NVIDIA宣布推出CUDA-XHPC
显卡硬件加速技术CUDARTX和TensorCore的应用
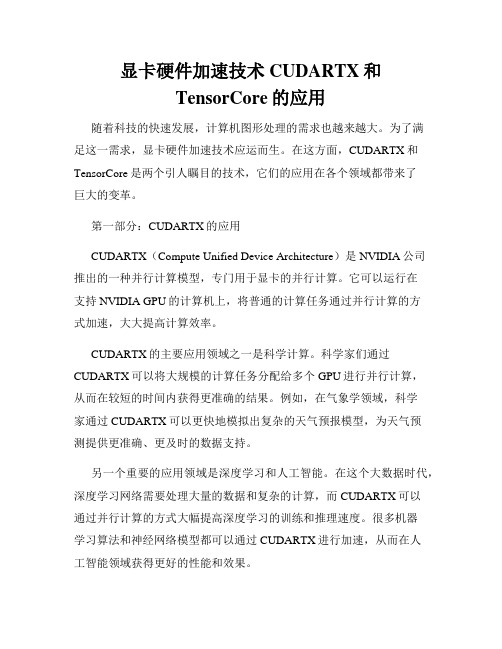
显卡硬件加速技术CUDARTX和TensorCore的应用随着科技的快速发展,计算机图形处理的需求也越来越大。
为了满足这一需求,显卡硬件加速技术应运而生。
在这方面,CUDARTX和TensorCore是两个引人瞩目的技术,它们的应用在各个领域都带来了巨大的变革。
第一部分:CUDARTX的应用CUDARTX(Compute Unified Device Architecture)是NVIDIA公司推出的一种并行计算模型,专门用于显卡的并行计算。
它可以运行在支持NVIDIA GPU的计算机上,将普通的计算任务通过并行计算的方式加速,大大提高计算效率。
CUDARTX的主要应用领域之一是科学计算。
科学家们通过CUDARTX可以将大规模的计算任务分配给多个GPU进行并行计算,从而在较短的时间内获得更准确的结果。
例如,在气象学领域,科学家通过CUDARTX可以更快地模拟出复杂的天气预报模型,为天气预测提供更准确、更及时的数据支持。
另一个重要的应用领域是深度学习和人工智能。
在这个大数据时代,深度学习网络需要处理大量的数据和复杂的计算,而CUDARTX可以通过并行计算的方式大幅提高深度学习的训练和推理速度。
很多机器学习算法和神经网络模型都可以通过CUDARTX进行加速,从而在人工智能领域获得更好的性能和效果。
第二部分:TensorCore的应用TensorCore是NVIDIA推出的一种专用硬件单元,用于加速矩阵乘法和深度学习中的张量运算。
它提供了极高的计算性能和能效,成为深度学习和神经网络算法的不可或缺的一部分。
TensorCore的主要应用之一是神经网络的加速。
神经网络算法中的大部分计算都可以通过矩阵乘法的方式进行高效的计算,并且这些计算都可以通过TensorCore来加速。
通过使用TensorCore,神经网络的训练和推理速度可以大幅提高,从而在人工智能领域取得更好的效果。
此外,TensorCore在科学计算中也有广泛的应用。
英伟达超算中心浸没式冷却液方案
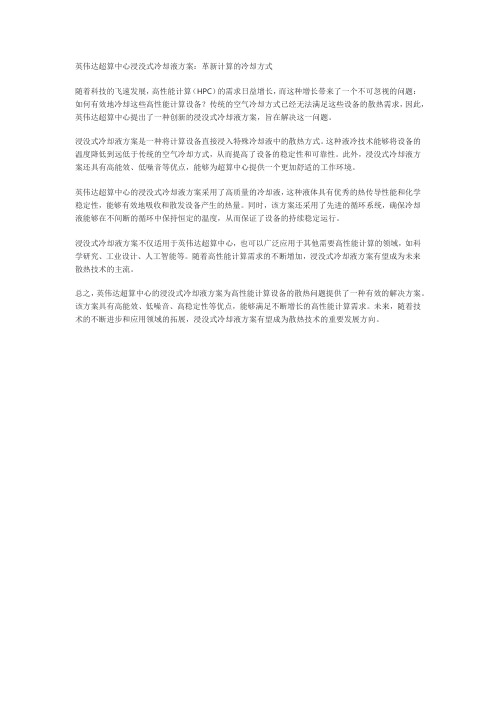
英伟达超算中心浸没式冷却液方案:革新计算的冷却方式
随着科技的飞速发展,高性能计算(HPC)的需求日益增长,而这种增长带来了一个不可忽视的问题:如何有效地冷却这些高性能计算设备?传统的空气冷却方式已经无法满足这些设备的散热需求,因此,英伟达超算中心提出了一种创新的浸没式冷却液方案,旨在解决这一问题。
浸没式冷却液方案是一种将计算设备直接浸入特殊冷却液中的散热方式。
这种液冷技术能够将设备的温度降低到远低于传统的空气冷却方式,从而提高了设备的稳定性和可靠性。
此外,浸没式冷却液方案还具有高能效、低噪音等优点,能够为超算中心提供一个更加舒适的工作环境。
英伟达超算中心的浸没式冷却液方案采用了高质量的冷却液,这种液体具有优秀的热传导性能和化学稳定性,能够有效地吸收和散发设备产生的热量。
同时,该方案还采用了先进的循环系统,确保冷却液能够在不间断的循环中保持恒定的温度,从而保证了设备的持续稳定运行。
浸没式冷却液方案不仅适用于英伟达超算中心,也可以广泛应用于其他需要高性能计算的领域,如科学研究、工业设计、人工智能等。
随着高性能计算需求的不断增加,浸没式冷却液方案有望成为未来散热技术的主流。
总之,英伟达超算中心的浸没式冷却液方案为高性能计算设备的散热问题提供了一种有效的解决方案。
该方案具有高能效、低噪音、高稳定性等优点,能够满足不断增长的高性能计算需求。
未来,随着技术的不断进步和应用领域的拓展,浸没式冷却液方案有望成为散热技术的重要发展方向。
NVIDIA宣布推出CUDA-X HPC
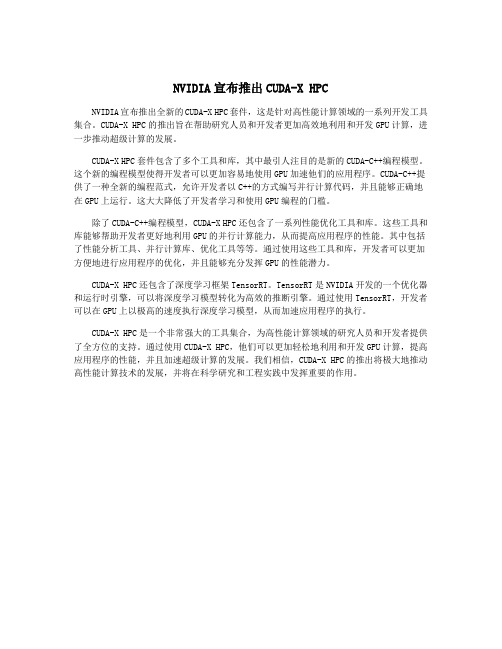
NVIDIA宣布推出CUDA-X HPCNVIDIA宣布推出全新的CUDA-X HPC套件,这是针对高性能计算领域的一系列开发工具集合。
CUDA-X HPC的推出旨在帮助研究人员和开发者更加高效地利用和开发GPU计算,进一步推动超级计算的发展。
CUDA-X HPC套件包含了多个工具和库,其中最引人注目的是新的CUDA-C++编程模型。
这个新的编程模型使得开发者可以更加容易地使用GPU加速他们的应用程序。
CUDA-C++提供了一种全新的编程范式,允许开发者以C++的方式编写并行计算代码,并且能够正确地在GPU上运行。
这大大降低了开发者学习和使用GPU编程的门槛。
除了CUDA-C++编程模型,CUDA-X HPC还包含了一系列性能优化工具和库。
这些工具和库能够帮助开发者更好地利用GPU的并行计算能力,从而提高应用程序的性能。
其中包括了性能分析工具、并行计算库、优化工具等等。
通过使用这些工具和库,开发者可以更加方便地进行应用程序的优化,并且能够充分发挥GPU的性能潜力。
CUDA-X HPC还包含了深度学习框架TensorRT。
TensorRT是NVIDIA开发的一个优化器和运行时引擎,可以将深度学习模型转化为高效的推断引擎。
通过使用TensorRT,开发者可以在GPU上以极高的速度执行深度学习模型,从而加速应用程序的执行。
CUDA-X HPC是一个非常强大的工具集合,为高性能计算领域的研究人员和开发者提供了全方位的支持。
通过使用CUDA-X HPC,他们可以更加轻松地利用和开发GPU计算,提高应用程序的性能,并且加速超级计算的发展。
我们相信,CUDA-X HPC的推出将极大地推动高性能计算技术的发展,并将在科学研究和工程实践中发挥重要的作用。
英伟达 tesla p100 应用性能指南- hpc 和深度学习应用说明书
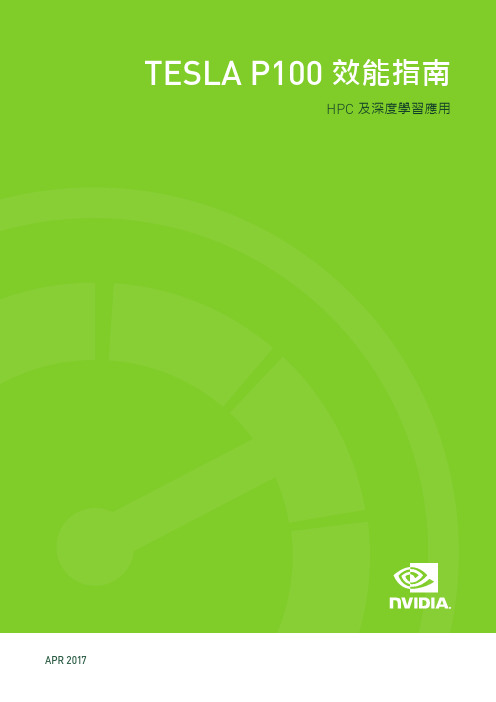
HPC 及深度學習應用APR 2017TESLA P100 效能指南現代的高效運算(HPC)資料中心是解決部分全球最重要之科學與工程挑戰的關鍵。
NVIDIA® Tesla®加速運算平台利用領先業界的應用程式支援這些現代化資料中心,促進 HPC 與 AI 工作負載。
Tesla P100 GPU 是現代資料中心的引擎,能以更少的伺服器展現突破性效能,進而實現更快的解析能力,並大幅降低成本。
每一個 HPC 資料中心都能自 Tesla 平台獲益。
在廣泛的領域中有超過 400 個HPC 應用程式,採用 GPU 最佳化,包括所有前 10 大 HPC 應用程式和各種主要深度學習架構。
採用加速 GPU 應用程式的研究領域包括:超過 400 個 HPC 應用及所有深度學習架構皆是採用加速 GPU。
>若想要取得最新 GPU 加速應用目錄,請造訪:/teslaapps>若想要立即在 GPU 上使用簡易指示,快速執行廣泛的加速應用,請造訪:/gpu-ready-apps分子動力(MD)代表 HPC 資料中心的大部分工作負載。
100% 頂尖 MD 應用皆是採用 GPU 加速,以使科學家能進行從前僅有 CPU 版本之傳統應用項目無法執行的模擬工作。
在執行 MD 應用時,配備 Tesla P100 GPU 的資料中心可節省高達 60% 的伺服器取得成本。
TESLA 平台及適用 MD 的 P100 的關鍵功能>搭載 P100 的伺服器,最多可取代 40 部適用 HOOMD-Blue、LAMMPS、AMBER、GROMACS 和 NAMD 等應用的 CPU 伺服器>100% 頂尖 MD 應用項目皆採用加速 GPU>FFT 和 BLAS 等關鍵數學程式庫>每一個 GPU 之單精度效能高達每秒 11 TFLOPS>每一個 GPU 之記憶體頻寬高達每秒 732 GB檢視所有相關的應用項目:/molecular-dynamics-appsHOOMD-BLUE循序寫入 GPU 的粒子動力封裝版本1.3.3加速功能CPU 和 GPU 可用版本延展性多 GPU 和多節點更多資訊/hoomd-blueLAMMPS典型粒子動力封裝版本2016加速功能Lennard-Jones、Gay-Berne、Tersoff 更多勢能延展性多 GPU 和多節點更多資訊/lammpsGROMACS模擬含複雜連結互動的生物模型分子版本5.1.2加速功能PME ,顯性與隱性溶劑延展性多 GPU 和多節點擴展至 4xP100更多資訊/gromacs黃色在生物分子上模擬分子動力的程式套件版本16.3加速功能PMEMD 顯性溶劑和 GB 、顯性及隱性溶劑、REMD 、aMD延展性多 GPU 和多節點更多資訊/amberNAMD專為高效模擬大分子系統而設計版本2.11加速功能PME 全靜電和眾多模擬功能延展性高達 100M 原子,多 GPU,擴展為 2xP100更多資訊/namd量子化學(QC)模擬是探索新藥物與原料的關鍵,且會耗費大部分 HPC 資料中心的工作負載。
cuda发展历程 -回复
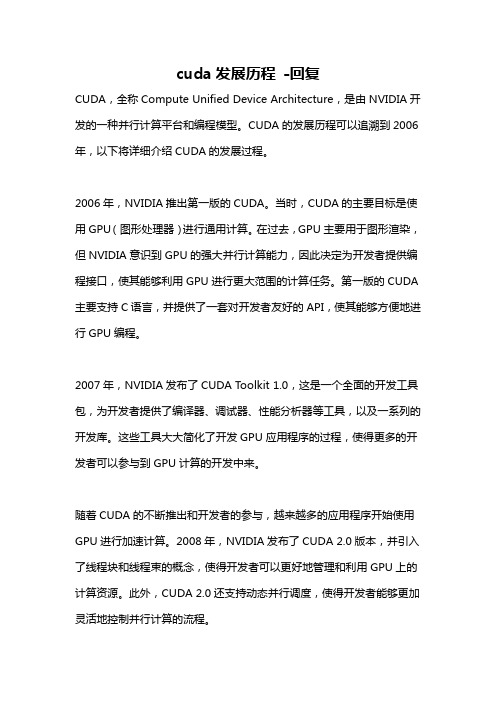
cuda发展历程-回复CUDA,全称Compute Unified Device Architecture,是由NVIDIA开发的一种并行计算平台和编程模型。
CUDA的发展历程可以追溯到2006年,以下将详细介绍CUDA的发展过程。
2006年,NVIDIA推出第一版的CUDA。
当时,CUDA的主要目标是使用GPU(图形处理器)进行通用计算。
在过去,GPU主要用于图形渲染,但NVIDIA意识到GPU的强大并行计算能力,因此决定为开发者提供编程接口,使其能够利用GPU进行更大范围的计算任务。
第一版的CUDA 主要支持C语言,并提供了一套对开发者友好的API,使其能够方便地进行GPU编程。
2007年,NVIDIA发布了CUDA Toolkit 1.0,这是一个全面的开发工具包,为开发者提供了编译器、调试器、性能分析器等工具,以及一系列的开发库。
这些工具大大简化了开发GPU应用程序的过程,使得更多的开发者可以参与到GPU计算的开发中来。
随着CUDA的不断推出和开发者的参与,越来越多的应用程序开始使用GPU进行加速计算。
2008年,NVIDIA发布了CUDA 2.0版本,并引入了线程块和线程束的概念,使得开发者可以更好地管理和利用GPU上的计算资源。
此外,CUDA 2.0还支持动态并行调度,使得开发者能够更加灵活地控制并行计算的流程。
在接下来的几年里,NVIDIA持续不断地更新和改进CUDA平台。
2010年,CUDA 3.0发布,引入了一种新的内存模型,即统一虚拟寻址(Unified Virtual Addressing,UVA)。
这一功能使得开发者可以更方便地在CPU 和GPU之间共享内存,并且不再需要显示地进行内存拷贝。
UVA的引入大大简化了编程的流程,提高了开发效率。
2012年,NVIDIA发布了CUDA 5.0版本,引入了动态并行调度的新特性,这使得开发者能够更好地响应计算需求的变化。
此外,CUDA 5.0还支持GPU加速的MPI(Message Passing Interface),使得CUDA可以更好地与传统的MPI编程模型结合起来,实现更高效的并行计算。
cuda发展历程

cuda发展历程CUDA(Compute Unified Device Architecture)是由NVIDIA 公司开发的一种并行计算平台和API模型。
以下是CUDA发展的主要里程碑:1. 2006年:NVIDIA发布了第一代CUDA架构,支持NVIDIA的GeForce 8系列和Tesla架构的显卡。
这一版本主要用于通用计算和图像处理。
2. 2007年:NVIDIA发布了CUDA 1.1版本,添加了对64位操作系统和64位浮点数的支持。
此外,还增加了对多GPU的支持。
3. 2008年:NVIDIA发布了CUDA 2.0版本,引入了CUDA C 编程语言,允许程序员使用类C语言的语法来编写并行计算代码。
此外,这一版本还引入了纹理内存、统一虚拟寻址和动态并行ism等功能。
4. 2010年:NVIDIA发布了CUDA 3.0版本,引入了GPU内存共享和主机线程同步等特性。
这一版本还大幅提升了GPU 和CPU之间的数据传输效率。
5. 2012年:NVIDIA发布了CUDA 5.0版本,引入了动态并行调度和GPUDirect技术,可以直接将数据从存储设备传输到GPU内存。
6. 2014年:NVIDIA发布了CUDA 6.0版本,增加了对动态并行ism和GPU内存引用计数的支持,提升了GPU的并行计算能力。
7. 2016年:NVIDIA发布了CUDA 8.0版本,引入了Pascal架构的显卡支持,这一架构在性能和能效方面都有显著改进。
8. 2020年:NVIDIA发布了CUDA 11.0版本,支持了NVIDIA的Ampere架构的显卡,这一版本在性能和功能上都有所提升。
目前,CUDA已经成为了广泛使用的并行计算平台,用于加速科学计算、机器学习、深度学习等领域。
同时,NVIDIA也在持续推进CUDA的发展,进一步提高GPU的计算能力和开发者的编程体验。
cuda 算力对应版本

cuda 算力对应版本CUDA(Compute Unified Device Architecture)是由NVIDIA推出的并行计算平台和编程模型,用于利用NVIDIA GPU进行通用目的计算。
CUDA算力对应版本指的是不同NVIDIA GPU设备的计算能力版本号,这个版本号代表了GPU的计算性能和功能特性。
以下是一些常见的CUDA算力对应版本:1. CUDA 1.x,这个版本对应的是早期的NVIDIA GPU,如GeForce 8800 GTX等,计算能力较低,通常用于简单的并行计算任务。
2. CUDA 2.x,这个版本对应的是一些较早期的Tesla架构GPU,计算能力相对较高,支持一些新的特性和指令集。
3. CUDA 3.x,这个版本对应的是Fermi架构的GPU,引入了更多的并行计算特性和性能优化,适合于复杂的并行计算任务。
4. CUDA5.x,这个版本对应的是Kepler架构的GPU,进一步提升了计算能力和能效比,支持动态并行调度等新特性。
5. CUDA6.x,这个版本对应的是Maxwell架构的GPU,提供了更高的能效比和性能表现,支持动态并行任务分配和共享内存等特性。
6. CUDA7.x,这个版本对应的是Pascal架构的GPU,引入了深度学习和机器学习等新特性,提供了更强大的并行计算能力。
7. CUDA 8.x,这个版本对应的是Volta架构的GPU,提供了更高的计算能力和更多的并行计算资源,适合于深度学习和科学计算等领域。
总的来说,CUDA算力对应版本代表了NVIDIA GPU的计算能力和性能特性,开发者可以根据自己的需求选择适合的CUDA版本来进行并行计算任务的开发和优化。
随着NVIDIA不断推出新的GPU架构,CUDA算力对应版本也会不断更新,提供更强大的计算能力和更丰富的功能特性。
NVIDIA Tesla P100 性能指南:HPC 和深度学习应用程序说明书
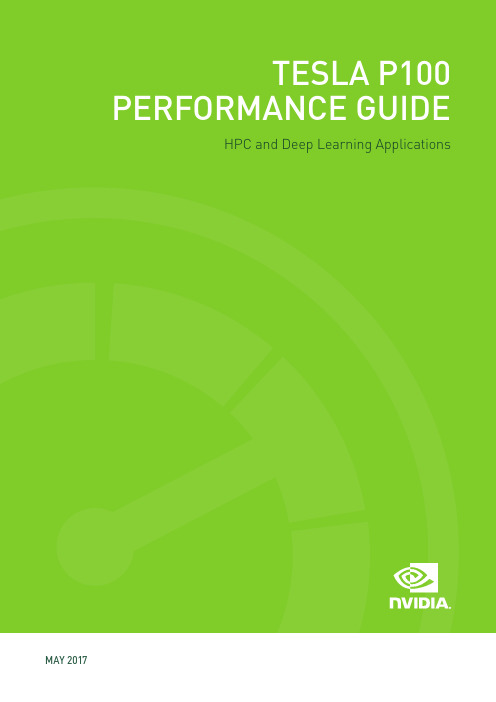
HPC and Deep Learning Applications MAY 2017TESLA P100 PERFORMANCE GUIDEModern high performance computing (HPC) data centers are key to solving some of the world’s most important scientific and engineering challenges. NVIDIA ® Tesla ® accelerated computing platform powers these modern data centers with the industry-leading applications to accelerate HPC and AI workloads. The Tesla P100 GPU is the engine of the modern data center , delivering breakthrough performance with fewer servers resulting in faster insights and dramatically lower costs.Every HPC data center can benefit from the Tesla platform. Over 400 HPC applications in a broad range of domains are optimized for GPUs, including all 10 of the top 10 HPC applications and every major deep learning framework.Over 400 HPC applications and all deep learning frameworks are GPU-accelerated.>To get the latest catalog of GPU-accelerated applications visit: /teslaapps >To get up and running fast on GPUs with a simple set of instructions for a wide range of accelerated applications visit: /gpu-ready-appsRESEARCH DOMAINS WITH GPU-ACCELERATED APPLICATIONS INCLUDE:Molecular Dynamics (MD) represents a large share of the workload in an HPC data center. 100% of the top MD applications are GPU-accelerated, enabling scientists to run simulations they couldn’t perform before with traditional CPU-only versions of these applications. When running MD applications, a data center with Tesla P100 GPUs can save up to 60% in server acquisition cost. KEY FEATURES OF THE TESLA PLATFORM AND P100FOR MD>Servers with P100 replace up to 40 CPU servers for applications such as HOOMD-Blue, LAMMPS, AMBER, GROMACS, and NAMD>100% of the top MD applications are GPU-accelerated>Key math libraries like FFT and BLAS>Up to 11 TFLOPS per second of single precision performance per GPU>Up to 732 GB per second of memory bandwidth per GPUView all related applications at:/molecular-dynamics-appsHOOMD-BLUEParticle dynamics package is written from the ground up for GPUsVERSION1.3.3ACCELERATED FEATURESCPU & GPU versions availableSCALABILITYMulti-GPU and Multi-NodeMORE INFORMATION/hoomd-blueLAMMPSClassical molecular dynamics packageVERSION2016ACCELERATED FEATURESLennard-Jones, Gay-Berne, Tersoff, many more potentialsSCALABILITYMulti-GPU and Multi-NodeMORE INFORMATION/lammpsGROMACSSimulation of biochemical molecules with complicated bond interactions VERSION5.1.2ACCELERATED FEATURESPME, Explicit, and Implicit Solvent SCALABILITYMulti-GPU and Multi-Node Scales to 4xP100MORE INFORMATION /gromacsAMBERSuite of programs to simulatemolecular dynamics on biomolecule VERSION16.3ACCELERATED FEATURESPMEMD Explicit Solvent & GB; Explicit& Implicit Solvent, REMD, aMD SCALABILITYMulti-GPU and Single-Node MORE INFORMATION /amberNAMDDesigned for high-performance simulation of large molecular systemsVERSION2.11ACCELERATED FEATURESFull electrostatics with PME and many simulation featuresSCALABILITYUp to 100M atom capable, Multi-GPU, Scales to 2xP100MORE INFORMATION/namdQuantum chemistry (QC) simulations are key to the discovery of new drugs and materials and consume a large part of the HPC data center's workload. 