Fuzzy Logic Meets DSPs
Fuzzy Logic and Systems
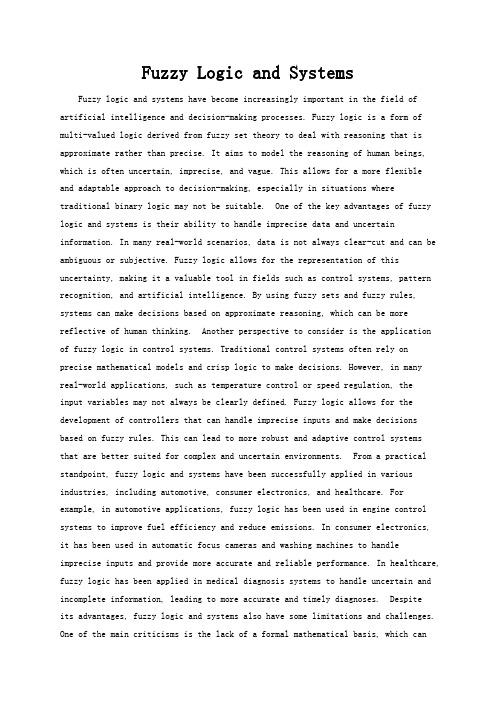
Fuzzy Logic and SystemsFuzzy logic and systems have become increasingly important in the field of artificial intelligence and decision-making processes. Fuzzy logic is a form of multi-valued logic derived from fuzzy set theory to deal with reasoning that is approximate rather than precise. It aims to model the reasoning of human beings, which is often uncertain, imprecise, and vague. This allows for a more flexibleand adaptable approach to decision-making, especially in situations wheretraditional binary logic may not be suitable. One of the key advantages of fuzzy logic and systems is their ability to handle imprecise data and uncertain information. In many real-world scenarios, data is not always clear-cut and can be ambiguous or subjective. Fuzzy logic allows for the representation of this uncertainty, making it a valuable tool in fields such as control systems, pattern recognition, and artificial intelligence. By using fuzzy sets and fuzzy rules, systems can make decisions based on approximate reasoning, which can be more reflective of human thinking. Another perspective to consider is the application of fuzzy logic in control systems. Traditional control systems often rely on precise mathematical models and crisp logic to make decisions. However, in manyreal-world applications, such as temperature control or speed regulation, theinput variables may not always be clearly defined. Fuzzy logic allows for the development of controllers that can handle imprecise inputs and make decisions based on fuzzy rules. This can lead to more robust and adaptive control systemsthat are better suited for complex and uncertain environments. From a practical standpoint, fuzzy logic and systems have been successfully applied in various industries, including automotive, consumer electronics, and healthcare. For example, in automotive applications, fuzzy logic has been used in engine control systems to improve fuel efficiency and reduce emissions. In consumer electronics,it has been used in automatic focus cameras and washing machines to handle imprecise inputs and provide more accurate and reliable performance. In healthcare, fuzzy logic has been applied in medical diagnosis systems to handle uncertain and incomplete information, leading to more accurate and timely diagnoses. Despiteits advantages, fuzzy logic and systems also have some limitations and challenges. One of the main criticisms is the lack of a formal mathematical basis, which canmake it difficult to analyze and design complex fuzzy systems. Additionally, the interpretation of fuzzy rules and membership functions can be subjective, leading to potential inconsistencies in the decision-making process. Furthermore, the computational complexity of fuzzy systems can be a challenge, especially in real-time applications where quick decisions are required. In conclusion, fuzzy logic and systems offer a valuable approach to dealing with uncertainty and imprecision in decision-making processes. By allowing for approximate reasoning and flexible control systems, fuzzy logic has found applications in a wide range of industries and has the potential to continue making significant contributions to the field of artificial intelligence. However, it is important to acknowledge the limitations and challenges associated with fuzzy logic and to continue research efforts to address these issues and further improve the effectiveness of fuzzy systems.。
Fuzzy Logic and Systems
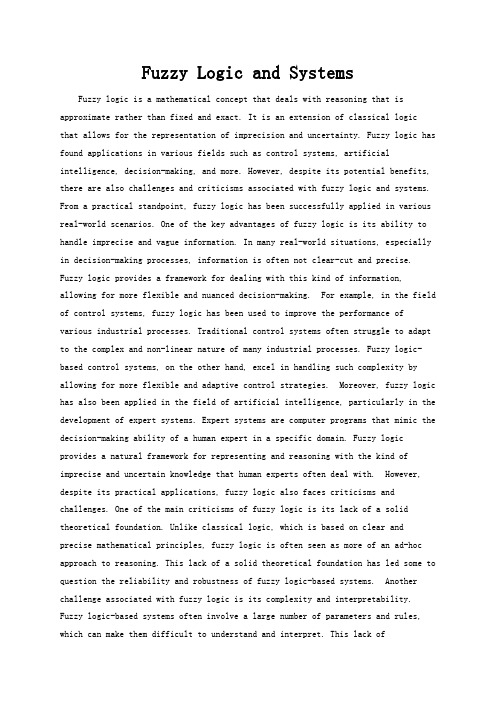
Fuzzy Logic and SystemsFuzzy logic is a mathematical concept that deals with reasoning that is approximate rather than fixed and exact. It is an extension of classical logicthat allows for the representation of imprecision and uncertainty. Fuzzy logic has found applications in various fields such as control systems, artificial intelligence, decision-making, and more. However, despite its potential benefits, there are also challenges and criticisms associated with fuzzy logic and systems. From a practical standpoint, fuzzy logic has been successfully applied in various real-world scenarios. One of the key advantages of fuzzy logic is its ability to handle imprecise and vague information. In many real-world situations, especially in decision-making processes, information is often not clear-cut and precise. Fuzzy logic provides a framework for dealing with this kind of information, allowing for more flexible and nuanced decision-making. For example, in the field of control systems, fuzzy logic has been used to improve the performance of various industrial processes. Traditional control systems often struggle to adapt to the complex and non-linear nature of many industrial processes. Fuzzy logic-based control systems, on the other hand, excel in handling such complexity by allowing for more flexible and adaptive control strategies. Moreover, fuzzy logic has also been applied in the field of artificial intelligence, particularly in the development of expert systems. Expert systems are computer programs that mimic the decision-making ability of a human expert in a specific domain. Fuzzy logic provides a natural framework for representing and reasoning with the kind of imprecise and uncertain knowledge that human experts often deal with. However, despite its practical applications, fuzzy logic also faces criticisms and challenges. One of the main criticisms of fuzzy logic is its lack of a solid theoretical foundation. Unlike classical logic, which is based on clear and precise mathematical principles, fuzzy logic is often seen as more of an ad-hoc approach to reasoning. This lack of a solid theoretical foundation has led some to question the reliability and robustness of fuzzy logic-based systems. Another challenge associated with fuzzy logic is its complexity and interpretability. Fuzzy logic-based systems often involve a large number of parameters and rules, which can make them difficult to understand and interpret. This lack oftransparency can be a significant barrier, especially in safety-critical applications where the reasoning behind a system's decisions needs to be clear and understandable. Moreover, there is also a challenge in terms of the computational overhead associated with fuzzy logic. Fuzzy logic-based systems can be computationally intensive, especially when dealing with a large amount of data and complex rule sets. This can limit the scalability and efficiency of fuzzy logic-based systems, particularly in real-time applications where quick decision-making is crucial. In conclusion, fuzzy logic and systems have both advantages and challenges. While fuzzy logic has been successfully applied in various real-world scenarios, it also faces criticisms related to its theoretical foundation, complexity, and computational overhead. Despite these challenges, fuzzy logic continues to be an important tool in dealing with imprecise and uncertain information, and ongoing research and development efforts aim to address these criticisms and further improve the practical applications of fuzzy logic.。
Fuzzy Logic and Systems

Fuzzy Logic and SystemsFuzzy logic is a type of logic that allows for the inclusion of uncertainty and imprecision in decision-making. It is an important tool in the field of artificial intelligence and has applications in various fields such as control systems, decision support systems, and pattern recognition. However, while fuzzy logic has its advantages, it also has its limitations and challenges. One of the main advantages of fuzzy logic is its ability to handle imprecise and vague information. In traditional logic, a statement is either true or false, but inreal-world situations, many statements are not black and white. Fuzzy logic allows for the representation of these shades of gray, making it a valuable tool for dealing with uncertainty. For example, in a temperature control system, fuzzylogic can be used to determine the appropriate level of heating or cooling based on imprecise inputs such as "warm" or "cool." Another advantage of fuzzy logic is its ability to model human reasoning. Human reasoning is often based on imprecise and uncertain information, and fuzzy logic provides a way to capture this reasoning process. This makes fuzzy logic a useful tool for building intelligent systems that can mimic human decision-making. However, fuzzy logic also has its limitations. One of the main challenges is the difficulty of defining fuzzy sets and rules. Unlike traditional logic, where rules are based on precise definitions, fuzzy logic rules are based on linguistic terms such as "very hot" or "slightly cold." This can make it challenging to create accurate and reliable fuzzy logic systems. Another limitation of fuzzy logic is its computational complexity. Fuzzy logic systems often require a large number of rules and a significant amount of computational power to process the fuzzy inputs and make decisions. This can make fuzzy logic systems slow and resource-intensive, especially in real-time applications. Despite these limitations, fuzzy logic has found widespread use in various fields. In control systems, fuzzy logic is used to create intelligent controllers that can adapt to changing environments and imprecise inputs. In decision support systems, fuzzy logic is used to model human reasoning and make decisions based on uncertain information. In pattern recognition, fuzzy logic is used to classify data that does not have clear boundaries. In conclusion, fuzzy logic is a powerful tool for dealing with uncertainty and imprecision in decision-making. It has advantages in its ability to handle vague information and model human reasoning, but it also has limitations in defining fuzzy sets and rules and its computational complexity. Despite these challenges, fuzzy logic has found widespread use in various fields and continues to be an important area of research in artificial intelligence and control systems.。
