外文翻译-不确定性数据挖掘:一种新的研究方向
人工智能领域中英文专有名词汇总
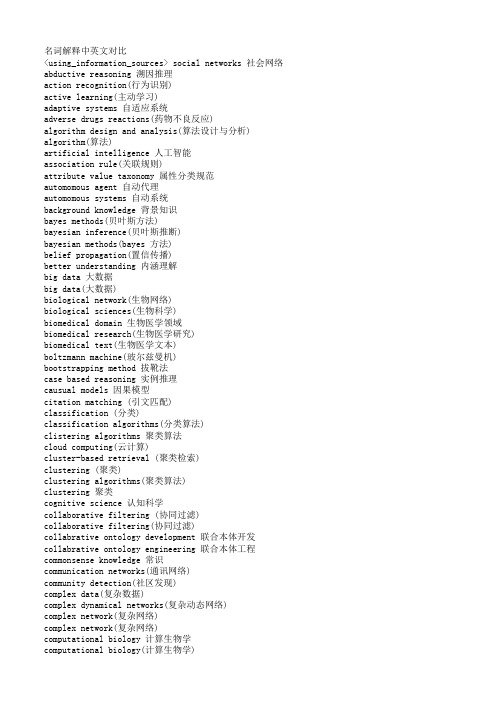
名词解释中英文对比<using_information_sources> social networks 社会网络abductive reasoning 溯因推理action recognition(行为识别)active learning(主动学习)adaptive systems 自适应系统adverse drugs reactions(药物不良反应)algorithm design and analysis(算法设计与分析) algorithm(算法)artificial intelligence 人工智能association rule(关联规则)attribute value taxonomy 属性分类规范automomous agent 自动代理automomous systems 自动系统background knowledge 背景知识bayes methods(贝叶斯方法)bayesian inference(贝叶斯推断)bayesian methods(bayes 方法)belief propagation(置信传播)better understanding 内涵理解big data 大数据big data(大数据)biological network(生物网络)biological sciences(生物科学)biomedical domain 生物医学领域biomedical research(生物医学研究)biomedical text(生物医学文本)boltzmann machine(玻尔兹曼机)bootstrapping method 拔靴法case based reasoning 实例推理causual models 因果模型citation matching (引文匹配)classification (分类)classification algorithms(分类算法)clistering algorithms 聚类算法cloud computing(云计算)cluster-based retrieval (聚类检索)clustering (聚类)clustering algorithms(聚类算法)clustering 聚类cognitive science 认知科学collaborative filtering (协同过滤)collaborative filtering(协同过滤)collabrative ontology development 联合本体开发collabrative ontology engineering 联合本体工程commonsense knowledge 常识communication networks(通讯网络)community detection(社区发现)complex data(复杂数据)complex dynamical networks(复杂动态网络)complex network(复杂网络)complex network(复杂网络)computational biology 计算生物学computational biology(计算生物学)computational complexity(计算复杂性) computational intelligence 智能计算computational modeling(计算模型)computer animation(计算机动画)computer networks(计算机网络)computer science 计算机科学concept clustering 概念聚类concept formation 概念形成concept learning 概念学习concept map 概念图concept model 概念模型concept modelling 概念模型conceptual model 概念模型conditional random field(条件随机场模型) conjunctive quries 合取查询constrained least squares (约束最小二乘) convex programming(凸规划)convolutional neural networks(卷积神经网络) customer relationship management(客户关系管理) data analysis(数据分析)data analysis(数据分析)data center(数据中心)data clustering (数据聚类)data compression(数据压缩)data envelopment analysis (数据包络分析)data fusion 数据融合data generation(数据生成)data handling(数据处理)data hierarchy (数据层次)data integration(数据整合)data integrity 数据完整性data intensive computing(数据密集型计算)data management 数据管理data management(数据管理)data management(数据管理)data miningdata mining 数据挖掘data model 数据模型data models(数据模型)data partitioning 数据划分data point(数据点)data privacy(数据隐私)data security(数据安全)data stream(数据流)data streams(数据流)data structure( 数据结构)data structure(数据结构)data visualisation(数据可视化)data visualization 数据可视化data visualization(数据可视化)data warehouse(数据仓库)data warehouses(数据仓库)data warehousing(数据仓库)database management systems(数据库管理系统)database management(数据库管理)date interlinking 日期互联date linking 日期链接Decision analysis(决策分析)decision maker 决策者decision making (决策)decision models 决策模型decision models 决策模型decision rule 决策规则decision support system 决策支持系统decision support systems (决策支持系统) decision tree(决策树)decission tree 决策树deep belief network(深度信念网络)deep learning(深度学习)defult reasoning 默认推理density estimation(密度估计)design methodology 设计方法论dimension reduction(降维) dimensionality reduction(降维)directed graph(有向图)disaster management 灾害管理disastrous event(灾难性事件)discovery(知识发现)dissimilarity (相异性)distributed databases 分布式数据库distributed databases(分布式数据库) distributed query 分布式查询document clustering (文档聚类)domain experts 领域专家domain knowledge 领域知识domain specific language 领域专用语言dynamic databases(动态数据库)dynamic logic 动态逻辑dynamic network(动态网络)dynamic system(动态系统)earth mover's distance(EMD 距离) education 教育efficient algorithm(有效算法)electric commerce 电子商务electronic health records(电子健康档案) entity disambiguation 实体消歧entity recognition 实体识别entity recognition(实体识别)entity resolution 实体解析event detection 事件检测event detection(事件检测)event extraction 事件抽取event identificaton 事件识别exhaustive indexing 完整索引expert system 专家系统expert systems(专家系统)explanation based learning 解释学习factor graph(因子图)feature extraction 特征提取feature extraction(特征提取)feature extraction(特征提取)feature selection (特征选择)feature selection 特征选择feature selection(特征选择)feature space 特征空间first order logic 一阶逻辑formal logic 形式逻辑formal meaning prepresentation 形式意义表示formal semantics 形式语义formal specification 形式描述frame based system 框为本的系统frequent itemsets(频繁项目集)frequent pattern(频繁模式)fuzzy clustering (模糊聚类)fuzzy clustering (模糊聚类)fuzzy clustering (模糊聚类)fuzzy data mining(模糊数据挖掘)fuzzy logic 模糊逻辑fuzzy set theory(模糊集合论)fuzzy set(模糊集)fuzzy sets 模糊集合fuzzy systems 模糊系统gaussian processes(高斯过程)gene expression data 基因表达数据gene expression(基因表达)generative model(生成模型)generative model(生成模型)genetic algorithm 遗传算法genome wide association study(全基因组关联分析) graph classification(图分类)graph classification(图分类)graph clustering(图聚类)graph data(图数据)graph data(图形数据)graph database 图数据库graph database(图数据库)graph mining(图挖掘)graph mining(图挖掘)graph partitioning 图划分graph query 图查询graph structure(图结构)graph theory(图论)graph theory(图论)graph theory(图论)graph theroy 图论graph visualization(图形可视化)graphical user interface 图形用户界面graphical user interfaces(图形用户界面)health care 卫生保健health care(卫生保健)heterogeneous data source 异构数据源heterogeneous data(异构数据)heterogeneous database 异构数据库heterogeneous information network(异构信息网络) heterogeneous network(异构网络)heterogenous ontology 异构本体heuristic rule 启发式规则hidden markov model(隐马尔可夫模型)hidden markov model(隐马尔可夫模型)hidden markov models(隐马尔可夫模型) hierarchical clustering (层次聚类) homogeneous network(同构网络)human centered computing 人机交互技术human computer interaction 人机交互human interaction 人机交互human robot interaction 人机交互image classification(图像分类)image clustering (图像聚类)image mining( 图像挖掘)image reconstruction(图像重建)image retrieval (图像检索)image segmentation(图像分割)inconsistent ontology 本体不一致incremental learning(增量学习)inductive learning (归纳学习)inference mechanisms 推理机制inference mechanisms(推理机制)inference rule 推理规则information cascades(信息追随)information diffusion(信息扩散)information extraction 信息提取information filtering(信息过滤)information filtering(信息过滤)information integration(信息集成)information network analysis(信息网络分析) information network mining(信息网络挖掘) information network(信息网络)information processing 信息处理information processing 信息处理information resource management (信息资源管理) information retrieval models(信息检索模型) information retrieval 信息检索information retrieval(信息检索)information retrieval(信息检索)information science 情报科学information sources 信息源information system( 信息系统)information system(信息系统)information technology(信息技术)information visualization(信息可视化)instance matching 实例匹配intelligent assistant 智能辅助intelligent systems 智能系统interaction network(交互网络)interactive visualization(交互式可视化)kernel function(核函数)kernel operator (核算子)keyword search(关键字检索)knowledege reuse 知识再利用knowledgeknowledgeknowledge acquisitionknowledge base 知识库knowledge based system 知识系统knowledge building 知识建构knowledge capture 知识获取knowledge construction 知识建构knowledge discovery(知识发现)knowledge extraction 知识提取knowledge fusion 知识融合knowledge integrationknowledge management systems 知识管理系统knowledge management 知识管理knowledge management(知识管理)knowledge model 知识模型knowledge reasoningknowledge representationknowledge representation(知识表达) knowledge sharing 知识共享knowledge storageknowledge technology 知识技术knowledge verification 知识验证language model(语言模型)language modeling approach(语言模型方法) large graph(大图)large graph(大图)learning(无监督学习)life science 生命科学linear programming(线性规划)link analysis (链接分析)link prediction(链接预测)link prediction(链接预测)link prediction(链接预测)linked data(关联数据)location based service(基于位置的服务) loclation based services(基于位置的服务) logic programming 逻辑编程logical implication 逻辑蕴涵logistic regression(logistic 回归)machine learning 机器学习machine translation(机器翻译)management system(管理系统)management( 知识管理)manifold learning(流形学习)markov chains 马尔可夫链markov processes(马尔可夫过程)matching function 匹配函数matrix decomposition(矩阵分解)matrix decomposition(矩阵分解)maximum likelihood estimation(最大似然估计)medical research(医学研究)mixture of gaussians(混合高斯模型)mobile computing(移动计算)multi agnet systems 多智能体系统multiagent systems 多智能体系统multimedia 多媒体natural language processing 自然语言处理natural language processing(自然语言处理) nearest neighbor (近邻)network analysis( 网络分析)network analysis(网络分析)network