《人工智能》第八章机器人规划
人工智能应用基础智慧树知到答案章节测试2023年

第一章测试1.()被称为“人工智能之父”。
() A:亚瑟·塞缪尔 B:约翰·冯·诺依曼 C:约翰·麦卡锡 D:唐纳德·赫布答案:C2.2016年3月9日至15日,谷歌AlphaGo机器人在围棋比赛中以比分()击败了世界冠军李世石。
() A:4:1 B:4:2 C:5:0 D:3:2 答案:A3.约瑟夫·魏岑鲍姆教授开发的(),实现了计算机与人通过文本进行交流。
() A:ELIZA B:谷歌Allo C:微软小冰 D:苹果Siri 答案:A4.在1986年,罗斯·昆兰提出了()概念,这是机器学习另一个主流的闪光点。
() A:感知机 B:决策树 C:BP D:随机森林答案:B5.首次提出“人工智能”是在()年。
() A:1946 B:1916 C:1956 D:1960 答案:B6.人工智能发展的驱动力包括()。
() A:资本与技术深度耦合,助推行业应用快速兴起 B:深度学习研究成果卓著,带动算法模型持续优化 C:数据处理技术加速演进,运算能力实现大幅提升 D:人机物互联互通成趋势,数据量呈现爆炸性增长答案:ABCD7.人工智能产业链关键技术,主要分哪三个核心层()。
() A:技术层 B:基础层 C:中间层 D:应用层答案:ABD8.克劳德·香农提出用二进制替代十进制运算,并将计算机分成了5大组件。
() A:对 B:错答案:B9.专家系统是一个含有大量的某个领域专家水平的知识与经验智能计算机程序系统,能够利用人类专家的知识和解决问题的方法来处理该领域问题.简而言之,专家系统是一种模拟人类专家解决领域问题的计算机程序系统。
() A:对 B:错答案:A第二章测试1.机器学习是人工智能的()。
() A:基础 B:根本 C:核心 D:其他都正确答案:C2.目标检测是对目标进行识别和( )。
() A:标注 B:定位 C:检测 D:学习答案:B3.深度学习的核心是 ( )。
《人工智能》课程教学大纲.doc
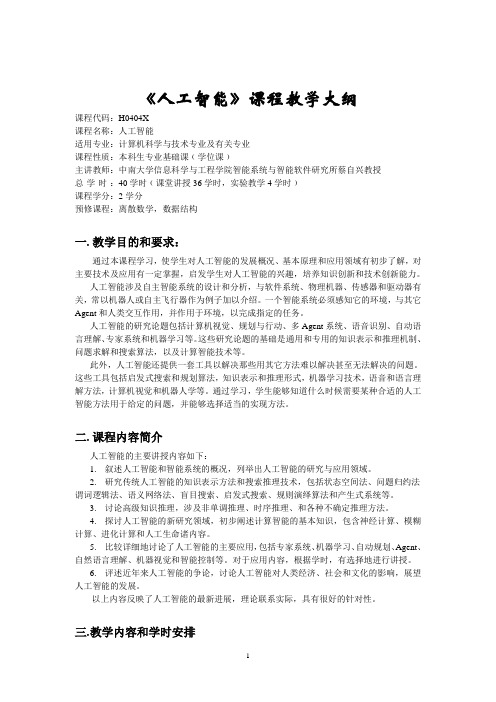
《人工智能》课程教学大纲课程代码:H0404X课程名称:人工智能适用专业:计算机科学与技术专业及有关专业课程性质:本科生专业基础课﹙学位课﹚主讲教师:中南大学信息科学与工程学院智能系统与智能软件研究所蔡自兴教授总学时:40学时﹙课堂讲授36学时,实验教学4学时﹚课程学分:2学分预修课程:离散数学,数据结构一.教学目的和要求:通过本课程学习,使学生对人工智能的发展概况、基本原理和应用领域有初步了解,对主要技术及应用有一定掌握,启发学生对人工智能的兴趣,培养知识创新和技术创新能力。
人工智能涉及自主智能系统的设计和分析,与软件系统、物理机器、传感器和驱动器有关,常以机器人或自主飞行器作为例子加以介绍。
一个智能系统必须感知它的环境,与其它Agent和人类交互作用,并作用于环境,以完成指定的任务。
人工智能的研究论题包括计算机视觉、规划与行动、多Agent系统、语音识别、自动语言理解、专家系统和机器学习等。
这些研究论题的基础是通用和专用的知识表示和推理机制、问题求解和搜索算法,以及计算智能技术等。
此外,人工智能还提供一套工具以解决那些用其它方法难以解决甚至无法解决的问题。
这些工具包括启发式搜索和规划算法,知识表示和推理形式,机器学习技术,语音和语言理解方法,计算机视觉和机器人学等。
通过学习,学生能够知道什么时候需要某种合适的人工智能方法用于给定的问题,并能够选择适当的实现方法。
二.课程内容简介人工智能的主要讲授内容如下:1.叙述人工智能和智能系统的概况,列举出人工智能的研究与应用领域。
2.研究传统人工智能的知识表示方法和搜索推理技术,包括状态空间法、问题归约法谓词逻辑法、语义网络法、盲目搜索、启发式搜索、规则演绎算法和产生式系统等。
3.讨论高级知识推理,涉及非单调推理、时序推理、和各种不确定推理方法。
4.探讨人工智能的新研究领域,初步阐述计算智能的基本知识,包含神经计算、模糊计算、进化计算和人工生命诸内容。
《走进人工智能》教学设计

《走进人工智能》教学设计教材分析:本节课是初中九年级信息技术第八章《走进人工智能》中的内容。
人工智能在生活中已经无处不在,它深刻影响着我们的生活。
越来越低的计算成本、科学家梦寐以求的海量数据,推动了“机器学习”的飞速发展,特别是深度学习的广泛应用,模式识别、自动驾驶、机器翻译、智能机器人等应用无处不在。
本节内容在机器人、物联网的基础之上,旨在让学生了解人工智能的起源与历史,感人工智能带给我们生活的便利,学会正确对待人工智能。
学情分析:本课面对的教学对象是初中九年级的学生,对知识的获取已经开始由感性认识提升到理性认识,已经具有一定的研究能力,探究新知的欲望也比较强烈,在日常的学习和生活中,也或多或少的接触过人工智能技术的应用,对这项技术充满好奇。
但是,他们对人工智能的了解更多的停留在日常学习和生活中的所见所闻,对人工智能的起源和概念缺乏系统的理解。
设计理念:本节是人工智能基础知识的教学,着重采用讲授法、体验法、讨论学习等方式,为了激发学生学习的兴趣,本人改变传统的教学,教师带领进入三个馆:能量加油馆、智能体验馆、全民辩论馆,以此来走进人工智能。
其中智能体验馆中通过三个体验活动:机器翻译--中英互译,人机大战之“五子棋大战”,人机对话---和siri聊天,通过这种体验的方式,让学生在一种愉快轻松的氛围中,丰富对人工智能的认知与理解,了解身边的人工智能技术。
感受人工智能神奇以及带给生活的便利,初步树立为科技做贡献的理想。
也通过辩论赛的方式,引导学生树立正确对待人工智能的意识,理解人与人工智能应该以怎样的关系相处。
教学目标:知识与技能(1)了解人工智能的历史、概念。
(2)了解人工智能在生活中的运用。
过程与方法教师带领进入三个馆:能量加馆、智能体验馆、全民辩论馆,以此来走进人工智能,其中智能体验馆中通过三个体验活动:机器翻译--中英互译,人机大战之“五子棋大战”,人机对话---和siri 聊天,通过这种体验的方式,了解身边的人工智能技术,丰富对人工智能的认识与理解。
机器人规划
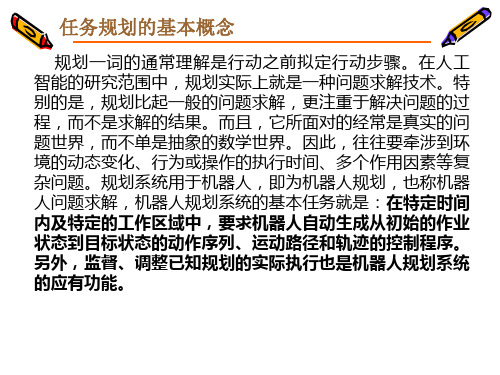
(7.3)
(7.4)
7.2.1三次多项式插值 关于四个系数的线性方程:
0 a0 3 f a0 a1t f a 2 t 2 a t f 3 f
0 a1 0 a1 2a 2 t f 3a 3 t 2 f
空间路径规划
当机器人的手抓、臂或本体要穿行于存在障碍物的外部世界, 去达到某个目标位置时,就需要在空间确定一条无碰撞的穿行 路径,这就是空间路径规划问题,也称为无碰路径规划问题。 与任务规划有所不同,在此,“规划”的含义实际上是直观地 求解带有约束的几何问题,而不是操作序列或行为步骤。另一 方面,如果把运动物体看作要研究的问题的某种状态,把障碍 物看作要研究的问题的约束条件,而无碰路径则为满足约束条 件的解,空间路径规划就是一种多约束的问题的求解过程。
(0) 0 (t f ) f
(0) 0 (t f ) 0
(7.1)
(7.2)
由此确定了一个三次多项式:
(t ) a0 a1t a2t 2 a3t 3
关节速度和加速度:
(t ) a1 2a 2 t 3a3t 2
(7.8)
7.2.2过路径点的三次多项式插值 重新求得三项式的系数:
a0 0 a1 0 3 2 1 a2 2 ( f 0 ) f 0 tf tf tf 2 1 a3 3 ( f 0 ) 2 ( 0 f ) tf tf
2.4用抛物线过渡的线性插值
按式(7.15)算出相应的tb
2t 2 4 ( ) t f 0 tb 2 2
(7.16)
人工智能本科习题

图8.22机械手堆积木规划问题
8-8指出你的过程结构空间求得的图8.23问题的路径,并叙述如何把你在上题中所得结论推广至包括旋转情况。
图8.23一个寻找路径问题
第一章绪论
1-1.什么是人工智能?试从学科和能力两方面加以说明。
1-2.在人工智能的发展过程中,有哪些思想和思潮起了重要作用?
