Semantic search of unstructured data using contextual network graphs
(完整版)图情常规术语(中英文对照)
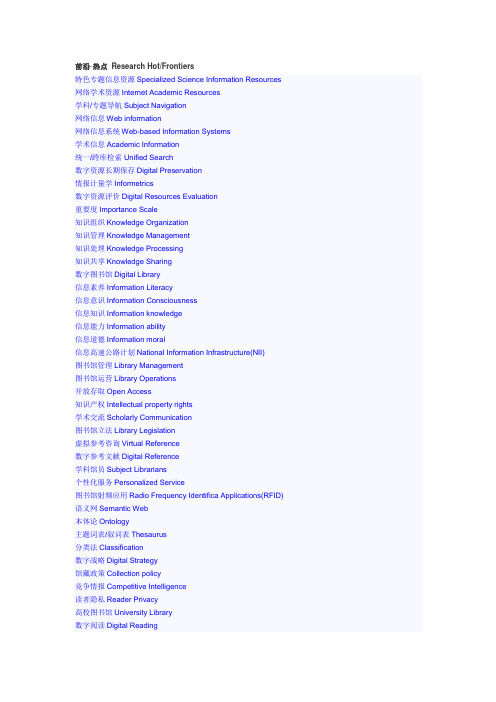
前沿·热点Research Hot/Frontiers特色专题信息资源Specialized Science Information Resources 网络学术资源Internet Academic Resources学科/专题导航Subject Navigation网络信息Web information网络信息系统Web-based Information Systems学术信息Academic Information统一/跨库检索Unified Search数字资源长期保存Digital Preservation情报计量学Informetrics数字资源评价Digital Resources Evaluation重要度Importance Scale知识组织Knowledge Organization知识管理Knowledge Management知识处理Knowledge Processing知识共享Knowledge Sharing数字图书馆Digital Library信息素养Information Literacy信息意识Information Consciousness信息知识Information knowledge信息能力Information ability信息道德Information moral信息高速公路计划National Information Infrastructure(NII)图书馆管理Library Management图书馆运营Library Operations开放存取Open Access知识产权Intellectual property rights学术交流Scholarly Communication图书馆立法Library Legislation虚拟参考咨询Virtual Reference数字参考文献Digital Reference学科馆员Subject Librarians个性化服务Personalized Service图书馆射频应用Radio Frequency Identifica Applications(RFID)语义网Semantic Web本体论Ontology主题词表/叙词表Thesaurus分类法Classification数字战略Digital Strategy馆藏政策Collection policy竞争情报Competitive Intelligence读者隐私Reader Privacy高校图书馆University Library数字阅读Digital Reading图像信息Image Information档案网站Archives website数字档案Digital Archive信息集成Information Integration社会网络分析Social Network Analysis网络图书馆Network Library整合性图书馆系统Integrated Library System图书馆联盟Library consortia复合图书馆Hybrid Library图书漂流Bookcrossing链接分析Link analysis信息伦理Information Ethics信息检索Information Retrieval信息安全Information Security信息构建Information Architecture捐赠政策Donation Policy图书馆学Library science图书Books期刊Journals/Periodicals报纸Newspapers百科全书Encyclopedia信息资源Information resources知识Knowledge道德规范ethics图书馆服务library service交叉学科interdisciplinary science美国图书馆运动American Library Movement图书馆学学术课程Academic courses in library science 采集管理Collection management信息系统技术Information systems and technology编目分类Cataloging and Classification保藏Preservation参考咨询Reference统计管理Statistics and management数据库管理Database management情报建设Information architecture知识管理Knowledge Management图书馆学分支学科Subdisciplines in library science人类情报行为Human Information Behaviors知识组织Knowledge Organization数字图书馆Digital libraries采集开发Collection development个人信息管理Personal information management(PIM)保存Preservation公共参考咨询Public reference and other services学术交流Scholarly communication信息计量学informetrics科学计量学scientometrics图书馆职位类型Types of library science professionals图书管理员Librarian档案保管员Archivist编目员Cataloger图书馆馆长Curator编索引员Indexers文摘员Abstractors研究员Researchers信息设计师Information architect信息代理商Information broker元数据设计师Metadata Architects元数据经理Metadata Managers保护员Conservators图书馆相关刊物Current issues in LIS图书馆员教育Education for librarianshipInformation policy信息交流技术Information communication technologies (ICT's) Information Society阅览公平Equity of AccessSustainability and ICT's儿童互联网保护法规Children's Internet Protection Act审查制度Censorship信息爆炸Information explosion信息扫盲Information literacy政府信息Government Information复制权Copyright知识产权Intellectual property rights知识自由Intellectual freedom数字分水岭Digital divide开架阅览Open accessPatriot Act公共借阅权Public lending rightSerials crisisCurrent digital/scanning technologies远程存取Remote access数字图书馆Digital libraries信息检索系统information retrieval system电子信息系统Electronic Information System数字格式digital formats数字参考文献Digital Reference计算机获取accessible by computers电子图书馆electronic library电子书eBooks虚拟图书馆virtual library有声读物audiobooks原生数字born-digital数字化digitizing物理收藏physical collections数字收藏digital collections美国记忆American Memory数字档案Internet Archive电子出版ePrint电子书目ibiblio珀尔修斯项目Project Perseus古藤堡项目Project Gutenbergsearch engines元数据Metadata牛津文本档案馆Oxford Text Archive光学符号识别optical character recognition深度链接资源deep web resources/invisible web搜索引擎蜘蛛search engine crawlersOAI-PMH协议Open Archives Initiative Protocol for Metadata Harvesting (OAI-PMH) Z39.50协议Z39.50网络计量学Webmetrics情报学(信息学)Information science/Informatics迭代过程iterative design processes美国医学图书馆National Library of MedicineDialogCompuserve特殊利益团体Special interest groupsPaul OtletHenri La Fontaine文献计量学Bibliometrics影响因子Journal Impact Factor重要度Importance Scale网页Rank值PageRank数据建模Data modeling数据模型理论data model theory数据模型实例data model instance数据库模型database model非结构化数据unstructured data文档管理Document management电子档案electronic documents电子图像electronic images群件Groupware社交软件Social softwareonline dating services社交网Social networksFriendster计算机支持协作computer-supported collaboration人机交互Human-computer interactionMan-machine interaction (MMI)Computer-human interaction (CHI)用户界面User interface情报建设Information architecture结构化信息structuring information用户体验设计user experience design信息系统设计information system design易用性usability信息伦理Information ethics隐私Privacy信息生命周期life-cycle of information所有权ownership安全security获取access公众community信息检索Information retrieval信息过载information overload单机数据库stand-alone databases超文本数据库hypertextually-networked databases数据检索data retrieval文档检索document retrieval文本检索text retrieval信息社会Information society知识经济knowledge economy后工业化社会post-industrial society知识社会knowledge society网络社会network society知识管理Knowledge management知识工程Knowledge engineering个人信息管理Personal Information Management语义网Semantic web网页内容web content自然语言natural language软件代理software agentsTim Berners-Lee资源描述框架Resource Description Framework (RDF)数据交换格式data interchange formats网页本体语言Web Ontology Language (OWL)用户为中心设计User-centered design可扩展标记语言XMLW3C标记语言markup language标准标记语言Standard Generalized Markup Language (SGML) RSSMathMLGraphMLXHTML可升级向量制图法Scalable Vector GraphicsMusicXML。
Exalead引领基于搜索应用的革命_达索

Exalead引领基于搜索应用的革命Exalead CloudView TMEnablement Discovery & Search致力于发现与搜索About Exalead®›Exalead®于2000年由搜索引擎先驱创立,现已成长为一家向企业和网络提供信息访问软件的全球供应商。
每月,全球250多家公司,1亿名有效用户使用Exalead的CloudView平台对信息资产进行搜索、查找和管理,部分用户包括桑格研究院(Sanger Institute)、泽思博(Jaspersoft)、普华永道、赛诺菲-安万特(Sanofi-Aventis)、捷富凯(Gefco)、标致雪铁龙集团、ViaMichelin、AFP、CSC(计算机科学公司)、黄页集团(Yellow PagesGroup)和美国国防部等。
›目前,Exalead正引领着搜索型应用(SBA)革命,以令人难以置信的简便性和较低的总体拥有成本,为异构企业信息云中的数据赋予全新的结构、意义和可访问性。
在SBA开发方面,Exalead与Capgemini、Sogeti、Logica、Keyrus、ST Groupe和Business & Decision开展了密切的协作。
›Exalead于2010年被达索系统并购。
Exalead在巴黎、旧金山、格拉斯哥、伦敦、阿姆斯特丹、米兰和法兰克福等地均设有办事处。
›更多信息请访问:/software。
3AgendaSearch Chronology 搜索技术发展史Point to point SQL search结构化查询语言搜索Keyword searchBrowsingDemocratized19701980199020002010Search (R)Evolution (演变与创新)?An extended CRM ? A WW community ? A geo-spatial search ?A cutting edge technology to search in non-textual format ? A smart operational BI tool ?SBA Revolution…Your favorite searching platform present every moment of your life…With or without “search bar”…Your favorite computing device presentevery moment of your life…AgendaExalead Desktop TM 从桌面即时进入所有数据Exalead CloudView TMConnecting the dots in the enterprise data cloud for 360°information accessExalead CloudView非并行结构内容检索开放的SOA 架构严谨的安全策略简单并强大的管理高效,可控Widget LibraryReorganise widgets by drag & dropContextual help and suggestions for variablesand propertiesWidget ConfigurationUse Previously defined data feeds inwidgetsWidget ,中文译名被称作是微件,是一小块可以在任意一个基于HTML 的Web 页面上执行的代码,它的表现形式可能是视频,地图,新闻,小游戏等等›Extracted from the CRM, mail databases , websites…›Dynamic ranking based on business rules to present the most relevant information›Latest Events and Tasks to complete, related to AcmeGlobal information on an account extracted from the CRM systemGlobal information on a company extracted from SalesforceRelated documents hosted on the filesystem of your companyCurrent status of the opportunities for that accountSearch for anyinformation (contact, account…)Customer ProjectsAgendaJim›美国人›毕业于哈佛大学›伦敦商学院MBARoger ›法国人…两人均›掌管电子制造业公司超过20年›有不俗的业务增长›吞并了多家竞争对手›拥有相似的IT环境….ITIT Eco SystemBoth need Marketing› 6 analysts are visiting distribution channels›2% of channel coverage = market representative›Analyse competition›Analyse pricing›Analyse merchandising impact ›Invest heavily in customer survey›Reports are generated every 6 months to adapt the strategy ›Is monitoring every channel web site ›Covers 80% of the industry›Monitors new competitive products and pricing trends›Is monitoring social network tounderstand consummers expectations ›Build