-rion is not appropriate in this case because the values for labeled data arefixed during training,and moreover likeli-hood doesn’t make sense for the unlabeled data because we do not have a generative model.We propose instead to use average label entropy as a heuristic criterion for parameter learning.The average label entropy of thefield is defined as(13) using the fact that.Both and are sub-matrices of.In the above derivation we use as label probabilities di-rectly;that is,class.If we incorpo-rate class prior information,or combine harmonic energy minimization with other classifiers,it makes sense to min-imize entropy on the combined probabilities.For instance, if we incorporate a class prior using CMN,the probability is given bylabeled set size a c c u r a c yFigure 3.Harmonic energy minimization on digits “1”vs.“2”(left)and on all 10digits (middle)and combining voted-perceptron with harmonic energy minimization on odd vs.even digits (right)Figure 4.Harmonic energy minimization on PC vs.MAC (left),baseball vs.hockey (middle),and MS-Windows vs.MAC (right)10trials.In each trial we randomly sample labeled data from the entire dataset,and use the rest of the images as unlabeled data.If any class is absent from the sampled la-beled set,we redo the sampling.For methods that incorpo-rate class priors ,we estimate from the labeled set with Laplace (“add one”)smoothing.We consider the binary problem of classifying digits “1”vs.“2,”with 1100images in each class.We report aver-age accuracy of the following methods on unlabeled data:thresh,CMN,1NN,and a radial basis function classifier (RBF)which classifies to class 1iff .RBF and 1NN are used simply as baselines.The results are shown in Figure 3.Clearly thresh performs poorly,because the values of are generally close to 1,so the major-ity of examples are classified as digit “1”.This shows the inadequacy of the weight function (1)based on pixel-wise Euclidean distance.However the relative rankings ofare useful,and when coupled with class prior information significantly improved accuracy is obtained.The greatest improvement is achieved by the simple method CMN.We could also have adjusted the decision threshold on thresh’s solution ,so that the class proportion fits the prior .This method is inferior to CMN due to the error in estimating ,and it is not shown in the plot.These same observations are also true for the experiments we performed on several other binary digit classification problems.We also consider the 10-way problem of classifying digits “0”through ’9’.We report the results on a dataset with in-tentionally unbalanced class sizes,with 455,213,129,100,754,970,275,585,166,353examples per class,respec-tively (noting that the results on a balanced dataset are sim-ilar).We report the average accuracy of thresh,CMN,RBF,and 1NN.These methods can handle multi-way classifica-tion directly,or with slight modification in a one-against-all fashion.As the results in Figure 3show,CMN again im-proves performance by incorporating class priors.Next we report the results of document categorization ex-periments using the 20newsgroups dataset.We pick three binary problems:PC (number of documents:982)vs.MAC (961),MS-Windows (958)vs.MAC,and base-ball (994)vs.hockey (999).Each document is minimally processed into a “tf.idf”vector,without applying header re-moval,frequency cutoff,stemming,or a stopword list.Two documents are connected by an edge if is among ’s 10nearest neighbors or if is among ’s 10nearest neigh-bors,as measured by cosine similarity.We use the follow-ing weight function on the edges:(16)We use one-nearest neighbor and the voted perceptron al-gorithm (Freund &Schapire,1999)(10epochs with a lin-ear kernel)as baselines–our results with support vector ma-chines are comparable.The results are shown in Figure 4.As before,each point is the average of10random tri-als.For this data,harmonic energy minimization performsmuch better than the baselines.The improvement from the class prior,however,is less significant.An explanation for why this approach to semi-supervised learning is so effec-tive on the newsgroups data may lie in the common use of quotations within a topic thread:document quotes partof document,quotes part of,and so on.Thus, although documents far apart in the thread may be quite different,they are linked by edges in the graphical repre-sentation of the data,and these links are exploited by the learning algorithm.7.1.Incorporating External ClassifiersWe use the voted-perceptron as our external classifier.For each random trial,we train a voted-perceptron on the la-beled set,and apply it to the unlabeled set.We then use the 0/1hard labels for dongle values,and perform harmonic energy minimization with(10).We use.We evaluate on the artificial but difficult binary problem of classifying odd digits vs.even digits;that is,we group “1,3,5,7,9”and“2,4,6,8,0”into two classes.There are400 images per digit.We use second order polynomial kernel in the voted-perceptron,and train for10epochs.Figure3 shows the results.The accuracy of the voted-perceptron on unlabeled data,averaged over trials,is marked VP in the plot.Independently,we run thresh and CMN.Next we combine thresh with the voted-perceptron,and the result is marked thresh+VP.Finally,we perform class mass nor-malization on the combined result and get CMN+VP.The combination results in higher accuracy than either method alone,suggesting there is complementary information used by each.7.2.Learning the Weight MatrixTo demonstrate the effects of estimating,results on a toy dataset are shown in Figure5.The upper grid is slightly tighter than the lower grid,and they are connected by a few data points.There are two labeled examples,marked with large symbols.We learn the optimal length scales for this dataset by minimizing entropy on unlabeled data.To simplify the problem,wefirst tie the length scales in the two dimensions,so there is only a single parameter to learn.As noted earlier,without smoothing,the entropy approaches the minimum at0as.Under such con-ditions,the results of harmonic energy minimization are usually undesirable,and for this dataset the tighter grid “invades”the sparser one as shown in Figure5(a).With smoothing,the“nuisance minimum”at0gradually disap-pears as the smoothing factor grows,as shown in FigureFigure5.The effect of parameter on harmonic energy mini-mization.(a)If unsmoothed,as,and the algorithm performs poorly.(b)Result at optimal,smoothed with(c)Smoothing helps to remove the entropy minimum. 5(c).When we set,the minimum entropy is0.898 bits at.Harmonic energy minimization under this length scale is shown in Figure5(b),which is able to dis-tinguish the structure of the two grids.If we allow a separate for each dimension,parameter learning is more dramatic.With the same smoothing of ,keeps growing towards infinity(we usefor computation)while stabilizes at0.65, and we reach a minimum entropy of0.619bits.In this case is legitimate;it means that the learning al-gorithm has identified the-direction as irrelevant,based on both the labeled and unlabeled data.Harmonic energy minimization under these parameters gives the same clas-sification as shown in Figure5(b).Next we learn’s for all256dimensions on the“1”vs.