Variable Structure Multiple Model Particle Filter for
运筹学英汉词汇ABC

运筹学英汉词汇(0,1) normalized ――0-1规范化Aactivity ――工序additivity――可加性adjacency matrix――邻接矩阵adjacent――邻接aligned game――结盟对策analytic functional equation――分析函数方程approximation method――近似法arc ――弧artificial constraint technique ――人工约束法artificial variable――人工变量augmenting path――增广路avoid cycle method ――避圈法Bbackward algorithm――后向算法balanced transportation problem――产销平衡运输问题basic feasible solution ――基本可行解basic matrix――基阵basic solution ――基本解basic variable ――基变量basic ――基basis iteration ――换基迭代Bayes decision――贝叶斯决策big M method ――大M 法binary integer programming ――0-1整数规划binary operation――二元运算binary relation――二元关系binary tree――二元树binomial distribution――二项分布bipartite graph――二部图birth and death process――生灭过程Bland rule ――布兰德法则branch node――分支点branch――树枝bridge――桥busy period――忙期Ccapacity of system――系统容量capacity――容量Cartesian product――笛卡儿积chain――链characteristic function――特征函数chord――弦circuit――回路coalition structure――联盟结构coalition――联盟combination me――组合法complement of a graph――补图complement of a set――补集complementary of characteristic function――特征函数的互补性complementary slackness condition ――互补松弛条件complementary slackness property――互补松弛性complete bipartite graph――完全二部图complete graph――完全图completely undeterministic decision――完全不确定型决策complexity――计算复杂性congruence method――同余法connected component――连通分支connected graph――连通图connected graph――连通图constraint condition――约束条件constraint function ――约束函数constraint matrix――约束矩阵constraint method――约束法constraint ――约束continuous game――连续对策convex combination――凸组合convex polyhedron ――凸多面体convex set――凸集core――核心corner-point ――顶点(角点)cost coefficient――费用系数cost function――费用函数cost――费用criterion ; test number――检验数critical activity ――关键工序critical path method ――关键路径法(CMP )critical path scheduling ――关键路径cross job ――交叉作业curse of dimensionality――维数灾customer resource――顾客源customer――顾客cut magnitude ――截量cut set ――截集cut vertex――割点cutting plane method ――割平面法cycle ――回路cycling ――循环Ddecision fork――决策结点decision maker决――策者decision process of unfixed step number――不定期决策过程decision process――决策过程decision space――决策空间decision variable――决策变量decision决--策decomposition algorithm――分解算法degenerate basic feasible solution ――退化基本可行解degree――度demand――需求deterministic inventory model――确定贮存模型deterministic type decision――确定型决策diagram method ――图解法dictionary ordered method ――字典序法differential game――微分对策digraph――有向图directed graph――有向图directed tree――有向树disconnected graph――非连通图distance――距离domain――定义域dominate――优超domination of strategies――策略的优超关系domination――优超关系dominion――优超域dual graph――对偶图Dual problem――对偶问题dual simplex algorithm ――对偶单纯形算法dual simplex method――对偶单纯形法dummy activity――虚工序dynamic game――动态对策dynamic programming――动态规划Eearliest finish time――最早可能完工时间earliest start time――最早可能开工时间economic ordering quantity formula――经济定购批量公式edge ――边effective set――有效集efficient solution――有效解efficient variable――有效变量elementary circuit――初级回路elementary path――初级通路elementary ――初等的element――元素empty set――空集entering basic variable ――进基变量equally liability method――等可能性方法equilibrium point――平衡点equipment replacement problem――设备更新问题equipment replacing problem――设备更新问题equivalence relation――等价关系equivalence――等价Erlang distribution――爱尔朗分布Euler circuit――欧拉回路Euler formula――欧拉公式Euler graph――欧拉图Euler path――欧拉通路event――事项expected value criterion――期望值准则expected value of queue length――平均排队长expected value of sojourn time――平均逗留时间expected value of team length――平均队长expected value of waiting time――平均等待时间exponential distribution――指数分布external stability――外部稳定性Ffeasible basis ――可行基feasible flow――可行流feasible point――可行点feasible region ――可行域feasible set in decision space――决策空间上的可行集feasible solution――可行解final fork――结局结点final solution――最终解finite set――有限集合flow――流following activity ――紧后工序forest――森林forward algorithm――前向算法free variable ――自由变量function iterative method――函数迭代法functional basic equation――基本函数方程function――函数fundamental circuit――基本回路fundamental cut-set――基本割集fundamental system of cut-sets――基本割集系统fundamental system of cut-sets――基本回路系统Ggame phenomenon――对策现象game theory――对策论game――对策generator――生成元geometric distribution――几何分布goal programming――目标规划graph theory――图论graph――图HHamilton circuit――哈密顿回路Hamilton graph――哈密顿图Hamilton path――哈密顿通路Hasse diagram――哈斯图hitchock method ――表上作业法hybrid method――混合法Iideal point――理想点idle period――闲期implicit enumeration method――隐枚举法in equilibrium――平衡incidence matrix――关联矩阵incident――关联indegree――入度indifference curve――无差异曲线indifference surface――无差异曲面induced subgraph――导出子图infinite set――无限集合initial basic feasible solution ――初始基本可行解initial basis ――初始基input process――输入过程Integer programming ――整数规划inventory policy―v存贮策略inventory problem―v货物存储问题inverse order method――逆序解法inverse transition method――逆转换法isolated vertex――孤立点isomorphism――同构Kkernel――核knapsack problem ――背包问题Llabeling method ――标号法latest finish time――最迟必须完工时间leaf――树叶least core――最小核心least element――最小元least spanning tree――最小生成树leaving basic variable ――出基变量lexicographic order――字典序lexicographic rule――字典序lexicographically positive――按字典序正linear multiobjective programming――线性多目标规划Linear Programming Model――线性规划模型Linear Programming――线性规划local noninferior solution――局部非劣解loop method――闭回路loop――圈loop――自环(环)loss system――损失制Mmarginal rate of substitution――边际替代率Marquart decision process――马尔可夫决策过程matching problem――匹配问题matching――匹配mathematical programming――数学规划matrix form ――矩阵形式matrix game――矩阵对策maximum element――最大元maximum flow――最大流maximum matching――最大匹配middle square method――平方取中法minimal regret value method――最小后悔值法minimum-cost flow――最小费用流mixed expansion――混合扩充mixed integer programming ――混合整数规划mixed Integer programming――混合整数规划mixed Integer ――混合整数规划mixed situation――混合局势mixed strategy set――混合策略集mixed strategy――混合策略mixed system――混合制most likely estimate――最可能时间multigraph――多重图multiobjective programming――多目标规划multiobjective simplex algorithm――多目标单纯形算法multiple optimal solutions ――多个最优解multistage decision problem――多阶段决策问题multistep decision process――多阶段决策过程Nn- person cooperative game ――n人合作对策n- person noncooperative game――n人非合作对策n probability distribution of customer arrive――顾客到达的n 概率分布natural state――自然状态nature state probability――自然状态概率negative deviational variables――负偏差变量negative exponential distribution――负指数分布network――网络newsboy problem――报童问题no solutions ――无解node――节点non-aligned game――不结盟对策nonbasic variable ――非基变量nondegenerate basic feasible solution――非退化基本可行解nondominated solution――非优超解noninferior set――非劣集noninferior solution――非劣解nonnegative constrains ――非负约束non-zero-sum game――非零和对策normal distribution――正态分布northwest corner method ――西北角法n-person game――多人对策nucleolus――核仁null graph――零图Oobjective function ――目标函数objective( indicator) function――指标函数one estimate approach――三时估计法operational index――运行指标operation――运算optimal basis ――最优基optimal criterion ――最优准则optimal solution ――最优解optimal strategy――最优策略optimal value function――最优值函数optimistic coefficient method――乐观系数法optimistic estimate――最乐观时间optimistic method――乐观法optimum binary tree――最优二元树optimum service rate――最优服务率optional plan――可供选择的方案order method――顺序解法ordered forest――有序森林ordered tree――有序树outdegree――出度outweigh――胜过Ppacking problem ――装箱问题parallel job――平行作业partition problem――分解问题partition――划分path――路path――通路pay-off function――支付函数payoff matrix――支付矩阵payoff――支付pendant edge――悬挂边pendant vertex――悬挂点pessimistic estimate――最悲观时间pessimistic method――悲观法pivot number ――主元plan branch――方案分支plane graph――平面图plant location problem――工厂选址问题player――局中人Poisson distribution――泊松分布Poisson process――泊松流policy――策略polynomial algorithm――多项式算法positive deviational variables――正偏差变量posterior――后验分析potential method ――位势法preceding activity ――紧前工序prediction posterior analysis――预验分析prefix code――前级码price coefficient vector ――价格系数向量primal problem――原问题principal of duality ――对偶原理principle of optimality――最优性原理prior analysis――先验分析prisoner’s dilemma――囚徒困境probability branch――概率分支production scheduling problem――生产计划program evaluation and review technique――计划评审技术(PERT) proof――证明proper noninferior solution――真非劣解pseudo-random number――伪随机数pure integer programming ――纯整数规划pure strategy――纯策略Qqueue discipline――排队规则queue length――排队长queuing theory――排队论Rrandom number――随机数random strategy――随机策略reachability matrix――可达矩阵reachability――可达性regular graph――正则图regular point――正则点regular solution――正则解regular tree――正则树relation――关系replenish――补充resource vector ――资源向量revised simplex method――修正单纯型法risk type decision――风险型决策rooted tree――根树root――树根Ssaddle point――鞍点saturated arc ――饱和弧scheduling (sequencing) problem――排序问题screening method――舍取法sensitivity analysis ――灵敏度分析server――服务台set of admissible decisions(policies) ――允许决策集合set of admissible states――允许状态集合set theory――集合论set――集合shadow price ――影子价格shortest path problem――最短路线问题shortest path――最短路径simple circuit――简单回路simple graph――简单图simple path――简单通路Simplex method of goal programming――目标规划单纯形法Simplex method ――单纯形法Simplex tableau――单纯形表single slack time ――单时差situation――局势situation――局势slack variable ――松弛变量sojourn time――逗留时间spanning graph――支撑子图spanning tree――支撑树spanning tree――生成树stable set――稳定集stage indicator――阶段指标stage variable――阶段变量stage――阶段standard form――标准型state fork――状态结点state of system――系统状态state transition equation――状态转移方程state transition――状态转移state variable――状态变量state――状态static game――静态对策station equilibrium state――统计平衡状态stationary input――平稳输入steady state――稳态stochastic decision process――随机性决策过程stochastic inventory method――随机贮存模型stochastic simulation――随机模拟strategic equivalence――策略等价strategic variable, decision variable ――决策变量strategy (policy) ――策略strategy set――策略集strong duality property ――强对偶性strong ε-core――强ε-核心strongly connected component――强连通分支strongly connected graph――强连通图structure variable ――结构变量subgraph――子图sub-policy――子策略subset――子集subtree――子树surplus variable ――剩余变量surrogate worth trade-off method――代替价值交换法symmetry property ――对称性system reliability problem――系统可靠性问题Tteam length――队长tear cycle method――破圈法technique coefficient vector ――技术系数矩阵test number of cell ――空格检验数the branch-and-bound technique ――分支定界法the fixed-charge problem ――固定费用问题three estimate approach一―时估计法total slack time――总时差traffic intensity――服务强度transportation problem ――运输问题traveling salesman problem――旅行售货员问题tree――树trivial graph――平凡图two person finite zero-sum game二人有限零和对策two-person game――二人对策two-phase simplex method ――两阶段单纯形法Uunbalanced transportation problem ――产销不平衡运输问题unbounded ――无界undirected graph――无向图uniform distribution――均匀分布unilaterally connected component――单向连通分支unilaterally connected graph――单向连通图union of sets――并集utility function――效用函数Vvertex――顶点voting game――投票对策Wwaiting system――等待制waiting time――等待时间weak duality property ――弱对偶性weak noninferior set――弱非劣集weak noninferior solution――弱非劣解weakly connected component――弱连通分支weakly connected graph――弱连通图weighed graph ――赋权图weighted graph――带权图weighting method――加权法win expectation――收益期望值Zzero flow――零流zero-sum game――零和对策zero-sum two person infinite game――二人无限零和对策。
