Note of Computer Vision- Algorithms and Applications#1
计算机机器视觉简历模板

计算机机器视觉简历模板Computer Vision Resume Template.Personal Information.Name: [Your Name]Email: [Your Email Address]Phone: [Your Phone Number]LinkedIn: [Your LinkedIn Profile URL]Objective.To obtain a challenging and rewarding position in the field of computer vision where I can apply my expertise in developing and implementing innovative solutions to complex problems.Skills.Image Processing and Analysis.Machine Learning and Deep Learning.Computer Vision Algorithms.Object Detection and Recognition.Scene Understanding and Segmentation.Image Manipulation and Enhancement.Python, C++, and CUDA.OpenCV, TensorFlow, and PyTorch.Experience.Computer Vision Engineer, ABC Company, [Start Date] [End Date]Developed and implemented computer vision models for real-time object detection and tracking.Designed and trained deep learning models using TensorFlow and PyTorch.Collaborated with a team of engineers to integrate computer vision solutions into production systems.Research Assistant, University of XYZ, [Start Date] [End Date]Conducted research on object recognition and image classification using deep learning techniques.Published papers in peer-reviewed conferences and journals.Presented research findings at academic conferences.Education.Master of Science in Computer Science, University of XYZ, [MM/YY] [MM/YY]GPA: [Your GPA]Bachelor of Science in Computer Science, ABC University, [MM/YY] [MM/YY]GPA: [Your GPA]Projects.Object Detection and Tracking System: Developed areal-time object detection and tracking system using YOLOv5 and OpenCV, achieving an accuracy of over 95%.Image Classification Model: Trained a deep learning model using TensorFlow to classify images into different categories with an accuracy of 98%.Self-Driving Car Simulator: Built a self-driving carsimulator using Python and OpenCV to test and evaluate computer vision algorithms for autonomous driving.Certifications.Certified Computer Vision Professional (CCVP)。
中国人工智能的发展情况英语作文

中国人工智能的发展情况英语作文全文共3篇示例,供读者参考篇1The Development of Artificial Intelligence in ChinaArtificial intelligence (AI) has become a focus of attention and investment in China in recent years. With the rapid development of technology, China has been making significant progress in the field of AI, from both government support and private sector innovation.In terms of government support, China has made AI a national priority. In 2017, the State Council of China released a plan to become the world leader in AI by 2030. The plan includes initiatives to increase investment in research and development, build a skilled workforce, and create a supportive regulatory environment for AI development. The Chinese government has also established AI research institutes, such as the National Engineering Laboratory for Deep Learning Technology and Application, to support the growth of the industry.In addition to government support, the private sector in China has been actively investing in AI research anddevelopment. Chinese tech giants like Baidu, Alibaba, and Tencent have all established AI research labs and are making significant investments in AI technologies. These companies are developing AI-powered products and services in a wide range of industries, from healthcare and finance to autonomous vehicles and smart cities.China is also becoming a global leader in AI research. Chinese researchers are publishing more AI research papers and patents than any other country, and Chinese universities are producing top talent in the field. Chinese AI companies are also collaborating with international partners to advance AI technologies and applications.However, there are challenges facing the development of AI in China. One major challenge is the lack of talent with expertise in AI. While China produces a large number of STEM graduates, there is a shortage of skilled AI professionals. The Chinese government is working to address this talent gap by implementing initiatives to train more AI professionals and attract top talent from around the world.Another challenge is the ethical and regulatory issues surrounding AI development. As AI technologies become more advanced, there are concerns about privacy, surveillance, andbias in AI algorithms. Chinese regulators are working to develop a framework for ethical AI development and deployment, but there are still many unresolved issues.Overall, China's development of AI is rapidly progressing, driven by government support, private sector investment, and a strong talent pool. As China continues to invest in AI technologies and applications, it will play an increasingly important role in shaping the future of AI on a global scale.篇2The Development of Artificial Intelligence in ChinaArtificial Intelligence (AI) has been a hot topic in recent years, with many countries racing to develop and implement AI technologies into various industries. China, as one of the world's leading economies, has also been making significant strides in the field of AI. In this essay, we will discuss the development of artificial intelligence in China, focusing on its current state, advancements, challenges, and future prospects.China's AI industry has been growing rapidly in recent years, with the Chinese government placing a strong emphasis on AI development as part of its national strategy. In 2017, the State Council of China released the "New Generation ArtificialIntelligence Development Plan," which outlined a roadmap for China to become the world leader in AI by 2030. This plan set ambitious goals for China to build a domestic AI industry worth nearly $150 billion by 2030 and to achieve major breakthroughs in AI technology.One of the key factors driving the growth of AI in China is the country's vast amount of data. With a population of over 1.4 billion people and a high rate of internet and smartphone usage, China generates massive amounts of data every day. This data is essential for training AI algorithms and developing AI applications in various industries, such as healthcare, finance, transportation, and manufacturing.In terms of AI research and development, China has been making significant advancements in machine learning, natural language processing, computer vision, and robotics. Chinese tech giants such as Baidu, Alibaba, Tencent, and Huawei have been investing heavily in AI research, hiring top AI talent, and collaborating with leading research institutions around the world. Chinese universities and research institutes have also been actively involved in AI research, publishing high-impact papers and participating in international AI competitions.China's AI industry has also been benefiting from the government's supportive policies and funding initiatives. The Chinese government has been investing billions of dollars in AI research and development, establishing AI research centers, incubators, and technology parks across the country. The government has also been promoting AI education and training programs to cultivate a skilled workforce in AI-related fields.Despite the rapid growth of China's AI industry, there are still some challenges and concerns that need to be addressed. One of the main challenges is the lack of transparency and ethical guidelines in AI development. There have been concerns about data privacy, algorithm bias, and the potential misuse of AI technology for surveillance and social control. It is important for China to establish clear regulations and ethical standards to ensure the responsible development and deployment of AI technologies.Another challenge facing China's AI industry is the shortage of talent. While China has a large pool of AI researchers and engineers, there is still a need for more skilled professionals in specialized areas such as deep learning, reinforcement learning, and computer vision. It is crucial for China to invest in AIeducation and training programs to develop a diverse and highly skilled workforce in AI technology.Looking ahead, the future prospects of China's AI industry are promising. With strong government support, abundant data resources, and a growing pool of AI talent, China iswell-positioned to become a global leader in AI innovation. Chinese companies are already making significant advancements in AI-powered applications, such as autonomous vehicles, medical diagnosis, and smart cities. By leveraging its strengths in AI research, development, and deployment, China has the potential to shape the future of AI technology and drivesocio-economic development in the years to come.In conclusion, the development of artificial intelligence in China has been progressing rapidly, fueled by strong government support, abundant data resources, and a growing pool of AI talent. While there are challenges and concerns that need to be addressed, the future prospects of China's AI industry are promising. With continued investment in AI research, education, and regulation, China has the potential to become a global leader in AI innovation and shape the future of AI technology worldwide. It will be interesting to see how China's AIindustry evolves in the coming years and what impact it will have on society and the economy.篇3The Development of Artificial Intelligence in ChinaArtificial Intelligence (AI) has been a rapidly growing field in China in recent years, with the country investing heavily in research, development, and talent training. As one of the world's leading technology hubs, China has made significant strides in AI innovation, applications, and commercialization.One of the key drivers of AI development in China is the government's strong support and investment in the industry. In 2017, the Chinese government announced a national AI development plan, outlining its vision to become the global leader in AI by 2030. This plan includes initiatives to build AI research centers, fund AI startups, and promote AI applications in various industries.China is also home to some of the world's leading AI companies, such as Baidu, Alibaba, Tencent, and Huawei. These companies have made substantial investments in AI research and development, and have developed innovative AI technologies in areas like autonomous driving, healthcare, finance, and robotics.In addition to the private sector, China's academic institutions have also played a crucial role in advancing AI research. Leading universities like Tsinghua University, Peking University, and the Chinese Academy of Sciences have established AI research centers and collaborations with industry partners to drive innovation in the field.Furthermore, China has been actively promoting the development of AI talent through initiatives like the "Thousand Talents Program" and the "One Thousand Young Talents" Program. These programs aim to attract top AI researchers and professionals from around the world to work in China and contribute to the country's AI ecosystem.With its fast-growing economy, large population, and technological expertise, China is well-positioned to become a global leader in AI. However, challenges remain, such as data privacy concerns, ethical issues, and international competition. Nevertheless, China's commitment to AI development and its strong ecosystem of research institutions, companies, and talent make it a powerhouse in the field of artificial intelligence. So, the future of China's AI industry looks promising, as the country continues to invest in research, innovation, and talent to drive its AI agenda forward.。
国际普遍公认的计算机学科期刊分级
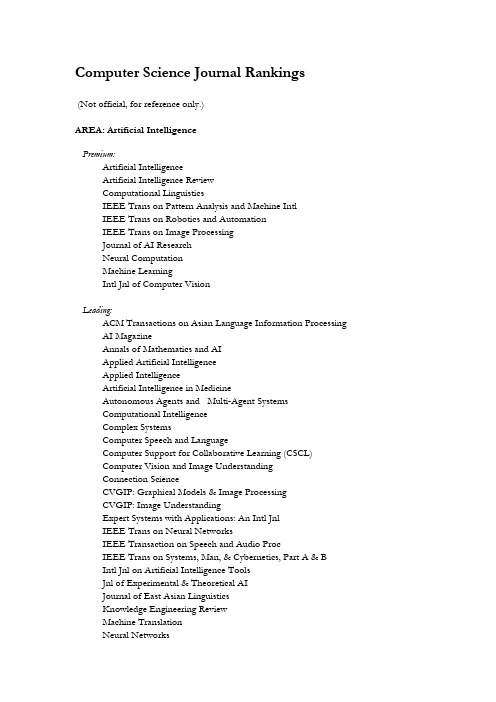
Computer Science Journal Rankings(Not official, for reference only.)AREA: Artificial IntelligencePremium:Artificial IntelligenceArtificial Intelligence ReviewComputational LinguisticsIEEE Trans on Pattern Analysis and Machine IntlIEEE Trans on Robotics and AutomationIEEE Trans on Image ProcessingJournal of AI ResearchNeural ComputationMachine LearningIntl Jnl of Computer VisionLeading:ACM Transactions on Asian Language Information ProcessingAI MagazineAnnals of Mathematics and AIApplied Artificial IntelligenceApplied IntelligenceArtificial Intelligence in MedicineAutonomous Agents and Multi-Agent SystemsComputational IntelligenceComplex SystemsComputer Speech and LanguageComputer Support for Collaborative Learning (CSCL)Computer Vision and Image UnderstandingConnection ScienceCVGIP: Graphical Models & Image ProcessingCVGIP: Image UnderstandingExpert Systems with Applications: An Intl JnlIEEE Trans on Neural NetworksIEEE Transaction on Speech and Audio ProcIEEE Trans on Systems, Man, & Cybernetics, Part A & BIntl Jnl on Artificial Intelligence ToolsJnl of Experimental & Theoretical AIJournal of East Asian LinguisticsKnowledge Engineering ReviewMachine TranslationNeural NetworksNetwork Computing in Neural SystemsPattern Analysis and ApplicationsPattern RecognitionNeurocomputingUser Modelling & User-Adapted Interaction: an Intl Jnl Reputable:Communications of COLIPSComputer Processing of Chinese & Oriental Languages Computers and Artificial IntelligenceCybernetics and SystemsEngineering Intelligent Systems for EE and CSExpert SystemsEvolutionary ComputationIntelligent Instruments & ComputersIntl Jnl for AI in EngineeringIntl Jnl of Applied Expert SystemsIntl Jnl of Approximate ReasoningIntl Jnl of Intelligent SystemsIntl Jnl of Neural SystemsIntl Jnl of Pattern Recognition & AIIntl Journal of Document Analysis and RecognitionIEEE Transactions on Fuzzy SystemsJournal of Intelligent and Fuzzy SystemsKnowledge Acquisition JnlKnowledge-Based SystemsKybernetikaNatural Language EngineeringNeural Computing & ApplicationsNetwork SocietyNeural Processing LettersPattern Recognition LettersIEE Proceedings: Vision, Image and Signal ProcSpeech CommunicationsJournal of Neural Network ComputingMinds and Machines: Jnl for AI, Philosopy and Cog. Sc Intl Jnl of Uncertainty, Fuzziness and KBSHeuristics: Jnl of Knowledge EngineeringEngineering Applications of AIJnl. of Japanese Soc. of AIAustralian Jnl of Intelligent Information Proc SysIntelligent Data AnalysisImage and Vision ComputingJournal of Artificial Neural NetworksNeural, Parallel and Scientific ComputationsRoboticaOthers:AIAA JournalCanadian Artificial IntelligenceJournal of Advanced RoboticsJournal of Artificial Intelligence in EducationJournal of Artificial Intelligence in Engineering, Automation, and Manufacturing Journal of Artificial Intelligence, Neural Networks and ComplexJournal of Computational AcousticsJournal of Computational NeuroscienceJournal of Computational VisionJournal of Card. ImagingJournal of CyberneticsJournal of Cybernetics and Information ScienceJournal of Design Automation of Embedded SystemsJournal of Knowledge-Based Intelligent Engineering SystemsJournal of Intelligent Robotic Systems: Theory and ApplicationsJournal of Systems Automation: Research and ApplicationsJournal of Automation and Remote ControlJournal of Intelligent Automation & Soft ComputingJournal of Intelligent Control and SystemsJournal of Intelligent and Robotic SystemsJournal of Intelligent ManufacturingJournal of Intelligent Systems EngineeringJournal of Intelligence SystemJournal of Intelligent TechnologyJournal of Literary and Linguistic ComputingJournal of Machine Vision and ApplicationsJournal of Man-Machine StudiesJournal on Neural and Mass-Parallel Computing and Information Systems Journal of Robotics and MechatronicsJournal of Robotic SystemsJournal of Robotics and Autonomous SystemsJournal of Robotics ResearchJournal of the Robotics Society of JapanJournal of Computational NeurologyInternational Journal of LexicographyJournal of intelligent ComputingInternational Journal of Intelligent Systems in Accounting Finance and Management International Journal of