“2”digits dataset.For this problem we minimize the entropy with CMN probabilities(15).We randomly pick a split of 92labeled and2108unlabeled examples,and start with all dimensions sharing the same as in previous ex-periments.Then we compute the derivatives of for each dimension separately,and perform gradient descent to min-imize the entropy.The result is shown in Table1.As entropy decreases,the accuracy of CMN and thresh both increase.The learned’s shown in the rightmost plot of Figure6range from181(black)to465(white).A small (black)indicates that the weight is more sensitive to varia-tions in that dimension,while the opposite is true for large (white).We can discern the shapes of a black“1”and a white“2”in thisfigure;that is,the learned parametersCMNstart97.250.73%0.654298.020.39%Table1.Entropy of CMN and accuracies before and after learning ’s on the“1”vs.“2”dataset.Figure6.Learned’s for“1”vs.“2”dataset.From left to right: average“1”,average“2”,initial’s,learned’s.exaggerate variations within class“1”while suppressing variations within class“2”.We have observed that with the default parameters,class“1”has much less variation than class“2”;thus,the learned parameters are,in effect, compensating for the relative tightness of the two classes in feature space.8.ConclusionWe have introduced an approach to semi-supervised learn-ing based on a Gaussian randomfield model defined with respect to a weighted graph representing labeled and unla-beled data.Promising experimental results have been pre-sented for text and digit classification,demonstrating that the framework has the potential to effectively exploit the structure of unlabeled data to improve classification accu-racy.The underlying randomfield gives a coherent proba-bilistic semantics to our approach,but this paper has con-centrated on the use of only the mean of thefield,which is characterized in terms of harmonic functions and spectral graph theory.The fully probabilistic framework is closely related to Gaussian process classification,and this connec-tion suggests principled ways of incorporating class priors and learning hyperparameters;in particular,it is natural to apply evidence maximization or the generalization er-ror bounds that have been studied for Gaussian processes (Seeger,2002).Our work in this direction will be reported in a future publication.ReferencesBelkin,M.,&Niyogi,P.(2002).Using manifold structure for partially labelled classification.Advances in Neural Information Processing Systems,15.Blum,A.,&Chawla,S.(2001).Learning from labeled and unlabeled data using graph mincuts.Proc.18th Interna-tional Conf.on Machine Learning.Boykov,Y.,Veksler,O.,&Zabih,R.(2001).Fast approx-imate energy minimization via graph cuts.IEEE Trans. on Pattern Analysis and Machine Intelligence,23. Chapelle,O.,Weston,J.,&Sch¨o lkopf,B.(2002).Cluster kernels for semi-supervised learning.Advances in Neu-ral Information Processing Systems,15.Chung,F.,&Yau,S.(2000).Discrete Green’s functions. Journal of Combinatorial Theory(A)(pp.191–214). Doyle,P.,&Snell,J.(1984).Random walks and electric networks.Mathematical Assoc.of America. Freund,Y.,&Schapire,R.E.(1999).Large margin classi-fication using the perceptron algorithm.Machine Learn-ing,37(3),277–296.Hull,J.J.(1994).A database for handwritten text recog-nition research.IEEE Transactions on Pattern Analysis and Machine Intelligence,16.Kondor,R.I.,&Lafferty,J.(2002).Diffusion kernels on graphs and other discrete input spaces.Proc.19th Inter-national Conf.on Machine Learning.Le Cun,Y.,Boser, B.,Denker,J.S.,Henderson, D., Howard,R.E.,Howard,W.,&Jackel,L.D.(1990). Handwritten digit recognition with a back-propagation network.Advances in Neural Information Processing Systems,2.Meila,M.,&Shi,J.(2001).A random walks view of spec-tral segmentation.AISTATS.Ng,A.,Jordan,M.,&Weiss,Y.(2001a).On spectral clus-tering:Analysis and an algorithm.Advances in Neural Information Processing Systems,14.Ng,A.Y.,Zheng,A.X.,&Jordan,M.I.(2001b).Link analysis,eigenvectors and stability.International Joint Conference on Artificial Intelligence(IJCAI). Seeger,M.(2001).Learning with labeled and unlabeled data(Technical Report).University of Edinburgh. Seeger,M.(2002).PAC-Bayesian generalization error bounds for Gaussian process classification.Journal of Machine Learning Research,3,233–269.Shi,J.,&Malik,J.(2000).Normalized cuts and image segmentation.IEEE Transactions on Pattern Analysis and Machine Intelligence,22,888–905.Szummer,M.,&Jaakkola,T.(2001).Partially labeled clas-sification with Markov random walks.Advances in Neu-ral Information Processing Systems,14.Weiss,Y.,,&Freeman,W.T.(2001).Correctness of belief propagation in Gaussian graphical models of arbitrary topology.Neural Computation,13,2173–2200.Yu,S.X.,&Shi,J.(2001).Grouping with bias.Advances in Neural Information Processing Systems,14.。
有限单元法基础
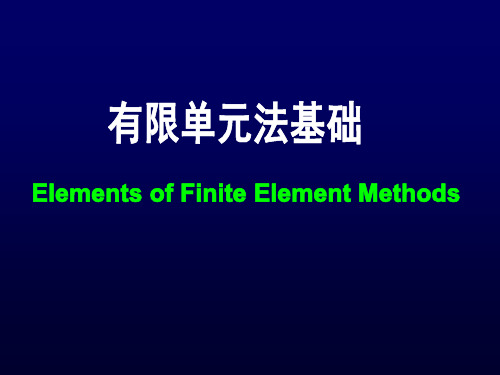
性体在各节点处的位移解。
3、单元分析---三角形单元
y
3.1 单元的结点位移和结点力向量
从离散化的网格中任取一个单元。三个结点 按反时针方向的顺序编号为:i, j, m。
结点坐标: (xi,yi) , (xj,yj) , (xm,ym) 结点位移: (ui,vi) , (uj,yj) , (um,vm) 共有6个自由度
单元位移插值函数: u(x, y) a1 a2 x a3 y
(3.1)
v(x, y) a4 a5x a6 y
插值函数的系数: a1 aiui a ju j amum / 2 A, a4 aivi a jv j amvm / 2 A,
a2 biui bju j bmum / 2 A, a5 bivi bjv j bmvm / 2 A,
um a1 a2 xm a3 ym , vm a4 a5 xm a6 ym ,
求解以上方程组得到以节点位移和节点坐标表示的6个参数:
a1 aiui a ju j amum / 2 A, a4 aivi a jv j amvm / 2 A, a2 biui bju j bmum / 2 A, a5 bivi bjv j bmvm / 2 A, a3 ciui c ju j cmum / 2 A, a6 civi c jv j cmvm / 2 A,
研究方法
从数学上讲它是微分方程边值问题(椭圆型微分方程、抛物型微分方程和双曲型微 分方程)的一种的数值解法,是一种将数学物理问题化为等价的变分问题的解法,并作 为一种通用的数值解法成为应用数学的一个重要分支。从物理上讲是将连续介质物理 场进行离散化,将无限自由度问题化为有限自由度问题的一种解方法。从固体力学上 认识,是瑞利-里兹法的推广。
生成代码的文本摘要(1)

⽣成代码的⽂本摘要(1)代码⽣成摘要该课题的当代进展,按照发展顺序排列:u IR检索技术该技术利⽤词性标注识别出最有可能体现代码特性的关键词;然后分析修正在词性标注过程中可能引⼊的错误;其次,对标识出的关键词进⾏降噪,以减少⽂本噪声带来的不利影响;最后,从关键词中选取若⼲个权值最⾼的词以组成代码摘要。
但是⾯临着关键词抽取困难等弊端。
⽂献:Automatic generation of natural language summaries for java classes.⽂献:Source code summarization technology based on syntactic analysis引⼊代码克隆检测技术检测相似⽚段,提取相似⽚段的注释信息。
⽂献:Clocom: Mining existing source code for automatic comment generation.u 建⽴源代码的语⾔模型:fault detection ⽂献:On the naturalness of buggy codecode completion ⽂献:A statistical semantic language model for source codecode summarization ⽂献:Summarizing source code using a neural attention model.u 基于深度学习&结合Attention机制l Attention机制的起源于计算机视觉⽂献:Recurrent Models of Visual Attentionl ⾸次将Attention机制⽤于NLP⽂献:Neural Machine Translation by Jointly Learning to Align and Translatel Goolge团队于2017引⼊了⾃注意⼒机制⽂献:Attention is All You Needl 将Attention机制⽤于⽣成代码摘要:n 深度学习模型:CODE-NN⽂献:Summarizing Source Code using a Neural Attention Model (师兄推荐)使⽤LSTM & Attention模型进⾏从源代码到⽂本摘要的过渡。
the_students_guide_to_cognitive_neuroscience 译本
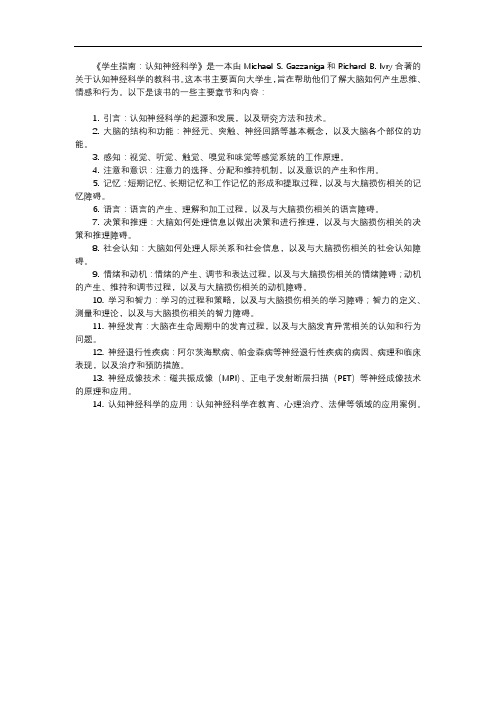
《学生指南:认知神经科学》是一本由Michael S. Gazzaniga和Richard B. Ivry合著的关于认知神经科学的教科书。
这本书主要面向大学生,旨在帮助他们了解大脑如何产生思维、情感和行为。
以下是该书的一些主要章节和内容:1. 引言:认知神经科学的起源和发展,以及研究方法和技术。
2. 大脑的结构和功能:神经元、突触、神经回路等基本概念,以及大脑各个部位的功能。
3. 感知:视觉、听觉、触觉、嗅觉和味觉等感觉系统的工作原理。
4. 注意和意识:注意力的选择、分配和维持机制,以及意识的产生和作用。
