Bioinformatics in The Drug Discovery Process
人类基因组计划名词解释生物信息学
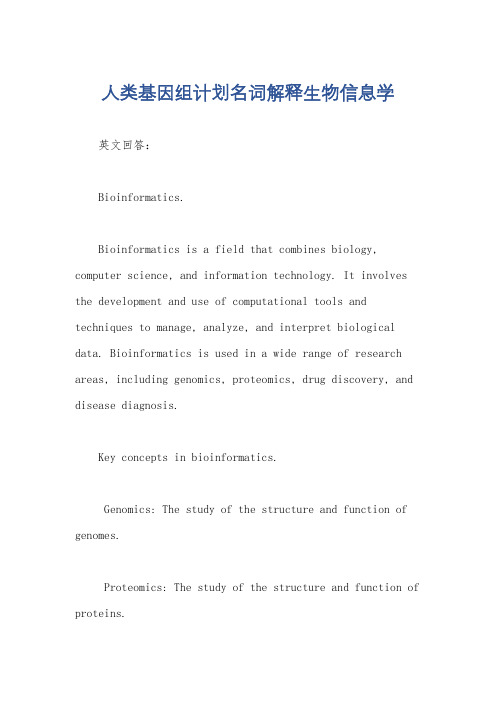
人类基因组计划名词解释生物信息学英文回答:Bioinformatics.Bioinformatics is a field that combines biology, computer science, and information technology. It involves the development and use of computational tools and techniques to manage, analyze, and interpret biological data. Bioinformatics is used in a wide range of research areas, including genomics, proteomics, drug discovery, and disease diagnosis.Key concepts in bioinformatics.Genomics: The study of the structure and function of genomes.Proteomics: The study of the structure and function of proteins.Transcriptomics: The study of the structure and function of transcripts.Metabolomics: The study of the structure and function of metabolites.Bioinformatics databases: Databases that store and manage biological data.Bioinformatics tools: Software tools that are used to analyze and interpret biological data.Applications of bioinformatics.Drug discovery: Bioinformatics is used to identify new drug targets and to design new drugs.Disease diagnosis: Bioinformatics is used to develop new diagnostic tests for diseases.Personalized medicine: Bioinformatics is used todevelop personalized treatment plans for patients.Evolutionary biology: Bioinformatics is used to study the evolution of species.Challenges in bioinformatics.Data explosion: The amount of biological data is growing rapidly, making it difficult to manage and analyze.Data integration: Biological data is often stored in different formats and in different databases, making it difficult to integrate and analyze.Algorithm development: New algorithms are needed to analyze and interpret complex biological data.Despite these challenges, bioinformatics is a rapidly growing field with the potential to revolutionize the way we understand and treat diseases.中文回答:生物信息学。
肽库筛选方法

肽库筛选方法Peptide library screening is a crucial step in drug discovery and development. The method involves the identification of peptides that possess a desired biological activity from a diverse pool of peptides. In order to effectively screen a peptide library, researchers utilize various techniques and strategies to increase the chances of finding the most promising candidates. One commonly used approach is high-throughput screening, which allows for the rapid testing of a large number of peptides simultaneously. This method is particularly useful in identifying peptides with specific binding properties or therapeutic potential.肽库筛选是药物发现和研发中的一个关键步骤。
该方法涉及从各种多样的肽中识别具有期望生物活性的肽。
为了有效地筛选肽库,研究人员利用各种技术和策略来增加找到最有前景候选物的机会。
一种常用的方法是高通量筛选,它可以同时快速测试大量肽。
这种方法特别适用于识别具有特定结合特性或治疗潜力的肽。
Another important consideration in peptide library screening is the selection of an appropriate screening assay. Different assays can beused to evaluate diverse aspects of peptide activity, such as binding affinity, enzymatic activity, or cellular response. By choosing the most suitable assay for the desired outcome, researchers can enhance the efficiency and accuracy of the screening process. Moreover, the development of novel screening assays tailored to specific targets or applications can further improve the success rate of peptide library screening.在肽库筛选中另一个重要的考虑因素是选择合适的筛选方法。
生物利用度在药物发展过程中的意义英语作文
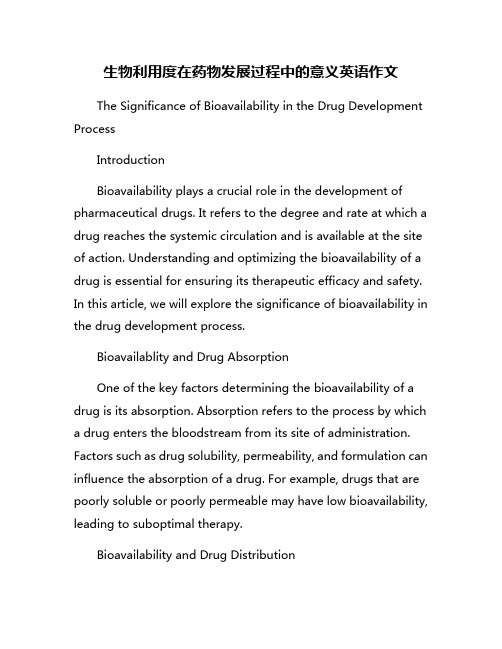
生物利用度在药物发展过程中的意义英语作文The Significance of Bioavailability in the Drug Development ProcessIntroductionBioavailability plays a crucial role in the development of pharmaceutical drugs. It refers to the degree and rate at which a drug reaches the systemic circulation and is available at the site of action. Understanding and optimizing the bioavailability of a drug is essential for ensuring its therapeutic efficacy and safety. In this article, we will explore the significance of bioavailability in the drug development process.Bioavailablity and Drug AbsorptionOne of the key factors determining the bioavailability of a drug is its absorption. Absorption refers to the process by which a drug enters the bloodstream from its site of administration. Factors such as drug solubility, permeability, and formulation can influence the absorption of a drug. For example, drugs that are poorly soluble or poorly permeable may have low bioavailability, leading to suboptimal therapy.Bioavailability and Drug DistributionAfter absorption, a drug is distributed throughout the body to its target tissues or organs. The distribution of a drug is influenced by factors such as protein binding, tissue permeability, and blood flow. Drugs that are highly bound to plasma proteins may have limited distribution and lower bioavailability. Understanding the distribution of a drug is important for optimizing its dosage and dosing regimen.Bioavailability and Drug MetabolismOnce a drug is absorbed and distributed, it undergoes metabolism in the liver and other tissues. Metabolism can modify the structure of a drug, making it more or less active. The bioavailability of a drug can be affected by its metabolism, as metabolites may have different pharmacokinetic properties than the parent drug. This can impact the efficacy and safety of a drug, highlighting the importance of considering metabolism in the drug