competitive strategy in real time15 名熟练人员 2 个决策人员Sales›Have an army of talented field sales ›Have a sophisticated salesenablement program›Have a sales incentives program ›Have an active online strategy›Consolidate customer data from every channel and online business›Use web technology to automate›Recommendation›Cross sell›Up sell›ProfilingBoth need CRMCover 2 millionscustomers in 3 geography with a team of45 peoples !Covers 20 millionscustomers in 23 geographies with a team of 3 peoples and 1 million euro in IT automationBoth need Decisional›Have invested in Business object›Have reduced reporting latency from 1 month to standardized weekly format ›Every Wednesday executive meeting analyze the reports and takeappropriate decision to solve theproblems ›Every field manager access every data needed to solve operational issues›Channels have immediate notification of any issues and possible workaroundHave increase channel satisfaction by 5%People have the right tool to take decision at the right time…Satisfaction of customer has been double…Implication of employs have been triple and revenue has been increasedfrom 15%IT›Which he bought in the time he could afford it in the mid 80’sROIInvest 3M€peryearInvest 300K€per yearJim needs a Ferrari !!!From Jim’s perspective his problem is very simple.It’s just about …But IT guys keep saying it’s NOT POSSIBLE(or at least too expensive)Getting some data ….And produce some nice screen with : …With easy navigation, search, etc…CRM recordsComments on the web Customer’s billTea Pot Design SheetsFeedbacks sessions transcripts …ListsPie-ChartsSummariesPicturesSearch Box Pie-Charts Tag Clouds…well any kind of dataJim’s philosophy : Spaghetti IT …Source SystemsAccess CRM DWBudgetDelivery SystemsSiebelSAP JDEScala Oracle PSMappingData WarehouseODSBusiness AnalystBIAnalyst SRO ReportMDMProduct InformationAccelerator ERP Client.DocExcelFIDMCustomerisbABCCRMCIMCR MODSOracleBIOff-line analysisRCKHow Exalead Revolutionizes ITLarge number of usersEase of use, traffic scalabilityUsersLimited number of usersUsage complexity, production costsDedicated resourcesDatamarts, additional hardwareHeavy one ‐shot developmentAgile applicationsSimple data access, use of standardweb technologiesGeneric data layerReal time data, high performance queryingStructured dataAll dataConnectors, structuration of dataStandard ArchiJim’s Architecture Roger’s ArchitectureAgility灵活Flexible, Agile,Days vs Months Performance性能Real time, millions of end-users, Terabytes of informationUsability可用性360°, Google-like, interactive,conversationalExalead Value Proposal : Our unique DNAHow can Exalead CloudView™help ?Perfect SBAComplete DIYWhen to SBA and not to SBA›Beware that a SBA is not› A replacement for your transactional applications 业务程序• A SBA won’t manage your workflows, lifecycles, and won’t modify theexisting systems› A good excuse to drop your business intelligence software • A SBA goal is not produce the pixel perfect highly complex final reportyou have to submit to the SEC(证券交易委员会)›Replace all your complex, historical business systems • A SBA goal is not to reproduce all the business logic of existingapplications. It’s to simplify it for information access›A SBA solves critical business issues by enablingeasy Search & Discovery into key data by key users 关键人员通过搜索关键数据来解决危急的业务问题Exalead CloudView›Exalead CloudView is a fully packaged platform to build SBA(Search Based Applications)面向服务的体系结构(Service-Oriented Architecture,SOA)Search Based Applications Key Characteristics-SBA 特性SEARCH AS YOUTYPEENRICH / STRUCTUREMERGE EXPLORE YOUR DATABASEFEDERATE / 360°OPERATIONAL REPORTING32Auto suggestionlanguage identificationspell checker related terms related queries 询问Synonyms 同义词cross language Lemmatization 异形词ontology Matcher 存在匹配faceted search Phonetic 语音学Data sourcesAuto Suggestion ExampleAuto completion ofuser query as they typeDB administrator is defined as synonym of Database Administrator This synonymy can be in one direction or both waysLemmatization...Cross Language Information RetrievalAfghanistanOntology Matcher : add an alternative indexing form to a string spanFor example, wecan use theontology matcherso that searchingany postal codewill also search forany citiesmatching thatcode.How to explore a database ?Traditional search on database iscomplicated:-Several fields to fill in-Need to know field valuesNatural language query on DatabaseExalead CloudView allows NaturalLanguage queries on structured dataInfer semantic from your database 从你的数据库里进行语义推断132Tabular facets46sentiment analysisnamed entityQueryMatcherFastRulesHTMLRelevantContextExtractorClustererCategorizerA powerful semantic analysis layerData sourcesProcessing PipelinesOver 300+ file formatDatenormalization,Metadataprocessing54languagessupportedSplit words,detect end ofphrase, …Detect wordtypes (noun,verb, …)Determinelemma andstem of thewordAssociate Synonyms for predefinedtokens Extract People,Location,Organization, …DetermineimportantKeywordsUse todeterminesimilardocuments,documents ofsame subjectmatters, …OthersSemanticprocessorssuch asSentimentAnalysis, …Based on yourown thesaurusGet the most out of your content Indexing SideRules Matcher Named Entitiesimage of use?Sentiment Analyzer。
sadea解析 -回复
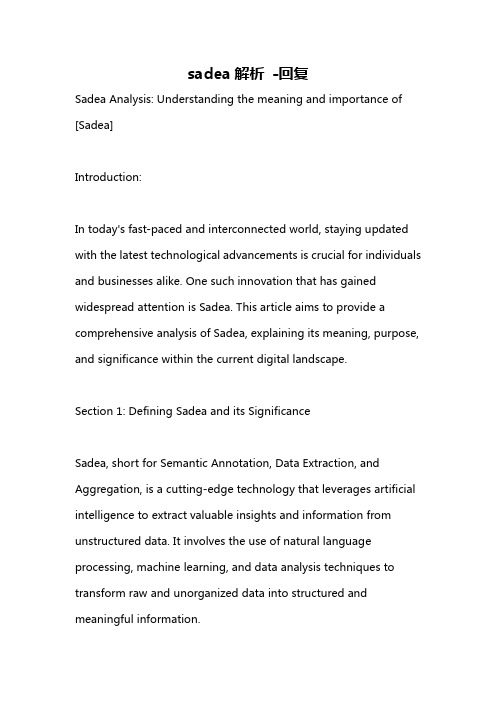
sadea解析-回复Sadea Analysis: Understanding the meaning and importance of [Sadea]Introduction:In today's fast-paced and interconnected world, staying updated with the latest technological advancements is crucial for individuals and businesses alike. One such innovation that has gained widespread attention is Sadea. This article aims to provide a comprehensive analysis of Sadea, explaining its meaning, purpose, and significance within the current digital landscape.Section 1: Defining Sadea and its SignificanceSadea, short for Semantic Annotation, Data Extraction, and Aggregation, is a cutting-edge technology that leverages artificial intelligence to extract valuable insights and information from unstructured data. It involves the use of natural language processing, machine learning, and data analysis techniques to transform raw and unorganized data into structured and meaningful information.The significance of Sadea lies in its ability to make sense of vast amounts of unstructured data that are generated daily. Unstructured data includes text, images, audio, and video files, which are often difficult to analyze due to their complex nature. By utilizing Sadea, businesses, researchers, and individuals can make data-driven decisions, gain insights into consumer behavior, develop innovative solutions, and enhance overall performance.Section 2: Key Components of SadeaSadea consists of three main components: semantic annotation, data extraction, and aggregation. Each component plays a crucial role in maximizing the potential of unstructured data.2.1 Semantic Annotation: This component focuses on understanding the meaning and context of individual data elements. By applying semantic tags or labels to unstructured data, Sadea enables machines to comprehend the content in a more human-like manner. This process involves techniques like named entity recognition, sentiment analysis, and topic modeling.2.2 Data Extraction: The data extraction component employs various algorithms and machine learning models to extract relevant information from unstructured data sources. By identifying patterns, keywords, and entities, Sadea can extract valuable insights and categorize data based on specific criteria. This automated extraction process saves time and resources for businesses and researchers, enabling them to focus on analyzing insights rather than spending time manually extracting data.2.3 Data Aggregation: The final component of Sadea involves aggregating the extracted data into a structured format. This allows for easy data integration and analysis. It combines data from multiple sources, eliminates redundancy, and presents the information in a manner that is understandable and actionable.Section 