Fracture detection in a carbonate reservoir
无损检测专业英语
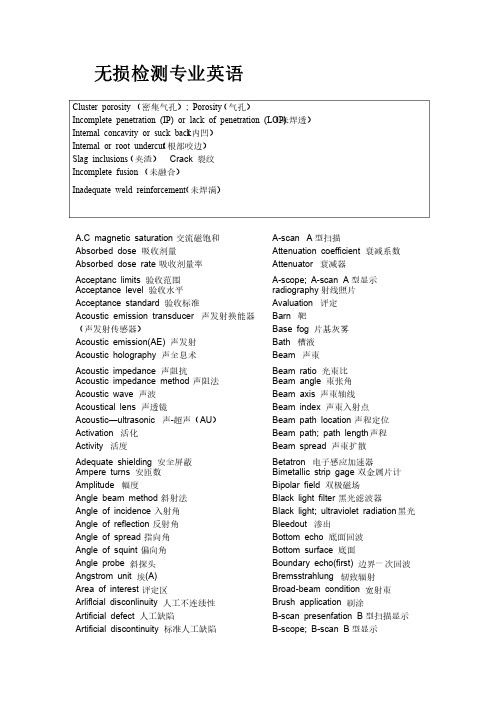
无损检测专业英语A.C magnetic saturation 交流磁饱和交流磁饱和 Absorbed dose 吸收剂量吸收剂量吸收剂量 Absorbed dose rate 吸收剂量率吸收剂量率 Acceptanc limits 验收范围验收范围验收范围 Acceptance level 验收水平验收水平验收水平 Acceptance standard 验收标准验收标准验收标准 Acoustic Acoustic emission emission emission transducer transducer 声发射换能器(声发射传感器)(声发射传感器)Acoustic emission(AE) 声发射声发射声发射 Acoustic holography 声全息术声全息术声全息术 Acoustic impedance 声阻抗声阻抗声阻抗 Acoustic impedance method 声阻法声阻法 Acoustic wave 声波声波声波 Acoustical lens 声透镜声透镜声透镜 Acoustic Acoustic——ultrasonic 声-超声(AU ) Activation 活化活化 Activity 活度活度 Adequate shielding 安全屏蔽安全屏蔽安全屏蔽 Ampere turns 安匝数安匝数安匝数 Amplitude 幅度幅度Angle beam method 斜射法斜射法 Angle of incidence 入射角入射角 Angle of reflection 反射角反射角 Angle of spread 指向角指向角 Angle of squint 偏向角偏向角 Angle probe 斜探头斜探头斜探头 Angstrom unit 埃(A) Area of interest 评定区评定区 Arliflcial disconlinuity 人工不连续性人工不连续性人工不连续性 Artificial defect 人工缺陷人工缺陷人工缺陷 Artificial discontinuity 标准人工缺陷标准人工缺陷标准人工缺陷A-scan A 型扫描型扫描Attenuation coefficient 衰减系数衰减系数衰减系数 Attenuator 衰减器衰减器 A-scope; A-scan A 型显示型显示 radiography 射线照片射线照片 Avaluation 评定评定 Barn 靶 Base fog 片基灰雾片基灰雾片基灰雾 Bath 槽液槽液 Beam 声束声束 Beam ratio 光束比光束比光束比 Beam angle 束张角束张角束张角 Beam axis 声束轴线声束轴线声束轴线 Beam index 声束入射点声束入射点声束入射点 Beam path location 声程定位声程定位 Beam path; path length 声程声程 Beam spread 声束扩散声束扩散声束扩散 Betatron 电子感应加速器电子感应加速器 Bimetallic strip gage 双金属片计双金属片计 Bipolar field 双极磁场双极磁场双极磁场 Black light filter 黑光滤波器黑光滤波器 Black light; ultraviolet radiation 黑光黑光 Bleedout 渗出渗出 Bottom echo 底面回波底面回波底面回波 Bottom surface 底面底面底面 Boundary echo(first) 边界一次回波边界一次回波边界一次回波 Bremsstrahlung 轫致辐射轫致辐射 Broad-beam condition 宽射束宽射束宽射束 Brush application 刷涂刷涂刷涂 B-scan presenfation B 型扫描显示型扫描显示 B-scope; B-scan B 型显示型显示Cluster porosity (密集气孔); Porosity (气孔)(气孔)Incomplete penetration (IP) or lack of penetration (LOP)(未焊透)(未焊透) Internal concavity or suck back (内凹)(内凹) Internal or root undercut (根部咬边)(根部咬边) Slag inclusions (夹渣)(夹渣) Crack 裂纹裂纹 Incomplete fusion (未融合)(未融合) Inadequate weld reinforcement (未焊满)C- scan C 型扫描型扫描 Calibration,instrument 设备校准设备校准 Capillary action 毛细管作用毛细管作用毛细管作用 Carrier fluid 载液载液载液 Carry over of penetrate 渗透剂移转渗透剂移转 Cathode 阴极阴极 Central conductor 中心导体中心导体中心导体 Central conductor method 中心导体法中心导体法 Characteristic curve 特性曲线特性曲线特性曲线 Characteristic curve of film 胶片特性曲线胶片特性曲线 Characteristic radiation 特征辐射特征辐射特征辐射 Chemical fog 化学灰雾化学灰雾化学灰雾 Cine-radiography 射线(活动)电影摄影术电影摄影术 Cintact pads 接触垫接触垫接触垫 Circumferential coils 圆环线圈圆环线圈圆环线圈 Circumferential field 周向磁场周向磁场周向磁场 Circumferential Circumferential magnetization magnetization magnetization method method 周向磁化法磁化法 Clean 清理清理 Clean- up 清除清除清除 Coercive force 矫顽力矫顽力矫顽力 Coi1,test 测试线圈测试线圈 Coil size 线圈大小线圈大小线圈大小 Coil spacing 线圈间距线圈间距线圈间距 Coil technique 线圈技术线圈技术线圈技术 Coil method 线圈法线圈法线圈法 Coilreference 线圈参考线圈参考 Collimator 准直器准直器Combined colour comtrast and fluorescent penetrant 着色荧光渗透剂着色荧光渗透剂 Compressed air drying 压缩空气干燥压缩空气干燥 Compressional wave 压缩波压缩波压缩波 Compton scatter 康普顿散射康普顿散射康普顿散射 Continuous emission 连续发射连续发射连续发射 Continuous linear array 连续线阵连续线阵 Continuous method 连续法连续法连续法 Continuous wave 连续波连续波连续波 Contrast 对比度对比度 Contrast agent 对比剂对比剂对比剂 Contrast aid 反差剂反差剂反差剂 Contrast sensitivity 对比灵敏度对比灵敏度对比灵敏度 Control echo 参考回波参考回波参考回波 Couplant 耦合剂耦合剂 Coupling 耦合耦合Coupling losses 耦合损失耦合损失耦合损失 Cracking 裂解裂解 Creeping wave 爬波爬波爬波 Critical angle 临界角临界角临界角 Cross section 横截面横截面横截面 Cross-drilled hole 横孔横孔横孔 Crystal 晶片晶片 C-scope; C-scan C 型显示型显示 Curie point 居里点居里点居里点 Current flow method 通电法通电法 Current induction method 电流感应法电流感应法 Current Current magnetization magnetization magnetization method method 电流磁化法Dead zone 盲区盲区盲区 Decay curve 衰变曲线衰变曲线衰变曲线 Decibel(dB) 分贝分贝 Defect 缺陷缺陷Defect resolution 缺陷分辨力缺陷分辨力缺陷分辨力 Defect detection sensitivity 缺陷检出灵敏度Defect resolution 缺陷分辨力缺陷分辨力缺陷分辨力 Definition 清晰度清晰度Definition , image image definition definition 清晰度,图像清晰度像清晰度Demagnetization 退磁退磁 Demagnetization factor 退磁因子退磁因子退磁因子 Demagnetizer 退磁装置退磁装置 Densitometer 黑度计黑度计 Density 黑度(底片)黑度(底片) Density comparison strip 黑度比较片黑度比较片 Detecting medium 检验介质检验介质检验介质 Detergent remover 洗净液洗净液洗净液 Developer 显像剂显像剂 Developer , agueons 水性显象剂水性显象剂 Developer , dry 干显象剂干显象剂 Developer , liquid film 液膜显象剂液膜显象剂液膜显象剂 Developer , nonaqueous (sus- pendible ) 非水(可悬浮)显象剂非水(可悬浮)显象剂 Developing time 显像时间显像时间显像时间 Development 显影显影 Diffraction mottle 衍射斑衍射斑衍射斑 Diffusion 扩散扩散 Digital Digital image image image acquisition acquisition acquisition system system 数字图像识别系统像识别系统Direct contact magnetization 直接接触磁化直接接触磁化 Direct exposure imaging 直接曝光成像直接曝光成像 Direct contact method 直接接触法直接接触法 Directivity 指向性指向性 Discontinuity 不连续性不连续性 Distance- Distance- gain- gain- gain- size-German size-German size-German AVG AVG 距离- 增益- 尺寸(DGS 德文为AVG ) Distance marker; time marker 距离刻度距离刻度 Dose equivalent 剂量当量剂量当量剂量当量 Dose rate meter 剂量率计剂量率计 Dosemeter 剂量计剂量计 Double crystal probe 双晶片探头双晶片探头 Double probe technique 双探头法双探头法 Double transceiver technique 双发双收法双发双收法 Double traverse technique 二次波法二次波法 Drain time 滴落时间滴落时间滴落时间 Drain time 流滴时间流滴时间流滴时间 Drift 漂移漂移 Dry method 干法干法干法 Dry powder 干粉干粉干粉 Dry technique 干粉技术干粉技术干粉技术 Dry developer 干显像剂干显像剂干显像剂 Dry developing cabinet 干显像柜干显像柜Dry method 干粉法干粉法干粉法 Drying oven 干燥箱干燥箱干燥箱 Drying station 干燥工位干燥工位干燥工位 Drying time 干燥时间干燥时间干燥时间 D-scope; D-scan D 型显示型显示 Dual search unit 双探头双探头 Dual-focus tube 双焦点管双焦点管双焦点管 Duplex-wire image quality indicator 双线像质指示器指示器 Duration 持续时间持续时间 Dwell time 停留时间停留时间停留时间 Dye penetrant 着色渗透剂着色渗透剂着色渗透剂 Dynamic leak test 动态泄漏检测动态泄漏检测 Dynamic Dynamic leakage leakage leakage measurement measurement 动态泄漏测量Dynamic range 动态范围动态范围动态范围 Dynamic radiography 动态射线透照术动态射线透照术动态射线透照术 Echo 回波回波Echo frequency 回波频率回波频率回波频率 Echo height 回波高度回波高度回波高度 Echo indication 回波指示回波指示回波指示 Echo transmittance of sound pressure 往复透过率复透过率Echo width 回波宽度回波宽度回波宽度 Eddy current 涡流涡流涡流 Eddy current flaw detector 涡流探伤仪涡流探伤仪 Eddy current testiog 涡流检测涡流检测 Edge 端面端面 Edge effect 边缘效应边缘效应边缘效应 Edge echo 棱边回波棱边回波棱边回波 Edge effect 边缘效应边缘效应边缘效应 Effective Effective depth depth depth penetration penetration (EDP ) 有效穿透深度效穿透深度Effective focus size 有效焦点尺寸有效焦点尺寸 Effective magnetic permeability 有效磁导率Effective permeability 有效磁导率有效磁导率有效磁导率 Effective reflection surface of flaw 缺陷有效反射面效反射面Effective resistance 有效电阻有效电阻有效电阻 Elastic medium 弹性介质弹性介质弹性介质 Electric displacement 电位移电位移电位移 Electrical center 电中心电中心电中心 Electrode 电极电极 Electromagnet 电磁铁电磁铁 Electro-magnetic Electro-magnetic acoustic acoustic acoustic transducer transducer 电磁声换能器磁声换能器Electromagnetic induction 电磁感应电磁感应电磁感应 Electromagnetic radiation 电磁辐射电磁辐射电磁辐射 Electromagnetic testing 电磁检测电磁检测电磁检测 Electro-mechanical Electro-mechanical coupling coupling coupling factor factor 机电耦合系数耦合系数Electron radiography 电子辐射照相术电子辐射照相术电子辐射照相术 Electron volt 电子伏恃电子伏恃电子伏恃 Electronic noise 电子噪声电子噪声电子噪声 Electrostatic spraying 静电喷涂静电喷涂静电喷涂 Emulsification 乳化乳化 Emulsification time 乳化时间乳化时间乳化时间 Emulsifier 乳化剂乳化剂 Encircling coils 环绕式线圈环绕式线圈环绕式线圈 End effect 端部效应端部效应端部效应 Energizing cycle 激励周期激励周期激励周期 Equalizing filter 均衡滤波器均衡滤波器均衡滤波器 Equivalent 当量当量 Equivalent I .Q. I. Sensitivity 象质指示器当量灵敏度当量灵敏度Equivalent Equivalent penetrameter penetrameter penetrameter sensifivty sensifivty 透度计当量灵敏度量灵敏度Equivalent method 当量法当量法当量法 Etching 浸蚀浸蚀 Evaluation 评定评定 Evaluation threshold 评价阈值评价阈值评价阈值 Event count 事件计数事件计数事件计数 Event count rate 事件计数率事件计数率Examination area 检测范围检测范围检测范围 Examination region 检验区域检验区域检验区域 Exhaust pressure/discharge pressure 排气压力Exposure 曝光曝光 Exposure chart 曝光曲线曝光曲线曝光曲线 Exposure fog 曝光灰雾曝光灰雾曝光灰雾 Exposure ,radiographic exposure 曝光,曝光,射线照相曝光照相曝光False indication 假指示假指示假指示 Feed-through coil 穿过式线圈穿过式线圈穿过式线圈 Field , resultant magnetic 复合磁场复合磁场复合磁场 Fill factor 填充系数填充系数填充系数 Film speed 胶片速度胶片速度胶片速度 Film badge 胶片襟章剂量计胶片襟章剂量计胶片襟章剂量计 Film base 片基片基片基 Film contrast 胶片对比度胶片对比度胶片对比度 Film gamma 胶片胶片γ值 Film processing 胶片冲洗加工胶片冲洗加工胶片冲洗加工 Film speed 胶片感光度胶片感光度胶片感光度 Film unsharpness 胶片不清晰度胶片不清晰度胶片不清晰度 Film viewing screen 观察屏观察屏 Filter 滤波器/滤光板滤光板 Final test 复探复探复探 Flat-bottomed hole 平底孔平底孔平底孔 Flat-bottomed hole equivalent 平底孔当量平底孔当量 Flaw 缺陷缺陷 Flaw echo 缺陷回波缺陷回波缺陷回波 Flexural wave 弯曲波弯曲波弯曲波 Fluorescence 荧光荧光Fluorescent examination method 荧光检验法荧光检验法 Fluorescent Fluorescent magnetic magnetic magnetic particle particle particle inspection inspection 荧光磁粉检验光磁粉检验Fluorescent light 荧光荧光荧光 Fluorescent magnetic powder 荧光磁粉荧光磁粉Fluorescent penetrant 荧光渗透剂荧光渗透剂荧光渗透剂 Fluorescent screen 荧光屏荧光屏荧光屏 Fluoroscopy 荧光检查法荧光检查法 Flux leakage field 磁通泄漏场磁通泄漏场 Flux lines 磁通线磁通线磁通线 Focal spot 焦点焦点焦点 Focal distance 焦距焦距焦距 Focus length 焦点长度焦点长度焦点长度 Focus size 焦点尺寸焦点尺寸焦点尺寸 Focus width 焦点宽度焦点宽度焦点宽度 Focus(electron) 电子焦点电子焦点 Focused beam 聚焦声束聚焦声束聚焦声束 Focusing probe 聚焦探头聚焦探头聚焦探头 Focus-to-film Focus-to-film distance(f.f.d) distance(f.f.d) 焦点-胶片距离(焦距)离(焦距) Fog 底片灰雾底片灰雾 Fog density 灰雾密度灰雾密度灰雾密度 Footcandle 英尺烛光英尺烛光 Freguency 频率频率 Frequency constant 频率常数频率常数频率常数 Fringe 干涉带干涉带 Front distance 前沿距离前沿距离前沿距离 Front distance of flaw 缺陷前沿距离缺陷前沿距离 Full- wave direct current (FWDC ) 全波直流直流 Furring 毛状迹痕毛状迹痕 Gage pressure 表压表压表压 Gain 增益增益Gamma radiography γ射线透照术射线透照术 Gamma ray source γ射线源射线源 Gamma ray source container γ射线源容器Gamma rays γ射线射线Gamma-ray radiographic equipment γ射线透照装置线透照装置 Gap scanning 间隙扫查间隙扫查间隙扫查 Gas 气体气体 Gate 闸门闸门 Gauss 高斯高斯Geiger-Muller counter 盖革盖革.弥勒计数器弥勒计数器 Geometric unsharpness 几何不清晰度几何不清晰度几何不清晰度 Gray(Gy) 戈瑞戈瑞 Grazing incidence 掠入射掠入射掠入射 Grazing angle 掠射角掠射角掠射角Half life 半衰期半衰期半衰期 Half- wave current (HW ) 半波电流半波电流 Half-value layer(HVL) 半值层半值层半值层 Half-value method 半波高度法半波高度法半波高度法 Halogen 卤素卤素Halogen leak detector 卤素检漏仪卤素检漏仪 Hard X-rays 硬X 射线射线 Hard-faced probe 硬膜探头硬膜探头硬膜探头 Harmonic analysis 谐波分析谐波分析谐波分析 Hermetically tight seal 气密密封气密密封 High vacuum 高真空高真空高真空 High energy X-rays 高能X 射线射线 Holography (optical) 光全息照相光全息照相光全息照相 Holography , acoustic 声全息声全息 Hydrophilic emulsifier 亲水性乳化剂亲水性乳化剂亲水性乳化剂 Hydrophilic remover 亲水性洗净剂亲水性洗净剂亲水性洗净剂 Hydrostatic text 流体静力检测流体静力检测流体静力检测 Hysteresis 磁滞磁滞 Hysteresis 磁滞磁滞 IACS IACS ID coil I D ID 线圈线圈 Image definition 图像清晰度图像清晰度图像清晰度 Image contrast 图像对比度图像对比度图像对比度 Image enhancement 图像增强图像增强图像增强 Image magnification 图像放大图像放大图像放大 Image quality 图像质量图像质量图像质量 Image quality indicator sensitivity 像质指示器灵敏度灵敏度Image quality indicator(IQI)/image quality indication 像质指示器像质指示器 Imaging line scanner 图像线扫描器图像线扫描器 Immersion probe 液浸探头液浸探头液浸探头 Immersion rinse 浸没清洗浸没清洗浸没清洗 Immersion testing 液浸法液浸法液浸法 Immersion time 浸没时间浸没时间浸没时间 Impedance 阻抗阻抗Impedance plane diagram 阻抗平面图阻抗平面图 Imperfection 不完整性不完整性Impulse eddy current testing 脉冲涡流检测脉冲涡流检测 Incremental permeability 增量磁导率增量磁导率增量磁导率 Indicated defect area 缺陷指示面积缺陷指示面积 Indicated defect length 缺陷指示长度缺陷指示长度 Indication 指示指示 Indirect exposure 间接曝光间接曝光间接曝光 Indirect magnetization 间接磁化间接磁化间接磁化 Indirect Indirect magnetization magnetization magnetization method method 间接磁化法Indirect scan 间接扫查间接扫查间接扫查 Induced field 感应磁场感应磁场感应磁场 Induced current method 感应电流法感应电流法 Infrared imaging system 红外成象系统红外成象系统 Infrared sensing device 红外扫描器红外扫描器 Inherent fluorescence 固有荧光固有荧光固有荧光 Inherent filtration 固有滤波固有滤波固有滤波 Initial permeability 起始磁导率起始磁导率起始磁导率 Initial pulse 始脉冲始脉冲始脉冲 Initial pulse width 始波宽度始波宽度 Inserted coil 插入式线圈插入式线圈插入式线圈 Inside coil 内部线圈内部线圈内部线圈 Inside- out testing 外泄检测外泄检测 Inspection 检查检查 Inspection medium 检查介质检查介质检查介质 Inspection Inspection frequency/ frequency/ frequency/ test test test frequency frequency 检测频率测频率Intensifying factor 增感系数增感系数增感系数 Intensifying screen 增感屏增感屏增感屏 Interface echo 界面回波界面回波界面回波 Interface trigger 界面触发界面触发界面触发 Interference 干涉干涉 Interpretation 解释解释 Ionization chamber 电离室电离室电离室 Ionization potential 电离电位电离电位电离电位 Ionization vacuum gage 电离真空计电离真空计 Ionography 电离射线透照术电离射线透照术 Isotope 同位素同位素 Kilo volt k v kv 千伏特千伏特 Kiloelectron volt k eV keV 千电子伏特千电子伏特 Lateral scan with oblique angle 斜平行扫查Latitude (of an emulsion) 胶片宽容度胶片宽容度 Lead screen 铅屏铅屏铅屏 Leak 泄漏孔泄漏孔 Leak artifact 泄漏器泄漏器泄漏器 Leak detector 检漏仪检漏仪检漏仪 Leak testtion 泄漏检测泄漏检测泄漏检测 Leakage field 泄漏磁场泄漏磁场泄漏磁场 Leakage rate 泄漏率泄漏率泄漏率 Light intensity 光强度光强度光强度Limiting resolution 极限分辨率极限分辨率极限分辨率 Line scanner 线扫描器线扫描器线扫描器 Line focus 线焦点线焦点线焦点 Line pair pattern 线对检测图线对检测图 Linear Linear (electron) (electron) (electron) accelerator(LINAC) accelerator(LINAC) 电子直线加速器线加速器Linear attenuation coefficient 线衰减系数线衰减系数 Linear scan 线扫查线扫查线扫查 Linearity (time time or or or distance distance ) 线性(时间或距离)距离)Linearity , anplitude 幅度线性幅度线性 Lines of force 磁力线磁力线 Lipophilic emulsifier 亲油性乳化剂亲油性乳化剂亲油性乳化剂 Lipophilic remover 亲油性洗净剂亲油性洗净剂亲油性洗净剂 Liquid penetrant examination 液体渗透检验液体渗透检验 Liquid film developer 液膜显像剂液膜显像剂 Local magnetization 局部磁化局部磁化局部磁化 Local magnetization method 局部磁化法局部磁化法 Local scan 局部扫查局部扫查局部扫查 Location 定位定位 Location accuracy 定位精度定位精度定位精度 Location computed 定位,计算定位,计算定位,计算 Location marker 定位标记定位标记定位标记 Location upon delta-T 时差定位时差定位 Location , clusfer 定位,群集定位,群集 Location ,continuous continuous AE AE AE signal signal 定位,连续AE 信号信号Longitudinal field 纵向磁场纵向磁场纵向磁场 Longitudinal magnetization method 纵向磁化法Longitudinal resolution 纵向分辨率纵向分辨率纵向分辨率 Longitudinal wave 纵波纵波纵波 Longitudinal wave probe 纵波探头纵波探头 Longitudinal wave technique 纵波法纵波法 Loss of back reflection 背面反射损失背面反射损失 Loss of back reflection 底面反射损失底面反射损失 Luminance 亮度亮度 Luminosity 流明流明Maga or million electron volts MeV 兆电子伏特Magnetic hysteresis 磁性滞后磁性滞后磁性滞后 Magnetic Magnetic particle particle particle field field field indication indication 磁粉磁场指示器示器 Magnetic particle inspection flaw indications 磁粉检验的伤显示磁粉检验的伤显示Magnetic circuit 磁路磁路磁路 Magnetic domain 磁畴磁畴磁畴 Magnetic field distribution 磁场分布磁场分布 Magnetic field indicator 磁场指示器磁场指示器 Magnetic field meter 磁场计磁场计 Magnetic field strength 磁场强度(H) Magnetic field/field ,magnetic 磁场磁场 Magnetic flux 磁通磁通磁通 Magnetic flux density 磁通密度磁通密度 Magnetic force 磁化力磁化力磁化力 Magnetic leakage field 漏磁场漏磁场 Magnetic leakage flux 漏磁通漏磁通 Magnetic moment 磁矩磁矩磁矩 Magnetic particle 磁粉磁粉磁粉 Magnetic particle indication 磁痕磁痕Magnetic particle testing/magnetic particle examination 磁粉检测磁粉检测 Magnetic permeability 磁导率磁导率磁导率 Magnetic permeability 磁导率磁导率磁导率 Magnetic pole 磁极磁极磁极 Magnetic saturataion 磁饱和磁饱和磁饱和 Magnetic saturation 磁饱和磁饱和磁饱和 Magnetic slorage meclium 磁储介质磁储介质 Magnetic writing 磁写磁写磁写 Magnetizing 磁化磁化 Magnetizing current 磁化电流磁化电流磁化电流 Magnetizing coil 磁化线圈磁化线圈磁化线圈 Magnetostrictive effect 磁致伸缩效应磁致伸缩效应磁致伸缩效应 Magnetostrictive Magnetostrictive transducer transducer 磁致伸缩换能器能器Main beam 主声束主声束主声束 Manual testing 手动检测手动检测手动检测 Markers 时标时标MA-scope; MA-scan M A MA 型显示型显示 Masking 遮蔽遮蔽 Mass Mass attcnuation attcnuation attcnuation coefficient coefficient 质量吸收系数Mass number 质量数质量数质量数 Mass spectrometer (M.S.) 质谱仪质谱仪 Mass Mass spectrometer spectrometer spectrometer leak detector leak detector 质谱检质谱检漏仪漏仪Mass spectrum 质谱质谱质谱 MDTD 最小可测温度差最小可测温度差Medium vacuum 中真空中真空中真空 Mega or million volt MV 兆伏特兆伏特Micro focus X - ray tube 微焦点X 光管光管 Microfocus radiography 微焦点射线透照术微焦点射线透照术微焦点射线透照术 Micrometre 微米微米 Micron of mercury 微米汞柱微米汞柱 Microtron 电子回旋加速器电子回旋加速器 Milliampere 毫安(mA ) Millimetre of mercury 毫米汞柱毫米汞柱 Minifocus x- ray tube 小焦点调射线管小焦点调射线管 Mode conversion 波型转换波型转换波型转换 Mode transformation 波型转换波型转换波型转换 Monitor 监控器监控器 Monochromatic 单色波单色波 Movement unsharpness 移动不清晰度移动不清晰度移动不清晰度 Moving beam radiography 可动射束射线透照术Multiaspect Multiaspect magnetization magnetization magnetization method method 多向磁化法Multidirectional magnetization 多向磁化多向磁化多向磁化 Multifrequency eddy current testiog 多频涡流检测检测Multiple back reflections 多次背面反射多次背面反射 Multiple reflections 多次反射多次反射多次反射 Multiple back reflections 多次底面反射多次底面反射 Multiple echo method 多次反射法多次反射法 Multiple probe technique 多探头法多探头法 Multiple triangular array 多三角形阵列多三角形阵列 Narrow beam condition 窄射束窄射束 NC NC Near field 近场近场近场 Near field length 近场长度近场长度 Near surface defect 近表面缺陷近表面缺陷 Net density 净黑度净黑度净黑度 Net density 净(光学)密度密度 Neutron 中子中子Neutron radiograhy 中子射线透照中子射线透照中子射线透照 Neutron radiography 中子射线透照术中子射线透照术中子射线透照术 Newton (N ) 牛顿牛顿 Noise 噪声噪声 Noise 噪声噪声Noise equivalent temperature difference (NETD ) 噪声当量温度差(NETD ) Nondcstructivc Nondcstructivc Examination Examination (NDE ) 无损试验Nondestructive Nondestructive Evaluation Evaluation (NDE ) 无损评价评价Nondestructive Inspection (NDI ) 无损检验Nondestructive T esting (NDT ) 无损检测无损检测 Nonferromugnetic material 非铁磁性材料非铁磁性材料非铁磁性材料 Nonrelevant indication 非相关指示非相关指示非相关指示 Non-screen-type film 非增感型胶片非增感型胶片非增感型胶片 Normal Normal incidence incidence 垂直入射(亦见直射声束)束)Normal permeability 标准磁导率标准磁导率标准磁导率 Normal beam method; straight beam method 垂直法垂直法 Normal probe 直探头直探头直探头 Object beam angle 物体光束角物体光束角 Object-film distance 被检体被检体-胶片距离胶片距离 Object 一film distance 物体物体- 胶片距离胶片距离 Over development 显影过度显影过度显影过度 Over emulsfication 过乳化过乳化过乳化 Overall magnetization 整体磁化整体磁化整体磁化 Overload recovery time 过载恢复时间过载恢复时间 Overwashing 过洗过洗 Oxidation fog 氧化灰雾氧化灰雾氧化灰雾 Pair production 电子对产生电子对产生电子对产生 Pair production 电子偶的产生电子偶的产生电子偶的产生 Parallel scan 平行扫查平行扫查平行扫查 Paramagnetic material 顺磁性材料顺磁性材料顺磁性材料 Parasitic echo 干扰回波干扰回波干扰回波 Partial pressure 分压分压分压 Particle content 磁悬液浓度磁悬液浓度磁悬液浓度 Particle velocity 质点质点(振动)速度速度 Pascal (Pa ) 帕斯卡(帕)帕斯卡(帕) Pascal Pascal cubic cubic cubic metres metres metres per per per second second 帕立方米每秒(Pa•m3/s ) Path length 光程长光程长光程长 Path length difference 光程长度差光程长度差 Pattern 探伤图形探伤图形 Peak current 峰值电流峰值电流峰值电流 Penetrameter 透度计透度计 Penetrameter sensitivity 透度计灵敏度透度计灵敏度透度计灵敏度 Penetrant 渗透剂渗透剂 Penetrant comparator 渗透对比试块渗透对比试块渗透对比试块 Penetrant flaw detection 渗透探伤渗透探伤Penetrant removal 渗透剂去除渗透剂去除渗透剂去除 Penetrant station 渗透工位渗透工位渗透工位 Penetrant , water- washable 水洗型渗透剂水洗型渗透剂水洗型渗透剂 Penetration 穿透深度穿透深度 Penetration time 渗透时间渗透时间渗透时间 Permanent magnet 永久磁铁永久磁铁永久磁铁 Permeability coefficient 透气系数透气系数 Permeability ,a-c 交流磁导率交流磁导率 Permeability ,d -c 直流磁导率直流磁导率 Phantom echo 幻象回波幻象回波幻象回波 Phase angle 相位角相位角相位角 Phase Phase controlled controlled controlled circuit circuit circuit breaker breaker 断电相位控制器制器Phase detection 相位检测相位检测相位检测 Phase hologram 相位全息相位全息相位全息 Phase sensitive detector 相敏检波器相敏检波器 Phase shift 相位移相位移相位移 Phase velocity 相速度相速度相速度 Phase-sensitive system 相敏系统相敏系统相敏系统 Phillips ionization gage 菲利浦电离计菲利浦电离计 Phosphor 荧光物质荧光物质 Photo fluorography 荧光照相术荧光照相术荧光照相术 Photoelectric absorption 光电吸收光电吸收光电吸收 Photographic emulsion 照相乳剂照相乳剂照相乳剂 Photographic fog 照相灰雾照相灰雾照相灰雾 Photostimulable luminescence 光敏发光光敏发光光敏发光 Piezoelectric effect 压电效应压电效应 Piezoelectric material 压电材料压电材料压电材料 Piezoelectric stiffness constant 压电劲度常数压电劲度常数 Piezoelectric stress constant 压电应力常数压电应力常数 Piezoelectric transducer 压电换能器压电换能器压电换能器 Piezoelectric voltage constant 压电电压常数压电电压常数 Pitch and catch technique 一发一收法一发一收法 Pixel size 象素尺寸象素尺寸象素尺寸 Pixel , disply size 象素显示尺寸象素显示尺寸象素显示尺寸 Planar array 平面阵平面阵(列) Plane wave 平面波平面波平面波 Plate wave 板波板波板波 Plate wave technique 板波法板波法 Point source 点源点源点源 Post emulsification 后乳化后乳化后乳化 Post emulsifiable penetrant 后乳化渗透剂后乳化渗透剂 Post-cleaning 后清除后清除 Post-cleaning 后清洗后清洗Powder 粉未粉未Powder blower 喷粉器喷粉器喷粉器 Powder blower 磁粉喷枪磁粉喷枪磁粉喷枪 Pre-cleaning 预清理预清理 Pressure difference 压力差压力差压力差 Pressure dye test 压力着色检测压力着色检测 Pressure probe 压力探头压力探头压力探头 Pressure testing 压力检测压力检测压力检测 Pressure- evacuation test 压力抽空检测压力抽空检测 Pressure mark 压痕压痕压痕 Pressure,design 设计压力设计压力 Pre-test 初探初探 Primary coil 一次线圈一次线圈一次线圈 Primary radiation 初级辐射初级辐射初级辐射 Probe gas 探头气体探头气体探头气体 Probe test 探头检测探头检测探头检测 Probe backing 探头背衬探头背衬探头背衬 Probe coil 点式线圈点式线圈点式线圈 Probe coil 探头式线圈探头式线圈探头式线圈 Probe coil clearance 探头线圈间隙探头线圈间隙 Probe index 探头入射点探头入射点探头入射点 Probe to weld distance 探头-焊缝距离焊缝距离 Probe/ search unit 探头探头 Process control radiograph 工艺过程控制的射线照相的射线照相Processing capacity 处理能力处理能力处理能力 Processing speed 处理速度处理速度处理速度 Prods 触头触头Projective radiography 投影射线透照术投影射线透照术投影射线透照术 Proportioning probe 比例探头比例探头比例探头 Protective material 防护材料防护材料防护材料 Proton radiography 质子射线透照质子射线透照质子射线透照 Pulse 脉冲波脉冲波 Pulse 脉冲脉冲Pulse echo method 脉冲回波法脉冲回波法 Pulse repetition rate 脉冲重复率脉冲重复率 Pulse amplitude 脉冲幅度脉冲幅度脉冲幅度 Pulse echo method 脉冲反射法脉冲反射法 Pulse energy 脉冲能量脉冲能量脉冲能量 Pulse envelope 脉冲包络脉冲包络脉冲包络 Pulse length 脉冲长度脉冲长度脉冲长度 Pulse repetition frequency 脉冲重复频率脉冲重复频率 Pulse tuning 脉冲调谐脉冲调谐脉冲调谐 Quadruple traverse technique 四次波法四次波法。
史上最全石油英语词汇(A~C)
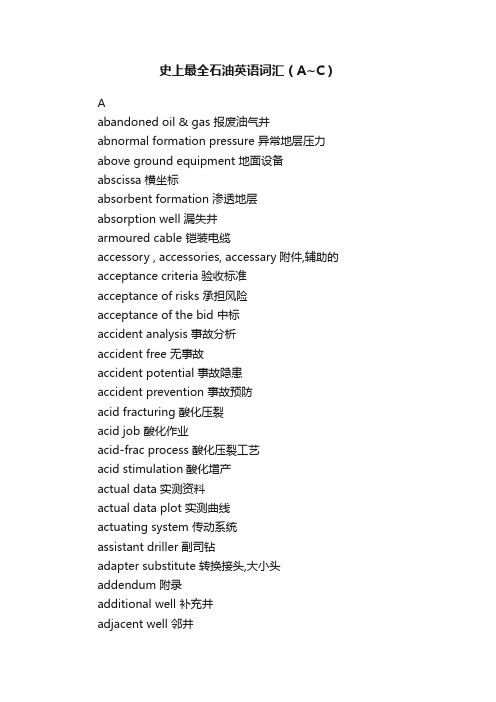
