Taxonomy-based Adaptive Web Search Method
(完整版)生态学(双语)专业英语单词(2)

K-对策者 K—strategistisn维超体积资源空间 n—dimensional hyper—volume n维生态位 n-dimensional nicheRaunkiaer定律 Law of Frequencyr-对策者 r-strategistis奥陶纪 Ordovician period白垩土草地 chalk grassland斑块 patch斑块性 patchiness斑块性种群 patchy population半荒漠 semi-desert半矩阵或星系图 constellation diagrams伴生种 companion species饱和密度 saturation density饱和期 asymptotic phase保护哲学 conservation philosophy北方针叶林 northern conifer forest被动取样假说 passive sampling hypothesis本能 instinct本能行为 instinctive behavior避敌 avoiding predator边缘效应 edge effect变异性 variability标志重捕法 mark recapture methods标准频度图解 frequency diagram表现型适应 phenotypic adaptation并行的 simultaneous并行同源 paralogy捕食 predation不重叠的 non—overlapping残存斑块 remnant patch残余廊道 remnant corridor操作性条件作用 operant conditioning草原生态系统 grassland system层次性结构 hierachical structure产卵和取食促进剂 oviposition and feeding stimulant 产业生态学 industry ecology长日照植物 long day plant超体积生态位 hyper—volume niche成本外摊 externalized cost程序化死亡 programmed cell death尺度效应 scaling effect抽彩式竞争 competive lottery臭氧层破坏 ozone layer destruction出生率 natality或birth rate初级生产 primary production初级生产力 primary productivity初级生产者 primary producer传感器 sensor串行的 serial垂直结构 vertical structure春化 vernalization次级生产 secondary production次级生产力 secondary productivity次生演替 secondary successon粗密度 crude density存活曲线 survival curve存活值 survival value存在度 presence搭载效应 hitchhiking effect大陆—岛屿型复合种群 mainland-island metapopulation 带状廊道 strip corridor单联 single linkage单体生物 unitary organism单位努力捕获量 catch per unit effort单元的 monothetic淡水生态系统 fresh water ecosystem氮循环 nitrogen cycling等级(系统)理论 hierarchy theory等级的 hierarchical底内动物 infauna底栖动物 benthos地表火 surface fire地带性生物群落 biome地理信息系统 geographic information system地面芽植物 hemicryptophytes地上芽植物 chamaephytes地植物学 geobotany第三纪 Tetiary period第四纪 Quaternary period点突变 genic mutation或point mutation电荷耦合器 charge coupled device, CCD顶极阶段 climax stage顶极群落 climax community顶极种 climax species动态率模型 dynamic pool model动态平衡理论 dynamic equilibrium theory动态生命表 dynamic life table动物痕迹的计数 counts of animal signs动物计数 counts of animals冻原 tundra短日照植物 short day plant断层 gaps多波段光谱扫描仪 multichannel spectrum scanner, MSS 多度 abundance多样化 variety多元的 poly thetic厄尔尼诺El Nino反馈feedback反射reflex泛化种generalist防卫行为defennce behavior访花昆虫flower visitor非等级的non—hierarchical非空间模型non-spatial model非内稳态生物non-homeostatic organism非平衡态复合种群nonequilibrium metapopulation非平衡态跟踪生境复合种群nonequilibrium habitat-tracking metapopulation非平衡态下降复合种群nonequilibrium declining metapopulation非生态位non-niche非生物环境physical environment非线性关系nonlinear分布dispersion分解者decomposer分支过程branching process分子分类学molecular taxonomy分子进化的中性理论the neutral theory of molecular evolution分子生态学molecular ecology分子系统学molecular systematics浮游动物plankton负反馈negative feedback)负荷量carrying capacity负相互作用negative interaction负选择negative selection附底动物epifauna复合种群metapopulation富营养化现象eutrohication改良relamation盖度coverage盖度比cover ratio干扰disturbance干扰斑块disturbance patch干扰廊道disturbance corridor干扰作用interference高度height高斯假说Coarse's hypothesis高斯理论Coarse's theory高位芽植物phanerophytes格林威尔造山运动Grenville Orogenesis 个体individual个体论概念individualistic concept更新renewal功能生态位functional niche攻击行为aggressive behavior构件modules构件生物modular organism关键种keystone species关联系数association coefficients光饱和点light saturation point光补偿点light compensation point光周期photoperiod过滤器filter哈德-温伯格原理Hardy—Weinberg principle 海洋生态系统Ocean ecosytem寒武纪Cambrian period旱生植物siccocolous河流廊道river corridor恒有度contancy红树林mangrove呼吸量respiration互利mutualism互利素synomone互利作用synomonal化感作用allelopathy化学防御chemical defence化学生态学chemical ecology化学物质allelochemicals化学隐藏chemocryptic划分的divisive环境environment环境伦理学environmental ethics环境容纳量environmental carryin capacity环境资源斑块environmental resource patch环境资源廊道environmental resource corridor 荒漠desert荒漠化desertification荒漠生态系统desert ecosystem黄化现象eitiolation phenomenon恢复生态学restoration ecology混沌学chaos混合型mixed type活动库exchange pool获得性行为acquired behavior机体论学派organismic school基础生态位Fundamental niche基质matrix极点排序法polar ordination集群型clumped寄生parasitism加速期accelerating phase价值value价值流value flow间接排序indirect ordination间接梯度分析indirect gradiant analysis减速期decelerating phase简单聚合法lumping碱性植物alkaline soil plant建群种constructive species接触化学感觉contact chemoreception解磷菌或溶磷菌Phosphate-solubiIizing Microorganisms, PSM 进化适应evolutionary adaptation经典型复合种群classic metapopulation经济密度economic density景观landscape景观格局landscape patten景观过程模型process based landscape model景观结构landscape structure景观空间动态模型spatial dynamic landscape model景观生态学landscape ecology净初级生产量net primary production竞争competition竞争排斥原理competition exclusion principle静态生命表static life table局部种群local population距离效应distance effect聚合的agglomerative均匀型uniform菌根mycorrhiza抗毒素phytoalexins可持续发展sustainable development 空间结构spatial structure空间模型spatial model空间生态位spatial niche空间异质性spatial heterogeneity 库pool矿产资源mineral resources廊道corridor离散性discrete利己素allomone利己作用allomona利他行为altruism利他作用kairomonal连续体continuum联想学习associative learning猎食行为hunting behavior林冠火crown fire磷循环phosphorus cycling零假说null hypothesis领悟学习insight learning领域性territoriality流flow绿色核算green accounting逻辑斯谛方程logistic equation铆钉假说Rivet hypothesis密度density密度比density ratio密度制约死亡density-dependent mortality 面积效应area effect灭绝extinction铭记imprinting模拟hametic模型modeling牧食食物链grazing food chain内禀增长率intrinsic rate of increase内稳态homeostasis内稳态生物homeostatic organisms内源性endogenous内在的intrinsic耐阴植物shade-enduring plants能量分配原则principle of energy allocation 能量流动energy flow能源资源energy resources能值emergy泥盆纪Devonian period拟寄生parasitoidism逆分析inverse analysis年龄分布age distribution年龄结构age structure年龄性别锥体age—sex pyramid年龄锥体age pyramids偶见种rare species排序ordination配额quota配偶选择mate selection偏害amensalism偏利commensalism频度frequency平衡选择balancing selection平台plantform平行进化parallel evolution栖息地habitat期望值外推法extrapolation by expected value 气候驯化acclimatisation器官organs亲本投资parental investment亲族选择kin selection趋光性phototaxis趋化性chemotaxis趋同进化convergent evolution趋性taxis趋异进化divergent evolution趋异适应radiation adaptation取食促进剂oviposition and feeding stimulant 取样调查法sampling methods去除取样法removal sampling全联法complete linkage全球global全球变暖global warnning全球定位系统global Positioning System全球生态学global ecology确限度fidelity群丛association群丛单位理论association unit theory群丛组association group群落community群落的垂直结构vertical structure群落生态学community ecology群落水平格局horizontal pattern群落外貌physiognomy群落演替succession群系formation群系组formation group热带旱生林tropical dry forest热带季雨林tropical seasonal rainforest热带稀树草原tropical savanna热带雨林tropical rainforest热力学第二定律second law of thermodynamics 热力学第一定律first law of thermodynamics 人工斑块introduced patch人工廊道introduced corridor人口调查法cencus technique人口统计学human demography日中性植物day neutral plant冗余redundancy冗余种假说Redundancy species hypothesis三叠纪Triassic period森林生态系统forest ecosystem熵值entropy value上渐线upper asymptotic社会性防卫行为defence behavior社会优势等级dominance hierarchy摄食行为feed behavior生活史life history生活史对策life history strategy生活小区biotope生活型life form生活周期life cycle生境habitat生境多样性假说habitat diversity hypothesis 生理出生率physiological natality生理死亡率physiological mortality生命表life table生态出生率ecological natality生态对策bionomic strategy生态反作用ecological reaction生态幅ecological amplitude生态工程ecological engineering生态工业ecological industry生态规划ecological planning生态恢复ecological restoration生态经济ecological economics生态旅游ecotourism生态密度ecological density生态农业ecological agriculture生态入侵ecological invasion生态设计ecological design生态适应ecological adaptation生态死亡率ecological mortality生态位niche生态位宽度niche breadth生态位相似性比例niche proportional similarity 生态位重叠niche overlap生态文明ecological civilization生态系统ecosystem生态系统产品ecosystem goods生态系统多样性ecosystem diversity生态系统服务ecosystem service生态系统生态学ecosystem ecology生态系统学ecosystemology生态型ecotype生态学ecology生态因子ecological factor生态元ecological unit生态作用ecological effect生物organism生物地球化学循环biogecochemical cycle生物多样性biodiversity生物量biomass生物潜能biotic potential生物群落biotic community,biome生物群落演替succession生殖潜能reproductive potential剩余空间residual space失共生aposymbiosis湿地wetland湿地生态系统wetland ecosystem湿地植物hygrophyte时间结构temporal structure实际出生率realized natality实际死亡率realized mortality食草动物herbivores食肉动物carnivores食物链food chain食物网food wed矢量vector适合度fitness适应辐射adaptive radiation适应值adaptive value适应组合adaptive suites收获理论harvest theory收益外泄externalized profit衰退型种群contracting population 水平格局horizontal pattern水土流失soil and water erosion 水循环water cycling瞬时增长率instantaneous rate死亡率mortality & death rate松散垂直耦连loose vertical coupling松散水平耦连loose horizontal coupling溯祖过程coalescent process溯祖理论coalescent theory酸性土理论acid soil plant酸雨acid rain随机型random碎屑食物链detritus food chainK-对策者K-strategistisn维超体积资源空间n-dimensional hyper—volume n维生态位n-dimensional nicheRaunkiaer定律Law of Frequencyr-对策者r-strategistis奥陶纪Ordovician period白垩土草地chalk grassland斑块patch斑块性patchiness斑块性种群patchy population半荒漠semi-desert半矩阵或星系图constellation diagrams伴生种companion species饱和密度saturation density饱和期asymptotic phase保护哲学conservation philosophy北方针叶林northern conifer forest被动取样假说passive sampling hypothesis本能instinct本能行为instinctive behavior避敌avoiding predator边缘效应edge effect变异性variability标志重捕法mark recapture methods标准频度图解frequency diagram表现型适应phenotypic adaptation并行的simultaneous并行同源paralogy捕食predation不重叠的non—overlapping残存斑块remnant patch残余廊道remnant corridor操作性条件作用operant conditioning草原生态系统grassland system层次性结构hierachical structure产卵和取食促进剂oviposition and feeding stimulant 产业生态学industry ecology长日照植物long day plant超体积生态位hyper—volume niche成本外摊externalized cost程序化死亡programmed cell death尺度效应scaling effect抽彩式竞争competive lottery臭氧层破坏ozone layer destruction出生率natality或birth rate初级生产primary production初级生产力primary productivity初级生产者primary producer传感器sensor串行的serial垂直结构vertical structure春化vernalization次级生产secondary production次级生产力secondary productivity次生演替secondary successon粗密度crude density存活曲线survival curve存活值survival value存在度presence搭载效应hitchhiking effect大陆—岛屿型复合种群mainland-island metapopulation 带状廊道strip corridor单联single linkage单体生物unitary organism单位努力捕获量catch per unit effort单元的monothetic淡水生态系统fresh water ecosystem氮循环nitrogen cycling等级(系统)理论hierarchy theory等级的hierarchical底内动物infauna底栖动物benthos地表火surface fire地带性生物群落biome地理信息系统geographic information system 地面芽植物hemicryptophytes地上芽植物chamaephytes地植物学geobotany第三纪Tetiary period第四纪Quaternary period点突变genic mutation或point mutation电荷耦合器charge coupled device, CCD顶极阶段climax stage顶极群落climax community顶极种climax species动态率模型dynamic pool model动态平衡理论dynamic equilibrium theory动态生命表dynamic life table动物痕迹的计数counts of animal signs动物计数counts of animals冻原tundra短日照植物short day plant断层gaps多波段光谱扫描仪multichannel spectrum scanner, MSS多度abundance多样化variety多元的poly thetic厄尔尼诺El Nino反馈feedback反射reflex泛化种generalist防卫行为defennce behavior访花昆虫flower visitor非等级的non—hierarchical非空间模型non-spatial model非内稳态生物non-homeostatic organism非平衡态复合种群nonequilibrium metapopulation非平衡态跟踪生境复合种群nonequilibrium habitat—tracking metapopulation非平衡态下降复合种群nonequilibrium declining metapopulation非生态位non—niche非生物环境physical environment非线性关系nonlinear分布dispersion分解者decomposer分支过程branching process分子分类学molecular taxonomy分子进化的中性理论the neutral theory of molecular evolution 分子生态学molecular ecology分子系统学molecular systematics浮游动物plankton负反馈negative feedback)负荷量carrying capacity负相互作用negative interaction负选择negative selection附底动物epifauna复合种群metapopulation富营养化现象eutrohication改良relamation盖度coverage盖度比cover ratio干扰disturbance干扰斑块disturbance patch干扰廊道disturbance corridor干扰作用interference高度height高斯假说Coarse's hypothesis高斯理论Coarse's theory高位芽植物phanerophytes格林威尔造山运动Grenville Orogenesis个体individual个体论概念individualistic concept更新renewal功能生态位functional niche攻击行为aggressive behavior构件modules构件生物modular organism关键种keystone species关联系数association coefficients光饱和点light saturation point光补偿点light compensation point光周期photoperiod过滤器filter哈德-温伯格原理Hardy—Weinberg principle 海洋生态系统Ocean ecosytem寒武纪Cambrian period旱生植物siccocolous河流廊道river corridor恒有度contancy红树林mangrove呼吸量respiration互利mutualism互利素synomone互利作用synomonal化感作用allelopathy化学防御chemical defence化学生态学chemical ecology化学物质allelochemicals化学隐藏chemocryptic划分的divisive环境environment环境伦理学environmental ethics环境容纳量environmental carryin capacity环境资源斑块environmental resource patch环境资源廊道environmental resource corridor 荒漠desert荒漠化desertification荒漠生态系统desert ecosystem黄化现象eitiolation phenomenon恢复生态学restoration ecology混沌学chaos混合型mixed type活动库exchange pool获得性行为acquired behavior机体论学派organismic school基础生态位Fundamental niche基质matrix极点排序法polar ordination集群型clumped寄生parasitism加速期accelerating phase价值value价值流value flow间接排序indirect ordination间接梯度分析indirect gradiant analysis减速期decelerating phase简单聚合法lumping碱性植物alkaline soil plant建群种constructive species接触化学感觉contact chemoreception解磷菌或溶磷菌Phosphate-solubiIizing Microorganisms, PSM 进化适应evolutionary adaptation经典型复合种群classic metapopulation经济密度economic density景观landscape景观格局landscape patten景观过程模型process based landscape model景观结构landscape structure景观空间动态模型spatial dynamic landscape model 景观生态学landscape ecology净初级生产量net primary production竞争competition竞争排斥原理competition exclusion principle静态生命表static life table局部种群local population距离效应distance effect聚合的agglomerative均匀型uniform菌根mycorrhiza抗毒素phytoalexins可持续发展sustainable development空间结构spatial structure空间模型spatial model空间生态位spatial niche空间异质性spatial heterogeneity库pool矿产资源mineral resources廊道corridor离散性discrete利己素allomone利己作用allomona利他行为altruism利他作用kairomonal连续体continuum联想学习associative learning猎食行为hunting behavior林冠火crown fire磷循环phosphorus cycling零假说null hypothesis领悟学习insight learning领域性territoriality流flow绿色核算green accounting逻辑斯谛方程logistic equation铆钉假说Rivet hypothesis密度density密度比density ratio密度制约死亡density-dependent mortality 面积效应area effect灭绝extinction铭记imprinting模拟hametic模型modeling牧食食物链grazing food chain内禀增长率intrinsic rate of increase内稳态homeostasis内稳态生物homeostatic organisms内源性endogenous内在的intrinsic耐阴植物shade-enduring plants能量分配原则principle of energy allocation 能量流动energy flow能源资源energy resources能值emergy泥盆纪Devonian period拟寄生parasitoidism逆分析inverse analysis年龄分布age distribution年龄结构age structure年龄性别锥体age-sex pyramid年龄锥体age pyramids偶见种rare species排序ordination配额quota配偶选择mate selection偏害amensalism偏利commensalism频度frequency平衡选择balancing selection平台plantform平行进化parallel evolution栖息地habitat期望值外推法extrapolation by expected value 气候驯化acclimatisation器官organs亲本投资parental investment亲族选择kin selection趋光性phototaxis趋化性chemotaxis趋同进化convergent evolution趋性taxis趋异进化divergent evolution趋异适应radiation adaptation取食促进剂oviposition and feeding stimulant 取样调查法sampling methods去除取样法removal sampling全联法complete linkage全球global全球变暖global warnning全球定位系统global Positioning System全球生态学global ecology确限度fidelity群丛association群丛单位理论association unit theory群丛组association group群落community群落的垂直结构vertical structure群落生态学community ecology群落水平格局horizontal pattern群落外貌physiognomy群落演替succession群系formation群系组formation group热带旱生林tropical dry forest热带季雨林tropical seasonal rainforest热带稀树草原tropical savanna热带雨林tropical rainforest热力学第二定律second law of thermodynamics 热力学第一定律first law of thermodynamics 人工斑块introduced patch人工廊道introduced corridor人口调查法cencus technique人口统计学human demography日中性植物day neutral plant冗余redundancy冗余种假说Redundancy species hypothesis三叠纪Triassic period森林生态系统forest ecosystem熵值entropy value上渐线upper asymptotic社会性防卫行为defence behavior社会优势等级dominance hierarchy摄食行为feed behavior生活史life history生活史对策life history strategy生活小区biotope生活型life form生活周期life cycle生境habitat生境多样性假说habitat diversity hypothesis 生理出生率physiological natality生理死亡率physiological mortality生命表life table生态出生率ecological natality生态对策bionomic strategy生态反作用ecological reaction生态幅ecological amplitude生态工程ecological engineering生态工业ecological industry生态规划ecological planning生态恢复ecological restoration生态经济ecological economics生态旅游ecotourism生态密度ecological density生态农业ecological agriculture生态入侵ecological invasion生态设计ecological design生态适应ecological adaptation生态死亡率ecological mortality生态位niche生态位宽度niche breadth生态位相似性比例niche proportional similarity 生态位重叠niche overlap生态文明ecological civilization生态系统ecosystem生态系统产品ecosystem goods生态系统多样性ecosystem diversity生态系统服务ecosystem service生态系统生态学ecosystem ecology生态系统学ecosystemology生态型ecotype生态学ecology生态因子ecological factor生态元ecological unit生态作用ecological effect生物organism生物地球化学循环biogecochemical cycle 生物多样性biodiversity生物量biomass生物潜能biotic potential生物群落biotic community,biome生物群落演替succession生殖潜能reproductive potential剩余空间residual space失共生aposymbiosis湿地wetland湿地生态系统wetland ecosystem湿地植物hygrophyte时间结构temporal structure实际出生率realized natality实际死亡率realized mortality食草动物herbivores食肉动物carnivores食物链food chain食物网food wed矢量vector适合度fitness适应辐射adaptive radiation适应值adaptive value适应组合adaptive suites收获理论harvest theory收益外泄externalized profit衰退型种群contracting population水平格局horizontal pattern水土流失soil and water erosion水循环water cycling瞬时增长率instantaneous rate死亡率mortality & death rate松散垂直耦连loose vertical coupling松散水平耦连loose horizontal coupling溯祖过程coalescent process溯祖理论coalescent theory酸性土理论acid soil plant酸雨acid rain随机型random碎屑食物链detritus food chainK-对策者K—strategistisn维超体积资源空间n-dimensional hyper—volume n维生态位n—dimensional nicheRaunkiaer定律Law of Frequencyr-对策者r-strategistis奥陶纪Ordovician period白垩土草地chalk grassland斑块patch斑块性patchiness斑块性种群patchy population半荒漠semi-desert半矩阵或星系图constellation diagrams伴生种companion species饱和密度saturation density饱和期asymptotic phase保护哲学conservation philosophy北方针叶林northern conifer forest被动取样假说passive sampling hypothesis 本能instinct本能行为instinctive behavior避敌avoiding predator边缘效应edge effect。