1-3.为什么能够用机器(计算机)模仿人的智能?
1-4.现在人工智能有哪些学派?它们的认知观是什么?
1-5.你认为应从哪些层次对认知行为进行研究?
1-6.人工智能的主要研究和应用领域是什么?其中,哪些是新的研究热点?
3-16下列语句是一些几何定理,把这些语句表示为基于规则的几何证明系统的产生式规则:
(1)两个全等三角形的各对应角相等。
(2)两个全等三角形的各对应边相等。
(3)各对应边相等的三角形是全等三角形。
(4)等腰三角形的两底角相等。
第四章计算智能(1):神经计算模糊计算
4-1计算智能的含义是什么?它涉及哪些研究分支?
5-2试述遗传算法的基本原理,并说明遗传算法的求解步骤。
5-3如何利用遗传算法求解问题,试举例说明求解过程。
5-4用遗传算法求的最大值
5-5进化策略是如何描述的?
5-6简述进化编程的机理和基本过程,并以四状态机为例说明进化编程的表示。
5-7遗传算法、进化策略和进化编程的关系如何?有何区别?
5-8人工生命是否从1987年开始研究?为什么?
2-10试构造一个描述你的寝室或办公室的框架系统。
第三章搜索推理技术
3-1什么是图搜索过程?其中,重排OPEN表意味着什么,重排的原则是什么?
3-2试举例比较各种搜索方法的效率。
第八章 Agent(艾真体)

湖南科技大学计算机学院
戴祖雄
5
(4)开放性。通过网络互连和系统的分布,便于扩 充系统规模,使系统具有比单个系统更大的开放 性和灵活性。 (5)容错性。系统具有较多的冗余处理节点、通信 路径和知识,能够使系统在出现故障时,仅仅通 过降低响应速度或求解精度,就可以保持系统正 常工作,提高工作可靠性。 (6)独立性。系统把求解任务归约为几个相对独立 的子任务,从而降低了各个处理节点和子系统问 题求解的复杂性,也降低了软件设计开发的复杂 性。
湖南科技大学计算机学院
戴祖雄
12
2.艾真体的要素 艾真体必须利用知识修改其内部状态(心理状态),以适应 环境变化和协作求解的需要。艾真体的行动受其心理状态驱 动。人类心理状态的要素有认知(信念、知识、学习等)、情 感(愿望、兴趣、爱好等)和意向(意图、目标、规划和承诺等) 三种。着重研究信念(belief)、愿望(desire)和意图(intention) 的关系及其形式化描述,力图建立艾真体的BDI(信念、愿望和 意图)模型,已成为艾真体理论模型研究的主要方向。 信念、愿望、意图与行为具有某种因果关系,如图8.2所 示。其中,信念描述艾真体对环境的认识,表示可能发生的状 态;愿望从信念直接得到,描述艾真体对可能发生情景的判断; 意图来自愿望,制约艾真体,是目标的组成部分。
湖南科技大学计算机学院
戴祖雄
7
上述对分布式人工智能的分类并非绝对和完善。 有些人认为MAS基本上就是分布式人工智能,DPS仅是 MAS研究的一个子集,他们提出,当满足下列三个假设 时,MAS就成为DPS系统:①Agent友好;②目标共同; ③集中设计。显然,持这种看法的人大大扩展了MAS的 研究和应用领域。正是由于MAS具有更大的灵活性,更 能体现人类社会的智能,更适应开放和动态的世界环境, 因而引起许多学科及其研究者的强烈兴趣和高度重视。 目前对Agent和MAS的研究有增无减,仍是一个研究 热点。要研究的问题包括Agent的概念、理论、分类、 模型、结构、语言、推理和通信等。
中职《信息技术》教学设计 第8章 任务1 初识人工智能
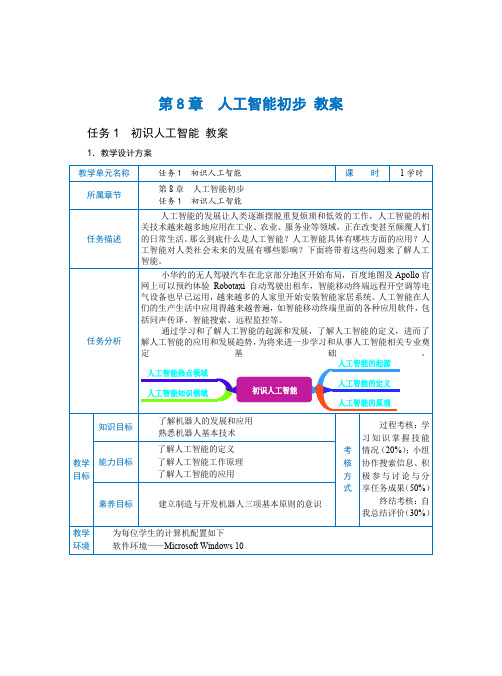
分组协作,搜集人工智能的知识领域和工作原理
分组
讨论
引导
启发
讲授
投影
屏幕
10
学生
讨论
分组讨论人工智能在生活中的应用。说一说导航软件是如何让出行更便捷的。
联系日常学习和生活,分组搜集并讨论问题记录讨论结果上传学习平台
人工智能定义
人工智能(Artificial Intelligence),英文缩写为AI。它是研究、开发用于模拟、延伸和扩展人的智能的理论、方法、技术及应用系统的一门新的技术科学。
人工智能是计算机科学的一个分支,它企图了解智能的实质,并生产出一种新的能以人类智能相似的方式做出反应的智能机器,来模拟人的某些思维过程和智能行为。该领域的研究包括机器人、语音识别、图像识别、自然语言处理和专家系统等,涉及计算机科学、心理学、哲学和语言学等学科,可以说几乎包含了自然科学和社会科学的所有学科,其范围已远远超出了计算机科学的范畴。人工智能技术本质上是以数学算法为核心,辅以计算机技术来模拟人的智能行为的技术。
第8章 人工智能初步 教案
任务
1
教学单元名称
任务1 初识人工智能
课 时
1学时
所属章节
第8章人工智能初步
任务1 初识人工智能
任务描述
人工智能的发展让人类逐渐摆脱重复烦琐和低效的工作,人工智能的相关技术越来越多地应用在工业、农业、服务业等领域,正在改变甚至颠覆人们的日常生活。那么到底什么是人工智能?人工智能具体有哪些方面的应用?人工智能对人类社会未来的发展有哪些影响?下面将带着这些问题来了解人工智能。
通过学习和了解人工智能的起源和发展,了解人工智能的定义,进而了解人工智能的应用和发展趋势,为将来进一步学习和从事人工智能相关专业奠定展和应用
第八章 人工智能技术应用场景

4.伦理与安全
随着技术的进步,人工智能在医疗行为 中的方方面面超越人类只是时间问题。到那 时是否应该在医疗过程中给予机器人自主权? 能否保证机器人进行医疗行为的安全性?智 能诊疗过程中如果出现了意外情况,责任应 该如何判定?这些都是智能医学的实现过程 中一定会遇到的问题,应当在政策和法律上 对相关问题提前做出约束和规范。
智慧交通中的核心技术
1.人工智能识别技术和无线传感网络技术
人工智能识别技术和无线传感技术是物体感知 和标识的主要方式,同时也是建设智慧交通 的核心技术。
2.云计算技术
智慧交通系统中的各个模块目前还处于一 种单独作战、信息分离的状态,无法促进各 个数据信息之间的有效连接,导致数据浪费 现象较为严重。智慧交通云就是以交通服务 领域为主要目标的一种融合云计算的管理技 术,它具有云计算中的资源统一分析、信息 安全与海量信息存储等优势,从而为城市交 通的数据管理和共享提供有效的渠道。
(4)智能化是制造自动化的发展方向,是先进制造业发展的重要形态。智能制造 (Intelligent Manufacturing,IM)是一种由智能机器和人类专家共同组成的人 机一体化智能系统,它在制造过程中能进行智能活动,诸如分析、推理、判断、 构思和决策等。通过人与智能机器的合作,可以扩大、延伸和部分地取代人类 专家在制造过程中的脑力劳动。
智慧交通中的人工智能应用
1.自动驾驶汽车 自动驾驶汽车(Self-driving Automobile)又称无人驾驶汽车、计算机驾驶汽
车或轮式移动机器人,是一种通过计算机系统实现无人驾驶的智能汽车。它依 靠人工智能、视觉计算、雷达、监控装置和全球定位系统协同合作,让计算机 可以在没有任何人类主动操作的情况下,自动安全地操作机动车辆。
人工智能(第八章)(Artificial intelligence (the eighth chapter))
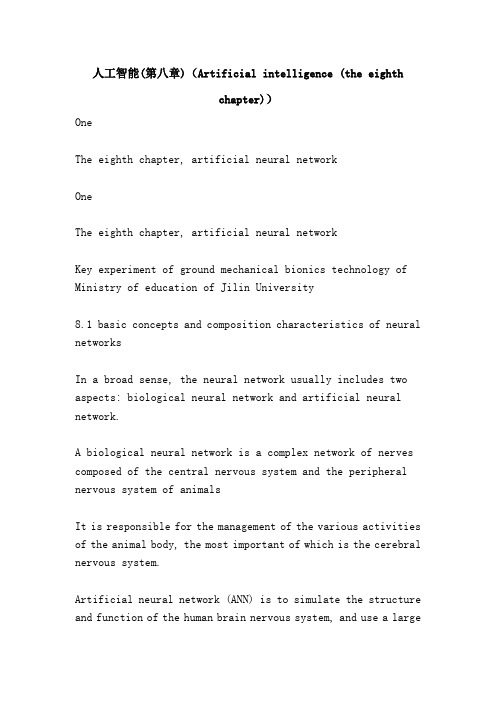
人工智能(第八章)(Artificial intelligence (the eighthchapter))OneThe eighth chapter, artificial neural networkOneThe eighth chapter, artificial neural networkKey experiment of ground mechanical bionics technology of Ministry of education of Jilin University8.1 basic concepts and composition characteristics of neural networksIn a broad sense, the neural network usually includes two aspects: biological neural network and artificial neural network.A biological neural network is a complex network of nerves composed of the central nervous system and the peripheral nervous system of animalsIt is responsible for the management of the various activities of the animal body, the most important of which is the cerebral nervous system.Artificial neural network (ANN) is to simulate the structure and function of the human brain nervous system, and use a largenumber of soft and hardware processing units,A network system built manually by extensive parallel interconnection.Structure and functional characteristics of 8.1.1 biological neuronsBiological neurons, usually called nerve cells, are made up of living beingsThe basic unit of the nervous system, referred to as a neuron.Neurons mainly consist of three parts, including cell bodies,The basic structures of axons and dendrites are shown here.1. the structure of biological neuronsBiological neuron structureTwoKey experiment of ground mechanical bionics technology of Ministry of education of Jilin UniversityFunctional properties of 2. neuronsFrom the point of view of biological cybernetics, neurons, which are the basic unit of control and information processing, haveList some features and features.(1) space-time integration function;The neuron has the function of time integration for the information transmitted by the same synapse at different time;The information of different synaptic afferents has spatial integration function. The combination of the two functions makes biological neurons possessSpatio temporal integration of input information processing functions.(2) the dynamic polarization of neuronsAlthough different neurons differ markedly in shape and function, most neurons do predictIn the direction of which information flows.(3) excitatory and inhibitory states;Neurons have two conventional working states, namely excitatory state and inhibitory state.