史上最全石油英语词汇(A~C)Aabandoned oil & gas 报废油气井abnormal formation pressure 异常地层压力above ground equipment 地面设备abscissa 横坐标absorbent formation 渗透地层absorption well 漏失井armoured cable 铠装电缆accessory , accessories, accessary附件,辅助的acceptance criteria 验收标准acceptance of risks 承担风险acceptance of the bid 中标accident analysis 事故分析accident free 无事故accident potential 事故隐患accident prevention 事故预防acid fracturing 酸化压裂acid job 酸化作业acid-frac process 酸化压裂工艺acid stimulation酸化增产actual data 实测资料actual data plot 实测曲线actuating system 传动系统assistant driller 副司钻adapter substitute 转换接头,大小头addendum 附录additional well 补充井adjacent well 邻井adjustment well 调整井adjust to zero 调零adjuster sub 调整短节adapter 适配器,接头automatic fine tuning 自动微调after-sale service 售后服务,后续服务after-treatment profile log 油井(增产)措施后生产剖面测井hole azimuth 井斜方向AL(acoustic logging)声波测井acoustic amplitude log,amplitude log声幅测井adjacent bed 围岩apparent resistivity视电阻率ACBL(acoustic cement bond log)声波水泥胶结测井activation logging活化测井ASC(after survey calibration)测后刻度amplitude type cement bond log声幅水泥胶结测井annular space log环空测井array sonic tool阵列声波测井仪acoustic wavetrain logging声波全波列测井alemite gun 黄油枪 alligator wrench 活动管钳alternator 交流发电机A-mast A形井架amplifier 放大器amplitude 振幅,声波幅度annulus(油套管)环形空间analog cable signal 模拟电缆信号anchor packer 卡瓦封隔器annual survey 年检annular blowout preventer环形防喷器annular pressure 环空压力annular pressure operated test valve环空压力控制测试阀annular reversing valve环空加压反循环阀annular space 环形空间annular water detector环空找水仪annular water injection反注,环空注入annulus blow out 环空井喷annulus completion fluid 环空完井液annulus (flow)line 环空(出油)管annulus fluid环空液体annulus logging环空测试annulus pack off环空封隔装置annulus pressure firing system环空压力起爆器annulus pressure response tool APR工具,(哈里伯顿)环空加压式测试阀annulus sample catcher环空取样器anticline背斜antiknock agent 防爆剂antivibration防震,抗震API pup joint API标准油管短节apparent wellbore radius有效井筒半径appraisal well 评价井aqueous saturation 含水饱和度APR(annulus pressure respondent)环空压力控制式测试工具area operations superintendent 地区作业监督armoured (electric) cable 铠装电缆artificial bottom hole 人工井底artificial fracture 人造裂缝artificial lift 机械采油assessment of bids 评标assessment well评价井assistant driller 副司钻assistant engineer助理工程师associated gas 伴生气assurance factor安全系数atmospheric service常压作业autocartograph 自动绘图仪autoignition自动点火AVL(acoustic velocity log )声速测井azimuth 井斜方位Bbit 钻头,刀片box 母扣,母接头block区块,滑轮车,断快back flow反循环洗井backup arm 推靠臂backup copy 副本bad earth 接地不良bail 提环,提捞back off 倒扣,解卡bailer捞砂筒bailing 提捞作业bailing bucket 提捞筒bar drop投棒bar drop sub 投杆接头,撞击接头barefoot completion 裸眼完井barefoot interval 裸眼井段barefoot well(bare hole)裸眼井bar fishing 打捞点火棒bar hydrostatic firing head 投棒压力起爆器basement rock基岩bastard connection 不合格的丝扣(接头)bastard thread不合格的丝扣behind completion date没有按期完工bit diameter钻头直径breakdown pressure 临界压力bailed dry (把井)掏空beam pumping unit 游梁式抽油机beam well (深井泵)抽油井bean up 放喷 bed of interest 目的层bellmouth喇叭口,锥形孔belly band 安全带bell nipple喇叭口短节bottom hole fluid sampler井底流体取样器bottom hole assembly 底部管柱结构BHC(borehole compensated sonic log )井眼补偿声波测井background radiation放射性本底植banana plug香蕉插头before calibration测前刻度BHTV(borehole televiewer)井下(声波)电视borehole fluid sampler井眼流体取样器bridle马笼头bradenhead flange 井口法兰bottom hole 井底,井下big hole perforator大孔眼射孔器borehole status井眼状况bottom-hole pressure ,closed井底关井压力bottom-hole pressure,flowing井底流动压力bottom-hole sample 井底取样,井下取样bottom hole sampler井底取样器bid bond投标保证金,投标保证书bidder 投标者bidding投标bidding documents投标文件,招标文件bidding procedure 投标程序bid opening开标big-entry-hole大射孔孔眼big-frac treatment(多层段)大型压裂big repair大修bill of materials材料清单bit hook打捞工具blank footage枪接头盲区blank off 油层封住,封堵blank tubing空油管,盲枪blasting cap 起爆雷管bleeding off pressure 放压block and tackle arrangement起下用滑车系统block diagram方框图,结构图block-squeeze分段挤水泥blowdown放压,衰竭式开采blowing out井喷blowing well自喷井blowout capping 控制井喷blowout hookup(井口)防喷装置blowout preventer 防喷器blank plug 丝堵,堵头blowout-prevention equipment防喷设备blowout-prevention procedure防喷措施bullets射孔弹blue sky exploratory well预探井bumper减震器back out(break out)卸开,松扣bobtail packer裸眼井测试封隔器bonding cable 接地电缆,地线booster传爆管bore diameter孔径,井眼直径bore frame井身结构borehole correction井眼校正borehole deviation井斜borehole effect井眼效应borehole face井壁borehole log 测井曲线,录井图borehole operation井下作业borehole pressure井筒压力borehole survey井下测量borehole wall井壁boring casing套管borings岩屑boring tower井架bottom hole turbine flowmeter井下涡轮流量计bottom nose (下井仪)尾端,(射孔)枪尾bottom-up firing枪尾起爆器box and pin公母接头box coupling接箍bridge plug桥塞by-pass旁通brake handle刹把breakdown 故障,事故break off pin type circulating sub断销式反循环阀breaking out pipe卸扣bridge plugging(打)桥塞封堵bridge the hole (在井中)打桥塞bring in a well完井投产bring out production投产,进行开采broken down 解堵brought in well投产井button switch按钮开关bushing 套筒 basket 打捞篮,水泥伞bottomed 下到井底borehole televiewer井下电视buffer减震器 build up压力恢复bubble 气泡,水泡bubble head泡泡头bubble point pressure饱和压力bucket rod抽油杆buck up紧扣build up of fluid液面恢复build the pressure打压build up of pressure压力恢复bull dog wrench管钳bullet gun射孔枪bullet hole射孔孔眼bumper spring 减震器弹簧burr毛刺,去毛刺by-pass pipe旁通管Ccontractor承包商C&F (cost and freight)成本加运费价,到岸价cement in place注水泥完毕cabin操作室cable armour电缆外皮cable bond(joint)电缆接头cable conductor缆芯cable configuration电缆规格cable continuity电缆通断情况cable core缆芯cable drum(reel)电缆滚筒cable duct 电缆槽cable fishing tool顿钻打捞工具cable formation test电缆地层测试cable head电缆头cable kinking电缆扭结cable-laid rope钢丝绳cable loop绳扣cable oil电缆油cable ringing电缆打结cable slack电缆松弛cable winch(work) 电缆绞车contract award date合同签定日期cake泥饼caliper井径仪calibration标定,调试,刻度calibrated标定过的calibrating jig刻度架caliper logging井径测量calling of bids招标callow表层camp equipment野外作业设备camp site施工现场cap rock盖层cap 雷管capping bed,cap formation盖层carbonate rock碳酸盐岩cased hole套管井cased hole completion 下套管完井cased hole formation tester套管井地层测试器case in 下套管cased well下套管井casing(case pipe)套管casing/cementation report套管固井报告casing cementing套管注水泥casing collar(coupling) 套管接箍CCL(casing collar locator)套管接箍测井casing grade套管钢级casing gun 电缆输送套管射孔枪casing head spool套管头四通casingless completion 无套管完井casing list 套管记录casing over pressure套管加压,环空加压casing packer套管封隔器casing perforation(套管)射孔casing perforation operator射孔作业工casing perforation service射孔作业casing perforation tool射孔器材casing perforation type射孔方式casing point下套管深度casing program套管程序casing setting depth套管下入深度casing shoe套管鞋casing top spacing套补距casing-tubing annulus油套环空casing wear套管损坏caution sign警告标志cave-in井壁坍塌core barrel取芯筒CBL(cement bond logging)水泥胶结测井carbon/oxygen logging碳氧比测井carbon/oxygen spectral logging 碳氧比能谱测井casing caliper log 套管内径测井casing inspection log套管探伤测井CBIL(circumferential borehole imaging logging system)环形井眼成象测井系统CET(cement evaluation tool)水泥评价测井channelling窜槽channelling detection找窜check shots检验地震测井completion date 完井日期cellar井口cellar connection(钻井)井口装置cemented to水泥返至cementing documentation固井设计cementing truck水泥车cement job quality固井质量cement sheath水泥环cement squeeze挤水泥cement top水泥返高centering device扶正器casing fluid level套管液面深度channel(射孔)孔道,管道,途径change over变扣接头change over sub大小头charge射孔弹,费用charge penetration射孔弹穿透深度charge strip(射孔)弹架charge type(射孔)弹型chief operator主操Chinese side中方christmas tree采油树christmas tree gauge井口压力表contract item合同条款casing inside diameter套管内径cement in place固井作业结束closed-in pressure关井压力circuit tester万用表clay 粘土caliper log井径测井claim 索赔claimer索赔人claim for breaking an agreement 违约赔偿claim for payment 要求付款claim indemnity索赔claim rejection拒赔 claystone粘土岩,泥岩clean out (清砂、清蜡)修井clean-out of well 洗井clean-out operation(service)洗井作业clean-out report修井报告clear working place安全工作区 clip绳卡closed-in关井closed-in well关闭井,停产井closed-in casing pressure关井套管压力closed-in tubing pressure关井油管压力cementer固井公司,固井队工人condensate凝析油coarse adjustment(control)粗调coarse -thread joint 粗扣接头change of contract合同变更casing outside diameter套管外径coiled rod 连续抽油杆coiled tubing连续油管,小油管coiled tubing service连续油管作业coiled tubing unit连续油管作业机coiled tubing operation小油管作业collar接箍,法兰盘,井口,钻铤collared hole下了表层套管的井combination collar(coupling)转换接箍combination tool组合下井仪commercial accumulation(deposit)有开采价值的油气藏commercial bed有开采价值的油气层commercial field有开采价值的油气田commingling production多层合采commissioning trial试用投产communication窜槽communication between zones窜层compensated density log补偿密度测井CDNT(compensated density-neutron tool)补偿地层密度中子测井仪compensated formation densilog补偿地层密度测井CNL(compensated neutron log)补偿中子测井compensated sonic log补偿声波测井conductivity log电导率测井conglomerate砾岩completion damage完井污染completion date完井日期completion evaluation完井评价completion fluid完井液completion operation 完井作业completion perforating完井射孔completion well test完井测试completion factor完井指数completion interval完井层段completion string完井管柱condensate field凝析气田condensate pool凝析油藏condensate well凝析油气井conductor line 导线conductor wire缆芯线connection end接头consent 插座 console面板constant maintenance定期保养consumable material消耗材料consumable accessories耗损件contact probe探头contact sonde(井下仪器的)探头container horizon(rock)含油层,储集层contamination污染continental shelf大陆架contingency意外情况contingency plan(procedures)应急措施contract area合同区contract award date 合同签定日期contract duration合同期限contracted block合同区contracted construction承包施工contract life合同有效期限contract(or)employee施工人员contract item合同条款contributing zone生产层control fluid压井液controlled directional well定向井control of well kick and blowout井涌井喷控制control panel remote遥控面板conventional DST常规地层测试conventional gun普通射孔器conventional operation常规作业conventional overbalanced perforating 常规正压射孔conventional pumping unit普通抽油机conventional well常规井conventional workover rig常规修井作业core barrel岩心筒core bit取心钻头CST(core sample taker)井壁取心器cored well取心井coupled tubing油管立柱coupling tubing connection油管接箍连接cement plug水泥塞casing pressure-closed关井套压casing pressure-flowing开井套压combination production logging组合测井crystal quartz gauge石英晶体压力crab(winch,crane)吊车,起重机crown block天车crooked stem 弯曲的管柱cross over短节,变扣接头cross over circulation 反循环cross over sub(joint)变扣接头,转换接头crowbar 撬杠crown platform天车平台CST(chronological sample taker,chronological sidewall core tool)井壁取心器cartage运费complete trip in hole 完成(仪器)下井作业cable telemetry system电缆遥测系统cable transmission system电缆传输系统current maintenance 日常维修cushion 液垫,测试垫customs海关,关税cut-off date截止日期。
改进的蚂蚁追踪裂缝检测算法及其应用研究
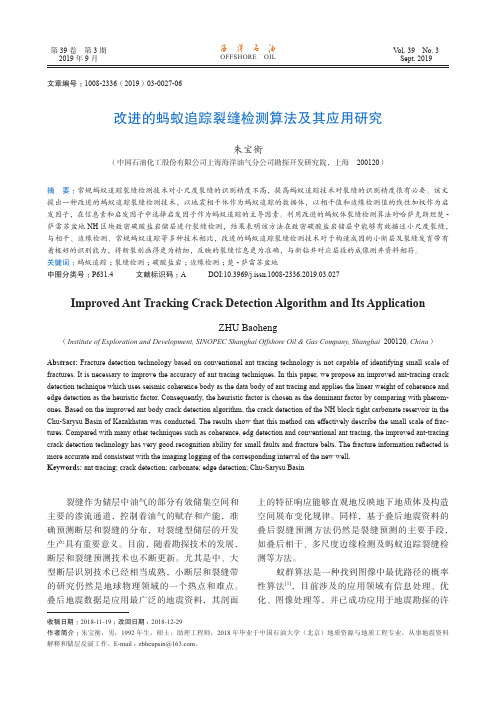
关键词 :蚂蚁追踪 ;裂缝检测 ;碳酸盐岩 ;边缘检测 ;楚 - 萨雷苏盆地
中图分类号 :P631.4
文献标识码 :A
DOI:10.3969/j.issn.1008-2336.2019.03.027
Improved Ant Tracking Crack Detection Algorithm and Its Application
萨雷苏盆地 NH 区块致密碳酸盐岩储层进行裂缝检测,结果表明该方法在致密碳酸盐岩储层中能够有效描述小尺度裂缝,
与相干、边缘检测、常规蚂蚁追踪等多种技术相比,改进的蚂蚁追踪裂缝检测技术对于构造成因的小断层及裂缝发育带有
着极好的识别能力,将断裂刻画得更为精细,反映的裂缝信息更为准确,与新钻井对应层段的成像测井资料相符。
第 39 卷 第 3 期 2019 年 9 月
文章编号 :1008-2336(2019)03-0027-06
OFFSHORE OIL
Vol. 39 No. 3 Sept. 2019
改进的蚂蚁追踪裂缝检测算法及其应用研究
朱宝衡
(中国石油化工股份有限公司上海海洋油气分公司勘探开发研究院,上海 200120)
收稿日期 :2018-11-19 ;改回日期 :2018-12-29 作者简介 :朱宝衡,男,1992 年生,硕士,助理工程师,2018 年毕业于中国石油大学(北京)地质资源与地质工程专业,从事地震资料 解释和储层反演工作。E-mail :zbhcupsin@。
ZHU Baoheng
(Institute of Exploration and Development, SINOPEC Shanghai Offshore Oil & Gas Company, Shanghai 200120, China)
碳酸盐岩储层裂缝智能预测技术及其应用
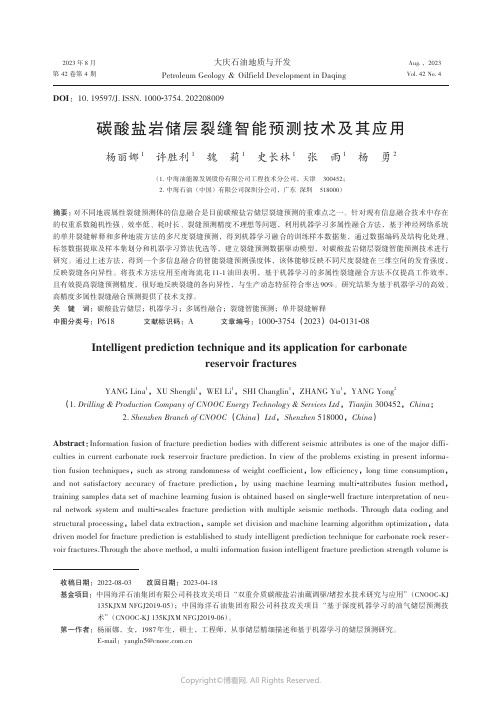
大庆石油地质与开发Petroleum Geology & Oilfield Development in Daqing2023 年 8 月第 42 卷第 4 期Aug. ,2023Vol. 42 No. 4DOI :10.19597/J.ISSN.1000-3754.202208009碳酸盐岩储层裂缝智能预测技术及其应用杨丽娜1 许胜利1 魏莉1 史长林1 张雨1 杨勇2(1.中海油能源发展股份有限公司工程技术分公司,天津300452;2.中海石油(中国)有限公司深圳分公司,广东 深圳518000)摘要: 对不同地震属性裂缝预测体的信息融合是目前碳酸盐岩储层裂缝预测的重难点之一。
针对现有信息融合技术中存在的权重系数随机性强、效率低、耗时长、裂缝预测精度不理想等问题,利用机器学习多属性融合方法,基于神经网络系统的单井裂缝解释和多种地震方法的多尺度裂缝预测,得到机器学习融合的训练样本数据集,通过数据编码及结构化处理、标签数据提取及样本集划分和机器学习算法优选等,建立裂缝预测数据驱动模型,对碳酸盐岩储层裂缝智能预测技术进行研究。
通过上述方法,得到一个多信息融合的智能裂缝预测强度体,该体能够反映不同尺度裂缝在三维空间的发育强度,反映裂缝各向异性。
将技术方法应用至南海流花11‑1油田表明,基于机器学习的多属性裂缝融合方法不仅提高工作效率,且有效提高裂缝预测精度,很好地反映裂缝的各向异性,与生产动态特征符合率达90%。
研究结果为基于机器学习的高效、高精度多属性裂缝融合预测提供了技术支撑。
关键词:碳酸盐岩储层;机器学习;多属性融合;裂缝智能预测;单井裂缝解释中图分类号:P618 文献标识码:A 文章编号:1000-3754(2023)04-0131-08Intelligent prediction technique and its application for carbonatereservoir fracturesYANG Lina 1,XU Shengli 1,WEI Li 1,SHI Changlin 1,ZHANG Yu 1,YANG Yong 2(1.Drilling & Production Company of CNOOC Energy Technology & Services Ltd ,Tianjin 300452,China ;2.Shenzhen Branch of CNOOC (China )Ltd ,Shenzhen 518000,China )Abstract :Information fusion of fracture prediction bodies with different seismic attributes is one of the major diffi‑culties in current carbonate rock reservoir fracture prediction. In view of the problems existing in present informa‑tion fusion techniques , such as strong randomness of weight coefficient , low efficiency , long time consumption , and not satisfactory accuracy of fracture prediction , by using machine learning multi -attributes fusion method , training samples data set of machine learning fusion is obtained based on single -well fracture interpretation of neu‑ral network system and multi -scales fracture prediction with multiple seismic methods. Through data coding and structural processing , label data extraction , sample set division and machine learning algorithm optimization , datadriven model for fracture prediction is established to study intelligent prediction technique for carbonate rock reser‑voir fractures.Through the above method, a multi information fusion intelligent fracture prediction strength volume is收稿日期:2022-08-03 改回日期:2023-04-18基金项目:中国海洋石油集团有限公司科技攻关项目“双重介质碳酸盐岩油藏调驱/堵控水技术研究与应用”(CNOOC -KJ135KJXM NFGJ2019-05);中国海洋石油集团有限公司科技攻关项目“基于深度机器学习的油气储层预测技术”(CNOOC -KJ 135KJXM NFGJ2019-06)。
灾后救援生命探测仪的现状和发展趋势
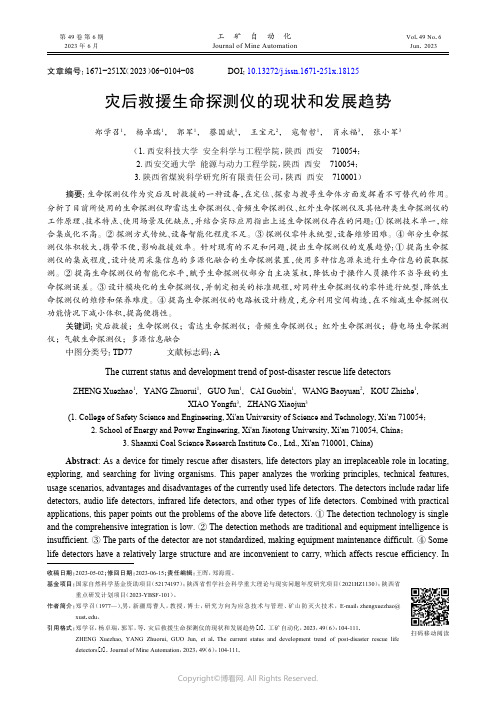
灾后救援生命探测仪的现状和发展趋势郑学召1, 杨卓瑞1, 郭军1, 蔡国斌1, 王宝元2, 寇智哲1, 肖永福3, 张小军3(1. 西安科技大学 安全科学与工程学院,陕西 西安 710054;2. 西安交通大学 能源与动力工程学院,陕西 西安 710054;3. 陕西省煤炭科学研究所有限责任公司,陕西 西安 710001)摘要:生命探测仪作为灾后及时救援的一种设备,在定位、探索与搜寻生命体方面发挥着不可替代的作用。
分析了目前所使用的生命探测仪即雷达生命探测仪、音频生命探测仪、红外生命探测仪及其他种类生命探测仪的工作原理、技术特点、使用场景及优缺点,并结合实际应用指出上述生命探测仪存在的问题:① 探测技术单一,综合集成化不高。
② 探测方式传统、设备智能化程度不足。
③ 探测仪零件未统型,设备维修困难。
④ 部分生命探测仪体积较大,携带不便,影响救援效率。
针对现有的不足和问题,提出生命探测仪的发展趋势:① 提高生命探测仪的集成程度,设计使用采集信息的多源化融合的生命探测装置,使用多种信息源来进行生命信息的获取探测。
② 提高生命探测仪的智能化水平,赋予生命探测仪部分自主决策权,降低由于操作人员操作不当导致的生命探测误差。
③ 设计模块化的生命探测仪,并制定相关的标准规程,对同种生命探测仪的零件进行统型,降低生命探测仪的维修和保养难度。
④ 提高生命探测仪的电路板设计精度,充分利用空间构造,在不缩减生命探测仪功能情况下减小体积,提高便携性。
关键词:灾后救援;生命探测仪;雷达生命探测仪;音频生命探测仪;红外生命探测仪;静电场生命探测仪;气敏生命探测仪;多源信息融合中图分类号:TD77 文献标志码:AThe current status and development trend of post-disaster rescue life detectorsZHENG Xuezhao 1, YANG Zhuorui 1, GUO Jun 1, CAI Guobin 1, WANG Baoyuan 2, KOU Zhizhe 1,XIAO Yongfu 3, ZHANG Xiaojun 3(1. College of Safety Science and Engineering, Xi'an University of Science and Technology, Xi'an 710054;2. School of Energy and Power Engineering, Xi'an Jiaotong University, Xi'an 710054, China ;3. Shaanxi Coal Science Research Institute Co., Ltd., Xi'an 710001, China)Abstract : As a device for timely rescue after disasters, life detectors play an irreplaceable role in locating,exploring, and searching for living organisms. This paper analyzes the working principles, technical features,usage scenarios, advantages and disadvantages of the currently used life detectors. The detectors include radar life detectors, audio life detectors, infrared life detectors, and other types of life detectors. Combined with practical applications, this paper points out the problems of the above life detectors. ① The detection technology is single and the comprehensive integration is low. ② The detection methods are traditional and equipment intelligence is insufficient. ③ The parts of the detector are not standardized, making equipment maintenance difficult. ④ Some life detectors have a relatively large structure and are inconvenient to carry, which affects rescue efficiency. In收稿日期:2023-05-02;修回日期:2023-06-15;责任编辑:王晖,郑海霞。
VoxelNet_ End-to-End Learning for Point Cloud Base
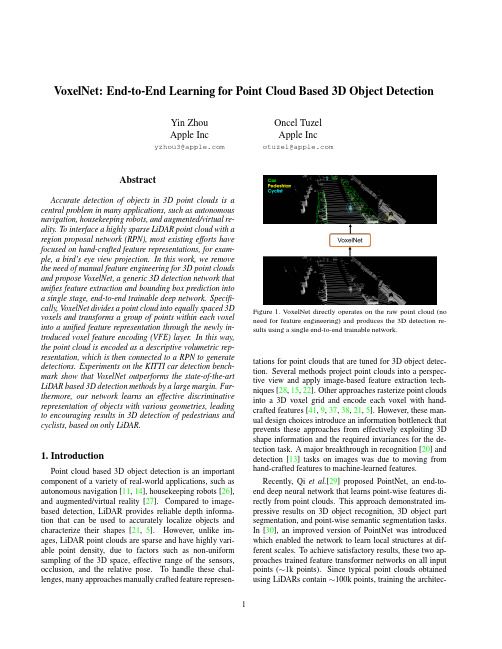
VoxelNet:End-to-End Learning for Point Cloud Based3D Object DetectionYin ZhouApple Inc****************Oncel TuzelApple Inc****************AbstractAccurate detection of objects in3D point clouds is a central problem in many applications,such as autonomous navigation,housekeeping robots,and augmented/virtual re-ality.To interface a highly sparse LiDAR point cloud with a region proposal network(RPN),most existing efforts have focused on hand-crafted feature representations,for exam-ple,a bird’s eye view projection.In this work,we remove the need of manual feature engineering for3D point clouds and propose VoxelNet,a generic3D detection network that unifies feature extraction and bounding box prediction into a single stage,end-to-end trainable deep network.Specifi-cally,VoxelNet divides a point cloud into equally spaced3D voxels and transforms a group of points within each voxel into a unified feature representation through the newly in-troduced voxel feature encoding(VFE)layer.In this way, the point cloud is encoded as a descriptive volumetric rep-resentation,which is then connected to a RPN to generate detections.Experiments on the KITTI car detection bench-mark show that VoxelNet outperforms the state-of-the-art LiDAR based3D detection methods by a large margin.Fur-thermore,our network learns an effective discriminative representation of objects with various geometries,leading to encouraging results in3D detection of pedestrians and cyclists,based on only LiDAR.1.IntroductionPoint cloud based3D object detection is an important component of a variety of real-world applications,such as autonomous navigation[11,14],housekeeping robots[26], and augmented/virtual reality[27].Compared to image-based detection,LiDAR provides reliable depth informa-tion that can be used to accurately localize objects and characterize their shapes[21,5].However,unlike im-ages,LiDAR point clouds are sparse and have highly vari-able point density,due to factors such as non-uniform sampling of the3D space,effective range of the sensors, occlusion,and the relative pose.To handle these chal-lenges,many approaches manually crafted featurerepresen-Figure1.V oxelNet directly operates on the raw point cloud(no need for feature engineering)and produces the3D detection re-sults using a single end-to-end trainable network.tations for point clouds that are tuned for3D object detec-tion.Several methods project point clouds into a perspec-tive view and apply image-based feature extraction tech-niques[28,15,22].Other approaches rasterize point clouds into a3D voxel grid and encode each voxel with hand-crafted features[41,9,37,38,21,5].However,these man-ual design choices introduce an information bottleneck that prevents these approaches from effectively exploiting3D shape information and the required invariances for the de-tection task.A major breakthrough in recognition[20]and detection[13]tasks on images was due to moving from hand-crafted features to machine-learned features.Recently,Qi et al.[29]proposed PointNet,an end-to-end deep neural network that learns point-wise features di-rectly from point clouds.This approach demonstrated im-pressive results on3D object recognition,3D object part segmentation,and point-wise semantic segmentation tasks.In[30],an improved version of PointNet was introduced which enabled the network to learn local structures at dif-ferent scales.To achieve satisfactory results,these two ap-proaches trained feature transformer networks on all input points(∼1k points).Since typical point clouds obtained using LiDARs contain∼100k points,training the architec-1Figure2.V oxelNet architecture.The feature learning network takes a raw point cloud as input,partitions the space into voxels,and transforms points within each voxel to a vector representation characterizing the shape information.The space is represented as a sparse 4D tensor.The convolutional middle layers processes the4D tensor to aggregate spatial context.Finally,a RPN generates the3D detection.tures as in[29,30]results in high computational and mem-ory requirements.Scaling up3D feature learning networks to orders of magnitude more points and to3D detection tasks are the main challenges that we address in this paper.Region proposal network(RPN)[32]is a highly opti-mized algorithm for efficient object detection[17,5,31, 24].However,this approach requires data to be dense and organized in a tensor structure(e.g.image,video)which is not the case for typical LiDAR point clouds.In this pa-per,we close the gap between point set feature learning and RPN for3D detection task.We present V oxelNet,a generic3D detection framework that simultaneously learns a discriminative feature represen-tation from point clouds and predicts accurate3D bounding boxes,in an end-to-end fashion,as shown in Figure2.We design a novel voxel feature encoding(VFE)layer,which enables inter-point interaction within a voxel,by combin-ing point-wise features with a locally aggregated feature. Stacking multiple VFE layers allows learning complex fea-tures for characterizing local3D shape information.Specif-ically,V oxelNet divides the point cloud into equally spaced 3D voxels,encodes each voxel via stacked VFE layers,and then3D convolution further aggregates local voxel features, transforming the point cloud into a high-dimensional volu-metric representation.Finally,a RPN consumes the vol-umetric representation and yields the detection result.This efficient algorithm benefits both from the sparse point struc-ture and efficient parallel processing on the voxel grid.We evaluate V oxelNet on the bird’s eye view detection and the full3D detection tasks,provided by the KITTI benchmark[11].Experimental results show that V oxelNet outperforms the state-of-the-art LiDAR based3D detection methods by a large margin.We also demonstrate that V oxel-Net achieves highly encouraging results in detecting pedes-trians and cyclists from LiDAR point cloud.1.1.Related WorkRapid development of3D sensor technology has moti-vated researchers to develop efficient representations to de-tect and localize objects in point clouds.Some of the earlier methods for feature representation are[39,8,7,19,40,33, 6,25,1,34,2].These hand-crafted features yield satisfac-tory results when rich and detailed3D shape information is available.However their inability to adapt to more complex shapes and scenes,and learn required invariances from data resulted in limited success for uncontrolled scenarios such as autonomous navigation.Given that images provide detailed texture information, many algorithms infered the3D bounding boxes from2D images[4,3,42,43,44,36].However,the accuracy of image-based3D detection approaches are bounded by the accuracy of the depth estimation.Several LIDAR based3D object detection techniques utilize a voxel grid representation.[41,9]encode each nonempty voxel with6statistical quantities that are de-rived from all the points contained within the voxel.[37] fuses multiple local statistics to represent each voxel.[38] computes the truncated signed distance on the voxel grid.[21]uses binary encoding for the3D voxel grid.[5]in-troduces a multi-view representation for a LiDAR point cloud by computing a multi-channel feature map in the bird’s eye view and the cylindral coordinates in the frontal view.Several other studies project point clouds onto a per-spective view and then use image-based feature encoding公众号DASOU-整理schemes[28,15,22].There are also several multi-modal fusion methods that combine images and LiDAR to improve detection accu-racy[10,16,5].These methods provide improved perfor-mance compared to LiDAR-only3D detection,particularly for small objects(pedestrians,cyclists)or when the objectsare far,since cameras provide an order of magnitude more measurements than LiDAR.However the need for an addi-tional camera that is time synchronized and calibrated with the LiDAR restricts their use and makes the solution more sensitive to sensor failure modes.In this work we focus on LiDAR-only detection.1.2.Contributions•We propose a novel end-to-end trainable deep archi-tecture for point-cloud-based3D detection,V oxelNet, that directly operates on sparse3D points and avoids information bottlenecks introduced by manual feature engineering.•We present an efficient method to implement V oxelNet which benefits both from the sparse point structure and efficient parallel processing on the voxel grid.