analysis(网络分析)network formation(组网)network structure(网络结构)network theory(网络理论)network topology(网络拓扑)network visualization(网络可视化)neural network(神经网络)neural networks (神经网络)neural networks(神经网络)nonlinear dynamics(非线性动力学)nonmonotonic reasoning 非单调推理nonnegative matrix factorization (非负矩阵分解) nonnegative matrix factorization(非负矩阵分解) object detection(目标检测)object oriented 面向对象object recognition(目标识别)object recognition(目标识别)online community(网络社区)online social network(在线社交网络)online social networks(在线社交网络)ontology alignment 本体映射ontology development 本体开发ontology engineering 本体工程ontology evolution 本体演化ontology extraction 本体抽取ontology interoperablity 互用性本体ontology language 本体语言ontology mapping 本体映射ontology matching 本体匹配ontology versioning 本体版本ontology 本体论open government data 政府公开数据opinion analysis(舆情分析)opinion mining(意见挖掘)opinion mining(意见挖掘)outlier detection(孤立点检测)parallel processing(并行处理)patient care(病人医疗护理)pattern classification(模式分类)pattern matching(模式匹配)pattern mining(模式挖掘)pattern recognition 模式识别pattern recognition(模式识别)pattern recognition(模式识别)personal data(个人数据)prediction algorithms(预测算法)predictive model 预测模型predictive models(预测模型)privacy preservation(隐私保护)probabilistic logic(概率逻辑)probabilistic logic(概率逻辑)probabilistic model(概率模型)probabilistic model(概率模型)probability distribution(概率分布)probability distribution(概率分布)project management(项目管理)pruning technique(修剪技术)quality management 质量管理query expansion(查询扩展)query language 查询语言query language(查询语言)query processing(查询处理)query rewrite 查询重写question answering system 问答系统random forest(随机森林)random graph(随机图)random processes(随机过程)random walk(随机游走)range query(范围查询)RDF database 资源描述框架数据库RDF query 资源描述框架查询RDF repository 资源描述框架存储库RDF storge 资源描述框架存储real time(实时)recommender system(推荐系统)recommender system(推荐系统)recommender systems 推荐系统recommender systems(推荐系统)record linkage 记录链接recurrent neural network(递归神经网络) regression(回归)reinforcement learning 强化学习reinforcement learning(强化学习)relation extraction 关系抽取relational database 关系数据库relational learning 关系学习relevance feedback (相关反馈)resource description framework 资源描述框架restricted boltzmann machines(受限玻尔兹曼机) retrieval models(检索模型)rough set theroy 粗糙集理论rough set 粗糙集rule based system 基于规则系统rule based 基于规则rule induction (规则归纳)rule learning (规则学习)rule learning 规则学习schema mapping 模式映射schema matching 模式匹配scientific domain 科学域search problems(搜索问题)semantic (web) technology 语义技术semantic analysis 语义分析semantic annotation 语义标注semantic computing 语义计算semantic integration 语义集成semantic interpretation 语义解释semantic model 语义模型semantic network 语义网络semantic relatedness 语义相关性semantic relation learning 语义关系学习semantic search 语义检索semantic similarity 语义相似度semantic similarity(语义相似度)semantic web rule language 语义网规则语言semantic web 语义网semantic web(语义网)semantic workflow 语义工作流semi supervised learning(半监督学习)sensor data(传感器数据)sensor networks(传感器网络)sentiment analysis(情感分析)sentiment analysis(情感分析)sequential pattern(序列模式)service oriented architecture 面向服务的体系结构shortest path(最短路径)similar kernel function(相似核函数)similarity measure(相似性度量)similarity relationship (相似关系)similarity search(相似搜索)similarity(相似性)situation aware 情境感知social behavior(社交行为)social influence(社会影响)social interaction(社交互动)social interaction(社交互动)social learning(社会学习)social life networks(社交生活网络)social machine 社交机器social media(社交媒体)social media(社交媒体)social media(社交媒体)social network analysis 社会网络分析social network analysis(社交网络分析)social network(社交网络)social network(社交网络)social science(社会科学)social tagging system(社交标签系统)social tagging(社交标签)social web(社交网页)sparse coding(稀疏编码)sparse matrices(稀疏矩阵)sparse representation(稀疏表示)spatial database(空间数据库)spatial reasoning 空间推理statistical analysis(统计分析)statistical model 统计模型string matching(串匹配)structural risk minimization (结构风险最小化) structured data 结构化数据subgraph matching 子图匹配subspace clustering(子空间聚类)supervised learning( 有support vector machine 支持向量机support vector machines(支持向量机)system dynamics(系统动力学)tag recommendation(标签推荐)taxonmy induction 感应规范temporal logic 时态逻辑temporal reasoning 时序推理text analysis(文本分析)text anaylsis 文本分析text classification (文本分类)text data(文本数据)text mining technique(文本挖掘技术)text mining 文本挖掘text mining(文本挖掘)text summarization(文本摘要)thesaurus alignment 同义对齐time frequency analysis(时频分析)time series analysis( 时time series data(时间序列数据)time series data(时间序列数据)time series(时间序列)topic model(主题模型)topic modeling(主题模型)transfer learning 迁移学习triple store 三元组存储uncertainty reasoning 不精确推理undirected graph(无向图)unified modeling language 统一建模语言unsupervisedupper bound(上界)user behavior(用户行为)user generated content(用户生成内容)utility mining(效用挖掘)visual analytics(可视化分析)visual content(视觉内容)visual representation(视觉表征)visualisation(可视化)visualization technique(可视化技术) visualization tool(可视化工具)web 2.0(网络2.0)web forum(web 论坛)web mining(网络挖掘)web of data 数据网web ontology lanuage 网络本体语言web pages(web 页面)web resource 网络资源web science 万维科学web search (网络检索)web usage mining(web 使用挖掘)wireless networks 无线网络world knowledge 世界知识world wide web 万维网world wide web(万维网)xml database 可扩展标志语言数据库附录 2 Data Mining 知识图谱(共包含二级节点15 个,三级节点93 个)间序列分析)监督学习)领域 二级分类 三级分类。
工业工程 外文期刊 翻译
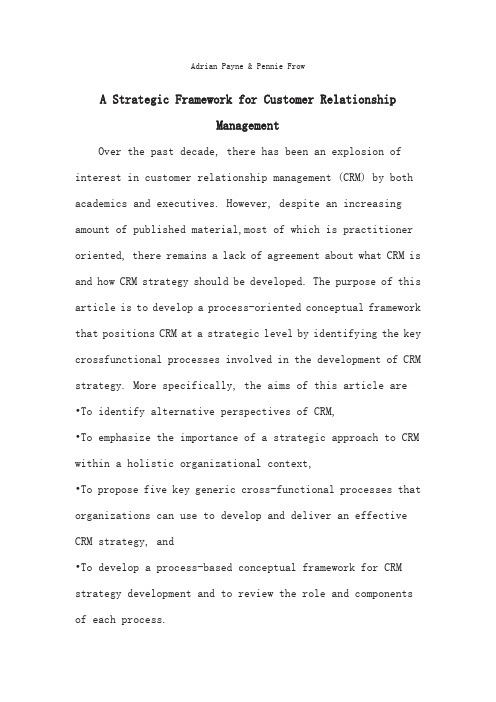
Adrian Payne & Pennie FrowA Strategic Framework for Customer RelationshipManagementOver the past decade, there has been an explosion of interest in customer relationship management (CRM) by both academics and executives. However, despite an increasing amount of published material,most of which is practitioner oriented, there remains a lack of agreement about what CRM is and how CRM strategy should be developed. The purpose of this article is to develop a process-oriented conceptual framework that positions CRM at a strategic level by identifying the key crossfunctional processes involved in the development of CRM strategy. More specifically, the aims of this article are •To identify alternative perspectives of CRM,•To emphasize the importance of a strategic approach to CRM within a holistic organizational context,•To propose five key generic cross-functional processes that organizations can use to develop and deliver an effective CRM strategy, and•To develop a process-based conceptual framework for CRM strategy development and to review the role and components of each process.We organize this article in three main parts. First, we explore the role of CRM and identify three alternative perspectives of CRM. Second, we consider the need for a cross -functional process-based approach to CRM. We develop criteria for process selection and identify five key CRM processes. Third, we propose a strategic conceptual framework that is constructed of these five processes and examine the components of each process.The development of this framework is a response to a challenge by Reinartz, Krafft, and Hoyer (2004), who criticize the severe lack of CRM research that takes a broader, more strategic focus. The article does not explore people issues related to CRM implementation. Customer relationship management can fail when a limited number of employees are committed to the initiative; thus, employee engagement and change management are essential issues in CRM implementation. In our discussion, we emphasize such implementation and people issues as a priority area for further research.CRM Perspectives and DefinitionThe term “customer relationship management” emerged in the information technology (IT) vendor community and practitioner community in the mid-1990s. It is often used todescribe technology-based customer solutions, such as sales force automation (SFA). In the academic community, the terms “relationship marketing and CRM are often used interchangeably (Parvatiyar and Sheth 2001). However,CRM is more commonly used in the context of technology solutions and has been described as “information-enabled relationship marketing” (Ryals and Payne 2001, p. 3).Zablah, Beuenger, and Johnston (2003, p. 116) suggest that CRM is “a philosophically-related offspring to relationship marketing which is for the most part neglected in the literature,”and they conclude that “further exploration of CRM and its related phenomena