development process.Bioavailability and Drug EliminationFinally, a drug is eliminated from the body through processes such as renal excretion, hepatic metabolism, and biliary excretion. The rate of elimination can affect the bioavailability of a drug, as drugs with a short half-life may require more frequent dosing to maintain therapeutic levels.Understanding the elimination of a drug is essential for optimizing its bioavailability and overall pharmacokinetics.Optimizing Bioavailability in Drug DevelopmentIn order to optimize the bioavailability of a drug, researchers can use various strategies during the drug development process. These include selecting appropriate drug formulations, enhancing drug solubility and permeability, and minimizing the impact of metabolism and elimination. By considering bioavailability early in the drug development process, researchers can design drugs with improved efficacy, safety, and patient compliance.ConclusionIn conclusion, bioavailability is a critical factor in the drug development process. Understanding and optimizing the bioavailability of a drug is essential for ensuring its therapeutic effectiveness and safety. By considering factors such as absorption, distribution, metabolism, and elimination, researchers can develop drugs with improved bioavailability and pharmacokinetic properties. Ultimately, by prioritizing bioavailability in drug development, researchers can enhance the quality and impact of pharmaceutical drugs.。
药物发现与计算机辅助设计简介
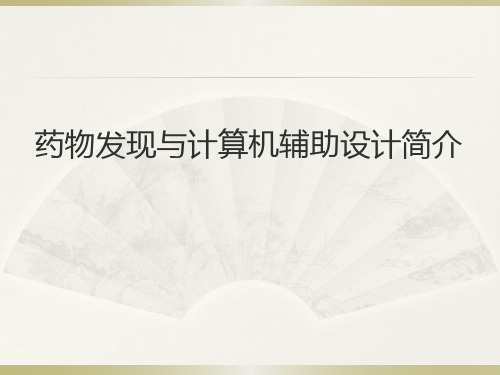
药物开发流程
The Development Phase
临床前研究 Pre-clinical Studies
相应参数
–药物的作用机制 –动物安全性和毒性(包括急性和慢性的毒性研究) –ADME参数(药代动力学) –生物利用度,口服生物利用度 –药物剂型试验
广泛的动物实验
药效&副作用
26
药物开发流程
Investigation New Drug
New Drug Appery & Development
时间分配
23
药物开发流程
Drug Discovery & Development
24
药物开发流程
The Discovery Phase
Market
80%-90% of potential drugs fail in the development phase (pre)clinical trials Causes: ADMET , efficacy and side effects
21
药物开发流程
Drug Discovery & Development
二期临床:
三期临床:
27
现代药物研究四大技术支柱
分子生物学、基因组学及蛋白质组学
Molecular Biology, Genomics and Proteomics 发现疾病靶标
28
现代药物研究四大技术支柱
组合化学 Combinatorial Chemistry
3222=24种组合
只有3/10的药物面市后收 入超过R&D花费
Bioinformatics信息
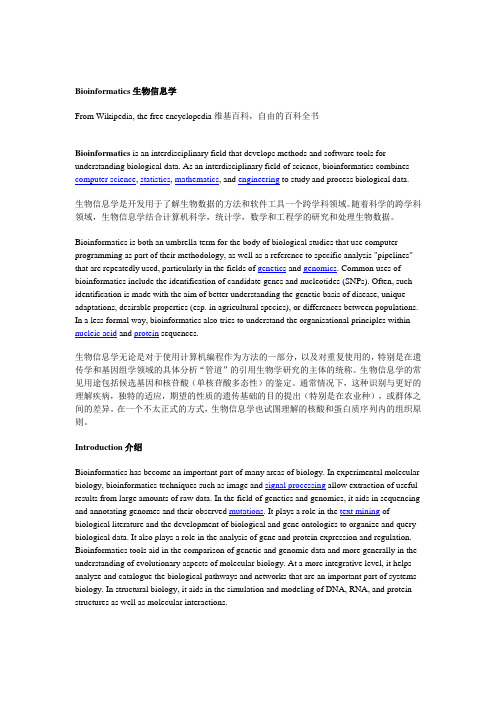
Bioinformatics生物信息学From Wikipedia, the free encyclopedia维基百科,自由的百科全书Bioinformatics is an interdisciplinary field that develops methods and software tools for understanding biological data. As an interdisciplinary field of science, bioinformatics combines computer science, statistics, mathematics, and engineering to study and process biological data.生物信息学是开发用于了解生物数据的方法和软件工具一个跨学科领域。
随着科学的跨学科领域,生物信息学结合计算机科学,统计学,数学和工程学的研究和处理生物数据。
Bioinformatics is both an umbrella term for the body of biological studies that use computer programming as part of their methodology, as well as a reference to specific analysis "pipelines" that are repeatedly used, particularly in the fields of genetics and genomics. Common uses of bioinformatics include the identification of candidate genes and nucleotides (SNPs). Often, such identification is made with the aim of better understanding the genetic basis of disease, unique adaptations, desirable properties (esp. in agricultural species), or differences between populations. In a less formal way, bioinformatics also tries to understand the organisational principles within nucleic acid and protein sequences.生物信息学无论是对于使用计算机编程作为方法的一部分,以及对重复使用的,特别是在遗传学和基因组学领域的具体分析“管道”的引用生物学研究的主体的统称。
药物筛选 英语
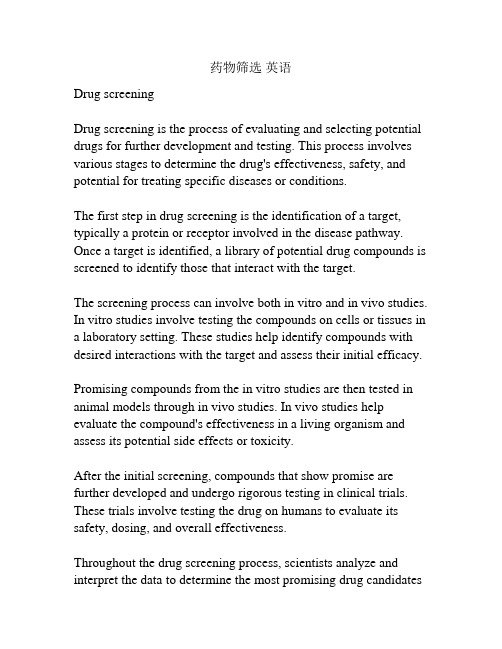
药物筛选英语Drug screeningDrug screening is the process of evaluating and selecting potential drugs for further development and testing. This process involves various stages to determine the drug's effectiveness, safety, and potential for treating specific diseases or conditions.The first step in drug screening is the identification of a target, typically a protein or receptor involved in the disease pathway. Once a target is identified, a library of potential drug compounds is screened to identify those that interact with the target.The screening process can involve both in vitro and in vivo studies. In vitro studies involve testing the compounds on cells or tissues in a laboratory setting. These studies help identify compounds with desired interactions with the target and assess their initial efficacy.Promising compounds from the in vitro studies are then tested in animal models through in vivo studies. In vivo studies help evaluate the compound's effectiveness in a living organism and assess its potential side effects or toxicity.After the initial screening, compounds that show promise are further developed and undergo rigorous testing in clinical trials. These trials involve testing the drug on humans to evaluate its safety, dosing, and overall effectiveness.Throughout the drug screening process, scientists analyze and interpret the data to determine the most promising drug candidatesfor further development. This includes considering factors such as efficacy, safety, pharmacokinetics, and the potential market demand.Overall, drug screening is a crucial step in the drug discovery and development process, helping researchers identify potential drugs that have the best chances of success in treating specific diseases or conditions.。