人工智能领域中英文专有名词汇总
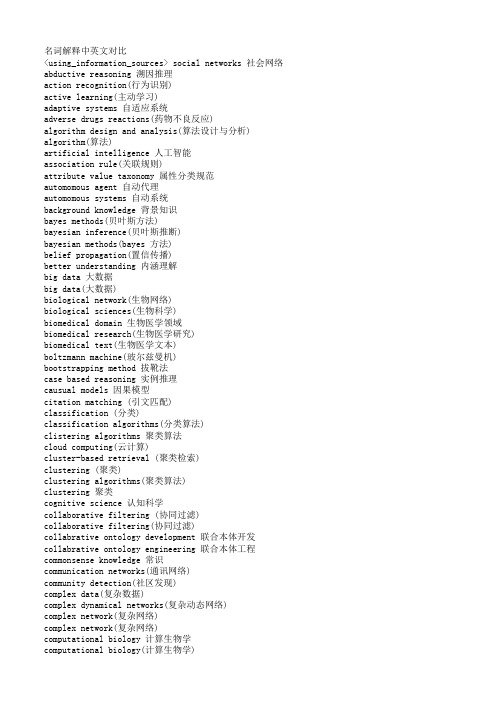
名词解释中英文对比<using_information_sources> social networks 社会网络abductive reasoning 溯因推理action recognition(行为识别)active learning(主动学习)adaptive systems 自适应系统adverse drugs reactions(药物不良反应)algorithm design and analysis(算法设计与分析) algorithm(算法)artificial intelligence 人工智能association rule(关联规则)attribute value taxonomy 属性分类规范automomous agent 自动代理automomous systems 自动系统background knowledge 背景知识bayes methods(贝叶斯方法)bayesian inference(贝叶斯推断)bayesian methods(bayes 方法)belief propagation(置信传播)better understanding 内涵理解big data 大数据big data(大数据)biological network(生物网络)biological sciences(生物科学)biomedical domain 生物医学领域biomedical research(生物医学研究)biomedical text(生物医学文本)boltzmann machine(玻尔兹曼机)bootstrapping method 拔靴法case based reasoning 实例推理causual models 因果模型citation matching (引文匹配)classification (分类)classification algorithms(分类算法)clistering algorithms 聚类算法cloud computing(云计算)cluster-based retrieval (聚类检索)clustering (聚类)clustering algorithms(聚类算法)clustering 聚类cognitive science 认知科学collaborative filtering (协同过滤)collaborative filtering(协同过滤)collabrative ontology development 联合本体开发collabrative ontology engineering 联合本体工程commonsense knowledge 常识communication networks(通讯网络)community detection(社区发现)complex data(复杂数据)complex dynamical networks(复杂动态网络)complex network(复杂网络)complex network(复杂网络)computational biology 计算生物学computational biology(计算生物学)computational complexity(计算复杂性) computational intelligence 智能计算computational modeling(计算模型)computer animation(计算机动画)computer networks(计算机网络)computer science 计算机科学concept clustering 概念聚类concept formation 概念形成concept learning 概念学习concept map 概念图concept model 概念模型concept modelling 概念模型conceptual model 概念模型conditional random field(条件随机场模型) conjunctive quries 合取查询constrained least squares (约束最小二乘) convex programming(凸规划)convolutional neural networks(卷积神经网络) customer relationship management(客户关系管理) data analysis(数据分析)data analysis(数据分析)data center(数据中心)data clustering (数据聚类)data compression(数据压缩)data envelopment analysis (数据包络分析)data fusion 数据融合data generation(数据生成)data handling(数据处理)data hierarchy (数据层次)data integration(数据整合)data integrity 数据完整性data intensive computing(数据密集型计算)data management 数据管理data management(数据管理)data management(数据管理)data miningdata mining 数据挖掘data model 数据模型data models(数据模型)data partitioning 数据划分data point(数据点)data privacy(数据隐私)data security(数据安全)data stream(数据流)data streams(数据流)data structure( 数据结构)data structure(数据结构)data visualisation(数据可视化)data visualization 数据可视化data visualization(数据可视化)data warehouse(数据仓库)data warehouses(数据仓库)data warehousing(数据仓库)database management systems(数据库管理系统)database management(数据库管理)date interlinking 日期互联date linking 日期链接Decision analysis(决策分析)decision maker 决策者decision making (决策)decision models 决策模型decision models 决策模型decision rule 决策规则decision support system 决策支持系统decision support systems (决策支持系统) decision tree(决策树)decission tree 决策树deep belief network(深度信念网络)deep learning(深度学习)defult reasoning 默认推理density estimation(密度估计)design methodology 设计方法论dimension reduction(降维) dimensionality reduction(降维)directed graph(有向图)disaster management 灾害管理disastrous event(灾难性事件)discovery(知识发现)dissimilarity (相异性)distributed databases 分布式数据库distributed databases(分布式数据库) distributed query 分布式查询document clustering (文档聚类)domain experts 领域专家domain knowledge 领域知识domain specific language 领域专用语言dynamic databases(动态数据库)dynamic logic 动态逻辑dynamic network(动态网络)dynamic system(动态系统)earth mover's distance(EMD 距离) education 教育efficient algorithm(有效算法)electric commerce 电子商务electronic health records(电子健康档案) entity disambiguation 实体消歧entity recognition 实体识别entity recognition(实体识别)entity resolution 实体解析event detection 事件检测event detection(事件检测)event extraction 事件抽取event identificaton 事件识别exhaustive indexing 完整索引expert system 专家系统expert systems(专家系统)explanation based learning 解释学习factor graph(因子图)feature extraction 特征提取feature extraction(特征提取)feature extraction(特征提取)feature selection (特征选择)feature selection 特征选择feature selection(特征选择)feature space 特征空间first order logic 一阶逻辑formal logic 形式逻辑formal meaning prepresentation 形式意义表示formal semantics 形式语义formal specification 形式描述frame based system 框为本的系统frequent itemsets(频繁项目集)frequent pattern(频繁模式)fuzzy clustering (模糊聚类)fuzzy clustering (模糊聚类)fuzzy clustering (模糊聚类)fuzzy data mining(模糊数据挖掘)fuzzy logic 模糊逻辑fuzzy set theory(模糊集合论)fuzzy set(模糊集)fuzzy sets 模糊集合fuzzy systems 模糊系统gaussian processes(高斯过程)gene expression data 基因表达数据gene expression(基因表达)generative model(生成模型)generative model(生成模型)genetic algorithm 遗传算法genome wide association study(全基因组关联分析) graph classification(图分类)graph classification(图分类)graph clustering(图聚类)graph data(图数据)graph data(图形数据)graph database 图数据库graph database(图数据库)graph mining(图挖掘)graph mining(图挖掘)graph partitioning 图划分graph query 图查询graph structure(图结构)graph theory(图论)graph theory(图论)graph theory(图论)graph theroy 图论graph visualization(图形可视化)graphical user interface 图形用户界面graphical user interfaces(图形用户界面)health care 卫生保健health care(卫生保健)heterogeneous data source 异构数据源heterogeneous data(异构数据)heterogeneous database 异构数据库heterogeneous information network(异构信息网络) heterogeneous network(异构网络)heterogenous ontology 异构本体heuristic rule 启发式规则hidden markov model(隐马尔可夫模型)hidden markov model(隐马尔可夫模型)hidden markov models(隐马尔可夫模型) hierarchical clustering (层次聚类) homogeneous network(同构网络)human centered computing 人机交互技术human computer interaction 人机交互human interaction 人机交互human robot interaction 人机交互image classification(图像分类)image clustering (图像聚类)image mining( 图像挖掘)image reconstruction(图像重建)image retrieval (图像检索)image segmentation(图像分割)inconsistent ontology 本体不一致incremental learning(增量学习)inductive learning (归纳学习)inference mechanisms 推理机制inference mechanisms(推理机制)inference rule 推理规则information cascades(信息追随)information diffusion(信息扩散)information extraction 信息提取information filtering(信息过滤)information filtering(信息过滤)information integration(信息集成)information network analysis(信息网络分析) information network mining(信息网络挖掘) information network(信息网络)information processing 信息处理information processing 信息处理information resource management (信息资源管理) information retrieval models(信息检索模型) information retrieval 信息检索information retrieval(信息检索)information retrieval(信息检索)information science 情报科学information sources 信息源information system( 信息系统)information system(信息系统)information technology(信息技术)information visualization(信息可视化)instance matching 实例匹配intelligent assistant 智能辅助intelligent systems 智能系统interaction network(交互网络)interactive visualization(交互式可视化)kernel function(核函数)kernel operator (核算子)keyword search(关键字检索)knowledege reuse 知识再利用knowledgeknowledgeknowledge acquisitionknowledge base 知识库knowledge based system 知识系统knowledge building 知识建构knowledge capture 知识获取knowledge construction 知识建构knowledge discovery(知识发现)knowledge extraction 知识提取knowledge fusion 知识融合knowledge integrationknowledge management systems 知识管理系统knowledge management 知识管理knowledge management(知识管理)knowledge model 知识模型knowledge reasoningknowledge representationknowledge representation(知识表达) knowledge sharing 知识共享knowledge storageknowledge technology 知识技术knowledge verification 知识验证language model(语言模型)language modeling approach(语言模型方法) large graph(大图)large graph(大图)learning(无监督学习)life science 生命科学linear programming(线性规划)link analysis (链接分析)link prediction(链接预测)link prediction(链接预测)link prediction(链接预测)linked data(关联数据)location based service(基于位置的服务) loclation based services(基于位置的服务) logic programming 逻辑编程logical implication 逻辑蕴涵logistic regression(logistic 回归)machine learning 机器学习machine translation(机器翻译)management system(管理系统)management( 知识管理)manifold learning(流形学习)markov chains 马尔可夫链markov processes(马尔可夫过程)matching function 匹配函数matrix decomposition(矩阵分解)matrix decomposition(矩阵分解)maximum likelihood estimation(最大似然估计)medical research(医学研究)mixture of gaussians(混合高斯模型)mobile computing(移动计算)multi agnet systems 多智能体系统multiagent systems 多智能体系统multimedia 多媒体natural language processing 自然语言处理natural language processing(自然语言处理) nearest neighbor (近邻)network analysis( 网络分析)network analysis(网络分析)network analysis(网络分析)network formation(组网)network structure(网络结构)network theory(网络理论)network topology(网络拓扑)network visualization(网络可视化)neural network(神经网络)neural networks (神经网络)neural networks(神经网络)nonlinear dynamics(非线性动力学)nonmonotonic reasoning 非单调推理nonnegative matrix factorization (非负矩阵分解) nonnegative matrix factorization(非负矩阵分解) object detection(目标检测)object oriented 面向对象object recognition(目标识别)object recognition(目标识别)online community(网络社区)online social network(在线社交网络)online social networks(在线社交网络)ontology alignment 本体映射ontology development 本体开发ontology engineering 本体工程ontology evolution 本体演化ontology extraction 本体抽取ontology interoperablity 互用性本体ontology language 本体语言ontology mapping 本体映射ontology matching 本体匹配ontology versioning 本体版本ontology 本体论open government data 政府公开数据opinion analysis(舆情分析)opinion mining(意见挖掘)opinion mining(意见挖掘)outlier detection(孤立点检测)parallel processing(并行处理)patient care(病人医疗护理)pattern classification(模式分类)pattern matching(模式匹配)pattern mining(模式挖掘)pattern recognition 模式识别pattern recognition(模式识别)pattern recognition(模式识别)personal data(个人数据)prediction algorithms(预测算法)predictive model 预测模型predictive models(预测模型)privacy preservation(隐私保护)probabilistic logic(概率逻辑)probabilistic logic(概率逻辑)probabilistic model(概率模型)probabilistic model(概率模型)probability distribution(概率分布)probability distribution(概率分布)project management(项目管理)pruning technique(修剪技术)quality management 质量管理query expansion(查询扩展)query language 查询语言query language(查询语言)query processing(查询处理)query rewrite 查询重写question answering system 问答系统random forest(随机森林)random graph(随机图)random processes(随机过程)random walk(随机游走)range query(范围查询)RDF database 资源描述框架数据库RDF query 资源描述框架查询RDF repository 资源描述框架存储库RDF storge 资源描述框架存储real time(实时)recommender system(推荐系统)recommender system(推荐系统)recommender systems 推荐系统recommender systems(推荐系统)record linkage 记录链接recurrent neural network(递归神经网络) regression(回归)reinforcement learning 强化学习reinforcement learning(强化学习)relation extraction 关系抽取relational database 关系数据库relational learning 关系学习relevance feedback (相关反馈)resource description framework 资源描述框架restricted boltzmann machines(受限玻尔兹曼机) retrieval models(检索模型)rough set theroy 粗糙集理论rough set 粗糙集rule based system 基于规则系统rule based 基于规则rule induction (规则归纳)rule learning (规则学习)rule learning 规则学习schema mapping 模式映射schema matching 模式匹配scientific domain 科学域search problems(搜索问题)semantic (web) technology 语义技术semantic analysis 语义分析semantic annotation 语义标注semantic computing 语义计算semantic integration 语义集成semantic interpretation 语义解释semantic model 语义模型semantic network 语义网络semantic relatedness 语义相关性semantic relation learning 语义关系学习semantic search 语义检索semantic similarity 语义相似度semantic similarity(语义相似度)semantic web rule language 语义网规则语言semantic web 语义网semantic web(语义网)semantic workflow 语义工作流semi supervised learning(半监督学习)sensor data(传感器数据)sensor networks(传感器网络)sentiment analysis(情感分析)sentiment analysis(情感分析)sequential pattern(序列模式)service oriented architecture 面向服务的体系结构shortest path(最短路径)similar kernel function(相似核函数)similarity measure(相似性度量)similarity relationship (相似关系)similarity search(相似搜索)similarity(相似性)situation aware 情境感知social behavior(社交行为)social influence(社会影响)social interaction(社交互动)social interaction(社交互动)social learning(社会学习)social life networks(社交生活网络)social machine 社交机器social media(社交媒体)social media(社交媒体)social media(社交媒体)social network analysis 社会网络分析social network analysis(社交网络分析)social network(社交网络)social network(社交网络)social science(社会科学)social tagging system(社交标签系统)social tagging(社交标签)social web(社交网页)sparse coding(稀疏编码)sparse matrices(稀疏矩阵)sparse representation(稀疏表示)spatial database(空间数据库)spatial reasoning 空间推理statistical analysis(统计分析)statistical model 统计模型string matching(串匹配)structural risk minimization (结构风险最小化) structured data 结构化数据subgraph matching 子图匹配subspace clustering(子空间聚类)supervised learning( 有support vector machine 支持向量机support vector machines(支持向量机)system dynamics(系统动力学)tag recommendation(标签推荐)taxonmy induction 感应规范temporal logic 时态逻辑temporal reasoning 时序推理text analysis(文本分析)text anaylsis 文本分析text classification (文本分类)text data(文本数据)text mining technique(文本挖掘技术)text mining 文本挖掘text mining(文本挖掘)text summarization(文本摘要)thesaurus alignment 同义对齐time frequency analysis(时频分析)time series analysis( 时time series data(时间序列数据)time series data(时间序列数据)time series(时间序列)topic model(主题模型)topic modeling(主题模型)transfer learning 迁移学习triple store 三元组存储uncertainty reasoning 不精确推理undirected graph(无向图)unified modeling language 统一建模语言unsupervisedupper bound(上界)user behavior(用户行为)user generated content(用户生成内容)utility mining(效用挖掘)visual analytics(可视化分析)visual content(视觉内容)visual representation(视觉表征)visualisation(可视化)visualization technique(可视化技术) visualization tool(可视化工具)web 2.0(网络2.0)web forum(web 论坛)web mining(网络挖掘)web of data 数据网web ontology lanuage 网络本体语言web pages(web 页面)web resource 网络资源web science 万维科学web search (网络检索)web usage mining(web 使用挖掘)wireless networks 无线网络world knowledge 世界知识world wide web 万维网world wide web(万维网)xml database 可扩展标志语言数据库附录 2 Data Mining 知识图谱(共包含二级节点15 个,三级节点93 个)间序列分析)监督学习)领域 二级分类 三级分类。
基于提前终止策略改进的运动估计算法

第 22卷第 7期2023年 7月Vol.22 No.7Jul.2023软件导刊Software Guide基于提前终止策略改进的运动估计算法朱鑫磊,汪伟(上海理工大学光电信息与计算机工程学院,上海 200093)摘要:针对HM-16.14中TZSearch标准算法存在的计算复杂度高、耗时相对较长等问题,提出一种基于提前终止策略的改进TZSearch算法。
首先,根据编码产生的率失真代价对编码单元、变换单元和预测单元的深度进行划分,有效避免了额外的划分深度;然后,在TZSearch初始网格搜索过程中,采用钻石搜索和六边形搜索两种搜索方式,根据运动矢量分布位置选择一种更为有效的方式,精确找出最佳匹配点;最后,使用OARP栅格搜索和精细搜索完成运动估计。
由实验结果可知,该方法与标准算法相比,平均降低了60%以上的TZSearch运动估计耗时,且基本不影响视频质量。
关键词:TZSearch算法;提前终止策略;栅格搜索;精细搜索;运动估计DOI:10.11907/rjdk.221887开放科学(资源服务)标识码(OSID):中图分类号:TP391.1 文献标识码:A文章编号:1672-7800(2023)007-0051-08A Modified Motion Estimation Algorithm Based on Early Termination StrategyZHU Xinlei, WANG Wei(School of Optical-Electrical and Computer Engineering, University of Shanghai for Science and Technology,Shanghai 200093, China)Abstract:Considering the high computational complexity and relatively long time consumption of the TZSearch standard algorithm within HM-16.14, an improved TZSearch algorithm based on early termination strategy is proposed to improve the efficiency of video coding. Firstly,the depth sorting of the coding unit, transform unit and prediction unit is calculated according to the performance of rate distortion, which can effectively decrease additional division depths. Secondly, two search methods, i.e. diamond search and hexagonal search, are employed within the initial grid search step of TZSearch in order to precisely find the best matching point according to the motion vector distribution. Finally,OARP raster search and fine search are used to acquire the motion estimation results. Compared with the standard algorithm, experimental re‐sults show that the proposed method reduces more than 60% motion estimation time consumption on average, yet keeps the similar video quali‐ty .Key Words:TZSearch algorithm; early termination strategy; raster search; fine search; motion estimation0 引言随着视频技术的快速发展,依靠视频传递信息变得越来越普及,这使得视频流数据在互联网传输中的占比越来越大。
基于多Agent的Web个性化信息检索模型

S p. 0 0 e 2 1
文 章 编 号 :62 7 1 (0 0 0 — 0 10 17 — 0 0 2 1 ) 3 0 2 — 5
基 于 多 A e t We gn的 b个 性 化 信 息 检 索模 型
梁 高卫
(邵 阳学院 信 息工程 系, 南 邵 阳 4 2 0 湖 2 0 0) 摘 要 :提 出 了一 种基 于 多 A e t的 W b个 性 化 信 息检 索系 统 的模 型 ( PR — A , 模 型利 用 gn e W IS M ) 该
第 7卷 第 3期 21 0 0年 9月
邵 阳学 院 学 报 ( 自然 科 学版 )
J un lo h o a gUnv st ( Naua ce c E io o ra fS a y n ieri y t rl in e dt n) S i
V0 . No. I7 3
领 域 的一 个 研 究 热点 . 当前 , 索 引擎 是 用 户 查 搜
个搜 索 满 足所 有 用 户 ” 的模 型 , 得 所 有 的 用 使
W b文档 测 试 分析 结 果表 明 ,该 模 型 可 以保 证 良好 的关键 词 集 的正 确 率 ,用 户查 询 满 意度 达 到 8 % e 0以
上 .