3: Real-World Applications of SadeaThe applications of Sadea are diverse and span across various industries. Some notable applications include:3.1 Business Intelligence: Sadea enables businesses to gather and analyze customer feedback, social media sentiments, andcompetitive intelligence. By extracting and aggregating data from multiple sources, businesses can make informed decisions regarding product development, marketing strategies, and customer engagement.3.2 Financial Analysis: Sadea can analyze financial reports, news articles, and industry trends to provide insights for investment decisions. By identifying patterns, trends, and anomalies in the data, financial analysts can make more accurate predictions and recommendations.3.3 Healthcare Research: Sadea has the potential to revolutionize healthcare research by extracting and analyzing patient records, medical literature, and clinical trials. This can lead to improved disease diagnosis, personalized treatment plans, and advancements in medical research.Section 4: Challenges and Future DirectionsDespite its promising capabilities, Sadea also faces several challenges. The most significant challenge lies in handling the vast amount of unstructured data being generated every day. Moreover,ensuring the accuracy and reliability of the extracted information is crucial.To overcome these challenges, ongoing research and development efforts are required. Future directions for Sadea include improving natural language processing algorithms, enhancing data visualization techniques, and developing robust data privacy and security measures.Conclusion:Sadea, with its semantic annotation, data extraction, and aggregation capabilities, has emerged as a powerful technology to extract insights from unstructured data. Its significance lies in its ability to transform raw information into structured and actionable intelligence. By leveraging Sadea, businesses, researchers, and individuals can gain a competitive advantage, make data-driven decisions, and foster innovation. As research and development efforts continue, Sadea is expected to play an increasingly vital role in the growth and success of organizations across variousindustries.。
一种神经机器翻译中稀有词模糊语义表示方法
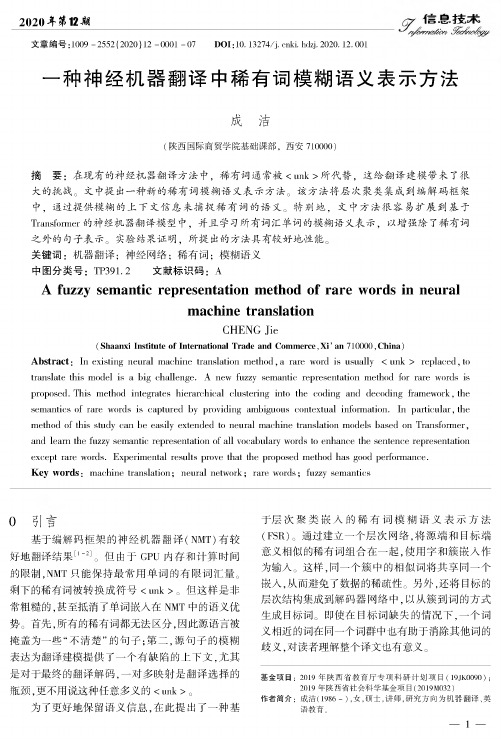
2020耳第12剧文章编号:1009-2552(2020)12-0001-07DOI:10.13274/ki.hdzj.2020.12.001一种神经机器翻译中稀有词模糊语义表示方法成洁(陕西国际商贸学院基础课部,西安710000)摘要:在现有的神经机器翻译方法中,稀有词通常被<unk>所代替,这给翻译建模带来了很大的挑战。
文中提出一种新的稀有词模糊语义表示方法。
该方法将层次聚类集成到编解码框架中,通过提供模糊的上下文信息来捕捉稀有词的语义。
特别地,文中方法很容易扩展到基于Transformer的神经机器翻译模型中,并且学习所有词汇单词的模糊语义表示,以增强除了稀有词之外的句子表示。
实验结果证明,所提出的方法具有较好地性能。
关键词:机器翻译;神经网络;稀有词;模糊语义中图分类号:TP391.2文献标识码:AA fuzzy semantic representation method of rare words in neuralmachine translationCHENG Jie(Shaanxi Institute of International Trade and Commerce,Xi'an710000,China) Abstract:In existing neural machine translation method,a rare word is usually<unk>replaced,to translate this model is a big challenge.A new fuzzy semantic representation method for rare words is proposed.This method integrates hierarchical clustering into the coding and decoding framework,the semantics of rare words is captured by providing ambiguous contextual information.In particular,the method of this study can be easily extended to neural machine translation models based on Transformer, and learn the fuzzy semantic representation of all vocabulary words to enhance the sentence representation except rare words.Experimental results prove that the proposed method has good performance.Key words:machine translation;neural network;rare words;fuzzy semantics0引言基于编解码框架的神经机器翻译(NMT)有较好地翻译结果[l-2]o但由于GPU内存和计算时间的限制,NMT只能保持最常用单词的有限词汇量o 剩下的稀有词被转换成符号<unk>。
OpenText IDOL自然语言问答系统介绍说明书
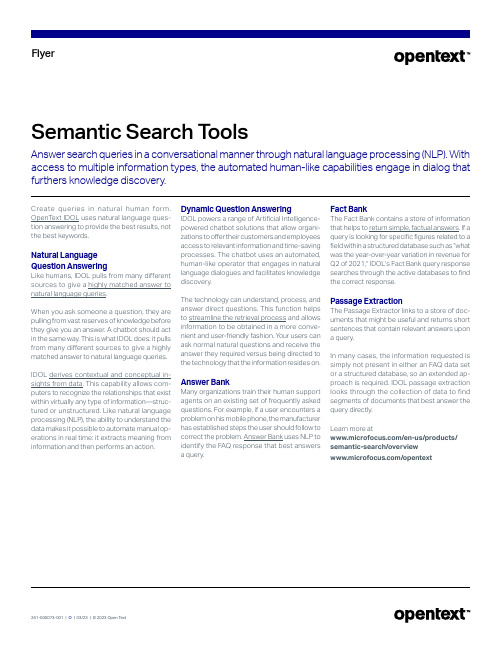
FlyerSemantic Search T oolsAnswer search queries in a conversational manner through natural language processing (NLP). With access to multiple information types, the automated humanlike capabilities engage in dialog that furthers knowledge discovery.Create queries in natural human form. OpenT ext IDOL uses natural language question answering to provide the best results, not the best keywords.Natural LanguageQuestion AnsweringLike humans, IDOL pulls from many different sources to give a highly matched answer to natural language queries.When you ask someone a question, they are pulling from vast reserves of knowledge before they give you an answer. A chatbot should act in the same way. This is what IDOL does: it pulls from many different sources to give a highly matched answer to natural language queries. IDOL derives contextual and conceptual insights from data. This capability allows computers to recognize the relationships that exist within virtually any type of information—structured or unstructured. Like natural language processing (NLP), the ability to understand the data makes it possible to automate manual operations in real time: it extracts meaning from information and then performs an action.Dynamic Question AnsweringIDOL powers a range of Artificial Intelligence-powered chatbot solutions that allow organizations to offer their customers and employeesaccess to relevant information and timesavingprocesses. The chatbot uses an automated,humanlike operator that engages in naturallanguage dialogues and facilitates knowledgediscovery.The technology can understand, process, andanswer direct questions. This function helpsto streamline the retrieval process and allowsinformation to be obtained in a more convenient and userfriendly fashion. Y our users canask normal natural questions and receive theanswer they required versus being directed tothe technology that the information resides on.Answer BankMany organizations train their human supportagents on an existing set of frequently askedquestions. For example, if a user encounters aproblem on his mobile phone, the manufacturerhas established steps the user should follow tocorrect the problem. Answer Bank uses NLP toidentify the FAQ response that best answersa query.Fact BankThe Fact Bank contains a store of informationthat helps to return simple, factual answers. If aquery is looking for specific figures related to afield within a structured database such as “whatwas the yearoveryear variation in revenue forQ2 of 2021,” IDOL’s Fact Bank query responsesearches through the active databases to findthe correct response.Passage ExtractionThe Passage Extractor links to a store of documents that might be useful and returns shortsentences that contain relevant answers upona query.In many cases, the information requested issimply not present in either an FAQ data setor a structured database, so an extended approach is required. IDOL passage extractionlooks through the collection of data to findsegments of documents that best answer thequery directly.Learn more at/en-us/products/semantic-search/overview/opentext 261-000073-001 | O | 03/23 | © 2023 Open T ext。
ieee transaction on knowledge en under review
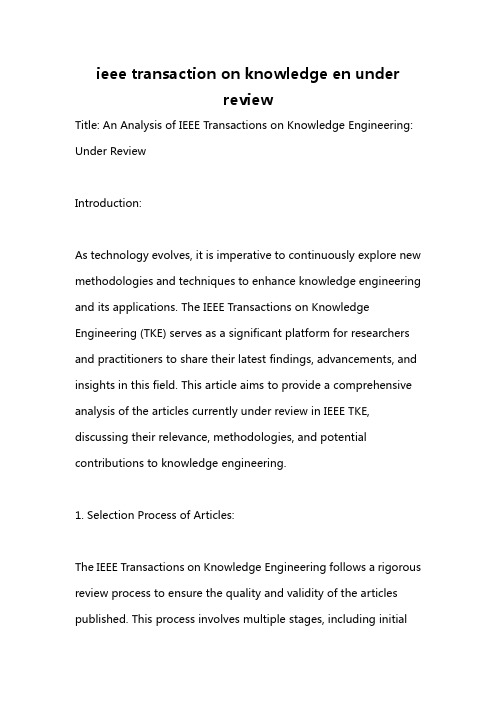
