A Flow Graph Formulation of Optimal Software Pipelining
美洲大蠊提取物胃漂浮缓释片制备研究

第49卷第10期2021年5月广㊀州㊀化㊀工Guangzhou Chemical IndustryVol.49No.10May.2021美洲大蠊提取物胃漂浮缓释片制备研究王㊀旭(云南省昆虫生物医药研发重点实验室(大理大学),大理大学药学院,云南㊀大理㊀671000)摘㊀要:以假酸浆子胶质为片剂辅料制备美洲大蠊提取物胃漂浮缓释片㊂在单因素试验的基础上,以美洲大蠊提取物胃漂浮缓释片中尿嘧啶体外释放百分率为评价指标进行正交试验优化美洲大蠊提取物胃漂浮缓释片处方㊂优化处方为美洲大蠊提取物10g,羟丙甲基纤维素K100M 22g,假酸浆子胶质冻干品4g,十八醇12g,乙基纤维素12g,1%硬脂酸镁㊂应用假酸浆子胶质制备的美洲大蠊提取物胃漂浮缓释片制备方法简单可行㊂关键词:美洲大蠊提取物;假酸浆子胶质;胃漂浮缓释片;制备㊀中图分类号:R9㊀文献标志码:A文章编号:1001-9677(2021)010-0070-04㊀㊀㊀㊀㊀㊀㊀㊀㊀㊀㊀㊀㊀作者简介:王旭,在读硕士研究生,专业方向为药物新剂与新技术型研究㊂Preparation of Intragastric Floating Sustained -ReleaseTablets of Periplaneta Americana ExtractWANG Xu(Yunnan Provincial Key Laboratory of Entomological Biopharmaceutical R &D (Dali University),College of Pharmacy,Dali University,Yunnan Dali 671000,China)Abstract :To prepare intragastric floating sustained -release tablets of Periplaneta americana extract with gum from Nicandra physaloides (L.)Gaertn seeds (GNPS)as tablet excipient.Based on single factor test,the orthogonal experiment was conducted to optimize the formulation of intragastric floating sustained -release tablets of Periplaneta americana extract (GFSRTPA),using the percentage released in vitro uracil in GFSRTPA as evaluation indexes.The optimal formulation of GFSRTPA was made up of Periplaneta americana extract 10g,HPMC K100M 22g,GNPS 4g,octadecanol 12g,ethylcellulose 12g,1%magnesium stearate.The prepared method of GFSRTPA with GNPS is simple and feasible.Key words :Periplaneta americana extract;gum from Nicandra physaloides (L.)Gaertn seeds;intragastric floating sustained -release tablets;preparation胃溃疡是一种常见的消化道疾病,一般认为其发病机制为侵袭因素和保护性因素之间失去平衡所导致[1]㊂美洲大蠊(Periplaneta americana Linnaeus),俗称蟑螂,近年研究表明美洲大蠊提取物对消化系统疾病如消化道溃疡㊁胃溃疡㊁上消化道出血等具有较好的疗效[2]㊂邹俊波等[3]研究表明美洲大蠊提取物有抗氧自由基及降低NO 含有量的作用,是其对抗乙醇诱导急性胃粘膜损伤的作用机制之一㊂康复新液是美洲大蠊提取物制成的液体制剂,用于治疗消化性溃疡,但药液会随胃排空很快离开病灶部位,作用时间较短,需一日服用三次㊂胃漂浮缓释片是服用后较长时间滞留胃液内㊁控制药物缓慢释放的制剂㊂制备美洲大蠊提取物胃漂浮缓释片,可以延长有效成分在胃内的滞留时间,提高有效成分在病灶部位的作用时间㊂李远辉[4]研究表明以康复新液冻干粉为主药的胃漂浮片具有良好的体外缓释行为㊂假酸浆子胶质(Gum from Nicandra physaloides (L.)Gaertn seeds,GNPS)无色㊁无味㊁无毒,在西南地区广为食用,俗称 冰粉 ㊂研究表明假酸浆子胶质是一种大分子果胶多糖,分子量为1.6ˑ106u [5]㊂课题组何海花等[6]对GNPS 制备美洲大蠊提取物胃漂浮缓释片(intragastric floating sustained -release tablets of Periplaneta americana extract,GFSRTPA)进行体外释药评价,具有良好的体外缓释特征㊂在此基础上,本文采用GNPS 为助漂剂制备GFSRTPA,通过单因素考察与正交试验优化处方制备GFSRTPA,为GNPS 应用于胃漂浮缓释片提供参考㊂1㊀仪器与材料1.1㊀仪㊀器Agilent1200型高效液相色谱仪,美国Agilent 公司;CP224C 型分析天平,奥豪斯仪器有限公司;RC8MD 型溶出实验仪,天津市天大天发科技有限公司;RZD -8D 型取样收集系统,天津天大天发科技有限公司;101-3E 型鼓风干燥机,北京市永光明医疗器械有限公司;YY0221-1995型旋转式压片机,上海天和制药机械厂;KQ5200DB 型超声波清洗器,昆山市超声仪器有限公司;GOLD -SIM 型冻干机,美国SIM 国际集团有限公司㊂1.2㊀药物与试剂美洲大蠊提取物,云南省昆虫生物医药研发重点实验室提供;假酸浆子胶质冻干品,自制;乙基纤维素(批号15-12),第49卷第10期王旭:美洲大蠊提取物胃漂浮缓释片制备研究71㊀安徽山河药用辅料股份有限公司;十八醇(批号105220151101),湖南尔康制药股份有限公司;HPMC K100M (批号200423),安徽山河药用辅料股份有限公司;HPMC K15M(批号200314),安徽山河药用辅料股份有限公司; HPMC K4M(批号190211),安徽山河药用辅料股份有限公司;尿嘧啶(批号100469-201302),中国食品药品检定研究所;其它试剂为分析纯㊂2㊀方法与结果2.1㊀胃漂浮缓释片的制备辅料过80目筛,采用等量递加法,将十八醇㊁乙基纤维素㊁HPMC㊁溶胀的假酸浆子胶质冻干品与美洲大蠊提取物混合,50%乙醇制软材,过16目筛制湿颗粒,50ħ烘干3h,整粒,加1%硬脂酸镁,压片,即得GFSRTPA㊂2.2㊀指标成分含量测定2.2.1㊀溶液的制备对照品溶液的配制:精密称取尿嘧啶对照品0.0133g,置于100mL容量瓶中,加入适量超纯水,超声溶解,加超纯水定容至刻度,即得尿嘧啶对照品溶液,浓度为133μg/mL㊂供试品溶液的配制:取GFSRTPA研磨成细粉,取0.34g 加入锥形瓶中,加入100mL超纯水,超声30min,取出,放置室温,抽滤,续滤液转移容置100mL容量瓶中,定容,即得供试品溶液㊂阴性样品溶液的配制:取辅料最大量加入锥形瓶中,加入100mL超纯水,超声30min,取出,放置室温,抽滤,取续滤液转移容置100mL容量瓶中,定容,即得阴性样品溶液㊂2.2.2㊀色谱条件色谱柱:ZORBAX SB-C18(4.6mmˑ250mm,5μm)色谱柱,含预柱ZORBAX SB-C18(4.6mmˑ12.5mm,5μm);流动相:(A)超纯水,(B)乙腈;梯度洗脱(0min,2%B;2min, 2%B;10min,10%B;20min,10%B;25min,2%B),流速0.6mL/min;DAD检测器,检测波长257nm,柱温25ħ,进样量10μL㊂图1㊀对照品,供试品和阴性样品的HPLC图Fig.1㊀HPLC chromatograms of reference,test sample andnegative sample分别精密移取对照品溶液㊁供试品溶液和阴性样品溶液1mL,过ϕ0.22μm滤膜,放入棕色进样瓶内,按上述色谱条件进高效液相色谱仪测定,结果见图1㊂2.2.3㊀线性关系取尿嘧啶对照品溶液,加入超纯水制成浓度为13.3㊁11.97㊁10.64㊁9.31㊁7.98㊁6.65㊁5.32㊁3.99㊁2.66㊁1.33㊁0.665μg/mL的尿嘧啶对照品溶液,过ϕ0.22μm滤膜,放入棕色进样瓶内,按色谱条件进样测定,记录峰面积,对照品的峰面积Y与含量X(μg)做线性回归,绘制标准曲线,计算回归方程㊂尿嘧啶线性方程为Y=7083.3X-8.7794,R2为0.9992,尿嘧啶在0.00665~0.133μg范围内线性关系良好㊂2.2.4㊀精密度试验取尿嘧啶对照品溶液1mL,过ϕ0.22μm滤膜,放入棕色进样瓶内,按色谱条件重复进样6次,记录尿嘧啶的峰面积,计算RSD㊂RSD为0.15%,表明精密度良好㊂2.2.5㊀稳定性试验取供试品溶液1mL,过ϕ0.22μm滤膜,放入棕色进样瓶内,按色谱条件测定,分别在0㊁1㊁2㊁4㊁6㊁8㊁10㊁12㊁24h 进样10μL,记录尿嘧啶的峰面积,计算RSD㊂RSD为1.38%,表明24h内稳定性良好㊂2.2.6㊀重复性试验按供试品溶液制备方法平行制备6份供试品溶液,取1mL 溶液,过ϕ0.22μm滤膜,放入棕色进样瓶内,按色谱条件进样,记录尿嘧啶的峰面积,计算RSD㊂RSD为1.31%,重复性好㊂2.2.7㊀加样回收率取6mL供试品溶液10份,一份进样测定含量,其余9份分为三组,即高㊁中㊁低三组,低剂量组加入0.05mL尿嘧啶对照品溶液,中剂量组加入0.1mL尿嘧啶对照品溶液,高剂量组加入0.15mL尿嘧啶对照品溶液,分别定容至10mL容量瓶中,过ϕ0.22μm滤膜,放入棕色进样瓶内,按色谱条件进样,计算尿嘧啶回收率,高㊁中㊁低三组的回收率分别为98.91%㊁99.80%㊁100.47%,在95%~105%范围内,RSD 为0.23%㊁0.76%㊁0.18%,加样回收率良好㊂2.3㊀体外释放度测定选择‘中国药典“2015年版四部通则中0931项下第二法桨法,以0.1moL/L盐酸500mL为释放介质,温度设定37ħ,转速为100r/min,取样时间设定为0.5㊁1㊁2㊁4㊁6㊁8㊁10㊁12h,在各取样点取样5mL,并及时补充同等温度的5mL新鲜释放介质,取样液水浴蒸干,1mL蒸馏水溶解,过ϕ0.22μm72㊀广㊀州㊀化㊀工2021年5月滤膜,续滤液进样,按色谱条件进样测定,计算药物释放百分率㊂2.4㊀单因素考察2.4.1㊀HPMC 型号对释放百分率影响固定其他条件不变,考察不同型号的HPMC K4M㊁HPMC K15M㊁HPMC K100M 对GFSRTPA 释放百分率的影响㊂按处方制备不同型号HPMC 的GFSRTPA,测定尿嘧啶释放百分率,考察不同型号的HPMC 对药物释放百分率的影响㊂以药物释放百分率对时间做图,结果见图2㊂图2㊀不同型号HPMC 的GFSRTPA 释放百分率曲线图Fig.2㊀Release percentage curve of GFSRTPA with differenttypes of HPMC 0.5h 时HPMC K4M㊁HPMC K15M㊁HPMC K100M 释放百分率分别为33.23%㊁28.61%㊁26.21%,其中HPMC K4M 型号释放百分率最大,HPMC K100M 型号释放百分率最小;2h 时HPMC K4M㊁HPMC K15M㊁HPMC K100M 释放百分率分别为48.59%㊁52.82%㊁47.66%,其中HPMC K15M 型号释放百分率最大,HPMC K100M 型号释放百分率最小;6h 时HPMC K4M㊁HPMC K15M㊁HPMC K100M 释放百分率分别为79.22%㊁78.84%㊁73.47%,其中HPMC K15M 型号释放百分率最大,HPMC K100M 型号释放百分率最小;10h 时HPMC K4M㊁HPMC K15M㊁HPMC K100M 释放百分率分别为85.57%㊁89.47%㊁85.10%,其中HPMC K4M 型号释放百分率最大,HPMC K100M 型号释放百分率最小㊂总体看各型号释放百分率相差不大,综合考虑,选择释放百分率较小的型号,故选择HPMC K100M㊂2.4.2㊀GNPS量对释放百分率影响图3㊀不同GNPS 用量的GFSRTPA 释放百分率曲线图Fig.3㊀Release percentage curve of GFSRTPA with differentGNPS dosages固定其他条件不变,选用HPMC 型号为HPMC K100M,将辅料等量递加混合均匀,选择GNPS 冻干品的用量为2㊁4㊁6g,制备GFSRTPA,测定尿嘧啶释放百分率,考察不同用量的GNPS 冻干品对药物释放百分率的影响㊂以药物释放百分率对时间做图,结果见图3㊂0.5h 时,GNPS 用量为2g㊁4g㊁6g 的释放百分率分别为26.87%㊁29.28%㊁30.92%,其中用量为6g 的释放百分率最大,用量为2g 的释放百分率最小;2h 时,GNPS 用量为2g㊁4g㊁6g 的释放百分率分别为48.49%㊁55.02%㊁55.04%,其中用量为6g 的释放百分率最大,用量为2g 的释放百分率最小;6h 时,GNPS 用量为2g㊁4g㊁6g 的释放百分率分别为78.26%㊁70.01%㊁84.80%,其中用量为6g 的释放百分率最大,用量为4g 的释放百分率最小;10h 时,GNPS 用量为2g㊁4g㊁6g 的释放百分率分别为83.09%㊁88.87%㊁92.97%,其中用量为6g 的释放百分率最大,用量为2g 的释放百分率最小㊂2.5㊀正交试验选用HPMC K100M㊁GNPS㊁十八醇和乙基纤维素的用量为考察因素,每个因素设定三个水平,进行正交设计,以尿嘧啶释放百分率为指标,根据2015版‘中国药典“四部缓控释剂指导原则,设定三个标准,2h 释放百分率(Q 2)以30%为标准;6h 释放百分率(Q 6)以60%为标准;10h 释放百分率(Q 10)以85%为标准,采用综合评分法对正交试验结果分析,即综合评分K 的计算公式为:K =|Q 2-30%|+|Q 6-60%|+|Q 10-85%|,K 值越小说明优化条件越佳[7]㊂因素水平见表1,正交试验结果见表2,方差分析见表3㊂表1㊀因素水平表Table 1㊀Factor level table水平因素A(HPMC K100M)B (GNPS)C (十八醇)D(乙基纤维素)122212122254151532861818表2㊀正交试验及结果Table 2㊀Orthogonal test and results实验号A B C D 实验结果K 1111140.832122229.163133356.724212362.115223134.856231235.747313265.928321340.269332138.75均值142.23756.28738.94338.143均值244.23334.75743.34043.607均值348.31043.73752.49753.030极差6.07321.53013.55414.887第49卷第10期王旭:美洲大蠊提取物胃漂浮缓释片制备研究73㊀表3㊀方差分析Table 3㊀Analysis of variance因素偏差平方和自由度F 比F 临界值显著性HPMC K100M 57.4912 1.0009.000GNPS701.684212.2059.000∗十八醇286.8682 4.9909.000乙基纤维素340.2602 5.9189.000误差57.492由表2可各因素对尿嘧啶释放百分率的影响为B>D>C>A,直观分析最佳处方为A 1B 2C 1D 1,方差分析结果表明,B 有显著性差异,拟定GFSRTPA 处方为HPMC K100M 22g,GNPS 4g,十八醇12g,乙基纤维素12g,美洲大蠊提取物10g,1%硬脂酸镁㊂2.6㊀验证试验按上述确定的最佳处方,制备三批GFSRTPA,测定尿嘧啶释放百分率㊂结果见图4㊂GFSRTPA 的12h 释放百分率为91.44%ʃ0.44%,结果表明,最佳处方的重现性良好㊂图4㊀三批GFSRTPA 尿嘧啶释放百分率曲线图Fig.4㊀Uracil release percentage curve of GFSRTPAin three batches3㊀结㊀论(1)胃漂浮片依据动力学平衡原理,亲水凝胶材料吸水膨胀在片剂表面形成凝胶,维持胃漂浮片的密度小于1,保持漂浮性,控制药物释放[8]㊂本实验用HPMC 为胃漂浮缓释片的骨架材料,以GNPS 为助漂剂,和其他辅料混合制备GFSRTPA,HPMC 遇水形成凝胶,控制药物释放㊂(2)胃漂浮片的体外释放度测定采用的装置通常为篮法[9]和桨法[10],因需要观察片剂的体外漂浮性能,故选用桨法装置㊂美洲大蠊的主要成分有氨基酸类㊁核苷类㊁有机酸㊁小分子肽㊁生物碱类物质㊁香豆素类化合物[11-16]等成分,尚未有研究指出美洲大蠊产生治疗作用的特定成分,因此,本实验选择核苷类中含量较高的尿嘧啶为指标成分,测定体外释放度㊂(3)实验通过单因素考察,进行正交试验设计筛选优化处方,确定了处方为HPMC K100M 22g,GNPS 4g,十八醇12g,乙基纤维素12g,美洲大蠊提取物10g,1%硬脂酸镁,采用湿法制粒压片法制备GFSRTPA,实验结果表明,12h 内GFSRTPA 缓慢释放药物,12h GFSRTPA 的尿嘧啶的体外释放百分率为91.44%ʃ0.44%,制备方法简单,易于操作㊂参考文献[1]㊀王莉,王娟.临床常用胃溃疡药物研究现状及进展[J].临床合理用药杂志,2011,4(14):178-180.[2]㊀李奇娟,王战国,刘巧,等.美洲大蠊研究现状及其研究中关键问题分析与展望[J].中国中药杂志,2018,43(07):1507-1516.[3]㊀邹俊波,桑文涛,王芳,等.美洲大蠊提取物对乙醇致小鼠急性胃溃疡的预防作用[J].中成药,2016,38(11):2325-2331.[4]㊀李远辉,冯建安,武亚晓,等.混料设计优化康复新胃漂浮片处方[J].中国实验方剂学杂志,2015,21(6):25-28.[5]㊀牛庆凤,王斌,李涛,等.假酸浆籽胶质多糖的结构及凝胶特性研究[J].现代食品科技,2015,31(09):68-73.[6]㊀何海花,于永杰,王旭,等.美洲大蠊提取物胃漂浮缓释片的体外释放度评价[J].世界最新医学信息文摘,2019,19(42):180-182.[7]㊀何利,刘巍,王冠华,等.基于正交试验优选芍药甘草汤胃漂浮缓释片处方工艺研究[J].亚太传统医药,2020,16(01):62-65.[8]㊀史振祺,蒋新国.胃漂浮片的研究进展[J].中国医药工业杂志,2003(04):45-48.[9]㊀付英杰,王建安,侯林,等.弥罗松酚胃漂浮片的制备[J].中成药,2014,36(8):1644-1648.[10]刘文,王群,陈中芬,等.戊己胃漂浮片中多指标成分的体外释放及体内滞留时间考察[J].中国实验方剂学杂志,2013,19(23):43-46.[11]吕娜,沈连刚,李广志,等.康复新液化学成分研究[J].中国现代中药,2017,19(04):488-490.[12]Neupert S,Predel R.Mass spectrometric analysis of single identified neurons of an insect [J ].Biochemical &Biophysical Research Communications,2005,327(3):645.[13]廖芳,朱伟,周洁,等.大孔吸附树脂纯化美洲大蠊多肽的工艺研究[J].中草药,2016,47(19):3420-3425.[14]黄博,敬勇,张秀娟,等.HPLC 法测定美洲大蠊药材中尿嘧啶㊁次黄嘌呤及肌苷的含量[J].中药材,2014,37(9):1537-1540.[15]焦春香,张成桂,刘光明.美洲大蠊醇提水溶性成分中挥发性成分的气相色谱-质谱分析[J].时珍国医国药,2012,23(11):2797-2798.[16]LUO S L,HUANG X J,WANG Y,et al.Isocoumarins from Americancockroach (Periplaneta americana)and their cytotoxic activities[J].Fitoterapia,2014,95(10):115-120.。
《人工智能-一种现代方法》第四版习题答案
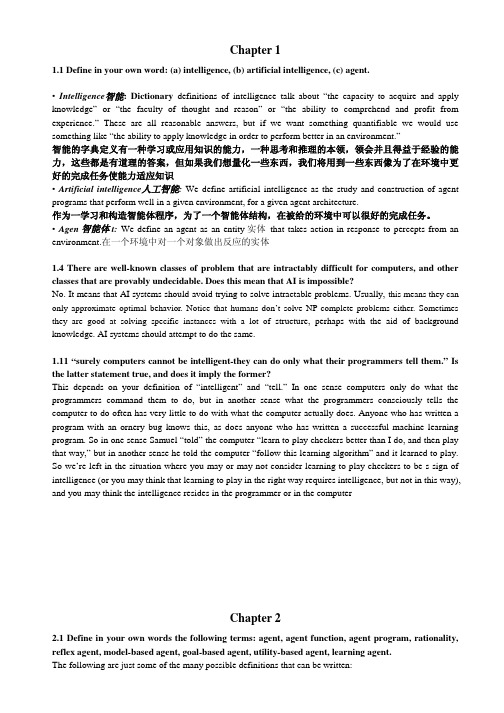
2.1 Define in your own words the following terms: agent, agent function, agent program, rationality, reflex agent, model-based agent, goal-based agent, utility-based agent, learning agent. The following are just some of the many possible definitions that can be written:
1.11 “surely computers cannot be intelligent-they can do only what their programmers tell them.” Is the latter statement true, and does it imply the former? This depends on your definition of “intelligent” and “tell.” In one sense computers only do what the programmers command them to do, but in another sense what the programmers consciously tells the computer to do often has very little to do with what the computer actually does. Anyone who has written a program with an ornery bug knows this, as does anyone who has written a successful machine learning program. So in one sense Samuel “told” the computer “learn to play checkers better than I do, and then play that way,” but in another sense he told the computer “follow this learning algorithm” and it learned to play. So we’re left in the situation where you may or may not consider learning to play checkers to be s sign of intelligence (or you may think that learning to play in the right way requires intelligence, but not in this way), and you may think the intelligence resides in the programmer or in the computer
Gurobi最优算法和启发式算法的融合
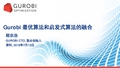
Gurobi 最优算法和启发式算法的融合顾宗浩GUROBI CTO, 联合创始人深圳, 2018年7月13日Outline•MIP Algorithm•Heuristics and (vs.) optimization •Gurobi heuristics•Non-LP based•LP based•Reformulation•Improvement•SubMIP and recursive•Features helping heuristics •Gurobi heuristic parameters •User input for Gurobi heuristics •MIP start/Multiple MIP starts•MIP hint•Partition heuristic•Heuristic callback•What to do with too big/hard models•MIP算法•启发式和(vs.)优化•Gurobi启发式算法•基于非LP•基于LP•模型改建•改进型•子MIP和递归•有助于启发式的功能•Gurobi 启发式参数•Gurobi启发式的用户输入功能•MIP 起始值/多个MIP起始值•MIP提示•分区启发式•启发式回调•如何处理太大/太难的模型概要Gurobi MIP Algorithms Gurobi 混合整数规划算法MIP Building Blocks 模块•Presolve•Tighten formulation and reduce problem size•Solve continuous relaxations•Ignoring integrality•Gives a bound on the optimal integral objective•Cutting planes•Cut off relaxation solutions•••Primal heuristics•Method, NodeMethodPresolve, PrePasses, AggFill, Aggregate, DualReductions, PreSparsify, ...Cuts, CutPasses, CutAggPasses, GomoryPasses, CliqueCuts, CoverCuts, FlowCoverCuts, ...预优化求解连续松弛模型切平面MIP Presolve预优化•Reduce problem size•Strengthen LP relaxationDisconnected•Identify problem sub-structures••Similar to LP presolve, but more powerful: 与LP 预优化类似,但更强大•Exploit integrality•Round fractional bounds and right hand sides•Lifting/coefficient strengthening•Probing•Does not need to preserve duality•We only need to be able to uncrush a primal solution•Neither a dual solution nor a basis needs to be uncrushedMIP –LP Relaxation LP 松弛问题MIP –Branching 分支LP based Branch-and-Bound 基于LP的分支定界法Upper BoundGAPLower BoundSolving a MIP Model 求解混合整数模型O b j e c t i v ePresolving Node SelectionNode PresolveLP Relaxation Cutting PlanesHeuristicsBranchingPresolving Node Selection LP Relaxation Cutting PlanesNode Presolve BranchingHeuristics Read MPS format model from file /models/mip/roll3000.mps.bz2Reading time = 0.03 secondsroll3000: 2295 rows, 1166 columns, 29386 nonzerosOptimize a model with 2295 rows, 1166 columns and 29386 nonzerosCoefficient statistics:Matrix range [2e-01, 3e+02]Objective range [1e+00, 1e+00]Bounds range [1e+00, 1e+09]RHS range [6e-01, 1e+03]Presolve removed 1308 rows and 311 columnsPresolve time: 0.08s Presolved: 987 rows, 855 columns, 19346 nonzerosVariable types: 211 continuous, 644 integer (545 binary)Root relaxation: objective 1.112003e+04, 1063 iterations, 0.03 secondsNodes | Current Node | Objective Bounds | WorkUnexpl | Obj Depth IntInf 0 0 11120.0279 0 154 -0 0 11526.8918 0 207 -0 0 11896.9710 0 190 -Presolving Node SelectionNode PresolveLP RelaxationCutting PlanesHeuristicsBranchingWhich open node should be processed next?Presolving Node SelectionLP RelaxationCutting PlanesNode PresolveBranching HeuristicsPresolved: 987 rows, 855 columns, 19346 nonzerosVariable types: 211 continuous, 644 integer (545 binary)Root relaxation: objective 1.112003e+04, 1063 iterations, 0.03 secondsNodes | Current Node | Objective Bounds | WorkDepth IntInf | Incumbent BestBd 0 0 11120.0279 0 154 -11120.0279 -0 0 11526.8918 0 207 -11526.8918 -0 0 11896.9710 0 190 -11896.9710 -H 327 218 13135.000000 12455.2162 5.18% 42.6 1sPresolving Node SelectionLP Relaxation Cutting Planes Node Presolve BranchingHeuristics Root relaxation: objective 1.112003e+04, 1063 iterations, 0.03 seconds Nodes | Current Node | Objective Bounds | WorkExpl Unexpl | Obj Depth IntInf 0 0 11120.0279 0 154 -0 0 11526.8918 0 207 -0 0 11896.9710 0 190 -0 0 12151.4022 0 190 -0 0 12278.3391 0 208 -5485 634 12885.3652 52 143 12890.0000 12829.0134 0.47% 54.5 25sCutting planes:Learned: 4Cover: 39Implied bound: 8Clique: 2MIR: 112Flow cover: 27GUB cover: 11Zero half: 91Explored 6808 nodes (357915 simplex iterations) in 27.17 secondsThread count was 4 (of 8 available processors)PresolvingNode Selection LP Relaxation Cutting Planes Node Presolve BranchingHeuristicsRoot relaxation: objective 1.112003e+04, 1063 iterations, 0.03 seconds0 0 11120.0279 0 154 -0 0 11526.8918 0 207 -0 0 11896.9710 0 190 -0 0 12448.7684 0 181 -H 0 0 16129.000000 12448.7684 22.8% -H 0 0 15890.000000 12448.7684 21.7% -0 2 12448.7684 0 181 15890.0000 12448.7684 21.7% -H 142 129 15738.000000 12450.7195 20.9% 43.8 1sH 212 189 14596.000000 12453.8870 14.7% 42.3 1sH 217 181 13354.000000 12453.8870 6.74% 42.6 1s * 234 181 40 13319.000000 12453.8870 6.50% 42.1 1sH 254 190 13307.000000 12453.8870 6.41% 41.3 1sH 284 194 13183.000000 12453.8870 5.53% 42.6 1s H 286 194 13169.000000 12453.8870 5.43% 42.7 1sBranch-and-Cut 分支切割Presolving Node SelectionLP Relaxation Cutting Planes Node PresolveBranchingHeuristics Presolved: 987 rows, 855 columns, 19346 nonzerosVariable types: 211 continuous, 644 integer (545 binary)Root relaxation: objective 1.112003e+04, 1063 iterations, 0.03 secondsNodes | Current Node | Objective Bounds | Work| Obj Depth IntInf | Incumbent BestBd 0 0 11120.0279 0 154 -0 0 11526.8918 0 207 -0 0 11896.9710 0 190 -H 0 0 15890.000000 12448.7684 21.7% -0 2 12448.7684 0 181 15890.0000 12448.7684 21.7% -...1066 702 12956.2676 31 192 13087.0000 12629.5426 3.50% 37.2 5s1097 724 12671.8285 8 147 13087.0000 12671.8285 3.17% 41.6 10s1135 710 12732.5601 32 126 12890.0000 12727.1362 1.26% 44.6 15s3416 887 12839.9880 46 136 12890.0000 12780.7059 0.85% 49.7 20s 5485 634 12885.3652 52 143 12890.0000 12829.0134 0.47% 54.5 25sHeuristics and (vs.) optimization 启发式和(对立)优化•优化是NP-hard•我们只应考虑启发式,但可行性问题也是NP-hard•理论上, 启发式和优化难度是一样的•许多实际问题经常被解到最优•我的优化问题很难解•所以我只开发了自己的启发式算法•那么你就是这次演讲的最佳听众•启发式算法和优化算法并不对立, 可以融合•我们将展示•Gurobi启发式的想法(ideas), 或许可以帮助您开发和改进您的启发式算法•如何融合Gurobi优化算法和您的启发式算法为您找到更好的解决方案GUROBI Heuristics GUROBI启发式算法Gurobi Heuristics 启发式算法•Gurobi has more than 30 heuristics有30多种启发式算法•Different types 不同种类•Non-LP based基于非LP•Enumerate, search, greedy, …•LP based基于LP•Rounding, fixing & diving, …•Reformulation模型改建•Zero-objective, min relaxation, …•Improvement改进型•RINS..•SubMIP and recursive子MIP和递归•Target heuristic, RINS, …•Problem specific针对具体问题的启发式•Fixed charge network heuristic•Features helping heuristics有助于启发式的功能•Pump reduceNon-LP Based Heuristics: Greedy基于非LP启发式算法:贪婪算法•Famous algorithms 着名算法•Optimal 最优的: shortest path最短的路径, min spanning tree最小生成树•Not optimal非最优的: 0-1 Knapsack 背包Max 10 u + 8 v + 11 x + 7 y + 5 zs.t. 3 u + 4 v + 6 x + 4 y + 3 z ≤ 14u, v, x, y, z are binary variablesSorting variables based on the ratio of the obj. coefficient and constraint coefficient, already sortedSetting variables to one based on the order until it become infeasible. Here setting y to one become infeasibleSo the solution is (u, v, x, y, z) = (1, 1, 1, 0, 0) with obj. value 29Optimal solution (u, v, x, y, z) = (1, 1, 0, 1, 1) with obj. value 30•Gurobi blind heuristics 盲目启发式(blind means not using LP relaxation solution)•Sort binary/integer variables based on some measure 用某种度量对二进制/整数变量进行排序•Fixing them in the greedy order 按贪婪顺序固定它们•Propagate fixing and bound changes for each fix 传播变量固定和收紧界值•Without it, it is almost impossible to find a feasible solution•Solve the remaining LP model, if there are continuous variables•LP based greedy heuristics 基于LP的贪婪算法•It is often more effective to use relaxation solution to sort variables 用松弛解对变量排序通常更有效Non-LP Based Heuristics 基于非LP启发式算法•They can find integer solutions quickly 有可能可以快速找到整数解•The quality of the solutions is often very poor 解的质量通常很差•One poor solution is good enough, poor solutions often won’t help overall optimization 一个差的解就足够了,差的解往往无法帮助整体优化•Multi cores and difficulty to parallelize the root node are the reasons to pay some attention to non-LP based heuristics 多核电脑和难以对根节点并行化是关注基于非LP的启发式算法的原因LP Based Heuristics 基于LP启发式算法•Rounding 取整法•Solve LP relaxation, round the solution values to nearest integer values 解LP松弛问题,将解值四舍五入到最接近的整数值•0-1 knapsack example (same example) 0-1背包例子(相同例子)Max 10 u + 8 v + 11 x + 7 y + 5 zs.t. 3 u + 4 v + 6 x + 4 y + 3 z ≤ 14u, v, x, y, z are binary variablesOptimal LP relaxation solution (u, v, x, y, z) = (1, 1, 1, ¼, 0)Rounded solution (u, v, x, y, z) = (1, 1, 1, 0, 0) is integer feasible with obj. value 29•Simple rounding won’t work well, especially for models with equalities简单的取整不会很好,特别是具有等式约束的模型•Consider integer values on both sides 考虑两边的整数值•Rounding with propagating, fixing variables and tightening bounds取整时需传播变量固定和收紧界值•Gurobi has several different versions of rounding heuristics Gurobi有多种不同版本的取整启发式算法•Most Gurobi heuristics are LP based or need LP relaxation solutions 大多数Gurobi启发式算法都是基于LP或需要LP松弛解•LP relaxation solution is very important for heuristics to get high quality solutions!LP松弛解对于启发式算法获取高质量解非常重要!