Speech TechnologyEngineering Design and Automation JournalInternational Journal of Machine Tools & ManufacturingInternational Journal of Corpus LinguisticsJournal of Chinese Information ProcessingAREA: Algorithms, Theory and Related AreasPremium:AlgorithmicaComputational ComplexityDiscrete & Computational GeometryIEEE Trans on Information TheoryInformation & ComputationJnl of AlgorithmsJnl of Computer and System SciencesJnl of the Association for Computing MachinerySIAM Jnl on ComputingMathematics of ORLeading:Acta InformaticaChicago Journal of Theoretical Computer ScienceComputational Logic (TOCL)Designs, Codes and CryptographyJnl of Symbolic ComputationJournal of Automated ReasoningJournal of Graph Algorithms and ApplicationsJournal of ComplexityJournal of CryptologyJOTA - J. of Optimization: Theory and ApplicationsMathematics of ComputationMathematical ProgrammingOptimization: A J. of Mathematical Programming and Operations Research ORSA Journal of ComputingNordic J of Computing (BIT)SIAM Journal on OptimizationRandom Structures & AlgorithmsTheoretical Computer ScienceReputable:Applicable Algebra in Eng., Comm., and ComputingApplied Maths and ComputationBIT: Computer Science and Numerical MathematicsBulletin of the European Assoc. for Theoretical CSComputational and Applied MathsComputers & Mathematics With ApplicationsCombinatorics, Probability & ComputingEuropean Journal of ORJournal of Computer and System Sciences InternationalIntl Jnl of Foundations of Computer ScienceOthers:Problem Solving TechnologiesJournal of Algebraic CombinatoricsJournal of Combinatorial DesignsJournal of Combinatorial OptimizationJournal of Experimental AlgorithmicsJournal of Electronic ImagingIntl J. for Numerical Methods in EngineeringSIAM Journal of Algorithms and Discrete MethodsSIAM Journal on Algebraic and Discrete MethodsSIAM Journal on Numerical AnalysisSIAM Journal on Matrix Analysis and ApplicationsNaval Journal of Operations ResearchJournal of SchedulingElectronic Journal of CombinatoricsJournal of Mathematical Modeling and Scientific Computing Journal of Mathematical Structures in Computer ScienceInternational Journal on Mathematical and Computer Models Journal of Global OptimizationJournal of Computational Statistics and Data AnalysisAREA: Hardware and ArchitecturePremium:IEEE Trans on Circuits and Systems IIEEE Trans on ComputersIntegration: The VLSI JnlVLSI DesignLeading:IEEE Trans on Circuits and Systems IIJnl of Microcomputer ApplicationsMicroprocessing and Microprogramming Reputable:Computer DesignDigital ProcessesElectronics Letters,EUROMICRO JnlJnl of Circuits, Systems and ComputersJnl of Electronics, Information and SystemsMicroprocessors and MicrosystemsSupercomputerIEEE Journal on Computer Architectures for Intelligent Machines Others:Signal ProcessingTechnical Journal of Digital Equipment CorporationThe Linux JournalIBM Application System/400 Technology JournalJournal of System ArchitectureNEC Technical JournalInternational Journal of Computer Aided VLSI DesignMRS Internet Journal of Nitride Semiconductor ResearchStandards and Interface JournalInternational Journal of Mini and MicrocomputersJournal of Microelectronic Systems IntegrationUser Modeling and User-Adapted InteractionThe X Resource: A Practical Journal of the X Window SystemHewlett-Packard JournalAREA: DatabasesPremium:ACM Trans on Database SystemsIEEE Trans on Knowledge & Data EngineeringJnl of Intell. Info Systems: Integrating AI and DB TechVLDB Intl JnlDistributed and Parallel DatabasesLeading:Data & Knowledge EngineeringInformation systemsJnl of Systems IntegrationJnl. of Data Mining & Knowledge DiscoveryIntl Jnl of Computer & Information SciencesIntl Jnl of Cooperative Information SystemsIntl Jnl of Intelligent & Cooperative Info. SystemsIntl Jnl of Geographic Information SystemsJournal of Information Processing and CyberneticsGeoinformaticaJournal on Digital LibrariesJournal of the American Society for Information ScienceJournal of Intelligent Information Systems Reputable:Data EngineeringKnowledge and Information SystemsAdvances in Engineering SoftwareInformation & Software TechnologyData BaseData Base ManagementDatabase and Network JournalJournal of Data WarehousingJournal of Combinatorics, Information and System SciencesInternational Journal of Information TechnologyTransactions of Information Processing Society of JapanSIGMOD RecordIEICE Data EngineeringOthers:Journal of Computing Information ScienceJournal of Information Science and EngineeringEuropean Journal of Information SystemsJournal of Databases ManagementDatabase for Advances in Information SystemsData ManagementDatamationGovernment Data SystemsJournal of Database AdministrationJournal of the Association for Education Data SystemsInformation Processing and ManagementJournal of Information Science: Principles and PracticeDatabase Programming and DesignScandinavian Journal of Information SystemAREA: Programming Languages and Software Engineering Premium:ACM Trans on Programming Languages & SystemsAnnals of Software EngineeringIEEE Trans on Software EngineeringJnl of Functional ProgrammingACM Trans on S/W Eng and MethodologyFormal Methods in System DesignLeading:The Jnl of Logic ProgrammingIEE Procs - SoftwareJnl of Software Maintenance: Research and PracticeHigher-Order and Symbolic Computation (previously known as LISP and Symbolic Computation)Software: Practice and ExperienceJnl of Functional and Logic ProgrammingThe Constraints JournalJournal of Logic and ComputationJournal of Programming LanguagesEmpirical Software EngineeringAutomated Software EngineeringFormal Aspects of ComputingObject-Oriented SystemsTheory and Practice of Object SystemsJournal of Object-Oriented ProgrammingIEEE Transactions on ReliabilityFuture Generations Computer Systems: FGCSReputable:Programming and Computer SoftwareScience of Computer ProgrammingJnl of Systems and SoftwareIntl Jnl on Software Engineering and Knowledge EngNew Generation ComputingSoftware Quality JournalSoftware Testing, Verification and ReliabilityComputer LanguagesRequirements Engineering JournalIEE Software Engineering JournalOthers:Journal of the Interest Group in Pure and Applied LogicNotre Dame Journal of Formal LogicJournal of Computer and Software EngineeringJournal of Structured ProgrammingInternational Journal on Software Tools for Technology Transfer Chinese Journal of Advanced Software ResearchJournal of Computing Systems in EngineeringJournal of Symbolic LogicProject Management JournalInternational Journal of Reliability, Quality, and SafetyJournal for Applied Nonclassical LogicThe Journal of Defense Software EngineeringComputer & Control Engineering JournalJournal of Logic, Language and InformationComputer Systems Engineering JournalJournal of Automata, Languages and CombinatoricsThe C Users JournalInformation Design JournalJava Developer's JournalC++ JournalFortran JournalJournal of Scientific ProgrammingLogic Journal of the IGPLJournal of Philosophical LogicJournal of Quality TechnologyInternational Journal of Technology ManagementSoftware Process Modeling and TechnologyJournal of Computers and TranslationJournal of C Language TranslationJournal of Electronic TestingSoftware Engineering NotesAREA: Software TechnologyPremium:ACM Trans on GraphicsACM Trans on Modeling & Computer SimulationComputer Aided Geometric DesignComputer-Aided DesignIEEE Trans on CAD of Integrated Circuits & SystemsIEEE Trans on Visualization and Computer GraphicsSIAM Jnl on Scientific and Statistical ComputingMultimedia SystemsPerformance EvaluationJournal of Visual Communication and Image Representation Leading:Computers & EducationACM Trans on Mathematical SoftwareHypermediaIntl Jnl of Modelling & SimulationIntl Jnl of Shape ModellingIntl Jnl on Computational Geometry & AppsSimulation & GamesSimulation & GamingVisual ComputerComputational Geometry - Theory and ApplicationsSimulationMultimedia Tools & ApplicationsIntl Jnl in Computer SimulationIntegrated Computer-Aided EngineeringInformation RetrievalReputable:Computer Graphics Forum: Jnl of the Europ As. for CGComputer & GraphicsIntl Jnl of Applied Software TechnologyJnl of Computational and Applied MathematicsMathematical and Computer ModellingMathematics and Computers in SimulationInternational Journal of Computer MathematicsSimulation Practice and TheoryThe New Review of Hypermedia & Multimedia: Apps & ResTrans of the Intl Assoc for Math and Comps in SimulnComputer Simulation: Modeling & AnalysisTrans of the Society for Computer SimulationJournal of Visual Languages and ComputingEngineering ComputationsSoftware - Concepts and ToolsJournal of Visualization and Computer AnimationThe International Journal of The Eurographics Association Others:Iranian Journal of Electrical and Computer EngineeringJournal of Digital ImagingJournal of Concurrent Engineering: Applications and ResearchDigital Technical JournalInterface Journal of New Music ResearchSPIE Journal of Electronic ImagingThe Journal of Electronic CommerceJournal of Graphics ToolsInternational Journal of Information Processing and Management Journal of Library AutomationThe Journal of Computer Game DesignJournal of DocumentationVirtual Prototyping JournalJournal of Computing and Information TechnologySIAM Journal on Scientific Computing.Journal of Computer Aided SurgeryJournal of Computer-Aided Molecular DesignJournal of the Virtual Reality SocietyJournal of Virtual Reality Research, Development and Applications Journal of Computational and Graphical StatisticsAREA: System TechnologyPremium:IEEE Trans on CommunicationsJnl of Parallel and Distributed ComputingACM Trans on Computer SystemsIEEE/ACM Trans on NetworkingACM Trans on Information SystemsIEEE Trans on Parallel and Distributed SystemsDistributed ComputingIEEE Jnl on Selected Areas in CommunicationInternational Journal of Parallel ProgrammingComputer Networks (Previously "& ISDN Networks")Real-time SystemsLeading:NetworksInternet Research - Electronic Networking Applications and Policy Computer CommunicationsJnl of Computer CommunicationsJnl of Network and Computer ApplicationsParallel ComputingMobile Networks and ApplicationsJnl of High Speed NetworksWireless NetworksQueueing SystemsThe Intl Jnl of Supercomputer ApplicationsConcurrency - Practice and ExperienceIEEE Journal of Internet ComputingACM Transactions on Information and System Security Reputable:Computers & SecurityIEE Proc. - CommunicationsIEICE Trans on Fundamentals of Elec., Comm., and Comp.IEICE Trans on CommunicationsMobile Computing and Communicatons ReviewDistributed Systems Engineering JnlIntl Jnl of High Speed ComputingWorld Wide Web JournalElectronic NetworkingIntl Jnl of Communication SystemsSignal Processing: Image CommunicationTelecommunication SystemsInternational Journal of Systems ScienceJournal of CryptographyInternet Security JournalJournal of Networks and Systems ManagementInternational Journal of Wireless Information NetworksAn Interactive Journal of Mobile ComputingOthers:Information & Systems TechnologyJnl of Computer SecurityParallel Processing LettersIBM Systems JournalThe International Journal of Computer and Telecommunications Networking USENIX Computing Systems JournalAT&T Technical JournalJournal of Internetworking: Research and ExperienceFujitsu Scientific and Technical JournalComputer Music JournalJournal of Computer-based InstructionICL Technical JournalInternational Journal of Imaging Systems and TechnologyInternational Journal of Digital and Analog Communication SystemJournal of Supercomputer Application of High Performance ComputingJournal of Decision Support SystemsJournal of Computer Research and DevelopmentBritish Telecommunications Technology JournalThe Journal of Interactive Media and EducationInternational Journal of Time-Critical Computing SystemsInternational. Journal of CAD/ CAM & Comp. GraphicsEducational Communication and Technology JournalThe International Journal on the Development and Application of Standards for Computers, Data Communications and InterfacesJournal of Electronic PublishingWeb JournalMicrosoft Systems JournalJournal of Data & Computer CommunicationJournal of SupercomputingJournal of Imaging TechnologyINESC Journal on Junior Activities in Science and Technology The Journal of Electronic Data InterchangeISPRS Journal of Photogrammetry and Remote SensingInternational Journal of CIMInternational Journal of Circuit Theory and ApplicationsJournal of Digital SystemsIVHS JournalJournal Design Automation and Fault Tolerant ComputingJournal of the American Voice I/O SocietyJournal of the Communications Research LabInternational Journal of Construction information Technology Journal of Computer Communication ReviewReal-Time Imaging JournalJournal of Lightwave TechnologyJournal of Advanced Automation TechnologyJournal of Technology TransferTI Technical JournalJournal of Imaging Science and TechnologyJournal of Mathematical Imaging and VisionThe journal of the Interactive Multimedia AssociationTransputer Communication JournalBell System Technical JournalJournal of Combinatorial DesignsJournal of Combinatorial OptimizationJournal of Reliable ComputingJournal of Digital Library ResearchAREA: General Computer Science and Related Journals Premium:Jnl of the American Medical Informatics Assoc (JAMIA)Journal of Combinatorial Theory (A & B)CombinatoricaSIAM Jnl on Discrete MathematicsOperations ResearchLeading:Communications of the ACMACM Computing SurveysProcs of the IEEEInformation Processing LettersIBM Journal of R & DComputers in Biomedical ResearchMethods of Information in MedicineMedical Decision MakingInternational Journal of General SystemsAdaptive BehaviorDiscrete Applied MathematicsDiscrete MathematicsJournal of Computational BiologyJournal of Molecular BiologyBioinformaticsReputable:Computer JournalComputer Applications in the BioSciences: CABIOS ComputingFuzzy Sets and SystemsIBS Computing QuarterlyInformation SciencesIntl Jnl of Computer Applications in TechnologyIntl Jnl of Computer System Science & EngineeringMedical InformaticsInternational Journal of Medical InformaticsJnl of Computer Science and TechnologyJnl of Computing and InformationJnl of Information ProcessingAustralian Computer JnlJournal on Universal Computer ScienceIEICE Trans on Infomation and SystemsComputer Methods and Programs in BiomedicineComputers in Biology and MedicineFinite Fields and their ApplicationsInstructional Science。
Computer vision( Wikipedia)(计算机视觉)
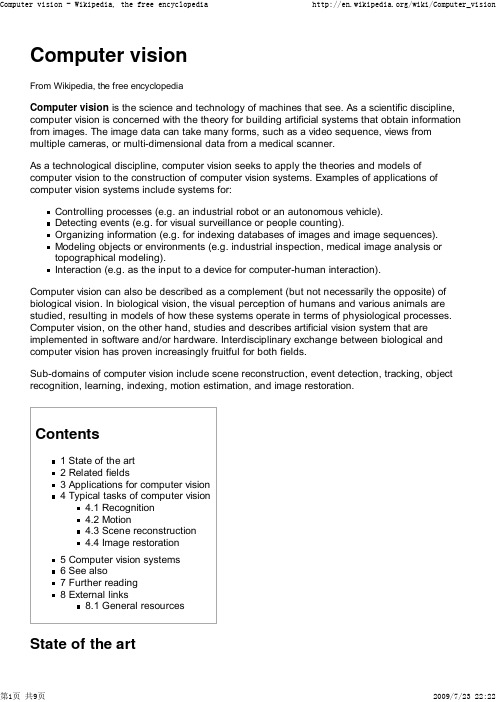