5. 记忆:短期记忆、长期记忆和工作记忆的形成和提取过程,以及与大脑损伤相关的记忆障碍。
6. 语言:语言的产生、理解和加工过程,以及与大脑损伤相关的语言障碍。
7. 决策和推理:大脑如何处理信息以做出决策和进行推理,以及与大脑损伤相关的决策和推理障碍。
8. 社会认知:大脑如何处理人际关系和社会信息,以及与大脑损伤相关的社会认知障碍。
9. 情绪和动机:情绪的产生、调节和表达过程,以及与大脑损伤相关的情绪障碍;动机的产生、维持和调节过程,以及与大脑损伤相关的动机障碍。
10. 学习和智力:学习的过程和策略,以及与大脑损伤相关的学习障碍;智力的定义、测量和理论,以及与大脑损伤相关的智力障碍。
11. 神经发育:大脑在生命周期中的发育过程,以及与大脑发育异常相关的认知和行为问题。
12. 神经退行性疾病:阿尔茨海默病、帕金森病等神经退行性疾病的病因、病理和临床表现,以及治疗和预防措施。
13. 神经成像技术:磁共振成像(MRI)、正电子发射断层扫描(PET)等神经成像技术的原理和应用。
14. 认知神经科学的应用:认知神经科学在教育、心理治疗、法律等领域的应用案例。
信息管理与信息系统专业英语Unit1~6 TextB 课文翻译

管理的角色和技能管理角色亨利·明茨伯格对执行者行为的研究让他得出这样的结论:经理都需要承担大量的角色。
一个角色是一组预期的行为对一个特定的位置。
明茨伯格的角色可以分为三大类如图1.1所示:信息角色(管理信息);人际角色(管理通过人)和决策角色(管理行动)。
每个角色代表活动经理承担最终完成的功能规划、组织、领导、控制。
重要的是要记住,真正的工作的管理不能练习作为一组独立的部分;所有的角色交互的方式在现实世界的管理。
图1.1 管理角色信息角色描述活动用来维持和发展一个信息网络。
这三个信息角色监督者、传播者和发言人。
监督者涉及从许多来源寻求当前的信息。
经理获得信息来自他人和扫描书面材料来保持消息灵通。
传播者和发言人的角色是正好相反。
经理把当前信息传递给他人,内部和外部的组织,才能使用它。
与授权趋势的低级别员工,很多经理都共享尽可能丰富的信息。
由于人际角色让经理们被叫去与众多组织和个人交互。
这三个人际角色是挂名首脑、领袖和交流与合作者。
这个挂名首脑角色专注于管理正式的和象征性的活动的部门或组织。
经理代表本组织在他或她作为单位的负责人的正式管理能力。
领导的作用是指经理的工作在激励下属,以满足单位的目标。
交流与合作者的作用来自于经理的责任与各种团体在组织内外交流。
一个例子是一个面对面讨论控制器和计划主管之间解决关于预算的一种误解。
决策角色指管理的决策过程。
这些角色通常需要概念以及人类的技能。
这四种管理角色都属于这一类企业家,障碍处理者,资源分配者,谈判代表。
一个管理者承担一个企业家的角色当他或她启动项目来提高部门或工作单位时。
当问题比如错过了交付关键客户的出现,经理必须采用一个障碍处理的角色。
决定如何分配单位的金钱、时间、材料和其他资源,称为经理的资源分配角色。
最后,谈判者角色指的是这种情况,经理必须代表单位和其他人的利益,如供应商、客户和政府。
根据一篇经典文章由罗伯特·l·卡茨,管理上的成功主要取决于性能而不是人格特质。
卡里加里博士名词解释
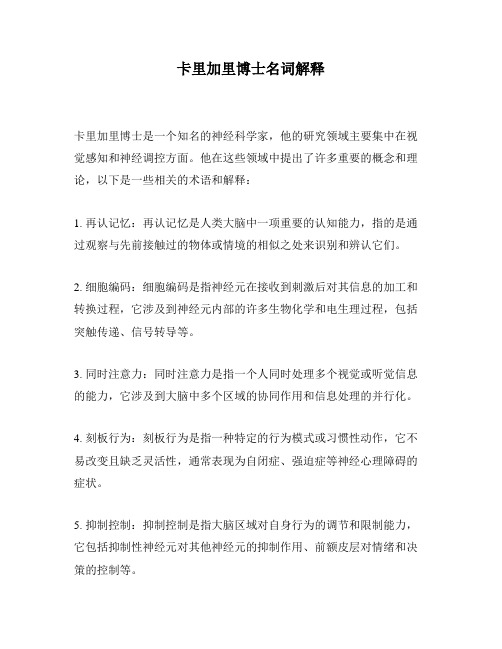
卡里加里博士名词解释
卡里加里博士是一个知名的神经科学家,他的研究领域主要集中在视觉感知和神经调控方面。
他在这些领域中提出了许多重要的概念和理论,以下是一些相关的术语和解释:
1. 再认记忆:再认记忆是人类大脑中一项重要的认知能力,指的是通过观察与先前接触过的物体或情境的相似之处来识别和辨认它们。
2. 细胞编码:细胞编码是指神经元在接收到刺激后对其信息的加工和转换过程,它涉及到神经元内部的许多生物化学和电生理过程,包括突触传递、信号转导等。
3. 同时注意力:同时注意力是指一个人同时处理多个视觉或听觉信息的能力,它涉及到大脑中多个区域的协同作用和信息处理的并行化。
4. 刻板行为:刻板行为是指一种特定的行为模式或习惯性动作,它不易改变且缺乏灵活性,通常表现为自闭症、强迫症等神经心理障碍的症状。
5. 抑制控制:抑制控制是指大脑区域对自身行为的调节和限制能力,它包括抑制性神经元对其他神经元的抑制作用、前额皮层对情绪和决策的控制等。
6. 知觉预测:知觉预测是指一个人根据自身的经验和知识,在接收到
部分信息时预测并补全其余的未知信息的过程,这个过程涉及到大脑
中的许多区域和神经机制。
7. 多感官整合:多感官整合是指神经系统将来自不同感觉通道的信息
整合起来,构成更全面、更准确的感知体验,它涉及到大脑中多个区
域的协同作用和信息融合的机制。
8. 神经干扰:神经干扰是指在大脑中某些区域的活动与其他区域的活
动发生冲突和干扰,在特定的条件下可能导致学习和行为表现的下降。
9. 神经调节:神经调节是指神经系统通过神经传递、神经调节剂等方
式对器官与组织的功能进行调节和平衡的过程,它与各个器官和系统
之间的相互关联密切相关。
神经科学核心辞汇翻译

精要速览系列(影印版)Instant Notes Neuroscience神经科学……………核心词汇翻译参考手册……………………按章节顺序:Section A Brain cellsA1 Neuron structureNeuron 神经细胞,神经元Subcellular organelles 亚细胞器Nissl body 尼氏体神经元中的粗面内质网形成的聚合物。
Neurite 神经突Axon 轴突Dendrite 树突Mitochondria 线粒体dendritic spines 树突脊树突在神经元上分化成几百个微小投射。
axon hillock 轴丘myelin sheath 髓鞘axon collalterals 轴突分枝terminals 突触末稍varicosities 曲张体microtubules 微管cytoskeleton 细胞骨架A2 Classes and numbers of neuronsMorphology 形态学Neurotranmitters 神经递质Unipolar 单极神经元仅有一个树突的神经元。
Bipolar 双极神经元Multipolar 多极神经元Pseudounipolar 假单极神经元生长出两个神经突,但随后融合Pyramidal cell 锥体细胞Purkinje cell 浦肯野氏细胞Projection neuron 投射神经元拥有长轴突的神经元Interneurons 中间神经元拥有短的轴突的神经元Afferent 传入Efferent 传出Sensory neuron 感觉神经元Motor neuron 运动神经元A3 Morphology of chemical synapseselectrical synapse 电突触chemical synapse 化学突触synaptic cleft 突触间隙axodendritic synapse 轴树突触轴突与树突之间的突触axosomatic synapse 轴体突触axoaxonal synapse 轴轴突触small clear synaptic vesicles(SSVs) 小清楚突触囊泡在突触前神经元存在的贮存递质的囊泡dense projections 致密突起active zone 活性区域postsynaptic density 突触致密物质large dense-core vesicles 庞大致密度中心囊泡A4 glial cells and myelinationGlial cells 胶质细胞是神经细胞的辅助细胞Astrocytes 星状细胞Oligodendrocytes 寡突细胞Schwann cells 许旺氏细胞围绕在神经细胞外的一种胶质细胞,形成髓鞘。
常用学术网址与链接(用于收集资料熟悉研究方向)(常更新)(发给研究生)

常用学术网址链接(用于收集资料熟悉研究方向)论坛部落类:1、小木虫论坛:/bbs/2、研学论坛/index.jsp3、子午学术论坛/bbs/index.php4、零点花园(内有大量基金报告)/bbs/5、科研基金网/6、5Q部落: 7、博士部落(包括求职、职务描绘、创业、科研资料、课题、论文、外语、计算机等,网页上推荐了不少好网站)8天下论坛9、清华BBS:10、科苑星空BBS:11、博研联盟/index.html(博士、博士后信息)12、源代码下载与搜索网站/(天空软件)13、软件性能分析程序VTune:分析在Intel芯片上运行的C或Fortran程序(高永华推荐)14、高校课件:15、FFTW: /用C语言编写的快速傅立叶变换程序(Fastest Fourier Transform in the West), 库文件及头文件放在E:\fftw. 使用时需将之拷贝至C:\Windows\system32研究机构与学者主页类:1.加州大学佰克利分校计算机系:2.北京邮电大学主页:有一些关于通信会议的信息及动态3.SVM用于语音识别[Mississippi state university institute for signal and information processing] Aravind ganapathiraju,Jonathan4.在北大bbs 上的语音处理:/学术讨论/语音语言处理5.Boosting 机器学习技术6.核ICA (另有机器学习的一些资源)7.郭天佑Tin-you KWOKt.hk/~jamesk朱海龙(博士)http://202.117.29.24/grzhy/zhuhailong/links.htm8.wiley出版社Springer出版社http://springer.de9.Christopher M.Bishop的主页/~cmbishop关于统计模式识别,写了一本书《Neural Networks for Pattern Recognition》10 Netlab的网址(一个机器学习与统计工具箱)/netlab/index.htmlncrg:神经计算研究组aston: aston大学(有博后职位)11高斯过程(Mackay Williams)/~carl.html(由Carl建立,也见105)12正则化网络(MITCBCL--Poggio)/projects/cbcl/13apnik的主页(提出了SVM)/info/vlad14ahba的主页(研究样条插值ANOV A 、RKHS等, 有博后职位) /~wahba/15Kernel Fisher discriminant [KFD]http://ida.first.gmd.de/hompages/mika/Fisher.html16SMO for LS-SVMS (贝叶斯与SVM).sg/~mpessk/publications.html17支持向量机、核方法(Cristianini)18Keerthi的主页(新加坡国立大学).sg/~mpessk19搜索国外FTP以及专业资料的网页20郭天佑的主页上有许多关于机器学习的链接有关于各种学术杂志的网站链接(统计神经网络方面)有研究神经网络、机器学习、统计方法、信息检索、文本分类、智能代理、手写体识别、计算机视觉及模式识别的机构及个人网址,有香港本地研究机构网址t.hk/~jamesk/others.html21数学资源22神经网络,神经计算研究资源/resource.html23Plivis: Probabilistic Hierachical interactive visualization 潜在变量分析软件包/Phivis24TM: generative topographic mapping 自组织映射(SOM)的概率统计方法/GTM25关于人工智能的参考书、学者、公司、研究组大全/ai.html26关于贝叶斯网的各种资源http://www-2.cs.cmu.2du/~stefann/Bayesian-learning.htm27R.Herbrich的主页(研究机器学习、贝叶斯点机器学习,目前在微软研究组http://stat.cs.tu-berlin.de/~ralfh28David J.C Mackay的主页(提出显著度框架,也研究GP和变分法等)/mackay/网站在加拿大的镜象:http://www//~mackay/README.html29Radford.M.Neal的主页(Monte Carlo模拟, 主页上有贝叶斯方法的程序) /~radford30一些书籍的pdf格式文件下载/theses/available31关于LS-SVMhttp://www.esat.kuleuven.ac.be/sista/lssvmlab/home.html32. IEEE主页:IEEE数据库:IEICE主页:(IEICE: The Institute of Electronics, Information and Communication Engineers) Spie主页:32SI公司主页(SCI是其主要产品)33中科院主页:中国科技信息网:34一个有许多数字书籍的ftp ftp://202.38.85.7535Chu Wei的主页(提出了SVM分段损失函数,主页上有源程序).sg/~chuweihttp://www.ai.univie.ac.at/~brain/pthesis/~chuwei36Beal的主页(关于Bayesian learning的变分法,主页上有源程序)/~bealE-mail: beal@37Nando的主页(MCMC, 变分推理,主页上有源程序)www.cs.ubc.ca/~nando/publications.html38Gatsby Computational Neuroscience Unit39Schölkopf 的最新主页(核方法的鼻祖)www.kyb.tuebingen.mpg.de/~bs40Tom Mitchell的主页(machine learning一书的作者,卡内基梅隆大学教授)/~tom/41卡内基梅隆大学CALD中心(机器学习,人机智能,属于计算机科学学院,center for Automated Learning and Discovery,同时有一些聚类、分类软件)42Mallick和Veerabhadram的主页(关于Bayesian+Spline)/~bmallick (教授)/~veera(研究生)43Denison的主页(关于Bayesian+Spline,写了一本书) /~dgtd44Holmes的主页(Bayesian+Spline,博士已毕业)/~ccholmes (提出MLS)45David Ruppert的主页(关于Bayesian+Spline,写了一本书Semiparametric regression, 主要研究方向:Penalized splines, MCMC, Semiparametric Modeling, Local Polynomial; Additive models; Spatial model; Interaction models)(资料已下载放在E盘)/~davidr46Zhou Ding-Xuan的主页(提出了RKHS的覆盖数、容量等).hk/~mazhou47(香港)大学教育资助委员会.hk48美国数学协会(AMS), 出版proceedings of AMS和Trans of AMS等49Grudic的主页(关于Machine learning,有博后职位)/~grudic50美国计算机协会中国电子学会51Thomas Strohmann的主页(关于Minimax Probability Machine)/~strohmanLanckriet的主页(关于Minimax Probability Machine)/~gert52关于贝叶斯和统计的网站,网站上有软件可下载的软件有:Belief Networks; Poly-Splines; MC Inference; Poly-mars53 Association for uncertainty in AI,在其resource中有一些链接,主要有Bayes net; Decision analysis; machine learning; PR等54 机器学习(ML)资源大全ML 软件; ML Benchmarks; ML papers, Bibliographies, Journals, Organization,研究ML公司,出版社, ML Conferences等网站上有相关专题:Inductive logic programming; Data mining; Conceptual clustering; Reinforcement learning; Genetic algorithm; NN; Computational learning http://www.ai.univie.ac.at/oefai/ml/ml-resources.html55 达夫特大学模式识别资源大全研究领域、期刊、书、文章、研究小组等介绍,Job announcement栏目里有大量博后职位http://www.ph.tn.tudelft.nl/PRInfo/index.html56 核方法主页由Shawe-Taylor建立,Kernel methods for pattern analysis一书的主页57一个机器学习资源更丰富的站点,Jobs栏目里有一些博后职位/~aha/research/machine-learning.html58 博后职位在线Current listings of post-docs online,另外在google中可直接链入“pos tdoctoral position”进行搜索,在北大、清华的BBS中也有博后版59 Michael I. Jordan的主页(徐雷的老师,有博后职位)/~jordan60徐雷的主页.hk/~lxu/61Arnaud Doucet的主页(研究Sequential Monte Carlo和Particle Filtering)Nando的老师,有博士后职位/~ad2/arnaud_doucet.html62统计多媒体学习组,Statistical Multimedia Learning Groupwww.cs.ubc.ca/nest/lci/sml63剑桥大学统计实验室(数学学院/数学统计系)64CMC资料大全/~mcmc65研究贝叶斯统计的学者主页,Bayesian Statistics Personal Web Pages /~madigan/bayes-people.html66新语丝(学术打假) 67中国科技在线科技咨询、科技成果(863计划,火炬计划等)、科研机构、科技资料68 新加坡高性能计算中心Institute of High Performance Computing, Singapore,有博后职位,由“a comparison of PCA,FPCA and ICA...”