•Example 例子Min 3 u + 8 v + 3 w + 2 x + 7 y + 5 zs.t. 3 u + 4 v -4 w + 8 x + 4 y + 3 z ≤ 95 u + 2 v + 4 x + 7 y + 9 z = 15u, v, x, y, z are non negative integer variables, w is a binary variable•Zero-objective heuristic 去目标启发式•Remove the objective and solve it as a feasible problem 删除目标并将其为可行问题去解•Hope that presolve can have more reductions and the resulting presolved model is easier to solve 希望预预优化可以使模型变的更小,并且最终的预优化模型更容易解•For this example, the reformulated model is 对于这个例子,重新改建的模型是Min 0s.t. 3 u + 4 v -4 w + 8 x + 4 y + 3 z ≤ 95 u + 2 v + 4 x + 7 y + 9 z = 15u, v, x, y, z are non negative integer variables, w is a binary variable•Variables x and v are parallel, x can be fixed to 0•Variable w can be fixed to 1, which will only help the feasibility of the first constraint•Minimum relaxation heuristic 最小松弛启发式•For each inequality, add one penalty variable for the constraint violation 对于每个不等式,加一个违反约束的惩罚变量•For each equality, add two penalty variables for the two directions of the violation对于每个等式,加两个违反约束的惩罚变量, 两个方向各一个•Then minimize the sum of violations 然后对违约总和求最小化•If the optimal solution has the sum = 0, then we find a feasible solution; otherwise the original model is infeasible 如果最优总和为0, 那我们找到一个可行解; 否则原始模型是不可行的•For this example, the reformulated model is 对于这个例子,重新改建的模型是Min r + s + ts.t. 3 u + 4 v -4 w + 8 x + 4 y + 3 z -r ≤ 95 u + 2 v + 4 x + 7 y + 9 z + s -t = 15u, v, x, y, z are non negative integer variablesw is a binary variabler, s, t are non negative continuous variablesRINS•Relaxation induced neighborhood search(RINS) 松弛诱导邻域搜索•Given the incumbent (the best integer solution found so far) and the current fractional solution of the node relaxation 给定现任整数解和节点松弛的当前分数解•Fix a variable if its incumbent value and its relaxation value agrees 如果变量的整数解值和松弛解值一致,则固定变量•Solve the partially fixed model as a subMIP将部分固定的模型作为子MIP去解•It is an improvement heuristics 这是一种改进型的启发式算法•It is the our most effective heuristic 这是我们最有效的启发式算法SubMIP and Recursive Solve 子MIP和递归•Many Gurobi heuristics will 许多Gurobi启发式算法会•Have a target to fix some percentage of variables, say 80% 设一个目标来固定一定比例的变量,比如80%•Fix one variable and then propagate 固定一个变量然后传播•Repeat fixing and propagating until the target is reached or it becomes infeasible 重复固定和传播,直到达到目标或它变得不可行•Solve it as a subMIP将其为子MIP去解•In the subMIP, it will call the same heuristics, so recursively 在子MIP中,它将以递归方式调用相同的启发式算法•It often works well and finds feasible solutions quickly 它通常非常有效,可迅速找到可行解Feasibility Pump Heuristic泵式缩减启发式•Fischetti, Glover and Lodi, 2004•Solve the relaxation and round the solution解松弛问题并舍入到整数解•Replace the objective to minimize the distance to the rounded solution (quadratic)目标换成到舍入整数解距离最小(二次)•Use L1 norm (sum |xj –xj*|), where x* is the rounded solution (linear)使用L1范数(sum | xj-xj* |),其中x *是取整解(线性)•If a binary variable xj = 0.3, then xj* = 0, then the objective part for xjis | xj–0| = xj, i.e. obj. coefficient is 1•If a binary variable xj= 0.7, then obj. coefficient will be -1•Solve the modified LP and repeat 解修改后的LP并重复•Until it hits some limit or the relaxation solution is integer feasible直到它达到一定限值或松弛解是整数可行的•Setting the limit to e.g. 10, i.e. solving the LP 10 times is expensive and it usually won’t be lucky例如将限值设为10,则需解LP10次, 很化时,通常很难运气好Pump Reduce泵式缩减•Motivated by feasibility pump heuristic 受泵式缩减启发式算法的启发•Observation 观察•Most models are dual degenerate, i.e. relaxation has alternative optimal solutions大多数模型对偶退化,即松弛问题有多个的最优解•Goal 目标• A relaxation solution with less fractional integer variables 有较少整数变量取分数值的松弛解•Possible zero fractional integer variables, but not the goal, so it isn’t heuristic 可能没有分数整数变量,但不是目标,所以它不是启发式的•Such relaxation solution helps heuristics and b&b significantly to find integer feasible solutions这样的松弛解很显著地帮助启发式和b&b找到可行的整数解•Steps步骤•Solve the relaxation and fix all variables with nonzero reduced costs, making sure to stay in the optimal space 解松弛问题,固定非零递减成本的所有变量, 确保保持在最优空间•Round the relaxation solution, replace the objective with L1 norm distance to the rounded solution舍入松弛解,目标换成到舍入整数解距离最小(L1 范数)•Solve the modified LP, round and repeat解修改后的LP并重复•Until it hits some limit or the number of fractional integer variables doesn’t go down直到它达到一定限值或取分数值的整数变量的数量不下降GUROBI Heuristic Parameters Gurobi 启发式参数Heuristic Parameters 启发式参数•Main MIP parameter 主要MIP参数, MIPFocus•Main heuristic parameter 主要启发式参数, Heuristics•Individual heuristic parameters 个别启发式参数•Other parameters affecting feasible solutions 影响可行解的其他参数MIPFocus•Define high-level solution strategy 定义解高层策略•Default默认, balance between finding new feasible solutions and proving that the current solution is optimal. 在找新的可行解和证明最优性之间取得平衡•= 1, more interested in finding feasible solutions quickly 更注重找到可行解•= 2, more attention on proving optimality 更注重证明最优性•= 3, focus on the objective bound 更注重目标界值Heuristics•Main heuristic parameter主要启发式参数•The parameter value is roughly the fraction of time that we will spend on heuristics 参数值大致是我们在启发式上花费时间的部分值•Default value默认值= 0.05•> 0.05, more aggressive, 1 most aggressive•< 0.05, less aggressive, 0 no heuristicsIndividual heuristic parameters个别启发式参数•Pump reduce 泵式缩减(or degenerate simplex moves), Degenmoves •Feasibility pump heuristic泵式缩减启发式, PumpPasses•Improvement heuristic parameters改进型的启发式参数•ImproveStartGap•ImproveStartNodes•ImproveStartTime(warning: not deterministic)•Minimum relaxation heuristic最小松弛启发式, MinRelNodes•RINS heuristic RINS启发式, RINS•Zero objective heuristic去目标启发式, ZeroObjNodesOther Parameters Affecting Heuristics影响启发式的其他参数•Nodes explored by sub-MIP heuristics, SubMIPNodes•Branch direction preference, BranchDir•Setting the value to 1 may help MIP diving to find a feasible solution more quickly•Tuning criterion, TuneCriterion•= 2 objective value, i.e. focusing more on finding good feasible solutionsUser Input for GUROBI Heuristics 启发式的用户输入功能MIP Start / Multiple MIP Starts MIP起始值/多个MIP起始值•User can provide a MIP start or multiple MIP starts (new in 8.0) 用户可以提供一个或多个MIP起始值(多个为8.0的新功能)• A good MIP start, even a partial solution often can produce a good feasible solution instantly 良好的MIP起始值,即使是部分解,也可以立即产生好的可行解•Useful when you have multiple partial solutions 有多个部分解可能会很有用•MIP solver will try to complete them, and will store the ones it finds•For distributed MIP, MIP starts will be evaluated on different machines 对于分布式MIP,将在不同的机器上评估MIP起始值Variable Hints MIP提示•Provide hints to the solver about which variable should take which value 向优化器提示哪个变量应采用哪个值•Guides heuristics and branching 指导启发式和分支•VarHintVal attribute 属性•Specifies a value for a variable 指定变量的值•VarHintPri attribute 属性•Specifies a level of confidence in this particular variable value 指定此特定变量值的置信度•Comparison to MIP start 与MIP起始值比较•MIP start is used to provide an initial feasible solution to the solver MIP起始值用于为优化器提供初始可行解•Is evaluated prior to starting the solution process•Provides incumbent if feasible•Does not influence solution process if it is not feasible•Variable Hints guide the search 变量提示指导搜索•High quality hints should lead to a high quality solution quickly•Either through heuristics or through branching•Affects the whole solution processPartition Heuristic分区启发式算法•User-specified local improvement heuristic 用户指定的局部改进型启发式算法•RINS is our most effective heuristic RINS是我们最有效的启发式算法•It is a sub-MIP heuristic 这是一个子MIP启发式算法•Fix a subset of the variables to incumbent values 将变量的子集固定为现任整数解的值•Solve the resulting MIP (recursively) 解生成的MIP(递归)•Reoptimizes over just that portion of the problem•Sub-MIP heuristics extremely effective in general 子MIP启发式算法一般非常有效•How to choose the sub-problem to reoptimize? 如何选择子问题进行重新优化?•RINS chooses automatically RINS自动选择•This feature allows user to make the choice 此功能允许用户做出选择•Example sub-problems:•All decisions related to a single time period•All decisions related to a single machine•All decisions related to physical sub-regions (e.g., Western US, Eastern US, etc.)MIP Heuristic Callback 启发式回调•Motivations•Our MIP solver is mostly a black box solver 我们的MIP求解器主要是黑盒求解器•We try to recognize some common structures, but very limited•Users know the structure of their model 用户知道他们模型的结构•Relaxation solutions help heuristics a lot 松弛解对启发式算法很有帮助•Knowledge of problem structure and the relaxation solutions often mean fast good feasible solutions 对问题结构和松弛解的了解通常意味着快速找到可行解•Heuristic callback•At each node, Gurobi will call back 在每个节点,Gurobi都会回调•Users can query the relaxation solution and use it to guide their heuristics 用户可以查询松弛解并使用它来指导他们的启发式算法•Users can provide a full or partial solution vector to Gurobi through callback 用户可以通过回调向Gurobi 提供完整或部分解•If it is partial, Gurobi will try to complete it 如果它是部分的,Gurobi将尝试完成它What to do with too big/hard models 如何处理太大/太难的模型Too Big/Hard Models, Really?太大/太难的模型,真的吗?•“My model is too big or too hard, I have no choice but heuristic”, really? “我的模型太大或太难,我别无选择,只有启发式算法”,真的吗?•Old MIP experiences don’t count 旧的MIP经验不算数•At the end of 80’s and earlier 90’s, people in electrical power industry concluded that MIP was a nicetool, which couldn’t solve real unit commitment model•Close to 2000, people revisited MIP technology and people now solve the unit commitment modelroutinely•The similar stories happened more and more•I tried open source solvers, they are hopeless 我试过开源优化器,没有希望解我的问题•We have a lot of users, who send us their models, since the open solver they used couldn’t find afeasible solution in hours. Gurobi often solved the models in less than one second•All open source solvers are way behind the state of art commercial solvers•Gurobi users often solve their MIP models with millions of variables/constraints Gurobi用户经常解有数百万个变量/约束的MIP模型•Our customer model sets have a lot of such models, many of them we can solve or find good solutions within 10% MIPGap.Gurobi MIP Library 模型集(4538 models)1101001000100001000001000000100000001000000001E+09C o l u m n sMIP 速度不断提高, 主要版本每次提高几乎两倍0.000.501.001.502.002.503.00V e r s i o n -t o -V e r s i o n S p e e d u p•Have you tried to solve the relaxation? 你试过解松弛问题吗?•LP relaxation is polynomial-time solvable LP松弛问题是多项式时间可解的•Gurobi has solved LP models with 100M+ variables/constraints Gurobi解过许多超过几亿个变量/约束的LP模型•Relaxation solution is often very useful for heuristics松弛解对启发式算法很有帮助•The objective value of the relaxation solution provides the bound, without it, it is hard to know how gooda heuristic solution is 松弛解提供目标界值,没有它,很难知道启发式解有多好•Have you tried to reduce the models?你试过减小模型吗?•Aggregate汇总•Daily schedule -> weekly schedule日计划->周计划•Decompose big model into smaller pieces 将大模型分解为较小的部分•World -> America, Europe and Asia 世界->美洲,欧洲和亚洲•Local improvement 局部改进•Use heuristic to generate an initial solution 使用启发式算法生成初始解•Use MIP to reoptimize over a portion of the model, like RINS 使用MIP重新优化模型的一部分,如RINS •No lower bound, but often produces very high quality global solutions 没有下限,但通常会产生非常高质量的整体解•Successful stories to combine optimization and heuristics融合优化和启发式的成功案例•MIP based heuristics 基于MIP的启发式算法•Rolling horizon heuristics 滚动时段启发式算法•Relax integrality of future periods•May aggregate future time periods•Solve smaller LP/MIP•Air taxi and mining•Local search heuristics 局部搜索启发式算法•In group of periods, machines etc, solve smaller LP/MIP•Lenstra et al., local search in combinatorial optimization•Lin-Kernighan heuristic for TSP 货郎担问题的启发式算法•Solve relaxation and use reduced costs to guide•Etc.Always Try MIP总是试试MIPConclusion结论•Always try Gurobi, it should be better than pure heuristics 试试Gurobi,它应该比纯启发式更好!。
热电联产中英文对照外文翻译文献
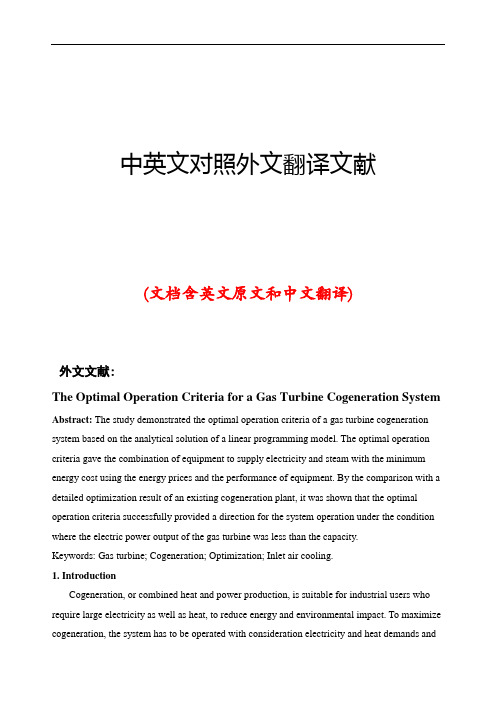
中英文对照外文翻译文献(文档含英文原文和中文翻译)外文文献:The Optimal Operation Criteria for a Gas Turbine Cogeneration System Abstract: The study demonstrated the optimal operation criteria of a gas turbine cogeneration system based on the analytical solution of a linear programming model. The optimal operation criteria gave the combination of equipment to supply electricity and steam with the minimum energy cost using the energy prices and the performance of equipment. By the comparison with a detailed optimization result of an existing cogeneration plant, it was shown that the optimal operation criteria successfully provided a direction for the system operation under the condition where the electric power output of the gas turbine was less than the capacity.Keywords: Gas turbine; Cogeneration; Optimization; Inlet air cooling.1. IntroductionCogeneration, or combined heat and power production, is suitable for industrial users who require large electricity as well as heat, to reduce energy and environmental impact. To maximize cogeneration, the system has to be operated with consideration electricity and heat demands andthe performance of equipment. The optimal operation of cogeneration systems is intricate in many cases, however, due to the following reasons. Firstly, a cogeneration system is a complex of multiple devices which are connected each other by multiple energy paths such as electricity, steam, hot water and chilled water. Secondly, the performance characteristics of equipment will be changed by external factors such as weather conditions.For example, the output and the efficiency of gas turbines depend on the inlet air temperature. Lastly,the optimal solution of operation of cogeneration systems will vary with the ratio of heat demand to electricity demand and prices of gas, oil and electricity.Because of these complexities of cogeneration systems, a number of researchers have optimal solutions of cogeneration systems using mathematical programming or other optimization techniques. Optimization work focusing on gas turbine cogeneration systems are as follows. Yokoyama et al. [1] presented optimal sizing and operational planning of a gas turbine cogeneration system using a combination of non-linear programming and mixed-integer linear programming methods. They showed the minimum annual total cost based on the optimization strategies. A similar technique was used by Beihong andWeiding [2] for optimizing the size of cogeneration plant. A numerical example of a gas turbine cogeneration system in a hospital was given and the minimization of annual total cost was illustrated. Kong et al. [3] analyzed a combined cooling, heating and power plant that consisted of a gas turbine, an absorption chiller and a heat recovery boiler. The energy cost of the system was minimized by a linear programming model and it was revealed that the optimal operational strategies depended on the load conditions as well as on the cost ratio of electricity to gas. Manolas et al. [4] applied a genetic algorithm (GA) for the optimization of an industrial cogeneration system, and examined the parameter setting of the GA on the optimization results. They concluded that the GA was successful and robust in finding the optimal operation of a cogeneration system.As well as the system optimization, the performance improvement of equipment brings energy cost reduction benefits. It is known that the electric power output and the efficiency of gas turbines decrease at high ambient temperatures. Some technical reports [5, 6] show that the electric power output of a gas turbine linearly decreases with the rise of the ambient temperature, and it varies about 5 % to 10 % with a temperature change of 10 ◦C. Therefore, cooling of the turbine inlet air enhances electric output and efficiency. Some studies have examined theperformance of the gas turbine with inlet air cooling as well as the effect of various cooling methods [7, 8, 9].The cooling can be provided without additional fuel consumption by evaporative coolers or by waste heat driven absorption chillers. The optimal operation of the system will be more complex, however, especially in the case of waste heat driven absorption chillers because the usage of the waste heat from the gas turbine has to be optimized by taking into consideration the performance of not only the gas turbine and the absorption chiller but also steam turbines, boilers and so on. The heat and electricity demands as well as the prices of electricity and fuels also influence the optimal operation.The purpose of our study is to provide criteria for optimal operation of gas turbine cogeneration systems including turbine inlet air cooling. The criteria give the minimum energy cost of the cogeneration system. The method is based on linear programming and theKuhn-Tucker conditions to examine the optimal solution, which can be applied to a wide range of cogeneration systems.2. The Criteria for the Optimal Operation of Gas Turbine Cogeneration SystemsThe criteria for the optimal operation of gas turbine cogeneration systems were examined from the Kuhn-Tucker conditions of a linear programming model [10]. A simplified gas turbine cogeneration system was modeled and the region where the optimal solution existed was illustrated on a plane of the Lagrange multipliers.2.1. The Gas Turbine Cogeneration System ModelThe gas turbine cogeneration system was expressed as a mathematical programming model. The system consisted of a gas turbine including an inlet air cooler and a heat recovery steam generator (HRSG), a steam turbine, an absorption chiller, a boiler and the electricity grid. Figure 1 shows the energy flow of the system. Electricity, process steam, and cooling for process or for air-conditioning are typical demands in industry, and they can be provided by multiple suppliers. In the analysis, cooling demands other than for inlet air cooling were not taken into account, and therefore, the absorption chiller would work only to provide inlet air cooling of the gas turbine. The electricity was treated as the electric power in kilowatts, and the steam and the chilled water were treated as the heat flow rates in kilowatts so that the energy balance can be expressed in the same units.Figure 1. The energy flow of the simplified gas turbine cogeneration system with the turbineinlet air cooling.The supplied electric power and heat flow rate of the steam should be greater than or equal to the demands, which can be expressed by Eqs. (1-2).(1)(2)where, xe and xs represent the electric power demand and the heat flow rate of the steam demand. The electric power supply from the grid, the gas turbine and the steam turbine are denoted by xG, xGT and xST, respectively. xB denotes the heat flow rate of steam from the boiler, and xAC denotes the heat flow rate of chilled water from the absorption chiller. The ratio of the heat flow rate of steam from the HRSG to the electric power from the gas turbine is denominated the steam to electricity ratio, and denoted by ρGT. Then, ρGTxGT represents the heat flow rate o f steam from the gas turbine cogeneration. The steam consumption ratios of the steam turbine and the absorption chiller are given as ωST and ωAC, respectively. The former is equivalent to the inverse of the efficiency based on the steam input, and the latter is equivalent to the inverse of the coefficient of performance.The inlet air cooling of the gas turbine enhances the maximum output from the gas turbine. By introducing the capacity of the gas turbine, XGT, the effect of the inlet air cooling was expressed by Eq. (3).(3).It was assumed that the increment of the gas turbine capacity was proportional to the heatflow rate of chilled water supplied to the gas turbine. The proportional constant is denoted byαGT.In addition to the enhancement of the gas turbine capacity, the inlet air cooling improves the electric efficiency of the gas turbine. Provided that the improvement is proportional to the heat flow rate of chilled water to the gas turbine, the fuel consumption of the gas turbine can be expressed as ωGTxGT¡βGTxAC, whereωGT is the fuel consumption ratio without the inlet air cooling and βGT is the improvement factor of the fuel consumption by the inlet air cooling. As the objective of the optimization is the minimization of the energy cost during a certain time period, Δt, the energy cost should be expressed as a function of xG, xGT, xST, xB and xAC. By defining the unit energy prices of the electricity, gas and oil as Pe, Pg and Po, respectively, the energy cost, C, can be given as:(4)where, ωB is the fuel consumpti on ratio of the boiler, which is equivalent to the inverse of the thermal efficiency.All the parameters that represent the characteristics of equipment, such as ωGT, ωST, ωAC, ωB, ρGT, αGT and βGT, were assumed to be constant so that the system could be m odeled by the linear programming. Therefore, the part load characteristics of equipment were linearly approximated.2.2. The Mathematical Formulation and the Optimal Solution From Eqs. (1–4), the optimization problem is formed as follows:(5)(6)(7)(8)where, x = (xG, xGT, xST, xB, xAC). Using the Lagrange multipliers, λ = (λ1, λ2, λ3), theobjectivefunction can be expressed by the Lagrangian, L(x,λ).