(4) plasticity of structure;The characteristics of synaptic transmission information are variable, and the transmission function can be enhanced withthe change of nerve impulse transmission modeWeak, so the connection between neurons is flexible, which is called structural plasticity.ThreeKey experiment of ground mechanical bionics technology of Ministry of education of Jilin University(5) pulse and potential signal conversionSynaptic interface has the function of pulse and potential signal conversion.(6) synaptic delay and refractory period;Synaptic transmission of information is delayed and refractory, requiring a certain time interval between two adjacent inputs,In the meantime, it does not affect incentives and does not convey information, which is called refractory period.(7) learning, forgetting and fatigueBecause of structural plasticity, synaptic transmission is enhanced, attenuated, and saturated, so nerve cells also respond accordinglyLearning, forgetting, or fatigue effects (saturation effects).The composition and structure of 8.1.2 artificial neural network1. artificial neural networkArtificial neural networks (Artificial, Neural, Nets, ANN) are composed of a large number of processing units that are extensively interconnectedArtificial neural networks are used to simulate the structure and function of the brain nervous system. These processing units are called artificial neurons.Artificial neural networks can be viewed as directed graphs with artificial neurons as nodes and connected by directed weighted arcs.In directed graphs, artificial neurons are the simulation of biological neurons, while directed arcs are simulations of axon synapse dendritic pairs,The weights of the directed arcs represent the strength of the interaction between the two artificial neurons that are interconnected.Key experiment of ground mechanical bionics technology of Ministry of education of Jilin UniversityAs shown in the figure for artificial neural network composed of sketch.It consists of multiple artificial neurons interconnected.The circle represents the cell body of the neuron; XRepresents the external input of the neuron; W is theThe connections between neurons and each input are strongDegrees are called connection weights.For an individual worker neuron in ANN, the input from other neurons is multiplied by the weights, and then the phasePlus. Compare all sums with the threshold level. When the sum is above the threshold level, the output is 1Then, the output is 0.As shown in the figure for artificial neural network composed of sketch.It consists of multiple artificial neurons interconnected.The circle represents the cell body of the neuron; XRepresents the external input of the neuron; W is theThe connections between neurons and each input are strongDegrees are called connection weights.For an individual worker neuron in ANN, the input from other neurons is multiplied by the weights, and then the phasePlus. Compare all sums with the threshold level. When the sum is above the threshold level, the output is 1Then, the output is 0.FiveKey experiment of ground mechanical bionics technology of Ministry of education of Jilin University2. the working process of artificial neuronsFor each neuron in an artificial neural network, it can accept a set of other neurons from the systemThe input signal, each input corresponds to one weight, and the weighted sum of all inputs determines the activation state of the neuron. thisInside, each right is equivalent to the synaptic strength of the synapse".For a neuron, it is assumed that information from other neurons, I, is Xi, and that they interact with the neuronWith intensity, that is, the connection weight is wi, i=0,1,... N-1, the internal threshold of the neuron is theta.Then the input of this neuron is: the output of the neuron is:.NOne)In the formula, Xi is the input of the I element, and wi is the interconnection weight between the I neuron and the neuron. F is called an excitation function or functionFunction that determines the output of a neuron.The output of the neuron is 1 or 0 depending on the sum of its inputs greater than or less than the internal threshold theta.NOneIZero.Sigma =F (.Y= Wixi Wixi =I Zero order Sigma =Wixi.NOneIs called activation value.IZero.Sigma=SixKey experiment of ground mechanical bionics technology of Ministry of education of Jilin UniversityThe excitation function generally has nonlinearcharacteristics. The commonly used nonlinear excitation functions have threshold type and piecewise linear type,The Sigmoid function type (referred to as "S") and the hyperbolic tangent type are shown in the figure.The threshold type function is also called the step function,It represents the relationship between the activation value Sigma and its output f (sigma). This two valued modelA neuron whose output is valued at 1 or 0, respectively, represents the excitatory and inhibitory states of neurons. At some point, GodThe state of the yuan is determined by the excitation function F.When the activation value is Sigma >0, the neuron is activated and excited, and its state f (sigma) is 1;When the activation value is Sigma <0, the neuron is not activated and enters a state of suppression, with the state f (sigma) of 0.F (sigma)Sigma 10F (sigma)Sigma 10F (sigma)Sigma 10F (sigma)Sigma 10-1 (a), threshold type (b), piecewise linear (c), Sigmoid function type (d), hyperbolic tangent typeThe excitation function generally has nonlinear