关 键 词 : e ; Aet个 性化 ; 息检 索 Wb 多 gn; 信 中图分 类 号 : P 9 T3 1 文献 标识 码 : A
n lg fmu t l tl g n g ns c mb n n ed s r t n o u r s a d e t ci gt eu e s p tn i tr s n h r ce i- o o y o l p e i e ie ta e t, o i i gt e c p i f e e , n xr t h s r ’ oe t i e e t a d e a a tr i n l h i o q i a n l a n s s
数据库英文参考文献(最新推荐120个)

由于我国经济的高速发展,计算机科学技术在当前各个科技领域中迅速发展,成为了应用最广泛的技术之一.其中数据库又是计算机科学技术中发展最快,应用最广泛的重要分支之一.它已成为计算机信息系统和计算机应用系统的重要技术基础和支柱。
下面是数据库英文参考文献的分享,希望对你有所帮助。
数据库英文参考文献一:[1]Nú?ez Matías,Weht Ruben,Nú?ez Regueiro Manuel. Searching for electronically two dimensional metals in high-throughput ab initio databases[J]. Computational Materials Science,2020,182.[2]Izabela Karsznia,Marta Przychodzeń,Karolina Sielicka. Methodology of the automatic generalization of buildings, road networks, forests and surface waters: a case study based on the Topographic Objects Database in Poland[J]. Geocarto International,2020,35(7).[3]Alankrit Chaturvedi. Secure Cloud Migration Challenges and Solutions[J]. Journal of Research in Science and Engineering,2020,2(4).[4]Ivana Nin?evi? Pa?ali?,Maja ?uku?i?,Mario Jadri?. Smart city research advances in Southeast Europe[J]. International Journal of Information Management,2020.[5]Jongseong Kim,Unil Yun,Eunchul Yoon,Jerry Chun-Wei Lin,Philippe Fournier-Viger. One scan based high average-utility pattern mining in static and dynamic databases[J]. Future Generation Computer Systems,2020.[6]Jo?o Peixoto Martins,António Andrade-Campos,Sandrine Thuillier. Calibration of Johnson-Cook Model Using Heterogeneous Thermo-Mechanical Tests[J]. Procedia Manufacturing,2020,47.[7]Anna Soriani,Roberto Gemignani,Matteo Strano. A Metamodel for the Management of Large Databases: Toward Industry 4.0 in Metal Forming[J]. Procedia Manufacturing,2020,47.[8]Ayman Elbadawi,Karim Mahmoud,Islam Y. Elgendy,Mohammed Elzeneini,Michael Megaly,Gbolahan Ogunbayo,Mohamed A. Omer,Michelle Albert,Samir Kapadia,Hani Jneid. Racial disparities in the utilization and outcomes of transcatheter mitral valve repair: Insights from a national database[J]. Cardiovascular Revascularization Medicine,2020.[9]Maurizio Boccia,Antonio Sforza,Claudio Sterle. Simple Pattern Minimality Problems: Integer Linear Programming Formulations and Covering-Based Heuristic Solving Approaches[J]. INFORMS Journal on Computing,2020.[10]. Inc.; Patent Issued for Systems And User Interfaces For Dynamic Access Of Multiple Remote Databases And Synchronization Of Data Based On User Rules (USPTO 10,628,448)[J]. Computer Technology Journal,2020.[11]. Bank of America Corporation; Patent Issued for System For Electronic Data Verification, Storage, And Transfer (USPTO 10,628,058)[J]. Computer Technology Journal,2020.[12]. Information Technology - Database Management; Data from Technical University Munich (TU Munich) Advance Knowledge in Database Management (Make the most out of your SIMD investments: counter control flow divergence in compiled query pipelines)[J]. Computer Technology Journal,2020.[13]. Information Technology - Database Management; Studies from Pontifical Catholic University Update Current Data on Database Management (General dynamic Yannakakis: conjunctive queries with theta joins under updates)[J]. Computer Technology Journal,2020.[14]Kimothi Dhananjay,Biyani Pravesh,Hogan James M,Soni Akshay,Kelly Wayne. Learning supervised embeddings for large scale sequence comparisons.[J]. PloS one,2020,15(3).[15]. Information Technology; Studies from University of California San Diego (UCSD) Reveal New Findings on Information Technology (A Physics-constrained Data-driven Approach Based On Locally Convex Reconstruction for Noisy Database)[J]. Information Technology Newsweekly,2020.[16]. Information Technology; Researchers from National Institute of Information and Communications Technology Describe Findings in Information Technology (Efficient Discovery of Weighted Frequent Neighborhood Itemsets in Very Large Spatiotemporal Databases)[J]. Information Technology Newsweekly,2020.[17]. Information Technology; Investigators at Gdansk University of Technology Report Findings in Information Technology (A Framework for Accelerated Optimization of Antennas Using Design Database and Initial Parameter Set Estimation)[J]. Information Technology Newsweekly,2020.[18]. Information Technology; Study Results from Palacky University Update Understanding of Information Technology (Evaluation of Replication Mechanisms on Selected Database Systems)[J]. Information Technology Newsweekly,2020.[19]Runfola Daniel,Anderson Austin,Baier Heather,Crittenden Matt,Dowker Elizabeth,Fuhrig Sydney,Goodman Seth,Grimsley Grace,Layko Rachel,MelvilleGraham,Mulder Maddy,Oberman Rachel,Panganiban Joshua,Peck Andrew,Seitz Leigh,Shea Sylvia,Slevin Hannah,Youngerman Rebecca,Hobbs Lauren. geoBoundaries: A global database of political administrative boundaries.[J]. PloS one,2020,15(4).[20]Dupré Damien,Krumhuber Eva G,Küster Dennis,McKeown Gary J. A performance comparison of eight commercially available automatic classifiers for facial affect recognition.[J]. PloS one,2020,15(4).[21]Partha Pratim Banik,Rappy Saha,Ki-Doo Kim. An Automatic Nucleus Segmentation and CNN Model based Classification Method of White Blood Cell[J]. Expert Systems With Applications,2020,149.[22]Hang Dong,Wei Wang,Frans Coenen,Kaizhu Huang. Knowledge base enrichment by relation learning from social tagging data[J]. Information Sciences,2020,526.[23]Xiaodong Zhao,Dechang Pi,Junfu Chen. Novel trajectory privacy-preserving method based on clustering using differential privacy[J]. Expert Systems With Applications,2020,149.[24]. Information Technology; Researchers at Beijing University of Posts and Telecommunications Have Reported New Data on Information Technology (Mining top-k sequential patterns in transaction database graphs)[J]. Internet Weekly News,2020.[25]Sunil Kumar Sharma. An empirical model (EM: CCO) for clustering, convergence and center optimization in distributive databases[J]. Journal of Ambient Intelligence and Humanized Computing,2020(prepublish).[26]Naryzhny Stanislav,Klopov Nikolay,Ronzhina Natalia,Zorina Elena,Zgoda Victor,Kleyst Olga,Belyakova Natalia,Legina Olga. A database for inventory of proteoform profiles: "2DE-pattern".[J]. Electrophoresis,2020.[27]Noel Varela,Jesus Silva,Fredy Marin Gonzalez,Pablo Palencia,Hugo Hernandez Palma,Omar Bonerge Pineda. Method for the Recovery of Images in Databases of Rice Grains from Visual Content[J]. Procedia Computer Science,2020,170.[28]Ahmad Rabanimotlagh,Prabhu Janakaraj,Pu Wang. Optimal Crowd-Augmented Spectrum Mapping via an Iterative Bayesian Decision Framework[J]. Ad Hoc Networks,2020.[29]Ismail Boucherit,Mohamed Ould Zmirli,Hamza Hentabli,Bakhtiar Affendi Rosdi. Finger vein identification using deeply-fused Convolutional Neural Network[J]. Journal of King Saud University - Computer and Information Sciences,2020.[30]Sachin P. Patel,S.H. Upadhyay. Euclidean Distance based Feature Ranking andSubset Selection for Bearing Fault Diagnosis[J]. Expert Systems With Applications,2020.[31]Julia Fomina,Denis Safikanov,Alexey Artamonov,Evgeniy Tretyakov. Parametric and semantic analytical search indexes in hieroglyphic languages[J]. Procedia Computer Science,2020,169.[32]Selvine G. Mathias,Sebastian Schmied,Daniel Grossmann. An Investigation on Database Connections in OPC UA Applications[J]. Procedia Computer Science,2020,170.[33]Abdourrahmane Mahamane Atto,Alexandre Benoit,Patrick Lambert. Timed-image based deep learning for action recognition in video sequences[J]. Pattern Recognition,2020.[34]Yonis Gulzar,Ali A. Alwan,Abedallah Zaid Abualkishik,Abid Mehmood. A Model for Computing Skyline Data Items in Cloud Incomplete Databases[J]. Procedia Computer Science,2020,170.[35]Xiaohan Yang,Fan Li,Hantao Liu. Deep feature importance awareness based no-reference image quality prediction[J]. Neurocomputing,2020.[36]Dilana Hazer-Rau,Sascha Meudt,Andreas Daucher,Jennifer Spohrs,Holger Hoffmann,Friedhelm Schwenker,Harald C. Traue. The uulmMAC Database—A Multimodal Affective Corpus for Affective Computing in Human-Computer Interaction[J]. Sensors,2020,20(8).[37]Tomá? Pohanka,Vilém Pechanec. Evaluation of Replication Mechanisms on Selected Database Systems[J]. ISPRS International Journal of Geo-Information,2020,9(4).[38]Verheggen Kenneth,Raeder Helge,Berven Frode S,Martens Lennart,Barsnes Harald,Vaudel Marc. Anatomy and evolution of database search engines-a central component of mass spectrometry based proteomic workflows.[J]. Mass spectrometry reviews,2020,39(3).[39]Moscona Leon,Casta?eda Pablo,Masrouha Karim. Citation analysis of the highest-cited articles on developmental dysplasia of the hip.[J]. Journal of pediatric orthopedics. Part B,2020,29(3).[40]Nasseh Daniel,Schneiderbauer Sophie,Lange Michael,Schweizer Diana,Heinemann Volker,Belka Claus,Cadenovic Ranko,Buysse Laurence,Erickson Nicole,Mueller Michael,Kortuem Karsten,Niyazi Maximilian,Marschner Sebastian,Fey Theres. Optimizing the Analytical Value of Oncology-Related Data Based on an In-Memory Analysis Layer: Development and Assessment of the Munich OnlineComprehensive Cancer Analysis Platform.[J]. Journal of medical Internet research,2020,22(4).数据库英文参考文献二:[41]Meiling Chai,Changgeng Li,Hui Huang. A New Indoor Positioning Algorithm of Cellular and Wi-Fi Networks[J]. Journal of Navigation,2020,73(3).[42]Mandy Watson. How to undertake a literature search: a step-by-step guide[J]. British Journal of Nursing,2020,29(7).[43]. Patent Application; "Memorial Facility With Memorabilia, Meeting Room, Secure Memorial Database, And Data Needed For An Interactive Computer Conversation With The Deceased" in Patent Application Approval Process (USPTO 20200089455)[J]. Computer Technology Journal,2020.[44]. Information Technology; Data on Information Technology Detailed by Researchers at Complutense University Madrid (Hr-sql: Extending Sql With Hypothetical Reasoning and Improved Recursion for Current Database Systems)[J]. Computer Technology Journal,2020.[45]. Science - Metabolomics; Study Data from Wake Forest University School of Medicine Update Knowledge of Metabolomics (Software tools, databases and resources in metabolomics: updates from 2018 to 2019)[J]. Computer Technology Journal,2020.[46]. Sigma Computing Inc.; Researchers Submit Patent Application, "GeneratingA Database Query To Dynamically Aggregate Rows Of A Data Set", for Approval (USPTO 20200089796)[J]. Computer Technology Journal,2020.[47]. Machine Learning; Findings on Machine Learning Reported by Investigators at Tongji University (Comparing Machine Learning Algorithms In Predicting Thermal Sensation Using Ashrae Comfort Database Ii)[J]. Computer Technology Journal,2020.[48]. Sigma Computing Inc.; "Generating A Database Query Using A Dimensional Hierarchy Within A Graphical User Interface" in Patent Application Approval Process (USPTO 20200089794)[J]. Computer Technology Journal,2020.[49]Qizhi He,Jiun-Shyan Chen. A physics-constrained data-driven approach based on locally convex reconstruction for noisy database[J]. Computer Methods in Applied Mechanics and Engineering,2020,363.[50]José A. Delgado-Osuna,Carlos García-Martínez,JoséGómez-Barbadillo,Sebastián Ventura. Heuristics for interesting class association rule mining a colorectal cancer database[J]. Information Processing andManagement,2020,57(3).[51]Edival Lima,Thales Vieira,Evandro de Barros Costa. Evaluating deep models for absenteeism prediction of public security agents[J]. Applied Soft Computing Journal,2020,91.[52]S. Fareri,G. Fantoni,F. Chiarello,E. Coli,A. Binda. Estimating Industry 4.0 impact on job profiles and skills using text mining[J]. Computers in Industry,2020,118.[53]Estrela Carlos,Pécora Jesus Djalma,Dami?o Sousa-Neto Manoel. The Contribution of the Brazilian Dental Journal to the Brazilian Scientific Research over 30 Years.[J]. Brazilian dental journal,2020,31(1).[54]van den Oever L B,Vonder M,van Assen M,van Ooijen P M A,de Bock G H,Xie X Q,Vliegenthart R. Application of artificial intelligence in cardiac CT: From basics to clinical practice.[J]. European journal of radiology,2020,128.[55]Li Liu,Deborah Silver,Karen Bemis. Visualizing events in time-varying scientific data[J]. Journal of Visualization,2020,23(2–3).[56]. Information Technology - Database Management; Data on Database Management Discussed by Researchers at Arizona State University (Architecture of a Distributed Storage That Combines File System, Memory and Computation In a Single Layer)[J]. Information Technology Newsweekly,2020.[57]. Information Technology - Database Management; New Findings from Guangzhou Medical University Update Understanding of Database Management (GREG-studying transcriptional regulation using integrative graph databases)[J]. Information Technology Newsweekly,2020.[58]. Technology - Laser Research; Reports from Nicolaus Copernicus University in Torun Add New Data to Findings in Laser Research (Nonlinear optical study of Schiff bases using Z-scan technique)[J]. Journal of Technology,2020.[59]Loeffler Caitlin,Karlsberg Aaron,Martin Lana S,Eskin Eleazar,Koslicki David,Mangul Serghei. Improving the usability and comprehensiveness of microbial databases.[J]. BMC biology,2020,18(1).[60]Caitlin Loeffler,Aaron Karlsberg,Lana S. Martin,Eleazar Eskin,David Koslicki,Serghei Mangul. Improving the usability and comprehensiveness of microbial databases[J]. BMC Biology,2020,18(1).[61]Dean H. Barrett,Aderemi Haruna. Artificial intelligence and machine learningfor targeted energy storage solutions[J]. Current Opinion in Electrochemistry,2020,21.[62]Chenghao Sun. Research on investment decision-making model from the perspective of “Internet of Things + Big data”[J]. Future Generation Computer Systems,2020,107.[63]Sa?a Adamovi?,Vladislav Mi?kovic,Nemanja Ma?ek,Milan Milosavljevi?,Marko ?arac,Muzafer Sara?evi?,Milan Gnjatovi?. An efficient novel approach for iris recognition based on stylometric features and machine learning techniques[J]. Future Generation Computer Systems,2020,107.[64]Olivier Pivert,Etienne Scholly,Grégory Smits,Virginie Thion. Fuzzy quality-Aware queries to graph databases[J]. Information Sciences,2020,521.[65]Javier Fernando Botía Valderrama,Diego José Luis Botía Valderrama. Two cluster validity indices for the LAMDA clustering method[J]. Applied Soft Computing Journal,2020,89.[66]Amer N. Kadri,Marie Bernardo,Steven W. Werns,Amr E. Abbas. TAVR VS. SAVR IN PATIENTS WITH CANCER AND AORTIC STENOSIS: A NATIONWIDE READMISSION DATABASE REGISTRY STUDY[J]. Journal of the American College of Cardiology,2020,75(11).[67]. Information Technology; Findings from P. Sjolund and Co-Authors Update Knowledge of Information Technology (Whole-genome sequencing of human remains to enable genealogy DNA database searches - A case report)[J]. Information Technology Newsweekly,2020.