ieee transaction on knowledge en underreviewTitle: An Analysis of IEEE Transactions on Knowledge Engineering: Under ReviewIntroduction:As technology evolves, it is imperative to continuously explore new methodologies and techniques to enhance knowledge engineering and its applications. The IEEE Transactions on Knowledge Engineering (TKE) serves as a significant platform for researchers and practitioners to share their latest findings, advancements, and insights in this field. This article aims to provide a comprehensive analysis of the articles currently under review in IEEE TKE, discussing their relevance, methodologies, and potential contributions to knowledge engineering.1. Selection Process of Articles:The IEEE Transactions on Knowledge Engineering follows a rigorous review process to ensure the quality and validity of the articles published. This process involves multiple stages, including initialsubmission, peer review, revision, and final decision by the editorial board. The articles currently under review have already passed the initial screening and are presently being evaluated by subject matter experts in the field of knowledge engineering.2. Research Topics and Relevance:The articles under review cover a wide range of topics related to knowledge engineering. These topics encompass various domains, such as natural language processing, machine learning, information retrieval, expert systems, ontology engineering, and semantic web technologies. The research topics reflect contemporary challenges and opportunities in advancing the field of knowledge engineering, addressing real-world problems and paving the way for innovative solutions.3. Methodological Approaches:The articles demonstrate diverse methodological approaches to enhance knowledge engineering. Some articles propose novel algorithms and models to improve existing techniques, while others explore the integration of different methodologies, such asmachine learning and ontological modeling. Several studies also focus on data collection and preprocessing techniques, considering the vital role of quality data in knowledge engineering endeavors. Additionally, a subset of the articles focuses on evaluating the performance and efficiency of existing knowledge engineering techniques.4. Contributions to Knowledge Engineering:The articles under review make significant contributions to the field of knowledge engineering. They bring forth new insights, algorithms, and methodologies that can enhance knowledge representation, reasoning, and decision-making processes. Some articles propose novel approaches to extract knowledge from large-scale unstructured data, empowering organizations to harness valuable information and drive data-informed decisions. Others investigate the development of intelligent systems that can adapt and learn from user interactions, ultimately improving the user experience and the accuracy of information retrieval.5. Future Directions:The articles currently under review pave the way for future research directions in knowledge engineering. They shed light on the potential for further advancements by combining different disciplines, such as artificial intelligence, cognitive science, and human-computer interaction. The integration of emerging technologies, such as deep learning and natural language processing, is also highlighted as a promising avenue for knowledge engineering. These future directions will foster the development of more intelligent and efficient knowledge engineering systems, enabling better decision-making, enhanced information retrieval, and improved user experiences.Conclusion:The articles currently under review in IEEE Transactions on Knowledge Engineering demonstrate the commitment of researchers and practitioners towards advancing the field. The diverse research topics, methodological approaches, and potential contributions discussed in this article emphasize the importance of continuous exploration and innovation in knowledge engineering. By bridging the gap between theory and practice, these articlesprovide invaluable insights that can shape the future of knowledge engineering, promoting advancements in various domains and enabling the development of intelligent systems capable of managing and utilizing knowledge effectively.。
Literature Review 英文文献综述模板
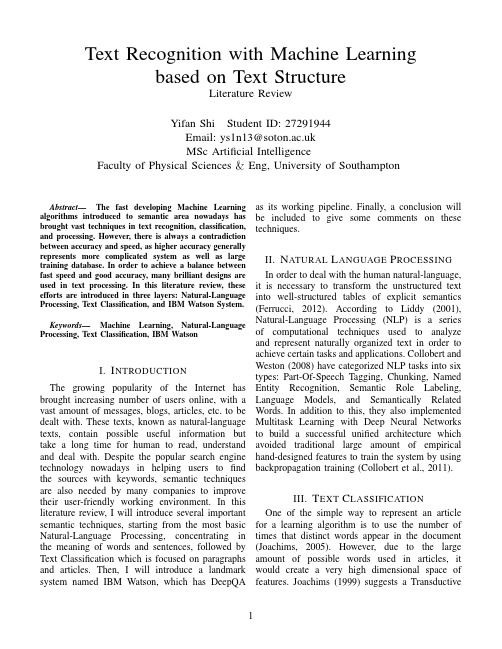
Text Recognition with Machine Learning based on Text StructureLiterature ReviewYifan Shi Student ID:27291944Email:ys1n13@MSc Artificial IntelligenceFaculty of Physical Sciences&Eng,University of SouthamptonAbstract—The fast developing Machine Learning algorithms introduced to semantic area nowadays has brought vast techniques in text recognition,classification, and processing.However,there is always a contradiction between accuracy and speed,as higher accuracy generally represents more complicated system as well as large training database.In order to achieve a balance between fast speed and good accuracy,many brilliant designs are used in text processing.In this literature review,these efforts are introduced in three layers:Natural-Language Processing,Text Classification,and IBM Watson System.Keywords—Machine Learning,Natural-Language Processing,Text Classification,IBM WatsonI.I NTRODUCTIONThe growing popularity of the Internet has brought increasing number of users online,with a vast amount of messages,blogs,articles,etc.to be dealt with.These texts,known as natural-language texts,contain possible useful information but take a long time for human to read,understand and deal with.Despite the popular search engine technology nowadays in helping users tofind the sources with keywords,semantic techniques are also needed by many companies to improve their user-friendly working environment.In this literature review,I will introduce several important semantic techniques,starting from the most basic Natural-Language Processing,concentrating in the meaning of words and sentences,followed by Text Classification which is focused on paragraphs and articles.Then,I will introduce a landmark system named IBM Watson,which has DeepQA as its working pipeline.Finally,a conclusion will be included to give some comments on these techniques.II.N ATURAL L ANGUAGE P ROCESSING In order to deal with the human natural-language, it is necessary to transform the unstructured text into well-structured tables of explicit semantics (Ferrucci,2012).According to Liddy(2001), Natural-Language Processing(NLP)is a series of computational techniques used to analyze and represent naturally organized text in order to achieve certain tasks and applications.Collobert and Weston(2008)have categorized NLP tasks into six types:Part-Of-Speech Tagging,Chunking,Named Entity Recognition,Semantic Role Labeling, Language Models,and Semantically Related Words.In addition to this,they also implemented Multitask Learning with Deep Neural Networks to build a successful unified architecture which avoided traditional large amount of empirical hand-designed features to train the system by using backpropagation training(Collobert et al.,2011).III.T EXT C LASSIFICATIONOne of the simple way to represent an article for a learning algorithm is to use the number of times that distinct words appear in the document (Joachims,2005).However,due to the large amount of possible words used in articles,it would create a very high dimensional space of features.Joachims(1999)suggests a TransductiveSupport Vector Machines to do classification because of its effective learning ability even in high dimensional feature space.Rather than using non-linear Support Vector Machine(SVM), Dumais et al.(1998)compared linear SVM with another four different learning algorithms which are Find Similar,Decision Trees,Naive Bayes, and Bayes Nets,which also supports SVM in text classification because of its high accuracy,fast speed as well as its simple model.Sebastiani(2002) also recommends Neural Network as a potential selection in text classification in that its accuracy is only slightly lower than SVM in comparison. The cross-document comparison of small pieces of text,using linguistic features such as noun phrases,and synonyms is introduced by Hatzivassiloglou et al.