characteristics. The commonly used nonlinear excitation functions have threshold type and piecewise linear type,The Sigmoid function type (referred to as "S") and the hyperbolic tangent type are shown in the figure.The threshold type function, also called the step function, represents the relation between the activation value Sigma and its output f (sigma). This two valued modelA neuron whose output is valued at 1 or 0, respectively, represents the excitatory and inhibitory states of neurons. At some point, GodThe state of the yuan is determined by the excitation function F.When the activation value is Sigma >0, the neuron is activated and excited, and its state f (sigma) is 1;When the activation value is Sigma <0, the neuron is not activated and enters a state of suppression, with the state f (sigma) of 0.F (sigma)Sigma 10F (sigma)Sigma 10F (sigma)Sigma 10F (sigma)Sigma 10-1 (a), threshold type (b), piecewise linear (c), Sigmoid function type (d), hyperbolic tangent typeSevenKey experiment of ground mechanical bionics technology of Ministry of education of Jilin UniversityPiecewise linear function can be regarded as one of the simplest nonlinear functions. It is characterized by limiting the range of functionsWithin a certain range, the input and output meet the linear relationship in a certain range and continue until the output is the mostDomain value. However, when the maximum value is reached, the output will no longer increase. This maximum is called saturation.The S type function is a nonlinear function with the maximum output value, whose output value is continuously valued in a certain range.Neurons whose activation functions are also saturated.The hyperbolic tangent type function is actually a special S type function whose saturation values are - 1 and 1.In artificial neural networks, different connections of neurons form different connection models of networks.3. structure of artificial neural networksCommon connection models are:Forward networkA network of feedback from the input layer to the output layerThere are interconnected networks in the layerAny two neurons in the network can be interconnected by the internet.Piecewise linear function can be regarded as one of the simplest nonlinear functions. It is characterized by limiting the range of functionsWithin a certain range, the input and output meet the linear relationship in a certain range and continue until the output is the mostDomain value. However, when the maximum value is reached, the output will no longer increase. This maximum is calledsaturation.The S type function is a nonlinear function with the maximum output value, whose output value is continuously valued in a certain range.Neurons whose activation functions are also saturated.The hyperbolic tangent type function is actually a special S type function whose saturation values are - 1 and 1.In the artificial neural network,The different connections of neurons form different connection models of networks.3. structure of artificial neural networksCommon connection models are:Forward networkA network of feedback from the input layer to the output layerThere are interconnected networks in the layerAny two neurons in the network can be interconnected by the internet.EightKey experiment of ground mechanical bionics technology of Ministry of education of Jilin University4. classification and main characteristics of artificial neural networksThe neural network model can obtain different classification results from different angles:According to the performance of the network, it can be divided into continuous and discrete networks, and can be divided into deterministic and stochastic networksAccording to the topological structure of the network, it can be divided into feedback network and no feedback network;According to the method of network learning, it can be divided into a learning network with teachers and a learning network without teachers;According to the properties of synaptic connections, they can be divided into first order linear associative networks and higher order nonlinear associated networks;Artificial neural networks have the following main characteristics:(1) it can simulate human's image thinking better;(2) large-scale parallel processing capability;(3) strong learning ability;(4) it has strong fault tolerance and association ability;(5) it is a large-scale, self-organizing and adaptive nonlinear dynamical system.NineKey experiment of ground mechanical bionics technology of Ministry of education of Jilin University8.2 perceptron model and its learning algorithmmethod8.2.1 perceptron modelPerceptron is one of the earliest artificial neural networks models designed and implemented.In 1957, American scholar Rosen Bo Larter (Rosenblatt) proposed a neural network model with self-learning abilityType perceptron model, which leads the research of neural network to engineering realization from pure theory.Perceptron model, also called single layer perceptron, consists of input part and output layer, and the output layer is its computing layer.In the single-layer perceptron model, the input part of the neuron is connected to the various neurons in the output layer. When the input section willWhen the input data is transmitted to the connected neuron, the output layer weights the input data and generates the product via the threshold functionGenerate a set of output data.As shown, a single layer perceptron model.X1x2xn.........Y1ym...Omega ijInput dataAdjustable connection weightsoutput dataMjxxynijiijnijiiji,..., 3,2,10,00111=.......