(9)According to the Kuhn-Tucker conditions, x and λ satisfy the following conditions at the optimal solution.(10)(11)(12)(13)The following inequalities are derived from Eq. (10).(14)(15)(16)(17)(18)Equation (11) means that xi > 0 if the derived expression concerning the supplier i satisfies the equali ty, otherwise, xi = 0. For example, xG has a positive value if λ1 equals PeΔt. If λ1 is less than PeΔt, then xG equals zero.With regard to the constraint g3(x), it is possible to classify the gas turbine operation into two conditions.The first one is the case where the electric power from the gas turbine is less than the capacity,which means xG < XGT + αGTxAC. The second one is the case where the electric power from the gas turbine is at the maximum, which means xGT = XGT + αGTxAC. We denominate the former and the latter conditions the operational conditions I and II, respectively. Due to Eq. (12) of the Kuhn-Tucker condition, λ3 = 0 on the operational condition I, and λ3 > 0 on the operational condition II.2.3. The Optimal Solution where the Electric Power from the Gas Turbine is less than theCapacityOn the operational condition I where xG < XGT + αGTxAC, Eqs. (14–18) can be drawn on the λ1-λ2 plane because λ3 equals zero. The region surrounded by the inequalities gives the feasible solutions, and the output of the supplier i has a positive value, i.e. xi > 0, when the solution exists on the line which represents the supplier i.Figure 2 illustrates eight cases of the feasible solution region appeared on the λ1-λ2 plane. The possible optimal solutions ar e marked as the operation modes “a” to “g”. The mode a appears in the case A, where the grid electricity and the boiler are chosen at the optimal operation. In the mode b,the boiler and the steam turbine satisfy the electric power demand and the heat flow rate of the steam demand. After the case C, the electric power from the gas turbine is positive at the optimal operation.In the case C, the optimal operation is the gas turbine only (mode c), the combination of the gas turbine and the boiler (mode d) or the combination of the gas turbine and the grid electricity (mode e). In this case, the optimal operation will be chosen by the ratio of the heat flow rate of the steam demand to the electric power demand, which will be discussed later. When the line which represents the boiler does not cross the gas turbine line in the first quadrant, which is the case C’, only the modes c and e appear as the possible optimal solutions. The modes f and g appear in the cases D and E, respectively. The suppliersThe cases A through E will occur depending on the performance parameters of the suppliers and the unit energy prices. The conditions of each case can be obtained from the graphical analysis. For example, the case A occurs if λ1 at the intersection of G and B is smaller than that at the intersection of GT and B, and is smaller than that at the intersection of ST and B. In addition, the line B has to be located above the line AC so that the feasible solution region exists. Then, the following conditions can be derived.(19)(20)(21)Equation (19) means that the gas cost to produce a certain quantity of electricity and steam with the gas turbine is higher than the total of the electricity and oil costs to purchase the same quantity of electricity from the grid and to produce the same quantity of steam with the boiler.Equation (20) means that the electricity cost to purchase a certain quantity of electricity is cheaper than the oil cost to produce the same quantity of electricity using the boiler and the steam turbine. Equation (21) indicates that the reduction of the gas cost by a certain quantity of the inlet air cooling should be smaller than the oil cost to provide the same quantity of cooling using the boiler and the absorption chiller. Otherwise, the optimal solution does not exist because the reduction of the gas cost is unlimited by the inlet air cooling using the absorption chiller driven by the boiler.Figure 2. The possible cases of the optimal solution on the operational condition ISimilar ly, the following conditions can be derived for the other cases. The condition given as Eq. (21) has to be applied to all the cases below.Case B:(22)(23)Equation (22) compares the production cost of the electricity and the steam between the gas and the oil. The gas cost to produce a certain quantity of electricity and steam by the gas turbine is higher than the oil cost to produce the same quantity of electricity and steam by thecombination of the boiler and the steam turbine. Equation (23) is the opposite of Eq. (20), which means that the oil cost to produce a certain quantity of electricity by the boiler and the steam turbine is cheaper than the purchase price of electricity.Case C:(24)(25)(26)(27)Equation (24) is the opposite case of Eq. (19). Equation (25) compares the boiler and the gas turbine regarding the steam production, which is related to the mode d. In the case C, the oil cos t for the boiler is cheaper than the gas cost for the gas turbine to produce a certain quantity of steam. If the gas cost is cheaper, mode d is not a candidate for the optimal sol ution, as illustrated in the case C’. Equations (26) and (27) evaluate the effectiveness of the steam turbine and the inlet air cooling by the absorption chiller,resp ectively. The grid electricity is superior to the steam turbine and to the inlet air cooling in this case.Case D:In addition to Eq. (25),(28)(29)(30)Similarly to the case C’, the case D’ occurs if the inequality sign of Eq. (25) is reversed. Equation (28) is the opposite case of Eq. (22), which is the comparison of the electricity production between gas and oil. Equation (29) is the opposite case of Eq. (26), which is the comparison of the steam turbine and grid electricity. The gas cost to produce a certain quantity of electricity by the combination of the gas turbine and the steam turbine is cheaper than the purchase cost of the same quantity of electricity from the grid. Equation (30) gives the condition where the steam turbine is more advantageous than the inlet air cooling by the absorption chiller. The left hand side of Eq. (30) represents an additional steam required for a certain quantity of electricity production by the inlet air cooling. Therefore, Eq. (30) insists that the steam required for a certain quantity of electricity production by the steam turbine is smaller than that requiredfor the same quantity of electricity production by the inlet air cooling in this case, and it is independent of energy prices.Case E:In addition to Eq.(25),(31)(32)The case E’ occurs if Eq. (25) is reversed. Equations (31) and (32) are the opposite cases of Eqs. (27)and (30), which give the conditions where the inlet air cooling is more advantageous compared with the alternative technologies. In this case, Eq. (28) is always satisfied because of Eqs. (21) and (32).The conditions discussed above can be arranged using the relative electricity price, Pe/Pg and the relative oil price, Po/Pg. The optimal cases to be chosen are graphically shown in Figure 3 on the Po/Pg-Pe/Pg plane. When Eq. (30) is valid, Figure 3 (a) should be applied. The inlet air cooling is not an optimal option in any case. When Eq. (32) is valid, the cases E and E’ appear on the plane and the steam turbine is never chosen, as depicted in Figure 3 (b). It is noteworthy that if the inlet air cooling cannot improve the gas turbine efficiency, i.e. βGT = 0, the inlet air cooling is never the optimal solution.As the cases C, D and E include three operation modes, another criterion for the selection of the optimal operation mode is necessary in those cases. The additional criterion is related with the steam to electricity ratio, and can be derived from the consideration below.In the c ases C, D and E, λ1 and λ2 have positive values. Therefore, two of the constraints given as Eqs. (6) and (7) take the equality conditions due to the Kuhn-Tucker condition Eq. (12). Then, the two equations can be solved simultaneously for two variables which have positive values at each mode.For the mode d, the simultaneous equations can be solved under xGT, xB > 0 and xG, xST, xAC = 0.Then, one can obtain xGT = xe and xB = xs ¡ ρGTxe. Because xB has a positive value, the following condition has to be satisfied for the mode d to be selected.(33)At the mode e, one can obtain xG = xe ¡ xs/ρGT and xGT = xs/ρGT, and the following condition can be drawn out of the former expression because xG is greater than zero at this mode.(34)Similar considerations can be applied to the cases D and E. Consequently, Eq. (33) is the condition for the mode d to be selected, while Eq. (34) is the condition for the modes e, f or g to be selected. Furthermore, it is obvious that the mode c has to be chosen if the steam to electricity ratio of the gas turbine is equal to the ratio of the heat flow rate of the steam demand to the electric power demand, i.e. ρGT = xs/xe.Equations (33) and (34) mean that when the steam to electricity ratio of the gas turbine is smaller than the ratio of the heat flow rate of the steam demand to the electric power demand, the gas turbine should be operated to meet the electric power demand. Then, the boiler should balance the heat flow rate of the steam supply with the demand. On the other hand, if the steam to electricity ratio of the gas turbine is larger than the ratio of the heat flow rate of the steam demand to the electric power demand,the gas turbine has to be operated to meet the heat flow rate of the steam demand. Then, the insufficient electric power supply from the gas turbine has to be compensated by either the grid (mode e), the steam turbine (mode f), or the inlet air cooling (mode g). There is no need of any auxiliary equipment to supply additional electric power or steam if the steam to electricity ratio of the gas turbine matches the demands.Figure 3. The optimal operation cases expressed on the relative oil price-relative electricity price plane (the operational condition I).2.4. The Optimal Solution where the Electric Power from the Gas Turbine is at the MaximumIn the operational condition II, the third constraint, Eq. (8), takes the equality condition and λ3 would have a positive value. Then, Eqs. (11) and (18) yields:(35)It is reasonable to assume that ρGT ¡ !AC ®GT > 0 and ωGT ¡ ¯GT ®GT > 0 in the case ofgas turbine cogeneration systems because of relatively low electric efficiency (¼ 25 %) and a high heat to electricity ratio (ρGT > 1.4). Then, the optimal solution cases c an be defined by a similar consideration to the operational condition I, and the newly appeared cases are illustrated in Figure 4. The cases F and G can occur in the operational condition II in addition to the cases A and B of the operational condition I. Similarly to the cases C’ and D’ of the operational condition I, the cases F’ and G’ can be defined where the mode h is excluded from the cases F and G, respectively.Figure 4. The optimal solution cases on the operational condition II.In the operational condition II, the conditions of the cases A and B are slightly different from those in the operational condition I, as given below.Case A:(36)(37)Case B:(38)(39)The conditions for the cases F and G are obtained as follows.Case F:(40)(41)(42)Case G:In addition to Eq. (41),(43)(44)The case s F’ and G’ occur whenthe inequality sign of Eq. (41) is reversed. Equations (36), (38),(40), (41), (42), (43) and (44) correspond to Eqs. (19), (22), (24), (25), (26), (28) and (29), respectively.In these equations, ωGT ¡ ¯GT®GTis substituted for ωGT, an d ρGT ¡ !AC®GTis substituted for ρGT.The optimal cases of the operational condition II are illustrated on the Po/Pg-Pe/Pg plane as shown in Figure 5. Unlike the operational condition I, there is no lower limit of the relative oil price for the optimal solution to exist. The line separating the cases F and G is determined by the multiple parameters.Basically, a larger ρGT or a smaller ωST lowers the line, which causes a higher possibility for the case G to be selected.Figure 5. The optimal operation cases expressed on the relative oil price-relative electricity price plane (the operational condition II).To find the optimal mode out of three operation modes included in the cases F or G, another strategy is necessary. The additional conditions can be found by a similar examination on the variables to that done for the cases C, D and E. In the operational condition II, three variables can be analytically solved by the constraints given as Eqs. (6), (7) and (8) taking equality conditions.In the mode g, only two variables, ωGT andωAC are positive and the other variables are equal to zero.Therefore, the analytical solutions of those in the operational condition II can be obtained from equations derived from Eqs. (6) and (7) as xGT = xe and xAC = (ρGTxe ¡xs)/ωA C. Then the third constraint gives the equality condition concerning xs/xe and XGT/xe as follows:(45)where, XGT/xe represents the ratio of the gas turbine capacity to the electricity demand, and XGT/xe ·1.For mode h, the condition where this mode should be selected is derived from the analytical solution of xB with xB > 0 as follows:(46)For the mode i, xG > 0 and xAC > 0 give the following two conditions.(47)(48)For the mode j, xST > 0 and xAC > 0 give the following conditions.(49)(50)The conditions given as Eqs. (45–50) are graphically shown in Figure 6. In the cases F and G,the operational condition II cannot be applied to the region of xsxe< ρGTXGT xeand xsxe<(ωST+ρGT)XGTxe¡ωST,respectively, because xAC becomes negative in this region. The optimal operation should be found under the operational condition I in this region.3. Comparison of the Optimal Operation Criteria with a Detailed Optimization ResultTo examine the applicability of the method explained in the previous section to a practical cogeneration system, the combination of the suppliers selected by the optimal operation criteria was compared with the results of a detailed optimization of an existing plant.3.1. An Example of an Existing Energy Center of a FactoryAn energy center of an existing factory is depicted in Figure 7. The factory is located in Aichi Prefecture, Japan, and produces car-related parts. The energy center produces electricity by a combined cycle of a gas turbine and a steam turbine. The gas turbine can be fueled with either gas or kerosene, and it is equipped with an inlet air cooler. The electric power distribution system of the factory is also linked to the electricity grid so that the electricity can be purchased in case the electric power supply from the energy center is insufficient.The steam is produced from the gas turbine and boilers. The high, medium or low pressure steam is consumed in the manufacturing process as well as for the driving force of the steam turbine and absorption chillers. The absorption chillers supply chilled water for the process, air conditioning and the inlet air cooling. One of the absorption chiller can utilize hot water recovered from the low temperature waste gas of the gas turbine to enhance the heat recovery efficiency of the system.Figure 6. The selection of the optimal operation mode in the cases of F and G.3.2. The Performance Characteristics of the EquipmentThe part load characteristics of the equipment were linearly approximated so that the system could be modeled by the linear programming. The approximation lines were derived from the characteristics of the existing machines used in the energy center.The electricity and the steam generation characteristics of the gas turbine and the HRSG are shown in Figure 8, for example. The electric capacity of the gas turbine increases with lower inlet air temperatures. The quantity of generated steam is also augmented with lower inlet air temperatures.In practice, it is known that the inlet air cooling is beneficial when the purchase of the grid electricity will exceed the power contract without the augmentation of the gas turbine capacity. Furthermore, the inlet air cooling is effective when the outdoor air temperature is higher than 11 ◦C. A part of the operation of the actual gas turbine system is based on the above judgement of the operator, which is also included in the detailed optimization model.3.3. The Detailed Optimization of the Energy CenterThe optimization of the system shown in Figure 7 was performed by a software tool developed for this system. The optimization method used in the tool is the linear programming method combined with the listed start-stop patterns of equipment and with the judgement whether the inlet air cooling is on oroff. The methodology used in the tool is fully described in the reference [11].Figure 7. An energy center of a factory.Figure 8. The performance characteristics of the gas turbine and the HRSG.The Detailed Optimization MethodThe energy flow in the energy center was modeled by the linear programming. The outputs of equipment were the variables to be optimized, whose values could be varied within the lower and upper limits. To make the optimization model realistic, it is necessary to take the start-stop patterns of the equipment into account. The start-stop patterns were generated according to thepossible operation conditions of the actual energy center, and 20 patterns were chosen for the enumeration. The optimal solution was searched by the combination of the enumeration of the start-stop patterns and the linear programming method. The list of the start-stop patterns of the gas turbine and the steam turbine is given in Figure 9.The demands given in the detailed optimization are shown in Figure 10 as the ratios of the heat flow rate of the steam demand to the electric power demand on a summer day with a large electric power demand and on a winter day with a small steam demand. On the summer day, the ratio of the heat flow rate of the steam demand to the electric power demand is at a low level throughout a day. While, it is high on the winter day, and during the hours 2 to 6, the ratio exceeds 1.4 that is the steam to electricity ratio of the gas turbine.Figure 9. The start-stop patterns of the gas turbine and the steam turbine.The Plant Operation Obtained by the Detailed OptimizationThe accumulated graphs shown in Figures 11 through 14 illustrate the electric power supply and the heat flow rate of the steam supply from equipment on the summer and winter days. On the summer day, the gas turbine and the steam turbine worked at the maximum load and the electric power demand was met by the purchase from the grid for most of the day except the hours 2 to 6, at which the electric power demand was small. The inlet air cooling of the gas turbine was used only at the hours 10 and 14, at which the peak of the electric power demand existed. The steam was mainly supplied by the gas turbine, and the boiler was used only if the total heat flow rate of the steam demands by the process, the steam turbine, and the absorption。
回溯分支限界
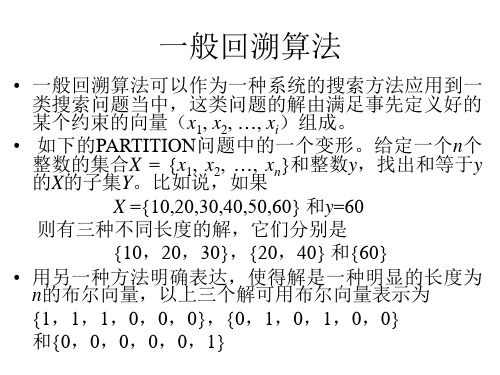
• Give each of the arcs an arc number, from 1 to m, as identification.