心理学英文术语

感觉记忆(SM)—sensory memory短期记忆(STM)—short-term M.长期记忆(LTM)—long-term memory复诵——rehearsal预示(激发)——priming童年失忆症——childhood amnesia视觉编码(表征)——visual code(representation)听觉编码—acoustic code运作记忆——working memory语意性知识—semantic knowledge记忆扫瞄程序—memory scanning procedure竭尽式扫瞄程序-exhaustive S.P.自我终止式扫瞄—self-terminated S.程序性知识—procedural knowledge命题(陈述)性知识——propositional(declarative)knowledge 情节(轶事)性知识—episodic K.讯息处理深度—depth of processing精致化处理—elaboration登录特殊性—coding specificity记忆术—mnemonic位置记忆法—method of loci字钩法—peg word(线)探索(测)(激发)字—prime关键词——key word命题思考——propositional thought心像思考——imaginal thought行动思考——motoric thought概念——concept原型——prototype属性——property特征——feature范例策略——exemplar strategy语言相对性(假说)—linguistic relativity th.音素——phoneme词素——morpheme(字词的)外延与内涵意义—denotative & connotative meaning (句子的)表层与深层结构—surface & deep structure语意分析法——semantic differential全句语言—holophrastic speech过度延伸——over-extension电报式语言—telegraphic speech关键期——critical period差异减缩法——difference reduction方法目的分析——means-ends analysis倒推——working backward动机——motive自由意志——free will决定论——determinism本能——instinct种属特有行为——species specific驱力——drive诱因——incentive驱力减低说——drive reduction th.恒定状态(作用)—homeostasis原级与次级动机—primary & secondary M. 功能独立—functional autonomy下视丘侧部(LH)—lateral hypothalamus 脂肪细胞说——fat-cell theory.下视丘腹中部(VMH)—ventromedial H 定点论——set point th.CCK———胆囊调节激素第一性征——primary sex characteristic第二性征——secondary sex characteristic自我效能期望—self-efficiency expectancy内在(发)动机—intrinsic motive外在(衍)动机—extrinsic motive成就需求——N. achievement需求层级—hierarchy of needs自我实现——self actualization冲突——conflict多项仪——polygraph肤电反应——GSR(认知)评估——(cognitive appraisal)脸部回馈假说——facial feedback hypothesis(生理)激发——arousal挫折-攻击假说——frustration-aggression hy.替代学习——vicarious learning短期记忆(STM)—short-term M.长期记忆(LTM)—long-term memory复诵——rehearsal预示(激发)——priming童年失忆症——childhood amnesia视觉编码(表征)——visual code(representation)听觉编码—acoustic code运作记忆——working memory语意性知识—semantic knowledge记忆扫瞄程序—memory scanning procedure竭尽式扫瞄程序-exhaustive S.P.自我终止式扫瞄—self-terminated S.程序性知识—procedural knowledge命题(陈述)性知识——propositional(declarative)knowledge 情节(轶事)性知识—episodic K.讯息处理深度—depth of processing精致化处理—elaboration登录特殊性—coding specificity记忆术—mnemonic位置记忆法—method of loci字钩法—peg word(线)探索(测)(激发)字—prime关键词——key word命题思考——propositional thought心像思考——imaginal thought行动思考——motoric thought概念——concept原型——prototype属性——property特征——feature范例策略——exemplar strategy语言相对性(假说)—linguistic relativity th.音素——phoneme词素——morpheme(字词的)外延与内涵意义—denotative & connotative meaning (句子的)表层与深层结构—surface & deep structure语意分析法——semantic differential全句语言—holophrastic speech过度延伸——over-extension电报式语言—telegraphic speech关键期——critical period差异减缩法——difference reduction方法目的分析——means-ends analysis倒推——working backward动机——motive自由意志——free will决定论——determinism本能——instinct种属特有行为——species specific驱力——drive诱因——incentive驱力减低说——drive reduction th.恒定状态(作用)—homeostasis原级与次级动机—primary & secondary M. 功能独立—functional autonomy下视丘侧部(LH)—lateral hypothalamus 脂肪细胞说——fat-cell theory.下视丘腹中部(VMH)—ventromedial H 定点论——set point th.CCK———胆囊调节激素第一性征——primary sex characteristic第二性征——secondary sex characteristic 自我效能期望—self-efficiency expectancy 内在(发)动机—intrinsic motive外在(衍)动机—extrinsic motive成就需求——N. achievement需求层级—hierarchy of needs自我实现——self actualization冲突——conflict多项仪——polygraph肤电反应——GSR(认知)评估——(cognitive appraisal)脸部回馈假说——facial feedback hypothesis(生理)激发——arousal挫折-攻击假说——frustration-aggression hy.替代学习——vicarious learning 发展——development先天——nature后天——nurture成熟——maturation(视觉)偏好法——preferential method习惯法——habituation视觉悬崖——visual cliff剥夺或丰富(环境)——deprivation or enrichment of env. 基模——schema同化——assimilation调适——accommodation平衡——equilibrium感觉动作期——sensorimotor stage物体永久性——objective permanence运思前期——preoperational st.保留概念——conservation道德现实主义——moral realism具体运思期——concrete operational形式运思期——formal operational st.前俗例道德——pre-conventional moral俗例道德——conventional moral超俗例道德——post-conventional moral气质——temperament依附——attachment性别认定——gender identity性别配合——sex typing性蕾期——phallic stage恋亲冲突—Oedipal conflict认同——identification社会学习——social learning情结——complex性别恒定——gender constancy青年期——adolescence青春期—— -puberty第二性征——secondary sex characteristics 认同危机——identity crisis定向统合——identity achievement早闭型统合——foreclosure未定型统合——moratorium迷失型统合——identity diffusion传承——generativity心理动力——psycho-dynamics心理分析——psychoanalysis行为论——behaviorism心理生物观——psycho-biological perspective 认知——cognition临床心理学家-clinical psychologist谘商——counseling人因工程——human factor engineering组织——organization潜意识——unconsciousness完形心理学——Gestalt psychology感觉——sensation知觉——perception实验法——experimental method独变项——independent variable依变项——dependent V.控制变项——control V.生理——physiology条件化——conditioning学习——learning比较心理学——comparative psy.发展——development社会心理学——social psy.人格——personality心理计量学—psychometrics受试(者)——subject 实验者预期效应—experimenter expectancy effect 双盲法——double—blind实地实验——field experiment相关——correlation调查——survey访谈——interview个案研究——case study观察——observation心理测验——psychological test纹理递变度——texture gradient注意——attention物体的组群——grouping of object型态辨识—pattern recognition形象-背景——figure-ground接近律——proximity相似律——similarity闭合律——closure连续律——continuity对称律——symmetry错觉——illusion幻觉——delusion恒常性——constancy大小——size形状——shape位置—— location单眼线索——monocular cue线性透视——linear- perspective 双眼线索——binocular cue深度——depth调节作用——accommodation 重迭——superposition双眼融合——binocular fusion 辐辏作用——convergence双眼像差——binocular disparity向度—— dimension自动效应——autokinetic effect运动视差—— motion parallax诱发运动—— induced motion闪光运动—— stroboscopic motion上下文、脉络-context人工智能——artificial intelligence A.I. 脉络关系作用-context effect模板匹配——template matching整合分析法——analysis-by-synthesis 丰富性——redundancy选择性——selective无意识的推论-unconscious inferences 运动后效——motion aftereffect特征侦测器—feature detector激发性——excitatory抑制性——inhibitory几何子——geons由上而下处理—up-down process由下而上处理——bottom-up process连结者模式——connectionist model联结失识症——associative agnosia脸孔辨识困难症——prosopagnosia意识——conscious(ness)意识改变状态——altered states of consciousness无意识——unconsciousness前意识——preconsciousness内省法——introspection边缘注意——peripheral attention多重人格——multiple personality午餐排队(鸡尾酒会)效应—lunch line(cocktail party)effect 自动化历程——automatic process解离——dissociate解离认同失常——dissociative identity disorder快速眼动睡眠——REM dream非快速眼动睡眠—NREM dream失眠——insomnia显性与隐性梦——manifest & latern content心理活动性psychoactive冥想——meditation抗药性——tolerance戒断——withdrawal感觉剥夺——sensory deprivation物质滥用——substance abuse成瘾——physical addiction物质依赖——sub. dependence戒断症状——withdrawal symptom兴奋剂——stimulant幻觉(迷幻)剂——hallucinogen镇定剂——sedative抑制剂——depressant酒精中毒引起谵妄—delirium tremens麻醉剂——narcotic催眠——hypnosis催眠后暗示——posthypnotic suggestion 催眠后失忆posthypnotic amnesia超心理学——parapsychology超感知觉extrasensory perception ESP 心电感应——telepathy超感视——clairvoyance预知——precognition心理动力—psycokinesis PK受纳器——receptor绝对阈——absolute threshold 差异阈——difference threshold 恰辨差——-JND韦伯律——Weber''s law心理物理——psychophysical 费雪纳定律——Fechner''s law 频率——frequency振幅——amplitude音频——pitch基音——fundamental tone倍音——overtone和谐音——harmonic音色——timbre白色噪音——white noise鼓膜——eardrum耳蜗——cochlea卵形窗—oval window圆形窗——round window前庭——vestibular sacs半规管——semicircular canals角膜——cornea水晶体——lens虹膜——iris瞳孔——pupil网膜——retina睫状肌——ciliary muscle调节作用——accommodation脊髓——spinal cord反射弧——reflex arc脑干——brain stem计算机轴性线断层扫描——CAT或CT PET——正子放射断层摄影MRI——磁共振显影延脑——medulla桥脑——pons小脑——cerebellum网状结构——reticular formation RAS——网状活化系统视丘——thalamus下视丘——hypothalamus大脑——cerebrum脑(下)垂体(腺)—pituitary gland脑半球——cerebral hemisphere皮质——cortex胼胝体——corpus callosum边缘系统——limbic system海马体——hippocampus杏仁核——amygdala中央沟——central fissure侧沟——lateral fissure脑叶——lobe同卵双生子——identical twins异卵双生子—fraternal twins古典制约——classical conditioning操作制约——operant conditioning非制约刺激—(US unconditioned stimulus 非制约反应—(UR)unconditioned R.制约刺激——(CS)conditioned S.制约反应——(CR)conditioned R.习(获)得——acquisition增强作用——reinforcement消除(弱)——extinction自(发性)然恢复——spontaneous recovery前行制约—forward conditioning同时制约——simultaneous conditioning回溯制约——backward cond.痕迹制约——trace conditioning延宕制约—delay conditioning类化(梯度)——generalization(gradient)区辨——discrimination(次级)增强物——(secondary)reinforcer嫌恶刺激——aversive stimulus试误学习——trial and error learning效果率——law of effect正(负)性增强物—positive(negative)rei.行为塑造—behavior shaping循序渐进——successive approximation自行塑造—autoshaping部分(连续)增强—partial(continuous)R定比(时)时制—fixed ratio(interval)schedule FR或FI变化比率(时距)时制—variable ratio(interval)schedule VR或VI 逃离反应——escape R.回避反应—avoidance response习得无助——learned helplessness顿悟——insight学习心向—learning set隐内(潜在)学习——latent learning 认知地图——cognitive map生理回馈——biofeedback敏感递减法-systematic desensitization 普里迈克原则—Premack''s principle 洪水法——flooding观察学习——observational learning 动物行为学——ethology敏感化—sensitization习惯化——habituation联结——association认知学习——cognitional L.观察学习——observational L.登录、编码——encoding保留、储存——retention提取——retrieval回忆——(free recall全现心像、照相式记忆——eidetic imagery、photographic memory . 舌尖现象(TOT)—tip of tongue再认——recognition再学习——relearning节省分数——savings外显与内隐记忆——explicit & implicit memory记忆广度——memory span组集——chunk序列位置效应——serial position effect起始效应——primacy effect新近效应——recency effect心(情)境依赖学习——state-dependent L.无意义音节—nonsense syllable顺向干扰——proactive interference逆向干扰——retroactive interference闪光灯记忆——flashbulb memory动机性遗忘——motivated forgetting器质性失忆症—organic amnesia阿兹海默症——Alzheimer''s disease近事(顺向)失忆症—anterograde amnesia旧事(逆向)失忆—retrograde A.高沙可夫症候群—korsakoff''s syndrome 凝固理论—consolidation。
变结构交互式多模型滤波和平滑算法
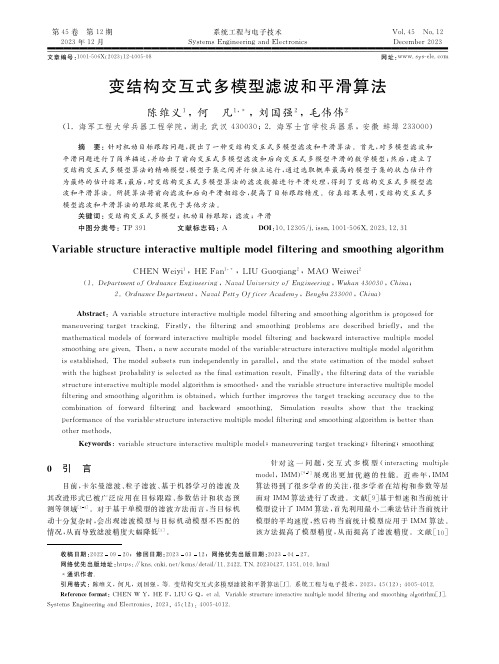
第45卷 第12期2023年12月系统工程与电子技术SystemsEngineeringandElectronicsVol.45 No.12December2023文章编号:1001 506X(2023)12 4005 08 网址:www.sys ele.com收稿日期:20220920;修回日期:20230312;网络优先出版日期:20230427。
网络优先出版地址:https:∥kns.cnki.net/kcms/detail/11.2422.TN.20230427.1351.010.html 通讯作者.引用格式:陈维义,何凡,刘国强,等.变结构交互式多模型滤波和平滑算法[J].系统工程与电子技术,2023,45(12):4005 4012.犚犲犳犲狉犲狀犮犲犳狅狉犿犪狋:CHENWY,HEF,LIUGQ,etal.Variablestructureinteractivemultiplemodelfilteringandsmoothingalgorithm[J].SystemsEngineeringandElectronics,2023,45(12):4005 4012.变结构交互式多模型滤波和平滑算法陈维义1,何 凡1, ,刘国强2,毛伟伟2(1.海军工程大学兵器工程学院,湖北武汉430030;2.海军士官学校兵器系,安徽蚌埠233000) 摘 要:针对机动目标跟踪问题,提出了一种变结构交互式多模型滤波和平滑算法。
首先,对多模型滤波和平滑问题进行了简单描述,并给出了前向交互式多模型滤波和后向交互式多模型平滑的数学模型;然后,建立了变结构交互式多模型算法的精确模型,模型子集之间并行独立运行,通过选取概率最高的模型子集的状态估计作为最终的估计结果;最后,对变结构交互式多模型算法的滤波数据进行平滑处理,得到了变结构交互式多模型滤波和平滑算法。
所提算法将前向滤波和后向平滑相结合,提高了目标跟踪精度。
自适应转移概率交互式多模型跟踪算法
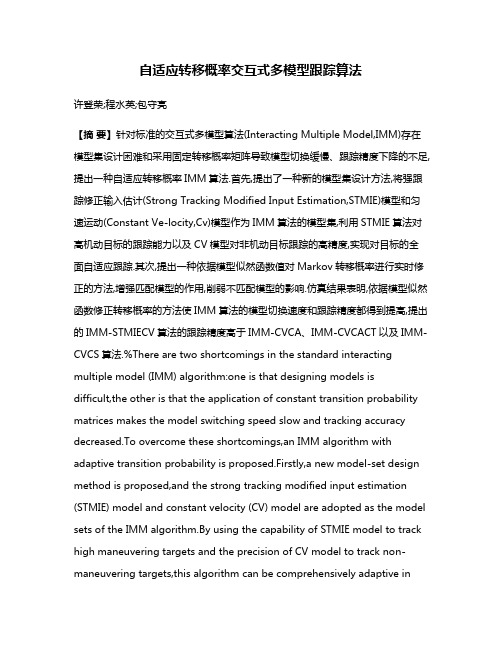
