单细胞基因测序
单细胞测序技术

单细胞测序技术单细胞测序技术是一项用于研究和识别单个细胞基因表达特征的先进技术。
通过单细胞测序,我们可以深入了解细胞之间的异质性,揭示细胞发育、功能和疾病发生等重要过程中的细胞类型和状态的差异。
本文将对单细胞测序技术的原理、应用和未来发展进行简要介绍。
单细胞测序技术的原理是将每个单个细胞分离并进行单独处理。
首先,使用一种特殊的方法将细胞分离成单个个体。
然后,通过开发出不同种类的单细胞测序技术,我们可以对每个细胞的基因表达进行测定。
这些测定包括了细胞DNA或RNA的测序,可以帮助我们研究细胞之间的基因调控变化。
单细胞测序技术的一个重要应用是在细胞组学研究中。
通过对各种类型的细胞进行全面的基因表达测定,我们可以更好地了解细胞类型的多样性和功能特性。
通过这种方法,研究人员可以更深入地研究发育过程中不同细胞类型的转录调控网络,揭示细胞发育和分化的分子机制。
此外,单细胞测序技术也有助于我们深入了解疾病的发生和发展机制。
通过对单个细胞的基因表达进行测定,我们可以发现疾病中细胞类型和状态的改变,揭示疾病发生的潜在机制和标志物。
这对于研究疾病的发展途径、发现新的治疗靶点以及开发个性化医学有重要意义。
随着单细胞测序技术的不断发展,越来越多的应用领域将受益于这项技术。
例如,单细胞测序技术在神经科学研究中的应用,可以帮助我们了解大脑中不同神经元亚型的功能和相互作用。
同时,在肿瘤学中,单细胞测序技术可以帮助我们研究肿瘤细胞异质性和治疗抵抗性,为开发个性化肿瘤治疗方案提供新的思路。
尽管单细胞测序技术具有很多潜力和应用前景,但是它也存在着一些挑战。
首先,单细胞的处理和测序过程相对复杂,需要使用高度敏感的技术和设备。
此外,由于细胞的数量和数据量的增加,数据分析的复杂性也大大增加。
因此,我们需要发展更高效和准确的数据处理和分析方法,以便从大量的细胞数据中提取有用的信息。
在未来,单细胞测序技术将继续发展和创新。
我们可以预见,随着技术的不断进步,单细胞测序的分辨率将进一步提高,从而能够更好地解析细胞内基因调控的变化。
单细胞基因测序技术

单细胞基因测序技术单细胞基因测序技术是近年来发展迅速的生物技术领域中的热门研究方向。
它的出现使得科学家们能够深入了解单个细胞的基因组信息及其在生物体中的作用,为精准医学和生物学研究提供了重要的工具。
本文将对单细胞基因测序技术进行详细解读,从技术原理、应用领域到发展趋势进行全面分析。
一、单细胞基因测序技术的原理和方法1. 原理单细胞基因测序技术是一种能够对单个细胞进行基因组测序的方法。
其原理是通过分离单个细胞,并将其DNA进行放大扩增,然后进行测序分析。
由于单细胞存在于复杂的细胞组织中,因此在分离和扩增过程中需要克服一些技术难题,如单细胞损伤、污染等问题。
2. 方法目前常用的单细胞基因测序技术包括单细胞RNA测序(scRNA-seq)、单细胞DNA测序(scDNA-seq)等。
scRNA-seq能够分析单个细胞的转录组信息,揭示细胞类型和功能的差异;而scDNA-seq则能够对单个细胞的基因组进行测序,探究遗传变异的发生和影响。
二、单细胞基因测序技术的应用领域1. 癌症研究单细胞基因测序技术在癌症研究中具有重要价值。
通过对肿瘤细胞进行单细胞基因测序可以揭示肿瘤内部的遗传变异和克隆演化过程,有助于精准化治疗策略的制定。
2. 免疫学研究单细胞基因测序技术可以帮助科学家们深入了解免疫细胞的转录组和表观组学特征,从而揭示不同类型免疫细胞的功能和相互作用,为免疫相关疾病的治疗提供理论支持。
3. 胚胎学研究在胚胎发育过程中,单细胞基因测序技术可以追踪细胞的分化过程,揭示胚胎细胞谱系发展的规律和机制,有助于揭示胚胎发育的分子调控网络。
三、单细胞基因测序技术的发展趋势1. 技术趋势随着测序技术的不断进步和成本的不断降低,单细胞基因测序技术将更加快速、准确、经济,同时提高数据量和分辨率。
2. 数据分析趋势随着单细胞测序数据的不断增加,数据分析方法也在不断完善,包括单细胞测序数据的质控、批次效应的消除、细胞类型的鉴别等。
单细胞测序前世今生 原理
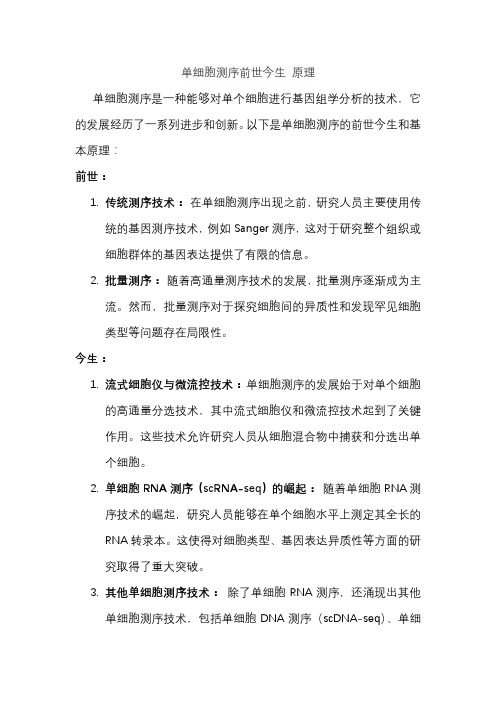
单细胞测序前世今生原理单细胞测序是一种能够对单个细胞进行基因组学分析的技术,它的发展经历了一系列进步和创新。
以下是单细胞测序的前世今生和基本原理:前世:1.传统测序技术:在单细胞测序出现之前,研究人员主要使用传统的基因测序技术,例如Sanger测序,这对于研究整个组织或细胞群体的基因表达提供了有限的信息。
2.批量测序:随着高通量测序技术的发展,批量测序逐渐成为主流。
然而,批量测序对于探究细胞间的异质性和发现罕见细胞类型等问题存在局限性。
今生:1.流式细胞仪与微流控技术:单细胞测序的发展始于对单个细胞的高通量分选技术,其中流式细胞仪和微流控技术起到了关键作用。
这些技术允许研究人员从细胞混合物中捕获和分选出单个细胞。
2.单细胞RNA测序(scRNA-seq)的崛起:随着单细胞RNA测序技术的崛起,研究人员能够在单个细胞水平上测定其全长的RNA转录本。
这使得对细胞类型、基因表达异质性等方面的研究取得了重大突破。
3.其他单细胞测序技术:除了单细胞RNA测序,还涌现出其他单细胞测序技术,包括单细胞DNA测序(scDNA-seq)、单细胞蛋白质测序(CITE-seq、REAP-seq)等,这些技术使得研究人员能够全面了解单个细胞的遗传信息和表观信息。
基本原理:1.单细胞分选:使用流式细胞仪或微流控技术对单个细胞进行高通量的分选和捕获。
2.细胞溶解与RNA扩增:对单个细胞进行细胞溶解,获取其RNA。
随后,通过反转录和扩增等步骤,将单细胞中的RNA扩增为足够的量进行测序。
3.测序:对扩增后的RNA进行高通量测序,得到大量的RNA序列信息。
4.数据分析:利用生物信息学工具对得到的数据进行分析,包括细胞类型鉴定、基因表达分析、细胞群体结构等。
单细胞测序技术的发展使得研究人员能够更全面、深入地理解单个细胞的生物学特性,为生命科学、医学研究等领域提供了强大的工具。
Nature:单细胞基因组测序

个体化医疗与精准诊断
个体化医疗
单细胞基因组测序可以揭示个体之间 的基因表达和变异差异,有助于实现 个体化医疗,为患者提供更加精准和 有效的治疗方案。
精准诊断
通过对患者的单细胞基因组数据进行 检测和分析,可以更加准确地诊断疾 病,提高诊断的准确性和可靠性。
药物研发与基因治疗
药物研发
单细胞基因组测序可以研究药物的基 因表达和变异效应,有助于发现新的 药物靶点和筛选出更有效的药物。
秘。
药物研发
单细胞测序技术将有助于更 快速、准确地筛选和开发新 药,降低药物研发成本和时 间。
THANKS
谢谢
通量
一次测序能够分析大量单细胞样本,具有高 通量。
02
CHAPTER
自然领域中的应用
物种进化研究
物种进化研究
单细胞基因组测序可以用于研究 物种的进化历程,通过比较不同 物种的单细胞基因组数据,可以 揭示物种之间的亲缘关系和进化 趋势。
物种分类
单细胞基因组测序可以提供更准 确的物种分类依据,通过对基因 组的比较和分析,可以更准确地 鉴定和分类物种。
技术发展历程
初始阶段
2009年,科学家首次实现了单细胞全基因组测 序。
进展阶段
随着技术的不断改进,单细胞测序的通量、分 辨率和灵敏度逐渐提高。
当前阶段
单细胞测序已经成为研究细胞异质性和基因表达的重要工具。
关键技术指标
分辨率
单细胞测序能够检测到单个细胞的基因表达 和变异情况,具有高分辨率。
灵敏度
能够检测到低丰度的基因表达和稀有变异, 具有高灵敏度。
02
疾病分类与诊断
03
药物筛选与作用机制
单细胞基因组测序可以揭示单个细胞 的基因表达和变异情况,有助于深入 了解疾病的发生机制,为疾病的预防 、诊断和治疗提供科学依据。
单细胞测序技术流程及其应用

单细胞测序技术流程及其应用单细胞测序技术是一种用于研究单个细胞基因组的高通量技术,可以帮助科研人员深入了解生物体内不同细胞之间的异质性和功能差异。
本文将介绍单细胞测序技术的流程和应用,并讨论其在生物医学研究、临床诊断和药物研发中的潜在应用价值。
一、单细胞测序技术流程1. 细胞样本的获取单细胞测序技术的第一步是获取待测细胞样本。
这些样本可以来自于组织、血液、脑脊液等身体部位,也可以来自于细胞培养物。
为了保证测序结果的准确性和代表性,科研人员需要尽量避免细胞样本的质量受到损害,避免污染其他非目标细胞的成分。
2. 单细胞的分离在获得细胞样本之后,科研人员需要利用细胞分离技术将单个细胞从混合细胞群中分离出来。
传统的细胞分离方法包括流式细胞术和荧光激活细胞分选技术,近年来也出现了微流控芯片技术和微流控钳技术等新型分离方法。
3. 单细胞的溶解和RNA提取分离出的单个细胞需要被溶解,并且提取RNA。
由于单细胞中RNA的含量非常少,因此科研人员需要运用高效的RNA提取技术,确保从单个细胞中提取到足够数量的RNA,并且不受到外源RNA的污染。
4. RNA的反转录和文库构建提取的RNA需要通过反转录酶进行反转录,合成成为cDNA。
之后,科研人员需要进行cDNA的文库构建,包括末端修复、连接连接子、文库扩增等步骤。
在这一步骤中,科研人员需要特别注意避免样本之间的混杂和文库构建过程中的错误。
5. 测序平台的选择与测序运行文库构建完成后,科研人员需要选择合适的测序平台进行测序运行。
常见的测序平台包括Illumina平台、Ion Torrent平台、PacBio平台等。
不同的平台具有不同的测序原理和测序效果,因此在选择平台时需要根据实际情况进行综合考量。
6. 数据分析与挖掘得到的测序数据需要进行严格的质控和数据分析,以确定单细胞的基因表达模式、转录组特征以及细胞间的差异。
在这一步骤中,科研人员需要借助生物信息学分析工具,对大量的数据进行筛选、过滤和整合,最终得出科学合理的结论。
单细胞基因测序技术

单细胞基因测序技术单细胞基因测序技术是一种先进的生物学技术,它能够对单个细胞进行基因测序,从而获取细胞水平的基因表达和变异信息。
该技术广泛应用于基础研究和临床诊断领域,如发育生物学、肿瘤研究、免疫学等。
下面将详细介绍单细胞基因测序技术的四个方面。
1.单细胞样本制备单细胞基因测序技术的第一步是单细胞样本制备。
该步骤主要包括细胞选取、细胞分散和细胞裂解等。
选取的细胞可以是新鲜的组织样本,也可以是经过培养的细胞。
在选取细胞的过程中,需要使用显微镜等设备,确保选取单个细胞。
然后将选取的细胞分散成单个细胞,并裂解以释放其内部的核酸。
2.建库和测序在单细胞基因测序技术中,建库和测序是关键步骤。
在建库阶段,从单细胞样本中提取的核酸被转化为适合测序的文库。
这个过程包括对核酸进行片段化、添加测序接头、进行PCR扩增等操作。
在测序阶段,文库中的核酸片段被序列化并产生测序数据。
目前常用的测序技术包括第二代测序技术和第三代测序技术。
3.数据分析和解读单细胞基因测序技术产生的数据需要进行深入的分析和解读。
这个过程包括对测序数据进行质控、比对和聚类等操作。
通过对数据的分析,可以获取每个细胞的基因表达情况、变异信息以及细胞间的差异等。
通过对这些数据进行分析,可以揭示细胞在分子水平上的行为和变化,为生物学研究和临床诊断提供有力支持。
4.应用领域单细胞基因测序技术的应用领域非常广泛。
在基础研究领域,该技术可以用于研究胚胎发育、肿瘤发生发展、免疫应答等生物学过程。
通过单细胞基因测序技术,科学家们可以追踪单个细胞的基因表达和变异情况,深入探究细胞间的差异和分化路径。
此外,单细胞基因测序技术还可以用于临床诊断,如无创产前诊断、肿瘤诊断和预后评估等。
通过该技术可以获取患者单个细胞的基因表达和变异信息,为疾病的早期发现和治疗提供依据。
总之,单细胞基因测序技术是一种强大的生物学工具,它能够提供单个细胞的基因表达和变异信息,为生物学研究和临床诊断提供有力支持。
单细胞测序t细胞亚型分类

单细胞测序t细胞亚型分类
单细胞测序是一种高通量的基因组学技术,可以用来研究单个
细胞的基因表达模式。
在研究T细胞亚型分类时,单细胞测序可以
帮助识别和分类不同类型的T细胞亚型,从而深入了解它们在免疫
系统中的功能和作用。
首先,单细胞测序可以通过分析单个T细胞的基因表达谱来确