•We conduct experiments on KITTI benchmark and show that V oxelNet produces state-of-the-art results in LiDAR-based car,pedestrian,and cyclist detection benchmarks.2.VoxelNetIn this section we explain the architecture of V oxelNet, the loss function used for training,and an efficient algo-rithm to implement the network.2.1.VoxelNet ArchitectureThe proposed V oxelNet consists of three functional blocks:(1)Feature learning network,(2)Convolutional middle layers,and(3)Region proposal network[32],as il-lustrated in Figure2.We provide a detailed introduction of V oxelNet in the following sections.2.1.1Feature Learning NetworkVoxel Partition Given a point cloud,we subdivide the3D space into equally spaced voxels as shown in Figure2.Sup-pose the point cloud encompasses3D space with range D, H,W along the Z,Y,X axes respectively.We define each voxel of size v D,v H,and v W accordingly.The resulting 3D voxel grid is of size D =D/v D,H =H/v H,W = W/v W.Here,for simplicity,we assume D,H,W are a multiple of v D,v H,v W.Grouping We group the points according to the voxel they reside in.Due to factors such as distance,occlusion,ob-ject’s relative pose,and non-uniform sampling,the LiDARFullyConnectedNeuralNetPoint-wiseInputPoint-wiseFeatureElement-wiseMaxpoolPoint-wiseConcatenateLocallyAggregatedFeaturePoint-wiseconcatenatedFeatureFigure3.V oxel feature encoding layer.point cloud is sparse and has highly variable point density throughout the space.Therefore,after grouping,a voxel will contain a variable number of points.An illustration is shown in Figure2,where V oxel-1has significantly more points than V oxel-2and V oxel-4,while V oxel-3contains no point.Random Sampling Typically a high-definition LiDAR point cloud is composed of∼100k points.Directly pro-cessing all the points not only imposes increased mem-ory/efficiency burdens on the computing platform,but also highly variable point density throughout the space might bias the detection.To this end,we randomly sample afixed number,T,of points from those voxels containing more than T points.This sampling strategy has two purposes,(1)computational savings(see Section2.3for details);and(2)decreases the imbalance of points between the voxels which reduces the sampling bias,and adds more variation to training.Stacked Voxel Feature Encoding The key innovation is the chain of VFE layers.For simplicity,Figure2illustrates the hierarchical feature encoding process for one voxel. Without loss of generality,we use VFE Layer-1to describe the details in the following paragraph.Figure3shows the architecture for VFE Layer-1.Denote V={p i=[x i,y i,z i,r i]T∈R4}i=1...t as a non-empty voxel containing t≤T LiDAR points,where p i contains XYZ coordinates for the i-th point and r i is the received reflectance.Wefirst compute the local mean as the centroid of all the points in V,denoted as(v x,v y,v z). Then we augment each point p i with the relative offset w.r.t. the centroid and obtain the input feature set V in={ˆp i= [x i,y i,z i,r i,x i−v x,y i−v y,z i−v z]T∈R7}i=1...t.Next, eachˆp i is transformed through the fully connected network (FCN)into a feature space,where we can aggregate in-formation from the point features f i∈R m to encode the shape of the surface contained within the voxel.The FCN is composed of a linear layer,a batch normalization(BN) layer,and a rectified linear unit(ReLU)layer.After obtain-ing point-wise feature representations,we use element-wise MaxPooling across all f i associated to V to get the locally aggregated feature˜f∈R m for V.Finally,we augmenteach f i with˜f to form the point-wise concatenated featureas f outi =[f T i,˜f T]T∈R2m.Thus we obtain the outputfeature set V out={f outi }i...t.All non-empty voxels areencoded in the same way and they share the same set of parameters in FCN.We use VFE-i(c in,c out)to represent the i-th VFE layer that transforms input features of dimension c in into output features of dimension c out.The linear layer learns a ma-trix of size c in×(c out/2),and the point-wise concatenation yields the output of dimension c out.Because the output feature combines both point-wise features and locally aggregated feature,stacking VFE lay-ers encodes point interactions within a voxel and enables thefinal feature representation to learn descriptive shape information.The voxel-wise feature is obtained by trans-forming the output of VFE-n into R C via FCN and apply-ing element-wise Maxpool where C is the dimension of the voxel-wise feature,as shown in Figure2.Sparse Tensor Representation By processing only the non-empty voxels,we obtain a list of voxel features,each uniquely associated to the spatial coordinates of a particu-lar non-empty voxel.The obtained list of voxel-wise fea-tures can be represented as a sparse4D tensor,of size C×D ×H ×W as shown in Figure2.Although the point cloud contains∼100k points,more than90%of vox-els typically are empty.Representing non-empty voxel fea-tures as a sparse tensor greatly reduces the memory usage and computation cost during backpropagation,and it is a critical step in our efficient implementation.2.1.2Convolutional Middle LayersWe use Conv M D(c in,c out,k,s,p)to represent an M-dimensional convolution operator where c in and c out are the number of input and output channels,k,s,and p are the M-dimensional vectors corresponding to kernel size,stride size and padding size respectively.When the size across the M-dimensions are the same,we use a scalar to represent the size e.g.k for k=(k,k,k).Each convolutional middle layer applies3D convolution,BN layer,and ReLU layer sequentially.The convolutional middle layers aggregate voxel-wise features within a pro-gressively expanding receptivefield,adding more context to the shape description.The detailed sizes of thefilters in the convolutional middle layers are explained in Section3.2.1.3Region Proposal NetworkRecently,region proposal networks[32]have become an important building block of top-performing object detec-tion frameworks[38,5,23].In this work,we make several key modifications to the RPN architecture proposed in[32], and combine it with the feature learning network and con-volutional middle layers to form an end-to-end trainable pipeline.The input to our RPN is the feature map provided by the convolutional middle layers.The architecture of this network is illustrated in Figure4.The network has three blocks of fully convolutional layers.Thefirst layer of each block downsamples the feature map by half via a convolu-tion with a stride size of2,followed by a sequence of con-volutions of stride1(×q means q applications of thefilter). After each convolution layer,BN and ReLU operations are applied.We then upsample the output of every block to a fixed size and concatanate to construct the high resolution feature map.Finally,this feature map is mapped to the de-sired learning targets:(1)a probability score map and(2)a regression map.2.2.Loss FunctionLet{a pos i}i=1...N pos be the set of N pos positive an-chors and{a neg j}j=1...N neg be the set of N neg negative anchors.We parameterize a3D ground truth box as (x g c,y g c,z g c,l g,w g,h g,θg),where x g c,y g c,z g c represent the center location,l g,w g,h g are length,width,height of the box,andθg is the yaw rotation around Z-axis.To re-trieve the ground truth box from a matching positive anchor parameterized as(x a c,y a c,z a c,l a,w a,h a,θa),we define the residual vector u∗∈R7containing the7regression tar-gets corresponding to center location∆x,∆y,∆z,three di-Voxel Input Feature BufferVoxel CoordinateBufferK T7Sparse TensorK31Voxel-wise FeatureK C 1Point CloudIndexingMemory CopyS t a c k e d V F EFigure 5.Illustration of efficient implementation.mensions ∆l,∆w,∆h ,and the rotation ∆θ,which are com-puted as:∆x =x g c −x a cd a ,∆y =y g c −y a c d a ,∆z =z gc −z a c h a ,∆l =log(l g l a ),∆w =log(w g w a ),∆h =log(h gh a ),(1)∆θ=θg −θawhere d a =(l a )2+(w a )2is the diagonal of the base of the anchor box.Here,we aim to directly estimate the oriented 3D box and normalize ∆x and ∆y homogeneously with the diagonal d a ,which is different from [32,38,22,21,4,3,5].We define the loss function as follows:L =α1N pos i L cls (p posi ,1)+β1N neg jL cls (p neg j ,0)+1N posiL reg (u i ,u ∗i )(2)where p pos i and p neg j represent the softmax output for posi-tive anchor a posi and negative anchor a neg j respectively,whileu i ∈R 7and u ∗i ∈R 7are the regression output and ground truth for positive anchor a pos i .The first two terms are the normalized classification loss for {a pos i }i =1...N pos and {a negj }j =1...N neg ,where the L cls stands for binary cross en-tropy loss and α,βare postive constants balancing the rel-ative importance.The last term L reg is the regression loss,where we use the SmoothL1function [12,32].2.3.Efficient ImplementationGPUs are optimized for processing dense tensor struc-tures.The problem with working directly with the point cloud is that the points are sparsely distributed across space and each voxel has a variable number of points.We devised a method that converts the point cloud into a dense tensor structure where stacked VFE operations can be processed in parallel across points and voxels.The method is summarized in Figure 5.We initialize aK ×T ×7dimensional tensor structure to store the voxel input feature buffer where K is the maximum number of non-empty voxels,T is the maximum number of points per voxel,and 7is the input encoding dimension for each point.The points are randomized before processing.For each point in the point cloud,we check if the corresponding voxel already exists.This lookup operation is done effi-ciently in O (1)using a hash table where the voxel coordi-nate is used as the hash key.If the voxel is already initial-ized we insert the point to the voxel location if there are less than T points,otherwise the point is ignored.If the voxel is not initialized,we initialize a new voxel,store its coordi-nate in the voxel coordinate buffer,and insert the point to this voxel location.The voxel input feature and coordinate buffers can be constructed via a single pass over the point list,therefore its complexity is O (n ).To further improve the memory/compute efficiency it is possible to only store a limited number of voxels (K )and ignore points coming from voxels with few points.After the voxel input buffer is constructed,the stacked VFE only involves point level and voxel level dense oper-ations which can be computed on a GPU in parallel.Note that,after concatenation operations in VFE,we reset the features corresponding to empty points to zero such that they do not affect the computed voxel features.Finally,using the stored coordinate buffer we reorganize the com-puted sparse voxel-wise structures to the dense voxel grid.The following convolutional middle layers and RPN oper-ations work on a dense voxel grid which can be efficiently implemented on a GPU.3.Training DetailsIn this section,we explain the implementation details of the V oxelNet and the training procedure.work DetailsOur experimental setup is based on the LiDAR specifi-cations of the KITTI dataset [11].Car Detection For this task,we consider point clouds within the range of [−3,1]×[−40,40]×[0,70.4]meters along Z,Y ,X axis respectively.Points that are projected outside of image boundaries are removed [5].We choose a voxel size of v D =0.4,v H =0.2,v W =0.2meters,which leads to D =10,H =400,W =352.We set T =35as the maximum number of randomly sam-pled points in each non-empty voxel.We use two VFE layers VFE-1(7,32)and VFE-2(32,128).The final FCN maps VFE-2output to R 128.Thus our feature learning net generates a sparse tensor of shape 128×10×400×352.To aggregate voxel-wise features,we employ three convo-lution middle layers sequentially as Conv3D(128,64,3,(2,1,1),(1,1,1)),Conv3D(64,64,3,(1,1,1),(0,1,1)),andConv3D(64,64,3,(2,1,1),(1,1,1)),which yields a4D ten-sor of size64×2×400×352.After reshaping,the input to RPN is a feature map of size128×400×352,where the dimensions correspond to channel,height,and width of the3D tensor.Figure4illustrates the detailed network ar-chitecture for this task.Unlike[5],we use only one anchor size,l a=3.9,w a=1.6,h a=1.56meters,centered at z a c=−1.0meters with two rotations,0and90degrees. Our anchor matching criteria is as follows:An anchor is considered as positive if it has the highest Intersection over Union(IoU)with a ground truth or its IoU with ground truth is above0.6(in bird’s eye view).An anchor is considered as negative if the IoU between it and all ground truth boxes is less than0.45.We treat anchors as don’t care if they have 0.45≤IoU≤0.6with any ground truth.We setα=1.5 andβ=1in Eqn.2.Pedestrian and Cyclist Detection The input range1is [−3,1]×[−20,20]×[0,48]meters along Z,Y,X axis re-spectively.We use the same voxel size as for car detection, which yields D=10,H=200,W=240.We set T=45 in order to obtain more LiDAR points for better capturing shape information.The feature learning network and con-volutional middle layers are identical to the networks used in the car detection task.For the RPN,we make one mod-ification to block1in Figure4by changing the stride size in thefirst2D convolution from2to1.This allowsfiner resolution in anchor matching,which is necessary for de-tecting pedestrians and cyclists.We use anchor size l a= 0.8,w a=0.6,h a=1.73meters centered at z a c=−0.6 meters with0and90degrees rotation for pedestrian detec-tion and use anchor size l a=1.76,w a=0.6,h a=1.73 meters centered at z a c=−0.6with0and90degrees rota-tion for cyclist detection.The specific anchor matching cri-teria is as follows:We assign an anchor as postive if it has the highest IoU with a ground truth,or its IoU with ground truth is above0.5.An anchor is considered as negative if its IoU with every ground truth is less than0.35.For anchors having0.35≤IoU≤0.5with any ground truth,we treat them as don’t care.During training,we use stochastic gradient descent (SGD)with learning rate0.01for thefirst150epochs and decrease the learning rate to0.001for the last10epochs. We use a batchsize of16point clouds.3.2.Data AugmentationWith less than4000training point clouds,training our network from scratch will inevitably suffer from overfitting. To reduce this issue,we introduce three different forms of data augmentation.The augmented training data are gener-ated on-the-fly without the need to be stored on disk[20].1Our empirical observation suggests that beyond this range,LiDAR returns from pedestrians and cyclists become very sparse and therefore detection results will be unreliable.Define set M={p i=[x i,y i,z i,r i]T∈R4}i=1,...,N as the whole point cloud,consisting of N points.We parame-terize a3D bouding box b i as(x c,y c,z c,l,w,h,θ),where x c,y c,z c are center locations,l,w,h are length,width, height,andθis the yaw rotation around Z-axis.We de-fineΩi={p|x∈[x c−l/2,x c+l/2],y∈[y c−w/2,y c+ w/2],z∈[z c−h/2,z c+h/2],p∈M}as the set con-taining all LiDAR points within b i,where p=[x,y,z,r] denotes a particular LiDAR point in the whole set M.Thefirst form of data augmentation applies perturbation independently to each ground truth3D bounding box to-gether with those LiDAR points within the box.Specifi-cally,around Z-axis we rotate b i and the associatedΩi with respect to(x c,y c,z c)by a uniformally distributed random variable∆θ∈[−π/10,+π/10].Then we add a translation (∆x,∆y,∆z)to the XYZ components of b i and to each point inΩi,where∆x,∆y,∆z are drawn independently from a Gaussian distribution with mean zero and standard deviation1.0.To avoid physically impossible outcomes,we perform a collision test between any two boxes after the per-turbation and revert to the original if a collision is detected. Since the perturbation is applied to each ground truth box and the associated LiDAR points independently,the net-work is able to learn from substantially more variations than from the original training data.Secondly,we apply global scaling to all ground truth boxes b i and to the whole point cloud M.Specifically, we multiply the XYZ coordinates and the three dimen-sions of each b i,and the XYZ coordinates of all points in M with a random variable drawn from uniform distri-bution[0.95,1.05].Introducing global scale augmentation improves robustness of the network for detecting objects with various sizes and distances as shown in image-based classification[35,18]and detection tasks[12,17].Finally,we apply global rotation to all ground truth boxes b i and to the whole point cloud M.The rotation is applied along Z-axis and around(0,0,0).The global ro-tation offset is determined by sampling from uniform dis-tribution[−π/4,+π/4].By rotating the entire point cloud, we simulate the vehicle making a turn.4.ExperimentsWe evaluate V oxelNet on the KITTI3D object detection benchmark[11]which contains7,481training images/point clouds and7,518test images/point clouds,covering three categories:Car,Pedestrian,and Cyclist.For each class, detection outcomes are evaluated based on three difficulty levels:easy,moderate,and hard,which are determined ac-cording to the object size,occlusion state,and truncation level.Since the ground truth for the test set is not avail-able and the access to the test server is limited,we con-duct comprehensive evaluation using the protocol described in[4,3,5]and subdivide the training data into a training setMethod ModalityCar Pedestrian CyclistEasy Moderate Hard Easy Moderate Hard Easy Moderate HardMono3D[3]Mono 5.22 5.19 4.13N/A N/A N/A N/A N/A N/A 3DOP[4]Stereo12.639.497.59N/A N/A N/A N/A N/A N/A VeloFCN[22]LiDAR40.1432.0830.47N/A N/A N/A N/A N/A N/A MV(BV+FV)[5]LiDAR86.1877.3276.33N/A N/A N/A N/A N/A N/A MV(BV+FV+RGB)[5]LiDAR+Mono86.5578.1076.67N/A N/A N/A N/A N/A N/A HC-baseline LiDAR88.2678.4277.6658.9653.7951.4763.6342.7541.06 V oxelNet LiDAR89.6084.8178.5765.9561.0556.9874.4152.1850.49 Table1.Performance comparison in bird’s eye view detection:average precision(in%)on KITTI validation set.Method ModalityCar Pedestrian CyclistEasy Moderate Hard Easy Moderate Hard Easy Moderate HardMono3D[3]Mono 2.53 2.31 2.31N/A N/A N/A N/A N/A N/A 3DOP[4]Stereo 6.55 5.07 4.10N/A N/A N/A N/A N/A N/A VeloFCN[22]LiDAR15.2013.6615.98N/A N/A N/A N/A N/A N/A MV(BV+FV)[5]LiDAR71.1956.6055.30N/A N/A N/A N/A N/A N/A MV(BV+FV+RGB)[5]LiDAR+Mono71.2962.6856.56N/A N/A N/A N/A N/A N/A HC-baseline LiDAR71.7359.7555.6943.9540.1837.4855.3536.0734.15 V oxelNet LiDAR81.9765.4662.8557.8653.4248.8767.1747.6545.11 Table2.Performance comparison in3D detection:average precision(in%)on KITTI validation set.and a validation set,which results in3,712data samples for training and3,769data samples for validation.The split avoids samples from the same sequence being included in both the training and the validation set[3].Finally we also present the test results using the KITTI server.For the Car category,we compare the proposed method with several top-performing algorithms,including image based approaches:Mono3D[3]and3DOP[4];LiDAR based approaches:VeloFCN[22]and3D-FCN[21];and a multi-modal approach MV[5].Mono3D[3],3DOP[4]and MV[5]use a pre-trained model for initialization whereas we train V oxelNet from scratch using only the LiDAR data provided in KITTI.To analyze the importance of end-to-end learning,we implement a strong baseline that is derived from the V ox-elNet architecture but uses hand-crafted features instead of the proposed feature learning network.We call this model the hand-crafted baseline(HC-baseline).HC-baseline uses the bird’s eye view features described in[5]which are computed at0.1m resolution.Different from[5],we in-crease the number of height channels from4to16to cap-ture more detailed shape information–further increasing the number of height channels did not lead to performance improvement.We replace the convolutional middle lay-ers of V oxelNet with similar size2D convolutional layers, which are Conv2D(16,32,3,1,1),Conv2D(32,64,3,2, 1),Conv2D(64,128,3,1,1).Finally RPN is identical in V oxelNet and HC-baseline.The total number of parame-ters in HC-baseline and V oxelNet are very similar.We train the HC-baseline using the same training procedure and data augmentation described in Section3.4.1.Evaluation on KITTI Validation SetMetrics We follow the official KITTI evaluation protocol, where the IoU threshold is0.7for class Car and is0.5for class Pedestrian and Cyclist.The IoU threshold is the same for both bird’s eye view and full3D evaluation.We compare the methods using the average precision(AP)metric. Evaluation in Bird’s Eye View The evaluation result is presented in Table1.V oxelNet consistently outperforms all the competing approaches across all three difficulty levels. HC-baseline also achieves satisfactory performance com-pared to the state-of-the-art[5],which shows that our base region proposal network(RPN)is effective.For Pedestrian and Cyclist detection tasks in bird’s eye view,we compare the proposed V oxelNet with HC-baseline.V oxelNet yields substantially higher AP than the HC-baseline for these more challenging categories,which shows that end-to-end learn-ing is essential for point-cloud based detection.We would like to note that[21]reported88.9%,77.3%, and72.7%for easy,moderate,and hard levels respectively, but these results are obtained based on a different split of 6,000training frames and∼1,500validation frames,and they are not directly comparable with algorithms in Table1. Therefore,we do not include these results in the table. Evaluation in3D Compared to the bird’s eye view de-tection,which requires only accurate localization of ob-jects in the2D plane,3D detection is a more challeng-ing task as it requiresfiner localization of shapes in3D space.Table2summarizes the comparison.For the class Car,V oxelNet significantly outperforms all other ap-proaches in AP across all difficulty levels.Specifically, using only LiDAR,V oxelNet significantly outperforms the。
jstd035声学扫描
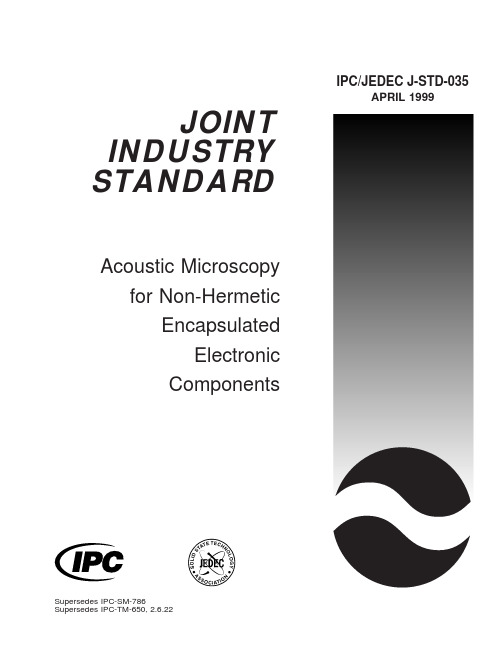