• The branching within each stage corresponds to the decision of either adding a certain arc to the solution arc set or not.
• introduce an arc-oriented branch and bound method, branching by using an arc or not.
A Binary Search Tree
• Define an m-stage binary search tree for problem with n nodes, where, for complete graph problem, m equals the number of pairs of nodes.
• Define traveling along left branch represents for choosing an arc and traveling along right branch represents for not choosing an arc.
指南中推荐意见的形成英文
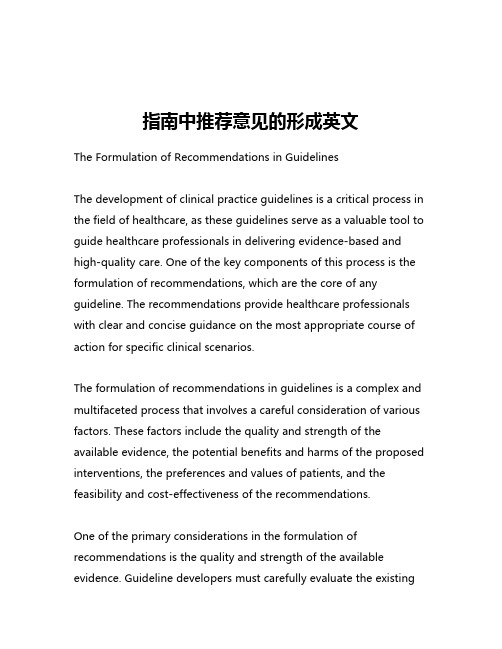
指南中推荐意见的形成英文The Formulation of Recommendations in GuidelinesThe development of clinical practice guidelines is a critical process in the field of healthcare, as these guidelines serve as a valuable tool to guide healthcare professionals in delivering evidence-based and high-quality care. One of the key components of this process is the formulation of recommendations, which are the core of any guideline. The recommendations provide healthcare professionals with clear and concise guidance on the most appropriate course of action for specific clinical scenarios.The formulation of recommendations in guidelines is a complex and multifaceted process that involves a careful consideration of various factors. These factors include the quality and strength of the available evidence, the potential benefits and harms of the proposed interventions, the preferences and values of patients, and the feasibility and cost-effectiveness of the recommendations.One of the primary considerations in the formulation of recommendations is the quality and strength of the available evidence. Guideline developers must carefully evaluate the existingresearch on the topic at hand, assessing the methodological rigor, study design, and overall quality of the evidence. This assessment is crucial in determining the confidence that can be placed in the recommendations.In cases where the available evidence is strong and of high quality, the recommendations can be made with a greater degree of confidence. However, in situations where the evidence is limited or of lower quality, guideline developers must exercise caution and consider the potential uncertainties and limitations of the available data. In such instances, the recommendations may be qualified or presented with a lower level of certainty.Another important factor in the formulation of recommendations is the consideration of the potential benefits and harms of the proposed interventions. Guideline developers must weigh the potential positive outcomes, such as improved patient outcomes, reduced morbidity, or decreased healthcare costs, against the potential negative consequences, such as adverse effects, increased resource utilization, or patient burden.This careful balancing act is essential in ensuring that the recommendations strike the right balance between promoting optimal patient care and minimizing potential harm. Guideline developers may also consider the preferences and values of patients,as these can play a significant role in the acceptability and uptake of the recommendations.The feasibility and cost-effectiveness of the recommendations are also crucial considerations in the formulation process. Guideline developers must assess whether the proposed interventions are practical to implement within the healthcare system, taking into account factors such as resource availability, infrastructure, and provider expertise. Additionally, the cost-effectiveness of the recommendations must be evaluated to ensure that they represent a wise use of healthcare resources.The process of formulating recommendations in guidelines is further complicated by the need to account for contextual factors, such as local healthcare systems, cultural norms, and resource constraints. Guideline developers must carefully consider how these factors may impact the applicability and implementation of the recommendations in different settings.To address this challenge, many guideline development organizations have adopted the Grading of Recommendations Assessment, Development, and Evaluation (GRADE) approach. The GRADE system provides a structured framework for evaluating the quality of evidence and the strength of recommendations, taking into account factors such as the risk of bias, the consistency ofresults, the directness of the evidence, and the precision of the estimates.By using the GRADE approach, guideline developers can provide healthcare professionals with clear and transparent information about the level of confidence they can have in the recommendations, as well as the rationale behind the formulation of those recommendations.In conclusion, the formulation of recommendations in clinical practice guidelines is a complex and multifaceted process that requires careful consideration of a range of factors. Guideline developers must balance the quality and strength of the available evidence, the potential benefits and harms of the proposed interventions, the preferences and values of patients, and the feasibility and cost-effectiveness of the recommendations. By adopting a structured and transparent approach, such as the GRADE system, guideline developers can ensure that the recommendations they provide are evidence-based, patient-centered, and ultimately effective in improving the quality of healthcare delivery.。
铁路网络阻塞问题优化建模——流量路由问题说明书

5th International Conference on Civil Engineering and Transportation (ICCET 2015)Railway Network Blocking Problem: An Optimization ModelingFormulation about Flow Routing ProblemHongpeng Ma1, a, Yixiang Yue2, b* and Congli Hao3, c1 School of Traffic and Transportation, Beijing Jiaotong University, Beijing 100044, China2 School of Traffic and Transportation, Beijing Jiaotong University, Beijing 100044, China3 School of Traffic and Transportation, Beijing Jiaotong University, Beijing 100044, Chinaa*****************.cn,b********************,c*****************.cn Keywords: Railroad; Road Network; Blocking Problem; OptimizationAbstract:In this paper, we mainly study on modeling formulation for railway network blocking problem. We propose model formulation for RBP. The objective function of RBP model is to minimize the costs of flow traveling and delay for the train in marshalling station, by deciding which block is built and specifying the assignment of commodities to these blocks, while observing limits on the reclassification capacity at each terminal. The model is solved using GAMS. The model is tested on a real-world railway network located in North of China, the computation results show that the model have the potential to apply and can yield the dramatic railroad’s operating costs saving. IntroductionThe Railway Blocking Problem (RBP) determines how to aggregate a large number of shipments into blocks of shipments as they travel from origins to destinations [1]. In other words, RBP determines which blocks should be built at each yard and what shipments should be placed in the block.Mathematically, RBP is a multi-commodity-flow, network-design, and routing problem. To solve RBP, we need to design the underlying blocking network and to route different commodities on the blocking network to minimize the transportation costs [2]. In RBP, each train will be assigned to a direct block, whose OD is the same as that of the shipment, to avoid unnecessary marshalling and delays. So there are some directed arcs between two terminals that are not necessarily connected by a physical link. However, blocking capacity at each yard, determined by available yard resources (hump yard equipment and shunting yard equipment), limits the maximum number of blocks and maximum car volume that each yard can handle, preventing railroads from assigning direct blocks for all trains. So aiming at delivering the flow with the fewest possible classifications, railroads develop RBP determining which blocks should be built at each yard and what shipments should be placed in each block [3, 4, 5, 6].RBP is one of the most important decision in freight railroads. A good solution of RBP can save railway operation costs of delivering all commodities. And these costs are usually broken down into car-handling costs associated with handling (or blocking) a car and car-miles costs associated with the movement of a car.There are some study about blocking problem of single railway line, Xu [7] proposed a 0-1 programming with the target of minimum balanced using of adaptation capacity and hour of freight train in marshalling station. And Yang [8] proposed 0-1 linear programming model and 0-1 quadratic program model.Compared with single railway line, the reality railroad network is much complicated. For example, number of transport plan about blocking problem in single line railroad is 1048576, but in railway network, the number may be million, even billion. So for complicated railroad network blocking problem, there are very few study in the field. Li [9] proposed Chance Constrained Programming with considering flow, assembly time and volatility of vehicle adaptation extra time consuming. Newton [10] and Newton et al. [11] modeled the blocking problem as a network-design model and formulated it as a MIP. Bodin [12] established Nonlinear Mixed Integer Programming model with the target ofminimum total cost of adaptation and transport, and proposed heuristic decomposition method. Yaghini [13] and Yue [14] proposed Ant Colony Optimization Algorithm to solve the railroad blocking problem.Their approaches focus on determining a near-optimal solution. However, many models only solve blocking problem of single railway network, such as 0-1 linear programming model. And the approaches for RBP can’t guarantee to get optimal solution in any case without considering factors, such as, empty car, flow pathway and service level. So it is very necessary to develop optimization modeling formulation of RBP. Model and Solution MethodNotation. i, j, n, k, q is macroscopic node index. And i, j, n correspond to physical marshalling station in a railway network. k is origin station of commodity and q is destination station of commodity in a railway network. ,i j c is flow transport cost between each pair OD(from station i to station j ), is proportional to mileage between the pair OD. ,i j c can be calculated by any empirical formula according to statistical curve fitting. Conversion coefficient that changing car-hour delay to cost is p, and the value of p is 80 in this study. ,i j m is accumulation delay when the directed block to station j is built in station i . The empirical formulation is shown as equation (1).,,i j i i j m s α=⨯ (1) Where i αis accumulation parameter of marshalling station i , it is a constant derived from statistical analysis by many years record data. ,i j s is number of cars for one train from station i to station j . i t is the save time of car passing station i without reclassification. ,i j u is re-classification capacity from station i to direction j . ,k q d is demand from station k to station q . M is a large number. ,i j b is directed-block index as equation (2).,there is directed block from station to statio 1,0otherwisen i j i j b i j ⎧=∀⎨⎩ (2)There are two decision variables: ,,,i j k q x and ,i j y . ,,,i j k q x is volume from station k to station q shipped using train from station i to station j . ,i j y is 0-1 binary variables as equation (3). ,there is train from station to station 1,0otherwisei j i j j y i ⎧=∀⎨⎩ (3)Formulation of RBP Model. The objective function of RBP model is to minimize the costs of flow traveling and delay for the train in marshalling stations, by deciding which block is built and specifying the assignment of commodities to these blocks, while observing limits on reclassification capacity at each terminal.The formulation of RBP is as follow.,,,,,,,,,,,,,,,,[()]i j k q i j i j i j i j j i j k q k j i j k qi jji k qkz min x c p m y b t x d =⨯+⨯⨯⨯+⨯-∑∑∑∑∑∑ (4)Subject to,,,,,,,i j k q k j j ki qx d u j k -≤∀∑ (5),,,,,,i j k qk qjxd i k q i k =∀=∑, (6),,,,,,,i j k qk qixd j k q j q =∀=∑ (7),,,,,,0,,,,i j k qn i k q jnxx i k q i q i k -=∀≠≠∑∑ (8),,,,,,,i j k q i jx My i j k q ≤∀ (9),,,0,,,i j k q x i j k q ≥∀ (10)The objective function of RBP model is to minimize the total cost consists of flow transport cost in railroad and delay in marshalling station. Constraint (5) is the hard constraint of reclassification capacity of the number of blocking cars satisfied reclassification capacity in every marshalling station. Constraint (6)、(7) and (8) is flow balance constraint. Constraint (9) ensures that if there is no train from station i to station j , volume from station k to station q shipped by train from station i to station j must be zero, which means if ,=0i j y , there is that ,,,=0i j k q x . Real World Case StudyRailway Network. Based on eight marshalling stations, the railroad network of North China is constructed to calculate as in Fig. 1. All intermediate stations isn’t shown in Fig. 1.Fig. 1.Case of railroad networkThe real world data collected is shown in Table 1, Table 2, Table 3 and Table 4. Station 2 is the center of railway network with five convergence directions, so accumulation delay of station 2 is detailed in Table 2.arrival leave 1 2 3 4 5 6 7 81 0 84 389 832 324 292 693 609 2 84 0 355 798 290 258 659 575 3 389 355 0 1103 465 563 964 874 4 832 798 1103 0 1038 1006 1407 1323 5 324 290 465 1038 0 430 831 536 6 292 258 563 1006 430 0 451 367 7 704 659 964 1537 831 451 0 402 8 609 575 874 1323 536 367 402 0Table 1. Flow transport cost between each pair OD [$/car] departure 1 3 4 5 6 7 8Number of cars of one train of station B[car] 49.3 49.3 52.6 42 41.8 50.5 52.3 Accumulation Parameter of station B[h/car] 9.1 10.1 8.2 9 9.3 10.6 4.1Accumulation delay of station B[h] 449 498 431 378 389 535 215Table 2. Accumulation delay of station 2 station 1 3 4 5 6 7 8Accumulation delay of other stations[h] 550 550 636 600 500 530 530Table 3. Accumulation delay of other marshalling stations station 1 2 3 4 5 6 7 8 t i [h/car] 3 2.9 4 4.7 3 3 4 4reclassificationcapacity[car]Station 2 321 - 651 1110 39 1125 1125 1125Station 6 1125 1125 1125 1125 88 - 700 1100Station 5 500 500 500 500 - 500 500 30 Model Testing and Result Analysis. We use General Algebraic Modeling System (GAMS) [15] to solve MIP model of RBP. And calculation time of RBP solved by GAMS are 30 seconds.To verify feasibility of model of RBP, we solve respectively the RBP with real flow data in 2013 and 2014. And the traffic demand between of each pair OD in 2013 and 2014 is shown in Table 5 and Table 6.Arrival leave 1 2 3 4 5 6 7 81 0 190 70 125 110 10 20 252 150 0 245 20 24 300 153 385 3 272 442 0 160 17 140 130 294 85 405 150 0 9 135 50 185 5 59 140 4 3 0 4 3 06 15 35 230 120 40 0 50 327 11 221 282 138 26 31 0 0 8 40 30 50 490 4 57 0 0 Table 5. Traffic demand between each pair OD in 2013 [car]Arrival leave 1 2 3 4 5 6 7 81 0 190 67 125 106 13 9 342 161 0 231 22 24 315 153 396 3 72 442 0 161 17 131 160 294 89 401 293 0 9 132 49 185 5 59 135 4 3 0 4 3 06 15 36 231 360 40 0 50 327 11 21 282 134 26 30 0 08 38 30 50 505 4 59 0 0 Table 6. Traffic demand between each pair OD in 2014 [car]We use GAMS to solve model of RBP. And the solution is shown in Table 7, Table 8, Table 9 and Table 10.i j k q ,,,i j k q x i j k q ,,,i j k q x i j k q ,,,i j k q x i j k q ,,,i j k q x1 2 1 2 150 2 4 2 4 405 3 2 3 4 150 6 2 6 1 13 1 2 1 4 85 2 4 3 4 150 3 2 3 5 4 6 2 6 2 300 1 2 1 5 59 2 4 5 4 9 3 2 3 8 50 6 2 6 3 140 1 2 1 6 15 2 4 6 4 135 3 6 3 6 230 6 2 6 4 135 1 2 1 7 11 2 4 7 4 50 3 7 3 7 282 6 5 6 5 4 1 2 1 8 40 2 5 1 5 59 4 2 4 1 125 6 5 7 5 3 1 3 1 3 272 2 5 2 5 140 4 2 4 2 20 6 7 5 7 26 2 1 2 1 190 2 5 3 5 4 4 2 4 3 160 6 7 6 7 31 2 1 3 1 70 2 5 4 5 3 4 2 4 5 3 6 8 6 8 57 2 1 4 1 125 2 6 1 6 15 4 6 4 6 120 7 2 7 1 20 2 1 5 1 14 2 6 2 6 35 4 6 4 7 134 7 2 7 2 153 2 1 6 1 13 2 6 4 6 120 4 8 4 8 490 7 2 7 3 130 2 1 7 1 20 2 7 1 7 11 5 1 5 1 96 7 2 7 4 50 21 8 12527 2 722152 5 11476 7 53i j k q ,,,i j k q x i j k q ,,,i j k q x i j k q ,,,i j k q x i j k q ,,,i j k q x 2 3 2 3 442 2 7 4 7 134 5 2 5 2 24 7 6 7 6 50 2 3 4 3 160 2 8 1 8 40 5 2 5 3 17 8 2 8 1 25 2 3 5 3 17 2 8 2 8 30 5 2 5 4 9 8 2 8 2 385 2 3 6 3 140 2 8 3 8 50 5 6 5 6 40 8 2 8 3 29 2 3 7 3 130 3 2 3 1 70 5 6 5 7 26 8 4 8 4 185 2 3 8 3 29 3 2 3 2 245 5 8 5 8 4 8 6 8 6 32 24 1 4 85 - - - - - - - - - - - - - - - Table 7. The Solution of RBP for variable ,,,i j k q x with real flow OD in 2013i j 1 2 3 4 5 6 7 81 0 1 1 0 0 0 0 02 1 0 1 1 1 1 1 13 0 1 0 0 0 1 1 04 0 1 0 0 0 0 0 15 1 1 0 0 0 1 0 16 0 1 0 0 1 0 1 17 0 1 0 0 0 1 0 08 0 1 0 1 0 1 0 0 Table 8. The solution of RBP for variable ,i j y with real flow OD in 2013The objective function value is:2013$5107135z = (11)i j k q ,,,i j k q x i j k q ,,,i j k q x i j k q ,,,i j k q x i j k q ,,,i j k q x 1 2 1 2 161 2 4 2 4 401 3 6 3 6 231 6 2 6 4 132 1 2 1 3 72 2 4 5 4 9 3 7 3 7 282 6 5 6 5 4 1 2 1 4 89 2 4 6 4 132 4 2 4 1 125 6 5 7 5 3 1 2 1 5 59 2 4 7 4 49 4 2 4 2 22 6 7 1 7 11 1 2 1 6 15 2 5 1 5 59 4 2 4 3 161 6 7 2 7 21 1 2 1 7 11 2 5 2 5 135 4 2 4 5 3 6 7 4 7 134 1 2 1 8 38 2 5 3 5 4 4 6 4 6 360 6 7 5 7 26 2 1 2 1 190 2 5 4 5 3 4 6 4 7 134 6 7 6 7 30 2 1 3 1 67 2 6 1 6 15 4 8 4 8 505 6 8 6 8 59 2 1 4 1 125 2 6 1 7 11 5 1 5 1 93 7 2 7 1 9 2 1 5 1 13 2 6 2 6 36 5 2 5 1 13 7 2 7 2 153 2 1 6 1 13 2 6 2 7 21 5 2 5 2 24 7 2 7 4 49 2 1 7 1 9 2 8 1 8 38 5 2 5 3 17 7 3 7 3 160 2 1 8 1 34 2 8 2 8 30 5 2 5 4 9 7 6 7 5 3 2 3 1 3 72 2 8 3 8 50 5 6 5 6 40 7 6 7 6 50 2 3 2 3 442 3 2 3 1 67 5 6 5 7 26 8 2 8 1 34 2 3 4 3 161 3 2 3 2 231 5 8 5 8 4 8 2 8 2 396 2 3 5 3 17 3 2 3 5 4 6 2 6 1 13 8 2 8 3 29 2 3 6 3 131 3 2 3 8 50 6 2 6 2 315 8 4 8 4 185 2 3 8 3 29 3 4 3 4 293 6 2 6 3 131 8 6 8 6 32 2 41 4 89 - - - - - - - - - - - - - - -Table 9.The solution of RBP for variable ,,,i j k q x with real flow OD in 2014 i j 1 2 3 4 5 6 7 81 0 1 0 0 0 0 0 02 1 0 1 1 1 1 0 1 i j 1 234567 83 0 1 0 1 0 1 1 04 0 1 0 0 0 1 0 15 1 1 0 0 0 1 0 16 0 1 0 0 1 0 1 17 0 1 1 0 0 1 0 08 0 1 0 1 0 1 0 0 Table 10. The solution of RBP for variable ,i j y with real flow OD in 2014The objective function value is:2014$5496920z = (12)To verify intuitively feasibility of model, we compare the solution of RBP in 2013 with the solution of RBP in 2014 in Fig. 2. And there are only directed train in Fig. 2.There is a directed train between two stationsPhysical Railway NetworkSolution of RBP in 2014Solution of RBP in 2013StationFig. 2. The solution comparisonComparing the solution of RBP in 2013 and 2014, the feasibility of the model can be verified from the following aspects:1. Some directed trains are canceled.If there are the loss of car-hours and cost, the directed train will be canceled. For example, there are 221 cars per day from station 2 to station 7 in 2013. But there are only 21 cars per day. Because of fewer flow, the directed train will cause the loss of car-hours and cost. So the directed train from station 2 to station 7 is canceled. 2. Some directed trains are built.If the directed block can save car-hours and cost, the directed trains will be built. For example, there are 150 cars per day from station 3 to station 4 in 2013. But there are only 293 cars per day. Because of more flow, the directed train will cause the save of car-hours and cost. So the directed train from 3 to 4 is built.The objective function of RBP model is to minimize the costs of flow traveling and delay for the train in marshalling station. So we need compare the solution with the now using RBP solution in 2014 to verify optimization of model.The flow of transit car with resorting and transit car without resorting of station 2 in 2014 is shown in Table 11 and Table 12.Arrival leave 1 3 4 5 6 7 81 0 32 72 7 9 6 23 3 47 0 61 13 36 43 84 28 79 05 46 17 66 5 5 1 2 0 1 0 0 6 1 19 135 2 0 0 2 7 7 31 52 2 1 0 08 6 1 215 2 1 0 0 Table 11. The flow of transit car with resorting in 2014 [car]Arrival leave 1 2 3 4 5 6 7 81 0 190 35 53 99 4 3 112 161 0 231 22 24 315 153 396 3 25 442 0 1004 95 117 21 4 61 401 214 0 4 86 32 119 5 54 135 3 1 0 3 3 0 6 14 36 212 225 38 0 50 307 4 21 251 82 24 29 0 08 32 30 49 290 2 58 0 0 Table 12. The flow of transit car without resorting in 2014 [car]And the objective function value of real-world in the condition of the same parameters isz (13) $6527430actualCompared with the now using RBP solution in 2014, the optimization of the model can be verified from the following aspects:1. Some directed trains are canceled.The new solution deletes 25 directed trains, saves total 9005 car-hours per day. For example, there are 13 cars per day from station 6 to station 1. Because of a directed block for the flow, there are 500 car-hours about car detention time under accumulation and 38 car-hours of the save time because of transit car without reclassification per day. The directed train from station 6 to station 1 causes the loss of 400 car-hours per day.2. Volume shipped by directed trains are added.The new solution adds volume shipped by directed trains, saves total 3538 car-hours per day.3. Cost Saving.The objective function value of formulation of RBP model is 5496920. And the objective function value of real-world in the condition of the same parameters is 6527430. Total cost saving is 1030510.4. Traffic flow adjustments.The solution considers the balance of railway line. And some flows in busy railway line are adjusted to other rail lines to improve whole network efficiency. Such as, in existing RBP solution in 2014, the flow from station 5 to station 6 pass station 2 with reclassification operation. But in solution of RBP model, a directed block between station 5 and station 6 is built to make full use of railway between station 5 and station 6.ConclusionsThis paper mainly focuses on Railway Blocking Problem in a network. We consider both transport cost and delay on marshaling station; and use GAMS to solve it. We give a case of 8 marshaling stations to test the model on the real world data. In the case, solution by our method can decrease 55% of car-hours and 16% of cost per day. In the meantime, we can optimize traffic flow to improve efficiency of the whole network. It is sure that our proposed models are effective, efficient and potential for application in a real world railway network.References[1] M Yaghini, et.al. Solving railroad blocking problem using ant colony optimization algorithm [J]. Applied Mathematical Modelling, 35(2011) 5579-5591.[2] R.K. Ahuja, et.al. Solving Real-Life Railroad Blocking Problems [J]. Interfaces, 37(2007) 404-419.[3] M Yaghinia and R Akhavan. Multicommodity Network Design Problem in Rail Freight Transportation Planning [J]. Procedia - Social and Behavioral Sciences, 43(2012) 728-739.[4] C Barnhart, et.al. Railroad Blocking: A Network Design Application [J]. Operations Research, 48(2000) 603-614.[5] Ahuja, et.al. Network Models in Railroad planning and scheduling [J]. Operation Research, 1(2005) 54-101.[6] A Balakrishnan, et.al. A Dual-ascent Procedure for Large-scale Uncapacitated Network Design [J]. Operations Research, 73(1989) 716-740.[7] H.Xu, et al. Study on the Model and Algorithm of the Formation Plan of Single Group Trains at Technical Service Stations (In Chinese) [J]. Journal of the China Railway Society, 28(2006) 12-17.[8] S.Yang, et al. An Artificial Neural Network Method for Marshalling Plan (In Chinese) [J]. Journal of Changsha Railway University, 20(2002) 79-84.[9] X.Li. Study on Optimization of Marshalling Plan and Flow Path Based on Uncertain Parameters (In Chinese) [D]. Southwest Jiaotong University, 2002.[10] H.N. Newton. Network Design under Budget Constraints with Application to the Railroad Blocking Problem [D]. Auburn University, 1996.[11] H.N. Newton, et.al. Constructing Railroad Blocking Plans to Minimize Handling Costs [J]. Transportation Science, 32(1998) 330-345.[12] L.Bodin, et.al. A Model for the Blocking of Trains [J]. Transportation Research Part B Methodological, 14(1980) 115-120.[13] M.Yaghini, et.al. Solving Railroad Blocking Problem Using Ant Colony Optimization Algorithm [J]. Applied Mathematical Modelling, 35(2011) 5579-5591.[14] Y.Yue, et.al. Multi-route Railroad Blocking Problem by Improved Model and Ant Colony Algorithm Real World [J]. Computers & Industrial Engineering, 60(2011) 34-42.[15] A.Brooke, et.al. GAMS Language Guide. 2006.。