Fuzzy Logic and Systems
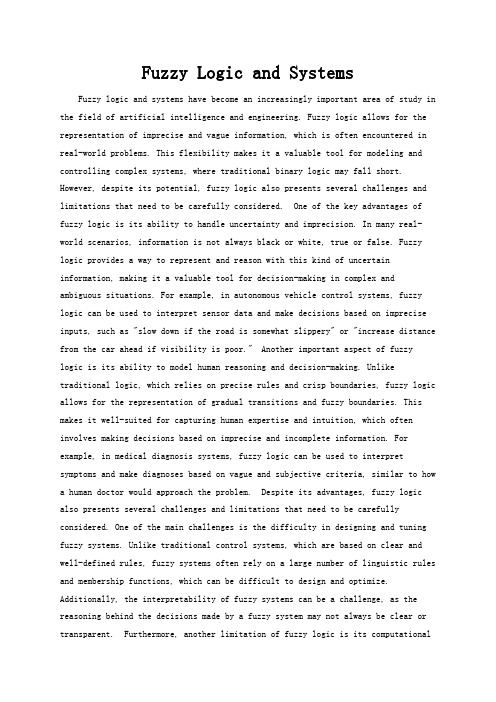
Fuzzy Logic and SystemsFuzzy logic and systems have become an increasingly important area of study in the field of artificial intelligence and engineering. Fuzzy logic allows for the representation of imprecise and vague information, which is often encountered in real-world problems. This flexibility makes it a valuable tool for modeling and controlling complex systems, where traditional binary logic may fall short. However, despite its potential, fuzzy logic also presents several challenges and limitations that need to be carefully considered. One of the key advantages of fuzzy logic is its ability to handle uncertainty and imprecision. In many real-world scenarios, information is not always black or white, true or false. Fuzzy logic provides a way to represent and reason with this kind of uncertain information, making it a valuable tool for decision-making in complex and ambiguous situations. For example, in autonomous vehicle control systems, fuzzy logic can be used to interpret sensor data and make decisions based on imprecise inputs, such as "slow down if the road is somewhat slippery" or "increase distance from the car ahead if visibility is poor." Another important aspect of fuzzylogic is its ability to model human reasoning and decision-making. Unlike traditional logic, which relies on precise rules and crisp boundaries, fuzzy logic allows for the representation of gradual transitions and fuzzy boundaries. This makes it well-suited for capturing human expertise and intuition, which often involves making decisions based on imprecise and incomplete information. For example, in medical diagnosis systems, fuzzy logic can be used to interpret symptoms and make diagnoses based on vague and subjective criteria, similar to how a human doctor would approach the problem. Despite its advantages, fuzzy logic also presents several challenges and limitations that need to be carefully considered. One of the main challenges is the difficulty in designing and tuning fuzzy systems. Unlike traditional control systems, which are based on clear and well-defined rules, fuzzy systems often rely on a large number of linguistic rules and membership functions, which can be difficult to design and optimize. Additionally, the interpretability of fuzzy systems can be a challenge, as the reasoning behind the decisions made by a fuzzy system may not always be clear or transparent. Furthermore, another limitation of fuzzy logic is its computationalcomplexity. Fuzzy systems often require a large number of rules and membership functions, which can lead to increased computational overhead. This can be particularly challenging in real-time applications, where fast decision-making is crucial. Additionally, the lack of a formal mathematical foundation for fuzzylogic can make it difficult to analyze and reason about the behavior of fuzzy systems, which can be a barrier to its widespread adoption in certain domains. In conclusion, fuzzy logic and systems offer a powerful and flexible framework for modeling and controlling complex and uncertain systems. Its ability to handle uncertainty and imprecision, as well as its capability to model human reasoning, make it a valuable tool for a wide range of applications. However, it also presents several challenges and limitations that need to be carefully considered, such as the difficulty in designing and tuning fuzzy systems, as well as its computational complexity. Despite these challenges, the potential of fuzzy logic in addressing real-world problems makes it an important area of study and research in the field of artificial intelligence and engineering.。
Fuzzy Logic and Systems
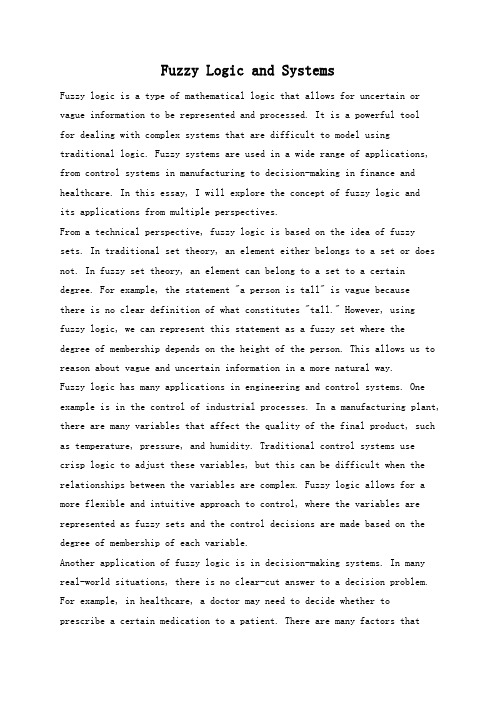
Fuzzy Logic and SystemsFuzzy logic is a type of mathematical logic that allows for uncertain or vague information to be represented and processed. It is a powerful toolfor dealing with complex systems that are difficult to model usingtraditional logic. Fuzzy systems are used in a wide range of applications, from control systems in manufacturing to decision-making in finance and healthcare. In this essay, I will explore the concept of fuzzy logic andits applications from multiple perspectives.From a technical perspective, fuzzy logic is based on the idea of fuzzy sets. In traditional set theory, an element either belongs to a set or does not. In fuzzy set theory, an element can belong to a set to a certain degree. For example, the statement "a person is tall" is vague becausethere is no clear definition of what constitutes "tall." However, using fuzzy logic, we can represent this statement as a fuzzy set where thedegree of membership depends on the height of the person. This allows us to reason about vague and uncertain information in a more natural way.Fuzzy logic has many applications in engineering and control systems. One example is in the control of industrial processes. In a manufacturing plant, there are many variables that affect the quality of the final product, such as temperature, pressure, and humidity. Traditional control systems usecrisp logic to adjust these variables, but this can be difficult when the relationships between the variables are complex. Fuzzy logic allows for a more flexible and intuitive approach to control, where the variables are represented as fuzzy sets and the control decisions are made based on the degree of membership of each variable.Another application of fuzzy logic is in decision-making systems. In many real-world situations, there is no clear-cut answer to a decision problem. For example, in healthcare, a doctor may need to decide whether toprescribe a certain medication to a patient. There are many factors thatcan influence this decision, such as the patient's medical history, age, and lifestyle. Fuzzy logic can be used to represent these factors as fuzzy sets and to make a decision based on the degree of membership of each factor.From a social perspective, fuzzy logic has the potential to improve decision-making and problem-solving in many areas. In healthcare, for example, it can help doctors make more informed decisions about treatment options. In finance, it can help investors make better decisions about which stocks to buy or sell. In education, it can help teachers design more effective learning programs for their students. However, there are also concerns about the use of fuzzy logic in decision-making. Critics arguethat it can be difficult to interpret the results of a fuzzy system, and that it may lead to decisions that are based on incomplete or biased information.From an ethical perspective, fuzzy logic raises questions about the role of technology in decision-making. Should we rely on machines to make decisions about our health, finances, and education? What are the ethicalimplications of using fuzzy logic to make decisions that affect people's lives? There is also a concern about the potential for bias in fuzzy systems. If the inputs to a fuzzy system are biased, the outputs will also be biased. This could lead to unfair or discriminatory decisions.In conclusion, fuzzy logic is a powerful tool for dealing with complex systems that are difficult to model using traditional logic. It has many applications in engineering, control systems, and decision-making. However, there are also concerns about the use of fuzzy logic in decision-making, particularly in terms of interpretability and bias. From a social perspective, fuzzy logic has the potential to improve decision-making and problem-solving in many areas, but it is important to consider the ethical implications of its use. Ultimately, the use of fuzzy logic should be guided by a commitment to fairness, transparency, and accountability.。
Fuzzy Logic and Systems