60% of the top QC applications are accelerated with GPUs today. When running QC applications, a data center's workload with Tesla P100 GPUs can save up to 40% in server acquisition cost.KEY FEATURES OF THE TESLA PLATFORM AND P100 FOR QC>Servers with P100 replace up to 36 CPU servers for applications such as VASP and LSMS>60% of the top QC applications are GPU-accelerated>Key math libraries like FFT and BLAS>Up to 5.3 TFLOPS per second of double precision performance per GPU>Up to 16 GB of memory capacity for large datasetsView all related applications at:/quantum-chemistry-appsVASPPackage for performing ab-initio quantum-mechanical molecular dynamics (MD) simulationsVERSION5.4.1ACCELERATED FEATURESRMM-DIIS, Blocked Davidson,K-points, and exact-exchangeSCALABILITYMulti-GPU and Multi-NodeMORE INFORMATION/vaspLSMSMaterials code for investigating the effects of temperature on magnetismVERSION3ACCELERATED FEATURES Generalized Wang-Landau methodSCALABILITYMulti-GPUMORE INFORMATION/lsmsFrom fusion energy to high energy particles, physics simulations span a wide range of applications in the HPC data center. Many of the top physics applications are GPU-accelerated, enabling insights previously not possible.A data center with Tesla P100 GPUs can save up to 70% in server acquisition cost when running GPU-accelerated physics applications.KEY FEATURES OF THE TESLA PLATFORM AND P100 FOR PHYSICS>Servers with P100 replace up to 50 CPU servers for applications such as GTC-P, QUDA, MILC and Chroma>Most of the top physics applications are GPU-accelerated>Up to 5.3 TFLOPS of double precision floating point performance>Up to 16 GB of memory capacity with up to 732 GB/s memory bandwidth View all related applications at:/physics-appsGTC-PA development code for optimization of plasma physicsVERSION2016ACCELERATED FEATURESPush, shift, and collisionSCALABILITYMulti-GPUMORE INFORMATION/gtc-pQUDAA library for Lattice Quantum Chromo Dynamics on GPUsVERSION2017ACCELERATED FEATURESAllSCALABILITYMulti-GPU and Multi-NodeMORE INFORMATION/qudaMILCLattice Quantum Chromodynamics(LQCD) codes simulate how elementalparticles are formed and bound bythe “strong force” to create largerparticles like protons and neutronsVERSION7.8.0ACCELERATED FEATURESStaggered fermions, Krylov solvers,and Gauge-link fatteningScales to 4xP100SCALABILITYMulti-GPU and Multi-NodeMORE INFORMATION/milcCHROMALattice Quantum Chromodynamics(LQCD)VERSION2016ACCELERATED FEATURESWilson-clover fermions, Krylovsolvers, and Domain-decompositionSCALABILITYMulti-GPUMORE INFORMATION/chroma APPLICATION PERFORMANCE GUIDE | PHYSICSGeoscience simulations are key to the discovery of oil and gas and performing geological modeling. Many of the top geoscience applications are accelerated with GPUs today. When running Geoscience applications, a data center with Tesla P100 GPUs can save up to 65% in server acquisition cost.KEY FEATURES OF THE TESLA PLATFORM AND P100 FOR GEOSCIENCE>Servers with P100 replace up to 50 CPU servers for applications such as RTM and SPECFEM 3D>Top Oil and Gas applications are GPU-accelerated>Up to 10.6 TFLOPS of single precision floating point performance>Up to 16 GB of memory capacity with up to 732 GB/s memory bandwidth View all related applications at:/oil-and-gas-appsRTMReverse time migration (RTM)modeling is a critical component inthe seismic processing workflow ofoil and gas explorationVERSION2016ACCELERATED FEATURESBatch algorithmSCALABILITYMulti-GPU and Multi-NodeSPECFEM 3DSimulates Seismic wave propagationVERSION7.0.0ACCELERATED FEATURESWilson-clover fermions, Krylovsolvers, and Domain-decompositionSCALABILITYMulti-GPU and Multi-NodeMORE INFORMATION/specfem3d-globe APPLICATION PERFORMANCE GUIDE | OIL AND GASSimulation is key to financial service firms offering the ability to drive their business faster, with better analytics at lower costs. Top finance applications are GPU-accelerated and can save up to 40% in server acquisition cost for a data center powered by Tesla P100 GPUs.KEY FEATURES OF THE TESLA PLATFORM AND P100 FOR FINANCE>Servers with P100 replace up to 12 CPU servers for applications such as STAC A2>Top finance applications are GPU-accelerated>Up to 5.3 TFLOPS of double precision floating point performance>Up to 16 GB of memory capacity with up to 732 GB/s memory bandwidth View all related applications at:/financial-appsSTAC A2Compute-intensive analyticworkloads involved in pricing and riskmanagementVERSION2016ACCELERATED FEATURESAllSCALABILITYMulti-GPU and Multi-NodeMORE INFORMATION/stac-a2 APPLICATION PERFORMANCE GUIDE | FINANCEDeep Learning is solving important scientific, enterprise, and consumer problems that seemed beyond our reach just a few years back. Every major deep learning framework is optimized for NVIDIA GPUs, enabling data scientists and researchers to leverage artificial intelligence for their work. When running deep learning frameworks, a data center with Tesla P100 GPUs can save up to 70% in server acquisition cost.KEY FEATURES OF THE TESLA PLATFORM AND P100 FOR DEEP LEARNING TRAINING>Caffe, TensorFlow, and CNTK are up to 3x faster with Tesla P100 compared to K80>100% of the top deep learning frameworks are GPU-accelerated>Up to 21.2 TFLOPS of native half precision floating point>Up to 16 GB of memory capacity with up to 732 GB/s memory bandwidth View all related applications at:/deep-learning-appsCAFFEA popular, GPU-accelerated DeepLearning framework developed at UCBerkeleyVERSION0.16ACCELERATED FEATURESFull framework acceleratedSCALABILITYMulti-GPUMORE INFORMATION/caffe APPLICATION PERFORMANCE GUIDE | DEEP LEARNINGTESLA P100 PRODUCT SPECIFICATIONSNVIDIA Tesla P100 forAssumptions and DisclaimersThe percentage of top applications that are GPU-accelerated is from top 50 app list in the i360 report: H PC Application Support for GPU Computing. Calculation of throughput and cost savings assumes a workload profile where applications benchmarked in the domain take equal compute cycles.。
cuda发展历程
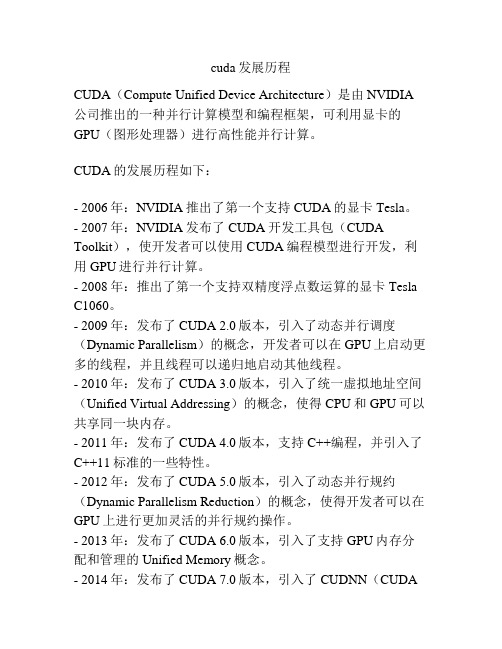
cuda发展历程CUDA(Compute Unified Device Architecture)是由NVIDIA 公司推出的一种并行计算模型和编程框架,可利用显卡的GPU(图形处理器)进行高性能并行计算。
CUDA的发展历程如下:- 2006年:NVIDIA推出了第一个支持CUDA的显卡Tesla。
- 2007年:NVIDIA发布了CUDA开发工具包(CUDA Toolkit),使开发者可以使用CUDA编程模型进行开发,利用GPU进行并行计算。
- 2008年:推出了第一个支持双精度浮点数运算的显卡Tesla C1060。
- 2009年:发布了CUDA 2.0版本,引入了动态并行调度(Dynamic Parallelism)的概念,开发者可以在GPU上启动更多的线程,并且线程可以递归地启动其他线程。
- 2010年:发布了CUDA 3.0版本,引入了统一虚拟地址空间(Unified Virtual Addressing)的概念,使得CPU和GPU可以共享同一块内存。
- 2011年:发布了CUDA 4.0版本,支持C++编程,并引入了C++11标准的一些特性。
- 2012年:发布了CUDA 5.0版本,引入了动态并行规约(Dynamic Parallelism Reduction)的概念,使得开发者可以在GPU上进行更加灵活的并行规约操作。
- 2013年:发布了CUDA 6.0版本,引入了支持GPU内存分配和管理的Unified Memory概念。
- 2014年:发布了CUDA 7.0版本,引入了CUDNN(CUDADeep Neural Network library),提供了一套高性能的深度学习库,用于加速神经网络的训练和推断。
- 2015年:发布了CUDA 7.5版本,引入了Dynamic Parallelism的改进和扩展,进一步提高了GPU的可编程性和灵活性。
- 2016年:发布了CUDA 8.0版本,引入了支持并行计算任务调度的异步处理流(Stream)机制,提供了更好的任务并行性和资源利用率。
CUDA 4.0 用户手册说明书
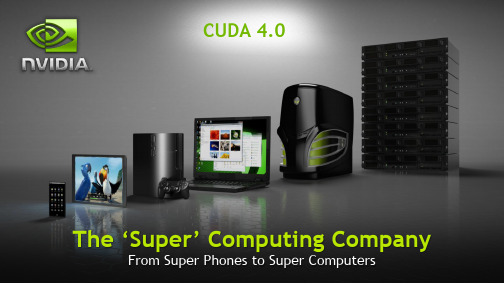