Secrets of Optical Flow Estimation and Their Principles
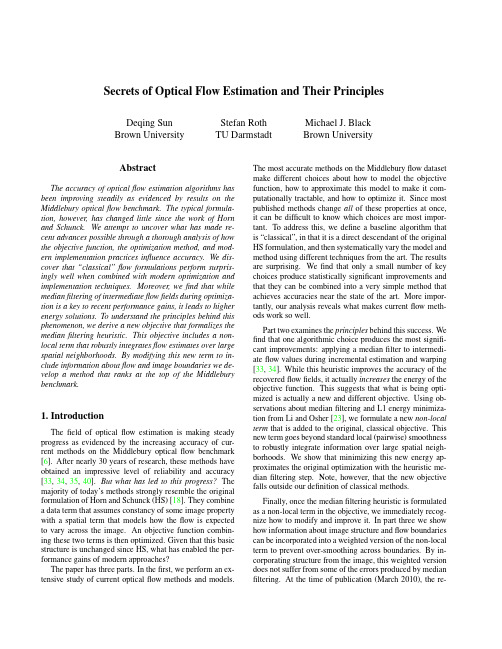
Secrets of Optical Flow Estimation and Their PrinciplesDeqing Sun Brown UniversityStefan RothTU DarmstadtMichael J.BlackBrown UniversityAbstractThe accuracy of opticalflow estimation algorithms has been improving steadily as evidenced by results on the Middlebury opticalflow benchmark.The typical formula-tion,however,has changed little since the work of Horn and Schunck.We attempt to uncover what has made re-cent advances possible through a thorough analysis of how the objective function,the optimization method,and mod-ern implementation practices influence accuracy.We dis-cover that“classical”flow formulations perform surpris-ingly well when combined with modern optimization and implementation techniques.Moreover,wefind that while medianfiltering of intermediateflowfields during optimiza-tion is a key to recent performance gains,it leads to higher energy solutions.To understand the principles behind this phenomenon,we derive a new objective that formalizes the medianfiltering heuristic.This objective includes a non-local term that robustly integratesflow estimates over large spatial neighborhoods.By modifying this new term to in-clude information aboutflow and image boundaries we de-velop a method that ranks at the top of the Middlebury benchmark.1.IntroductionThefield of opticalflow estimation is making steady progress as evidenced by the increasing accuracy of cur-rent methods on the Middlebury opticalflow benchmark [6].After nearly30years of research,these methods have obtained an impressive level of reliability and accuracy [33,34,35,40].But what has led to this progress?The majority of today’s methods strongly resemble the original formulation of Horn and Schunck(HS)[18].They combine a data term that assumes constancy of some image property with a spatial term that models how theflow is expected to vary across the image.An objective function combin-ing these two terms is then optimized.Given that this basic structure is unchanged since HS,what has enabled the per-formance gains of modern approaches?The paper has three parts.In thefirst,we perform an ex-tensive study of current opticalflow methods and models.The most accurate methods on the Middleburyflow dataset make different choices about how to model the objective function,how to approximate this model to make it com-putationally tractable,and how to optimize it.Since most published methods change all of these properties at once, it can be difficult to know which choices are most impor-tant.To address this,we define a baseline algorithm that is“classical”,in that it is a direct descendant of the original HS formulation,and then systematically vary the model and method using different techniques from the art.The results are surprising.Wefind that only a small number of key choices produce statistically significant improvements and that they can be combined into a very simple method that achieves accuracies near the state of the art.More impor-tantly,our analysis reveals what makes currentflow meth-ods work so well.Part two examines the principles behind this success.We find that one algorithmic choice produces the most signifi-cant improvements:applying a medianfilter to intermedi-ateflow values during incremental estimation and warping [33,34].While this heuristic improves the accuracy of the recoveredflowfields,it actually increases the energy of the objective function.This suggests that what is being opti-mized is actually a new and different ing ob-servations about medianfiltering and L1energy minimiza-tion from Li and Osher[23],we formulate a new non-local term that is added to the original,classical objective.This new term goes beyond standard local(pairwise)smoothness to robustly integrate information over large spatial neigh-borhoods.We show that minimizing this new energy ap-proximates the original optimization with the heuristic me-dianfiltering step.Note,however,that the new objective falls outside our definition of classical methods.Finally,once the medianfiltering heuristic is formulated as a non-local term in the objective,we immediately recog-nize how to modify and improve it.In part three we show how information about image structure andflow boundaries can be incorporated into a weighted version of the non-local term to prevent over-smoothing across boundaries.By in-corporating structure from the image,this weighted version does not suffer from some of the errors produced by median filtering.At the time of publication(March2010),the re-sulting approach is ranked1st in both angular and end-point errors in the Middlebury evaluation.In summary,the contributions of this paper are to(1)an-alyze currentflow models and methods to understand which design choices matter;(2)formulate and compare several classical objectives descended from HS using modern meth-ods;(3)formalize one of the key heuristics and derive a new objective function that includes a non-local term;(4)mod-ify this new objective to produce a state-of-the-art method. In doing this,we provide a“recipe”for others studying op-ticalflow that can guide their design choices.Finally,to en-able comparison and further innovation,we provide a public M ATLAB implementation[1].2.Previous WorkIt is important to separately analyze the contributions of the objective function that defines the problem(the model) and the optimization algorithm and implementation used to minimize it(the method).The HS formulation,for example, has long been thought to be highly inaccurate.Barron et al.[7]reported an average angular error(AAE)of~30degrees on the“Yosemite”sequence.This confounds the objective function with the particular optimization method proposed by Horn and Schunck1.When optimized with today’s meth-ods,the HS objective achieves surprisingly competitive re-sults despite the expected over-smoothing and sensitivity to outliers.Models:The global formulation of opticalflow intro-duced by Horn and Schunck[18]relies on both brightness constancy and spatial smoothness assumptions,but suffers from the fact that the quadratic formulation is not robust to outliers.Black and Anandan[10]addressed this by re-placing the quadratic error function with a robust formula-tion.Subsequently,many different robust functions have been explored[12,22,31]and it remains unclear which is best.We refer to all these spatially-discrete formulations derived from HS as“classical.”We systematically explore variations in the formulation and optimization of these ap-proaches.The surprise is that the classical model,appropri-ately implemented,remains very competitive.There are many formulations beyond the classical ones that we do not consider here.Significant ones use oriented smoothness[25,31,33,40],rigidity constraints[32,33], or image segmentation[9,21,41,37].While they deserve similar careful consideration,we expect many of our con-clusions to carry forward.Note that one can select among a set of models for a given sequence[4],instead offinding a “best”model for all the sequences.Methods:Many of the implementation details that are thought to be important date back to the early days of op-1They noted that the correct way to optimize their objective is by solv-ing a system of linear equations as is common today.This was impractical on the computers of the day so they used a heuristic method.ticalflow.Current best practices include coarse-to-fine es-timation to deal with large motions[8,13],texture decom-position[32,34]or high-orderfilter constancy[3,12,16, 22,40]to reduce the influence of lighting changes,bicubic interpolation-based warping[22,34],temporal averaging of image derivatives[17,34],graduated non-convexity[11]to minimize non-convex energies[10,31],and medianfilter-ing after each incremental estimation step to remove outliers [34].This medianfiltering heuristic is of particular interest as it makes non-robust methods more robust and improves the accuracy of all methods we tested.The effect on the objec-tive function and the underlying reason for its success have not previously been analyzed.Least median squares estima-tion can be used to robustly reject outliers inflow estimation [5],but previous work has focused on the data term.Related to medianfiltering,and our new non-local term, is the use of bilateralfiltering to prevent smoothing across motion boundaries[36].The approach separates a varia-tional method into twofiltering update stages,and replaces the original anisotropic diffusion process with multi-cue driven bilateralfiltering.As with medianfiltering,the bi-lateralfiltering step changes the original energy function.Models that are formulated with an L1robust penalty are often coupled with specialized total variation(TV)op-timization methods[39].Here we focus on generic opti-mization methods that can apply to any model andfind they perform as well as reported results for specialized methods.Despite recent algorithmic advances,there is a lack of publicly available,easy to use,and accurateflow estimation software.The GPU4Vision project[2]has made a substan-tial effort to change this and provides executablefiles for several accurate methods[32,33,34,35].The dependence on the GPU and the lack of source code are limitations.We hope that our public M ATLAB code will not only help in un-derstanding the“secrets”of opticalflow,but also let others exploit opticalflow as a useful tool in computer vision and relatedfields.3.Classical ModelsWe write the“classical”opticalflow objective function in its spatially discrete form asE(u,v)=∑i,j{ρD(I1(i,j)−I2(i+u i,j,j+v i,j))(1)+λ[ρS(u i,j−u i+1,j)+ρS(u i,j−u i,j+1)+ρS(v i,j−v i+1,j)+ρS(v i,j−v i,j+1)]}, where u and v are the horizontal and vertical components of the opticalflowfield to be estimated from images I1and I2,λis a regularization parameter,andρD andρS are the data and spatial penalty functions.We consider three different penalty functions:(1)the quadratic HS penaltyρ(x)=x2;(2)the Charbonnier penaltyρ(x)=√x2+ 2[13],a dif-ferentiable variant of the L1norm,the most robust convexfunction;and(3)the Lorentzianρ(x)=log(1+x22σ2),whichis a non-convex robust penalty used in[10].Note that this classical model is related to a standard pairwise Markov randomfield(MRF)based on a4-neighborhood.In the remainder of this section we define a baseline method using several techniques from the literature.This is not the“best”method,but includes modern techniques and will be used for comparison.We only briefly describe the main choices,which are explored in more detail in the following section and the cited references,especially[30].Quantitative results are presented throughout the remain-der of the text.In all cases we report the average end-point error(EPE)on the Middlebury training and test sets,de-pending on the experiment.Given the extensive nature of the evaluation,only average results are presented in the main body,while the details for each individual sequence are given in[30].3.1.Baseline methodsTo gain robustness against lighting changes,we follow [34]and apply the Rudin-Osher-Fatemi(ROF)structure texture decomposition method[28]to pre-process the in-put sequences and linearly combine the texture and struc-ture components(in the proportion20:1).The parameters are set according to[34].Optimization is performed using a standard incremental multi-resolution technique(e.g.[10,13])to estimateflow fields with large displacements.The opticalflow estimated at a coarse level is used to warp the second image toward thefirst at the nextfiner level,and aflow increment is cal-culated between thefirst image and the warped second im-age.The standard deviation of the Gaussian anti-aliasingfilter is set to be1√2d ,where d denotes the downsamplingfactor.Each level is recursively downsampled from its near-est lower level.In building the pyramid,the downsampling factor is not critical as pointed out in the next section and here we use the settings in[31],which uses a factor of0.8 in thefinal stages of the optimization.We adaptively de-termine the number of pyramid levels so that the top level has a width or height of around20to30pixels.At each pyramid level,we perform10warping steps to compute the flow increment.At each warping step,we linearize the data term,whichinvolves computing terms of the type∂∂x I2(i+u k i,j,j+v k i,j),where∂/∂x denotes the partial derivative in the horizon-tal direction,u k and v k denote the currentflow estimate at iteration k.As suggested in[34],we compute the deriva-tives of the second image using the5-point derivativefilter1 12[−180−81],and warp the second image and its deriva-tives toward thefirst using the currentflow estimate by bicu-bic interpolation.We then compute the spatial derivatives ofAvg.Rank Avg.EPEClassic-C14.90.408HS24.60.501Classic-L19.80.530HS[31]35.10.872BA(Classic-L)[31]30.90.746Adaptive[33]11.50.401Complementary OF[40]10.10.485Table1.Models.Average rank and end-point error(EPE)on the Middlebury test set using different penalty functions.Two current methods are included for comparison.thefirst image,average with the warped derivatives of the second image(c.f.[17]),and use this in place of∂I2∂x.For pixels moving out of the image boundaries,we set both their corresponding temporal and spatial derivatives to zero.Af-ter each warping step,we apply a5×5medianfilter to the newly computedflowfield to remove outliers[34].For the Charbonnier(Classic-C)and Lorentzian (Classic-L)penalty function,we use a graduated non-convexity(GNC)scheme[11]as described in[31]that lin-early combines a quadratic objective with a robust objective in varying proportions,from fully quadratic to fully robust. Unlike[31],a single regularization weightλis used for both the quadratic and the robust objective functions.3.2.Baseline resultsThe regularization parameterλis selected among a set of candidate values to achieve the best average end-point error (EPE)on the Middlebury training set.For the Charbonnier penalty function,the candidate set is[1,3,5,8,10]and 5is optimal.The Charbonnier penalty uses =0.001for both the data and the spatial term in Eq.(1).The Lorentzian usesσ=1.5for the data term,andσ=0.03for the spa-tial term.These parameters arefixed throughout the exper-iments,except where mentioned.Table1summarizes the EPE results of the basic model with three different penalty functions on the Middlebury test set,along with the two top performers at the time of publication(considering only published papers).The clas-sic formulations with two non-quadratic penalty functions (Classic-C)and(Classic-L)achieve competitive results de-spite their simplicity.The baseline optimization of HS and BA(Classic-L)results in significantly better accuracy than previously reported for these models[31].Note that the analysis also holds for the training set(Table2).At the time of publication,Classic-C ranks13th in av-erage EPE and15th in AAE in the Middlebury benchmark despite its simplicity,and it serves as the baseline below.It is worth noting that the spatially discrete MRF formulation taken here is competitive with variational methods such as [33].Moreover,our baseline implementation of HS has a lower average EPE than many more sophisticated methods.Avg.EPE significance p-value Classic-C0.298——HS0.38410.0078Classic-L0.31910.0078Classic-C-brightness0.28800.9453HS-brightness0.38710.0078Classic-L-brightness0.32500.2969Gradient0.30500.4609Table2.Pre-Processing.Average end-point error(EPE)on the Middlebury training set for the baseline method(Classic-C)using different pre-processing techniques.Significance is always with respect to Classic-C.4.Secrets ExploredWe evaluate a range of variations from the baseline ap-proach that have appeared in the literature,in order to illu-minate which may be of importance.This analysis is per-formed on the Middlebury training set by changing only one property at a time.Statistical significance is determined using a Wilcoxon signed rank test between each modified method and the baseline Classic-C;a p value less than0.05 indicates a significant difference.Pre-Processing.For each method,we optimize the regu-larization parameterλfor the training sequences and report the results in Table2.The baseline uses a non-linear pre-filtering of the images to reduce the influence of illumina-tion changes[34].Table2shows the effect of removing this and using a standard brightness constancy model(*-brightness).Classic-C-brightness actually achieves lower EPE on the training set than Classic-C but significantly lower accuracy on the test set:Classic-C-brightness= 0.726,HS-brightness=0.759,and Classic-L-brightness =0.603–see Table1for comparison.This disparity sug-gests overfitting is more severe for the brightness constancy assumption.Gradient only imposes constancy of the gra-dient vector at each pixel as proposed in[12](i.e.it robustly penalizes Euclidean distance between image gradients)and has similar performance in both training and test sets(c.f. Table8).See[30]for results of more alternatives. Secrets:Some form of imagefiltering is useful but simple derivative constancy is nearly as good as the more sophisti-cated texture decomposition method.Coarse-to-fine estimation and GNC.We vary the number of warping steps per pyramid level andfind that3warping steps gives similar results as using10(Table3).For the GNC scheme,[31]uses a downsampling factor of0.8for non-convex optimization.A downsampling factor of0.5 (Down-0.5),however,has nearly identical performance Removing the GNC step for the Charbonnier penalty function(w/o GNC)results in higher EPE on most se-quences and higher energy on all sequences(Table4).This suggests that the GNC method is helpful even for the con-vex Charbonnier penalty function due to the nonlinearity ofAvg.EPE significance p-value Classic-C0.298——3warping steps0.30400.9688Down-0.50.2980 1.0000w/o GNC0.35400.1094Bilinear0.30200.1016w/o TA VG0.30600.1562Central derivativefilter0.30000.72667-point derivativefilter[13]0.30200.3125Bicubic-II0.29010.0391GC-0.45(λ=3)0.29210.0156GC-0.25(λ=0.7)0.2980 1.0000MF3×30.30500.1016MF7×70.30500.56252×MF0.3000 1.00005×MF0.30500.6875w/o MF0.35210.0078Classic++0.28510.0078 Table3.Model and Methods.Average end-point error(EPE)on the Middlebury training set for the baseline method(Classic-C) using different algorithm and modelingchoices.Figure1.Different penalty functions for the spatial terms:Char-bonnier( =0.001),generalized Charbonnier(a=0.45and a=0.25),and Lorentzian(σ=0.03).the data term.Secrets:The downsampling factor does not matter when using a convex penalty;a standard factor of0.5isfine. Some form of GNC is useful even for a convex robust penalty like Charbonnier because of the nonlinear data term. Interpolation method and derivatives.Wefind that bicu-bic interpolation is more accurate than bilinear(Table3, Bilinear),as already reported in previous work[34].Re-moving temporal averaging of the gradients(w/o TA VG), using Central differencefilters,or using a7-point deriva-tivefilter[13]all reduce accuracy compared to the base-line,but not significantly.The M ATLAB built-in function interp2is based on cubic convolution approximation[20]. The spline-based interpolation scheme[26]is consistently better(Bicubic-II).See[30]for more discussions. Secrets:Use spline-based bicubic interpolation with a5-pointfilter.Temporal averaging of the derivatives is proba-bly worthwhile for a small computational expense. Penalty functions.Wefind that the convex Charbonnier penalty performs better than the more robust,non-convex Lorentzian on both the training and test sets.One reason might be that non-convex functions are more difficult to op-timize,causing the optimization scheme tofind a poor local(a)With medianfiltering(b)Without medianfilteringFigure2.Estimatedflowfields on sequence“RubberWhale”using Classic-C with and without(w/o MF)the medianfiltering step. Color coding as in[6].