A third field which plays an important role is neurobiology, specifically the study of the biological vision system. Over the last century, there has been an extensive study of eyes, neurons, and the brain structures devoted to processing of visual stimuli in both humans and various animals. This has led to a coarse, yet complicated, description of how "real" vision systems operate in order to solve certain vision related tasks. These results have led to a subfield within computer vision where artificial systems are designed to mimic the processing and behaviour of biological systems, at different levels of complexity. Also, some of the learning-based methods developed within computer vision have their background in biology.Yet another field related to computer vision is signal processing. Many methods for processing of one-variable signals, typically temporal signals, can be extended in a natural way to processing of two-variable signals or multi-variable signals in computer vision. However, because of the specific nature of images there are many methods developed within computer vision which have no counterpart in the processing of one-variable signals. A distinct character of these methods is the fact that they are non-linear which, together with the multi-dimensionality of the signal, defines a subfield in signal processing as a part of computer vision.Beside the above mentioned views on computer vision, many of the related research topics can also be studied from a purely mathematical point of view. For example, many methods in computer vision are based on statistics, optimization or geometry. Finally, a significant part of the field is devoted to the implementation aspect of computer vision; how existing methods can be realized in various combinations of software and hardware, or how these methods can be modified in order to gain processing speed without losing too much performance.The fields most closely related to computer vision are image processing, image analysis, robot vision and machine vision. There is a significant overlap in the range of techniques and applications that these cover. This implies that the basic techniques that are used and developed in these fields are more or less identical, something which can be interpreted as there is only one field with different names. On the other hand, it appears to be necessary for research groups, scientific journals, conferences and companies to present or market themselves as belonging specifically to one of these fields and, hence, various characterizations which distinguish each of the fields from the others have been presented.The following characterizations appear relevant but should not be taken as universally accepted: Image processing and image analysis tend to focus on 2D images, how to transform one image to another, e.g., by pixel-wise operations such as contrast enhancement, localoperations such as edge extraction or noise removal, or geometrical transformations such as rotating the image. This characterization implies that image processing/analysis neither require assumptions nor produce interpretations about the image content.Computer vision tends to focus on the 3D scene projected onto one or several images, e.g., how to reconstruct structure or other information about the 3D scene from one or several images. Computer vision often relies on more or less complex assumptions about the scene depicted in an image.Machine vision tends to focus on applications, mainly in industry, e.g., vision basedautonomous robots and systems for vision based inspection or measurement. This implies that image sensor technologies and control theory often are integrated with the processing of image data to control a robot and that real-time processing is emphasized by means of efficient implementations in hardware and software. It also implies that the externalconditions such as lighting can be and are often more controlled in machine vision thanthey are in general computer vision, which can enable the use of different algorithms.There is also a field called imaging which primarily focus on the process of producingexploration is already being made with autonomous vehicles using computer vision, e. g., NASA's Mars Exploration Rover.Other application areas include:Support of visual effects creation for cinema and broadcast, e.g., camera tracking(matchmoving).Surveillance.Typical tasks of computer visionEach of the application areas described above employ a range of computer vision tasks; more or less well-defined measurement problems or processing problems, which can be solved using a variety of methods. Some examples of typical computer vision tasks are presented below.RecognitionThe classical problem in computer vision, image processing and machine vision is that of determining whether or not the image data contains some specific object, feature, or activity. This task can normally be solved robustly and without effort by a human, but is still not satisfactorily solved in computer vision for the general case: arbitrary objects in arbitrary situations. The existing methods for dealing with this problem can at best solve it only for specific objects, such as simple geometric objects (e.g., polyhedrons), human faces, printed or hand-written characters, or vehicles, and in specific situations, typically described in terms of well-defined illumination, background, and pose of the object relative to the camera.Different varieties of the recognition problem are described in the literature:Recognition: one or several pre-specified or learned objects or object classes can berecognized, usually together with their 2D positions in the image or 3D poses in the scene.Identification: An individual instance of an object is recognized. Examples: identification ofa specific person's face or fingerprint, or identification of a specific vehicle.Detection: the image data is scanned for a specific condition. Examples: detection ofpossible abnormal cells or tissues in medical images or detection of a vehicle in anautomatic road toll system. Detection based on relatively simple and fast computations is sometimes used for finding smaller regions of interesting image data which can be further analyzed by more computationally demanding techniques to produce a correctinterpretation.Several specialized tasks based on recognition exist, such as:Content-based image retrieval: finding all images in a larger set of images which have a specific content. The content can be specified in different ways, for example in terms ofsimilarity relative a target image (give me all images similar to image X), or in terms ofhigh-level search criteria given as text input (give me all images which contains manyhouses, are taken during winter, and have no cars in them).Pose estimation: estimating the position or orientation of a specific object relative to the camera. An example application for this technique would be assisting a robot arm inretrieving objects from a conveyor belt in an assembly line situation.Optical character recognition (or OCR): identifying characters in images of printed orhandwritten text, usually with a view to encoding the text in a format more amenable toediting or indexing (e.g. ASCII).MotionSeveral tasks relate to motion estimation, in which an image sequence is processed to produce an estimate of the velocity either at each points in the image or in the 3D scene. Examples of such tasks are:Egomotion: determining the 3D rigid motion of the camera.Tracking: following the movements of objects (e.g. vehicles or humans).Scene reconstructionGiven one or (typically) more images of a scene, or a video, scene reconstruction aims at computing a 3D model of the scene. In the simplest case the model can be a set of 3D points. More sophisticated methods produce a complete 3D surface model.Image restorationThe aim of image restoration is the removal of noise (sensor noise, motion blur, etc.) from images. The simplest possible approach for noise removal is various types of filters such as low-pass filters or median filters. More sophisticated methods assume a model of how the local image structures look like, a model which distinguishes them from the noise. By first analysing the image data in terms of the local image structures, such as lines or edges, and then controlling the filtering based on local information from the analysis step, a better level of noise removal is usually obtained compared to the simpler approaches.Computer vision systemsThe organization of a computer vision system is highly application dependent. Some systems are stand-alone applications which solve a specific measurement or detection problem, while other constitute a sub-system of a larger design which, for example, also contains sub-systems for control of mechanical actuators, planning, information databases, man-machine interfaces, etc. The specific implementation of a computer vision system also depends on if its functionality ispre-specified or if some part of it can be learned or modified during operation. There are, however, typical functions which are found in many computer vision systems.Image acquisition: A digital image is produced by one or several image sensors, which, besides various types of light-sensitive cameras, include range sensors, tomographydevices, radar, ultra-sonic cameras, etc. Depending on the type of sensor, the resultingimage data is an ordinary 2D image, a 3D volume, or an image sequence. The pixel values typically correspond to light intensity in one or several spectral bands (gray images orcolour images), but can also be related to various physical measures, such as depth,absorption or reflectance of sonic or electromagnetic waves, or nuclear magneticresonance.Pre-processing: Before a computer vision method can be applied to image data in order to extract some specific piece of information, it is usually necessary to process the data inorder to assure that it satisfies certain assumptions implied by the method. Examples are Re-sampling in order to assure that the image coordinate system is correct.Noise reduction in order to assure that sensor noise does not introduce falseinformation.Contrast enhancement to assure that relevant information can be detected.Scale-space representation to enhance image structures at locally appropriate scales.Feature extraction: Image features at various levels of complexity are extracted from theimage data. Typical examples of such features areLines, edges and ridges.Localized interest points such as corners, blobs or points.More complex features may be related to texture, shape or motion.Detection/Segmentation: At some point in the processing a decision is made about which image points or regions of the image are relevant for further processing. Examples are Selection of a specific set of interest pointsSegmentation of one or multiple image regions which contain a specific object ofinterest.High-level processing: At this step the input is typically a small set of data, for example a set of points or an image region which is assumed to contain a specific object. Theremaining processing deals with, for example:Verification that the data satisfy model-based and application specific assumptions.Estimation of application specific parameters, such as object pose or object size.Classifying a detected object into different categories.See alsoActive visionArtificial intelligence Digital image processing Image processing List of computer visiontopicsMachine learningMachine visionMachine Vision GlossaryMedical imagingPattern recognitionTopological data analysisFurther readingSorted alphabetically with respect to first author's family namePedram Azad, Tilo Gockel, Rüdiger Dillmann (2008). Computer Vision - Principles andPractice. Elektor International Media BV. ISBN 0905705718. /book.html.Dana H. Ballard and Christopher M. Brown (1982). Computer Vision. Prentice Hall. ISBN 0131653164. /rbf/BOOKS/BANDB/bandb.htm.Wilhelm Burger and Mark J. Burge (2007). Digital Image Processing: An AlgorithmicApproach Using Java. Springer. ISBN 1846283795 and ISBN 3540309403./.James L. Crowley and Henrik I. Christensen (Eds.) (1995). Vision as Process. Springer-Verlag. ISBN 3-540-58143-X and ISBN 0-387-58143-X.E. Roy Davies (2005). Machine Vision : Theory, Algorithms, Practicalities. MorganKaufmann. ISBN 0-12-206093-8.Olivier Faugeras (1993). Three-Dimensional Computer Vision, A Geometric Viewpoint. MIT Press. ISBN 0-262-06158-9.R. Fisher, K Dawson-Howe, A. Fitzgibbon, C. Robertson, E. Trucco (2005). Dictionary of Computer Vision and Image Processing. John Wiley. ISBN 0-470-01526-8.David A. Forsyth and Jean Ponce (2003). Computer Vision, A Modern Approach. Prentice Hall. ISBN 0-12-379777-2.Gösta H. Granlund and Hans Knutsson (1995). Signal Processing for Computer Vision.Kluwer Academic Publisher. ISBN 0-7923-9530-1.Richard Hartley and Andrew Zisserman (2003). Multiple View Geometry in Computer Vision.Cambridge University Press. ISBN 0-521-54051-8.Berthold Klaus Paul Horn (1986). Robot Vision. MIT Press. ISBN 0-262-08159-8.Fay Huang, Reinhard Klette and Karsten Scheibe (2008). Panoramic Imaging - Sensor-Line Cameras and Laser Range-Finders. Wiley. ISBN 978-0-470-06065-0.Bernd Jähne and Horst Haußecker (2000). Computer Vision and Applications, A Guide for Students and Practitioners. Academic Press. ISBN 0-13-085198-1.Bernd Jähne (2002). Digital Image Processing. Springer. ISBN 3-540-67754-2.Reinhard Klette, Karsten Schluens and Andreas Koschan (1998). Computer Vision - Three-Dimensional Data from Images. Springer, Singapore. ISBN 981-3083-71-9.Tony Lindeberg (1994). Scale-Space Theory in Computer Vision. Springer. ISBN0-7923-9418-6. http://www.nada.kth.se/~tony/book.html.David Marr (1982). Vision. W. H. Freeman and Company. ISBN 0-7167-1284-9.Gérard Medioni and Sing Bing Kang (2004). Emerging Topics in Computer Vision. Prentice Hall. ISBN 0-13-101366-1.Tim Morris (2004). Computer Vision and Image Processing. Palgrave Macmillan. ISBN0-333-99451-5.Nikos Paragios and Yunmei Chen and Olivier Faugeras (2005). Handbook of Mathematical Models in Computer Vision. Springer. ISBN 0-387-26371-3.Azriel Rosenfeld and Avinash Kak (1982). Digital Picture Processing. Academic Press. ISBN 0-12-597301-2.Linda G. Shapiro and George C. Stockman (2001). Computer Vision. Prentice Hall. ISBN 0-13-030796-3.Milan Sonka, Vaclav Hlavac and Roger Boyle (1999). Image Processing, Analysis, andMachine Vision. PWS Publishing. ISBN 0-534-95393-X.Emanuele Trucco and Alessandro Verri (1998). Introductory Techniques for 3-D Computer Vision. Prentice Hall. ISBN 0132611082.External linksGeneral resourcesKeith Price's Annotated Computer Vision Bibliography (/Vision-Notes/bibliography/contents.html) and the Official Mirror Site Keith Price's AnnotatedComputer Vision Bibliography (/bibliography/contents.html)USC Iris computer vision conference list (/Information/Iris-Conferences.html)Retrieved from "/wiki/Computer_vision"Categories: Artificial intelligence | Computer visionThis page was last modified on 30 June 2009 at 03:36.Text is available under the Creative Commons Attribution/Share-Alike License; additional terms may apply. See Terms of Use for details.Wikipedia® is a registered trademark of the Wikimedia Foundation, Inc., a non-profitorganization.。
计算机视觉领域的letter
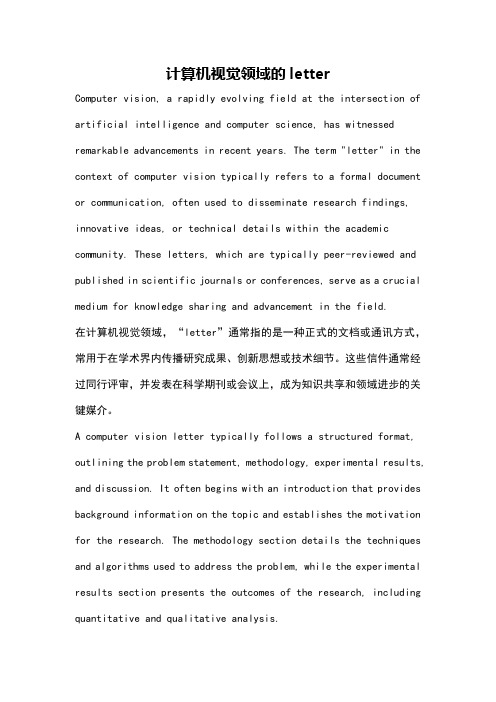
计算机视觉领域的letterComputer vision, a rapidly evolving field at the intersection of artificial intelligence and computer science, has witnessed remarkable advancements in recent years. The term "letter" in the context of computer vision typically refers to a formal document or communication, often used to disseminate research findings, innovative ideas, or technical details within the academic community. These letters, which are typically peer-reviewed and published in scientific journals or conferences, serve as a crucial medium for knowledge sharing and advancement in the field.在计算机视觉领域,“letter”通常指的是一种正式的文档或通讯方式,常用于在学术界内传播研究成果、创新思想或技术细节。
这些信件通常经过同行评审,并发表在科学期刊或会议上,成为知识共享和领域进步的关键媒介。
A computer vision letter typically follows a structured format, outlining the problem statement, methodology, experimental results, and discussion. It often begins with an introduction that provides background information on the topic and establishes the motivation for the research. The methodology section details the techniques and algorithms used to address the problem, while the experimental results section presents the outcomes of the research, including quantitative and qualitative analysis.计算机视觉领域的信件通常采用结构化格式,概述问题陈述、方法、实验结果和讨论。
Computer-Vision计算机视觉英文ppt
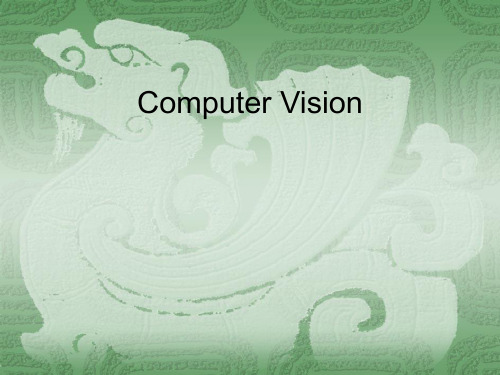
Its mainstream research is divided into three stages:
Stage 1: Research on the visual basic method ,which take the model world as the main object;
Stage 2: Research on visual model ,which is based on the computational theory;
the other is to rebuild the three dimensional object according to the two-dimensional projection images .
