基于ENVI的TM与SAR数据融合处理流程介绍
基于小波纹理信息的星载SAR图像与TM图像的数据融合
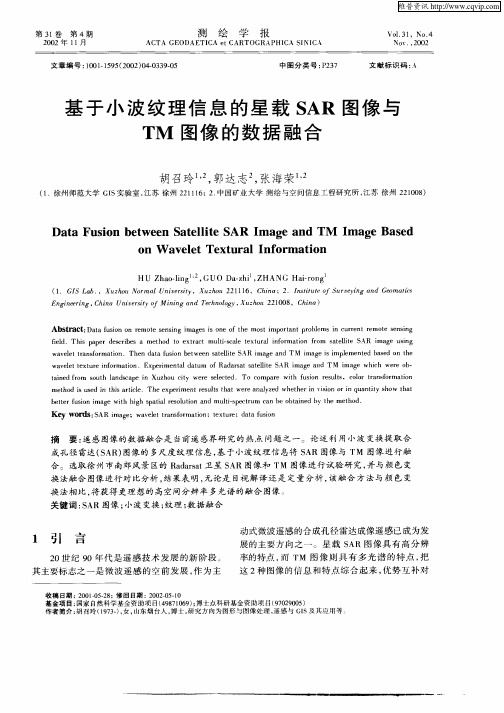
中 图 分 类 号 :2 7 P 3
文献标识码 : A
基于小波纹理信息的星载 S AR 图像 与 T 图像 的 数 据 融 合 M
胡 召 玲 , 达 志2张 海 荣 2 2郭 ,
(. 1 徐州 师范大学 G S实验室 , I 江苏 徐 州 2 1 1 ;2 中国矿业 大学 测绘 与空间信息工 程研究所 , 2 16 . 江苏 徐州 2 10 ) 2 0 8
Ke r : AR i g ;wa ee rn fr t n e t r ;d t u in y wo ds S ma e v ltta somai ;txu e aaf s o o
摘 要 : 遥感 图像 的数据 融 合是 当前遥 感界 研 究的 热 点 问题 之 一 。论 述 利 用 小 波 变换 提 取 合
i d.Th s p p r d s r e t o o e t c mu t s ae t x u a n o ma in fo s tl t S fl e i a e ec i s a me h d t x r t b a l — l e t r i f r t r m a e i ic l o l e AR ma e u i g i g sn w v l tt n f r t n.Th n d t u i n b t e a e i AR i g n a ee r so ma i a o e aa f s e we n s t l t S ma e a d TM ma e i i l me t d b s d o h o e i g s mpe n e a e n t e wa ee e t r n o ma in.E p rme t a u fRa a s ts tl t AR ma e a d TM ma e wh c r b v ltt x u e i f r t o x e i n a d t m o d ra a el e S l i i g n i g ih we e o ・ t ie r m o t a d a e i z o i r ee td. To c mp r t u i n r s t ,c l r ta so ma i n a n d fo s u h l n s p n Xu h u ct we e s lce c v o a e wi f s e u s o o r n f r t h o l o me h d i u e n t i a t l .Th x e i n e u t t a r n y e e h ri ii n o n q a tt h w t a t o s s d i h s ri e c e e p rme trs l h twe ea a z d wh t e vso ri u n iy s o h t s l n b te u i n i g t i h s a i eo u i n a d mut s e tu c n b b a n d b h t o . e t rf so ma e wih h g p t rs l t n li p c r m a e o ti e y t e me h d l a o —
融合方法介绍
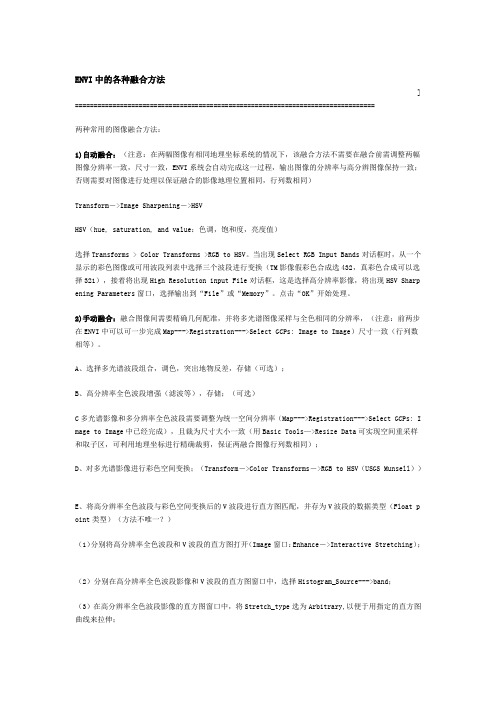
ENVI中的各种融合方法] ================================================================================两种常用的图像融合方法:1)自动融合:(注意:在两幅图像有相同地理坐标系统的情况下,该融合方法不需要在融合前需调整两幅图像分辨率一致,尺寸一致,ENVI系统会自动完成这一过程,输出图像的分辨率与高分辨图像保持一致;否则需要对图像进行处理以保证融合的影像地理位置相同,行列数相同)Transform->Image Sharpening->HSVHSV(hue, saturation, and value:色调,饱和度,亮度值)选择Transforms > Color Transforms >RGB to HSV。
当出现Select RGB Input Bands对话框时,从一个显示的彩色图像或可用波段列表中选择三个波段进行变换(TM影像假彩色合成选432,真彩色合成可以选择321),接着将出现High Resolution input File对话框,这是选择高分辨率影像,将出现HSV Sharp ening Parameters窗口,选择输出到“File”或“Memory”。
点击“OK”开始处理。
2)手动融合:融合图像间需要精确几何配准,并将多光谱图像采样与全色相同的分辨率,(注意:前两步在ENVI中可以可一步完成Map--->Registration--->Select GCPs: Image to Image)尺寸一致(行列数相等)。
A、选择多光谱波段组合,调色,突出地物反差,存储(可选);B、高分辨率全色波段增强(滤波等),存储;(可选)C多光谱影像和多分辨率全色波段需要调整为统一空间分辨率(Map--->Registration--->Select GCPs: I mage to Image中已经完成),且裁为尺寸大小一致(用Basic Tools—>Resize Data可实现空间重采样和取子区,可利用地理坐标进行精确裁剪,保证两融合图像行列数相同);D、对多光谱影像进行彩色空间变换;(Transform->Color Transforms->RGB to HSV(USGS Munsell))E、将高分辨率全色波段与彩色空间变换后的V波段进行直方图匹配,并存为V波段的数据类型(Float p oint类型)(方法不唯一?)(1)分别将高分辨率全色波段和V波段的直方图打开(Image窗口:Enhance->Interactive Stretching);(2)分别在高分辨率全色波段影像和V波段的直方图窗口中,选择Histogram_Source--->band;(3)在高分辨率全色波段影像的直方图窗口中,将Stretch_type选为Arbitrary,以便于用指定的直方图曲线来拉伸;(4)用鼠标将V波段影像直方图的输入(Input Histogram标签)拖动至在高分辨率全色波段影像的直方图的输出窗口(Output Histogram)中,然后点击Apply;(5)在V波段的直方图窗口中,选择Options->Histogram Parameters,记录下Histogram Min和Hist ogram Max两个值;(6)在高分辨率全色波段影像的直方图窗口中,选择File—>Export Stretch,将刚才记下的两个值分别填入Output Min和Output Max中;再将“Output Data Type”改为“Floating Point”,然后给定文件名存储;F、彩色空间变换的反变换。
实验报告二 使用ENVI进行TM和SPOT数据融合 - 副本

《遥感原理与应用》实验报告实验内容:遥感影像的融合学生姓名:李明明学号:2010104306学院班级:地科学院10测绘工程(2)班指导教师姓名:方刚指导教师职称:副教授实验报告二使用ENVI进行TM和SPOT数据融合一、实验目的理解数据融合的基本原理,数据融合的过程,掌握ENVI环境下数据融合的方法。
二、实验内容1.手动数据融合2.自动数据融合三、实验准备数据:文件夹bldr_reg、lontmspFile 自动融合数据手动融合数据bldrtm_m.hdrbldrtm_m.imgbldrtm_m.pts lon_spotbldr_sp.hdr lon_spot.ers bldr_sp.img lon_tmbldr_sp.grd lon_tm.ers bldr_sp.ann copyright.txt bldr_sp.sta四、实验步骤使用ENVI进行数据融合的文件若带地理坐标要进行空间重采样,如果不带地理坐标,对同一地理区域,要具有相同的像素大小,同样的图像大小,和相同的方向。
在这个练习中使用的文件不带地理坐标,因此对低分辨率图像要求具有相同的像素大小,对高空间分辨率图像要用最近邻重采样。
一、读取并显示ERMapper影像1.选择File → Open External File → IP Software → ER Mapper,浏览到lontmsp 子目录,选择文件lon_tm.ers并打开。
2.在available bands list中点击RGB颜色单选按钮,点击顺序为Red Layer、Green Layer 和Blue Layer,然后点击Load RGB,显示一个真色彩Landsat TM 影像,如图1。
3.选择File → Open External File→IP Software → ER Mapper, 浏览到lontmsp 子目录,选择文件lon_spot.ers并打开。
TM和SAR遥感图像的不同层次融合分类比较

TM和SAR遥感图像的不同层次融合分类比较
于秀兰;钱国蕙
【期刊名称】《遥感技术与应用》
【年(卷),期】1999(14)3
【摘要】多传感器遥感图像为分类技术提供了更多的地物特征信息,有助于提高分类精度,增强计算机自动解译的能力,减少遥感图像的后处理时间。
给出了TM和SAR遥感图像根据信息处理所在的不同层次融合分类特征的选取及分类方法,并从融合分类的精度和计算时间消耗上对哈尔滨市附近地区512×512大小的TM和JERS1的SAR图像的不同层次融合的分类进行比较。
【总页数】6页(P38-43)
【关键词】遥感图像;TM;SAR;图像处理;图像融合
【作者】于秀兰;钱国蕙
【作者单位】哈尔滨工业大学航天电子与光电工程系
【正文语种】中文
【中图分类】TP751
【相关文献】
1.SAR与TM影像融合及在BP神经网络分类中的应用 [J], 张海龙;蒋建军;吴宏安;解修平
2.TM和SAR遥感图象特征层融合分类方法的研究 [J], 于秀兰;钱国蕙
3.多光谱和SAR遥感图像融合分类的特征选取 [J], 于秀兰;钱国蕙;贾晓光
4.不同极化方式SAR与TM融合影像滨海湿地分类精度比较 [J], 王霄鹏;张杰;马毅;任广波
5.SAR和TM图像主成分变换融合中不同主分量替换的比较 [J], 杨存建;许?;张增祥
因版权原因,仅展示原文概要,查看原文内容请购买。
ENVI中的融合方法
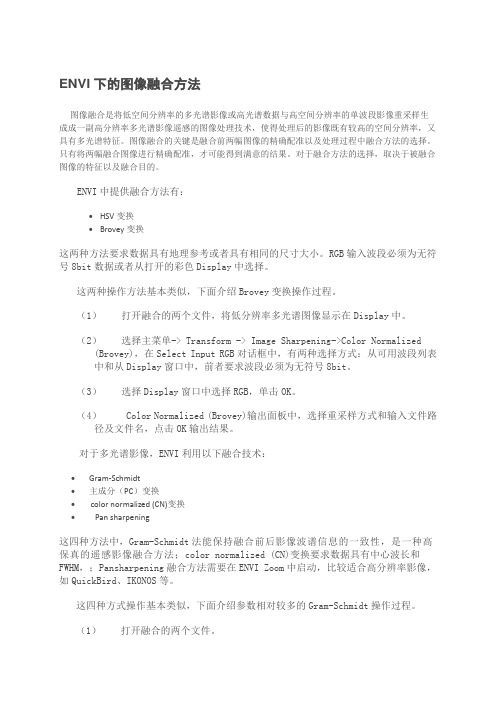
ENVI下的图像融合方法图像融合是将低空间分辨率的多光谱影像或高光谱数据与高空间分辨率的单波段影像重采样生成成一副高分辨率多光谱影像遥感的图像处理技术,使得处理后的影像既有较高的空间分辨率,又具有多光谱特征。
图像融合的关键是融合前两幅图像的精确配准以及处理过程中融合方法的选择。
只有将两幅融合图像进行精确配准,才可能得到满意的结果。
对于融合方法的选择,取决于被融合图像的特征以及融合目的。
ENVI中提供融合方法有:∙HSV变换∙Brovey变换这两种方法要求数据具有地理参考或者具有相同的尺寸大小。
RGB输入波段必须为无符号8bit数据或者从打开的彩色Display中选择。
这两种操作方法基本类似,下面介绍Brovey变换操作过程。
(1)打开融合的两个文件,将低分辨率多光谱图像显示在Display中。
(2)选择主菜单-> Transform -> Image Sharpening->Color Normalized (Brovey),在Select Input RGB对话框中,有两种选择方式:从可用波段列表中和从Display窗口中,前者要求波段必须为无符号8bit。
(3)选择Display窗口中选择RGB,单击OK。
(4) Color Normalized (Brovey)输出面板中,选择重采样方式和输入文件路径及文件名,点击OK输出结果。
对于多光谱影像,ENVI利用以下融合技术:∙Gram-Schmidt∙主成分(PC)变换∙color normalized (CN)变换∙Pan sharpening这四种方法中,Gram-Schmidt法能保持融合前后影像波谱信息的一致性,是一种高保真的遥感影像融合方法;color normalized (CN)变换要求数据具有中心波长和FWHM,;Pansharpening融合方法需要在ENVI Zoom中启动,比较适合高分辨率影像,如QuickBird、IKONOS等。
基于ENVI的SAR数据处理流程介绍
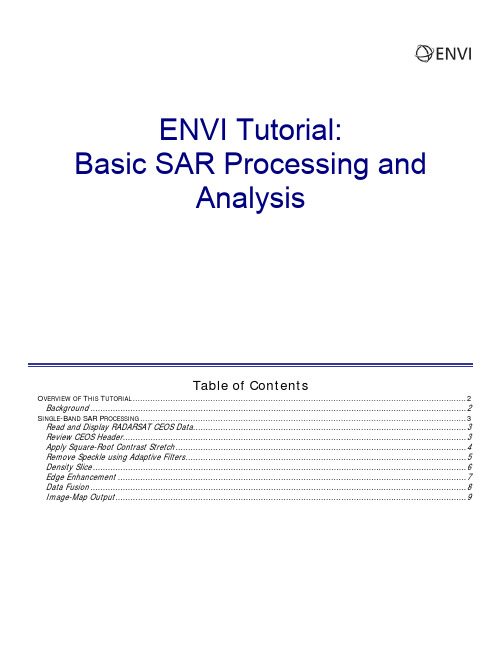
ENVI Tutorial:Basic SAR Processing andAnalysisTable of ContentsO VERVIEW OF T HIS T UTORIAL (2)Background (2)S INGLE-B AND SAR P ROCESSING (3)Read and Display RADARSAT CEOS Data (3)Review CEOS Header (3)Apply Square-Root Contrast Stretch (4)Remove Speckle using Adaptive Filters (5)Density Slice (6)Edge Enhancement (7)Data Fusion (8)Image-Map Output (9)Overview of This TutorialThis tutorial is designed to give you a working knowledge of ENVI’s basic tools for processing single-band synthetic aperture radar (SAR) data such as RADARSAT, ERS-1, and JERS-1.Files Used in This TutorialENVI Resource DVD: envidata\rsat_subFile Descriptionlea_01.001 RADARSAT leader filebonnrsat.img (.hdr) RADARSAT image subsetrsi_f1.img (.hdr) Frost filter resultdslice.dsr Density slice filersi_f2.img (.hdr) Laplacian filter resultrsi_f3.img (.hdr) Laplacian filter result with 90% add-backrsi_fus.img (.hdr) Simulated fused TM and RADARSATrsi_map.jpg RADARSAT map composition exampleBackgroundUse the Radar menu in ENVI to access standard and advanced tools for analysis of detected radar images and advanced SAR systems such as NASA/Jet Propulsion Laboratory's (JPL's) fully polarimetric AIRSAR and SIR-C systems. ENVI can process ERS-1, JERS-1, RADARSAT, SIR-C, X-SAR, and AIRSAR data and any other detected SAR dataset. In addition, ENVI is designed to handle radar data distributed in the CEOS format.Most standard ENVI processing functions are inherently radar-capable, including all display capabilities, stretching, color manipulations, classification, registration, filters, geometric rectification, and so on. Additional specialized tools are provided for analyzing polarimetric radar data. A typical processing flow may include reviewing the CEOS header, reading the CEOS data, displaying and contrast stretching, removing speckle using an adaptive filter, density slicing, edge enhancement, data fusion, and map composition.Single-Band SAR ProcessingThis section describes a typical single-band SAR processing scenario from data input through processing and analysis, to publication-quality or map output. You will use a subsetted RADARSAT 1 Path Image, Fine Beam 2, from December 17, 1995, Bonn, Germany.Read and Display RADARSAT CEOS DataENVI provides the tools to read generic CEOS data tapes and RADARSAT data from both tape and CD-ROM. To read data from tape, select File → Tape Utilities → Read Known Tape Formats → RADARSAT CEOS. To read original RADARSAT data from disk or CD, select Radar → Open/Prepare Radar File → RADARSAT. For this tutorial, a RADARSAT image subset has already been extracted.1.From the ENVI main menu bar, select File→Open Image