(1999).The similarity of two paragraphs is defined by the same action conducted on the same object by the same actor. Therefore,drawing features according to nouns and verbs would generally conclude a paragraph into several primitive elements.In addition to the similar primitive elements,restrictions such as ordering, distances and primitive(matching noun and verb pairs)are also implemented to exclude weakly related features.The feature selection methods can effectively reduce the dimensions of dataset (Ikonomakis,2005)while keeping the performance of classification.To make sure which words are to be kept,an Evaluation function has been introduced by Soucy and Mineau(2003)to measure how much information we can get by classifying through a single word.Another improvement by Han et al. (2004)is to use Principal Component Analysis (PCA)to reduce the dimension in transformation of features.Nigam and Mccallum(2000)combine Expectation-Maximization and Naive Bayes classifier to train the classifier with certain amount of labeled texts followed by large amount of unlabeled documents,which realizes the automatic training without huge amount of hand-designed training data.IV.IBM W ATSONThe IBM Watson project has shown us that computer system in open-domain question-answering(QA)is possible to beat human champions in Jeopardy.As Ferrucci(2012) mentioned,the structure of Watson is more complicated than any single agent as it has hundreds of algorithms working together,in the way that Minsky(1988)introduced in Society of Mind.Generally,Watson consists of parts which are DeepQA,Natural Language Processing(NLP), Machine Learning(ML),and Semantic Web and Cloud Computing(Gliozzo et al.,2013).The DeepQA system analyzes the question by different algorithms,giving different interpretations of questions and forming queries for each question (Ferrucci,2012).It provides all the possible answers to the question with the evidences and the scores for each candidate,which would generate a ranking of candidate answers with the likelihood of correctness.The Machine Learning algorithms are used to train the weights in its evaluating and analyzing algorithms(Gliozzo et al.,2013).The clue that Watson uses in searching is named as lexical answer type(LAT),which tells Watson what the question is asking about and what kind of things it needs to look for.Before doing searching, it would generate prior knowledge of type label, known as‘direction’,to each candidate answer and search evidences for and against this‘type direction’(Ferrucci,2012).The DeepQA also has a high requirement in Grammar-based and syntactic analysis techniques,for example,relation extraction techniques in getting possible relations between words,based on a rule-based approach.In addition,the ability of breaking the question down into sub-questions by logics also improved Watsons performance(Ferrucci,2012),which enables Watson tofind results for each smaller questions and combine them together.In correspondence to the ability of breaking down questions,it can also generate the score for the original question based on the evidence for sub-questions.To simulate human knowledge,Watson also uses self-contained database.However,this requirement has led to its great hardware cost.Watson also needs to do automatic text analysis and knowledge extraction to update its database,because of the enormous amount of work and the insurance ofinput-knowledge accuracy.However,the use of self-contained database is costly,that only few institutions can afford the hardware expense,which makes the application of Watson expensive.Another limitation is that the structured resource is relatively narrow compared with vast unstructured natural-language texts.One of the possible improvement is to use online data and ordinary online search engine tofind possible related articles and analyze them with PC clients.Despite the tradeoff between accuracy and cost,because of the possible the unreal data and incorrect information online,it makes the technique more realizable in general.V.C ONCLUSIONAs can be seen from the content above,most techniques used in text analysis are based on‘word feature’extraction,word types,and relations, which are all semantic techniques.While Watson also uses searching techniques tofind the exact answer shown in text.However,the machines lack the ability to conclude the main idea in a paragraph,which is more related with abstract logic thinking.While the way that human read concerns not only on vocabularies and meanings, but also the structure of paragraph and the location of sentences,for example,thefirst sentence in the paragraph usually guides the following content, which helps tell the significance of the sentences and words.Therefore,using machine learning to analyze the structure of an article and combining with the meaning of every sentence might generate the ability to conclude the main idea,which can be used in text scanning and classification.R EFERENCES[1]S.Dumais,J.Platt,D.Heckerman,and M.Sahami,InductiveLearning Algorithms and Representations for Text Categoriza-tion,Proceedings of the seventh international conference on Information and knowledge management,pp-148-155,1998. [2]T.Joachims,Text Categorization with Support Vector Machines:Learning with Many Relevant,ECML-98Proceedings of the10th European Conference on Machine Learning,pp-137-142,1998.[3]T.Joachims,Transductive Inference for Text Classification usingSupport Vector Machines,International Conference on Machine Learning(ICML),pp-200-209,1999.[4]V.Hatzivassiloglou,J.Klavans,and E.Eskin,Detecting TextSimilarity Over Short Passages:Exploring Linguistic Feature Combinations Via Machine Learning,Joint SIGDAT Conference on Empirical Methods in Natural Language Processing and Very Large Corpora,2000.[5]K.Nigam,Text Classification from Labeled and Unlabeled Doc-uments using EM,Machine Learning,V olume39,pp-103134, 2000.[6] E.Liddy,Natural Language Processing,In Encyclopedia ofLibrary and Information Science,2nd Ed.NY.Marcel Decker, Inc,2001.[7]S.Tong and D.Koller,Support Vector Machine Active Learningwith Applications to Text Classification,Journal of Machine Learning Research pp-45-66,2001.[8] F.Sebastiani,Machine Learning in Automated Text Categoriza-tion,ACM Computing Surveys(CSUR),Issue1,V olume34, pp-1-47,2002.[9]P.Soucy and G.Mineau,Feature Selection Strategies for TextCategorization,AI2003,LNAI2671,pp-505-509,2003. [10]X.Han,G.Zu,W.Ohyama,T.Wakabayashi,and F.Kimura,Accuracy Improvement of Automatic Text Classification Based on Feature Transformation and Multi-classifier Combination, LNCS,V olume3309,pp.463-468,Jan2004.[11]M.Ikonomakis,S.Kotsiantis,V.and Tampakas,Text Classifica-tion using Machine Learning Techniques,WSEAS Transactions on Computers,Issue8,V olume4,pp-966-974,2005.[12]R.Collobert and J.Weston,unified architecture for natural lan-guage processing:deep neural networks with multitask learning, ICML’08Proceedings of the25th international conference on Machine learning,ACM New York,USA,Pages160-167,2008.[13]R.Collobert,J.Weston,L.Bottou,M.Karlen,K.Kavukcuoglu,and P.Kuksa Natural Language Processing(Almost)from Scratch,Journal of Machine Learning Research,V olume12,pp-2493-2537,2011.[14] A.Gliozzo,O.Biran,S.Patwardhan,and K.McKeown,Seman-tic Technologies in IBM Watson,The10th International Semantic Web Conference,Bonn,Germany,2011.[15] D.Ferrucci,Introduction to“This is Watson”,IBM Journal ofResearch and Development,V olume56Number3/4,pp-1:1-1:15 May/July2012.[16]G.Tesauro,D.Gondek,J.Lenchner,J.Fan,and J.Prager,Simulation,learning,and optimization techniques in Watsons game strategies,IBM Journal of Research and Development, V olume56,Number3/4,pp-16:116:11,2012.。
Semantic Web Query Languages

More expression testing (date-time support, for example)
Using DESCRIBE clauses to return descriptions of the resources matching the query part. Enables sorting. Specify OPTIONAL triple or graph query patterns Testing the absence, or non-existence, of tuples.
Query File
Query File
Executing SPARQL Queries Using Jena and Java
Set class path, this may differ according to Jena version. Write your java program and execute it. Using Jena and Java gives you the ability to process query output in the way you like. Example program
SeRQL )Sesame RDF Query Language(
Based on several existing languages, most notably RQL, RDQL and N3. SeRQL is easier to parse than RQL. Missing functions: eg. aggregation (minimum, maximum, average, count) SeRQL is not safe as it provides various recursive built-infunctions.
- 1、下载文档前请自行甄别文档内容的完整性,平台不提供额外的编辑、内容补充、找答案等附加服务。
- 2、"仅部分预览"的文档,不可在线预览部分如存在完整性等问题,可反馈申请退款(可完整预览的文档不适用该条件!)。
- 3、如文档侵犯您的权益,请联系客服反馈,我们会尽快为您处理(人工客服工作时间:9:00-18:30)。