CUDA 4.0The ‘Super’ Computing Company From Super Phones to Super ComputersCUDA 4.0 for Broader Developer AdoptionCUDA 4.0Application Porting Made SimplerRapid Application PortingUnified Virtual Addressing Faster Multi-GPU ProgrammingNVIDIA GPUDirect™ 2.0 Easier Parallel Programming in C++ThrustCUDA 4.0: Highlights•Share GPUs across multiple threads •Single thread access to all GPUs •No-copy pinning of system memory •New CUDA C/C++ features•Thrust templated primitives library •NPP image/video processing library •Layered TexturesEasier Parallel Application Porting•Auto Performance Analysis •C++ Debugging•GPU Binary Disassembler•cuda-gdb for MacOSNew & ImprovedDeveloper Tools•NVIDIA GPUDirect™ v2.0•Peer-to-Peer Access •Peer-to-Peer Transfers•Unified Virtual AddressingFasterMulti-GPU ProgrammingEasier Porting of Existing ApplicationsShare GPUs across multiple threadsEasier porting of multi-threaded appspthreads / OpenMP threads share a GPULaunch concurrent kernels fromdifferent host threadsEliminates context switching overheadNew, simple context management APIsOld context migration APIs still supported Single thread access to all GPUs Each host thread can now access allGPUs in the systemOne thread per GPU limitation removedEasier than ever for applications totake advantage of multi-GPUSingle-threaded applications can nowbenefit from multiple GPUsEasily coordinate work across multipleGPUs (e.g. halo exchange)No-copy Pinning of System MemoryReduce system memory usage and CPU memcpy() overheadEasier to add CUDA acceleration to existing applications Just register malloc’d system memory for async operations and then call cudaMemcpy() as usualAll CUDA-capable GPUs on Linux or WindowsRequires Linux kernel 2.6.15+ (RHEL 5)Before No-copy Pinning With No-copy Pinning Extra allocation and extra copy requiredJust register and go!cudaMallocHost(b) memcpy(b, a) memcpy(a, b) cudaFreeHost(b)cudaHostRegister(a)cudaHostUnregister(a)cudaMemcpy() to GPU, launch kernels, cudaMemcpy() from GPU malloc(a)New CUDA C/C++ Language FeaturesC++ new/deleteDynamic memory managementC++ virtual functionsEasier porting of existing applicationsInline PTXEnables assembly-level optimizationC++ Templatized Algorithms & Data Structures (Thrust) Powerful open source C++ parallel algorithms & data structures Similar to C++ Standard Template Library (STL)Automatically chooses the fastest code path at compile time Divides work between GPUs and multi-core CPUsParallel sorting @ 5x to 100x fasterData Structures •thrust::device_vector •thrust::host_vector •thrust::device_ptr •Etc.Algorithms •thrust::sort •thrust::reduce •thrust::exclusive_scan •Etc.NVIDIA Performance Primitives (NPP) library10x to 36x faster image processingInitial focus on imaging and video related primitivesGPU-Accelerated Image ProcessingData exchange & initialization Set, Convert, CopyConstBorder, Copy, Transpose, SwapChannelsColor ConversionRGB To YCbCr (& vice versa),ColorTwist, LUT_LinearThreshold & Compare OpsThreshold, CompareStatisticsMean, StdDev, NormDiff, MinMax,Histogram,SqrIntegral, RectStdDev Filter FunctionsFilterBox, Row, Column, Max, Min, Median, Dilate, Erode, SumWindowColumn/RowGeometry TransformsMirror, WarpAffine / Back/ Quad,WarpPerspective / Back / Quad, ResizeArithmetic & Logical OpsAdd, Sub, Mul, Div, AbsDiffJPEGDCTQuantInv/Fwd, QuantizationTableLayered Textures – Faster Image ProcessingIdeal for processing multiple textures with same size/format Large sizes supported on Tesla T20 (Fermi) GPUs (up to 16k x 16k x 2k)e.g. Medical Imaging, Terrain Rendering (flight simulators), etc.Faster PerformanceReduced CPU overhead: single binding for entire texture arrayFaster than 3D Textures: more efficient filter cachingFast interop with OpenGL / Direct3D for each layerNo need to create/manage a texture atlasNo sampling artifactsLinear/Bilinear filtering applied only within a layerCUDA 4.0: Highlights•Auto Performance Analysis •C++ Debugging•GPU Binary Disassembler•cuda-gdb for MacOSNew & ImprovedDeveloper Tools•Share GPUs across multiple threads •Single thread access to all GPUs •No-copy pinning of system memory •New CUDA C/C++ features•Thrust templated primitives library •NPP image/video processing library •Layered TexturesEasier Parallel Application Porting•NVIDIA GPUDirect™ v2.0•Peer-to-Peer Access •Peer-to-Peer Transfers•Unified Virtual AddressingFasterMulti-GPU ProgrammingNVIDIA GPUDirect™:Towards Eliminating the CPU Bottleneck•Direct access to GPU memory for 3rd party devices•Eliminates unnecessary sys mem copies & CPU overhead•Supported by Mellanox and Qlogic •Up to 30% improvement in communication performanceVersion 1.0for applications that communicateover a network•Peer-to-Peer memory access, transfers & synchronization•Less code, higher programmer productivityVersion 2.0for applications that communicatewithin a nodeBefore NVIDIA GPUDirect™ v2.0Required Copy into Main MemoryGPU 1GPU 1 MemoryGPU 2GPU 2 MemoryPCI-eCPUChip System MemoryTwo copies required:1. cudaMemcpy(GPU2, sysmem)2. cudaMemcpy(sysmem, GPU1)NVIDIA GPUDirect™ v2.0:Peer-to-Peer CommunicationDirect Transfers between GPUsGPU 1GPU 1 MemoryGPU 2GPU 2 MemoryPCI-eCPUChip System MemoryOnly one copy required:1. cudaMemcpy(GPU2, GPU1)GPUDirect v2.0: Peer-to-Peer CommunicationDirect communication between GPUsFaster - no system memory copy overheadMore convenient multi-GPU programmingDirect TransfersCopy from GPU0 memory to GPU1 memoryWorks transparently with UVADirect AccessGPU0 reads or writes GPU1 memory (load/store)Supported on Tesla 20-series and other Fermi GPUs 64-bit applications on Linux and Windows TCCUnified Virtual AddressingEasier to Program with Single Address SpaceNo UVA: Multiple Memory SpacesUVA : Single Address SpaceSystem MemoryCPU GPU 0 GPU 0 MemoryGPU 1 GPU 1 MemorySystem MemoryCPU GPU 0 GPU 0 Memory GPU 1GPU 1 MemoryPCI-ePCI-e0x0000 0xFFFF0x0000 0xFFFF0x0000 0xFFFF0x00000xFFFFUnified Virtual AddressingOne address space for all CPU and GPU memoryDetermine physical memory location from pointer valueEnables libraries to simplify their interfaces (e.g. cudaMemcpy)Supported on Tesla 20-series and other Fermi GPUs64-bit applications on Linux and Windows TCCBefore UVA With UVASeparate options for each permutation One function handles all cases cudaMemcpyHostToHost cudaMemcpyHostToDevice cudaMemcpyDeviceToHost cudaMemcpyDeviceToDevicecudaMemcpyDefault(data location becomes an implementation detail)CUDA 4.0: Highlights•NVIDIA GPUDirect™ v2.0•Peer-to-Peer Access •Peer-to-Peer Transfers•Unified Virtual AddressingFasterMulti-GPU Programming•Share GPUs across multiple threads •Single thread access to all GPUs •No-copy pinning of system memory •New CUDA C/C++ features•Thrust templated primitives library •NPP image/video processing library •Layered TexturesEasier Parallel Application Porting•Auto Performance Analysis •C++ Debugging•GPU Binary Disassembler•cuda-gdb for MacOSNew & ImprovedDeveloper ToolsAutomated Performance Analysis in Visual ProfilerSummary analysis & hintsSessionDeviceContextKernelNew UI for kernel analysisIdentify limiting factorAnalyze instruction throughputAnalyze memory throughputAnalyze kernel occupancyNew Features in cuda-gdbFermidisassemblyBreakpoints on all instances of templated functionsC++ symbols shown in stack trace viewNow available for both Linux and MacOSinfo cuda threadsautomatically updated in DDD(cuobjdump)cuda-gdb Now Available for MacOSDetails @ /object/cuda-gdb.htmlNVIDIA Parallel Nsight™ Pro 1.5ProfessionalCUDA Debugging ✓Compute Analyzer ✓CUDA / OpenCL Profiling ✓Tesla Compute Cluster (TCC) Debugging ✓Tesla Support: C1050/S1070 or higher ✓Quadro Support: G9x or higher ✓Windows 7, Vista and HPC Server 2008 ✓Visual Studio 2008 SP1 and Visual Studio 2010 ✓OpenGL and OpenCL Analyzer ✓DirectX 10 & 11 Analyzer, Debugger & Graphics✓inspectorGeForce Support: 9 series or higher ✓CUDA Registered Developer ProgramAll GPGPU developers should become NVIDIA Registered Developers Benefits include:Early Access to Pre-Release SoftwareBeta software and librariesCUDA 4.0 Release Candidate available nowSubmit & Track Issues and BugsInteract directly with NVIDIA QA engineersNew benefits in 2011Exclusive Q&A Webinars with NVIDIA EngineeringExclusive deep dive CUDA training webinarsIn-depth engineering presentations on pre-release softwareAdditional Information…CUDA Features OverviewCUDA Developer Resources from NVIDIACUDA 3rd Party EcosystemPGI CUDA x86GPU Computing Research & EducationNVIDIA Parallel Developer ProgramGPU Technology Conference 2011CUDA Features OverviewNew in CUDA 4.0Hardware Features ECC Memory Double PrecisionNative 64-bit Architecture Concurrent Kernel Execution Dual Copy Engines6GB per GPU supportedOperating System Support MS Windows 32/64 Linux 32/64 Mac OS X 32/64Designed for HPC Cluster Management GPUDirectT esla Compute Cluster (TCC) Multi-GPU supportGPUDirect tm (v 2.0)Peer-Peer CommunicationPlatformC supportNVIDIA C CompilerCUDA C Parallel Extensions Function Pointers Recursion Atomics malloc/freeC++ supportClasses/Objects Class Inheritance PolymorphismOperator Overloading Class Templates Function Templates Virtual Base Classes NamespacesFortran supportCUDA Fortran (PGI)Unified Virtual Addressing C++ new/deleteC++ Virtual FunctionsProgramming ModelNVIDIA Library SupportComplete math.h Complete BLAS Library (1, 2 and 3)Sparse Matrix Math LibraryRNG LibraryFFT Library (1D, 2D and 3D)Video Decoding Library (NVCUVID)Video Encoding Library (NVCUVENC)Image Processing Library (NPP)Video Processing Library (NPP) 3rd Party Math Libraries CULA T ools (EM Photonics) MAGMA