定它们的亚型。
T细胞可以分为多个亚型,如辅助T细胞(Th细胞)、细胞毒性T细胞(Tc细胞)和调节性T细胞(Treg细胞)等。
通过单细胞测序,可以识别并区分这些不同的亚型,从而了解它们
在免疫反应中的不同作用。
其次,单细胞测序还可以帮助发现新的T细胞亚型。
通过对大
量单个T细胞的基因表达数据进行聚类和分析,可以发现一些新的、以往未被发现的T细胞亚型。
这些新的亚型可能具有特定的免疫调
节功能,对于理解免疫系统的调节机制具有重要意义。
此外,单细胞测序还可以揭示T细胞亚型在疾病发展和治疗中
的作用。
通过比较健康人和患病个体中T细胞亚型的差异,可以发
现一些与疾病发展相关的T细胞亚型变化,为疾病的诊断和治疗提
供新的线索。
总的来说,单细胞测序在T细胞亚型分类中具有重要的应用前景,可以帮助我们更全面地了解T细胞的多样性和功能,为免疫系统的研究和临床应用提供重要的支持。
单细胞基因测序技术
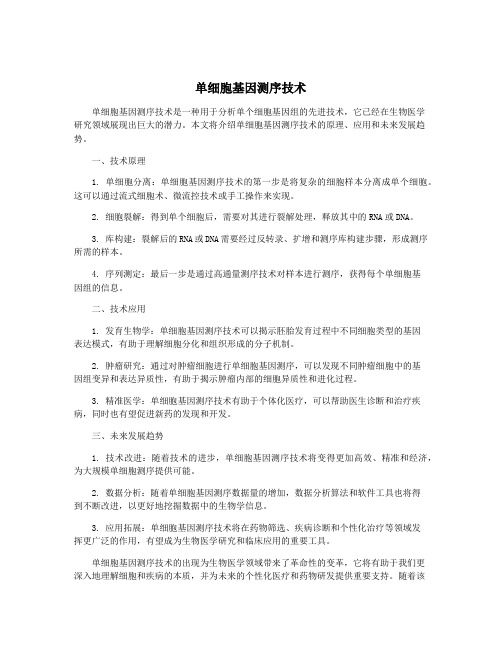
单细胞基因测序技术单细胞基因测序技术是一种用于分析单个细胞基因组的先进技术,它已经在生物医学研究领域展现出巨大的潜力。
本文将介绍单细胞基因测序技术的原理、应用和未来发展趋势。
一、技术原理1. 单细胞分离:单细胞基因测序技术的第一步是将复杂的细胞样本分离成单个细胞。
这可以通过流式细胞术、微流控技术或手工操作来实现。
2. 细胞裂解:得到单个细胞后,需要对其进行裂解处理,释放其中的RNA或DNA。
3. 库构建:裂解后的RNA或DNA需要经过反转录、扩增和测序库构建步骤,形成测序所需的样本。
4. 序列测定:最后一步是通过高通量测序技术对样本进行测序,获得每个单细胞基因组的信息。
二、技术应用1. 发育生物学:单细胞基因测序技术可以揭示胚胎发育过程中不同细胞类型的基因表达模式,有助于理解细胞分化和组织形成的分子机制。
2. 肿瘤研究:通过对肿瘤细胞进行单细胞基因测序,可以发现不同肿瘤细胞中的基因组变异和表达异质性,有助于揭示肿瘤内部的细胞异质性和进化过程。
3. 精准医学:单细胞基因测序技术有助于个体化医疗,可以帮助医生诊断和治疗疾病,同时也有望促进新药的发现和开发。
三、未来发展趋势1. 技术改进:随着技术的进步,单细胞基因测序技术将变得更加高效、精准和经济,为大规模单细胞测序提供可能。
2. 数据分析:随着单细胞基因测序数据量的增加,数据分析算法和软件工具也将得到不断改进,以更好地挖掘数据中的生物学信息。
3. 应用拓展:单细胞基因测序技术将在药物筛选、疾病诊断和个性化治疗等领域发挥更广泛的作用,有望成为生物医学研究和临床应用的重要工具。
单细胞基因测序技术的出现为生物医学领域带来了革命性的变革,它将有助于我们更深入地理解细胞和疾病的本质,并为未来的个性化医疗和药物研发提供重要支持。
随着该技术的不断发展和应用,相信它将在未来的生物医学研究和临床实践中发挥越来越重要的作用。
单细胞测序技术课件
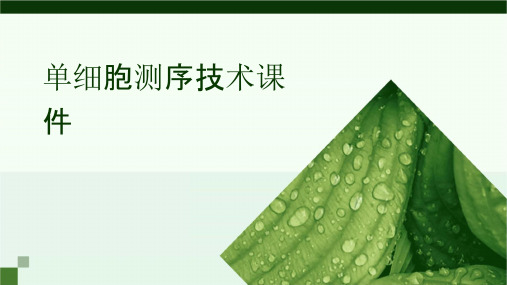
逆转录与cDNA合成 使用逆转录酶将建原理
将逆转录合成的cDNA进行一 录
• 单细胞测序技术概述 • 单细胞测序技术原理 • 单细胞测序实验设计 • 单细胞测序数据分析 • 单细胞测序技术的应用案例 • 单细胞测序技术的未来发展与挑战
contents
01
单细胞测序技术概述
定义与特点
定义
单细胞测序技术是一种高通量的分子 生物学技术,可以对单个细胞进行基 因组、转录组或表观组测序,以揭示 进行酶切、连接和文 库构建,以便后续的测序 分析。
技术优势与局限性
优势
能够对单个细胞进行基因组或转录组 分析,分辨率高,能够揭示细胞异质 性。
局限性
由于技术复杂度高,成本较高,且存 在一定的误差率。
03
单细胞测序实验设计
实验准备
确定研究目标
在开始单细胞测序实验前,需要明确研究目标,例如鉴定特定组 织或疾病中的细胞类型、分析细胞发育过程等。
预测模型构建
基于单细胞测序数据,构建预测模型,用于 疾病诊断、药物筛选和个性化治疗等。
技术伦理与法规问题
数据隐私保护
确保单细胞测序数据的隐私保护,防止数据泄露和 滥用。
伦理审查与知情同意
建立严格的伦理审查机制,确保单细胞测序技术的 合理使用和伦理规范。
法规监管
制定相关法规和政策,规范单细胞测序技术的研发 和应用,保障科技发展的安全和可控性。
应用领域
基础研究
01
用于揭示细胞发育、分化、功能和相互作用的机制,以及探索
疾病发生、发展和治疗的分子机制。
单细胞测序原始结果
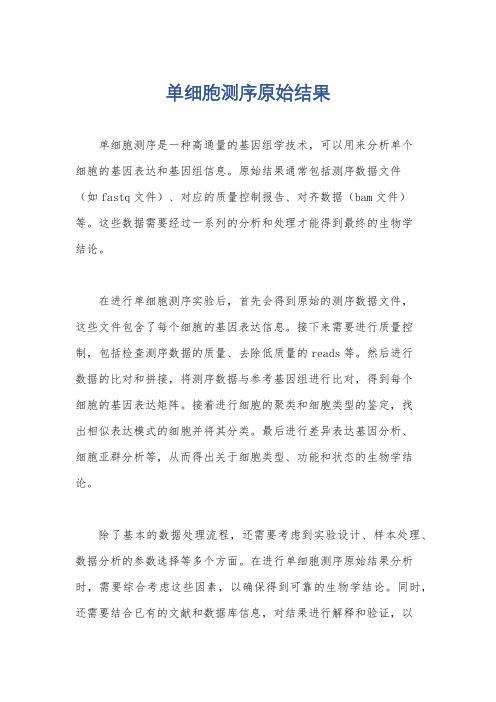
单细胞测序原始结果
单细胞测序是一种高通量的基因组学技术,可以用来分析单个
细胞的基因表达和基因组信息。
原始结果通常包括测序数据文件
(如fastq文件)、对应的质量控制报告、对齐数据(bam文件)等。
这些数据需要经过一系列的分析和处理才能得到最终的生物学
结论。
在进行单细胞测序实验后,首先会得到原始的测序数据文件,
这些文件包含了每个细胞的基因表达信息。
接下来需要进行质量控制,包括检查测序数据的质量、去除低质量的reads等。
然后进行
数据的比对和拼接,将测序数据与参考基因组进行比对,得到每个
细胞的基因表达矩阵。
接着进行细胞的聚类和细胞类型的鉴定,找
出相似表达模式的细胞并将其分类。
最后进行差异表达基因分析、
细胞亚群分析等,从而得出关于细胞类型、功能和状态的生物学结论。
除了基本的数据处理流程,还需要考虑到实验设计、样本处理、数据分析的参数选择等多个方面。
在进行单细胞测序原始结果分析时,需要综合考虑这些因素,以确保得到可靠的生物学结论。
同时,还需要结合已有的文献和数据库信息,对结果进行解释和验证,以
得出科学的结论。
总的来说,单细胞测序原始结果的分析需要综合考虑实验技术、生物学知识和数据处理方法,以得出可靠的结论。
单细胞测序技术相关知识点
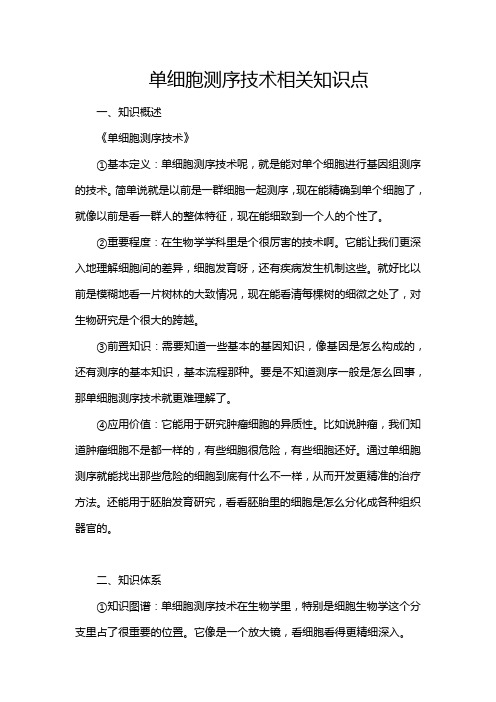
单细胞测序技术相关知识点一、知识概述《单细胞测序技术》①基本定义:单细胞测序技术呢,就是能对单个细胞进行基因组测序的技术。
简单说就是以前是一群细胞一起测序,现在能精确到单个细胞了,就像以前是看一群人的整体特征,现在能细致到一个人的个性了。
②重要程度:在生物学学科里是个很厉害的技术啊。
它能让我们更深入地理解细胞间的差异,细胞发育呀,还有疾病发生机制这些。
就好比以前是模糊地看一片树林的大致情况,现在能看清每棵树的细微之处了,对生物研究是个很大的跨越。
③前置知识:需要知道一些基本的基因知识,像基因是怎么构成的,还有测序的基本知识,基本流程那种。
要是不知道测序一般是怎么回事,那单细胞测序技术就更难理解了。
④应用价值:它能用于研究肿瘤细胞的异质性。
比如说肿瘤,我们知道肿瘤细胞不是都一样的,有些细胞很危险,有些细胞还好。
通过单细胞测序就能找出那些危险的细胞到底有什么不一样,从而开发更精准的治疗方法。
还能用于胚胎发育研究,看看胚胎里的细胞是怎么分化成各种组织器官的。
二、知识体系①知识图谱:单细胞测序技术在生物学里,特别是细胞生物学这个分支里占了很重要的位置。
它像是一个放大镜,看细胞看得更精细深入。
②关联知识:和基因编辑技术有关联,因为都涉及基因层面的操作研究。
也和细胞培养技术有关,毕竟得有细胞来源才能做单细胞测序。
③重难点分析:掌握难度嘛,说实话我觉得它概念理解不容易,因为涉及到很多微观的东西。
然后分析结果也不轻松,数据很多很复杂。
关键点就是准确地采集单细胞,还有很好地分析那些测序数据。
④考点分析:考试中要是考细胞相关的,挺重要的。
可能考查单细胞测序技术原理,或者给个细胞相关疾病的场景,问如何用单细胞测序技术解决问题。
三、详细讲解【理论概念类】①概念辨析:单细胞测序技术核心就是精确到单个细胞的基因测序,和传统测序相比,传统测序把细胞当一个整体测,它是一个一个来。
就像数一堆硬币,传统只能称出一堆的重量,单细胞测序能数清楚每个硬币。
单细胞测序实验方案

单细胞测序实验方案一、实验目的。
咱为啥要做单细胞测序呢?就是想看看单个细胞里面那些基因在搞啥小动作,每个细胞可都有自己的小秘密呢,这个实验就能把这些秘密挖出来。
二、实验材料准备。
# (一)样本。
1. 细胞来源。
首先得确定从哪儿弄细胞。
是从组织里分离呢,还是已经有培养好的细胞系。
比如说,要是研究肿瘤,就从肿瘤组织里把细胞小心地分离出来,这就像从一个复杂的小社会(肿瘤组织)里挑出一个个小居民(细胞)。
2. 样本数量。
那得准备多少细胞呢?这得看咱的研究有多“贪心”。
如果想研究得全面细致,那就多准备点,但是也不能太多,不然就像一群人挤在一个小房间里,乱套了。
一般来说,根据实验设计,几千个到几万个细胞可能就够了。
# (二)试剂。
1. 细胞裂解液。
2. 反转录试剂(如果做RNA Seq)它能把RNA这个小信使变成可以被测序仪读懂的DNA,就像把一种语言(RNA语言)翻译成另一种语言(DNA语言)。
3. 测序文库构建试剂。
这可是构建测序高速公路的材料。
没有它,咱就没法把细胞里的基因信息整整齐齐地送到测序仪那里去。
4. 单细胞捕获试剂(如果是单细胞分离捕获的方法)这个试剂就像一个个小陷阱,专门用来抓住单个细胞,可不能让细胞溜走了。
# (三)仪器设备。
1. 显微镜(如果需要手动挑选单细胞)这是咱的小眼睛,用来在细胞的小世界里找那些长得特别的细胞,就像在人群里找那个穿红衣服的人一样。
2. 离心机。
它是个大力士,能把细胞或者细胞里面的东西按照重量分开,重的在下面,轻的在上面。
3. PCR仪(如果有PCR步骤)这个就像一个复印机,能把少量的DNA或者RNA复制好多好多份,这样才有足够的量去测序。
4. 测序仪。
这是整个实验的大明星,它能把细胞的基因信息一个一个读出来,就像一个超级读书郎。
三、实验步骤。
# (一)单细胞分离。
1. 组织消化法(如果从组织开始)把组织切成小块,就像切菜一样。
然后放到含有消化酶的溶液里,让消化酶像小剪刀一样把细胞之间的连接剪断,这样细胞就一个个分开了。
单细胞测序技术及其在基因组学研究中的应用

单细胞测序技术及其在基因组学研究中的应用基因组学是研究生物体的全部基因组构成和基因功能调控的分支学科。
近年来,单细胞测序技术被广泛应用于基因组学研究中,能够解决常规测序技术所无法识别的罕见细胞亚群和个体差异等问题。
本文将介绍单细胞测序技术的原理和应用,并分析其在基因组学研究中的优势。
一、单细胞测序技术的原理单细胞测序技术是通过分离单个细胞,并在保证细胞完整性的前提下对其进行基因组、转录组或表观基因组测序。
常用的单细胞测序技术主要包括单细胞PCR和单细胞测序法两种。
1. 单细胞PCR技术单细胞PCR技术是通过微操纵系统将单个细胞的DNA或RNA扩增到数百万倍,然后对扩增产物进行常规的基因组测序或转录组测序,从而获得单个细胞的遗传信息。
该技术优点在于从单个细胞中可以扩增出大量的DNA或RNA,但存在扩增偏差和错配的问题。
2. 单细胞测序法单细胞测序法主要包括单细胞WGA、单细胞RNA-seq和单细胞ATAC-seq等多种方法。
其中,单细胞WGA技术通过DNA扩增的方式获得单细胞基因组全景图谱,单细胞RNA-seq技术则是通过RNA逆转录将单个细胞中的RNA转成cDNA,再进行测序。
单细胞ATAC-seq技术可以测定基因组上的开放染色质区域,从而确定单个细胞中的表观基因组测序。
二、单细胞测序技术在基因组学研究中的应用单细胞测序技术已经在多种生命科学领域得到广泛应用。
下面介绍一些常见的应用:1. 发现细胞亚群单细胞测序技术可以挖掘细胞种群中的不同亚群,例如癌细胞中的肿瘤干细胞亚群等。
这种分析有助于深入理解细胞生物学过程,并且有助于开发个性化治疗策略。
2. 探索细胞发育和分化单细胞测序可以揭示不同发育阶段的胚胎干细胞的分化过程,或者在特定治疗中的干细胞的不同反应,从而得到单个细胞生命过程中的遗传图谱,促进对生命的认识。
3. 发现个体差异单细胞测序技术可以准确检测出细胞水平的遗传变异,例如突变和重排等,从而确定细胞间的个体差异。
基因组学研究中的单细胞测序数据分析技巧
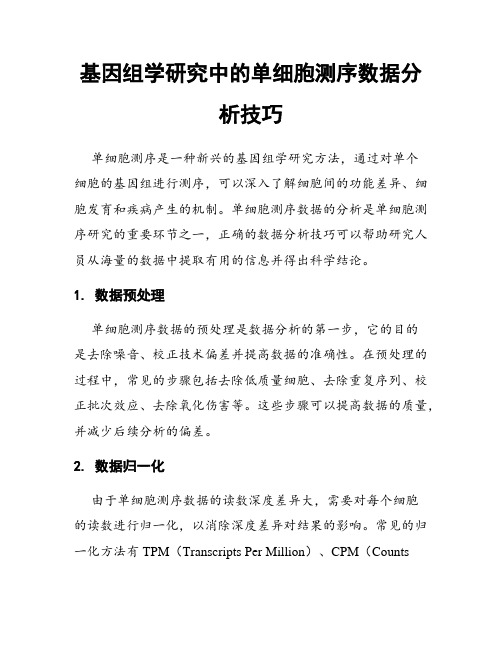