is not only warranted but also desperately needed.”A significant problem that many organizations deciding to adopt CRM face stems from the great deal of confusion about what constitutes CRM. In interviews with executives, which formed part of our research process (we describe this process subsequently), we found a wide range of views about what CRM means. To some, it meant direct mail, a loyalty card scheme, or a database, whereas others envisioned it as a help desk or a call center. Some said that it was about populating a data warehouse or undertaking data mining; others considered CRM an e-commerce solution,such as the use of a personalization engine on the Internet or a relational database for SFA. This lack of a widely accepted and appropriate definition of CRM can contribute to the failure of a CRM project when an organization views CRM from a limited technology perspective or undertakes CRM on a fragmented basis. The definitions and descriptions of CRM that different authors and authorities use vary considerably, signifying a variety of CRM viewpoints. To identify alternative perspectives of CRM, we considered definitions and descriptions of CRM from a range of sources, which we summarize in the Appendix. We excluded other, similar definitions from this List.Process Identification and the CRM FrameworkWe began by identifying possible generic CRM processes from the CRM and related business literature. We then discussed these tentative processes interactively with the groups of executives. The outcome of this work was a short list of seven processes. We then used the expert panel of experienced CRM executives who had assisted in the development of the process selection schema to nominate the CRM processes that they considered important and to agree on those that were the most relevant and generic. After an initial group workshop, eachpanel member independently completed a list representing his or her view of the key generic processes that met the six previously agreed-on process criteria. The data were fed back to this group, and a detailed discussion followed to help confirm our understanding of the process categories.As a result of this interactive method, five CRM processes that met the selection criteria were identified; all five were agreed on as important generic processes by more than two-thirds of the group in the first iteration. Subsequently, we received strong confirmation of these as key generic CRM processes by several of the other groups of managers. The resultant five generic processes were (1) the strategy development process, (2) the value creation process, (3) the multichannel integration process, (4) the information management process, and (5) the performance assessment process.We then incorporated these five key generic CRM processes into a preliminary conceptual framework. This initial framework and the development of subsequent versions were both informed by and further refined by our interactions with two primary executive groups.客户关系的管理框架在过去的十年里,管理层和学术界对客户关系管理(CRM)的兴趣激增。
外文翻译-不确定性数据挖掘:一种新的研究方向
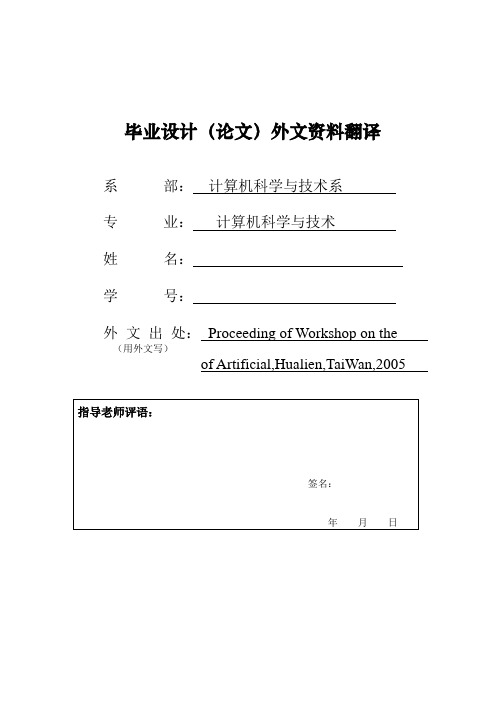
毕业设计(论文)外文资料翻译系部:计算机科学与技术系专业:计算机科学与技术姓名:学号:外文出处:Proceeding of Workshop on the (用外文写)of Artificial,Hualien,TaiWan,2005不确定性数据挖掘:一种新的研究方向Michael Chau1, Reynold Cheng2, and Ben Kao31:商学院,香港大学,薄扶林,香港2:计算机系,香港理工大学九龙湖校区,香港3:计算机科学系,香港大学,薄扶林,香港摘要由于不精确测量、过时的来源或抽样误差等原因,数据不确定性常常出现在真实世界应用中。
目前,在数据库数据不确定性处理领域中,很多研究结果已经被发表。
我们认为,当不确定性数据被执行数据挖掘时,数据不确定性不得不被考虑在内,才能获得高质量的数据挖掘结果。
我们称之为“不确定性数据挖掘”问题。
在本文中,我们为这个领域可能的研究方向提出一个框架。
同时,我们以UK-means 聚类算法为例来阐明传统K-means算法怎么被改进来处理数据挖掘中的数据不确定性。
1.引言由于测量不精确、抽样误差、过时数据来源或其他等原因,数据往往带有不确定性性质。
特别在需要与物理环境交互的应用中,如:移动定位服务[15]和传感器监测[3]。
例如:在追踪移动目标(如车辆或人)的情境中,数据库是不可能完全追踪到所有目标在所有瞬间的准确位置。
因此,每个目标的位置的变化过程是伴有不确定性的。
为了提供准确地查询和挖掘结果,这些导致数据不确定性的多方面来源不得不被考虑。
在最近几年里,已有在数据库中不确定性数据管理方面的大量研究,如:数据库中不确定性的表现和不确定性数据查询。
然而,很少有研究成果能够解决不确定性数据挖掘的问题。
我们注意到,不确定性使数据值不再具有原子性。
对于使用传统数据挖掘技术,不确定性数据不得不被归纳为原子性数值。
再以追踪移动目标应用为例,一个目标的位置可以通过它最后的记录位置或通过一个预期位置(如果这个目标位置概率分布被考虑到)归纳得到。
聚类分析文献英文翻译

电气信息工程学院外文翻译英文名称:Data mining-clustering译文名称:数据挖掘—聚类分析专业:自动化姓名:****班级学号:****指导教师:******译文出处:Data mining:Ian H.Witten, EibeFrank 著二○一○年四月二十六日Clustering5.1 INTRODUCTIONClustering is similar to classification in that data are grouped. However, unlike classification, the groups are not predefined. Instead, the grouping is accomplished by finding similarities between data according to characteristics found in the actual data. The groups are called clusters. Some authors view clustering as a special type of classification. In this text, however, we follow a more conventional view in that the two are different. Many definitions for clusters have been proposed:●Set of like elements. Elements from different clusters are not alike.●The distance between points in a cluster is less than the distance betweena point in the cluster and any point outside it.A term similar to clustering is database segmentation, where like tuple (record) in a database are grouped together. This is done to partition or segment the database into components that then give the user a more general view of the data. In this case text, we do not differentiate between segmentation and clustering. A simple example of clustering is found in Example 5.1. This example illustrates the fact that that determining how to do the clustering is not straightforward.As illustrated in Figure 5.1, a given set of data may be clustered on different attributes. Here a group of homes in a geographic area is shown. The first floor type of clustering is based on the location of the home. Homes that are geographically close to each other are clustered together. In the second clustering, homes are grouped based on the size of the house.Clustering has been used in many application domains, including biology, medicine, anthropology, marketing, and economics. Clustering applications include plant and animal classification, disease classification, image processing, pattern recognition, and document retrieval. One of the first domains in which clustering was used was biological taxonomy. Recent uses include examining Web log data to detect usage patterns.When clustering is applied to a real-world database, many interesting problems occur:●Outlier handling is difficult. Here the elements do not naturally fallinto any cluster. They can be viewed as solitary clusters. However, if aclustering algorithm attempts to find larger clusters, these outliers will beforced to be placed in some cluster. This process may result in the creationof poor clusters by combining two existing clusters and leaving the outlier in its own cluster.● Dynamic data in the database implies that cluster membership may change over time.● Interpreting the semantic meaning of each cluster may be difficult. With classification, the labeling of the classes is known ahead of time. However, with clustering, this may not be the case. Thus, when the clustering process finishes creating a set of clusters, the exact meaning of each cluster may not be obvious. Here is where a domain expert is needed to assign a label or interpretation for each cluster.● There is no one correct answer to a clustering problem. In fact, many answers may be found. The exact number of clusters required is not easy to determine. Again, a domain expert may be required. For example, suppose we have a set of data about plants that have been collected during a field trip. Without any prior knowledge of plant classification, if we attempt to divide this set of data into similar groupings, it would not be clear how many groups should be created.● Another related issue is what data should be used of clustering. Unlike learning during a classification process, where there is some a priori knowledge concerning what the attributes of each classification should be, in clustering we have no supervised learning to aid the process. Indeed, clustering can be viewed as similar to unsupervised learning.We can then summarize some basic features of clustering (as opposed to classification):● The (best) number of clusters is not known.