(a)(w/MF)energy502,387and(b)(w/o MF)energy449,290.The medianfiltering step helps reach a so-lution free from outliers but with a higher energy.optimum.We investigate a generalized Charbonnier penalty functionρ(x)=(x2+ 2)a that is equal to the Charbon-nier penalty when a=0.5,and non-convex when a<0.5 (see Figure1).We optimize the regularization parameterλagain.Wefind a slightly non-convex penalty with a=0.45 (GC-0.45)performs consistently better than the Charbon-nier penalty,whereas more non-convex penalties(GC-0.25 with a=0.25)show no improvement.Secrets:The less-robust Charbonnier is preferable to the Lorentzian and a slightly non-convex penalty function(GC-0.45)is better still.Medianfiltering.The baseline5×5medianfilter(MF 5×5)is better than both MF3×3[34]and MF7×7but the difference is not significant(Table3).When we perform5×5medianfiltering twice(2×MF)orfive times(5×MF)per warping step,the results are worse.Finally,removing the medianfiltering step(w/o MF)makes the computedflow significantly less accurate with larger outliers as shown in Table3and Figure2.Secrets:Medianfiltering the intermediateflow results once after every warping iteration is the single most important secret;5×5is a goodfilter size.4.1.Best PracticesCombining the analysis above into a single approach means modifying the baseline to use the slightly non-convex generalized Charbonnier and the spline-based bicu-bic interpolation.This leads to a statistically significant improvement over the baseline(Table3,Classic++).This method is directly descended from HS and BA,yet updated with the current best optimization practices known to us. This simple method ranks9th in EPE and12th in AAE on the Middlebury test set.5.Models Underlying Median FilteringOur analysis reveals the practical importance of median filtering during optimization to denoise theflowfield.We ask whether there is a principle underlying this heuristic?One interesting observation is thatflowfields obtained with medianfiltering have substantially higher energy than those without(Table4and Figure2).If the medianfilter is helping to optimize the objective,it should lead to lower energies.Higher energies and more accurate estimates sug-gest that incorporating medianfiltering changes the objec-tive function being optimized.The insight that follows from this is that the medianfil-tering heuristic is related to the minimization of an objective function that differs from the classical one.In particular the optimization of Eq.(1),with interleaved medianfiltering, approximately minimizesE A(u,v,ˆu,ˆv)=(2)∑i,j{ρD(I1(i,j)−I2(i+u i,j,j+v i,j))+λ[ρS(u i,j−u i+1,j)+ρS(u i,j−u i,j+1)+ρS(v i,j−v i+1,j)+ρS(v i,j−v i,j+1)]}+λ2(||u−ˆu||2+||v−ˆv||2)+∑i,j∑(i ,j )∈N i,jλ3(|ˆu i,j−ˆu i ,j |+|ˆv i,j−ˆv i ,j |),whereˆu andˆv denote an auxiliaryflowfield,N i,j is the set of neighbors of pixel(i,j)in a possibly large area andλ2 andλ3are scalar weights.The term in braces is the same as theflow energy from Eq.(1),while the last term is new. This non-local term[14,15]imposes a particular smooth-ness assumption within a specified region of the auxiliary flowfieldˆu,ˆv2.Here we take this term to be a5×5rectan-gular region to match the size of the medianfilter in Classic-C.A third(coupling)term encouragesˆu,ˆv and u,v to be the same(c.f.[33,39]).The connection to medianfiltering(as a denoising method)derives from the fact that there is a direct relation-ship between the median and L1minimization.Consider a simplified version of Eq.(2)with just the coupling and non-local terms,where E(ˆu)=λ2||u−ˆu||2+∑i,j∑(i ,j )∈N i,jλ3|ˆu i,j−ˆu i ,j |.(3)While minimizing this is similar to medianfiltering u,there are two differences.First,the non-local term minimizes the L1distance between the central value and allflow values in its neighborhood except itself.Second,Eq.(3)incorpo-rates information about the data term through the coupling equation;medianfiltering theflow ignores the data term.The formal connection between Eq.(3)and medianfil-tering3is provided by Li and Osher[23]who show that min-2Bruhn et al.[13]also integrated information over a local region in a global method but did so for the data term.3Hsiao et al.[19]established the connection in a slightly different way.Classic-C 0.5890.7480.8660.502 1.816 2.317 1.126 1.424w/o GNC 0.5930.7500.8700.506 1.845 2.518 1.142 1.465w/o MF0.5170.7010.6680.449 1.418 1.830 1.066 1.395Table 4.Eq.(1)energy (×106)for the optical flow fields computed on the Middlebury training set .Note that Classic-C uses graduated non-convexity (GNC),which reduces the energy,and median filtering,which increases it.imizing Eq.(3)is related to a different median computationˆu (k +1)i,j=median (Neighbors (k )∪Data)(4)where Neighbors (k )={ˆu (k )i ,j }for (i ,j )∈N i,j and ˆu (0)=u as well as Data ={u i,j ,u i,j ±λ3λ2,u i,j±2λ3λ2···,u i,j ±|N i,j |λ32λ2},where |N i,j |denotes the (even)number of neighbors of (i,j ).Note that the set of “data”values is balanced with an equal number of elements on either side of the value u i,j and that information about the data term is included through u i,j .Repeated application of Eq.(4)converges rapidly [23].Observe that,as λ3/λ2increases,the weighted data val-ues on either side of u i,j move away from the values of Neighbors and cancel each other out.As this happens,Eq.(4)approximates the median at the first iterationˆu (1)i,j ≈median (Neighbors (0)∪{u i,j }).(5)Eq.(2)thus combines the original objective with an ap-proximation to the median,the influence of which is con-trolled by λ3/λ2.Note in practice the weight λ2on thecoupling term is usually small or is steadily increased from small values [34,39].We optimize the new objective (2)by alternately minimizingE O (u ,v )=∑i,jρD (I 1(i,j )−I 2(i +u i,j ,j +v i,j ))+λ[ρS (u i,j −u i +1,j )+ρS (u i,j −u i,j +1)+ρS (v i,j −v i +1,j )+ρS (v i,j −v i,j +1)]+λ2(||u −ˆu ||2+||v −ˆv ||2)(6)andE M (ˆu ,ˆv )=λ2(||u −ˆu ||2+||v −ˆv ||2)(7)+∑i,j ∑(i ,j )∈N i,jλ3(|ˆu i,j −ˆu i ,j |+|ˆv i,j −ˆv i ,j |).Note that an alternative formulation would drop the cou-pling term and impose the non-local term directly on u and v .We find that optimization of the coupled set of equations is superior in terms of EPE performance.The alternating optimization strategy first holds ˆu ,ˆv fixed and minimizes Eq.(6)w.r.t.u ,v .Then,with u ,v fixed,we minimize Eq.(7)w.r.t.ˆu ,ˆv .Note that Eqs.(3)andAvg.EPE significancep -value Classic-C0.298——Classic-C-A0.30500.8125Table 5.Average end-point error (EPE)on the Middlebury train-ing set is shown for the new model with alternating optimization (Classic-C-A ).(7)can be minimized by repeated application of Eq.(4);weuse this approach with 5iterations.We perform 10steps of alternating optimizations at every pyramid level and change λ2logarithmically from 10−4to 102.During the first and second GNC stages,we set u ,v to be ˆu ,ˆv after every warp-ing step (this step helps reach solutions with lower energy and EPE [30]).In the end,we take ˆu ,ˆv as the final flow field estimate.The other parameters are λ=5,λ3=1.Alternatingly optimizing this new objective function (Classic-C-A )leads to similar results as the baseline Classic-C (Table 5).We also compare the energy of these solutions using the new objective and find the alternat-ing optimization produces the lowest energy solutions,as shown in Table 6.To do so,we set both the flow field u ,v and the auxiliary flow field ˆu ,ˆv to be the same in Eq.(2).In summary,we show that the heuristic median filter-ing step in Classic-C can now be viewed as energy min-imization of a new objective with a non-local term.The explicit formulation emphasizes the value of robustly inte-grating information over large neighborhoods and enables the improved model described below.6.Improved ModelBy formalizing the median filtering heuristic as an ex-plicit objective function,we can find ways to improve it.While median filtering in a large neighborhood has advan-tages as we have seen,it also has problems.A neighborhood centered on a corner or thin structure is dominated by the surround and computing the median results in oversmooth-ing as illustrated in Figure 3(a).Examining the non-local term suggests a solution.For a given pixel,if we know which other pixels in the area be-long to the same surface,we can weight them more highly.The modification to the objective function is achieved by introducing a weight into the non-local term [14,15]:∑i,j ∑(i ,j )∈N i,jw i,j,i ,j (|ˆu i,j −ˆu i ,j |+|ˆv i,j −ˆv i ,j |),(8)where w i,j,i ,j represents how likely pixel i ,j is to belongto the same surface as i,j .。
分支定价算法
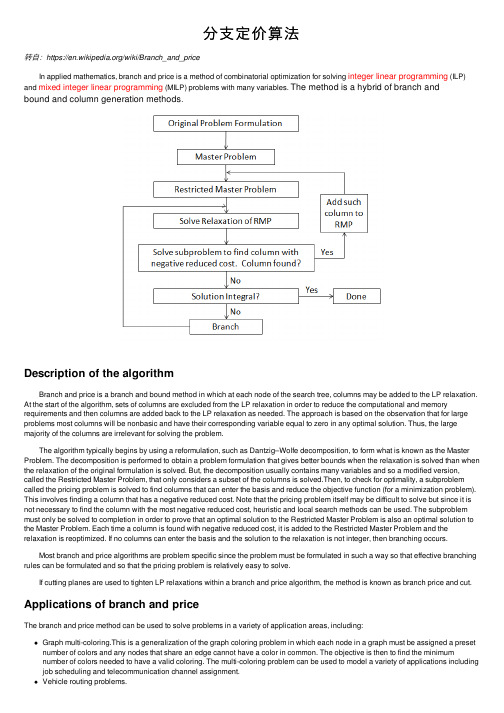
分⽀定价算法转⾃:https:///wiki/Branch_and_price In applied mathematics, branch and price is a method of combinatorial optimization for solving integer linear programming (ILP) and mixed integer linear programming (MILP) problems with many variables. The method is a hybrid of branch andbound and column generation methods.Description of the algorithm Branch and price is a branch and bound method in which at each node of the search tree, columns may be added to the LP relaxation. At the start of the algorithm, sets of columns are excluded from the LP relaxation in order to reduce the computational and memory requirements and then columns are added back to the LP relaxation as needed. The approach is based on the observation that for large problems most columns will be nonbasic and have their corresponding variable equal to zero in any optimal solution. Thus, the large majority of the columns are irrelevant for solving the problem. The algorithm typically begins by using a reformulation, such as Dantzig–Wolfe decomposition, to form what is known as the Master Problem. The decomposition is performed to obtain a problem formulation that gives better bounds when the relaxation is solved than when the relaxation of the original formulation is solved. But, the decomposition usually contains many variables and so a modified version, called the Restricted Master Problem, that only considers a subset of the columns is solved.Then, to check for optimality, a subproblem called the pricing problem is solved to find columns that can enter the basis and reduce the objective function (for a minimization problem). This involves finding a column that has a negative reduced cost. Note that the pricing problem itself may be difficult to solve but since it is not necessary to find the column with the most negative reduced cost, heuristic and local search methods can be used. The subproblem must only be solved to completion in order to prove that an optimal solution to the Restricted Master Problem is also an optimal solution to the Master Problem. Each time a column is found with negative reduced cost, it is added to the Restricted Master Problem and the relaxation is reoptimized. If no columns can enter the basis and the solution to the relaxation is not integer, then branching occurs. Most branch and price algorithms are problem specific since the problem must be formulated in such a way so that effective branching rules can be formulated and so that the pricing problem is relatively easy to solve. If cutting planes are used to tighten LP relaxations within a branch and price algorithm, the method is known as branch price and cut. Applications of branch and priceThe branch and price method can be used to solve problems in a variety of application areas, including:Graph multi-coloring.This is a generalization of the graph coloring problem in which each node in a graph must be assigned a preset number of colors and any nodes that share an edge cannot have a color in common. The objective is then to find the minimum number of colors needed to have a valid coloring. The multi-coloring problem can be used to model a variety of applications including job scheduling and telecommunication channel assignment.Vehicle routing problems.Generalized assignment problem.。
卡梅伦液压数据手册(第 20 版)说明书
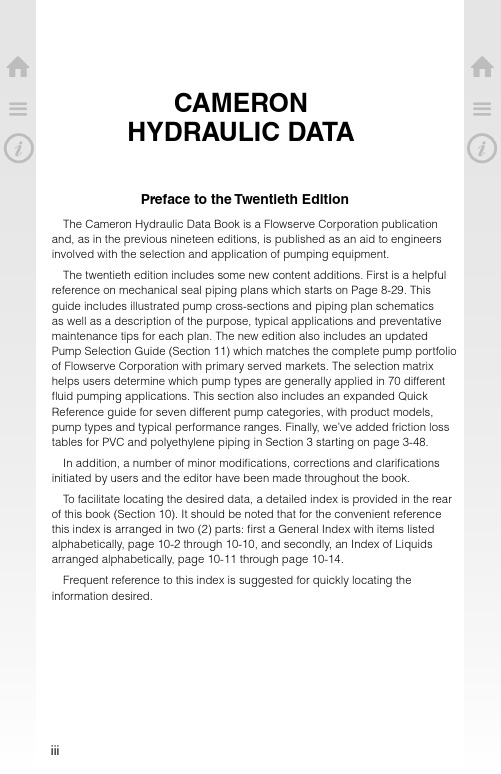
iv
⌂
CONTENTS OF SECTION 1
☰ Hydraulics
⌂ Cameron Hydraulic Data ☰
Introduction. . . . . . . . . . . . . ................................................................ 1-3 Liquids. . . . . . . . . . . . . . . . . . . ...................................... .......................... 1-3
4
Viscosity etc.
Steam data....................................................................................................................................................................................... 6
1 Liquid Flow.............................................................................. 1-4
Viscosity. . . . . . . . . . . . . . . . . ...................................... .......................... 1-5 Pumping. . . . . . . . . . . . . . . . . ...................................... .......................... 1-6 Volume-System Head Calculations-Suction Head. ........................... 1-6, 1-7 Suction Lift-Total Discharge Head-Velocity Head............................. 1-7, 1-8 Total Sys. Head-Pump Head-Pressure-Spec. Gravity. ...................... 1-9, 1-10 Net Positive Suction Head. .......................................................... 1-11 NPSH-Suction Head-Life; Examples:....................... ............... 1-11 to 1-16 NPSH-Hydrocarbon Corrections.................................................... 1-16 NPSH-Reciprocating Pumps. ....................................................... 1-17 Acceleration Head-Reciprocating Pumps. ........................................ 1-18 Entrance Losses-Specific Speed. .................................................. 1-19 Specific Speed-Impeller. .................................... ........................ 1-19 Specific Speed-Suction...................................... ................. 1-20, 1-21 Submergence.. . . . . . . . . ....................................... ................. 1-21, 1-22 Intake Design-Vertical Wet Pit Pumps....................................... 1-22, 1-27 Work Performed in Pumping. ............................... ........................ 1-27 Temperature Rise. . . . . . . ...................................... ........................ 1-28 Characteristic Curves. . ...................................... ........................ 1-29 Affinity Laws-Stepping Curves. ..................................................... 1-30 System Curves.. . . . . . . . ....................................... ........................ 1-31 Parallel and Series Operation. .............................. ................. 1-32, 1-33 Water Hammer. . . . . . . . . . ...................................... ........................ 1-34 Reciprocating Pumps-Performance. ............................................... 1-35 Recip. Pumps-Pulsation Analysis & System Piping...................... 1-36 to 1-45 Pump Drivers-Speed Torque Curves. ....................................... 1-45, 1-46 Engine Drivers-Impeller Profiles. ................................................... 1-47 Hydraulic Institute Charts.................................... ............... 1-48 to 1-52 Bibliography.. . . . . . . . . . . . ...................................... ........................ 1-53
SPE-159919译文
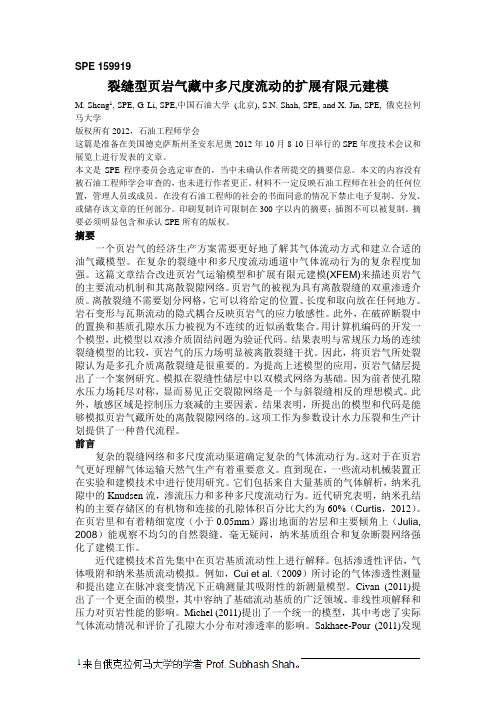
SPE 159919裂缝型页岩气藏中多尺度流动的扩展有限元建模M. Sheng1, SPE, G. Li, SPE,中国石油大学(北京), S.N. Shah, SPE, and X. Jin, SPE, 俄克拉何马大学版权所有2012,石油工程师学会这篇是准备在美国德克萨斯州圣安东尼奥2012年10月8-10日举行的SPE年度技术会议和展览上进行发表的文章。
本文是SPE程序委员会选定审查的,当中未确认作者所提交的摘要信息。
本文的内容没有被石油工程师学会审查的,也未进行作者更正。
材料不一定反映石油工程师在社会的任何位置,管理人员或成员。
在没有石油工程师的社会的书面同意的情况下禁止电子复制、分发、或储存该文章的任何部分。
印刷复制许可限制在300字以内的摘要;插图不可以被复制。
摘要必须明显包含和承认SPE所有的版权。
摘要一个页岩气的经济生产方案需要更好地了解其气体流动方式和建立合适的油气藏模型。
在复杂的裂缝中和多尺度流动通道中气体流动行为的复杂程度加强。
这篇文章结合改进页岩气运输模型和扩展有限元建模(XFEM)来描述页岩气的主要流动机制和其离散裂隙网络。
页岩气的被视为具有离散裂缝的双重渗透介质。
离散裂缝不需要划分网格,它可以将给定的位置、长度和取向放在任何地方。
岩石变形与瓦斯流动的隐式耦合反映页岩气的应力敏感性。
此外,在破碎断裂中的置换和基质孔隙水压力被视为不连续的近似函数集合。
用计算机编码的开发一个模型,此模型以双渗介质固结问题为验证代码。
结果表明与常规压力场的连续裂缝模型的比较,页岩气的压力场明显被离散裂缝干扰。
因此,将页岩气所处裂隙认为是多孔介质离散裂缝是很重要的。
为提高上述模型的应用,页岩气储层提出了一个案例研究。
模拟在裂缝性储层中以双模式网络为基础。
因为前者使孔隙水压力场耗尽对称,显而易见正交裂隙网络是一个与斜裂缝相反的理想模式。
此外,敏感区域是控制压力衰减的主要因素。
结果表明,所提出的模型和代码是能够模拟页岩气藏所处的离散裂隙网络的。
HighRe solutions for incompressible flow using the Navier-Stokes equations and a Multigrid Method
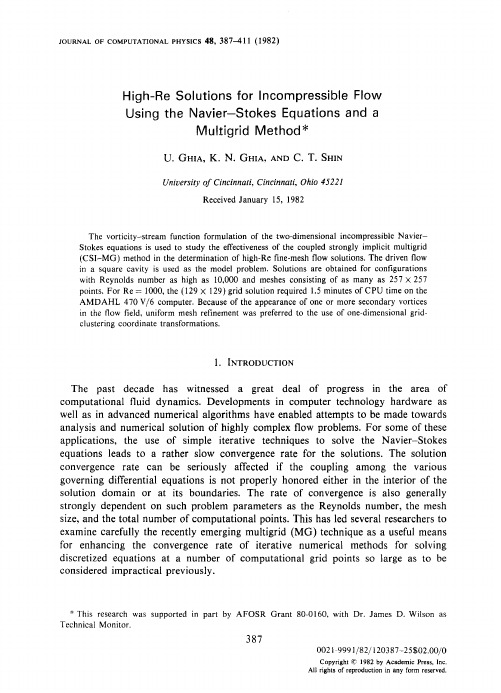
2. BASIC PRINCIPLE OF MULTIGRID TECHNIQUE Following Brandt and Dinar [7], the continuous differential problem considered is a system of I partial differential equations represented symbolically as Lj CT(X)= Fj(~), with the m boundary conditions Bi o(2) = G,(Z), i = 1, 2,..., m, X E aD, (2.2) j= 1,2 ,..., 1, YED, (2.1)
where 0 = (U, , U, ,..., U,) are the unknown variables, X = (x,, x2,..., xd) are the d independent variables of the d-dimensional problem, Fj and Gi are known functions on domain D and its boundary c?D, respectively, and Lj and Bi are general differential operators. A finite-difference solution to the problem described by Eqs. (2.1) and (2.2) is desired in a computational domain with grid spacing h. With a superscript h to denote the finite-difference approximation, the linear system of algebraic equations resulting from a selected difference scheme can be represented as (2.3) A conventional iterative technique for solving Eq. (2.3) consists of repeated sweeps of some relaxation scheme, the simplest being the Gauss-Seidel scheme, until convergence is achieved. It is often experienced that the convergence of the method is fast only for the first few iterations. This phenomenon can be explained if one considers a Fourier analysis of the error. Brandt (51 has thus estimated the
外文翻译--基于优化的牛顿—拉夫逊法和牛顿法的潮流计算
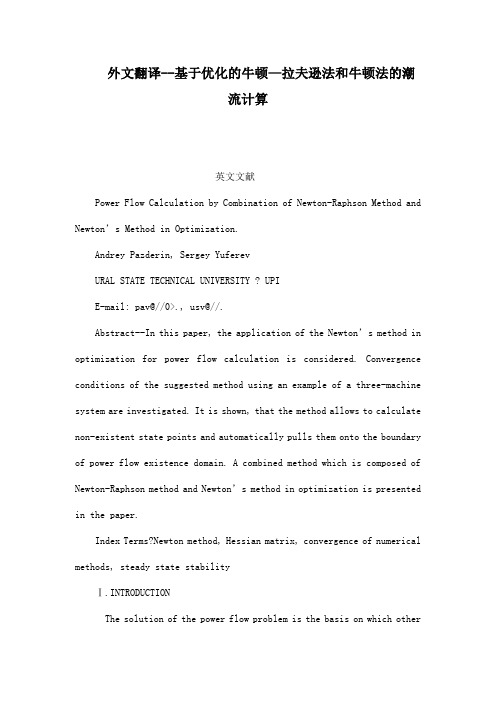
外文翻译--基于优化的牛顿—拉夫逊法和牛顿法的潮流计算英文文献Power Flow Calculation by Combination of Newton-Raphson Method and Newton’s Method in Optimization.Andrey Pazderin, Sergey YuferevURAL STATE TECHNICAL UNIVERSITY ? UPIE-mail: pav@//0>., usv@//.Abstract--In this paper, the application of the Newton’s method in optimization for power flow calculation is considered. Convergence conditions of the suggested method using an example of a three-machine system are investigated. It is shown, that the method allows to calculate non-existent state points and automatically pulls them onto the boundary of power flow existence domain. A combined method which is composed of Newton-Raphson method and Newton’s method in optimization is presented in the paper.Index Terms?Newton method, Hessian matrix, convergence of numerical methods, steady state stabilityⅠ.INTRODUCTIONThe solution of the power flow problem is the basis on which otherproblems of managing the operation and development of electrical power systems EPS are solved. The complexity of the problem of power flow calculation is attributed to nonlinearity of steady-state equations system and its high dimensionality, which involves iterative methods. The basic problem of the power flow calculation is that of the solution feasibility and iterative process convergence [1].The desire to find a solution which would be on the boundary of the existence domain when the given nodal capacities are outside the existence domain of the solution, and it is required to pull the state point back onto the feasibility boundary, motivates to develop methods and algorithms for power flow calculation, providing reliable convergence to the solution.The algorithm for the power flow calculation based on the Newton's method in optimization allows to find a solution for the situation when initial data are outside the existence domain and to pull the operation point onto the feasibility boundary by an optimal path. Also it is possible to estimate a static stability margin by utilizing Newton's method in optimization.As the algorithm based on the Newton’s method in optimization has considerable computational cost and power control cannot be realized in all nodes, the algorithm based on the combination of the Newton-Raphson met hods and the Newton’s method in optimization is offered to be utilizedfor calculating speed, enhancing the power flow calculation.II. THEORETICAL BACKGROUNDA.Steady-state equationsThe system of steady-state equations, in general, can be expressed as follows: where is the vector of parameters given for power flow calculation. In power flow calculation, real and reactive powers are set in each bus except for the slack bus. Ingeneration buses, the modulus of voltage can be fixed. WX,Y is the nonlinear vector function of steady-state equations. Variables Y define the quasi-constant parameters associated with an equivalent circuit of an electrical network. X is a required state vector, it defines steady state of EPS. The dimension of the state vector coincides with the number of nonlinear equations of the system 1. There are various known forms of notation of the steady-state equations. Normally, they are nodal-voltage equations in the form of power balance or in the form of current balance. Complex quantities in these equations can be presented in polar or rectangular coordinates, which leads to a sufficiently large variety forms of the steady-state equations notation. There are variable methods of a nonlinear system of steady-state equations solution. They are united by the incremental vector of independent variables ΔX being searched and the condition of convergence being assessed at each iteration.B. The Newton's method in optimizationAnother way of solving the problem of power flow calculation is related to defining a zero minimum of objective function of squares sum of discrepancies of steady-stateequations:2?The function minimum 2 is reached at the point where derivatives on all required variables are equal to zero: 3It is necessary to solve a nonlinear set of equations 3 to find the solution for the problem. Calculating the power flow, which is made by the system of the linear equations with a Hessian matrix at each iteration, is referred to as the Newton'smethod in optimization [4]: 4The Hessian matrix contains two items: 5During the power flow calculation, the determinant of Hessian matrix is positive round zero and negative value of a determinant of Jacobian .This allows to find the state point during the power flow calculation, when initial point has been outside of the existence domain.The convergence domain of the solution of the Newton's optimization method is limited by a positive value of the Hessian matrix determinant. The iterative process even for a solvable operating point can converge to an incorrectsolution if initial approximation has been outside convergencedomain. This allows to estimate a static stability margin of the state and to find the most