自适应转移概率交互式多模型跟踪算法许登荣;程水英;包守亮【摘要】针对标准的交互式多模型算法(Interacting Multiple Model,IMM)存在模型集设计困难和采用固定转移概率矩阵导致模型切换缓慢、跟踪精度下降的不足,提出一种自适应转移概率IMM算法.首先,提出了一种新的模型集设计方法,将强跟踪修正输入估计(Strong Tracking Modified Input Estimation,STMIE)模型和匀速运动(Constant Ve-locity,Cv)模型作为IMM算法的模型集,利用STMIE算法对高机动目标的跟踪能力以及CV模型对非机动目标跟踪的高精度,实现对目标的全面自适应跟踪.其次,提出一种依据模型似然函数值对Markov转移概率进行实时修正的方法,增强匹配模型的作用,削弱不匹配模型的影响.仿真结果表明,依据模型似然函数修正转移概率的方法使IMM算法的模型切换速度和跟踪精度都得到提高,提出的IMM-STMIECV算法的跟踪精度高于IMM-CVCA、IMM-CVCACT以及IMM-CVCS算法.%There are two shortcomings in the standard interacting multiple model (IMM) algorithm:one is that designing models is difficult,the other is that the application of constant transition probability matrices makes the model switching speed slow and tracking accuracy decreased.To overcome these shortcomings,an IMM algorithm with adaptive transition probability is proposed.Firstly,a new model-set design method is proposed,and the strong tracking modified input estimation (STMIE) model and constant velocity (CV) model are adopted as the model sets of the IMM algorithm.By using the capability of STMIE model to track high maneuvering targets and the precision of CV model to track non-maneuvering targets,this algorithm can be comprehensively adaptive intarget tracking.Secondly,a new method is proposed to modify the Markov transition probability in real time based on the likelihood values of the models,which enhances the effect of the matching model,and weakens the influence of the mismatched model.Simulation results show that the new method improves model switching speed and tracking precision of IMM algorithm,and the tracking precision of IMM-STM1ECV algorithm is higher than that of IMM-CVCA,IMM-CVCACT and IMM-CVCS algorithms.【期刊名称】《电子学报》【年(卷),期】2017(045)009【总页数】8页(P2113-2120)【关键词】机动目标跟踪;交互式多模型算法;Markov转移概率;修正的输入估计法;强跟踪【作者】许登荣;程水英;包守亮【作者单位】国防科技大学电子对抗学院,安徽合肥230037;国防科技大学电子对抗学院,安徽合肥230037;国防科技大学电子对抗学院,安徽合肥230037【正文语种】中文【中图分类】TN95机动目标跟踪[1~5]是目标跟踪领域的重点和难点问题,相关学者对此问题展开了广泛的研究.最开始采用的是自适应单模型算法对机动目标进行跟踪,然后发展为带机动检测的单模型跟踪算法,紧接着的是多模型算法,并逐渐成为机动目标跟踪的主流方法.多模型算法总结起来可分为三代[6]:自主多模型(AutonomousMultiple Model,AMM)估计、协作式多模型(Cooperating MultipleModel,CMM)估计、变结构多模型(Variable-Structure Multiple Model,VSMM)估计.在所有的多模型算法中,IMM算法通过合理的假设管理,一般被认为是混合系统中有效的混合估计方式,并得到了广泛的应用[7~9].虽然IMM算法被成功地应用,但是IMM算法跟踪性能仍受两个方面的限制.第一,由于IMM采用固定模型集,而现有的目标跟踪算法大多是基于模型的,因而IMM算法跟踪性能的好坏很大程度取决于所选的模型集.模型集的选择存在这样一个困境,即为了提高滤波精度需要尽可能多的增加模型,但是太多的模型除了大大增加计算量之外,反而降低了估计器的性能.第二,IMM算法中的Markov转移概率矩阵决定着输入交互的作用程度,一般根据先验信息人为选取为固定的主对角占优矩阵[10],不能依据后验信息对转移概率进行实时调整,不符合实际情况.当先验信息不确定时,采用固定Markov转移概率矩阵会造成跟踪精度的下降,因此,对Markov转移概率的实时估计一直是国内外研究的一个热点问题[10~13].本文针对IMM算法性能受限的两个方面而展开研究.首先,为了弥补IMM算法在模型集设计方面的不足,提出一种新颖的模型集设计方法.将STMIE算法与IMM算法相结合,把STMIE模型和CV模型作为IMM算法的模型集,利用STMIE算法对高机动目标的跟踪能力以及CV模型对非机动目标跟踪的高精度,实现对目标的全面自适应跟踪;同时针对STMIE算法采取扩维方法估计目标机动加速度导致与CV模型的状态向量维数不一致,采用无偏混合[14]方法.其次,针对采用IMM算法采取固定Markov转移概率矩阵会造成跟踪精度的下降的问题,提出一种简单有效的依据模型似然函数值在线修正转移概率的方法,并给出相应的递推公式.仿真结果校验了本文算法的优越性.2.1 修正的输入估计(Modified Input Estimation,MIE)算法在无先验信息条件下,假设机动目标离散的状态方程和量测方程可表示为:式中:Fk为状态转移矩阵;Gk为输入控制矩阵;Γk为过程噪声分布矩阵;Hk+1为量测矩阵;uk为目标未知的加速度;wk、vk分别为零均值的白色过程噪声和量测噪声序列,协方差矩阵分别为Qk和Rk,且任意时刻的wk与vk不相关.以二维情况为例,假设为k时刻目标的状态向量,zk=[xk yk]T为观测向量,则:其中T为采样间隔,由于Fk、Gk、Γk、Hk+1为时不变矩阵,为方便起见,省略时间下标,下文中简写为F、G、Γ、H.若将未知输入向量uk扩展为状态向量xk的一个新的分量,就可将式(1)的机动目标状态方程转化为非机动的目标状态方程,扩展的状态方程为[4]:量测方程为:zk+1=Hxk+1+vk+1=H(Fxk+Guk+Γwk)+vk+1=[HF HG][xkuk]T+HΓwk+vk+1定义HΓwk+vk+1.则可得扩维后的状态方程和量测方程:由式(6)、(7)即可利用卡尔曼滤波对目标的状态进行递推估计.2.2 强跟踪多重渐消因子在目标高机动或连续机动时,目标的加速度幅度变化大,容易造成滤波器发散.文献[5]结合强跟踪思想对MIE算法进行了改进.即引入强跟踪滤波器时变的多重渐消因子对滤波器的预测协方差矩阵进行调整,进而调节滤波器的增益矩阵.引入多重渐消因子的一步预测协方差矩阵的计算公式为:而可采用如下计算方法:式中:tr为求矩阵迹的算子;αi≥1(i=1,2,…,n)均为预先选定的常数,由先验信息确定;η为弱化因子,可以使状态估计值更加的平滑,通常根据经验选取;为矩阵Mk+1的对角线元素;Vk+1为实际输出残差序列的协方差矩阵,在实际中是未知的,可通过下式进行估算:式中为理论输出的残差序列,0<ρ≤1为遗忘因子,通常取ρ=0.95.2.3 强跟踪输入估计算法滤波步骤由上面的分析可以得到STMIE算法滤波流程如下[4,5]:步骤1 一步预测状态的一步预测:协方差的一步预测为:步骤2 一步更新状态的一步更新协方差的一步更新其中=HΓQk+1ΓTHT+Rk+2由式(19)可知,扩维后滤波器的增益由预测协方差矩阵和共同决定.通常当目标高机动时,滤波器的残差迅速增大,一步预测协方差可以通过多重渐消因子矩阵λk+1而及时调整,进而使滤波增益能得到合理地调整,显著增强了MIE算法对高机动目标的跟踪能力.3.1 IMM-STMIECV算法模型集的设计是多模型估计中最重要的问题之一,传统的IMM算法一般选用CV 模型和匀加速度(Constant Acceleration,CA)模型的组合作为模型集进行混合估计或者选用CV模型、CA模型以及转弯速率未知的协同转弯(Coordinate Turn,CT)模型[15]作为模型集进行混合估计,来实现对目标非机动时的精确跟踪以及目标加速或转弯机动时的跟踪.另外采用相关噪声模型(如当前统计(Current Statistical,CS)模型)和CV模型的组合也比较常见,但当目标高机动时或者连续机动时,往往会因为模型竞争或模型不匹配导致滤波估计精度不理想.STMIE算法在目标多种机动情况下相比其他通用型跟踪算法拥有更高的跟踪精度,但降低了对非机动目标的滤波精度,因而从理论上来说,选用STMIE模型和CV模型的组合的作为IMM算法的模型集就能实现对目标的全面自适应跟踪,并记采用这种模型集设计的IMM算法为IMM-STMIECV算法.但由于二维空间中CV模型的状态维数为4,而STMIE模型扩维后状态向量的维数为6,状态维数不一致,而IMM算法要实现状态和协方差的混合交互,所有状态向量必须转换成一致的状态变量[16].模型状态维数不同以及状态元素不一致时如何实现IMM算法的混合估计在文献[14,16,17]都有所提及,本文采用的是文献[14]提出的无偏混合方法,该算法在混合估计过程中不会带来偏差,且具有更好的一致性.3.2 IMM-STMIECV算法步骤IMM估计算法是递推进行的.每步递推包括4个步骤,即模型条件重初始化、模型条件滤波、模型概率更新、估计融合.现给出IMM-STMIECV算法由k-1时刻到k 时刻的递推步骤.步骤1 模型条件重初始化首先计算混合概率,然后采用2.1节介绍的无偏混合方法进行混合估计,即可得到重初始化的状态和协方差矩阵.步骤2 模型条件滤波由步骤1得到CV模型和STMIE模型估计的初始条件.然后每个模型按如下步骤进行递推其中式(22)~(27)中,上标j表示其中的第j个模型,下同,j∈{1,2,…,M},M为模型总个数,此处M=2.步骤3 模型概率更新=Pr{rk=j|Zk}==式中步骤4 估计融合需要注意的是由于STMIE算法中因此估计的状态向量取决于zk+1,造成了一个采样间隔的延迟;而基于CV模型的滤波器k时刻的状态更新值取决于zk.即模型条件滤波中STMIE模型是利用k+1时刻的量测更新k时刻的状态和协方差,而CV模型是利用k时刻的量测进行状态和协方差更新.因此模型似然函数的计算分别利用了k时刻和k+1时刻的量测,因而实际上对式(28)的模型概率计算进行了近似处理.由于量测值为位置信息,在采样间隔不是太大且目标离传感器较远时,其相对大小改变较小,这种近似是合理的.上述IMM算法中假定系统模型切换过程是一个Markov过程,即模型跳变服从Markov链[7],设Markov概率转移矩阵为则:式中:M为模型的总个数,rk=j表示k时刻系统匹配的模型为j模型表示模型i转移到模型j的概率,且对于任意i∈{1,2,…,M}有标准的IMM算法中Markov转移概率是预先给定的,但是由于目标的机动性和先验信息不确定,这种预先设定的Markov矩阵并不能很好的反应目标真实运动模式的切换,造成估计误差增大[11].因此在滤波过程中需要利用后验信息对IMM算法的转移概率进行自适应修正,使之符合实际情况.文献[10]和文献[13]分别提出利用模型概率变化的后验信息和利用误差压缩率[13]的后验信息对模型转移概率进行修正,而本文提出一种根据模型似然函数值对模型转移概率进行修正的方法.模型似然函数值中包含了当前的量测信息,其大小很好的反应了模型与目标真实运动模式匹配程度.假设k时刻子模型j的模型似然函数值为其值相对其他模型似然函数值越大说明子模型j与目标真实运动状态越匹配,则其他模型向这一匹配模型的转移概率就应越大,反之,其他模型转向这一模型概率就应越小,而本文就是基于这思想对转移概率进行自适应调整.其具体方法为:对Markov概率转移矩阵的元素利用如下方法进行修正,即:式中为调节因子,控制调节的快慢.考虑到k时刻某一模型转向其他模型的概率总和为1,因此需要利用这一性质对式(33)进行修正.即:从式(34)可以看到,若k时刻模型j的模型似然函数值比模型i的大,即子模型j比子模型i与目标真实状态更匹配,通过修正后由模型i转移到模型j的概率也随之增大,因而在下一时刻滤波前的模型交互中,模型似然函数值大的子模型在交互估计输出中所占的比重更大;相反,若k时刻模型j的模型似然函数值比模型i的小,通过修正后由模型i转移到模型j的概率也随之减小,模型似然函数值小的子模型在交互估计输出中所占的比重减小.通过这种利用量测信息实时自适应地修正模型转移概率的方法,增强了匹配模型的作用,压缩了非匹配模型的影响.但是式(34)的转移概率设计仍存在不足,可能导致矩阵主对角元素的某些值越来越大,而某些值会越来越小,即匹配模型转移到自身的概率会越来越大,而不匹配模型转移到自身的概率会越来越小.但当目标机动使得不匹配模型变成匹配模型时,会导致当前匹配模型转移到之前的匹配模型的概率仍很大,转移到自身的概率仍较小,造成模型切换比较缓慢.鉴于此,对式(34)做如下调整,在自适应调整转移概率的同时保持Markov矩阵的主对角占优.对Markov矩阵的主对角线元素设定一个阈值Th,若主对角线元素则对其所在行的元素作如下修改:若则其所在行的元素保持不变.显然,经过修正后仍满足且的要求.同时经过式(34)、(35)修正,模型切换过程中不仅能更多地利用匹配模型的信息,削弱非匹配模型的影响,而且能增强算法的稳定性,使跟踪精度和收敛速度都得到提高.图1为提出的自适应转移概率IMM-STMIECV算法流程图.从两个方面来验证本文提出算法的性能,首先验证本文提出的转移概率自适应算法的性能,然后验证本文提出模型集设计方法性能的好坏.5.1 转移概率自适应修正算法性能分析选择文献[6]中的机动场景3来测试本文提出的自适应转移概率算法的性能,并同时与标准的IMM算法、文献[10]提出的依据模型概率的变化修正转移概率以及文献[13]提出的根据误差压缩率修正转移概率的方法进行对比分析.目标从x0=[8000,600,8000,600](m,m/s,m,m/s)出发,并在开始的20s匀速运动.接下来,目标在21~110s内进行协同转弯运动,转弯速率为1/30(rad/s).在此之后,目标保持匀速直线运动50s直至运动结束.此处目标只有两种运动模式,即匀速运动和协同转弯运动.为了测试这4种算法的性能,同时考虑到需要比较模型切换速度以及模型概率估计,所有算法都采用匹配模型集,即所有IMM算法都采用CV模型和转弯角速度已知( ω= 1/30(rad/s))的CT模型作为IMM算法的模型集.CV、CT模型过程噪声协方差矩阵为QCV=ΓCVdiag(qcv1,qcv2)(ΓCV)T,QCT=ΓCTdiag(qct1,qct2)(ΓCT)T其中:qcv1=qcv2=qct1=qct2=0.01m2·s-4.本文算法的调节因子γ=1/2,Markov概率转移矩阵主对角线元素的阈值Th=0.7.4种算法的初始Markov概率转移矩阵及模型初始概率为:量测为线性量测,即zk=[xk yk]T,量测协方差矩阵为R=diag(1002, 1002),采样间隔T=1s.采用两点初始化[18]的方法,Monte Carlo仿真实验100次.图2为4种方法的均方根误差(RMSE)曲线,图3给出了单次实验中各算法的模型概率变化曲线.仿真结果表明,3种时变转移概率算法的跟踪性相比标准的IMM算法都用不同程度的提升.但相对来说,本文方法拥有更好的跟踪性能,具体表现在:(1)拥有更高的跟踪精度,从图2可以看到,无论是位置均方根误差还是速度均方根,本文方法的稳态误差都是最小的,跟踪精度较标准的IMM算法有大幅度提升,且好于文献[10]和文献[13]方法.(2)模型切换更迅速,收敛速度更快,模型概率估计更加准确.本文方法完成模型切换所用的时间平均只有7s左右,标准的IMM算法和文献[10]方法需要15s左右的时间才能完成模型切换,而文献[13]方法需要30s左右的时间才能实现从一个模型到另一个模型的过渡.在模型概率估计方面,本文方法拥有绝对的优势,远好于其他三种方法,除最开始10s左右滤波器在调整导致模型概率估计不太准确外,其他时间模型概率变化与目标真实的模式的变化十分接近.说明算法增强了匹配模型的作用,削弱了非匹配模型的影响.本文方法的缺陷在于峰值误差(最大误差)较大,尤其是当目标转弯运动变为匀速运动时,误差增大明显.原因在于本文提出的自适应转移概率IMM算法极大的削弱了非匹配模型的作用,但当目标机动非匹配模型变成匹配模型时,模型切换有一定时间的滞后,导致模型切换过程中误差较大.5.2 本文算法模型集设计性能分析上述实验为已知目标真实运动模式的情况下采用匹配模型集验证本文提出的自适应转移概率方法对标准的IMM算法性能的提高.但是现实情况中,目标的机动类型,机动强度以及何时机动都是未知的,因而IMM算法存在模型集设计的难题.但目标可能的运动模式概括起来又是已知的,即主要有匀速运动,匀加速运动,以及转弯运动.因而传统的IMM算法采用CV、CA模型的组合或CV、CA、CT模型的组合实现对机动目标跟踪,利用相关噪声模型与CV模型的组合也比较常见.但是由于目标的机动程度未知,上述几种方法的性能通常不太理想,为此本文提出一种新颖的模型集设计方法,下面对本文提出模型集设计的性能进行验证.选取了包含三种运动模式的目标连续机动的运动场景,用本文提出的IMM-STMIECV算法、IMM-CVCA算法、IMM-CVCACT算法以及IMM-CVCS算法分别对目标进行跟踪,并以均方根误差为性能评价指标.需要指出的是,这里的CT模型是转弯角速度未知的转弯模型,其状态方程是非线性的,利用扩展卡尔曼滤波(Extended Kalman Filter,EKF)算法进行滤波.假设目标初始状态x0=[10000,-160,2000,50](m,m/s,m,m/s),在二维平面内运动120s.分别在0~20s、40~60s、110~120s进行匀速运动,20~40s和60~80s进行匀速转弯运动,80~110s进行匀加速运动,20~40s,60~80s时角速度分别为π/18 (rad/s)和-π/20 (rad/s),80~110s时两个方向的加速度分别为5m/s2,5m/s2.图4为目标运动的真实航迹图.各算法的参数设置如下:CV模型的噪声协方差与4.1节相同,CA模型的过程噪声协方差为QCA=ΓCAdiag(qca1,qca2)(ΓCA)T,CT模型的过程噪声协方差为QCT=ΓCTdiag(qct1,qct2,qct3)(ΓCT)T,式中:qca1=qca2=10m2·s-4;qct1=qct2=0.01m2·s-4,qct3=0.001rad2·s-4;STMIE中wk的方差为预先选定的常数αi=1,弱化因子η=1.同时为了比较的公平性,4种IMM算法都采用本文提出的自适应转移概率方法.IMM-STMIECV、IMM-CVCA、IMM-CVCS的模型初始概率都为[0.5,0.5]T、IMM-CVCACT算法模型初始概率为[1/3,1/3,1/3]T.4中算法的初始Markov概率转移矩阵分别为:自适应转移概率算法的调节因子γ=1/3,Markov概率转移矩阵主对角线元素的阈值Th=0.7.量测为线性量测,即zk=[xk yk]T,量测协方差矩阵为R=diag(1002, 1002),采样间隔T=1s.采用三点初始化[18]方法,Monte Carlo实验200次.图5分别显示的是这四种算法的位置和速度均方根误差曲线,同时对各算法观测时间内平均均方根误差,峰值误差(20s以后)等进行了统计,结果如表1所示.表1统计的结果表明,本文算法的平均位置均方根误差和位置峰值误差都最小,而平均速度均方根误差和速度峰值误差和IMM-CVCACT算法相当,但小于IMM-CVCA算法以及IMM-CVCS算法.从图5可以看到,无论目标在哪种运动模式,本文算法的位置均方根误差几乎都是这几种算法中最小的,而速度均方根误差只有在目标转弯机动时稍大于IMM-CVCACT算法,说明采用STMIE模型和CV模型的组合的IMM算法具有良好的适应性,能实现对目标的全面自适应跟踪,跟踪精度较传统的模型集设计方法得到了显著的提高.而在收敛速度方面,虽然当目标机动时,本文方法的跟踪误差也明显增大,但能迅速收敛,误差保持在一个较小的值,而其他方法收敛速度相对较慢,且目标机动时跟踪精度低.另外,采用STMIE模型和CV模型组合的设计,在量测误差相同但机动性更强的条件下,算法的峰值误差较4.1节得到了改善,原因在于STMIE也能实现对匀速目标的跟踪,而转弯角速度已知的CT模型不能实现对匀速目标的跟踪.综上,本文提出的自适应转移概率IMM-STMIECV算法由于采用新颖的模型集设计同时依据量测信息实时修正转移概率,算法能实现对目标全面的自适应跟踪,无论目标是否机动,相比其他三种算法跟踪精度更高,模型切换迅速,收敛速度快,同时只采用两个跟踪模型,算法复杂度相对较低.算法的跟踪性能较传统方法有较大的提高,是一种性能较为优越的机动目标跟踪算法.本文针对机动目标跟踪问题,在分析标准IMM算法存在的不足的基础上,提出一种自适应转移概率的交互式多模型算法.仿真结果表明采用STMIE模型和CV模型的组合,能提高IMM算法的适应性,无论目标是否机动以及机动程度如何,在无目标任何先验信息的条件下相对其他通用型跟踪算法拥有更高的跟踪精度.采用自适应转移概率算法,能根据量测信息实时对转移概率进行修正,增强匹配模型的作用,削弱非匹配模型的影响,使模型切换更迅速更合理,对模型概率的估计更加准确,收敛速度更快,算法的跟踪精度得到了显著的提高.【相关文献】[1]Xu L,Li X R,Duan Z.Hybrid grid multiple-model estimation with application to maneuvering target tracking[J].IEEE Transactions on Aerospace and Electronic Systems,2016,52(1):122-136.[2]Sithiravel R,Mcdonald M,Balaji B,et al.Multiple model spline probability hypothesis density filter[J].IEEE Transactions on Aerospace and Electronic Systems,2016,52(3):1210-1226.[3]Nemeth C,Fearnhead P,Mihaylova L.Sequential monte carlo methods for state and parameter estimation in abruptly changing environments[J].IEEE Transactions on Signal Processing,2014,62(5):1245-1255.[4]Khaloozadeh H,Karsaz A.Modified input estimation technique for tracking maneuvering targets[J].IET Radar Sonar Navigation,2009,3(1):30-41.[5]杨金龙,姬红兵,樊振华.强跟踪输入估计概率假设密度多机动目标跟踪算法[J].