Fuzzy Logic and SystemsFuzzy logic and systems have become increasingly important in various fields, including engineering, artificial intelligence, and decision-making processes. This approach allows for a more flexible and nuanced way of dealing with uncertainty and imprecision, which are common in real-world situations. However, despite its benefits, fuzzy logic also presents some challenges and limitations that need to be carefully considered. From an engineering perspective, fuzzylogic provides a powerful tool for dealing with complex and uncertain systems. Traditional binary logic is often too rigid and unable to capture the nuances of real-world situations. Fuzzy logic, on the other hand, allows for the representation of vague concepts and gradual transitions, making it well-suitedfor applications such as control systems, pattern recognition, and decision support. In the field of artificial intelligence, fuzzy logic has also found widespread use. It enables machines to make decisions based on imprecise or incomplete information, mimicking human reasoning processes. This is particularly valuable in situations where precise rules or data are difficult to come by, such as in natural language processing or image recognition. By incorporating fuzzy logic, AI systems can better handle the uncertainties inherent in these tasks. Moreover, in the realm of decision-making, fuzzy logic offers a more natural and intuitive way of modeling human judgment. Traditional decision-making models often rely on strict rules and precise measurements, which may not accurately capture the complexity of real-life scenarios. Fuzzy logic allows decision-makers to express their preferences in a more flexible manner, taking into account multiple factors and degrees of certainty. However, despite its many advantages, fuzzy logic also has its limitations. One of the main challenges is the difficulty in defining fuzzy sets and rules in a way that accurately captures the underlying system. This process often requires a deep understanding of the specific domain and may involve a significant amount of trial and error. Additionally,interpreting and explaining fuzzy logic-based systems to non-experts can be challenging, as the results are not always straightforward and may seem counterintuitive. Another limitation of fuzzy logic is its computational complexity. While modern computing power has largely mitigated this issue, thereare still situations where the processing demands of fuzzy logic systems can be prohibitive. This is particularly relevant in real-time applications, where decisions need to be made quickly and efficiently. Balancing the expressive power of fuzzy logic with its computational cost is an ongoing challenge for researchers and practitioners. Furthermore, the subjective nature of fuzzy logic can also bea double-edged sword. While it allows for a more human-like way of reasoning, it also introduces a level of subjectivity and ambiguity that may not always be desirable. This is especially important in safety-critical systems, where the consequences of imprecise or unclear reasoning can be severe. Striking the right balance between flexibility and rigor is a key concern in the practicalapplication of fuzzy logic. In conclusion, fuzzy logic and systems offer a valuable approach to dealing with uncertainty and imprecision in various domains. Its flexibility and ability to capture the nuances of real-world situations makeit a powerful tool for engineers, AI researchers, and decision-makers. However,the challenges of defining fuzzy sets and rules, managing computational complexity, and dealing with subjectivity and ambiguity must be carefully considered. By addressing these limitations, fuzzy logic can continue to play a crucial role in addressing the complexities of the modern world.。
Fuzzy Logic and Systems
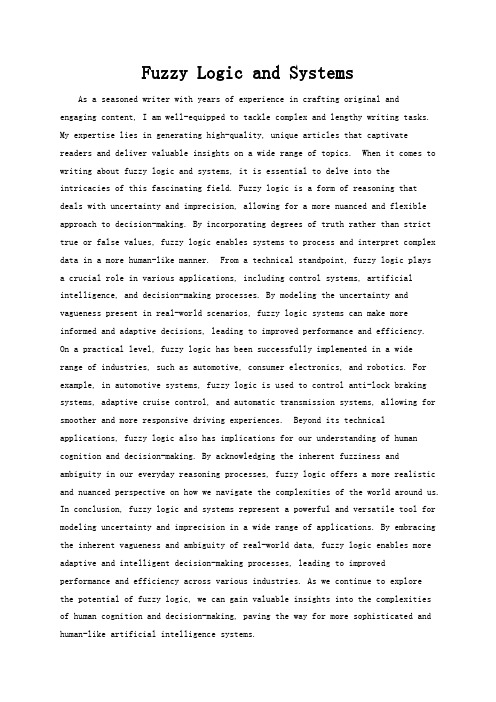
Fuzzy Logic and SystemsAs a seasoned writer with years of experience in crafting original andengaging content, I am well-equipped to tackle complex and lengthy writing tasks. My expertise lies in generating high-quality, unique articles that captivatereaders and deliver valuable insights on a wide range of topics. When it comes to writing about fuzzy logic and systems, it is essential to delve into theintricacies of this fascinating field. Fuzzy logic is a form of reasoning thatdeals with uncertainty and imprecision, allowing for a more nuanced and flexible approach to decision-making. By incorporating degrees of truth rather than strict true or false values, fuzzy logic enables systems to process and interpret complex data in a more human-like manner. From a technical standpoint, fuzzy logic playsa crucial role in various applications, including control systems, artificial intelligence, and decision-making processes. By modeling the uncertainty and vagueness present in real-world scenarios, fuzzy logic systems can make more informed and adaptive decisions, leading to improved performance and efficiency. On a practical level, fuzzy logic has been successfully implemented in a widerange of industries, such as automotive, consumer electronics, and robotics. For example, in automotive systems, fuzzy logic is used to control anti-lock braking systems, adaptive cruise control, and automatic transmission systems, allowing for smoother and more responsive driving experiences. Beyond its technical applications, fuzzy logic also has implications for our understanding of human cognition and decision-making. By acknowledging the inherent fuzziness andambiguity in our everyday reasoning processes, fuzzy logic offers a more realistic and nuanced perspective on how we navigate the complexities of the world around us. In conclusion, fuzzy logic and systems represent a powerful and versatile tool for modeling uncertainty and imprecision in a wide range of applications. By embracing the inherent vagueness and ambiguity of real-world data, fuzzy logic enables more adaptive and intelligent decision-making processes, leading to improvedperformance and efficiency across various industries. As we continue to explorethe potential of fuzzy logic, we can gain valuable insights into the complexitiesof human cognition and decision-making, paving the way for more sophisticated and human-like artificial intelligence systems.。
Fuzzy Logic and Systems
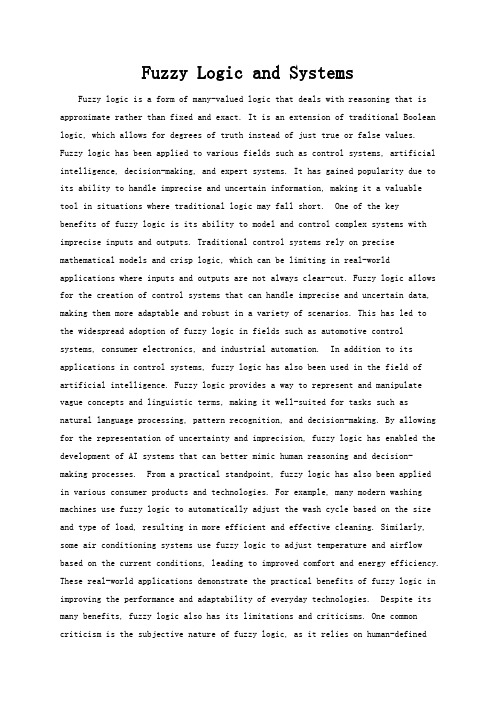
Fuzzy Logic and SystemsFuzzy logic is a form of many-valued logic that deals with reasoning that is approximate rather than fixed and exact. It is an extension of traditional Boolean logic, which allows for degrees of truth instead of just true or false values. Fuzzy logic has been applied to various fields such as control systems, artificial intelligence, decision-making, and expert systems. It has gained popularity due to its ability to handle imprecise and uncertain information, making it a valuabletool in situations where traditional logic may fall short. One of the keybenefits of fuzzy logic is its ability to model and control complex systems with imprecise inputs and outputs. Traditional control systems rely on precise mathematical models and crisp logic, which can be limiting in real-world applications where inputs and outputs are not always clear-cut. Fuzzy logic allows for the creation of control systems that can handle imprecise and uncertain data, making them more adaptable and robust in a variety of scenarios. This has led to the widespread adoption of fuzzy logic in fields such as automotive control systems, consumer electronics, and industrial automation. In addition to its applications in control systems, fuzzy logic has also been used in the field of artificial intelligence. Fuzzy logic provides a way to represent and manipulate vague concepts and linguistic terms, making it well-suited for tasks such asnatural language processing, pattern recognition, and decision-making. By allowing for the representation of uncertainty and imprecision, fuzzy logic has enabled the development of AI systems that can better mimic human reasoning and decision-making processes. From a practical standpoint, fuzzy logic has also been appliedin various consumer products and technologies. For example, many modern washing machines use fuzzy logic to automatically adjust the wash cycle based on the size and type of load, resulting in more efficient and effective cleaning. Similarly, some air conditioning systems use fuzzy logic to adjust temperature and airflow based on the current conditions, leading to improved comfort and energy efficiency. These real-world applications demonstrate the practical benefits of fuzzy logic in improving the performance and adaptability of everyday technologies. Despite its many benefits, fuzzy logic also has its limitations and criticisms. One common criticism is the subjective nature of fuzzy logic, as it relies on human-definedlinguistic variables and rules. This can lead to ambiguity and inconsistency in the design and implementation of fuzzy systems, potentially impacting their reliability and effectiveness. Additionally, the computational complexity of fuzzy logic systems can be a barrier to their widespread adoption, as they may require more resources and processing power compared to traditional crisp logic systems. In conclusion, fuzzy logic has emerged as a valuable tool for handling imprecise and uncertain information in a variety of fields. Its ability to model complex systems, handle vague concepts, and improve the performance of consumer products has made it a popular choice for engineers and researchers. While it has its limitations, the practical benefits of fuzzy logic are clear, and its continued development and application are likely to have a significant impact on the future of technology and decision-making.。
Fuzzy Logic and Systems
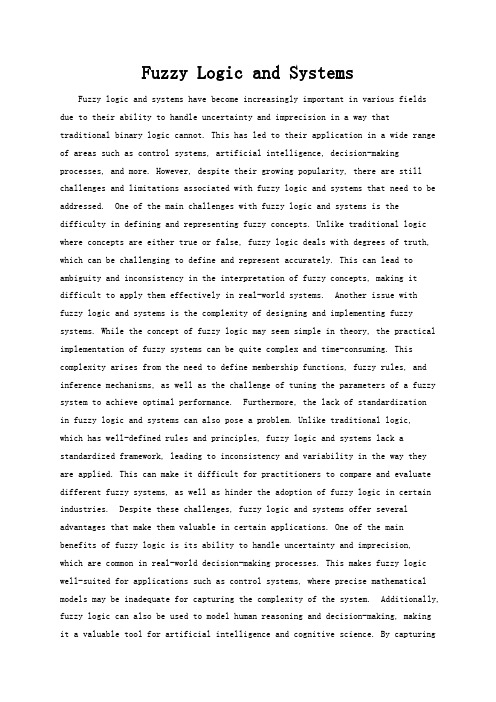