JOINT INDUSTRY STANDARDAcoustic Microscopy for Non-HermeticEncapsulatedElectronicComponents IPC/JEDEC J-STD-035APRIL1999Supersedes IPC-SM-786 Supersedes IPC-TM-650,2.6.22Notice EIA/JEDEC and IPC Standards and Publications are designed to serve thepublic interest through eliminating misunderstandings between manufacturersand purchasers,facilitating interchangeability and improvement of products,and assisting the purchaser in selecting and obtaining with minimum delaythe proper product for his particular need.Existence of such Standards andPublications shall not in any respect preclude any member or nonmember ofEIA/JEDEC or IPC from manufacturing or selling products not conformingto such Standards and Publications,nor shall the existence of such Standardsand Publications preclude their voluntary use by those other than EIA/JEDECand IPC members,whether the standard is to be used either domestically orinternationally.Recommended Standards and Publications are adopted by EIA/JEDEC andIPC without regard to whether their adoption may involve patents on articles,materials,or processes.By such action,EIA/JEDEC and IPC do not assumeany liability to any patent owner,nor do they assume any obligation whateverto parties adopting the Recommended Standard or ers are alsowholly responsible for protecting themselves against all claims of liabilities forpatent infringement.The material in this joint standard was developed by the EIA/JEDEC JC-14.1Committee on Reliability Test Methods for Packaged Devices and the IPCPlastic Chip Carrier Cracking Task Group(B-10a)The J-STD-035supersedes IPC-TM-650,Test Method2.6.22.For Technical Information Contact:Electronic Industries Alliance/ JEDEC(Joint Electron Device Engineering Council)2500Wilson Boulevard Arlington,V A22201Phone(703)907-7560Fax(703)907-7501IPC2215Sanders Road Northbrook,IL60062-6135 Phone(847)509-9700Fax(847)509-9798Please use the Standard Improvement Form shown at the end of thisdocument.©Copyright1999.The Electronic Industries Alliance,Arlington,Virginia,and IPC,Northbrook,Illinois.All rights reserved under both international and Pan-American copyright conventions.Any copying,scanning or other reproduction of these materials without the prior written consent of the copyright holder is strictly prohibited and constitutes infringement under the Copyright Law of the United States.IPC/JEDEC J-STD-035Acoustic Microscopyfor Non-Hermetic EncapsulatedElectronicComponentsA joint standard developed by the EIA/JEDEC JC-14.1Committee on Reliability Test Methods for Packaged Devices and the B-10a Plastic Chip Carrier Cracking Task Group of IPCUsers of this standard are encouraged to participate in the development of future revisions.Contact:EIA/JEDEC Engineering Department 2500Wilson Boulevard Arlington,V A22201 Phone(703)907-7500 Fax(703)907-7501IPC2215Sanders Road Northbrook,IL60062-6135 Phone(847)509-9700Fax(847)509-9798ASSOCIATION CONNECTINGELECTRONICS INDUSTRIESAcknowledgmentMembers of the Joint IPC-EIA/JEDEC Moisture Classification Task Group have worked to develop this document.We would like to thank them for their dedication to this effort.Any Standard involving a complex technology draws material from a vast number of sources.While the principal members of the Joint Moisture Classification Working Group are shown below,it is not possible to include all of those who assisted in the evolution of this Standard.To each of them,the mem-bers of the EIA/JEDEC and IPC extend their gratitude.IPC Packaged Electronic Components Committee ChairmanMartin FreedmanAMP,Inc.IPC Plastic Chip Carrier Cracking Task Group,B-10a ChairmanSteven MartellSonoscan,Inc.EIA/JEDEC JC14.1CommitteeChairmanJack McCullenIntel Corp.EIA/JEDEC JC14ChairmanNick LycoudesMotorolaJoint Working Group MembersCharlie Baker,TIChristopher Brigham,Hi/FnRalph Carbone,Hewlett Packard Co. Don Denton,TIMatt Dotty,AmkorMichele J.DiFranza,The Mitre Corp. Leo Feinstein,Allegro Microsystems Inc.Barry Fernelius,Hewlett Packard Co. Chris Fortunko,National Institute of StandardsRobert J.Gregory,CAE Electronics, Inc.Curtis Grosskopf,IBM Corp.Bill Guthrie,IBM Corp.Phil Johnson,Philips Semiconductors Nick Lycoudes,MotorolaSteven R.Martell,Sonoscan Inc. Jack McCullen,Intel Corp.Tom Moore,TIDavid Nicol,Lucent Technologies Inc.Pramod Patel,Advanced Micro Devices Inc.Ramon R.Reglos,XilinxCorazon Reglos,AdaptecGerald Servais,Delphi Delco Electronics SystemsRichard Shook,Lucent Technologies Inc.E.Lon Smith,Lucent Technologies Inc.Randy Walberg,NationalSemiconductor Corp.Charlie Wu,AdaptecEdward Masami Aoki,HewlettPackard LaboratoriesFonda B.Wu,Raytheon Systems Co.Richard W.Boerdner,EJE ResearchVictor J.Brzozowski,NorthropGrumman ES&SDMacushla Chen,Wus Printed CircuitCo.Ltd.Jeffrey C.Colish,Northrop GrummanCorp.Samuel J.Croce,Litton AeroProducts DivisionDerek D-Andrade,Surface MountTechnology CentreRao B.Dayaneni,Hewlett PackardLaboratoriesRodney Dehne,OEM WorldwideJames F.Maguire,Boeing Defense&Space GroupKim Finch,Boeing Defense&SpaceGroupAlelie Funcell,Xilinx Inc.Constantino J.Gonzalez,ACMEMunir Haq,Advanced Micro DevicesInc.Larry A.Hargreaves,DC.ScientificInc.John T.Hoback,Amoco ChemicalCo.Terence Kern,Axiom Electronics Inc.Connie M.Korth,K-Byte/HibbingManufacturingGabriele Marcantonio,NORTELCharles Martin,Hewlett PackardLaboratoriesRichard W.Max,Alcatel NetworkSystems Inc.Patrick McCluskey,University ofMarylandJames H.Moffitt,Moffitt ConsultingServicesRobert Mulligan,Motorola Inc.James E.Mumby,CibaJohn Northrup,Lockheed MartinCorp.Dominique K.Numakura,LitchfieldPrecision ComponentsNitin B.Parekh,Unisys Corp.Bella Poborets,Lucent TechnologiesInc.D.Elaine Pope,Intel Corp.Ray Prasad,Ray Prasad ConsultancyGroupAlbert Puah,Adaptec Inc.William Sepp,Technic Inc.Ralph W.Taylor,Lockheed MartinCorp.Ed R.Tidwell,DSC CommunicationsCorp.Nick Virmani,Naval Research LabKen Warren,Corlund ElectronicsCorp.Yulia B.Zaks,Lucent TechnologiesInc.IPC/JEDEC J-STD-035April1999 iiTable of Contents1SCOPE (1)2DEFINITIONS (1)2.1A-mode (1)2.2B-mode (1)2.3Back-Side Substrate View Area (1)2.4C-mode (1)2.5Through Transmission Mode (2)2.6Die Attach View Area (2)2.7Die Surface View Area (2)2.8Focal Length(FL) (2)2.9Focus Plane (2)2.10Leadframe(L/F)View Area (2)2.11Reflective Acoustic Microscope (2)2.12Through Transmission Acoustic Microscope (2)2.13Time-of-Flight(TOF) (3)2.14Top-Side Die Attach Substrate View Area (3)3APPARATUS (3)3.1Reflective Acoustic Microscope System (3)3.2Through Transmission AcousticMicroscope System (4)4PROCEDURE (4)4.1Equipment Setup (4)4.2Perform Acoustic Scans..........................................4Appendix A Acoustic Microscopy Defect CheckSheet (6)Appendix B Potential Image Pitfalls (9)Appendix C Some Limitations of AcousticMicroscopy (10)Appendix D Reference Procedure for PresentingApplicable Scanned Data (11)FiguresFigure1Example of A-mode Display (1)Figure2Example of B-mode Display (1)Figure3Example of C-mode Display (2)Figure4Example of Through Transmission Display (2)Figure5Diagram of a Reflective Acoustic MicroscopeSystem (3)Figure6Diagram of a Through Transmission AcousticMicroscope System (3)April1999IPC/JEDEC J-STD-035iiiIPC/JEDEC J-STD-035April1999This Page Intentionally Left BlankivApril1999IPC/JEDEC J-STD-035 Acoustic Microscopy for Non-Hermetic EncapsulatedElectronic Components1SCOPEThis test method defines the procedures for performing acoustic microscopy on non-hermetic encapsulated electronic com-ponents.This method provides users with an acoustic microscopy processflow for detecting defects non-destructively in plastic packages while achieving reproducibility.2DEFINITIONS2.1A-mode Acoustic data collected at the smallest X-Y-Z region defined by the limitations of the given acoustic micro-scope.An A-mode display contains amplitude and phase/polarity information as a function of time offlight at a single point in the X-Y plane.See Figure1-Example of A-mode Display.IPC-035-1 Figure1Example of A-mode Display2.2B-mode Acoustic data collected along an X-Z or Y-Z plane versus depth using a reflective acoustic microscope.A B-mode scan contains amplitude and phase/polarity information as a function of time offlight at each point along the scan line.A B-mode scan furnishes a two-dimensional(cross-sectional)description along a scan line(X or Y).See Figure2-Example of B-mode Display.IPC-035-2 Figure2Example of B-mode Display(bottom half of picture on left)2.3Back-Side Substrate View Area(Refer to Appendix A,Type IV)The interface between the encapsulant and the back of the substrate within the outer edges of the substrate surface.2.4C-mode Acoustic data collected in an X-Y plane at depth(Z)using a reflective acoustic microscope.A C-mode scan contains amplitude and phase/polarity information at each point in the scan plane.A C-mode scan furnishes a two-dimensional(area)image of echoes arising from reflections at a particular depth(Z).See Figure3-Example of C-mode Display.1IPC/JEDEC J-STD-035April1999IPC-035-3 Figure3Example of C-mode Display2.5Through Transmission Mode Acoustic data collected in an X-Y plane throughout the depth(Z)using a through trans-mission acoustic microscope.A Through Transmission mode scan contains only amplitude information at each point in the scan plane.A Through Transmission scan furnishes a two-dimensional(area)image of transmitted ultrasound through the complete thickness/depth(Z)of the sample/component.See Figure4-Example of Through Transmission Display.IPC-035-4 Figure4Example of Through Transmission Display2.6Die Attach View Area(Refer to Appendix A,Type II)The interface between the die and the die attach adhesive and/or the die attach adhesive and the die attach substrate.2.7Die Surface View Area(Refer to Appendix A,Type I)The interface between the encapsulant and the active side of the die.2.8Focal Length(FL)The distance in water at which a transducer’s spot size is at a minimum.2.9Focus Plane The X-Y plane at a depth(Z),which the amplitude of the acoustic signal is maximized.2.10Leadframe(L/F)View Area(Refer to Appendix A,Type V)The imaged area which extends from the outer L/F edges of the package to the L/F‘‘tips’’(wedge bond/stitch bond region of the innermost portion of the L/F.)2.11Reflective Acoustic Microscope An acoustic microscope that uses one transducer as both the pulser and receiver. (This is also known as a pulse/echo system.)See Figure5-Diagram of a Reflective Acoustic Microscope System.2.12Through Transmission Acoustic Microscope An acoustic microscope that transmits ultrasound completely through the sample from a sending transducer to a receiver on the opposite side.See Figure6-Diagram of a Through Transmis-sion Acoustic Microscope System.2April1999IPC/JEDEC J-STD-0353IPC/JEDEC J-STD-035April1999 3.1.6A broad band acoustic transducer with a center frequency in the range of10to200MHz for subsurface imaging.3.2Through Transmission Acoustic Microscope System(see Figure6)comprised of:3.2.1Items3.1.1to3.1.6above3.2.2Ultrasonic pulser(can be a pulser/receiver as in3.1.1)3.2.3Separate receiving transducer or ultrasonic detection system3.3Reference packages or standards,including packages with delamination and packages without delamination,for use during equipment setup.3.4Sample holder for pre-positioning samples.The holder should keep the samples from moving during the scan and maintain planarity.4PROCEDUREThis procedure is generic to all acoustic microscopes.For operational details related to this procedure that apply to a spe-cific model of acoustic microscope,consult the manufacturer’s operational manual.4.1Equipment Setup4.1.1Select the transducer with the highest useable ultrasonic frequency,subject to the limitations imposed by the media thickness and acoustic characteristics,package configuration,and transducer availability,to analyze the interfaces of inter-est.The transducer selected should have a low enough frequency to provide a clear signal from the interface of interest.The transducer should have a high enough frequency to delineate the interface of interest.Note:Through transmission mode may require a lower frequency and/or longer focal length than reflective mode.Through transmission is effective for the initial inspection of components to determine if defects are present.4.1.2Verify setup with the reference packages or standards(see3.3above)and settings that are appropriate for the trans-ducer chosen in4.1.1to ensure that the critical parameters at the interface of interest correlate to the reference standard uti-lized.4.1.3Place units in the sample holder in the coupling medium such that the upper surface of each unit is parallel with the scanning plane of the acoustic transducer.Sweep air bubbles away from the unit surface and from the bottom of the trans-ducer head.4.1.4At afixed distance(Z),align the transducer and/or stage for the maximum reflected amplitude from the top surface of the sample.The transducer must be perpendicular to the sample surface.4.1.5Focus by maximizing the amplitude,in the A-mode display,of the reflection from the interface designated for imag-ing.This is done by adjusting the Z-axis distance between the transducer and the sample.4.2Perform Acoustic Scans4.2.1Inspect the acoustic image(s)for any anomalies,verify that the anomaly is a package defect or an artifact of the imaging process,and record the results.(See Appendix A for an example of a check sheet that may be used.)To determine if an anomaly is a package defect or an artifact of the imaging process it is recommended to analyze the A-mode display at the location of the anomaly.4.2.2Consider potential pitfalls in image interpretation listed in,but not limited to,Appendix B and some of the limita-tions of acoustic microscopy listed in,but not limited to,Appendix C.If necessary,make adjustments to the equipment setup to optimize the results and rescan.4April1999IPC/JEDEC J-STD-035 4.2.3Evaluate the acoustic images using the failure criteria specified in other appropriate documents,such as J-STD-020.4.2.4Record the images and thefinal instrument setup parameters for documentation purposes.An example checklist is shown in Appendix D.5IPC/JEDEC J-STD-035April19996April1999IPC/JEDEC J-STD-035Appendix AAcoustic Microscopy Defect Check Sheet(continued)CIRCUIT SIDE SCANImage File Name/PathDelamination(Type I)Die Circuit Surface/Encapsulant Number Affected:Average%Location:Corner Edge Center (Type II)Die/Die Attach Number Affected:Average%Location:Corner Edge Center (Type III)Encapsulant/Substrate Number Affected:Average%Location:Corner Edge Center (Type V)Interconnect tip Number Affected:Average%Interconnect Number Affected:Max.%Length(Type VI)Intra-Laminate Number Affected:Average%Location:Corner Edge Center Comments:CracksAre cracks present:Yes NoIf yes:Do any cracks intersect:bond wire ball bond wedge bond tab bump tab leadDoes crack extend from leadfinger to any other internal feature:Yes NoDoes crack extend more than two-thirds the distance from any internal feature to the external surfaceof the package:Yes NoAdditional verification required:Yes NoComments:Mold Compound VoidsAre voids present:Yes NoIf yes:Approx.size Location(if multiple voids,use comment section)Do any voids intersect:bond wire ball bond wedge bond tab bump tab lead Additional verification required:Yes NoComments:7IPC/JEDEC J-STD-035April1999Appendix AAcoustic Microscopy Defect Check Sheet(continued)NON-CIRCUIT SIDE SCANImage File Name/PathDelamination(Type IV)Encapsulant/Substrate Number Affected:Average%Location:Corner Edge Center (Type II)Substrate/Die Attach Number Affected:Average%Location:Corner Edge Center (Type V)Interconnect Number Affected:Max.%LengthLocation:Corner Edge Center (Type VI)Intra-Laminate Number Affected:Average%Location:Corner Edge Center (Type VII)Heat Spreader Number Affected:Average%Location:Corner Edge Center Additional verification required:Yes NoComments:CracksAre cracks present:Yes NoIf yes:Does crack extend more than two-thirds the distance from any internal feature to the external surfaceof the package:Yes NoAdditional verification required:Yes NoComments:Mold Compound VoidsAre voids present:Yes NoIf yes:Approx.size Location(if multiple voids,use comment section)Additional verification required:Yes NoComments:8Appendix BPotential Image PitfallsOBSERV ATIONS CAUSES/COMMENTSUnexplained loss of front surface signal Gain setting too lowSymbolization on package surfaceEjector pin knockoutsPin1and other mold marksDust,air bubbles,fingerprints,residueScratches,scribe marks,pencil marksCambered package edgeUnexplained loss of subsurface signal Gain setting too lowTransducer frequency too highAcoustically absorbent(rubbery)fillerLarge mold compound voidsPorosity/high concentration of small voidsAngled cracks in package‘‘Dark line boundary’’(phase cancellation)Burned molding compound(ESD/EOS damage)False or spotty indication of delamination Low acoustic impedance coating(polyimide,gel)Focus errorIncorrect delamination gate setupMultilayer interference effectsFalse indication of adhesion Gain set too high(saturation)Incorrect delamination gate setupFocus errorOverlap of front surface and subsurface echoes(transducerfrequency too low)Fluidfilling delamination areasApparent voiding around die edge Reflection from wire loopsIncorrect setting of void gateGraded intensity Die tilt or lead frame deformation Sample tiltApril1999IPC/JEDEC J-STD-0359Appendix CSome Limitations of Acoustic MicroscopyAcoustic microscopy is an analytical technique that provides a non-destructive method for examining plastic encapsulated components for the existence of delaminations,cracks,and voids.This technique has limitations that include the following: LIMITATION REASONAcoustic microscopy has difficulty infinding small defects if the package is too thick.The ultrasonic signal becomes more attenuated as a function of two factors:the depth into the package and the transducer fre-quency.The greater the depth,the greater the attenuation.Simi-larly,the higher the transducer frequency,the greater the attenu-ation as a function of depth.There are limitations on the Z-axis(axial)resolu-tion.This is a function of the transducer frequency.The higher the transducer frequency,the better the resolution.However,the higher frequency signal becomes attenuated more quickly as a function of depth.There are limitations on the X-Y(lateral)resolu-tion.The X-Y(lateral)resolution is a function of a number of differ-ent variables including:•Transducer characteristics,including frequency,element diam-eter,and focal length•Absorption and scattering of acoustic waves as a function of the sample material•Electromechanical properties of the X-Y stageIrregularly shaped packages are difficult to analyze.The technique requires some kind offlat reference surface.Typically,the upper surface of the package or the die surfacecan be used as references.In some packages,cambered packageedges can cause difficulty in analyzing defects near the edgesand below their surfaces.Edge Effect The edges cause difficulty in analyzing defects near the edge ofany internal features.IPC/JEDEC J-STD-035April1999 10April1999IPC/JEDEC J-STD-035Appendix DReference Procedure for Presenting Applicable Scanned DataMost of the settings described may be captured as a default for the particular supplier/product with specific changes recorded on a sample or lot basis.Setup Configuration(Digital Setup File Name and Contents)Calibration Procedure and Calibration/Reference Standards usedTransducerManufacturerModelCenter frequencySerial numberElement diameterFocal length in waterScan SetupScan area(X-Y dimensions)Scan step sizeHorizontalVerticalDisplayed resolutionHorizontalVerticalScan speedPulser/Receiver SettingsGainBandwidthPulseEnergyRepetition rateReceiver attenuationDampingFilterEcho amplitudePulse Analyzer SettingsFront surface gate delay relative to trigger pulseSubsurface gate(if used)High passfilterDetection threshold for positive oscillation,negative oscillationA/D settingsSampling rateOffset settingPer Sample SettingsSample orientation(top or bottom(flipped)view and location of pin1or some other distinguishing characteristic) Focus(point,depth,interface)Reference planeNon-default parametersSample identification information to uniquely distinguish it from others in the same group11IPC/JEDEC J-STD-035April1999Appendix DReference Procedure for Presenting Applicable Scanned Data(continued) Reference Procedure for Presenting Scanned DataImagefile types and namesGray scale and color image legend definitionsSignificance of colorsIndications or definition of delaminationImage dimensionsDepth scale of TOFDeviation from true aspect ratioImage type:A-mode,B-mode,C-mode,TOF,Through TransmissionA-mode waveforms should be provided for points of interest,such as delaminated areas.In addition,an A-mode image should be provided for a bonded area as a control.12Standard Improvement FormIPC/JEDEC J-STD-035The purpose of this form is to provide the Technical Committee of IPC with input from the industry regarding usage of the subject standard.Individuals or companies are invited to submit comments to IPC.All comments will be collected and dispersed to the appropriate committee(s).If you can provide input,please complete this form and return to:IPC2215Sanders RoadNorthbrook,IL 60062-6135Fax 847509.97981.I recommend changes to the following:Requirement,paragraph number Test Method number,paragraph numberThe referenced paragraph number has proven to be:Unclear Too RigidInErrorOther2.Recommendations forcorrection:3.Other suggestions for document improvement:Submitted by:Name Telephone Company E-mailAddress City/State/ZipDate ASSOCIATION CONNECTING ELECTRONICS INDUSTRIESASSOCIATION CONNECTINGELECTRONICS INDUSTRIESISBN#1-580982-28-X2215 Sanders Road, Northbrook, IL 60062-6135Tel. 847.509.9700 Fax 847.509.9798。
金属的英语单词
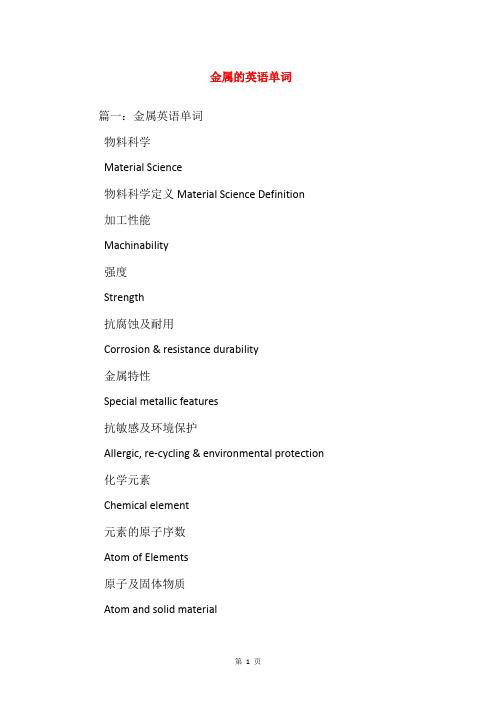
金属的英语单词篇一:金属英语单词物料科学Material Science物料科学定义Material Science Definition加工性能Machinability强度Strength抗腐蚀及耐用Corrosion & resistance durability金属特性Special metallic features抗敏感及环境保护Allergic, re-cycling & environmental protection 化学元素Chemical element元素的原子序数Atom of Elements原子及固体物质Atom and solid material原子的组成、大小、体积和单位图表The size, mass, charge of an atom, and is particles (Pronton,Nentron and Electron)原子的组织图Atom Constitutes周期表Periodic Table原子键结Atom Bonding金属与合金Metal and Alloy铁及非铁金属Ferrous & Non Ferrous Metal金属的特性Features of Metal晶体结构Crystal Pattern晶体结构,定向格子及单位晶格Crystal structure, Space lattice & Unit cellX线结晶分析法X –ray crystal analyics method金属结晶格子Metal space lattice格子常数Lattice constant米勒指数Mill's Index金相及相律Metal Phase and Phase Rule固熔体Solid solution置换型固熔体Substitutional type solid solution 插入型固熔体Interstital solid solution金属间化物Intermetallic compound金属变态Transformation变态点Transformation Point磁性变态Magnetic Transformation同素变态Allotropic Transformation合金平衡状态Thermal Equilibrium相律Phase Rule自由度Degree of freedom临界温度Critical temperture共晶Eutectic包晶温度Peritectic Temperature包晶反应Peritectic Reaction包晶合金Peritectic Alloy亚共晶体Hypoeutetic Alloy过共晶体Hyper-ectectic Alloy金属的相融、相融温度、晶体反应及合金在共晶合金、固熔孻共晶合金及偏晶反应的比较Equilibrium Comparision金属塑性Plastic Deformation滑动面Slip Plan畸变Distortion硬化Work Hardening退火Annealing回复柔软Crystal Recovery再结晶Recrystallization金属材料的性能及试验Properties & testing of metal化学性能Chemical Properties物理性能Physical Properties颜色Colour磁性Magnetisum比电阻Specific resistivity & specific resistance比重Specific gravity & specific density比热Specific Heat热膨胀系数Coefficient of thermal expansion导热度Heat conductivity机械性能Mechanical properties屈服强度(降伏强度) (Yield strangth)弹性限度、阳氏弹性系数及屈服点elastic limit, Yeung's module of elasticity to yield point 伸长度Elongation断面缩率Reduction of area金属材料的试验方法The Method of Metal inspection不破坏检验篇二:金属材料相关英语词汇物料科学Material Science物料科学定义Material Science Definition加工性能Machinability强度Strength抗腐蚀及耐用Corrosion & resistance durability金属特性Special metallic features抗敏感及环境保护Allergic, re-cycling & environmental protection 化学元素Chemical element元素的原子序数Atom of Elements原子及固体物质Atom and solid material原子的组成、大小、体积和单位图表The size, mass, charge of an atom, and is particles (Pronton,Nentronand Electron)原子的组织图Atom Constitutes周期表Periodic Table原子键结Atom Bonding金属与合金Metal and Alloy铁及非铁金属Ferrous & Non Ferrous Metal金属的特性Features of Metal晶体结构Crystal Pattern晶体结构,定向格子及单位晶格Crystal structure, Space lattice & Unit cellX线结晶分析法X –ray crystal analyics method金属结晶格子Metal space lattice格子常数Lattice constant米勒指数Mill's Index金相及相律Metal Phase and Phase Rule固熔体Solid solution置换型固熔体Substitutional type solid solution 插入型固熔体Interstital solid solution金属间化物Intermetallic compound金属变态Transformation变态点Transformation Point磁性变态Magnetic Transformation同素变态Allotropic Transformation合金平衡状态Thermal Equilibrium相律Phase Rule自由度Degree of freedom临界温度Critical tempertureEutectic包晶温度Peritectic Temperature包晶反应Peritectic Reaction包晶合金Peritectic Alloy亚共晶体Hypoeutetic Alloy过共晶体Hyper-ectectic Alloy金属的相融、相融温度、晶体反应及合金在共晶合金、固熔孻共晶合金及偏晶反应的比较Equilibrium Comparision金属塑性Plastic Deformation滑动面Slip Plan畸变Distortion硬化Work Hardening退火Annealing回复柔软Crystal Recovery再结晶Recrystallization金属材料的性能及试验Properties & testing of metal化学性能Chemical Properties物理性能Physical PropertiesColour磁性Magnetisum比电阻Specific resistivity & specific resistance比重Specific gravity & specific density比热Specific Heat热膨胀系数Coefficient of thermal expansion导热度Heat conductivity机械性能Mechanical properties屈服强度(降伏强度) (Yield strangth)弹性限度、阳氏弹性系数及屈服点elastic limit, Yeung's module of elasticity to yield point 伸长度Elongation断面缩率Reduction of area金属材料的试验方法The Method of Metal inspection 不破坏检验Non –destructive inspections 渗透探伤法Penetrate inspection磁粉探伤法Magnetic particle inspection放射线探伤法Radiographic inspection超声波探伤法Ultrasonic inspection显微观察法Microscopic inspection破坏的检验Destructive Inspection冲击测试Impact Test疲劳测试Fatigue Test潜变测试Creep Test潜变强度Creeps Strength第壹潜变期Primary Creep第二潜变期Secondary Creep第三潜变期Tertiary Creep主要金属元素之物理性质Physical properties of major Metal Elements工业标准及规格–铁及非铁金属Industrial Standard –Ferrous & Non –ferrous Metal磁力Magnetic简介General软磁Soft Magnetic硬磁Hard Magnetic磁场Magnetic Field磁性感应Magnetic Induction透磁度Magnetic Permeability磁化率Magnetic Susceptibility (Xm)磁力(Magnetic Force)及磁场(Magnetic Field)是因物料里的电子(Electron)活动而产生抗磁体、顺磁体、铁磁体、反铁磁体及亚铁磁体Diamagnetism, Paramagnetic, Ferromagnetism,Antiferromagnetism &Ferrimagnetism抗磁体Diamagnetism磁偶极子Dipole负磁力效应Negative effect顺磁体Paramagnetic正磁化率Positive magnetic susceptibility铁磁体Ferromagnetism转变元素Transition element交换能量Positive energy exchange外价电子Outer valence electrons化学结合Chemical bond自发上磁Spontaneous magnetization磁畴Magnetic domain相反旋转Opposite span比较抗磁体、顺磁体及铁磁体Comparison of Diamagnetism, Paramagnetic & Ferromagnetism反铁磁体Antiferromagnetism亚铁磁体Ferrimagnetism磁矩magnetic moment净磁矩Net magnetic moment钢铁的主要成份The major element of steel钢铁用"碳"之含量来分类Classification of Steel according to Carbon contents铁相Steel Phases钢铁的名称Name of steel纯铁体Ferrite渗碳体Cementitle奥氏体Austenite(转载自第一范文网,请保留此标记。