控制理论与应用,2011,28(8):1164-1170.Yang Jin-long,Ji Hong-bing,Fan Zhen-hua.Strong tracking modified input estimation probability hypothesis density for multiple maneuvering targets tracking [J].Journal of Control Theory and Applications,2011,28(8):1164-1170.(in Chinese)[6]Lan J,Li X R,Mu C.Best model augmentation for variable-structure multiple-model estimation[J].Aerospace and Electronic Systems,IEEE Transactions on,2011,47(3):2008-2025.[7]Li W,Jia Y.An information theoretic approach to interacting multiple model estimation[J].IEEE Transactions on Aerospace and Electronic Systems,2015,51(3):1811-1825.[8]刘先省,胡振涛,金勇,等.基于粒子优化的多模型粒子滤波算法[J].电子学报,2010,38(2):301-306. Liu Xian-xing,Hu Zhen-tao,Jin Yong,et al.A novel multiple model particle filter algorithm based on particle optimization[J].Acta Electronica Sinica,2010,38(2):301-306.(in Chinese) [9]万九卿,梁旭,马志峰.基于自适应观测模型交互多模型粒子滤波的红外机动目标跟踪[J].电子学报,2011,39(3):602-608.Wan Jiu-qing,Liang Xu,Ma Zhi-feng.Infrared maneuvering target tracking based on IMM-PF with adaptive observation model[J].Acta Electronica Sinica,2011,39(3):602-608.(in Chinese)[10]郭志,董春云,蔡远利,等.时变转移概率IMM-SRCKF机动目标跟踪算法[J].系统工程与电子技术,2015(1):24-30.Guo Zhi,Dong Chun-yun,Cai Yuan-li,et al.Time-varying transition probability based IMM-SRCKF algorithm for maneuvering target tracking[J].Journal of Systems Engineering and Electronics,2015(1):24-30.(in Chinese)[11]Wang G.ML Estimation of transition probabilities in jump markov systems via convex optimization[J].IEEE Transactions on Aerospace and Electronic Systems,2010,46(3):1492-1502.[12]Guo R,Shen M,Huang D,et al.Recursive estimation of transition probabilities for jump Markov linear systems under minimum Kullback-Leibler divergence criterion[J].Iet Control Theory & Applications,2015,9(17):2491-2499.[13]臧荣春,崔平远.马尔可夫参数自适应IFIMM算法研究[J].电子学报,2006,34(3):521-524. Zang Rong-chun,Cui Ping-yuan.Research on adaptive markov parameter IFIMM algorithm[J].Acta Electronica Sinica,2006,34(3):521-524.(in Chinese)[14]Yuan T,Bar-Shalom Y,Willett P,et al.A multiple IMM estimation approach with unbiased mixing for thrusting projectiles[J].IEEE Transactions on Aerospace Electronic Systems,2012,48(4):3250-3267.[15]Zhu L,Cheng X.High manoeuvre target tracking in coordinated turns[J].IET Radar Sonar Navigation,2015,9(8):1078-1087.[16]Blackman S S,Popoli R.Design and Analysis of Modern TrackingSystems[M].Boston:Artech House,1999.224-240.[17]Farrell W.Interacting multiple model filter for tactical ballistic missile tracking[J].IEEE Transactions on Aerospace and Electronic Systems,2008,44(2):418-426.[18]何友,修建娟,关欣,等.雷达数据处理及应用(第三版)[M].北京:电子工业出版社,2013.44-47.。
多元回归模型英语

多元回归模型英语Multivariate Regression ModelMultivariate regression analysis is a powerful statistical technique that allows researchers to examine the relationship between multiple independent variables and a single dependent variable. This approach is particularly useful in situations where there are multiple factors that may influence a particular outcome or phenomenon. By considering the combined effects of these variables, researchers can gain a more comprehensive understanding of the underlying dynamics and make more accurate predictions.One of the key advantages of multivariate regression is its ability to account for the complexities of real-world situations. In many cases, the relationship between a dependent variable and a single independent variable may be oversimplified or incomplete. By incorporating multiple predictors, the multivariate regression model can capture the nuances and interactions that shape the outcome of interest.The general form of a multivariate regression model can be expressed as:Y = β₀ + β₁X₁ + β₂X₂ + ... + βₖXₖ + εWhere:- Y is the dependent variable- X₁, X₂, ..., Xₖ are the independent variables- β₀ is the y-intercept (the value of Y when all Xs are zero)- β₁, β₂, ..., βₖ are the regression coefficients, which r epresent the change in Y associated with a one-unit change in the corresponding X, holding all other variables constant- ε is the error term, which represents the unexplained variation in YThe regression coefficients in a multivariate model can be interpreted as the partial regression coefficients, meaning they represent the unique contribution of each independent variable to the prediction of the dependent variable, while controlling for the effects of the other variables in the model.One of the key assumptions of multivariate regression is that the independent variables are linearly related to the dependent variable. This means that the relationship between each X and Y can be approximated by a straight line. Additionally, the model assumes that the e rrors (ε) are normally distributed, have a mean of zero, and have constant variance (homoscedasticity).To assess the overall fit of the multivariate regression model, researchers often use the coefficient of determination, or R-squared (R²). This statist ic represents the proportion of the total variation in the dependent variable that is explained by the independent variables in the model. A higher R² indicates a better fit, with a value of 1 indicating that the model explains all of the variation in the dependent variable.In addition to the overall model fit, researchers may also examine the statistical significance of individual regression coefficients using t-tests or F-tests. These tests help determine whether the observed relationships between the independent variables and the dependent variable are likely to have occurred by chance or are statistically significant.Multivariate regression models have a wide range of applications across various fields, including economics, social sciences, healthcare, and engineering. For example, in the field of marketing, a multivariate regression model might be used to investigate the factors that influence customer satisfaction, such as product quality, customer service, and pricing. In the healthcare sector, a multivariate model could be used to examine the relationship between patient characteristics (e.g., age, gender, medical history) and the likelihood of a particular health outcome.One of the key challenges in using multivariate regression is the issue of multicollinearity, which occurs when two or more independent variables are highly correlated with each other. This can lead to unstable and unreliable estimates of the regression coefficients, making it difficult to determine the unique contribution of each variable. Researchers often use diagnostic tools, such as the variance inflation factor (VIF), to detect and address multicollinearity in their models.Despite these challenges, multivariate regression remains a valuable tool for researchers and analysts who are interested in understanding the complex relationships between multiple variables. By incorporating multiple predictors, this approach can provide a more comprehensive and nuanced understanding of the factors that influence a particular outcome or phenomenon.。
多自变量 结构方程
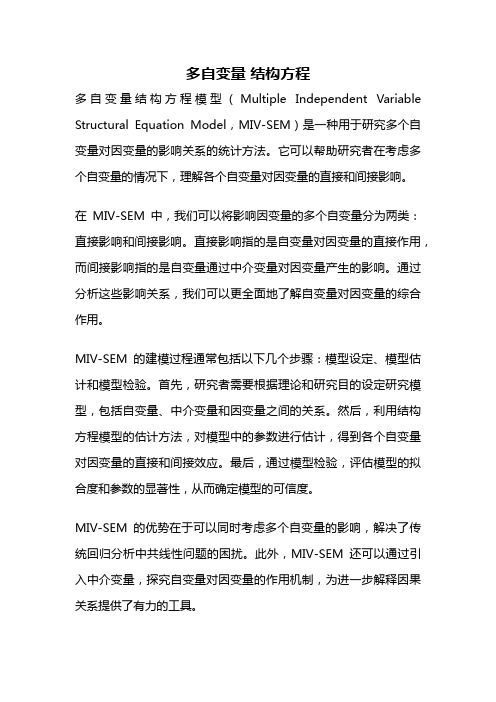
多自变量结构方程多自变量结构方程模型(Multiple Independent Variable Structural Equation Model,MIV-SEM)是一种用于研究多个自变量对因变量的影响关系的统计方法。
它可以帮助研究者在考虑多个自变量的情况下,理解各个自变量对因变量的直接和间接影响。
在MIV-SEM中,我们可以将影响因变量的多个自变量分为两类:直接影响和间接影响。
直接影响指的是自变量对因变量的直接作用,而间接影响指的是自变量通过中介变量对因变量产生的影响。
通过分析这些影响关系,我们可以更全面地了解自变量对因变量的综合作用。
MIV-SEM的建模过程通常包括以下几个步骤:模型设定、模型估计和模型检验。
首先,研究者需要根据理论和研究目的设定研究模型,包括自变量、中介变量和因变量之间的关系。
然后,利用结构方程模型的估计方法,对模型中的参数进行估计,得到各个自变量对因变量的直接和间接效应。
最后,通过模型检验,评估模型的拟合度和参数的显著性,从而确定模型的可信度。
MIV-SEM的优势在于可以同时考虑多个自变量的影响,解决了传统回归分析中共线性问题的困扰。
此外,MIV-SEM还可以通过引入中介变量,探究自变量对因变量的作用机制,为进一步解释因果关系提供了有力的工具。
然而,MIV-SEM也存在一些限制。
首先,MIV-SEM对样本量要求较高,通常需要较大的样本才能得到可靠的结果。
其次,模型设定需要基于充分的理论依据,否则可能导致模型的拟合度不佳。
此外,MIV-SEM对数据的要求较高,需要满足变量间的线性关系假设和正态分布假设。
在实际应用中,研究者需要在使用MIV-SEM之前仔细考虑研究问题的特点和数据的可行性。
如果研究问题涉及多个自变量,且自变量之间存在相互影响或中介作用,那么MIV-SEM是一种很好的分析工具。
通过MIV-SEM的应用,我们可以更加全面地了解自变量对因变量的影响关系,为相关领域的研究和实践提供科学依据。
利用红外光谱仪的网球比赛运动目标跟踪方法
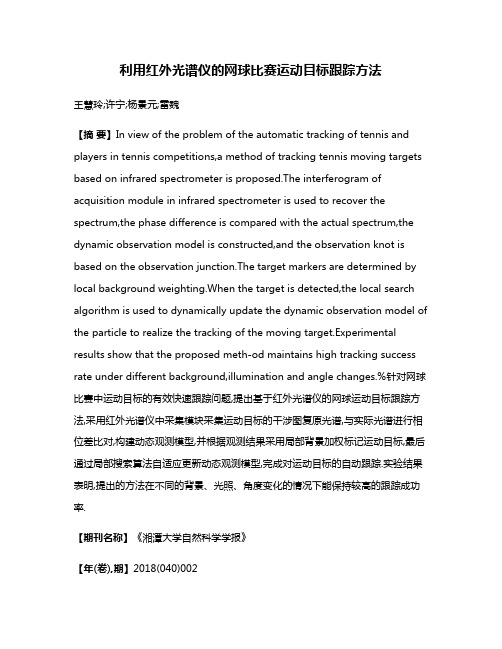
利用红外光谱仪的网球比赛运动目标跟踪方法王慧玲;许宁;杨景元;雷魏【摘要】In view of the problem of the automatic tracking of tennis and players in tennis competitions,a method of tracking tennis moving targets based on infrared spectrometer is proposed.The interferogram of acquisition module in infrared spectrometer is used to recover the spectrum,the phase difference is compared with the actual spectrum,the dynamic observation model is constructed,and the observation knot is based on the observation junction.The target markers are determined by local background weighting.When the target is detected,the local search algorithm is used to dynamically update the dynamic observation model of the particle to realize the tracking of the moving target.Experimental results show that the proposed meth-od maintains high tracking success rate under different background,illumination and angle changes.%针对网球比赛中运动目标的有效快速跟踪问题,提出基于红外光谱仪的网球运动目标跟踪方法,采用红外光谱仪中采集模块采集运动目标的干涉图复原光谱,与实际光谱进行相位差比对,构建动态观测模型,并根据观测结果采用局部背景加权标记运动目标,最后通过局部搜索算法自适应更新动态观测模型,完成对运动目标的自动跟踪.实验结果表明,提出的方法在不同的背景、光照、角度变化的情况下能保持较高的跟踪成功率.【期刊名称】《湘潭大学自然科学学报》【年(卷),期】2018(040)002【总页数】4页(P46-49)【关键词】红外光谱仪;网球;背景加权;局部搜索;目标跟踪【作者】王慧玲;许宁;杨景元;雷魏【作者单位】西藏大学教育学院,西藏拉萨850000;西藏大学信息科学技术学院,西藏拉萨850000;西藏大学教育学院,西藏拉萨850000;西藏大学教育学院,西藏拉萨850000【正文语种】中文【中图分类】O657网球比赛中,对球及球员的有效快速跟踪对于比赛视频的录制至关重要,而自动跟踪球和球员面临着一些挑战.如球在不同角度或视野中都非常小,且很多时候球可能是不可见的.尤其在跟踪网球的背景下,由于球不仅小而且速度快,噪声是一个很大的问题,图像中经常出现噪声,干扰目标检测的过程[1-2].文献[3]提出一种背景差分检测法,能够消除部分背景噪声,但是不能消除全部噪声,且通常需要额外的滤波操作.为了克服由于拍摄的图像质量差而引起的限制,文献[4]提出了一种改进的背景差分检测方法,使用背景差分检测获得二值图像映射和测量集,并应用高斯混合模型处理目标跟踪中的一些不确定性因素,但是在跟踪检测大的图像或物体时,会消耗更多的处理时间.文献[5]中提出了基于多假设跟踪器的数据关联方法,对一组离散度检测信号进行分区用以跟踪,但是并没有对多目标进行有效跟踪.文献[6]提出了针对球员的检测和跟踪方法,通过支持向量分类获得球员的准确球场分割区域,并采用粒子滤波器进一步提升性能.文献[7]针对网球视频的自动标注提出了网球的自动分析方法,将视频延伸为具有精度的单个视频截图,在合适的位置分类球的区域轨迹.该方法对慢速球具有较好的跟踪效果,但是在光照影响或快速球的情况下,效果欠佳.文献[8]使用网球比赛的自动标注技术结合粒子滤波器,对网球运动进行平滑化识别和轨迹观察,这种方法一般只适用于跟踪候选网球.本文提出一种基于红外光谱仪的网球运动目标跟踪方法.1 基于红外光谱仪的运动目标跟踪方法首先采集运动目标的干涉图复原光谱,将其与实际光谱进行相位差比对,接着构建动态观测模型,在创建完这个模型之后,在当前帧和下一帧之间利用局部背景加权确定目标标记,在所创建的背景图像中执行逻辑与操作并获得图像加权结果,最后利用局部搜索算法动态更新粒子动态观测模型,提出的方法框架如图1所示.1.1 红外光谱仪采集处理红外光谱仪采用PCI的多通道采集卡结合CPLD作为控制核心,对运动目标进行多路同步采集,采用32位AADC数模转换芯片作为数模转换,并用程控增益放大器进行信号放大[9].采集时通过切光的办法减少自然光干扰,并通过相敏检测与可编程方法器的方法提高信号信噪比,改善对弱信号的采集.完整的光谱图不仅需要使用动镜扫描较长的距离,还要求在较小的间隔内对干涉图进行抽样.但是民用光谱仪的动镜扫描距离是有限的,其干涉图信号光程差也是有限的.因此需要对多边采集到的运动目标进行傅里叶变换,但在光程差截取范围之外的干涉信息将丢失,造成变换后的光谱图与理想值存在差距.本文通过旁瓣操作来减小误差,还原红外光谱图信息.首先通过单色光分析运动目标截止对光谱复原的影响,其傅里叶变换为单色谱线.单色光经过截止后相当于原函数乘以一矩形窗函数,对其做傅里叶变换后会在单色谱线两边出现逐渐减弱的旁瓣振荡,若要更好地光谱复原,需要尽可能减小旁瓣.文中采用旁瓣函数代替矩形函数实现对运动目标的截取,可以缓和矩形窗函数截止造成的不连续性.由采集模块采集干涉图复原的光谱与实际光谱存在一定的相位差,相位误差将造成干涉图函数中的余弦项发生变化.干涉图数据点采集漂移引起的相位误差通常在单边带干涉信号采集中出现,因此采用Forman相位矫正法[10],先计算短双边干涉图的相位误差函数,再通过误差函数与干涉图卷积得到双边干涉图,最后对双边干涉图做傅里叶变换实现相位矫正.1.2 动态观测模型动态观测模型负责管理运动目标图像连续帧之间状态的演变,假设动态观测模型p(Xt|Xt-1),公式如下:Xt=Xt-1+Vt+ε(x.y.h.a).