● There may not be any a priori knowledge concerning the clusters.● Cluster results are dynamic.The clustering problem is stated as shown in Definition 5.1. Here we assume that the number of clusters to be created is an input value, k. The actual content (and interpretation) of each cluster,j k ,1j k ≤≤, is determined as a result of the function definition. Without loss of generality, we will view that the result of solving a clustering problem is that a set of clusters is created: K={12,,...,k k k k }.D EFINITION 5.1.Given a database D ={12,,...,n t t t } of tuples and an integer value k , the clustering problem is to define a mapping f : {1,...,}D k → where each i t is assigned to one cluster j K ,1j k ≤≤. A cluster j K , contains precisely those tuples mapped to it; that is, j K ={|(),1,i i j t f t K i n =≤≤and i t D ∈}.A classification of the different types of clustering algorithms is shown in Figure 5.2. Clustering algorithms themselves may be viewed as hierarchical or partitional. With hierarchical clustering, a nested set of clusters is created. Each level in the hierarchy has a separate set of clusters. At the lowest level, each item is in its own unique cluster. At the highest level, all items belong to the same cluster. With hierarchical clustering, the desired number of clusters is not input. With partitional clustering, the algorithm creates only one set of clusters. These approaches use the desired number of clusters to drive how the final set is created. Traditional clustering algorithms tend to be targeted to small numeric database that fit into memory .There are, however, more recent clustering algorithms that look at categorical data and are targeted to larger, perhaps dynamic, databases. Algorithms targeted to larger databases may adapt to memory constraints by either sampling the database or using data structures, which can be compressed or pruned to fit into memory regardless of the size of the database. Clustering algorithms may also differ based on whether they produce overlapping or nonoverlapping clusters. Even though we consider only nonoverlapping clusters, it is possible to place an item in multiple clusters. In turn, nonoverlapping clusters can be viewed as extrinsic or intrinsic. Extrinsic techniques use labeling of the items to assist in the classification process. These algorithms are the traditional classification supervised learning algorithms in which a special input training set is used. Intrinsic algorithms do not use any a priori category labels, but depend only on the adjacency matrix containing the distance between objects. All algorithms we examine in this chapter fall into the intrinsic class.The types of clustering algorithms can be furthered classified based on the implementation technique used. Hierarchical algorithms can becategorized as agglomerative or divisive. ”Agglomerative ” implies that the clusters are created in a bottom-up fashion, while divisive algorithms work in a top-down fashion. Although both hierarchical and partitional algorithms could be described using the agglomerative vs. divisive label, it typically is more associated with hierarchical algorithms. Another descriptive tag indicates whether each individual element is handled one by one, serial (sometimes called incremental), or whether all items are examined together, simultaneous. If a specific tuple is viewed as having attribute values for all attributes in the schema, then clustering algorithms could differ as to how the attribute values are examined. As is usually done with decision tree classification techniques, some algorithms examine attribute values one at a time, monothetic. Polythetic algorithms consider all attribute values at one time. Finally, clustering algorithms can be labeled base on the mathematical formulation given to the algorithm: graph theoretic or matrix algebra. In this chapter we generally use the graph approach and describe the input to the clustering algorithm as an adjacency matrix labeled with distance measure.We discuss many clustering algorithms in the following sections. This is only a representative subset of the many algorithms that have been proposed in the literature. Before looking at these algorithms, we first examine possible similarity measures and examine the impact of outliers.5.2 SIMILARITY AND DISTANCE MEASURESThere are many desirable properties for the clusters created by a solution to a specific clustering problem. The most important one is that a tuple within one cluster is more like tuples within that cluster than it is similar to tuples outside it. As with classification, then, we assume the definition of a similarity measure, sim(,i l t t ), defined between any two tuples, ,i l t t D . This provides a more strict and alternative clustering definition, as found in Definition 5.2. Unless otherwise stated, we use the first definition rather than the second. Keep in mind that the similarity relationship stated within the second definition is a desirable, although not always obtainable, property.A distance measure, dis(,i j t t ), as opposed to similarity, is often used inclustering. The clustering problem then has the desirable property that given a cluster,j K ,,jl jm j t t K ∀∈ and ,(,)(,)i j jl jm jl i t K sim t t dis t t ∉≤.Some clustering algorithms look only at numeric data, usually assuming metric data points. Metric attributes satisfy the triangular inequality. The cluster can then be described by using several characteristic values. Given a cluster, m K of N points { 12,,...,m m mN t t t }, we make the following definitions [ZRL96]:Here the centroid is the “middle ” of the cluster; it need not be an actual point in the cluster. Some clustering algorithms alternatively assume that the cluster is represented by one centrally located object in the cluster called a medoid . The radius is the square root of the average mean squared distance from any point in the cluster to the centroid, and of points in the cluster. We use the notation m M to indicate the medoid for cluster m K .Many clustering algorithms require that the distance between clusters (rather than elements) be determined. This is not an easy task given that there are many interpretations for distance between clusters. Given clusters i K and j K , there are several standard alternatives to calculate the distance between clusters. A representative list is:● Single link : Smallest distance between an element in onecluster and an element in the other. We thus havedis(,i j K K )=min((,))il jm il i j dis t t t K K ∀∈∉and jm j i t K K ∀∈∉.● Complete link : Largest distance between an element in onecluster and an element in the other. We thus havedis(,i j K K )=max((,))il jm il i j dis t t t K K ∀∈∉and jm j i t K K ∀∈∉.● Average : Average distance between an element in onecluster and an element in the other. We thus havedis(,i j K K )=((,))il jm il i j mean dis t t t K K ∀∈∉and jm j i t K K ∀∈∉.