关键药物靶点筛选 英语

关键药物靶点筛选英语Key Drug Target Screening in Modern Therapeutics.Drug target screening is a crucial step in the drug discovery process, aiming to identify specific molecules or biological structures that can be modulated to treat or prevent diseases. With the ever-evolving field of biomedicine, the importance of target screening has become increasingly apparent, as it holds the potential to revolutionize healthcare by developing more effective and precise therapeutics.1. Importance of Drug Target Screening.Drug target screening is essential for several reasons. Firstly, it helps to identify the most relevant biological targets that are involved in the pathogenesis of a particular disease. By understanding the underlying biological mechanisms, researchers can design drugs that are more specific and less likely to cause unwanted sideeffects.Secondly, target screening enables the prioritization of targets based on their therapeutic potential. This is achieved by assessing the expression levels of targets in diseased tissues, their involvement in key biological processes, and their amenability to modulation by drugs.Lastly, target screening facilitates the identification of novel drug candidates. By screening large libraries of compounds for their ability to interact with specific targets, researchers can discover new leads that may lead to the development of innovative drugs.2. Methods of Drug Target Screening.Several methods are employed for drug target screening, each with its own advantages and limitations.2.1 High-throughput Screening (HTS)。
代谢组学 计算机药学方法体系
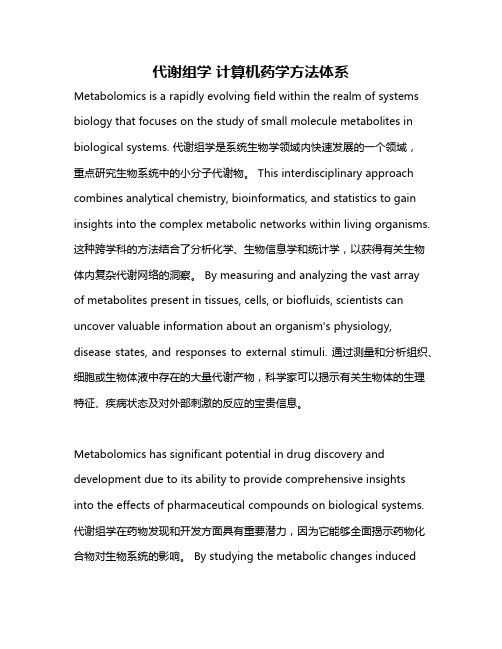
代谢组学计算机药学方法体系Metabolomics is a rapidly evolving field within the realm of systems biology that focuses on the study of small molecule metabolites in biological systems. 代谢组学是系统生物学领域内快速发展的一个领域,重点研究生物系统中的小分子代谢物。
This interdisciplinary approach combines analytical chemistry, bioinformatics, and statistics to gain insights into the complex metabolic networks within living organisms. 这种跨学科的方法结合了分析化学、生物信息学和统计学,以获得有关生物体内复杂代谢网络的洞察。
By measuring and analyzing the vast arrayof metabolites present in tissues, cells, or biofluids, scientists can uncover valuable information about an organism's physiology, disease states, and responses to external stimuli. 通过测量和分析组织、细胞或生物体液中存在的大量代谢产物,科学家可以揭示有关生物体的生理特征、疾病状态及对外部刺激的反应的宝贵信息。
Metabolomics has significant potential in drug discovery and development due to its ability to provide comprehensive insightsinto the effects of pharmaceutical compounds on biological systems. 代谢组学在药物发现和开发方面具有重要潜力,因为它能够全面揭示药物化合物对生物系统的影响。
生物利用度在药物发展过程中的意义英语作文
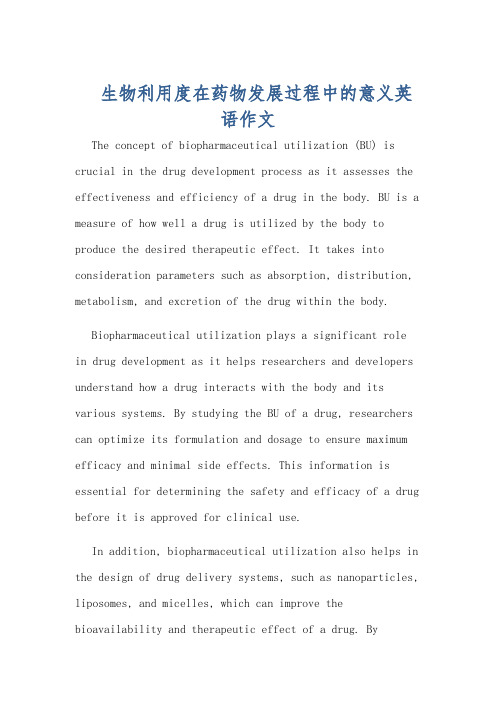
生物利用度在药物发展过程中的意义英语作文The concept of biopharmaceutical utilization (BU) is crucial in the drug development process as it assesses the effectiveness and efficiency of a drug in the body. BU is a measure of how well a drug is utilized by the body to produce the desired therapeutic effect. It takes into consideration parameters such as absorption, distribution, metabolism, and excretion of the drug within the body.Biopharmaceutical utilization plays a significant rolein drug development as it helps researchers and developers understand how a drug interacts with the body and its various systems. By studying the BU of a drug, researchers can optimize its formulation and dosage to ensure maximum efficacy and minimal side effects. This information is essential for determining the safety and efficacy of a drug before it is approved for clinical use.In addition, biopharmaceutical utilization also helps in the design of drug delivery systems, such as nanoparticles, liposomes, and micelles, which can improve the bioavailability and therapeutic effect of a drug. Byenhancing the BU of a drug, these delivery systems can increase its concentration at the target site and reduceoff-target effects.Overall, biopharmaceutical utilization is a critical aspect of drug development that ensures the safety, efficacy, and optimal utilization of drugs in the body. By understanding how a drug is utilized by the body, researchers can improve the design and delivery of drugs to maximize their therapeutic potential and minimize potential risks.生物利用度在药物发展过程中的意义非常重要,它评估药物在体内产生所需治疗效果的效力和效率。
基于生物信息学方法发现潜在药物靶标
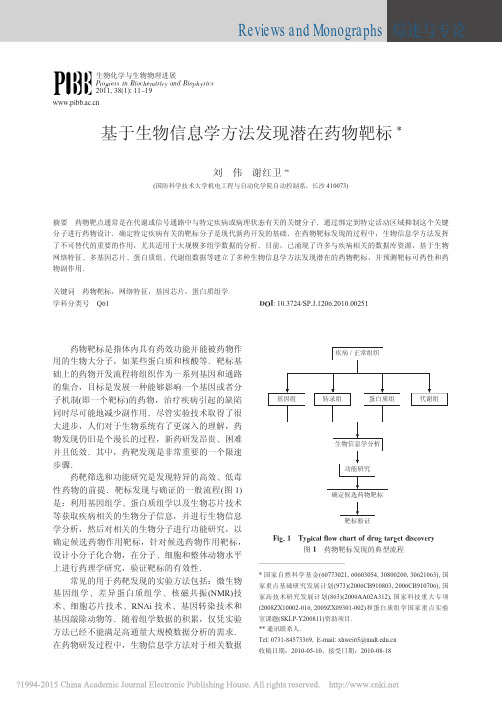
基因芯片技术指将大量(通常每平方厘米点阵 密度高于 400)探针分子固定于支持物上与标记的 样品分子进行杂交,检测每个探针分子的杂交信号 强度,进而获取样品分子的数量和序列信息.由于 基因芯片技术的高通量、快速、平行化等特点,使 得疾病相关的基因芯片数据资源非常丰富,利用基 因芯片数据挖掘潜在药物靶标成为一种重要的途 径.例如,在 GEO 数据库的基础上,Hu 等[8]建立 了大规模的疾病 - 药物对应网络,帮助有效地识别 药物靶标.
2011; 38 (1)
刘伟,等:基于生物信息学方法发现潜在药物靶标
·13·
2 用于药靶发现的生物信息学方法
2.1 基因组方法 丰富的基因组学数据为药靶发现提供了基础,
目前已有多种方法可用于寻找新的药物靶标[6].其 中,最常用的方法是同源搜索,采用序列比对软件 寻找候选基因与已知癌症基因之间的序列同源性, 如 BLAST 或基于隐马尔科夫的 HMMER 软件包 等.然而,新的靶标与已知癌症基因的序列可能并 不相似.因此,有必要分析已知药靶中更为普遍的 结构特征,如信号肽、跨膜结构域或蛋白激酶域. 此类生物信息学工具包括预测信号肽的 SignalP 和 预测跨膜结构域的 TMHMM.此外,还可以使用 基因预测程序从人类基因组序列中预测新基因,寻 找 全 新 的 药 物 靶 标 , 常 用 的 程 序 是 Genescan 和 Grail.
·12·
生物化学与生物物理进展 Prog. Biochem. Biophys.
2011; 38 (1)
的存储、分析和处理,以及如何有效地发现和验证 新的药靶,发挥了重要的作用.本文首先介绍可用 于药靶发现的数据库资源,包括疾病相关的基因数 据库、候选药靶数据库和基因芯片数据库等,其次 讨论了基于多种组学数据进行药物靶标发现的生物 信息学方法,如基于基因组、基因表达谱、蛋白质 组、代谢组的方法以及整合多组学数据的系统生物 学方法,再次描述了生物信息学方法在药物靶标验 证方面的应用,主要是预测蛋白质可药性以及药物 副作用,最后是总结和展望.