Semantic Search of Unstructured Data using ContextualNetwork GraphsMaciej Ceglowski, Aaron Coburn, and John CuadradoNational Institute for Technology and Liberal EducationMiddlebury College, Middlebury, Vermont, 05753 USA{mceglows, acoburn}@drjlc@Abstract. The authors present a graph-based algorithm for searching potentiallylarge collections of unstructured data, and discuss its implementation as asearch engine designed to offer advanced relevance feedback features to userswho may have limited familiarity with search tools. The technique, whichclosely resembles the spreading activation network model described by ScottPreece, uses a term-document matrix to generate a bipartite graph of term anddocument nodes representing the document collection. This graph can besearched by a simple recursive procedure that distributes energy from an initialquery node. Nodes that acquire energy above a specified threshold comprise theresult set. Initial results on live collections suggest that this technique mayoffer performance comparable to latent semantic indexing (LSI), while avoidingsome of that technique’s computational pitfalls. Both the algorithm and itsimplementation in a production Web environment are discussed.1. IntroductionThe rapid growth of online information has proved both a blessing and a burden, as information retrieval systems struggle to keep up with a flood of new material. This information overload is particularly acute in the academic community, where domain experts need to perform advanced searches, but frequently lack the expertise and training needed to make productive use of tools like Boolean query syntax and regular expressions.Current metadata creation standards for the humanities, such as SCORM [1] or Dublin Core [2], require such intensive human effort that, for many institutions, the costs of data markup are prohibitive.This situation has created an urgent need for automated search tools that can search large data collections based on semantic content. While techniques such as latent semantic indexing (LSI) have shown great promise in enabling this kind of content discovery, it is not clear that they can scale well to large, dynamic document collection.The National Institute for Technology and Liberal Education (NITLE), with generous support from the Andrew W. Mellon Foundation, has been working to make usable, advanced search tools available to students and scholars. Our initial work on adapting LSI for general use has led to the rediscovery of a search algorithm first described in 1981 [3] which shows great promise in offering results comparable in quality to LSI, without the concomitant computational overhead.In this paper we describe both the new algorithm and its initial implementation as an open-source, Web based search service designed for maximum usability.2. Latent Semantic IndexingLatent semantic indexing (LSI) is a vector-space technique that has shown great promise in addressing the problems of polysemy and synonymy in text collections, offering improved recall over standard keyword searches. One of the most striking features of LSI is the ability to return relevant results for queries even when there is no exact keyword match [4]. Because the LSI vector model is a purely mathematical representation, the indexing technique is not limited to any particular language. Indeed, recent work has shown that LSI can be usefully extended to non-text domains, including log file analysis [5] and protein structure prediction [6].Several features of vector space models in general, and LSI in particular, allow for the design of relevance feedback features that would be difficult to implement in a standard search engine. These include ‘find similar’ links for individual documents in a result set, as well as the ability to run an iterative search by re-querying the engine with a set of relevant results. Suitably implemented, these kinds of feedback features can reduce or eliminate the need to train users in an elaborate query syntax.For all its advantages, LSI also presents some drawbacks. The poor scalability of the singular value decomposition (SVD) algorithm remains an obstacle to indexing very large collections. While techniques have been developed for making incremental updates to a scaled collection, these changes typically cannot exceed a certain threshold without triggering a rebuild [7,8]. These constraints make LSI ill suited to the kinds of large, rapidly changing document collections typically found on the Web.A further disadvantage to LSI is the difficulty in interpreting the underlying reduced term space [4]. This makes it difficult to select an optimum number of singular values to retain in the SVD for a given collection, or allow domain expert adjustment of relevance values in the reduced space once the SVD has been calculated.These shortcomings have led us to seek out an indexing scheme that retains the advantages of LSI, while presenting fewer computational and conceptual obstacles.3. Contextual Network GraphsA standard step in LSI is the creation of a term-document matrix (TDM), which is essentially a weighted lookup table of term frequency data for the entire document collection. In LSI, this matrix is interpreted as a high-dimensional vector space.An alternative interpretation of this matrix is possible, however, with the TDM representing a bipartite graph of term and document nodes where each non-zero value in the TDM corresponds to an edge connecting a term node to a document node. In this model, every term is connected to all of the documents in which the term appears, and every document has a link to each term contained in that document. The weightedfrequency values in the TDM correspond to weights placed on the edges of the graph. We call this construct a contextual network graph.As an example, consider the miniature document collection in table 1, and its associated term list in table 2.Table 1. A sample document collection with associated node labelsNode Document content1Glacial ice often appears blue.2Glaciers are made up of fallen snow3Firn is an intermediate state between snow and glacial ice.4Ice shelves occur when ice sheets extend over the sea.5Glaciers and ice sheets calve icebergs into the sea.6Firn is half as dense as sea water.7Icebergs are chunks of glacial ice under water.Table 2. A labeled term list derived from the collection in table 1. All terms occur across at least two documents in the parent collection.Node Term Occurrence counta glacial ice3b ice5c glacier2d snow2e firn2f ice sheet2g sea3h water2i iceberg2j sheet2We can represent this collection of terms and documents as a contextual network with the topology shown in figure 1.Fig. 1.Sample contextual network graph indicating connections between the documents in table 1 and content terms in table 2While similar in spirit to IR approaches like conceptual graphs [10], the contextual network graph does not encode any information about grammatical or hierarchical relationships between terms. Its structure is determined purely by term co-occurrence across the collection.Each edge in the graph has a strength assigned to it whose magnitude depends on our choice of the local and global term weighting scheme used in generating the TDM. The only constraint on weighting schemes is that all edge weights must fall in the interval (0,1).We can search the collection represented by this graph by energizing a query node and allowing the energy to propagate to other nodes along the edges of the graph based on a set of simple rules. The total energy deposited at any given node in the graph will depend both on the number of paths between it and the query node, as well as the relative strength of the connections along those paths. This corresponds to the intuition that documents that share many rare terms are likely to be semantically related. It also enables the same kind of enhanced recall provided by LSI, since a query on a particular keyword may still reach a document that does not contain the word itself, but is closely linked to other documents that do.Because the initial