● Centroid : If cluster have a representative centroid, then thecentroid distance is defined as the distance between the centroids.We thus have dis(,i j K K )=dis(,i j C C ), where i C is the centroidfor i K and similarly for j C .Medoid : Using a medoid to represent each cluster, thedistance between the clusters can be defined by the distancebetween the medoids: dis(,i j K K )=(,)i j dis M M5.3 OUTLIERSAs mentioned earlier, outliers are sample points with values much different from those of the remaining set of data. Outliers may represent errors in the data (perhaps a malfunctioning sensor recorded an incorrect data value) or could be correct data values that are simply much different from the remaining data. A person who is 2.5 meters tall is much taller than most people. In analyzing the height of individuals, this value probably would be viewed as an outlier.Some clustering techniques do not perform well with the presence of outliers. This problem is illustrated in Figure 5.3. Here if three clusters are found (solid line), the outlier will occur in a cluster by itself. However, if two clusters are found (dashed line), the two (obviously) different sets of data will be placed in one cluster because they are closer together than the outlier. This problem is complicated by the fact that many clustering algorithms actually have as input the number of desired clusters to be found.Clustering algorithms may actually find and remove outliers to ensure that they perform better. However, care must be taken in actually removing outliers. For example, suppose that the data mining problem is to predict flooding. Extremely high water level values occur very infrequently, and when compared with the normal water level values may seem to be outliers. However, removing these values may not allow the data mining algorithms to work effectively because there would be no data that showed that floods ever actually occurred.Outlier detection, or outlier mining, is the process of identifying outliers in a set of data. Clustering, or other data mining, algorithms may then choose to remove or treat these values differently. Some outlier detection techniques are based on statistical techniques. These usually assume that the set of data follows a known distribution and that outliers can be detected by well-known tests such as discordancy tests. However, thesetests are not very realistic for real-world data because real-world data values may not follow well-defined data distributions. Also, most of these tests assume single attribute value, and many attributes are involved in real-world datasets. Alternative detection techniques may be based on distance measures.聚类分析5.1简介聚类分析与分类数据分组类似。
数据挖掘英语
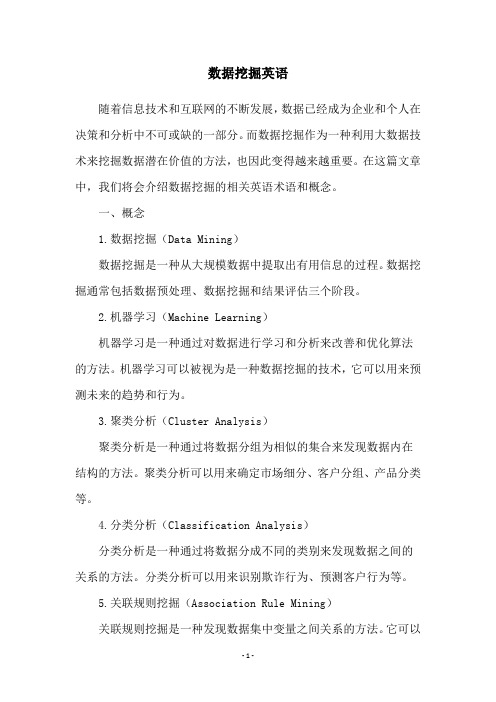
数据挖掘英语随着信息技术和互联网的不断发展,数据已经成为企业和个人在决策和分析中不可或缺的一部分。
而数据挖掘作为一种利用大数据技术来挖掘数据潜在价值的方法,也因此变得越来越重要。
在这篇文章中,我们将会介绍数据挖掘的相关英语术语和概念。
一、概念1.数据挖掘(Data Mining)数据挖掘是一种从大规模数据中提取出有用信息的过程。
数据挖掘通常包括数据预处理、数据挖掘和结果评估三个阶段。
2.机器学习(Machine Learning)机器学习是一种通过对数据进行学习和分析来改善和优化算法的方法。
机器学习可以被视为是一种数据挖掘的技术,它可以用来预测未来的趋势和行为。
3.聚类分析(Cluster Analysis)聚类分析是一种通过将数据分组为相似的集合来发现数据内在结构的方法。
聚类分析可以用来确定市场细分、客户分组、产品分类等。
4.分类分析(Classification Analysis)分类分析是一种通过将数据分成不同的类别来发现数据之间的关系的方法。
分类分析可以用来识别欺诈行为、预测客户行为等。
5.关联规则挖掘(Association Rule Mining)关联规则挖掘是一种发现数据集中变量之间关系的方法。
它可以用来发现购物篮分析、交叉销售等。
6.异常检测(Anomaly Detection)异常检测是一种通过识别不符合正常模式的数据点来发现异常的方法。
异常检测可以用来识别欺诈行为、检测设备故障等。
二、术语1.数据集(Dataset)数据集是一组数据的集合,通常用来进行数据挖掘和分析。
2.特征(Feature)特征是指在数据挖掘和机器学习中用来描述数据的属性或变量。
3.样本(Sample)样本是指从数据集中选取的一部分数据,通常用来进行机器学习和预测。
4.训练集(Training Set)训练集是指用来训练机器学习模型的样本集合。
5.测试集(Test Set)测试集是指用来测试机器学习模型的样本集合。
翻译专业毕业论文研究方向探索
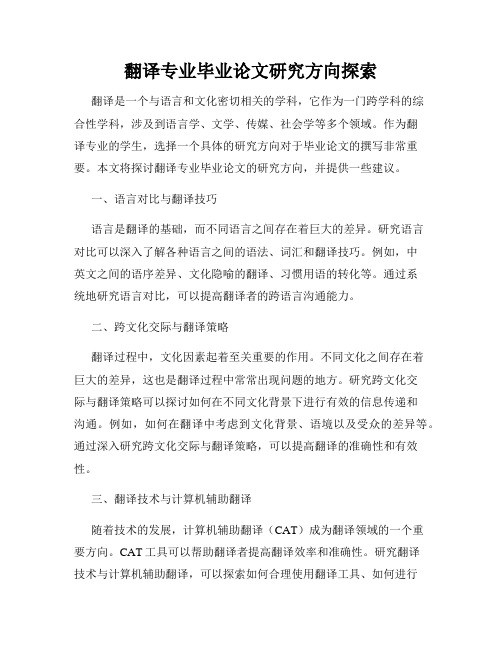
翻译专业毕业论文研究方向探索翻译是一个与语言和文化密切相关的学科,它作为一门跨学科的综合性学科,涉及到语言学、文学、传媒、社会学等多个领域。
作为翻译专业的学生,选择一个具体的研究方向对于毕业论文的撰写非常重要。
本文将探讨翻译专业毕业论文的研究方向,并提供一些建议。
一、语言对比与翻译技巧语言是翻译的基础,而不同语言之间存在着巨大的差异。
研究语言对比可以深入了解各种语言之间的语法、词汇和翻译技巧。
例如,中英文之间的语序差异、文化隐喻的翻译、习惯用语的转化等。
通过系统地研究语言对比,可以提高翻译者的跨语言沟通能力。
二、跨文化交际与翻译策略翻译过程中,文化因素起着至关重要的作用。
不同文化之间存在着巨大的差异,这也是翻译过程中常常出现问题的地方。
研究跨文化交际与翻译策略可以探讨如何在不同文化背景下进行有效的信息传递和沟通。
例如,如何在翻译中考虑到文化背景、语境以及受众的差异等。
通过深入研究跨文化交际与翻译策略,可以提高翻译的准确性和有效性。
三、翻译技术与计算机辅助翻译随着技术的发展,计算机辅助翻译(CAT)成为翻译领域的一个重要方向。
CAT工具可以帮助翻译者提高翻译效率和准确性。
研究翻译技术与计算机辅助翻译,可以探索如何合理使用翻译工具、如何进行术语管理以及如何利用机器翻译等技术提高翻译效果。
此外,还可以研究自然语言处理技术在翻译过程中的应用。
四、专业文本翻译与行业应用翻译工作广泛应用于各个行业,而不同领域的专业文本存在着各自的特点和难点。
研究专业文本翻译与行业应用可以探讨如何在特定领域内进行准确且流畅的翻译。
例如,法律文件、医学文献、商务合同等。
通过研究专业文本翻译与行业应用,可以提高翻译者在特定领域内的工作能力和竞争力。
五、翻译教育与专业能力培养翻译教育是培养翻译专业人才的关键环节。
研究翻译教育与专业能力培养可以探讨如何有效地进行翻译教学和实践,并培养学生的综合素质与专业技能。
例如,探讨翻译教学方法、实习机制以及评估体系等。
市场调研方法外文文献及翻译
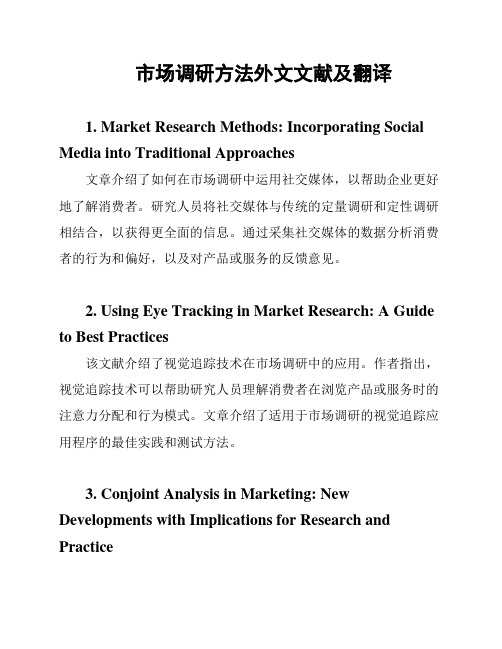
市场调研方法外文文献及翻译1. Market Research Methods: Incorporating Social Media into Traditional Approaches文章介绍了如何在市场调研中运用社交媒体,以帮助企业更好地了解消费者。
研究人员将社交媒体与传统的定量调研和定性调研相结合,以获得更全面的信息。
通过采集社交媒体的数据分析消费者的行为和偏好,以及对产品或服务的反馈意见。
2. Using Eye Tracking in Market Research: A Guide to Best Practices该文献介绍了视觉追踪技术在市场调研中的应用。
作者指出,视觉追踪技术可以帮助研究人员理解消费者在浏览产品或服务时的注意力分配和行为模式。
文章介绍了适用于市场调研的视觉追踪应用程序的最佳实践和测试方法。
3. Conjoint Analysis in Marketing: New Developments with Implications for Research and Practice这篇文章介绍了一种被称为 "共轭分析" 的调研方法,该方法可以帮助研究人员了解消费者在购买某种产品或服务时的偏好和决策过程。
文献称,共轭分析已经成为市场营销领域最为普遍的工具之一。
文章还介绍了最新的研究和在实践中的应用,并探讨了一些特定情况下共轭分析的限制。
4. Qualitative Market Research: An International Journal这个杂志专注于定性市场调研方法。
它包括与确定消费者需求、分析竞争对手、建立品牌等相关的研究。
文章强调定性市场调研可以提供深入的见解和对产品或服务的更清晰的理解,帮助企业做出更明智的营销和业务决策。
每一期都包括来自该领域的专家的文章,并提供案例研究和最佳实践。
5. Use of Artificial Intelligence Techniques in Market Research: A Review该文献介绍了如何使用人工智能技术进行市场调研。
大数据挖掘外文翻译文献
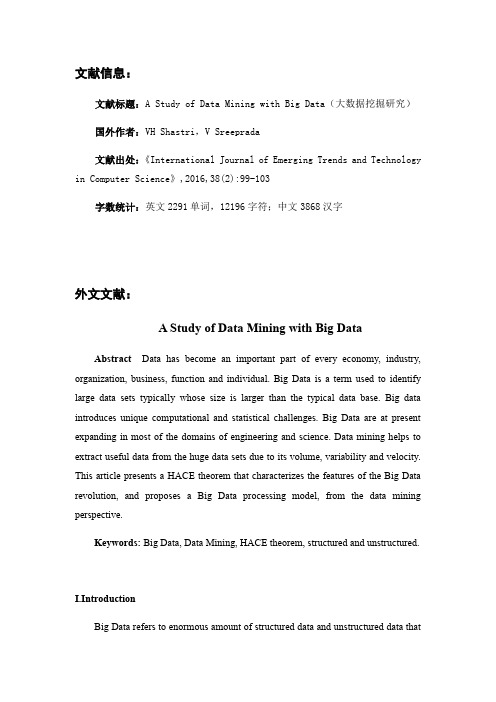
文献信息:文献标题:A Study of Data Mining with Big Data(大数据挖掘研究)国外作者:VH Shastri,V Sreeprada文献出处:《International Journal of Emerging Trends and Technology in Computer Science》,2016,38(2):99-103字数统计:英文2291单词,12196字符;中文3868汉字外文文献:A Study of Data Mining with Big DataAbstract Data has become an important part of every economy, industry, organization, business, function and individual. Big Data is a term used to identify large data sets typically whose size is larger than the typical data base. Big data introduces unique computational and statistical challenges. Big Data are at present expanding in most of the domains of engineering and science. Data mining helps to extract useful data from the huge data sets due to its volume, variability and velocity. This article presents a HACE theorem that characterizes the features of the Big Data revolution, and proposes a Big Data processing model, from the data mining perspective.Keywords: Big Data, Data Mining, HACE theorem, structured and unstructured.I.IntroductionBig Data refers to enormous amount of structured data and unstructured data thatoverflow the organization. If this data is properly used, it can lead to meaningful information. Big data includes a large number of data which requires a lot of processing in real time. It provides a room to discover new values, to understand in-depth knowledge from hidden values and provide a space to manage the data effectively. A database is an organized collection of logically related data which can be easily managed, updated and accessed. Data mining is a process discovering interesting knowledge such as associations, patterns, changes, anomalies and significant structures from large amount of data stored in the databases or other repositories.Big Data includes 3 V’s as its characteristics. They are volume, velocity and variety. V olume means the amount of data generated every second. The data is in state of rest. It is also known for its scale characteristics. Velocity is the speed with which the data is generated. It should have high speed data. The data generated from social media is an example. Variety means different types of data can be taken such as audio, video or documents. It can be numerals, images, time series, arrays etc.Data Mining analyses the data from different perspectives and summarizing it into useful information that can be used for business solutions and predicting the future trends. Data mining (DM), also called Knowledge Discovery in Databases (KDD) or Knowledge Discovery and Data Mining, is the process of searching large volumes of data automatically for patterns such as association rules. It applies many computational techniques from statistics, information retrieval, machine learning and pattern recognition. Data mining extract only required patterns from the database in a short time span. Based on the type of patterns to be mined, data mining tasks can be classified into summarization, classification, clustering, association and trends analysis.Big Data is expanding in all domains including science and engineering fields including physical, biological and biomedical sciences.II.BIG DATA with DATA MININGGenerally big data refers to a collection of large volumes of data and these data are generated from various sources like internet, social-media, business organization, sensors etc. We can extract some useful information with the help of Data Mining. It is a technique for discovering patterns as well as descriptive, understandable, models from a large scale of data.V olume is the size of the data which is larger than petabytes