速度向量Vt的噪声ε(x.y.h.a)满足零均值高斯分布,将Vt和Xt替换,获得动态观测模型:p(Zt|Xt)表示模型的观测似然函数,通过观测似然分配权重给每个粒子即第i个粒子的模型采用16×16×16正态分布图HRef进行编码,令为的正态分布图,故观测似然定义为:上式中,λ为常量因子,确保粒子权重总和为为直方图交叉核,定义为选择具有最大似然的粒子,反过来推断目标跟踪状态.1.3 背景加权目标标记背景在目标跟踪中作用很大,对于两个相似的目标,利用背景往往可以很好地区分,因此将目标背景信息融入到目标跟踪中非常必要[11],目标背景模型的权重计算公式如下:式中是常量化系数,qu(y)和表示目标彩色直方图的概率密度.其中概率密度函数表示为式(1),提出的方法运用直方图向量表征及其背景加权以对多目标进行划分和标记.(1)1.4 局部搜索算法局部搜索算法是通过考虑当前和下帧之间的差分来找到候选网球的技术.选择局部搜索的原因是网球通常是快速移动的,它将在连续帧中占据一个完全不同的像素集,在该连续帧中,缓慢移动的目标会有重叠.估计球和球员的灰度水平,需要考虑灯光、阴影和距离变化等影响因素.先获得最佳跟踪边界,再通过当前和前一时间步优化速度向量为:XBest,t和XBest,t-1分别为时间步t和t-1的最佳配置,若粒子直方图交叉核相对于d*的平均值小于T,则更新粒子集.为了跟踪效果更好,基于局部搜索算法迭代更新获取的最佳粒子,并执行偏移运动以得到新的最佳解,直至最佳解逼近运动目标的似然值.2 实验结果实验在来自澳大利亚网球公开赛和温布尔顿公开赛的视频序列上进行,实验采用式(2)计算跟踪的成功率:(2)式中ROIG表示实况状态,ROID表示检测状态.在所有帧中计算成功率,在每个帧中,当这个分数大于0.5时,则将其当作如表1所示的成功.实验中检测了1000个帧,其中有50%的帧可以观测到网球,实验在不同照明条件、角度、速度下检测球和球员的跟踪效果,并将本文的方法与文献[4]、文献[6]、文献[7]的方法进行对比.表1 目标跟踪成功率Tab.1 The success rate of target tracking网球比赛文献[4]文献[6]文献[7]本文的方法澳大利亚网球公开赛67.23%84.52%86.78%93.64%温布尔顿网球公开赛69.79%83.14%82.85%95.12%从表1可以看出,本文的方法目标跟踪成功率保持在了90%以上,比文献[4]、文献[6]、文献[7]方法的成功率均高.这是因为提出的方法通过动态观测模型提高了识别球和球员的跟踪精度,并采用目标加权对背景进行目标标记,提高了目标的跟踪成功率.3 结论本文提出一种基于红外光谱仪的网球运动目标跟踪方法,采用红外光谱仪中采集模块采集运动目标的干涉图复原光谱,与实际光谱进行相位差比对,构建动态观测模型,采用局部背景加权标记运动目标,最后通过局部搜索算法自适应更新动态观测模型,完成对运动目标的自动跟踪.实验在来自澳大利亚网球公开赛和温布尔顿公开赛的视频序列上进行.实验结果表明,提出的方法在不同的背景、光照、角度变化的情况下能保持较高的跟踪成功率.参考文献[1] 邢运龙, 李艾华, 崔智高,等. 改进核相关滤波的运动目标跟踪算法[J]. 红外与激光工程, 2016, 45(6): 214-221.[2] 毕笃彦, 库涛, 查宇飞,等. 基于颜色属性直方图的尺度目标跟踪算法研究[J]. 电子与信息学报, 2016, 38(5):1099-1106.[3] XIAO J, STOLKIN R, LEONARDIS A. Single target tracking using adaptive clustered decision trees and dynamic multi-level appearance models[J]. Pattern Recognition, 2017, 103(5): 4978-4987.[4] 王刘彭, 易年余. 线性有限元误差的L~2范数估计及其应用[J]. 湘潭大学自然科学学报, 2018, 40(1): 35-40.[5] CAMPBELL M. Quantifying the behavior of fish in response to a moving camera vehicle by using benthic stereo cameras and target tracking[J]. Fishery Bulletin-National Oceanic and Atmospheric Administration, 2017, 115(3):343-354.[6] DONG P, JING Z, GONG D, et al. Maneuvering multi-target tracking based on variable structure multiple model GMCPHD filter[J]. Signal Processing, 2017, 141(12):158-167.[7] 桑月标, 马庆功. 网球视频中基于背景模型的目标跟踪方法[J]. 湘潭大学自然科学学报, 2017, 39(3): 111-114.[8] 周明, 涂宏斌. 一种基于改进粒子滤波的多目标检测与跟踪方法[J]. 华东交通大学学报, 2016, 33(2): 121-126.[9] 祁兴普, 陈通, 陈斌. 基于Android微型近红外光谱仪实时检测云系统的实现[J]. 食品安全质量检测学报, 2016, 7(5): 1864-1869.[10] 陆军, 张艺竞, 王成成,等. 相位偏移gamma矫正方法的结构光三维视觉测量技术[J]. 哈尔滨工业大学学报, 2017, 46(9): 182-188.[11] FAN X, XU Z, ZHANG J. Infrared dim and small target background suppression based on improved gradient inverse weighting filter[J]. Opto-Electronic Engineering, 2017, 44(7): 719-724.。
统计学 英语术语
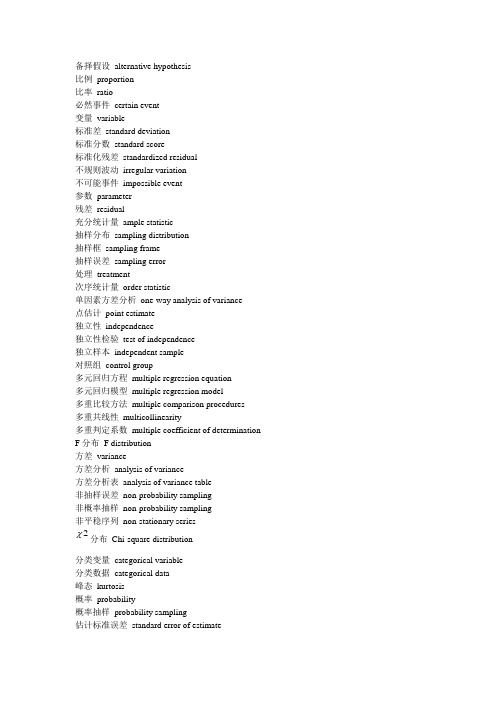
备择假设 alternative hypothesis比例 proportion比率 ratio必然事件 certain event变量 variable标准差 standard deviation标准分数 standard score标准化残差 standardized residual不规则波动 irregular variation不可能事件 impossible event参数 parameter残差 residual充分统计量 ample statistic抽样分布 sampling distribution抽样框 sampling frame抽样误差 sampling error处理 treatment次序统计量 order statistic单因素方差分析 one-way analysis of variance点估计 point estimate独立性 independence独立性检验 test of independence独立样本 independent sample对照组 control group多元回归方程 multiple regression equation多元回归模型 multiple regression model多重比较方法 multiple comparison procedures多重共线性 multicollinearity多重判定系数 multiple coefficient of determinationF 分布 F distribution方差 variance方差分析 analysis of variance方差分析表 analysis of variance table非抽样误差 non-probability sampling非概率抽样 non-probability sampling非平稳序列 non-stationary series2 分布 Chi-square distribution分类变量 categorical variable分类数据 categorical data峰态 kurtosis概率 probability概率抽样 probability sampling估计标准误差 standard error of estimate估计的多元回归方程estimated multiple regression equation 估计的回归方程estimated regression equation估计量estimator估计值estimated value股票价格指数stock price index观测数据observational data回归方程regression equation回归模型regression model基本事件elementary event极差range几何平均数geometric mean季节变动seasonal fluctuation假设检验hypothesis testing渐近分布asymptotic distribution交互作用interaction截面数据cross-sectional data均方mean square均值mean拉氏指数Laspeyres index累积频数cumulative frequencies离散系数coefficient of variation离散型随机变量discrete random variable连续型随机变量continuous random variable列联表contingency table列联系数coefficient of contingency临界值critical value零售价格指数retail price index拟合优度检验goodness of fit test帕氏指数Paasche index判定系数coefficient of determination匹配样本matched sample偏态skewness频数frequency频数分布frequency distribution平均差mean deviation平均增长率average rate of increase平稳序列stationary series期望值expected value区间估计interval estimate趋势trend时间序列time series时间序列数据time series data实验数据experimental data实验组experimental group数值型变量metric variable数值型数据metric data双因素方差分析two-way analysis of variance顺序变量rand variable顺序数据rank data四分位差quartile deviation四分位数quartile随机化区组设计randomized block design随机事件random eventt 分布t distribution条件概率conditional probability统计量statistic统计学statistics调整的多重判定系数adjusted multiple coefficient of determination V相关系数V correlation coefficient无偏性unbiasedness显著性水平significance level相关关系correlation相关系数correlation coefficient消费价格指数consumer price index循环波动cyclical fluctuation样本sample一致性consistency移动平均法moving average异众比率variation ratio因变量dependent variable因子factor因子设计factorial design有效性efficiency预测区间估计prediction interval estimate原假设null hypothesis增长率growth rate指数index number指数平滑法exponential smoothing置信区间confidence interval置信区间估计confidence interval estimate置信水平confidence level中位数median中心极限定理cental limit theorem众数mode自变量independent variable自由度degree of freedom总平方和sum of squares for total总体population组间平方和sum of squares for factor A 组距class width组内平方和sum of squares for error组中值class midpoint最小二乘法method of least squares。
向量自回归模型英语

向量自回归模型英语English:Vector autoregression (VAR) model is a multivariate time series model that extends the idea of univariate autoregression to multiple time series variables. In a VAR model, each variable is regressed on a lagged version of itself and on the lagged values of all other variables in the system. This allows capturing the dynamic interdependencies among the variables over time. VAR models are widely used in econometrics, finance, and other fields to analyze the joint behavior of multiple time series variables, forecast future values, and conduct policy analysis. Estimation of VAR models involves techniques like ordinary least squares (OLS), maximum likelihood estimation (MLE), or Bayesian methods. The selection of lag order in VAR models is crucial and often determined using information criteria such as Akaike Information Criterion (AIC) or Bayesian Information Criterion (BIC). Additionally, impulse response analysis and forecast error variance decomposition are commonly employed to assess the dynamic interactions and forecast accuracy of VAR models.Translated content:向量自回归(VAR)模型是一种多变量时间序列模型,它将单变量自回归的思想扩展到多个时间序列变量。
基于VS-IMM算法的A-SMGCS场面运动目标跟踪
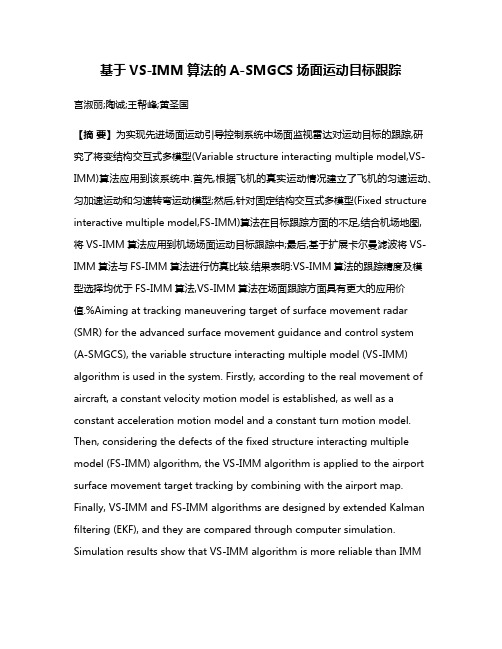
基于VS-IMM算法的A-SMGCS场面运动目标跟踪宫淑丽;陶诚;王帮峰;黄圣国【摘要】为实现先进场面运动引导控制系统中场面监视雷达对运动目标的跟踪,研究了将变结构交互式多模型(Variable structure interacting multiple model,VS-IMM)算法应用到该系统中.首先,根据飞机的真实运动情况建立了飞机的匀速运动、匀加速运动和匀速转弯运动模型;然后,针对固定结构交互式多模型(Fixed structure interactive multiple model,FS-IMM)算法在目标跟踪方面的不足,结合机场地图,将VS-IMM算法应用到机场场面运动目标跟踪中;最后,基于扩展卡尔曼滤波将VS-IMM算法与FS-IMM算法进行仿真比较.结果表明:VS-IMM算法的跟踪精度及模型选择均优于FS-IMM算法,VS-IMM算法在场面跟踪方面具有更大的应用价值.%Aiming at tracking maneuvering target of surface movement radar (SMR) for the advanced surface movement guidance and control system (A-SMGCS), the variable structure interacting multiple model (VS-IMM) algorithm is used in the system. Firstly, according to the real movement of aircraft, a constant velocity motion model is established, as well as a constant acceleration motion model and a constant turn motion model. Then, considering the defects of the fixed structure interacting multiple model (FS-IMM) algorithm, the VS-IMM algorithm is applied to the airport surface movement target tracking by combining with the airport map. Finally, VS-IMM and FS-IMM algorithms are designed by extended Kalman filtering (EKF), and they are compared through computer simulation. Simulation results show that VS-IMM algorithm is more reliable than IMMalgorithm on tracking accuracy. So VS-IMM algorithm has the greater value on surface maneuvering target tracking.【期刊名称】《南京航空航天大学学报》【年(卷),期】2012(044)001【总页数】6页(P118-123)【关键词】目标跟踪;交互式多模型算法;扩展卡尔曼滤波【作者】宫淑丽;陶诚;王帮峰;黄圣国【作者单位】南京航空航天大学民航学院,南京,210016;南京航空航天大学民航学院,南京,210016;南京航空航天大学民航学院,南京,210016;南京航空航天大学民航学院,南京,210016【正文语种】中文【中图分类】V351机场场面监视是保障机场上的航空器和车辆运行安全的基础技术。
常用回归模型

分层数据 面板数据 二分类变量(binomial variable) 截面数据 分层数据 面板数据 截面 (二项分布或多项式分布) (categorical data) 定序变量 (ordinal variable)
分层数据 面板数据 截面数据 分层数据 面板数据
纵贯数据 + 删截 (longitudinal data: 事件史分析:Cox 模型(event history analysis) censoring+time-dependent covariate)
注:1、针对整群(cluster)抽样数据,可采用 Huber-White 标准误调整法或采用 STATA 中的调查估计分析法(survey estimator,命令都以 svy 开头,如 svyreg) 。 2、 针对删截数据 (censoring) , 可采用 Tobit 模型 (Tobin’s probit model) ; 针对截除数据 (truncation) 与内生生性 (endogenous) 样本选择问题, 可采用 Heckman 选择模型 (Heckman selection model: Heckit model) 、内生性变换模型(endogenous switching regression)或倾向分分析法(propensity score matching analysis) 。
常用回归模型简介
因变量类型(DV) 连续变量 (正态分布) (continuous variable) 数据类型(data type) 截面数据(cross-section data) 分层数据(multilevel data) 面板数据(panel data) 截面数据 计数变量 (Poisson 分布) (count data) 常用模型(common model) 普通线性回归(multiple linear regression) 分层线性模型(固定+随机效应)(multilevel model: random+fixed effect) 随机效应及固定效应模型(random effect and fixed effect model) 1、Poisson 回归(Poisson regression) 2、负二项回归(negative binomial) 3、零膨胀模型(ZIP & ZINB) 4、Hurdle 模型(Hurdle model) 分层回归模型(固定+随机效应) 随机效应及固定效应模型 普通二分类 logit 模型(binary logit model) 分层二分类 logit 模型(固定+随机效应) 随机效应及固定效应模型 普通多分类 logit/probit 模型/条件 logit 模型(multinomial logit model/conditional logit model) 分层多分类 logit 模型(固定+随机效应) 随机效应及固定效应模型 普通定序 logit/probit 模型(ordered logit/probit model) 分层定序 logit 模型(固定+随机效应) 随机效应及固定效应模型 备注
《计量经济学导论》ch3
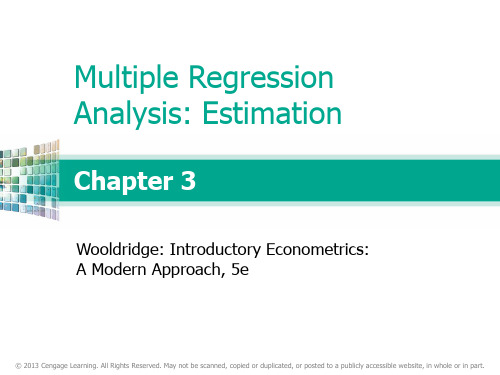
Now measures effect of education explicitly holding experience fixed
All other factors…
Hourly wage
Years of education
Labor market experience
Average family income of students at this school
Other factors
Per student spending is likely to be correlated with average family income at a given high school because of school financing
Dependent variable, explained variable, response variable,…
Independent variables, explanatory variables, regressors,…
Error term, disturbance, unobservables,…
Omitting average family income in regression would lead to biased estimate of the effect of spending on average test scores