Heterogeneous LAPACK IMSL (Rogue Wave) VSIPL (GPU VSIPL) Thrust C++ LibraryTemplated Performance Primitives LibraryParallel LibrariesNVIDIA Developer T oolsParallel Nsightfor MS Visual Studio cuda-gdb Debugger with multi-GPU support CUDA/OpenCL Visual Profiler CUDA Memory Checker CUDA DisassemblerGPU Computing SDKNVMLCUPTI 3rd Party Developer T ools Allinea DDT RogueWave /T otalview Vampir T auCAPS HMPPParallel Nsight Pro 1.5Development T oolsCUDA Developer Resources from NVIDIALibraries and EnginesMath LibrariesCUFFT, CUBLAS, CUSPARSE, CURAND, math.h 3rd Party LibrariesCULA LAPACK, VSIPL NPP Image LibrariesPerformance primitives for imagingApp Acceleration Engines Ray Tracing: Optix, iRayVideo Encoding / DecodingNVCUVENC / VCUVIDDevelopmentT oolsCUDA T oolkit Complete GPU computing development kit cuda-gdbGPU hardware debuggingcuda-memcheck Identifies memory errors cuobjdumpCUDA binary disassembler Visual ProfilerGPU hardware profiler for CUDA C and OpenCLParallel Nsight ProIntegrated developmentenvironment for Visual StudioSDKs and Code SamplesGPU Computing SDKCUDA C/C++, DirectCompute, OpenCL code samples and documentationBooksCUDA by Example GPU Computing Gems Programming Massively Parallel Processors Many more…Optimization GuidesBest Practices for GPU computing and graphics developmentCUDA 3rd Party EcosystemParallel DebuggersMS Visual Studio withParallel Nsight ProAllinea DDT DebuggerT otalView Debugger Parallel Performance T ools ParaT ools VampirTrace TauCUDA Performance T ools PAPIHPC T oolkit Cloud Providers Amazon EC2Peer 1OEM’sDellHPIBMInfiniband Providers MellanoxQLogicCluster Management Platform HPCPlatform Symphony Bright Cluster manager Ganglia Monitoring System Moab Cluster SuiteAltair PBS ProJob SchedulingAltair PBSproTORQUEPlatform LSFMPI LibrariesComing soon…PGI CUDA FortranPGI Accelerator (C/Fortran) PGI CUDA x86CAPS HMPPpyCUDA (Python) Tidepowerd (C#) JCuda (Java)Khronos OpenCLMicrosoft DirectCompute3rd Party Math Libraries CULA T ools (EM Photonics) MAGMA Heterogeneous LAPACK IMSL (Rogue Wave)VSIPL (GPU VSIPL)NAGCluster T ools Parallel LanguageSolutions & APIs Parallel T ools Compute Platform ProvidersPGI CUDA x86 Compiler BenefitsDeploy CUDA apps onlegacy systems without GPUsLess code maintenancefor developersTimelineApril/May 1.0 initial releaseDevelop, debug, test functionalityAug 1.1 performance releaseMulticore, SSE/AVX supportProven Research Vision John Hopkins University Nanyan University Technical University-Czech CSIRO SINTEF HP Labs ICHECBarcelona SuperComputer Center Clemson University Fraunhofer SCAIKarlsruhe Institute Of TechnologyWorld Class Research Leadership and Teaching University of Cambridge Harvard University University of Utah University of Tennessee University of MarylandUniversity of Illinois at Urbana-Champaign Tsinghua UniversityTokyo Institute of Technology Chinese Academy of Sciences National Taiwan University Georgia Institute of TechnologyGPGPU Education 350+ UniversitiesAcademic Partnerships / FellowshipsGPU Computing Research & EducationMass. Gen. Hospital/NE Univ North Carolina State University Swinburne University of Tech. Techische Univ. Munich UCLAUniversity of New Mexico University Of Warsaw-ICMVSB-Tech University of Ostrava And more coming shortly.“Don’t kid yourself. GPUs are a game-changer.” said Frank Chambers, a GTC conference attendee shopping for GPUs for his finite element analysis work. “What we are seeing here is like going from propellers to jet engines. That made transcontinental flights routine. Wide access to this kind of computing power is making things like artificial retinas possible, and that wasn’t predicted to happen until 2060.”- Inside HPC (Sept 22, 2010)GPU Technology Conference 2011October 11-14 | San Jose, CAThe one event you can’t afford to miss▪Learn about leading-edge advances in GPU computing▪Explore the research as well as the commercial applications▪Discover advances in computational visualization▪T ake a deep dive into parallel programmingWays to participate▪Speak – share your work and gain exposure as a thought leader▪Register – learn from the experts and network with your peers▪Exhibit/Sponsor – promote your company as a key player in the GPU ecosystem。
CUDA C++ 编程指南版本12.0 NVIDIA 2023年2月21日说明书
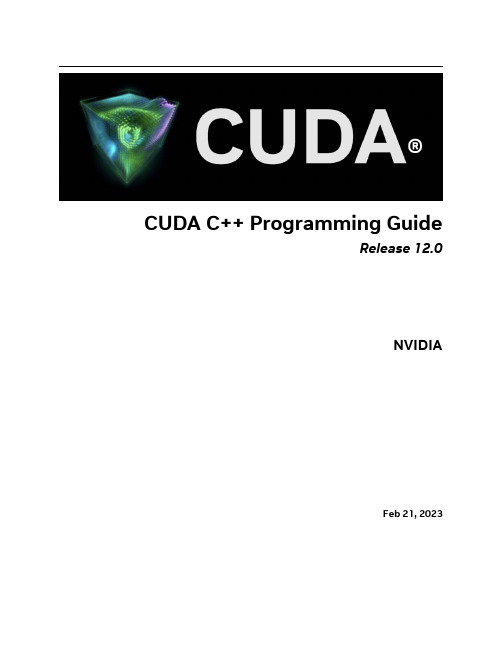
Just-in-Time Compilation . . . . . . . . . . . . . . . . . . . . . . . . . . . . . . . . . . . . 22
6.1.2 Binary Compatibility . . . . . . . . . . . . . . . . . . . . . . . . . . . . . . . . . . . . . . 22
5.3 Memory Hierarchy . . . . . . . . . . . . . . . . . . . . . . . . . . . . . . . . . . . . . . . . . . . . 16
5.4 Heterogeneous Programming . . . . . . . . . . . . . . . . . . . . . . . . . . . . . . . . . . . . . 17
6.1.1 Compilation Workflow . . . . . . . . . . . . . . . . . . . . . . . . . . . . . . . . . . . . . 22
Offline Compilation . . . . . . . . . . . . . . . . . . . . . . . . . . . . . . . . . . . . . . . 22
6.2.8 Asynchronous Concurrent Execution . . . . . . . . . . . . . . . . . . . . . . . . . . . . 45 Concurrent Execution between Host and Device . . . . . . . . . . . . . . . . . . . . . 46 Concurrent Kernel Execution . . . . . . . . . . . . . . . . . . . . . . . . . . . . . . . . . 46 Overlap of Data Transfer and Kernel Execution . . . . . . . . . . . . . . . . . . . . . . 47 Concurrent Data Transfers . . . . . . . . . . . . . . . . . . . . . . . . . . . . . . . . . . . 47 Streams . . . . . . . . . . . . . . . . . . . . . . . . . . . . . . . . . . . . . . . . . . . . . . 47 Creation and Destruction . . . . . . . . . . . . . . . . . . . . . . . . . . . . . . . 47 Default Stream . . . . . . . . . . . . . . . . . . . . . . . . . . . . . . . . . . . . . . 48 Explicit Synchronization . . . . . . . . . . . . . . . . . . . . . . . . . . . . . . . . 49 Implicit Synchronization . . . . . . . . . . . . . . . . . . . . . . . . . . . . . . . . 49 Overlapping Behavior . . . . . . . . . . . . . . . . . . . . . . . . . . . . . . . . . . 49 Host Functions (Callbacks) . . . . . . . . . . . . . . . . . . . . . . . . . . . . . . . 50 Stream Priorities . . . . . . . . . . . . . . . . . . . . . . . . . . . . . . . . . . . . . 51 Programmatic Dependent Launch and Synchronization . . . . . . . . . . . . . . . . . 51 Background . . . . . . . . . . . . . . . . . . . . . . . . . . . . . . . . . . . . . . . . 51 API Description . . . . . . . . . . . . . . . . . . . . . . . . . . . . . . . . . . . . . . 52 CUDA Graphs . . . . . . . . . . . . . . . . . . . . . . . . . . . . . . . . . . . . . . . . . . . 53 Graph Structure . . . . . . . . . . . . . . . . . . . . . . . . . . . . . . . . . . . . . 54 Creating a Graph Using Graph APIs . . . . . . . . . . . . . . . . . . . . . . . . . 55 Creating a Graph Using Stream Capture . . . . . . . . . . . . . . . . . . . . . . 56 Updating Instantiated Graphs . . . . . . . . . . . . . . . . . . . . . . . . . . . . . 58 Using Graph APIs . . . . . . . . . . . . . . . . . . . . . . . . . . . . . . . . . . . . . 61 Device Graph Launch . . . . . . . . . . . . . . . . . . . . . . . . . . . . . . . . . . 62 Events . . . . . . . . . . . . . . . . . . . . . . . . . . . . . . . . . . . . . . . . . . . . . . . . 69 Creation and Destruction . . . . . . . . . . . . . . . . . . . . . . . . . . . . . . . 69 Elapsed Time . . . . . . . . . . . . . . . . . . . . . . . . . . . . . . . . . . . . . . . 70 Synchronous Calls . . . . . . . . . . . . . . . . . . . . . . . . . . . . . . . . . . . . . . . . 70
NVIDIA HPC SDK安装指南说明书
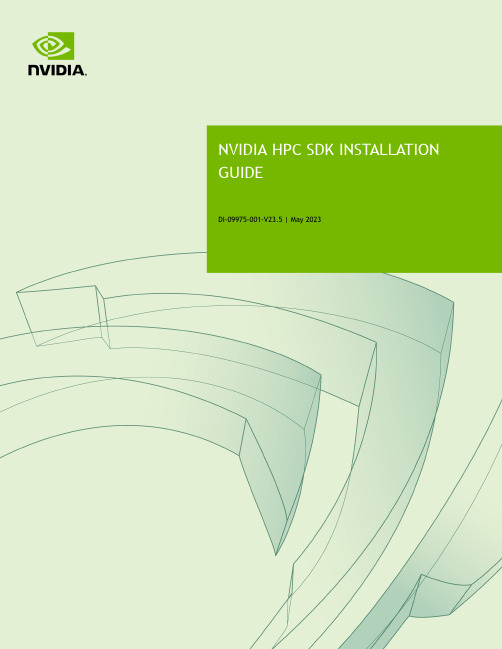