File. A file selection dialog appears.2.Navigate to envidata\rsat_sub and select bonnrsat.img. Click Open.3.In the Available Bands List, select the Gray Scale radio button and click Load Band. The following figure showsthe subsetted RADARSAT image of Bonn, Germany, with a 2% linear stretch applied. These data were acquired during the RADARSAT commissioning phase and should not be used for scientific analysis or interpretation. Data are copyright, RADARSAT, 1995.Review CEOS HeaderMany SAR datasets are distributed in CEOS format. ENVI provides generic tools to read CEOS headers and display CEOS header information on the screen. ENVI also has tools specifically designed to read RADARSAT CEOS headers, which contain additional information.1.From the ENVI main menu bar, select Radar→Open/Prepare Radar File→View RADARSAT Header. Afile selection dialog appears.2.Select the RADARSAT leader file lea_01.001. A CEOS Header Report dialog appears.3.Browse the information in the CEOS Header Report, then close the dialog when you are finished.Apply Square-Root Contrast StretchRadar data typically cover a large range of data values. As seen above, default linear stretches do not perform a very good job of enhancing the contrast in most radar images. ENVI’s square-root stretch spreads out radar data over a given range of gray scales better than other types of stretches, resulting in an improved display of radar images.1.From the Display group menu bar, select Enhance→[Image] Square Root. The stretch is applied based onthe statistics of the data in the Image window. The following figure shows a square-root stretch of the BonnRADARSAT image. Compare to the linear contrast stretch above.2.In the Available Bands List, click Display #1 and select New Display. Click Load Band again to display theimage with the default 2% linear stretch.3.From a Display group menu bar, select Tools→Link→Link Displays. Click OK to link the square-root imagewith the 2% linear stretch image. Click in an Image window to toggle between the two images.Remove Speckle using Adaptive FiltersAdaptive filters remove radar speckle from images without seriously affecting the spatial characteristics of the data. The Frost-filtered image shown below is a considerable improvement over the unfiltered data. The Frost filter, an exponentially damped, circularly symmetric filter that uses local statistics, is used to reduce speckle while preserving edges in the data. The pixel being filtered is replaced with a value calculated based on the distance from the filter center, the damping factor, and the local variance.1.From the ENVI main menu bar, select Radar→Adaptive Filters→Frost. A Frost Filter Input File dialogappears.2.Select bonnrsat.img and click OK. A Frost Filter Parameters dialog appears.e the default Filter Size (3x3) and Damping Factor (1.0). Enter an output filename and click OK.4.In the Available Bands List, select the new Frost-filtered image and click Load Band. Or, load the pre-generatedfile rsi_f1.img to a new display group and apply a square-root stretch.5.From a Display group menu bar, select Tools→Link→Link Displays. Click OK to link the Frost-filtered imagewith the 2% linear stretch image. Click in an Image window to toggle between the two images.Density SliceDensity slicing visually enhances radar differences based on image brightness. The density-sliced image below has four levels, with higher radar backscatter in the warmer colors.1.From the Display group menu bar associated with the Frost-filtered image, select Tools→Color Mapping→Density Slice. A Density Slice Band Choice dialog appears.2.Select the name of your Frost-filtered image and click OK. A Density Slice dialog appears.3.From the Density Slice dialog menu bar, select File→Restore Ranges. A file selection dialog appears.4.Select dslice.dsr and click Open.5.Click Apply in the Density Slice dialog. Use image-linking and dynamic overlays to compare the density-slicedimage to the gray scale images.6.When you are finished, close the Density Slice dialog.Edge EnhancementA Laplacian filter is a convolution filter that enhances edges in SAR and other data types. A 5 x 5 filter has the following kernel:0 0 -1 0 00 -1 -2 -1 0-1 -2 16 -2 -10 -1 -2 -1 00 0 -1 0 01.From the ENVI main menu bar, select Filter→Convolutions and Morphology.2.From the the Convolutions and Morphology Tool dialog menu bar, select Convolutions→Laplacian.3.Set the Kernel Size field to 5.4.Click Quick Apply. An input band selection dialog appears. Select bonnrsat.img and click OK. Or, view thepre-generated file rsi_f2.dat. Applying the kernel in this manner strongly enhances the edges while losing most of the radiometric information in the image.5.Repeat Steps 1-4, but set the Image Add Back field to 90.6.Click Quick Apply. Or, view the pre-generated file rsi_f3.dat. The following figure shows Laplacian filterresults (left) and 90% Add Back results (right):e image linking and dynamic overlays to compare these results with the original gray scale images.8.When you are finished, close the Convolutions and Morphology Tool dialog.9.From the ENVI main menu bar, select File→Exit.Data FusionSAR data complement other types of data by providing spatial information that other data do not contain. Conversely, SAR data do not have the composition expressed in multispectral, optical data. Therefore, a combined SAR/optical data analysis is usually required.You can use an intensity-hue-saturation (IHS) transform to combine a multispectral, color-composite image with a monochromatic SAR-sharpening band. ENVI provides a simple tool to conduct data merging using IHS.See the Landsat TM and SAR Data Fusion tutorial for more information, and view the pre-generated, fused TM/SAR file rsi_fus.img.Image-Map OutputOutput from ENVI image processing usually consists of a map-oriented, scaled image-map for presentation or visual analysis and interpretation. Radar data can be used in a map composition, just like any other dataset. See the Map Registration and Map Composition tutorials for more information.。
ENVI 5.2+SARscape5.1安装说明

ENVI 5.2+SARscape5.1安装指南(试用许可)Esri中国信息技术有限公司2015年01月技术支持热线:400-819-2881 转7 E-mail:envi-idl@目录ENVI 5.2安装指南 (1)Esri中国信息技术有限公司 (1)1软硬件要求 (2)1.1硬件要求 (2)1.2软件要求 (2)2安装软件 (2)2.1运行安装文件 (2)2.2安装确认 (3)2.3产品选择 (4)2.4开始安装 (5)2.5安装许可 (6)3验证 (9)1软硬件要求1.1硬件要求硬盘空间:5.0G以上交换空间:2.0G以上内存:512M及以上(建议1024M以上)CPU:Intel/AMD 64-bit显示设备:8bit(24bit或以上)SUN CG3, GX,SX,3D+800*600*256建议(1024*768*32)1.2软件要求•操作系统:(详见支持操作系统说明文档)Window XP SP2,Vista,7,8,10Mac OS X (32-bit and 64-bit) 10.8 10.9LINUX Kernel 2.6.32,glibc 2.122安装软件本说明书的安装过程是在Windows 8 64位操作系统下完成的,其他Windows 系统类似。
2.1运行安装文件插入光盘,如不自动运行请双击光盘内的autorun.exe,在弹出界面中选择64-bit ENVI(如果是网站下载,双击运行IDL84ENVI52win64.exe,本说明即使用此安装介质完成)。
注:如操作系统中缺少必要的组件MSVC_SP1_x64,安装程序会自动安装。
安装ENVI/IDL需要管理员权限,如下图所示。
图2-1 提示管理员权限运行图2-2准备安装2.2安装确认图2-3确认安装图2-4 接受安装协议选择安装路径,默认路径是C:\Program Files\Exelis图2-5 选择安装路径2.3产品选择可选的产品包括ENVI、ENVI LiDAR,IDL产品是必须安装。
(完整版)03-SAR数据基本处理
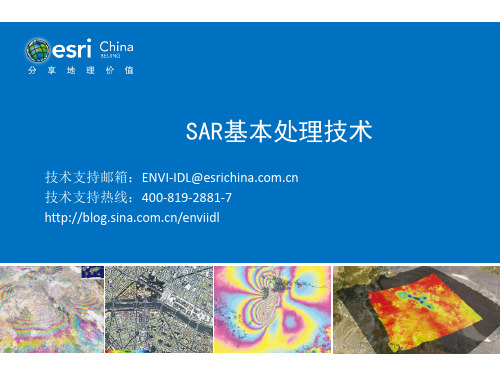
– 距离引起的损失(R3): 接收功率要由传播过程中从远到近的距离 变化校正
辐射定标——结果
• 一般采用以下命名
– Beta Nought (ß°) 雷达亮度(反射率)系数,在斜距方向每单位面 积的反射率单位是无量纲的。这种归一化的优点是不需要入射角 (如散射面积A)
– Sigma Nought (so), 后向散射系数,就是通常说的散射体反射回来 的雷达强度,单位是dB,Sigma nought的定义是假设入射到水平面, 其差异与入射角、波长、极化、散射体的物理性质有关
– Gamma (g) ,用入射角归一化的后向散射系数
辐射归一化
• 严格的定标之后,可识别在距离方向的后向散射系数。因 为地物反射的能量取决于入射角,实际上,获取数据的幅 宽越大,在距离向的后向散射系数的变化越大,这种变化 和散射体的物理特性有关,是不能被校正的,只能通过一 些相对的方法来弥补,如标准化
SAR基本处理技术
技术支持邮箱:ENVI-IDL@ 技术支持热线:400-819-2881-7 /enviidl
主要内容
1. 数据导入 2. 单景雷达影像处理 3. 多时相雷达影像处理
1.数据导入
数据导入
• 目的:生成SARscape识别的数据格式 • 支持SAR数据, 光学数据,高程数据
– 局部入射角校正
– 叠掩/阴影处理
左-后向散射系数,中-局部入射角地图,右-叠掩/阴影地图
练习:地理编码和辐射定标
• 工具:/SARscape/Basic/Intensity Processing/Geocoding/Geocoding and Radiometric Calibration
实验五 使用ENVI进行TM和SPOT数据融合
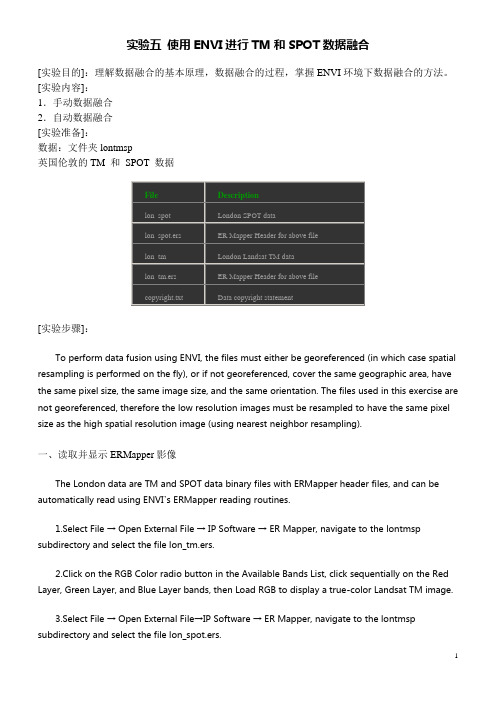
实验五使用ENVI进行TM和SPOT数据融合[实验目的]:理解数据融合的基本原理,数据融合的过程,掌握ENVI环境下数据融合的方法。