计算生物学英文
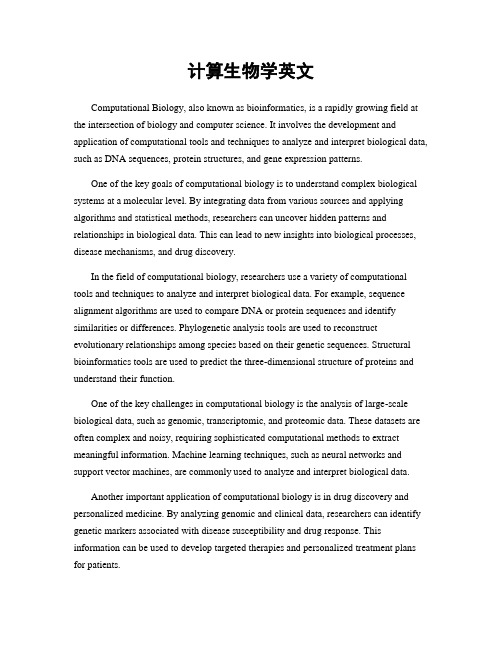
计算生物学英文Computational Biology, also known as bioinformatics, is a rapidly growing field at the intersection of biology and computer science. It involves the development and application of computational tools and techniques to analyze and interpret biological data, such as DNA sequences, protein structures, and gene expression patterns.One of the key goals of computational biology is to understand complex biological systems at a molecular level. By integrating data from various sources and applying algorithms and statistical methods, researchers can uncover hidden patterns and relationships in biological data. This can lead to new insights into biological processes, disease mechanisms, and drug discovery.In the field of computational biology, researchers use a variety of computational tools and techniques to analyze and interpret biological data. For example, sequence alignment algorithms are used to compare DNA or protein sequences and identify similarities or differences. Phylogenetic analysis tools are used to reconstruct evolutionary relationships among species based on their genetic sequences. Structural bioinformatics tools are used to predict the three-dimensional structure of proteins and understand their function.One of the key challenges in computational biology is the analysis of large-scale biological data, such as genomic, transcriptomic, and proteomic data. These datasets are often complex and noisy, requiring sophisticated computational methods to extract meaningful information. Machine learning techniques, such as neural networks and support vector machines, are commonly used to analyze and interpret biological data.Another important application of computational biology is in drug discovery and personalized medicine. By analyzing genomic and clinical data, researchers can identify genetic markers associated with disease susceptibility and drug response. This information can be used to develop targeted therapies and personalized treatment plans for patients.Overall, computational biology plays a crucial role in advancing our understanding of complex biological systems and accelerating biomedical research. By combining the power of computational tools and biological knowledge, researchers can address key biological questions and make new discoveries that can lead to improved human health and well-being.。
人类基因组计划名词解释生物信息学

英文回答:Bioinformatics, an interdisciplinary field that integrates biology andputer science, is dedicated to the interpretation and analysis ofplex biological data, specifically those associated with DNA, RNA, and protein sequences. This area of study employsputational techniques to effectively store, organize, and analyze extensive sets of biological data, such as genomic sequences, in order to extract meaningful insights and valuable information. Bioinformatics plays a crucial role in various domains of biological research, including genomic analysis, evolutionary biology, drug discovery, and personalized medicine. With the rapid advancement of high-throughput sequencing technologies, which generate copious amounts of biological data necessitating sophisticatedputational tools for analysis and interpretation, the importance of bioinformaticshas greatly escalated.生物信息学是一个跨学科领域,融合了生物学和截肢科学,致力于解释和分析复杂生物数据,特别是与DNA,RNA,蛋白质序列相关的数据。
bioinformatics analysis is a technique
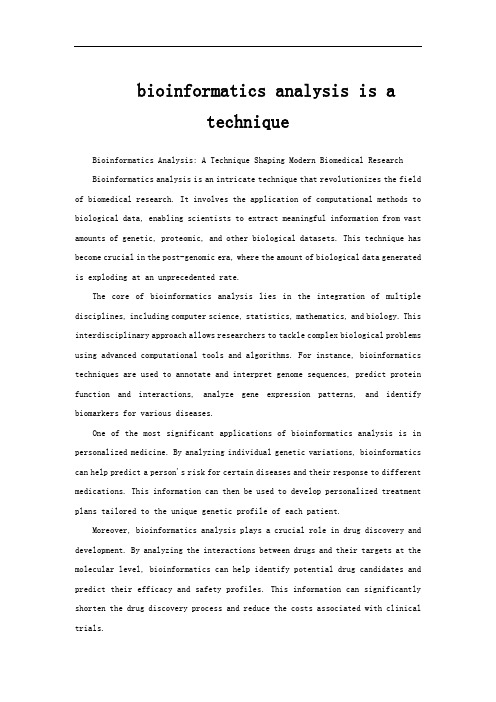
bioinformatics analysis is atechniqueBioinformatics Analysis: A Technique Shaping Modern Biomedical ResearchBioinformatics analysis is an intricate technique that revolutionizes the field of biomedical research. It involves the application of computational methods to biological data, enabling scientists to extract meaningful information from vast amounts of genetic, proteomic, and other biological datasets. This technique has become crucial in the post-genomic era, where the amount of biological data generated is exploding at an unprecedented rate.The core of bioinformatics analysis lies in the integration of multiple disciplines, including computer science, statistics, mathematics, and biology. This interdisciplinary approach allows researchers to tackle complex biological problems using advanced computational tools and algorithms. For instance, bioinformatics techniques are used to annotate and interpret genome sequences, predict protein function and interactions, analyze gene expression patterns, and identify biomarkers for various diseases.One of the most significant applications of bioinformatics analysis is in personalized medicine. By analyzing individual genetic variations, bioinformatics can help predict a person's risk for certain diseases and their response to different medications. This information can then be used to develop personalized treatment plans tailored to the unique genetic profile of each patient.Moreover, bioinformatics analysis plays a crucial role in drug discovery and development. By analyzing the interactions between drugs and their targets at the molecular level, bioinformatics can help identify potential drug candidates and predict their efficacy and safety profiles. This information can significantly shorten the drug discovery process and reduce the costs associated with clinical trials.In addition to its applications in personalized medicine and drug discovery, bioinformatics analysis also has numerous other uses. It can be used to study the evolution of species, the mechanisms of gene regulation, and the interactions between different biological systems. Bioinformatics analysis is also essential in the field of epidemiology, where it helps track the spread of diseases and identify potential outbreaks.In conclusion, bioinformatics analysis is a technique that has revolutionized biomedical research. Its interdisciplinary nature and the use of advanced computational methods have enabled researchers to extract meaningful information from vast amounts of biological data. This information has led to breakthroughs in personalized medicine, drug discovery, and other areas of biomedical research, promising better health outcomes and improved quality of life for millions of people.。
医药研发常用英语词汇大全
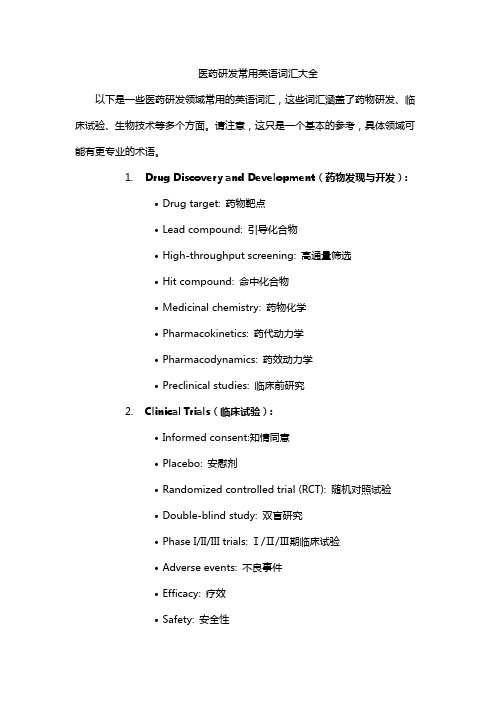
医药研发常用英语词汇大全以下是一些医药研发领域常用的英语词汇,这些词汇涵盖了药物研发、临床试验、生物技术等多个方面。
请注意,这只是一个基本的参考,具体领域可能有更专业的术语。
1.Drug Discovery and Development(药物发现与开发):•Drug target: 药物靶点•Lead compound: 引导化合物•High-throughput screening: 高通量筛选•Hit compound: 命中化合物•Medicinal chemistry: 药物化学•Pharmacokinetics: 药代动力学•Pharmacodynamics: 药效动力学•Preclinical studies: 临床前研究2.Clinical Trials(临床试验):•Informed consent:知情同意•Placebo: 安慰剂•Randomized controlled trial (RCT): 随机对照试验•Double-blind study: 双盲研究•Phase I/II/III trials: Ⅰ/Ⅱ/Ⅲ期临床试验•Adverse events: 不良事件•Efficacy: 疗效•Safety: 安全性3.Biotechnology(生物技术):•Recombinant DNA technology: 重组DNA技术•Genetically modified organism (GMO): 转基因生物•Cloning: 克隆•Gene therapy: 基因治疗•Stem cells: 干细胞•Bioprocessing: 生物加工•Bioinformatics: 生物信息学4.Regulatory Affairs(法规事务):•Regulatory submission: 法规提交•Investigational New Drug (IND): 新药申请•New Drug Application (NDA): 新药上市申请•Good Manufacturing Practice (GMP): 良好生产规范•Good Clinical Practice (GCP): 良好临床实践5.Pharmacology(药理学):•Receptor: 受体•Agonist: 激动剂•Antagonist: 拮抗剂•Pharmacogenetics: 药理遗传学•Toxicology: 毒理学6.Quality Control(质量控制):•Batch release: 批释放•Quality assurance: 质量保证•Certificate of Analysis (CoA): 分析证书•Stability testing: 稳定性测试这些词汇只是医药研发领域中的一小部分,具体的词汇会根据不同的子领域而有所不同。
生物信息学课件英文原版课件
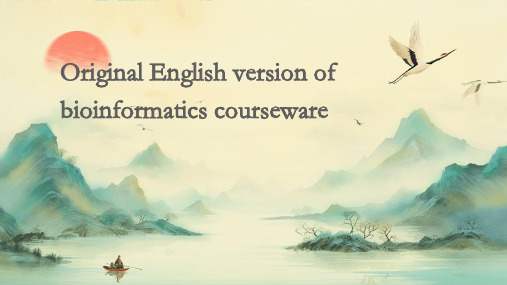
• Introduction to Bioinformatics • Genomics • Proteomics • The Application of Bioinformatics in
Medicine • The Future Development of
The research field of bioinformatics
Summary: Research Field of Bioinformatics
Detailed description: The research fields of bioinformatics are very extensive, including genomics, proteomics, systems biology, evolutionary biology, epigenetics, etc. These fields of research all involve the acquisition, processing, analysis, and interpretation of biological data, as well as the role of these data in understanding biological processes and disease mechanisms.