[68]. Information Technology; New Findings from P. Yan and Co-Researchers in the Area of Information Technology Described (BrainEXP: a database featuring with spatiotemporal expression variations and co-expression organizations in human brains)[J]. Information Technology Newsweekly,2020.[69]. IDERA; IDERA Database Tools Expand Support for Cloud-Hosted Databases[J]. Information Technology Newsweekly,2020.[70]Adrienne Warner,David A. Hurley,Jonathan Wheeler,Todd Quinn. Proactive chat in research databases: Inviting new and different questions[J]. The Journal of Academic Librarianship,2020,46(2).[71]Chidentree Treesatayapun. Discrete-time adaptive controller based on IF-THEN rules database for novel architecture of ABB IRB-1400[J]. Journal of the Franklin Institute,2020.[72]Tian Fang,Tan Han,Cheng Zhang,Ya Juan Yao. Research and Construction of the Online Pesticide Information Center and Discovery Platform Based on Web Crawler[J]. Procedia Computer Science,2020,166.[73]Dinusha Vatsalan,Peter Christen,Erhard Rahm. Incremental clustering techniques for multi-party Privacy-Preserving Record Linkage[J]. Data & Knowledge Engineering,2020.[74]Ying Xin Liu,Xi Yuan Li. Design and Implementation of a Business Platform System Based on Java[J]. Procedia Computer Science,2020,166.[75]Akhilesh Kumar Bajpai,Sravanthi Davuluri,Kriti Tiwary,Sithalechumi Narayanan,Sailaja Oguru,Kavyashree Basavaraju,Deena Dayalan,Kavitha Thirumurugan,Kshitish K. Acharya. Systematic comparison of the protein-protein interaction databases from a user's perspective[J]. Journal of Biomedical Informatics,2020,103.[76]P. Raveendra,V. Siva Reddy,G.V. Subbaiah. Vision based weed recognition using LabVIEW environment for agricultural applications[J]. Materials Today: Proceedings,2020,23(Pt 3).[77]Christine Rosati,Emily Bakinowski. Preparing for the Implementation of an Agnis Enabled Data Reporting System and Comprehensive Research Level Data Repository for All Cellular Therapy Patients[J]. Biology of Blood and Marrow Transplantation,2020,26(3).[78]Zeiser Felipe André,da Costa Cristiano André,Zonta Tiago,Marques Nuno M C,Roehe Adriana Vial,Moreno Marcelo,da Rosa Righi Rodrigo. Segmentation of Masses on Mammograms Using Data Augmentation and Deep Learning.[J]. Journal of digital imaging,2020.[79]Dhaked Devendra K,Guasch Laura,Nicklaus Marc C. Tautomer Database: A Comprehensive Resource for Tautomerism Analyses.[J]. Journal of chemical information and modeling,2020,60(3).[80]Pian Cong,Zhang Guangle,Gao Libin,Fan Xiaodan,Li Fei. miR+Pathway: the integration and visualization of miRNA and KEGG pathways.[J]. Briefings in bioinformatics,2020,21(2).数据库英文参考文献三:[81]Marcello W. M. Ribeiro,Alexandre A. B. Lima,Daniel Oliveira. OLAP parallel query processing in clouds with C‐ParGRES[J]. Concurrency and Computation: Practice and Experience,2020,32(7).[82]Li Gao,Peng Lin,Peng Chen,Rui‐Zhi Gao,Hong Yang,Yun He,Jia‐Bo Chen,Yi ‐Ge Luo,Qiong‐Qian Xu,Song‐Wu Liang,Jin‐Han Gu,Zhi‐Guang Huang,Yi‐Wu Dang,Gang Chen. A novel risk signature that combines 10 long noncoding RNAs to predict neuroblastoma prognosis[J]. Journal of Cellular Physiology,2020,235(4).[83]Julia Krzykalla,Axel Benner,Annette Kopp‐Schneider. Exploratory identification of predictive biomarkers in randomized trials with normal endpoints[J]. Statistics in Medicine,2020,39(7).[84]Jianye Ching,Kok-Kwang Phoon. Measuring Similarity between Site-Specific Data and Records from Other Sites[J]. ASCE-ASME Journal of Risk and Uncertainty in Engineering Systems, Part A: Civil Engineering,2020,6(2).[85]Anne Kelly Knowles,Justus Hillebrand,Paul B. Jaskot,Anika Walke. Integrative, Interdisciplinary Database Design for the Spatial Humanities: the Case of the Holocaust Ghettos Project[J]. International Journal of Humanities and Arts Computing,2020,14(1-2).[86]Sheng-Feng Sung,Pei-Ju Lee,Cheng-Yang Hsieh,Wan-Lun Zheng. Medication Use and the Risk of Newly Diagnosed Diabetes in Patients with Epilepsy: A Data Mining Application on a Healthcare Database[J]. Journal of Organizational and End User Computing (JOEUC),2020,32(2).[87]Rashkovits Rami,Lavy Ilana. Students' Difficulties in Identifying the Use of Ternary Relationships in Data Modeling[J]. International Journal of Information and Communication Technology Education (IJICTE,2020,16(2).[88]Yusuf Akhtar,Dipti Prasad Mukherjee. Context-based ensemble classification for the detection of architectural distortion in a digitised mammogram[J]. IET Image Processing,2020,14(4).[89]Gurpreet Kaur,Sukhwinder Singh,Renu Vig. Medical fusion framework using discrete fractional wavelets and non-subsampled directional filter banks[J]. IET Image Processing,2020,14(4).[90]Qian Liu,Bo Jiang,Jia-lei Zhang,Peng Gao,Zhi-jian Xia. Semi-supervised uncorrelated dictionary learning for colour face recognition[J]. IET Computer Vision,2020,14(3).[91]Yipo Huang,Leida Li,Yu Zhou,Bo Hu. No-reference quality assessment for live broadcasting videos in temporal and spatial domains[J]. IET Image Processing,2020,14(4).[92]Panetta Karen,Wan Qianwen,Agaian Sos,Rajeev Srijith,Kamath Shreyas,Rajendran Rahul,Rao Shishir Paramathma,Kaszowska Aleksandra,Taylor Holly A,Samani Arash,Yuan Xin. A Comprehensive Database for Benchmarking Imaging Systems.[J]. IEEE transactions on pattern analysis and machine intelligence,2020,42(3).[93]Rahnev Dobromir,Desender Kobe,Lee Alan L F,Adler William T,Aguilar-Lleyda David,Akdo?an Ba?ak,Arbuzova Polina,Atlas Lauren Y,Balc? Fuat,Bang Ji Won,Bègue Indrit,Birney Damian P,Brady Timothy F,Calder-Travis Joshua,Chetverikov Andrey,Clark Torin K,Davranche Karen,Denison Rachel N,Dildine Troy C,Double Kit S,Duyan Yaln A,Faivre Nathan,Fallow Kaitlyn,Filevich Elisa,Gajdos Thibault,Gallagher Regan M,de Gardelle Vincent,Gherman Sabina,Haddara Nadia,Hainguerlot Marine,Hsu Tzu-Yu,Hu Xiao,Iturrate I?aki,Jaquiery Matt,Kantner Justin,Koculak Marcin,Konishi Mahiko,Ko? Christina,Kvam Peter D,Kwok Sze Chai,Lebreton Ma?l,Lempert Karolina M,Ming Lo Chien,Luo Liang,Maniscalco Brian,Martin Antonio,Massoni Sébastien,Matthews Julian,Mazancieux Audrey,Merfeld Daniel M,O'Hora Denis,Palser Eleanor R,Paulewicz Borys?aw,Pereira Michael,Peters Caroline,Philiastides Marios G,Pfuhl Gerit,Prieto Fernanda,Rausch Manuel,Recht Samuel,Reyes Gabriel,Rouault Marion,Sackur Jér?me,Sadeghi Saeedeh,Samaha Jason,Seow Tricia X F,Shekhar Medha,Sherman Maxine T,Siedlecka Marta,Skóra Zuzanna,Song Chen,Soto David,Sun Sai,van Boxtel Jeroen J A,Wang Shuo,Weidemann Christoph T,Weindel Gabriel,WierzchońMicha?,Xu Xinming,Ye Qun,Yeon Jiwon,Zou Futing,Zylberberg Ariel. The Confidence Database.[J]. Nature human behaviour,2020,4(3).[94]Taipalus Toni. The Effects of Database Complexity on SQL Query Formulation[J]. Journal of Systems and Software,2020(prepublish).[95]. Information Technology; Investigators from Deakin University Target Information Technology (Conjunctive query pattern structures: A relational database model for Formal Concept Analysis)[J]. Computer Technology Journal,2020.[96]. Machine Learning; Findings from Rensselaer Polytechnic Institute Broaden Understanding of Machine Learning (Self Healing Databases for Predictive Risk Analytics In Safety-critical Systems)[J]. Computer Technology Journal,2020.[97]. Science - Library Science; Investigators from Cumhuriyet University Release New Data on Library Science (Scholarly databases under scrutiny)[J]. Computer Technology Journal,2020.[98]. Information Technology; Investigators from Faculty of Computer Science and Engineering Release New Data on Information Technology (FGSA for optimal quality of service based transaction in real-time database systems under different workload condition)[J]. Computer Technology Journal,2020.[99]Muhammad Aqib Javed,M.A. Naveed,Azam Hussain,S. Hussain. Integrated data acquisition, storage and retrieval for glass spherical tokamak (GLAST)[J]. Fusion Engineering and Design,2020,152.[100]Vinay M.S.,Jayant R. Haritsa. Operator implementation of Result Set Dependent KWS scoring functions[J]. Information Systems,2020,89.[101]. Capital One Services LLC; Patent Issued for Computer-Based Systems Configured For Managing Authentication Challenge Questions In A Database And Methods Of Use (USPTO 10,572,653)[J]. Journal of Robotics & Machine Learning,2020.[102]Ikawa Fusao,Michihata Nobuaki. In Reply to Letter to the Editor Regarding "Treatment Risk for Elderly Patients with Unruptured Cerebral Aneurysm from a Nationwide Database in Japan".[J]. World neurosurgery,2020,135.[103]Chen Wei,You Chao. Letter to the Editor Regarding "Treatment Risk for Elderly Patients with Unruptured Cerebral Aneurysm from a Nationwide Database in Japan".[J]. World neurosurgery,2020,135.[104]Zhitao Xiao,Lei Pei,Lei Geng,Ying Sun,Fang Zhang,Jun Wu. Surface Parameter Measurement of Braided Composite Preform Based on Faster R-CNN[J]. Fibers and Polymers,2020,21(3).[105]Xiaoyu Cui,Ruifan Cai,Xiangjun Tang,Zhigang Deng,Xiaogang Jin. Sketch‐based shape‐constrained fireworks simulation in head‐mounted virtual reality[J]. Computer Animation and Virtual Worlds,2020,31(2).[106]Klaus B?hm,Tibor Kubjatko,Daniel Paula,Hans-Georg Schweiger. New developments on EDR (Event Data Recorder) for automated vehicles[J]. Open Engineering,2020,10(1).[107]Ming Li,Ruizhi Chen,Xuan Liao,Bingxuan Guo,Weilong Zhang,Ge Guo. A Precise Indoor Visual Positioning Approach Using a Built Image Feature Database and Single User Image from Smartphone Cameras[J]. Remote Sensing,2020,12(5).[108]Matthew Grewe,Phillip Sexton,David Dellenbach. Use Risk‐Based Asset Prioritization to Develop Accurate Capital Budgets[J]. Opflow,2020,46(3).[109]Jose R. Salvador,D. Mu?oz de la Pe?a,D.R. Ramirez,T. Alamo. Predictive control of a water distribution system based on process historian data[J]. Optimal Control Applications and Methods,2020,41(2).[110]Esmaeil Nourani,Vahideh Reshadat. Association extraction from biomedicalliterature based on representation and transfer learning[J]. Journal of Theoretical Biology,2020,488.[111]Ikram Saima,Ahmad Jamshaid,Durdagi Serdar. Screening of FDA approved drugs for finding potential inhibitors against Granzyme B as a potent drug-repurposing target.[J]. Journal of molecular graphics & modelling,2020,95.[112]Keiron O’Shea,Biswapriya B. Misra. Software tools, databases and resources in metabolomics: updates from 2018 to 2019[J]. Metabolomics,2020,16(D1).[113]. Information Technology; Researchers from Virginia Polytechnic Institute and State University (Virginia Tech) Describe Findings in Information Technology (A database for global soil health assessment)[J]. Energy & Ecology,2020.[114]Moosa Johra Muhammad,Guan Shenheng,Moran Michael F,Ma Bin. Repeat-Preserving Decoy Database for False Discovery Rate Estimation in Peptide Identification.[J]. Journal of proteome research,2020,19(3).[115]Huttunen Janne M J,K?rkk?inen Leo,Honkala Mikko,Lindholm Harri. Deep learning for prediction of cardiac indices from photoplethysmographic waveform: A virtual database approach.[J]. International journal for numerical methods in biomedical engineering,2020,36(3).[116]Kunxia Wang,Guoxin Su,Li Liu,Shu Wang. Wavelet packet analysis for speaker-independent emotion recognition[J]. Neurocomputing,2020.[117]Fusao Ikawa,Nobuaki Michihata. In Reply to Letter to the Editor Regarding “Treatment Risk for Elderly Patients with Unruptured Cerebral Aneurysm from a Nationwide Database in Japan”[J]. World Neurosurgery,2020,135.[118]Wei Chen,Chao You. Letter to the Editor Regarding “Treatment Risk for Elderly Patients with Unruptured Cerebral Aneurysm from a Nationwide Database in Japan”[J]. World Neurosurgery,2020,135.[119]Lindsey A. Parsons,Jonathan A. Jenks,Andrew J. Gregory. Accuracy Assessment of National Land Cover Database Shrubland Products on the Sagebrush Steppe Fringe[J]. Rangeland Ecology & Management,2020,73(2).[120]Jing Hua,Yilu Xu,Jianjun Tang,Jizhong Liu,Jihao Zhang. ECG heartbeat classification in compressive domain for wearable devices[J]. Journal of Systems Architecture,2020,104.以上就是关于数据库英文参考文献的全部内容,希望看完后对你有所启发。
自然语言处理在信息检索中的最新进展
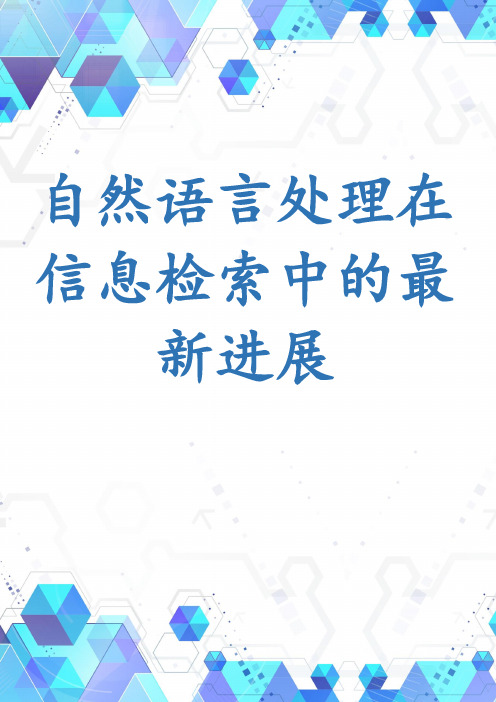
自然语言处理在信息检索中的最新进展自然语言处理在信息检索中的最新进展自然语言处理(NLP)是人工智能领域中的一个重要分支,它致力于使计算机能够理解和处理人类自然语言的方式。
在信息检索领域,NLP的最新进展为提高搜索引擎的准确性和用户体验提供了巨大的潜力。
本文将逐步介绍NLP在信息检索中的最新进展。
第一步:文本预处理和词嵌入NLP在信息检索中的第一步是对文本进行预处理。
这包括去除停用词、标点符号和特殊字符,对文本进行分词以及将文本转换为小写。
预处理后,文本需要通过词嵌入技术转换为向量表示。
词嵌入是一种将单词映射到低维向量空间的技术,它能够保留单词之间的语义关系。
近年来,基于深度神经网络的词嵌入方法如Word2Vec和BERT取得了显著的进展。
第二步:查询理解和语义匹配在信息检索中,用户输入的查询语句需要被准确理解并与文档进行语义匹配。
传统的基于关键词匹配的方法存在一定的局限性。
最新的NLP技术通过使用预训练的模型,如BERT和ELMo,可以更准确地理解查询的语义。
这些模型能够将查询语句转换为向量表示,并与文档进行语义匹配,从而提高搜索结果的准确性。
第三步:知识图谱和实体识别知识图谱是一种将实体、关系和属性组织成图形结构的方法。
最新的NLP技术可以通过实体识别和关系抽取从文本中提取实体和关系,并构建知识图谱。
这使得搜索引擎可以更好地理解文档中的实体,并根据实体之间的关系提供更准确的搜索结果。
第四步:多模态检索和跨语言检索NLP最新的进展还包括多模态检索和跨语言检索。
多模态检索是指在搜索引擎中集成多种媒体内容,如图像、音频和视频。
通过使用深度学习技术,可以将多种媒体内容转换为向量表示,并将其与文本进行匹配。
跨语言检索是指在不同语言之间进行搜索。
最新的NLP技术可以实现将查询语句从一种语言翻译到另一种语言,并在目标语言中进行搜索。
第五步:个性化搜索和推荐系统最新的NLP技术还广泛应用于个性化搜索和推荐系统中。
适应性绩效研究简述

适应性绩效研究简述摘要适应性绩效是绩效研究中除任务绩效和关系绩效外的第三个维度,主要是对人的适应环境的能力进行的研究,对人力资源管理有一定的影响。
本文首先简述了适应性绩效研究提出的背景,接着对适应性绩效国内研究进行了一个简单的述评,最后提出一些研究展望。
关键词适应性适应性绩效研究简述中图分类号:c390 文献标识码:a一、适应性绩效提出的背景适应性本身并不是一个新的概念,但由于当今组织的变革速度加快和变革的种类增加,致使这种变化的、动态的组织特征对员工的适应性提出了更高的要求,适应性绩效也由此应运而生。
针对这种情况,最先由allworth & hesketh(1997)指出不但要强调任务绩效和关系绩效,而且要增加员工应对环境变化的适应性绩效成分(adaptive performance),他们通过两个大样本(n=317,n=368)的研究,证实在任务绩效和关系绩效之外,适应性绩效确实独立存在,这一结论充实和完善了工作绩效理论。
campell(1999)也指出,其早期工作绩效结构框架的确遗漏了员工适应新情境和工作要求的适应性绩效这一个应该包含的重要成份,随后适应性绩效理论在学术界被广泛的研究。
二、适应性绩效国外研究简述在适应性绩效这一概念提出之后,学术界对适应性绩效的研究越来越重视,在国外的研究中,适应性绩效的概念也相应出现了一些不同的版本,但是对于适应性绩效定义的研究,最具有权威并且影响力最大的还是pulakos等人的观点。
pulakos等人(2000)在美国《应用心理学》杂志上发表了“工作场所中的适应性:适应性绩效分类的开发”一文,介绍了适应性绩效系统研究的结果。
他们在有关适应性研究(如个体层次、群体层次和组织层次的适应性研究;角色弹性;个体自我管理等研究)的基础上,提出了8个维度的适应性绩效。
这八个维度的名称及维度定义如下:(一)处理紧急事件或危机情境。
能采取合适的紧急措施应对生活中的威胁、危险或危急状态;快速分析各种备选方案以应对危险、危机等;应用清晰、集中的思维快速决策;控制情感、客观性地关注当前情境;必要时,逐步采取行动解决危险或危机。
Usage-based adaptable taxonomy
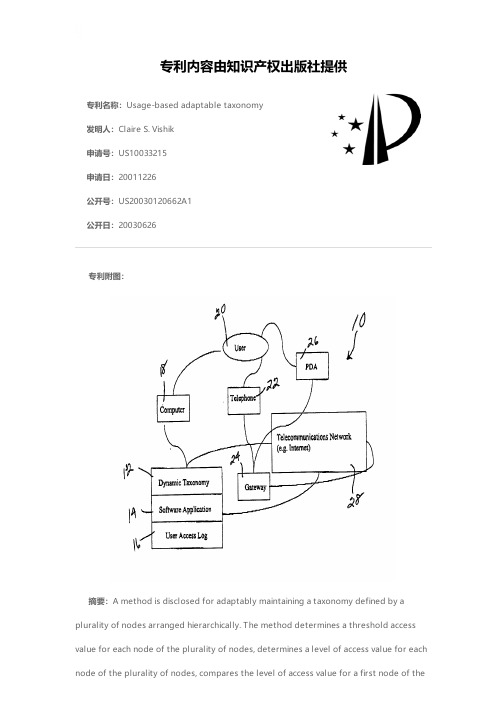
专利名称:Usage-based adaptable taxonomy发明人:Claire S. Vishik申请号:US10033215申请日:20011226公开号:US20030120662A1公开日:20030626专利内容由知识产权出版社提供专利附图:摘要:A method is disclosed for adaptably maintaining a taxonomy defined by a plurality of nodes arranged hierarchically. The method determines a threshold access value for each node of the plurality of nodes, determines a level of access value for each node of the plurality of nodes, compares the level of access value for a first node of theplurality of nodes with the threshold access value for the first node of the plurality of nodes, and if the level of access value for the first node is less than the threshold access value for the first node, merges the first node with a related node arranged laterally to the first node in the hierarchical arrangement, and compares the level of access value for the first node of the plurality of nodes with the threshold access value for a second node of the plurality of nodes, and if the level of access value for the first node is greater than the threshold access value for the second node, promotes the first node to a higher level in the hierarchical arrangement than the second node, and if the level of access value for the first node is less than the threshold access value for the second node, demotes the first node to a lower level in the hierarchical arrangement than the second node.申请人:VISHIK CLAIRE S.更多信息请下载全文后查看。