一文发现69芬兰HUT,Helsinki University of Technology,Neural Networks Research center,Laboratory of computer and information,有博后职位www.cis.hut.fi/jobs70 ICA研究主页关于ICA的程序,研究人员,论文等(ICA for communication)http://www.cis.hut.fi/projects/ica71 SOM研究主页http://www.cis.hut.fi/projects/somtoolbox72tefan Harmeling的主页(研究基于核的盲源分离)http://www.first.fhg.de/~harmeli/index.html73.Muller的主页(研究SVM)http://www.first.fhg.de/persons/mueller.klaus-robert.html73iehe的主页(提出了关于盲源分离的一种新方法TDSEP)http://www.first.fhg.de/~ziehe/74王力波的主页(南洋理工大学博士,人工神经网络,软计算).sg/home/elpwang75Roman Rosipal的主页(研究核偏最小二乘KPLS)http://aiolos.um.savba.sk/rosi76IEEE北京地区分会/relations/IEEE%20BJ/index.htm77.周志华的主页(南京大学计算机系教授,研究机器学习)/people/zhouzh78.C. K .I. Williams的主页(研究高斯过程)/homes/ckiw79.P. Sollich的主页(研究贝叶斯学习)/~psollich80.Carl Edward Rasmussen的主页(研究高斯过程,建立了一个高斯过程网站)/~edward81 Santa Fe 时间序列预测分析竞赛(由Andreas主持)/~andreas/Time-Series/SantaFe.html82 Andreas的主页/~andreas83张志华t.hk/~zhzhangResearch interests(1)Bayesian Statistics (mixture model\graphical models(2)Machine learning (KM\spectral-graph)(3)Applications84 Dit-Yan-Yeung 的主页(Kwok的老师)t.hk/faculty/dyyeung/index.html85I Group at UST (Yeung是其中一员)t.hk/aigroup86 NIPS (neural information processing systems)/web/groups/NIPS(可下载NIPS会议集全文)87 JMLR(Journal of machine learning research) 杂志的主页/projects/jmlr能下载全文88 Neural Computation杂志的主页89 David Dowe的主页(研究混合模型)有各种混合模型的介绍与软件,比如GMM、Gamma分布的混合、对数分布的混合、Poisson分布的混合、Weibull分布的混合等.au/~dld/cluster.html90 Nell. D. Lawrence的主页(Bishop的学生,提出高斯过程潜变量模型GPLVM)/neil/ (老主页)/~neil(新主页)91 F. R. Bach的主页(提出KICA,将核方法与图模型结合)/~fbach92 Avrim Blum的主页(卡内基梅隆大学教授,研究机器学习)/~avrim93 学习理论大全,包括各种兴趣组、参考书、邮件列表,资源,COLT链接等94R.Schapire的主页(研究boosting)/~schapire95 T.Hastie的主页(主曲线的提出者,《统计学习基础》一书的作者)/~hastie/96.J.Friedman的主页(MARS、投影寻踪等方法的提出者)/people/faculty/friedman.html97最小最大概率机研究者主页nckriet: /~gertT.Strohmann: /~strohman98中国人工智能网99Kevin Murphy 的主页(研究概率图模型和贝叶斯网,并将之应用于计算机视觉有一个matlab工具箱BNT)/~murphyk或www.cs.ubc.ca/~murphyk100人脸识别学术网站/databases101 Sam Roweis的主页(多伦多大学助教,研究统计机器学习,主页上有NSPS,MNITS等手写体库和人脸库)/~roweis/102中国学术会议在线(网站内有很多国际会议消息)/index.jsp103史忠值的主页(中科院计算所信息处理实验室)104中科院数学与系统科学研究院105 A.Ronjyakotomam的主页(提出了小波核)http://asi.insa-rouen.fr/~arakotom106 Elad Yom-Tov的主页(与Duda和Strok开发了一个分类工具箱,《Computer Manual in Matlab to Accompany Pattern Classification》书的作者,该书是Duda模式分类一书的配套)/index.html107 G.Stork的主页(Pattern Classification一书的作者)/~stork108 Colin Fyfe的主页(研究SOM及其核版本、主曲线等)/fyfe-ci0/109 Dominique Mantinez的主页(提出基于核的盲源分离KBSS) http://www.loria.fr/~dmartine110 Andreas Ziehc的主页(KBSS 关于盲源分离的资料链接) http://idafirst.gmd.de/~Ziehe/research.html111 盲源分离欧洲项目(BLISS: Blind source separation and application) http://www.cis.inpg.fr/pages-paperso/bliss/index.php112 Gao Junbin 的主页(用贝叶斯方法实现SVM,有博后职位)http://athene.riv.csu.au/~jbgao/jbgao@.au113南安普敦大学电子与计算机科学系信号、图像、系统中心(有博后职位)/people114 David Zhang (张大鹏,香港理工大学教授,研究生物统计学, 有博后职位).hk/~csdzhang115自动生成计算机领域内的论文:/scigen116周志华的“机器学习与数据挖掘”研究组, 有机器学习领域内一些研究杂志与研究机构的链接/index_cn.htm117英文学术论文润色,检查可读性、语法、拼写、清晰度118黄德双的主页(中科大教授,中科院合肥智能机械研究所智能计算实验室)/119一个关于通信的ftp: 162.105.75.232有程序代码/书籍资料/通信文献/协议标准120一个关于DSP之类的ftp: http://202.38.73.175121合众达电子(关于DSP的入门网站)122、微波技术网/出国留学类:1、国外留学信息:国家留学网:/(国家留学基金委)中国留学网:/publish/portal0/tab171/2、欧洲中国留学生之家:3、我爱英语网:/tl/4、飞跃重洋:/5、英语学习太傻网:地球物理类:1、SEP: Stanford exploration project 斯坦福大学地震勘探工程以Claerbout为首的研究小组,网页内有源代码,人员介绍等。
普林斯顿 结构生物 类脑计算 经费

《普林斯顿:结构生物与类脑计算的探索与发展》一、引言在当今科技飞速发展的时代,结构生物学和类脑计算作为两大前沿交叉学科,对人类社会和科学技术发展产生了深远的影响。
作为这一领域的先锋,普林斯顿大学一直致力于对结构生物与类脑计算进行探索与发展。
本文将从普林斯顿大学的视角出发,全面评估和解析普林斯顿在这一领域的最新研究成果,并共享对结构生物与类脑计算的个人理解和观点。
二、普林斯顿:结构生物的前沿研究1. 普林斯顿在结构生物领域的权威地位作为一所拥有丰富研究资源和顶尖科研团队的顶尖大学,普林斯顿在结构生物领域拥有显著的研究优势。
在高分辨率结构生物学、蛋白质折叠与组装、大分子相互作用等方面,普林斯顿都有着卓越的研究成果和学术地位。
2. 普林斯顿的结构生物研究成果通过对普林斯顿在结构生物学领域的研究成果进行深入探讨,我们可以发现,普林斯顿在解析高分辨率蛋白质结构、研究生命大分子的结构与功能、探索生命活动的分子机制等方面取得了突破性进展。
这些成果不仅为生命科学领域的发展提供了重要的理论和实践支持,同时也为人类社会的生物医药、食品安全等方面带来了巨大的影响和推动力。
三、普林斯顿:类脑计算的前沿研究1. 普林斯顿在类脑计算领域的研究方向作为类脑计算领域的佼佼者,普林斯顿在神经科学、人工智能、认知科学等领域的研究成果备受关注。
通过对普林斯顿在类脑计算领域的研究方向进行全面评估,我们可以发现,普林斯顿在神经元信号传导模型、神经网络结构与功能的模拟、认知计算理论等方面取得了令人瞩目的成就。
2. 普林斯顿的类脑计算研究成果普林斯顿在类脑计算领域的研究成果不仅涉及基础理论研究,还包括脑-机器接口技术、人工智能系统的优化与应用、大规模脑网络行为建模等领域。
这些成果为人工智能、神经科学、认知计算等领域的交叉研究提供了重要的理论和技术支持,推动了类脑计算技术的迅速发展和应用。
四、结构生物与类脑计算的交叉研究1. 普林斯顿在结构生物与类脑计算交叉研究的探索普林斯顿在结构生物与类脑计算交叉研究的探索成果丰硕,涉及蛋白质与神经元的相互作用、大脑认知功能的生物结构基础、类脑计算技术在生物医学领域的应用等诸多领域。
脑部神经元格子的计算模型研究
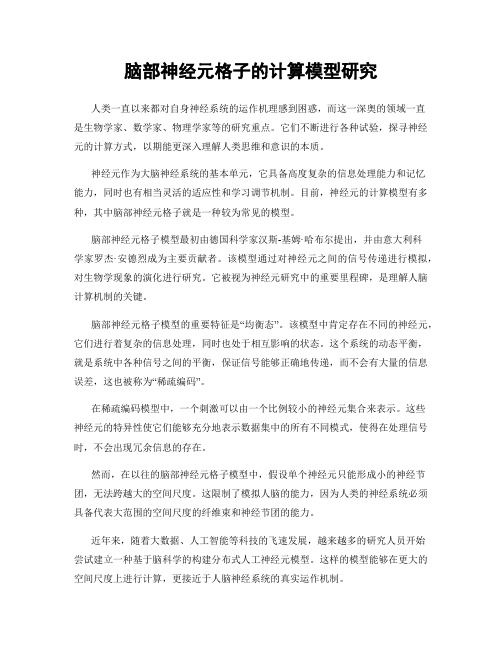
脑部神经元格子的计算模型研究人类一直以来都对自身神经系统的运作机理感到困惑,而这一深奥的领域一直是生物学家、数学家、物理学家等的研究重点。
它们不断进行各种试验,探寻神经元的计算方式,以期能更深入理解人类思维和意识的本质。
神经元作为大脑神经系统的基本单元,它具备高度复杂的信息处理能力和记忆能力,同时也有相当灵活的适应性和学习调节机制。
目前,神经元的计算模型有多种,其中脑部神经元格子就是一种较为常见的模型。
脑部神经元格子模型最初由德国科学家汉斯-基姆·哈布尔提出,并由意大利科学家罗杰·安德烈成为主要贡献者。
该模型通过对神经元之间的信号传递进行模拟,对生物学现象的演化进行研究。
它被视为神经元研究中的重要里程碑,是理解人脑计算机制的关键。
脑部神经元格子模型的重要特征是“均衡态”。
该模型中肯定存在不同的神经元,它们进行着复杂的信息处理,同时也处于相互影响的状态。
这个系统的动态平衡,就是系统中各种信号之间的平衡,保证信号能够正确地传递,而不会有大量的信息误差,这也被称为“稀疏编码”。
在稀疏编码模型中,一个刺激可以由一个比例较小的神经元集合来表示。
这些神经元的特异性使它们能够充分地表示数据集中的所有不同模式,使得在处理信号时,不会出现冗余信息的存在。
然而,在以往的脑部神经元格子模型中,假设单个神经元只能形成小的神经节团,无法跨越大的空间尺度。
这限制了模拟人脑的能力,因为人类的神经系统必须具备代表大范围的空间尺度的纤维束和神经节团的能力。
近年来,随着大数据、人工智能等科技的飞速发展,越来越多的研究人员开始尝试建立一种基于脑科学的构建分布式人工神经元模型。
这样的模型能够在更大的空间尺度上进行计算,更接近于人脑神经系统的真实运作机制。
为此,科学家们不断在脑部神经元格子模型的架构上进行创新,引入新的机器学习算法和数据分析方法。
他们同样也在自然智能计算中,引入神经科学的理论和计算模型,以提高机器学习和主流人工智能算法的性能。
课文参考译文 (1)-信息科学与电子工程专业英语(第2版)-吴雅婷-清华大学出版社
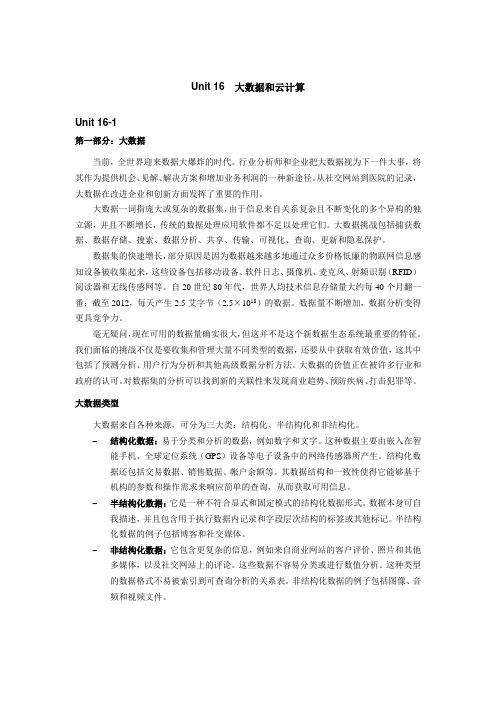
Unit 16 大数据和云计算Unit 16-1第一部分:大数据当前,全世界迎来数据大爆炸的时代。
行业分析师和企业把大数据视为下一件大事,将其作为提供机会、见解、解决方案和增加业务利润的一种新途径。
从社交网站到医院的记录,大数据在改进企业和创新方面发挥了重要的作用。
大数据一词指庞大或复杂的数据集,由于信息来自关系复杂且不断变化的多个异构的独立源,并且不断增长,传统的数据处理应用软件都不足以处理它们。
大数据挑战包括捕获数据、数据存储、搜索、数据分析、共享、传输、可视化、查询、更新和隐私保护。
数据集的快速增长,部分原因是因为数据越来越多地通过众多价格低廉的物联网信息感知设备被收集起来,这些设备包括移动设备、软件日志、摄像机、麦克风、射频识别(RFID)阅读器和无线传感网等。
自20世纪80年代,世界人均技术信息存储量大约每40个月翻一番;截至2012,每天产生2.5艾字节(2.5×1018)的数据。
数据量不断增加,数据分析变得更具竞争力。
毫无疑问,现在可用的数据量确实很大,但这并不是这个新数据生态系统最重要的特征。
我们面临的挑战不仅是要收集和管理大量不同类型的数据,还要从中获取有效价值,这其中包括了预测分析、用户行为分析和其他高级数据分析方法。
大数据的价值正在被许多行业和政府的认可。
对数据集的分析可以找到新的关联性来发现商业趋势、预防疾病、打击犯罪等。
大数据类型大数据来自各种来源,可分为三大类:结构化、半结构化和非结构化。
-结构化数据:易于分类和分析的数据,例如数字和文字。
这种数据主要由嵌入在智能手机、全球定位系统(GPS)设备等电子设备中的网络传感器所产生。
结构化数据还包括交易数据、销售数据、帐户余额等。
其数据结构和一致性使得它能够基于机构的参数和操作需求来响应简单的查询,从而获取可用信息。
-半结构化数据:它是一种不符合显式和固定模式的结构化数据形式。
数据本身可自我描述,并且包含用于执行数据内记录和字段层次结构的标签或其他标记。
ucl英文简介
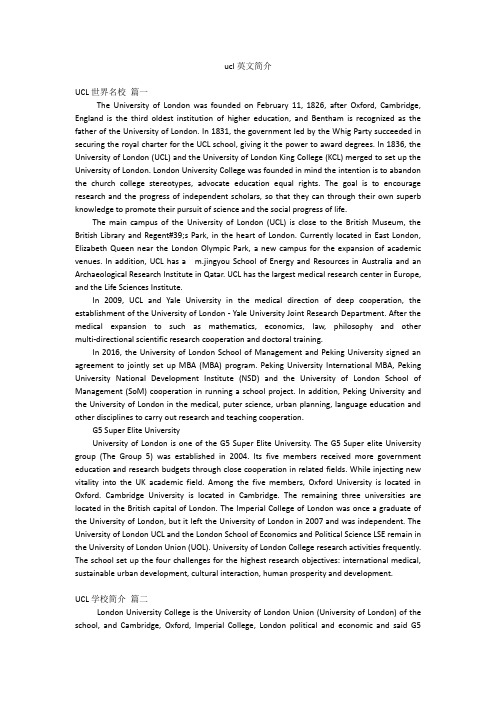