基因组学研究中的单细胞测序数据分析技巧单细胞测序是一种新兴的基因组学研究方法,通过对单个细胞的基因组进行测序,可以深入了解细胞间的功能差异、细胞发育和疾病产生的机制。
单细胞测序数据的分析是单细胞测序研究的重要环节之一,正确的数据分析技巧可以帮助研究人员从海量的数据中提取有用的信息并得出科学结论。
1. 数据预处理单细胞测序数据的预处理是数据分析的第一步,它的目的是去除噪音、校正技术偏差并提高数据的准确性。
在预处理的过程中,常见的步骤包括去除低质量细胞、去除重复序列、校正批次效应、去除氧化伤害等。
这些步骤可以提高数据的质量,并减少后续分析的偏差。
2. 数据归一化由于单细胞测序数据的读数深度差异大,需要对每个细胞的读数进行归一化,以消除深度差异对结果的影响。
常见的归一化方法有TPM(Transcripts Per Million)、CPM(CountsPer Million)和DESeq2等。
这些方法可以校正细胞间的差异,使得不同细胞之间的比较更加准确。
3. 细胞分类与聚类单细胞测序数据中含有多个细胞的信息,需要将这些细胞根据其基因表达模式进行分类和聚类。
常见的细胞分类和聚类方法有PCA(Principal Component Analysis)、t-SNE(t-distributed Stochastic Neighbor Embedding)和PCA与t-SNE结合等。
通过这些方法可以将具有相似基因表达模式的细胞聚集在一起,从而得到不同类型的细胞簇。
4. 基因差异表达分析单细胞测序数据分析中的一个关键问题是确定在不同细胞簇之间的差异表达基因。
常见的基因差异表达分析方法有DESeq2、edgeR和limma等。
通过这些方法可以确定哪些基因在不同细胞簇之间的表达量有显著差异,从而进一步了解不同细胞类型的功能和特征。
5. 基因调控网络分析单细胞测序数据可以用于构建细胞的基因调控网络,揭示细胞内基因表达的调控机制。
单细胞测序原理

单细胞测序原理单细胞测序是一种用于研究单个细胞基因组的技术,它可以揭示细胞间的功能和表达差异,帮助科学家更好地理解生物学系统的复杂性。
单细胞测序技术的原理主要包括细胞分离、细胞裂解、DNA 或RNA提取、建库、测序和数据分析等步骤。
首先,细胞分离是单细胞测序的第一步,它可以通过机械分离或荧光激活细胞分选等方法将单个细胞从混合细胞群中分离出来,确保每个细胞都可以被独立地进行测序。
接下来,细胞裂解是将细胞膜破裂,释放出细胞内的DNA或RNA。
这一步通常使用酶解法或化学法,将细胞内的核酸释放出来,为后续的提取工作做准备。
然后,DNA或RNA提取是将细胞内的DNA或RNA提取出来,以供后续的建库和测序。
提取方法可以根据实验需要选择不同的试剂盒或试剂进行操作。
建库是将提取得到的DNA或RNA进行文库构建,这一步主要包括末端修复、连接连接适配体、文库富集等步骤,以确保测序时能够得到高质量的测序数据。
测序是将建库后的样品进行高通量测序,以获取每个细胞的基因组序列信息。
目前常用的测序技术包括Illumina测序、PacBio 测序、Ion Torrent测序等,它们可以快速、准确地获取大量的基因组数据。
最后,数据分析是将测序得到的数据进行处理和分析,包括序列比对、基因表达分析、单细胞聚类、细胞轨迹分析等步骤,以挖掘出细胞间的功能和表达差异,从而揭示生物学系统的复杂性。
总的来说,单细胞测序技术的原理是通过一系列的步骤将单个细胞的基因组信息提取出来,并进行高通量测序和数据分析,以揭示细胞间的功能和表达差异,帮助科学家更好地理解生物学系统的复杂性。
这项技术的发展将为生物医学研究和临床诊断带来革命性的变革,有望在癌症治疗、干细胞研究、免疫学等领域发挥重要作用。
单细胞测序原理 概述及解释说明
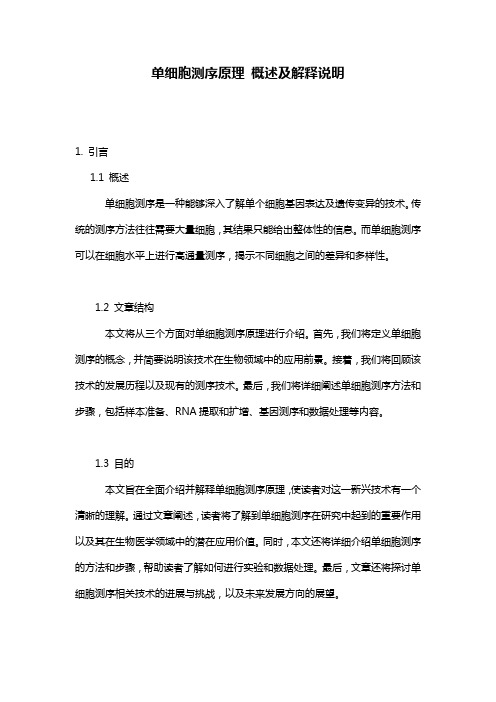
单细胞测序原理概述及解释说明1. 引言1.1 概述单细胞测序是一种能够深入了解单个细胞基因表达及遗传变异的技术。
传统的测序方法往往需要大量细胞,其结果只能给出整体性的信息。
而单细胞测序可以在细胞水平上进行高通量测序,揭示不同细胞之间的差异和多样性。
1.2 文章结构本文将从三个方面对单细胞测序原理进行介绍。
首先,我们将定义单细胞测序的概念,并简要说明该技术在生物领域中的应用前景。
接着,我们将回顾该技术的发展历程以及现有的测序技术。
最后,我们将详细阐述单细胞测序方法和步骤,包括样本准备、RNA提取和扩增、基因测序和数据处理等内容。
1.3 目的本文旨在全面介绍并解释单细胞测序原理,使读者对这一新兴技术有一个清晰的理解。
通过文章阐述,读者将了解到单细胞测序在研究中起到的重要作用以及其在生物医学领域中的潜在应用价值。
同时,本文还将详细介绍单细胞测序的方法和步骤,帮助读者了解如何进行实验和数据处理。
最后,文章还将探讨单细胞测序相关技术的进展与挑战,以及未来发展方向的展望。
2. 单细胞测序原理:2.1 单细胞测序的定义:单细胞测序是一种基因组学研究方法,它可以在单个细胞级别上分析其DNA或RNA的序列。
传统的基因测序方法通常是对大量细胞进行测序,从而获得平均表达水平或突变频率等整体信息。
与之相反,单细胞测序使我们能够了解每个单个细胞的遗传信息和功能特征,可以揭示不同细胞类型之间的异质性。
2.2 测序技术的发展历程:随着高通量测序技术的快速发展,单细胞测序也得到了长足地进展。
最初,单细胞测序主要使用PCR扩增来获得足够数量的DNA或RNA以进行后续测序分析。
然而,这种方法存在偏差和错误放大等问题。
近年来,出现了许多新兴的单细胞测序技术,如SMART-seq、MARS-seq、DroNc-seq和10x Genomics等。
这些技术利用微流控芯片、体积限制及其他改良步骤来提高PCR扩增效率和减少偏差。
2.3 单细胞测序的应用领域:单细胞测序广泛应用于生命科学的多个领域。
基因组学中单细胞测序技术与数据分析教程
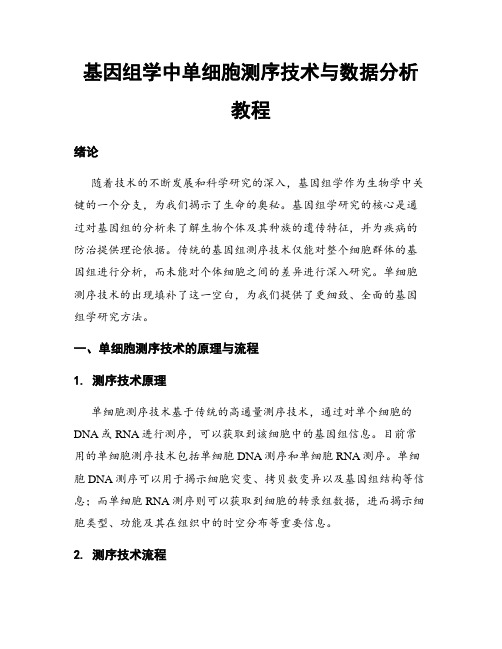
基因组学中单细胞测序技术与数据分析教程绪论随着技术的不断发展和科学研究的深入,基因组学作为生物学中关键的一个分支,为我们揭示了生命的奥秘。
基因组学研究的核心是通过对基因组的分析来了解生物个体及其种族的遗传特征,并为疾病的防治提供理论依据。
传统的基因组测序技术仅能对整个细胞群体的基因组进行分析,而未能对个体细胞之间的差异进行深入研究。
单细胞测序技术的出现填补了这一空白,为我们提供了更细致、全面的基因组学研究方法。
一、单细胞测序技术的原理与流程1. 测序技术原理单细胞测序技术基于传统的高通量测序技术,通过对单个细胞的DNA或RNA进行测序,可以获取到该细胞中的基因组信息。
目前常用的单细胞测序技术包括单细胞DNA测序和单细胞RNA测序。
单细胞DNA测序可以用于揭示细胞突变、拷贝数变异以及基因组结构等信息;而单细胞RNA测序则可以获取到细胞的转录组数据,进而揭示细胞类型、功能及其在组织中的时空分布等重要信息。
2. 测序技术流程单细胞测序技术的流程包括细胞样品的准备、细胞的捕获与分离、细胞的裂解与提取、DNA或RNA的扩增与测序、数据的质控和分析等步骤。
在细胞样品的准备中,需要选择适当的组织或细胞来源,并进行样品的准备与保存。
细胞的捕获与分离是单细胞测序的关键步骤,常用的方法包括流式细胞术、微流控排序和单细胞微操作技术等。
细胞的裂解与提取需要使用特定的方法来破解细胞,提取其中的DNA或RNA,并进行纯化。
扩增与测序则是通过PCR等方法来扩增并测序样品中的DNA或RNA,以获取基因组信息。
最后,对测得的数据进行质控和分析,可以了解到细胞的基因组结构、表达水平等信息。
二、单细胞测序数据分析教程1. 数据预处理在进行单细胞测序数据分析前,需要对原始数据进行预处理。
预处理流程包括数据清洗、去除低质量序列、去除重复序列、去除污染序列等环节,以确保后续分析的准确性。
常用的预处理工具包括FastQC、Trimmomatic等。
单细胞测序,取交集

单细胞测序,取交集
单细胞测序是一种高通量的基因组学技术,用于研究单个细胞的基因表达和基因组特征。
取交集通常是指在两个或多个数据集中找到共同的元素或特征。
在单细胞测序中,取交集可能涉及到不同细胞类型之间的共同基因表达特征,或者在不同实验条件下的共同基因表达模式。
从数据分析的角度来看,取交集可以帮助研究人员找到在不同样本或条件下共同存在的基因表达模式,有助于识别细胞类型的共同特征或者不同条件下的共同调控机制。
这有助于我们理解细胞的功能和特征,并且可以为疾病研究提供重要线索。
在实际操作中,取交集通常需要进行数据整合和比较分析。
研究人员可能会使用生物信息学工具或编程语言如R或Python来对单细胞测序数据进行处理,找出共同的基因表达特征或细胞类型。
这可能涉及到基因表达矩阵的比较、聚类分析、差异表达基因的筛选等多个步骤。
此外,取交集的过程也需要考虑数据的质量和一致性,以及统计学上的显著性。
在进行取交集操作时,研究人员需要注意数据处
理的标准化和校正,以及如何控制假阳性发现的风险。
总的来说,单细胞测序取交集是一个复杂而重要的数据分析过程,它可以帮助我们发现细胞的共同特征和调控机制,为细胞生物学和疾病研究提供重要的信息。
单细胞测序分析流程
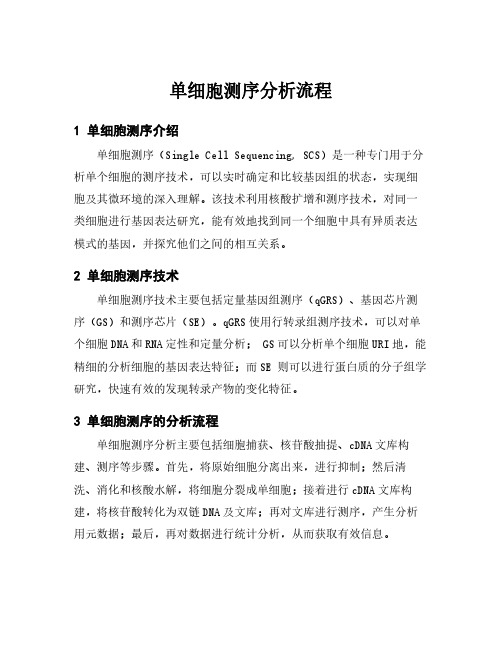
单细胞测序分析流程
1单细胞测序介绍
单细胞测序(Single Cell Sequencing,SCS)是一种专门用于分析单个细胞的测序技术,可以实时确定和比较基因组的状态,实现细胞及其微环境的深入理解。
该技术利用核酸扩增和测序技术,对同一类细胞进行基因表达研究,能有效地找到同一个细胞中具有异质表达模式的基因,并探究他们之间的相互关系。
2单细胞测序技术
单细胞测序技术主要包括定量基因组测序(qGRS)、基因芯片测序(GS)和测序芯片(SE)。
qGRS使用行转录组测序技术,可以对单个细胞DNA和RNA定性和定量分析;GS可以分析单个细胞URI地,能精细的分析细胞的基因表达特征;而SE则可以进行蛋白质的分子组学研究,快速有效的发现转录产物的变化特征。
3单细胞测序的分析流程
单细胞测序分析主要包括细胞捕获、核苷酸抽提、cDNA文库构建、测序等步骤。
首先,将原始细胞分离出来,进行抑制;然后清洗、消化和核酸水解,将细胞分裂成单细胞;接着进行cDNA文库构建,将核苷酸转化为双链DNA及文库;再对文库进行测序,产生分析用元数据;最后,再对数据进行统计分析,从而获取有效信息。
4单细胞测序的应用
单细胞测序可以用于进行多种基因表达研究,可用于研究细胞临床医学学习,如原发性肿瘤研究,对肿瘤细胞分化、分型以及预后分析等具有重要意义。
而且,可以通过对单细胞测序数据的深入分析,了解细胞间通讯网络的动态演变,从而更深入的了解细胞的行为特性及发育规律。
单细胞测序技术不但为研究肿瘤细胞特异性提供全面的基础数据,而且对药物研发等也有重要的价值。
单细胞测序在生物学中的应用

单细胞测序在生物学中的应用随着科技的不断进步,单细胞测序技术越来越受到关注。
单细胞测序可以帮助我们更好地了解细胞之间的异同,探究生物体内各种复杂的生物学过程。
单细胞测序技术可以应用于生物学的多个领域,本文将就此进行探讨。
1. 单细胞基因组测序单细胞基因组测序可以帮助我们更好地了解单个细胞的基因组信息,包括基因型、拷贝数变异、染色体畸变等。
这种技术特别适用于研究癌症等疾病,因为癌症细胞常常会出现染色体畸变等不同于正常细胞的情况。
通过单细胞基因组测序,我们可以全面了解每个细胞的基因组信息,为癌症等疾病的研究提供数据支持。
2. 单细胞转录组测序通过单细胞转录组测序,我们可以全面了解单个细胞内各类RNA的表达情况,包括mRNA、ncRNA等。
这种技术可在细胞组成和细胞功能等方面提供全面的信息,帮助我们更好地了解细胞的分化、功能和生长发育等生物学过程。
此外,单细胞转录组测序还可以用于研究人类疾病的发生和发展机制,为疾病的早期预测、诊断和治疗提供数据支持。
3. 单细胞表观遗传测序单细胞表观遗传测序主要用于研究细胞内的表观遗传变异,如DNA甲基化和组蛋白修饰等。
这种技术可配合前两种技术研究细胞的分化和功能,探究表观遗传变异与多种疾病之间的关联,如心血管疾病、癌症等。