pharmaceuticals. For example, in the field of medicine, genomics can be used to diagnose genetic diseases, predict drug responses, and personalize healthcare. In the field of agriculture, genomics can be used to improve crop and livestock varieties, increase yield and resistance.
疾病差异基因表达英文

疾病差异基因表达英文Disease-specific gene expression: A deep dive into the molecular mechanisms.Diseases are often caused by complex interactions between genetic and environmental factors. Among these, the dysregulation of gene expression plays a pivotal role in the pathogenesis of various diseases. Disease-specific gene expression refers to the altered expression patterns of genes that are uniquely associated with a particular disease. Understanding the molecular mechanisms underlying these changes is crucial for developing effective diagnostic tools and therapeutic strategies.The study of disease-specific gene expression involves the analysis of gene expression profiles in diseased tissues or cells compared to their normal counterparts. This can be achieved through various high-throughput techniques such as microarrays, next-generation sequencing, and proteomics. These technologies allow researchers tomeasure the expression levels of thousands of genes simultaneously, providing a comprehensive view of the transcriptional landscape in diseased tissues.One of the key challenges in the field is the identification of disease-specific gene expression signatures. These signatures refer to the unique combinations of genes that are differentially expressed in a particular disease. The identification of these signatures requires the integration of bioinformatics tools and statistical methods to filter out the most relevant genes from the vast amount of data generated by high-throughput techniques.Once identified, disease-specific gene expression signatures can be used for various applications. Firstly, they can serve as biomarkers for disease diagnosis. By measuring the expression levels of specific genes inpatient samples, doctors can accurately diagnose diseases at an early stage, enabling timely treatment and improved patient outcomes.Secondly, disease-specific gene expression signatures can be used to understand the pathogenesis of diseases. By analyzing the functions and interactions of these genes, researchers can gain insights into the molecular mechanisms underlying the development and progression of diseases. This knowledge can then be used to design targeted therapeutic strategies that aim to modulate the expression of these genes.Moreover, disease-specific gene expression signatures can also be used to predict patient outcomes and responses to treatment. By correlating gene expression patterns with clinical outcomes, researchers can identify subgroups of patients who are more likely to respond favorably to a particular treatment. This information can guide clinicians in making personalized treatment decisions for their patients.In addition, disease-specific gene expression signatures can serve as targets for drug discovery and development. By identifying the genes that are dysregulated in a particular disease, researchers can focus theirefforts on developing drugs that can modulate the expression of these genes. This approach has led to the discovery of several novel therapeutics that are currently being used to treat diseases such as cancer, inflammatory diseases, and neurological disorders.In conclusion, the study of disease-specific gene expression has opened new avenues for understanding the pathogenesis of diseases and developing effective diagnostic tools and therapeutic strategies. With the advent of advanced technologies and bioinformatics methods, we are poised to make further progress in this field and bring about improvements in patient care and outcomes.。
Drug discovery and development-药物发现与发展
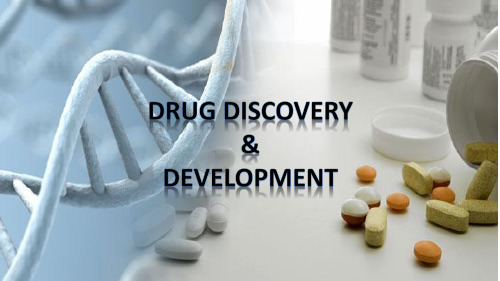
Registration:
• The Ministry of health & Family Welfare and the Ministry of Chemicals & Fertilizers have major role in regulation of IPM. • NDA must be submitted to DCGI • Phase III study reported to CDL, Kolkata • Package inserted approved by DCI • Marketing approval from FDA
1920s, 30s
• Vitamins • Vaccines
1960s
• Breakthrough in Etiology
1990s
• Robotics • Automation
1940s
• Antibiotic Era • R&D Boost due to WW2
1950s
• New technology, • Discovery of DNA
Today's NPV($mn) 5,807 4,953 4,666 4,332 4,241
6
7 8 9
DR Cysteamine
AMR 101 Eliquis Eliquis
Undisclosed
Undisclosed
Phase III
Phase III
4,155
4,052 3,836 3,592 3,250
Target Selection Lead Discovery Medicinal Chemistry In Vitro Studies In Vivo Studies Clinical Trials
生物利用度在药物发展过程中的意义英语作文

生物利用度在药物发展过程中的意义英语作文The significance of biological utilization in the drug development process lies in its ability to identify, understand, and leverage the natural resources and processes within living organisms to develop new drugs and therapies. By studying and harnessing the biological activities of various organisms, researchers can discover novel compounds, understand disease mechanisms, and develop new treatment modalities.Biological utilization involves the exploration of diverse natural sources such as plants, animals, and microorganisms for their potential therapeutic properties. For example, many traditional medicines have their origins in plant-based remedies, and modern drug discovery continues to draw inspiration from natural sources. By understanding the biological activities of these natural compounds, scientists can modify and optimize them to create more effective and safer drugs.In addition, biological utilization plays a crucial role in understanding disease processes and identifying potential drugtargets. By studying the biological pathways and molecular mechanisms involved in various diseases, researchers can identify key molecules and processes that can be targeted for therapeutic intervention. This knowledge is essential for developing new drugs that can effectively modulate disease processes and improve patient outcomes.Furthermore, biological utilization also encompasses the study of how drugs and therapies interact with living organisms. This includes understanding drug metabolism, pharmacokinetics, and drug interactions within the body. By studying these aspects, researchers can optimize drug dosing regimens, minimize side effects, and improve drug efficacy.Overall, biological utilization is essential in drug development as it provides a wealth of natural resources and insights that can lead to the discovery of new drugs, the understanding of disease processes, and the optimization of drug therapies. By harnessing the power of living organisms, researchers can continue to advance the field of drug development and improve patient care.生物利用度在药物发展过程中的意义在于其能够识别、理解和利用生物体内的自然资源和过程,以开发新药物和疗法。
bioinformatics 医学英语

bioinformatics 医学英语Bioinformatics is an interdisciplinary field that combines biology, computer science, and information technology to analyze and interpret biological data. In the context of medicine, bioinformatics focuses on using computational tools and methods to make sense of vast amounts of genomic, transcriptomic, proteomic, and other biological data to better understand various diseases and develop personalized treatment strategies.Bioinformaticians in medicine use algorithms, statistical models, and machine learning techniques to analyze and compare DNA sequences, identify genes and protein structures, predict protein functions, and study gene expression patterns. They also develop databases and software tools for storing, managing, and analyzing biological data, allowing researchers to access and share valuable information.By leveraging bioinformatics tools and techniques, researchers and clinicians can uncover novel insights into the underlying causes of diseases, discover biomarkers for early disease detection, and develop targeted therapies. It has been particularly useful in cancer research, where bioinformatics is used to identify specific gene mutations and genetic variations that drive cancer growth, helping to develop targeted therapies and personalized treatment plans. Overall, bioinformatics in medicine plays a crucial role in advancing our understanding of diseases, improving diagnosis and treatment, and ultimately paving the way for precision medicine.。
- 1、下载文档前请自行甄别文档内容的完整性,平台不提供额外的编辑、内容补充、找答案等附加服务。
- 2、"仅部分预览"的文档,不可在线预览部分如存在完整性等问题,可反馈申请退款(可完整预览的文档不适用该条件!)。
- 3、如文档侵犯您的权益,请联系客服反馈,我们会尽快为您处理(人工客服工作时间:9:00-18:30)。