ucl英文简介UCL世界名校篇一The University of London was founded on February 11, 1826, after Oxford, Cambridge, England is the third oldest institution of higher education, and Bentham is recognized as the father of the University of London. In 1831, the government led by the Whig Party succeeded in securing the royal charter for the UCL school, giving it the power to award degrees. In 1836, the University of London (UCL) and the University of London King College (KCL) merged to set up the University of London. London University College was founded in mind the intention is to abandon the church college stereotypes, advocate education equal rights. The goal is to encourage research and the progress of independent scholars, so that they can through their own superb knowledge to promote their pursuit of science and the social progress of life.The main campus of the University of London (UCL) is close to the British Museum, the British Library and Regent#39;s Park, in the heart of London. Currently located in East London, Elizabeth Queen near the London Olympic Park, a new campus for the expansion of academic venues. In addition, UCL has a m.jingyou School of Energy and Resources in Australia and an Archaeological Research Institute in Qatar. UCL has the largest medical research center in Europe, and the Life Sciences Institute.In 2009, UCL and Yale University in the medical direction of deep cooperation, the establishment of the University of London - Yale University Joint Research Department. After the medical expansion to such as mathematics, economics, law, philosophy and other multi-directional scientific research cooperation and doctoral training.In 2016, the University of London School of Management and Peking University signed an agreement to jointly set up MBA (MBA) program. Peking University International MBA, Peking University National Development Institute (NSD) and the University of London School of Management (SoM) cooperation in running a school project. In addition, Peking University and the University of London in the medical, puter science, urban planning, language education and other disciplines to carry out research and teaching cooperation.G5 Super Elite UniversityUniversity of London is one of the G5 Super Elite University. The G5 Super elite University group (The Group 5) was established in 2004. Its five members received more government education and research budgets through close cooperation in related fields. While injecting new vitality into the UK academic field. Among the five members, Oxford University is located in Oxford. Cambridge University is located in Cambridge. The remaining three universities are located in the British capital of London. The Imperial College of London was once a graduate of the University of London, but it left the University of London in 2007 and was independent. The University of London UCL and the London School of Economics and Political Science LSE remain in the University of London Union (UOL). University of London College research activities frequently. The school set up the four challenges for the highest research objectives: international medical, sustainable urban development, cultural interaction, human prosperity and development.UCL学校简介篇二London University College is the University of London Union (University of London) of the school, and Cambridge, Oxford, Imperial College, London political and economic and said G5Super Elite University, on behalf of the UK#39;s top scientific research strength, Economic strength.The University of London not only has the world#39;s leading medical school, the School of Economics, the School of Architecture, its theoretical physics and mathematics, space science, statistics, life sciences, putational neuroscience, puter science, machine learning and artificial intelligence, electrical and electronic engineering, chemistry And the chemical engineering, law, geography, education, social and humanities and other fields of outstanding achievements also famous in the world. At the beginning of the school has a medical school, mathematics and physics, engineering science and social science college, and gradually expanded to 11 colleges.The University of London is one of the largest and most prehensive research universities in the UK. The strengths of the Institute have very strict application conditions. It gives a very small proportion of first degree, and the annual elimination rate is very impressive. One of the highest standards of the university.University of London set up the first department of economics in the United Kingdom, the relevant areas in the world recognized the leading position. 2014 British official academic rankings REF, the London University College of Economics Department of Research GPA for the British first, Research Power for the British fourth. The University of London and the London School of Economics and Economics are known as the Gemini of British economics today.UCL School of Mathematics and Physical Sciences has a world-class research facilities, in the theoretical physics, mathematics and statistics and other disciplines have a solid foundation, the College has been the birth of three Fields Award winner. In addition, the establishment of space science laboratory (MSSL), UCL has bee the world#39;s first space physics research one of the universities. In addition, according to the United States USnews ranked 2016 global university professional ranking, the University of London puter science professional ranked second in Europe, the first in the UK.The Bartlett School of Architecture is one of the most prestigious colleges of the University of London and one of the world#39;s most prestigious architectural colleges. 2017 QS architecture ranked second in the world, second only to the Massachusetts Institute of Technology and Architecture. The University of London School of Education (IOE) is a European-based teacher education research carrier, with a world-class research scholar in the field of education, in 2017 the world#39;s ranking of QS education ranked first.UCL Life Sciences and Clinical Science Research, one of the largest and most prestigious medical and life science research aggregates in the UK, is known worldwide for its cutting-edge teaching and research. At the same time the National Medical Research Center (NIMR) is also located in UCL, is one of the world#39;s leading biomedical research centers. Computing Neuroscience is second in the world, after Harvard, is a globally recognized leader.UCL Engineering Science Institute, is the world#39;s first set up a professional electrical and electronic engineering college, EEE professional is the inventor of vacuum tube John Ambrose Fleming established. At the same time UCL is also the first time the United Kingdom established a physical and chemical undergraduate teaching laboratory, and innovation in engineering teaching university. Electrical and electronic engineering, mechanical engineering, chemical engineering and civil engineering and other engineering, in the UK official 2014 excellent research framework REF, are ranked in the top ten BritishThe University of London and the University of London, Cambridge University, ImperialCollege