In a simple regression model, effect of per student spending would partly include the effect of family income on test scores
多层线性模型在追踪研究中的应用-追踪的多水平模型 ppt课件
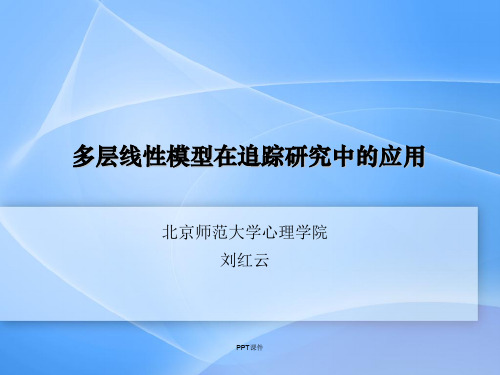
多层线性模型在追踪研究中的应用
北京师范大学心理学院 刘红云
level-1, E
0.312 0.097
-----------------------------------------------------------------------------
Page 37
PPT课件
非线性变化趋势
Page 38
PPT课件
固定部分
Final estimation of fixed effects
0i 00 01 (性别) 0(2 退缩行为) u0i
1i 10 1(1 性别) 1(2 退缩行为) u1i
可以用来自变量(如判断性别差异、有无退缩行为)对自我观念的变化有无趋势及影响程度
Page 14
PPT课件
Page 15
00 表示第二水平预测变量取值为 0 时,水平 1 截距的总体均值,如 这里表示退缩行为得分为 0 的女生三年级时自我概念的平均分; 01 表示在控制另外一个第二水平预测变量退缩行为时,男生相对女 生截距的差异,即男女生初始状态(三年级)时的差异; 02 表示在控制性别影响时,退缩行为每变化一个单位,自我概念截 距(初始状态,即三年级)的差异; 10 表示第二水平预测变量取值为 0 时,水平 1 斜率的总体均值,如 表示退缩行为得分为 0 的女生自我概念变化的平均斜率; 11 表示在控制另一个第二水平预测变量‘退缩行为’时,男生相对女 生变化速度的平均差异。 12 表示在控制性别影响时,退缩行为每变化一个单位,自我概念斜 率的平均差异。 随机部分 00 表示在控制性别和退缩行为后,第一水平截距对应的残 差的方差; 11 表示在控制性别和退缩行为后,第一水平斜率残差的方
“回归分析”
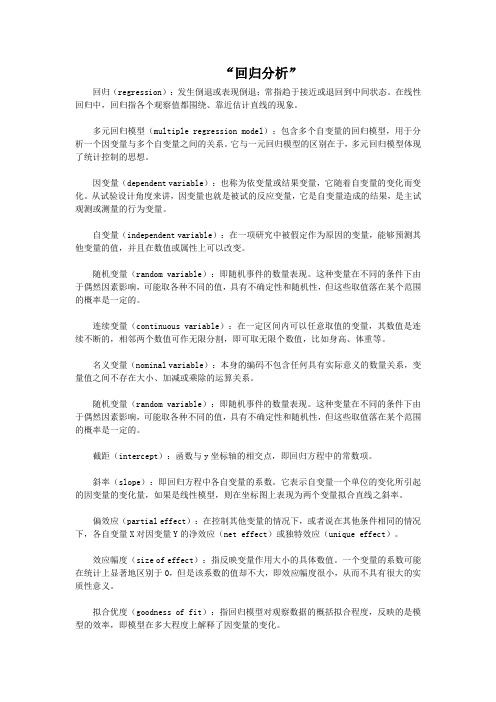
“回归分析”回归(regression):发生倒退或表现倒退;常指趋于接近或退回到中间状态。
在线性回归中,回归指各个观察值都围绕、靠近估计直线的现象。
多元回归模型(multiple regression model):包含多个自变量的回归模型,用于分析一个因变量与多个自变量之间的关系。
它与一元回归模型的区别在于,多元回归模型体现了统计控制的思想。
因变量(dependent variable):也称为依变量或结果变量,它随着自变量的变化而变化。
从试验设计角度来讲,因变量也就是被试的反应变量,它是自变量造成的结果,是主试观测或测量的行为变量。
自变量(independent variable):在一项研究中被假定作为原因的变量,能够预测其他变量的值,并且在数值或属性上可以改变。
随机变量(random variable):即随机事件的数量表现。
这种变量在不同的条件下由于偶然因素影响,可能取各种不同的值,具有不确定性和随机性,但这些取值落在某个范围的概率是一定的。
连续变量(continuous variable):在一定区间内可以任意取值的变量,其数值是连续不断的,相邻两个数值可作无限分割,即可取无限个数值,比如身高、体重等。
名义变量(nominal variable):本身的编码不包含任何具有实际意义的数量关系,变量值之间不存在大小、加减或乘除的运算关系。
随机变量(random variable):即随机事件的数量表现。
这种变量在不同的条件下由于偶然因素影响,可能取各种不同的值,具有不确定性和随机性,但这些取值落在某个范围的概率是一定的。
截距(intercept):函数与y坐标轴的相交点,即回归方程中的常数项。
斜率(slope):即回归方程中各自变量的系数。
它表示自变量一个单位的变化所引起的因变量的变化量,如果是线性模型,则在坐标图上表现为两个变量拟合直线之斜率。
偏效应(partial effect):在控制其他变量的情况下,或者说在其他条件相同的情况下,各自变量X对因变量Y的净效应(net effect)或独特效应(unique effect)。
excel中structure equation model -回复
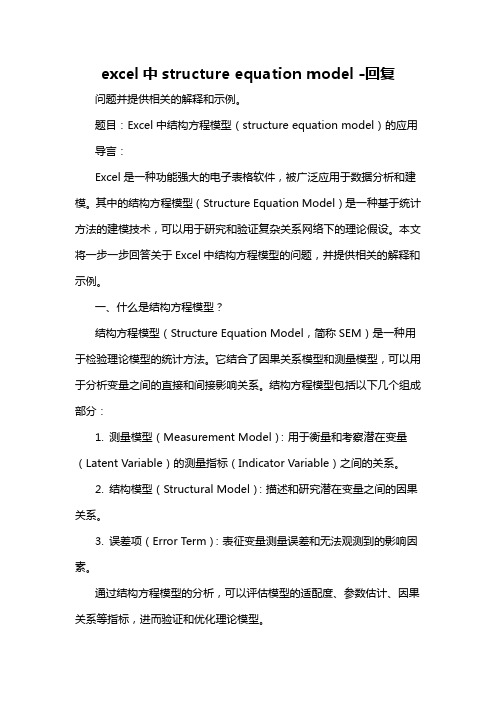
excel中structure equation model -回复问题并提供相关的解释和示例。
题目:Excel中结构方程模型(structure equation model)的应用导言:Excel是一种功能强大的电子表格软件,被广泛应用于数据分析和建模。
其中的结构方程模型(Structure Equation Model)是一种基于统计方法的建模技术,可以用于研究和验证复杂关系网络下的理论假设。
本文将一步一步回答关于Excel中结构方程模型的问题,并提供相关的解释和示例。
一、什么是结构方程模型?结构方程模型(Structure Equation Model,简称SEM)是一种用于检验理论模型的统计方法。
它结合了因果关系模型和测量模型,可以用于分析变量之间的直接和间接影响关系。
结构方程模型包括以下几个组成部分:1. 测量模型(Measurement Model):用于衡量和考察潜在变量(Latent Variable)的测量指标(Indicator Variable)之间的关系。
2. 结构模型(Structural Model):描述和研究潜在变量之间的因果关系。
3. 误差项(Error Term):表征变量测量误差和无法观测到的影响因素。
通过结构方程模型的分析,可以评估模型的适配度、参数估计、因果关系等指标,进而验证和优化理论模型。
二、在Excel中如何建立结构方程模型?在Excel中,我们可以使用专门的插件或者相关函数来建立结构方程模型。
下面是建立结构方程模型的一般步骤:1. 准备数据:首先,收集所需的数据,并准备好适合结构方程模型分析的数据格式。
通常,数据应包括潜在变量的测量指标数据和其他相关变量的数据。
2. 编码数据:对于潜在变量的测量指标数据,需要进行编码或者转换成Excel可以处理的数据格式。
例如,如果使用Likert量表进行测量,可以将1、2、3、4、5等编码为不同的数值。
3. 建立测量模型:使用测量模型分析工具,如因子分析、验证性因子分析等,建立测量模型。
数学专业词汇及翻译

一、字母顺序表 (1)二、常用的数学英语表述 (7)三、代数英语(高端) (13)一、字母顺序表1、数学专业词汇Aabsolute value 绝对值 accept 接受 acceptable region 接受域additivity 可加性 adjusted 调整的 alternative hypothesis 对立假设analysis 分析 analysis of covariance 协方差分析 analysis of variance 方差分析 arithmetic mean 算术平均值 association 相关性 assumption 假设 assumption checking 假设检验availability 有效度average 均值Bbalanced 平衡的 band 带宽 bar chart 条形图beta-distribution 贝塔分布 between groups 组间的 bias 偏倚 binomial distribution 二项分布 binomial test 二项检验Ccalculate 计算 case 个案 category 类别 center of gravity 重心 central tendency 中心趋势 chi-square distribution 卡方分布 chi-square test 卡方检验 classify 分类cluster analysis 聚类分析 coefficient 系数 coefficient of correlation 相关系数collinearity 共线性 column 列 compare 比较 comparison 对照 components 构成,分量compound 复合的 confidence interval 置信区间 consistency 一致性 constant 常数continuous variable 连续变量 control charts 控制图 correlation 相关 covariance 协方差 covariance matrix 协方差矩阵 critical point 临界点critical value 临界值crosstab 列联表cubic 三次的,立方的 cubic term 三次项 cumulative distribution function 累加分布函数 curve estimation 曲线估计Ddata 数据default 默认的definition 定义deleted residual 剔除残差density function 密度函数dependent variable 因变量description 描述design of experiment 试验设计 deviations 差异 df.(degree of freedom) 自由度 diagnostic 诊断dimension 维discrete variable 离散变量discriminant function 判别函数discriminatory analysis 判别分析distance 距离distribution 分布D-optimal design D-优化设计Eeaqual 相等 effects of interaction 交互效应 efficiency 有效性eigenvalue 特征值equal size 等含量equation 方程error 误差estimate 估计estimation of parameters 参数估计estimations 估计量evaluate 衡量exact value 精确值expectation 期望expected value 期望值exponential 指数的exponential distributon 指数分布 extreme value 极值F factor 因素,因子 factor analysis 因子分析 factor score 因子得分 factorial designs 析因设计factorial experiment 析因试验fit 拟合fitted line 拟合线fitted value 拟合值 fixed model 固定模型 fixed variable 固定变量 fractional factorial design 部分析因设计 frequency 频数 F-test F检验 full factorial design 完全析因设计function 函数Ggamma distribution 伽玛分布 geometric mean 几何均值 group 组Hharmomic mean 调和均值 heterogeneity 不齐性histogram 直方图 homogeneity 齐性homogeneity of variance 方差齐性 hypothesis 假设 hypothesis test 假设检验Iindependence 独立 independent variable 自变量independent-samples 独立样本 index 指数 index of correlation 相关指数 interaction 交互作用 interclass correlation 组内相关 interval estimate 区间估计 intraclass correlation 组间相关 inverse 倒数的iterate 迭代Kkernal 核 Kolmogorov-Smirnov test柯尔莫哥洛夫-斯米诺夫检验 kurtosis 峰度Llarge sample problem 大样本问题 layer 层least-significant difference 最小显著差数 least-square estimation 最小二乘估计 least-square method 最小二乘法 level 水平 level of significance 显著性水平 leverage value 中心化杠杆值 life 寿命 life test 寿命试验 likelihood function 似然函数 likelihood ratio test 似然比检验linear 线性的 linear estimator 线性估计linear model 线性模型 linear regression 线性回归linear relation 线性关系linear term 线性项logarithmic 对数的logarithms 对数 logistic 逻辑的 lost function 损失函数Mmain effect 主效应 matrix 矩阵 maximum 最大值 maximum likelihood estimation 极大似然估计 mean squared deviation(MSD) 均方差 mean sum of square 均方和 measure 衡量 media 中位数 M-estimator M估计minimum 最小值 missing values 缺失值 mixed model 混合模型 mode 众数model 模型Monte Carle method 蒙特卡罗法 moving average 移动平均值multicollinearity 多元共线性multiple comparison 多重比较 multiple correlation 多重相关multiple correlation coefficient 复相关系数multiple correlation coefficient 多元相关系数 multiple regression analysis 多元回归分析multiple regression equation 多元回归方程 multiple response 多响应 multivariate analysis 多元分析Nnegative relationship 负相关 nonadditively 不可加性 nonlinear 非线性 nonlinear regression 非线性回归 noparametric tests 非参数检验 normal distribution 正态分布null hypothesis 零假设 number of cases 个案数Oone-sample 单样本 one-tailed test 单侧检验 one-way ANOVA 单向方差分析 one-way classification 单向分类 optimal 优化的optimum allocation 最优配制 order 排序order statistics 次序统计量 origin 原点orthogonal 正交的 outliers 异常值Ppaired observations 成对观测数据paired-sample 成对样本parameter 参数parameter estimation 参数估计 partial correlation 偏相关partial correlation coefficient 偏相关系数 partial regression coefficient 偏回归系数 percent 百分数percentiles 百分位数 pie chart 饼图 point estimate 点估计 poisson distribution 泊松分布polynomial curve 多项式曲线polynomial regression 多项式回归polynomials 多项式positive relationship 正相关 power 幂P-P plot P-P概率图predict 预测predicted value 预测值prediction intervals 预测区间principal component analysis 主成分分析 proability 概率 probability density function 概率密度函数 probit analysis 概率分析 proportion 比例Qqadratic 二次的 Q-Q plot Q-Q概率图 quadratic term 二次项 quality control 质量控制 quantitative 数量的,度量的 quartiles 四分位数Rrandom 随机的 random number 随机数 random number 随机数 random sampling 随机取样random seed 随机数种子 random variable 随机变量 randomization 随机化 range 极差rank 秩 rank correlation 秩相关 rank statistic 秩统计量 regression analysis 回归分析regression coefficient 回归系数regression line 回归线reject 拒绝rejection region 拒绝域 relationship 关系 reliability 可*性 repeated 重复的report 报告,报表 residual 残差 residual sum of squares 剩余平方和 response 响应risk function 风险函数 robustness 稳健性 root mean square 标准差 row 行 run 游程run test 游程检验Sample 样本 sample size 样本容量 sample space 样本空间 sampling 取样 sampling inspection 抽样检验 scatter chart 散点图 S-curve S形曲线 separately 单独地 sets 集合sign test 符号检验significance 显著性significance level 显著性水平significance testing 显著性检验 significant 显著的,有效的 significant digits 有效数字 skewed distribution 偏态分布 skewness 偏度 small sample problem 小样本问题 smooth 平滑 sort 排序 soruces of variation 方差来源 space 空间 spread 扩展square 平方 standard deviation 标准离差 standard error of mean 均值的标准误差standardization 标准化 standardize 标准化 statistic 统计量 statistical quality control 统计质量控制 std. residual 标准残差 stepwise regression analysis 逐步回归 stimulus 刺激 strong assumption 强假设 stud. deleted residual 学生化剔除残差stud. residual 学生化残差 subsamples 次级样本 sufficient statistic 充分统计量sum 和 sum of squares 平方和 summary 概括,综述Ttable 表t-distribution t分布test 检验test criterion 检验判据test for linearity 线性检验 test of goodness of fit 拟合优度检验 test of homogeneity 齐性检验 test of independence 独立性检验 test rules 检验法则 test statistics 检验统计量 testing function 检验函数 time series 时间序列 tolerance limits 容许限total 总共,和 transformation 转换 treatment 处理 trimmed mean 截尾均值 true value 真值 t-test t检验 two-tailed test 双侧检验Uunbalanced 不平衡的 unbiased estimation 无偏估计 unbiasedness 无偏性 uniform distribution 均匀分布Vvalue of estimator 估计值 variable 变量 variance 方差 variance components 方差分量 variance ratio 方差比 various 不同的 vector 向量Wweight 加权,权重 weighted average 加权平均值 within groups 组内的ZZ score Z分数2. 