Bioinformatics in the Drug Discovery ProcessSarah Pollock and Hershel M. SaferCompugen Ltd.72 Pinchas Rosen Street, Tel Aviv 69512, Israel{ sarah, hersh } @compugen.co.ilIntroduction ─ Bioinformatics is the part of molecular biology that involves working with biological data, typically using computers, with the goal of enabling and accelerating biological research. It is ubiquitous in drug discovery; few if any projects are computer-free. Bioinformatics spans a wide range of activities: data capture, automated recording of experimental results; data storage and access, using a multitude of databases and query tools; data analysis; and visualization of raw data and analytical results. Although the first two categories rely on computer skills, the others also require mathematics, molecular biology, and biochemistry. Until recently, most people working in bioinformatics started in one discipline and picked up the others on the job. New interdisciplinary programs of study, though, integrate these subjects.Bioinformatics is web-centric. Most academic groups and many companies make computing tools and data available on the web. An online supplement to this article (/science/armc-2001.htm) contains links to relevant websites. Key databases are also reviewed in each year’s first issue of Nucleic Acids Research (1).This article briefly reviews recent bioinformatics publications, with a focus on tools and databases used to speed drug target discovery and selection. Subjects not covered include genetic, physical, and radiation hybrid mapping; genetics; genotyping and high-throughput screening; molecular and cellular simulation; and automation.WHOLE-GENOME SEQUENCE ANALYSISThe newly available human genome sequence includes the sequence of almost every gene. Initial versions of the transcriptome, the expression level of transcribed sequences in particular tissues under specific conditions, and of the proteome, the proteins in a cell under given conditions and their interactions, are also available. These data are transforming biology and the pharmaceutical industry. Manual analysis of such vast amounts of data is impractical; computerized tools are needed to reap the full benefit of these resources. Genomics has become a major source of drug targets, and bioinformatics is crucial for finding and validating novel targets so as to minimize investment in laboratory resources.Sequencing the Human Genome ─ The most important recent genomics papers are about the draft sequencing of the human genome (2, 3). Two versions were published: one by the Human Genome Project (HGP), which includes 20 sequencing centers in six countries, and one by the company Celera. The primary sequencing articles are accompanied by articles describing bioinformatics analyses of the data.Current technology can sequence DNA segments of up to about 1,000 base pairs (bp; kb for thousand bp and Mb for million bp). A longer stretch is sequenced by breaking it into small fragments, sequencing one or both ends of each, and joining the pieces, so-called shotgun sequencing. The process of joining short sequences is called fragment assembly; it relies on the assertion that two sequences with virtually identical regions are likely from the same location, in the absence of complications from repeats and sequencing errors. Assembly tools include Phrap, FAK, the TIGR assembler, and CAP (4-7). The HGP divided the genome into pieces of 200-350 kb that were sequenced individually and then joined. Celera, though, fragmented the entire genome. Previous assemblers reconstructed entire microbial genomes, but the Celera Assembler was the first to apply whole-genome shotgun sequencing to a target this complex (8). Published in Annual Reports in Medicinal Chemistry, 2001 © Academic Press2 Section─ Topics in Biology Allen, Ed.VThe HGP sequence and automatically-generated annotation are freely available from the websites of the National Center for Biotechnology Information (NCBI), Ensembl, and UCSC. The Celera sequence is available from the Celera website under conditions described there. Comparisons of the sequences reveal that they are globally similar but have local discrepancies, probably mostly the result of mis-assembly (9, 10) This review can mention only a few of the many interesting conclusions. The most striking finding may be the number of protein-coding genes, which both groups estimated as 30,000-40,000. This is lower than the previously estimated 70,000-120,00 genes (11), though recent analyses presaged this result (12, 13). These numbers are surprising because they are not much larger than the 20,000 genes in the nematode or the 12,000 in the fruit fly. This shows that genome complexity does not depend only on the number of genes. Alternative splicing seems to be key to the greater intricacy of humans (14). Analysis also revealed evidence of horizontal transfer of bacterial genes into vertebrates as well as extremely long duplicated regions.Sequencing Other Genomes ─ More than 600 organisms are being sequenced and several dozen genomes are complete. One criterion in choosing an organism to sequence is its medical importance. Microbial and viral genomes help identify drug targets in pathogens; eukaryotic genomes advance understanding of human genetics and physiology. Plant and animal sequencing have also led to significant advances in sequencing and bioinformatics technologies.A gene’s genomic context, including nearby genes and transcription control regions, is needed to understand the gene’s function. Comparative genomics, i.e., comparing conserved sequence regions in multiple organisms, uses conservation of sequence and higher-order genomic structure to gain insight into gene function. PipMaker compares long DNA sequences to identify conserved regions without prior knowledge of gene structures (15). The Artemis Comparison Tool (ACT), a DNA sequence viewer, displays genomic structure as well as similarities and differences between two genomes (16).NCBI provides tools for comparative genomics in addition to comprehensive cross-links between various databases (17). HomoloGene includes curated and calculated orthologues and homologues in six eukaryotes; LocusLink is an interface for queries about their genetic loci. The Human/Mouse Homology Maps display syntenic regions, i.e., regions that contain similar genes, usually in the same order.Clusters of Orthologous Groups (COGs) are phylogenetic classifications of proteins from 34 complete genomes in 26 major phylogenetic lineages (18). BLAST (see below) is used to find the best match for each protein in each genome. Proteins that are best hits for each other are clustered. Clustered proteins are likely to have similar functions;a COG is a cluster with proteins from three or more lineages. COGs yield functional predictions for poorly characterized genomes. Results do not depend on BLAST cutoff scores and so are unaffected by different evolutionary times between pairs of genomes. Sequence Alignment Tools ─ Database searches to find similar sequences that are putative homologues, i.e., that appear to have a common evolutionary ancestor, are at the core of sequence analysis. Proteins with similar sequences or domains typically have similar structure and often function, though the converse is not always true. Proteins that are at least 25% similar are generally homologous (19).Almost synonymous with database searching, Basic Local Alignment Search Tool (BLAST) may be the most widely used bioinformatics tool (20). BLAST is optimized for speed but sacrifices only minimal sensitivity in searching databases. More sensitive algorithms like that of Smith and Waterman find optimal alignments but are slower (21). Newer tools address features of nucleotide sequences (22, 23), modeling phenomena such as frame shifts, codon insertions and deletions, and introns. Improved sensitivity comes at the price of computation time, though hardware accelerators make some such algorithms reasonable for high-throughput environments.Chap. ? Bioinformatics Pollock, Safer 3 Distinguishing signal from background noise is difficult when looking for homologues that are extremely distant on the evolutionary time scale. Sensitivity is improved by focusing on a protein’s functional domains, which may have distinct origins and functions. Multiple alignment of sequences from a domain in several organisms reveals patterns of conservation, including highly conserved residues, and the patterns can be used to recognize distant homologues. Databases that use patterns are based on multiple alignments that have been either manually optimized or created automatically (24-27). InterPro centralizes protein information from many databases (28).Some new tools use existing sequence databases. BLAST now accommodates sequence gaps. Another version combines alignments into a position-specific scoring matrix and searches with that matrix. This iterative process yields a Position-Specific Iterated version (PSI-BLAST) (29) that provides improved annotation (30). Sequence queries can also be compared to collections of position-specific scoring matrices (31).GenBank contains more than 11 billion bp in more than 10 million sequences and continues to grow exponentially. A BLAST search against all of GenBank takes three times as long as it did a year ago! MEGABLAST compares large sets of long nucleotide sequences up to 10 times as fast as do previous programs, but is best for comparing sequences that differ because of sequencing errors (32). The race between database growth and search speed continues; algorithmic and hardware improvements will be needed to continue the pace of biological discovery.Recognizing and Predicting Genes and Other Sequence Features ─ One post-sequencing challenge is comprehensive genome annotation, including at least the putative start, stop, and structure of each gene, and preferably information about transcription regulatory regions. Similarity searches can identify some novel genes, but many human genes are not very similar to known sequences from other organisms.Ab initio gene prediction finds genes in a (typically eukaryotic) genomic sequence using sequence only, without knowing expressed or translated regions, though possibly using known genes as examples. This is challenging, as eukaryotic genes are