4. 单细胞蛋白组测序单细胞蛋白组测序可以全面了解单个细胞内不同蛋白之间的表达情况和相互关系。
这种技术可配合前三种技术研究细胞和疾病之间的关联,如研究癌症细胞的蛋白组变异,探究癌症细胞的分化和功能等。
总之,单细胞测序技术在生物学的应用领域十分广泛,如癌症研究、病毒感染研究、分子进化研究、系统生物学研究等。
通过综合多种单细胞测序技术,我们可以全面了解细胞的组成和功能,为了解复杂的生物学过程提供更加全面和深入的数据支持。
- 1、下载文档前请自行甄别文档内容的完整性,平台不提供额外的编辑、内容补充、找答案等附加服务。
- 2、"仅部分预览"的文档,不可在线预览部分如存在完整性等问题,可反馈申请退款(可完整预览的文档不适用该条件!)。
- 3、如文档侵犯您的权益,请联系客服反馈,我们会尽快为您处理(人工客服工作时间:9:00-18:30)。
Highthroughput with microfluidic dropletbarcodingThe application of single-cell genome sequencing to large cell populations has beenhindered by technical challenges in isolating single cells during genome preparation. Herewe present single-cell genomic sequencing (SiC-seq), which uses droplet microfluidics toisolate, fragment, and barcode the genomes of single cells, followed by Illumina sequencingof pooled DNA.We demonstrate ultra-high sequencing throughput of >50,000 cells per runin a synthetic community of Gram negative and Gram-positive bacteria and fungi. Thesequenced genomes can be sorted in silico based on characteristic sequences.We use thisapproach to analyze the distributions of antibiotic resistance genes, virulence factors, andphage sequences in microbial communities from an environmental sample.The ability toroutinely sequence large populations of single cells will enable the de-convolution of geneticheterogeneity in diverse cell populations.Main:Organisms are living expressions of their genomes and, hence, genome sequencing is apowerful way to study how they grow and function.Organisms are phenotypically diverse,and this diversity is mirrored by heterogeneity at the genomic level and plays important rolesin populations as a whole, particularly among populations of single cells.A commonchallenge when applying single cell sequencing to heterogeneous systems is that they oftencontain massive numbers of cells: a centimeter-sized tumor can contain hundreds of millionsof cancer cells1, while a milliliter of seawater can contain millions of microbes2.Moreover,each cell has a tiny quantity of DNA, making itchallenging to accurately amplify andsequence singlecells.The sparseness of the sampling limits the questions that canbe addressed, with the majority of findings relating to the most abundant subpopulations. Amethod that could markedly increase the number of cells sequenced at the single cell levelwould impact a broad range of problems across biology where heterogeneity is important.Droplet microfluidics enables millions of independent picoliterreactions, and has recentlybeen used to deep sequence single DNA molecules10, tag nucleosomes to enable single-cellChIP-seq11, and to profile the transcriptomes of single cells all at high throughput. However, sequencing the genomes of single cells presents unique challenges, becausegenomic DNA must be purified from the cellular matter and processed through a series ofenzymatic steps to prepare it for sequencing.Consequently, while droplet microfluidicsprovides the potential for sequencing of single cell genomes at ultra high-throughput, noapproach for accomplishing this has yet been described.We describe a method for single cell genome sequencing at ultrahigh-throughput (SiC-seq)using droplet microfluidics.In SiC-seq, we encapsulate cells in hydrogel microspheres(microgels) that are permeable to molecules with hydraulic diameters smaller than the poresize, including enzymes, detergents, and small molecules, but sterically trap large moleculessuch as genomic DNA.This allows us to use a series of “washes” on encapsulated cells, toperform the requisite steps of cell lysis and genome processing, while maintainingcompartmentalization of each genome.Using a combination of microgel andmicrofluidicprocessing steps, we lyse the cells, fragment the genomes, and attach unique barcodes toallfragments, in a workflow that processes >50,000 cells in a few hours.pooled and sequenced,and the reads grouped bybarcode,providing a library of single cell genomesthat can be subjected to additionaldownstreamprocessing, including demographiccharacterization and in silico cytometry (Fig. 1ResultsSiC-seq workflowThe principal strategy of SiC-seq is to label all DNAfragments originating from the samegenome with asequence identifier (barcode) unique to that cell.The resultant products arechimeric, comprising abarcode sequence covalently linked to a randomfragment of the cellgenome.The barcodes allow all reads belonging to a givencell to be identified throughshared sequence.We use libraries of barcode droplets containing thebarcode sequences thatare merged with the genomecontaining droplets to be barcoded10.To prepare a barcodedroplet library, we encapsulateinto droplets at limiting dilution, oligonucleotidescomprising15 random bases flanked by constantsequences with PCR reagents andprimerscomplementary to the constant regions of thebarcodes with one side containing the IlluminaP7flow cell adapter (Fig. 2a)16.The droplets are then thermal cycled to amplify thebarcodesequences via digital droplet PCR, generating~10 million barcode droplets in a few hours.Before the single cell genomes can be barcoded, theymust be physically isolated, purified,and fragmented.To accomplish this, we encapsulate