and terabytes. The scale and rise of size makes it difficult to store and analyse using traditional tools. Big Data should be used to mine large amounts of data within the predefined period of time. Traditional database systems were designed to address small amounts of data which were structured and consistent, whereas Big Data includes wide variety of data such as geospatial data, audio, video, unstructured text and so on.Big Data mining refers to the activity of going through big data sets to look for relevant information. To process large volumes of data from different sources quickly, Hadoop is used. Hadoop is a free, Java-based programming framework that supports the processing of large data sets in a distributed computing environment. Its distributed supports fast data transfer rates among nodes and allows the system to continue operating uninterrupted at times of node failure. It runs Map Reduce for distributed data processing and is works with structured and unstructured data.III.BIG DATA characteristics- HACE THEOREM.We have large volume of heterogeneous data. There exists a complex relationship among the data. We need to discover useful information from this voluminous data.Let us imagine a scenario in which the blind people are asked to draw elephant. The information collected by each blind people may think the trunk as wall, leg as tree, body as wall and tail as rope. The blind men can exchange information with each other.Figure1: Blind men and the giant elephantSome of the characteristics that include are:i.Vast data with heterogeneous and diverse sources: One of the fundamental characteristics of big data is the large volume of data represented by heterogeneous and diverse dimensions. For example in the biomedical world, a single human being is represented as name, age, gender, family history etc., For X-ray and CT scan images and videos are used. Heterogeneity refers to the different types of representations of same individual and diverse refers to the variety of features to represent single information.ii.Autonomous with distributed and de-centralized control: the sources are autonomous, i.e., automatically generated; it generates information without any centralized control. We can compare it with World Wide Web (WWW) where each server provides a certain amount of information without depending on other servers.plex and evolving relationships: As the size of the data becomes infinitely large, the relationship that exists is also large. In early stages, when data is small, there is no complexity in relationships among the data. Data generated from social media and other sources have complex relationships.IV.TOOLS:OPEN SOURCE REVOLUTIONLarge companies such as Facebook, Yahoo, Twitter, LinkedIn benefit and contribute work on open source projects. In Big Data Mining, there are many open source initiatives. The most popular of them are:Apache Mahout:Scalable machine learning and data mining open source software based mainly in Hadoop. It has implementations of a wide range of machine learning and data mining algorithms: clustering, classification, collaborative filtering and frequent patternmining.R: open source programming language and software environment designed for statistical computing and visualization. R was designed by Ross Ihaka and Robert Gentleman at the University of Auckland, New Zealand beginning in 1993 and is used for statistical analysis of very large data sets.MOA: Stream data mining open source software to perform data mining in real time. It has implementations of classification, regression; clustering and frequent item set mining and frequent graph mining. It started as a project of the Machine Learning group of University of Waikato, New Zealand, famous for the WEKA software. The streams framework provides an environment for defining and running stream processes using simple XML based definitions and is able to use MOA, Android and Storm.SAMOA: It is a new upcoming software project for distributed stream mining that will combine S4 and Storm with MOA.Vow pal Wabbit: open source project started at Yahoo! Research and continuing at Microsoft Research to design a fast, scalable, useful learning algorithm. VW is able to learn from terafeature datasets. It can exceed the throughput of any single machine networkinterface when doing linear learning, via parallel learning.V.DATA MINING for BIG DATAData mining is the process by which data is analysed coming from different sources discovers useful information. Data Mining contains several algorithms which fall into 4 categories. They are:1.Association Rule2.Clustering3.Classification4.RegressionAssociation is used to search relationship between variables. It is applied in searching for frequently visited items. In short it establishes relationship among objects. Clustering discovers groups and structures in the data.Classification deals with associating an unknown structure to a known structure. Regression finds a function to model the data.The different data mining algorithms are:Table 1. Classification of AlgorithmsData Mining algorithms can be converted into big map reduce algorithm based on parallel computing basis.Table 2. Differences between Data Mining and Big DataVI.Challenges in BIG DATAMeeting the challenges with BIG Data is difficult. The volume is increasing every day. The velocity is increasing by the internet connected devices. The variety is also expanding and the organizations’ capability to capture and process the data is limited.The following are the challenges in area of Big Data when it is handled:1.Data capture and storage2.Data transmission3.Data curation4.Data analysis5.Data visualizationAccording to, challenges of big data mining are divided into 3 tiers.The first tier is the setup of data mining algorithms. The second tier includesrmation sharing and Data Privacy.2.Domain and Application Knowledge.The third one includes local learning and model fusion for multiple information sources.3.Mining from sparse, uncertain and incomplete data.4.Mining complex and dynamic data.Figure 2: Phases of Big Data ChallengesGenerally mining of data from different data sources is tedious as size of data is larger. Big data is stored at different places and collecting those data will be a tedious task and applying basic data mining algorithms will be an obstacle for it. Next we need to consider the privacy of data. The third case is mining algorithms. When we are applying data mining algorithms to these subsets of data the result may not be that much accurate.VII.Forecast of the futureThere are some challenges that researchers and practitioners will have to deal during the next years:Analytics Architecture:It is not clear yet how an optimal architecture of analytics systems should be to deal with historic data and with real-time data at the same time. An interesting proposal is the Lambda architecture of Nathan Marz. The Lambda Architecture solves the problem of computing arbitrary functions on arbitrary data in real time by decomposing the problem into three layers: the batch layer, theserving layer, and the speed layer. It combines in the same system Hadoop for the batch layer, and Storm for the speed layer. The properties of the system are: robust and fault tolerant, scalable, general, and extensible, allows ad hoc queries, minimal maintenance, and debuggable.Statistical significance: It is important to achieve significant statistical results, and not be fooled by randomness. As Efron explains in his book about Large Scale Inference, it is easy to go wrong with huge data sets and thousands of questions to answer at once.Distributed mining: Many data mining techniques are not trivial to paralyze. To have distributed versions of some methods, a lot of research is needed with practical and theoretical analysis to provide new methods.Time evolving data: Data may be evolving over time, so it is important that the Big Data mining techniques should be able to adapt and in some cases to detect change