History of computer vision
1950s: in this period , statistical pattern recognition is most applied in computer vision , it mainly focuse on the analysis and identification of two-dimensional image,such as: optical character recognition, the surface of the workpiece, the analysis and interpretation of the aerial image.
2024年华为人工智能方向HCIA考试复习题库(含答案)
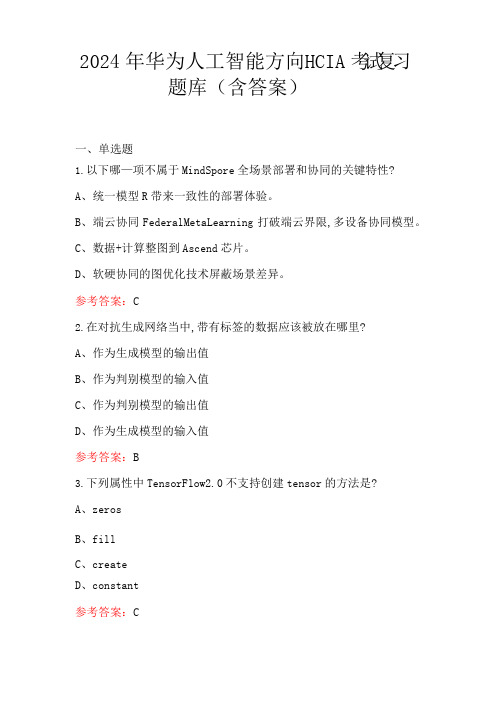
A、Dropout
B、正则化 C、梯度下降 D、交叉验证 参考答案:C 33.以下哪个不是图像识别服务的应用? A、目标检测 B、智能相册 C、场景分析 D、语音合成 参考答案:D 34.以下关于机器学习描述正确的是? A、深度学习是机器学习的一个分支
C、空字符 D、recapture 参考答案:C 49.以下列哪一项不属于语音识别场景的应用? A、会议记录
B、电话回访 C、口语测评 D、人脸识别 E、智能音箱 参考答案:D 50.tensorFlow2.0 中可用于张量合并的方法有?
A、split B、join C、concat D、unstack
A、图方法
B、源码转换 C、运算符重载 参考答案:B 16.梯度下降算法中,损失函数曲面上轨迹最混乱的算法是以下哪种 算法?
A、SGD B、BGD C、MGD D、MBGD
参考答案:A 17.长短记忆网络是基于循环神经网络发展而来的,长短神经网络主 要解决了循环神经网路的什么问题?
A、过拟合问题 B、梯度爆炸问题 C、欠拟合问题 D、梯度消失问题 参考答案:D 18.以下哪一项不是 ModelArts 中训练平台的主要任务? A、算法开发 B、收集数据 C、模型评估 D、模型可视化
2024 年华为人工智能方向HCIA 考试复习 题库(含答案)
一、单选题 1.以下哪—项不属于 MindSpore 全场景部署和协同的关键特性? A、统一模型 R 带来一致性的部署体验。 B、端云协同 FederalMetaLearning 打破端云界限,多设备协同模型。 C、数据+计算整图到 Ascend 芯片。 D、软硬协同的图优化技术屏蔽场景差异。 参考答案:C 2.在对抗生成网络当中,带有标签的数据应该被放在哪里? A、作为生成模型的输出值 B、作为判别模型的输入值 C、作为判别模型的输出值 D、作为生成模型的输入值 参考答案:B 3.下列属性中 TensorFlow2.0 不支持创建 tensor 的方法是? A、zeros B、fill C、create D、constant 参考答案:C
顶级期刊排名

顶级期刊排名Get:小虫 21:07:18/doc/878268801.html/loi/coli小虫 21:07:40/doc/878268801.html/science?_ob=PublicationURL&_tockey=%23TOC%2356 17%232010%23998259981%232520777%23FLP%23&_cdi=5617 &_pubType=J&_auth=y&_acct=C000050165&_version=1&_urlVersion=0&_userid=1002903& md5=fa794527d6fa620604 73b0b181eb1ab4小虫 21:07:46/doc/878268801.html/RankList?entitytype=4&domainID=5&last=0&start=1&end=100小虫 21:07:54/doc/878268801.html/citation.cfm?id=J1206&picked=prox小虫 21:08:00/doc/878268801.html/xpl/RecentIssue.jsp?punumber=9670小虫 21:08:08/doc/878268801.html/doi/10.1111/coin.2010.26.issue-4/issuetocAREA: Artificial IntelligencePremium:Artificial IntelligenceComputational LinguisticsIEEE Trans on Pattern Analysis and Machine IntlIEEE Trans on Robotics and AutomationIEEE Trans on Image ProcessingJournal of AI ResearchNeural ComputationMachine LearningIntl Jnl of Computer V isionIEEE Trans on Neural NetworksLeading:Artificial Intelligence ReviewACM Transactions on Asian Language Information ProcessingAI MagazineAnnals of Mathematics and AIApplied Artificial IntelligenceApplied IntelligenceArtificial Intelligence in Medic ineAutonomous Agents and Multi-Agent SystemsComputational IntelligenceComplex SystemsComputer Speech and LanguageComputer Support for Collaborative Learning (CSCL)Computer V ision and Image UnderstandingConnection ScienceCVGIP: Graphical Models & Image Processing CVGIP: Image UnderstandingExpert Systems with Applications: An Intl JnlIEEE Transaction on Speech and Audio ProcIEEE Trans on Systems, Man, & Cybernetics, Part A & B Intl Jnl on Artificial Intelligence ToolsJnl of Experimental & Theoretical AIJournal of East Asian LinguisticsKnowledge Engineering ReviewMachine TranslationNeural NetworksNetwork Computing in Neural SystemsPattern Analysis and ApplicationsPattern RecognitionNeurocomputingUser Modelling & User-Adapted Interaction: an Intl JnlReputable:Communications of COLIPSComputer Processing of Chinese & Oriental Languages Computers and Artificial IntelligenceCybernetics and SystemsEngineering Intelligent Systems for EE and CSExpert SystemsEvolutionary ComputationIntelligent Instruments & ComputersIntl Jnl for AI in EngineeringIntl Jnl of Applied Expert SystemsIntl Jnl of Approximate ReasoningIntl Jnl of Intelligent SystemsIntl Jnl of Neural SystemsIntl Jnl of Pattern Recognition & AIIntl Journal of Document Analysis and Recognition IEEE Transactions on Fuzzy SystemsJournal of Intelligent and Fuzzy SystemsKnowledge Acquisition JnlKnowledge-Based SystemsKybernetikaNatural Language EngineeringNeural Computing & ApplicationsNetwork SocietyNeural Processing LettersPattern Recognition LettersIEE Proceedings: Vision, Image and Signal Proc Speech CommunicationsJournal of Neural Network ComputingMinds and Machines: Jnl for AI, Philosopy and Cog. ScIntl Jnl of Uncertainty, Fuzziness and KBSHeuristics: Jnl of Knowledge EngineeringEngineering Applications of AIJnl. of Japanese Soc. of AIAustralian Jnl of Intelligent Information Proc SysIntelligent Data AnalysisImage and V ision ComputingJournal of Artificial Neural NetworksNeural, Parallel and Scientific ComputationsRoboticaOthers:AIAA JournalCanadian Artificial IntelligenceJournal of Advanced RoboticsJournal of Artificial Intelligence in EducationJournal of Artificial Intelligence in Engineering, Automation, and Manufacturing Journal of Artificial Intelligence, Neural Networks and ComplexJournal of Computational AcousticsJournal of Computational NeuroscienceJournal of Computational VisionJournal of Card. ImagingJournal of CyberneticsJournal of Cybernetics and Information ScienceJournal of Design Automation of Embedded SystemsJournal of Knowledge-Based Intelligent Engineering Systems Journal of Intelligent Robotic Systems: Theory and ApplicationsJournal of Systems Automation: Research and Applications Journal of Automation and Remote ControlJournal of Intelligent Automation & Soft ComputingJournal of Intelligent Control and SystemsJournal of Intelligent and Robotic SystemsJournal of Intelligent ManufacturingJournal of Intelligent Systems EngineeringJournal of Intelligence SystemJournal of Intelligent TechnologyJournal of Literary and Linguistic ComputingJournal of Machine V ision and ApplicationsJournal of Man-Machine StudiesJournal on Neural and Mass-Parallel Computing and Information Systems Journal of Robotics and Mechatronics Journal of Robotic SystemsJournal of Robotics and Autonomous SystemsJournal of Robotics ResearchJournal of the Robotics Society of JapanJournal of Computational NeurologyInternational Journal of LexicographyJournal of intelligent ComputingInternational Journal of Intelligent Systems in Accounting Finance and Management International Journal of SpeechTechnologyEngineering Design and Automation JournalInternational Journal of Machine Tools & ManufacturingInternational Journal of Corpus LinguisticsJournal of Chinese Information ProcessingAREA: Algorithms, Theory and Related AreasPremium:AlgorithmicaComputational ComplexityDiscrete & Computational GeometryIEEE Trans on Information TheoryInformation & ComputationJnl of AlgorithmsJnl of Computer and System SciencesJnl of the Association for Computing MachinerySIAM Jnl on ComputingACM Trans on Computational Logic (TOCL)Leading:Acta InformaticaChicago Journal of Theoretical Computer ScienceDesigns, Codes and CryptographyJnl of Symbolic ComputationJournal of Automated ReasoningJournal of Graph Algorithms and ApplicationsJournal of ComplexityJournal of CryptologyJOTA - J. of Optimization: Theory and ApplicationsMathematics of ComputationMathematical ProgrammingOptimization: A J. of Mathematical Programming andOperations ResearchORSA Journal of ComputingNordic J of Computing (BIT)SIAM Journal on OptimizationRandom Structures & AlgorithmsTheoretical Computer ScienceReputable:Applicable Algebra in Eng., Comm., and ComputingApplied Maths and ComputationBIT: Computer Science and Numerical MathematicsBulletin of the European Assoc. for Theoretical CSComputational and Applied MathsComputers & Mathematics With ApplicationsCombinatorics, Probability & ComputingEuropean Journal of ORJournal of Computer and System Sciences International Intl Jnl of Foundations of Computer ScienceOthers:Problem Solving T echnologiesJournal of Algebraic CombinatoricsJournal of Combinatorial DesignsJournal of Combinatorial OptimizationJournal of Experimental AlgorithmicsJournal of Electronic ImagingIntl Jnl for Numerical Methods in EngineeringSIAM Journal of Algorithms and Discrete MethodsSIAM Journal on Algebraic and Discrete MethodsSIAM Journal on Numerical AnalysisSIAM Journal on Matrix Analysis and ApplicationsNaval Journal of Operations ResearchJournal of SchedulingElectronic Journal of CombinatoricsJournal of Mathematical Modeling and Scientific Computing Journal of Mathematical Structures in Computer ScienceInternational Journal on Mathematical and Computer ModelsJournal of Global OptimizationJournal of Computational Statistics and Data Analysis微软学术搜索总结的人工智能(AI)领域顶级期刊2010-07-08 20:16Publications CitationsA I - Artificial Intelligence2502 96833 J AIR - Journal of Artificial Intelligence Research515 11757 I JRR - International Journal of Robotic Research1394 17018 A IM - AI Magazine1146 13937 A AMAS - Autonomous Agents and Multi-Agent Systems339 5119 A ROBOTS - Autonomous Robots583 7211 A LIFE - Artificial Life440 5637 J AR - Journal of Automated Reasoning766 7982 J IIS - Journal of Intelligent Information Systems448 5312 E XPERT - IEEE Expert / IEEE Intelligent Systems1578 13138 A IR - Artificial Intelligence Review495 4054 A MAI - Annals of Mathematics and Artificial Intelligence848 5688 A AI - Applied Artificial Intelligence809 5076 JETAI - Journal of Experimental and Theoretical Artificial407 3064 IntelligenceA RTMED - Artificial Intelligence in Medicine865 5196 J VCIR- Journal of Visual Communication and Image Representation472 3114 A ICOM - AI Communications422 2526 I JAR - International Journal of Approximate Reasoning1102 4756 I JIS - International Journal of Intelligent Systems828 3718 A IL - Artificial Intelligence and Law268 1304 J ournal of Scientific Computing 610 2171 International Journal of Humanoid Robotics167 473 J ournal of Field Robotics377 716 J IFS - Journal of Intelligent and Fuzzy Systems426 607 R obotica559 461 I JHIS - International Journal of Hybrid Intelligent Systems125 92 IJMTM - International Journal of Manufacturing Technology and447 164 ManagementI JSN - International Journal of Security and Networks114 80A nnual Reviews in Control56 46I JIQ - International Journal of Information Quality35 22A CM SIGSMALL Newsletter23 13I EEE Transactions Automation Science and Engineering64 13I JIA - International Journal of Information Acquisition173 11J OCCH - ACM Journal on Computing and Cultural Heritage18 3A CM SIGMOD Digital Symposium Collection 4 0A CM SIGPC Notes计算机视觉、IP、AI方向的一些顶级会议和期刊收藏计算机视觉方面的三大国际会议是ICCV, CVPR和ECCV,统称之为ICE。
如何成为人工智能工程师英语作文
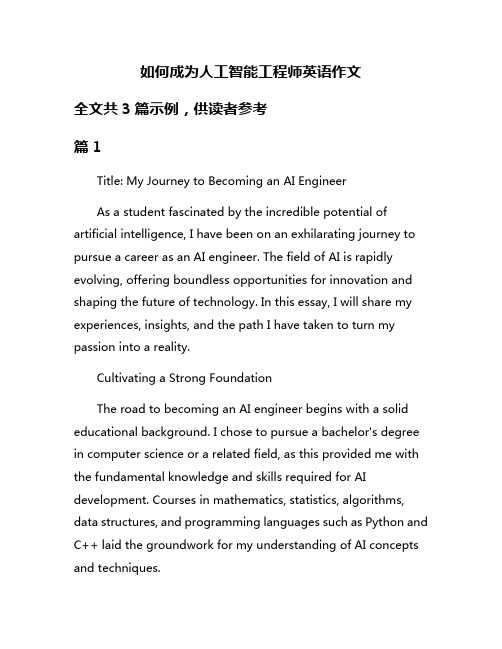
如何成为人工智能工程师英语作文全文共3篇示例,供读者参考篇1Title: My Journey to Becoming an AI EngineerAs a student fascinated by the incredible potential of artificial intelligence, I have been on an exhilarating journey to pursue a career as an AI engineer. The field of AI is rapidly evolving, offering boundless opportunities for innovation and shaping the future of technology. In this essay, I will share my experiences, insights, and the path I have taken to turn my passion into a reality.Cultivating a Strong FoundationThe road to becoming an AI engineer begins with a solid educational background. I chose to pursue a bachelor's degree in computer science or a related field, as this provided me with the fundamental knowledge and skills required for AI development. Courses in mathematics, statistics, algorithms, data structures, and programming languages such as Python and C++ laid the groundwork for my understanding of AI concepts and techniques.However, my learning extended far beyond the classroom. I invested countless hours in self-study, devouring online resources, tutorials, and research papers to stay up-to-date with the latest advancements in AI. The field is constantly evolving, and staying abreast of new developments is crucial for success.Hands-on Experience through Projects and InternshipsTheoretical knowledge alone is insufficient; hands-on experience is equally important. Throughout my academic journey, I actively sought opportunities to apply my learning through personal projects and internships. These practical experiences allowed me to tackle real-world challenges, experiment with different AI techniques, and develop a portfolio showcasing my skills.One of my most rewarding projects involved building a chatbot using natural language processing (NLP) techniques. This project not only deepened my understanding of NLP but also taught me valuable lessons in data preprocessing, model training, and deployment. Additionally, I participated in several AI competitions and hackathons, which pushed me to think critically, work collaboratively, and deliver solutions under time constraints.Internships at AI-focused companies or research labs further enriched my practical experience. Working alongside experienced professionals, I gained insights into industry best practices, project management, and the challenges of implementing AI solutions at scale. These internships also provided me with