Fuzzy Logic and SystemsFuzzy logic and systems have become increasingly important in various fields due to their ability to handle uncertainty and imprecision in a way thattraditional binary logic cannot. This has led to their application in a wide range of areas such as control systems, artificial intelligence, decision-making processes, and more. However, despite their growing popularity, there are still challenges and limitations associated with fuzzy logic and systems that need to be addressed. One of the main challenges with fuzzy logic and systems is thedifficulty in defining and representing fuzzy concepts. Unlike traditional logic where concepts are either true or false, fuzzy logic deals with degrees of truth, which can be challenging to define and represent accurately. This can lead to ambiguity and inconsistency in the interpretation of fuzzy concepts, making it difficult to apply them effectively in real-world systems. Another issue with fuzzy logic and systems is the complexity of designing and implementing fuzzy systems. While the concept of fuzzy logic may seem simple in theory, the practical implementation of fuzzy systems can be quite complex and time-consuming. This complexity arises from the need to define membership functions, fuzzy rules, and inference mechanisms, as well as the challenge of tuning the parameters of a fuzzy system to achieve optimal performance. Furthermore, the lack of standardizationin fuzzy logic and systems can also pose a problem. Unlike traditional logic,which has well-defined rules and principles, fuzzy logic and systems lack a standardized framework, leading to inconsistency and variability in the way they are applied. This can make it difficult for practitioners to compare and evaluate different fuzzy systems, as well as hinder the adoption of fuzzy logic in certain industries. Despite these challenges, fuzzy logic and systems offer several advantages that make them valuable in certain applications. One of the mainbenefits of fuzzy logic is its ability to handle uncertainty and imprecision,which are common in real-world decision-making processes. This makes fuzzy logic well-suited for applications such as control systems, where precise mathematical models may be inadequate for capturing the complexity of the system. Additionally, fuzzy logic can also be used to model human reasoning and decision-making, makingit a valuable tool for artificial intelligence and cognitive science. By capturingthe nuances and uncertainties present in human cognition, fuzzy logic can help bridge the gap between human and machine intelligence, leading to more human-like and intelligent systems. In conclusion, while fuzzy logic and systems offer several advantages in handling uncertainty and imprecision, they also present challenges in defining and representing fuzzy concepts, designing and implementing fuzzy systems, and lack of standardization. Despite these challenges, the unique capabilities of fuzzy logic make it a valuable tool in various fields, and ongoing research and development efforts are aimed at addressing the limitations associated with fuzzy logic and systems. As our understanding of fuzzy logic continues to evolve, it is likely that we will see even greater adoption and application of fuzzy logic in the future.。
Fuzzy Logic and Systems

Fuzzy Logic and SystemsFuzzy logic, a fascinating realm within the field of artificial intelligence, deals with the concept of partial truth, where the truth value can range between completely true and completely false. Unlike traditional binary sets, which rely on crisp boundaries, fuzzy logic embraces ambiguity and uncertainty, mirroring the complexities of human reasoning and decision-making. At the heart of fuzzy logic lies the concept of fuzzy sets. These sets differ from classical sets in their membership function. While classical sets operate on a binary principle – an element either belongs to the set or it doesn't – fuzzy sets introduce degrees of membership. An element can partially belong to a set, with a membership value between 0 and 1, reflecting the degree to which it embodies the set's characteristics. This nuanced approach enables fuzzy logic to model conceptsthat are inherently imprecise, such as 'tall' or 'hot'. Take, for instance, the concept of temperature. In classical logic, a temperature is either 'hot' or'cold'. Fuzzy logic, however, allows for a smoother transition between these states, recognizing that there are degrees of 'hotness' and 'coldness'. A temperature might be considered 'somewhat hot' or 'fairly cold', reflecting the natural ambiguity of human perception. The implementation of fuzzy logic hinges on linguistic variables, which represent concepts with imprecise meanings. These variables are defined by fuzzy sets, each associated with a specific linguistic term. For example, the variable 'temperature' could be defined by fuzzy sets like 'cold', 'warm', and 'hot', each with its corresponding membership function, mapping temperature values to degrees of membership within the set. The application of fuzzy logic extends to various fields, notably in control systems. Fuzzy logic controllers excel in situations where precise mathematical models are challenging to develop or where human expertise plays a crucial role. Consider a washing machine that adjusts its washing cycle based on the perceived dirtiness of the clothes. Fuzzy logic enables the controller to interpret linguistic inputslike 'slightly dirty' or 'very dirty' and translate them into appropriate actions, mirroring the intuitive decision-making process of a human operator. In conclusion, fuzzy logic, with its capacity to handle uncertainty and ambiguity, offers a powerful tool for modeling complex systems and mimicking human reasoning.Its ability to bridge the gap between crisp, digital systems and the fuzzy, analog world makes it invaluable in a wide range of applications, from control systems to decision-making processes. As we continue to explore the potential of artificial intelligence, fuzzy logic stands as a testament to the power of embracing the nuanced and imprecise nature of human thought.。
Fuzzy Logic and Systems

Fuzzy Logic and SystemsFuzzy logic and systems have become increasingly important in various fields such as engineering, artificial intelligence, and decision-making processes. Fuzzy logic is a form of multi-valued logic that deals with reasoning that is approximate rather than fixed and exact. It allows for a more flexible approach to decision-making and problem-solving, taking into account the uncertainty and imprecision that often exists in real-world situations. One perspective on fuzzy logic and systems is its application in engineering. Fuzzy logic has been used in various engineering applications such as control systems, image processing, and pattern recognition. In control systems, fuzzy logic allows for the development of controllers that can handle imprecise inputs and provide more accurate andflexible control. In image processing and pattern recognition, fuzzy logic can be used to deal with the uncertainty and variability that exists in real-world images and patterns, allowing for more robust and accurate recognition and processing. Another perspective on fuzzy logic and systems is its role in artificial intelligence. Fuzzy logic provides a way to represent and reason with uncertainand imprecise information, which is essential in many AI applications. For example, in natural language processing, fuzzy logic can be used to handle the ambiguityand vagueness that often exists in human language, allowing for more accurate understanding and interpretation of text. In expert systems, fuzzy logic can be used to represent and reason with the imprecise and uncertain knowledge that experts often use in decision-making processes. Fuzzy logic and systems also play a crucial role in decision-making processes. In many real-world situations, decision-making involves dealing with imprecise and uncertain information. Fuzzy logic provides a way to model and reason with this type of information, allowingfor more flexible and accurate decision-making. For example, in financialdecision-making, fuzzy logic can be used to model and reason with the impreciseand uncertain information that often exists in financial markets, allowing for more robust and accurate decision-making. From a philosophical perspective, fuzzy logic challenges the traditional binary view of truth and falsity. It recognizes that in many real-world situations, the truth value of a statement is not simply true or false, but rather exists on a spectrum of truth. This more nuanced view oftruth and reasoning can have profound implications for our understanding of knowledge and reality, challenging traditional notions of certainty and precision. In conclusion, fuzzy logic and systems have become increasingly important in various fields such as engineering, artificial intelligence, and decision-making processes. Its ability to handle uncertainty and imprecision makes it a valuable tool for modeling and reasoning with real-world information. From engineering applications to artificial intelligence and decision-making processes, fuzzy logic provides a more flexible and accurate approach to solving complex problems. Its philosophical implications also challenge traditional views of truth and reasoning, opening up new possibilities for understanding knowledge and reality.。
Fuzzy Logic and Systems
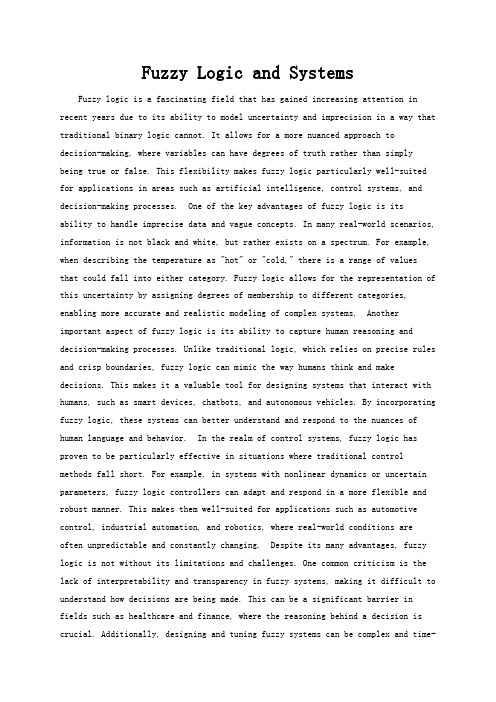