[实验内容]:1.手动数据融合2.自动数据融合[实验准备]:数据:文件夹lontmsp英国伦敦的TM 和SPOT 数据[实验步骤]:To perform data fusion using ENVI, the files must either be georeferenced (in which case spatial resampling is performed on the fly), or if not georeferenced, cover the same geographic area, have the same pixel size, the same image size, and the same orientation. The files used in this exercise are not georeferenced, therefore the low resolution images must be resampled to have the same pixel size as the high spatial resolution image (using nearest neighbor resampling).一、读取并显示ERMapper影像The London data are TM and SPOT data binary files with ERMapper header files, and can be automatically read using ENVI’s ERMapper reading routines.1.Select File → Open External File → IP Software → ER Mapper, navigate to the lontmsp subdirectory and select the file lon_tm.ers.2.Click on the RGB Color radio button in the Available Bands List, click sequentially on the Red Layer, Green Layer, and Blue Layer bands, then Load RGB to display a true-color Landsat TM image.3.Select File →Open External File→IP Software →ER Mapper, navigate to the lontmsp subdirectory and select the file lon_spot.ers.4.Click on the Gray Scale radio button in the Available Bands List, click on the Pseudo Layer band, select New Display from the pull-down Display menu, then click Load Band to display the gray scale SPOT image.二、调整影像大小1.Click first on the SPOT image band in the Available Bands List and note its spatial dimensions (2820 x 1569) and then click on the Landsat TM and note its spatial dimensions (1007 x 560). The Landsat data have a spatial pixel size of 28 meters, while the SPOT data are 10 meter spatial resolution. The Landsat image has to be resized by a factor of2.8 to create 10 m data that matches the SPOT data.2.Select Basic Tools → Resize Data (Spatial/Spectral) and choose the lon_tm image then click OK. Enter a value of 2.8 into the xfac text box in the Resize Data Parameters dialog. Enter a value of 2.8009 into the yfac text box (the value of 2.8009 rather than 2.8 must be used to add an extra pixel to the y dimension so the images will match exactly). This difference is insignificant for the purposes of this exercise, but might be important for an actual application. Enter an output filename and click OK to resize the TM image.3.Display the resized image and select Tools →Link →Link Displays to link the resized TM image and the SPOT Panchromatic image. Use the dynamic overlay to compare the two images.三、手动HSI数据融合1.HSV正变换1)Select Transform →Color Transforms →RGB to HSV from the ENVI main menu, and select the resized TM data as the RGB image from the Display. Enter an output filename and click OK to perform the transform.2)Display the Hue, Saturation, and Value images as gray scale images or an RGB.2.拉伸SPOT影像并替换TM的数值波段1)Select Basic Tools →Stretch Data from the ENVI main menu, click on the lon_spot file and then OK.2)In the Output Data portion of the Data Stretching dialog, enter the value 0 for the Min and 1.0 for the Max values, enter an output filename, and click OK to stretch the SPOT data to floating point data with a range of 0 to 1.0.3.HSV反变换1)Select Transform → Color Transforms → HSV to RGB from the ENVI main menu, and select the transformed TM Hue and Saturation bands as the H and S bands for the transformation.2)Choose the stretched SPOT data as the V band for the transform, click OK. Enter an output filename into the HSV to RGB Parameters dialog and click OK to perform the inverse transform.4.结果显示1)Click on the RGB Color radio button in the Available Bands List, click sequentially on the transformed R, G, and B bands, then click the Load RGB button to display a fused true-color Landsat TM/SPOT image.2)Display the fused image and select Tools → Link → Link Displays to link with the resized TM image and the SPOT Panchromatic image. Use the dynamic overlay to compare the images.四、自动HSV变换融合1.Select Transform → Image Sharpening → HSV from the ENVI main menu.2.If you have the resized TM color image displayed, choose the appropriate display in the Select Input RGB dialog. Otherwise, choose the “Red Layer”, “Green Layer”, and “Blue Layer”from the resized TM Image in the Select Input RGB Input Bands dialog and click OK.3.Choose the SPOT image in the High Resolution Input File dialog and click OK.4.Enter the output file name lontmsp.img and click OK in the HSV Sharpening Parameters dialog.五、结果显示、链接和比较5.Load the fused color image into a new display by selecting the RGB Color radio button in the Available Bands List dialog, selecting the R, G, and B bands from the new file and clicking Load RGB.pare the HSV sharpened color image to the original TM color composite, to the SPOT data, and to the manual data fusion result by selecting Tools →Link Displays →Link from the Main Display Window menu bar.六、结束ENVI。
ENVI对SAR数据的预处理过程详细版
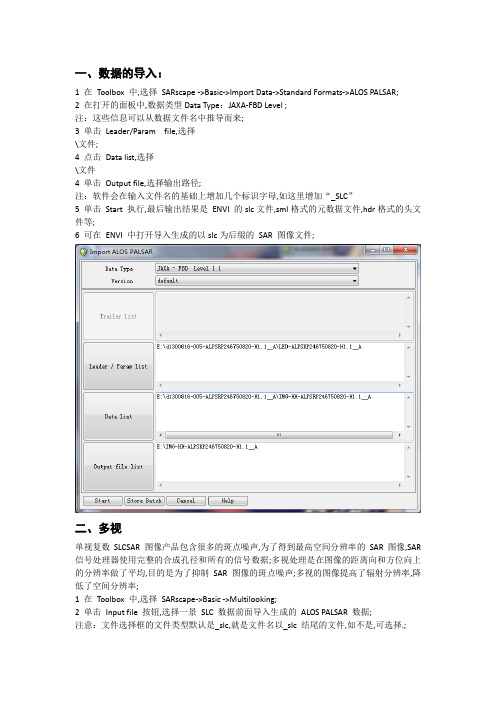
一、数据的导入:1 在Toolbox 中,选择SARscape ->Basic->Import Data->Standard Formats->ALOS PALSAR;2 在打开的面板中,数据类型Data Type:JAXA-FBD Level ;注:这些信息可以从数据文件名中推导而来;3 单击Leader/Param file,选择\文件;4 点击Data list,选择\文件4 单击Output file,选择输出路径;注:软件会在输入文件名的基础上增加几个标识字母,如这里增加“_SLC”5 单击Start 执行,最后输出结果是ENVI 的slc文件,sml格式的元数据文件,hdr格式的头文件等;6 可在ENVI 中打开导入生成的以slc为后缀的SAR 图像文件;二、多视单视复数SLCSAR 图像产品包含很多的斑点噪声,为了得到最高空间分辨率的SAR图像,SAR 信号处理器使用完整的合成孔径和所有的信号数据;多视处理是在图像的距离向和方位向上的分辨率做了平均,目的是为了抑制SAR 图像的斑点噪声;多视的图像提高了辐射分辨率,降低了空间分辨率;1 在Toolbox 中,选择SARscape->Basic ->Multilooking;2 单击Input file 按钮,选择一景SLC 数据前面导入生成的ALOS PALSAR 数据;注意:文件选择框的文件类型默认是_slc,就是文件名以_slc 结尾的文件,如不是,可选择.;3 设置:方位向视数Azimuth Looks:5,距离向视数Range Looks:1注:详细的计算方法如下所述;另外,单击Look 按钮可以估算视数;4 Border Resize 选项,选择此项,会对检测结果边缘中的无效值,进而重新计算输出图像的大小;这里不选择;5 输出路径会依据软件默认参数设置自动添加或自行修改,单击Start 按钮执行;6 计算完之后在Display 中显示结果,可以看到图像的斑点噪声得到的抑制,但是降低了空间分辨率注:方位向视数Azimuth Looks;距离向视数Range Looks视数跟距离向分辨率、方位向分辨率以及中心入射角有关;精确的计算方法如下:地面分辨率= pixel spacing slant range / sinincidence angle•pixel spacing azimuth = 方向分辨率•pixel spacing slant range = 距离分辨率•incidence angle scene centre = °•地距的距离分辨率:/ sin° = m,距离向视数为1•地距的方位向分辨率经过多视后保持与地距的距离分辨率一致•=5,因此方位向视数为5三、滤波从连贯SAR 传感器中获取的图像都有斑点噪声,SARscape 提供两大类滤波,用于单波段雷达图像的滤波和多时相雷达图像滤波;1 Toolbox 中,选择SARscape->Basic->Filtering->Single Image;2 打开Filtering Single Image 面板,单击Input file 按钮,选择前面得到的多视处理结果;3 有8 中滤波供选择,选Frost,同时会打开Frost Lee/Refined Lee 对话框;4 在Frost Lee/Refined Lee 对话框中,分别设置Azimuth Window Size 和Range WindowSize:95 单击Start 执行;四、地理编码和辐射定标SAR 系统是测量发射和返回脉冲的功率比,这个比值就是后向散射被投影为斜距几何;由于不同SAR 传感器或者不同接收模式,为了更好的对比SAR 图像几何和辐射特征,需要将SAR 数据从斜距或地距投影转换为地理坐标投影制图参考系;1 Toolbox 中,选择SARscape->Basic->Geocoding->Geocoding and Radiometric Calibration;2 在Geocoding and Radiometric Calibration 面板中,单击Input file 按钮,选择前面Frost滤波做的结果;3 Cartographic System,设置输出投影参数;5 像元大小GRID SIZE:x:25,y:25;6 重采样方法RESAMPLE:有5 中方法供选择,从左到右精度提高,但是速度越慢;这里选择Optimal Resolution;7 选择辐射定标Radiometric Calibration8 其他可选项:辐射归一化Radometric,局部入射角校正Local Incidence Angle、叠掩/阴影处理Layover/Shadow9 单击Start 执行;五、镶嵌工具在Toolbox中启动/Mosaicking/Seamless Mosaic;1)点击左上的加号如图添加需要镶嵌的影像数据;2添加进来之后可以看到数据的位置和重叠关系和影像轮廓线;3勾选Show Preview,可以预览镶嵌效果;在Data Ignore Value一览输入透明值,这里输入0;如下图为0值透明的效果;匀色处理:提供的匀色方法是直方图匹配;1在Corlor Correction选项中,勾选Histogram Matching:•∙Overlap Area Only:重叠区直方图匹配•∙Entire Scene:整景影像直方图匹配2在Main选项中,Color Matching Action中右键设置参考Reference和校正Adjust,根据预览效果确定参考图像;接边线与羽化接边线包括自动和手动绘制两种方法,也可以结合起来使用;1 选择下拉菜单Seamlines->Auto Generate Seamlines,自动绘制接边线,如下图所示,自动裁剪掉TM边缘“锯齿”;2 自动生成的接边线比较规整,可以明显看到由于颜色不同而显露的接边线;下拉菜单Seamlines-> Start editing seamlines,编辑接边线,可以在接边处绘制多边形,之后自动将绘制的多边形作为新的接边线;输出结果切换到Export选项,这是输出文件名、路径、格式、波段、背景值、重采样方法等信息;六、融合不同传感器图像融合下面以SPOT4 的10米全色波段和Landsat5 TM 30m多光谱的融合操作为例,学习图像融合操作流程,数据存放在"…\08.图像融合\数据\TM与spot"中;1选择File > Open,将SPOT4数据和Landsat TM数据分别打开;2在Toolbox中,打开 / Image Sharpening /Gram-Schmidt Pan Sharpening,在文件选择框中分别选择作为低分辨率影像Low Spatial和作为高分辨率影像High Spatial,单击OK;打开Pan Sharpening Parameters面板;3在Pan Sharpening Parameters面板中,选择传感器类型Sensor:Unknown,重采样方法Resampling:Cubic Convolution,输出格式为:ENVI;4选择输出路径及文件名,单击OK执行融合处理;注:进度条显示在右下角;相同传感器图像融合对于高分辨率影像,同样可以Gram-Schmidt Pansharping融合方法达到更好的效果,下面以QuickBird影像为例介绍这种融合方法,数据存放在"…\08.