complex and less than 2% of the human genome codes for proteins. RefSeq, a non-redundant, curated database of mRNA and protein sequences, contains examples that illustrate this complexity (17): genes have many exons (median 7 / mean 8.8), long introns (1,023 bp / 3,365 bp, with large variance, and many longer than 50 kb), short internal exons (122 bp / 145 bp), and short coding sequences (1,100 bp / 1,340 bp). They also stretch over long genomic extents (14 kb / 27 kb) and are widely separated (2).Methods such as Grail2, GenScan, Genie, and HMMGene (33-36) use probabilistic pattern recognition methods to identify protein coding regions based on gene-related patterns of DNA composition. The latter two programs also integrate sequence similarity to known coding regions and expressed sequence tags (see below).EST analysis ─ An expressed sequence tag (EST) is a partial expressed sequence from a (generally anonymous) cDNA. Large collections of ESTs promised a shortcut to finding most genes before the genome sequence was available and were to obviate difficulties of computational gene prediction (37). Although their early promise was not completely fulfilled, ESTs are quite useful. Examination of EST assemblies provided the first hints of the prevalence of alternative splicing in the human genome (38, 39) and led to the detection of many putative SNPs (see below) (40, 41). ESTs are useful molecular landmarks and those from multiple tissues can be used to answer questions about tissue-specific genes and to identify novel proteins (42, 43).GenBank contains more than three million human and almost two million mouse EST sequences, and proprietary collections include millions more. ESTs are typically short, 3’-skewed, and of relatively low quality; many represent only the 3’ UTR; and the databases are highly redundant and poorly annotated. As a result, searching EST databases directly is not terribly efficient. More useful information is found by clustering4 Section─ Topics in Biology Allen, Ed.VESTs and mRNAs based on sequence overlaps, yielding sequences that are longer, of higher accuracy, and that better represent the underlying genes. Databases of EST clusters include UniGene (44), the TIGR Human Gene Index (45), and STACK (46); they are compared in (47). GeneNest adds to UniGene by providing consensus sequences and visualization (48). A current challenge is integrating EST clusters and genomic data to improve gene and transcript predictions by eliminating EST artifacts. Polymorphism analysis ─ Most common sequence variation, or polymorphism, consists of single nucleotide polymorphisms (SNPs), mutations that replace one base pair with another. SNPs serve as genetic markers to track familial inheritance; some are functionally important, affecting disease susceptibility and response to medication (49). The dbSNP database (50) holds more than 1.5 million unique SNPs, more than half from The SNP Consortium (51). About 1.42 million have been mapped to the human genome using MEGABLAST (52). To find SNPs, several copies of DNA from a region, preferably from ethnically diverse individuals, are compared for sequence differences. SNP detection technology and the use of SNPs in genotyping are reviewed in (53).Phred (54), a basecalling program for sequencer traces, estimates an error probability for each base, and this quality score can help differentiate polymorphism from sequencing errors. Other errors can be found by aligning sequences to a high-quality reference sequence. The neighborhood quality standard (NQS) requires high Phred scores and good alignment to the reference sequence around a putative polymorphic site (51). POLYBAYES uses the NQS information and the multiple alignment of ESTs in a cluster to model the probability that a site is polymorphic (55); this allows it to detect paralogous genes as well as sequencing errors.METABOLIC AND REGULATORY PATHWAYS AND NETWORKS The previous section focuses on individual genes and proteins. Understanding how proteins interact, though, is crucial to drug development, as modifying one protein’s behavior affects others as well. Gene expression is often used as a surrogate for protein expression, as the former is easier to measure with current technologies, but they do not correlate perfectly (56). Both are used to model cellular dynamics (57, 58). Gene Expression Profiling ─ Gene expression is measured to find specific genes that are differentially expressed and groups of genes that are co-regulated (59). At present, the most popular technology for measuring gene expression is DNA “chips” or “microarrays,” which perform massively parallel Northern blots (60). Where scientists previously studied a few genes at a time, chips allow efficient genome-wide analysis of expression variation. Expression can also be measured with non-microarray methods, including Serial Analysis of Gene Expression (SAGE) and variants (61). Chip and SAGE results agree well on both absolute and relative expression levels (62), though proper analysis is needed to account for SAGE experimental biases (63-65).A differentially expressed gene may be implicated in the phenotypic differences between samples (66). A typical experiment compares expression at different developmental stages (67, 68), between normal and disease states (69, 70), or under differing environmental conditions (71). The EST cluster databases described above can also be used for expression profiling across multiple libraries (72).Co-regulation is recognized from similar expression levels in multiple states. Many methods have been used to measure profile similarity and to cluster genes with similar expression patterns (73-76). Some works cluster tissues as well, as co-regulation of genes associated with multiple regulatory mechanisms may only be recognized by examining a subset of the tissues (77).A common difficulty in prescribing medical treatment is differentiating between diseases with similar phenotypes. Microarray analyses can distinguish between normal and tumor tissue or between cancers (78-81). The latter paper includes an algorithmChap. ? Bioinformatics Pollock, Safer 5 that identifies multiple cancers in tissues without foreknowledge of different diseases. Microarrays are also used to compare cell lines. Cell line clusters based on expression levels differ from those based on drug responses (82); changes in expression can have striking effects on drug sensitivity and resistance. An elegant use of microarrays is to verify and supplement computational predictions of gene structure (83).Promoters, portions of genes that contain information to turn genes on and off, can be identified using clusters from microarray analysis (84). A motif that appears upstream of co-regulated genes may be a promoter (85-88). Promoters and expression clusters can be uncovered simultaneously (89, 90). Upstream regions of similar genes from multiple organisms can also be compared, with the required level of motif conservation depending on the phylogenetic distance between the organisms (91). Proteomics ─ Proteomics studies networks of proteins by measuring, among other things, protein expression. Protein activity is regulated by post-translational modification and degradation; these cannot yet be predicted from DNA sequence. Proteomics measures protein expression directly, not via gene expression, thus achieving better accuracy. Current work uses 2-dimensional polyacrylamide gel electrophoresis (2D-PAGE) and mass spectrometry. New separation and characterization technologies, such as protein microarrays and high-throughput chromatography, are being developed.In 2D-PAGE, samples to be compared are run through separate gels; corresponding spots that look different may indicate differential expression. Comparing spots has been time-consuming and error-prone, but new tools vastly improve both speed and accuracy (92). Spot intensity is measured to estimate expression level and mass spectrometry is used to identify proteins from spots of interest (93). Tools for analyzing gel images and mass spectra are described in (94). Proteins from organisms that are not well represented in protein databases can sometimes be identified based on proteins from other species using mutation-tolerant methods (95). Differential expression can be measured by labeling samples with different tags (96).Control sequences (standards) are typically used to calibrate mass spectrometry measurements. Internal standards added to samples can confound identification; external standards in different spots may not yield sufficient precision. Both suffer because calibration varies even over the time needed to process a sample plate. A recent approach corrects for systematic deviations by first using a low-precision database search. The proteins found are used to estimate calibration parameters and a second search uses greater precision, taking account of the recalibration (97). Metabolic and Regulatory Networks ─ Cellular processes result in large part from proteins interacting with each other and with other cellular components. Some research tries to identify interactions; other efforts use interactions to reverse-engineer metabolic and regulatory networks. Appropriate intervention points for therapeutic agents can be gleaned from an understanding of these networks. Comprehensive protein-protein interaction maps have been published for