Fuzzy Logic and SystemsFuzzy logic is a fascinating field that has gained increasing attention in recent years due to its ability to model uncertainty and imprecision in a way that traditional binary logic cannot. It allows for a more nuanced approach todecision-making, where variables can have degrees of truth rather than simply being true or false. This flexibility makes fuzzy logic particularly well-suited for applications in areas such as artificial intelligence, control systems, and decision-making processes. One of the key advantages of fuzzy logic is itsability to handle imprecise data and vague concepts. In many real-world scenarios, information is not black and white, but rather exists on a spectrum. For example, when describing the temperature as "hot" or "cold," there is a range of valuesthat could fall into either category. Fuzzy logic allows for the representation of this uncertainty by assigning degrees of membership to different categories, enabling more accurate and realistic modeling of complex systems. Another important aspect of fuzzy logic is its ability to capture human reasoning and decision-making processes. Unlike traditional logic, which relies on precise rules and crisp boundaries, fuzzy logic can mimic the way humans think and make decisions. This makes it a valuable tool for designing systems that interact with humans, such as smart devices, chatbots, and autonomous vehicles. By incorporating fuzzy logic, these systems can better understand and respond to the nuances of human language and behavior. In the realm of control systems, fuzzy logic has proven to be particularly effective in situations where traditional control methods fall short. For example, in systems with nonlinear dynamics or uncertain parameters, fuzzy logic controllers can adapt and respond in a more flexible and robust manner. This makes them well-suited for applications such as automotive control, industrial automation, and robotics, where real-world conditions are often unpredictable and constantly changing. Despite its many advantages, fuzzy logic is not without its limitations and challenges. One common criticism is the lack of interpretability and transparency in fuzzy systems, making it difficult to understand how decisions are being made. This can be a significant barrier in fields such as healthcare and finance, where the reasoning behind a decision is crucial. Additionally, designing and tuning fuzzy systems can be complex and time-consuming, requiring expertise in both the domain and fuzzy logic itself. In conclusion, fuzzy logic is a powerful and versatile tool that offers a more flexible and nuanced approach to modeling uncertainty and imprecision. Its ability to capture human reasoning and decision-making processes makes it a valuable asset in a wide range of applications, from artificial intelligence to control systems. While there are challenges to overcome, the potential benefits of fuzzy logic are clear, and its continued development and application are likely to shape thefuture of technology and decision-making.。
Fuzzy Logic and Systems
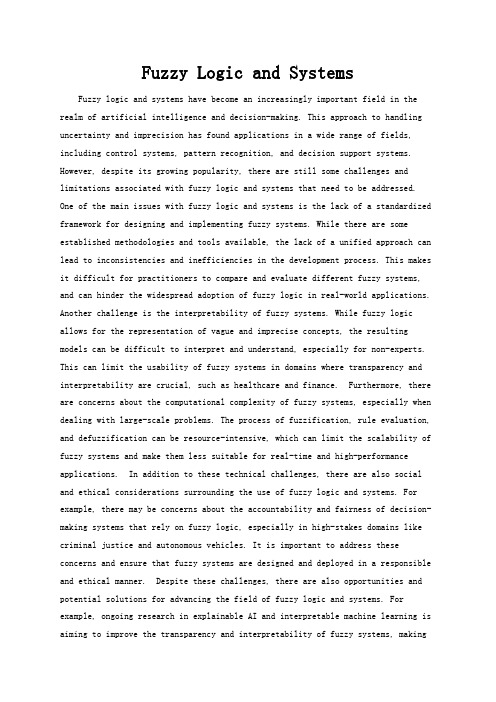
Fuzzy Logic and SystemsFuzzy logic and systems have become an increasingly important field in the realm of artificial intelligence and decision-making. This approach to handling uncertainty and imprecision has found applications in a wide range of fields, including control systems, pattern recognition, and decision support systems. However, despite its growing popularity, there are still some challenges and limitations associated with fuzzy logic and systems that need to be addressed. One of the main issues with fuzzy logic and systems is the lack of a standardized framework for designing and implementing fuzzy systems. While there are some established methodologies and tools available, the lack of a unified approach can lead to inconsistencies and inefficiencies in the development process. This makes it difficult for practitioners to compare and evaluate different fuzzy systems, and can hinder the widespread adoption of fuzzy logic in real-world applications. Another challenge is the interpretability of fuzzy systems. While fuzzy logic allows for the representation of vague and imprecise concepts, the resulting models can be difficult to interpret and understand, especially for non-experts. This can limit the usability of fuzzy systems in domains where transparency and interpretability are crucial, such as healthcare and finance. Furthermore, there are concerns about the computational complexity of fuzzy systems, especially when dealing with large-scale problems. The process of fuzzification, rule evaluation, and defuzzification can be resource-intensive, which can limit the scalability of fuzzy systems and make them less suitable for real-time and high-performance applications. In addition to these technical challenges, there are also social and ethical considerations surrounding the use of fuzzy logic and systems. For example, there may be concerns about the accountability and fairness of decision-making systems that rely on fuzzy logic, especially in high-stakes domains like criminal justice and autonomous vehicles. It is important to address these concerns and ensure that fuzzy systems are designed and deployed in a responsible and ethical manner. Despite these challenges, there are also opportunities and potential solutions for advancing the field of fuzzy logic and systems. For example, ongoing research in explainable AI and interpretable machine learning is aiming to improve the transparency and interpretability of fuzzy systems, makingthem more accessible to a wider audience. Similarly, advancements in computing technologies, such as parallel processing and cloud computing, can help alleviate the computational burden of fuzzy systems, enabling their use in more demanding applications. Moreover, the development of standardized frameworks and best practices for designing and implementing fuzzy systems can help improve consistency and efficiency in the field. By establishing common guidelines and methodologies, practitioners can more easily compare and evaluate different fuzzy systems, leading to better quality and more reliable applications. In conclusion, while fuzzy logic and systems offer a powerful approach to handling uncertainty and imprecision, there are still challenges and limitations that need to be addressed. From technical concerns about interpretability and scalability tosocial and ethical considerations, there are various aspects that require attention. However, with ongoing research and advancements in technology, there are opportunities to overcome these challenges and further advance the field of fuzzy logic and systems. It will be crucial for researchers, practitioners, and policymakers to work together to address these issues and ensure the responsible and effective use of fuzzy systems in diverse applications.。
Fuzzy Logic and Systems
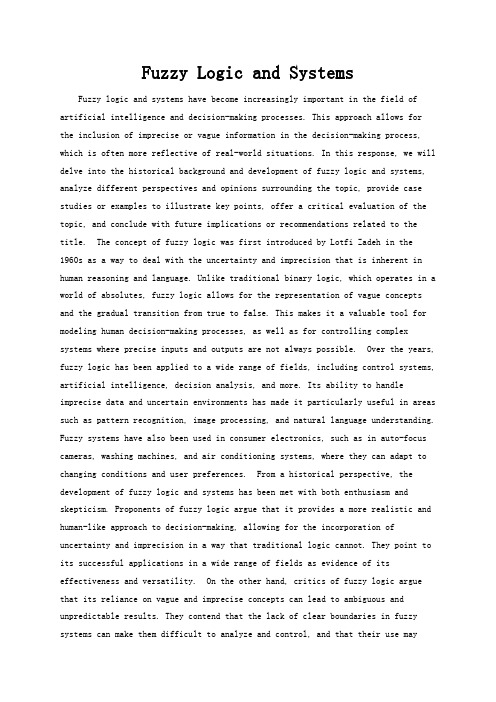
Fuzzy Logic and SystemsFuzzy logic and systems have become increasingly important in the field of artificial intelligence and decision-making processes. This approach allows for the inclusion of imprecise or vague information in the decision-making process, which is often more reflective of real-world situations. In this response, we will delve into the historical background and development of fuzzy logic and systems, analyze different perspectives and opinions surrounding the topic, provide case studies or examples to illustrate key points, offer a critical evaluation of the topic, and conclude with future implications or recommendations related to the title. The concept of fuzzy logic was first introduced by Lotfi Zadeh in the 1960s as a way to deal with the uncertainty and imprecision that is inherent in human reasoning and language. Unlike traditional binary logic, which operates in a world of absolutes, fuzzy logic allows for the representation of vague concepts and the gradual transition from true to false. This makes it a valuable tool for modeling human decision-making processes, as well as for controlling complex systems where precise inputs and outputs are not always possible. Over the years, fuzzy logic has been applied to a wide range of fields, including control systems, artificial intelligence, decision analysis, and more. Its ability to handle imprecise data and uncertain environments has made it particularly useful in areas such as pattern recognition, image processing, and natural language understanding. Fuzzy systems have also been used in consumer electronics, such as in auto-focus cameras, washing machines, and air conditioning systems, where they can adapt to changing conditions and user preferences. From a historical perspective, the development of fuzzy logic and systems has been met with both enthusiasm and skepticism. Proponents of fuzzy logic argue that it provides a more realistic and human-like approach to decision-making, allowing for the incorporation of uncertainty and imprecision in a way that traditional logic cannot. They point to its successful applications in a wide range of fields as evidence of its effectiveness and versatility. On the other hand, critics of fuzzy logic argue that its reliance on vague and imprecise concepts can lead to ambiguous and unpredictable results. They contend that the lack of clear boundaries in fuzzy systems can make them difficult to analyze and control, and that their use maylead to a loss of rigor and precision in decision-making processes. However, it is important to note that these criticisms have not prevented the widespread adoption of fuzzy logic and systems in various industries and academic fields. One example of the successful application of fuzzy logic is in