valuable networking opportunities and potential job prospects upon graduation.Specializing in AI SubfieldsThe field of AI is vast, encompassing numerous subfields such as machine learning, deep learning, computer vision, natural language processing, and robotics. While acquiring a broad understanding of AI is essential, specializing in one or more subfields can be advantageous. By focusing my efforts, I aimed to develop in-depth expertise and become a subject matter expert in my chosen area of interest.For instance, my fascination with computer vision led me to explore techniques like convolutional neural networks (CNNs) and object detection algorithms. I dedicated significant time to studying state-of-the-art models, implementing them in personal projects, and contributing to open-source computer vision libraries. This focused approach not only enhanced myskills but also made me more attractive to potential employers seeking specialized AI talent.Embracing Continuous Learning and ResearchThe field of AI is constantly evolving, with new algorithms, techniques, and frameworks emerging regularly. As an AI engineer, embracing a mindset of continuous learning and staying up-to-date with the latest research is paramount. I made it a habit to regularly attend AI conferences, workshops, and seminars to learn from industry experts and researchers.Additionally, I actively engaged with the AI community through online forums, social media groups, and open-source projects. Collaborating with like-minded individuals, sharing knowledge, and contributing to open-source initiatives not only expanded my understanding but also allowed me to establish a professional network and build a reputation within the AI community.Developing Soft Skills and Interdisciplinary KnowledgeWhile technical skills are undoubtedly crucial for an AI engineer, developing soft skills and interdisciplinary knowledge is equally important. Effective communication, teamwork, and problem-solving abilities are vital for collaborating withcross-functional teams, articulating complex ideas, and translating business requirements into AI solutions.Furthermore, AI increasingly intersects with various domains, such as healthcare, finance, and transportation. Gaining knowledge in these domains can provide valuable context and enable me to develop more impactful and relevant AI applications. By actively seeking opportunities to work on interdisciplinary projects and collaborating with domain experts, I aimed to broaden my perspective and increase my value as an AI professional.Ethical Considerations and Responsible AIAs AI systems become more prevalent and influential, it is essential to consider the ethical implications and potential biases that can arise. Throughout my journey, I made a conscious effort to understand the ethical principles and guidelines surrounding AI development and deployment.I studied topics such as algorithmic bias, privacy and data protection, transparency and accountability, and the societal impact of AI. By incorporating these considerations into my projects and decision-making processes, I aimed to develop AI solutions that are not only technically sophisticated but also ethical, responsible, and aligned with societal values.ConclusionBecoming an AI engineer is a challenging yet rewarding pursuit. It requires a combination of technical expertise, practical experience, continuous learning, and a commitment to ethical and responsible AI development. My journey has been filled with countless hours of study, hands-on projects, internships, and a relentless pursuit of knowledge.While the path has not been easy, the opportunities and potential impact of AI continue to inspire and motivate me. As I embark on my professional career, I remain committed to pushing the boundaries of what is possible with AI, contributing to groundbreaking innovations, and shaping a future where AI is a powerful force for positive change.篇2How to Become an AI EngineerArtificial Intelligence (AI) is rapidly transforming the world we live in, from the way we communicate and access information to how we work and solve problems. As a student fascinated by this cutting-edge technology, I have been exploring the path to becoming an AI engineer – a profession that promises to be both intellectually stimulating and highly rewarding.AI engineers are the masterminds behind the development and implementation of intelligent systems that can perceive, learn, reason, and take actions to achieve specific goals. They combine their knowledge of computer science, mathematics, and data analysis to create algorithms that enable machines to mimic human-like intelligence and decision-making capabilities. As the demand for AI solutions continues to surge across various industries, the role of an AI engineer has become increasingly crucial.The journey to becoming an AI engineer is a challenging yet exhilarating one, requiring a unique blend of technical skills, problem-solving abilities, and an unwavering passion for innovation. In this essay, I will share my insights and recommendations for aspiring AI engineers, based on extensive research and discussions with professionals in the field.Education and Academic Preparation:The foundation for a career in AI engineering begins with a strong academic background in computer science, mathematics, and statistics. Many universities now offer specialized programs in AI, machine learning, or data science, which provide students with a comprehensive understanding of the theoretical and practical aspects of these disciplines.Pursuing a bachelor's degree in computer science, with a focus on courses such as algorithms, data structures, programming languages, and computer architecture, is a common starting point. Additionally, taking elective courses in areas like machine learning, neural networks, and natural language processing can provide valuable exposure toAI-specific concepts.For those seeking a more in-depth understanding and advanced training, pursuing a master's degree or a doctoral program in AI, machine learning, or a related field can be highly beneficial. These graduate-level programs delve into the latest research and techniques, equipping students with the specialized knowledge and skills required to tackle complex AI challenges.Building a Strong Technical Skillset:Alongside a robust academic foundation, aspiring AI engineers must cultivate a diverse set of technical skills. Proficiency in programming languages such as Python, Java,C++, and R is essential, as these languages are widely used in AI development and implementation.Understanding and applying machine learning algorithms, including supervised and unsupervised learning techniques, is acritical component of an AI engineer's toolkit. Mastering popular machine learning frameworks and libraries, such as TensorFlow, Keras, PyTorch, and scikit-learn, will significantly enhance the ability to develop and deploy AI models effectively.Additionally, expertise in data analysis, data manipulation, and data visualization is crucial, as AI systems heavily rely on large volumes of data for training and optimization. Familiarity with tools like Pandas, NumPy, and Matplotlib can aid in data preprocessing, exploration, and visualization tasks.Hands-on Experience and Project-Based Learning:Theoretical knowledge alone is not sufficient to excel as an AI engineer. Practical experience through internships, personal projects, and participation in coding challenges or competitions is invaluable for honing skills and gaining real-world exposure.Internships at companies or research labs specializing in AI can provide invaluable opportunities to work on real-world problems, collaborate with experienced professionals, and gain insights into industry best practices. Additionally, personal projects focused on developing AI applications, such as chatbots, image recognition systems, or predictive models, candemonstrate initiative, creativity, and a deep understanding of AI concepts.Participating in coding challenges, hackathons, or online competitions related to AI and machine learning can also be highly beneficial. These events not only allow for the application and testing of skills but also foster collaboration,problem-solving abilities, and exposure to diverse perspectives and approaches.Continuous Learning and Professional Development:The field of AI is rapidly evolving, with new techniques, frameworks, and applications emerging constantly. As an AI engineer, embracing a mindset of continuous learning and professional development is crucial to staying ahead of the curve.Attending conferences, workshops, and seminars focused on AI and machine learning can provide invaluable opportunities to learn from industry experts, network with peers, and stay informed about the latest trends and advancements. Additionally, actively participating in online communities, forums, and discussion groups can facilitate knowledge sharing and exposure to diverse perspectives.Pursuing certifications or specialized training programs offered by industry leaders or educational institutions can further enhance professional credibility and expertise. These programs often cover emerging topics, advanced techniques, and best practices, enabling AI engineers to stay relevant and competitive in the job market.Collaboration and Communication Skills:While technical proficiency is paramount, successful AI engineers must also possess strong collaboration and communication skills. AI projects often involve interdisciplinary teams comprising data scientists, software engineers, domain experts, and stakeholders from various backgrounds.Effective communication is essential for articulating complex technical concepts, conveying insights derived from data analysis, and collaborating seamlessly with team members. AI engineers should be adept at presenting their work, discussing challenges, and actively seeking feedback to improve their solutions.Furthermore, the ability to bridge the gap between technical and non-technical stakeholders is crucial. AI engineers must be able to translate complex algorithms and technical jargon intoclear and accessible language, enabling effectivedecision-making and fostering trust in AI solutions.Ethical Considerations and Responsible AI:As AI systems become increasingly integrated into various aspects of our lives, it is essential to consider the ethical implications and potential risks associated with their development and deployment. AI engineers must be aware of the ethical principles and guidelines governing the responsible use of AI technologies.Understanding the concepts of fairness, accountability, transparency, and privacy in AI systems is crucial to mitigating potential biases, ensuring ethical decision-making, and protecting individual rights. AI engineers should be actively involved in discussions and initiatives aimed at promoting responsible AI practices, fostering trust in the technology, and addressing societal concerns.In conclusion, becoming an AI engineer is a rewarding and challenging journey that requires a strong foundation in computer science, mathematics, and statistics, coupled with a diverse set of technical skills, practical experience, and a commitment to continuous learning. By embracing collaboration, effective communication, and ethical considerations, aspiring AIengineers can contribute to the development of intelligent systems that positively impact individuals, businesses, and society as a whole.