of Technology, Southampton University, London King College jointly formed the British Science and Engineering South Union (SES-5), the academic research institutions for the cohesion of knowledge and resources Construction of the group. This is one of the most powerful scientific alliances and groups in the UK and one of the world#39;s leading scientific and engineering research centers.UCL简介篇三University College London (University College London), referred to as UCL, founded in 1826, located in London, England, is a world famous world famous school. It is the University of London (University of London, referred to as UOL) School of creation, and Cambridge University, Oxford University, Imperial College of Technology, London School of Economics and Economics and said G5 Super Elite University.Today, there are 32 Nobel laureates and 3 Fields winners in Alumni at the University of London (UCL), and there are many areas such as science, politics, and culture. celebrity. Including the father of fiber father Kao, father of telephone munication Alexander Bell, DNA discoverer father of biomedicalism Francis Crick, architectural telemunications core Peter Cook, artificial intelligence AlphaGo The founder of Daimies Hassabis and David Silva, the literary master Tagore, the father of India Mahatma Gandhi and Ito Hiroshi and so on.The University of London has a number of leading research institutions, including the National Medical Research Center (NIMR), Europe#39;s leading space exploration laboratory (MSSL), and the world-renowned Gatsby Computational Neuroscience Center (GCNU). Its main campus is close to the British Museum, the British Library, King#39;s Cross Station and Regent#39;s Park, in the heart of London.The United States in 2017 USnews ranked 23rd in the world, the first four in the United Kingdom; 2017 Shanghai Jiaotong University World University Academic Rank (ARWU) ranked the world#39;s 17th, the first three in England; 2017 QS World University Ranking Ranked No. 7 in the world, third place in the UK; in the UK by the official University of 2014 scientific research rankings (REF), the University of London ranked second in the UK, second only to Oxford University.。
大脑记忆的编码单元
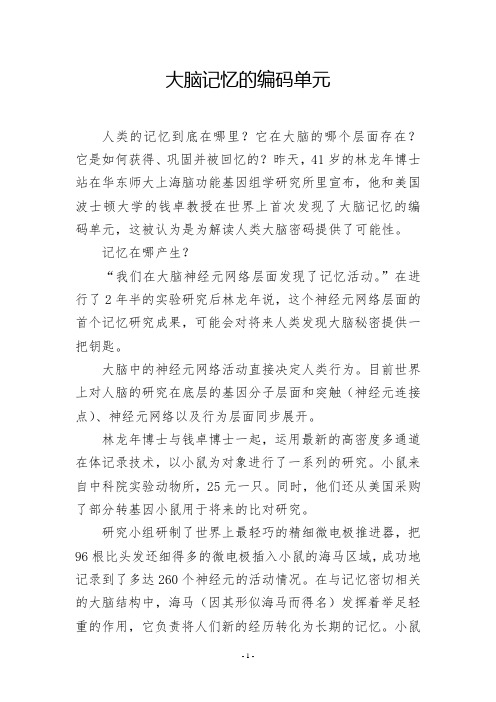
大脑记忆的编码单元人类的记忆到底在哪里?它在大脑的哪个层面存在?它是如何获得、巩固并被回忆的?昨天,41岁的林龙年博士站在华东师大上海脑功能基因组学研究所里宣布,他和美国波士顿大学的钱卓教授在世界上首次发现了大脑记忆的编码单元,这被认为是为解读人类大脑密码提供了可能性。
记忆在哪产生?“我们在大脑神经元网络层面发现了记忆活动。
”在进行了2年半的实验研究后林龙年说,这个神经元网络层面的首个记忆研究成果,可能会对将来人类发现大脑秘密提供一把钥匙。
大脑中的神经元网络活动直接决定人类行为。
目前世界上对人脑的研究在底层的基因分子层面和突触(神经元连接点)、神经元网络以及行为层面同步展开。
林龙年博士与钱卓博士一起,运用最新的高密度多通道在体记录技术,以小鼠为对象进行了一系列的研究。
小鼠来自中科院实验动物所,25元一只。
同时,他们还从美国采购了部分转基因小鼠用于将来的比对研究。
研究小组研制了世界上最轻巧的精细微电极推进器,把96根比头发还细得多的微电极插入小鼠的海马区域,成功地记录到了多达260个神经元的活动情况。
在与记忆密切相关的大脑结构中,海马(因其形似海马而得名)发挥着举足轻重的作用,它负责将人们新的经历转化为长期的记忆。
小鼠海马脑区只有半粒米大小,科学家们将实验的探测区域放在海马脑区的CA1细胞层,也就是信息的“输出层”,这里大约有紧密排列的20万至30万个神经元。
“这一步非常重要。
”林龙年博士介绍说,假如只能观察到几个神经元,就谈不上对神经元群体的编码进行分析了。
而传统的方法在小鼠上只能记录到几个至20几个神经元。
人类大脑是一个由约140亿个神经元组成的繁复的神经网络。
迄今为止,对大脑功能的解读,就像在基因密码破译之前人们只能通过基因的表型间接了解遗传信息一样,脑科学家们目前只能通过对行为的测试来间接检测记忆的形成。
技术上的突破使科学家能够通过检测大脑编码单元的活动状态直接解读大脑在学习过程中记忆的形成。
基于神经网络的多特征轻度认知功能障碍检测模型
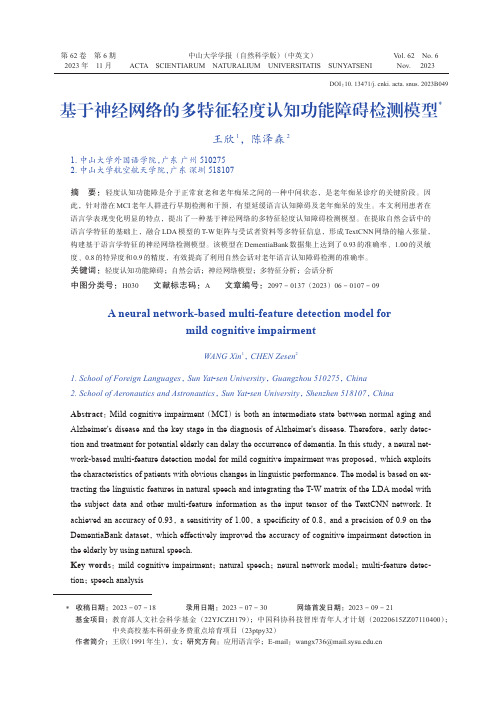
第 62 卷第 6 期2023 年11 月Vol.62 No.6Nov.2023中山大学学报(自然科学版)(中英文)ACTA SCIENTIARUM NATURALIUM UNIVERSITATIS SUNYATSENI基于神经网络的多特征轻度认知功能障碍检测模型*王欣1,陈泽森21. 中山大学外国语学院,广东广州 5102752. 中山大学航空航天学院,广东深圳 518107摘要:轻度认知功能障是介于正常衰老和老年痴呆之间的一种中间状态,是老年痴呆诊疗的关键阶段。
因此,针对潜在MCI老年人群进行早期检测和干预,有望延缓语言认知障碍及老年痴呆的发生。
本文利用患者在语言学表现变化明显的特点,提出了一种基于神经网络的多特征轻度认知障碍检测模型。
在提取自然会话中的语言学特征的基础上,融合LDA模型的T-W矩阵与受试者资料等多特征信息,形成TextCNN网络的输入张量,构建基于语言学特征的神经网络检测模型。
该模型在DementiaBank数据集上达到了0.93的准确率、1.00的灵敏度、0.8的特异度和0.9的精度,有效提高了利用自然会话对老年语言认知障碍检测的准确率。
关键词:轻度认知功能障碍;自然会话;神经网络模型;多特征分析;会话分析中图分类号:H030 文献标志码:A 文章编号:2097 - 0137(2023)06 - 0107 - 09A neural network-based multi-feature detection model formild cognitive impairmentWANG Xin1, CHEN Zesen21. School of Foreign Languages, Sun Yat-sen University, Guangzhou 510275, China2. School of Aeronautics and Astronautics, Sun Yat-sen University, Shenzhen 518107, ChinaAbstract:Mild cognitive impairment (MCI) is both an intermediate state between normal aging and Alzheimer's disease and the key stage in the diagnosis of Alzheimer's disease. Therefore, early detec‐tion and treatment for potential elderly can delay the occurrence of dementia. In this study, a neural net‐work-based multi-feature detection model for mild cognitive impairment was proposed, which exploits the characteristics of patients with obvious changes in linguistic performance. The model is based on ex‐tracting the linguistic features in natural speech and integrating the T-W matrix of the LDA model with the subject data and other multi-feature information as the input tensor of the TextCNN network. It achieved an accuracy of 0.93, a sensitivity of 1.00, a specificity of 0.8, and a precision of 0.9 on the DementiaBank dataset, which effectively improved the accuracy of cognitive impairment detection in the elderly by using natural speech.Key words:mild cognitive impairment; natural speech; neural network model; multi-feature detec‐tion; speech analysisDOI:10.13471/ki.acta.snus.2023B049*收稿日期:2023 − 07 − 18 录用日期:2023 − 07 − 30 网络首发日期:2023 − 09 − 21基金项目:教育部人文社会科学基金(22YJCZH179);中国科协科技智库青年人才计划(20220615ZZ07110400);中央高校基本科研业务费重点培育项目(23ptpy32)作者简介:王欣(1991年生),女;研究方向:应用语言学;E-mail:******************第 62 卷中山大学学报(自然科学版)(中英文)轻度认知障碍(MCI,mild cognitive impair‐ment)是一种神经系统慢性退行性疾病,也是阿尔茨海默病(AD,Alzheimer's disease)的早期关键阶段。
脑神经计算技术探索

脑神经计算技术探索一、脑神经计算技术概述脑神经计算技术是指基于神经生理学与计算机科学理论,并运用现代计算机技术和大数据技术模拟和分析人类大脑结构和功能,实现对人脑活动、神经疾病机制及神经系统行为的研究和探索。
近年来,随着计算机技术和神经科学研究的发展,脑神经计算技术也得到持续的改进和发展,成为了神经科学和计算机科学交叉应用的重要领域之一。
二、脑神经计算技术的研究方法脑神经计算技术的研究方法包括两个方面:一是利用计算机技术对大脑的信号和数据进行处理和分析,二是采用实验手段进行人脑活动、神经疾病机制和神经系统行为等的研究。
其中,基于计算机科学和技术的脑神经计算技术主要包括以下四个方面:1. 脑结构成像技术:利用MRI等医学成像技术对人脑进行成像,实现对脑结构、脑区分布和连接模式等信息的分析和绘制。
2. 脑电图技术:脑电图技术通过电极测量人脑发出的电信号,采集脑电波数据,通过对数据的分析识别脑电活动的波形类型、频率等参数,研究脑电活动及其与人体功能的关系。
3. 神经网络模型技术:神经网络模型技术是将计算机科学原理和人脑神经元特性相结合,建立神经网络或深度学习模型,实现对脑神经计算过程的模拟和研究。
4. 功能磁共振技术:功能磁共振技术是一种性能优良的无创脑成像技术,它可以检测脑血液氧合水平的变化,测量脑功能区域的活动,可用来研究诸如注意、语言、记忆、认知和情绪等方面。
三、脑神经计算技术的应用领域脑神经计算技术在医学、神经科学、认知科学、工程学等领域都有广泛的应用。
例如,在医学方面,脑神经计算技术可以用于诊断神经系统疾病,如癫痫、帕金森病等;在神经科学领域,脑神经计算技术可以用于研究记忆、情绪、注意力和思维等人脑智力行为;在认知科学领域,脑神经计算技术可以用于研究人类思维和意识的物理基础和机制;在工程学领域,脑神经计算技术可以用于机器人和人机接口技术的研发。
四、脑神经计算技术的展望随着人工智能技术和大数据技术的不断发展,脑神经计算技术将在未来得到进一步的应用和发展。
- 1、下载文档前请自行甄别文档内容的完整性,平台不提供额外的编辑、内容补充、找答案等附加服务。
- 2、"仅部分预览"的文档,不可在线预览部分如存在完整性等问题,可反馈申请退款(可完整预览的文档不适用该条件!)。
- 3、如文档侵犯您的权益,请联系客服反馈,我们会尽快为您处理(人工客服工作时间:9:00-18:30)。