<.Aged.=Sigma sigma ==Theta, Omega, theta, OmegaififThe input and output satisfy the following excitation functions:Key experiment of ground mechanical bionics technology of Ministry of education of Jilin UniversityLearning algorithm of 8.2.2 single layer perceptron modelRosenblatt proposed the learning algorithm of connection weight parameter in perceptron modelFirst, the join weights and thresholds are initialized tosmaller nonzero random numbers;Then input the input with n connection weights into the network;By weighted processing, if the output is different from the desired output, the connection weights are consideredThe number is adjusted automatically according to an algorithm;After many times of repetition, until the difference between the output and the desired output meets the requirements.Single layer perceptron specific learning algorithm (consider only one output):Let Xi (T) be the input of the time t perceptron (i=1,2,... (n), Omega I (T) is the corresponding connection weight, and Y (T) is practicalThe output of D (T) is the desired output, and the output of sensor or 1, or 1, is the single layer perceptronThe learning algorithm is:(1) initialize join weights and thresholds. Give Omega I (T) (i=1,2),... (n) and theta give a smaller non-zero sum, respectivelyRandom numbers are used as their initial values. Omega I (0) is the connection weight of the first input of the t=0 at I, and theta is the outputThreshold in a node.ElevenKey experiment of ground mechanical bionics technology of Ministry of education of Jilin University(2) enter a training parameter X = (x1 (T), X2 (t),... Xn (T) and expected output D (t).(3) calculate the actual output of the networkY (T)=F(sigma (n) Omega I(T) Xi(T) thetaI=1,2,..., n)One(4) calculate the difference between actual output and expected outputDEL=d (T) -y (T)If DEL< epsilon (epsilon is a small positive number), network training ends; otherwise, step (5).(5) adjust the connection weight asOmega = Omega I (t+1) I (T) + [d (T) -y (T)]xi (t (i=1,2),... N).The 0< is less than or equal to 1, a gain factor is used to control the speed change, also known as the gain or learning speed.(6) return (2).The above algorithm shows that the learning of network connection weights is an iterative process, which is in the first (2) step (6) stepRepeat until the error between the actual output of the network and the desired output is met. At this point, incomeThe weighted value of the network is I (i=1,2,... (n) is thenetwork connection parameter that is learned by training data.TwelveKey experiment of ground mechanical bionics technology of Ministry of education of Jilin University8.2.3 multilayer perceptionimplementOne or more layers of processing units are added between the input and output layers of the single layer perceptron to form two layers or moreLayer perceptron.The new layer is called the hidden layer, and the processing unit in the hidden layer is called the hidden unit.The hidden unit acts as a specific detector, which extracts the effective feature information contained in the input pattern, which is processed by the output unitThe patterns are linearly separable.The architecture of a two - layer perceptron is shown in the figure................Y1ynx1x2xnAdjustable weightWeight fixationoutput layerhidden layerInput partIn this figure, the perceptron has two layers of connection, the input partThe connection weights between the hidden layer and the hidden layer units are fixed randomlyFixed value, not adjustable, only output layer and hidden layer unitThe connection weight between the two layers is adjustable.ThirteenKey experiment of ground mechanical bionics technology of Ministry of education of Jilin UniversityMultilayer perceptron can take many disadvantages of single layer perceptron, and some of the problems that can not be solved by some single layer perceptron,It can be solved in a multilayer perceptron.For example, the application of two layer perceptron can solve the XOR logic problem, as shown in the figure.YOutput layer, where the threshold of the output layer neuron is assumed to be 0.5, for each deityThe link weights between the elements are shown in the figureX11-1-111x21The hidden layer is x11=1 * x10+ (-1) * x20-0.5ElevenX21=1 * X20 (-1 * x10-0.5)Input unitbranchY=1 * x11+1 * x21-0.5X10X20The corresponding identification area is shown in the figure.Because each neuron in the hidden layer can have its own half of the plane that can be identifiedAnd the output layer neuron makes the logic of the output of the hidden layerAnd "arithmetic", so its output can be used to identify the hidden layer identifiedA convex polygon formed by the intersection of half and half planes.A2B2A1B1FourteenKey experiment of ground mechanical bionics technology of Ministry of education of Jilin University8.3 back propagation model and its learning algorithmmethod8.3.1 back propagation model and its network structureThe back-propagation model, also known as the B-P(Back-Propagation) model, is used in forward multilayer neural networksThe back-propagation learning algorithm consists of Rumelhart (D.Rumelhart) and McLelland (MeClelland)Proposed 1985.The network structure of B-P algorithm is a forward multilayer network. The network contains not only input and