energy dissipates as it spreads over the graph (the requirement that energy dissipate is the reason for the constraint on edge weights), results for any search are localized to a single region of the graph, with important implications for scalability.In the example above, a search on ‘iceberg’ would begin by activating the node corresponding to the query term, in this case node i. This query node is assigned a default starting energy E, which is distributed to neighbor nodes according to the following algorithm:1 procedure energize( energy E, node n k ) {2energy(n k) := energy(n k) + E3E':= E / degree of n k4if ( E' > T ) {5for each node n j in N k {6E" := E' * e jk7energize( E", n j )8}9}10 }Where N k is the set of all neighbor nodes of n k, e jk is the weight of the edge connecting nodes n j and n k, T is a constant threshold value, and energy(n k) is a data structure that stores node energy values for the duration of a query. Note that this version of the algorithm performs a depth-first traversal of the graph. In the case where N k is sorted by decreasing edge weight, the traversal is also best-first. The algorithm may be suitably modified to perform a breadth-first traversal; the optimal traversal strategy is a topic for further study.In our example, the query consists of a single term, and the search terminates after the energy from the initial node has been distributed as far as it can go before dippingbelow the threshold T.In the case of queries consisting of multiple nodes, the procedure would be repeated for each query node in turn, with the final energy values for nodes in the graph a superimposition of the individual searches. Note that the query may consist of any combination of term and document nodes.Although our graph does not include nodes for singleton terms that occur in only one document, it is a straightforward matter to keep a list of these terms and substitute the appropriate document node for any singleton term in a query.Once all the nodes in a query have been processed, results are gathered in a collection step, with nodes sorted by reverse order of accumulated energy.Nodes with the highest energy values will correspond to documents and terms that are semantically closest to the original query. This result set will consist of both document and term nodes. The search interface may choose not to display nearest term results, or may use them as a relevance feedback feature.In addition to the traversal order, there are several other tunable parameters in the algorithm. The starting energy E and threshold energy T are both arbitrary values; the larger the starting energy, and the lower the limit threshold, the further the initial node energy will spread in the graph. The mechanism for distributing energy among neighbor nodes in line 3 and the weighting step in line 6 may also be altered.3.1. Preliminary ObservationsWork is ongoing to evaluate the quality of contextual network search (CNS) compared to keyword and LSI approaches. Preliminary results on live collections of Civil War articles [10] and protein sequence data [11] indicate that the CNS offers comparable results to an LSI search engine built from the same term-document matrix. Both CNS and LSI can return semantically related documents that do not contain an exact keyword match; however, CNS tends to favor keyword over non-keyword matches more than LSI.3.2. Distributed SearchThe algorithm described above can be distributed in two ways, both to cope with very large collections, and to improve performance for queries containing a large number of nodes. The two distribution techniques are complementary.The first technique involves partitioning the graph into subgraphs, and distributing the subgraphs across a computing cluster controlled by a master server. The master server must have a full map of graph connectivity, but need not store any information about node energies or edge weights. It distributes queries by passing them along to the slave server responsible for the portion of the graph containing the query node, as well as handling cases where searches cross subgraph boundaries. The master server collects and collates results from all slave servers involved in a given search into a single result set.For cases where queries contain a large number of document or term nodes (this can occur frequently in relevance feedback searches, as well as searches that involve large segments of text pasted into a Web form), it is possible to improve search speed by searching in parallel over multiple copies of the graph, and superimposing theresulting node energies. This approach overcomes one weakness of CNS compared to vector space techniques: while the limiting factor for vector space comparisons is the time required to parse the query into a pseudo-document vector, search time in the contextual network graph model grows as a function of the number of nodes in the query.In principle, it is possible to combine both the graph segmentation and parallel query approach given a sufficiently large computing cluster.3.3. Insertions and deletionsInsertion and removal of documents into the collection does not pose the same kinds of problems for CNS as it does for vector model approaches. For additions, the graph server simply has to parse the new documents, and add additional connections between document nodes and existing term nodes. Because singleton terms that occur in only one document are not included in the graph, the list of singleton terms has to be checked when a new document is parsed, to see if any of the terms need to be promoted to full term node status.Documents can also be removed from the collection on the fly, by excising the appropriate document nodes and checking the graph for any new singleton term nodes, which are relegated back to the singleton list.Depending on the document normalization and term weighting parameters used in generating the term-document matrix, addition or removal of nodes from the graph may require that edge weights be recalculated for the entire collection. However, this calculation is much less onerous than the kind of full rebuild required in LSI, and there are several ways to mitigate its impact. Edge weights can be updated on a rolling basis if necessary.4. ImplementationThe techniques described in this paper have been successfully implemented in a live Internet search engine covering a collection of Civil War-era newspaper articles, as well as on several test collections of both text and biological data [10,11,12]. The search engines are designed to be modular and to run on multiple servers and platforms, if necessary, to maximize flexibility. The relevance feedback features made possible by CNS form an important part of the user interface.4.1. System ArchitectureSearch, indexing, and interface components in the system are decoupled from one another, and communicate across a series of network interfaces. This means that the document data, search engine and user interface need not be located on the same network.Fig 2. Schematic of document processing and search component design The system is built around a central document repository, which stores all of the document and term data for the collection. The documents in this repository are processed in an initial indexing step, described below, to create the graph server, after which the search component is completely independent of the document repository. The repository is implemented as a MySQL database with a Perl interface that runs as a Web service, listening for document requests over XML-RPC.The graph query server is a C++ program that loads the actual contextual network graph, and searches the collection. This server uses a very simple protocol to minimize overhead – it listens on a Unix socket for a list of one or more query nodes, and returns a list of result nodes and energy values in response. Nodes are represented as strings in the form ‘d123’ or ‘t456’, depending on whether the node is a document