DI-09975-001-V23.5 | May 2023TABLE OF CONTENTS Chapter 1. Installations on Linux (1)1.1. Prepare to Install on Linux (1)1.2. Installation Steps for Linux (2)1.3. End-user Environment Settings (4)This section describes how to install the HPC SDK in a generic manner on Linux x86_64, OpenPOWER, or Arm Server systems with NVIDIA GPUs. It covers both local and network installations.For a complete description of supported processors, Linux distributions, and CUDA versions please see the HPC SDK Release Notes.1.1. Prepare to Install on LinuxLinux installations require some version of the GNU Compiler Collection (including gcc, g++, and gfortran compilers) to be installed and in your $PATH prior to installing HPC SDK software. For HPC compilers to produce 64-bit executables, a 64-bit gcc compiler must be present. For C++ compiling and linking, the same must be true for g++. To determine if such a compiler is installed on your system, do the following:1.Create a hello.c program.#include <stdio.h>int main() {printf("hello, world!\n");return 0;}pile with the -m64 option to create a 64-bit executable.$ gcc -m64 -o hello_64_c hello.cRun the file command on the produced executable. The output should looksimilar to the following:$ file ./hello_64_chello_64_c: ELF 64-bit LSB executable, x86-64, version 1 (SYSV), forGNU/Linux 2.6.9, dynamically linked (uses shared libs), for GNU/Linux2.6.9, not stripped3.For support with C++ compilation, g++ version4.4 is required at a minimum. Amore recent version will suffice. Create a hello.cpp program and invoke g++ with the -m64 argument. Make sure you are able to compile, link, and run the simple hello.cpp program first before proceeding.#include <iostream>int main() {std::cout << "hello, world!\n";return 0;}$ g++ -m64 -o hello_64_cpp hello.cppThe file command on the hello_64_cpp binary should produce similar results as the C example.Any changes to your gcc compilers requires you to reinstall the HPC SDK.For cluster installations, access to all the nodes is required. In addition, you should be able to connect between nodes using rsh or ssh, including to/from the same node you are on. The hostnames for each node should be the same as those in the cluster machine list for the system (machines.LINUX file).In a typical local installation, the default installation base directory is /opt/nvidia/ hpc_sdk.If you choose to perform a network installation, you should specify:‣ A shared file system for the installation base directory. All systems using the compilers should use a common pathname.‣ A second directory name that is local to each of the systems where the HPC compilers and tools are used. This local directory contains the libraries to use when compiling and running on that machine. Use the same pathname on every system, and point to a private (i.e. non-shared) directory location.This directory selection approach allows a network installation to support a networkof machines running different versions of Linux. If all the platforms are identical, the shared installation location can perform a standard installation that all can use.T o Prepare for the Installation:‣After downloading the HPC SDK installation package, bring up a shell command window on your system.The installation instructions assume you are using csh, sh, ksh, bash, or somecompatible shell. If you are using a shell that is not compatible with one of these shells, appropriate modifications are necessary when setting environment variables.‣Verify you have enough free disk space for the HPC SDK installation.‣The uncompressed installation packages requires 9.5 GB of total free disk space for the HPC SDK slim packages, and 20 GB for the HPC SDK multi pacakges.1.2. Installation Steps for LinuxFollow these instructions to install the software:1.Unpack the HPC SDK software.In the instructions that follow, replace <tarfile> with the name of the file that you downloaded.Use the following command sequence to unpack the tar file before installation.% tar xpfz <tarfile>.tar.gzThe tar file will extract an install script and an install_components folder to a directory with the same name as the tar file.2.Run the installation script(s).Install the compilers by running [sudo] ./install from the <tarfile> directory.Important The installation script must run to completion to properly install thesoftware.To successfully run this script to completion, be prepared to do the following:‣Determine whether to perform a local installation or a network installation.‣Define where to place the installation directory. The default is /opt/nvidia/ hpc_sdk.Linux users have the option of automating the installation of the HPC compilersuite without interacting with the usual prompts. This may be useful in a largeinstitutional setting, for example, where automated installation of HPC compilersover many systems can be efficiently done with a script.To enable the silent installation feature, set the appropriate environment variables prior to running the installation script. These variables are as follows:The HPC SDK installation scripts install all of the binaries, tools, and libraries for the HPC SDK in the appropriate subdirectories within the specified installation directory.3.Review documentation.NVIDIA HPC Compiler documentation is available online in both HTML and PDF formats.plete network installation tasks.Skip this step if you are not installing a network installation.For a network installation, you must run the local installation script on each system on the network where the compilers and tools will be available for use.If your installation base directory is /opt/nvidia/hpc_sdk and /usr/nvidia/ shared/23.5 is the common local directory, then run the following commands on each system on the network./opt/nvidia/hpc_sdk/$NVARCH/23.5/compilers/bin/makelocalrc -x /opt/nvidia/ hpc_sdk/$NVARCH/23.5 \-net /usr/nvidia/shared/23.5These commands create a system-dependent file localrc.machinename inthe /opt/nvidia/hpc_sdk/$NVARCH/23.5/compilers/bin directory. The commands also create the following three directories containing libraries and shared objects specific to the operating system and system libraries on that machine: /usr/nvidia/shared/23.5/lib/usr/nvidia/shared/23.5/liblf/usr/nvidia/shared/23.5/lib64The makelocalrc command does allow the flexibility of having local directorieswith different names on different machines. However, using the same directory ondifferent machines allows users to easily move executables between systems thatuse NVIDIA-supplied shared libraries.Installation of the HPC SDK for Linux is now complete. For assistance with difficulties related to the installation, please reach out on the NVIDIA Developer Forums.The following sections contain information detailing the directory structure of the HPC SDK installation, and instructions for end-users to initialize environment and path settings to use the compilers and tools.1.3. End-user Environment SettingsAfter the software installation is complete, each user’s shell environment must be initialized to use the HPC SDK.Each user must issue the following sequence of commands to initialize the shellenvironment before using the HPC SDK.The HPC SDK keeps version numbers under an architecture type directory, e.g.Linux_x86_64/23.5. The name of the architecture is in the form of `uname -s`_`uname -m`. For OpenPOWER and Arm Server platforms the expected architecture name is "Linux_ppc64le" and "Linux_aarch64" respectively. The guide below sets the value of thenecessary uname commands to "NVARCH", but you can explicitly specify the name of the architecture if desired.To make the HPC SDK available:In csh, use these commands:% setenv NVARCH `uname -s`_`uname -m`% setenv NVCOMPILERS /opt/nvidia/hpc_sdk% setenv MANPATH "$MANPATH":$NVCOMPILERS/$NVARCH/23.5/compilers/man% set path = ($NVCOMPILERS/$NVARCH/23.5/compilers/bin $path)In bash, sh, or ksh, use these commands:$ NVARCH=`uname -s`_`uname -m`; export NVARCH$ NVCOMPILERS=/opt/nvidia/hpc_sdk; export NVCOMPILERS$ MANPATH=$MANPATH:$NVCOMPILERS/$NVARCH/23.5/compilers/man; export MANPATH $ PATH=$NVCOMPILERS/$NVARCH/23.5/compilers/bin:$PATH; export PATHOnce the 64-bit compilers are available, you can make the OpenMPI commands and man pages accessible using these commands.% set path = ($NVCOMPILERS/$NVARCH/23.5/comm_libs/mpi/bin $path)% setenv MANPATH "$MANPATH":$NVCOMPILERS/$NVARCH/23.5/comm_libs/mpi/manAnd the equivalent in bash, sh, and ksh:$ export PATH=$NVCOMPILERS/$NVARCH/23.5/comm_libs/mpi/bin:$PATH$ export MANPATH=$MANPATH:$NVCOMPILERS/$NVARCH/23.5/comm_libs/mpi/manNoticeALL NVIDIA DESIGN SPECIFICATIONS, REFERENCE BOARDS, FILES, DRAWINGS, DIAGNOSTICS, LISTS, AND OTHER DOCUMENTS (TOGETHER AND SEPARATEL Y, "MATERIALS") ARE BEING PROVIDED "AS IS." NVIDIA MAKES NO WARRANTIES, EXPRESSED, IMPLIED, STATUTORY, OR OTHERWISE WITH RESPECT TO THE MATERIALS, AND EXPRESSL Y DISCLAIMS ALL IMPLIED WARRANTIES OF NONINFRINGEMENT, MERCHANTABILITY, AND FITNESS FOR A PARTICULAR PURPOSE.Information furnished is believed to be accurate and reliable. However, NVIDIA Corporation assumes no responsibility for the consequences of use of such information or for any infringement of patents or other rights of third parties that may result from its use. No license is granted by implication of otherwise under any patent rights of NVIDIA Corporation. Specifications mentioned in this publication are subject to change without notice. This publication supersedes and replaces all other information previously supplied. NVIDIA Corporation products are not authorized as critical components in life support devices or systems without express written approval of NVIDIA Corporation.TrademarksNVIDIA, the NVIDIA logo, CUDA, CUDA-X, GPUDirect, HPC SDK, NGC, NVIDIA Volta, NVIDIA DGX, NVIDIA Nsight, NVLink, NVSwitch, and T esla are trademarks and/ or registered trademarks of NVIDIA Corporation in the U.S. and other countries. Other company and product names may be trademarks of the respective companies with which they are associated.Copyright© 2013–2023 NVIDIA Corporation. All rights reserved.NVIDIA HPC SDK。