perilous path of its weighting.III. INVESTIGATIONS ON THE TEST SCHEMEConvergence of the Newton's method in optimization with a full Hessian matrix has been investigated. Calculations were made based on program MathCAD for a network comprising three buses the parameters of which are presented in Figure 1.Dependant variables were angles of vectors of bus voltage 1 and 2 ,independent variables were capacities in nodes 1 and 2, and absolute values of voltages of nodes 1, 2 and 3 were fixed.Fig. 1 ? The Test schemeIn Figure 2, the boundary of existence domain for a solution of the steady-state is presented in angular coordinates δ1-δ2. This boundary conforms to a positive value of the Jacobian determinant:As a result of the power flow calculation based on the Newton method in optimization, the angle values have been received, these values corresponding to the given capacities in Fig.2 generation is positive and loading is negative.For the state points which are inside the existence domain, the objective function 2 has been reduced to zero. For the state points which are on the boundary of the existence domain, objective function 2 has not been reduced to zero and the calculated values of capacities differed fromthe given capacities.Fig. 2 ? Domain of Existence for a SolutionFig.3 - Boundary of existence domain In Fig.3, the boundary of the existence domain is presented in coordinates of capacities P1-P2. State points occurring on the boundary of the existence domain 6 have been set by the capacities which were outside the existence domain. As a result of power flow calculation by minimization 2 based on the Newton's method in optimization, the iterative process converges to the nearest boundary point. It is due to the fact that surfaces of the equal level of objective function 2 in coordinates of nodal capacities are proper circles for threemachine system having the centre on the point defined by given values of nodal capacitiesThe graphic interpretation of surfaces of the equal level of objective function for operating point state with 13000 MW loading bus 1 and 15000 MW generating bus 2 is presented in Fig.3.Hessian matrix is remarkable in its being not singular on the boundary of existence domain. The determinant of a Hessian matrix 5 is positive around zero and a negative value of the Jacobian matrix determinant. This fact allows the power flow to be calculated even for the unstable points which are outside existence domain. The iterative process based on the system of the linear equations 4 solution has converged to the critical stability point within 3-5 iteration. Naturally,the iterative process based on Newton-Rapson method is divergent for such unsolvable operating points.The convergence domain of the method under consideration has been investigated. What is meant is that not all unsolvable operating points will be pulled onto theboundary of existence domain. A certain threshold having been exceeded the iterative process has begun to converge to the imaginary solution with angles exceeding 360It is necessary to note that to receive a critical stability operating point in case when initial nodal capacities are set outside the boundary of the existence domain, there is no necessity to make any additional terms as the iterative process converges naturally to the nearest boundary point.Pulling the operation point onto feasibility boundary is not always possible by the shortest and optimal path. There are a number of constraints, such as impossibility of load consumption increase at buses, constraints of generation shedding/gaining at stations. Load following capability of generator units is various, consequently for faster pulling the operation point onto the feasibility boundary it is necessary to carry out this pulling probably by longer, but faster path.The algorithm provides possibility of path correction of pulling. It is carried out by using of the weighting coefficients, which define degree of participation of eachnode in total control action. For this purpose diagonal matrix A of the weighting coefficients for each node is included into the objective function 2:All diagonal elements of the weighting coefficient matrix A should be greater-than zero:When initial approximation lies into the feasibility domain, coefficients are not influence on the computational process and on the result.In the figure 4 different paths of the pulling the same operation point onto feasibility boundary depending on the weighting coefficients are presented. Paths are presented for two different operating points.In tables I and II effect of weighting coefficients on the output computation is presented. In tables I and II k1 and k2 are weighting coefficient for buses 1 and 2, respectively.TABLE IWEIGHTING COEFFICIENT EFFECT ON OUTPUT COMPUTATION FOR INITIAL SET CAPACITIES P1 -13000 MW AND P2 15000 MWCoefficients ,MW ,MW ,deg ,deg1,1 -7800 9410 -45 555,1 -8600 8080 -69 250.005,1 -5700 10140 -1 93TABLE IIWEIGHTING COEFFICIENT EFFECT ON OUTPUT COMPUTATION FOR INITIAL SET CAPACITIES P1 -8000 MW AND P2 -5000 MWCoefficients ,MW ,MW ,deg ,deg1,1 -4360 -1680 -92 -800.01,1 -1050 -4920 -76 -941,0.35 5800 0 -99 -71Fig.4 - Paths of pulling the operation point onto the feasibility boundaryIV. COMBINATION OF METHODSIf to compare the Newton’s method in optimization for power flow calculation with newton-Raphson using a Jacobian matrix, the method computational costs on eachiteration will be several times greater as the property of Hessian matrix being filled up by nonzero elements 2.5-3 times greater than with Jacobian one. Each row of Jacobian matrix corresponding to any bus contains nonzero elements corresponding to all incident buses of the scheme. Each row of Hessian matrix contains nonzero elements in the matrix corresponding not only to the neighboring buses, but also their neighbors. However, it is possible to compensate this disadvantage through the combination Newton-Rap son method with Newton’s method in optimization. It means that the part of nodes can be calculated by conventional Newtonmethod, and the remaining buses will be computed by Newton’s method in optimization. The first group of passive nodes consists of buses in which it is not possible to changenodal capacity or it is not expedient. Hence, emergency control actions are possible only in a small group of buses supplying with telecontrol. Most of the nodes includingpurely transit buses are passive. Active nodes are generating buses in which operating actions are provided. Such approach allows to fix nodal capacity for all passive buses of the scheme which have been calculated by Newton-Rap son method. In active buses which have been calculated by Newton’s method in optimization, deviations from set values of nodal capacity are possible. These deviations can be considered as control action. The power flow calculation algorithm based on combination Newton ? Ra phson method and Newton’s method in optimization can be presented as follows:1.The linear equation system with Jacobian matrix is generated for all buses of the scheme.2. The solution process of the linear equation system with Jacobian is started by utilizing the Gauss method for all passive buses. Factorization of the linear equations system is terminated when all passive buses are eliminated. Factorizedequations are kept.3.The nodal admittance matrix is generated from not factorized the part of Jacobian matrix corresponding to active buses. This admittance matrix contains parameters of the equivalent network which contains only active buses.4.The linear equation system with Hessian matrix 4 is generated for the obtained equivalent by Newton’s method in optimization.5.The linear equation system with Hessian matrix is calculated and changes of independent variables are defined for active buses.6.Factorized equations of passive buses are calculated, and changes of independent variables are defined for passive buses.7.The vector of independent variables is updated using the changes of independent variables for all buses.8. New nodal capacities in all buses of the network are defined; constraints are checked; if it necessary, the list of active buses will be corrected.9. Convergence of the iterative process is checked. If changes of variables are significant, it is necessary to return to item 1.Taking into account the number of active buses in the network aren’t large, computationa l costs of such algorithm slightly exceed computational costs of the Newton-Rapson method.V. CONCLUSION1. The power flow calculation of an electric network by minimizingthe square sum of discrepancies of nodal capacities based on the Newton's method in optimizationmaterially increases the productivity of deriving a solution for heavy in terms of conditions of stability states and the unstable states outside the existence domain of the solution.2. During the power flow calculation, the determinant of Hessian matrix is positive around zero and negative value of the Jacobian matrix determinant. The iterative process naturally converges to the nearest marginal state point during the power flow calculation, when the initial operating point has been outside of the existence domain.3. There is a possibility of control action correction for the pulling operation point onto feasibility boundary by using matrix of weighting coefficients.4. Utilization of the combined method for power flow calculation all ows to use all advantages of Newton’s method in optimization and to provide high calculating speed.5. In case when the setting nodal powers are outside the existence domain, there are discrepancies in the active buses, which can be considered as control actions for pulling the state point onto the feasibility boundary. When the initial state point is inside the existence domain, the iterative process converges with zero discrepancies for both active and passive buses.中文翻译基于优化的牛顿??拉夫逊法和牛顿法的潮流计算摘要??在本文中,考虑到了优化的牛顿法在潮流计算中的应用。
混悬剂的制备以及质量检查的内容流程图
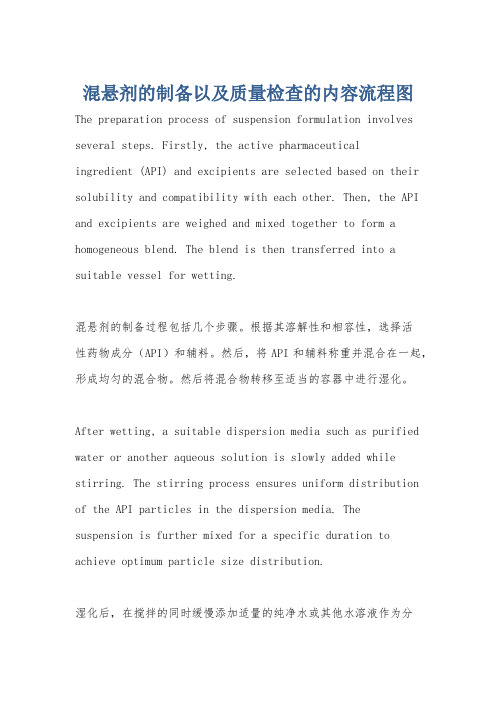
混悬剂的制备以及质量检查的内容流程图The preparation process of suspension formulation involves several steps. Firstly, the active pharmaceuticalingredient (API) and excipients are selected based on their solubility and compatibility with each other. Then, the API and excipients are weighed and mixed together to form a homogeneous blend. The blend is then transferred into a suitable vessel for wetting.混悬剂的制备过程包括几个步骤。
根据其溶解性和相容性,选择活性药物成分(API)和辅料。
然后,将API和辅料称重并混合在一起,形成均匀的混合物。
然后将混合物转移至适当的容器中进行湿化。
After wetting, a suitable dispersion media such as purified water or another aqueous solution is slowly added while stirring. The stirring process ensures uniform distribution of the API particles in the dispersion media. The suspension is further mixed for a specific duration to achieve optimum particle size distribution.湿化后,在搅拌的同时缓慢添加适量的纯净水或其他水溶液作为分散介质。
aqueous parenteral preparations
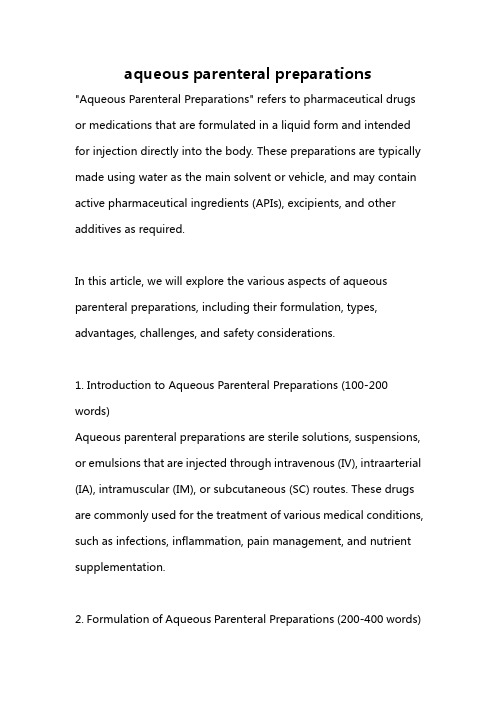
aqueous parenteral preparations "Aqueous Parenteral Preparations" refers to pharmaceutical drugs or medications that are formulated in a liquid form and intended for injection directly into the body. These preparations are typically made using water as the main solvent or vehicle, and may contain active pharmaceutical ingredients (APIs), excipients, and other additives as required.In this article, we will explore the various aspects of aqueous parenteral preparations, including their formulation, types, advantages, challenges, and safety considerations.1. Introduction to Aqueous Parenteral Preparations (100-200 words)Aqueous parenteral preparations are sterile solutions, suspensions, or emulsions that are injected through intravenous (IV), intraarterial (IA), intramuscular (IM), or subcutaneous (SC) routes. These drugs are commonly used for the treatment of various medical conditions, such as infections, inflammation, pain management, and nutrient supplementation.2. Formulation of Aqueous Parenteral Preparations (200-400 words)Formulating aqueous parenteral preparations involves careful consideration of several factors, including the solubility of the active pharmaceutical ingredient (API), stability, pH, tonicity, compatibility with excipients, and viscosity.The solubility of the API is crucial, as it determines the concentration of the drug in the final product. APIs with limited solubility may require additional solubilizing agents or complexation techniques to improve their dissolution in water.Stability is another important factor to consider during formulation. Aqueous parenteral preparations should remain stable throughout their shelf life, without undergoing degradation, precipitation, or the formation of particulate matter.pH also plays a vital role in formulation. Most drugs have a narrow pH range for optimal stability, and the pH of the formulation must be adjusted accordingly. Buffering agents are used to maintain the desired pH and prevent any pH-induced degradation of the drug.Tonicity refers to the osmolarity of the formulation. It is important to match the tonicity of the formulation with that of the body fluidsto minimize discomfort and potential tissue damage upon injection.Viscosity is another consideration, as it affects the injectability and ease of administration of the parenteral preparation. Formulators may employ viscosity-enhancing agents to achieve the desired flow properties.3. Types of Aqueous Parenteral Preparations (300-500 words) Aqueous parenteral preparations can be classified based on their physical form, mode of drug release, and intended route of administration.- Solutions: These preparations contain one or more API dissolved in water. Solutions are clear and compatible with a wide range of pharmaceutical ingredients. They are commonly used for the administration of small-molecule drugs and, in some cases, can be administered as bolus or continuous infusions.- Suspensions: These preparations consist of solid particles uniformly dispersed in water. Suspensions are used when the API is insoluble in water or to provide a slow-release profile. They requireshaking or agitation before administration and are often used for depot injections or long-acting formulations.- Emulsions: Emulsions are biphasic systems consisting of small droplets of oil dispersed in water or vice versa. They are used to enhance the solubility or stability of lipophilic drugs, as well as for controlled-release formulations. Emulsions are typically used for IV administration.- Liposomes: Liposomes are vesicles composed of one or more lipid bilayers that encapsulate the drug. They are used for the delivery of lipophilic drugs, as well as to protect the drug from degradation or enhance its targeting to specific tissues. Liposomes can be administered via various parenteral routes, including IV and SC.- Nanoparticles: These are colloidal particles ranging in size from 1 to 1000 nanometers that encapsulate or adsorb the drug. Nanoparticles can be made from various materials, such as lipids, polymers, or metals, and are used for targeted drug delivery or sustained release.4. Advantages and Challenges of Aqueous Parenteral Preparations(500-700 words)Aqueous parenteral preparations offer several advantages compared to other dosage forms, including:- Rapid onset of action: Injected drugs bypass the digestive system and are quickly absorbed into the bloodstream, leading to rapid onset of action.- Precise dosing: Parenteral administration allows for accurate and precise delivery of the drug, which is especially important for medications with a narrow therapeutic index.- Increased bioavailability: The absence of first-pass metabolism enhances the bioavailability of drugs administered parenterally, as they are directly delivered to the systemic circulation.- Flexibility in drug formulation: The aqueous nature of these preparations allows for easy incorporation of a wide range of APIs, excipients, and additives, making them suitable for various therapeutic applications.Despite the advantages, there are also challenges associated withaqueous parenteral preparations, including:- Sterility: Parenteral preparations must be formulated under strict aseptic conditions to prevent microbial contamination.- Stability: Maintaining the stability of the drug in the formulation can be challenging due to factors such as pH, temperature, and interaction with other components.- Tissue irritation: Some drugs or excipients may cause local tissue reactions or irritation at the injection site. It is important to select suitable excipients and adjust the formulation parameters to minimize this risk.- Compatibility: Compatibility studies are essential to ensure that the drug and excipients in the formulation do not interact and cause stability or efficacy issues.- Administration pain: Certain formulations or drug concentrations can cause pain or discomfort upon injection. Proper formulation design and use of suitable solvents or buffering agents can help alleviate this issue.5. Safety Considerations for Aqueous Parenteral Preparations (200-300 words)Aqueous parenteral preparations must adhere to regulatory guidelines and safety standards to ensure patient safety. Key considerations include:- Sterility assurance: Manufacturers must follow Good Manufacturing Practices (GMP) and conduct rigorous sterility testing to ensure the absence of microbial contamination.- Pyrogen testing: Pyrogens are substances that can cause fever when introduced into the body. Aqueous parenteral preparations should be subjected to pyrogen testing to ensure they are free from pyrogenic contaminants.- Packaging and labeling: Proper packaging materials and labeling protocols should be employed to protect the integrity and identity of the parenteral preparations throughout their shelf life.- Storage and transportation: Storage conditions, such as temperature and light exposure, should be strictly controlled tomaintain the stability and efficacy of the preparations. Additionally, proper transportation practices must be followed to prevent any damage or compromise of the product.- Product recalls and adverse event reporting: In case of any manufacturing defects or adverse events associated with the use of aqueous parenteral preparations, manufacturers must have robust recall and reporting systems in place to promptly address any safety concerns.In conclusion, aqueous parenteral preparations play a vital role in delivering drugs directly into the body for rapid action, increased bioavailability, and precise dosing. Formulating these preparations requires careful consideration of various factors such as solubility, stability, tonicity, pH, and viscosity. Different types of aqueous parenteral preparations, including solutions, suspensions, emulsions, liposomes, and nanoparticles, offer flexibility in drug delivery options. However, challenges related to sterility, stability, compatibility, and administration pain necessitate adherence to regulatory guidelines and safety standards to ensure the utmostpatient safety in the use of these preparations." (1500-2000 words)。
英文制药工艺流程

英文制药工艺流程Pharmaceutical Process FlowThe pharmaceutical industry plays a crucial role in the development, production, and distribution of medicines that save lives and improve the quality of life for people around the world. One of the key aspects of the pharmaceutical industry is the manufacturing process, which involves a series of steps to ensure the safety, efficacy, and quality of the final product. In this article, we will discuss the general process flow of pharmaceutical manufacturing.1. Research and Development: The first step in the pharmaceutical process is research and development (R&D), where scientists and researchers identify potential drug candidates and conduct extensive studies to understand their properties, efficacy, and safety. This involves the synthesis of new chemical compounds or the modification of existing compounds to create new drugs. R&D also includes preclinical testing, such as in vitro and animal studies, to assess the safety and effectiveness of the drug candidates.2. Formulation and Pre-Formulation: Once a drug candidate is identified, the next step is formulation and pre-formulation. During this stage, scientists determine the optimal dosage form of the drug, such as tablets, capsules, or injections, and develop a formulation that ensures the drug's stability, bioavailability, and patient acceptability. Pre-formulation studies focus on understanding the physical and chemical properties of the drug and determining the best excipients and manufacturing processes to achieve the desired formulation.3. Manufacturing: After formulation, the drug moves into the manufacturing phase. This involves scaling up the production from laboratory-scale to commercial-scale. The manufacturing process depends on the dosage form and can be divided into several steps. For solid dosage forms, such as tablets, powders or granules are mixed with excipients and compressed into tablets. For liquid dosage forms, such as syrups or suspensions, active pharmaceutical ingredients (APIs) are dissolved or suspended in a suitable vehicle. Sterile dosage forms, like injections or eye drops, require strict aseptic processing to prevent contamination.4. Quality Control: Throughout the manufacturing process, quality control is crucial to ensure that the final product meets the required standards and specifications. This involves the testing of raw materials, intermediates, and final products for chemical, physical, and microbiological parameters. Quality control also includes stability studies to determine the shelf life of the drug and ensure that it maintains its safety and efficacy over time.5. Packaging and Labeling: Once the final product has been manufactured and passed quality control, it is ready for packaging and labeling. The packaging must be designed to protect the drug from degradation, moisture, and tampering while maintaining its stability. The labeling includes important information such as drug name, strength, dosage instructions, and any necessary warnings or precautions.6. Distribution: After packaging, the pharmaceutical product is ready for distribution to pharmacies, hospitals, or other healthcarefacilities. This requires careful handling and storage to maintain the integrity and quality of the drugs. Distribution also involves proper documentation, including batch records, shipping records, and tracking systems, to ensure traceability and accountability.In conclusion, the pharmaceutical manufacturing process involves several stages, from research and development to distribution. Each step is crucial in ensuring the safety, efficacy, and quality of the final product. By following a well-defined process flow, pharmaceutical companies can produce medicines that meet regulatory standards and contribute to the health and well-being of individuals worldwide.。