single cells inagarose microgels usinga two-stream co-flow dropletmaker, which merges a cell suspension stream with amoltenagarose stream, forming a droplet consistingof an equal volume of both streams (Fig. 2b andSupplementary Fig. 1a).The droplet maker runs at ~10 kHz, allowing us togenerate~10 million ~22 μm diameter droplets in ~20minutes in a total volume of aqueous emulsionof ~60μL.Hence, droplet generation is fast and the total volume consumed small, allowingus to load cells at a rate of 1:10 to minimize multi-cell encapsulation.microgels are then transferred from oil to aqueouscarrier phase to besubjected to cell lysis and genomepurification.To lyse the cells, we incubate the microgelsovernightin a mixture of lytic enzymes, digesting theprotective microbial cell walls (Onlinemethods).We then incubate them in a mixture of detergents andproteases for 30 minutes,solubilizing lipids and digesting proteins, preservingonly high molecular weight genomicDNA, which weverify by staining with SYBR green dye (Fig. 2c).To fragment the genomesand attach the universalsequences to act as PCR handles, we re-encapsulatethe gels in theNextera® reaction (Fig 2dandSupplementary Fig. 1b).Because the transposases aredimeric, the fragmentedgenome remains intact as a macromolecular complex,remainingsterically encased within the hydrogelnetwork (Supplementary Fig. 2) 17.Nevertheless, were-encapsulate the gels into separatedroplets during fragmentation to ensure that there isnocross-contamination of DNA between the gels.After the genomes are purified and fragmented, theyare barcoded for sequencing.We use amicrofluidic device that merges eachmicrogel-containing droplet with dropletscontainingPCR reagents and a barcode droplet (Fig.2e andSupplementary Fig. 1c).The resultingdroplets, which containfragmented-genome and barcode DNA are collectedinto a PCR tubeand thermal cycled, splicing thebarcode sequences onto the genomic fragmentsviacomplementarity through the PCR handles addedby the transposase.At this point, thespliced fragments contain both theP5 and P7 Illumina sequencing adaptor requiredforsequencing on the Illumina platforms.We remove droplets that coalesce duringthermalcycling using a micropipette, then theremaining droplets are chemically merged andtheircontents pooled and prepared for sequencing(Online methods).Validation of SiC-seq on an artificial microbial communityThe objective of SiC-seq is to provide single cell genomic sequences bundled in barcodegroups.To validate that SiC-seq generates single cell barcode groups, we applied it to anartificial microbial community containing three Gram-negative bacteria, five Gram-positivebacteria, and two yeasts, which are typically difficult cell types to lyse.We prepared asingle-cell library from this community using SiC-seq and sequenced it on an IlluminaMiSeq, yielding ~6 million single-end reads of 150 bp after quality filtering.We groupedreads by barcode and discard groups with < 50 reads yielding the final 48,989 barcodegroups (Fig. 3a).Each barcode group represents a low-coverage genome of a cell, with asequencing depth of ~0.1% to ~1% (Supplementary Fig. 3)To determine whether the barcode groups indeed correspond to single cells, we mapped allreads to the reference genomes of the ten species.If two microbes reside within the samebarcode group, reads will map to two genomes.We defined a group purity score as thefraction of reads mapping to the most mapped reference (the ideal barcode group has apurity score of 1.0).The distribution of purity scores is strongly skewed to high values withthe majority of purity score over 0.95 suggesting that most barcode groups represent singlecells; this result is consis;tent even taking into account the different genome sizes of the tenspecies (Fig. 3b and Supplementary Fig. 4) as well as when purity is examined individuallyfor each species(Supplementary Fig. 5).To determine whether SiC-seq barcodes abundances reflect the organism abundances in thedataset, we compared abundance estimates calculated via short-read alignment, k-mer basedsequence classification, and counting under bright-field microscopy (Fig 3 andSupplementary Fig. 7).We found that all methods are in reasonable agreement when readsare pooled and analyzed in bulk and when species identities are assigned to each barcodebased on the most commonly mapped species in a group.This demonstrates that SiC-seqenables estimation of species abundance in a microbial population consistent with acceptedmetagenomic methods. Sequencing the genome of a single cell typically incurs coverage distribution bias18 due touneven amplification of DNA starting from a single genome copy.To investigate coveragedistribution bias in SiC-seq, we plotted the normalized coverage distribution for readsaggregated from all barcode groups for each microbe (Fig. 3d, 3e, and Supplementary Fig.8). With the exception of coverage gaps due to low abundances of cells of certain specieswithin the standard microbial community, we observed no substantial coverage bias.Thisindicates that the sampling of each genome within a barcode group is random, so that whenall groups are overlaid, a uniform distribution is