the field of automotive engineering. Fuzzy logic has been used to develop advanced driver assistance systems (ADAS), which can interpret and respond to ambiguous or uncertain situations on the road. For example, a fuzzy logic system can analyze sensor data to determine the appropriate level of braking force in response to changing road conditions, traffic patterns, and driver behavior. This allows for a more adaptive and human-like approach to vehicle control, improving safety and performance in a wide range of driving scenarios. Another example of the use of fuzzy logic is in the field of medical diagnosis. Fuzzy systems have been applied to interpret diagnostic test results and patient symptoms, allowing for the consideration of uncertain or ambiguous information in the diagnostic process. This can lead to more accurate and nuanced diagnoses, particularly in cases where traditional binary logic may struggle to account for the complexity and variability of human health. Despite its successful applications, fuzzy logic and systems are not without their drawbacks. One of the main criticisms of fuzzy logic is its lack of transparency and interpretability. Unlike traditional logic, which operates on clear rules and principles, fuzzy systems can be difficult to understand and analyze, particularly as they become more complex. This can lead to challenges in validating and verifying the behavior of fuzzy systems, as well as in communicating their decisions to end-users or stakeholders. Another potential drawback of fuzzy logic is its reliance on expert knowledge and input. Fuzzy systems often require the development of rules and membership functions based on the expertise of human operators, which can be subjective and difficult to formalize. This can lead to challenges in maintaining and updating fuzzy systems, particularly as the underlying domain knowledge evolves or changes over time. Looking ahead, the future implications of fuzzy logic and systems are likely to be shaped by ongoing advancements in artificial intelligence, machine learning, and data science. As these fields continue to evolve, there is an opportunity to integrate fuzzy logic with other approaches, such as deep learning andreinforcement learning, to create more robust and adaptive decision-making systems. This may involve the development of hybrid models that combine the strengths of fuzzy logic with the scalability and automation of machine learning, allowing for the representation of uncertainty and imprecision in large-scale and complex environments. In conclusion, fuzzy logic and systems have become an important and influential approach to decision-making and control in a wide range of fields. While they have been met with both enthusiasm and skepticism, their successful applications in areas such as automotive engineering and medical diagnosis demonstrate their effectiveness and versatility. However, it is important to acknowledge the potential drawbacks of fuzzy logic, including challenges in transparency and interpretability, as well as in the reliance on expert knowledge. Looking ahead, the future implications of fuzzy logic are likely to be shaped by ongoing advancements in artificial intelligence and machine learning, offering opportunities to create more robust and adaptive decision-making systems. As the field continues to evolve, there is potential for fuzzy logic to play a key rolein addressing the challenges of uncertainty and imprecision in complex and dynamic environments.。
Fuzzy Logic and Systems
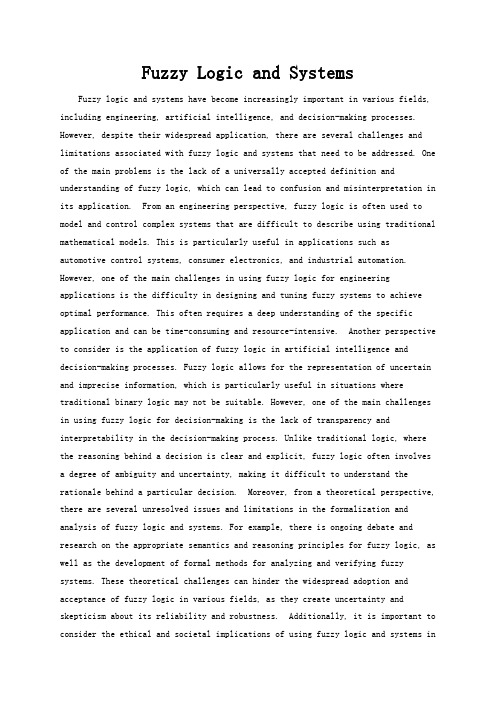
Fuzzy Logic and SystemsFuzzy logic and systems have become increasingly important in various fields, including engineering, artificial intelligence, and decision-making processes. However, despite their widespread application, there are several challenges and limitations associated with fuzzy logic and systems that need to be addressed. One of the main problems is the lack of a universally accepted definition and understanding of fuzzy logic, which can lead to confusion and misinterpretation in its application. From an engineering perspective, fuzzy logic is often used to model and control complex systems that are difficult to describe using traditional mathematical models. This is particularly useful in applications such as automotive control systems, consumer electronics, and industrial automation. However, one of the main challenges in using fuzzy logic for engineering applications is the difficulty in designing and tuning fuzzy systems to achieve optimal performance. This often requires a deep understanding of the specific application and can be time-consuming and resource-intensive. Another perspective to consider is the application of fuzzy logic in artificial intelligence and decision-making processes. Fuzzy logic allows for the representation of uncertain and imprecise information, which is particularly useful in situations where traditional binary logic may not be suitable. However, one of the main challenges in using fuzzy logic for decision-making is the lack of transparency and interpretability in the decision-making process. Unlike traditional logic, where the reasoning behind a decision is clear and explicit, fuzzy logic often involves a degree of ambiguity and uncertainty, making it difficult to understand the rationale behind a particular decision. Moreover, from a theoretical perspective, there are several unresolved issues and limitations in the formalization and analysis of fuzzy logic and systems. For example, there is ongoing debate and research on the appropriate semantics and reasoning principles for fuzzy logic, as well as the development of formal methods for analyzing and verifying fuzzy systems. These theoretical challenges can hinder the widespread adoption and acceptance of fuzzy logic in various fields, as they create uncertainty and skepticism about its reliability and robustness. Additionally, it is important to consider the ethical and societal implications of using fuzzy logic and systems indecision-making processes. The use of fuzzy logic in applications such as autonomous vehicles, medical diagnosis, and financial forecasting can have significant impacts on human lives and well-being. Therefore, it is crucial to ensure that fuzzy systems are designed and used in a responsible and ethical manner, taking into account the potential risks and consequences of their decisions. In conclusion, while fuzzy logic and systems offer many benefits and opportunities in various fields, there are also several challenges and limitations that need to be addressed. From engineering and practical applications to theoretical and ethical considerations, it is important to carefully evaluate the use of fuzzy logic and systems and work towards overcoming the obstacles that may hinder their successful implementation. By addressing these challenges, we can harness the full potential of fuzzy logic and systems while ensuring their reliability, transparency, and ethical use.。
Fuzzy Logic and Systems
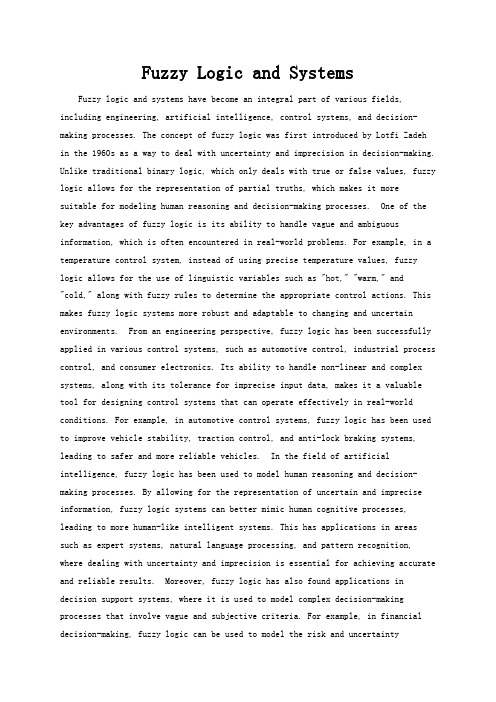
Fuzzy Logic and SystemsFuzzy logic and systems have become an integral part of various fields, including engineering, artificial intelligence, control systems, and decision-making processes. The concept of fuzzy logic was first introduced by Lotfi Zadeh in the 1960s as a way to deal with uncertainty and imprecision in decision-making. Unlike traditional binary logic, which only deals with true or false values, fuzzy logic allows for the representation of partial truths, which makes it moresuitable for modeling human reasoning and decision-making processes. One of the key advantages of fuzzy logic is its ability to handle vague and ambiguous information, which is often encountered in real-world problems. For example, in a temperature control system, instead of using precise temperature values, fuzzy logic allows for the use of linguistic variables such as "hot," "warm," and "cold," along with fuzzy rules to determine the appropriate control actions. This makes fuzzy logic systems more robust and adaptable to changing and uncertain environments. From an engineering perspective, fuzzy logic has been successfully applied in various control systems, such as automotive control, industrial process control, and consumer electronics. Its ability to handle non-linear and complex systems, along with its tolerance for imprecise input data, makes it a valuable tool for designing control systems that can operate effectively in real-world conditions. For example, in automotive control systems, fuzzy logic has been used to improve vehicle stability, traction control, and anti-lock braking systems, leading to safer and more reliable vehicles. In the field of artificial intelligence, fuzzy logic has been used to model human reasoning and decision-making processes. By allowing for the representation of uncertain and imprecise information, fuzzy logic systems can better mimic human cognitive processes, leading to more human-like intelligent systems. This has applications in areas such as expert systems, natural language processing, and pattern recognition, where dealing with uncertainty and imprecision is essential for achieving accurate and reliable results. Moreover, fuzzy logic has also found applications in decision support systems, where it is used to model complex decision-making processes that