基于深度学习的局部实例搜索
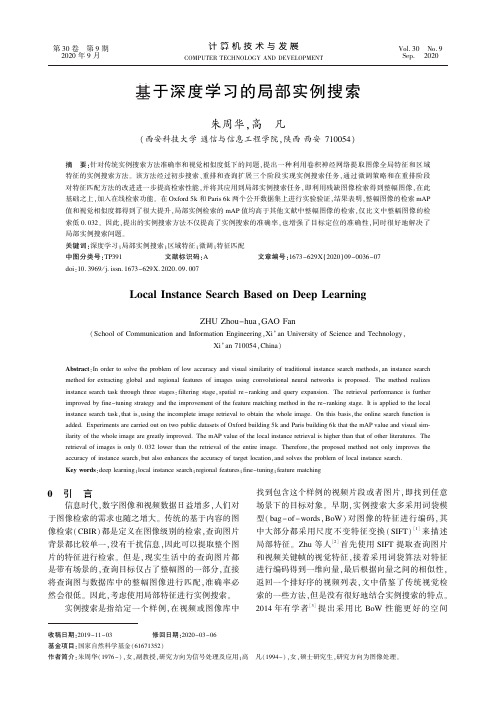
收稿日期:2019-11-03 修回日期:2020-03-06基金项目:国家自然科学基金(61671352)作者简介:朱周华(1976-),女,副教授,研究方向为信号处理及应用;高 凡(1994-),女,硕士研究生,研究方向为图像处理㊂基于深度学习的局部实例搜索朱周华,高 凡(西安科技大学通信与信息工程学院,陕西西安710054)摘 要:针对传统实例搜索方法准确率和视觉相似度低下的问题,提出一种利用卷积神经网络提取图像全局特征和区域特征的实例搜索方法㊂该方法经过初步搜索㊁重排和查询扩展三个阶段实现实例搜索任务,通过微调策略和在重排阶段对特征匹配方法的改进进一步提高检索性能,并将其应用到局部实例搜索任务,即利用残缺图像检索得到整幅图像,在此基础之上,加入在线检索功能㊂在Oxford 5k 和Paris 6k 两个公开数据集上进行实验验证,结果表明,整幅图像的检索mAP 值和视觉相似度都得到了很大提升,局部实例检索的mAP 值均高于其他文献中整幅图像的检索,仅比文中整幅图像的检索低0.032㊂因此,提出的实例搜索方法不仅提高了实例搜索的准确率,也增强了目标定位的准确性,同时很好地解决了局部实例搜索问题㊂关键词:深度学习;局部实例搜索;区域特征;微调;特征匹配中图分类号:TP 391 文献标识码:A 文章编号:1673-629X (2020)09-0036-07doi :10.3969/j.issn.1673-629X.2020.09.007Local Instance Search Based on Deep LearningZHU Zhou -hua ,GAO Fan(School of Communication and Information Engineering ,Xi ’an University of Science and Technology ,Xi ’an 710054,China )Abstract :In order to solve the problem of low accuracy and visual similarity of traditional instance search methods ,an instance search method for extracting global and regional features of images using convolutional neural networks is proposed.The method realizes instance search task through three stages :filtering stage ,spatial re -ranking and query expansion.The retrieval performance is further improved by fine -tuning strategy and the improvement of the feature matching method in the re -ranking stage.It is applied to the local instance search task ,that is ,using the incomplete image retrieval to obtain the whole image.On this basis ,the online search function is added.Experiments are carried out on two public datasets of Oxford building 5k and Paris building 6k that the mAP value and visual sim⁃ilarity of the whole image are greatly improved.The mAP value of the local instance retrieval is higher than that of other literatures.The retrieval of images is only 0.032lower than the retrieval of the entire image.Therefore ,the proposed method not only improves the accuracy of instance search ,but also enhances the accuracy of target location ,and solves the problem of local instance search.Key words :deep learning ;local instance search ;regional features ;fine -tuning ;feature matching0 引 言信息时代,数字图像和视频数据日益增多,人们对于图像检索的需求也随之增大㊂传统的基于内容的图像检索(CBIR )都是定义在图像级别的检索,查询图片背景都比较单一,没有干扰信息,因此可以提取整个图片的特征进行检索㊂但是,现实生活中的查询图片都是带有场景的,查询目标仅占了整幅图的一部分,直接将查询图与数据库中的整幅图像进行匹配,准确率必然会很低㊂因此,考虑使用局部特征进行实例搜索㊂实例搜索是指给定一个样例,在视频或图像库中找到包含这个样例的视频片段或者图片,即找到任意场景下的目标对象㊂早期,实例搜索大多采用词袋模型(bag -of -words ,BoW )对图像的特征进行编码,其中大部分都采用尺度不变特征变换(SIFT )[1]来描述局部特征㊂Zhu 等人[2]首先使用SIFT 提取查询图片和视频关键帧的视觉特征,接着采用词袋算法对特征进行编码得到一维向量,最后根据向量之间的相似性,返回一个排好序的视频列表,文中借鉴了传统视觉检索的一些方法,但是没有很好地结合实例搜索的特点㊂2014年有学者[3]提出采用比BoW 性能更好的空间第30卷 第9期2020年9月 计算机技术与发展COMPUTER TECHNOLOGY AND DEVELOPMENT Vol.30 No.9Sep. 2020Fisher向量[4]和局部特征聚合描述符(VLAD)[5]来描述SIFT特征的空间关系,从而进行实例检索㊂随着深度学习的发展,深度卷积神经网络特征被广泛应用于计算机视觉的各个领域,如图像分类[6-7]㊁语音识别[8]等,均取得了不错的效果,因此有学者也将其引入到图像检索领域㊂起初,研究者们利用神经网络的全连接层特征进行图像检索[9],后来很多研究者开始转向卷积层特征的研究[10],并且证明卷积层特征的性能更好㊂Eva等人[11]采用词袋模型对卷积神经网络(CNNs)提取的特征进行编码,然后分别进行初次搜索,局部重排,扩展查询,从而实现实例检索㊂实例检索需采用局部特征实现,因此许多生成区域信息的方法相继出现,最简单的是滑动窗口,之后有学者提出使用Selective Search生成物体候选框[12-13],但是这些方法将生成候选区域和特征提取分开进行㊂Faster R-CNN[14]是一个端到端的网络,它可以同时提取卷积层特征和生成候选区域㊂文献[15]提出将微调之后的目标检测网络Faster R-CNN应用到实例检索中,使用区域提议网络(region proposal network, RPN)生成候选区域,从而得到查询图区域特征与数据库图像区域特征,特征匹配之后排序得到检索结果,在两个建筑物数据集上取得了不错的效果㊂何涛在其论文中[16]针对Faster R-CNN网络效率较低的问题提出了端到端的深度区域哈希网络(DRH),使用VGG16作为特征提取器,滑动窗口和RPN网络得到候选区域,并将两种方法进行对比,整个网络最后阶段对特征进行哈希编码并计算汉明距离进行排序,从而得到检索结果,文中为了排除不同场景㊁不同光照和拍照角度产生的干扰,使用局部信息进行检索㊂以上两篇文献尽管均使用局部信息进行检索,但都是为了排除干扰信息将查询图中的目标标记出来,查询图依然是整幅图像㊂实际搜索图像时某些图片会有残缺,此时就无法通过标记目标进行检索,因此实例检索除了常见的利用局部特征进行整幅图像的检索之外,局部图像的检索亦有着非常重要的现实意义㊂现有的检索方法的检索效果不是很理想,因此文中针对以上两个问题首先改进整幅图像的检索并提高其检索性能,之后利用图像的部分信息(例如建筑物的顶部㊁嫌疑人的部分特征等)检索得到整幅图像,实现局部图像的检索㊂同时,考虑到实际检索时,输入均为一幅图像,输出为一组图像,因此,文中在局部实例检索的基础之上加入在线检索功能,可以实现局部图像的实时搜索㊂因此,主要有以下两大方面的贡献和创新:(1)基于深度学习的实例搜索㊂一方面,通过微调策略提高了实例搜索的精确度;另一方面,针对候选框得分(scores)和余弦距(cosine)两种相似性度量方法存在的不足,提出将两种方法相结合,以获得更好的检索效果㊂(2)基于深度学习的局部实例搜索㊂由于局部图像的检索具有重大的现实意义,将全局实例搜索算法应用在局部实例检索任务中,即利用残缺图片信息搜索得到整幅图像,并加入在线检索功能,输入局部查询图,便可以得到查询结果和所属建筑物的名字㊂1 相关理论1.1 基于Faster R-CNN的区域特征提取如图1所示,Faster R-CNN由卷积层(Conv layers),RPN网络,RoI pooling,分类和回归四部分构成㊂图1 Faster R-CNN结构 卷积层用于提取图像特征,可以选择不同结构的网络,输入为整张图片,输出为提取的特征称为feature maps㊂文中采用VGG16[7]的中间卷积层作为特征提取器㊂RPN网络用于推荐候选区域,这个网络是用来代替之前的selective search[13]的,输入为图片,输出为多个矩形区域以及对应每个矩形区域含有物体的概率㊂首先将一个3*3的滑窗在feature maps上滑动,每个窗口中心点映射到原图并生成9种矩形框(9anchor boxes),之后进入两个同级的1*1卷积,一个分支通过softmax进行二分类,判断anchor boxes属于foreground还是background㊂另一分支计算anchor㊃73㊃ 第9期 朱周华等:基于深度学习的局部实例搜索boxes的bounding box regression的偏移量㊂Proposal 层结合两个分支的输出信息去冗余后保留N个候选框,称为proposals㊂传统的CNN网络,输入图像尺寸必须是固定大小的,但是Faster R-CNN的输入是任意大小的,RoI pooling作用是根据候选区域坐标在特征图上映射得到区域特征并将其pooling成固定大小的输出,即对每个proposal提取固定尺寸的特征图㊂分类和回归模块,一方面通过全连接层和softmax 层确定每个proposal的类别,另一方面回归更加精确的目标检测框,输出候选区域在图像中的精确坐标㊂1.2 微调Faster R-CNN微调,即用预训练模型重新训练自己的数据,现有的VGG16FasterR-CNN预训练模型主要是基于VOC2007数据集中20类常见的物体预训练得到的㊂文中的数据集是建筑物,与VOC2007图片相似度和特征都相差较大,如果依然采用预训练模型,效果必然不好,再加上从头开始训练,需要大量的数据㊁时间和计算资源㊂而文中所选用的数据集较小,因此需要进行微调,这样不仅可以节省大量时间和计算资源,同时还可以得到一个较好的模型㊂微调主要分为以下三个步骤:(1)数据预处理㊂首先需要对数据集进行数据清洗,主要去除无效值和纠正错误数据,提高数据质量㊂其次,由于文中的数据集较小且每类样本分布不均衡,因此选择性地对每类图片作图像增强处理,其中图像增强方法包括水平翻转,增加高斯噪声,模糊处理,改变图像对比度㊂最后将每幅图片中的目标样例标记出来㊂(2)建立训练集和测试集㊂一般是按一定的比例进行分配,但是文中选用的数据集存在样本不均衡的问题,有些类别特别多,有些类别特别少㊂由于是将所有图片全部放入同一个文件夹,然后依次读取样本分配训练集和测试集,如果按比例分配,小类样本参与训练的机会就会比大类少,训练出来的模型将会偏向于大类,使得大类性能好,小类性能差㊂平衡采样策略就是把样本按类别分组,每个类别生成一个样本列表,制作训练集时从各个类别所对应的样本列表中随机选择样本,这样可以保证每个类别参与训练的机会比较均衡㊂(3)修改相关网络参数,进行训练㊂主要修改网络文件中的类别数和类名,然后不断调节超参数,使性能达到最好㊂1.3 实例搜索实例检索一般经过初次搜索,局部重排,扩展查询三部分完成㊂初次搜索首先提取查询图和数据库所有图像的全局特征,然后计算特征之间的相似度,最后经过排序得到初步的检索结果㊂文中提取VGG16网络的最后一个卷积层(Conv5_3)特征㊂局部重排是将初次搜索得到的前K幅图片作为新的数据库进行重排,基本思路是提取查询图和数据库的区域特征并进行匹配,根据匹配结果进行排序从而得到查询结果㊂这里查询图和数据库的区域特征提取方法不同,其中,查询图的区域特征是用groundtruth 给定的边界框对整幅图像特征进行裁剪得到的,而数据库的区域特征是经过RPN网络和RoI pooling池化得到的特征(pool5)㊂扩展查询(query expansion,QE)是取出局部重排返回的前K个结果,对其特征求和取平均作为新的查询图,再做一次检索,属于重排的一种㊂2 实 验2.1 数据集和评价指标(1)实验环境㊂文中所有实验均在NVIDIA GeForce RTX2080上进行,所用系统为Ubuntu18.04,使用的深度学习框架为Caffe,编程语言为Python㊂(2)数据集介绍㊂在两个公开的建筑物数据集Oxford[17]和Paris[18]上进行实验㊂其中Oxford包含5063张图片,Paris包含6412张图片,但是有20张被损坏,因此有6392张图片可用㊂两个数据集都来自Flickr,共有11类建筑物,同一种建筑物有5张查询图,因此每个数据集总共有55张查询图,除此之外,两个数据集相同类别建筑物的场景㊁拍照角度和光照都有所不同,而且有很多本来不是同一种建筑物但是从表面看上去却非常相似的图片㊂(3)评价指标㊂平均精度均值(mean average precision,mAP)是一个反映了图像检索整体性能的指标,如式(1)和(2)所示㊂MAP=∑N k=1AP查询图的个数(1) AP=∑N k=1(P(k)㊃rel(k))相关图片个数(2)其中,N表示返回结果总个数,P(k)表示返回结果中第k个位置的查准率,rel(k)表示返回结果中第k个位置的图片是否与查询图相关,相关为1,不相关为0㊂MAP是多次查询后,对每次检索的平均精度AP值求㊃83㊃ 计算机技术与发展 第30卷和取平均㊂这里对是否相关做进一步解释:两个数据集的groundtruth有三类,分别是good,ok和junk,如果检索结果在good和ok中,则判为与查询图相关,如果在junk中,则判为不相关㊂2.2 基于深度学习的实例检索2.2.1 方 法先尝试使用VGG16Faster R-CNN预训练模型进行检索,在两个数据集上MAP值仅0.5左右,接着文中使用微调策略,只冻结了前两个卷积层,更新了之后所有的网络层权值,通过不断调参,训练一个精度尽可能高的模型㊂其中,微调过程中分别采用数据增强和平衡采样技术对数据进行处理㊂具体地,对于Oxford 数据集,建筑物radcliffe_camera的数量高达221张,而建筑物pitt_rivers仅有6张,其他9类样本数量在7至78之间不等,数量差距相当大,因此,选择性地对数量小的6类样本通过上面提到的方法进行数据增强,使小类样本数量增大㊂对其进行数据增强之后,虽然样本数量差距缩小,但是依然存在不均衡的问题,如果将所有样本放入一个文件夹中,按比例分配训练集和测试集,则依然会导致训练出来的模型小类性能差的问题㊂因此,将每类样本生成一个列表,再从每个列表中随机选取一定数量的样本作为该类的训练样本㊂除此之外,文献[15]在局部重排部分使用了两种特征匹配方法,一种是直接利用候选框对应得分(scores)进行排序㊂数据库中每幅图片经过Proposal layer会得到300个区域提议(proposal)的坐标和对应得分,找到查询图对应类的最高得分作为查询图和数据库每幅图片的相似度,再从高到低进行排序就可以得到检索结果㊂另一种是利用余弦距(cosine)进行排序㊂数据库中的每幅图片经过RoI pooling可以得到300个特征向量,计算查询图与数据库中每幅图片的300个区域特征的余弦距,最小距离对应的候选框即就是和查询图最相似的区域提议,然后把所有的最小距离再从小到大进行排序,就可以得到相似度排序㊂第一种方法虽然得到的边界框(bounding box regression)定位较准,mAP值也很高,但是视觉相似度并不是很高㊂而且根据候选框得分进行排序,每类建筑物的得分和排序都是一定的,因此每次相同类别的不同查询返回结果都是相同的,不会根据查询图片的不同而返回不同的排序㊂第二种方法得到的检索结果,图像相似度很高,但是边界框定位不是很准确㊂文中将两种方法结合(scores+cosine),利用余弦距计算相似度并排序,选择得分最高的候选框进行目标定位,这样既解决了视觉相似度不够的问题,也解决了相同类别的不同查询返回结果相同的问题,同时又解决了目标定位不准的问题㊂2.2.2 参数设置本节主要讨论扩展查询中的参数k对实验结果的影响,并选取一个最优值作为本实验的默认值㊂在特征匹配方法选用余弦距的情况下,在两个数据集上分别测试了k等于3㊁4㊁5㊁6㊁7时的mAP值,实验结果如表1所示㊂表1 不同k值对mAP值的影响k Oxford Paris30.9090.88940.9100.88850.9100.88560.9120.88970.9120.888 从表1综合来看,文中选用6作为k的默认值㊂2.2.3 结果与分析表2是文中与其他文献mAP值的对比,最后两项是文中三种方法的实验结果㊂可以看出,相比于其他文献,文中方法的检索性能得到很大的提升,比目前最好的方法分别高出6.1%和4%,说明文中方法优于其他检索方法㊂文中方法与文献[15]所采用方法类似,但结果却得到了很大的改善,通过分析认为,虽然所用数据集都相同,但是生成的训练集和测试集完全不同,而且文中采用数据增强方法,使得样本的数量增加了3~4倍,使用平衡采样的方法保证小类样本可以得到和大类样本同样的训练机会㊂除此之外,网络超参数对于模型的影响非常大,因此文中对参数进行调优,使得训练出来的模型更好㊂表2 文中方法与其他方法的mAP值对比方法Oxford ParisR.Tao等人[3]0.765-Razavian等人[10]0.5560.697Eva等人[11]0.7390.819CA-SR[15]0.7720.824CS-SR[15]0.7860.842DRH[16]0.8510.849Ours(scores)0.9030.890 Ours(cosine/cosine+scores)0.9120.890 图2是两组对比图,图(a)和图(b)是对建筑物all_ souls和louvre分别用候选框得分和余弦距进行排序的结果,其中,左边1列是查询图,右边5列是查询返回结果,表示与查询相关(下同)㊂图3是all_souls的两个不同查询用得分进行排序得到的检索结果㊂图4是将两种匹配方法结合得到的检索结果㊂从图2可以看出利用候选框得分排序得到的结果目标定位较准确,但是返回结果的背景㊁光照㊁拍照角㊃93㊃ 第9期 朱周华等:基于深度学习的局部实例搜索度㊁颜色㊁对比度和样例大小与查询图相差很大,而利用余弦距排序得到的结果候选框定位不是很准,但从背景㊁样例大小等视觉角度来看相似度较高㊂图3中all _souls 的两个不同查询图返回结果中不仅图片一样,而且顺序也相同㊂因此可以看出两种方法各有缺陷㊂图2 不同建筑物的同一个查询分别利用得分和余弦距得到的排序图3 建筑物all _souls 的两个不同查询根据得分得到的排序图4是将两种方法结合得到的返回结果,与图2相比视觉相似度提高了,目标定位也更准确了,与图3相比,同一个建筑物的两个不同查询返回结果也会根据查询图片的不同而改变,且从表2最后一行可以看出该方法比使用候选框得分在Oxford 上得到的mAP 值高0.009,与使用余弦距得到的mAP 值相同㊂因此认为,以上提出的特征匹配方法得到的返回结果在不降低mAP 值的基础上提高了检索的准确率㊂图4 综合得分和余弦距两种方法得到的检索结果2.3 基于深度学习的局部实例检索2.3.1 方 法本节局部图像的检索是建立在2.2节基础之上的,正是由于整幅图像检索采用候选区域特征实现,局部图像的检索才得以实现㊂较之于整幅图像的检索,局部图像的检索具有同样重大的现实意义㊂生活中常会因为某个原因使图片变得残缺,且难以识别,那么此时就需要使用局部图像检索得到原始图像的完整信息㊂比如,通过某建筑物的顶部搜索得到整幅图像从而识别该建筑物㊂或者可以应用在刑侦工作中,当摄像机捕获到的是某犯罪嫌疑人的部分特征时,可以通过已有的部分特征在图像库或者其他摄像头下搜索得到该嫌疑人的完整信息㊂由于目前没有一个现成的残缺图像库,因此本节利用截图工具对整幅图像作裁剪处理以模拟残缺图像,即从图像库选取不同实例通过裁剪得到不同大小,不同背景,不同角度,不同颜色的局部查询图㊂由于图像库图像都是整幅图像,在尺寸和包含的信息方面与局部查询图相差很大,因此局部检索最大的难点在于如何处理局部图像㊂2.2节会对输入图片统一进行缩放,那么局部查询图片输入后,先进行放大,则会导致原始输入图像失真,提取特征后再对其进行裁剪又会进一步丢失大部分图像信息,因此根本无法得到正确的检索结果㊂文中对其进行以下处理:即输入查询图后,先将局部查询填充至与数据库图像相同大小(图像库的图像基本都是1024*768或者768*1024大小的),这样对图像进行统一缩放,提取特征,按比例㊃04㊃ 计算机技术与发展 第30卷裁剪之后,得到的正是局部图像的特征,再与图像库匹配,则会输出正确的排序结果㊂本节输入为建筑物的局部图像,输出为局部查询所属的建筑物图像,并且会标记出局部查询在所属建筑物中的位置㊂目前,很多文献(如[15])都比较注重算法的研究,基本都采用离线的形式实现图像检索,不仅离线建立特征库,查询图也是成批输入到网络中进行离线检索,得到的结果也是成批保存起来,可是实际应用中,一般都是将查询图逐幅输入进行实时检索,因此文中在前文基础之上,加入了在线检索功能,最后实现在线局部实例检索㊂2.3.2 结果与分析如图5是通过裁剪建筑物radcliffe _camera 和triomphe 的原图,得到的5个不同查询图,分别选取一幅进行检索,得到了完整的建筑物,且标记出了局部查询图像在整个建筑物中的位置,如图6所示㊂最终mAP 值分别为0.880和0.857㊂从检索结果可以看出返回结果的视觉相似度极高,目标定位准确,且mAP 值高于其他文献中整幅图像的检索准确率㊂因此,可以证明文中提出的全局搜索算法在局部图像检索任务中亦能取得很好的效果㊂图5 两种建筑物的五个局部查询图图6 两组局部查询的检索结果在此之前,只有文献[19]为了证明行人重识别系统的普适性,使用CaffeNet 和VGG 16两个网络模型在Oxford 数据集上对局部建筑物图像进行了测试,得到的mAP 值分别为0.662和0.764,远低于文中的准确率㊂因此提出的局部实例搜索的性能良好㊂在线检索功能按照输入图片,可得到查询结果和查询建筑物的名字,且文中在没有使用任何编码算法的情况下,在两个数据集上检索一幅图的平均耗时分别为5.7s 和7s ,经检测,90%的时间都耗费在利用特征向量计算相似度部分㊂如图7所示,分别是bodleian 和eiffel 的检索结果和总耗时㊂图7 在线检索结果3摇结束语为了进一步提高实例检索性能,针对以往的利用候选框得分和余弦距进行特征匹配的不足,提出将两种方法结合,即利用余弦距计算相似度并排序,选择得分最高的候选框进行目标定位㊂并使用微调策略重新训练预训练模型从而使其适用于文中的实例检索㊂相比于其他方法,文中采用的方法在性能方面有明显的提升㊂在此基础之上,利用残缺图像搜索得到整幅图像,性能高于其他文献整幅图像的检索,且仅比文中整幅图像检索低0.032,实验结果证明提出的全局搜索算法同样适用于局部图像检索任务㊂之后加入在线检索功能,在没有任何编码的情况下检索一幅图像平均耗时仅需5.7s ~7s ㊂在未来的工作中,可以进一步加入编码模块,以提高检索速度,并且可以在更大的数据集上进行测试㊂参考文献:[1] LOWE D G.Object recognition from local scale -invariant fea⁃tures [C ]//International conference on computer vision.㊃14㊃ 第9期 朱周华等:基于深度学习的局部实例搜索Kerkyra,Greece:IEEE,1999:1150-1157.[2] ZHU C,SATOH rge vocabulary quantization for searchinginstances from videos[C]//International conference on multime⁃dia retrieval.New York,NY,United States:ACM,2012:1-8.[3] TAO R,GAVVES E,SNOEK C G M,et al.Locality in genericinstance search from one example[C]//Conference on computer vision and pattern recognition.Columbus,OH,USA:IEEE, 2014:2099-2106.[4] PERRONNIN F,LIU Y,SÁNCHEZ J,et rge-scale imageretrieval with compressed Fisher vectors[C]//Conference on computer vision and pattern recognition.San-Francisco,CA, USA:IEEE,2010:3384-3391.[5] GONG Y,WANG L,GUO R,et al.Multi-scale orderless poolingof deep convolutional activation features[C]//European confer⁃ence on computer vision.Zürich,Switzerland:Springer,2014: 392-407.[6] KRIZHEVSKY A,SUTSKEVER I,HINTON G E.Imagenetclassification with deep convolutional neural networks[C]//Neural information processing systems.New York,NY,United States:ACM,2012:1097-1105.[7] SIMONYAN K,ZISSERMAN A.Very deep convolutional net⁃works for large-scale image recognition[C]//Computer vision and pattern recognition.Boston,MA,USA:IEEE,2015:1-14.[8] HINTON G,DENG L,YU D,et al.Deep neural networks for a⁃coustic modeling in speech recognition[J].IEEE Signal Process⁃ing Magazine,2012,29(6):82-97.[9] WAN J,WANG D,HOI S C H,et al.Deep learning for content-based image retrieval:a comprehensive study[C]//International conference on multimedia.New York,NY,United States:ACM, 2014:157-166.[10]RAZAVIAN A S,SULLIVAN J,CARLSSON S,et al.Visual in⁃stance retrieval with deep convolutional networks[J].ITE Trans⁃actions on Media Technology and Applications,2016,4(3):251-258.[11]MOHEDANO E,MCGUINNESS K,O’CONNOR N E,et al.Bags of local convolutional features for scalable instance search[C]//International conference on multimedia retrieval.NewYork,NY,United States:ACM,2016:327-331. [12]UIJLINGS J R R,VAN DE SANDE K E A,GEVERS T,et al.Selective search for object recognition[J].International Journal of Computer Vision,2013,104(2):154-171.[13]GIRSHICK R,DONAHUE J,DARRELL T,et al.Rich feature hi⁃erarchies for accurate object detection and semantic segmentation[C]//Computer vision and pattern recognition.Columbus,OH,USA:IEEE,2014:580-587.[14]REN S,HE K,GIRSHICK R,et al.Faster R-CNN:towards real-time object detection with region proposal networks[C]//Neural information processing systems.New York,NY,United States: ACM,2015:91-99.[15]SALVADOR A,GIRÓ-I-NIETO X,MARQUÉS F,et al.FasterR-CNN features for instance search[C]//Conference on com⁃puter vision and pattern recognition s Vegas,NV, USA:IEEE,2016:9-16.[16]何 涛.基于深度学习和哈希的图像检索的方法研究[D].成都:电子科技大学,2018.[17]PHILBIN J,CHUM O,ISARD M,et al.Object retrieval withlarge vocabularies and fast spatial matching[C]//Conference on computer vision and pattern recognition.Minneapolis,MN,USA: IEEE,2007:1-8.[18]PHILBIN J,CHUM O,ISARD M,et al.Lost in quantization:im⁃proving particular object retrieval in large scale image databases[C]//Conference on computer vision and pattern recognition.Anchorage,AK,USA:IEEE,2008:1-8.[19]ZHENG Z,ZHENG L,YANG Y.A discriminatively learnedCNN embedding for person re-identification[J].Multimedia Computing,Communications,and Applications,2017,14(1):1-20.㊃24㊃ 计算机技术与发展 第30卷。
Measuring Information Architecture

Metadata usage in Epicurious
Ingredient
Dish
Cuisine
Prepare
Recipe
Metadata usage in Epicurious
Ingredient
Dish
Cuisine
Prepare
I
Select
Dish
Questions I want to answer
• • • • How many facets are allowable? Should facets be mixed and matched? How much is too much? How shrchies be revealed? • How should free-text search be integrated?
– How can an information architecture be designed to successfully convey scent during navigation?
– Simple measures of surface properties can fairly accurately predict website ratings. – Have not yet analyzed site-level architecture
Why measure?