最优化方法词汇英汉对照表Aactive constraint 活动约束 active set method 活动集法 analytic gradient 解析梯度approximate 近似 arbitrary 强制性的 argument 变量 attainment factor 达到因子Bbandwidth 带宽 be equivalent to 等价于 best-fit 最佳拟合 bound 边界Ccoefficient 系数 complex-value 复数值 component 分量 constant 常数 constrained 有约束的constraint 约束constraint function 约束函数continuous 连续的converge 收敛 cubic polynomial interpolation method三次多项式插值法 curve-fitting 曲线拟合Ddata-fitting 数据拟合 default 默认的,默认的 define 定义 diagonal 对角的 direct search method 直接搜索法 direction of search 搜索方向 discontinuous 不连续Eeigenvalue 特征值 empty matrix 空矩阵 equality 等式 exceeded 溢出的Ffeasible 可行的 feasible solution 可行解 finite-difference 有限差分 first-order 一阶GGauss-Newton method 高斯-牛顿法 goal attainment problem 目标达到问题 gradient 梯度 gradient method 梯度法Hhandle 句柄 Hessian matrix 海色矩阵Independent variables 独立变量inequality 不等式infeasibility 不可行性infeasible 不可行的initial feasible solution 初始可行解initialize 初始化inverse 逆 invoke 激活 iteration 迭代 iteration 迭代JJacobian 雅可比矩阵LLagrange multiplier 拉格朗日乘子 large-scale 大型的 least square 最小二乘 least squares sense 最小二乘意义上的 Levenberg-Marquardt method 列文伯格-马夸尔特法line search 一维搜索 linear 线性的 linear equality constraints 线性等式约束linear programming problem 线性规划问题 local solution 局部解M medium-scale 中型的 minimize 最小化 mixed quadratic and cubic polynomialinterpolation and extrapolation method 混合二次、三次多项式内插、外插法multiobjective 多目标的Nnonlinear 非线性的 norm 范数Oobjective function 目标函数 observed data 测量数据 optimization routine 优化过程optimize 优化 optimizer 求解器 over-determined system 超定系统Pparameter 参数 partial derivatives 偏导数 polynomial interpolation method 多项式插值法Qquadratic 二次的 quadratic interpolation method 二次内插法 quadratic programming 二次规划Rreal-value 实数值 residuals 残差 robust 稳健的 robustness 稳健性,鲁棒性S scalar 标量 semi-infinitely problem 半无限问题 Sequential Quadratic Programming method 序列二次规划法 simplex search method 单纯形法 solution 解 sparse matrix 稀疏矩阵 sparsity pattern 稀疏模式 sparsity structure 稀疏结构 starting point 初始点 step length 步长 subspace trust region method 子空间置信域法 sum-of-squares 平方和 symmetric matrix 对称矩阵Ttermination message 终止信息 termination tolerance 终止容限 the exit condition 退出条件 the method of steepest descent 最速下降法 transpose 转置Uunconstrained 无约束的 under-determined system 负定系统Vvariable 变量 vector 矢量Wweighting matrix 加权矩阵3 样条词汇英汉对照表Aapproximation 逼近 array 数组 a spline in b-form/b-spline b样条 a spline of polynomial piece /ppform spline 分段多项式样条Bbivariate spline function 二元样条函数 break/breaks 断点Ccoefficient/coefficients 系数cubic interpolation 三次插值/三次内插cubic polynomial 三次多项式 cubic smoothing spline 三次平滑样条 cubic spline 三次样条cubic spline interpolation 三次样条插值/三次样条内插 curve 曲线Ddegree of freedom 自由度 dimension 维数Eend conditions 约束条件 input argument 输入参数 interpolation 插值/内插 interval取值区间Kknot/knots 节点Lleast-squares approximation 最小二乘拟合Mmultiplicity 重次 multivariate function 多元函数Ooptional argument 可选参数 order 阶次 output argument 输出参数P point/points 数据点Rrational spline 有理样条 rounding error 舍入误差(相对误差)Sscalar 标量 sequence 数列(数组) spline 样条 spline approximation 样条逼近/样条拟合spline function 样条函数 spline curve 样条曲线 spline interpolation 样条插值/样条内插 spline surface 样条曲面 smoothing spline 平滑样条Ttolerance 允许精度Uunivariate function 一元函数Vvector 向量Wweight/weights 权重4 偏微分方程数值解词汇英汉对照表Aabsolute error 绝对误差 absolute tolerance 绝对容限 adaptive mesh 适应性网格Bboundary condition 边界条件Ccontour plot 等值线图 converge 收敛 coordinate 坐标系Ddecomposed 分解的 decomposed geometry matrix 分解几何矩阵 diagonal matrix 对角矩阵 Dirichlet boundary conditions Dirichlet边界条件Eeigenvalue 特征值 elliptic 椭圆形的 error estimate 误差估计 exact solution 精确解Ggeneralized Neumann boundary condition 推广的Neumann边界条件 geometry 几何形状geometry description matrix 几何描述矩阵 geometry matrix 几何矩阵 graphical user interface(GUI)图形用户界面Hhyperbolic 双曲线的Iinitial mesh 初始网格Jjiggle 微调LLagrange multipliers 拉格朗日乘子Laplace equation 拉普拉斯方程linear interpolation 线性插值 loop 循环Mmachine precision 机器精度 mixed boundary condition 混合边界条件NNeuman boundary condition Neuman边界条件 node point 节点 nonlinear solver 非线性求解器 normal vector 法向量PParabolic 抛物线型的 partial differential equation 偏微分方程 plane strain 平面应变 plane stress 平面应力 Poisson's equation 泊松方程 polygon 多边形 positive definite 正定Qquality 质量Rrefined triangular mesh 加密的三角形网格 relative tolerance 相对容限 relative tolerance 相对容限 residual 残差 residual norm 残差范数Ssingular 奇异的二、常用的数学英语表述1.Logic∃there exist∀for allp⇒q p implies q / if p, then qp⇔q p if and only if q /p is equivalent to q / p and q are equivalent2.Setsx∈A x belongs to A / x is an element (or a member) of Ax∉A x does not belong to A / x is not an element (or a member) of AA⊂B A is contained in B / A is a subset of BA⊃B A contains B / B is a subset of AA∩B A cap B / A meet B / A intersection BA∪B A cup B / A join B / A union BA\B A minus B / the diference between A and BA×B A cross B / the cartesian product of A and B3. Real numbersx+1 x plus onex-1 x minus onex±1 x plus or minus onexy xy / x multiplied by y(x - y)(x + y) x minus y, x plus yx y x over y= the equals signx = 5 x equals 5 / x is equal to 5x≠5x (is) not equal to 5x≡y x is equivalent to (or identical with) yx ≡ y x is not equivalent to (or identical with) yx > y x is greater than yx≥y x is greater than or equal to yx < y x is less than yx≤y x is less than or equal to y0 < x < 1 zero is less than x is less than 10≤x≤1zero is less than or equal to x is less than or equal to 1| x | mod x / modulus xx 2 x squared / x (raised) to the power 2x 3 x cubedx 4 x to the fourth / x to the power fourx n x to the nth / x to the power nx −n x to the (power) minus nx (square) root x / the square root of xx 3 cube root (of) xx 4 fourth root (of) xx n nth root (of) x( x+y ) 2 x plus y all squared( x y ) 2 x over y all squaredn! n factorialx ^ x hatx ¯ x barx ˜x tildex i xi / x subscript i / x suffix i / x sub i∑ i=1 n a i the sum from i equals one to n a i / the sum as i runs from 1 to n of the a i4. Linear algebra‖ x ‖the norm (or modulus) of xOA →OA / vector OAOA ¯ OA / the length of the segment OAA T A transpose / the transpose of AA −1 A inverse / the inverse of A5. Functionsf( x ) fx / f of x / the function f of xf:S→T a function f from S to Tx→y x maps to y / x is sent (or mapped) to yf'( x ) f prime x / f dash x / the (first) derivative of f with respect to xf''( x ) f double-prime x / f double-dash x / the second derivative of f with r espect to xf'''( x ) triple-prime x / f triple-dash x / the third derivative of f with respect to xf (4) ( x ) f four x / the fourth derivative of f with respect to x∂f ∂ x 1the partial (derivative) of f with respect to x1∂ 2 f ∂ x 1 2the second partial (derivative) of f with respect to x1∫ 0 ∞the integral from zero to infinitylimx→0 the limit as x approaches zerolimx→0 + the limit as x approaches zero from abovelimx→0 −the limit as x approaches zero from belowlog e y log y to the base e / log to the base e of y / natural log (of) ylny log y to the base e / log to the base e of y / natural log (of) y一般词汇数学mathematics, maths(BrE), math(AmE)公理axiom定理theorem计算calculation运算operation证明prove假设hypothesis, hypotheses(pl.)命题proposition算术arithmetic加plus(prep.), add(v.), addition(n.)被加数augend, summand加数addend和sum减minus(prep.), subtract(v.), subtraction(n.)被减数minuend减数subtrahend差remainder乘times(prep.), multiply(v.), multiplication(n.)被乘数multiplicand, faciend乘数multiplicator积product除divided by(prep.), divide(v.), division(n.)被除数dividend除数divisor商quotient等于equals, is equal to, is equivalent to 大于is greater than小于is lesser than大于等于is equal or greater than小于等于is equal or lesser than运算符operator数字digit数number自然数natural number整数integer小数decimal小数点decimal point分数fraction分子numerator分母denominator比ratio正positive负negative零null, zero, nought, nil十进制decimal system二进制binary system十六进制hexadecimal system权weight, significance进位carry截尾truncation四舍五入round下舍入round down上舍入round up有效数字significant digit无效数字insignificant digit代数algebra公式formula, formulae(pl.)单项式monomial多项式polynomial, multinomial系数coefficient未知数unknown, x-factor, y-factor, z-factor 等式,方程式equation一次方程simple equation二次方程quadratic equation三次方程cubic equation四次方程quartic equation不等式inequation阶乘factorial对数logarithm指数,幂exponent乘方power二次方,平方square三次方,立方cube四次方the power of four, the fourth power n次方the power of n, the nth power开方evolution, extraction二次方根,平方根square root三次方根,立方根cube root四次方根the root of four, the fourth root n次方根the root of n, the nth root集合aggregate元素element空集void子集subset交集intersection并集union补集complement映射mapping函数function定义域domain, field of definition值域range常量constant变量variable单调性monotonicity奇偶性parity周期性periodicity图象image数列,级数series微积分calculus微分differential导数derivative极限limit无穷大infinite(a.) infinity(n.)无穷小infinitesimal积分integral定积分definite integral不定积分indefinite integral有理数rational number无理数irrational number实数real number虚数imaginary number复数complex number矩阵matrix行列式determinant几何geometry点point线line面plane体solid线段segment射线radial平行parallel相交intersect角angle角度degree弧度radian锐角acute angle直角right angle钝角obtuse angle平角straight angle周角perigon底base边side高height三角形triangle锐角三角形acute triangle直角三角形right triangle直角边leg斜边hypotenuse勾股定理Pythagorean theorem钝角三角形obtuse triangle不等边三角形scalene triangle等腰三角形isosceles triangle等边三角形equilateral triangle四边形quadrilateral平行四边形parallelogram矩形rectangle长length宽width附:在一个分数里,分子或分母或两者均含有分数。
multivariable prediction model

multivariable prediction modelA multivariate prediction model is a type of statistical model used to predict a target variable based on multiple input variables. These models are commonly used in various fields, including economics, finance, marketing, and engineering, to make predictions and informed decisions.The basic idea behind a multivariate prediction model is to identify the relationships between the input variables and the target variable using historical data. By analyzing these relationships, the model can learn the patterns and trends in the data and use them to make predictions for new data points.There are several types of multivariate prediction models, including linear regression, logistic regression, decision trees, random forests, and neural networks. The choice of model depends on the nature of the data, the预测目标, and the available computing resources.In general, the process of building a multivariate prediction model involves the following steps:1. Data collection and preprocessing: Gather relevant data and clean and transform it as necessary to prepare it for modeling.2. Feature selection: Identify the most relevant input variables that have a significant impact on the target variable.3. Model training: Use the selected features and historical data to train the model, adjusting the model parameters to optimize its performance.4. Model evaluation: Assess the performance of the trained model using validation data and metrics such as accuracy, precision, recall, and F1-score.5. Model deployment: If the model performs well, it can be deployed to make predictions on new data.It is important to note that multivariate prediction models are not perfect and may suffer from overfitting, underfitting, or other biases. Therefore, it is essential to validate and evaluate the model's performance using appropriate techniques and to continuously monitor and update the model as new data becomes available.。
多项式有限差分方法说明书
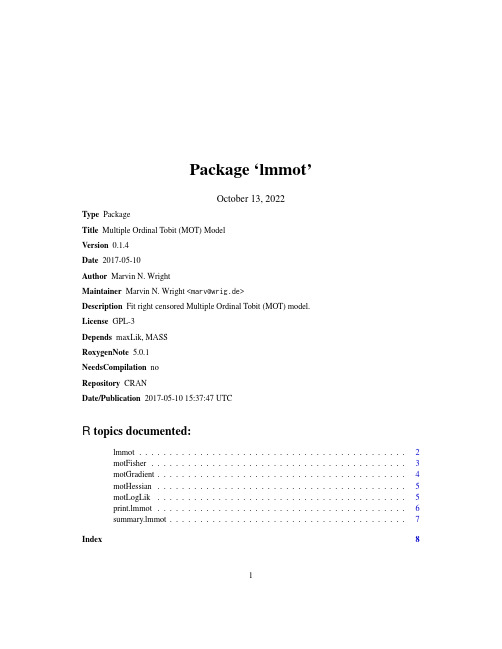