煤岩识别技术发展综述
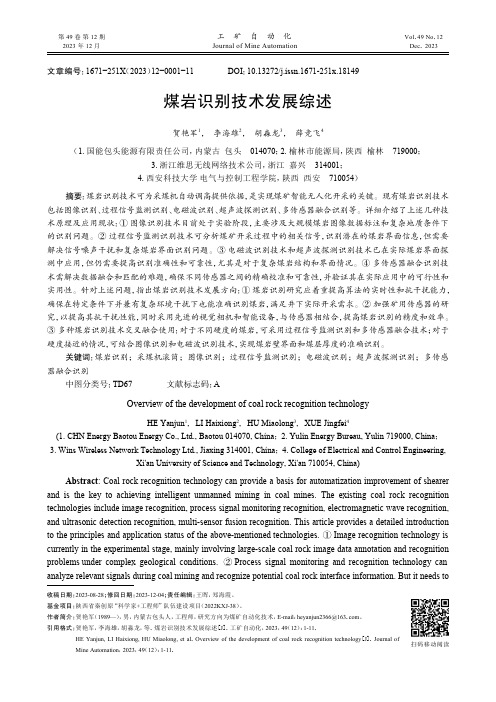
煤岩识别技术发展综述贺艳军1, 李海雄2, 胡淼龙3, 薛竞飞4(1. 国能包头能源有限责任公司,内蒙古 包头 014070;2. 榆林市能源局,陕西 榆林 719000;3. 浙江维思无线网络技术公司,浙江 嘉兴 314001;4. 西安科技大学 电气与控制工程学院,陕西 西安 710054)摘要:煤岩识别技术可为采煤机自动调高提供依据,是实现煤矿智能无人化开采的关键。
现有煤岩识别技术包括图像识别、过程信号监测识别、电磁波识别、超声波探测识别、多传感器融合识别等。
详细介绍了上述几种技术原理及应用现状:① 图像识别技术目前处于实验阶段,主要涉及大规模煤岩图像数据标注和复杂地质条件下的识别问题。
② 过程信号监测识别技术可分析煤矿开采过程中的相关信号,识别潜在的煤岩界面信息,但需要解决信号噪声干扰和复杂煤岩界面识别问题。
③ 电磁波识别技术和超声波探测识别技术已在实际煤岩界面探测中应用,但仍需要提高识别准确性和可靠性,尤其是对于复杂煤岩结构和界面情况。
④ 多传感器融合识别技术需解决数据融合和匹配的难题,确保不同传感器之间的精确校准和可靠性,并验证其在实际应用中的可行性和实用性。
针对上述问题,指出煤岩识别技术发展方向:① 煤岩识别研究应着重提高算法的实时性和抗干扰能力,确保在特定条件下并兼有复杂环境干扰下也能准确识别煤岩,满足井下实际开采需求。
② 加强矿用传感器的研究,以提高其抗干扰性能,同时采用先进的视觉相机和智能设备,与传感器相结合,提高煤岩识别的精度和效率。
③ 多种煤岩识别技术交叉融合使用:对于不同硬度的煤岩,可采用过程信号监测识别和多传感器融合技术;对于硬度接近的情况,可结合图像识别和电磁波识别技术,实现煤岩壁界面和煤层厚度的准确识别。
关键词:煤岩识别;采煤机滚筒;图像识别;过程信号监测识别;电磁波识别;超声波探测识别;多传感器融合识别中图分类号:TD67 文献标志码:AOverview of the development of coal rock recognition technologyHE Yanjun 1, LI Haixiong 2, HU Miaolong 3, XUE Jingfei 4(1. CHN Energy Baotou Energy Co., Ltd., Baotou 014070, China ; 2. Yulin Energy Bureau, Yulin 719000, China ;3. Wins Wireless Network Technology Ltd., Jiaxing 314001, China ; 4. College of Electrical and Control Engineering,Xi'an University of Science and Technology, Xi'an 710054, China)Abstract : Coal rock recognition technology can provide a basis for automatization improvement of shearer and is the key to achieving intelligent unmanned mining in coal mines. The existing coal rock recognition technologies include image recognition, process signal monitoring recognition, electromagnetic wave recognition,and ultrasonic detection recognition, multi-sensor fusion recognition. This article provides a detailed introduction to the principles and application status of the above-mentioned technologies. ① Image recognition technology is currently in the experimental stage, mainly involving large-scale coal rock image data annotation and recognition problems under complex geological conditions. ② Process signal monitoring and recognition technology can analyze relevant signals during coal mining and recognize potential coal rock interface information. But it needs to收稿日期:2023-08-28;修回日期:2023-12-04;责任编辑:王晖,郑海霞。
NDT英汉无损检测词汇
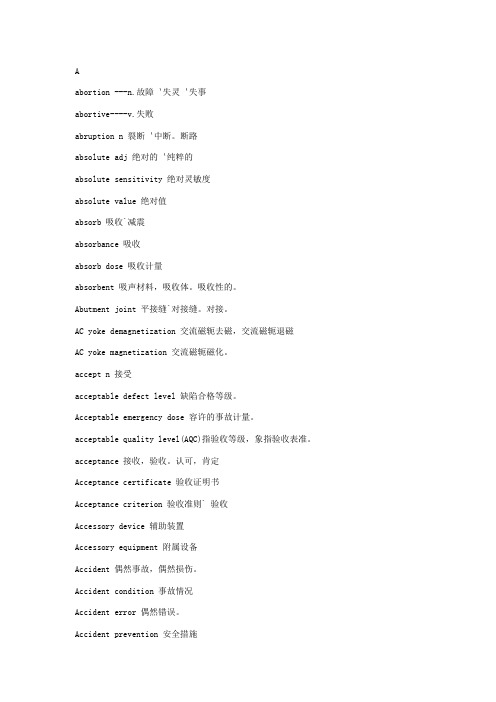
Aabortion ---n.故障`失灵`失事abortive----v.失败abruption n 裂断`中断。
断路absolute adj 绝对的`纯粹的absolute sensitivity 绝对灵敏度absolute value 绝对值absorb 吸收`减震absorbance 吸收absorb dose 吸收计量absorbent 吸声材料,吸收体。
吸收性的。
Abutment joint 平接缝`对接缝。
对接。
AC yoke demagnetization 交流磁轭去磁,交流磁轭退磁AC yoke magnetization 交流磁轭磁化。
accept n 接受acceptable defect level 缺陷合格等级。
Acceptable emergency dose 容许的事故计量。
acceptable quality level(AQC)指验收等级,象指验收表准。
acceptance 接收,验收。
认可,肯定Acceptance certificate 验收证明书Acceptance criterion 验收准则` 验收Accessory device 辅助装置Accessory equipment 附属设备Accident 偶然事故,偶然损伤。
Accident condition 事故情况Accident error 偶然错误。
Accident prevention 安全措施Accidental exposure 偶然曝光Accidental radiation injury偶然辐射伤害。
Accumulate dose 总剂量,累计剂量。
Accumulator battery 蓄电池Accumulator cell 蓄电池Acetic acid 醋酸,乙酸。
ACDS(acoustic crack detection system)声裂纹检测系统,声裂纹检测装置,声裂纹检测仪。
航空发动机压气机叶片损伤分析与监控对策

试验研究_________________Nfffl D O I:ia.l l973/wsjc202101005航空发动机压气机叶片损伤分析与监控对策张海兵\张泰峰、郭奇z(1.海军航空大学青岛校区航空机械工程与指挥系,青岛266041;2.北京航空工程技术研究中心,北京100076)摘要:针对发动机在服役过程中,压气机叶片因外物打伤而产生裂纹、掉块等缺陷的问题,运用基于断口分析和能谱分析的失效分析方法,查明了导致发动机压气机叶片损伤的机理和根本 原因,提出了集内窥检测和涡流检测于一体的监控对策。
通过检测试验分析了叶片损伤图像和检 测信号的典型特征,找出了缺陷的涡流信号显示与实际缺陷大小的对应关系,为准确可靠地定量判 断叶片损伤提供了基准。
关键词:航空发动机;压气机;叶片;损伤中图分类号:TG115.28 文献标志码:A 文章编号=1000-6656(2021)01-0015-04Damage analysis and monitoring measures of compressor blades of an aero engineZHANG Haibing1 . ZHANG Taifeng , GUO Qi2(1. Department of Aviation Mechanical Engineering and Command, Naval Aeronautical University Qingdao Campus,Qingdao 266041, China;2. Beijing Aeronautical Technology Research Center,Beijing 100076, China) Abstract:During the service of the engine, the compressor blades are damaged by foreign objects, resulting in cracks, falling blocks and other defects, this paper uses the failure analysis method based on fracture analysis technology and energy spectrum analysis technology to find out the mechanism and root cause of compressor blade damage,and puts forward the integrated endoscopic detection and eddy current detection in one monitoring strategy. The typical characteristics of blade damage image and damage detection signal are analyzed through inspection test, and the corresponding relationship between eddy current signal display of defect and actual size is found out, which provides a reference for accurate and reliable quantitative judgment.K ey w ords:aero engine ;compressor ;blade ;damage压气机是航空发动机动力装置的主要组成部分 和关键部件,是大气流经燃气动力装置的首要通道,其性能对航空发动机的性能将产生直接影响。
石油英语词汇
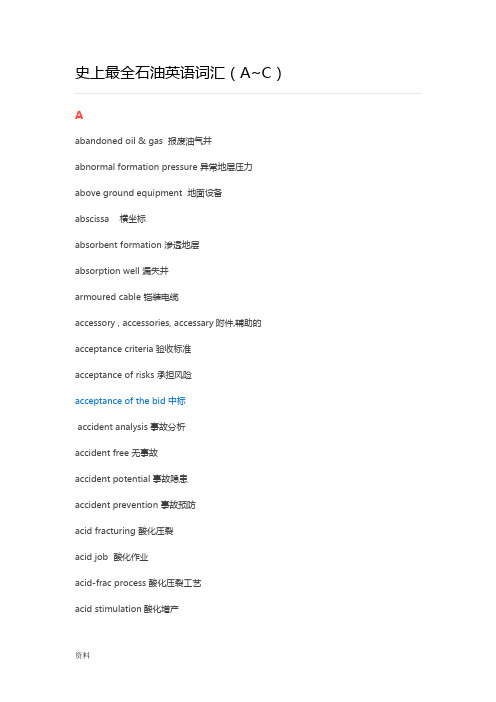
史上最全石油英语词汇(A~C)Aabandoned oil & gas 报废油气井abnormal formation pressure 异常地层压力above ground equipment 地面设备abscissa 横坐标absorbent formation 渗透地层absorption well 漏失井armoured cable 铠装电缆accessory , accessories, accessary附件,辅助的acceptance criteria 验收标准acceptance of risks 承担风险acceptance of the bid 中标accident analysis 事故分析accident free 无事故accident potential 事故隐患accident prevention 事故预防acid fracturing 酸化压裂acid job 酸化作业acid-frac process 酸化压裂工艺acid stimulation酸化增产actual data 实测资料actual data plot 实测曲线actuating system 传动系统assistant driller 副司钻adapter substitute 转换接头,大小头addendum 附录additional well 补充井adjacent well 邻井adjustment well 调整井adjust to zero 调零adjuster sub 调整短节adapter 适配器,接头automatic fine tuning 自动微调after-sale service 售后服务,后续服务after-treatment profile log 油井(增产)措施后生产剖面测井hole azimuth 井斜方向AL(acoustic logging)声波测井acoustic amplitude log,amplitude log声幅测井adjacent bed 围岩apparent resistivity视电阻率ACBL(acoustic cement bond log)声波水泥胶结测井activation logging活化测井ASC(after survey calibration)测后刻度amplitude type cement bond log声幅水泥胶结测井annular space log环空测井array sonic tool阵列声波测井仪acoustic wavetrain logging声波全波列测井alemite gun 黄油枪alligator wrench 活动管钳alternator 交流发电机A-mast A形井架amplifier 放大器amplitude 振幅,声波幅度annulus(油套管)环形空间analog cable signal 模拟电缆信号anchor packer 卡瓦封隔器annual survey 年检annular blowout preventer环形防喷器annular pressure 环空压力annular pressure operated test valve环空压力控制测试阀annular reversing valve环空加压反循环阀annular space 环形空间annular water detector环空找水仪annular water injection反注,环空注入annulus blow out 环空井喷annulus completion fluid 环空完井液annulus (flow)line 环空(出油)管annulus fluid环空液体annulus logging环空测试annulus pack off环空封隔装置annulus pressure firing system环空压力起爆器annulus pressure response tool APR工具,(哈里伯顿)环空加压式测试阀annulus sample catcher环空取样器anticline背斜antiknock agent 防爆剂antivibration防震,抗震API pup joint API标准油管短节apparent wellbore radius有效井筒半径appraisal well 评价井aqueous saturation 含水饱和度APR(annulus pressure respondent)环空压力控制式测试工具area operations superintendent 地区作业监督armoured (electric) cable 铠装电缆artificial bottom hole 人工井底artificial fracture 人造裂缝artificial lift 机械采油assessment of bids 评标assessment well评价井assistant driller 副司钻assistant engineer助理工程师associated gas 伴生气assurance factor安全系数atmospheric service常压作业autocartograph 自动绘图仪autoignition自动点火AVL(acoustic velocity log )声速测井azimuth 井斜方位Bbit 钻头,刀片box 母扣,母接头block区块,滑轮车,断快back flow反循环洗井backup arm 推靠臂backup copy 副本bad earth 接地不良bail 提环,提捞back off 倒扣,解卡bailer捞砂筒bailing 提捞作业bailing bucket 提捞筒bar drop投棒bar drop sub 投杆接头,撞击接头barefoot completion 裸眼完井barefoot interval 裸眼井段barefoot well(bare hole)裸眼井bar fishing 打捞点火棒bar hydrostatic firing head 投棒压力起爆器basement rock基岩bastard connection 不合格的丝扣(接头)bastard thread不合格的丝扣behind completion date没有按期完工bit diameter钻头直径breakdown pressure 临界压力bailed dry (把井)掏空beam pumping unit 游梁式抽油机beam well (深井泵)抽油井bean up 放喷bed of interest 目的层bellmouth喇叭口,锥形孔belly band 安全带bell nipple喇叭口短节bottom hole fluid sampler井底流体取样器bottom hole assembly 底部管柱结构BHC(borehole compensated sonic log )井眼补偿声波测井background radiation放射性本底植banana plug香蕉插头before calibration测前刻度BHTV(borehole televiewer)井下(声波)电视borehole fluid sampler井眼流体取样器bridle马笼头bradenhead flange 井口法兰bottom hole 井底,井下big hole perforator大孔眼射孔器borehole status井眼状况bottom-hole pressure ,closed井底关井压力bottom-hole pressure,flowing井底流动压力bottom-hole sample 井底取样,井下取样bottom hole sampler井底取样器bid bond投标保证金,投标保证书bidder 投标者bidding投标bidding documents投标文件,招标文件bidding procedure 投标程序bid opening开标big-entry-hole大射孔孔眼big-frac treatment(多层段)大型压裂big repair大修bill of materials材料清单bit hook打捞工具blank footage枪接头盲区blank off 油层封住,封堵blank tubing空油管,盲枪blasting cap 起爆雷管bleeding off pressure 放压block and tackle arrangement起下用滑车系统block diagram方框图,结构图block-squeeze分段挤水泥blowdown放压,衰竭式开采blowing out井喷blowing well自喷井blowout capping 控制井喷blowout hookup(井口)防喷装置blowout preventer 防喷器blank plug 丝堵,堵头blowout-prevention equipment防喷设备blowout-prevention procedure防喷措施bullets射孔弹blue sky exploratory well预探井bumper减震器back out(break out)卸开,松扣bobtail packer裸眼井测试封隔器bonding cable 接地电缆,地线booster传爆管bore diameter孔径,井眼直径bore frame井身结构borehole correction井眼校正borehole deviation井斜borehole effect井眼效应borehole face井壁borehole log 测井曲线,录井图borehole operation井下作业borehole pressure井筒压力borehole survey井下测量borehole wall井壁boring casing套管borings岩屑boring tower井架bottom hole turbine flowmeter井下涡轮流量计bottom nose (下井仪)尾端,(射孔)枪尾bottom-up firing枪尾起爆器box and pin公母接头box coupling接箍bridge plug桥塞by-pass旁通brake handle刹把breakdown 故障,事故break off pin type circulating sub断销式反循环阀breaking out pipe卸扣bridge plugging(打)桥塞封堵bridge the hole (在井中)打桥塞bring in a well完井投产bring out production投产,进行开采broken down 解堵brought in well投产井button switch按钮开关bushing 套筒basket 打捞篮,水泥伞bottomed 下到井底borehole televiewer井下电视buffer减震器build up压力恢复bubble 气泡,水泡bubble head泡泡头bubble point pressure饱和压力bucket rod抽油杆buck up紧扣build up of fluid液面恢复build the pressure打压build up of pressure压力恢复bull dog wrench管钳bullet gun射孔枪bullet hole射孔孔眼bumper spring 减震器弹簧burr毛刺,去毛刺by-pass pipe旁通管Ccontractor承包商C&F (cost and freight)成本加运费价,到岸价cement in place注水泥完毕cabin操作室cable armour电缆外皮cable bond(joint)电缆接头cable conductor缆芯cable configuration电缆规格cable continuity电缆通断情况cable core缆芯cable drum(reel)电缆滚筒cable duct 电缆槽cable fishing tool顿钻打捞工具cable formation test电缆地层测试cable head电缆头cable kinking电缆扭结cable-laid rope钢丝绳cable loop绳扣cable oil电缆油cable ringing电缆打结cable slack电缆松弛cable winch(work) 电缆绞车contract award date合同签定日期cake泥饼caliper井径仪calibration标定,调试,刻度calibrated标定过的calibrating jig刻度架caliper logging井径测量calling of bids招标callow表层camp equipment野外作业设备camp site施工现场cap rock盖层cap 雷管capping bed,cap formation盖层carbonate rock碳酸盐岩cased hole套管井cased hole completion 下套管完井cased hole formation tester套管井地层测试器case in 下套管cased well下套管井casing(case pipe)套管casing/cementation report套管固井报告casing cementing套管注水泥casing collar(coupling) 套管接箍CCL(casing collar locator)套管接箍测井casing grade套管钢级casing gun 电缆输送套管射孔枪casing head spool套管头四通casingless completion 无套管完井casing list 套管记录casing over pressure套管加压,环空加压casing packer套管封隔器casing perforation(套管)射孔casing perforation operator射孔作业工casing perforation service射孔作业casing perforation tool射孔器材casing perforation type射孔方式casing point下套管深度casing program套管程序casing setting depth套管下入深度casing shoe套管鞋casing top spacing套补距casing-tubing annulus油套环空casing wear套管损坏caution sign警告标志cave-in井壁坍塌core barrel取芯筒CBL(cement bond logging)水泥胶结测井carbon/oxygen logging碳氧比测井carbon/oxygen spectral logging 碳氧比能谱测井casing caliper log 套管内径测井casing inspection log套管探伤测井CBIL(circumferential borehole imaging logging system)环形井眼成象测井系统CET(cement evaluation tool)水泥评价测井channelling窜槽channelling detection找窜check shots检验地震测井completion date 完井日期cellar井口cellar connection(钻井)井口装置cemented to水泥返至cementing documentation固井设计cementing truck水泥车cement job quality固井质量cement sheath水泥环cement squeeze挤水泥cement top水泥返高centering device扶正器casing fluid level套管液面深度channel(射孔)孔道,管道,途径change over变扣接头change over sub大小头charge射孔弹,费用charge penetration射孔弹穿透深度charge strip(射孔)弹架charge type(射孔)弹型chief operator主操Chinese side中方christmas tree采油树christmas tree gauge井口压力表contract item合同条款casing inside diameter套管内径cement in place固井作业结束closed-in pressure关井压力circuit tester万用表clay 粘土caliper log井径测井claim 索赔claimer索赔人claim for breaking an agreement 违约赔偿claim for payment 要求付款claim indemnity索赔claim rejection拒赔claystone粘土岩,泥岩clean out (清砂、清蜡)修井clean-out of well 洗井clean-out operation(service)洗井作业clean-out report修井报告clear working place安全工作区clip绳卡closed-in关井closed-in well关闭井,停产井closed-in casing pressure关井套管压力closed-in tubing pressure关井油管压力cementer固井公司,固井队工人condensate凝析油coarse adjustment(control)粗调coarse -thread joint 粗扣接头change of contract合同变更casing outside diameter套管外径coiled rod 连续抽油杆coiled tubing连续油管,小油管coiled tubing service连续油管作业coiled tubing unit连续油管作业机coiled tubing operation小油管作业collar接箍,法兰盘,井口,钻铤collared hole下了表层套管的井combination collar(coupling)转换接箍combination tool组合下井仪commercial accumulation(deposit)有开采价值的油气藏commercial bed有开采价值的油气层commercial field有开采价值的油气田commingling production多层合采commissioning trial试用投产communication窜槽communication between zones窜层compensated density log补偿密度测井CDNT(compensated density-neutron tool)补偿地层密度中子测井仪compensated formation densilog补偿地层密度测井CNL(compensated neutron log)补偿中子测井compensated sonic log补偿声波测井conductivity log电导率测井conglomerate砾岩completion damage完井污染completion date完井日期completion evaluation完井评价completion fluid完井液completion operation 完井作业completion perforating完井射孔completion well test完井测试completion factor完井指数completion interval完井层段completion string完井管柱condensate field凝析气田condensate pool凝析油藏condensate well凝析油气井conductor line 导线conductor wire缆芯线connection end接头consent 插座console面板constant maintenance定期保养consumable material消耗材料consumable accessories耗损件contact probe探头contact sonde(井下仪器的)探头container horizon(rock)含油层,储集层contamination污染continental shelf大陆架contingency意外情况contingency plan(procedures)应急措施contract area合同区contract award date 合同签定日期contract duration合同期限contracted block合同区contracted construction承包施工contract life合同有效期限contract(or)employee施工人员contract item合同条款contributing zone生产层control fluid压井液controlled directional well定向井control of well kick and blowout井涌井喷控制control panel remote遥控面板conventional DST常规地层测试conventional gun普通射孔器conventional operation常规作业conventional overbalanced perforating 常规正压射孔conventional pumping unit普通抽油机conventional well常规井conventional workover rig常规修井作业core barrel岩心筒core bit取心钻头CST(core sample taker)井壁取心器cored well取心井coupled tubing油管立柱coupling tubing connection油管接箍连接cement plug水泥塞casing pressure-closed关井套压casing pressure-flowing开井套压combination production logging组合测井crystal quartz gauge石英晶体压力crab(winch,crane)吊车,起重机crown block天车crooked stem 弯曲的管柱cross over短节,变扣接头cross over circulation 反循环cross over sub(joint)变扣接头,转换接头crowbar 撬杠crown platform天车平台CST(chronological sample taker,chronological sidewall core tool)井壁取心器cartage运费complete trip in hole 完成(仪器)下井作业cable telemetry system电缆遥测系统cable transmission system电缆传输系统current maintenance 日常维修cushion 液垫,测试垫customs海关,关税cut-off date截止日期Ddrilling and production platform钻井采油平台drift angle(定向井)倾角data acquisition equipment数据采集装置daily capacity(output,production,rate)日产量daily servicing日常维护damaged formation受污染地层damaged well污染井damaged well productivity污染井产能damaged zone污染带damaged factor污染系数damage skin 损害表皮damper减震器damping spring减震弹簧data readback数据回放day to day operation日常业务drill collar钻铤dead time 停工时间dead well停产井dead work 准备工作,非生产性工作decenter 偏心decentralized casing 偏心套管decentralized gun偏心射孔器decentralized wellhead偏心井口decentralizing device偏心装置decline factor递减系数decline rate 递减速度decline exponent递减系数deep penetrating gun深穿透射孔枪deep penetration charge 深穿透射孔弹deep penetrator深井射孔弹ILD(deep induction log),deep investigation induction log深感应测井LLD(deep laterolog)深侧向测井depth mark of cable电缆深度记号Dewar flask,Dewar thermos bottle测井保温瓶default clause违约条款defective casing/liner cementation固井不合格defective insulation绝缘不良deficient well低效井deflected hole(well)斜井,定向井degree of damage污染程度degree of dip倾角degree of reserve recovery采出程度degree of saturation饱和度dehydrated crude脱水原油deliverability产能,产油delivery point供应点demodulator检波器demobilization复员,遣散demobilization cost遣散费demonstrated reserves探明储量demurrage charge(fee)延迟费density log密度测井deparaffin清蜡depleted field枯竭油田depleted oil sand枯竭油层depth in下入深度depreciation charge折旧费depth correction校深depth discrepancy深度误差depth of bottom hole measurement井底测量深度,测量仪器下入深度depth of plunger泵挂深度depth of setting下入深度depth out 起出深度depth sounding测液面深度derrick井架,钻塔derrick apples井架落物derrick floor钻台derrick guy(wire)井架绷绳derrick lowering放倒井架derrick raising立井架desanding device除砂装置description of reservoir油藏描述design pressure 设计压力desired flow rate 预期产量desired location 预定位置desired setting position预定坐封位置detector检波器,引爆器,雷管detail design施工设计detailed rules and regulations细则detail specifications详细说明书detecting head 探头,探针detention time 停留时间deterioration老化,磨损determining depth of fluid探测液面深度detonating cord导爆索detonation爆燃,爆炸detonator 雷管deviation井斜development program开发方案development well开发井deviated hole(well)斜井,定向井direct hydrocarbon indication直接油气显示down hole power supply井下供电DAL(digital acoustic log)数字阵列声波difference between reservoir pressure and injection pressure 注水压差differential pressure压差differential pressure firing head压差起爆器digital multimeter数字万用表digital integrated circuit数字集成电路DCBIL(digitally circumferential borehole imaging log)数字井周成像diminishing pressure递减压力diphase双相diphasic flow 双相流动dipper捞砂筒diplog地层倾角测井diplog tool-4 arm四臂地层倾角测井仪dipmeter地层倾角测井仪dip倾角DDL(direct digital logging)数控测井distance of “zone”mark零长dipping rod量油杆dipping tub量油管direct-current generator直流发电机direct flushing正循环洗井direct grounding直接接地directional drilling定向钻井directives for construction施工指令director general 总裁,总监disabling accident严重伤害事故displaced well生产井disruptive test击穿实验,耐压实验distance between wells井距distance between rotary table and wellhead联入distance between tubing and bushing油补距distance measurement遥测distance of zero mark零长distillate field凝析气田distinguishability分辨率division 刻度,分公司dividing range (油水,油气)分界面dog-leg狗腿depth logger测井深度dual lower casing 下部双层套管DDL(dual laterolog)双侧向测井dual induction focused log双感应聚焦测井dual induction-laterolog双感应—侧向测井dual induction-laterolog 8双感应—八侧向测井DIL(dual induction log)双感应测井dual laterolog-microspherically focused log双感应—微球形聚焦测井dual-spaced density log双源距密度测井DSN(dual-spaced neutron log)双源距中子测井dual-spacing thermal decay time log双源距中子寿命测井dual lower tubing下部双层油管drilling mud钻井液depth offset深度补偿drilled out钻出,钻碎(水泥塞)dolomite(dolostone)白云岩date of availability有效期限drilling-out cement(plug)钻水泥塞drilling-out depth钻水泥塞深度dog house井口值班房double O-ring seal双O形圈密封double drum drawworks(hoist),double drum rope winch双滚筒绞车dog shift夜班double-shift work两班倒downflow well注水井downhole blow-out preventer井下防喷器downhole calibration井下标定,现场调试downhole completion equipment井下完井装置down hole completion fluid 井下完井液down hole equipment井下工具downhole instrument,downhole probe,downhole tool下井仪器downhole operation井下作业downhole instrument cable井下电缆down hole sampling井底取样downhole string井下管柱downhole television井下电视downhole testing井下测试downhole test string井下测试管柱downhole water flow regulator井下配水器downhole test tool,downhole well test equipment井下测试工具downhole remedial operation井下大修作业,修井作业down hole tools installation井下工具连接down setting packer stroke封隔器坐封下滑距离downslide下滑down time(downtime)停工时间deepen加深draft agreement协议草案draft contract合同草本drawworks unit绞车drillable bridge plug可钻式桥塞drift diameter gauge,drift size gauge tool通井规drillable packer可钻式封隔器drill collar stand钻铤立柱drilled proved reserves钻井探明储量driller司钻driller's log 司钻记录,钻井记录drilling completion program钻井完井方案drilling crew 钻井队drilling foreman钻井队长drilling induced damage钻井引起的损害drilling returns钻井返出流体drilling rig,drill unit钻机drilling supervisor钻井监督drill pipe frozen卡钻DST(drill stem test)钻杆测试drowned well水淹井drum spool绞车滚筒dry well干井dry pipe空油管drillsite井场,钻井工地daily service report日报DST interval(地层)测试井段DST personnel测试人员DST/TCP(drillstem test/tubing conveyed perforating)油管传输射孔与地层测射联作DST string(地层)测试管串DSTT(drillstem test tool)中途测试工具,地层测试器duration of test测试周期duration of the contract合同期限duty cycle作业周期dynamic log动态测井,生产测井Eearly field life 油田开发初期earth bar接地棒earthing wire地线eccentric annulus偏心环空eccentric injection mandrel偏心配水器edge water flood边缘注水edge water incursion边水侵入edge well边缘井evacuation,escape and rescue 人员撤离,逃生和救护engineering flow diagram工艺流程图effluent pressure井口流体压力efflux射流,流出egress pressure出口压力external-internal upset内外加厚的(管子)electrical logging电测electrical detonator电雷管electrical line unit电缆试井装置electric line operation电缆作业electric line set packer电缆坐封封隔器electric monoconductor单芯电缆electric wireline unit operation电缆绞车操作EL(electric log)电法测井ES(electric survey)电法勘探electrode电极electrode pad电极极板electromagnetic flowmeter电磁流量计electronic cartridge电子线路短节elevator plug提升短节elevator吊卡emergency decree 安全技术规程emergency measures应急措施emergency escape应急出口emergency shutdown紧急关井,紧急停工employment contract雇用合同employment dismissal解雇endless tubing连续油管enhanced oil recovery强化采油,提高采收率enhanced recovery scheme强化采油方案enhanced water injection强化注水environmental pollution环境污染environmental conservation环境保护environmental regulations环保规定end of date截止日期equipment component list设备元件明细表equipment list设备清单equipment maintenance设备维修保养equipment malfunction设备故障equipment operating instructions设备操作说明equipment out设备发运equipment specification manual设备技术手册erected cost安装费用erection of derrick立井架erection schedule安装进程error band误差范围error correction误差校正estimated reserves控制储量external upset外加厚evaluation criterion评价标准evaluation of bid评标evaluation reservoir油藏评价evaluation well评价井exploration well探井examination and acception验收exceptional well特殊井excessive load超载excessive pressure超压executive cost施工费用exhibit 展示,附加条款expanded packer膨胀式封隔器expected reserves预计储量expendable gun一次性射孔器expendable plug一次性桥塞expiration of the contract合同期满expendable through-tubing perforator一次性过油管射孔器exploitable reserve可采储量exploitation plan开采方案exploitation velocity开采速度exploitation well开发井exploitation drilling 勘探钻井exploratory well探井exploitation of oil and gas field油气田勘探exploitation targets勘探目的层explored reserves探明储量explosive炸药explosive charge射孔弹,炸药explosive deterioration炸药失效extinguisher灭火器extension coupling加长短节extension mechanical firing head机械点火头extension well扩边井extent of formation damage地层损害程度external thread 公扣external upset tubing外加厚油管extra security额外保险extreme over balance超正压(射孔)eye diameter孔眼直径eyehole孔眼Ffirst aid 急救face of the wellbore井壁facsimile传真factor of porosity孔隙度factor of saturation 饱和度failure-free operation正常运行,安全操作fault block断块fault block hydrocarbon reservoir断块油藏faulty operation误操作flowing casing pressure 开井时套压FBS(full-bore spinner flowmeter)全径涡轮流量计fracture conductivity裂缝导流能力fire