• To learn what works … and what doesn‟t • To test hypotheses • My current interest:
WebPresence Sites
low
ServiceOriented Sites
基于多级全局信息传递模型的视觉显著性检测
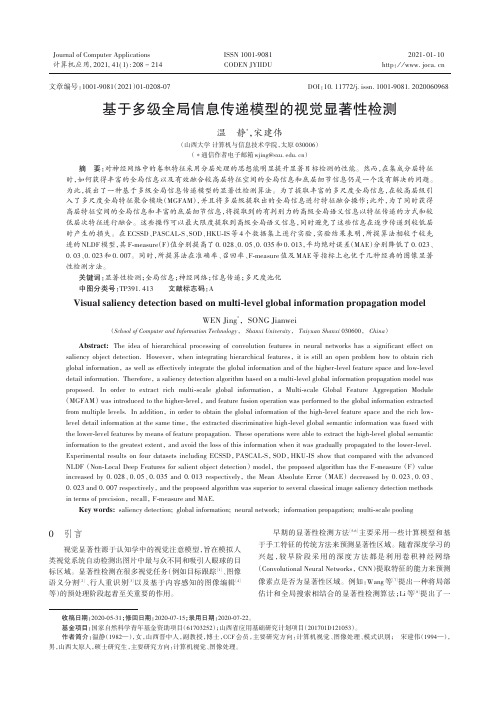
2021⁃01⁃10计算机应用,Journal of Computer Applications 2021,41(1):208-214ISSN 1001⁃9081CODEN JYIIDU http ://基于多级全局信息传递模型的视觉显著性检测温静*,宋建伟(山西大学计算机与信息技术学院,太原030006)(∗通信作者电子邮箱wjing@ )摘要:对神经网络中的卷积特征采用分层处理的思想能明显提升显著目标检测的性能。
然而,在集成分层特征时,如何获得丰富的全局信息以及有效融合较高层特征空间的全局信息和底层细节信息仍是一个没有解决的问题。
为此,提出了一种基于多级全局信息传递模型的显著性检测算法。
为了提取丰富的多尺度全局信息,在较高层级引入了多尺度全局特征聚合模块(MGFAM ),并且将多层级提取出的全局信息进行特征融合操作;此外,为了同时获得高层特征空间的全局信息和丰富的底层细节信息,将提取到的有判别力的高级全局语义信息以特征传递的方式和较低层次特征进行融合。
这些操作可以最大限度提取到高级全局语义信息,同时避免了这些信息在逐步传递到较低层时产生的损失。
在ECSSD 、PASCAL -S 、SOD 、HKU -IS 等4个数据集上进行实验,实验结果表明,所提算法相较于较先进的NLDF 模型,其F -measure (F )值分别提高了0.028、0.05、0.035和0.013,平均绝对误差(MAE )分别降低了0.023、0.03、0.023和0.007。
同时,所提算法在准确率、召回率、F -measure 值及MAE 等指标上也优于几种经典的图像显著性检测方法。
关键词:显著性检测;全局信息;神经网络;信息传递;多尺度池化中图分类号:TP391.413文献标志码:AVisual saliency detection based on multi -level global information propagation modelWEN Jing *,SONG Jianwei(School of Computer and Information Technology ,Shanxi University ,Taiyuan Shanxi 030600,China )Abstract:The idea of hierarchical processing of convolution features in neural networks has a significant effect onsaliency object detection.However ,when integrating hierarchical features ,it is still an open problem how to obtain rich global information ,as well as effectively integrate the global information and of the higher -level feature space and low -leveldetail information.Therefore ,a saliency detection algorithm based on a multi -level global information propagation model was proposed.In order to extract rich multi -scale global information ,a Multi -scale Global Feature Aggregation Module(MGFAM )was introduced to the higher -level ,and feature fusion operation was performed to the global information extracted from multiple levels.In addition ,in order to obtain the global information of the high -level feature space and the rich low -level detail information at the same time ,the extracted discriminative high -level global semantic information was fused with the lower -level features by means of feature propagation.These operations were able to extract the high -level global semantic information to the greatest extent ,and avoid the loss of this information when it was gradually propagated to the lower -level.Experimental results on four datasets including ECSSD ,PASCAL -S ,SOD ,HKU -IS show that compared with the advanced NLDF (Non -Local Deep Features for salient object detection )model ,the proposed algorithm has the F -measure (F )valueincreased by 0.028、0.05、0.035and 0.013respectively ,the Mean Absolute Error (MAE )decreased by 0.023、0.03、0.023and 0.007respectively ,and the proposed algorithm was superior to several classical image saliency detection methods in terms of precision ,recall ,F -measure and MAE.Key words:saliency detection;global information;neural network;information propagation;multi -scale pooling引言视觉显著性源于认知学中的视觉注意模型,旨在模拟人类视觉系统自动检测出图片中最与众不同和吸引人眼球的目标区域。
vqa研究方向总结
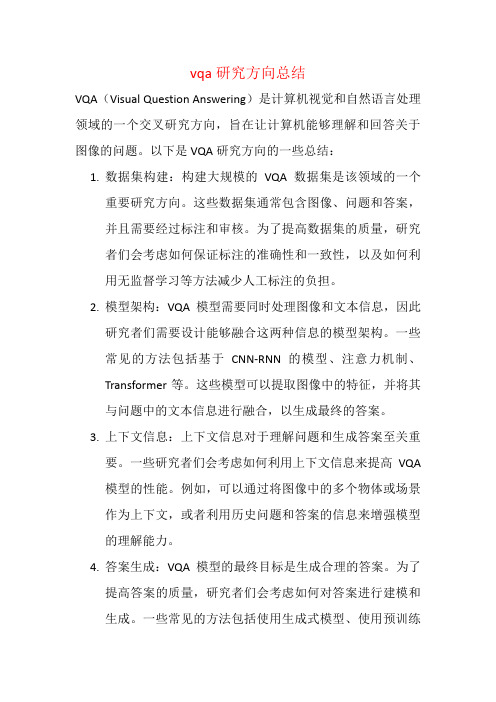
vqa研究方向总结VQA(Visual Question Answering)是计算机视觉和自然语言处理领域的一个交叉研究方向,旨在让计算机能够理解和回答关于图像的问题。
以下是VQA研究方向的一些总结:1.数据集构建:构建大规模的VQA数据集是该领域的一个重要研究方向。
这些数据集通常包含图像、问题和答案,并且需要经过标注和审核。
为了提高数据集的质量,研究者们会考虑如何保证标注的准确性和一致性,以及如何利用无监督学习等方法减少人工标注的负担。
2.模型架构:VQA模型需要同时处理图像和文本信息,因此研究者们需要设计能够融合这两种信息的模型架构。
一些常见的方法包括基于CNN-RNN的模型、注意力机制、Transformer等。
这些模型可以提取图像中的特征,并将其与问题中的文本信息进行融合,以生成最终的答案。
3.上下文信息:上下文信息对于理解问题和生成答案至关重要。
一些研究者们会考虑如何利用上下文信息来提高VQA 模型的性能。
例如,可以通过将图像中的多个物体或场景作为上下文,或者利用历史问题和答案的信息来增强模型的理解能力。
4.答案生成:VQA模型的最终目标是生成合理的答案。
为了提高答案的质量,研究者们会考虑如何对答案进行建模和生成。
一些常见的方法包括使用生成式模型、使用预训练的语言模型等。
此外,为了确保生成的答案是合理的,研究者们还会考虑如何对答案进行评估和排名。
5.多模态学习:多模态学习是VQA领域的一个重要研究方向。
由于图像和文本是两种不同的模态,因此如何将它们有效地结合起来是一个挑战。
一些常见的方法包括使用注意力机制、特征融合等。
此外,为了进一步提高多模态学习的效果,研究者们还会考虑如何利用无监督学习等方法从大量未标注数据中学习有用的特征和知识。
6.可解释性和可视化:为了更好地理解VQA模型的运行机制和性能瓶颈,研究者们会考虑如何对模型进行可解释性和可视化。
例如,可以通过可视化模型中的特征图、注意力权重等来了解模型关注的重点和存在的问题,从而进一步优化模型的性能。
入侵植物喜旱莲子草_生物学_生态学及管理_潘晓云

植 物 分 类 学 报 45 (6): 884–900(2007)doi:10.1360/aps06134 Acta Phytotaxonomica Sinica ———————————2006-08-28收稿, 2007-03-12收修改稿。
基金项目: 国家自然科学基金(30400052); 国家重点基础研究发展计划资助(2006CB403305) (Supported by the National Natural Science Foundation of China, Grant No. 30400052; National Basic Research Program of China, Grant No. 2006CB403305 )。
* 通讯作者(Author for correspondence. E-mail: jkchen@; Tel.: 86-21-65642468; Fax: 86-21-65642468)。
入侵植物喜旱莲子草——生物学、生态学及管理1潘晓云 1耿宇鹏 2Alejandro SOSA 1张文驹 1李 博 1陈家宽*1(生物多样性和生态工程教育部重点实验室, 复旦大学生物多样性科学研究所, 长江河口湿地生态系统野外科学 观测研究站 上海 200433)2(南美生物防治实验室 布宜诺斯艾利斯 1686)Invasive Alternanthera philoxeroides : biology, ecology andmanagement1PAN Xiao-Yun 1GENG Yu-Peng 2Alejandro SOSA 1ZHANG Wen-Ju 1LI Bo 1CHEN Jia-Kuan * 1(Ministry of Education Key Laboratory for Biodiversity Science & Ecological Engineering , Institute of Biodiversity Science ,Fudan University , Coastal Ecosystems Research Station of Yangtze River Estuary , Shanghai 200433, China) 2(South American Biological Control Laboratory , USDA-ARS , Hurlingham-Buenos Aires 1686, Argentina) Abstract In this review, we present a detailed account of Alternanthera philoxeroides (alligatorweed), including A. philoxeroides description, intraspecific variation from native to introduced regions, its life history strategies, invasion mechanisms, and management strategies. Alternanthera philoxeroides is a herbaceous amphibious weed of Amaranthaceae, native to South America, distributed from Buenos Aires Province (39° S) to south Brazil. It was first described by Martius in 1826, and consists of several taxa in both its native and non-native ranges. Current knowledge indicates that two forms of alligator weed exist in Argentina: A. philoxeroides f. philoxeroides in the southern range and A. philoxeroides f. angustifolia in the northern range. In Argentina, both forms set fruits and produce viable seeds. Alternanthera philoxeroides is now found as a serious weed from tropical to warm temperate regions, including the USA, China, India, South-East Asia, Australia and New Zealand. It is thought to have been brought to China during the 1930s, and later widely cultivated and spread in southern China as fodder during 1950s. The invasions of alligatorweed in China have caused considerable concerns, and now it is one of the 12 most harmful alien invasive species in China. Alligatorweed is found on stationary and slow moving water bodies, creeks, channels, riverbanks and associated areas that are occasionally flooded. It can also be found in terrestrial habitats as a pasture weed within urban environments. Alligatorweed does not produce viable seed in China and reproduces vegetatively from vegetative fragments (stems, rhizome or root tubes), which can be transported by water movement, boats, machinery and vehicles, and in hay. Movement between river catchments is common because of the human activities. Alligatorweed forms a floating mass which spreads out over the water. Its growth disrupts the ecology of banks and shallows and crowds out other plant species, restricts water flow, increases sedimentation, aggravates flooding, limits access and use by man and provides a favorable breeding area for disease vectors. We need better understanding of the biology and ecology of alligatorweed to assess the efficiency of control methods in any theoretical framework. According to the6期潘晓云等: 入侵植物喜旱莲子草——生物学、生态学及管理885 knowledge of the life history strategy of alligatorweed, we suggest that metapopulation theory is a good tool to improve management efficiency from watershed and regional perspectives. Key words alligatorweed (Alternanthera philoxeroides), biological invasion, dispersal, disturbance, intraspecific variation, life history, metapopulation, watershed management.摘要喜旱莲子草Alternanthera philoxeroides原产于南美洲, 属于苋科Amaranthaceae莲子草属Alternanthera。
2.2参考文献

2.2参考⽂献[1.1] O.D.Faugeras and G.Toscani, The Calibration Problem for Stereo, Proc. of IEEE Conference of Computer Vision and Pattern Recognition, pp 15-20, 1986.[1.2] O.D.Faugeras and G.Toscani,Camera Calibration for 3D Computer V ision, Proc. Of International Workshop on Industrial Application of Machine Vision and Machine Intellegence, pp.240-247, Japan, 1987[1.3] S.J.Manbank and O.D.Faugeras, A Theory of Self-Calibration of a Moving Camera., International Journal of Computer V ision, V ol.8:2,pp.123-151,1992.[1.4] S.D.Ma, A Self-Calibration Technique for Active Vision System, IEEE Trans. Robotics and Automation,Feb.,1996.[1.5] Y.C.Shiu and S.Ahmad, Calibration of Wrist-Mounted Robotic Sensors by Solving Homogenous Transform Equations of Form AX=XB, IEEETrans. Robotics and Automation,V ol.5,No.1,pp 16-29,January,1989.[1.6] Z. Zhang, A Flexible New Technique for Camera Calibration, IEEE Transactions on Pattern Analysis and Machine Intelligence,22(11):1330–1334, 2000.[2.1] Barnard S T,Fischler M A.Computational stereo[J].ACM Computing Surveys,1982,14(4):553-572.[2.2] Dhond U R,Aggarwal J K.Structure from stereo-A review[J].IEEE Trans on Systems,Man andCybernetics,1989,19(6):1489-1510.[2.3] Faugeras O.What can be seen in three dimensions with an uncalibrated stereo rig[C].Proc of the 2nd European Confon Computer Vision.Santa Margherita Ligure,1992:563-578.[2.4] Koschan A.What is new in computational stereo since 1989:A survey of current stereo papers [R].Berlin:Technical University of Berlin. 1993.[2.5] Scharstein D,Szeliski R.A taxonomy and evaluation of dense two-frame stereo correspondence algorithms[J].Int J of Computer V ision,2002,47(1):7-42.[2.6] Brown M Z,Burschka D,Hager G D.A dvances in computational stereo[J].IEEE Trans on Pattern Analysis and Machine Intelligence,2003,25(8):993-1008.[2.7] V enkateswar V,Chellappa R.Hierarchical stereo and motion correspondence using feature groupings[J].Int J of Computer V ision,1995,15(3):245-269.[2.8] Mahlmann K,Maier D,Hesser J,eta1.Calculating dense disparity maps from color stereo images,an efficient implementation[J].Int J of Computer Vision,2002,47(i-3):79-88.[2.9]Kolmogorov V,Zabin R.What energy functions can be minimized via graph cuts? [J].IEEE Trans on Pattern Analysis and Machine Intelligence,2004,26(2):147-159.[2.10] Gong M,Y ang Y H.Fast unambiguous stereo matching using reliability-based dynamic programming[J].IEEE Trans on Pattern Analysis and Machine Intelligence,2005,27(6):998-1003.[2.11]徐奕,周军,周源华.基于⼩波及动态规划的相位匹配[J].上海交通⼤学学报,2003,37(3):388-392.[2.12]Goulermas J Y,Liatsis P,Fernando T.A constrained nonlinear energy minimization framework for the regularization of the stereo correspondence problem [J].IEEE Trans on Circuits and Systems for V ideo Technology,2005,15(4):550-565.[2.13] V eksler O.Fast variable window for stereo correspondence using integral images[C].Proc of IEEE Conf on Computer V ision and Pattern Recognition.Madison,2003:556-561.[2.14]Wang L,Kang S B,Shum H Y.Cooperative segmentation and stereo using perspective space search [C].Proc of Asian Conf on Computer V ision.Jeju Island,2004:366-371.[2.15]Y oon K J,Kweon I S.Locally adaptive support-weight approach for visual correspondence search [C].Proc of IEEE Conf on Computer Vision and Pattern Recognition.SanDiego,2005:924-931.[2.16] Zabih R,Woodfill J.Non-parametric local transforms for computing visual correspondence[C].Procofthe 3rd European Conf on Computer Vision.Stockholm,l994:150-l58.[2.17] Birchfield S,Tomasi C.Multiway cut for stereo and motion with slanted surfaces [C].Proc of Int Conf on Computer Vision.GreeP.e,1999:489-495.[2.18] Boykov Y,V eksler O,Zabih R.Markovr and omfields with efficient approximations[C].Proc of IEEE Conf on Computer V ision and Pattern Recognition.Santa Barbara,1998:648-655.[2.19]Kim H,Sohn K.Hierarchical disparity estimation with energy-based regularization[C].Proc of Int Conf on Image Processing.Barcelona,2003:373-376.[2.20] Prince J D,Eagle R A.Weighted directional energy model of human stereo correspondence [J].V ision Research,2000,40(9):1143-1155.[2.21] Muquit M A,Shibahara T,Aoki T.A high-accuracy passive 3D measurement system using phase-based image matching [J].IEICE Trans on Fundamentals of Electronics,Communications and Computer Sciences,2006,E89-A(3):686-697.2006,E89-A(3):686-697.[2.22] Fleet D J,Jepson A D.Stability of phase information [J].IEEE Trans on:Pattern Analysis and Machine Intelligence,1993,15(12):1253-1268.[2.23] 徐彦君,杜利民,侯⾃强.基于相位的尺度⾃适应⽴体匹配⽅法[J].电⼦学报,1999,27(7):38-41.[2.24] Birchfield S,Tomasi C.Depth discontinuities by pixel-to-pixelstereo [C].Proc of IEEE Int Conf on Computer V ision.Bombay,1998:1073-1080.[2.25] Ohta Y,Kanade T.Stereo by intra- and inter-scan line search using dynamic programming [J].IEEE Trans on Pattern Analysis and Machine Intelligence,1985,7(2):139-i54.[2.26] Cox I J,Hingorani S L,Rao S B,eta1.A maximum likelihood stereo algorithm [J].Computer V ision and Image Understanding,1996,63(3):542-567.[2.27]Bobick A F,Intille S S.Large occlusion stereo[J].Int J of Computer V ision,1999,33(3):181-200.[2.28] Lei C,Seizer J,Y ang Y H.Region-tree based stereo using dynamic programming optimization [C].Proc of IEEE Conf on Computer V ision and Pattern Recognition.New Y ork,2006:378-385.[2.29]Cox I J,Roy S.A maximum-flow formulation of the N-camera stereo correspondence problem [C].Proc of the 6th Int Conf on Computer V ision.Bombay,1998:492-499.[2.30]Boykov Y,V eksler O,Zabih R.Fast approximate energy minimization via graph cuts [J].IEEE Trans on Pattern Analysis and Machine Intelligence,2001,23(11):1222-1239.[2.31]王年,范益政,鲍⽂霞,等.基于图割的图像匹配算法[J].电⼦学报,2006,34(2):232-236.[2.32]Boykov Y,Kolmogorov V.An experimental comparison of min-cut/max-flow algorithms for energy minimization in vision [J].IEEE Trans on Pattern Analysis and Machine Intelligence,2004,26(9):1124-1137.