Package‘lmmot’October13,2022Type PackageTitle Multiple Ordinal Tobit(MOT)ModelVersion0.1.4Date2017-05-10Author Marvin N.WrightMaintainer Marvin N.Wright<************>Description Fit right censored Multiple Ordinal Tobit(MOT)model.License GPL-3Depends maxLik,MASSRoxygenNote5.0.1NeedsCompilation noRepository CRANDate/Publication2017-05-1015:37:47UTCR topics documented:lmmot (2)motFisher (3)motGradient (4)motHessian (5)motLogLik (5)print.lmmot (6)summary.lmmot (7)Index812lmmot lmmot Multiple Ordinal Tobit ModelDescriptionFit right censored Multiple Ordinal Tobit(MOT)model.Usagelmmot(formula,data=sys.frame(sys.parent()),threshold,stdEr="fisher",...)Argumentsformula Object of class formula describing the model.data Optional data frame or environment containing the variables in the model.threshold Vector of thresholds in the model.stdEr Method for standard error e"fisher"for estimation using the in-verse of the Fisher information matrix or"hessian"for estimation using the Hes-sian matrix....Further arguments passed to the maximum likelihood estimation function max-Lik.DetailsFit right censored Multiple Ordinal Tobit(MOT)model.The model is a right censored Tobit model with multiple ordinal categories for latent values above the threshold,the threshold is therefore replaced by a threshold vector.For the latent variable a linear model with independent and identically distributed non-systematic and homoscedastic errors is assumed.If the threshold is of length1,the model is equivalent to the standard right censored Tobit model.The data isfitted with the Maximum Likelihood method.Valuelmmot object:maxLik object with additionalfields:•censoring:Number of obeservations in the censoring intervals.•fisherInfo:Fisher information matrix.•stdEr:Standard errors for estimated coefficients.•tval:Value for t statistic in Wald test.•pval:p-value in Wald test.•fitted.values:Fitted values of the estimated model.•residuals:Residuals of the estimated model.motFisher3 Author(s)Marvin N.WrightSee Alsolm maxLikExamples#Random data for xN<-100x<-rnorm(N,25,10)#Simulate data for latent variable ystar with simple linear modelbeta_0<-60beta_1<-1sigma<-8ystar<-beta_0+beta_1*x+rnorm(N,0,sigma)#Simulate censoring for observed variable yy<-ystary[y>=100]<-100y[(y>=90)&(y<100)]<-90y[(y>=80)&(y<90)]<-80#MOT regression with observed variable ymot.fit<-lmmot(y~x,threshold=c(80,90,100))#Show detailssummary(mot.fit)#Compare real data with model fitplot(x,ystar)abline(coefficients(mot.fit)[1:2])motFisher Fisher information for mot modelDescriptionFisher information matrix for right censored Multiple Ordinal Tobit(MOT)model.UsagemotFisher(param,xx,tau)4motGradientArgumentsparam parameter vector:(beta_0,beta_1,...,beta_m,sigma).xx design matrix of the model.tau threshold vector from tau_1to tau_K.Valuefisher information matrix,summarized over all observations.Author(s)Marvin WrightSee AlsolmmotmotGradient Gradient of log-Likelihood for mot modelDescriptionGradient of log-Likelihood for right censored Multiple Ordinal Tobit(MOT)model.UsagemotGradient(param,xx,y,tau)Argumentsparam parameter vector:(beta_0,beta_1,...,beta_m,sigma).xx design matrix of the model.y observation vector.tau threshold vector from tau_1to tau_K.Valuegradient of log-likelihood,vector with all observations.Author(s)Marvin WrightSee AlsolmmotmotHessian5 motHessian Hessian matrix of log-Likelihood for mot modelDescriptionHessian matrix of log-Likelihood for right censored Multiple Ordinal Tobit(MOT)model.UsagemotHessian(param,xx,y,tau)Argumentsparam parameter vector:(beta_0,beta_1,...,beta_m,sigma).xx design matrix of the model.y observation vector.tau threshold vector from tau_1to tau_K.Valuehessian matrix,summarized over all observations.Author(s)Marvin WrightSee AlsolmmotmotLogLik log-likelihood for mot modelDescriptionLog-Likelihood for multiple ordinal right censored Multiple Ordinal Tobit(MOT)model.UsagemotLogLik(param,xx,y,tau)6print.lmmotArgumentsparam parameter vector:(beta_0,beta_1,...,beta_m,sigma).xx design matrix of the model.y observation vector.tau threshold vector from tau_1to tau_K.Valuelog-likelihood,vector with all observations.Author(s)Marvin WrightSee Alsolmmotprint.lmmot Print lmmot objectDescriptionPrint lmmot object.Usage##S3method for class lmmotprint(x,digits=max(3,getOption("digits")-3),...)Argumentsx lmmot object to print.digits number of decimal digits to print....further arguments passed to or from other methods.Author(s)Marvin WrightSee Alsolm lmmotsummary.lmmot7 summary.lmmot Summary if lmmot objectDescriptionPrint details about lmmot object.Usage##S3method for class lmmotsummary(object,digits=max(3,getOption("digits")-3),...)Argumentsobject lmmot object to print.digits number of decimal digits to print....further arguments passed to or from other methods.Author(s)Marvin WrightSee Alsolm lmmotIndexlm,3,6,7lmmot,2,4–7maxLik,2,3motFisher,3motGradient,4motHessian,5motLogLik,5print.lmmot,6summary.lmmot,78。
多变量自回归
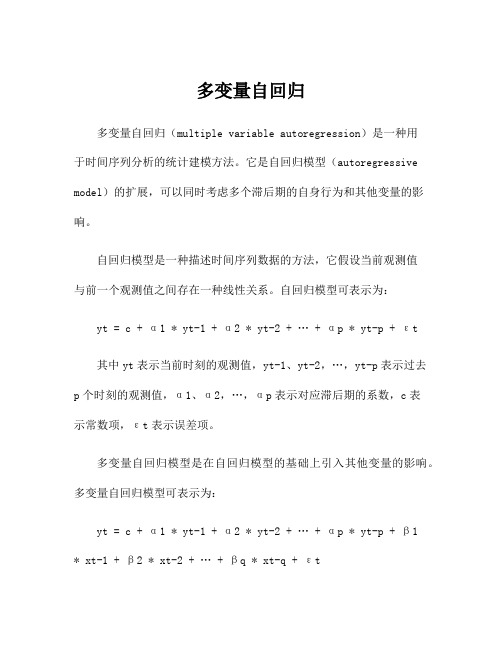
多变量自回归多变量自回归(multiple variable autoregression)是一种用于时间序列分析的统计建模方法。
它是自回归模型(autoregressive model)的扩展,可以同时考虑多个滞后期的自身行为和其他变量的影响。
自回归模型是一种描述时间序列数据的方法,它假设当前观测值与前一个观测值之间存在一种线性关系。
自回归模型可表示为:yt = c + α1 * yt-1 + α2 * yt-2 + … + αp * yt-p + εt其中yt表示当前时刻的观测值,yt-1、yt-2,…,yt-p表示过去p个时刻的观测值,α1、α2,…,αp表示对应滞后期的系数,c表示常数项,εt表示误差项。
多变量自回归模型是在自回归模型的基础上引入其他变量的影响。
多变量自回归模型可表示为:yt = c + α1 * yt-1 + α2 * yt-2 + … + αp * yt-p + β1* xt-1 + β2 * xt-2 + … + βq * x t-q + εt其中xt-1、xt-2,…,xt-q表示其他变量在过去q个时刻的观测值,β1、β2,…,βq表示其他变量的系数。
多变量自回归模型的建立需要考虑以下几个步骤:1.数据收集:收集时间序列数据和其他相关变量的观测值。
2.模型选择:根据收集的数据选择适合的多变量自回归模型。
可以使用统计方法(如信息准则)或经验判断来选择合适的滞后阶数p和q。
3.参数估计:使用最小二乘法或其他估计方法估计模型的参数。
参数估计的目标是使观测值与预测值之间的误差最小化。
4.模型诊断:对估计的模型进行诊断,检查模型的拟合程度和残差项的性质。
常用的诊断方法包括检查残差序列的平稳性、自相关性、正态性等。
5.模型预测:使用估计的模型进行未来观测值的预测。
可以使用递归方法或直接求解模型方程得到预测值。
多变量自回归模型在实际应用中有广泛的应用。
例如,在经济学中,可以使用多变量自回归模型来分析经济变量之间的关系,预测经济指标的未来走势;在气象学中,可以使用多变量自回归模型来描述气温、湿度、风速等变量之间的关系,预测未来的天气变化。
- 1、下载文档前请自行甄别文档内容的完整性,平台不提供额外的编辑、内容补充、找答案等附加服务。
- 2、"仅部分预览"的文档,不可在线预览部分如存在完整性等问题,可反馈申请退款(可完整预览的文档不适用该条件!)。
- 3、如文档侵犯您的权益,请联系客服反馈,我们会尽快为您处理(人工客服工作时间:9:00-18:30)。
I.
INTRODUCTION
The problem of tracking maneuvering radar target has attracted much attention in recent years. Nonlinearity, non-Gaussianity and maneuvering often exist in target tracking problems. The Kalman filter is the optimal solution to the Bayesian estimation problem for a given linear system with additive Gaussian noise sources of known statistics. An extended Kalman filter (EKF) or an unscented Kalman filter (UKF) is usually implemented for weak nonlinearity. Another group of popular techniques is the class of sequential Monte Carlo methods, also known as the particle filters (PF), which can be applied to problems with arbitrary nonlinearities in dynamics and measurements. It is generally difficult to use a single model to represent the motion of a maneuvering target. Hence, multiple model (MM) based approaches are often used for maneuvering target tracking. In particular, the IMM algorithm is widely accepted as one of the most cost-effective dynamic MM methods. IMM use a fixed set of models at each time. When these algorithms are applied to solve real-world problems, it is often the case that the use of only a small number of models is not good enough. The use of more models increases the computational burden considerably with the performance not remarkable
Abstract-This paper presents two methods for maneuvering radar target tracking, one is called variable structure multiple model particle filter (VSMMPF), which combines the variable structure multiple model (VSMM) filter with a Sampling-Importance Resampling (SIR) particle filter (PF); The other one is called modified adaptive gird multiple model (AGMM), which is suitable when model parameter scope is unknown beforehand. PF is used to deal with problems with arbitrary nonlinearities and non-Gaussian noise. Thus the combination of VSMM and PF is able to deal with problems of nonlinearities, non-Gaussian and maneuvering. As one of the VSMM algorithms, Grid adaptation (GA) is unique one which can produce new model-set that are not predefined and model parameter can be any value within continuous model space. However, it only used to tracking maneuvering target in 2D coordinate turn system. Our modified AGMM could track generic planar maneuvering target. Simulation results indicate that performance of the algorithm VSMMPF outperforms interacting multiple model (IMM) PF and the AGMM PF which combines AGMM with PF runs well especially when model scope is unknown in advance. Index terms: Variable structure, particle filter, adaptive grid, radar target tracking
Variable Structure Multiple Model Particle Filter for Maneuvering Radar Target Tracking
Min Zhang, Weidong Chen
Department of Electronics Engineering and Information Science, University of Science & Technology of China, Hefei, P.R.China, 230027
Given the following model of a stochastic hybrid system x(k ) = Fk j−1 x(k − 1) + Gkj−1 wkj −1
z (k ) = H kj x (k ) + vkj Where x(k ) is the system base state vector, z (k ) is the noisy measurement vector, w and v are the mode-dependent process and measurement random noise sequences, which assumedபைடு நூலகம்to be mutually independent with covariance, respectively, Qk and Rk ,. The random parameter j is the
improves. In [1], the concept of VSMM estimation is introduced and several variable structure algorithms are proposed. A series of VSMM algorithms are developed by Li and his team [2-5]. Model-set adaptation, which involves determination of multiple candidate model-sets and selection of best model-set from candidate sets, is an important approach for VSMM in recent years. In [1], the MM algorithms are formulated in a graph theoretic framework. Three representative model-set adaptation approaches, Model-group switching (MGS), likely–model set (LMS) and GA are all belong to these schemes. Compared with MGS and LMS approaches, GA is unique one for its ability to produce new model-set, which are not predefined and model parameter can be any value within continuous mode space, in real time. In [6], for maneuvering aircraft (MA) tracking problem, two VSMM algorithms with different model-set adaptation approaches, (switching grid) SG IMM and adaptive grid (AG) IMM, has been designed. In [7], a new method for tracking a maneuvering target called IMMPF is proposed. The basic idea is to combine the IMM approach with a PF approach. In the derivation of the standard IMM filter, a merging and filtering process are defined. It adopts a regularized particle filter for this filtering step, and performs the merging step on these probability densities, represented by a Gaussian mixture. In this paper, a modified AGMM approach used to track planar maneuvering target and a new approach called VSMMPF, which combines VSMM with PF, are proposed. In contrast to existed MM algorithms, the proposed approaches can deal with the tracking problems with nonlinear dynamics, nonlinear measurements and maneuvers. Simulation results show that the modified AGMM is better than LMS. VSMMPF outperforms IMMPF when the model parameter scope is unknown beforehand, and the AGMMPF has a well performance when scope is unknown in advance. II. SYSTEM SETUP