output nodes, but also networkAnd contains one or more layers of hidden (layer) nodes, as shown.X1x2xn.........Y1ym... Y1When the information is input to the network, the information is transmitted from the input layer to the hidden layer first, and then the characteristic function is applied to it,And then spread to the next hidden layer. This layer is passed down until the output node layer is finally output.In the meantime, the excitation function of each layer is differentiable, and the S type function is generally used.Key experiment of ground mechanical bionics technology of Ministry of education of Jilin UniversityLearning algorithms for 8.3.2 backpropagation networksmethodThe purpose of the B-P algorithm is to adjust the connection weights of the network, so that the network is adjusted to any inputCapable of getting the desired output.The learning process consists of forward propagation and reverse propagation. Forward propagation is used to calculate the forward network, that is, to an input letterAfter the network calculation, the output is obtained; the back propagation is used to transfer errors by layer by layer, and the connection rights between neurons are modifiedThe output of the network can reach the desired error requirement after the input information is calculated.Sigma.Kkkyye2')Twenty-oneActivation function. Is the node, function jxfIfOOWIjjiiij here)= = (sigma)Let Oi be the output of the node i in the network, Ij is the input of the node j, and Wij is the connection weight from node i to node j,YK and YK, respectively, the actual output and expected output of the node K on the B-P network output layer.For node j, if the node I is its upper node and the I is connected to j, thenActual output and expected outputThe error E is defined as:Correction formula of connection weight:....'''=......Delta sigma = mkmmkkkkjkjkkkjkkWIfkIfyyOWIIeW When the hidden layer node isWhen the output layer node isDelta, beta, beta, beta)(())KAmong them, beta is the proportionality factor.(1)(2)(3)(4)Key experiment of ground mechanical bionics technology of Ministry of education of Jilin UniversityNYstartSelect a set of training examples,Each sample consists of two parts: the input information and the desired outputSelect a sample from the training sample set and enter the input information into the networkForward propagation: calculates the output of the neurons in each hidden layer and output layer of the networkEndThe delta values of each node in the output layer are calculated in the first half of the formula (3),The connection weights of the neurons in the hidden layer are adjusted according to the formulaBack propagation: each hidden layer is calculated layer by layer in the second half of formula (3)The delta value of the upper neuron, and the connection weight of each neuron is adjusted according to the formula (2)NDoes the error E meet the requirements?Given input vectors and output vectorsAccording to formula (2) the actual output and expected output error eSets the connection weights for the training example and initial values for the thresholdAll of the training examples are centralizedHave you finished the sample yet?Flow chart of YB-P learning algorithmNYstartSelect a set of training examples, each of which consists of two parts, the input information and the desired outputSelect a sample from the training sample set and enter the input information into the networkForward propagation: calculates the output of the neurons in each hidden layer and output layer of the networkEndThe delta values of each node in the output layer are calculated in the first half of the formula (3),The connection weights of the neurons in the hidden layer are adjusted according to the formulaBack propagation: each hidden layer is calculated layer by layer in the second half of formula (3)The delta value of the upper neuron, and the connection weight of each neuron is adjusted according to the formula (2)NDoes the error E meet the requirements?Given input vectors and output vectorsAccording to formula (2) the actual output and expected output error eSets the connection weights for the training example and initial values for the thresholdAll of the training examples are centralizedHave you finished the sample yet?Flow chart of YB-P learning algorithmKey experiment of ground mechanical bionics technology of Ministry of education of Jilin UniversityThe B-P algorithm has many advantages, such as solid theoretical foundation, rigorous derivation process, clear physical concept and good generalityIt is a better algorithm for training forward multilayer networks.B-P algorithm also has some disadvantages, mainly in:(1) the convergence speed of the learning algorithm is slow, and it usually takes thousands of iterations, and with the training sample dimensionWith the increase in number, network performance will become worse.(2) the number of nodes in the network selection there is no theoretical guidance.