图像融合\数据\Quikbird"中;中,pansharping融合方法就是Gram-Schmidt Pan Sharpening选项;1File > Open,打开影像文件和;2在Toolbox中,打开 / Image Sharpening /Gram-Schmidt Pan Sharpening,在文件选择框中分别选择作为低分辨率影像Low Spatial和作为高分辨率影像High Spatial,单击OK;打开Pan Sharpening Parameters面板;3在Pan Sharpening Parameters面板中,选择传感器类型Sensor:QuickBird,重采样方法Resampling:Cubic Convolution,输出格式为:ENVI;注:传感器类型Sensor中还包括:GeoEye-1、Goktruk-2、IKONOS、landsat8_oli、landsat8_tirs、NPP VIIRS、Pleiades-1A/B、QuickBird、UI:GSS:Sensorrasat、Spot-6、Landsat ETM、WordlView-1/2;4选择输出路径及文件名,单击OK执行融合处理;注:进度条显示在右下角;七、裁剪规则图像裁剪规则裁剪,是指裁剪图像的边界范围是一个矩形,这个矩形范围获取途径包括:行列号、左上角和右下角两点坐标、图像文件、ROI/矢量文件;规则分幅裁剪功能在很多的处理处理过程中都可以启动Spatial Subset;下面介绍其中一种规则分幅裁剪过程;以TM影像为例,图像存放在"…\10.图像裁剪\数据"中;1File > Open打开图像,按Linear2%拉伸显示;2File > Save As,进入File Selection面板,选择Spatial Subset选项,打开右侧裁剪区域选择功能;有多种方法确定裁剪区域:使用当前可视区域确定裁剪区域:单击Use View Extent,自动读取主窗口中显示的区域;通过文件确定裁剪区域:可以选择一个矢量或者栅格等外部文件,自动读取外部文件的区域;点击右下角Subset By File,单击Open file 按钮,选择矢量数据"矢量.shp"作为裁剪范围手动交互确定裁剪区域:可以通过输入行列数Columns和Rows确定裁剪尺寸,按住鼠标左键拖动图像中的红色矩形框来移动以行列数确定的裁剪区域;也可以直接用鼠标左键按钮红色边框拖动来确定裁剪尺寸以及位置4可以看到裁剪区域信息,左侧Spectral Subset按钮还可以选择输出波段子集,这里默认不修改,单击OK;5选择输出路径及文件名,单击OK,完成规则图像裁剪过程不规则图像裁剪1打开图像,按Linear2%拉伸显示;2在Layer Manager中选中文件,单击鼠标右键,选择New Region Of Interest,打开Region of interest ROI Tool面板;3在Region of interest ROI Tool面板中点击按钮,在图像上绘制多边形,绘制大致为北京老皇城二环范围内的多边形,作为裁剪区域;可以修改感兴趣区名称ROIName、感兴趣区颜色ROI Color等,也可以根据需求绘制若干个多边形,当绘制多个感兴趣区时利用可以进行删减4在Region of interest ROI Tool面板中,选择File-> Save as,保存绘制的多边形ROI,选择保存的路径和文件名;5在Toolbox中,打开Regions of Interest/ Subset Data from ROIs;6在Select Input File对话框中,选择,打开Subset Data from ROIs Parameters面板;7在Subset Data from ROIs Parameters面板中,设置以下参数:Select Input ROIs:选择刚才生成的矢量文件roi1Mask pixels output of ROI: YesMask Background Value背景值:08选择输出路径和文件名,单击OK执行图像裁剪;。
基于ENVI的极化SAR数据处理流程介绍
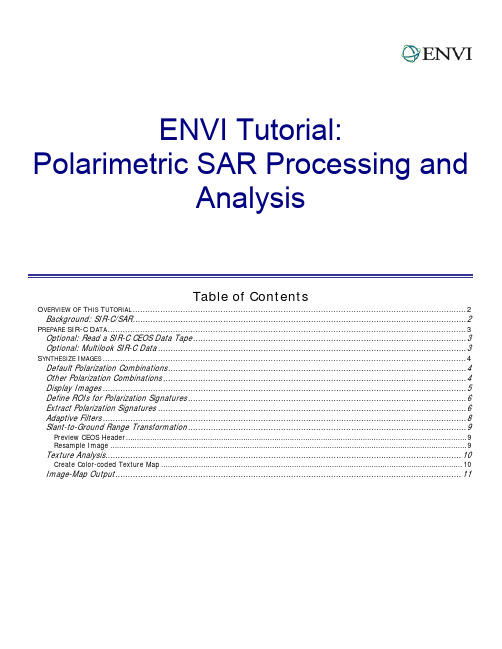
ENVI Tutorial: Polarimetric SAR Processing andAnalysisTable of ContentsO VERVIEW OF T HIS T UTORIAL (2)Background: SIR-C/SAR (2)P REPARE SIR-C D ATA (3)Optional: Read a SIR-C CEOS Data Tape (3)Optional: Multilook SIR-C Data (3)S YNTHESIZE I MAGES (4)Default Polarization Combinations (4)Other Polarization Combinations (4)Display Images (5)Define ROIs for Polarization Signatures (6)Extract Polarization Signatures (6)Adaptive Filters (8)Slant-to-Ground Range Transformation (9)Preview CEOS Header (9)Resample Image (9)Texture Analysis (10)Create Color-coded Texture Map (10)Image-Map Output (11)Overview of This TutorialThis tutorial demonstrates the use of ENVI’s tools for analyzing polarimetric synthetic aperture radar (SAR) data. You will multilook Spaceborne Imaging Radar-C (SIR-C) from Death Valley, California; synthesize images, define ROIs for (and extract) polarization signatures, use adaptive filters, perform slant-to-ground range transformation, use texture analysis, and create an output image-map.Files Used in This TutorialENVI Tutorial Data DVD: envidata\ndv_sircFile Descriptionndv_l.cdp L-band SIR-C subset in ENVI compressed data product (.cdp) formatpol_sig.roi Region of interest (ROI) fileBackground: SIR-C/SARSIR-C is a polarimetric SAR instrument that uses two microwave wavelengths: L-band (24 cm) and C-band (6 cm). The SIR-C radar system was flown as a science experiment on the Space Shuttle Endeavor in April (SRL-1) and October 1994 (SRL-2), collecting high-quality SAR data over many sites around the world. (A second radar system, XSAR, was also flown on this mission, but these data are neither discussed nor processed here.) Additional information about SIR-C is available on the NASA/JPL Imaging Radar Home Page at /.Prepare SIR-C DataThe data used in this tutorial are a subset of L-band Single Look Complex (SLC) SIR-C data that cover the northern part of Death Valley, including Stovepipe Wells, a site of active sand dunes and extensive alluvial fans at the base of mountains. These data were preprocessed by reading and subsetting from tape and multilooking (averaging) to 13 m square pixels. The data are provided in ENVI compressed data product (.cdp) format. This non-image format is similar to the tape format and cannot be viewed until images are synthesized for specific polarizations.The first two functions described in this example—reading the data tape and multilooking—were already applied to the SIR-C data. The steps are provided here only for completeness if you want to learn more about the processes. Skip to the Synthesizing Images section if you are not interested in data preparation.Optional: Read a SIR-C CEOS Data Tape1.From the ENVI main menu bar, select File→Tape Utilities→Read Known Tape Formats→SIR-C CEOS.The SIRC Format - Load Tape dialog appears.2.Enter the tape device name and accept the default record size of 65,536. Click OK. The tape is scanned todetermine what SIR-C files it contains. A dialog appears to let you select the desired datasets. By default, ENVI reads all of the data files on the tape.3.If you do not want to read all of the data files, click Clear, then select the check box next to each desired file.Click OK.4.You can independently subset and multilook the selected data files as they are being read from tape. However,you should perform multilooking on disk (unless you have insufficient disk space) as this function is extremely slow from tape.5.Select a filename, then click Spatial Subset or Multi-Look to enter parameters for the data file. Enter anoutput filename. Each input file must have an output filename. By convention, the output filenames should take the form filename_c.cdp and filename_l.cdp for the C- and L-bands, respectively. The SIR-C data areread from the tape, and one compressed scattering matrix output file is created for selected each dataset.Optional: Multilook SIR-C DataMultilooking is a method for reducing speckle noise in SAR data and for changing the size of a SAR file. You can multilook SIR-C data to a specified number of looks, number of lines and samples, or azimuth and range resolutions. The SIR-C file used in this tutorial was a single-look dataset with a range resolution of 13 m and an azimuth size of 5 m. Multilooking has already been performed in the azimuth direction to make 13 m square pixel sizes. Instructions on multilooking are included here only for completeness.1.From the ENVI main menu bar, select Radar→Polarimetric Tools→Multilook Compressed Data→SIR-C Multilook. An Input Data Product Files dialog appears.2.Click Open File and select an input file. ENVI detects whether the file contains L- or C- band data and displaysthe filename in the appropriate field of the dialog. Click OK.3.Select the file to multilook by selecting the check box next to the name. You can select multiple files.4.Enter any one of three values—number of looks, number of pixels, or pixel size—and the other two are calculatedautomatically. Integer and floating-point number of looks are supported.5.Enter the desired Samples (range) and Lines (azimuth) values.6.Enter a base filename and click OK.Synthesize ImagesThe SIR-C quad-polarization data provided with this tutorial and available on tape from JPL are in a non-image, compressed format. Accordingly, images of the SIR-C data must be mathematically synthesized from the compressed scattering matrix data. You can synthesize images with any transmit and receive polarization combinations you want.1.From the ENVI main menu bar, select Radar→Polarimetric Tools→Synthesize SIR-C Data. An InputProduct Data Files dialog appears.2.Click Open File. A file selection dialog appears.3.Navigate to envidata\ndv_sirc and select ndv_l.cdp. Click Open. When the filename appears in theSelected Files L: field, click OK. The Synthesize Parameters dialog appears.Default Polarization CombinationsFour standard transmit/receive polarization combinations—HH, VV, HV, and TP—are listed in the Select Bands to Synthesize list of the Synthesize Parameters dialog. By default, all of these bands are selected to be synthesized.1.Enter ndv_l.syn in the Enter Output Filename field.2.Click the Output Data Type drop-down list and select Byte. This scales the output data to byte values. (If youwill be performing quantitative analysis, the output should remain in floating-point format.) Click OK. Afterprocessing is complete, four bands corresponding to the four polarization combinations are added to the Available Bands List.Other Polarization CombinationsThe transmit and receive ellipticity and orientation angles determine