cuda .c后缀 -回复
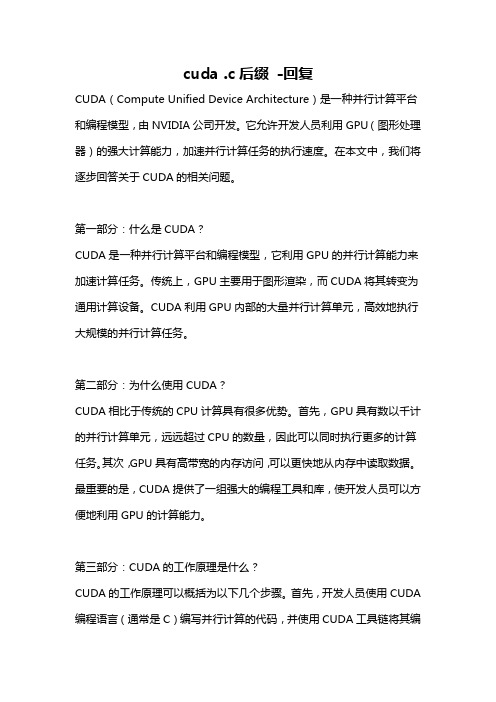
cuda .c后缀-回复CUDA(Compute Unified Device Architecture)是一种并行计算平台和编程模型,由NVIDIA公司开发。
它允许开发人员利用GPU(图形处理器)的强大计算能力,加速并行计算任务的执行速度。
在本文中,我们将逐步回答关于CUDA的相关问题。
第一部分:什么是CUDA?CUDA是一种并行计算平台和编程模型,它利用GPU的并行计算能力来加速计算任务。
传统上,GPU主要用于图形渲染,而CUDA将其转变为通用计算设备。
CUDA利用GPU内部的大量并行计算单元,高效地执行大规模的并行计算任务。
第二部分:为什么使用CUDA?CUDA相比于传统的CPU计算具有很多优势。
首先,GPU具有数以千计的并行计算单元,远远超过CPU的数量,因此可以同时执行更多的计算任务。
其次,GPU具有高带宽的内存访问,可以更快地从内存中读取数据。
最重要的是,CUDA提供了一组强大的编程工具和库,使开发人员可以方便地利用GPU的计算能力。
第三部分:CUDA的工作原理是什么?CUDA的工作原理可以概括为以下几个步骤。
首先,开发人员使用CUDA 编程语言(通常是C)编写并行计算的代码,并使用CUDA工具链将其编译成机器代码。
然后,开发人员将数据从主机(CPU)内存复制到设备(GPU)内存中。
接下来,开发人员将并行计算的任务分解成多个线程块,每个线程块包含多个线程。
每个线程独立地执行计算任务,并访问设备内存中的数据。
最后,开发人员将计算结果从设备内存复制回主机内存,并进行后续处理。
第四部分:如何使用CUDA进行并行计算?要使用CUDA进行并行计算,首先需要安装NVIDIA的显卡驱动和CUDA 开发工具包。
然后,开发人员可以使用CUDA编程语言编写并行计算的代码。
在代码中,需要使用特定的语法和API来定义并行计算任务,并操作设备内存中的数据。
编写完代码后,使用CUDA工具链进行编译,并将生成的可执行文件在GPU上运行。
cuda饱和乘法
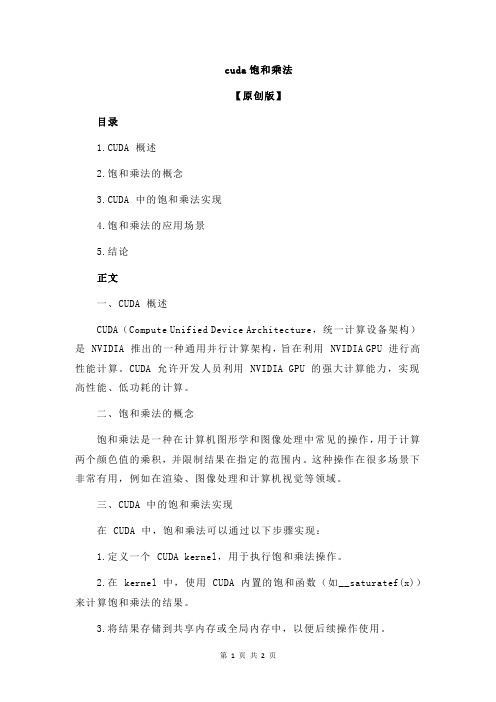
cuda饱和乘法【原创版】目录1.CUDA 概述2.饱和乘法的概念3.CUDA 中的饱和乘法实现4.饱和乘法的应用场景5.结论正文一、CUDA 概述CUDA(Compute Unified Device Architecture,统一计算设备架构)是 NVIDIA 推出的一种通用并行计算架构,旨在利用 NVIDIA GPU 进行高性能计算。
CUDA 允许开发人员利用 NVIDIA GPU 的强大计算能力,实现高性能、低功耗的计算。
二、饱和乘法的概念饱和乘法是一种在计算机图形学和图像处理中常见的操作,用于计算两个颜色值的乘积,并限制结果在指定的范围内。
这种操作在很多场景下非常有用,例如在渲染、图像处理和计算机视觉等领域。
三、CUDA 中的饱和乘法实现在 CUDA 中,饱和乘法可以通过以下步骤实现:1.定义一个 CUDA kernel,用于执行饱和乘法操作。
2.在 kernel 中,使用 CUDA 内置的饱和函数(如__saturatef(x))来计算饱和乘法的结果。
3.将结果存储到共享内存或全局内存中,以便后续操作使用。
四、饱和乘法的应用场景饱和乘法在很多实际应用中都有广泛的应用,例如:1.计算机图形学:在渲染管线中,饱和乘法用于计算纹理和颜色的乘积,以实现更好的渲染效果。
2.图像处理:在图像处理中,饱和乘法可以用于实现颜色调整、滤波等操作。
3.计算机视觉:在计算机视觉领域,饱和乘法可以用于实现图像增强、特征提取等任务。
五、结论CUDA 作为一种通用并行计算架构,提供了强大的计算能力。
在 CUDA 中实现饱和乘法,可以充分利用 GPU 的计算能力,实现高效、快速的计算。
NVIDIA HPC SDK 23.5版发布说明说明书

RN-09976-001-V23.5 | May 2023TABLE OF CONTENTS Chapter 1. What's New (1)Chapter 2. Release Component Versions (2)Chapter 3. Supported Platforms (4)3.1. Platform Requirements for the HPC SDK (4)3.2. Supported CUDA T oolchain Versions (5)Chapter 4. Known Limitations (6)Chapter 5. Deprecations and Changes (7)LIST OF TABLEST able 1 HPC SDK Release Components (2)T able 2 HPC SDK Platform Requirements (4)Welcome to the 23.5 version of the NVIDIA HPC SDK, a comprehensive suite of compilers and libraries enabling developers to program the entire HPC platform, from the GPU foundation to the CPU and out through the interconnect. The 23.5 release of the HPC SDK includes new features as well as important functionality and performance improvements.‣Environment variables for controlling how the OpenACC runtime controls memory allocations are available. Please refer to the Using OpenACC section of the the HPC Compilers Users Guide for more details.‣HPC-X and OpenMPI 4 have been updated to work with CUDA 12 and CUDA 11.HPX-X 2.15 is included to work with CUDA 12 and HPC-X 3.14 works with CUDA11. New modulefiles, "nvhpc-hpcx-cuda12" and "nvhpc-hpcx-cuda11" are included.Updated OpenMPI 4.1.5 libraries are included with CUDA 12 and CUDA 12.‣The HPC Compilers now provide the -gpu=sm_XY option to include SASS following the behavior of nvcc's --gpu-architecture=sm_80.‣Using the -MD and -MMD options will now cause the HPC Compilers to output a .d file if the -o is specified.‣The CUDA compatibility files for CUDA 12 are now being shipped with the HPC SDK. Please refer to the CUDA Documentation for usage instructions.The NVIDIA HPC SDK 23.5 release contains the following versions of each component: T able 1 HPC SDK Release ComponentsRelease Component Versions3.1. Platform Requirements for the HPC SDK T able 2 HPC SDK Platform RequirementsSupported PlatformsPrograms generated by the HPC Compilers for x86_64 processors require a minimumof AVX instructions, which includes Sandy Bridge and newer CPUs from Intel, as well as Bulldozer and newer CPUs from AMD. POWER 8 and POWER 9 CPUs from the POWER architecture are supported. The HPC SDK includes support for v8.1+ Server Class Arm CPUs that meet the requirements appendix E specified in the SBSA 7.1 specification.The HPC Compilers are compatible with gcc and g++ and use the GCC C and C++ libraries; the minimum compatible versions of GCC are listed in Table 2. The minimum system requirements for CUDA and NVIDIA Math Library requirements are available in the NVIDIA CUDA Toolkit documentation.3.2. Supported CUDA T oolchain VersionsThe NVIDIA HPC SDK uses elements of the CUDA toolchain when building programs for execution with NVIDIA GPUs. Every HPC SDK installation package puts the required CUDA components into an installation directory called [install-prefix]/ [arch]/[nvhpc-version]/cuda.An NVIDIA CUDA GPU device driver must be installed on a system with a GPU before you can run a program compiled for the GPU on that system. The NVIDIA HPC SDK does not contain CUDA Drivers. You must download and install the appropriate CUDA Driver from NVIDIA , including the CUDA Compatibility Platform if that is required. The nvaccelinfo tool prints the CUDA Driver version in its output. You can use it to find out which version of the CUDA Driver is installed on your system.The NVIDIA HPC SDK 23.5 includes the following CUDA toolchain versions:‣CUDA 11.0‣CUDA 11.8‣CUDA 12.1The minimum required CUDA driver versions are listed in the table in Section 3.1.‣The -Mipa option has been disabled starting with the 23.3 version of the HPC Compilers.‣The latest version of cuSolverMp bundled with this release has two new dependencies on UCC and UCX libraries. To execute a program linked againstcuSolverMP, please use the “nvhpc-hpcx-cuda12” environment module for theHPC-X library, or set the environment variable LD_LIBRARY_PATH as follows:LD_LIBRARY_PATH=${NVHPCSDK_HOME}/comm_libs/hpcx/latest/ucc/lib:${NVHPCSDK_HOME}/comm_libs/11.8/hpcx/latest/ucx/lib:$LD_LIBRARY_PATH ‣If not using the provided modulefiles, prior to using HPC-X, users should take care to source the hpcx-init.sh script: $ . /[install-path]/Linux_x86_64/dev/comm_libs/ hpcx/hpcx-2.11/hpcx-init.sh Then, run the hpcx_load function defined by thisscript: $ hpcx_load These actions will set important environment variables thatare needed when running HPC-X. The following warning from HPC-X whilerunning an MPI job – “WARNING: Open MPI tried to bind a process but failed.This is a warning only; your job will continue, though performance may bedegraded” – is a known issue, and may be worked around as follows: exportOMPI_MCA_hwloc_base_binding_policy=""‣Fortran derived type objects with zero-size derived type allocatable components that are used in sourced allocation or allocatable assignment may result in a runtime segmentation violation.‣When using -stdpar to accelerate C++ parallel algorithms, the algorithm calls cannot include virtual function calls or function calls through a function pointer, cannot use C++ exceptions, can only dereference pointers that point to the heap, and must use random access iterators (raw pointers as iterators work best).‣Beginning with the HPC SDK 23.7, the deprecated CUDA_HOME environment variable will not affect the HPC Compilers.‣The -ta=tesla, -Mcuda, -Mcudalib options for the HPC Compilers have been deprecated.‣Support for the RHEL 7-based operating systems will be removed in the HPC SDK version 23.7, corresponding with the upstream end-of-life (EOL).‣In an upcoming release the HPC SDK will bundle only CUDA 11.8 and the latest version of the CUDA 12.x series. Codepaths in the HPC Compilers that supportCUDA versions older than 11.0 will no longer be tested or maintained.‣Support for the Ubuntu 18.04 operating system will be removed in the HPC SDK version 23.5, corresponding with the upstream end-of-life (EOL).‣Support for CUDA Fortran textures is deprecated in CUDA 11.0 and 11.8, and has been removed from CUDA 12.‣cudaDeviceSynchronize() in CUDA Fortran has been deprecated, and support has been removed from device code. It is still supported in host code.‣Starting with the 21.11 version of the NVIDIA HPC SDK, the HPC-X package is no longer shipped as part of the packages made available for the POWER architecture.‣Starting with the 21.5 version of the NVIDIA HPC SDK, the -cuda option for NVC+ + and NVFORTRAN no longer automatically links the NVIDIA GPU math libraries.Please refer to the -cudalib option.‣HPC Compiler support for the Kepler architecture of NVIDIA GPUs was deprecated starting with the 21.3 version of the NVIDIA HPC SDK.