Saccharomyces cerevisiae (98) and Helicobacter pylori (99). Groups are working on maps for other organisms and for specific pathways in humans.Cross-species comparisons can be used to infer interactions. A phylogenetic profile is a list of species in which a protein is expressed. Proteins with the same profile are likely to be in a pathway that occurs only in certain organisms (100). Conservation of relative gene position identifies sets of genes that occur together, in the same order, in multiple species (101); this approach has been more informative in prokaryotes than in eukaryotes (102). Domain fusion looks for domains from two proteins in one organism that are contained in a single protein in a second organism (103, 104). Fusions may have an evolutionary advantage from more efficient interactions if the domains are in a common pathway. The protein with fused domains is called a “Rosetta stone” because it helps explain the relationship between the separated proteins in the other organism.6 Section─ Topics in Biology Allen, Ed.VStudying all tissues under all conditions to elucidate all interactions is a tall order. Recent work draws on existing research reports. Text mining tools extract interactions reported in document collections such as Medline. One approach is to compile a list of proteins and look for entries separated by appropriate verbs, such as two protein names with “phosphorylate” in between (105-107). A second approach parses text completely and looks for interactions (108, 109). The latter is more comprehensive but existing tools cannot fully evaluate all text. Other tools skip parsing altogether, using co-occurrence of protein names to deduce functional relationships (110, 111).Network complexity is illustrated by the Boehringer Mannheim “Biochemical Pathways” wall charts. Most reconstruction focuses on pathways, non-branching portions of networks. Databases of reconstructed pathways include KEGG (112), EcoCyc and MetaCyc (113), and DIP (114). Reconstructing pathways from interaction data is quite difficult. Some tools simultaneously recognize interactions and reconstruct pathways (115-117), often using statistical techniques (118-121).Mathematical models incorporate gene and protein expression to deduce pathway structure. Stoichiometric, thermodynamic, biochemical, and other constraints are used to model expression over time (122-127). Comparing pathways can yield functional insights; comparisons based on sequence (128) and on Enzyme Commission numbers of pathway components (129) have been developed. Reconstructed networks can predict output compounds based on input nutrients and required precursors (130).PROTEIN SECONDARY AND TERTIARY STRUCTURE Predicting protein three-dimensional structure is an important open problem not only in bioinformatics, but in general high performance computing. Structure being the key to function, determining a protein’s structure is a key step toward elucidating its role. The subfield of protein-ligand docking is useful in rational drug design. Laboratory prediction is time consuming and expensive, so researchers have been working on computerized prediction for several decades. Exact computational prediction is difficult (131, 132), but sophisticated algorithms to find approximate solutions continue to be developed.The Critical Assessment of Methods of Protein Structure Prediction (CASP) tracks progress in this field bi-annually. Computational groups predict structures of proteins whose structures have been found in the laboratory before the latter results are released. The predictions are assessed by experts. Tools are classified as using one of three approaches: comparative modeling looks for amino acid similarity to proteins of known structure; fold recognition predicts folds in regions that do not share amino acid similarity with known structures; and ab initio tools work directly from physical principles. Results of CASP 3 defined the technology as of 1998 (133-135). CASP 4 took place in 2000, and the upcoming publication of its results will define the current state of the art.WORKING WITH COMPLEX DATASETSThe difficulties of genomic analysis are compounded by data complexities. Datasets are large, ill-structured, and noisy. Combining data from multiple sources is challenging, as even basic terms have multiple meanings. A gene, for example, may refer to the stretch of genomic DNA that encodes a protein (sometimes including the promoter), the exons in that region, the coding sequence only, the resulting mRNA transcript, etc. Data Management and Integration ─ Most genomic data are stored in flat files or commercial relational databases, but object-oriented databases are finding increasing use. AceDB, a non-commercial database created as part of the Caenorhabditis elegans sequencing project, has a flexible data model that is easily extended to handle new kinds of data (136). It includes displays and other tools for use with genomic data.Gene expression, as a new research area, has benefited from integrating databases that are geographically dispersed, with different formats and terminology. Standards for storing, reporting, and exchanging data have been proposed (137-139); an alternative isChap. ? Bioinformatics Pollock, Safer 7 to combine data sources in a centralized, curated database (140, 141). Data storagestandards have also been proposed for protein interactions and pathways (142).Groups working in established areas often cannot retrofit standards to their existing databases. One way to enhance interoperability is to add standard, object-oriented interfaces (143-146). Another is to create tools that access distributed databases, but present a user interface that makes the databases appear integrated (147). A standard for exchanging many kinds of biological data, based on XML, is described in (148).Several databases contain different kinds of data integrated by locus. Often these contain similar data from different sources, such as multiple radiation hybrid maps (149). Sometimes they include different kinds of data (51, 150-153) or more complex representations, such as the genes contained in a metabolic pathway (154) or data on protein interaction and function (155). InterPro is perhaps the most comprehensive of such databases, and includes web links to many data sources (28).Gene Ontologies ─ Database integration is crucial for comparative genomics, large-scale inference of characteristics of novel genes in one organism from known properties of genes in other organisms. This requires automatic combination of different data sources. Ontologies are structured, controlled vocabularies. An ontology for biological function in Escherichia coli is described in (156). More recently, the Gene Ontology Consortium has built ontologies describing a gene’s molecular function, the biological processes that use it, and its cellular location (157). Tools for sharing ontologies exist (158), but results of using ontologies for database integration have yet to be published. Visualization ─ Computational methods cannot yet rival the ability of the human eye to pick out patterns. Many visualization tools show annotated genomic sequences. An early example is Genotator (159), which shows color-coded annotations such as predicted genes, promoters, splice sites, and putative homologies in parallel to the sequence. Different levels of detail can be viewed by zooming in and out. Artemis is a newer tool that allows calculations on the sequence or its CDS features, such as G+C content and amino acid properties such as hydrophobicity (16). This area continues to develop rapidly, with new tools constantly emerging. A recent focus is comparing both sequence and rearrangements, as discussed above (160-162).Most recent visualization tools build on the availability of web browsers. Re-usable interface components, typically in Java, for displaying common genomic objects are available (163, 164). Displays of 3D molecular structures may be the most striking visualization tools; experience with these and other display tools helps researchers understand how to take advantage of visual perception in the design of new tools (165). Acknowledgments ─ We thank A. Diber, M. Edelman, Z. Fligelman, R. Gill-More, N. Kaplan, G. Naveh, and P. Safer for their help.References1. Special issue: Biological databases, Nucleic Acids Res., 29, 1 (2001).2. International Human Genome Sequencing Consortium, Nature, 409, 860 (2001).3. J.C. Venter, M.D. Adams, E.W. Myers, P.W. Li, et al., Science, 291, 1304 (2001).4. P. Green, /UWGC/analysistools/phrap.htm, 2001.5. E.W. Myers, J. Comput. Biol., 2, 275 (1995).6. The Institute for Genomic Research, /softlab/assembler/, 2001.7. X. Huang and A. Madan, Genome Res., 9, 868 (1999).8. E.W. Myers, G.G. Sutton, A.L. Delcher, I.M. Dew, et al., Science, 287, 2196 (2000).9. M. Olivier, A. Aggarwal, J. Allen, A.A. Almendras, et al., Science, 291, 1298 (2001).10. J. Aach, M.L. Bulyk, G.M. Church, J. Comander, et al., Nature, 409, 856 (2001).11. F. Liang, I. Holt, G. Pertea, S. Karamycheva, et al., Nat. Genet., 25, 239 (2000).12. B. Ewing and P. Green, Nat. Genet., 25, 232 (2000).13. H. Roest Crollius, O. Jaillon, A. Bernot, C. Dasilva, et al., Nat. Genet., 25, 235 (2000).14. R. Sorek and M. Amitai, Nat. Biotechnol., 19, 196 (2001).15. S. Schwartz, Z. Zhang, K.A. Frazer, A. Smit, et al., Genome Res., 10, 577 (2000).。