the polarization of the radar wave used to synthesize an image. The ellipticity angle falls between -45 and 45 degrees and determines the “fatness” of the ellipse. The orientation angle is measured with respect to horizontal and ranges from 0 to 180 degrees. You can synthesize images of non-default polarization combinations by entering the desired parameters as follows.1.From the ENVI main menu bar, select Radar→Polarimetric Tools→Synthesize SIR-C Data. The filendv_l.cdp should still appear in the Selected Files field. Click OK. The Synthesize Parameters dialog appears.2.Enter -45 in both the Transmit Ellip and Receive Ellip fields and 135 in the Transmit Orien and ReceiveOrien fields.3.Click Add Combination. This will produce a right-hand circular polarization image.4.Enter 0 in both the Transmit Ellip and Receive Ellip fields and 30 in the Transmit Orien and Receive Orienfields.5.Click Add Combination. This will produce a linear polarization with an orientation angle of 30 degrees.6.Click Clear under the list of polarization combinations to turn off synthesis of the standard polarization bands,which have already been generated.7.Select the Yes radio button for Output in dB? This will produce images that are in decibels with values typicallybetween –50 and 0.8.In the Enter Output Filename field, enter ndv_l2.syn and click OK. After processing is complete, two bandscorresponding to the polarization combinations are added to the Available Bands List.Display Images1.In the Available Bands List, select [L-TP] under ndv_l.syn and click Load Band. The SIR-C, L-band, total-power image appears in a new display group.2.From the Display group menu bar, select Enhance→Interactive Stretching. A histogram plot windowappears, which shows the current stretch (between the vertical dotted lines on the input histogram) and thecorresponding DN values in the text fields.3.Drag the dotted vertical lines to change the stretch, or enter the desired DN values into the appropriate fields.4.Enter 5 in the left Stretch field and 95 in the right field.5.From the histogram menu bar, select Stretch Type→Gaussian. Click Apply. A Gaussian stretch is appliedwith a 5% low and high cutoff.6.Generate and compare linear and square-root stretches.7.To display a color composite, select the RGB Color radio button in the Available Bands List. Select[L-HH], [L-VV], and [L-HV] in sequential order under ndv_l.syn.8.Click Display #1 and select New Display. Click Load RGB to display the HH band in red, VV in green, and HVin blue. The color variations in the images are caused by variations in the radar reflectivity of the surfaces. The bright areas in the sand dunes are caused by scattering of the radar waves by vegetation (mesquite bushes). The alluvial fans show variations in surface texture due to age and composition of the rock materials.9.Adjust the stretch as desired (Gaussian and square-root stretches work well on all three bands).10.Close the histogram plot window and Display #2 when you are finished. Keep Display #1 open for later exercises.Define ROIs for Polarization SignaturesYou can extract polarization signatures from a SIR-C compressed scattering matrix for a region of interest (ROI) or a single pixel in a polarimetric radar image. Define ROIs by selecting pixels or by drawing lines or polygons within an image.1.From the Display group menu bar, select Overlay→Region of Interest. An ROI Tool dialog appears.2.Four ROIs were previously defined and saved for use in extracting polarization signatures for this tutorial. Fromthe ROI Tool dialog menu bar, select File→Restore ROIs. A file selection dialog appears.3.Select pol_sig.roi. A dialog box appears, stating that the regions were restored. Click OK.4.Regions named veg, fan, sand, and desert pvt appear in the table in the ROI Tool and are drawn in the displaygroup.5.To draw your own ROI, select ROI_Type→Polygon, Polyline, or Point from the ROI Tool menu bar.6.Click New Region, enter a name, and choose a color.Draw polygons by clicking the left mouse button in the display group to select the endpoints of linesegments, or by holding down the left mouse button and moving the cursor for continuous drawing. Clickthe right mouse button once to close the polygon and a second time to accept the polygon.Draw polylines in the same manner as polygons. Click the left mouse button to define the line endpointsand click the right button to end the polyline and a second time to accept the polyline.Point mode is used to select individual pixels. Click the left mouse button to add the pixel currently underthe cursor to the ROI.You can select multiple polygons, lines, and pixels for each ROI.7.Repeat Step 6 to draw a second ROI. You can save the ROIs to a file and restore them later by selecting File →Save ROI from the ROI Tool dialog menu bar.Extract Polarization SignaturesPolarization signatures are 3D representations of the complete radar scattering characteristics of the surface for a pixel or average of pixels. They show the backscatter response at all combinations of transmit and receive polarizations and are represented as either co-polarized or cross-polarized. Co-polarized signatures have the same transmit and receive polarizations. Cross-polarized signatures have orthogonal transmit and receive polarizations. Polarization signatures are extracted from the compressed scattering matrix data using the ROIs for pixel locations. Polarization signatures are displayed in viewer dialogs, as shown on the next page. To extract your own polarization signatures, perform the following steps.1.From the ENVI main menu bar, select Radar→Polarimetric Tools→Extract Polarization Signatures→SIR-C. The filename ndv_l.cdp should appear in the Input Data Product Files dialog. If not, click Open File and select this file. Click OK. The Polsig Parameters dialog appears.2.Select the four ROIs (veg, fan, sand, and desert pvt) by clicking Select All Items.3.Select the Memory radio button and click OK. Four Polarization Signature Viewer dialogs appear, one for eachROI. The polarization signatures are displayed as 3D wire mesh surface plots and as 2D gray scale images. The X and Y axes represent ellipticity and orientation angles, respectively. You can selectively plot the vertical axis as intensity, normalized intensity, or dB by selecting Polsig_Data from the Polarization Signature Viewer dialog menu bar.4.Polarization signature statistics appear at the bottom of each Polarization Signature Viewer dialog. Notice therange of intensity values for the different surfaces. The smoother surfaces (sand and desert pvt) have low Z values. The rough surfaces (fan and veg) have higher Z values. The minimum intensity indicates the pedestal height of the polarization signature. The rougher surfaces have more multiple scattering and therefore higher pedestal heights than the smoother surfaces. The shape of the signature also indicates the scatteringcharacteristics. Signatures with a peak in the middle show a Bragg-type (resonance) scattering mechanism.5.In any given Polarization Signature Viewer dialog, change the Z-axis by selecting Polsig_Data→Normalizedfrom the Polarization Signature Viewer dialog menu bar. This normalizes the signature by dividing by itsmaximum; the signature is plotted between 0 and 1. This representation shows the difference in pedestal heights and shapes better, but it removes the absolute intensity differences.Alternately, select Polsig_Data → Co-Pol and Cross-Pol to toggle between co-polarized and cross-polarized signatures.e the left mouse button to drag a 2D cursor on the polarization signature image on the right side of the plot.Note the corresponding 3D cursor in the polarization plot.7.Click-and-drag any axis to rotate the polarization signature.8.You can optionally output the signatures to a file or printer by selecting File→Save Plot As or File→Printfrom the Polarization Signature Viewer dialog menu bar.9.Close the Polarization Signature Viewer and ROI Tool dialogs when you are finished.Adaptive FiltersAdaptive filters are used