involve vague and subjective criteria. For example, in financial decision-making, fuzzy logic can be used to model the risk and uncertaintyassociated with investment decisions, allowing for more informed and robust decision-making processes. Similarly, in medical diagnosis, fuzzy logic can be used to handle the uncertainty and imprecision associated with symptoms and test results, leading to more accurate and reliable diagnoses. Despite its many advantages, fuzzy logic also has its limitations and challenges. One of the main criticisms of fuzzy logic is its lack of a solid mathematical foundation, whichhas led to concerns about its reliability and robustness in certain applications. Additionally, designing fuzzy logic systems can be complex and time-consuming, requiring a deep understanding of the problem domain and the appropriate selection of linguistic variables and fuzzy rules. Moreover, the interpretability of fuzzy logic systems can be a challenge, as the reasoning and decision-making processes are often not transparent, which can be a concern in safety-critical applications. In conclusion, fuzzy logic and systems have become an important tool for dealing with uncertainty and imprecision in various fields. Its ability to handle vagueand ambiguous information, along with its applications in engineering, artificial intelligence, and decision support systems, has made it a valuable and versatile tool for modeling complex real-world problems. However, its lack of a solid mathematical foundation, complexity in design, and challenges in interpretability are important factors to consider when applying fuzzy logic in practical applications. Nonetheless, the potential for fuzzy logic to improve the robustness and adaptability of intelligent systems makes it a promising area for further research and development.。
Fuzzy Logic and Systems

Fuzzy Logic and SystemsFuzzy logic and systems have become increasingly important in various fields, including engineering, artificial intelligence, and decision-making processes. The concept of fuzzy logic was first introduced by Lotfi Zadeh in the 1960s as a wayto deal with the uncertainty and imprecision that often exist in real-world problems. Unlike traditional binary logic, which only deals with true or false values, fuzzy logic allows for the representation of partial truths, which makesit more suitable for modeling human reasoning and decision-making. One of the key advantages of fuzzy logic is its ability to handle vague and ambiguous information, which is common in many real-world applications. This is particularly useful in areas such as control systems, where precise mathematical models may not always be available or practical. By allowing for the representation of uncertain and imprecise information, fuzzy logic can help improve the performance and robustness of control systems, leading to more efficient and reliable outcomes. In addition to its applications in control systems, fuzzy logic has also found use in fields such as pattern recognition, image processing, and natural language processing. In these areas, the ability of fuzzy logic to handle uncertainty and imprecision can be particularly valuable, as it allows for more flexible and human-like reasoning. For example, in image processing, fuzzy logic can be used to model humanperception and reasoning, leading to more accurate and natural-looking results. However, despite its many advantages, fuzzy logic also has its limitations and challenges. One of the main criticisms of fuzzy logic is its reliance onsubjective input from experts, which can lead to inconsistencies and biases in the modeling process. Additionally, the computational complexity of fuzzy logic systems can be a barrier to their widespread adoption, particularly in real-time applications where speed is crucial. Another potential challenge for fuzzy logicis its lack of a solid theoretical foundation, which can make it difficult to analyze and understand the behavior of fuzzy systems. This has led to some skepticism and criticism from traditional logicians and mathematicians, who argue that fuzzy logic is not based on a rigorous mathematical framework. Despite these challenges, the potential benefits of fuzzy logic and systems are significant, and ongoing research and development in this area continue to expand theirapplicability and effectiveness. As technology continues to advance, the ability of fuzzy logic to handle uncertainty and imprecision will become increasingly valuable, particularly in the context of emerging technologies such as autonomous vehicles, robotics, and smart systems. In conclusion, fuzzy logic and systems have emerged as a powerful and versatile tool for dealing with uncertainty and imprecision in various real-world applications. While they have their limitations and challenges, the potential benefits of fuzzy logic in improving the performance and flexibility of control systems, as well as in other areas such as pattern recognition and natural language processing, make them a valuable area of research and development. As technology continues to advance, the ability of fuzzy logic to model human-like reasoning and decision-making will become increasingly important, making it an area of study with significant potential for future impact.。
Fuzzy Logic and Systems

Fuzzy Logic and SystemsFuzzy Logic and Systems have become increasingly popular in various fields due to their ability to handle uncertainty and imprecision. This technology is basedon the concept of fuzzy sets, which allows for a more flexible and realistic representation of data. In this response, I will discuss the importance of fuzzy logic and systems from multiple perspectives. From a technical perspective, fuzzy logic and systems offer several advantages. Firstly, they provide a powerful tool for dealing with imprecise and uncertain information. Unlike traditional binary logic, which only allows for true or false values, fuzzy logic allows for degrees of truth. This means that it can handle situations where the boundaries between true and false are blurred, enabling a more nuanced representation of data. Furthermore, fuzzy logic and systems are particularly useful in situations where human expertise and intuition are required. By using linguistic variables andfuzzy rules, these systems can model human reasoning and decision-making processes. This makes them highly suitable for applications such as expert systems, where human-like decision-making is desired. From a practical perspective, fuzzy logic and systems have a wide range of applications. One notable example is in the field of control systems. Fuzzy control systems can handle complex and nonlinear systems, making them suitable for controlling processes that are difficult to model mathematically. This has led to their use in various industries, including automotive, aerospace, and manufacturing. Another important application of fuzzy logic and systems is in the field of artificial intelligence (AI). Fuzzy systems can be used to model uncertain and imprecise knowledge, making them useful inareas such as natural language processing and machine learning. By incorporating fuzzy logic into AI algorithms, we can enhance their ability to handle real-world data and make more accurate predictions. From a societal perspective, fuzzy logic and systems have the potential to improve decision-making processes and enhance human-machine interactions. By incorporating fuzzy logic into decision support systems, we can provide more realistic and flexible recommendations to users. This can be particularly valuable in domains such as healthcare, finance, and transportation, where decisions often involve uncertainty and multiple conflicting objectives. Furthermore, fuzzy logic can help bridge the gap between human andmachine reasoning. By modeling human-like decision-making processes, fuzzy systems can make machines more understandable and easier to interact with. This can lead to increased trust and acceptance of AI technologies, as users can better understand and relate to the decision-making processes employed by these systems. In conclusion, fuzzy logic and systems offer numerous advantages from technical, practical, and societal perspectives. Their ability to handle uncertainty and imprecision makes them a valuable tool in various fields, including control systems, artificial intelligence, and decision support systems. By incorporating fuzzy logic into these applications, we can improve their performance, enhance human-machine interactions, and make more informed decisions. As the demand for intelligent systems continues to grow, the importance of fuzzy logic and systems will only increase in the future.。
- 1、下载文档前请自行甄别文档内容的完整性,平台不提供额外的编辑、内容补充、找答案等附加服务。
- 2、"仅部分预览"的文档,不可在线预览部分如存在完整性等问题,可反馈申请退款(可完整预览的文档不适用该条件!)。
- 3、如文档侵犯您的权益,请联系客服反馈,我们会尽快为您处理(人工客服工作时间:9:00-18:30)。