(3) from a mathematical point of view, the B-P algorithm is a gradient steepest descent method, which may cause local minimaQuestions. When there is a local pole hour, the error meets the requirement on the surface, but the solution is not necessarilyIs the real solution to the problem. Therefore, the B-P algorithm is incomplete.Example of 8.3.3 reverse propagation calculationThe most important step in the B-P learning algorithm is to compute the connection between neurons in the network before training to the multilayer networkThe modified weight quantity Wjk. In order to calculate the delta Wjk, calculated in the backward delta is very important.The following example illustrates the calculation of delta in reverse propagation.EighteenKey experiment of ground mechanical bionics technology of Ministry of education of Jilin UniversityExample: here is a simple forward propagation network that uses the B-P algorithm to determine the weights of each connectionThe calculation method is as followsOneFourX1x22W13W233W34W355y1y2As you can see from the diagram:The reverse propagation is calculated as follows: I3=W13x1+W23x2O3=F(I3)(1) calculation;EI=WOO=Y=F(I)FourThree hundred and forty-three Forty-oneFour .W.E.E.I3 .e I= WO O=Y=F(I)=.D = xFiveThree hundred and fifty-three Fifty-twoFiveOne hundred and thirty-one.W...IThirteenThree hundred and thirteen ThreeTwelveTwoE=([Y1'.Y1)+(Y2'.Y2) ].E.E..e Two =.D = x TwoThirty-two.W.I..ITwenty-threeThree hundred and twenty-three (2) calculation;Delta4.=..IeFour =(Y1 .Y1 ')F'(I4) .E.E.I4 .=D = OThreeForty-three.W.I..I.EThirty-fourFour hundred and thirty-four FourDelta ==(Y.Y ')F'(I)FiveTwo hundred and twenty-five .I5.EE.I5.=.D = O ThreeFifty-three Delta = (delta W Delta + W )F'(I).W.I..IThree million four hundred and thirty-four thousand five hundred and thirty-fiveThreeThirty-fiveFive hundred and thirty-fiveKey experiment of ground mechanical bionics technology of Ministry of education of Jilin University8.4 Hopfield model and its learning algorithmThe feedforward network described earlier lacks dynamic processing capability, and hence its computational power is not strong enough.。
AI机器人的自主决策与行为规划

AI机器人的自主决策与行为规划随着人工智能(AI)技术的不断发展,AI机器人在许多领域表现出了强大的潜力和应用前景。
其中一个重要的方面是AI机器人的自主决策与行为规划能力。
本文将探讨AI机器人在自主决策和行为规划方面的应用和挑战,并展望未来的发展方向。
一、AI机器人的自主决策能力AI机器人的自主决策能力是指机器人能够自主地根据环境和任务要求做出决策,并采取相应的行动。
这种能力的核心是机器学习和推理。
通过对大量数据的学习和分析,AI机器人可以识别模式、预测趋势,并根据这些信息做出决策。
在实际应用中,AI机器人的自主决策能力被广泛应用于自动驾驶、智能家居、医疗保健等领域。
例如,自动驾驶汽车可以根据路况、交通规则和实时数据来做出决策,保证行车安全和效率。
智能家居系统可以根据用户的喜好和习惯来调节室内环境,提供舒适的居住体验。
然而,AI机器人的自主决策也面临一些挑战。
首先是数据的质量和数量。
机器学习和推理需要大量的高质量数据来训练模型和算法,但获取这些数据并不容易。
其次是决策过程的透明度和解释性。
AI机器人的决策过程通常是黑盒子,很难向人类解释其决策的原因和依据。
二、AI机器人的行为规划能力AI机器人的行为规划能力是指机器人可以根据自主决策制定和执行行为计划。
这种能力是基于AI机器人对环境、任务和自身能力的感知和理解。
通过感知和理解,机器人可以根据任务目标和约束条件来生成可行的行动序列,并选择最佳的行为策略。
行为规划在工业生产、物流配送、服务机器人等领域有着广泛的应用。
例如,在工业生产中,AI机器人可以根据生产线的配置和要求,规划机械臂的运动轨迹和物料传递路径,实现高效率的生产。
在物流配送中,AI机器人可以根据货物的位置和目的地,规划最短的配送路径,并避开障碍物和交通拥堵。
然而,AI机器人的行为规划也面临一些挑战。
首先是环境的复杂性和不确定性。
现实世界中的环境往往是动态和不确定的,AI机器人需要能够处理和适应各种变化的情况。
人工智能导论 课件 PPT -第八章 智能体与智能机器人
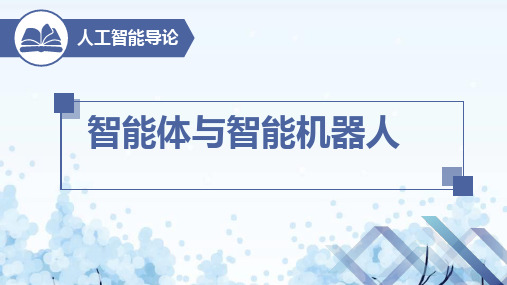
智能体的PEAS分析
智能体
性能
环境
执行器
传感器
无人驾驶汽 安全性、时间、 道路、其他 转向、加速器、 相机、声呐、GPS、速度计、
车
合法驾驶、舒适 汽车、行人、 制动器、信号、 里程计、加速度计、引擎传感
性
路标
喇叭、显示器 器、操作盘
网上购物
价格、质量、合 网站、厂商、 商品展示、跟随 网页(文本、图像、脚本)
8.2.1多智能体协商
在多智能体系统中,如果每个智能体都是自利的(使 自身获利最大),那么每个智能体的最优策略组合未 必是多智能体系统的最优策略。这反映了多智能体系 统中个体利益与集体利益相冲突的矛盾本质。
1、纳什均衡
纳什均衡
假设有两个小偷A和B一起私闯民宅偷盗被警察抓获。警方将两 人分别置于不同的两个房间内进行审讯,针对每一个犯罪嫌疑人, 警方给出的政策是: ➢ 如果一个犯罪嫌疑人坦白了罪行,交出了赃物,于是证据确凿,
完全 单个 确定 阵发 半动态 连续
网上购物 部分 单个 随机 顺序 半动态 离散
8.1.2智能体的结构
智能体的结构是建构智能体的方法学,即将智能体分 为不同的模块并描述模块之间的交互关系。
1、智能体的抽象结构
智能体的抽象结构
2、智能体的分类
智能体的分类
简单反射智能体
基于模型的反射智能体
智能体的定义
著名智能体理论研究学者Wooldridge(伍德里奇)博士等在 讨论智能体时,则提出“弱定义”和“强定义”二种定义方法: 弱定义智能体是指具有自治性、反应性、主动性、社会性和进化 性等基本特性的智能体;强定义智能体是指不仅具有弱定义中的 基本特性,而且具有移动性、通信能力、理性或其它特性的智能 体。
儿童ai小车课程设计

儿童ai小车课程设计一、课程目标知识目标:1. 学生能理解AI小车的基本工作原理,掌握相关的编程知识。
2. 学生能够描述AI小车的主要部件及其功能。
3. 学生能够理解并运用传感器收集环境数据,处理数据以控制AI小车的行为。
技能目标:1. 学生能够通过小组合作,完成AI小车的组装和编程。
2. 学生能够运用逻辑思维和问题解决能力,设计并实现AI小车在不同环境下的自动行驶策略。
3. 学生能够运用信息技术工具,对AI小车进行调试和优化,提高其性能。
情感态度价值观目标:1. 学生培养对科学技术的兴趣,增强对人工智能的认识和好奇心。
2. 学生在团队合作中,学会互相尊重、支持和沟通,培养团队精神和责任感。
3. 学生通过课程学习,认识到人工智能在生活中的应用,激发创新精神和实践能力。
课程性质:本课程为实践性强的跨学科课程,结合信息技术、工程技术和数学知识。
学生特点:四年级学生,具有一定的逻辑思维能力和动手操作能力,对新鲜事物充满好奇。
教学要求:教师需引导学生主动探究,鼓励学生动手实践,关注学生的个别差异,提供有针对性的指导。
在教学过程中,注重培养学生的创新能力、团队合作精神和解决问题的能力。
通过课程目标的实现,使学生获得实际的学习成果。
二、教学内容1. AI小车基础知识:介绍AI小车的基本构成,包括电机、传感器、控制板等,让学生理解各部分的功能和相互关系。
- 教材章节:第三章《认识机器人》- 内容列举:机器人基本构成、各部件作用。
2. 编程基础:学习编程软件的使用,掌握基本的编程语法和逻辑,为AI小车编写控制程序。
- 教材章节:第五章《编程入门》- 内容列举:编程环境搭建、基本编程语法、逻辑控制。
3. AI小车组装与调试:分组进行AI小车的组装,学习使用传感器进行环境感知,调试程序,实现小车的基本功能。
- 教材章节:第六章《机器人组装与调试》- 内容列举:组装技巧、传感器应用、程序调试。
4. 自动行驶策略设计:运用数学知识和逻辑思维,设计AI小车在不同环境下的自动行驶策略。
工业机器人职业规划书 6篇

工业机器人职业规划书6篇工业机器人职业规划书 1前言:我以前对职业规划从没有真正的考虑深思过,因为“计划永远赶不上变化”,我认为与其花时间规划自己今后的人生还不如花时间在各种能增加自己实力的事情上。
但在借鉴古今中外的企业大家的职业历程之时,我发现他们的成功经验有共同的一条,那就是:规划自己的职业,做到刚踏入社会的短短几年就能成为该行业的佼佼者。
职业生涯的规划使自己能够更好地了解自己的优势和存在的不足,使自己在大学期间有针对性的学习,提高自己的能力,真正做到“取长补短”,成为该行业的佼佼者。
以下我将从认识自我、所学专业概述、定位职业、择优职业目标与路径、行动计划与策略等方面来规划我的职业生涯,以使我能够在这个竞争激烈的社会不断提升自我完善自我!一、认识自我1. 行为风格:稳重、踏实、接受新事物的能力与适应力比较强。
肯吃苦耐劳,做事有责任心,对于自己做出的承诺尽自己的全力兑现,有较好的自控能力。
2. 个性特征:乐观主义者,自信、正直、善良,喜欢结交朋友,喜欢挑战,对生活、学习充满热情,有着强烈进取心和较强的自学能力。
3. 兴趣爱好:喜欢立体设计、运动、游览名胜,此外还喜欢自己设计制造东西。
4. 综合分析:出于对科学技术的向往和热爱,和对机器复杂结构神秘感的好奇,我毅然选择了机械设计制造及其自动化这个专业。
希望用自己的双手亲自揭开它的什么感,亲自制造出更高端、更便利的机械产品。
在入大学这一段时间内,我积极学习机械制图,在学习中爱上它,用自己的双手画出设计出自己喜欢的机械产品。
二、所学专业概机械设计制造及其自动化专业培养具备机械设计制造基础知识与应用能力,能在工业生产第一线从事机械制造领域内的设计制造、科技开发、应用研究、以机械设计与制造为基础,融入计算机科学、信息技术、自动控制技术的交叉学科,主要任务是运用先进设计制造技术的理论与方法,解决现代工程领域中的复杂技术问题,以实现产品智能化的设计与制造,运行管理和经营销售等方面工作的高级工程技术人才。
《人工智能》课程教学大纲(本科)
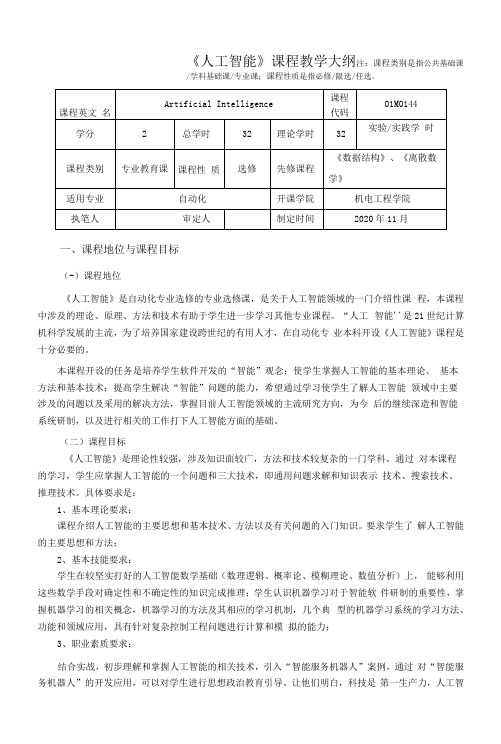
《人工智能》课程教学大纲注:课程类别是指公共基础课/学科基础课/专业课;课程性质是指必修/限选/任选。
一、课程地位与课程目标(-)课程地位《人工智能》是自动化专业选修的专业选修课,是关于人工智能领域的一门介绍性课程,本课程中涉及的理论、原理、方法和技术有助于学生进一步学习其他专业课程。
“人工智能''是21世纪计算机科学发展的主流,为了培养国家建设跨世纪的有用人才,在自动化专业本科开设《人工智能》课程是十分必要的。
本课程开设的任务是培养学生软件开发的“智能”观念;使学生掌握人工智能的基本理论、基本方法和基本技术;提高学生解决“智能”问题的能力,希望通过学习使学生了解人工智能领域中主要涉及的问题以及采用的解决方法,掌握目前人工智能领域的主流研究方向,为今后的继续深造和智能系统研制,以及进行相关的工作打下人工智能方面的基础。