to reduce the speckle noise in a radar image while preserving the texture information. Statistics are calculated for each kernel and used as input into the filter, allowing the filter to adapt to different textures within the image.1.From the ENVI main menu bar, select Radar→Adaptive Filters→Gamma. A Gamma Filter Input File dialogappears with a list of open files. You can apply a filter to an entire file or to an individual band.2.In the Gamma Filter Input File dialog, click the Select by toggle button to choose Band.3.Select [L-HH] under ndv_l.syn and click OK. The Gamma Filter Parameters dialog appears.4.Accept the default values, and select the Memory radio button. Click OK.5.In the Available Bands List, click Display #1 and select New Display. Select the Gray Scale radio button,select the new band name (Gamma), and click Load Band.6.From the Display group menu bar, select Enhance→[Image] Square Root.7.In the Available Bands List, click Display #2 and select Display #1. Select [L-HH] under ndv_l.syn, andclick Load Band.8.From the Display #1 menu bar, select Enhance→[Image] Square Root.9.From any Display group menu bar, select Tools→Link→ Link Displays. The Link Displays dialog appears.Click OK to link the gamma-filtered L-HH image (Display #2) with the original L-HH image (Display #1).10.Click in an Image window to toggle between the two images, using the dynamic overlay feature. The figure belowshows a portion of the original image (left) and the gamma-filtered image (right).11.Close Display #2 when you are finished. Leave Display #1 (ndv_l.syn) open for the next exercise.Slant-to-Ground Range TransformationA radar system looks to the side and records the locations of objects using the distance from the sensor to the object along the line of sight, rather than along the surface. An image collected using this geometry is referred to as a slant range image. Slant range radar data have a systematic geometric distortion in the range direction. The true, or ground range, pixel sizes vary across the range direction because of the changing incident angles. This makes the image appear compressed in the near range, relative to what it would look like if all of the pixels covered the same area on the ground. Slant-to-ground range correction for SIR-C is performed on synthesized images. In other words, the correction is not performed on the entire SIR-C compressed data product file. However, this file does store the required information in the CEOS header about the sensor orientation.Preview CEOS Header1.From the ENVI main menu bar, select Radar→Open/Prepare Radar File→View Generic CEOS Header. Afile selection dialog appears. You must select the original unsynthesized data file from which to extract thenecessary information.2.Select ndv_l.cdp and click Open. A CEOS Header Report dialog appears. Scroll down and note that the linespacing (azimuth direction) is 5.2 m, while the pixel spacing (slant range direction) is 13.32 m. Close the CEOS Header Report dialog when you are finished reviewing it.Next, you will use the Slant-to-Ground-Range function to resample the image to square 13.32 m pixels, thusremoving slant range geometric distortion.Resample Image3.From the ENVI main menu bar, select Radar→Slant to Ground Range→SIR-C. A file selection dialogappears.4.Select ndv_l.cdp and click Open. The Slant Range Correction Input File dialog appears.5.Select ndv_l.syn and click OK. The Slant to Ground Range Correction Dialog appears. ENVI automaticallypopulates the Instrument height (km), Near range distance (km), and Slant range pixel size (m) fields withinformation from the CEOS header.6.Enter 13.32 in the Output pixel size (m) field to generate square ground-range pixels.7.From the Resampling Method drop-down list, select Bilinear.8.In the Enter Output Filename field, enter ndv_gr.img. Click OK. The input image is resampled to square13.32 m pixels. Four new bands appear in the Available Bands List. Band 1 of the resampled image correspondsto the L-HH band of the original, slant-range image (ndv_l.syn), Band 2 corresponds to L-VV, etc.9.In the Available Bands List, click Display #1 and select New Display.10.Select a band from the resampled image and click Load Band. The resampled image appears in Display #2.Make sure Display #1 (ndv_l.syn) shows the corresponding polarization band.pare the two images.12.When you are finished comparing images, close Display #2. Keep Display #1 (ndv_l.syn) open for the nextexercise.Texture AnalysisTexture is a measure of the spatial variation in the gray levels in the image, as a function of scale. ENVI calculates texture based on a processing window size you specify. The texture measures demonstrated in this tutorial are Occurrence Measures, including data range, mean, variance, entropy, and skewness. These terms are explained in ENVI Help. Texture is best calculated for radar data with no resampling or filtering applied.1.From the ENVI main menu bar, select Radar→Texture Filters→Occurrence Measures. A Texture InputFile dialog appears.2.Click the Select By toggle button to choose Band. Select [L-HH] under ndv_l.syn and click OK. An OccurrenceTexture Parameters dialog appears.3.Deselect all of the Textures to Compute options except for Data Range.4.Set the Processing Window: Rows and Cols to 7 and 7.5.In the Enter Output Filename field, enter ndv_hh.tex and click OK.Create Color-coded Texture Map6.In the Available Bands List, click Display #1 and select New Display.7.Select Data Range under ndv_hh.tex and click Load Band.8.From the Display #2 menu bar, select Enhance→[Image] Square Root.9.From any Display group menu bar, select Tools→Link→ Link Displays. The Link Displays dialog appears.Click OK to link the original image (Display #1) with the colored texture image (Display #2).10.Click in an Image window to toggle between the two images.11.Double-click inside an Image window to display the Cursor Location/Value tool. Examine the data values in thetextured image, and compare these to the original image.12.From the Display #2 menu bar, select Tools→Color Mapping→Density Slice. A Density Slice Band Choicedialog appears.13.Select the Data Range band and click OK. A Density Slice dialog appears.14.Accept the default ranges by clicking Apply.15.Try creating your own density-sliced image and view the results.16.Keep Display #2 open for the next exercise.Image-Map OutputIn this exercise, you will create a map of your color-coded textured image and add a border and map key.1.From the Display #2 menu bar, select Overlay→Annotation. An Annotation dialog appears.2.From the Annotation dialog menu bar, select Options→Set Display Borders.3.In the Display Borders dialog, enter 100 in the upper field, and leave the remaining fields 0.4.Click Border Color and select Items 1:20→White. Click OK. This adds a 100-pixel white border at the top ofthe image.5.Move the Image box in the Scroll window to the top of the image containing the border.6.Enter a map title in the empty field in the Annotation dialog. Set the Size value to 16. Click the Color box onceto select black.7.Click in the Image window to show the map title, then move it inside the white border to the far left. Right-clickto lock the map title in place. You can place multiple text items on the image in this manner, and you can change their font size, type, color, and thickness as desired.8.From the Annotation dialog menu bar, select Object→Color Ramp.9.Enter Min and Max values of 0 and 255 respectively, set Inc to 4, and set the font Size to 14 to annotate thecolor ramp.10.Click in the Image window to show the map key, move it inside the white border to the far right, then right-clickto lock it in place. The following figure shows a sample map; your results may be different.11.Save the image to a PostScript file by selecting File→Save Image As→Postscript File from the Display #2menu bar. An Output Display to PostScript File dialog appears.12.Leave the default values, and enter an output filename or accept the default name of ndv_hh.ps. Click OK.Or, output the map directly to your printer by selecting File → Print from the Display #2 menu bar.13.When you are finished, select File→Exit from the ENVI main menu bar.。