Lightnin MagMixer MBE系列混合器产品说明书
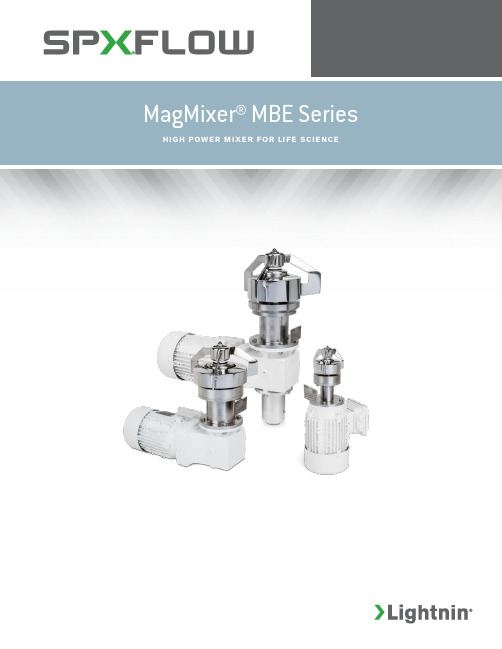
A Standard for Cleanability, Durability and Performance - MagMixer ® MBEMAG M I X E R M B E S E R I E S The big advantage of Lightnin’s magnetic agitator is the complete separation of the interior of the tank from the outside. In contrast to conventional agitators, there is no shaft penetrating the tank and therefore no mechanical seal. This eliminates the risks of leaks and microbial contamination and the need for special maintenance that are associated withconventional agitators. We have developed our magnetic agitators with special emphasis on optimizing their cleanability, which is essential for sterile processes. The MBE Series conforms to EC 1935 and AMSE BPE confirming the design of these agitators are qualified for such applications.Bottom-mounted magnetic agitators are state of the art for low-viscosity liquids in pharmaceutical and biotechnology production. The compact design, low maintenance and high reliability guarantee trouble-free production. Using a bottom-mounted agitator also frees up space on the tank lid for sensors, valves and sight glasses.Based in Charlotte, North Carolina, SPX FLOW is a leading global supplier of highly engineered flow components, process equipment and turn-key systems, along with the related aftermarket parts and services, into the food and beverage, power and energy and industrial end markets. SPX FLOW has more than $2 billion in annual revenues and approximately 8,000 employees with operations in over 35 countries and sales in over 150 countries around the world. T o learn more about SPX FLOW, please visit our website at A World Leader in Industrial Mixing since 1923, Lightnin has over 90 years of unrivaled experience in industrial mixing technology, process knowledge, and technological innovation. Lightnin enjoys a global reputation for durable, long-lasting mixers, agitators, aerators, and flocculators for fluid process systems. We offer a full spectrum of impeller designs for diverse applications. In addition, we offer a worldwide service network, mixer repair, gearbox repair, and replacement parts programs. Look to Lightnin for knowledge,technology, and service excellence.About SPX FLOWAbout LightninDairy, Food & Beverage P harmaceutical Fine ChemicalsM B E: OPE N D E S I G N & OPTI MAL FLOW TH R OU G H R OTOROpen design with excellent cleaning: hub and magnetic rotor are connected only by the impeller blades.The ceramic bearings are oversized (in diameter and height), product-lubricated and consist of outer (silicon carbide) and inner (zirconium oxide) bearing. This results in exceptional stability, good emergency running properties and particle generation below detectable levels.Ease of maintenance - ceramic bearing parts can be replaced by users on site; no spare rotor needed.A very large gap between the rotor and the containment shell maximizes flow through the gap and minimizes shear stress.CFD-engineered mixing: fluid is drawn from above and pumped radially. Perfect for mixing solid powders into liquids; rapid breakdown of temperature and concentration gradients, ensures good heating and cooling.M B E: MAG N ETI C I M PE LLE R SNew and stronger magnetic materials enable us to reach a higher transferable torque for the same geometry of the drive and rotor. Lightnin offers a wide range of drive sizes with operating torques from 30 Ncm to 300 Nm (2.65lbf.in to 2655lbf.in) to suit a wide range of applications.Lightnin MBE magnetic impellers ensure that the optimal formulation of the product is achieved throughout the whole volume. When the product is being transferred out of the vessel, the homogeneity of the mixed product is maintained reliably down to the last drop.The open design enables easy cleaning (CIP) and sterilization (SIP). Lightnin experts will help you select the best impeller variant for your process, the best drive unit and the most suitable installation option (welded or Plug In containment shell).Magnetic ImpellersDesign Features & Functions of Bottom-Mounted Magnetic AgitatorsB EAR I N G S• Zirconium oxide inner bearing: less risk of breakage, resistantto damage by sudden settling of the rotor• Silicon carbide outer bearing: with channels in the face side for better lubrication of the bottom contact surface andenhanced cleaning (CIP)• Run-dry capability with the rotor type MBE: Magnetic lifting of the impeller reduces the load on the bearing surface so thatthe agitator can be kept running while the vessel is emptied(mixing down to the last drop)LOW E R I N G D EVI CEAgitators size from MBE200 and upwards are supplied with aspecial lowering mechanism that withdraws the drive magnet outof the containment shell.B E N E FITS OF TH ER ETRACTAB LE D R IVE MAG N ETControlled removal and safe insertion of the impeller due to withdrawl of the drive magnet. The drive unit remains in positionwhile the magnetic drive rotor is lowered out of the containment shell. Avoids damage to the ceramic bearings. Improved safety: The device protects against the crushing hazard involved in placing theagitator head on the bearing, and thus meets the demand of theEC Machinery Directive for designed protection against injury.R E M OVAB LE CONTAI N M E NT S H E LL (PLU G-I N)As an alternative to a version with a flange for welding into the vessel, the agitator can be supplied with a removable containment shell. This can facilitate maintenance. This practical Plug In solutionis becoming more popular and makes it easier to switch fromshaft-driven agitators with mechanical seals to magnetic agitators.E LE CTR OP OLI S H I N G OFSTAI N LE S S STE E L S U R FACE SAs well as mechanical polishing to two levels, we also electropolishas standard so that we can meet the growing demand for thehighest possible surface quality.E XTRA LAR G E PLU G I N FLAN G EThe Plug In flange is also available with a large-diameter flange which allows the whole agitator head to be extracted from the vessel through the bottom opening.I M PE LLE R S PE E D M ON ITOR I N GSometimes a problem inside the tank or an operating error can lead to forces on the agitator head that exceed the maximum tranmissible torque, so that the magnetic coupling decouples. In this situation the agitator head stops turning although the drive is still running. T o monitor this issue, we offer an optional contact-free rpm sensor for the agitator head.ATTACH M E NT OF TH ED R IVE S H E LL BY TR I CLAM PAttaching the drive with a TriClamp fitting enables it to be removed quickly without tools, eg: when using the agitator with an autoclavable tank. As another alternative, bayonet fittings are also possible.E XTRACTOR TOOLAn optional tool for the removable containment shell (Plug In). The tool enables the containment shell to be removed from the tank easily and gently.Reliable and easy to maintainFor safety reasons, the speed sensor is included as standard in agitators for use in ATEXZone 0 (directive 2014/34/EU)5T echnical SpecificationsW ETTE D MATE RIALSStandard Max. Temp.: 280F (138°C)Standard Dry Running for 15 minutes at reduced speedStandard pH range: 1-14 OptionalImpeller removal toolOther Drive Options Stainless SteelTri-Clamp connectionTAN K PLATE / FLAN G E M OU NTI N G OPTI ON SWelded in Tank Plate - T ank plate welded to vessel by customer, Mixer bolts to plate, impeller sits on tank plate bearing postPlug in Tank Plate - Modified tank plate welded to vessel by customer, Mixer with coupling and bearing inserts into tankplate, Impeller sits on bearing D R IVE U N ITS7MBE 12D 80/3.150.090.1211701000140/37MBE 25D 105/4.130.180.241170950350/92MBE 25130/5.120.180.241110550500/132MBE 50165/6.490.370.4961105201,200/317MBE 75190/7.480.550.738854602,500/660MBE 100250/9.840.75 1.0068534014,500/3,830MBE 200300/11.81 1.50 2.0128530028,000/7,397MBE 300350/13.78 2.20 2.958528531,000/8,189MBE 550400/15.74 4.00 5.3648527025,000/6,604MBE 750450/17.72 5.507.3768527040,000/10,567MBE 1000500/19.697.5010.068523660,000/15,850MAG M I X E R M B E S E R I ESD R IVE PE D E STAL 316SS StandardG EAR E D M OTORWhite FDA Compliant Epoxy Paint IP55, IE3 Motor - Voltage to suit ATEX option available IP65, IP66, IP67 availableStainless Steel drive option availableW E LD PLATE Welds to vessel wall(Removable Plug In option available)AG ITATOR I M PE LLE R with integrated NeFeB magnets B EAR I N G SFemale bearing SiC (Silicon Carbide)Male bearing Zr02 (Zirconium Oxide)Options availableD R IVE MAG N ETSO-R I N GR ETAI N I N G B OLT with sealing O-ringD R IVE U N ITSGlobal LocationsS PX FLOW - LI G HTN I N & PLE NTY M I X E R S135 Mt. Read Blvd.Rochester, NY 14611P: +1 (888) 649-2378 (MIX-BEST) (US and Canada) or +1 (585) 436-5550F: +1 (585) 436-5589E:********************•/lightninSPX FLOW, Inc. reserves the right to incorporate our latest design and material changes without notice or obligation. Design features, materials of construction and dimen-sional data, as described in this bulletin, are provided for your information only and should not be relied upon unless confirmed in writing. Please contact your local sales representative for product availability in your region. For more information visit .The green “ ” and “ ” are trademarks of SPX FLOW, Inc.LIG-B-971 Version: 04/2018COPYRIGHT © 2018 SPX FLOW, Inc.AM E R I CAS S PX FLOW135 Mt. Read Blvd.Rochester, NY 14611P: +1 585 436 5550S PX FLOW B RA Z I LRua João Daprat231 São Bernardo do Campo SP 09600-010, Brazil P: +55 11 2127 8278S PX FLOW CH I LESPX FLOW Chile Limitada Av. Ricardo Lyon 222 of. 503Providencia - Santiago, Chile P: +56 2 8969 320S PX FLOW M E X I COAPV Soluciones Integrales, SA de CV Amargura # 60 Primer Piso Col. Lomas de La Herradura 52785 Huixquilucan, Edo de Mexico P: +52 55 5293 9048E M EA S PX FLOWOcean House, T owers Business Park Didsbury, Manchester M20 2YY, UK P: +44 161 249 1170S PX FLOW AFR I CAUnit 12BGrowthpoint Office Park, T onetti Street Midrand, South Africa P: +27 11 207 3700S PX FLOW M I D D LE EAST FZ EP.O Box 299745, Downtown Jebel Ali The Galleries 4Dubai, U.A.E.P: +971 4 8143400APACS PX FLOW PTY LTDSuite 2.3, Quad 28 Parkview DriveHomebush Bay NSW 2127, Australia P: +61 2 9763 4900S PX FLOW CH I NA7F, Nanfung T ower 1568 Hua Shan Road Shanghai 200052, China P: +86 (21) 2208 5888S PX FLOW S I N GAP OR E20 Pioneer Crescent, #06-01West Park BizCentral Singapore 628555P: +65 6264 4366。
- 1、下载文档前请自行甄别文档内容的完整性,平台不提供额外的编辑、内容补充、找答案等附加服务。
- 2、"仅部分预览"的文档,不可在线预览部分如存在完整性等问题,可反馈申请退款(可完整预览的文档不适用该条件!)。
- 3、如文档侵犯您的权益,请联系客服反馈,我们会尽快为您处理(人工客服工作时间:9:00-18:30)。
A Flow Graph Formulation of Optimal Software PipeliningDirk FimmelDresden University of Technology/IEE, 01062Dresden,Germanyfimmel@iee1.et.tu-dresden.deJan MüllerDresden University of Technology/IEE, 01062Dresden,Germanymuellerj@iee1.et.tu-dresden.deAbstractWe present a new approach to the loop scheduling problem, which excels previous solutions in two important aspects: The resource constraints are formulated usingflow graphs, and the initiation interval is treated as a rational vari-able.The approach supports heterogeneous processor ar-chitectures and pipelined functional units,and the Integer Linear Programming implementation produces an optimum loop schedule,whereby a minimum is achieved.Ourflow graph model facilitates the cyclic binding of loop operations to functional pared to previous research results, the solution can provide faster loop schedules and a signifi-cant reduction of the problem complexity and solution time.1.IntroductionRecent developments in processor design indicate that pro-cessor architectures become more and more heterogeneous. The addition of new functional units to processor kernels (e.g.MMX,SSE,and SSE2extensions of Intel Pentium processors)allows to operate more units in parallel. These developments raise the challenge to maximally ex-ploit available resources,and tofind operating schedules with minimum latencies.Current compilers,using heuris-tics for resource exploitation and loop scheduling,offer good solutions to these tasks[1,18].However,for the production of program libraries and for computationally in-tensive algorithms,the best solutions are needed,even if the compilation may take longer than with heuristic ap-proaches.This substantiates the use of Integer Linear Pro-gramming formulations[2,4,12,13,14],which always produce optimal solutions.The usual loop scheduling method,called Software Pipelin-ing[15,18],handles program loops with single assignments of operation results to loop variables.The result is an execu-tion schedule periodic in,the so-called initiation interval. It is well-known that a minimization of results in a min-imum loop latency[11].Despite the discrete time steps in a schedule,the initiation interval may be a rational number2.Problem descriptionIn this section we introduce our way of representing the pro-gram loops,the processor model,the objective of the loop scheduling,and the constraints resulting from the loop and the processor.Here we present a"conventional"resource constraint formulation which is given for the sake of com-pleteness and comparison with the formulations usingflow graphs(Section3).2.1.Loop and schedule representationIn this paper we restrict our attention to single loops:for toend for,(1)where are single valued operations,and are state-ments(assignments),are dependence distances be-tween statements;we use indices for iterations.We suppose that the loop has a single assignment form,i.e. each instance of a variable is assigned only once in the loop.The single assignment form may result from the appli-cation of an array dataflow analysis and a renaming of vari-ables[5].Then all data dependences areflow dependences which can be represented in a reduced dependence graph (RDG).In this RDG,the statements form the vertices ;directed edges are data depen-dences weighted by the dependence distances. The source of an edge is called and the sink.In our loop scheduling,the aim is tofind an-periodic schedule,i.e.a set of starting times:is called initiation interval. The expressionsare individual time offsets for each statement. Since we are bound to discrete time steps(clocks),we have to adapt fractional times using the operator.Example1[Loop Representation]As an example for the loop representation and the RDG,we choose the second or-der wave digitalfilter[7]shown in Figure1.The operation of statement,for example,is an addition;depends only on through variable(with a dependence distance of),since is not being assigned in the loop.2.2.Functional unitsAtfirst we have to introduce a bunch of variables describing functional units and relationships between them.A set of functional units(types)available for the computationfor toFigure1.Program code and reduced depen-dence graph of Example1of the operations of statements is supposed to be given.Theset of units that are able to execute a particular statement,is denoted as.Each functional unit is associated with a latency,defining the time between the start of the computation and the availability of the result,and with anoffset time,defining the minimum time between the start of two computations if has a pipeline architecture (otherwise).The set of statements that can be executed in is called .To implement the binding of statements to resources, wefinally introduce a function:,expressing that statement is evaluated by the functional unit if,and a function:,expressing that statement is evaluated by the instance of unit type.The number of instances of is denoted as.For convenience,we use the abbreviationsand.Example2[Functional units]Since this might have beena bit confusing,we explain the variables in a constructed example.Imagine a processor having four parts,two ALUs,one multiplier,and one adder(all non-pipelined). An ALU can execute multiplications,subtractions,and additions,the multiplier can only multiply,the Adder can add and subtract,i.e.with the statements of Ex-ample1:.Statement could then be exe-cuted by functional units.A multiplication takes three cycles in the multiplier andfour cycles in the ALU,the addition/subtraction takes al-ways one cycle:If then statement is executed in an ALU; if in addition then it is executed in the second (instance of the)ALU.2.3.Objective functionThe aim of loop scheduling is usually to minimize the looplatency:being the difference between thefirst start of a statement’sexecution and the last ending of an execution.In[11]we de-duced that the minimization of is equivalent to the mini-(5) mization of the initiation intervalwith being the cardinality of set,i.e.the number ofinstances being busy at a time must not exceed the numberof available instances.We introduce the decomposition1Modulo operator:The following conditions have to be satisfied:1.,i.e.each vertex has exactly one positive(incoming)edge and exactly one negative (outgoing)edge,2.,i.e.statements con-nected by an edge are bound to the same func-tional unit.The edges are weighted by,and the vertices by such that:(8) with4.Integer Linear ProgramIn this section we construct an Integer Linear Program to determine a scheduling with a minimum initiation interval .In the following analyses we change from a rational as a fraction of two integer variables and,to a rationalvariable.This difference was less important for thederivations in the previous sections(since a rational num-ber is the fraction of two integers),however,it brings about some advantages for the linear programming implementa-tion of our approach:We have to cope with fewer inequal-ities and(now only binary and rational)variables.We can renounce the binary decomposition of,or the treatment of as a constant,as in[6,11].Furthermore,a rational variable improves the condition of the linear program. We start at introducing an equivalent scheduling function ,which is followed by re-formulations of the causality constraints and the resource constraints for both static and cyclic binding,the latter using theflow graph approach(the "conventional"approach of cyclic binding has been dis-cussed extensively in[11]).We prove the validity of the program by showing that the constraints of Section2follow from the constraints of that program.4.1.Scheduling functionWe introduce a new scheduling function,as in[6]:(11) Theorem3With,the causality constraints(3)follow from(12).4.3.Resource constraints with static bindingFor the implementation of resource constraints with static binding(Section 2.5.1)the original formulation is lin-earized,and appropriate expressions for the binding func-tions and in(4)and for the resource constraints are found.This derivation is discussed in detail in[9].4.4.Resource constraints with aflow graph formu-lation of cyclic bindingFirst we show that a formulation of edge and vertex weights using leads to the formulation in Definition3.1. Theorem5WithWhen searching for the minimum of the objective function, will become zero if,as demanded in (13).At last we implement the edge weights.Here we use variables to express,since from(13),if,andif:(21)Similarly to(20),will become zero if,as demanded in(13).This concludes the re-formulation of the resource con-straints as a set of linear inequalities.4.5.Estimation of boundsWe have used bounds on several variables in the preceeding sections,which are now to be estimated.An upper bound of is given when the loop body of one iteration is executed sequentially,i.e.;however,this is very pessimistic. The maximum utilization of resources gives rise to a lower bound,where is a cycle in,and.The upper bound of[11],and of the flow graph implementation with a rational(Section4.4). Example4[Program:WDF](Examples1,3continued). For the scheduling of the loop shown in Figure1,a target processor having one adder(Add)and one multiplier(Mul), with latencies of one and three cycles,respectively,is sup-posed to be available.We determine the following schedul-ing functions,represented graphically in Figure2:The respectiveflow graph is shown in Figure3.Example5[Program:Calland[3]]In this example(Fig-ure4),two identical ALUs are given.The latencies for for toend forFigure4.Program code&RDG of Example5 the operations are,,, ,,and.We get the follow-ing scheduling functions,shown graphically in Figure5:Resources Iteration Iteration IterationFigure5.Schedule of Example5 Example6[Program:van Dongen[21]]In this example (Figure6)we consider two given adders having a pipeline architecture.The evaluation of an addition takes two cycles; an adder can start the next evaluation after one cycle.The resulting scheduling functions,as shown in Figure7,are:With the rational we have a schedule speed-up of 9.1%compared to the best integral solution.Let us now discuss the technical results of the three exam-ples’scheduling.We compare parameters of the linear pro-gramming solution for cyclic binding.In the case of static binding,the solution times are shorter,of course.However, the schedule of Example5has a greater latency than with cyclic binding,since idle sections are added to comply with the dependences.The results of static binding solutions are discussed in detail in[11].Example()() 4(WDF)664193(72)640.2125(Calland)1098110(144)540.1246(van Dongen)720 4.3(64)130 1.710Theflow graph formulation is better suited for a linear pro-gramming implementation than previous formulations,such that the number of variables and constraints is greatly re-duced,and the optimum solution is obtained significantly faster.The second major improvement was achieved by the treat-ment of the initiation interval as a rational variable,re-placing the integer representation of,or two separate vari-ables and,。