Fuzzy Logic Meets DSPs ____________________________________________IntroductionThe concept of fuzzy logic was born in 1965 when Lotfi Zadeh, a professor at University of California Berkeley published a paper on fuzzy sets. Fuzzy logic is based on imprecision, specifically, the way peo-ple make decisions on imprecise, and non-numerical information. Fuzzy logic mimics the way humans make decisions using linguistic reasoning. In a later paper, Zadeh introduced the concept of linguistic variables.Linguistic variables are described by words, rather than a value like normal Boolean variables. Forinstance, the linguistic variable "temperature" may have "cold," "medium," and "hot" defining its range of values. Combining linguistic variables with "IF-THEN" rules paved the way for implementing the theory to control real applications.Fuzzy ControlFuzzy logic uses a different approach than conventional controllers. Conventional Proportional, Integral,and Differential (PID) controllers model the desired system or process being controlled. Alternatively, in a fuzzy logic controller, it is the human operator's behavior that is modeled. The PID controller uses a set of differential equations to analytically model the system. It is the solution to these equations that tells the PID controller how to adjust the system. In a fuzzy controller, adjustments are handled by a fuzzy rule-based expert system (an expert system is a logical model of an expert human operator's reasoning to con-trol the system). The shift in focus from the process to the operator changes the whole approach to control problems.A typical fuzzy controller is composed of membership functions, rules, and a defuzzification function.Membership functions form the basis of a fuzzy logic controller. They are used to convert non-fuzzy data to fuzzy data. Moreover, membership functions are a set of fuzzy variables. For example, a range of tem-peratures may be represented by a fuzzy variable subset "cold," "medium," and "hot." These three subsets are mapped onto the entire range of the variable "temperature." This mapping produces a fuzzified mem-bership function. Figure 1 shows the membership set for the variable "temperature."I P Impatiens Publications4028 Pleasant Avenue SouthMpls, MN 55409-1545 (612) 822-5985e-mail: impub@ White Paper1Cold Medium HotFigure 1Fuzzy membership set for thevariable "termperature."Fuzzy controller inference rules take the form of "IF variable x is 'positive large,' THEN change the control variable y by the amount 'negative large.'" Inference rules actually represent a collection of several rules or compositional rule of inference. The IF portion of the rule is called the consequent, and the statement following the THEN is called the conclusion. For example, a rule that says if the error of a controller and the error's rate of change are "positive small," then the controlling variable is set to "negative medium." Figure 2 illustrates this compositional rule. Note that this rule may implicitly represent another rule like "positive large" error and rate of change for a "negative large" change in control.The resulting fuzzy output must be defuzzified to produce a non-fuzzy or “crisp” output. The crisp output is determined by evaluating all the activated "THEN" clauses of each rule (also referred to as "fired" rules), and applying a defuzzification function to them. The most commonly used defuzzification function is the centroid function. The centroid function is also called the center-of-gravity function. This function produces a median crisp value from all the fired rules. Specifically, it works by summing all fired conclu-sions and mapping the weighted sum to the output variable's membership function to produce a crisp out-put. Figure 3 shows the centroid defuzzification based on Figure 2.A fuzzy logic controller or fuzzy engine code has the advantage of being shorter than their PID controllers. In some cases they only require 250 bytes of code to implement a two input, one output controller. This translates into less cost for computing and faster response times than traditional controllers.As you can see, fuzzy controllers are much easier to read and understand than using a set of differential equations! Additionally, fuzzy controllers are simpler than classical controllers. That is because they can tolerate some imprecision when dealing with the desired system. This ease of use translates into lower costs and faster time to market. That is why so many companies are using fuzzy logic controllers in their applications.00++++----Zero error Small positive error Medium negative Small negative Figure 2A compositional fuzzy rule.0+-Actual output value:Figure 3Centroid based defuzzification.Real-World Fuzzy Logic ApplicationsFrom Zadeh's seminal paper, which has been translated into thousands of fuzzy-based applications over the past 40 years, fuzzy systems have found their way into products ranging from anti-lock brakes to washing machines. The reason for the widespread use of fuzzy logic lies in that they are easier to design than con-ventional PID controllers, and cheaper to produce as well.Fuzzy logic is widely used in Japan. Alternatively, in the US, fuzzy logic has been branded with "impre-cise" and thus has a stigma associated with it. On the contrary, fuzzy logic is often more precise than PID systems. For instance, compared to PID in motor control, fuzzy logic is easier and more accurate than tra-ditional implementations when dealing with non-linear control. Thus, many US companies quietlyacknowledge this, and have been building devices that make use of fuzzy logic for the past decade.Many international and Fortune 500 companies currently use fuzzy logic. Applications are not limited only to control. Fuzzy applications are used in a wide range of these companies’products. The following is a partial list of applications using fuzzy logic:Automatic TransmissionAnti-lock Braking System (ABS)ThermostatsAuto-focus CamcordersAnti-jitter CamcordersSecure Credit Card TransactionsServo Motor ControllersProgrammable Logic Controllers (PLCs)Advanced AvionicsAutomated Beverage Can InspectionWashing MachinesElevator ControllersAutomobile Engine Idle ControlInformation Retrieval SystemsRefrigerator Defrost SystemsAir ConditionersFuzzy Shower ControllersSubway ControllersFuzzy Stock Trading SystemsPilot-less V oice-Controlled HelicopterCement Kiln ControllersConclusionSpectrum Digital provides a complete fuzzy logic development system for motor/motion control. The FLSK includes the eZdsp TM development board, two CD ROMs, an article on the implementation of a fuzzy logic controller, the book: Design & Development of Fuzzy Logic Controllers, and the TeachFuzz Fuzzy logic Simulator.The FLSK is an ideal development environment for fuzzy logic control applications such as motor and motor/motion control. Developing a fuzzy logic controller is as simple as describing the system using English IF-THEN rules, simulating the fuzzy system using TeachFuzz, transferring the tuned fuzzy engine to the C-code template, tailoring the C-code to support application specifics, compiling the code using CCS, downloading object code to the eZdsp TM target, and debugging the application. The FLSK provides you with an easy and flexible method to create control applications. The TMS320F2812 is an excellent choice for developing a wide range of applications due to its high-level of integration, 150 MIPs perfor-mance, and its excellent development tools.Fuzzy Logic DefinitionsCentroid calculation function:Used for producing an exact output value by calculating the center of gravity of the union of areas bound by membership functions and the input variable axes.Defuzzificaton:a general method for determining the best exact or "crisp" output of a given fuzzy set. The defuzzification method uses the centroid calculation function or a similar function to generate a crisp output.Fuzzy Control System:Control system based on fuzzy IF-THEN rules that utilizes fuzzy sets for input and out-put.Fuzzy Inference System:A collection of fuzzy IF-THEN rules.Fuzzy Logic: Logic that uses linguistic variables to describe a system. Examples include: "fast," "slow," and "medium."Fuzzy Set: Any set that allows its members to have different "grades" of membership. Each member may be expressed by a continuous number between zero and one: [0,1]. This contrasts to Boolean logic that limits set members to a value of either zero or one.Fuzzy System: A system whose variables range over states that are fuzzy sets.Membership Function: The mapping of a fuzzy set that associates each set member with its grade of member-ship.ReferencesMiller, Byron. The Design and Development of Fuzzy Logic Controllers. Minneapolis, MN: Impatiens Publications, 1997V on Altrock, Constantin. Fuzzy Logic and NeuroFuzzy Applications Explained. Englewood Cliffs, NJ: Prentice Hall, 1995.Brubaker, David. "Fuzzy-logic basics: intuitive rules replace complex math," EDN June 18, 1992, p. 111. Brubaker, David. "Fuzzy-logic system solves control problem," EDN June 18, 1992, p. 121.Brubaker, David. "Design and simulate your own fuzzy setpoint controller," EDN January 5, 1995, p. 167. Brubaker, David. "The expert called in sick," EDN October 12, 1995, p. 207.Corbin, Jerry. "A Fuzzy Logic-Based Financial Transaction System," ESP, December 1994, p. 24.Klir, George & Schwartz Daniel. "Fuzzy logic flowers in Japan, IEEE Spectrum JULY1992, p. 32.Miller, Byron. "A Top-Down Approach to Fuzzy Logic Design," Embedded Systems Programming, July 1998, p. 52.Miller, Byron. "Fuzzy Logic Does Real Time on the DSP," DDJ, July 2004, p. 25.Sibigtroth, Jim. "Exploring New Fuzzy Logic Secrets," Embedded Systems Programming, August 1995, p. 44. Simon, Dan. "Fuzzy Control," ESP July, 2003, p. 55.Slutsker, Gary. "Why fuzzy logic is good business," Forbes, May 13, 1991, p. 120.。