Yee Whye Teh Department of Computer Science University of TorontoToronto M5S2Z9Canadaywteh@Geoffrey E.HintonGatsby Computational Neuroscience UnitUniversity College LondonLondon WC1N3AR U.K.hinton@ AbstractWe describe a neurally-inspired,unsupervised learning algorithm thatbuilds a non-linear generative model for pairs of face images from thesame individual.Individuals are then recognized byfinding the highestrelative probability pair among all pairs that consist of a test image andan image whose identity is known.Our method compares favorably withother methods in the literature.The generative model consists of a singlelayer of rate-coded,non-linear feature detectors and it has the propertythat,given a data vector,the true posterior probability distribution overthe feature detector activities can be inferred rapidly without iteration orapproximation.The weights of the feature detectors are learned by com-paring the correlations of pixel intensities and feature activations in twophases:When the network is observing real data and when it is observingreconstructions of real data generated from the feature activations.1IntroductionFace recognition is difficult when the number of individuals is large and the test and train-ing images of an individual differ in expression,lighting or the date on which they were taken.In addition to being an important application,face recognition allows us to evaluate different kinds of algorithm for learning to recognize or compare objects,since it requires accurate representation offine discriminative features in the presence of relatively large within-individual variations.This is made even more difficult when there are very few exemplars of each individual.We start by describing a new unsupervised learning algorithm for a restricted form of Boltz-mann machine[1].We then show how to generalize the generative model and the learning algorithm to deal with real-valued pixel intensities and rate-coded feature detectors.We then consider alternative ways of applying the rate-coded model to face recognition.2Inference and learning in Restricted Boltzmann MachinesA Restricted Boltzmann machine[2]has a layer of visible units and a single layer of hid-den units with no hidden-to-hidden connections.Inference in an RBM is much easier thanin a general Boltzmann machine and it is also much easier than in a causal belief net be-cause there is no explaining away[3].There is therefore no need to perform any iteration to determine the activities of the hidden units.The hidden states,,are conditionally in-dependent given the visible states,,and the distribution of is given by the standard logistic function:The replica trick can be interpreted as a cheap way of simulating an ensemble of neurons by assuming they have identical weights.Alternatively,it can be seen as a way of simulating a single neuron over a time interval in which it may produce multiple spikes that constitute a rate-code.For this reason we call the model“RBMrate”.We assumed that the visible units can produce up to10spikes and the hidden units can produce up to100spikes.We also made two further approximations:We replaced and in Eq.3by their expected values and we used the expected value of when computing the probability of activiation of the hidden units.However,we continued to use the stochastically chosen integerfiring rates of the hidden units when computing the one-step reconstructions of the data,so the hidden activities cannot transmit an unbounded amount of information from the data to the reconstruction.A simple way to use RBMrate for face recognition is to train a single model on the training set,and identify a face byfinding the gallery image that produces a hidden activity vector that is most similar to the one produced by the face.This is how eigenfaces are used for recognition,but it does not work well because it does not take into account the fact that some variations across faces are important for recognition,while some variations are not. To correct this,we instead trained an RBMrate model on pairs of different images of the same individual,and then we used this model of pairs to decide which gallery image is best paired with the test image.To account for the fact that the model likes some individual face images more than others,we define thefit between two faces and aswhere is the goodness score of the image pair under the model.The goodness score is the negative free energy which is an additive function of the total input received by each hidden unit.Each hidden unit has a set of weights going to each image in the pair,and weight-sharing is not used,hence.However,to preserve symmetry,each pair of images of the same individual in the training set has a reversed pair in the set.We trained the model with100hidden units on1000image pairs(500distinct pairs)for2000iterations in batches of100,with a learning rate of for the weights,a learning rate of for the biases,and a momentum of.One advantage of eigenfaces over correlation is that once the test image has been converted into a vector of eigenface activations,comparisons of test and gallery images can be made in the low-dimensional space of eigenface activations rather than the high-dimensional space of pixel intensities.The same applies to our face-pair network.The total input to each hidden unit from each gallery image can be precomputed and stored,while the total input from a test image only needs to be computed once for comparisons with all gallery images.4The FERET databaseOur version of the FERET database contained1002frontal face images of429individuals taken over a period of a few years under varying lighting conditions.Of these images,818 are used as both the gallery and the training set and the remaining184are divided into four, disjoint test sets:The expression test set contains110images of different individuals.These individuals all have another image in the training set that was taken with the same lighting conditions at the same time but with a different expression.The training set also includes a further 244pairs of images that differ only in expression.The days test set contains40images that come from20individuals.Each of these individuals has two images from the same session in the training set and two images taken in a session4days later or earlier in the test set.A further28individuals were photographed 4days apart and all112of these images are in the training set.a)b)c)d)e)f)by all ofthewere included in the training set.The glasses test set contains14images of7different individuals.Each of these individ-uals has two images in the training set that were taken in another session on the same day. The training and test pairs for an individual differ in that one pair has glasses and the other does not.The training set includes a further24images,half with glasses and half without, from6more individuals.The frontal face images include the whole head,parts of the shoulder and neck,and back-ground.Instead of training on the whole images,which contain much irrelevant informa-tion,we trained on face images that were normalized as shown infigure1.Masking out all of the background inevitably looses the contour of the face which contains much discrim-inative information.The histogram equalization step removes most lighting effects,but it also removes some relevant information like the skin tone.For the best performance,the contour shape and skin tone would have to be used as an additional source of discriminative information.Some examples of the processed images are shown infigure2.5Comparative resultsWe compared RBMrate with four popular face recognition methods.Thefirst and simplest is correlation[5],which returns the similarity score as the angle between two images represented as vectors of pixel