first. For example, the data stream mining field has very powerful techniques for this task.Compression: Dealing with Big Data, the quantity of space needed to store it is very relevant. There are two main approaches: compression where we don’t loose anything, or sampling where we choose what is thedata that is more representative. Using compression, we may take more time and less space, so we can consider it as a transformation from time to space. Using sampling, we are loosing information, but the gains inspace may be in orders of magnitude. For example Feldman et al use core sets to reduce the complexity of Big Data problems. Core sets are small sets that provably approximate the original data for a given problem. Using merge- reduce the small sets can then be used for solving hard machine learning problems in parallel.Visualization: A main task of Big Data analysis is how to visualize the results. As the data is so big, it is very difficult to find user-friendly visualizations. New techniques, and frameworks to tell and show stories will be needed, as for examplethe photographs, infographics and essays in the beautiful book ”The Human Face of Big Data”.Hidden Big Data: Large quantities of useful data are getting lost since new data is largely untagged and unstructured data. The 2012 IDC studyon Big Data explains that in 2012, 23% (643 exabytes) of the digital universe would be useful for Big Data if tagged and analyzed. However, currently only 3% of the potentially useful data is tagged, and even less is analyzed.VIII.CONCLUSIONThe amounts of data is growing exponentially due to social networking sites, search and retrieval engines, media sharing sites, stock trading sites, news sources and so on. Big Data is becoming the new area for scientific data research and for business applications.Data mining techniques can be applied on big data to acquire some useful information from large datasets. They can be used together to acquire some useful picture from the data.Big Data analysis tools like Map Reduce over Hadoop and HDFS helps organization.中文译文:大数据挖掘研究摘要数据已经成为各个经济、行业、组织、企业、职能和个人的重要组成部分。
外文翻译----什么是数据挖掘

什么是数据挖掘?简单地说,数据挖掘是从大量的数据中提取或“挖掘”知识。
该术语实际上有点儿用词不当。
注意,从矿石或砂子中挖掘黄金叫做黄金挖掘,而不是叫做矿石挖掘。
这样,数据挖掘应当更准确地命名为“从数据中挖掘知识”,不幸的是这个有点儿长。
“知识挖掘”是一个短术语,可能它不能反映出从大量数据中挖掘的意思。
毕竟,挖掘是一个很生动的术语,它抓住了从大量的、未加工的材料中发现少量金块这一过程的特点。
这样,这种用词不当携带了“数据”和“挖掘”,就成了流行的选择。
还有一些术语,具有和数据挖掘类似但稍有不同的含义,如数据库中的知识挖掘、知识提取、数据/模式分析、数据考古和数据捕捞。
许多人把数据挖掘视为另一个常用的术语—数据库中的知识发现或KDD的同义词。
而另一些人只是把数据挖掘视为数据库中知识发现过程的一个基本步骤。
知识发现的过程由以下步骤组成:1)数据清理:消除噪声或不一致数据,2)数据集成:多种数据可以组合在一起,3)数据选择:从数据库中检索与分析任务相关的数据,4)数据变换:数据变换或统一成适合挖掘的形式,如通过汇总或聚集操作,5)数据挖掘:基本步骤,使用智能方法提取数据模式,6)模式评估:根据某种兴趣度度量,识别表示知识的真正有趣的模式,7)知识表示:使用可视化和知识表示技术,向用户提供挖掘的知识。
数据挖掘的步骤可以与用户或知识库进行交互。
把有趣的模式提供给用户,或作为新的知识存放在知识库中。
注意,根据这种观点,数据挖掘只是整个过程中的一个步骤,尽管是最重要的一步,因为它发现隐藏的模式。
我们同意数据挖掘是知识发现过程中的一个步骤。
然而,在产业界、媒体和数据库研究界,“数据挖掘”比那个较长的术语“数据库中知识发现”更为流行。
因此,在本书中,选用的术语是数据挖掘。
我们采用数据挖掘的广义观点:数据挖掘是从存放在数据库中或其他信息库中的大量数据中挖掘出有趣知识的过程。
基于这种观点,典型的数据挖掘系统具有以下主要成分:数据库、数据仓库或其他信息库:这是一个或一组数据库、数据仓库、电子表格或其他类型的信息库。
近二十年来国际翻译学研究的核心、热点及前沿-应用语言学论文-语言学论文
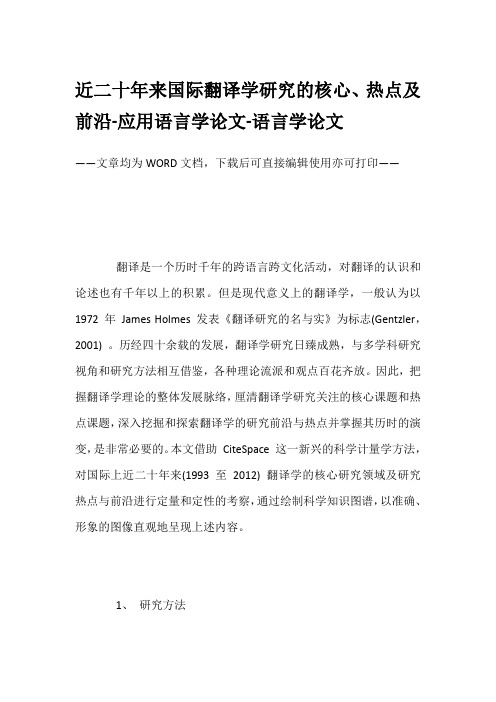
近二十年来国际翻译学研究的核心、热点及前沿-应用语言学论文-语言学论文——文章均为WORD文档,下载后可直接编辑使用亦可打印——翻译是一个历时千年的跨语言跨文化活动,对翻译的认识和论述也有千年以上的积累。
但是现代意义上的翻译学,一般认为以1972 年James Holmes 发表《翻译研究的名与实》为标志(Gentzler,2001) 。
历经四十余载的发展,翻译学研究日臻成熟,与多学科研究视角和研究方法相互借鉴,各种理论流派和观点百花齐放。
因此,把握翻译学理论的整体发展脉络,厘清翻译学研究关注的核心课题和热点课题,深入挖掘和探索翻译学的研究前沿与热点并掌握其历时的演变,是非常必要的。
本文借助CiteSpace 这一新兴的科学计量学方法,对国际上近二十年来(1993 至2012) 翻译学的核心研究领域及研究热点与前沿进行定量和定性的考察,通过绘制科学知识图谱,以准确、形象的图像直观地呈现上述内容。
1、研究方法近年来,伴随着信息社会的来临和知识的式增长,科学知识图谱(绘制) (mapping knowledge domains) 这一新兴的交叉研究领域(Shiffrin &B rner,2004: 5183) 异军突起,帮助我们在海量信息中有效获取知识、发现知识和探测知识前沿。
本研究借助科学知识图谱绘制的利器美国德雷赛尔大学(Drexel University) 陈超美博士开发的CiteSpace 软件系统对数据进行分析。
2、数据来源为保证数据的质量,本研究将数据来源定位于翻译学研究中刊载大量专业论文且利用率较高的少数权威国际期刊。
权威期刊的选定主要依据欧洲翻译研究协会(European Society forTranslation Studies) 、国际翻译和跨文化研究协会(InternationalAssociation for Translation and Intercultural Studies,简称IATIS) 和纽约大学图书馆的相关推荐。
电子商务与旅游中英文对照外文翻译文献
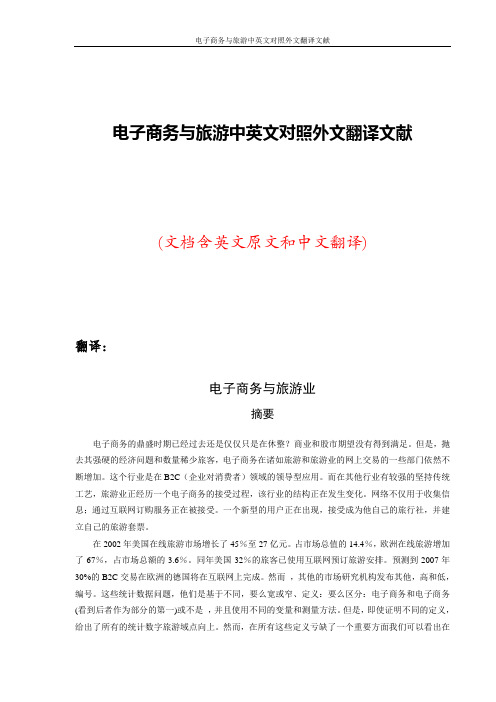
电子商务与旅游中英文对照外文翻译文献(文档含英文原文和中文翻译)翻译:电子商务与旅游业摘要电子商务的鼎盛时期已经过去还是仅仅只是在休整?商业和股市期望没有得到满足。
但是,抛去其强硬的经济问题和数量稀少旅客,电子商务在诸如旅游和旅游业的网上交易的一些部门依然不断增加。
这个行业是在B2C(企业对消费者)领域的领导型应用。
而在其他行业有较强的坚持传统工艺,旅游业正经历一个电子商务的接受过程,该行业的结构正在发生变化。
网络不仅用于收集信息;通过互联网订购服务正在被接受。
一个新型的用户正在出现,接受成为他自己的旅行社,并建立自己的旅游套票。
在2002年美国在线旅游市场增长了45%至27亿元。
占市场总值的14.4%,欧洲在线旅游增加了67%,占市场总额的3.6%。
同年美国32%的旅客已使用互联网预订旅游安排。
预测到2007年30%的B2C交易在欧洲的德国将在互联网上完成。
然而,其他的市场研究机构发布其他,高和低,编号。
这些统计数据问题,他们是基于不同,要么宽或窄、定义:要么区分:电子商务和电子商务(看到后者作为部分的第一)或不是,并且使用不同的变量和测量方法。
但是,即使证明不同的定义,给出了所有的统计数字旅游域点向上。
然而,在所有这些定义亏缺了一个重要方面我们可以看出在旅游案例:他们是所有交易和商业导向和忽略了这个事实,即网络也是一个中等的好奇心、创建社区或刚一件有趣的事,所有这一切都可能发生,也可能不会获得业务。
特别是旅游产品与情感体验,有趣但并不仅仅是业务。
一、关于这个行业旅游及旅游业作为一个全球或者说全球化的行业体现了非常具体的特点:1.旅行和观光的代表了大约11%世界范围内(GDP)旅游卫星账户后举行的世界旅游的方法和旅游委员会);2.将有十亿国外游客在2010年世界旅游组织,平均而言,旅游增长速度超过其他的经济部门;3. 作为一个伞业它涉及到许多部门,如文化或体育运动,超过30种不同的工业部件,服务已确认的旅行者;4.解释了整个行业的非均质性,因为它的中小企业结构(尤其是拍照的时候目的地的观点),它有一个巨大的重要性了区域发展。
数据挖掘技术的应用与研究
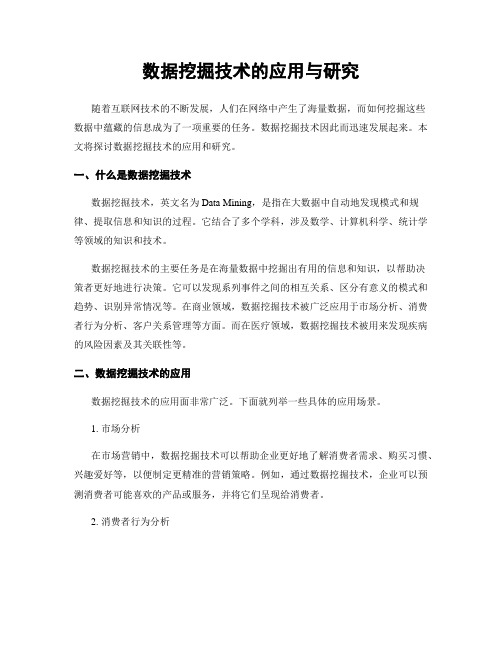
数据挖掘技术的应用与研究随着互联网技术的不断发展,人们在网络中产生了海量数据,而如何挖掘这些数据中蕴藏的信息成为了一项重要的任务。
数据挖掘技术因此而迅速发展起来。
本文将探讨数据挖掘技术的应用和研究。
一、什么是数据挖掘技术数据挖掘技术,英文名为Data Mining,是指在大数据中自动地发现模式和规律、提取信息和知识的过程。
它结合了多个学科,涉及数学、计算机科学、统计学等领域的知识和技术。
数据挖掘技术的主要任务是在海量数据中挖掘出有用的信息和知识,以帮助决策者更好地进行决策。
它可以发现系列事件之间的相互关系、区分有意义的模式和趋势、识别异常情况等。
在商业领域,数据挖掘技术被广泛应用于市场分析、消费者行为分析、客户关系管理等方面。
而在医疗领域,数据挖掘技术被用来发现疾病的风险因素及其关联性等。
二、数据挖掘技术的应用数据挖掘技术的应用面非常广泛。
下面就列举一些具体的应用场景。
1. 市场分析在市场营销中,数据挖掘技术可以帮助企业更好地了解消费者需求、购买习惯、兴趣爱好等,以便制定更精准的营销策略。
例如,通过数据挖掘技术,企业可以预测消费者可能喜欢的产品或服务,并将它们呈现给消费者。
2. 消费者行为分析数据挖掘技术可以帮助企业更好地了解消费者的行为模式,并提高客户忠诚度。
例如,在电子商务领域中,利用数据挖掘技术,可以通过分析用户的购物历史和浏览记录,为用户推荐更感兴趣的商品。
3. 客户关系管理通过分析客户数据和行为,数据挖掘技术可以建立更精准的客户画像,以实现更好的客户关系管理。
例如,在银行领域中,银行可以通过数据挖掘技术分析客户的信用记录、银行卡消费记录等数据,为客户提供更加个性化的服务。
4. 欺诈检测利用数据挖掘技术,企业可以快速发现欺诈性行为,并采取相应的措施。
例如,在信用卡领域中,企业可以通过数据挖掘技术分析用户的消费记录、交易类型等信息,及时发现问题交易并对其进行识别和拦截。
三、数据挖掘技术的研究在数据挖掘技术的研究方面,主要有以下几个方向。
abms的名词解释

abms的名词解释ABMS(Agent-Based Modelling and Simulation)是一种基于智能体的建模和仿真方法。
它是一种模拟社会或自然系统中个体行为和交互的技术。
ABMS的成功应用可以追溯到二十世纪七八十年代的计算机科学和人工智能领域,随着计算能力的提高和软件工具的发展,ABMS在近年来得到了广泛应用和研究。
在传统的建模和仿真方法中,通常通过数学方程式来表示和描述系统的行为和动态。
然而,这种方法往往忽略了系统中个体之间的相互作用和反馈机制,从而限制了对复杂系统的理解和预测能力。
ABMS正是为了解决这一问题而产生的一种新型建模和仿真方法。
ABMS的核心思想是将系统看作由个体智能体组成的集合,每个智能体都具有自己的特征、状态和行为规则。
这些智能体可以通过感知环境、与其他智能体进行交互以及根据预定的规则进行决策来模拟真实世界。
ABMS能够模拟多种复杂系统,如城市交通、社会网络、生态系统、金融市场等。
通过对智能体的建模,ABMS可以更好地理解系统中个体的行为模式、相互作用和决策过程,从而推断整个系统的行为和演变。
ABMS的应用领域非常广泛。
在城市规划中,ABMS可以用于模拟交通流量,优化交通信号控制,减少交通拥堵;在社会科学中,ABMS可以用于研究社会网络、群体行为和意见传播等问题;在生物学和生态学领域,ABMS可以用于模拟生物进化、种群动态和生态系统的演变。
与传统建模方法相比,ABMS具有以下几个优点:1. 能够模拟复杂系统的多样性和异质性。
由于ABMS关注个体智能体的行为规则和决策过程,它可以更好地模拟和理解现实世界中的多样性和异质性。
2. 能够模拟系统的动态演变和反馈机制。
ABMS通过模拟个体之间的相互作用和决策过程,可以捕捉系统演变的动态性以及反馈机制的作用。
3. 能够进行实验和预测。
ABMS可以对系统进行实验和敏感性分析,通过调整智能体的行为规则和参数,并观察系统的响应来推测系统的未来行为。
翻译学的最新研究成果和应用前景

翻译学的最新研究成果和应用前景翻译学是语言学的重要分支,研究翻译的原理、规律和方法,探讨翻译在跨文化交流中的作用和应用。
近年来,随着全球化的发展,翻译学研究日益深入,不断涌现出最新的研究成果和应用前景。
一、翻译学研究的最新成果1. 机器翻译技术的发展随着人工智能技术的快速发展,机器翻译技术变得越来越成熟,已经可以应用于各类场景中。
最新的机器翻译技术,采用了深度学习、神经网络等方法,大幅提高了翻译质量和效率。
2. 翻译质量评估的研究翻译质量评估是翻译学中非常重要的一环,可以帮助翻译者和机器翻译系统提高翻译质量和准确度。
最新的研究成果包括:基于人类判断的客观评估方法、基于语义相似度的自动评估方法、基于平行语料库的评估方法等。
3. 跨文化翻译研究跨文化翻译是翻译学研究中的一个重要领域,涉及到语言、文化、习惯、价值观等方面的问题。
最新的研究成果包括:跨文化交际能力的提高、语用学在翻译中的应用、文化因素对翻译的影响等。
二、翻译学的应用前景1. 机器翻译在商务领域的应用机器翻译技术的发展,并非要取代人工翻译,而是为了更好地配合和辅助人工翻译的工作。
在商务领域中,机器翻译可以帮助企业翻译商业文件、邮件、合同等文档,提高企业的工作效率和国际化水平。
2. 翻译在旅游领域的应用随着旅游业的发展,翻译在旅游领域中的应用越来越广泛。
旅游翻译可以帮助游客与当地居民和旅游工作者沟通交流,了解当地文化和习俗,提高游客的游览体验和满意度。
3. 翻译在教育领域的应用翻译在教育领域中的应用,可以帮助学生更好地了解外语知识和文化背景,提高他们的语言水平和跨文化交际能力。
教育翻译不仅可以用于外语教学,还可以作为学科知识翻译和学术研究翻译的一种重要手段。
总之,翻译学的最新研究成果和应用前景是十分广泛和丰富的。
未来,随着技术的不断进步和应用场景的扩大,翻译学将在跨领域和跨文化交流中扮演越来越重要的角色。
外文翻译--数据挖掘在CRM中运用

附录一调研报告数据挖掘在CRM中运用(1)通过数据挖掘获得新的客户。
在CRM中首先应识别潜在客户,然后将他们转化为客户。
Big Bank and Credit Card(BB&CC)公司每年通过邮递的方式开展25 次促销活动,每次给一百万人提供申请信用卡的机会,BB&CC 公司会将信用高的申请者接受为服务对象,最终只有1%的申请者成为用户。
BB&CC公司所面临的挑战是如何让邮递促销活动更加有效。
首先,BB&CC公司抽取了一个50,000人的样本,做了一个测试。
在样本测试结果分析的基础上建立了两个模型,一个用来预测谁将填写申请表(使用决策树方法),另一个是信用评估模型(使用神经网络方法)。
从剩下的950,000 个人中再次抽取700,000个样本,使用模型找出哪些人会对促销活动做出反应,并且具有良好的信用。
结果如下:包括建模型时用的50,000 共抽取了750,000个样本,其中9,000 个申请者被接受,接受率从1%上升到了1.2%。
数据挖掘虽然不能准确的识别哪10,000个申请者最终会成为用户,但是可以促使营销活动更加有效。
(2)通过数据挖掘使用交叉销售提高现有客户的价值。