篇3Title: The Path to Becoming an AI EngineerAs a student passionate about the fascinating field of artificial intelligence (AI), I have spent countless hours researching and dreaming about the possibility of pursuing a career as an AI engineer. The rapid advancements in this domain have captured my imagination, and I am determined to contribute to the development of intelligent systems that can revolutionize various aspects of our lives.To embark on this exhilarating journey, one must possess a strong foundation in computer science, mathematics, and statistics. These core disciplines form the backbone of AI, enabling us to understand and manipulate the complex algorithms and models that drive intelligent systems.Computer science provides the fundamental principles of programming, data structures, and algorithms, which are essential for building and optimizing AI applications. A firm grasp of programming languages such as Python, Java, and C++is crucial, as they serve as the tools for implementing AI solutions. Additionally, knowledge of computer architecture and operating systems is invaluable, as it allows for efficient utilization of hardware resources.Mathematics plays a pivotal role in AI, particularly in areas like linear algebra, calculus, and probability theory. Linear algebra forms the foundation for understanding and manipulating high-dimensional data, which is prevalent in AI applications such as computer vision and natural language processing. Calculus is indispensable for optimizing complex models and understanding the behavior of neural networks. Probability theory, on the other hand, underpins many AI techniques, including Bayesian inference and decision-making under uncertainty.Statistics is another vital component of AI, as it enables us to extract meaningful insights from data and make accurate predictions. Concepts like hypothesis testing, regression analysis, and data visualization are invaluable for understanding and interpreting the results of AI models. Furthermore, a strong grasp of machine learning techniques, such as supervised and unsupervised learning, reinforcement learning, and deeplearning, is essential for building intelligent systems capable of learning from data and making data-driven decisions.Beyond the technical skills, aspiring AI engineers must cultivate a deep understanding of the ethical implications and societal impacts of AI. As AI systems become increasingly integrated into various aspects of our lives, it is crucial to address issues such as privacy, bias, and the potential for misuse or unintended consequences. Ethical considerations should be at the forefront of AI development, ensuring that these powerful technologies are harnessed for the betterment of humanity.To gain practical experience and hone my skills, I plan to actively participate in AI-related projects, competitions, and internships. Hands-on experience is invaluable in this field, as it allows me to apply theoretical concepts to real-world problems and develop a deeper understanding of the challenges and intricacies involved in building AI systems.Furthermore, I intend to actively engage with the AI community by attending conferences, workshops, and meetups. This will not only provide me with opportunities to learn from experts in the field but also enable me to network withlike-minded individuals and stay abreast of the latest developments and emerging trends in AI.The path to becoming an AI engineer is undoubtedly challenging, but the rewards are immense. As an AI engineer, I will have the opportunity to push the boundaries of what is possible, solving complex problems and creating intelligent systems that can positively impact various domains, such as healthcare, finance, transportation, and beyond.Imagine developing AI-powered medical diagnostic tools that can accurately detect diseases at early stages, potentially saving countless lives. Or envision creating intelligent transportation systems that can optimize traffic flow, reducing congestion and emissions. The possibilities are endless, and the potential for making a significant impact on society is truly inspiring.However, it is important to acknowledge that the journey to becoming an AI engineer is not a solitary endeavor. Collaboration and teamwork are essential in this field, as AI projects often require a diverse range of expertise and perspectives. By working alongside experts from various domains, such as domain specialists, data scientists, and software engineers, we can create more robust and effective AI solutions.In conclusion, the road to becoming an AI engineer is paved with challenges and opportunities. It requires a solid foundation in computer science, mathematics, and statistics, coupled with a deep understanding of AI techniques and a commitment to ethical practices. However, with dedication, continuous learning, and a passion for pushing the boundaries of what is possible, the rewards are immense. As an AI engineer, I will have the privilege of shaping the future of intelligent systems and contributing to the development of groundbreaking technologies that can transform our world for the better.。
江苏省南京市第一中学2024届高三上学期暑期阶段性测试英语试题
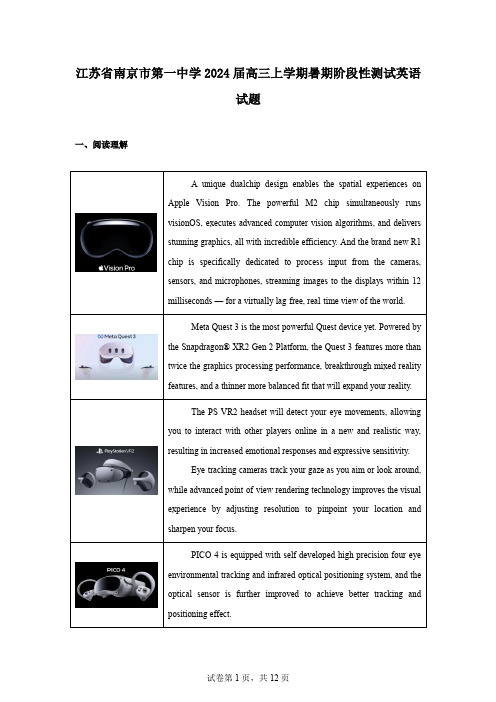
江苏省南京市第一中学2024届高三上学期暑期阶段性测试英语试题一、阅读理解1.Which unique technological feature of the Apple Vision Pro enables an unprecedented mixed reality experience for users?A.Eye-tracking system.B.Dual-chip design.C.Optical sensors.D.High-definition display.2.Which of the following statements accurately compares the capabilities of the four products mentioned?A.Apple Vision Pro is the only one with a dualchip design.B.Meta Quest 3 offers the highest graphics processing performance.C.PS VR2 detects eye movements for online interaction.D.PICO 4 lacks a high-precision tracking system.3.If Simon wanted to buy a product to play a game, which of the following products would be his first choice?A.Apple Vision Pro.B.Meta Quest 3.C.PS VR2.D.PICO 4.“Digital art with iPad and Apple Pencil has helped to expand my creative thinking.”Apichaya "Bim" Wannakit is starting her fourth year in painting, sculpture, and graphic art at Silpakorn University in Bangkok, in the heart of the modern Thai art scene. Growing up in Northeast Thailands creative community in Ubon Ratchatani, Bim developed her passion for art at a young age.She took inspiration from anime like "Dragon Ball Z" cartoons she saw on television and the "Manga" comic books she collected. She put years of hands-on practice into her illustrations using brushes, watercolors, and oil paints. In high school, she discovered even more ways to express herself and expand her creative palette when she started using iPad with Apple Pencil.“I quickly adapted to the precision and flexibility of digital painting using Apple Pencil,” Bim says. “It brought my entire art kit into one magical tool, without sacrificing the natural feel of traditional mediums. It allows me a new way to bring my ideas to life.”When it was time to get ready for university, Bim used iPad to bring her art portfolio together, capturing and storing all her mixed media digitally in iCloud. Bim says, “I initially chose Apple technology because of its cloud service for file management and simple-to-learn design interface — iPad has made my life easier.”Today she uses Procreate on iPad with Apple Pencil for all her creative workflows. Features such as QuickShape and StreamLine with Apple Pencil enable her to quickly add layers, outlines, colors, and shadows in an immersive and playful way. “My love for traditional painting and digital art brings me a new sense of balance. My Apple devices help to make this possible from anywhere.”iPad also supports her daily academic work. Bim uses Apple Pencil with GoodNotes for note-taking, and Microsoft Word on iPad for creating art descriptions. “In class, I use iPad Pro to present my work,” Bim says. “And the 12.9-inch screen is large enough for my professor to view my art comfortably.”“Through my art, I want to tell a story that inspires others and creates a space for experimental ideas.”4.What does Bim Wannakit value most about using Apple devices for her art?A.The cost-effectiveness of the devices.B.The ability to create a balance between traditional and digital art.C.The simplicity of the design interface.D.The ease of file management through cloud services.5.Why did Bim choose to use iPad and Apple Pencil for her artistic work?A.They replicated the feel of traditional art materials exactly.B.They provided a cheaper alternative to professional art tools.C.They allowed her to combine digital flexibility with a natural drawing feel.D.They were the only tools available to her at the time.6.According to the article, how has Bim's artistic practice been enhanced by digital tools?A.She can now create larger artworks than before.B.She has access to a wider range of colors and textures.C.She can share her work instantly with a global audience.D.She can experiment with new techniques and styles more easily.7.Bim mentioned that GoodNotes and Microsoft Word have been helpful in her academic work. What are the main uses of these tools?A.Creating detailed sketches for her art projects.B.Organizing her research and writing academic papers.C.Editing photos for her online art gallery.D.Designing promotional materials for art exhibitions.We explore large-scale training of generative models on video data. Specifically, we train text-conditional diffusion models jointly on videos and images of variable durations, resolutions and aspect ratios. We leverage a transformer architecture that operates on spacetime patches of video and image latent codes. Our largest model, Sora, is capable of generating a minute of high fidelity video. Our results suggest that scaling video generation models is a promising path towards building general purpose simulators of the physical world.We train a network that reduces the dimensionality of visual data. This network takes raw video as input and outputs a latest representation that is compressed both temporally and spatially. Sora is trained on and subsequently generates videos within this compressed latent space. We also train a corresponding decoder model that maps generated latents back to pixel space.All of the results above and in our landing page show text-to-video samples. But Sora can also be prompted with other inputs, such as pre-existing images or video. This capability enables Sora to perform a wide range of image and video editing tasks—creating perfectly looping video, animating static images, extending videos forwards or backwards in time, etc.Sora is also capable of generating images. We do this by arranging patches of Gaussian noise in a spatial grid with a temporal extent of one frame. The model can generate images of variable sizes—up to 2048*2048 resolution.We find that video models exhibit a number of interesting emergent capabilities when trained at scale. These capabilities enable Sora to simulate some aspects of people, animals and environments from the physical world. These properties emerge without any explicit inductivebiases for 3D, objects, etc.—they are purely phenomena of scale.We believe the capabilities Sora has today demonstrate that continued scaling of video models is a promising path towards the development of capable simulators of the physical and digital world, and the objects, animals and people that live within them.8.What is the meaning of the underlined words in the text?A.the original raw video data.B.a compressed version of the video data.C.the process of reducing video quality.D.the spatial and temporal dimensions of a video.9.What is the main content of Paragraph 3?A.Sora's ability to generate high-resolution images.B.The process of training Sora on compressed latent space.C.Sora's various applications in image and video editing.D.The emergence of interesting capabilities in video models.10.Which of the following best describes the overall goal of the research described in the passage?A.To create realistic images and videos using only text prompts.B.To develop a general-purpose simulator capable of simulating various aspects of thephysical world.C.To train a network that can compress video data without losing quality.D.To explore the potential of transformer architectures in video and image generation tasks. 11.What is the author's attitude towards the future development of Sora?A.Skeptical.B.Optimistic.C.Neutral.D.Uncertain.Electric vehicles (EVs) are a strong weapon in the world's efforts against global warming. But the effects of EVs depend on what country you are in. In some nations, electric vehicles lead to the release of more carbon gasses than gasoline cars, new research shows.The Radiant Energy Group (REG) compared gas emissions caused by a gasoline vehicle and from charging an electric vehicle. The study compared the emissions caused by charging a Tesla Model 3 to drive 100 kilometers with the emissions coming from an average gasoline cardriven the same distance.Countries where charging an electric vehicle is cleaner than driving a gasoline-powered car use a lot of hydroelectric, nuclear or solar power.Sales of electric cars are rising the fastest in Europe. Data from REG suggests that EVs in Poland and Kosovo actually create more carbon emissions because their electric systems depend so much on coal.In other European countries, however, EVs result in reduced emissions. The carbon gas reduction depends on what energy supplies electricity systems and the time of day vehicles are charged.The countries with the biggest carbon gas savings from EVs use a lot of nuclear and hydroelectric power. An EV driver in Germany reduces greenhouse gas emissions by 55 percent over a gasoline car. Germany uses a mix of renewable energy and coal to produce electricity.Germany and Spain create a lot of electricity from the sun and wind. But the sun and wind do not add to a country's electricity system equally throughout the day.For this reason, the amount of carbon emissions saved by driving an EV depends on the time of day it is being charged. Charging in the afternoon, when there is more sun and wind, saves 16 to 18 percent more carbon than at night when electricity systems are more likely to be using gas or coal.Automakers including General Motors, Stellantis and V olkswagen have set targets to sell mainly electric vehicles in Europe in the coming years. U.S. car manufacturer General Motors said it will have all new electric cars by 2022.12.Which of the following statements is NOT true according to the passage?A.The amount of carbon emissions saved by EVs depends on the source of electricity used for charging.B.Charging EVs during daylight hours with renewable energy sources can cause more carbon savings.C.The time of day when EVs are charged can significantly affect their carbon footprint.D.General Motors plans to sell only gasoline-powered cars by 2022 in the United States. 