obtained.We further inspected thedistribution of reads in individual barcode groups and found no substantial bias(Supplementary Fig. 9). We believe thatcoverage bias is minimal because each genomeisamplified in a tiny volume of ~65 pL, which has been shown to curtail bias-inducingrunaway of exponential amplification19.amplified genomes, the amplification of each genomecan be limited by the tinyvolume while still producingsufficient total DNA for sequencing.SiC-seq data analysis with in silico cytometryThe genomic sequences generated using SiC-seq aregrouped according to single cells,which iscomplementary to the sequences generated fromshotgun metagenomic sequencing.Existing computational tools are ill-suited to analyzethese data because they do not exploitthe single cellbarcode information unique to SiC-seq.To address this, we utilize a sequenceanalysis pipelinein which reads are organized hierarchically as barcodegroups, generating aSingle Cell Reads database(SiC-Reads) (Supplementary Fig. 10).To build SiC-Reads, wefilter raw sequences by quality,group them by barcode, and estimate ataxonomicclassification of each group usingphylogenetic profilers.We also estimate a purity scoreequal to the fraction ofreads mapping to the dominant taxon within theclassifiable set.Additional properties of barcode groups and reads,such as presence of sequencescorresponding toantibiotic resistance genes, can be added to thedatabase as they arediscovered during analysis.The massive set of single cell genomes present inSiC-Reads provides new opportunities fordiscoveringassociations between sequences within single cells, ina process we dub in silico cytometry.SiC-Reads comprises a collection of single cellgenomes that can be sorted insilico, analogous towhat is commonly done with flow cytometry onsingle cells. Thedatabase can be sorted repeatedly tomine for correlations between differentgeneticsequences and structures. Moreover, as newassociations are learned, new sorting parameterscanbe defined, enabling discoveries without having torepeat the experiment.resistance in microbesTo demonstrate in silico cytometry, we used SiC-seq to sequence a microbial community recovered from coastal seawater of San Francisco (Online methods). We obtained ~8 millionreads of 150 bp length after quality filtering(representing of ~55% of raw reads), withwhich we generated a SiC-Reads database(Supplementary Fig. 10). Using a phylogeneticprofiler, 601,348 (6.89%) of reads werebacteria, 0.04% archaea, and 0.16% viruses (Supplementary Fig. 11a). Barcode groups were assigned a taxonomic classification based on the reads they contained, following the rulethat more than 10% of reads in a barcode group must be classified, and the assigned classification is the taxon with the most supporting reads. Most barcode groups were high purity based on the classifiable sequences (~91% average), in accordance with our controlsample (~94% average) (Supplementary Fig. 11b). Using this SiC-reads database, we demonstrate in silico cytometry by exploring the distribution of antibiotic resistance,virulence factors, and phage sequences in the microbial community.Antibiotic resistance has become increasingly common and represents a significant threat to global human health20. While antibiotic resistance genes can be identified in mostenvironments by short-read sequencing, scant information on how they are distributedamong taxa is available, because obtaining this information usually requires testing or whole genome sequencing of single species; however, culture conditions for most species have notbeen identified, precluding such analyses.To determine the distribution of antibiotic resistance genes among taxa in our dataset, we searched our SiC-Reads database for known antibiotic resistance genes, finding 1,081(0.012% of reads), representing 108 (0.30%) ofbarcode groups. The taxonomic distributionof antibiotic resistance genes in our database has a clear structure, although it does notcorrelate with what is known from genomes in public databases (Fig. 4a and SupplementaryFig. 12a). This is unsurprising as differences are expected in the natural coastlineenvironment compared to the environment of isolated and sequenced strains. The mostabundant taxa associated with antibiotic resistance Array are not the most abundant communitymembers overall, suggesting that in this communitycertain taxa tend to associate more withantibiotic resistance genes.Association of virulence factors with hostbacteriaVirulence factors, like antibiotic resistance genes, areimportant genetic factors indetermining the threat that specific microbes pose tohuman health. Many opportunisticpathogens reside in natural communities in theenvironment and cause outbreaks whentransmitted to a suitable host21. Monitoring anddetecting potentially pathogenic microbes isimportant for public health. Like antibioticsresistance genes, traditional methods can detectthe presence of these genes but not their taxonomicdistribution.To examine the taxonomic distribution of virulencefactors in our dataset, we searched ourcoastal microbial community database for knownvirulence factor genes, yielding matches in1,949 (0.022%) reads