TM与SAR图像融合处理及在高原区调中的应用
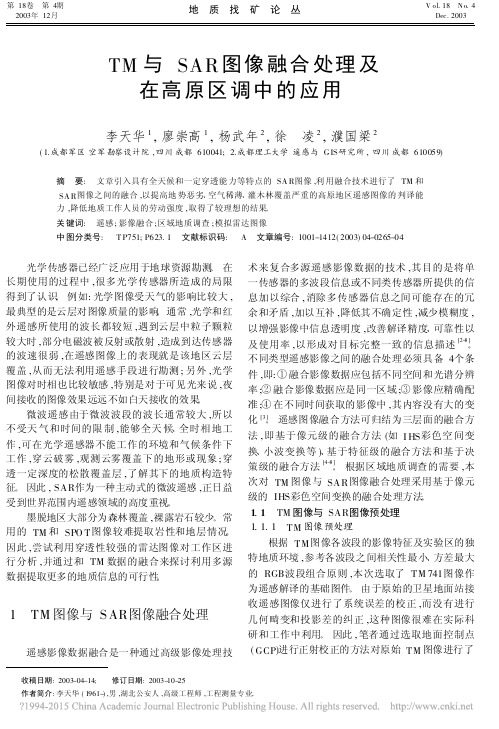
TM 与SAR 图像融合处理及在高原区调中的应用李天华1,廖崇高1,杨武年2,徐 凌2,濮国梁2(1.成都军区空军勘察设计院,四川成都610041; 2.成都理工大学遥感与G IS 研究所,四川成都610059)摘 要: 文章引入具有全天候和一定穿透能力等特点的SA R 图像,利用融合技术进行了TM 和SA R 图像之间的融合,以提高地势恶劣、空气稀薄、灌木林覆盖严重的高原地区遥感图像的判译能力,降低地质工作人员的劳动强度,取得了较理想的结果。
关键词: 遥感;影像融合;区域地质调查;模拟雷达图像中图分类号: T P751;P623.1 文献标识码: A 文章编号:1001-1412(2003)04-0265-04 光学传感器已经广泛应用于地球资源勘测。
在长期使用的过程中,很多光学传感器所造成的局限得到了认识。
例如:光学图像受天气的影响比较大,最典型的是云层对图像质量的影响。
通常,光学和红外遥感所使用的波长都较短,遇到云层中粒子颗粒较大时,部分电磁波被反射或散射,造成到达传感器的波速很弱,在遥感图像上的表现就是该地区云层覆盖,从而无法利用遥感手段进行勘测;另外,光学图像对时相也比较敏感,特别是对于可见光来说,夜间接收的图像效果远远不如白天接收的效果。
微波遥感由于微波波段的波长通常较大,所以不受天气和时间的限制,能够全天候、全时相地工作,可在光学遥感器不能工作的环境和气候条件下工作,穿云破雾,观测云雾覆盖下的地形或现象;穿透一定深度的松散覆盖层,了解其下的地质构造特征。
因此,SAR 作为一种主动式的微波遥感,正日益受到世界范围内遥感领域的高度重视。
墨脱地区大部分为森林覆盖,裸露岩石较少。
常用的TM 和SPO T 图像较难提取岩性和地层情况。
因此,尝试利用穿透性较强的雷达图像对工作区进行分析,并通过和TM 数据的融合来探讨利用多源数据提取更多的地质信息的可行性。
1 TM 图像与S AR 图像融合处理遥感影像数据融合是一种通过高级影像处理技术来复合多源遥感影像数据的技术,其目的是将单一传感器的多波段信息或不同类传感器所提供的信息加以综合,消除多传感器信息之间可能存在的冗余和矛盾,加以互补,降低其不确定性,减少模糊度,以增强影像中信息透明度,改善解译精度、可靠性以及使用率,以形成对目标完整一致的信息描述[2-8]。
利用envi进行tm影像监督分类详细操作步骤
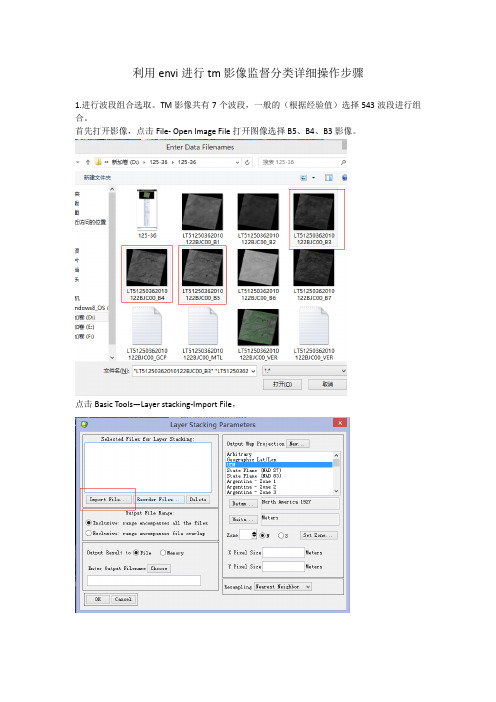
利用envi进行tm影像监督分类详细操作步骤1.进行波段组合选取。
TM影像共有7个波段,一般的(根据经验值)选择543波段进行组合。
首先打开影像,点击File- Open Image File打开图像选择B5、B4、B3影像。
点击Basic Tools—Layer stacking-Import File,分别双击B5、B4、B3,然后点击Choose选择合成影像的路径并进行命名。
在ABL里,对应RGB选择合成影像的B5、B4、B3波段,点击Load RGB,显示影像2、选择样本训练区点击Basic Tools-Region Of Interest-ROI Tool点击New Region确定分类样本,水体、居民地、道路、耕地、森林,为了选择精确选择Zoom 窗口进行选择。
选择水体后,开始在zoom里选样本,点击右键结束,可在不同区域的水体上选择样本选择合适水体样本后,则依次选取居民地等地物的样本3、验证样本样本选择完成之后,需要对样本从定量和定性上分别进行评价。
(1)定量Options-Compute ROI Separability-选择合成影像-ok点击select all items-ok该工具来计算任意类别间的统计距离,一般的,参数的值在0~2.0之间,大于1.9说明样本之间可分离性好;小于1.8大于1.4属于合格样本;小于1.4需要重新选择样本;小于1考虑将两类样本合成一类样本。
多次试验,道路不易单独提取,建议进行人工后期提取。
(2)定性点击File-Export ROIs to n-D Visualizer,选择影像点击ok-Select all items-ok在n-DContrs对话框点击1、2、3,变成白色之后点击start,在visualizer 会出现动态变化的样本聚类情况,如下图,看样本的集中度,越高代表样本越好。
3、进行监督分类点击Classification-Supervised-Maximum Likelihood(最大似然法),选择影像,点击ok选择要分的类,设置好输出路径。
基于ENVI的多源遥感影像数据融合
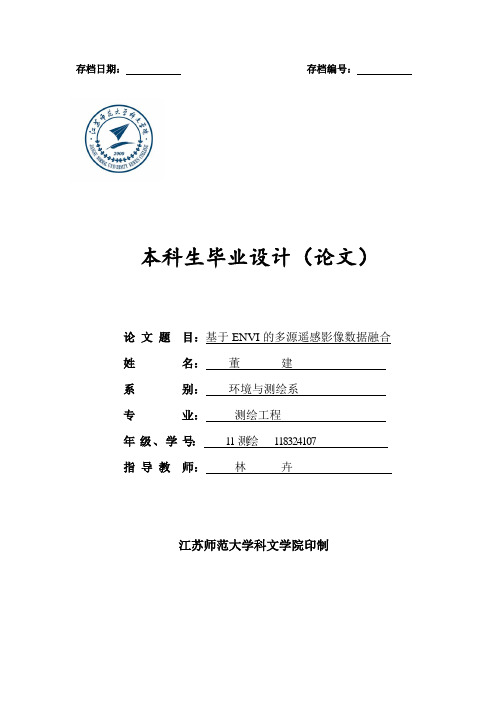
存档日期:存档编号:本科生毕业设计(论文)论文题目:基于ENVI的多源遥感影像数据融合*名:**系别:环境与测绘系专业:测绘工程年级、学号: 11 测绘 1***********师:**江苏师范大学科文学院印制摘要在世界遥感技术领域极速发展的情况下,对于用卫星传感器来观测和获取某一地域遥感影像数据的方法越来越多,此时多时相、多平台、高光谱和高分辨率卫星等影像数据大量涌现,并且在有关地学的多个领域都用到了这些影像数据。
多源遥感影像融合技术在处理怎么使各种有差别的的遥感影像既保存着各自重要的使用特点和对象,同时也会将其局限性缩至最小这方面是最有效的途径之一。
并且在不同的学科范畴都涉及到多源遥感影像数据融合技术,因此该技术被不断地完善与推行。
而当前在世界上对该技术还尚未形成一套完善的理论与方法,于是目前在遥感领域研究的一个重要的研究技术就是周密处理与剖析遥感信息。
本文基于ENVI对多源遥感影像数据融合的研究。
第一章介绍了遥感影像数据融合的基本理论知识和本论文内容研究的背景和意义,以及国内外目前对遥感图像融合技术的发展现状。
第二章是对数据融合三个层次(像素级融合、决策级融合和特征级融合)的对比介绍,像素级融合、决策级融合和特征级融合,另外还展示了每个层次数据处理流程图。
第三章对像素级融合中加权融合法变换、Brovery 变换、IHS变换和PCA变换等融合方法进行了分析比较。
第四章介绍了融合的评价指标(主观评价、客观评价)。
第五章通过对Quickbird多光谱影像的4、3、2波段和Quickbird全色影像的1波段数据的进行融合实验,对实验结果进行分析,总结出这四种融合方法的特点和适用范围,得到更准确,更可靠、更安全的估计和判断,为相关工作提供帮助。
关键词:数据融合;ENVI;IHS变换;PCA变换;评价指标;多源遥感影像;AbstractFast development in the field of remote sensing technology in the world, with satellite sensors to measure and obtain a regional method of remote sensing image data is becoming more and more this time multi temporal, multi platform, hyperspectral and high-resolution satellite , and in the study of the image data used in these areas. Multi-source remote sensing image fusion technology in dealing with how to make a variety of discriminating the use of remote sensing image is kept their important characteristics and objects, and at the same time its limitations will be shrunk to a minimum is one of the most effective way. And in different disciplines category involves multi-source remote sensing image data fusion technology, thus being constantly perfected and the implementation of the technology. And the current in the world and also with the technology has not yet formed a perfect theory and method, so the current research in the field of remote sensing is an important research techniques careful processing and analysis of remote sensing information.This article is based on ENVI of multi-source remote sensing image data fusion research. The first chapter introduces the basic theoretical knowledge of remote sensing image data fusion and the content of this thesis research background and significance, as well as the present situation on the development of remote sensing image fusion technology at home and abroad. Second chapter on three levels of data fusion (pixel level fusion and decision level fusion and feature level fusion) contrast, pixel level fusion and decision level fusion and feature level fusion, it also shows the data processing flow chart of each level. The third chapter of weighted fusion method in pixel level fusion transformation, Brovery transformation, IHS transform and PCA transform fusion method are analyzed and compared. The fourth chapter of the fusion evaluation (subjective evaluation and objective evaluation). Fifth chapter through to the Quickbird multispectral image of 4, 3, 2 band and Quickbird panchromatic image 1 band data fusion experiments, analysis of the experimental results, summarized the characteristics of the four fusion method and the applicable scope, get more accurate, more reliable and safer estimates and judgment, to offer help for related work.Key words:Data Fusion;ENVI;IHS transform;PCA transform;Evaluation;Multi-source remote sensing image目录摘要 (Ⅰ)Abstract (Ⅱ)1 绪论 (1)1.1 概述 (1)1.2 选题研究的背景与意义 (1)1.3 国内外研究现状 (2)1.3.1 国外研究现状 (2)1.3.2国内研究现状 (3)1.4论文结构 (4)2 多源遥感影像数据融合 (5)2.1 层次分类 (5)2.2 像素级融合 (5)2.3特征级融合 (5)2.4 决策级融合 (6)3 影像融合的常用方法 (10)3.1 融合常用方法分类 (10)3.2 加权融合法 (11)3.3 Brovery 变换法 (11)3.4 IHS 变换 (11)3.5 PCA变换 (13)3.6方法比较 (14)4 融合影像质量评价 (15)4.1 主观评价 (16)4.2 客观评价 (17)4.2.1 均值 (18)4.2.2 标准差 (18)4.2.3 信息熵 (18)4.2.4 平均梯度 (18)4.2.5 相关系数 (18)5 融合实验数据分析 (20)6 总结与展望 (25)6.1总结 (25)6.2展望 (25)7致谢 (27)1 绪论1.1 概述在二十世纪七十年代美国最早提出了数据融合(Data Fusion)的概念,然而那时人们并没有对其足够重视,直到进入80 年代以后在军事中广泛应用直接促使其快速发展[1]。
envi图像融合
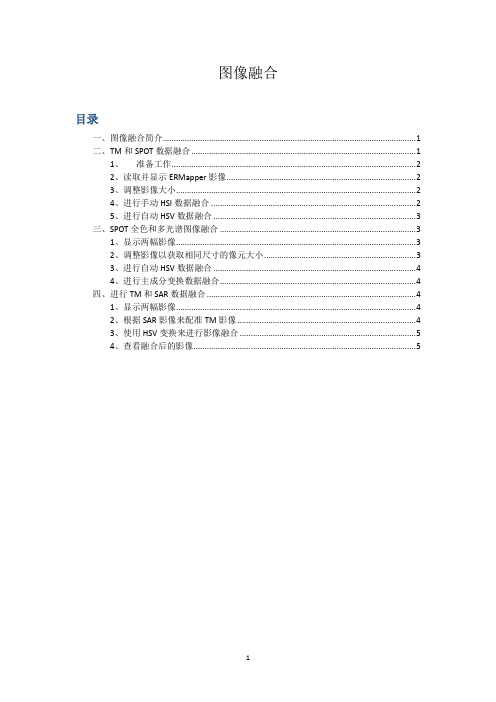
图像融合目录一、图像融合简介 (1)二、TM和SPOT数据融合 (1)1、准备工作 (2)2、读取并显示ERMapper影像 (2)3、调整影像大小 (2)4、进行手动HSI数据融合 (2)5、进行自动HSV数据融合 (3)三、SPOT全色和多光谱图像融合 (3)1、显示两幅影像 (3)2、调整影像以获取相同尺寸的像元大小 (3)3、进行自动HSV数据融合 (4)4、进行主成分变换数据融合 (4)四、进行TM和SAR数据融合 (4)1、显示两幅影像 (4)2、根据SAR影像来配准TM影像 (4)3、使用HSV变换来进行影像融合 (5)4、查看融合后的影像 (5)一、图像融合简介数据融合是将多幅影像组合到单一合成影像的处理过程。
一般使用高空间分辨率的全色影像或单一波段的雷达影像来增强多光谱影像的空间分辨率。
图像融合的关键是融合前两幅图像的精确配准以及处理过程中融合方法的选择。
●只有将两幅融合图像进行精确配准,才可能得到满意的结果。
●对于融合方法的选择,取决于被融合图像的特征以及融合目的。
ENVI中提供的融合方法有:HSV变换、Brovey变换、乘积运算(CN)、主成分(PC)变换、Gram-Schmidt Pan Sharpening(GS)。
表1 融合方法说明二、TM和SPOT数据融合数据一:lon_spot :London SPOT数据lon_spot.ers :ER Mapper的头文件lon_tm :London Landsat TM数据lon_tm.ers :ER Mapper的头文件1、准备工作为了在ENVI中进行数据融合,一般要求影像文件含有地理坐标(在这种情况下,空间重采样会自动进行),否则影像必须覆盖同一地理区域,并且有相同的像素大小、影像大小以及相同的方位。
由于London数据没有含有地理坐标,因而低空间分辨率的影像必须重采样,使其和高分辨率的影像有相同的像素大小(使得两幅影像的像素一一对应)。
《ENVI卫星影像处理全流程》

Landsat TM 影像处理完整流程ENVI(The Environment for Visualizing Images),由美国系统研究公司(Research System INC.)开发。
一. 界面系统介绍1. 主菜单:菜单项,File、Basic Tool、Classification、Tranform、Spectral实习所涉及的(粗略介绍)2. Help 工具的使用3. 主菜单设置(preferences):内存设置二. 文件的存取与显示1.图像显示由一组三个不同的图像窗口组成:主图像窗口、滚动窗口、缩放窗口。
1)主图像Image窗口:(400*400)100%显示(全分辨率显示)scroll的方框,可交互式分析、查询信息。
主图像窗口内的功能菜单:在主图像窗口内点击鼠标右键,切换隐藏子菜单的开启和关闭。
该"Functions" 菜单控制所有的ENVI交互显示功能,这包括:图像链接和动态覆盖;空间和波谱剖面图;对比度拉伸;彩色制图;诸如ROI的限定、光标位置和值、散点图和表面图等交互特征;诸如注记、网格、图像等值线和矢量层等的覆盖(叠置);动画以及显示特征。
2)滚动Scroll窗口:全局,重采样(降低分辨率)显示一幅图像。
只有要显示的图像比主图像窗口能显示的图象大时,才会出现滚动窗口。
滚动窗口位置和大小最初在envi.cfg 文件中被设置并且可以被修改。
3)缩放Zoom窗口:(200*200)显示image的方框。
缩放系数(用户自定义)出现在窗口标题栏的括号中。
2.图像的头文件资料的获取和编辑ENVI:File>>Edit ENVI Header,选择相应的文件。
从Header Info 对话框里,你可以点击Edit Attributes 下拉菜单中的选项,调用编辑特定文件头参数的独立对话框。
这些参数包括波段名、波长、地图信息等。
3.图像的存取File > Open Image File. 当你打开任何文件,可用波段列表(ABL)自动地出现。
ENVI图像融合
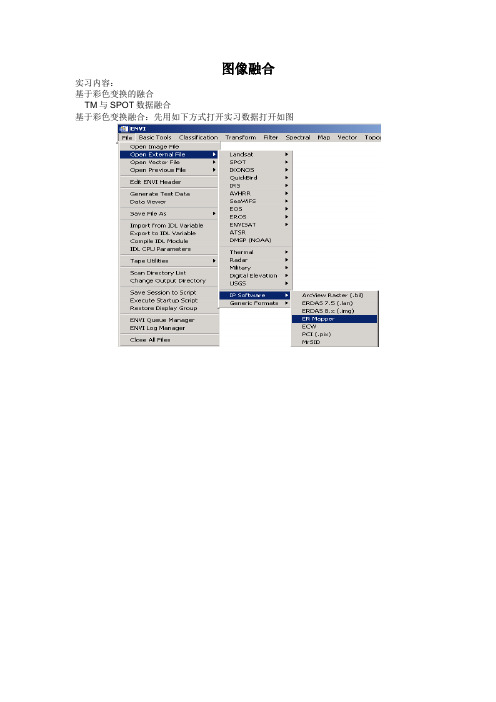
图像融合
实习内容:
基于彩色变换的融合
TM与SPOT数据融合
基于彩色变换融合:先用如下方式打开实习数据打开如图
要先对TM数据进行数据重采样
出现如下对话框,双击TM图像
点OK,出现如下: 保存
再做彩色正变化,
得到如
下的对话框,将重采样的数据对应的赋到RGB上,如上图,点OK.再保存.再做SPOT图的拉伸,先打开SPOT图象
点照片右键
得到
再
得到将Output Min输0,Output Max 输1,将Output Data Type改成 Floating Point,保存.再做彩色逆变换
将彩色变换H S两层赋给逆变
换,将拉伸赋到V层,点OK,保存即可
利用系统做彩色融合: 得到
如下的对话框如上图那样赋值点OK.