13.What can be inferred from the fact that car manufacturers like General Motors, Stellantis, and V olkswagen have set targets to sell mainly electric vehicles in Europe?A.The demand for EVs in Europe is expected to decrease in the near future.B.These manufacturers believe that EVs will become the norm in Europe in the coming years.C.Europe has banned the sale of gasoline-powered cars entirely.D.These manufacturers are not confident in the long-term viability of EVs.14.Based on the information in the passage, which of the following is a potential challenge for the widespread adoption of EVs?A.The limited range of EVs compared to gasoline-powered cars.B.The high initial cost of EVs compared to traditional vehicles.C.The inconsistency of renewable energy sources for EV charging.D.The lack of charging stations in rural areas.15.Which of the following would be the most suitable title for the passage?A.The Environmental Impact of Electric Vehicle Charging.B.The Global Shift to Electric Vehicle Adoption.C.The Economics of Electric Vehicle Ownership.D.The Future of Renewable Energy in Automobiles.In the rapidly advancing world of today, the concept of lifelong learning has become increasingly relevant. 16 Rather, it is essential to continuously adapt and learn throughout one's life. This essay aims to explore the significance of lifelong learning in the modern era, discussing its impact on personal growth, career development, and societal progress.17 As technology and knowledge continue to evolve, it is important to stay updated with new information and ideas. By engaging in lifelong learning, individuals can enhance their cognitive abilities, broaden their horizons, and develop a growth mindset. This, in turn, leads to a more fulfilling and enriching life experience.Lifelong learning is essential for career development. In the modern job market, employers increasingly value candidates who possess the ability to adapt to change and learn new skills quickly. Lifelong learners are more likely to stay relevant and competitive in their field, as they are constantly improving their knowledge and skills. 18Moreover, lifelong learning contributes significantly to societal progress. As individualscontinuously acquire new knowledge and skills, they are able to contribute more effectively to their communities and countries. 19 Lifelong learning also fosters a culture of inclusivity and diversity, as it encourages individuals from different backgrounds and experiences to learn and grow together.In conclusion, lifelong learning is an indispensable aspect of life in the modern era. 20 By committing to lifelong learning, individuals can stay updated with new knowledge and ideas, enhance their cognitive abilities, and contribute more effectively to their communities and countries. Therefore, it is important for us to cultivate a habit of lifelong learning and encourage it among our peers and future generations.A.Additionally, they demonstrate a commitment to personal and professional development. B.With the development of science and technology, humans will continue to be replaced by AI. C.Lifelong learning is crucial for personal growth.D.This can lead to innovations, economic growth, and cultural richness.E.It is essential for personal growth, career development, and societal progress. F.Therefore, only lifelong learning, in order to maintain the leading position.G.It is no longer sufficient to acquire knowledge and skills during one's formal education.二、完形填空Once upon a time, there was a small village hidden deep in the mountains. The villagers led a simple life, depending mostly on farming for their 21 . One day, a young man named Jack arrived in the village. He was 22 and eager to explore the surrounding mountains.Jack quickly 23 with the villagers and became a part of their community. He was fascinated by the 24 tales of the mountains and the mysterious creatures that were said to inhabit them. Determined to find out the truth, Jack decided to embark on a journey of 25 .Before leaving, the villagers warned him of the dangers that awaited him in the mountains. They told him about the 26 weather, the treacherous paths, and the wild animals that roamed the area. But Jack was not 27 . He packed his belongings, bid farewell to the villagers, and started his journey.As he climbed higher and higher, the weather became increasingly 28 . The pathswere slippery and difficult to navigate. But Jack persevered, determined to reach the top of the mountain.After days of 29 , Jack finally reached the summit. The view was breathtaking, with rolling hills and dense forests stretching out as far as the eye could see. But as he was taking in the 30 , he heard a strange sound coming from the bushes nearby.Curious, Jack approached the bushes and peeked inside. To his surprise, he saw a small, 31 creature with big eyes and furry ears. The creature seemed 32 at first, but then it slowly approached Jack, sniffing the air curiously.Jack realized that this was one of the mysterious creatures the villagers had talked about. He felt a sense of 33 and excitement wash over him. He gently reached out his hand and the creature cautiously sniffed it, 34 allowing Jack to pet it.From that day on, Jack and the creature became close friends. They explored the mountains together, 35 each other's company. The villagers were amazed when Jack returned with his newfound friend and shared his adventures with them.The experience taught Jack the value of courage, perseverance, and friendship. It also showed him that there was more to the world than what he had imagined, and that there was always something new and exciting to discover.21.A.income B.entertainment C.inspiration D.experience 22.A.adventurous B.cautious C.lazy D.timid 23.A.argued B.competed C.bonded D.disagreed 24.A.boring B.ordinary C.amazing D.strange 25.A.discovery B.escape C.adventure D.research 26.A.unpredictable B.pleasant C.calm D.mild 27.A.discouraged B.frightened C.delighted D.satisfied 28.A.severe B.comfortable C.warm D.changeable 29.A.hiking B.struggling C.resting D.searching 30.A.scene B.creature C.challenge D.danger 31.A.fierce B.ugly C.cute D.powerful 32.A.aggressive B.nervous C.confident D.happy 33.A.wonder B.relief C.pride D.pity34.A.eventually B.immediately C.suddenly D.frequently 35.A.seeking B.enjoying C.avoiding D.tolerating三、语法填空阅读下面短文,在空白处填入1个适当的单词或括号内单词的正确形式。
Computer Vision Techniques

Computer Vision Techniquesrefer to the methods and algorithms used in the field of computer vision, which is a branch of artificial intelligence that enables computers to interpret and understand the visual world. are widely used in various applications such as image and video processing, object recognition, medical image analysis, autonomous vehicles, surveillance, robotics, and more.One of the fundamental tasks in computer vision is image classification, which involves categorizing images into different classes or categories based on their visual content. This task is usually accomplished using deep learning models, such as convolutional neural networks (CNNs). CNNs are a type of neural network that is specifically designed for processing images, and they have been extremely successful in various computer vision tasks due to their ability to learn hierarchical representations of visual data.Another important computer vision technique is object detection, which involves identifying and localizing objects of interest within an image or video stream. Object detection is commonly used in applications like self-driving cars, surveillance systems, and image search engines. One popular approach to object detection is the use of region-based convolutional neural networks (R-CNNs) or their variants, such as Faster R-CNN and Mask R-CNN. These models can accurately detect and classify objects in real-time while providing precise bounding box coordinates.Furthermore, image segmentation is a computer vision technique that partitions an image into multiple segments or regions based on pixel intensity, color, texture, or other visual features. Semantic segmentation assigns a class label to each pixel in an image, while instance segmentation differentiates between individual objects in the same class. Deep learning models like U-Net and Mask R-CNN have been widely used for image segmentation tasks, such as medical image analysis and scene understanding.Additionally, object tracking is an essential computer vision technique that involves locating and following a specific object over time in a sequence of images or videoframes. Object tracking is crucial in applications like video surveillance, visual tracking, and augmented reality. Tracking algorithms utilize techniques such as Kalman filtering, optical flow, and deep learning-based tracking methods to estimate the position, size, and motion of objects in consecutive frames accurately.In conclusion, computer vision techniques play a vital role in enabling machines to interpret and understand visual information, revolutionizing a wide range of industries and applications. By leveraging deep learning models, such as CNNs, R-CNNs, and segmentation networks, computers can perform complex visual tasks with high accuracy and efficiency. As the field of computer vision continues to advance, we can expect even more innovative applications and breakthroughs in the near future.。
基于多尺度各向异性高斯核主方向角度变化的角点检测

2021年第05期(总第221期)《蓿窟画蓿基于多尺度各向异性高斯核主方向角度变化的角点检测王天赋,任劫,章为川,晁凯(西安工程大学大学电子信息学院,陕西西安710048)摘要:角点检测在图像分析和计算机视觉领域有着及其重要的作用。
各向异性高斯方向导数(AG D Ds)可以很好的提取 不同方向的图像局部灰度变化信息,并且具有很强的噪声鲁棒性,在对不同尺度下的轮康信息和各向异性高斯方向导数 进行研究后,将两者相结合提出了一种基于多尺度各向异性高斯核主方向角度变化的角点检测算法。
该算法可以有效 的降低角点检测的误检率。
实验采用了两幅标注了真实角点测试图,在无噪声和加入不同等级噪声的情况下,对该算法 和其它三种经典的角点检测算法从角点检测的能力和角点定位的精度进行对比。
实验表明,研究所得的算法对于角点 的检测具有更好的噪声稳健性和更低的误检率。
关键词:角点检测;图像轮摩;各向异性高斯核;多尺度;鲁棒性中图分类号:TP391.41 文献标识码:B文章编号=2096-9759(2021)05-0032-04A muHkeale coma-detection v ia ai^ledifi»iHice ofpnnc^le directions of a nis»tropk Gaussian daivativesW ang T ianfu,Ren Jie9Zhang W eichuan,Chao kai(School o f Electronics and Inform ation,X i;an Polytechnic University,X i^an710048, China)A bstract:Comer detection plays an important role in image analysis and computer vision.Anisotropic Gaussian directionalderivatives(AGDDs)can extract the local gray change inform ation o f images in different directions,and has strong noise ro-bustness.A fter studying the contour inform ation and anisotropic Gaussian directional derivatives in different scales,a comer detection a lg o ritto based on multi-scale anisottopic Gaussian kernel principal direction angle change is proposed.The algor-ithm can effectively reduce the false detection rate o f comer detection.Two real comer test images are used in the experiment.In the case o f no noise and adding different levels o f noise,the comer detection ability and comer positioning accuracy o f the algorithm are compared w ith other three classical comer detection algorithms.Experimental results show that the proposed al圓gorithm has better noise robustness and lower false detection rate for comer detection.Key words:comer detection;image contours;anisotropic Gaussian kernel;m ulti-scale;robustness〇引言角点中包含了图像中重要的结构信息,是图像的基本特 征。
计算机视觉算法与应用的一些测试数据集和源码站点
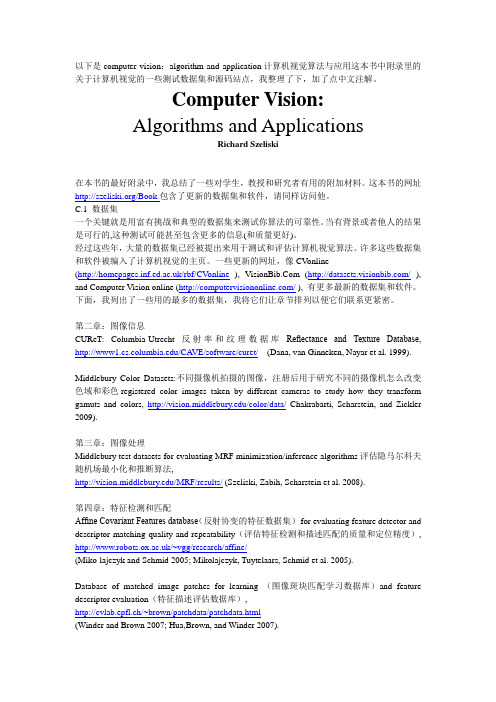
以下是computer vision:algorithm and application计算机视觉算法与应用这本书中附录里的关于计算机视觉的一些测试数据集和源码站点,我整理了下,加了点中文注解。