intensities.This performed better than using the Euclidean distance as a score.The second method is eigenfaces[6],whichfirst projects the images onto the principalFigure3:Error rates of all methods on all test sets.component subspaces,then returns the similarity score as the angle between the projected images.We used199principal components,since we get better results as we increase the number of components.We also omitted thefirst principal component,as we determined manually that it encodes simply for lighting conditions.This improved the recognition performances on all the probe sets except for expression.The third method isfisherfaces[7].Instead of projecting the images onto the subspace of the principal components,which maximizes the variance among the projected images,fisherfaces projects the images onto a subspace which,at the same time,maximizes the between individual variances and minimizes the within individual variances in the training set.We used a subspace of dimension200.Thefinal method,which we shall call ppca,is proposed by Pentland et al[8].This method models differences between images of the same individual as a PPCA[9],and differences between images of different individuals as another PPCA.Then given a difference image,it returns as the similarity score the likelihood ratio of the difference image under the two P-PCA models.It was the best performing algorithm in the September1996FERET test[10] and is consistently worse than RBMrate on our test sets.We used10and30dimensional PPCAs for thefirst and second model respectively.These are the same numbers used by Pentland et al and gives the best results.Figure3shows the error rates of allfive methods on all four test sets.Correlation and eigenfaces perform poorly on expression,probably because they do not attempt to ignore the within-individual variations,whereas the other methods do.All the models did very poorly on the months test set which is unfortunate as this is the test set that is most like real applications.When the error rate of the best match is high,it is interesting to compare methods by considering the rate at which correct matches appear in the top few images.On months,for example,RBMrate appears to be worse than correlation but it is far more likely to have the right answer in its top20matches.However,the months test set is tiny so the differences are unreliable.Figure4shows that after our preprocessing,Figure4:On the left is a probe image from months and on the right are the top8 matches to the probe returned by RBMrate.Most human observers cannotfind the correct match within these8.human observers also have great difficulty with the months test set,probably because the task is intrinsically difficult and is made even harder by the loss of contour and skin tone information combined with the misleading oval contour produced by masking out all of the background.6Receptivefields learned by RBMrateThe top half offigure5shows the weights of a few of the hidden units after training.All the units encode global features,probably because the image normalization ensures that there are strong long range correlations in pixel intensities.The maximum size of the weights is,with most weights having magnitudes smaller than.Note,however,that the hidden unit activations range from0to100.On the left are4units exhibiting interesting features and on the right are4units chosen at random.The top unit of thefirst column seems to be encoding the presence of moustache in both faces.The bottom unit seems to be coding for prominent right eyebrows in both faces.Note that these are facial features which often remain constant across images of the same individual.In the second column are two features which seem to encode for different facial expressions in the two faces.The right side of the top unit encodes a smile while the left side is expresionless.This is reversed in the bottom unit.So the network has discovered some features which are fairly constant across images in the same class,and some features which can differ substantially within a class.Inspired by[11],we tried to enforce local features by restricting the weights to be non-negative.The bottom half offigure5shows some of the hidden receptivefields learned by RBMrate when trained with non-negative weights.Except for the4features on the left, all other features are local and code for features like mouth shape changes(third column) and eyes and cheeks(fourth column).The4features on the left are much more global and clearly capture the fact that the direction of the lighting can differ for two images of the same person.Unfortunately,constraining the weights to be non-negative strongly limits the representational power of RBMrate and makes it worse than all the other methods on all the test sets(except for ppca on months).7ConclusionsWe have introduced a new method for face recognition based on a non-linear generative model.The non-linear generative model can be very complex,yet retains the efficiency required for applications.Good performance is obtained on the FERET database.There is plenty of room for further development using prior knowledge to constrain the weights or additional layers of hidden units to model the correlations of feature detector activities.[1]G.E.Hinton.Training products of experts by minimizing contrastive divergence.TechnicalReport GCNU TR2000-004,Gatsby Computational Neuroscience Unit,University College London,2000.[2]rmation processing in dynamical systems:Foundations of harmony theory.In D.E.Rumelhart and J.L.McClelland,editors,Parallel Distributed Processing:Explorations in the Microstructure of Cognition.Volume1:Foundations.MIT Press,1986.[3]J.Pearl.Probabilistic reasoning in intelligent systems:networks of plausible inference.MorganKaufmann Publishers,San Mateo CA,1988.[4]G.E.Hinton and T.J.Sejnowski.Learning and relearning in boltzmann machines.In D.E.Rumelhart and J.L.McClelland,editors,Parallel Distributed Processing:Explorations in the Microstructure of Cognition.Volume1:Foundations.MIT Press,1986.[5]R.Brunelli and T.Poggio.Face recognition:features versus templates.IEEE Transactions onPattern Analysis and Machine Intelligence,15(10):1042–1052,1993.[6]M.Turk and A.Pentland.Eigenfaces for recognition.Journal of Cognitive Neuroscience,3(1):71–86,1991.[7]P.N.Belmumeur,J.P.Hespanha,and D.J.Kriegman.Eigenfaces versusfisherfaces:recogni-tion using class specific linear projection.In European Conference on Computer Vision,1996.[8] B.Moghaddam,W.Wahid,and A.Pentland.Beyond eigenfaces:probabilistic matching for facerecognition.In IEEE International Conference on Automatic Face and Gesture Recognition, 1998.[9]M.E.Tipping and C.M.Bishop.Probabilistic principal component analysis.Technical ReportNCRG/97/010,Neural Computing Research Group,Aston University,1997.[10]P.J.Phillips,H.Moon,P.Rauss,and S.A.Rizvi.The FERET september1996database andevaluation procedure.In International Conference on Audio and Video-based Biometric Person Authentication,1997.[11] D.Lee and H.S.Seung.Learning the parts of objects by non-negative matrix factorization.Nature,401,October1999.。