in 101 (0.28%) barcode groupsconsisting of 29 prevalent virulencefactors distributed among 13 microbial genera. Theabundances of taxa where virulencefactors were found did not reflect that of the totalpopulation, suggesting that certain generatend to carry more virulence factors than others. Toquantify this, we calculated the virulencefactor ratio, the ratio between the number of barcodegroups containing virulence factors andthe total number of barcodes in the community forthat species, and normalized the results tothe highest virulence factor ratio for comparison (Fig.4b). Haemophilus and Escherichiastand out amongst all species, both of which areknown opportunistic human pathogens.Comparing the virulence factor ratios of the San Francisco coastline community with ones calculated for publicly-available whole genomes, and down sampled to match our per-cellread depth (Supplementary Fig. 12b), we found that the ratios are higher for the publicgenomes, an expected result given that isolated and sequenced genomes are are biasedtowards pathogenic strains.Determining transduction potential between bacteriaMany virulent bacterial strains are thought to arise from horizontal gene transfer aided bycross-infection of bacteriophages. Phages can modify the genomes of their hosts, leaving acopy of their own genome behind or transporting fragments of one species to another in aprocess known as transduction22,23. Characterizing the distribution of these mobile elementsis challenging in an ecological context because confident identification of foreign genomic fragments within a specific host requires sequencing large numbers of cultures of singlespecies or single cells. Nevertheless, this information is valuable for understanding howbacteria transfer genetic material in general, and how virulent new strains may emerge viathis mechanism.To explore transduction in the microbial community, we searched the SiC-Reads database ofthe San Francisco coastal community for barcode groups containing phage sequences. Aphage sequence found in a bacterial genome is evidence of infection, an association that isnormally extremely difficult to make for uncultivable microbes and their likely uncultivableinfecting phages. We found matches in 6,805 (0.078%) reads representing 260 (0.72%)barcode groups and 106 phage genomes. Since transduction can occur between two hostcells that can be infected by the same phage, the potential for transduction depends on thelikelihood of phages infecting both hosts. To visualize this, we plot the normalized sum ofthe number of times we detect the sequences matching to the same phage in two bacterialtaxa, normalized by the number of barcode groups in those taxa (Fig. 4c). According to this analysis, Delftia and Neisseria, which are closest related out of the taxa in our analysis, havethe highest potential for transduction. The dearth of representative phage genomes indatabases and the limited sequence information per barcode group, limits the accuracy ofthis approach. Therefore, higher coverage of the genomes and better phage genomedatabases are required to definitively identify the phages that are found in the database. Nevertheless, SiC-seq‟s ability to detect these sequences and correlate them within singlegenomes can provide a useful approach to studying phage-host interactions.DiscussionSiC-seq generates a metagenomic database grouped by single cell genomes amenable torepeated mining via in silico cytometry, for rapid hypothesis generation and testing. Wedemonstrated its use in measuring the distributions of antibiotic resistance genes, virulencefactors, and transduction potential in microbial communities. The ability to sequence allcells in a sample without the need to culture is a powerful aspect of SiC-seq that should aidin our ability to characterize t he …microbial dark matter‟.The barcoded nature of SiC-seq data necessitates additional quality control of measures forthe data, in addition to the quality control measures utilized in standard sequencing. First,the barcode reads themselves must be of high quality, thus eliminating any reads containinglow quality barcode sequences, regardless of the quality of the genomic sequences. Second,barcode groups must be quality controlled to remove small-sized barcode groups, which arethe result of mutations in the barcode sequences and background contamination of freeDNA. These quality control measures together resultin a typical yield of ~55% of raw readscontributing to the SiC-reads database. Improvements in yield can be made by, for example,computationally ident ifying reads with mutated barcodes …correcting‟ their sequence, but wehave found only modest improvements in yields using this method alone10.The taxonomic classification of microbes remains an integral part of studying communitydynamics, from ecosystems on Earth to those residing in and on our bodies24,25. However,the taxonomic classification of short reads is error prone, due to the diversity of microbes inmost communities and the high degree of horizontal gene transfer that mixes genomicelements in unpredictable ways. SiC-seq improves upon traditional metagenomicssequencing in addressing