Computer Vision:Algorithms and ApplicationsRichard Szeliski在本书的最好附录中,我总结了一些对学生,教授和研究者有用的附加材料。
这本书的网址/Book包含了更新的数据集和软件,请同样访问他。
C.1 数据集一个关键就是用富有挑战和典型的数据集来测试你算法的可靠性。
当有背景或者他人的结果是可行的,这种测试可能甚至包含更多的信息(和质量更好)。
经过这些年,大量的数据集已经被提出来用于测试和评估计算机视觉算法。
许多这些数据集和软件被编入了计算机视觉的主页。
一些更新的网址,像CV online(/rbf/CV online), (/), and Computer Vision online (/ ), 有更多最新的数据集和软件。
下面,我列出了一些用的最多的数据集,我将它们让章节排列以便它们联系更紧密。
第二章:图像信息CUReT: Columbia-Utrecht 反射率和纹理数据库Reflectance and Texture Database, /CA VE/software/curet/(Dana, van Ginneken, Nayar et al. 1999).Middlebury Color Datasets:不同摄像机拍摄的图像,注册后用于研究不同的摄像机怎么改变色域和彩色registered color images taken by different cameras to study how they transform gamuts and colors, /color/data/Chakrabarti, Scharstein, and Zickler 2009).第三章:图像处理Middlebury test datasets for evaluating MRF minimization/inference algorithms评估隐马尔科夫随机场最小化和推断算法,/MRF/results/ (Szeliski, Zabih, Scharstein et al. 2008).第四章:特征检测和匹配Affine Covariant Features database(反射协变的特征数据集)for evaluating feature detector and descriptor matching quality and repeatability(评估特征检测和描述匹配的质量和定位精度), /~vgg/research/affine/(Miko-lajczyk and Schmid 2005; Mikolajczyk, Tuytelaars, Schmid et al. 2005).Database of matched image patches for learning (图像斑块匹配学习数据库)and feature descriptor evaluation(特征描述评估数据库),http://cvlab.epfl.ch/~brown/patchdata/patchdata.html(Winder and Brown 2007; Hua,Brown, and Winder 2007).第五章;分割Berkeley Segmentation Dataset(分割数据库)and Benchmark of 1000 images labeled by 30 humans,(30个人标记的1000副基准图像)along with an evaluation,/Research/Projects/CS/vision/grouping/segbench/(Martin, Fowlkes, Tal et al. 2001).Weizmann segmentation evaluation database of 100 grayscale images with ground truth segmentations,http://www.wisdom.weizmann.ac.il/~vision/Seg Evaluation DB/index.html(Alpert, Galun, Basri et al. 2007).第八章:稠密运动估计The Middlebury optic flow evaluation(光流评估)Web site,/flow/data/(Baker, Scharstein, Lewis et al. 2009).The Human-Assisted Motion Annotation database,(人类辅助运动数据库)/celiu/motionAnnotation/(Liu, Freeman, Adelson et al. 2008)第十章:计算机摄像学High Dynamic Range radiance(辐射)maps, /Research/HDR/(De-bevec and Malik 1997).Alpha matting evaluation Web site, / (Rhemann, Rother, Wanget al. 2009).第十一章:Stereo correspondence立体对应Middlebury Stereo Datasets and Evaluation, /stereo/(Scharstein and Szeliski 2002).Stereo Classification(立体分类)and Performance Evaluation(性能评估)of different aggregation(聚类)costs for stereo matching(立体匹配),http://www.vision.deis.unibo.it/spe/SPEHome.aspx(Tombari, Mat-toccia, Di Stefano et al. 2008).Middlebury Multi-View Stereo Datasets,/mview/data/(Seitz,Curless, Diebel et al. 2006).Multi-view and Oxford Colleges building reconstructions,/~vgg/data/data-mview.html .Multi-View Stereo Datasets, http://cvlab.epfl.ch/data/strechamvs/(Strecha, Fransens,and Van Gool 2006).Multi-View Evaluation, http://cvlab.epfl.ch/~strecha/multiview/ (Strecha, von Hansen,Van Gool et al. 2008).第十二章:3D重建HumanEva: synchronized video(同步视频)and motion capture (动作捕捉)dataset for evaluation of articulated human motion, /humaneva/Sigal, Balan, and Black 2010).第十三章:图像渲染The (New) Stanford Light Field Archive, /(Wilburn, Joshi,Vaish et al. 2005).Virtual Viewpoint Video: multi-viewpoint video with per-frame depth maps,/en-us/um/redmond/groups/ivm/vvv/(Zitnick, Kang, Uytten- daele et al. 2004).第十四章:识别查找一系列的视觉识别数据库,在表14.1–14.2.除了那些,这里还有:Buffy pose classes, /~vgg/data/buffy pose classes/ and Buffy stickmen V2.1, /~vgg/data/stickmen/index.html(Ferrari,Marin- Jimenez, and Zisserman 2009; Eichner and Ferrari 2009).H3D database of pose/joint annotated photographs of humans,/~lbourdev/h3d/(Bourdev and Malik 2009).Action Recognition Datasets, /projects/vision/action, has point-ers to several datasets for action and activity recognition, as well as some papers.(有一些关于人活动和运动的数据库和论文)The human action database athttp://www.nada.kth.se/cvap/actions/包含更多的行动序列。
计算机视觉课程教学大纲
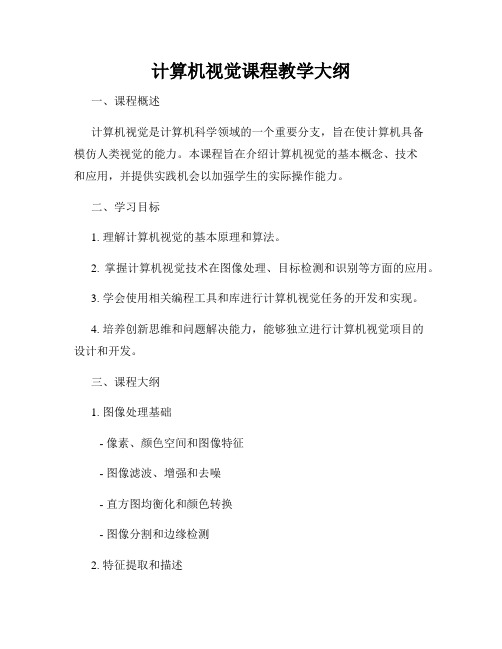
计算机视觉课程教学大纲一、课程概述计算机视觉是计算机科学领域的一个重要分支,旨在使计算机具备模仿人类视觉的能力。
本课程旨在介绍计算机视觉的基本概念、技术和应用,并提供实践机会以加强学生的实际操作能力。
二、学习目标1. 理解计算机视觉的基本原理和算法。
2. 掌握计算机视觉技术在图像处理、目标检测和识别等方面的应用。
3. 学会使用相关编程工具和库进行计算机视觉任务的开发和实现。
4. 培养创新思维和问题解决能力,能够独立进行计算机视觉项目的设计和开发。
三、课程大纲1. 图像处理基础- 像素、颜色空间和图像特征- 图像滤波、增强和去噪- 直方图均衡化和颜色转换- 图像分割和边缘检测2. 特征提取和描述- 尺度空间和兴趣点检测- 特征描述算法(SIFT、SURF等)- 特征匹配和重建3. 目标检测与识别- 目标检测的基本概念和方法- Haar特征和级联分类器- 图像分类和深度学习方法- 目标跟踪和行为分析4. 三维视觉- 三维重建和立体匹配- 摄像机标定和姿态估计- 深度传感器和点云处理5. 计算机视觉应用- 人脸检测与识别- 视频分析与视频跟踪- 视觉SLAM(同时定位与地图构建) - 医学图像处理与辅助诊断四、实践项目本课程将结合实践项目,供学生运用所学知识解决实际问题,并提供指导和反馈。
五、评估方式1. 平时表现与作业(30%):包括课堂讨论、作业完成情况等。
2. 实践项目(40%):根据项目难度、创新性、完成度等进行评估。
3. 期末考试(30%):对学生对整个课程内容的掌握情况进行考察。
六、教材与参考资料1. 主教材:- Richard Szeliski. "Computer Vision: Algorithms and Applications" (第二版),Springer出版社,2010年。
2. 参考资料:- Simon J. D. Prince. "Computer Vision: Models, Learning, and Inference",Cambridge出版社,2012年。
英语 科技英语写作习题和答案
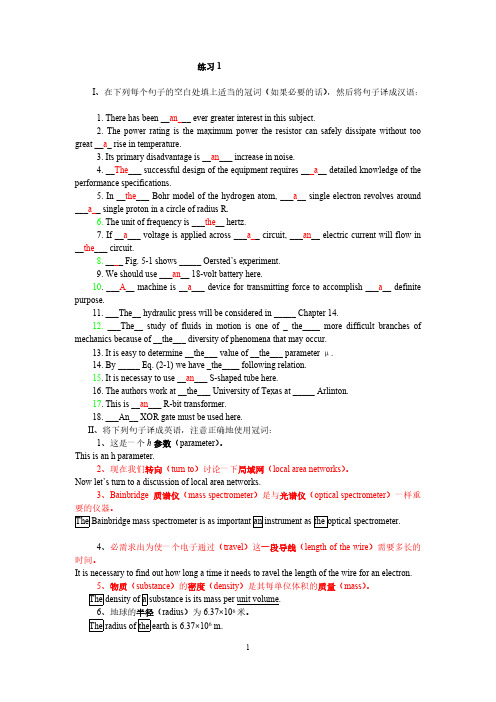
(ohm)。
3、其误差(error)为1012分之六。
4、这台计算机所储存的信息比那台多三倍。
(要求把“计算机”用作为主语的“主谓宾”结构的句型)5、月球的质量为地球的1/ 80.6、不久的将来对这种设备的需求量(demand)为现在的20倍。
7、这个元件(component)上的电压为零点几伏特(volt)。
8、现在其内部的压力是原来的三分之一。
III、将下列小段的内容译成英语:温度这一概念对我们大家来说都是熟悉的。
这是因为我们的身体对温差是非常灵敏的。
当我们拾起(pick up)一块冰时,我们感到冷,因为其温度低于我们手的温度。
在喝了一杯咖啡后,我们可能把它说成(refer to … as …)“热的”,“温的”(lukewarm)或“很糟的”(atrocious)。
在头两种情况下,至少我们在描述其温度超过(exceed)我们的(温度的)程度。
IV、根据所给的汉语文本,改正各英语文本中的错误:1、【汉语原文】这个电容器上的电压为零点零几伏特。
【英语文本】The voltage on this capacitor is zero point zero several volt.2、【汉语原文】这物体比那物体重四倍。
【英语文本】This object is four times heavy than that one is.3、【汉语原文】除非另有说明,我们假设使用的是硅管、I CBO可以忽略不计。
【英语文本】 Unless otherwise stated, it is assumed that silicon transistors are used andI CBO can be neglected.4、【汉语原文】这个电路的优点是结构简单、容易调整。
【英语文本】This circuit has the advantage of simpe in structure、easy to adjust.5、【汉语原文】图1、2、3画出了这个过程。
计算机视觉方向面试自我介绍
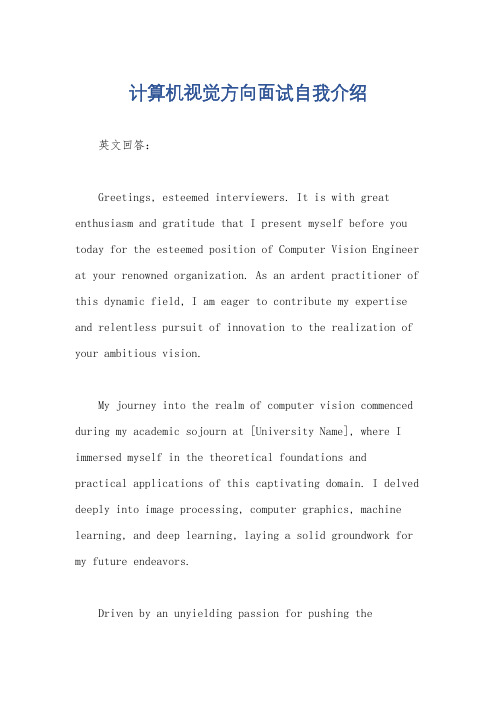
计算机视觉方向面试自我介绍英文回答:Greetings, esteemed interviewers. It is with great enthusiasm and gratitude that I present myself before you today for the esteemed position of Computer Vision Engineer at your renowned organization. As an ardent practitioner of this dynamic field, I am eager to contribute my expertise and relentless pursuit of innovation to the realization of your ambitious vision.My journey into the realm of computer vision commenced during my academic sojourn at [University Name], where I immersed myself in the theoretical foundations andpractical applications of this captivating domain. I delved deeply into image processing, computer graphics, machine learning, and deep learning, laying a solid groundwork for my future endeavors.Driven by an unyielding passion for pushing theboundaries of what is possible, I embarked upon a research project centered around the development of novel algorithms for object detection and recognition. This endeavor culminated in a peer-reviewed publication in[Journal/Conference Name], which garnered significant recognition within the scientific community.Upon graduating with honors, I embarked upon a professional career at [Company Name], where I honed myskills as a Computer Vision Engineer. I played an instrumental role in the development of cutting-edge solutions for a diverse range of industries, including healthcare, autonomous vehicles, and retail. My responsibilities encompassed the design, implementation,and evaluation of computer vision algorithms, ensuringtheir robustness, efficiency, and accuracy.Beyond my technical capabilities, I am also a highly motivated and results-oriented individual with a proven ability to thrive in both independent and collaborative settings. I am proficient in a wide array of programming languages and software tools, including Python, C++, OpenCV,and TensorFlow.I am particularly drawn to your organization's unwavering commitment to innovation and its reputation as a global leader in the development of world-changing technologies. I am confident that my skills and experience would make me a valuable asset to your team, enabling me to make significant contributions to your ongoing success.Thank you for granting me the opportunity to introduce myself. I eagerly anticipate the chance to further discuss my qualifications and demonstrate how my passion for computer vision aligns with the strategic objectives of your esteemed organization.中文回答:各位尊敬的面试官,大家好!非常荣幸有机会向大家介绍我自己,并申请贵公司计算机视觉工程师的职位。
边缘检测梯度算法
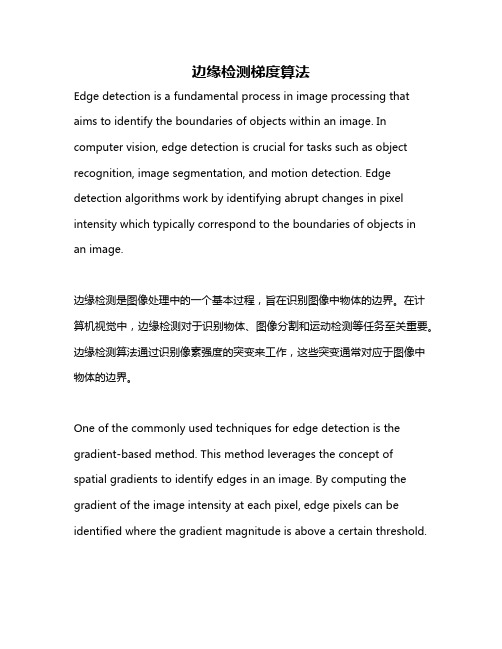
边缘检测梯度算法Edge detection is a fundamental process in image processing that aims to identify the boundaries of objects within an image. In computer vision, edge detection is crucial for tasks such as object recognition, image segmentation, and motion detection. Edge detection algorithms work by identifying abrupt changes in pixel intensity which typically correspond to the boundaries of objects inan image.边缘检测是图像处理中的一个基本过程,旨在识别图像中物体的边界。
在计算机视觉中,边缘检测对于识别物体、图像分割和运动检测等任务至关重要。
边缘检测算法通过识别像素强度的突变来工作,这些突变通常对应于图像中物体的边界。
One of the commonly used techniques for edge detection is the gradient-based method. This method leverages the concept of spatial gradients to identify edges in an image. By computing the gradient of the image intensity at each pixel, edge pixels can be identified where the gradient magnitude is above a certain threshold.The gradient direction can also provide information about the orientation of the edge.用于边缘检测的常用技术之一是基于梯度的方法。
人工智能:一种现代的方法第3版罗素课后答案
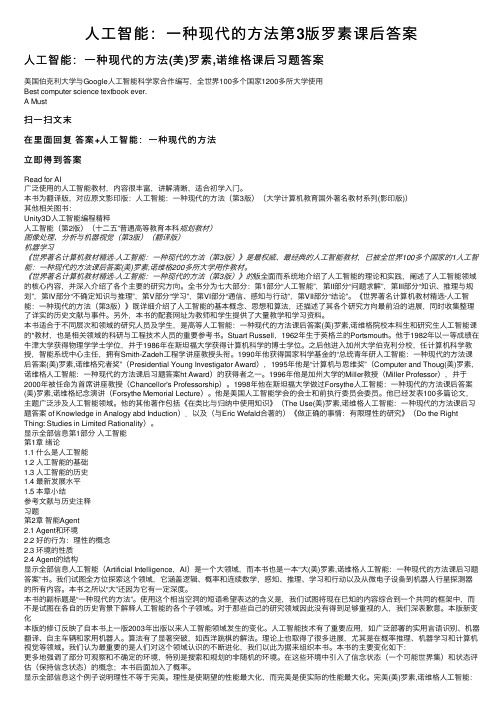
⼈⼯智能:⼀种现代的⽅法第3版罗素课后答案⼈⼯智能:⼀种现代的⽅法(美)罗素,诺维格课后习题答案美国伯克利⼤学与Google⼈⼯智能科学家合作编写,全世界100多个国家1200多所⼤学使⽤Best computer science textbook ever.A Must扫⼀扫⽂末在⾥⾯回复答案+⼈⼯智能:⼀种现代的⽅法⽴即得到答案Read for AI⼴泛使⽤的⼈⼯智能教材,内容很丰富,讲解清晰,适合初学⼊门。
本书为翻译版,对应原⽂影印版:⼈⼯智能:⼀种现代的⽅法(第3版)(⼤学计算机教育国外著名教材系列(影印版))其他相关图书:Unity3D⼈⼯智能编程精粹⼈⼯智能(第2版)(⼗⼆五”普通⾼等教育本科规划教材)图像处理、分析与机器视觉(第3版)(翻译版)机器学习《世界著名计算机教材精选·⼈⼯智能:⼀种现代的⽅法(第3版)》是最权威、最经典的⼈⼯智能教材,已被全世界100多个国家的1⼈⼯智能:⼀种现代的⽅法课后答案(美)罗素,诺维格200多所⼤学⽤作教材。
《世界著名计算机教材精选·⼈⼯智能:⼀种现代的⽅法(第3版)》的版全⾯⽽系统地介绍了⼈⼯智能的理论和实践,阐述了⼈⼯智能领域的核⼼内容,并深⼊介绍了各个主要的研究⽅向。
全书分为七⼤部分:第1部分“⼈⼯智能”,第II部分“问题求解”,第III部分“知识、推理与规划”,第IV部分“不确定知识与推理”,第V部分“学习”,第VI部分“通信、感知与⾏动”,第VII部分“结论”。
《世界著名计算机教材精选·⼈⼯智能:⼀种现代的⽅法(第3版)》既详细介绍了⼈⼯智能的基本概念、思想和算法,还描述了其各个研究⽅向最前沿的进展,同时收集整理了详实的历史⽂献与事件。
另外,本书的配套⽹址为教师和学⽣提供了⼤量教学和学习资料。
本书适合于不同层次和领域的研究⼈员及学⽣,是⾼等⼈⼯智能:⼀种现代的⽅法课后答案(美)罗素,诺维格院校本科⽣和研究⽣⼈⼯智能课的*教材,也是相关领域的科研与⼯程技术⼈员的重要参考书。
人工智能应用案例

人工智能应用案例【导言】在当今信息技术快速发展的时代,人工智能(Artificial Intelligence,简称AI)作为一种前沿技术,逐渐渗透到各个领域,为我们的生活带来了革命性的改变。
本文将介绍几个人工智能应用的典型案例,以展示其在不同领域的应用和对社会带来的意义。
【案例一:自然语言处理】自然语言处理(Natural Language Processing,简称NLP)是人工智能领域的重要分支,它使计算机能够理解和处理自然语言。
一个典型的NLP应用案例是智能客服系统。
以某电商平台为例,该平台运用了NLP技术使其客服系统能够自动识别用户问题并给出相应的解答。
通过对大量的用户问题和答案进行学习和分析,系统能够提供高效的客户服务,并随着时间的推移逐渐提升自己的解答能力。
这一应用极大地提高了客户满意度,同时也减少了人工客服人员的工作负担。
【案例二:机器视觉】机器视觉(Computer Vision)是指让计算机能够感知和理解图像和视频的能力。
一个典型的机器视觉应用案例是智能安防系统。
通过安装摄像头和利用图像处理算法,智能安防系统可以准确识别出人、车辆或其他物体,并对异常行为进行实时监控和报警。
这种系统可以应用于各种场合,如社区、商场、银行等,有效地提升了安全性和防范能力。
此外,机器视觉还可以用于无人驾驶汽车、医疗影像诊断等领域,为人类带来便利和安全。
【案例三:智能音箱】智能音箱作为智能家居的重要组成部分,内置了人工智能助手,可以通过语音指令实现多种功能,如播放音乐、查询天气、控制家电等等。
其中,语音识别和语音合成是关键技术。
智能音箱通过将语音信号转化为文字,然后交由人工智能算法进行处理,最终实现指令识别和执行。
这一应用方便了人们的生活,提高了家居智能化的程度。
【案例四:智能金融】人工智能在金融领域的应用也非常广泛。
例如,智能投顾系统能够根据投资者的风险承受能力和投资偏好,通过分析市场数据和历史走势,自动给出个性化的投资建议。
机器视觉应用工程师简历范文
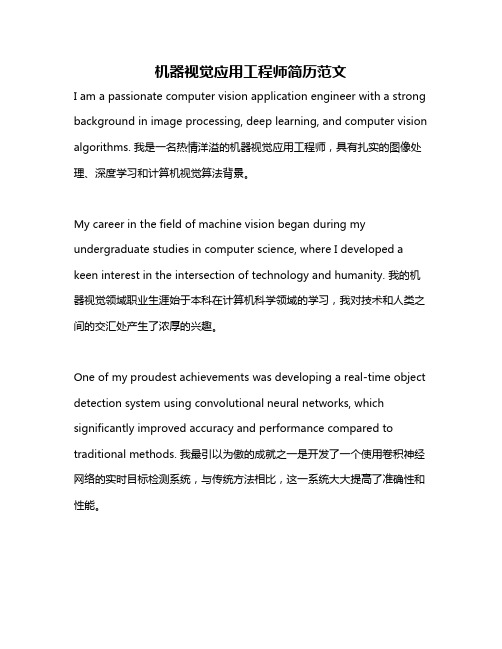
机器视觉应用工程师简历范文I am a passionate computer vision application engineer with a strong background in image processing, deep learning, and computer vision algorithms. 我是一名热情洋溢的机器视觉应用工程师,具有扎实的图像处理、深度学习和计算机视觉算法背景。
My career in the field of machine vision began during my undergraduate studies in computer science, where I developed a keen interest in the intersection of technology and humanity. 我的机器视觉领域职业生涯始于本科在计算机科学领域的学习,我对技术和人类之间的交汇处产生了浓厚的兴趣。
One of my proudest achievements was developing a real-time object detection system using convolutional neural networks, which significantly improved accuracy and performance compared to traditional methods. 我最引以为傲的成就之一是开发了一个使用卷积神经网络的实时目标检测系统,与传统方法相比,这一系统大大提高了准确性和性能。
I have worked on various projects involving facial recognition, object tracking, and image segmentation, which have allowed me to hone my skills in developing innovative computer vision solutions. 我参与了涉及人脸识别、目标跟踪和图像分割的各种项目,这让我得以提升自己在开发创新的计算机视觉解决方案方面的技能。
- 1、下载文档前请自行甄别文档内容的完整性,平台不提供额外的编辑、内容补充、找答案等附加服务。
- 2、"仅部分预览"的文档,不可在线预览部分如存在完整性等问题,可反馈申请退款(可完整预览的文档不适用该条件!)。
- 3、如文档侵犯您的权益,请联系客服反馈,我们会尽快为您处理(人工客服工作时间:9:00-18:30)。
感光景物的話,景物上一點投影
的位置使影像成像清晰。
在更進一步,為了控制曝光,我們可以加入一個Shutter以及一個aperture,aperture用以控制進入光的角度(即針孔的大小),Shutter
制曝光的時間。
接下來我們來看一下aperture,一般上
f-stop表示,在照相機上則標示為
aperture的數字越大,代表光圈
aperture)開的越小,所以進光量也越小。
與景深間的關係,所謂
當然,Lenses的好壞與種類可能會影響成像的品例如品質不好的鏡頭可能到成影像的扭曲。
魚眼鏡頭也會造成類似的效果。
Digital camera
aperture
& shutter
的作用是將光的資訊轉成電流的資訊,轉換的過程我們之前所談的,都是單色的情況,要得到彩色的
color
的意思。
一般由數位
•一般相機在拍照時,處理的流程如下:
,因此是一個一對一的遞增函式,我們可以假設
檔,得到曝光response function以後,我們就可以很容易地從
得出真正的曝光量。
需注意有些相機可以輸出
格式的檔案,內容是不經由response function而由
接取得的曝光值,對這樣的相機我們就不需要大費周章去
response function了。