(二)课程目标《人工智能》是理论性较强,涉及知识面较广,方法和技术较复杂的一门学科。
通过对本课程的学习,学生应掌握人工智能的一个问题和三大技术,即通用问题求解和知识表示技术、搜索技术、推理技术。
具体要求是:1、基本理论要求:课程介绍人工智能的主要思想和基本技术、方法以及有关问题的入门知识。
要求学生了解人工智能的主要思想和方法;2、基本技能要求:学生在较坚实打好的人工智能数学基础(数理逻辑、概率论、模糊理论、数值分析)上,能够利用这些数学手段对确定性和不确定性的知识完成推理;学生认识机器学习对于智能软件研制的重要性,掌握机器学习的相关概念,机器学习的方法及其相应的学习机制,几个典型的机器学习系统的学习方法、功能和领域应用,具有针对复杂控制工程问题进行计算和模拟的能力;3、职业素质要求:结合实战,初步理解和掌握人工智能的相关技术,引入“智能服务机器人”案例,通过对“智能服务机器人”的开发应用,可以对学生进行思想政治教育引导。
让他们明白,科技是第一生产力,人工智能作为一个关键技术,会影响一个国家的格局和国际竞争力。
《人工智能》-第八章 机器人规划
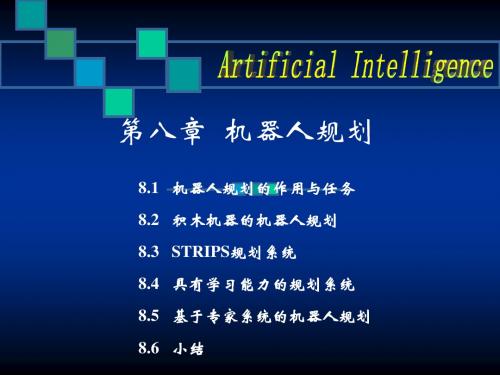
HANDEMPTY:机械手为空手。
中南大学 智能系统与智能软件研究所
7
8.2 积木世界的机器人规划 机械手
B
C A
A B C
机械手
(a)初始布局
(b)目标布局
图8.4 积木世界的机器人问题
图8.4(a)所示为初始布局的机器人问题。 目标在于建立一个积木堆,其中,积木B堆在积木 C上面,积木 A又堆在积木B上面,如图8.4(b)所示。
在这个例子中机器人能够执行的动作举例如下: unstack(a,b):把堆放在积木b上的积木a拾起。在进行这
个动作之前,要求机器人的手为空手,而且积木a的顶上 是空的。 stack(a,b):把积木a堆放在积木b上。动作之前要求机械 手必须已抓住积木a,而且积木b顶上必须是空的。 pickup(a):从桌面上拾起积木a,并抓住它不放。在动 作之前要求机械手为空手,而且积木a顶上没有任何东西。 putdown(a):把积木a放置到桌面上。要求动作之前机械 手已抓住积木a。
中南大学 智能系统与智能软件研究所
23
问题分解途径及方法
把某些比较复杂的问题分解为一些比较小的子问 题。有两条能够实现这种分解的重要途径。 第一条重要途径是当从一个问题状态移动到下一 个状态时,无需计算整个新的状态,而只要考虑 状态中可能变化了的那些部分。 第二条重要途径是把单一的困难问题分割为几个 有希望的较为容易解决的子问题。
22
8.6 小结
本章在说明了机器人规划的作用和任务后,主要 讨论了几种机器人规划的方法:
规划演绎法。 逻辑演算和通用搜索法 具有学习能力的规划系统 基于专家系统的规划
值得指出:第一,机器人规划已发展为综合应用 多种方法的规划。第二,机器人规划方法已广泛 应用于图象处理、计算机视觉等各领域。第三, 机器人规划尚有一些进一步研究的问题,如多机 器人协调规划和实时规划等。
- 1、下载文档前请自行甄别文档内容的完整性,平台不提供额外的编辑、内容补充、找答案等附加服务。
- 2、"仅部分预览"的文档,不可在线预览部分如存在完整性等问题,可反馈申请退款(可完整预览的文档不适用该条件!)。
- 3、如文档侵犯您的权益,请联系客服反馈,我们会尽快为您处理(人工客服工作时间:9:00-18:30)。
中南大学 智能系统与智能软件研究所
move(X,Y,Z)
CLEAR(X) CLEAR(Y) ON(X,Z) ONTABLE(Y) ONTABLE(z) 11
图8.5 表示move动作的搜索树
8.2 积木世界的机器人规划
move(x,y,z):把物体x从物体y上面移到物体z上面。 先决条件:CLEAR(x), CLEAR(z),ON(x,y) 删除表: ON(x,z), CLEAR(z) 添加表: ON(x,z), CLEAR(y)
如果move为此机器人仅有的操作符或适用动作,那
么,可以生成如图8.5所示的搜索图或搜索树。
22
8.6 小结
本章在说明了机器人规划的作用和任务后,主要 讨论了几种机器人规划的方法:
规划演绎法。 逻辑演算和通用搜索法 具有学习能力的规划系统 基于专家系统的规划
值得指出:第一,机器人规划已发展为综合应用 多种方法的规划。第二,机器人规划方法已广泛 应用于图象处理、计算机视觉等各领域。第三, 机器人规划尚有一些进一步研究的问题,如多机 器人协调规划和实时规划等。
中南大学 智能系统与智能软件研究所
6
8.2 积木世界的机器人规划
研究内容是综合机器人的动作序列,即在某个给定 初始情况下,经过某个动作序列而达到指定的目标。 机器人问题的状态描述和目标描述均可用谓词逻辑 公式构成:
ON(a,b):积木a在积木b之上。
ONTABLE(a):积木a在桌面上。 CLEAR(a):积木a顶上没有任何东西。
中南大学 智能系统与智能软件研究所
23
人有了知识,就会具备各种分析能力, 明辨是非的能力。 所以我们要勤恳读书,广泛阅读, 古人说“书中自有黄金屋。 ”通过阅读科技书籍,我们能丰富知识, 培养逻辑思维能力; 通过阅读文学作品,我们能提高文学鉴赏水平, 培养文学情趣; 通过阅读报刊,我们能增长见识,扩大自己的知识面。 有许多书籍还能培养我们的道德情操, 给我们巨大的精神力量, 鼓舞我们前进。
中南大学 智能系统与智能软件研究所
15
8.4 具有学习能力的规划系统
8.4.1 PULP-Ⅰ系统的结构与操作方式
PULP-Ⅰ系统的结构
字典 输入 SENEF 程序 模型 过程 方块
响应 “字典”是英语词 图 8.14 PULP-Ⅰ系统的总体结构 汇的集合。 “模型”部分包括模型世界 物体现有状态的事实。 “过程”集中了予先准备好的过程知识。 “方块”集中了LISP程序,它配合“规划”对“模型” 进行搜索和修正。
中南大学 智能系统与智能软件研究所
8
8.2 积木世界的机器人规划
初始布局可由下列谓词公式的合取来表示:
CLEAR(B):积木B顶部为空 CLEAR(C):积木C顶部为空 ON(C,A):积木C堆在积木A上 ONTABLE(A):积木A置于桌面上
ONTABLE(B):积木B置于桌面上
HANDEMPTY:机械手为空手
操作方法:应用状态空间表示和中间-结局分析。
中南大学 智能系统与智能软件研究所
14
8.4 具有学习能力的规划系统
PULP-Ⅰ机器人规划系统 PULP-Ⅰ机器人规划系统是一种具有学习能力的 系统,它采用管理式学习,其作用原理是建立在类 比(analogue)的基础上的。 STRIPS的弱点 需要极其大量计算机内存和时间等。 应用具有学习能力的规划系统能够克服这一缺点。
工作日规划 上午子规划 去上班 阅读文章 中午子规划 吃夹心面包 下午子规划 阅读 写作 回家
2
中南大学 智能系统与智能软件研究所
图8.1 子规划的分层结构例子
8.1自动规划的作用与任务
规划的作用
规划可用来监控问题求解过程,并能够在造成较 大的危害之前发现差错。规划的好处可归纳为简化 搜索、解决目标矛盾以及为差错补偿提供基础。
20
8.5 基于专家系统的机器人规划
基于规划的机器人规划专家系统由5个部分组成,
如图8.20所示。 知识库 控制策略 推理机 知识获取 解释与说明
基于规则的专家系统的目标就是要通过逐条执行
规则及其有关操作来逐步地改变总数据库的状况, 直到得到一个可接受的数据库(称为目标数据库)为 止。
输入命令
规划
响应
图8.16 规划方式下PULP-Ⅰ系统的结构
中南大学 智能系统与智能软件研究所
18
8.5 基于专家系统的机器人规划
机器人规划专家系统就是用专家系统的结构和技 术建立起来的机器人规划系统。
管理式学习能力的机器人规划系统的不足:
表达子句的语义网络结构过于 复杂。 与复杂的系统内部数据结构有关的是, PULP-Ⅰ系统具有许多子系统。而且需要 花费大量时间来编写程序。 尽管PULP-Ⅰ系统的执行速度要比 STRIPS 系列快得多,然而它仍然不够快。
中南大学 智能系统与智能软件研究所
13
8.3 STRIPS规划系统
STRIPS的介绍
STRIPS是由Fikes、Hart和Nilsson3人在1981及 1982研究成功的,它是夏凯(Shakey)机器人程序控制 系统的一个组成部分。
STRIPS系统组成
世界模型:为一阶谓词演算公式; 操作符(F规则):包括先决条件、删除表和添加表;
问题分解途径及方法
把某些比较复杂的问题分解为一些比较小的子问 题。有两条能够实现这种分解的重要途径。 第一条重要途径是当从一个问题状态移动到下一 个状态时,无需计算整个新的状态,而只要考虑 状态中可能变化了的那些部分。 第二条重要途径是把单一的困难问题分割为几个 有希望的较为容易解决的子问题。
中南大学 智能系统与智能软件研究所
12
8.2 积木世界的机器人规划
从初始状态开始,正向地依次读出连接弧线上 的F规则,我们就得到一个能够达到目标状态的动作 序列(如图8.6中粗线所示)于下: {unstack(C,A), putdown(C), pickup(B), stack(B,C), pickup(A), stack(A,B)} 就把这个动作序列叫做达到这个积木世界机器 人问题目标的规划。
在这个例子中机器人能够执行的动作举例如下: unstack(a,b):把堆放在积木b上的积木a拾起。在进行这
个动作之前,要求机器人的手为空手,而且积木a的顶上 是空的。 stack(a,b):把积木a堆放在积木b上。动作之前要求机械 手必须已抓住积木a,而且积木b顶上必须是空的。 pickup(a):从桌面上拾起积木a,并抓住它不放。在动 作之前要求机械手为空手,而且积木a顶上没有任何东西。 putdown(a):把积木a放置到桌面上。要求动作之前机械 手已抓住积木a。
中南大学 智能系统与智能软件研究所
4
8.2 积木世界的机器人规划
8.2.1 积木世界的机器人问题
积木世界由一些有标记 的立方形积木,互相堆 迭在一起构成;机器人 有个可移动的机械手, 它可以抓起积木块并移 动积木从一处至另一处。
中南大学 智能系统与智能软件研究所
机械手
C B A
5
8.2 积木世界的机器人规划
中南大学 智能系统与智能软件研究所
9
8.2 积木世界的机器人规划
8.2.2 用F规则求解规划序列
STRIPS规划系统的规则由3部分组成:
第一部分是先决条件。 第二部分是一个叫做删除表的谓词。
第三部分叫做添加表。
中南大学 智能系统与智能软件研究所
10
8.2 积木世界的机器人规划
例:表示堆积木的例子中move这个动作,如下所示:
19
中南大学 智能系统与智能软件研究所
8.5.1系统结构和规划机理
系统结构及规划机理
工具 专家系统 知识获取
机 问题输入或 新情况描述 用 器 户 人 接 回答问题或 用 建议与解释 口 户
知识库
控制 策略 推理机
机 器 人 专 家 说明与分析 知 识
图8.20 机器人规划专家系统的结构
中南大学 研究所
规划与学习
16
8.4 具有学习能力的规划系统
PULP-Ⅰ系统的操作方式
PULP-Ⅰ系统具有两种操作方式: 学习方式 在学习方式下,输入 至系统的知识是由操作人 员或者所谓“教师”提供 的。图8.15表示出在学习 方式下的系统操作。
中南大学 智能系统与智能软件研究所
机器人的4个动作(或操作符)可用STRIPS形式表示如下: stack(X,Y) 先决条件和删除表: HOLDING(X)∧CLEAR(Y) 添加表: HANDEMPTY,ON(X,Y) unstack(X,Y) 先决条件: HANDEMPTY∧ON(X,Y)∧CLEAR(X) 删除表: ON(X,Y),HANDEMPTY 添加表: HOLDING(X),CLEAR(Y) pickup(X) 先决条件:ONTABLE(X)∧CLEAR(X)∧HANDEMPTY 删除表: ONTABLE(X)∧HANDENPTY 添加表: HOLDING(X) putdown(X) 先决条件和删除表: HOLDING(X) 添加表: ONTABLE(X),HANDEMPTY
中南大学 智能系统与智能软件研究所
3
8.1自动规划的作用与任务
8.1.2 机器人规划系统的任务与方法
在规划系统中,必须具有执行下列各项任务的方法: 根据最有效的启发信息,选择应用于下一步的最好规则。 应用所选取的规则来计算由于应用该规则而生成的新状
态。 对所求得的解答进行检验。 检验空端,以便舍弃它们,使系统的求解工作向着更有 效的方向进行。 检验殆正确的解答,并应用具体的技术使之完全正确。
中南大学 智能系统与智能软件研究所