得到如下选择SPOT图点OK.。
ENVI对SAR数据的预处理过程详细版
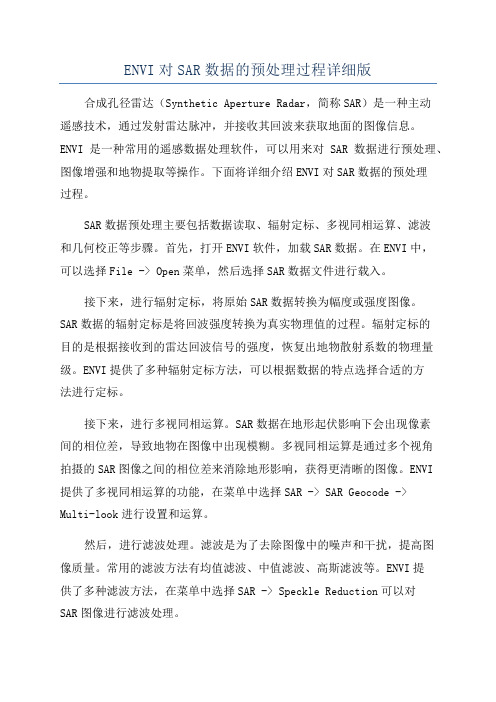
ENVI对SAR数据的预处理过程详细版合成孔径雷达(Synthetic Aperture Radar,简称SAR)是一种主动遥感技术,通过发射雷达脉冲,并接收其回波来获取地面的图像信息。
ENVI是一种常用的遥感数据处理软件,可以用来对SAR数据进行预处理、图像增强和地物提取等操作。
下面将详细介绍ENVI对SAR数据的预处理过程。
SAR数据预处理主要包括数据读取、辐射定标、多视同相运算、滤波和几何校正等步骤。
首先,打开ENVI软件,加载SAR数据。
在ENVI中,可以选择File -> Open菜单,然后选择SAR数据文件进行载入。
接下来,进行辐射定标,将原始SAR数据转换为幅度或强度图像。
SAR数据的辐射定标是将回波强度转换为真实物理值的过程。
辐射定标的目的是根据接收到的雷达回波信号的强度,恢复出地物散射系数的物理量级。
ENVI提供了多种辐射定标方法,可以根据数据的特点选择合适的方法进行定标。
接下来,进行多视同相运算。
SAR数据在地形起伏影响下会出现像素间的相位差,导致地物在图像中出现模糊。
多视同相运算是通过多个视角拍摄的SAR图像之间的相位差来消除地形影响,获得更清晰的图像。
ENVI提供了多视同相运算的功能,在菜单中选择SAR -> SAR Geocode ->Multi-look进行设置和运算。
然后,进行滤波处理。
滤波是为了去除图像中的噪声和干扰,提高图像质量。
常用的滤波方法有均值滤波、中值滤波、高斯滤波等。
ENVI提供了多种滤波方法,在菜单中选择SAR -> Speckle Reduction可以对SAR图像进行滤波处理。
最后,进行几何校正。
几何校正是将SAR图像与地理坐标系统对齐,使其与其他地理信息数据进行叠加和分析。
ENVI提供了几何校正的功能,在菜单中选择SAR -> SAR Geocode -> Geocoding进行设置和处理。
在进行SAR数据预处理时,还需要注意一些事项。
- 1、下载文档前请自行甄别文档内容的完整性,平台不提供额外的编辑、内容补充、找答案等附加服务。
- 2、"仅部分预览"的文档,不可在线预览部分如存在完整性等问题,可反馈申请退款(可完整预览的文档不适用该条件!)。
- 3、如文档侵犯您的权益,请联系客服反馈,我们会尽快为您处理(人工客服工作时间:9:00-18:30)。
ENVI Tutorial:Landsat TM and SAR DataFusionTable of ContentsO VERVIEW OF T HIS T UTORIAL (2)D ATA F USION (3)Preparing Images (3)R OME,I TALY,D ATA F USION E XAMPLE (4)Read and Display Images (4)Register the TM image to the ERS-2 image (4)Perform HSI Transform to Fuse Data (5)Display and Compare Results (5)Overview of This TutorialThis tutorial is designed to demonstrate selected ENVI data fusion capabilities. You will co-register Landsat Thematic Mapper (TM) data and ERS-2 synthetic aperture radar (SAR) data of Rome, Italy using image-to-image registration. You will fuse the two datasets using a hue-saturation-intensity (HSI) color transform, and you will compare the fused data to the individual datasets.ERS-2 and Landsat images used in this tutorial are provided courtesy of the European Space Agency (ESA) and Eurimage (used with permission) and may not be redistributed without explicit permission from these organizations.For additional data fusion details, please see ENVI Help.Files Used in This TutorialENVI Resource DVD: envidata/rometm_ersFile Descriptionrome_tm (.hdr) Landsat TM data of Rome, Italyrome_ers2 (.hdr) ERS-2 SAR data of Rome Italyromr_tm.pts Ground control points (GCPs) for image-to-imageregistrationData FusionData fusion is the process of combining multiple image layers into a single composite image. It is commonly used to enhance the spatial resolution of multispectral datasets using high spatial resolution panchromatic or single-band SAR data.The following sections demonstrate the preparation required to fuse image datasets in ENVI, and how to perform data fusion.Preparing ImagesTo perform data fusion in ENVI, the files must either be georeferenced (in which case spatial resampling is performed on the fly), or, if not georeferenced, cover the same geographic area, have the same pixel size, have the same image size, and have the same orientation. The files used in this exercise are not georeferenced. Therefore, the low spatial resolution images must be resampled to have the same pixel size as the high spatial resolution image (using nearest-neighbor resampling).Rome, Italy, Data Fusion ExampleRead and Display Images1.From the ENVI main menu bar, select File→ Open Image File. Navigate to envidata\rometm_ers andselect rome_ers2. Click Open. This file contains ERS-2 SAR data.2.In the Available Bands List, select the Gray Scale radio button. Select Band 1 under rome_ers2 and click LoadBand.3.From the ENVI main menu bar, select File→Open Image File. Select rome_tm. Click Open. This file containsLandsat TM data.4.In the Available Bands List, click Display #1 and select New Display.5.Select the RGB Color radio button. Select Band 4, Band 3, and Band 2 in sequential order. Click Load RGB todisplay rome_tm as a false-color composite into Display #2.Following is a comparison of the Landsat TM false-color composite (left) and the ERS-2 SAR gray scale image(right):Register the TM image to the ERS-2 image1.From the ENVI main menu bar, select Map →Registration→Select GCPs: Image-to-Image. An Image toImage Registration dialog appears.2.Under Base Image, select Display #1 (ERS-2 data). Under Warp Image, select Display #2 (TM data). ClickOK. A Ground Control Points Selection dialog appears.3.From the Ground Control Points Selection dialog menu bar, select File→Restore GCPs from ASCII. A fileselection dialog appears.4.Select rome_tm.pts and click Open.5.Pre-selected GCPs are loaded into both the TM and ERS-2 display groups. Review the positions of these points inboth images for accuracy, and observe the total RMS error listed at the bottom of the Ground Control PointsSelection dialog.6.Click Show List. In the Image to Image GCP List that appears, scroll to the right and review the RMS values foreach GCP. These GCPs are sufficient for a quick registration and for this exercise; however, adding more GCPs will improve the match between images. See the tutorial Image Georeferencing and Registration for additional details about performing image-to-image registration. From the Ground Control Points Selection dialog menu bar, select File→Cancel.7.From the Ground Control Points Selection dialog menu bar, select Options→Warp File. A file selection dialogappears. Select rome_tm and click OK to warp all seven TM bands to match the ERS-2 data. A RegistrationParameters dialog appears.8.Enter the following values for Output Image Extent:Upper Left X: 1Upper Left Y: 1Output Samples: 5134Output Lines: 55499.Accept the default values for the remaining fields. In the Enter Output Filename field, enter register_tm.Click OK to perform the image-to-image registration.10.In the Available Bands List, click Display #2 and select New Display.11.In the Available Bands List, select the RGB Color radio button. Select Warp bands 4, 3, and 2 underregister_tm and click Load RGB to display the registered TM image as a false-color composite in Display #3.Perform HSI Transform to Fuse Data1.From the ENVI main menu bar, select Transform→Image Sharpening→HSV. A Select Input RGB dialogappears.2.Select Display #3 (which contains register_tm) and click OK. A High Resolution Input File appears.3.Select Band 1 under rome_ers2 and click OK. An HSV Sharpening Parameters dialog appears.4.In the Enter Output Filename field, enter rome_fused.img and click OK.Display and Compare Results1.In the Available Bands List, select the RGB Color radio button. Click Display #2.2.Select the HSV Sharp R, G, and B bands under rome_fused.img in sequential order. Click Load RGB to loadthe HSV-sharpened, fused, color image into Display #2, replacing the original TM image.Following is a subset of the fused image:3.From a Display group menu bar, select Tools→Link→Link Displays. A Link Displays dialog appears.4.Click OK to link Display #1 (original ERS-2 image), Display #2 (fused image), and Display #3 (registered TMimage). Compare these three images.5.Try fusing other color composites with the ERS-2 data as above and compare the results.6.When you are finished, exit ENVI.。