Guns and Rouses(G&R)公司销售的产品是:仿迫击炮与大炮的室外花盆和仿大口径手枪与长枪的室内花盆。
产品表被发往12,000,000个家庭。
当客户电话定购某个产品时,(G&R)公司会积极的推销其它的产品——交叉销售。
但是,(G&R)公司发现只有1/3的客户允许他们提出建议,最终的交叉销售率不足1%,并招致了一片抱怨声。
为此B&R公司想确定到底是哪些人在定购某个产品的同时需要其他的产品。
G&R公司建立了两个数据挖掘模型,一个是用来预测某个客户是否会被建议触怒,另一个用来预测什么样的建议会被很好的接受。
数据挖掘模型使用客户信息数据库中客户的信息和新的客户信息,告诉销售代表哪种人可以采用交叉销售的方式以及建议什么产品。
数据挖掘论文中英文翻译
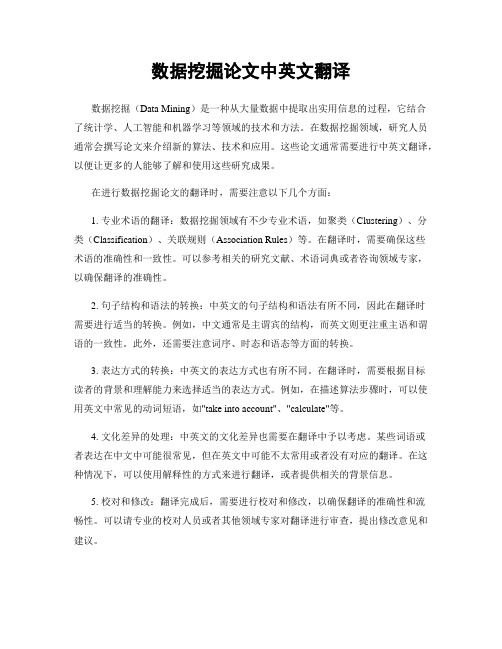
数据挖掘论文中英文翻译数据挖掘(Data Mining)是一种从大量数据中提取出实用信息的过程,它结合了统计学、人工智能和机器学习等领域的技术和方法。
在数据挖掘领域,研究人员通常会撰写论文来介绍新的算法、技术和应用。
这些论文通常需要进行中英文翻译,以便让更多的人能够了解和使用这些研究成果。
在进行数据挖掘论文的翻译时,需要注意以下几个方面:1. 专业术语的翻译:数据挖掘领域有不少专业术语,如聚类(Clustering)、分类(Classification)、关联规则(Association Rules)等。
在翻译时,需要确保这些术语的准确性和一致性。
可以参考相关的研究文献、术语词典或者咨询领域专家,以确保翻译的准确性。
2. 句子结构和语法的转换:中英文的句子结构和语法有所不同,因此在翻译时需要进行适当的转换。
例如,中文通常是主谓宾的结构,而英文则更注重主语和谓语的一致性。
此外,还需要注意词序、时态和语态等方面的转换。
3. 表达方式的转换:中英文的表达方式也有所不同。
在翻译时,需要根据目标读者的背景和理解能力来选择适当的表达方式。
例如,在描述算法步骤时,可以使用英文中常见的动词短语,如"take into account"、"calculate"等。
4. 文化差异的处理:中英文的文化差异也需要在翻译中予以考虑。
某些词语或者表达在中文中可能很常见,但在英文中可能不太常用或者没有对应的翻译。
在这种情况下,可以使用解释性的方式来进行翻译,或者提供相关的背景信息。
5. 校对和修改:翻译完成后,需要进行校对和修改,以确保翻译的准确性和流畅性。
可以请专业的校对人员或者其他领域专家对翻译进行审查,提出修改意见和建议。
总之,数据挖掘论文的中英文翻译需要综合考虑专业术语、句子结构、表达方式、文化差异等方面的因素。
通过准确翻译和流畅表达,可以让更多的人理解和应用这些研究成果,推动数据挖掘领域的发展。
数据挖掘中的名词解释
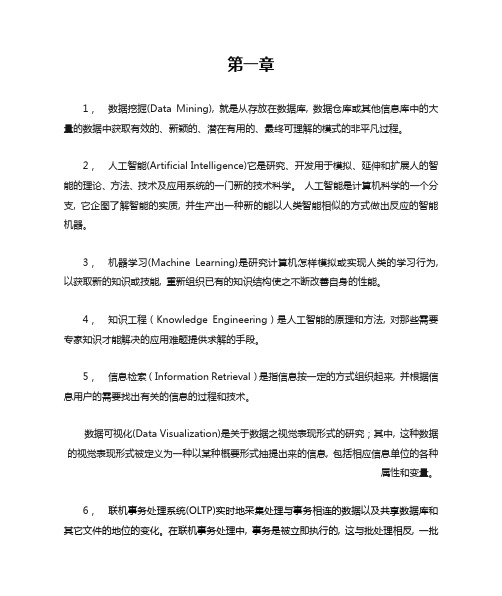
第一章1,数据挖掘(Data Mining), 就是从存放在数据库, 数据仓库或其他信息库中的大量的数据中获取有效的、新颖的、潜在有用的、最终可理解的模式的非平凡过程。
2,人工智能(Artificial Intelligence)它是研究、开发用于模拟、延伸和扩展人的智能的理论、方法、技术及应用系统的一门新的技术科学。
人工智能是计算机科学的一个分支, 它企图了解智能的实质, 并生产出一种新的能以人类智能相似的方式做出反应的智能机器。
3,机器学习(Machine Learning)是研究计算机怎样模拟或实现人类的学习行为, 以获取新的知识或技能, 重新组织已有的知识结构使之不断改善自身的性能。
4,知识工程(Knowledge Engineering)是人工智能的原理和方法, 对那些需要专家知识才能解决的应用难题提供求解的手段。
5,信息检索(Information Retrieval)是指信息按一定的方式组织起来, 并根据信息用户的需要找出有关的信息的过程和技术。
数据可视化(Data Visualization)是关于数据之视觉表现形式的研究;其中, 这种数据的视觉表现形式被定义为一种以某种概要形式抽提出来的信息, 包括相应信息单位的各种属性和变量。
6,联机事务处理系统(OLTP)实时地采集处理与事务相连的数据以及共享数据库和其它文件的地位的变化。
在联机事务处理中, 事务是被立即执行的, 这与批处理相反, 一批事务被存储一段时间, 然后再被执行。
7,8, 联机分析处理(OLAP)使分析人员, 管理人员或执行人员能够从多角度对信息进行快速一致, 交互地存取, 从而获得对数据的更深入了解的一类软件技术。
决策支持系统(decision support)是辅助决策者通过数据、模型和知识, 以人机交互方式进行半结构化或非结构化决策的计算机应用系统。
它为决策者提供分析问题、建立模型、模拟决策过程和方案的环境, 调用各种信息资源和分析工具, 帮助决策者提高决策水平和质量。
互联网金融中英文对照外文翻译文献
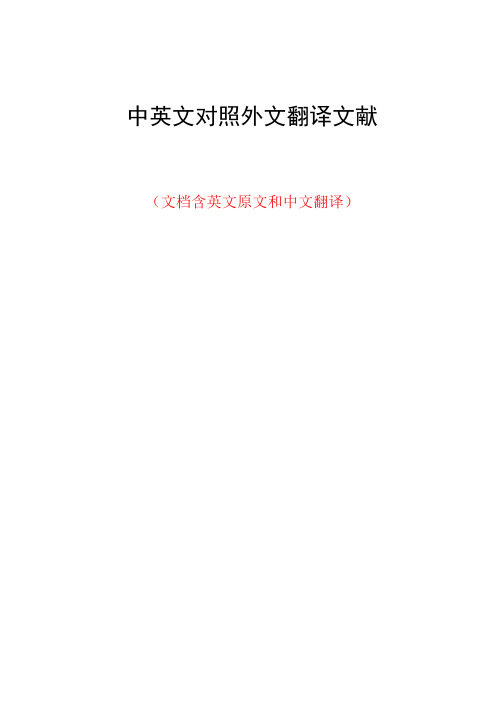
中英文对照外文翻译文献(文档含英文原文和中文翻译)互联网金融对传统金融的影响渗透与融合,是技术发展与市场规律要求的体现,是不可逆转的趋势。
互联网带给传统金融的不仅仅是低成本与高效率,更在于一种创新的思维模式和对用户体验的不懈追求。
而传统金融行业要去积极应对。
互联网金融,对于这样一片足以改变世界的巨大蓝海,是非常值得投入精力去理顺其发展脉络,去从现有的商业模式中发现其发展前景的。
“互联网金融”属于最新的业态形式,对互联网金融进行探讨研究的文献不少,但多缺乏系统性与实践性。
因此本文根据互联网行业实践性较强的特点,对市场上的几种业务模式进行概括分析,并就传统金融行业如何积极应对互联网金融浪潮给出了分析与建议,具有较强的现实意义。
2互联网金融的产生背景互联网金融是以互联网为资源平台,以大数据和云计算为基础的新金融模式。
互联网金融借助于互联网技术、移动通信技术来实现资金融通、支付和信息中介等业务,是传统金融业与以互联网为代表的现代信息科技(移动支付、云计算、数据挖掘、搜索引擎和社交网络等)相结合产生的新兴领域。
不管是互联网金融还是金融互联网,只是战略上的区别,并没有严格定义区分。
随着金融与互联网的相互渗透与相互融合,互联网金融可以泛指一切通过互联网技术来实现资金融通的行为。
互联网金融是互联网与传统金融相互渗透和融合的产物,这种崭新的金融模式有着深刻的产生背景。
互联网金融的出现既源于金融主体对于降低成本的强烈渴求,也离不开现代信息技术迅猛发展提供的技术支撑。
2.1需求型拉动因素传统金融市场存在严重的信息不对称,极大的提高了交易风险;移动互联网的发展逐步改变了人们的金融消费习惯,对服务效率和体验的要求越来越高;此外,运营成本的不断上升,都刺激着金融主体对于金融创新与改革的渴求;这种由需求拉动的因素,成为互联网金融产生的强大内在推动力。
2.2供给型推动因素数据挖掘、云计算以及搜索引擎等技术的发展、金融与互联网机构的技术平台的革新、企业逐利性的混业经营等,为传统金融业的转型和互联网企业向金融领域渗透提供了可能,为互联网金融的产生和发展提供了外在的技术支撑,成为一种外化的拉动力。
- 1、下载文档前请自行甄别文档内容的完整性,平台不提供额外的编辑、内容补充、找答案等附加服务。
- 2、"仅部分预览"的文档,不可在线预览部分如存在完整性等问题,可反馈申请退款(可完整预览的文档不适用该条件!)。
- 3、如文档侵犯您的权益,请联系客服反馈,我们会尽快为您处理(人工客服工作时间:9:00-18:30)。
毕业设计(论文)外文资料翻译系部:计算机科学与技术系专业:计算机科学与技术姓名:学号:外文出处:Proceeding of Workshop on the (用外文写)of Artificial,Hualien,TaiWan,2005不确定性数据挖掘:一种新的研究方向Michael Chau1, Reynold Cheng2, and Ben Kao31:商学院,香港大学,薄扶林,香港2:计算机系,香港理工大学九龙湖校区,香港3:计算机科学系,香港大学,薄扶林,香港摘要由于不精确测量、过时的来源或抽样误差等原因,数据不确定性常常出现在真实世界应用中。
目前,在数据库数据不确定性处理领域中,很多研究结果已经被发表。
我们认为,当不确定性数据被执行数据挖掘时,数据不确定性不得不被考虑在内,才能获得高质量的数据挖掘结果。
我们称之为“不确定性数据挖掘”问题。
在本文中,我们为这个领域可能的研究方向提出一个框架。
同时,我们以UK-means 聚类算法为例来阐明传统K-means算法怎么被改进来处理数据挖掘中的数据不确定性。
1.引言由于测量不精确、抽样误差、过时数据来源或其他等原因,数据往往带有不确定性性质。
特别在需要与物理环境交互的应用中,如:移动定位服务[15]和传感器监测[3]。
例如:在追踪移动目标(如车辆或人)的情境中,数据库是不可能完全追踪到所有目标在所有瞬间的准确位置。
因此,每个目标的位置的变化过程是伴有不确定性的。
为了提供准确地查询和挖掘结果,这些导致数据不确定性的多方面来源不得不被考虑。
在最近几年里,已有在数据库中不确定性数据管理方面的大量研究,如:数据库中不确定性的表现和不确定性数据查询。
然而,很少有研究成果能够解决不确定性数据挖掘的问题。
我们注意到,不确定性使数据值不再具有原子性。
对于使用传统数据挖掘技术,不确定性数据不得不被归纳为原子性数值。
再以追踪移动目标应用为例,一个目标的位置可以通过它最后的记录位置或通过一个预期位置(如果这个目标位置概率分布被考虑到)归纳得到。
不幸地是,归纳得到的记录与真实记录之间的误差可能会严重也影响挖掘结果。
图1阐明了当一种聚类算法被应用追踪带有不确定性位置的移动目标时所发生的问题。
图1(a)表示一组目标的真实数据,而图1(b)则表示记录的已过时的这些目标的位置。
如果这些实际位置是有效的话,那么它们与那些从过时数据值中得到的数据集群有明显差异。
如果我们仅仅依靠记录的数据值,那么将会很多的目标可能被置于错误的数据集群中。
更糟糕地是,一个群中的每一个成员都有可能改变群的质心,因此导致更多的错误。
图1 数据图图1.(a)表示真实数据划分成的三个集群(a、b、c)。
(b)表示的有些目标(隐藏的)的记录位置与它们真实的数据不一样,因此形成集群a’、b’、c’和c”。
注意到a’集群中比a集群少了一个目标,而b’集群中比b集群多一个目标。
同时,c也误拆分会为c’和c”。
(c)表示方向不确定性被考虑来推测出集群a’,b’和c。
这种聚类产生的结果比(b)结果更加接近(a)。
我们建议将不确定性数据的概率密度函数等不确定性信息与现有的数据挖掘方法结合,这样在实际数据可利用于数据挖掘的情况下会使得挖掘结果更接近从真实数据中获得的结果。
本文研究了不确定性怎么通过把数据聚类当成一种激励范例使用使得不确定性因素与数据挖掘相结合。
我们称之为不确定性数据挖掘问题。
在本文中,我们为这个领域可能的研究方向提出一个框架。
文章接下来的结构如下。
第二章是有关工作综述。
在第三章中,我们定义了不确定性数据聚类问题和介绍我们提议的算法。
第四章将呈现我们算法在移动目标数据库的应用。
详细地的实习结果将在第五章解释。
最后在第六章总结论文并提出可能的研究方向。
2.研究背景近年来,人们对数据不确定性管理有明显的研究兴趣。
数据不确定性被为两类,即已存在的不确定生和数值不确定性。
在第一种类型中,不管目标或数据元组存在是否,数据本身就已经存在不确定性了。
例如,关系数据库中的元组可能与能表现它存在信任度的一个概率值相关联[1,2]。
在数据不确定性类型中,一个数据项作为一个封闭的区域,与其值的概率密度函数(PDF)限定了其可能的值[3,4,12,15]。
这个模型可以被应用于量化在不断变化的环境下的位置或传感器数据的不精密度。
在这个领域里,大量的工作都致力于不精确查找。
例如,在[5]中,解决不确定性数据范围查询的索引方案已经被提出。
在[4]中,同一作者提出了解决邻近等查询的方案。
注意到,所有工作已经把不确定性数据管理的研究结果应用于简化数据库查询中,而不是应用于相对复杂的数据分析和挖掘问题中。
在数据挖掘研究中,聚类问题已经被很好的研究。
一个标准的聚类过程由5个主要步骤组成:模式表示,模式定义,模式相似度量的定义,聚类或分组,数据抽象和造工评核[10]。
只有小部分关于数据挖掘或不确定性数据聚类的研究被发表。
Hamdan与Govaert已经通过运用EM算法解决使混合密度适合不确定性数据聚类的问题 [8]。
然而,这个模型不能任意地应用于其他聚类算法因为它相当于为EM定制的。
在数据区间的聚类也同样被研究。
像城区距离或明考斯基距离等不同距离测量也已经被用来衡量两个区间的相似度。
在这些测量的大多数中,区间的概率密度函数并没有被考虑到。
另外一个相关领域的研究就是模糊聚类。
在模糊逻辑中的模糊聚类研究已经很久远了[13]。
在模糊聚类中,一个是数据簇由一组目标的模糊子集组成。
每个目标与每个簇都有一个“归属关系度”。
换言之,一个目标可以归属于多个簇,与每个簇均有一个度。
模糊C均值聚类算法是一种最广泛的使用模糊聚类方法[2,7]。
不同的模糊聚类方法已被应用在一般数据或模糊数据中来产生的模糊数据簇。
他们研究工作是基于一个模糊数据模型的,而我们工作的开展则基于移动目标的不确定性模型。
3.不确定数据的分类在图2中,我们提出一种分类法来阐述数据挖掘方法怎么根据是否考虑数据不准确性来分类。
有很多通用的数据挖掘技术,如: 关联规则挖掘、数据分类、数据聚类。
当然这些技术需要经过改进才能用于处理不确定性技术。
此外,我们区分出数据聚类的两种类型:硬聚类和模糊聚类。
硬聚类旨在通过考虑预期的数据来提高聚类的准确性。
另一方面,模糊聚类则表示聚类的结果为一个“模糊”表格。
模糊聚类的一个例子是每个数据项被赋予一个被分配给数据簇的任意成员的概率。
图2. 不确定性数据挖掘的一种分类例如,当不确定性被考虑时,会发生一个有意思的问题,即如何在数据集中表示每个元组和关联的不确定性。
而且,由于支持和其他指标的概念需要重新定义,不得不考虑改进那些著名的关联规则挖掘算法(如Apriori)。
同样地,在数据分类和数据聚集中,传统算法由于未将数据不确定性考虑在内而导致不能起作用。
不得不对聚类质心、两个目标的距离、或目标与质心的距离等重要度量作重新定义和进行更深的研究。
4.不确定性数据聚类实例在这个章节中,我们将以不确定性数据挖掘的例子为大家介绍我们在不确定性数据聚类中的研究工作。
这将阐明我们在改进传统数据挖掘算法以适合不确定性数据问题上的想法。
4.1 问题定义用S 表示V 维向量x i 的集合,其中i=1到n ,这些向量表示在聚类应用中被考虑的所有记录的属性值。
每个记录o i 与一个概率密度函数f i (x)相联系,这个函数就是o i 属性值x 在时间t 时刻的概率密度函数。
我们没有干涉这个不确定性函数的实时变化,或记录的概率密度函数是什么。
平均密度函数就是一个概率密度函数的例子,它描述“大量不确定性”情景中是最糟的情况[3]。
另一个常用的就是高斯分布函数,它能够用于描述测量误差[12,15]。
聚类问题就是在数据集簇C j (j 从1到K )找到一个数据集C ,其中C j 由基于相似性的平均值c j 构成。
不同的聚类算法对应不对的目标函数,但是大意都是最小化同一数据集目标间的距离和最大化不同数据集目标间的距离。
数据集内部距离最小化也被视为每个数据点之间距离x i 以及x i 与对应的C j 中平均值c j 距离的最小化。
在论文中,我们只考虑硬聚类,即,每个目标只分配给一个一个集群的一个元素。
4.2 均值聚类在精确数据中的应用这个传统的均值聚类算法目的在于找到K(也就是由平均值c j 构成数据集簇C j )中找到一个数据集C 来最小化平方误差总和(SSE )。
平方误差总和通常计算如下:∑∑=∈-K j x i j ji x c 1C 2 (1)|| . ||表示一个数据点x i 与数据集平均值c j 的距离试题。
例如,欧氏距离定义为:∑=-=-V i i i y x y x 12(2)一个数据集C i 的平均值(质心)由下面的向量公式来定义:∑∈=j C i i j i x C c 1 (3)均值聚类算法如下:1. Assign initial values for cluster means c 1 to c K2. repeat3. for i = 1 to n do4. Assign each data point x i to cluster C j where || c j - x i || is the minimum.5. end for6. for j = 1 to K do7. Recalculate cluster mean c j of cluster C j8. end for9. until convergence10. return C收敛可能基于不同的质心来确定。
一些收敛性判别规则例子包括:(1)当平方误差总和小于某一用户专用临界值,(2)当在一次迭代中没有一个目标再分配给不同的数据集和(3)当迭代次数还达到预期的定义的最大值。
4.3 K-means 聚类在不确定性数据中的应用为了在聚类过程中考虑数据不确定性,我们提出一种算法来实现最小化期望平方误差总和E(SSE)的目标。
注意到一个数据对象x i 由一个带有不确定性概率密度f(x i )的不确定性区域决定。
给定一组数据群集,期望平方误差总和可以计算如下:()ii K j C i ij Kj C i ij Kj C i i j dx x f x c x c E x c E j jj )(121212∑∑∑∑∑∑=∈=∈=∈-=-=⎪⎪⎭⎫ ⎝⎛- (4) 数据集平均值可以如下给出: ()∑⎰∑∑∈∈∈==⎪⎪⎭⎫ ⎝⎛=j jj C i ii i j C i i j C i i j j dx x f x C x E C x C E c )(111 (5) 我们到此将提出一种新K-means 算法,即UK-means ,来实现不确定性数据聚类。
1. Assign initial values for cluster means c 1 to c K2. repeat3. for i = 1 to n do4. Assign each data point x i to cluster C j where E(|| c j - x i ||) is the minimum.5. end for6. for j = 1 to K do7. Recalculate cluster mean c j of cluster C j8. end for9. until convergence10. return CUK-mean 聚类算法与K-means 聚类算法的最大不同点在于距离和群集的计算。