control equipment消防设备FDL(fluid density log)流体密度测井FDT(fluid density tool)流体密度测井仪flushed zone冲洗带FIL(fluid identification log)流体识别测井,含水率测井flow diagram 流程图field development plan油田开发方案feasibility study可行性研究felt油毛毡formation evaluation measurement-while-drilling随钻测试与地层评价formation evaluation while drilling随钻地层评价formation fluid sampler地层流体取样器formation fluid test地层流体测试field acquisition现场采集field adjustment现场调试field analysis现场解释field conduct现场实施field connection现场连接field environment野外条件field lighting工地照明field job design现场作业设计field maintenance现场维护field office值班室field operation现场作业field process现场施工field record(notes)现场记录field staff现场施工人员field situation油气田位置field type facility现场设施field well生产井field work野外作业FIL(fracture identification log)裂缝识别测井filling in well加密井final work收尾工作find bottom探井底fine adjustment,fine turning微调finish barefooted裸眼完井finishing drilling完钻fire escape,fire exit安全出口fire extinction灭火fire foam producing machine泡沫灭火器fire protection防火firing head点火装置firing pin点火撞针first aid急救first-hand information第一手资料first party甲方fish落鱼,井下落物fishing 打捞fishing basket打捞篮fishing job(operation)打捞作业fishing tool打捞工具fishing grab打捞爪fishing rod打捞棒fishing service打捞作业队fishing up打捞出fissure 裂缝fissured formation裂缝性地层fissured oil field裂缝性油田fissured reservoir裂缝性油气藏fissure fault裂缝断层fixed pulley定滑轮flame damper(snuffer)灭火器flame igniter点火装置flange法兰盘flank water drive边水驱动flank waterflooding边缘注水flank well油田边缘井flare opening喇叭口flexible connection揉性连接flood development注水开发flood effectiveness注水效果flooding network注采井网flooding well network注水井网floodout水淹floorman钻工flow capacity地层系数,产能系数flow head井口装置,测试井口flow column油管柱flowing bean(nipple)油嘴flowing tubing pressure开井油压flowing well自喷井flow rate log流量测井flow well开井测试,开井放喷fluid column cushion测试液垫,水垫fluid column pressure液柱压力fluid level液面深度flush collar平口接箍flushing fluid洗井液,钻井液flowmeter流量计field operation handbook,field operating manual现场操作手册force outage事故停机foreign side外方foreman工头,领班formation caving井壁坍塌formation contaminant地层污染formation evaluation地层评价formation fluid pressure地层流体压力formation fluid sampling地层流体取样formation fracturing 地层压裂formation of interest目的层formation sand flow地层出砂formation solid control防砂formation stimulation油层增产措施formation tester地层测试器FAL(formation - analysis log)地层分析测井FDC(formation density compensated)补偿地层密度测井仪formation density log地层密度测井FDT(formation density tool)地层密度测井仪formation to be tested测试层段,测试井段formation water resistivity地层水电阻率formation water salinity地层水矿化度fountain well自喷井four-arm caliper四臂井径仪four-way piece四通fire plug消防栓formation pressure gradient地层压力梯度fracture fluid 压裂液fracture job压裂作业fracture裂缝,压裂fracture acidizing压裂酸化fractured hydrocarbon reservoir裂缝油藏fractured distribution裂缝分布fractured well裂缝井,压裂井fracture fluid压裂液fracture permeability裂缝渗透率fracture porosity裂缝孔隙度FIL(fracture identification log)裂缝识别测井fracture log裂缝测井fracturing technology压裂工艺fracturing tool压裂工具fracturing truck(unit)压裂车freeze in卡钻,卡死full bore(flow,opening,port)全通径fullbore tool全通径工具GGeiger counter盖革计数管gas condensate凝析油,凝析气gamma ray spectrum log 自然伽马能谱测井gas condensate field 凝析气田gas condensate reservoir凝析油储量gas condensate well凝析气井gaseous well气井gas injection(input)well注气井gas lift气举gas lift equipment气举设备gas lift installation气举装置gas lift production气举采油gas lift string气举管柱gas lift well气举井gas mask防毒面具gas/oil horizon(interface,surface)油气界面gas-oil separation油气分离gas prospecting天然气勘探gauge cock压力表gauge reading仪表读数gauge ring通井规,通径规gauging nipple测量短节gun azimuth射孔枪方位ground equipment地面设备general purpose常用的,通用的general safety hazard一般事故general sketch(drawing)示意图geological base map井位图geological exploration地质勘探geological information地质资料geologic anomaly地质异常geologic condition地质条件geologic description地质描述geologic feature地质特征geologic framework(structure)地质构造geologic interpretation地质解释geologic profile(section)地质剖面geologic reserve地质储量geologic trap地质圈闭geophysical exploration地球物理勘探geopressured well高压井geophone检波器geophysical prospecting,geophysical survey地球物理勘探,物探general field engineer现场总工程师go in the hole下入井内go dead(油气井)停止自喷gun offset射孔枪偏位go fishing 进行打捞general operating specification一般操作规程gun perforation射孔gun perforator射孔枪gradient survey梯度测量gin滑车gradient sonde梯度电极系gradiomanometer压差密度仪grand slam大满贯测井解释方法gravity logging重力测井graph plotter绘图仪graveyard shift夜班grease gun黄油枪grease oil润滑油gamma ray log自然伽马测井gross record原始记录gross thickness总厚度ground protection接地保护ground support aquipment地面辅助设备good show of oil and gas油气显示良好guard electrode logging屏蔽电极测井,聚焦电阻率测井,侧向测井gun射孔枪gun carrier弹架,枪身gun perforated casing 射孔套管gun perforated completion射孔完井gun positioning射孔器定位gun stand-off枪身扶正gun system射孔设备gusher well自喷井,失控井Hhydraulic液压的,液压设备Halliburton turbine(flow)meter哈里伯顿涡轮流量计hard-boiled hat,hard hat,hardhat helmet安全帽hard disk硬盘handling sub提升短节hazard identification危险标志hazardous area(location)危险区域hazardous operation有害作业hazardous situation report事故报告hazardous substance有害物质hydrocarbon油气horizontal displacement水平位移headquaters总部headman组长,队长head space间距,安全距离head well puller修井队长heapstead井口房heavy crude oil,heavy oil重油,稠油heavy oil recovery稠油开采heavy weight tubing加厚油管heavy weight drilling pipe加厚钻杆HEGF(high energy gas fracturing)高能气体压裂helical shot pattern螺旋布孔格式helper副司钻heptcable七芯电缆hermetic seal,hermetization密封heterogeneous非均质的,多相的high-angle大斜度high definition,high resolution高分辨率high density pattern perforation高孔密射孔high-inclination well,high-deviated well,high angle deviated hole大斜度井high pressure gas well高压气井high productivity well,high rate producing well高产井HDT(high-resolution dipmeter tool)高分辨率地层倾角仪HDIL(high definition induction logging)高分辨率阵列感应high risk industry高危险行业high service高技术服务high-temperature service高温作业high water-cut layer高含水油层high-water-cut oil-producing well高含水产油井high-water-cut producer高含水油井high water-cut stage(油田)高含水期high water-cut well高含水井high wax content oil高含蜡原油historical well analysis老井分析hydraulically operated equipment液压驱动设备hoist提升,起钻hoistman绞车司机hole anomaly井眼不规则hole blow井喷hole caliper井径仪hole cleaning井眼清洗hole condition井眼条件hole deflection(deviation)井斜hole gauge通井规hole section井段hole pattern井网hole spacing井距hole structure井身结构hole toe 井底hole top井口hollow carrier射孔器枪身hollow carrier gun有枪身射孔器hook wrench钩头扳手hoot-owl shift(tour)大夜班horizontal deviation水平位移horizontal drainhole,horizontal well 水平井horizontal hole section水平井段horizontal well test水平井测试horsestone夹层horizontal drilling水平钻井hot work operational guideline火工作业操作规程hot work permitting procedure火工许可程序high pressure high temperature well test高温高压井测试hookwall packer卡瓦封隔器hydra-cushion shock tool液压减震工具hydraulically-powered winch液压绞车hydrocarbon release油气泄漏hydrocarbon resources油气资源hydrocarbon show油气显示hydrocarbon trap油气圈闭hydroseal液压密封Iinspection & acceptance record验收记录initial depth起始深度idle cost停工损失idle equipment(unit)闲置设备instrument engineer仪修工程师ignition voltage点火电压induction electrical log,induction electrical survey,induction log感应测井immediate vicinity of wellbore近井地带invitation for bid招标igneous rock火成岩igniter(ignitor)引爆装置igniter system,ignition system点火系统,点火装置impact damper减震器imperforated未射孔的。
特征更新的动态图卷积表面损伤点云分割方法

第41卷 第4期吉林大学学报(信息科学版)Vol.41 No.42023年7月Journal of Jilin University (Information Science Edition)July 2023文章编号:1671⁃5896(2023)04⁃0621⁃10特征更新的动态图卷积表面损伤点云分割方法收稿日期:2022⁃09⁃21基金项目:国家自然科学基金资助项目(61573185)作者简介:张闻锐(1998 ),男,江苏扬州人,南京航空航天大学硕士研究生,主要从事点云分割研究,(Tel)86⁃188****8397(E⁃mail)839357306@;王从庆(1960 ),男,南京人,南京航空航天大学教授,博士生导师,主要从事模式识别与智能系统研究,(Tel)86⁃130****6390(E⁃mail)cqwang@㊂张闻锐,王从庆(南京航空航天大学自动化学院,南京210016)摘要:针对金属部件表面损伤点云数据对分割网络局部特征分析能力要求高,局部特征分析能力较弱的传统算法对某些数据集无法达到理想的分割效果问题,选择采用相对损伤体积等特征进行损伤分类,将金属表面损伤分为6类,提出一种包含空间尺度区域信息的三维图注意力特征提取方法㊂将得到的空间尺度区域特征用于特征更新网络模块的设计,基于特征更新模块构建出了一种特征更新的动态图卷积网络(Feature Adaptive Shifting⁃Dynamic Graph Convolutional Neural Networks)用于点云语义分割㊂实验结果表明,该方法有助于更有效地进行点云分割,并提取点云局部特征㊂在金属表面损伤分割上,该方法的精度优于PointNet ++㊁DGCNN(Dynamic Graph Convolutional Neural Networks)等方法,提高了分割结果的精度与有效性㊂关键词:点云分割;动态图卷积;特征更新;损伤分类中图分类号:TP391.41文献标志码:A Cloud Segmentation Method of Surface Damage Point Based on Feature Adaptive Shifting⁃DGCNNZHANG Wenrui,WANG Congqing(School of Automation,Nanjing University of Aeronautics and Astronautics,Nanjing 210016,China)Abstract :The cloud data of metal part surface damage point requires high local feature analysis ability of the segmentation network,and the traditional algorithm with weak local feature analysis ability can not achieve the ideal segmentation effect for the data set.The relative damage volume and other features are selected to classify the metal surface damage,and the damage is divided into six categories.This paper proposes a method to extract the attention feature of 3D map containing spatial scale area information.The obtained spatial scale area feature is used in the design of feature update network module.Based on the feature update module,a feature updated dynamic graph convolution network is constructed for point cloud semantic segmentation.The experimental results show that the proposed method is helpful for more effective point cloud segmentation to extract the local features of point cloud.In metal surface damage segmentation,the accuracy of this method is better than pointnet++,DGCNN(Dynamic Graph Convolutional Neural Networks)and other methods,which improves the accuracy and effectiveness of segmentation results.Key words :point cloud segmentation;dynamic graph convolution;feature adaptive shifting;damage classification 0 引 言基于深度学习的图像分割技术在人脸㊁车牌识别和卫星图像分析领域已经趋近成熟,为获取物体更226吉林大学学报(信息科学版)第41卷完整的三维信息,就需要利用三维点云数据进一步完善语义分割㊂三维点云数据具有稀疏性和无序性,其独特的几何特征分布和三维属性使点云语义分割在许多领域的应用都遇到困难㊂如在机器人与计算机视觉领域使用三维点云进行目标检测与跟踪以及重建;在建筑学上使用点云提取与识别建筑物和土地三维几何信息;在自动驾驶方面提供路面交通对象㊁道路㊁地图的采集㊁检测和分割功能㊂2017年,Lawin等[1]将点云投影到多个视图上分割再返回点云,在原始点云上对投影分割结果进行分析,实现对点云的分割㊂最早的体素深度学习网络产生于2015年,由Maturana等[2]创建的VOXNET (Voxel Partition Network)网络结构,建立在三维点云的体素表示(Volumetric Representation)上,从三维体素形状中学习点的分布㊂结合Le等[3]提出的点云网格化表示,出现了类似PointGrid的新型深度网络,集成了点与网格的混合高效化网络,但体素化的点云面对大量点数的点云文件时表现不佳㊂在不规则的点云向规则的投影和体素等过渡态转换过程中,会出现很多空间信息损失㊂为将点云自身的数据特征发挥完善,直接输入点云的基础网络模型被逐渐提出㊂2017年,Qi等[4]利用点云文件的特性,开发了直接针对原始点云进行特征学习的PointNet网络㊂随后Qi等[5]又提出了PointNet++,针对PointNet在表示点与点直接的关联性上做出改进㊂Hu等[6]提出SENET(Squeeze⁃and⁃Excitation Networks)通过校准通道响应,为三维点云深度学习引入通道注意力网络㊂2018年,Li等[7]提出了PointCNN,设计了一种X⁃Conv模块,在不显著增加参数数量的情况下耦合较远距离信息㊂图卷积网络[8](Graph Convolutional Network)是依靠图之间的节点进行信息传递,获得图之间的信息关联的深度神经网络㊂图可以视为顶点和边的集合,使每个点都成为顶点,消耗的运算量是无法估量的,需要采用K临近点计算方式[9]产生的边缘卷积层(EdgeConv)㊂利用中心点与其邻域点作为边特征,提取边特征㊂图卷积网络作为一种点云深度学习的新框架弥补了Pointnet等网络的部分缺陷[10]㊂针对非规律的表面损伤这种特征缺失类点云分割,人们已经利用各种二维图像采集数据与卷积神经网络对风扇叶片㊁建筑和交通工具等进行损伤检测[11],损伤主要类别是裂痕㊁表面漆脱落等㊂但二维图像分割涉及的损伤种类不够充分,可能受物体表面污染㊁光线等因素影响,将凹陷㊁凸起等损伤忽视,或因光照不均匀判断为脱漆㊂笔者提出一种基于特征更新的动态图卷积网络,主要针对三维点云分割,设计了一种新型的特征更新模块㊂利用三维点云独特的空间结构特征,对传统K邻域内权重相近的邻域点采用空间尺度进行区分,并应用于对金属部件表面损伤分割的有用与无用信息混杂的问题研究㊂对邻域点进行空间尺度划分,将注意力权重分组,组内进行特征更新㊂在有效鉴别外邻域干扰特征造成的误差前提下,增大特征提取面以提高局部区域特征有用性㊂1 深度卷积网络计算方法1.1 包含空间尺度区域信息的三维图注意力特征提取方法由迭代最远点采集算法将整片点云分割为n个点集:{M1,M2,M3, ,M n},每个点集包含k个点:{P1, P2,P3, ,P k},根据点集内的空间尺度关系,将局部区域划分为不同的空间区域㊂在每个区域内,结合局部特征与空间尺度特征,进一步获得更有区分度的特征信息㊂根据注意力机制,为K邻域内的点分配不同的权重信息,特征信息包括空间区域内点的分布和区域特性㊂将这些特征信息加权计算,得到点集的卷积结果㊂使用空间尺度区域信息的三维图注意力特征提取方式,需要设定合适的K邻域参数K和空间划分层数R㊂如果K太小,则会导致弱分割,因不能完全利用局部特征而影响结果准确性;如果K太大,会增加计算时间与数据量㊂图1为缺损损伤在不同参数K下的分割结果图㊂由图1可知,在K=30或50时,分割结果效果较好,K=30时计算量较小㊂笔者选择K=30作为实验参数㊂在分析确定空间划分层数R之前,简要分析空间层数划分所应对的问题㊂三维点云所具有的稀疏性㊁无序性以及损伤点云自身噪声和边角点多的特性,导致了点云处理中可能出现的共同缺点,即将离群值点云选为邻域内采样点㊂由于损伤表面多为一个面,被分割出的损伤点云应在该面上分布,而噪声点则被分布在整个面的两侧,甚至有部分位于损伤内部㊂由于点云噪声这种立体分布的特征,导致了离群值被选入邻域内作为采样点存在㊂根据采用DGCNN(Dynamic Graph Convolutional Neural Networks)分割网络抽样实验结果,位于切面附近以及损伤内部的离群值点对点云分割结果造成的影响最大,被错误分割为特征点的几率最大,在后续预处理过程中需要对这种噪声点进行优先处理㊂图1 缺损损伤在不同参数K 下的分割结果图Fig.1 Segmentation results of defect damage under different parameters K 基于上述实验结果,在参数K =30情况下,选择空间划分层数R ㊂缺损损伤在不同参数R 下的分割结果如图2所示㊂图2b 的结果与测试集标签分割结果更为相似,更能体现损伤的特征,同时屏蔽了大部分噪声㊂因此,选择R =4作为实验参数㊂图2 缺损损伤在不同参数R 下的分割结果图Fig.2 Segmentation results of defect damage under different parameters R 在一个K 邻域内,邻域点与中心点的空间关系和特征差异最能表现邻域点的权重㊂空间特征系数表示邻域点对中心点所在点集的重要性㊂同时,为更好区分图内邻域点的权重,需要将整个邻域细分㊂以空间尺度进行细分是较为合适的分类方式㊂中心点的K 邻域可视为一个局部空间,将其划分为r 个不同的尺度区域㊂再运算空间注意力机制,为这r 个不同区域的权重系数赋值㊂按照空间尺度多层次划分,不仅没有损失核心的邻域点特征,还能有效抑制无意义的㊁有干扰性的特征㊂从而提高了深度学习网络对点云的局部空间特征的学习能力,降低相邻邻域之间的互相影响㊂空间注意力机制如图3所示,计算步骤如下㊂第1步,计算特征系数e mk ㊂该值表示每个中心点m 的第k 个邻域点对其中心点的权重㊂分别用Δp mk 和Δf mk 表示三维空间关系和局部特征差异,M 表示MLP(Multi⁃Layer Perceptrons)操作,C 表示concat 函数,其中Δp mk =p mk -p m ,Δf mk =M (f mk )-M (f m )㊂将两者合并后输入多层感知机进行计算,得到计算特征系数326第4期张闻锐,等:特征更新的动态图卷积表面损伤点云分割方法图3 空间尺度区域信息注意力特征提取方法示意图Fig.3 Schematic diagram of attention feature extraction method for spatial scale regional information e mk =M [C (Δp mk ‖Δf mk )]㊂(1) 第2步,计算图权重系数a mk ㊂该值表示每个中心点m 的第k 个邻域点对其中心点的权重包含比㊂其中k ∈{1,2,3, ,K },K 表示每个邻域所包含点数㊂需要对特征系数e mk 进行归一化,使用归一化指数函数S (Softmax)得到权重多分类的结果,即计算图权重系数a mk =S (e mk )=exp(e mk )/∑K g =1exp(e mg )㊂(2) 第3步,用空间尺度区域特征s mr 表示中心点m 的第r 个空间尺度区域的特征㊂其中k r ∈{1,2,3, ,K r },K r 表示第r 个空间尺度区域所包含的邻域点数,并在其中加入特征偏置项b r ,避免权重化计算的特征在动态图中累计单面误差指向,空间尺度区域特征s mr =∑K r k r =1[a mk r M (f mk r )]+b r ㊂(3) 在r 个空间尺度区域上进行计算,就可得到点m 在整个局部区域的全部空间尺度区域特征s m ={s m 1,s m 2,s m 3, ,s mr },其中r ∈{1,2,3, ,R }㊂1.2 基于特征更新的动态图卷积网络动态图卷积网络是一种能直接处理原始三维点云数据输入的深度学习网络㊂其特点是将PointNet 网络中的复合特征转换模块(Feature Transform),改进为由K 邻近点计算(K ⁃Near Neighbor)和多层感知机构成的边缘卷积层[12]㊂边缘卷积层功能强大,其提取的特征不仅包含全局特征,还拥有由中心点与邻域点的空间位置关系构成的局部特征㊂在动态图卷积网络中,每个邻域都视为一个点集㊂增强对其中心点的特征学习能力,就会增强网络整体的效果[13]㊂对一个邻域点集,对中心点贡献最小的有效局部特征的边缘点,可以视为异常噪声点或低权重点,可能会给整体分割带来边缘溢出㊂点云相比二维图像是一种信息稀疏并且噪声含量更大的载体㊂处理一个局域内的噪声点,将其直接剔除或简单采纳会降低特征提取效果,笔者对其进行低权重划分,并进行区域内特征更新,增强抗噪性能,也避免点云信息丢失㊂在空间尺度区域中,在区域T 内有s 个点x 被归为低权重系数组,该点集的空间信息集为P ∈R N s ×3㊂点集的局部特征集为F ∈R N s ×D f [14],其中D f 表示特征的维度空间,N s 表示s 个域内点的集合㊂设p i 以及f i 为点x i 的空间信息和特征信息㊂在点集内,对点x i 进行小范围内的N 邻域搜索,搜索其邻域点㊂则点x i 的邻域点{x i ,1,x i ,2, ,x i ,N }∈N (x i ),其特征集合为{f i ,1,f i ,2, ,f i ,N }∈F ㊂在利用空间尺度进行区域划分后,对空间尺度区域特征s mt 较低的区域进行区域内特征更新,通过聚合函数对权重最低的邻域点在图中的局部特征进行改写㊂已知中心点m ,点x i 的特征f mx i 和空间尺度区域特征s mt ,目的是求出f ′mx i ,即中心点m 的低权重邻域点x i 在进行邻域特征更新后得到的新特征㊂对区域T 内的点x i ,∀x i ,j ∈H (x i ),x i 与其邻域H 内的邻域点的特征相似性域为R (x i ,x i ,j )=S [C (f i ,j )T C (f i ,j )/D o ],(4)其中C 表示由输入至输出维度的一维卷积,D o 表示输出维度值,T 表示转置㊂从而获得更新后的x i 的426吉林大学学报(信息科学版)第41卷特征㊂对R (x i ,x i ,j )进行聚合,并将特征f mx i 维度变换为输出维度f ′mx i =∑[R (x i ,x i ,j )S (s mt f mx i )]㊂(5) 图4为特征更新网络模块示意图,展示了上述特征更新的计算过程㊂图5为特征更新的动态图卷积网络示意图㊂图4 特征更新网络模块示意图Fig.4 Schematic diagram of feature update network module 图5 特征更新的动态图卷积网络示意图Fig.5 Flow chart of dynamic graph convolution network with feature update 动态图卷积网络(DGCNN)利用自创的边缘卷积层模块,逐层进行边卷积[15]㊂其前一层的输出都会动态地产生新的特征空间和局部区域,新一层从前一层学习特征(见图5)㊂在每层的边卷积模块中,笔者在边卷积和池化后加入了空间尺度区域注意力特征,捕捉特定空间区域T 内的邻域点,用于特征更新㊂特征更新会降低局域异常值点对局部特征的污染㊂网络相比传统图卷积神经网络能获得更多的特征信息,并且在面对拥有较多噪声值的点云数据时,具有更好的抗干扰性[16],在对性质不稳定㊁不平滑并含有需采集分割的突出中心的点云数据时,会有更好的抗干扰效果㊂相比于传统预处理方式,其稳定性更强,不会发生将突出部分误分割或漏分割的现象[17]㊂2 实验结果与分析点云分割的精度评估指标主要由两组数据构成[18],即平均交并比和总体准确率㊂平均交并比U (MIoU:Mean Intersection over Union)代表真实值和预测值合集的交并化率的平均值,其计算式为526第4期张闻锐,等:特征更新的动态图卷积表面损伤点云分割方法U =1T +1∑Ta =0p aa ∑Tb =0p ab +∑T b =0p ba -p aa ,(6)其中T 表示类别,a 表示真实值,b 表示预测值,p ab 表示将a 预测为b ㊂总体准确率A (OA:Overall Accuracy)表示所有正确预测点p c 占点云模型总体数量p all 的比,其计算式为A =P c /P all ,(7)其中U 与A 数值越大,表明点云分割网络越精准,且有U ≤A ㊂2.1 实验准备与数据预处理实验使用Kinect V2,采用Depth Basics⁃WPF 模块拍摄金属部件损伤表面获得深度图,将获得的深度图进行SDK(Software Development Kit)转化,得到pcd 格式的点云数据㊂Kinect V2采集的深度图像分辨率固定为512×424像素,为获得更清晰的数据图像,需尽可能近地采集数据㊂选择0.6~1.2m 作为采集距离范围,从0.6m 开始每次增加0.2m,获得多组采量数据㊂点云中分布着噪声,如果不对点云数据进行过滤会对后续处理产生不利影响㊂根据统计原理对点云中每个点的邻域进行分析,再建立一个特别设立的标准差㊂然后将实际点云的分布与假设的高斯分布进行对比,实际点云中误差超出了标准差的点即被认为是噪声点[19]㊂由于点云数据量庞大,为提高效率,选择采用如下改进方法㊂计算点云中每个点与其首个邻域点的空间距离L 1和与其第k 个邻域点的空间距离L k ㊂比较每个点之间L 1与L k 的差,将其中差值最大的1/K 视为可能噪声点[20]㊂计算可能噪声点到其K 个邻域点的平均值,平均值高出标准差的被视为噪声点,将离群噪声点剔除后完成对点云的滤波㊂2.2 金属表面损伤点云关键信息提取分割方法对点云损伤分割,在制作点云数据训练集时,如果只是单一地将所有损伤进行统一标记,不仅不方便进行结果分析和应用,而且也会降低特征分割的效果㊂为方便分析和控制分割效果,需要使用ArcGIS 将点云模型转化为不规则三角网TIN(Triangulated Irregular Network)㊂为精确地分类损伤,利用图6 不规则三角网模型示意图Fig.6 Schematic diagram of triangulated irregular networkTIN 的表面轮廓性质,获得训练数据损伤点云的损伤内(外)体积,损伤表面轮廓面积等㊂如图6所示㊂选择损伤体积指标分为相对损伤体积V (RDV:Relative Damege Volume)和邻域内相对损伤体积比N (NRDVR:Neighborhood Relative Damege Volume Ratio)㊂计算相对平均深度平面与点云深度网格化平面之间的部分,得出相对损伤体积㊂利用TIN 邻域网格可获取某损伤在邻域内的相对深度占比,有效解决制作测试集时,将因弧度或是形状造成的相对深度判断为损伤的问题㊂两种指标如下:V =∑P d k =1h k /P d -∑P k =1h k /()P S d ,(8)N =P n ∑P d k =1h k S d /P d ∑P n k =1h k S ()n -()1×100%,(9)其中P 表示所有点云数,P d 表示所有被标记为损伤的点云数,P n 表示所有被认定为损伤邻域内的点云数;h k 表示点k 的深度值;S d 表示损伤平面面积,S n 表示损伤邻域平面面积㊂在获取TIN 标准包络网视图后,可以更加清晰地描绘损伤情况,同时有助于量化损伤严重程度㊂笔者将损伤分为6种类型,并利用计算得出的TIN 指标进行损伤分类㊂同时,根据损伤部分体积与非损伤部分体积的关系,制定指标损伤体积(SDV:Standard Damege Volume)区分损伤类别㊂随机抽选5个测试组共50张图作为样本㊂统计非穿透损伤的RDV 绝对值,其中最大的30%标记为凹陷或凸起,其余626吉林大学学报(信息科学版)第41卷标记为表面损伤,并将样本分类的标准分界值设为SDV㊂在设立以上标准后,对凹陷㊁凸起㊁穿孔㊁表面损伤㊁破损和缺损6种金属表面损伤进行分类,金属表面损伤示意图如图7所示㊂首先,根据损伤是否产生洞穿,将损伤分为两大类㊂非贯通伤包括凹陷㊁凸起和表面损伤,贯通伤包括穿孔㊁破损和缺损㊂在非贯通伤中,凹陷和凸起分别采用相反数的SDV 作为标准,在这之间的被分类为表面损伤㊂贯通伤中,以损伤部分平面面积作为参照,较小的分类为穿孔,较大的分类为破损,而在边缘处因腐蚀㊁碰撞等原因缺角㊁内损的分类为缺损㊂分类参照如表1所示㊂图7 金属表面损伤示意图Fig.7 Schematic diagram of metal surface damage表1 损伤类别分类Tab.1 Damage classification 损伤类别凹陷凸起穿孔表面损伤破损缺损是否形成洞穿××√×√√RDV 绝对值是否达到SDV √√\×\\S d 是否达到标准\\×\√\2.3 实验结果分析为验证改进的图卷积深度神经网络在点云语义分割上的有效性,笔者采用TensorFlow 神经网络框架进行模型测试㊂为验证深度网络对损伤分割的识别准确率,采集了带有损伤特征的金属部件损伤表面点云,对点云进行预处理㊂对若干金属部件上的多个样本金属面的点云数据进行筛选,删除损伤占比低于5%或高于60%的数据后,划分并装包制作为点云数据集㊂采用CloudCompare 软件对样本金属上的损伤部分进行分类标记,共分为6种如上所述损伤㊂部件损伤的数据集制作参考点云深度学习领域广泛应用的公开数据集ModelNet40part㊂分割数据集包含了多种类型的金属部件损伤数据,这些损伤数据显示在510张总点云图像数据中㊂点云图像种类丰富,由各种包含损伤的金属表面构成,例如金属门,金属蒙皮,机械构件外表面等㊂用ArcGIS 内相关工具将总图进行随机点拆分,根据数据集ModelNet40part 的规格,每个独立的点云数据组含有1024个点,将所有总图拆分为510×128个单元点云㊂将样本分为400个训练集与110个测试集,采用交叉验证方法以保证测试的充分性[20],对多种方法进行评估测试,实验结果由单元点云按原点位置重新组合而成,并带有拆分后对单元点云进行的分割标记㊂分割结果比较如图8所示㊂726第4期张闻锐,等:特征更新的动态图卷积表面损伤点云分割方法图8 分割结果比较图Fig.8 Comparison of segmentation results在部件损伤分割的实验中,将不同网络与笔者网络(FAS⁃DGCNN:Feature Adaptive Shifting⁃Dynamic Graph Convolutional Neural Networks)进行对比㊂除了采用不同的分割网络外,其余实验均采用与改进的图卷积深度神经网络方法相同的实验设置㊂实验结果由单一损伤交并比(IoU:Intersection over Union),平均损伤交并比(MIoU),单一损伤准确率(Accuracy)和总体损伤准确率(OA)进行评价,结果如表2~表4所示㊂将6种不同损伤类别的Accuracy 与IoU 进行对比分析,可得出结论:相比于基准实验网络Pointet++,笔者在OA 和MioU 方面分别在贯通伤和非贯通伤上有10%和20%左右的提升,在整体分割指标上,OA 能达到90.8%㊂对拥有更多点数支撑,含有较多点云特征的非贯通伤,几种点云分割网络整体性能均能达到90%左右的效果㊂而不具有局部特征识别能力的PointNet 在贯通伤上的表现较差,不具备有效的分辨能力,导致分割效果相对于其他损伤较差㊂表2 损伤部件分割准确率性能对比 Tab.2 Performance comparison of segmentation accuracy of damaged parts %实验方法准确率凹陷⁃1凸起⁃2穿孔⁃3表面损伤⁃4破损⁃5缺损⁃6Ponitnet 82.785.073.880.971.670.1Pointnet++88.786.982.783.486.382.9DGCNN 90.488.891.788.788.687.1FAS⁃DGCNN 92.588.892.191.490.188.6826吉林大学学报(信息科学版)第41卷表3 损伤部件分割交并比性能对比 Tab.3 Performance comparison of segmentation intersection ratio of damaged parts %IoU 准确率凹陷⁃1凸起⁃2穿孔⁃3表面损伤⁃4破损⁃5缺损⁃6PonitNet80.582.770.876.667.366.9PointNet++86.384.580.481.184.280.9DGCNN 88.786.589.986.486.284.7FAS⁃DGCNN89.986.590.388.187.385.7表4 损伤分割的整体性能对比分析 出,动态卷积图特征以及有效的邻域特征更新与多尺度注意力给分割网络带来了更优秀的局部邻域分割能力,更加适应表面损伤分割的任务要求㊂3 结 语笔者利用三维点云独特的空间结构特征,将传统K 邻域内权重相近的邻域点采用空间尺度进行区分,并将空间尺度划分运用于邻域内权重分配上,提出了一种能将邻域内噪声点降权筛除的特征更新模块㊂采用此模块的动态图卷积网络在分割上表现出色㊂利用特征更新的动态图卷积网络(FAS⁃DGCNN)能有效实现金属表面损伤的分割㊂与其他网络相比,笔者方法在点云语义分割方面表现出更高的可靠性,可见在包含空间尺度区域信息的注意力和局域点云特征更新下,笔者提出的基于特征更新的动态图卷积网络能发挥更优秀的作用,而且相比缺乏局部特征提取能力的分割网络,其对于点云稀疏㊁特征不明显的非贯通伤有更优的效果㊂参考文献:[1]LAWIN F J,DANELLJAN M,TOSTEBERG P,et al.Deep Projective 3D Semantic Segmentation [C]∥InternationalConference on Computer Analysis of Images and Patterns.Ystad,Sweden:Springer,2017:95⁃107.[2]MATURANA D,SCHERER S.VoxNet:A 3D Convolutional Neural Network for Real⁃Time Object Recognition [C]∥Proceedings of IEEE /RSJ International Conference on Intelligent Robots and Systems.Hamburg,Germany:IEEE,2015:922⁃928.[3]LE T,DUAN Y.PointGrid:A Deep Network for 3D Shape Understanding [C]∥2018IEEE /CVF Conference on ComputerVision and Pattern Recognition (CVPR).Salt Lake City,USA:IEEE,2018:9204⁃9214.[4]QI C R,SU H,MO K,et al.PointNet:Deep Learning on Point Sets for 3D Classification and Segmentation [C]∥IEEEConference on Computer Vision and Pattern Recognition (CVPR).Hawaii,USA:IEEE,2017:652⁃660.[5]QI C R,SU H,MO K,et al,PointNet ++:Deep Hierarchical Feature Learning on Point Sets in a Metric Space [C]∥Advances in Neural Information Processing Systems.California,USA:SpringerLink,2017:5099⁃5108.[6]HU J,SHEN L,SUN G,Squeeze⁃and⁃Excitation Networks [C ]∥IEEE Conference on Computer Vision and PatternRecognition.Vancouver,Canada:IEEE,2018:7132⁃7141.[7]LI Y,BU R,SUN M,et al.PointCNN:Convolution on X⁃Transformed Points [C]∥Advances in Neural InformationProcessing Systems.Montreal,Canada:NeurIPS,2018:820⁃830.[8]ANH VIET PHAN,MINH LE NGUYEN,YEN LAM HOANG NGUYEN,et al.DGCNN:A Convolutional Neural Networkover Large⁃Scale Labeled Graphs [J].Neural Networks,2018,108(10):533⁃543.[9]任伟建,高梦宇,高铭泽,等.基于混合算法的点云配准方法研究[J].吉林大学学报(信息科学版),2019,37(4):408⁃416.926第4期张闻锐,等:特征更新的动态图卷积表面损伤点云分割方法036吉林大学学报(信息科学版)第41卷REN W J,GAO M Y,GAO M Z,et al.Research on Point Cloud Registration Method Based on Hybrid Algorithm[J]. Journal of Jilin University(Information Science Edition),2019,37(4):408⁃416.[10]ZHANG K,HAO M,WANG J,et al.Linked Dynamic Graph CNN:Learning on Point Cloud via Linking Hierarchical Features[EB/OL].[2022⁃03⁃15].https:∥/stamp/stamp.jsp?tp=&arnumber=9665104. [11]林少丹,冯晨,陈志德,等.一种高效的车体表面损伤检测分割算法[J].数据采集与处理,2021,36(2):260⁃269. LIN S D,FENG C,CHEN Z D,et al.An Efficient Segmentation Algorithm for Vehicle Body Surface Damage Detection[J]. Journal of Data Acquisition and Processing,2021,36(2):260⁃269.[12]ZHANG L P,ZHANG Y,CHEN Z Z,et al.Splitting and Merging Based Multi⁃Model Fitting for Point Cloud Segmentation [J].Journal of Geodesy and Geoinformation Science,2019,2(2):78⁃79.[13]XING Z Z,ZHAO S F,GUO W,et al.Processing Laser Point Cloud in Fully Mechanized Mining Face Based on DGCNN[J]. ISPRS International Journal of Geo⁃Information,2021,10(7):482⁃482.[14]杨军,党吉圣.基于上下文注意力CNN的三维点云语义分割[J].通信学报,2020,41(7):195⁃203. YANG J,DANG J S.Semantic Segmentation of3D Point Cloud Based on Contextual Attention CNN[J].Journal on Communications,2020,41(7):195⁃203.[15]陈玲,王浩云,肖海鸿,等.利用FL⁃DGCNN模型估测绿萝叶片外部表型参数[J].农业工程学报,2021,37(13): 172⁃179.CHEN L,WANG H Y,XIAO H H,et al.Estimation of External Phenotypic Parameters of Bunting Leaves Using FL⁃DGCNN Model[J].Transactions of the Chinese Society of Agricultural Engineering,2021,37(13):172⁃179.[16]柴玉晶,马杰,刘红.用于点云语义分割的深度图注意力卷积网络[J].激光与光电子学进展,2021,58(12):35⁃60. CHAI Y J,MA J,LIU H.Deep Graph Attention Convolution Network for Point Cloud Semantic Segmentation[J].Laser and Optoelectronics Progress,2021,58(12):35⁃60.[17]张学典,方慧.BTDGCNN:面向三维点云拓扑结构的BallTree动态图卷积神经网络[J].小型微型计算机系统,2021, 42(11):32⁃40.ZHANG X D,FANG H.BTDGCNN:BallTree Dynamic Graph Convolution Neural Network for3D Point Cloud Topology[J]. Journal of Chinese Computer Systems,2021,42(11):32⁃40.[18]张佳颖,赵晓丽,陈正.基于深度学习的点云语义分割综述[J].激光与光电子学,2020,57(4):28⁃46. ZHANG J Y,ZHAO X L,CHEN Z.A Survey of Point Cloud Semantic Segmentation Based on Deep Learning[J].Lasers and Photonics,2020,57(4):28⁃46.[19]SUN Y,ZHANG S H,WANG T Q,et al.An Improved Spatial Point Cloud Simplification Algorithm[J].Neural Computing and Applications,2021,34(15):12345⁃12359.[20]高福顺,张鼎林,梁学章.由点云数据生成三角网络曲面的区域增长算法[J].吉林大学学报(理学版),2008,46 (3):413⁃417.GAO F S,ZHANG D L,LIANG X Z.A Region Growing Algorithm for Triangular Network Surface Generation from Point Cloud Data[J].Journal of Jilin University(Science Edition),2008,46(3):413⁃417.(责任编辑:刘俏亮)。
学术英语习题及部分答案
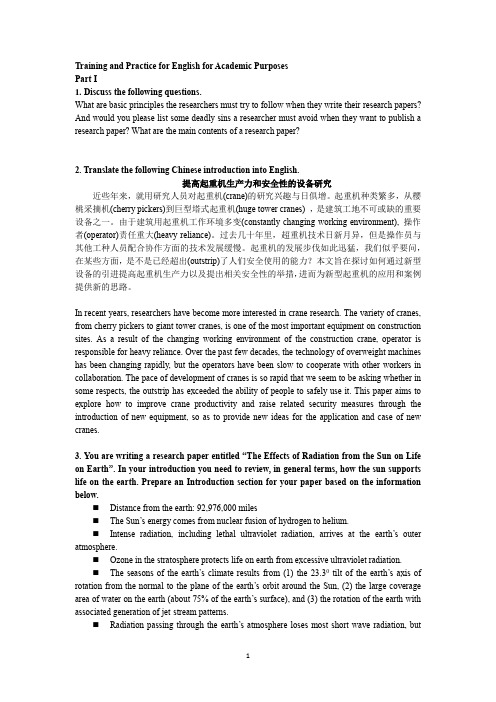