this challenge because taxonomic identification can be made basedon hundreds of reads within a barcode group. Advanced strategies can be applied to estimatetaxonomy of a barcode group, including Bayesian probabilistic ones based on classificationof each read in the group, or ones weighted towards specific taxonomic markers. With eventhis improvement, accurate classification is difficult because the vast majority of sequencesremain unclassifiable and the classification of sequences are biased towards well-sequencedtaxa in the databases. As genome coverage improves in future iterations of SiC-seq,taxonomic classification of barcode groups should become more confident and precise,potentially arriving at strain level classifications under certain circumstances. It is worthnoting that taxonomic classification with SiC-seq is also subject to the fundamentallimitations of reference based classification paradigms where the classification is only asaccurate as the match between the sample and the references. Hence, like traditionalmethods, SiC-seq phylogenetic profiling will become more reliable and complete with theexpanding database of reference genomes.The degree of genome coverage impacts the usefulness of single cell data, including theability to generate assemblies or identify characteristic sequences for in silico cytometry. Alimitation of SiC-seq is that, while the number of cells sequenced far exceeds currentlydescribed methods, the coverage per cell is significantly lower. Therefore, dropouts incoverage and false negatives can be expected in in silico cytometry analysis. For abundantorganisms with a random distribution of coverage in each barcode group, the system isrobust to dropouts because results are averaged over many barcode groups. For example,approximately 7,000 Alteromonas barcode groups were taken into account to determine theantibioticresistance profile for Alteromonas bacteria. However, for less abundant species,such as Haemophilus, more dropouts can be expected because there may not be enough totalsequence information to detect a specific genetic factor. For this reason, the analysis of SiCreads databases should be limited to relative comparisons of species within the database, andthe abundance of target genes within subpopulations should be normalized to the number ofbarcode groups in the subpopulation. It is worth noting that the dropout phenomenon is notunique to SiC-seq data, but all metagenomic sequencing data where the subpopulation to beanalyzed represents a very small fraction.Although coverage can be increased by sequencing more reads, the coverage per cell perbarcode group will be below 100%. This is because the method begins with a single genomecopy without amplification and losses incurred during enzymatic and microfluidicprocessing are irrevocable, thus limiting the maximum coverage attainable. In futureiterations of SiC-seq, coverage may be increased by pre-amplifying genomes prior toprocessing, for example, with multiple displacement amplification in droplets7. Additionally,different strategies for barcoding genomes may yield higher coverage, such as recentlydescribed combinatorial indexing via transposase libraries40, which should be applicable tosingle cell genomes encapsulated in microgels.The de novo assembly of whole genomes from metagenomics sequences is a common goalin the field of metagenomics. Mate-paired sequencing can be used to bridge contigs inametagenomics sequencing dataset and potentially assemble whole genomes given sufficientcoverage26. Though powerful, the method is limited by the required micrograms of startingDNA that can be difficult to obtain from microbial ecosystems. Furthermore, many matepaired reads are required to assemble a whole genome, since each mate-pair bridges onlytwo contigs. SiC-seq data improves on mate-paired sequencing in this respect by requiringminimal amounts of sample as well as enabling the bridging of multiple contigs per barcodegroup. Consequently, SiC-seq should allow generation of draft genomes from shotgunmetagenomic data with far less DNA input requirement and sequencing effort.While we focused on microbial communities, SiC-seq is also applicable to populations ofmammalian cells, where it can have a more direct impact on human health. The groupedreads provided by SiC-seq should afford the information required to determine copy-numbervariations within the genome, which is relevant to cancer27. The enormous size ofmammalian genomes, however, limits the number of cells that can be sequenced for a targetlevel of coverage. Nevertheless, as the cost of sequencing continues to decrease, more cellscan be sequenced to greater depth, creating opportunities for characterizing mammaliantissues, cell-by-cell.SiC-seq method is a means to isolate and barcode large DNA molecules, irrespective of theentity from which they originate. While we have focused on cells, similar approaches can beapplied to any entities whose genomes can be trapped and processed within the gel matrix.SiC-seq‟s ability to build and mine large databases of genomes grouped by single cellsshould contribute to the characterization of heterogeneity across biology.。