‣Support for the KNL architecture of multicore CPUs in the NVIDIA HPC SDK was removed in the HPC SDK version 21.3.NVIDIA HPC SDK Release Notes Version 23.5 | 7NoticeALL NVIDIA DESIGN SPECIFICATIONS, REFERENCE BOARDS, FILES, DRAWINGS, DIAGNOSTICS, LISTS, AND OTHER DOCUMENTS (TOGETHER AND SEPARATEL Y, "MATERIALS") ARE BEING PROVIDED "AS IS." NVIDIA MAKES NO WARRANTIES, EXPRESSED, IMPLIED, STATUTORY, OR OTHERWISE WITH RESPECT TO THE MATERIALS, AND EXPRESSL Y DISCLAIMS ALL IMPLIED WARRANTIES OF NONINFRINGEMENT, MERCHANTABILITY, AND FITNESS FOR A PARTICULAR PURPOSE.Information furnished is believed to be accurate and reliable. However, NVIDIA Corporation assumes no responsibility for the consequences of use of such information or for any infringement of patents or other rights of third parties that may result from its use. No license is granted by implication of otherwise under any patent rights of NVIDIA Corporation. Specifications mentioned in this publication are subject to change without notice. This publication supersedes and replaces all other information previously supplied. NVIDIA Corporation products are not authorized as critical components in life support devices or systems without express written approval of NVIDIA Corporation.TrademarksNVIDIA, the NVIDIA logo, CUDA, CUDA-X, GPUDirect, HPC SDK, NGC, NVIDIA Volta, NVIDIA DGX, NVIDIA Nsight, NVLink, NVSwitch, and T esla are trademarks and/ or registered trademarks of NVIDIA Corporation in the U.S. and other countries. Other company and product names may be trademarks of the respective companies with which they are associated.Copyright© 2013–2023 NVIDIA Corporation. All rights reserved.NVIDIA HPC SDK。
NVIDIA HPC Compilers Support Services Quick Start

DQ-10081-001-V001 | Novemberr 2020HPC Compiler Support Services Quick Start Guide provides minimal instructionsfor accessing NVIDIA® portals as well as downloading and installing the supported software. If you need complete instructions for installation and use of the software, please refer to the HPC SDK Installation Guide and HPC Compilers Documentation for your version of the HPC SDK software, or PGI Documentation for legacy PGI software. After your order for NVIDIA HPC Compiler Support Service is processed, youwill receive an order confirmation message from NVIDIA. This message contains information that you need for accessing NVIDIA Enterprise and Licensing Portalsand getting your NVIDIA software from the NVIDIA Licensing Portal. To log in to the NVIDIA Licensing Portal, you must have an NVIDIA Enterprise Account.1.1. Your Order Confirmation MessageAfter your order for NVIDIA HPC Compiler Support Services is processed, you will receive an order confirmation message to which your NVIDIA Entitlement Certificate is attached.Your NVIDIA Entitlement Certificate contains your order information.Your NVIDIA Entitlement Certificate also provides instructions for using the certificate.To get the support for your NVIDIA HPC Compiler Support Services , you must have an NVIDIA Enterprise Account.For a HPC Compiler Support Services renewal, you should already have an NVIDIAEnterprise AccountIf you do not have an account, follow the Register link in the instructions for using the certificate to create your account. For details, see the next section, Creating your NVIDIA Enterprise Account.If you already have an account, follow the Login link in the instructions for using the certificate to log in to the NVIDIA Enterprise Application Hub.1.2. Creating your NVIDIA Enterprise AccountIf you do not have an NVIDIA Enterprise Account, you must create an account to be able to log in to the NVIDIA Licensing Portal.If you already have an account, skip this task and go to Downloading Your NVIDIA HPCSDK or PGI Software.Before you begin, ensure that you have your order confirmation message.1.In the instructions for using your NVIDIA Entitlement Certificate, follow the Register link.2.Fill out the form on the NVIDIA Enterprise Account Registration page and click Register.A message confirming that an account has been created appears and an e-mail instructing you to set your NVIDIA password is sent to the e-mail address you provided.3.Open the e-mail instructing you to set your password and click SET PASSWORDAfter you have set your password during the initial registration process, you willbe able to log in to your account within 15 minutes. However, it may take up to 24business hours for your entitlement to appear in your account.For your account security, the SET PASSWORD link in this e-mail is set to expire in 24 hours.4.Enter and re-enter your new password, and click SUBMIT.A message confirming that your password has been set successfully appears.You will land on the Application Hub with access to both NVIDIA Licensing Portal and NVIDIA Enterprise Support Portal.2.1. Downloading Your NVIDIA HPC SDK or PGI SoftwareBefore you begin, ensure that you have your order confirmation message and have created an NVIDIA Enterprise Account.1.Visit the NVIDIA Enterprise Application Hub by following the Login link in the instructions for using your NVIDIA Entitlement Certificate or when prompted after setting the password for your NVIDIA Enterprise Account.2.When prompted, provide your e-mail address and password, and click LOGIN.3.On the NVIDIA APPLICATION HUB page that opens, click NVIDIA LICENSING PORTAL.The NVIDIA Licensing Portal dashboard page opens.Your entitlement might not appear on the NVIDIA Licensing Portal dashboard pageuntil 24 business hours after you set your password during the initial registrationprocess.4.In the left navigation pane of the NVIDIA Licensing Portal dashboard, click SOFTWARE DOWNLOADS.5.On the Product Download page that opens, follow the Download link for the release, platform, version and package type of NVIDIA software that you wish to use, for example, NVIDIA HPC SDK for Linux/x86-64 RPM version 20.11.If you don't see the release of NVIDIA HPC SDK or PGI software that you wish to use, click ALL A V AILABLE to see a list of all NVIDIA HPC SDK and PGI softwareavailable for download. The “Product” box can be used to select only HPC SDK (“HPC”) or PGI. Use the drop-down lists or the search box to further filter the software listed.For PGI software, the following archive versions are available:Linux x86-64: 10.2 to 20.4Linux OpenPOWER: 16.1 to 20.4Windows: 18.10 to 20.4 (command line only)The last PGI release was version 20.4. Product descriptions may not match those onthe legacy PGI website, but provided packages contain the most features available.Some older versions of PGI are no longer available to new customers and are notprovided here.6.When prompted to accept the license for the software that you are downloading, click AGREE & DOWNLOAD.7.When the browser asks what it should do with the file, select the option to save the file.8.For PGI software only, you will also need to download a License Key. This is not required for HPC SDK software.1.Navigate to the SOFTWARE DOWNLOADS page as described in step 4 above2.Search for “PGI License Key” and download the License File for your platform.This is a text file that contains instructions for use. Open with any text editor.3.Save this file for use after installing the PGI software as described in the nextsection.2.2. Installing Your NVIDIA HPC SDK or PGI Software1.HPC SDK Software1.Install per the instructions in the Installation Guide for your version available athttps:///hpc-sdk/.2.There are no License Files or License Servers to setup for the HPC SDK2.PGI Software1.Install per the instructions in the Installation Guide for your version available athttps:///hpc-sdk/pgi-compilers/, skipping any steps regardinginstallation of License Files or License Servers.2.After installation is complete, follow the instructions included within the LicenseFile from step 8 in section 2.1 above. This typically involves renaming the License File to “license.dat” for x86 platforms or “license.pgi” for OpenPOWER, andplacing it in the top level PGI installation directory, e.g., /opt/pgi, replacing any existing License File that may already exist.NoticeALL NVIDIA DESIGN SPECIFICATIONS, REFERENCE BOARDS, FILES, DRAWINGS, DIAGNOSTICS, LISTS, AND OTHER DOCUMENTS (TOGETHER AND SEPARATELY, "MATERIALS") ARE BEING PROVIDED "AS IS." NVIDIA MAKES NO WARRANTIES, EXPRESSED, IMPLIED, STATUTORY, OR OTHERWISE WITH RESPECT TO THE MATERIALS, AND EXPRESSLY DISCLAIMS ALL IMPLIED WARRANTIES OF NONINFRINGEMENT, MERCHANTABILITY, AND FITNESS FOR A PARTICULAR PURPOSE.Information furnished is believed to be accurate and reliable. However, NVIDIA Corporation assumes no responsibility for the consequences of use of such information or for any infringement of patents or other rights of third parties that may result from its use. No license is granted by implication of otherwise under any patent rights of NVIDIA Corporation. Specifications mentioned in this publication are subject to change without notice. This publication supersedes and replaces all other information previously supplied. NVIDIA Corporation products are not authorized as critical components in life support devices or systems without express written approval of NVIDIA Corporation.TrademarksNVIDIA, the NVIDIA logo, CUDA, CUDA-X, GPUDirect, HPC SDK, NGC, NVIDIA Volta, NVIDIA DGX, NVIDIA Nsight, NVLink, NVSwitch, and Tesla are trademarks and/or registered trademarks of NVIDIA Corporation in the U.S. and other countries. Other company and product names may be trademarks of the respective companies with which they are associated.Copyright© 2020 NVIDIA Corporation. All rights reserved.。