Training and Practice for English for Academic PurposesPart I1.Discuss the following questions.What are basic principles the researchers must try to follow when they write their research papers? And would you please list some deadly sins a researcher must avoid when they want to publish a research paper? What are the main contents of a research paper?2. Translate the following Chinese introduction into English.提高起重机生产力和安全性的设备研究近些年来,就用研究人员对起重机(crane)的研究兴趣与日俱增。
起重机种类繁多,从樱桃采摘机(cherry pickers)到巨型塔式起重机(huge tower cranes) ,是建筑工地不可或缺的重要设备之一。
由于建筑用起重机工作环境多变(constantly changing working environment), 操作者(operator)责任重大(heavy reliance)。
过去几十年里,超重机技术日新月异,但是操作员与其他工种人员配合协作方面的技术发展缓慢。
起重机的发展步伐如此迅猛,我们似乎要问,在某些方面,是不是已经超出(outstrip)了人们安全使用的能力?本文旨在探讨如何通过新型设备的引进提高起重机生产力以及提出相关安全性的举措,进而为新型起重机的应用和案例提供新的思路。
In recent years, researchers have become more interested in crane research.The variety of cranes, from cherry pickers to giant tower cranes, is one of the most important equipment on construction sites.As a result of the changing working environment of the construction crane, operator is responsible for heavy reliance.Over the past few decades, the technology of overweight machines has been changing rapidly, but the operators have been slow to cooperate with other workers in collaboration.The pace of development of cranes is so rapid that we seem to be asking whether in some respects, the outstrip has exceeded the ability of people to safely use it.This paper aims to explore how to improve crane productivity and raise related security measures through the introduction of new equipment, so as to provide new ideas for the application and case of new cranes.3. You are writing a research paper entitled “The Effects of Radiation from the Sun on Life o n Earth”. In your introduction you need to review, in general terms, how the sun supports life on the earth. Prepare an Introduction section for your paper based on the information below.⏹Distance from the earth: 92,976,000 miles⏹The Sun’s energy comes from nuclear fusion of hydrogen to helium.⏹Intense radiation, including lethal ultraviolet radiation, arrives at the earth’s outer atmosphere.⏹Ozone in the stratosphere protects life on earth from excessive ultraviolet radiation.⏹The seasons of the earth’s climate results from (1) the 23.30tilt of the earth’s axis of rotation from the normal to the plane of the earth’s orbit around the Sun, (2) the large coverage area of water on the earth (about 75% of the earth’s surface), an d (3) the rotation of the earth with associated generation of jet-stream patterns.⏹Radiation passing through the earth’s atmosphere loses most short-wave radiation, butsome arriving at the surface is converted into infrared radiation which is then trapped by water vapor and other tri-atomic molecules in the troposphere and stratosphere, causing global warming.Life on earth is maintained from photosynthesis and conversion of carbon dioxide to oxygen by plants.4.Translate the following parts of sentences in Introduction into proper English.(1)过去对……的研究工作说明……The previous work on … has indicated that…(2)A在1932年做了关于……的早期研究。
C反应蛋白检测与临床诊断思路

(b) Ribbon diagram of the crystal structure, showing the lectin fold and the two calcium atoms (spheres) in the ligand-binding site of each protomer .
CRP--急性时相反应的一个极灵敏的指标
各种组织损伤
急性心肌梗死
栓塞
创伤
感染
炎症
P
外科手术 肿瘤浸润
R
风湿病
C
炎症:
6~12h可检测升高
18~24h达到高峰
可达正常水平的数十 至数百倍,甚至2000 倍。
CRP
是炎症或组织损
伤的非特异性标 志物
CRP--急性时相反应的一个极灵敏的指标
半衰期短,5~7h,达峰值后,以每 天近50%的速度迅速趋向正常,随着 病情的缓解,病变消退、组织结构和 功能的恢复,其浓度可迅速降至正常 水平,与病情变化同步且与病情的严 重程度具有相关性。
CRP变化的临床思路
9.良恶性胸腔积液的鉴别诊断
炎性胸腔积液CRP水平>结核性胸腔积液 CRP水平>恶性胸腔积液CRP水平
血、胸液CRP< 5.0mg/L见于癌性胸腔积液不 支持结核性胸腔积液
结核性胸液患者血/胸液CRP< 1 癌性胸液患者血/胸液CRP基本上≥1
CRP变化的临床思路
10.免疫性疾病的判断
detection limit探测极限
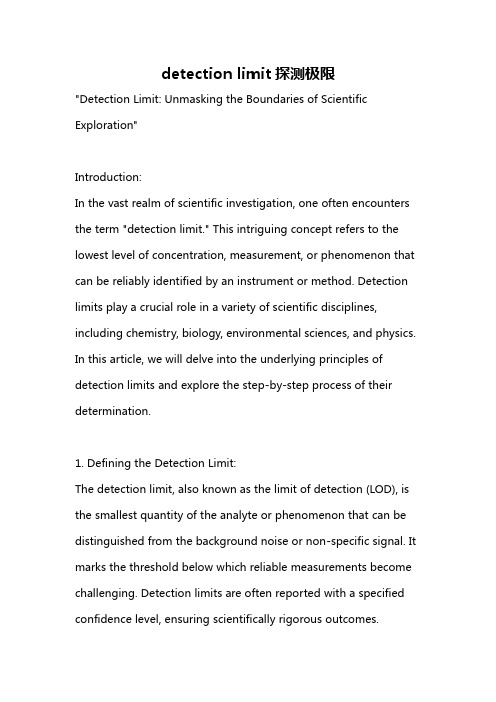
detection limit探测极限"Detection Limit: Unmasking the Boundaries of Scientific Exploration"Introduction:In the vast realm of scientific investigation, one often encounters the term "detection limit." This intriguing concept refers to the lowest level of concentration, measurement, or phenomenon that can be reliably identified by an instrument or method. Detection limits play a crucial role in a variety of scientific disciplines, including chemistry, biology, environmental sciences, and physics. In this article, we will delve into the underlying principles of detection limits and explore the step-by-step process of their determination.1. Defining the Detection Limit:The detection limit, also known as the limit of detection (LOD), is the smallest quantity of the analyte or phenomenon that can be distinguished from the background noise or non-specific signal. It marks the threshold below which reliable measurements become challenging. Detection limits are often reported with a specified confidence level, ensuring scientifically rigorous outcomes.2. Significance of Detection Limits:Accurate determination of detection limits is paramount in various scientific applications. From environmental monitoring to pharmaceutical research, detection limits allow scientists to differentiate between trace amounts of substances, quantitatively analyze analytes, and set boundaries for safety regulations. These limits aid in tracking pollution levels, identifying disease markers, ensuring product quality, and much more.3. Factors Affecting Detection Limits:Several key factors directly impact the determination and improvement of detection limits. These factors include instrument specifications, background noise levels, analyte concentration, sample matrix, detection technique, and data analysis methods. Understanding these factors and their interactions is vital for optimizing detection limits.4. Methodology for Determining Detection Limits:The process of determining detection limits involves a systematic approach to quantify the lowest detectable concentration or value. Here is a step-by-step exploration of this methodology:a. Selecting the Analyte and Background Matrix:The first step is to identify the analyte of interest and the medium in which it is present. Different analytes and matrices impose varying challenges and may require specific approaches for successful detection.b. Instrument Calibration:Calibrating the instrument or method to be used is essential before determining the detection limit. This includes obtaining a range of known standards with concentrations both above and below the expected detection limit. By analyzing these calibration standards, a calibration curve can be constructed.c. Determining the Signal-to-Noise Ratio:The signal-to-noise ratio (S/N) is calculated by comparing the measured signal from the analyte to the background noise level. A higher S/N ratio indicates a more favorable signal strength relative to noise, hence lowering the detection limit.d. Performing Replicate Measurements:To ensure robustness and reliability, multiple replicatemeasurements at different analyte concentrations or values should be performed. This allows for statistical analysis, enhancing the accuracy of the detection limit determination.e. Statistical Analysis:Using statistical tools, such as regression analysis or hypothesis testing, the obtained measurements and their uncertainties are analyzed. These statistical calculations aid in establishing rigorous detection limits with appropriate confidence levels.5. Improving Detection Limits:Once the detection limit is determined, researchers strive to lower it to enhance the efficiency and sensitivity of their methods. Strategies for improving detection limits include optimizing sample preparation techniques, reducing background noise, enhancing signal amplification, employing advanced instruments, and utilizing cutting-edge data analysis techniques.Conclusion:Detection limits are essential boundaries that enable scientists to explore the minute details of their subjects of interest. Byunderstanding the underlying principles and employing astep-by-step methodology for their determination, researchers continue to unravel the mysteries of the natural world and contribute to scientific progress. The pursuit of lower detection limits challenges scientists to refine their techniques, pushing the boundaries of scientific exploration further with each breakthrough.。
壳层结构 英文缩写

壳层结构英文缩写The Layered Structure of ShellsThe natural world is filled with a diverse array of structures, each uniquely adapted to serve the needs of the organisms that inhabit them. One particularly fascinating example of this is the layered structure of shells found in various marine and terrestrial species. These intricate and often visually striking formations serve a multitude of essential functions, from protection against predators to the facilitation of essential biological processes.At the core of these shells lies a fundamental structural design known as the layered or laminated structure. This architecture is characterized by the presence of multiple distinct layers, each with its own unique properties and functions. The outermost layer, often referred to as the periostracum, is typically a thin, tough, and durable material that serves as the primary line of defense against physical and environmental stresses.Beneath the periostracum lies a layer known as the prismatic layer, which is composed of tightly packed, elongated crystals of calcium carbonate. These crystals are arranged in a highly organized andefficient manner, providing the shell with remarkable strength and resistance to fracture. The prismatic layer is often responsible for the distinctive patterns and textures observed on the surface of many shells, adding to their visual appeal and serving as a means of species identification.The innermost layer of the shell is known as the nacreous layer, or "mother-of-pearl." This layer is composed of alternating sheets of calcium carbonate and a protein-based material called conchiolin. The intricate arrangement of these materials results in a highly reflective and iridescent surface, which can range in color from brilliant white to a stunning array of hues, including blues, greens, and purples.The layered structure of shells serves a multitude of essential functions for the organisms that produce them. The outer periostracum layer provides protection against physical damage, abrasion, and the corrosive effects of the surrounding environment. The prismatic layer, with its remarkable strength and durability, helps to shield the organism from predators and the stresses of their habitat. The innermost nacreous layer, with its unique optical properties, can play a role in camouflage, signaling, and even the regulation of light and heat within the organism's body.In addition to these functional benefits, the layered structure ofshells also holds significant importance in the realms of science and technology. The study of these intricate structures has provided valuable insights into the principles of biomineralization, the process by which living organisms produce inorganic materials like calcium carbonate. This knowledge has led to the development of innovative materials and technologies, ranging from advanced ceramics to self-healing coatings and protective surfaces.Moreover, the layered structure of shells has also been a source of inspiration for engineers and designers, who have sought to mimic the efficiency and resilience of these natural structures in the creation of new materials and products. The concept of biomimicry, or the imitation of nature's designs, has become an increasingly important approach in fields such as materials science, architecture, and engineering.In conclusion, the layered structure of shells is a remarkable example of the ingenious design and adaptations found in the natural world. These intricate formations serve a multitude of essential functions for the organisms that produce them, while also providing valuable insights and inspiration for scientific and technological advancements. As we continue to explore and understand the complexity of these natural structures, we may unlock new possibilities for innovation and discovery, further expanding our knowledge and appreciation of the wonders of the natural world.。
基于深度残差网络和近红外光谱的煤矸石智能识别
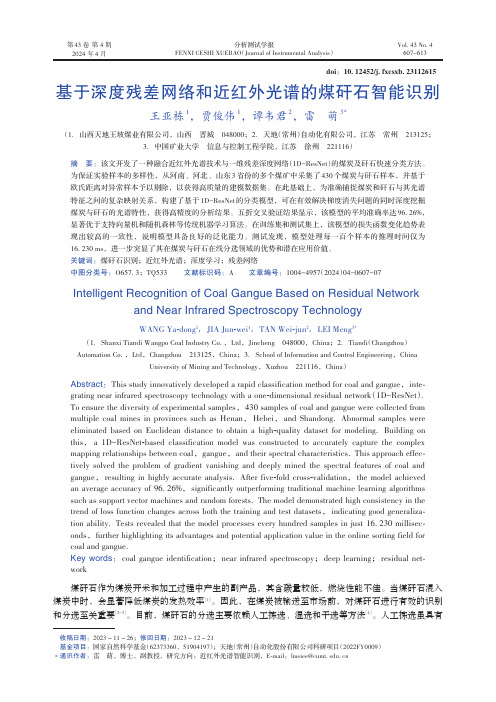
第43 卷第 4 期2024 年4 月Vol.43 No.4607~613分析测试学报FENXI CESHI XUEBAO(Journal of Instrumental Analysis)基于深度残差网络和近红外光谱的煤矸石智能识别王亚栋1,贾俊伟1,谭韦君2,雷萌3*(1.山西天地王坡煤业有限公司,山西晋城 048000;2.天地(常州)自动化有限公司,江苏常州213125;3.中国矿业大学 信息与控制工程学院,江苏徐州221116)摘要:该文开发了一种融合近红外光谱技术与一维残差深度网络(1D-ResNet)的煤炭及矸石快速分类方法。
为保证实验样本的多样性,从河南、河北、山东3省份的多个煤矿中采集了430个煤炭与矸石样本,并基于欧氏距离对异常样本予以剔除,以获得高质量的建模数据集。
在此基础上,为准确捕捉煤炭和矸石与其光谱特征之间的复杂映射关系,构建了基于1D-ResNet的分类模型,可在有效解决梯度消失问题的同时深度挖掘煤炭与矸石的光谱特性,获得高精度的分析结果。
五折交叉验证结果显示,该模型的平均准确率达96.26%,显著优于支持向量机和随机森林等传统机器学习算法。
在训练集和测试集上,该模型的损失函数变化趋势表现出较高的一致性,说明模型具备良好的泛化能力。
测试发现,模型处理每一百个样本的推理时间仅为16.230 ms,进一步突显了其在煤炭与矸石在线分选领域的优势和潜在应用价值。
关键词:煤矸石识别;近红外光谱;深度学习;残差网络中图分类号:O657.3;TQ533文献标识码:A 文章编号:1004-4957(2024)04-0607-07Intelligent Recognition of Coal Gangue Based on Residual Networkand Near Infrared Spectroscopy TechnologyWANG Ya-dong1,JIA Jun-wei1,TAN Wei-jun2,LEI Meng3*(1.Shanxi Tiandi Wangpo Coal Industry Co.,Ltd,Jincheng 048000,China;2.Tiandi(Changzhou)Automation Co.,Ltd,Changzhou 213125,China;3.School of Information and Control Engineering,ChinaUniversity of Mining and Technology,Xuzhou 221116,China)Abstract:This study innovatively developed a rapid classification method for coal and gangue,inte⁃grating near infrared spectroscopy technology with a one-dimensional residual network(1D-ResNet). To ensure the diversity of experimental samples,430 samples of coal and gangue were collected from multiple coal mines in provinces such as Henan,Hebei,and Shandong.Abnormal samples were eliminated based on Euclidean distance to obtain a high-quality dataset for modeling.Building on this,a 1D-ResNet-based classification model was constructed to accurately capture the complex mapping relationships between coal,gangue,and their spectral characteristics. This approach effec⁃tively solved the problem of gradient vanishing and deeply mined the spectral features of coal and gangue,resulting in highly accurate analysis.After five-fold cross-validation,the model achieved an average accuracy of 96.26%,significantly outperforming traditional machine learning algorithms such as support vector machines and random forests. The model demonstrated high consistency in the trend of loss function changes across both the training and test datasets,indicating good generaliza⁃tion ability. Tests revealed that the model processes every hundred samples in just 16.230 millisec⁃onds,further highlighting its advantages and potential application value in the online sorting field for coal and gangue.Key words:coal gangue identification;near infrared spectroscopy;deep learning;residual net⁃work煤矸石作为煤炭开采和加工过程中产生的副产品,其含碳量较低,燃烧性能不佳。
基于高光谱技术的现场车漆物证识别模型研究

第42 卷第 7 期2023 年7 月Vol.42 No.7817~824分析测试学报FENXI CESHI XUEBAO(Journal of Instrumental Analysis)基于高光谱技术的现场车漆物证识别模型研究张浩,高树辉*(中国人民公安大学侦查学院,北京100038)摘要:该文基于光谱检测可无损成像、操作简单的优点,探索了高光谱成像技术结合深度残差收缩网络识别现场车漆物证的方法。
以现场常见的白色车漆碎片为研究对象,采集了18种不同车型共54个车漆样本的高光谱图像,对图像进行10 × 10像素融合,形成19 740个像素的反射光谱。
结合高光谱数据的特点,建立了针对性的一维深度残差收缩网络(1D-DRSN)识别模型。
实验结果表明,该模型在训练集和测试集上的准确率分别为99.5%和98.6%,损失函数值分别下降到0.093和0.106收敛。
相比一维卷积神经网络(1D-CNN)模型和支持向量机(SVM)、随机森林(RF)、逻辑回归(LR)3种传统的机器学习方法,该模型的分类效果和精度明显提高。
通过对高光谱数据的深度挖掘区分了来自不同品牌或型号的白色车漆样本。
该研究解决了实战难题,是现场车身油漆碎片物证检验技术和方法的有力补充。
关键词:高光谱成像技术;深度残差收缩网络;机器学习;现场车漆物证;智能识别中图分类号:O657.3;O436文献标识码:A 文章编号:1004-4957(2023)07-0817-08Research on Identification Model for Car Paint Material EvidenceBased on Hyperspectral TechnologyZHANG Hao,GAO Shu-hui*(School of Investigation,People’s Public Security University of China,Beijing 100038,China)Abstract:Vehicle’s paint chips are one of the common physical evidences at the scene of criminal cas⁃es and traffic accidents. Identification and recognition of such physical evidences have been an impor⁃tant part of the research of public security technicians as they might contain some important information about the vehicles involved.Traditional instrumental analysis methods are cumbersome and cannot meet the demand for rapid nondestructive testing of physical evidence at the scene.Based on the advan⁃tages of nondestructive imaging and simple operation of spectral detection,a hyperspectral imaging technology combined with deep residual shrinkage network was explored to identify on-site car paint evi⁃dence,in order to improve detection efficiency and optimize detection means.The hyperspectral imag⁃es of 54 car paint samples from 18 different car models were collected,and the images were fused with 10 × 10 pixels to form a reflection spectrum of 19 740 pixels.Combined with the characteristics of hy⁃perspectral data,a targeted one-dimensional deep residual shrinkage network(1D-DRSN) recognition model was established in this paper.The results showed that 1D-DRSN model has 99.5% and 98.6% accuracy for the training and test sets,with the loss function values decreasing to 0.093 and 0.106,respectively.1D-DRSN was significantly better than 1D convolutional neural network(1D-CNN) model and three traditional machine learning methods,including support vector machine(SVM),random for⁃est(RF) and logistic regression(LR).The white car paint samples from different brands or models are distinguished by deep mining of hyperspectral data.This study was applied to solving the practical challenges,and it will be a powerful complement to the existing techniques and methods in the detec⁃tion and classification of vehicle’s paint fragments from the scene.Key words:hyperspectral imaging technique;deep residual shrinkage network;machine learning;car paint evidence from the scene;intelligent identification在刑事案件和交通事故现场勘查中,常能发现汽车车身油漆碎片等物证,这些物证携带有涉事车辆的相关信息,是法庭科学物证检验的重要内容之一。
利用地震P波确定煤层瓦斯富集带的分布
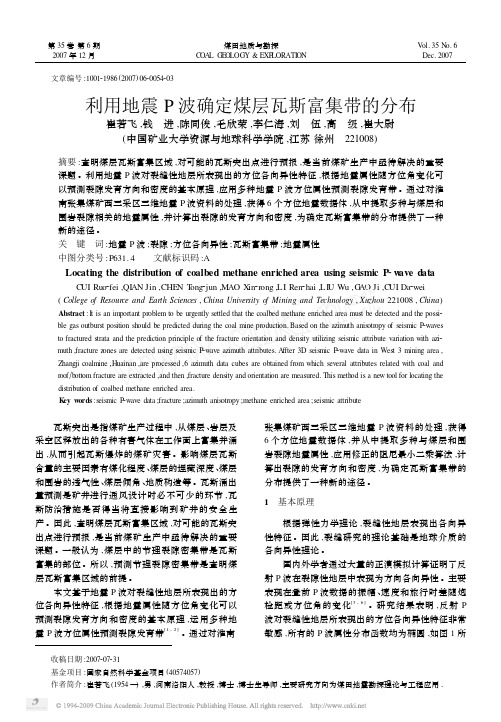
文章编号:100121986(2007)0620054203利用地震P 波确定煤层瓦斯富集带的分布崔若飞,钱 进,陈同俊,毛欣荣,李仁海,刘 伍,高 级,崔大尉(中国矿业大学资源与地球科学学院,江苏徐州 221008)摘要:查明煤层瓦斯富集区域,对可能的瓦斯突出点进行预报,是当前煤矿生产中亟待解决的重要课题。
利用地震P 波对裂缝性地层所表现出的方位各向异性特征,根据地震属性随方位角变化可以预测裂隙发育方向和密度的基本原理,应用多种地震P 波方位属性预测裂隙发育带。
通过对淮南张集煤矿西三采区三维地震P 波资料的处理,获得6个方位地震数据体,从中提取多种与煤层和围岩裂隙相关的地震属性,并计算出裂隙的发育方向和密度,为确定瓦斯富集带的分布提供了一种新的途径。
关 键 词:地震P 波;裂隙;方位各向异性;瓦斯富集带;地震属性中图分类号:P631.4 文献标识码:ALocating the distribution of coalbed methane enriched area using seismic P 2w ave dataC UI Ruo 2fei ,QI AN Jin ,CHE N T ong 2jun ,MAO X in 2rong ,LI Ren 2hai ,LI U Wu ,G AO Ji ,C UI Da 2wei (College o f Resource and Earth Sciences ,China Univer sity o f Mining and Technology ,Xuzhou 221008,China )Abstract :I t is an im portant problem to be urgently settled that the coalbed methane enriched area must be detected and the possi 2ble gas outburst position should be predicted during the coal mine production.Based on the azimuth anis otropy of seismic P 2waves to fractured strata and the prediction principle of the fracture orientation and density utilizing seismic attribute variation with azi 2muth ,fracture zones are detected using seismic P 2wave azimuth attributes.A ffter 3D seismic P 2wave data in West 3mining area ,Zhangji coalmine ,Huainan ,are processed ,6azimuth data cubes are obtained from which several attributes related with coal and roof Πbottom fracture are extracted ,and then ,fracture density and orientation are measured.This method is a new tool for locating the distribution of coalbed enriched area.K ey w ords :seismic P 2wave data ;fracture ;azimuth anis otropy ;methane enriched area ;seismic attribute 瓦斯突出是指煤矿生产过程中,从煤层、岩层及采空区释放出的各种有害气体在工作面上富集并涌出,从而引起瓦斯爆炸的煤矿灾害。
- 1、下载文档前请自行甄别文档内容的完整性,平台不提供额外的编辑、内容补充、找答案等附加服务。
- 2、"仅部分预览"的文档,不可在线预览部分如存在完整性等问题,可反馈申请退款(可完整预览的文档不适用该条件!)。
- 3、如文档侵犯您的权益,请联系客服反馈,我们会尽快为您处理(人工客服工作时间:9:00-18:30)。