or term node. The graph server can also be asked to raise or lower its minimum energy threshold, to control the sensitivity of the search.The search server correlates results from the graph server with document data obtained from the document repository, and organizes the data into a format suitable for display by the human interface. The search server is responsible for converting natural language queries to node lists to pass along to the graph server, as well taking care of details such as providing short extracts of long documents, and paginating result sets for the benefit of the human interface. This server is implemented in Perl and also runs as a Web service, listening for queries over an XML-RPC protocol.The user interface can take a variety of forms; in its current implementation, it runs as a Perl Web application. This consists of a single query box and search button, with relevance feedback features provided as part of each result set. Documents in a result set are displayed with a clickable ‘find similar’ link, allowing for effective horizontal navigation through search results. Users are also given a ‘document basket’ – a session-based store they can populate with documents encountered in the course of their search. The basket has its own find similar link, letting users search on the aggregate of all documents in the saved set. The basket metaphor, familiar fromonline shopping sites, gives users an intuitive method for performing an iterative search on the collection.The most unusual relevance feedback item is a list of similar terms displayed with each result set. Since CNS returns both document and term nodes, displaying a list of ‘nearest terms’ for a query is trivial. While these terms are clickable, their more important function is in keeping users oriented. By glancing at the list of similar terms, users can get a feel for the semantic neighborhood of their query.4.2. Pre-processingWe assume that the raw documents in the repository are in plain text or in a Web markup format, such as XML or HTML. Documents are cleaned using a suite of Perl regular expressions to strip formatting and remove markup. They are then sent through the appropriate parser. For English language text, we use a part-of-speech (POS) tagger in tandem with a regular expression for finding maximal noun phrases to generate our term list [13]. Unlike more traditional stop list + stemming approaches, this approach allows us to discriminate between polysemous words whose meaning changes depending on the part of speech (e.g., ‘fly’ as a verb or noun), as well as extract multi-word noun phrases and proper names (‘hot rod’, ‘Rip Van Winkle’). Noun phrase extraction is greedy and recursive, so that a single noun phrase can be parsed into multiple terms.Our POS tagger [14] uses a stored lexicon with statistical information about word and part-of-speech usage for English derived from the Penn Treebank Project [15], an annotated corpus of text developed by the Linguistic Data Consortium. The tagger uses stored probability data in conjunction with a bigram Hidden Markov Model (HMM) of POS occurrence, assigning a tag t i to a word w i according to the following formula:t i = argmax P( t j | t i-1 ) P( w i | t j ) . (1)jA metric based on word morphology supplements the HMM for words not appearing in the lexicon.The term list derived from the parsing step is used to generate a standard term-document matrix (TDM). In our implementation, we weight TDM values according to the formula:e ij = g j ln(f ij)n i c j , (2) whereg j is a global term weight determined by inverse document frequency, f ij is the frequency of term j in document i, n j is a document normalization factor, and c j is the number of words in term j.6. Further WorkWe are currently evaluating CNS against both LSI results and human-classified collections from TREC to obtain empirical estimates of search quality. In addition to these ongoing tests, we are pursuing several promising directions of study.6.1. Scalability.In its current implementation, the graph server requires approximately 1GB of RAM for every 20K documents (95K terms), running on a desktop Linux server.We are currently working on implementing the distributed search techniques described earlier, using a cluster of commodity PCs over a Gigabit Ethernet connection to field queries on very large document collections. At this time, available memory rather than processing speed seems to be the limiting factor on graph size.6.2. Representing Internal LinksThe graph data model allows for the potential inclusion of document-to-document links, whether in the form of internal references to other documents, or as explicitly defined links added to the collection model by a human curator. While patterns of internal citations in a document collection have previously been studied [14], contextual networks provide a natural framework for combining content and link-based similarity. Both inherent and user-defined internal links are features that would be difficult to represent in a vector-space model.The addition of intra-document links causes two major changes to the model - the graph is no longer bipartite, and part of the graph becomes directed. The possible implications of these changes are unclear.It is also possible to conceive of internal links between term nodes, for example, as an implementation of cross-language search.6.3. ClusteringSeveral techniques are available for segmenting the network graph and assigning documents to content clusters. We are currently experimenting with ways to segment very large collections into smaller sub-collections in an effort at auto-categorization, both in the text and protein domain, as well as approaches to graph clustering that will form the subject of a future paper.6.4. Non-text collectionsOur work with LSI in the protein domain has shown encouraging results for context-based prediction of protein structure based on sequence. We have every reason to believe similar results will obtain from a contextual network model, while allowing us to work with larger data collections.References1.SCORM: /specification.asp2.Dublin Core: 3.Preece, Scott. “A spreading activation network model for information retrieval”PhD thesis, CS Dept., Univ. of Illinios, Urbana, IL. 1981.4.Deerwester, S., Dumais, S., Furnas, G., Landauer, T. and Harshman, R., “Indexingby latent semantic analysis”, Journal of the American Society for Information Science, 1990, pp. 391-407.5.Quesada, J., Kintsch, W. and Gomez, E. “A Computational Theory of ComplexProblem Solving Using Latent Semantic Analysis” In W.D. Gray & C.D. Schunn (Eds.) Proceedings of the 24th Annual Conference of the Cognitive Science Society, Fairfax, VA. Lawrence Erlbaum Associates, Mahwah, NJ, 2002, pp.750-755.6. Ceglowski, M. and Cuadrado, J. Slide presentation, O’Reilly BioinformaticsConference, February 2003./cs/bio2003/view/e_sess/34067.Berry, M., Drmac, Z. and Jessup E. “Matrices, Vector Spaces, and InformationRetrieval”, SIAM Review. Vol. 41, No. 2, pp. 335-362.8.Simon, H. and Zha, H. “On Updating Problems in Latent Semantic Indexing”,Technical Report No. CSE-97-011, Department of Computer Science andEngineering, Pennsylvania State University, 1997.9.Montes-y-Gómez, M., López-López, A. and Gelbukh, A. “Information Retrievalwith Conceptual Graph Matching”, Lecture Notes in Computer Science, Vol.1873, Springer Verlag, 2000, pp. 312-321.10.University of Virginia Valley of the Shadow Project search engine./cns/uva.pl11.PDB Toxin search demo. /cns/toxins.pl12.Steven Johnson research notes. /cns/sbj.pl13.Bader, R., Callahan, M., Grim, D., Krause, J. and Pottenger, W., "The role of theHDDI TM collection builder in hierarchical distributed dynamic indexing", Workshop on Text Mining, Chicago, 2001, pp. 23-30.14.Lingua::EN::Tagger, a Perl part-of-speech tagger for English text./author/MCEGLOWS/15.Marcus, M., Santorini, B., Marcinkiewicz, M.A., and Taylor, A. TreeBank-3.Linguistic Data Consortium, 1999.。