cuda纹理乘以旋转矩阵
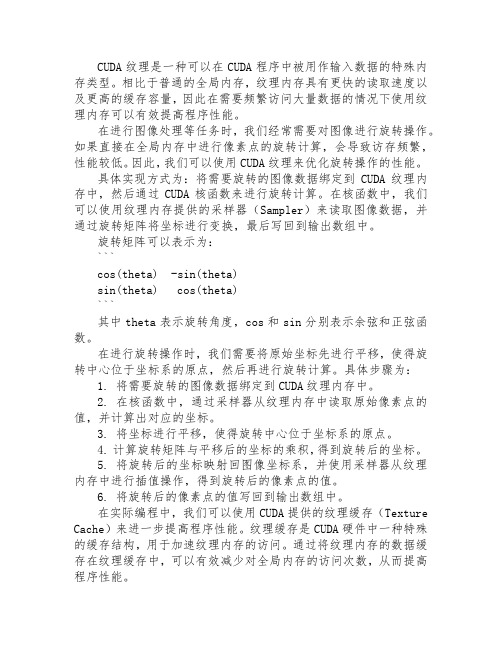
CUDA纹理是一种可以在CUDA程序中被用作输入数据的特殊内存类型。
相比于普通的全局内存,纹理内存具有更快的读取速度以及更高的缓存容量,因此在需要频繁访问大量数据的情况下使用纹理内存可以有效提高程序性能。
在进行图像处理等任务时,我们经常需要对图像进行旋转操作。
如果直接在全局内存中进行像素点的旋转计算,会导致访存频繁,性能较低。
因此,我们可以使用CUDA纹理来优化旋转操作的性能。
具体实现方式为:将需要旋转的图像数据绑定到CUDA纹理内存中,然后通过CUDA核函数来进行旋转计算。
在核函数中,我们可以使用纹理内存提供的采样器(Sampler)来读取图像数据,并通过旋转矩阵将坐标进行变换,最后写回到输出数组中。
旋转矩阵可以表示为:```cos(theta) -sin(theta)sin(theta) cos(theta)```其中theta表示旋转角度,cos和sin分别表示余弦和正弦函数。
在进行旋转操作时,我们需要将原始坐标先进行平移,使得旋转中心位于坐标系的原点,然后再进行旋转计算。
具体步骤为:1. 将需要旋转的图像数据绑定到CUDA纹理内存中。
2. 在核函数中,通过采样器从纹理内存中读取原始像素点的值,并计算出对应的坐标。
3. 将坐标进行平移,使得旋转中心位于坐标系的原点。
4. 计算旋转矩阵与平移后的坐标的乘积,得到旋转后的坐标。
5. 将旋转后的坐标映射回图像坐标系,并使用采样器从纹理内存中进行插值操作,得到旋转后的像素点的值。
6. 将旋转后的像素点的值写回到输出数组中。
在实际编程中,我们可以使用CUDA提供的纹理缓存(Texture Cache)来进一步提高程序性能。
纹理缓存是CUDA硬件中一种特殊的缓存结构,用于加速纹理内存的访问。
通过将纹理内存的数据缓存在纹理缓存中,可以有效减少对全局内存的访问次数,从而提高程序性能。
除了纹理内存和纹理缓存,还有一些其他的技术可以用于优化CUDA程序的性能,例如常量内存、共享内存等。
NVIDIA Quadro RTX 8000 和 RTX 6000 专用服务器版设计、制造、测试和授
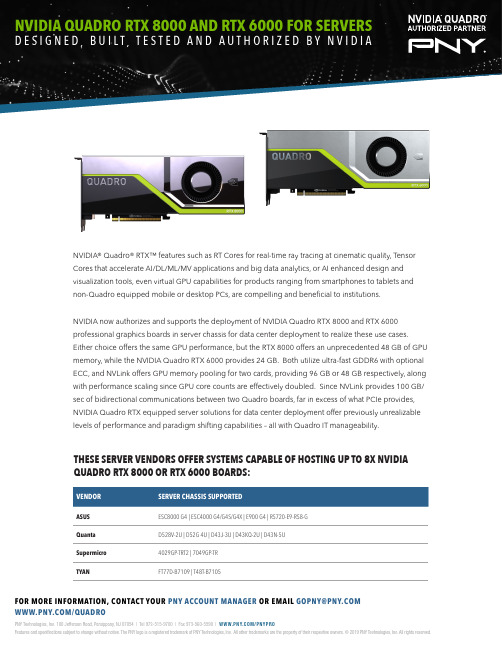
NVIDIA QUADRO RTX 8000 AND RTX 6000 FOR SERVERSD E S I G N E D,B U I L T,T E S T E D A N D A U T H O R I Z E D B Y N V I D I ANVIDIA® Quadro® RTX™ features such as RT Cores for real-time ray tracing at cinematic quality, TensorCores that accelerate AI/DL/ML/MV applications and big data analytics, or AI enhanced design andvisualization tools, even virtual GPU capabilities for products ranging from smartphones to tablets andnon-Quadro equipped mobile or desktop PCs, are compelling and beneficial to institutions.NVIDIA now authorizes and supports the deployment of NVIDIA Quadro RTX 8000 and RTX 6000professional graphics boards in server chassis for data center deployment to realize these use cases.Either choice offers the same GPU performance, but the RTX 8000 offers an unprecedented 48 GB of GPUmemory, while the NVIDIA Quadro RTX 6000 provides 24 GB. Both utilize ultra-fast GDDR6 with optionalECC, and NVLink offers GPU memory pooling for two cards, providing 96 GB or 48 GB respectively, alongwith performance scaling since GPU core counts are effectively doubled. Since NVLink provides 100 GB/sec of bidirectional communications between two Quadro boards, far in excess of what PCIe provides,NVIDIA Quadro RTX equipped server solutions for data center deployment offer previously unrealizablelevels of performance and paradigm shifting capabilities – all with Quadro IT manageability.VENDOR SERVER CHASSIS SUPPORTEDASUS ESC8000 G4 | ESC4000 G4/G4S/G4X | E900 G4 | RS720-E9-RS8-GQuanta D528V-2U | D52G 4U | D43J-3U | D43KQ-2U | D43N-5USupermicro4029GP-TRT2 | 7049GP-TRTYAN FT77D-B7109 | T48T-B7105THESE SERVER VENDORS OFFER SYSTEMS CAPABLE OF HOSTING UP TO 8X NVIDIAQUADRO RTX 8000 OR RTX 6000 BOARDS:FOR MORE INFORMATION, CONTACT YOUR PNY ACCOUNT MANAGER OR EMAILHERE ARE SOME ESSENTIAL NVIDIA QUADRO RTX 8000 AND RTX 6000SPECIFICATIONS WHEN USING ONE, TWO, FOUR, OR EIGHT GRAPHICS BOARDS:NVIDIA QUADRO RTX 8000 AND RTX 6000 POWERED SERVERS SUPPORT A WIDE ARRAY OF MARKETS AND SOLUTIONS:CUDA Cores 460892161843236864RT Cores 72144288576Tensor Cores 576115223044608RTX-OPS 84T168T336T672TRays Cast10 Giga Rays/Sec 20 Giga Rays/Sec 40 Giga Rays/Sec 80 Giga Rays/Sec Peak FP32 Performance 16.3 TFLOPS 32.6 TFLOPS 65.2 TFLOPS 130.4 TFLOPS Peak FP16 Performance 32.6 TFLOPS 65.2 TFLOPS 130.4 TFLOPS 260.8 TFLOPS Peak INT8 Performance 206.1 TOPS 412.2 TOPS 824.4 TOPS 1684.8 TOPS Deep Learning TFLOPS 130.5 Tensor TFLOPS 261.0 Tensor TFLOPS 522.0 Tensor TFLOPS 1044.0 Tensor TFLOPS Board Power Consumption 295 W 590 W 1180 W 2360 W RTX 8000 GPU Memory 48 GB 96 GB 192 GB 384 GB RTX 6000 GPU Memory 24 GB48 GB96 GB192 GBNVLink Bandwidth100 GB/sec Bidirectional | Between 2x GPUsWorkloadWorkstations for Design and VisualizationOffline Rendering, On-Demand Viewport Rendering, Workstations and Render Nodes Workstations for Data Science R&DWorkstations for HPC Compute and VisualizationDevelopment Platforms for AR/VR over 5GISV Software Hypervisor, ISV ApplicationsRenderer, ISV Applications, HypervisorData Science Software, HypervisorHPC Applications, Hypervisor AR/VR Applications,Development Tools, Hypervisor NVIDIA Software Quadro vDWS, CUDA-X AI, OptiXQuadro vDWS, CUDA-X AI, OptiXQuadro vDWS, CUDA-X AI, NGC ContainersQuadro vDWS, NGC ContainersQuadro vDWS, Development ToolsNVLink Bandwidth 100 GB/sec Bidirectional | Between 2x GPUs Server EnclosureASUS, Quanta, Supermicro and TYAN Qualified SystemsFOR MORE INFORMATION, CONTACT YOUR PNY ACCOUNT MANAGER OR EMAIL *************NVIDIA Quadro RTX 8000 and RTX 6000 fueled servers deliver exponential power at a fraction of the cost of CPU-based alternatives. For rendering the RTX solution is typically 1/4th the cost, for AI 1/5th the cost, and for HPC 1/7th the cost. To learn more about how NVIDIA Quadro RTX servers can enhance innova-tion, boost productivity, and realize significant operational efficiencies, please email *************.。
cuda 对gpu的要求 -回复
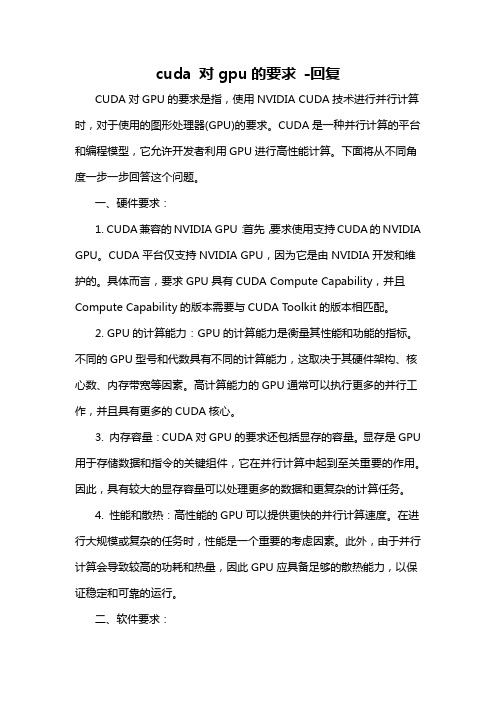
cuda 对gpu的要求-回复CUDA对GPU的要求是指,使用NVIDIA CUDA技术进行并行计算时,对于使用的图形处理器(GPU)的要求。
CUDA是一种并行计算的平台和编程模型,它允许开发者利用GPU进行高性能计算。
下面将从不同角度一步一步回答这个问题。
一、硬件要求:1. CUDA兼容的NVIDIA GPU:首先,要求使用支持CUDA的NVIDIA GPU。
CUDA平台仅支持NVIDIA GPU,因为它是由NVIDIA开发和维护的。
具体而言,要求GPU具有CUDA Compute Capability,并且Compute Capability的版本需要与CUDA Toolkit的版本相匹配。
2. GPU的计算能力:GPU的计算能力是衡量其性能和功能的指标。
不同的GPU型号和代数具有不同的计算能力,这取决于其硬件架构、核心数、内存带宽等因素。
高计算能力的GPU通常可以执行更多的并行工作,并且具有更多的CUDA核心。
3. 内存容量:CUDA对GPU的要求还包括显存的容量。
显存是GPU 用于存储数据和指令的关键组件,它在并行计算中起到至关重要的作用。
因此,具有较大的显存容量可以处理更多的数据和更复杂的计算任务。
4. 性能和散热:高性能的GPU可以提供更快的并行计算速度。
在进行大规模或复杂的任务时,性能是一个重要的考虑因素。
此外,由于并行计算会导致较高的功耗和热量,因此GPU应具备足够的散热能力,以保证稳定和可靠的运行。
二、软件要求:1. CUDA Toolkit:为了使用CUDA进行并行计算,需要安装NVIDIA 的CUDA Toolkit。
CUDA Toolkit是一个开发工具集,提供了编译器、调试器、性能分析器等工具,以及与GPU交互的API和库。
根据不同的CUDA 版本,可能需要与特定版本的GPU和驱动程序相匹配。
2. 驱动程序:为了让CUDA正常工作,还需要安装适用于GPU的最新驱动程序。
- 1、下载文档前请自行甄别文档内容的完整性,平台不提供额外的编辑、内容补充、找答案等附加服务。
- 2、"仅部分预览"的文档,不可在线预览部分如存在完整性等问题,可反馈申请退款(可完整预览的文档不适用该条件!)。
- 3、如文档侵犯您的权益,请联系客服反馈,我们会尽快为您处理(人工客服工作时间:9:00-18:30)。
新闻News 栏目编辑|齐健
qij@
NVIDIA宣布推出CUDA-X HPC
日前,NVIDIA宣布推出CUDA-X HPC,这是一个集合多种库、工具、编译器与API于一体的集大成者,可帮助开发者们解决全球最具挑战性的难题。
与在GTC Silicon Valley 2019大会上发布的CUDA-X AI类似,CUDA-X HPC 也是建立在NVIDIA CUDA并行计算平台和编程模型的顶层之上。
CUDA-X HPC搭载了HPC所必需的经过高度调优的内核。
针对线性代数、并行算法、信号与图像的GPU加速库,为计算物理学、化学、分子动力学和地震波勘测等领域内的计算密集型应用奠定了坚实基础。
持续扩充的CUDA-X HPC库列表会定期更新,并精心调优,以便充分利用诸如混合精度运算等新型算法。
列表内的线性代数库包含业内最佳的BLAS、Math和SOLVER库,为编程人员提供了广泛的功能性与灵活性。
此外,CUDA-X HPC还拥有一系列其它库,包括:适用于经优化的张量原语(cuTENSOR)、快速傅里叶变换(cuFFT)、处理图像与信号的性能基元(NPP)、并行算法与数据结构(Thrust)以及多GPU配置(NCCL)。
CUDA-X HPC还含有NVIDIA Nsight开发者工具,用于提供一流的GPU调试与剖析。
作为系统级低开销性能分析工具,Nsight Systems有助于开发者找出系统瓶颈,而Nsight Compute则是面向CUDA应用的交互式内核分析器。
其编译器支持C/C++、Python与FORTRAN等流行语言。
锐捷网络云办公4.0解决方案发布
2019年6月12日,锐捷网络在京举行媒体发布会,重磅推出云办公4.0解决方案。
云办公4.0解决方案以更高的性能配置和更强的操作系统兼容,提供了革命式的云桌面应用体验,是实现”替代商用PC”愿景的全新解决方案。
锐捷云办公致力于满足不同用户群体的业务需求,构建了融合VDI与IDV两大技术架构的全场景解决方案。
云办公4.0在硬件上做了更体贴的设计,如快拆、一体机单独的屏幕开关、调节键等;在交付方面,推出了基于正版操作系统的黄金镜像,让云桌面实施变得非常简单,甚至超越PC;在操作系统兼容性上,实现了在最新的CPU体系上运行Windows XP和Win7,兼容老旧业务系统。
此外,实际应用中,云桌面的运维方和使用方经常提出一些从技术角度相互矛盾的需求。
比较典型的是“用户个性化数据的保留“与”桌面软件的批量更新“。
这些需求很合理,但从实现的角度看,却难上加难。
因为批量维护和个性化的使用,就像是天平的两端,难以平衡。
锐捷使用Windows系统分层技术,将用户需求产品化。
云办公4.0全新的应用分发功能可以实现系统补丁、应用程序和系统配置的批量更新,并且不会丢失任何用户数据。
同时,该功能不会限制对于母镜像的编辑。
云办公4.0在VDI 与IDV两个技术架构的融合方案创新,从性能、设计、交付、兼容和运维等方面都实现了巨大突破,为用户提供了商用PC的替代之选。
欧特克在线商城正式上线
2019年6月10日,欧特克软件(中国)有限公司宣布,首家在线商城在中国正式上线。
继成功过渡到订阅模式后,欧特克又提供了全新的销售平台和便捷安全的交易方式,从而进一步拓宽了消费者的购买渠道。
目前,该在线商城已上架AutoCAD、Revit和Maya等十余款业界领先软件,用户可通过支付宝支付的形式以1年期和3年期的形式订阅软件,商城由欧特克的经销商——数位河商贸(上海)有限公司运营。
欧特克在全球拥有超过2亿用户,在线商城的开通将为更多的中国制造商、建筑师和工程师提供先进的工具和技术,让他们能够以不同以往的方式进行设计和制造。
欧特克软件(中国)有限公司董事长及大中华区总经理李邵建表示:“欧特克始终以客户为中心,通过坚持不懈地创新来简化客户流程。
转变为订阅模式是从软件拥有成本的角度出发,但我们并未就此止步。
从转变订阅模式以来,我们一直致力于加快并简化欧特克软件的购买流程,这也是我们与数位河商贸合作开设在线商城的原因。
同时,中国的企业和消费者引领着全球电子商务的趋势,我们相信,全新的线上渠道带来的便利性将得到用户的支持,今后,他们可以通过在线商城迅速获得自己需要的软件。
”
2019年6月中国制造业采购经理指数为49.4% 2019年6月30日,中国物流与采购联合会、国家统计局服务业调查中心发布,2019年6月中国制造业采购经理指数(PMI)为49.4%,与上月持平,其中供需分项指数小幅下降,但降幅较上月有所收窄。
2019年6月市场需求增势略有放缓,企业生产保持扩张,中小型企业生产经营活动增势回升,新动能发展态势较好,经济趋稳运行具备基础。
2019年第7期-05-。