GEOPHYSICS,VOL.64,NO.4(JULY-AUGUST 1999);P .1266–1276,14FIGS.Fracture detection in a carbonate reservoir using a variety of seismic methodsMaria A.P´erez ∗,Vladimir Grechka ‡,and Reinaldo J.Michelena ∗ABSTRACTWe combine various methods to estimate fracture ori-entation in a carbonate reservoir located in southwest Venezuela.The methods we apply include the 2-D ro-tation analysis of 2-D P-S data along three different az-imuths,amplitude-variation-with-offset (A VO)of 2-D P -wave data along the same three azimuths,normal-moveout (NMO)analysis of the same 2-D data,and both 3-D azimuthal A VO and NMO analysis of 3-D P -wave data recorded in the same field.The results of all methods are compared against mea-sures of fracture orientation obtained from Formation microScanner logs recorded at four different locations in the field,regional and local measures of maximum horizontal stress,and the alignment of the major faults that cross the field.P-S data yield fracture orientations that follow the regional trend of the maximum horizontal stress,and are consistent with fracture orientations measured in the wells around the carbonate reservoir.Azimuthal A VO analysis yields a similar regional trend as that obtained from the P-S data,but the resolution is lower.Local variations in fracture orientation derived from 3-D A VO show good correlation with local structural changes.In contrast,due to the influence of a variety of factors,in-cluding azimuthal anisotropy and lateral heterogeneity in the overburden,azimuthal NMO analysis over the 3-D P -wave data yields different orientations compared to those obtained by other methods.It is too early to say which particular method is more appropriate and reliable for fracture characterization.The answer will depend on factors that range from local geological con-ditions to additional costs for acquiring new information.INTRODUCTIONFracture characterization is an important part of reservoir development,in particular for carbonate reservoirs.Different techniques have been used to estimate fracture orientation and density.Traditionally,S -waves generated at the surface and recorded by three component geophones (either with vertical seismic profiling or surface geometries)have been used for this purpose (Alford,1986).However,since acquisition and pro-cessing of S -waves is costly and the availability of shear waves sources is limited,different alternatives have been considered.Recently,P-S converted waves have become more popular because they are expected to contain the same information as S-S waves but can be generated with compressional sources,which makes the acquisition not only inexpensive but also less labor intensive than S-S waves recording.Garotta and Granger (1988)and Ata and Michelena (1995)showed examples of the use of P-S converted waves to estimate fracture orientation.P-S waves,however,are more cumbersome to use than non-Manuscript received by the Editor February 24,1998;revised manuscript received January 19,1999.Published on line.∗PDVSA-Intevep,Aptdo 76343,Caracas 1070A,Venezuela.E-mail:maru@;michelen@.‡Center for Wave Phenomena,Dept.of Geophysics,Colorado School of Mines,Golden,Colorado 80401.E-mail:vgrechka@.c 1999Society of Exploration Geophysicists.All rights reserved.converted waves because of the asymmetry of the ray path,and they are more expensive to record and process than conven-tional P-P waves.Since many areas in the world are already covered by 3-D P -wave data,various authors have focused their attention on the use of this existing information to estimate fracture prop-erties.A modeling exercise presented by Mallick and Frazer (1991)shows that the amplitude-variation-with-offset (A VO)response of P -waves can be affected by the presence of frac-tures depending on the relative orientation between fractures and the recording line.Lefeuvre (1994),Lynn et al.(1995),P´erez and Gibson (1996),and P´erez et al.(1999)presented ex-amples that confirmed Mallick and Frazer’s (1991)predictions.R ¨uger (1996)developed the theory behind these observations and showed how to estimate other fracture properties besides orientation.A more recent line of research initiated by Grechka and Tsvankin (1996)is devoted to the estimation of fracture1266Fracture Detection Using Seismic Methods 1267properties from the normal-moveout (NMO)analysis of mul-tiazimuth P -wave data.Corrigan et al.(1996)successfully ap-plied these ideas to field data.All the previously mentioned methods have their own limi-tations and do not necessarily yield the same results when ap-plied in the same area.They are influenced by different sources of noise that need to be properly considered and reduced to obtain accurate results.In this study,we compare the results of different seismic methods used to estimate fracture orientation when applied to different data sets recorded in the same field.The methods we use are the rotational analysis of converted waves,azimuthal A VO analysis,and NMO ellipticity.The results obtained from different methods generally agree (except that for 3-D NMO ellipticity),follow one of the fracture sets detected with For-mation microScanners (FMS)logs,and coincide with the trend of the maximum horizontal stress in the area.THE STUDY AREAMaporal field is located in the north-central part of the Barinas-Apure Basin,near the Andes,in Venezuela.Struc-turally,Maporal field is a dome slightly extended toward the northeast.Geologically,the sediments are nearly flat-lying,dip-ping toward the northeast at approximately 4◦.The target zone is the “O”member of the Escandalosa Formation.This mem-ber is a 25-m-thick fractured limestone located at a depth of approximately 3000m (2.32s).Since fractures seem to control production from the Escandalosa Formation,reservoir engi-neers decided to continue the exploitation of the field using horizontal wells oriented perpendicularly to the densest frac-ture systems.Seismic data were recorded to help engineers achieve theirgoal.F IG .1.Maximum horizontal stress (inward facing arrows)from break-out orientation logs at wells 16,17,20,and 23.The rose diagrams indicate fracture orientation and density from FMS logs in the same wells.Existing well log information in the field provides good back-ground information about the reservoir and its fracture prop-erties,and can be used to calibrate the results obtained from the seismic data.Well data include gamma-ray,resistivity,and dipole sonic logs,which provided information about P -and S -waves around the target.FMS logs were used to estimate fracture orientation,fracture density,and orientation of maxi-mum horizontal stress at four different wells.The rose diagrams in Figure 1shows the presence of different fracture systems in the area.However,the orientation of the maximum horizontal stress measured in the same wells is constant across the field.The open fracture system tends to be the one parallel or quasi-parallel to the orientation of maximum horizontal stress.AVAILABLE DATAThe data sets used for this study consisted of a 3-D P -wave survey recorded over an area (640km 2)much larger than the area of interest to help in the characterization of other adjacent reservoirs,and three 10-km 2-D 3-C lines centered in the area of interest (Figure 2).The multicomponent acquisition was per-formed right after the 3-D acquisition finished and,therefore,the results of the analysis of one data set were not used to help the design of the other.The three 2-D 3-C multicomponent lines were centered over the area of interest with an intersection point coinciding with a well location.For calibration purposes,each line intersected,or was close to,at least one additional well with log information.Previous P -wave 2-D seismic data were used to identify two nearly orthogonal faults systems that cross the field (Figure 1).The azimuths of two of the multicomponent lines were almost parallel to these systems,whereas the other line bisected them,forming an angle of approximately 45◦with each.A noise-1268Perez et al.spread test was used to optimize the design and enhance the quality of the converted waves.The 3-D seismic data were collected using a swath geome-try with a shot line perpendicular to eight receivers lines.A bin spacing of 80m,a fold of approximately 40traces,and a maximum offset of 3626m were acquired.A subset of 25km 2from the original 3-D data set centered at the intersection point of the 2-D multicomponent lines was used for this study.Su-perbins of 240×240m 2were formed to insure adequate cov-erage in offset and azimuth for A VO and NMOanalysis.F IG .2.Study area over 2-D and 3-Dsurveys.F IG .3.Typical supergather from 3-D data.The bin size is 240×240m 2.The processing sequence of both data sets was designed to preserve relative amplitude,maximize frequency band,and op-timize velocity analyses for all components.Refraction statics estimated from a P -wave refraction survey were applied to both 2-D and 3-D data.Converted wave statics in the 2-D 3-C data were corrected by using P -wave statics and various iterations of residual statics.Figure 3shows a typical CDP supergather from the 3-D data.Only f-k filtering in the shot domain has been applied to these data to eliminate surface waves.Notice how the presence of static noise,or possible azimuthal anisotropy,Fracture Detection Using Seismic Methods 1269influences the continuity of all reflections.Reflections from the top of the target (Escandalosa Formation)are located at 2.32s.Figure 4shows a 2-D 3C,raw common-shot gather recorded over line 2.Notice the presence of energy in both horizontal components,which is an indication of azimuthal anisotropy.Michelena et al.(1994)performed modeling to demonstrate that azimuthal anisotropy,not heterogeneity,is responsible for the energy observed in the transverse component in this area.As shown by Ata and Michelena (1995),fracture orientation changes across the field,which explains why not all 3-C records show energy in the transverse component.Converted waves from the top of Escandalosa Formation are indicated by the arrow at 3.8s.Figure 5shows the structural map of the top of the Escandalosa Formation interpreted from the 3-D P -wave seis-mic data.This map confirms the gentle nature of the structural variations in the field already known from previous 2-D P -wave data.As we mentioned before,this structure dips 4◦toward the northeast.DATA ANALYSISWe estimate fracture orientation by applying different meth-ods to the different data sets available.We start by analyzing the P-S converted waves in the multicomponent data.Then,we analyze the A VO and NMO responses of P -waves recorded in the vertical component of the 2-D 3-C data around the intersec-tion point of the three lines.Finally,we examine the azimuthal variations of A VO and NMO responses of the P -waves for each bin in the 3-D data.This section describes these results.2-D dataRotation analysis of P-S converted waves.—Figure 6shows portions of migrated horizontal components around threedif-F IG .4.3-C raw common-shot gather recorded over line 2of the 2-D multicomponent lines.Converted waves from the top of Escandalosa Formation are indicated by the arrow at 3.8s in the horizontal components.ferent points in the field.Notice that points located on lines 1and 3clearly show that the horizontal component parallel to line 3arrives earlier than the other component,which is what we expected from the direction of the maximum hori-zontal stress in the field.Remember that the orientation of the faster shear arrival generally coincides with the direction of maximum horizontal stress.After interpreting the migrated sections of radial and trans-verse components,we compute rms amplitudes in a window around the target.Then,we perform rotational analysis based on the amplitude ratio between the two horizontal components (Ata and Michelena,1995)to estimate fracture orientation for each common conversion point (CCP)of the three lines.From the angles estimated at each CCP ,we obtain new angles for points outside the multicomponent lines using 2-D spline in-terpolation.The orientation of the fastest shear arrival happens to be approximately constant for all depths across the field.Figure 7shows a smoothed map with the results of the ro-tational analysis plus interpolation.Each arrow indicates the local fracture orientation (fracture strike)estimated from con-verted waves.The colors show the same structural map pre-sented in Figure 5.Since the azimuths between the lines have been interpolated,orientations presented in this map are more reliable along and in the neighborhood of the three lines.As we can see,fracture orientation follows the trend of the maximum horizontal stress in the area.Notice that the estimated fracture orientation follows the direction of one of the fracture systems present in the area.Azimuthal A VO from 2-D data.—For the 2-D P -wave data,we perform conventional A VO analysis over CDP gathers lo-cated along each line close to the intersection point.We ob-tain the sections of A VO gradient and A VO intercept for each line.Figure 8shows the A VO gradient sections around the1270Perez et al.intersection point along almost perpendicular lines 1and 3.Notice the different A VO gradients for the reflection from the bottom of the reservoir along these two lines.No significant differences are observed in A VO response from the top ofthe F IG .5.Structural map of the top of Escandalosa Formation interpreted from the 3-D seismic data.Colors indicatetwo-way traveltime inseconds.F IG .6.Horizontal components from three different locations in the field.reservoir.Figure 9is a graph of A VO gradient versus A VO intercept.Gradients for lines 1and 2(nearly perpendicular to fracture orientation)are positive and higher than gradients along line 3(which is nearly parallel to the fracture orientationFracture Detection Using Seismic Methods 1271estimated from converted waves).As expected,the A VO in-tercept is almost the same for all lines.This result is consistent with the observations of Mallick and Frazer (1991)and John-son (1995)that,in the presence of azimuthal anisotropy,A VO characteristics along different shooting directions are similar for near-normal reflections and may differ for wide-angle re-flections.The exact direction of the maximum A VO gradient was esti-mated from the three CDP gathers located at the intersection point of the multicomponent lines.We use a formula derived by R ¨uger (1996)for the reflection coefficients in transversely isotropic media with a horizontal symmetry axis.The estimated azimuth of the maximum A VO gradient is 56◦.Since it is ex-pected to be perpendicular to fracture orientation,we obtain the fracture azimuth as 146◦(Figure 10).As we can see,fracture orientation estimated using this technique also follows the re-gional maximum horizontal stress and is close to the orientation obtained from the analysis of the converted waves (Figure 7).NMO ellipticity from 2-D data.—From the same three gath-ers used to obtain the orientation of the maximum A VO gradient,we estimate the parameters that describe the best-fitting horizontal ellipse of the NMO velocities for all azimuths (Grechka and Tsvankin,1996).The NMO ellipse obtained from this analysis is shown in Figure 11.The azimuth of the major axis is 125◦.The major axis corresponds to the maxi-mum NMO velocity that is expected to coincide with fracture orientation.The differences between maximum and minimum velocities are around 5%,but the axes of the ellipse have been exaggerated to give a better idea of its orientation.In this case,the orientation of the major axis of the NMO ellipse also fol-lows the regional trend of the maximum horizontal stress in thearea.F IG .7.Fracture orientation from rotational analysis of converted waves.3-D dataAzimuthal A VO from 3-D data.—3-D P -wave data was gath-ered using a bin size of 240×240m 2to achieve the coverage needed in both offset and azimuth to perform azimuthal A VO analysis.The orientation of the maximum A VO gradient was estimated for each superbin based on the amplitudes located within a time window that follows the structural interpretation of the top of Escandalosa Formation (Figure 5).Figure 12shows smoothed results of the azimuthal A VO analysis for each superbin.The arrows in Figure 12are ori-ented according to these new,smoothed angles.As we can see,the estimated orientations follow closely the local structural changes,but the general trend is still close to the regional max-imum horizontal stress.Areas with abrupt changes in structure seem to have a more erratic A VO response when compared to other areas in the field.Notice the similarity between the results obtained with converted waves (Figure 7)and 3-D azimuthal A VO analysis (Figure 12).There are differences,however,between the two results,especially in the northwest part of the area.No inter-polation was used to generate Figure 12because A VO analysis was performed at equally spaced grid points.On the contrary,to generate Figure 7,we interpolated the azimuths measured along the lines for all grid points where no information was available.In principle,this can create unrealistic orientations in areas surrounded by angles that represent the same direc-tion but different orientations (0◦and 180◦,for instance),which is the case in the northwest part of the area.No attempt was made to change these angles in the data before interpolation.NMO ellipticity from 3-D data.—3-D NMO analysis was per-formed for the same superbins used in the 3-D azimuthal A VO1272Perez et al.F IG.8.A VO gradient sections around the interception point for lines1and3.Notice how the A VO response changes around thebottom of the reservoir located at2.370s.Fracture Detection Using Seismic Methods 1273analysis.Figure 13shows the result of this process:the ori-entation of the semimajor axes of NMO ellipses at the target for each CDP .The general trend in the orientation of these axes is not as expected from all our previous results,which F IG .9.A VO intercept versus A VO gradient for lines 1,2,and 3at the intersection point of the 2-D multicomponentlines.F IG .10.Orientation perpendicular to the maximum A VO gradient at the intersection point of the 2-D multi-component lines.This result is compared to the orientation of line 3,which is nearly parallel to the orientation of maximum horizontal stress in the area.followed the orientation of the maximum horizontal stress more closely.In Figure 13,the differences between the semi-major and semiminor axes of the NMO ellipse are of the order of 3%.1274Perez et al.We can speculate about various reasons to explain the ori-entations of NMO ellipses for each CDP .First,the NMO el-lipses include a cumulative influence of the overburden from the surface to the target.We did not do layer stripping toobtain F IG .11.Orientation of the NMO ellipse from 2-D P -wave data at the intersection point of the 2-D multicom-ponentlines.F IG .12.Fracture orientation from 3-D azimuthal A VO analysis.The arrows indicate the local orientation of theperpendicular to the maximum A VO gradient.interval velocities because the target is too thin (25m)com-pared to its depth and,therefore,the error amplification due to stripping is expected to be severe.Second,since the ellipticities (i.e.,the elongations of NMO ellipses)are small (about 3%),Fracture Detection Using Seismic Methods 1275the ellipse orientation becomes a poorly determined quantity,so that the estimated ellipse azimuths may be inaccurate.The third issue is that we believe that the proper way to remove the effects of near-surface azimuthal anisotropy is by doing inde-pendent static corrections for each azimuth,which we didonly F IG .13.Fracture orientation from 3-D NMO ellipticity.The arrows indicate the local orientation of the maximumaxis for the NMOellipse.F IG teral velocity variations of the isotropic NMO velocity at the bottom of the reservoir.for the 2-D data.The effect of static variations,near-surface anisotropy,and azimuthal anisotropy in the subsurface that affect the NMO velocities cannot be separated with static cor-rection methods that analyze simultaneously all azimuths in a superbin.We found that after applying such corrections for any1276Perez et al.depth,lateral coherency of events was improved considerably, but differences between major and minor axes of NMO el-lipses were reduced to less than0.01%.For this reason,we did not remove static corrections in the data used to generate Fig-ure13.Finally,the influence of lateral heterogeneity may make azimuthal variation of the NMO velocity elliptical even in the absence of anisotropy.Figure14shows lateral variations of the isotropic NMO velocity(e.g.,a circular approximation of NMO ellipse)estimated from conventional velocity analysis around the target.Even though the changes in these velocities are not that large,they may distort our inferences about P-wave az-imuthal anisotropy which are made under the assumption that the medium is laterally homogeneous.CONCLUSIONSWe have applied a variety of methods to estimate frac-ture orientation using different kinds of surface seismic data recorded over the same reservoir.Most of the results from dif-ferent methods(rotation analysis of P-S-wave2-D data,and azimuthal A VO and NMO analysis of2-D and3-D P-wave data)captured the regional orientation of maximum horizon-tal stress in thefield;however,when compared locally,the different methods may yield somewhat different answers.Lo-cal changes seem to be controlled by structural variations across thefield.Even though the reservoir has several frac-ture systems,most of the applied methods picked the one that is closer to the orientation of the maximum horizontal stress.The NMO ellipticity analysis over the3-D data produced results different than those produced by other methods.Al-though several reasons may explain such differences,we be-lieve that the most important ones include near-surface az-imuthal anisotropy and overburden effects that could not be removed,and lateral velocity variations that may affect the es-timated orientations.We believe static corrections should be performed independently for different azimuth ranges instead of correcting for all azimuths simultaneously.By doing az-imuthally independent static corrections,we will avoid elim-inating the effects of anisotropy in the subsurface that can be treated as near-surface variations if all azimuths are treated at once.The answer to the question of whether there is a most appropriate and reliable method of fracture characterization depends on a variety of issues that need to be carefully ana-lyzed.Ideally,if we have the resources to acquire multicom-ponent data,we should use this option that has proven its ef-fectiveness in estimating not only fracture orientation but also fracture density.If we have only3-D P-wave data with good amplitude control,our results suggest that3-D azimuthal A VO analysis is more robust than3-D azimuthal NMO analysis.If we have only2-D P-wave data recorded along intersecting lines, we can use the results of azimuthal A VO and NMO analysis at the intersection points to obtain a low-resolution estimate of the fracture orientation.Such orientation may help to design further3-D P-wave or multicomponent seismic acquisitions that yield higher resolution estimates.In any case,we advise always using independent information that may be available from cores,well logs,and geology to assess the reliability of the results obtained with any particular method before drawing anyfinal conclusions.ACKNOWLEDGMENTSWe thank PDVSA-Intevep for permission to publish this work.We also thank Luis Lugo,Hector Pineda,and Elias Ata for their help in processing the data.We thank Ken Craft, Richard VanDok,and Gerard Beaudoin for their comments and suggestions that helped to improve the quality of this manuscript.REFERENCESAlford,R.M.,1986,Shear data in the presence of azimuthal anisotropy: 56th Ann.Internat.Mtg.,Soc.Expl.Geophys.,Expanded Abstract, 476–479.Ata,E.,and Michelena,R.J.,1995,Mapping distribution of fractures in a reservoir with P-S converted waves:The Leading Edge,12,664–676.Corrigan,D.,Withers,R.,Darnall,J.,and Skopinski,T.,1996,Analysis of amplitude versus offset to detect gas-oil contacts in the Arabian Gulf:66th Ann.Internat.Mtg.,Soc.Expl.Geophys.,Expanded Ab-stract,1834–1837.Garotta,R.,and Granger,P.Y.,1988,Acquisition and processing of3C ×3-D data using converted waves:58th Ann.Internat.Mtg.,Soc. Expl.Geophys.,Expanded Abstract,657–658.Grechka,V.,and Tsvankin,I.,1996,3D description of normal moveout in anisotropic media:66th Ann.Internat.Mtg.,Soc.Expl.Geophys., Expanded Abstracts,1487–1490.Johnson,W.E.,1995,Direct detection of gas in pre-Tertiary sedi-ments?:The Leading Edge,14,119–122.Lefeuvre,F.,1994,Fracture related anisotropy detection and analysis:“And if the P-waves were enough?”:64th Ann.Internat.Mtg.,Soc. Expl.Geophys.,Expanded Abstracts,942–944.Lynn,H.,Simon,K.M.,Layman,M.,Schneider,R.,Bates,C.R.,and Jones,M.,1995,Use of anisotropy in P-wave and S-wave data for fracture characterization in a naturally fractured gas reservoir:The Leading Edge,14,887–893.Mallick,S.,and Frazer,L.N.,1991,Reflection/transmission coefficients and azimuthal anisotropy in marine seismic studies:Geophys.J.In-ternat.,105,241–252.Michelena,R.J.,Ata,E.,and Sierra,J.,1994,Exploiting P-S con-verted waves:Part1,Modeling the effects of anisotropy and hetero-geneities:64Ann.Internat.Mtg.,Soc.Expl.Geophys.,Expanded Abstract,236–239.P´erez,M.,and Gibson,R.,1996,Detection of fracture orientation us-ing azimuthal variation of P-wave A VO responses:Barinas Field (Venezuela):66th Ann.Internat.Mtg.,Soc.Expl.Geophys.,Ex-panded Abstracts,1353–1356.P´erez,M.,Gibson,R.,and T¨oksoz,N.,1999,Detection of Fracture ori-entation from azimuthal variation of P-wave A VO responses:Geo-physics,64,1253–1267,this issue.R¨uger,A.,1996,Reflection coefficients and azimuthal A VO analysis in anisotropic media:Ph.D.thesis,Colorado School of Mines.。