[2.33] Ruichek Y.Multi level and neural-network-based stereo-matching method for real-time obstacle detection using linear cameras[J].IEEE Trans on Intelligent Transportation Systems,2005,6(1):54-62.[2.34] Hua X J,Y okomichi M,Kono M.Stereo correspondence using color based on competitive-cooperative neural networks[C].Proc of the 6th Int Conf on Parallel and Distributed Computing,Applications and Technologies.Denver,2005:856-860.[2.35]Wang B.Chung R,Shen C.Genetic algorithm-based stereo vision with no block-partitioning of input images[C].Proc IEEE Int Symposium on Computational Intelligence in Robotics and Automation.Kobe,2003:830-836.[2.36]Gong M,Y ang Y H.Genetic-based stereo algorithm and disparity map evaluation [J].Int J of ComputerVision,2002,47(1-3):63-77.[2.37]Zitnick L C,Kanade T.A cooperative algorithm for stereo matching and occlusion detection[J].IEEE Trans on Pattern Analysis and Machine Intelligence,2000,22(7):675-681.[2.38]Ruichek Y,Issa H,Postaire JG,etal.Towards real-time obstacle detection using a hierarchicaldecomposition methodology for stereo matching with a genetic algorithm[C].Proc of the 16th IEEE Int Conf on Tools with Artificial Intelligence.Boca Raton,2004:138-147.[2.39]Scharstein D,Szeliski R.Stereo matching with nonlinear diffusion[J].Int J of Computer Vision,1998,28(2):155-174.[2.40] Lee S H,Kanatsugu Y,Park J I.MAP-based stochastic diffusion for stereo matching and line fieldsestimation[J].Int J of Computer V ision,2002,47(1-3):195-218.[2.41]Sun J,Zheng N N,Shum H Y.Stereo matching using belief propagation[J].IEEE Trans on Pattern Analysis and Machine Intelligence,2003,25(7):787-800.[2.42]Felzenszwalb P F,Huttenlocher D P.Efficient belief propagation for early vision[C].Proc of IEEE Conf on Computer V ision and Pattern Recognition.Washington,2004:261-268.[2.43]Klaus A,Sormann M,Karner K.Segment-based stereo matching using belief propagation and a self-adapting dissimilarity measure[C].Proc of the 18th Int Conf on Pattern Recognition.HongKong,2006:15-18.[2.44] Wang L,Liao M,Gong M,etal.High-qualityreal-time stereo using adaptive cost aggregation and dynamic programming[C].3rd Int Symposiumon 3D DataProcessing,Visualization and Transmission.NorthCarolina,2006:798-805.[2.45]Birchfield B,Natarajan B,Tromasi C.correspondence as energy-based segmentation[J].Image and Vision Computing,2007,25(8):1329-1340.[2.46]Deng Y,Y ang Q,Linx,etal.Stereo correspondence with occlusion handling in a symmetric patch-based graph-cuts model[J].IEEE Trans on Pattern Analysis and Machine Intelligence,2007,29(6):1068-1079.[2.47]Binaghi E,Gallo I,Marino G,etal.Neural adaptive stereo matching[J].Pattern RecognitionLetters,2004,25(15):1743-1758.[2.48]Bleyer M,Gelautz M.Graph-cut-based stereo matching using image segmentation with symmetrical treatment of occlusions[J].Signal Processing:Image Communication,2007,22(2):127-143.[2.49]Gong M,Y ang Y H.Real-time stereo matching using orthogonal reliability-based dynamic programming[J].IEEE Trans on Image Processing,2007,16(3):879-884.[2.50]Ambrosio G,Gonzalez J.Extracting and matching perceptual groups for hierarchical stereo vision[C].Proc of the 15th Int Conf on Pattern Recognition.Barcelona,2000:542-545.[2.51]Desolneux A,Moisan I,Morel J M.Gestalt theory and computer vision[R].Paris:CMLA,2002.。
基于网络药理学预测白藜芦醇治疗阿尔茨海默症的关键潜在靶点
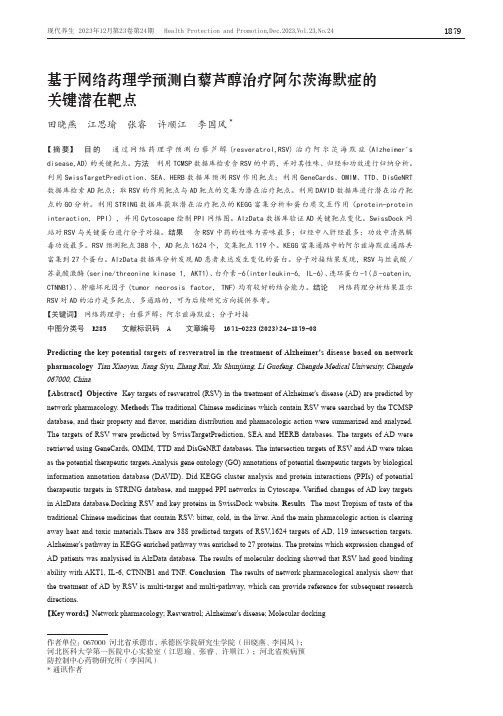
基于网络药理学预测白藜芦醇治疗阿尔茨海默症的关键潜在靶点田晓燕 江思瑜 张睿 许顺江 李国风*【摘要】目的通过网络药理学预测白藜芦醇(resveratrol,RSV)治疗阿尔茨海默症(Alzheimer's disease,AD)的关键靶点。
方法 利用TCMSP数据库检索含RSV的中药,并对其性味、归经和功效进行归纳分析。
利用SwissTargetPrediction、SEA、HERB数据库预测RSV作用靶点;利用GeneCards、OMIM、TTD、DisGeNRT 数据库检索AD靶点;取RSV的作用靶点与AD靶点的交集为潜在治疗靶点。
利用DAVID数据库进行潜在治疗靶点的GO分析。
利用STRING数据库获取潜在治疗靶点的KEGG富集分析和蛋白质交互作用(protein-protein interaction, PPI),并用Cytoscape绘制PPI网络图。
AlzData数据库验证AD关键靶点变化。
SwissDock网站对RSV与关键蛋白进行分子对接。
结果含RSV中药的性味为苦味最多;归经中入肝经最多;功效中清热解毒功效最多。
RSV预测靶点388个,AD靶点1624个,交集靶点119个。
KEGG富集通路中的阿尔兹海默症通路共富集到27个蛋白。
AlzData数据库分析发现AD患者表达发生变化的蛋白。
分子对接结果发现,RSV与丝氨酸/苏氨酸激酶(serine/threonine kinase 1, AKT1)、白介素-6(interleukin-6, IL-6)、连环蛋白-1(β-catenin, CTNNB1)、肿瘤坏死因子(tumor necrosis factor, TNF)均有较好的结合能力。
结论网络药理分析结果显示RSV对AD的治疗是多靶点、多通路的,可为后续研究方向提供参考。
【关键词】 网络药理学;白藜芦醇;阿尔兹海默症;分子对接中图分类号 R285文献标识码 A 文章编号1671-0223(2023)24-1879-08Predicting the key potential targets of resveratrol in the treatment of Alzheimer's disease based on network pharmacology Tian Xiaoyan, Jiang Siyu, Zhang Rui, Xu Shunjiang, Li Guofeng. Chengde Medical University, Chengde 067000, China【Abstract】Objective Key targets of resveratrol (RSV) in the treatment of Alzheimer's disease (AD) are predicted by network pharmacology. Methods The traditional Chinese medicines which contain RSV were searched by the TCMSP database, and their property and flavor, meridian distribution and phamacologic action were summarized and analyzed. The targets of RSV were predicted by SwissTargetPrediction, SEA and HERB databases. The targets of AD were retrieved using GeneCards, OMIM, TTD and DisGeNRT databases. The intersection targets of RSV and AD were taken as the potential therapeutic targets.Analysis gene ontology (GO) annotations of potential therapeutic targets by biological information annotation database (DAVID). Did KEGG cluster analysis and protein interactions (PPIs) of potential therapeutic targets in STRING database, and mapped PPI networks in Cytoscape. Verified changes of AD key targets in AlzData database.Docking RSV and key proteins in SwissDock website. Results The most Tropism of taste of the traditional Chinese medicines that contain RSV: bitter, cold, in the liver. And the main phamacologic action is clearing away heat and toxic materials.There are 388 predicted targets of RSV,1624 targets of AD, 119 intersection targets. Alzheimer's pathway in KEGG enriched pathway was enriched to 27 proteins. The proteins which expression changed of AD patients was analysised in AlzData database. The results of molecular docking showed that RSV had good binding ability with AKT1, IL-6, CTNNB1 and TNF. Conclusion The results of network pharmacological analysis show that the treatment of AD by RSV is multi-target and multi-pathway, which can provide reference for subsequent research directions.【Key words】 Network pharmacology; Resveratrol; Alzheimer's disease; Molecular docking作者单位:067000 河北省承德市,承德医学院研究生学院 (田晓燕、李国风);河北医科大学第一医院中心实验室(江思瑜、张睿、许顺江);河北省疾病预防控制中心药物研究所(李国风)*通讯作者现代科学研究认为,阿尔兹海默症(Alzheimer disease,AD)是一种不可逆的退行性神经疾病,临床上多以记忆力障碍、执行能力障碍以及人格变化等为特征,是老年性痴呆的最主要因素。
三目组合视觉系统在乒乓球机器人上的研究与实现

0引言目前乒乓球机器人已经有了三四十年的研究历史[1],从1983年第一款具有乒乓球轨迹追踪的机器人问世以来,世界对乒乓球机器人视觉的研究就没有停止。
乒乓球直径小、运动速度快,用乒乓球作为载体研究乒乓球机器人需要了解乒乓球的运动特性,对乒乓球运动轨迹、落点进行可靠跟踪预测。
国外对于乒乓球机器人的研究较早,有四目、双目等多种形式的视觉系统,但当时PC 硬件发展落后,图像处理速度跟不上,使得计算的乒乓球坐标和预测乒乓球的轨迹没能得到很好的保证[2]。
国内在这方面研究相对较晚,尽管已经有一些性能较好的机器人可以实现多回合人机对打,但以机器视觉为基础研究乒乓球的运动识别仍有很高的研究意义。
本文采用的2+1式的三目组合式视觉系统,使用双目作为立体匹配解析距离,单目进行乒乓球的识别追踪,最后,由PC 拟合出高精度乒乓球运动轨迹,对乒乓球落点进行预测,具有较好的稳定性和精确度。
1系统总体方案在三目组合式视觉系统中,单目摄像头由树莓派4B 控制,双目摄像头直接与MiniPC(小型主机)相连。
树莓派得到的二维坐标数据会写入一个本地文件,通过UDP 传输至Mini PC,PC 将UDP(UDP 即用户数据报协议)解包与双目摄像头匹配解析的距离数据传送至C++程序中,实时解析三维坐标保存为二进制数据文件,而后Python 脚本通过读取文件中的三维坐标生成三维轨迹图,并进行轨迹预测及落点预测,最后通过USB-TTL 向下位机发送处理好的坐标数据,如图1所示。
图1 系统总体方案2摄像头标定摄像头采用棋盘标定板作为标定数据来源,并准备了15组标定图对摄像头进行标定[3]。
这里对比使用OpenCV (开源计算机视觉库)标定和Matlab 标定。
2.1 OpenCV 标定OpenCV 标定程序输入参数较为复杂,为了使标定结果更加直观,本文使用了Qt(一个跨平台的C++图形用户界面应用程序开发框架)重新写了一个标定程序Stereo Calibrator(双目标定器),在图形界面下使用标定。
基于周期采样的分布式动态事件触发优化算法
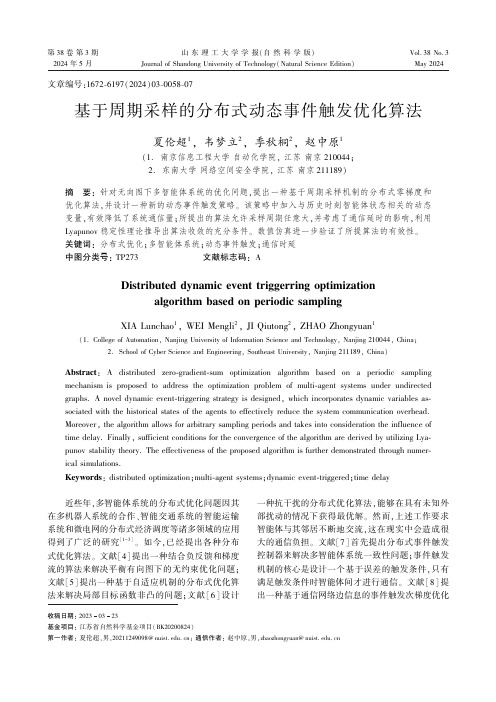
第38卷第3期2024年5月山东理工大学学报(自然科学版)Journal of Shandong University of Technology(Natural Science Edition)Vol.38No.3May 2024收稿日期:20230323基金项目:江苏省自然科学基金项目(BK20200824)第一作者:夏伦超,男,20211249098@;通信作者:赵中原,男,zhaozhongyuan@文章编号:1672-6197(2024)03-0058-07基于周期采样的分布式动态事件触发优化算法夏伦超1,韦梦立2,季秋桐2,赵中原1(1.南京信息工程大学自动化学院,江苏南京210044;2.东南大学网络空间安全学院,江苏南京211189)摘要:针对无向图下多智能体系统的优化问题,提出一种基于周期采样机制的分布式零梯度和优化算法,并设计一种新的动态事件触发策略㊂该策略中加入与历史时刻智能体状态相关的动态变量,有效降低了系统通信量;所提出的算法允许采样周期任意大,并考虑了通信延时的影响,利用Lyapunov 稳定性理论推导出算法收敛的充分条件㊂数值仿真进一步验证了所提算法的有效性㊂关键词:分布式优化;多智能体系统;动态事件触发;通信时延中图分类号:TP273文献标志码:ADistributed dynamic event triggerring optimizationalgorithm based on periodic samplingXIA Lunchao 1,WEI Mengli 2,JI Qiutong 2,ZHAO Zhongyuan 1(1.College of Automation,Nanjing University of Information Science and Technology,Nanjing 210044,China;2.School of Cyber Science and Engineering,Southeast University,Nanjing 211189,China)Abstract :A distributed zero-gradient-sum optimization algorithm based on a periodic sampling mechanism is proposed to address the optimization problem of multi-agent systems under undirected graphs.A novel dynamic event-triggering strategy is designed,which incorporates dynamic variables as-sociated with the historical states of the agents to effectively reduce the system communication overhead.Moreover,the algorithm allows for arbitrary sampling periods and takes into consideration the influence oftime delay.Finally,sufficient conditions for the convergence of the algorithm are derived by utilizing Lya-punov stability theory.The effectiveness of the proposed algorithm is further demonstrated through numer-ical simulations.Keywords :distributed optimization;multi-agent systems;dynamic event-triggered;time delay ㊀㊀近些年,多智能体系统的分布式优化问题因其在多机器人系统的合作㊁智能交通系统的智能运输系统和微电网的分布式经济调度等诸多领域的应用得到了广泛的研究[1-3]㊂如今,已经提出各种分布式优化算法㊂文献[4]提出一种结合负反馈和梯度流的算法来解决平衡有向图下的无约束优化问题;文献[5]提出一种基于自适应机制的分布式优化算法来解决局部目标函数非凸的问题;文献[6]设计一种抗干扰的分布式优化算法,能够在具有未知外部扰动的情况下获得最优解㊂然而,上述工作要求智能体与其邻居不断地交流,这在现实中会造成很大的通信负担㊂文献[7]首先提出分布式事件触发控制器来解决多智能体系统一致性问题;事件触发机制的核心是设计一个基于误差的触发条件,只有满足触发条件时智能体间才进行通信㊂文献[8]提出一种基于通信网络边信息的事件触发次梯度优化㊀算法,并给出了算法的指数收敛速度㊂文献[9]提出一种基于事件触发机制的零梯度和算法,保证系统状态收敛到最优解㊂上述事件触发策略是静态事件触发策略,即其触发阈值仅与智能体的状态相关,当智能体的状态逐渐收敛时,很容易满足触发条件并将生成大量不必要的通信㊂因此,需要设计更合理的触发条件㊂文献[10]针对非线性系统的增益调度控制问题,提出一种动态事件触发机制的增益调度控制器;文献[11]提出一种基于动态事件触发条件的零梯度和算法,用于有向网络的优化㊂由于信息传输的复杂性,时间延迟在实际系统中无处不在㊂关于考虑时滞的事件触发优化问题的文献很多㊂文献[12]研究了二阶系统的凸优化问题,提出时间触发算法和事件触发算法两种分布式优化算法,使得所有智能体协同收敛到优化问题的最优解,并有效消除不必要的通信;文献[13]针对具有传输延迟的多智能体系统,提出一种具有采样数据和时滞的事件触发分布式优化算法,并得到系统指数稳定的充分条件㊂受文献[9,14]的启发,本文提出一种基于动态事件触发机制的分布式零梯度和算法,与使用静态事件触发机制的文献[15]相比,本文采用动态事件触发机制可以避免智能体状态接近最优值时频繁触发造成的资源浪费㊂此外,考虑到进行动态事件触发判断需要一定的时间,使用当前状态值是不现实的,因此,本文使用前一时刻状态值来构造动态事件触发条件,更符合逻辑㊂由于本文采用周期采样机制,这进一步降低了智能体间的通信频率,但采样周期过长会影响算法收敛㊂基于文献[14]的启发,本文设计的算法允许采样周期任意大,并且对于有时延的系统,只需要其受采样周期的限制,就可得到保证多智能体系统达到一致性和最优性的充分条件㊂最后,通过对一个通用示例进行仿真,验证所提算法的有效性㊂1㊀预备知识及问题描述1.1㊀图论令R表示实数集,R n表示向量集,R nˑn表示n ˑn实矩阵的集合㊂将包含n个智能体的多智能体系统的通信网络用图G=(V,E)建模,每个智能体都视为一个节点㊂该图由顶点集V={1,2, ,n}和边集E⊆VˑV组成㊂定义A=[a ij]ɪR nˑn为G 的加权邻接矩阵,当a ij>0时,表明节点i和节点j 间存在路径,即(i,j)ɪE;当a ij=0时,表明节点i 和节点j间不存在路径,即(i,j)∉E㊂D=diag{d1, ,d n}表示度矩阵,拉普拉斯矩阵L等于度矩阵减去邻接矩阵,即L=D-A㊂当图G是无向图时,其拉普拉斯矩阵是对称矩阵㊂1.2㊀凸函数设h i:R nңR是在凸集ΩɪR n上的局部凸函数,存在正常数φi使得下列条件成立[16]:h i(b)-h i(a)- h i(a)T(b-a)ȡ㊀㊀㊀㊀φi2 b-a 2,∀a,bɪΩ,(1)h i(b)- h i(a)()T(b-a)ȡ㊀㊀㊀㊀φi b-a 2,∀a,bɪΩ,(2) 2h i(a)ȡφi I n,∀aɪΩ,(3)式中: h i为h i的一阶梯度, 2h i为h i的二阶梯度(也称黑塞矩阵)㊂1.3㊀问题描述考虑包含n个智能体的多智能体系统,假设每个智能体i的成本函数为f i(x),本文的目标是最小化以下的优化问题:x∗=arg minxɪΩðni=1f i(x),(4)式中:x为决策变量,x∗为全局最优值㊂1.4㊀主要引理引理1㊀假设通信拓扑图G是无向且连通的,对于任意XɪR n,有以下关系成立[17]:X T LXȡαβX T L T LX,(5)式中:α是L+L T2最小的正特征值,β是L T L最大的特征值㊂引理2(中值定理)㊀假设局部成本函数是连续可微的,则对于任意实数y和y0,存在y~=y0+ω~(y -y0),使得以下不等式成立:f i(y)=f i(y0)+∂f i∂y(y~)(y-y0),(6)式中ω~是正常数且满足ω~ɪ(0,1)㊂2㊀基于动态事件触发机制的分布式优化算法及主要结果2.1㊀考虑时延的分布式动态事件触发优化算法本文研究具有时延的多智能体系统的优化问题㊂为了降低智能体间的通信频率,提出一种采样周期可任意设计的分布式动态事件触发优化算法,95第3期㊀㊀㊀㊀㊀㊀㊀㊀㊀㊀㊀㊀夏伦超,等:基于周期采样的分布式动态事件触发优化算法其具体实现通信优化的流程图如图1所示㊂首先,将邻居和自身前一触发时刻状态送往控制器(本文提出的算法),得到智能体的状态x i (t )㊂然后,预设一个固定采样周期h ,使得所有智能体在同一时刻进行采样㊂同时,在每个智能体上都配置了事件检测器,只在采样时刻检查是否满足触发条件㊂接着,将前一采样时刻的智能体状态发送至构造的触发器中进行判断,当满足设定的触发条件时,得到触发时刻的智能体状态x^i (t )㊂最后,将得到的本地状态x^i (t )用于更新自身及其邻居的控制操作㊂由于在实际传输中存在时延,因此需要考虑满足0<τ<h 的时延㊂图1㊀算法实现流程图考虑由n 个智能体构成的多智能体系统,其中每个智能体都能独立进行计算和相互通信,每个智能体i 具有如下动态方程:x ㊃i (t )=-1h2f i (x i )()-1u i (t ),(7)式中u i (t )为设计的控制算法,具体为u i (t )=ðnj =1a ij x^j (t -τ)-x ^i (t -τ)()㊂(8)㊀㊀给出设计的动态事件触发条件:θi d i e 2i (lh )-γq i (lh -h )()ɤξi (lh ),(9)q i (t )=ðnj =1a ij x^i (t -τ)-x ^j (t -τ)()2,(10)㊀㊀㊀ξ㊃i (t )=1h[-μi ξi (lh )+㊀㊀㊀㊀㊀δi γq i (lh -h )-d i e 2i (lh )()],(11)式中:d i 是智能体i 的入度;γ是正常数;θi ,μi ,δi 是设计的参数㊂令x i (lh )表示采样时刻智能体的状态,偏差变量e i (lh )=x i (lh )-x^i (lh )㊂注释1㊀在进行动态事件触发条件设计时,可以根据不同的需求为每个智能体设定不同的参数θi ,μi ,δi ,以确保其能够在特定的情境下做出最准确的反应㊂本文为了方便分析,选择为每个智能体设置相同的θi ,μi ,δi ,以便更加清晰地研究其行为表现和响应能力㊂2.2㊀主要结果和分析由于智能体仅在采样时刻进行事件触发条件判断,并在达到触发条件后才通信,因此有x ^i (t -τ)=x^i (lh )㊂定理1㊀假设无向图G 是连通的,对于任意i ɪV 和t >0,当满足条件(12)时,在算法(7)和动态事件触发条件(9)的作用下,系统状态趋于优化解x ∗,即lim t ңx i (t )=x ∗㊂12-β2φm α-τβ2φm αh -γ>0,μi+δi θi <1,μi-1-δi θi >0,ìîíïïïïïïïï(12)式中φm =min{φ1,φ2}㊂证明㊀对于t ɪ[lh +τ,(l +1)h +τ),定义Lyapunov 函数V (t )=V 1(t )+V 2(t ),其中:V 1(t )=ðni =1f i (x ∗)-f i (x i )-f ᶄi (x i )(x ∗-x i )(),V 2(t )=ðni =1ξi (t )㊂令E (t )=e 1(t ), ,e n (t )[]T ,X (t )=x 1(t ), ,x n (t )[]T ,X^(t )=x ^1(t ), ,x ^n (t )[]T ㊂对V 1(t )求导得V ㊃1(t )=1h ðni =1u i (t )x ∗-x i (t )(),(13)由于ðni =1ðnj =1a ij x ^j (t -τ)-x ^i (t -τ)()㊃x ∗=0成立,有V ㊃1(t )=-1hX T (t )LX ^(lh )㊂(14)6山东理工大学学报(自然科学版)2024年㊀由于㊀㊀X (t )=X (lh +τ)-(t -lh -τ)X ㊃(t )=㊀㊀㊀㊀X (lh )+τX ㊃(lh )+t -lh -τhΓ1LX^(lh )=㊀㊀㊀㊀X (lh )-τh Γ2LX^(lh -h )+㊀㊀㊀㊀(t -lh -τ)hΓ1LX^(lh ),(15)式中:Γ1=diag (f i ᶄᶄ(x ~11))-1, ,(f i ᶄᶄ(x ~1n ))-1{},Γ2=diag (f i ᶄᶄ(x ~21))-1, ,(f i ᶄᶄ(x ~2n))-1{},x ~1iɪ(x i (lh +τ),x i (t )),x ~2i ɪ(x i (lh ),x i (lh+τ))㊂将式(15)代入式(14)得㊀V ㊃1(t )=-1h E T (lh )LX ^(lh )-1hX ^T (lh )LX ^(lh )+㊀㊀㊀τh2Γ2X ^T (lh -h )L T LX ^(lh )+㊀㊀㊀(t -lh -τ)h2Γ1X ^T (lh )L T LX ^(lh )㊂(16)根据式(3)得(f i ᶄᶄ(x ~i 1))-1ɤ1φi,i =1, ,n ㊂即Γ1ɤ1φm I n ,Γ2ɤ1φmI n ,φm =min{φ1,φ2}㊂首先对(t -lh -τ)h2Γ1X ^T (lh )L T LX ^(lh )项进行分析,对于t ɪ[lh +τ,(l +1)h +τ),基于引理1和式(3)有(t -lh -τ)h2Γ1X ^T (lh )L T LX ^(lh )ɤβhφm αX ^T (lh )LX ^(lh )ɤβ2hφm αðni =1q i(lh ),(17)式中最后一项根据X^T (t )LX ^(t )=12ðni =1q i(t )求得㊂接着分析τh2Γ2X ^(lh -h )L T LX ^(lh ),根据引理1和杨式不等式有:τh2Γ2X ^T (lh -h )L T LX ^(lh )ɤ㊀㊀㊀㊀τβ2h 2φm αX ^T (lh -h )LX ^(lh -h )+㊀㊀㊀㊀τβ2h 2φm αX ^T (lh )LX ^(lh )ɤ㊀㊀㊀㊀τβ4h 2φm αðni =1q i (lh -h )+ðni =1q i (lh )[]㊂(18)将式(17)和式(18)代入式(16)得㊀V ㊃1(t )ɤβ2φm α+τβ4φm αh -12()1h ðni =1q i(lh )+㊀㊀㊀τβ4φm αh ðni =1q i (lh -h )+1h ðni =1d i e 2i(lh )㊂(19)根据式(11)得V ㊃2(t )=-ðni =1μih ξi(lh )+㊀㊀㊀㊀ðni =1δihγq i (lh -h )-d i e 2i (lh )()㊂(20)结合式(19)和式(20)得V ㊃(t )ɤ-12-β2φm α-τβ4φm αh ()1h ðni =1q i (lh )+㊀㊀㊀㊀τβ4φm αh 2ðn i =1q i (lh -h )+γh ðni =1q i (lh -h )-㊀㊀㊀㊀1h ðni =1(μi -1-δi θi)ξi (lh ),(21)因此根据李雅普诺夫函数的正定性以及Squeeze 定理得㊀V (l +1)h +τ()-V (lh +τ)ɤ㊀㊀㊀-12-β2φm α-τβ4φm αh()ðni =1q i(lh )+㊀㊀㊀τβ4φm αh ðni =1q i (lh -h )+γðni =1q i (lh -h )-㊀㊀㊀ðni =1(μi -1-δiθi)ξi (lh )㊂(22)对式(22)迭代得V (l +1)h +τ()-V (h +τ)ɤ㊀㊀-12-β2φm α-τβ2φm αh-γ()ðl -1k =1ðni =1q i(kh )+㊀㊀τβ4φm αh ðni =1q i (0h )-㊀㊀12-β2φm α-τβ4φm αh()ðni =1q i(lh )-㊀㊀ðlk =1ðni =1μi -1-δiθi()ξi (kh ),(23)进一步可得㊀lim l ңV (l +1)h -V (h )()ɤ㊀㊀㊀τβ4φm αh ðni =1q i(0h )-16第3期㊀㊀㊀㊀㊀㊀㊀㊀㊀㊀㊀㊀夏伦超,等:基于周期采样的分布式动态事件触发优化算法㊀㊀㊀ðni =1(μi -1-δi θi )ðl =1ξi (lh )-㊀㊀㊀12-β2φm α-τβ2φm αh-γ()ð l =1ðni =1q i(lh )㊂(24)由于q i (lh )ȡ0和V (t )ȡ0,由式(24)得lim l ң ðni =1ξi (lh )=0㊂(25)基于ξi 的定义和拉普拉斯矩阵的性质,可以得到每个智能体的最终状态等于相同的常数,即lim t ңx 1(t )= =lim t ңx n (t )=c ㊂(26)㊀㊀由于目标函数的二阶导数具有以下性质:ðni =1d f ᶄi (x i (t ))()d t =㊀㊀㊀㊀-ðn i =1ðnj =1a ij x ^j (t )-x ^i (t )()=㊀㊀㊀㊀-1T LX^(t )=0,(27)式中1=[1, ,1]n ,所以可以得到ðni =1f i ᶄ(x i (t ))=ðni =1f i ᶄ(x ∗i )=0㊂(28)联立式(26)和式(28)得lim t ңx 1(t )= =lim t ңx n (t )=c =x ∗㊂(29)㊀㊀定理1证明完成㊂当不考虑通信时延τ时,可由定理1得到推论1㊂推论1㊀假设通信图G 是无向且连通的,当不考虑时延τ时,对于任意i ɪV 和t >0,若条件(30)成立,智能体状态在算法(7)和触发条件(9)的作用下趋于最优解㊂14-n -1φm -γ>0,μi+δi θi <1,μi-1-δi θi >0㊂ìîíïïïïïïïï(30)㊀㊀证明㊀该推论的证明过程类似定理1,由定理1结果可得14-β2φm α-γ>0㊂(31)令λn =βα,由于λn 是多智能体系统的全局信息,因此每个智能体很难获得,但其上界可以根据以下关系来估计:λn ɤ2d max ɤ2(n -1),(32)式中d max =max{d i },i =1, ,n ㊂因此得到算法在没有时延情况下的充分条件:14-n -1φm -γ>0㊂(33)㊀㊀推论1得证㊂注释2㊀通过定理1得到的稳定性条件,可以得知当采样周期h 取较小值时,由于0<τ<h ,因此二者可以抵消,从而稳定性不受影响;而当采样周期h 取较大值时,τβ2φm αh项可以忽略不计,因此从理论分析可以得出允许采样周期任意大的结论㊂从仿真实验方面来看,当采样周期h 越大,需要的收剑时间越长,但最终结果仍趋于优化解㊂然而,在文献[18]中,采样周期过大会导致稳定性条件难以满足,即算法最终难以收敛,无法达到最优解㊂因此,本文提出的算法允许采样周期任意大,这一创新点具有重要意义㊂3㊀仿真本文对一个具有4个智能体的多智能体网络进行数值模拟,智能体间的通信拓扑如图2所示㊂采用4个智能体的仿真网络仅是为了初步验证所提算法的有效性㊂值得注意的是,当多智能体的数量增加时,算法的时间复杂度和空间复杂度会增加,但并不会影响其有效性㊂因此,该算法在更大规模的多智能体网络中同样适用㊂成本函数通常选择凸函数㊂例如,在分布式传感器网络中,成本函数为z i -x 2+εi x 2,其中x 表示要估计的未知参数,εi 表示观测噪声,z i 表示在(0,1)中均匀分布的随机数;在微电网中,成本函数为a i x 2+b i x +c i ,其中a i ,b i ,c i 是发电机成本参数㊂这两种情境下的成本函数形式不同,但本质上都是凸函数㊂本文采用论文[19]中的通用成本函数(式(34)),用于证明本文算法在凸函数上的可行性㊂此外,通信拓扑图结构并不会影响成本函数的设计,因此,本文的成本函数在分布式网络凸优化问题中具有通用性㊂g i (x )=(x -i )4+4i (x -i )2,i =1,2,3,4㊂(34)很明显,当x i 分别等于i 时,得到最小局部成本函数,但是这不是全局最优解x ∗㊂因此,需要使用所提算法来找到x ∗㊂首先设置重要参数,令φm =16,γ=0.1,θi =1,ξi (0)=5,μi =0.2,δi =0.2,26山东理工大学学报(自然科学版)2024年㊀图2㊀通信拓扑图x i (0)=i ,i =1,2,3,4㊂图3为本文算法(7)解决优化问题(4)时各智能体的状态,其中设置采样周期h =3,时延τ=0.02㊂智能体在图3中渐进地达成一致,一致值为全局最优点x ∗=2.935㊂当不考虑采样周期影响时,即在采样周期h =3,时延τ=0.02的条件下,采用文献[18]中的算法(10)时,各智能体的状态如图4所示㊂显然,在避免采样周期的影响后,本文算法具有更快的收敛速度㊂与文献[18]相比,由于只有当智能体i 及其邻居的事件触发判断完成,才能得到q i (lh )的值,因此本文采用前一时刻的状态值构造动态事件触发条件更符合逻辑㊂图3㊀h =3,τ=0.02时算法(7)的智能体状态图4㊀h =3,τ=0.02时算法(10)的智能体状态为了进一步分析采样周期的影响,在时延τ不变的情况下,选择不同的采样周期h ,其结果显示在图5中㊂对比图3可以看出,选择较大的采样周期则收敛速度减慢㊂事实上,这在算法(7)中是很正常的,因为较大的h 会削弱反馈增益并减少固定有限时间间隔中的控制更新次数,具体显示在图6和图7中㊂显然,当选择较大的采样周期时,智能体的通信频率显著下降,同时也会导致收敛速度减慢㊂因此,虽然采样周期允许任意大,但在收敛速度和通信频率之间需要做出权衡,以选择最优的采样周期㊂图5㊀h =1,τ=0.02时智能体的状态图6㊀h =3,τ=0.02时的事件触发时刻图7㊀h =1,τ=0.02时的事件触发时刻最后,固定采样周期h 的值,比较τ=0.02和τ=2时智能体的状态,结果如图8所示㊂显然,时延会使智能体找到全局最优点所需的时间更长,但由于其受采样周期的限制,最终仍可以对于任意有限延迟达成一致㊂图8㊀h =3,τ=2时智能体的状态36第3期㊀㊀㊀㊀㊀㊀㊀㊀㊀㊀㊀㊀夏伦超,等:基于周期采样的分布式动态事件触发优化算法4 结束语本文研究了无向图下的多智能体系统的优化问题,提出了一种基于动态事件触发机制的零梯度和算法㊂该机制中加入了与前一时刻智能体状态相关的动态变量,避免智能体状态接近最优值时频繁触发产生的通信负担㊂同时,在算法和触发条件设计中考虑了采样周期的影响,在所设计的算法下,允许采样周期任意大㊂对于有时延的系统,在最大允许传输延迟小于采样周期的情况下,给出了保证多智能体系统达到一致性和最优性的充分条件㊂今后拟将本算法向有向图和切换拓扑图方向推广㊂参考文献:[1]杨洪军,王振友.基于分布式算法和查找表的FIR滤波器的优化设计[J].山东理工大学学报(自然科学版),2009,23(5):104-106,110.[2]CHEN W,LIU L,LIU G P.Privacy-preserving distributed economic dispatch of microgrids:A dynamic quantization-based consensus scheme with homomorphic encryption[J].IEEE Transactions on Smart Grid,2022,14(1):701-713.[3]张丽馨,刘伟.基于改进PSO算法的含分布式电源的配电网优化[J].山东理工大学学报(自然科学版),2017,31(6):53-57.[4]KIA S S,CORTES J,MARTINEZ S.Distributed convex optimization via continuous-time coordination algorithms with discrete-time communication[J].Automatica,2015,55:254-264.[5]LI Z H,DING Z T,SUN J Y,et al.Distributed adaptive convex optimization on directed graphs via continuous-time algorithms[J]. IEEE Transactions on Automatic Control,2018,63(5):1434 -1441.[6]段书晴,陈森,赵志良.一阶多智能体受扰系统的自抗扰分布式优化算法[J].控制与决策,2022,37(6):1559-1566. [7]DIMAROGONAS D V,FRAZZOLI E,JOHANSSON K H.Distributed event-triggered control for multi-agent systems[J].IEEE Transactions on Automatic Control,2012,57(5):1291-1297.[8]KAJIYAMA Y C,HAYASHI N K,TAKAI S.Distributed subgradi-ent method with edge-based event-triggered communication[J]. IEEE Transactions on Automatic Control,2018,63(7):2248 -2255.[9]LIU J Y,CHEN W S,DAI H.Event-triggered zero-gradient-sum distributed convex optimisation over networks with time-varying topol-ogies[J].International Journal of Control,2019,92(12):2829 -2841.[10]COUTINHO P H S,PALHARES R M.Codesign of dynamic event-triggered gain-scheduling control for a class of nonlinear systems [J].IEEE Transactions on Automatic Control,2021,67(8): 4186-4193.[11]CHEN W S,REN W.Event-triggered zero-gradient-sum distributed consensus optimization over directed networks[J].Automatica, 2016,65:90-97.[12]TRAN N T,WANG Y W,LIU X K,et al.Distributed optimization problem for second-order multi-agent systems with event-triggered and time-triggered communication[J].Journal of the Franklin Insti-tute,2019,356(17):10196-10215.[13]YU G,SHEN Y.Event-triggered distributed optimisation for multi-agent systems with transmission delay[J].IET Control Theory& Applications,2019,13(14):2188-2196.[14]LIU K E,JI Z J,ZHANG X F.Periodic event-triggered consensus of multi-agent systems under directed topology[J].Neurocomputing, 2020,385:33-41.[15]崔丹丹,刘开恩,纪志坚,等.周期事件触发的多智能体分布式凸优化[J].控制工程,2022,29(11):2027-2033. [16]LU J,TANG C Y.Zero-gradient-sum algorithms for distributed con-vex optimization:The continuous-time case[J].IEEE Transactions on Automatic Control,2012,57(9):2348-2354. [17]LIU K E,JI Z J.Consensus of multi-agent systems with time delay based on periodic sample and event hybrid control[J].Neurocom-puting,2016,270:11-17.[18]ZHAO Z Y.Sample-baseddynamic event-triggered algorithm for op-timization problem of multi-agent systems[J].International Journal of Control,Automation and Systems,2022,20(8):2492-2502.[19]LIU J Y,CHEN W S.Distributed convex optimisation with event-triggered communication in networked systems[J].International Journal of Systems Science,2016,47(16):3876-3887.(编辑:杜清玲)46山东理工大学学报(自然科学版)2024年㊀。
S T A P L E 目 标 跟 踪 算 法
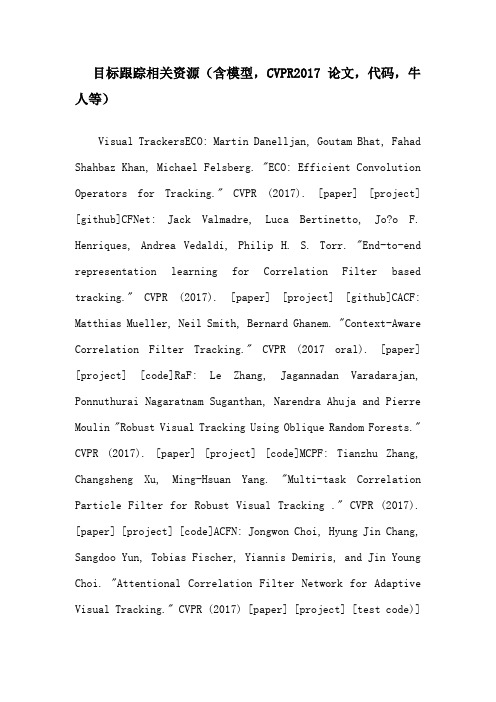
目标跟踪相关资源(含模型,CVPR2017论文,代码,牛人等)Visual TrackersECO: Martin Danelljan, Goutam Bhat, Fahad Shahbaz Khan, Michael Felsberg. "ECO: Efficient Convolution Operators for Tracking." CVPR (2017). [paper] [project] [github]CFNet: Jack Valmadre, Luca Bertinetto, Jo?o F. Henriques, Andrea Vedaldi, Philip H. S. Torr. "End-to-end representation learning for Correlation Filter based tracking." CVPR (2017). [paper] [project] [github]CACF: Matthias Mueller, Neil Smith, Bernard Ghanem. "Context-Aware Correlation Filter Tracking." CVPR (2017 oral). [paper] [project] [code]RaF: Le Zhang, Jagannadan Varadarajan, Ponnuthurai Nagaratnam Suganthan, Narendra Ahuja and Pierre Moulin "Robust Visual Tracking Using Oblique Random Forests." CVPR (2017). [paper] [project] [code]MCPF: Tianzhu Zhang, Changsheng Xu, Ming-Hsuan Yang. "Multi-task Correlation Particle Filter for Robust Visual Tracking ." CVPR (2017). [paper] [project] [code]ACFN: Jongwon Choi, Hyung Jin Chang, Sangdoo Yun, Tobias Fischer, Yiannis Demiris, and Jin Young Choi. "Attentional Correlation Filter Network for Adaptive Visual Tracking." CVPR (2017) [paper] [project] [test code)][training code]LMCF: Mengmeng Wang, Yong Liu, Zeyi Huang. "Large Margin Object Tracking with Circulant Feature Maps." CVPR (2017). [paper] [zhihu]ADNet: Sangdoo Yun, Jongwon Choi, Youngjoon Yoo, Kimin Yun, Jin Young Choi. "Action-Decision Networks for Visual Tracking with Deep Reinforcement Learning ." CVPR (2017). [paper] [project]CSR-DCF: Alan Luke?i?, Tomá? Vojí?, Luka ?ehovin, Ji?í Matas, Matej Kristan. "Discriminative Correlation Filter with Channel and Spatial Reliability." CVPR (2017). [paper][code]BACF: Hamed Kiani Galoogahi, Ashton Fagg, Simon Lucey. "Learning Background-Aware Correlation Filters for Visual Tracking." CVPR (2017). [paper]Bohyung Han, Jack Sim, Hartwig Adam "BranchOut: Regularization for Online Ensemble Tracking with Convolutional Neural Networks." CVPR (2017).SANet: Heng Fan, Haibin Ling. "SANet: Structure-Aware Network for Visual Tracking." CVPRW (2017). [paper] [project] [code]DNT: Zhizhen Chi, Hongyang Li, Huchuan Lu, Ming-Hsuan Yang. "Dual Deep Network for Visual Tracking." TIP (2017). [paper]DRT: Junyu Gao, Tianzhu Zhang, Xiaoshan Yang, Changsheng Xu. "Deep Relative Tracking." TIP (2017). [paper]BIT: Bolun Cai, Xiangmin Xu, Xiaofen Xing, Kui Jia, Jie Miao, Dacheng Tao. "BIT: Biologically Inspired Tracker." TIP (2016). [paper] [project][github]SiameseFC: Luca Bertinetto, Jack Valmadre, Jo?o F. Henriques, Andrea Vedaldi, Philip H.S. Torr. "Fully-Convolutional Siamese Networks for Object Tracking." ECCV workshop (2016). [paper] [project] [github]GOTURN: David Held, Sebastian Thrun, Silvio Savarese. "Learning to Track at 100 FPS with Deep Regression Networks." ECCV (2016). [paper] [project] [github]C-COT: Martin Danelljan, Andreas Robinson, Fahad Khan, Michael Felsberg. "Beyond Correlation Filters: Learning Continuous Convolution Operators for Visual Tracking." ECCV (2016). [paper] [project] [github]CF+AT: Adel Bibi, Matthias Mueller, and Bernard Ghanem. "Target Response Adaptation for Correlation Filter Tracking." ECCV (2016). [paper] [project]MDNet: Nam, Hyeonseob, and Bohyung Han. "Learning Multi-Domain Convolutional Neural Networks for Visual Tracking." CVPR (2016). [paper] [VOT_presentation] [project] [github]SINT: Ran Tao, Efstratios Gavves, Arnold W.M. Smeulders. "Siamese Instance Search for Tracking." CVPR (2016). [paper] [project]SCT: Jongwon Choi, Hyung Jin Chang, Jiyeoup Jeong, Yiannis Demiris, and Jin Young Choi. "Visual Tracking Using Attention-Modulated Disintegration and Integration." CVPR (2016). [paper] [project]STCT: Lijun Wang, Wanli Ouyang, Xiaogang Wang, and Huchuan Lu. "STCT: Sequentially TrainingConvolutional Networks for Visual Tracking." CVPR (2016). [paper] [github]SRDCFdecon: Martin Danelljan, Gustav H?ger, Fahad Khan, Michael Felsberg. "Adaptive Decontamination of the Training Set: A Unified Formulation for Discriminative Visual Tracking." CVPR (2016). [paper] [project]HDT: Yuankai Qi, Shengping Zhang, Lei Qin, Hongxun Yao, Qingming Huang, Jongwoo Lim, Ming-Hsuan Yang. "Hedged Deep Tracking." CVPR (2016). [paper] [project]Staple: Luca Bertinetto, Jack Valmadre, Stuart Golodetz, Ondrej Miksik, Philip H.S. Torr. "Staple: Complementary Learners for Real-Time Tracking." CVPR (2016). [paper] [project] [github]DLSSVM: Jifeng Ning, Jimei Yang, Shaojie Jiang, Lei Zhang and Ming-Hsuan Yang. "Object Tracking via Dual Linear Structured SVM and Explicit Feature Map." CVPR (2016). [paper] [code] [project]CNT: Kaihua Zhang, Qingshan Liu, Yi Wu, Minghsuan Yang. "Robust Visual Tracking via Convolutional Networks Without Training." TIP (2016). [paper] [code]DeepSRDCF: Martin Danelljan, Gustav H?ger, Fahad Khan, Michael Felsberg. "Convolutional Features for Correlation Filter Based Visual Tracking." ICCV workshop (2015). [paper] [project]SRDCF: Martin Danelljan, Gustav H?ger, Fahad Khan, Michael Felsberg. "Learning Spatially Regularized Correlation Filters for Visual Tracking." ICCV (2015). [paper][project]CNN-SVM: Seunghoon Hong, Tackgeun You, Suha Kwak and Bohyung Han. "Online Tracking by Learning Discriminative Saliency Map with Convolutional Neural Network ." ICML (2015) [paper] [project]CF2: Chao Ma, Jia-Bin Huang, Xiaokang Yang and Ming-Hsuan Yang. "Hierarchical Convolutional Features for Visual Tracking." ICCV (2015) [paper] [project] [github]FCNT: Lijun Wang, Wanli Ouyang, Xiaogang Wang, and Huchuan Lu. "Visual Tracking with Fully Convolutional Networks." ICCV (2015). [paper] [project] [github]LCT: Chao Ma, Xiaokang Yang, Chongyang Zhang, Ming-Hsuan Yang. "Long-term Correlation Tracking." CVPR (2015). [paper] [project] [github]RPT: Yang Li, Jianke Zhu and Steven C.H. Hoi. "Reliable Patch Trackers: Robust Visual Tracking by Exploiting Reliable Patches." CVPR (2015). [paper] [github]CLRST: Tianzhu Zhang, Si Liu, Narendra Ahuja, Ming-Hsuan Yang, Bernard Ghanem."Robust Visual Tracking Via Consistent Low-Rank Sparse Learning." IJCV (2015). [paper] [project] [code]DSST: Martin Danelljan, Gustav H?ger, Fahad Shahbaz Khan and Michael Felsberg. "Accurate Scale Estimation for Robust Visual Tracking." BMVC (2014). [paper] [PAMI] [project]MEEM: Jianming Zhang, Shugao Ma, and Stan Sclaroff. "MEEM: Robust Tracking via Multiple Experts using Entropy Minimization." ECCV (2014). [paper] [project]TGPR: Jin Gao,Haibin Ling, Weiming Hu, Junliang Xing. "Transfer Learning Based Visual Tracking with Gaussian Process Regression." ECCV (2014). [paper] [project]STC: Kaihua Zhang, Lei Zhang, Ming-Hsuan Yang, David Zhang. "Fast Tracking via Spatio-Temporal Context Learning." ECCV (2014). [paper] [project]SAMF: Yang Li, Jianke Zhu. "A Scale Adaptive Kernel Correlation Filter Tracker with Feature Integration." ECCV workshop (2014). [paper] [github]KCF: Jo?o F. Henriques, Rui Caseiro, Pedro Martins, Jorge Batista. "High-Speed Tracking with Kernelized Correlation Filters." TPAMI (2015). [paper] [project]OthersRe3: Daniel Gordon, Ali Farhadi, Dieter Fox. "Re3 : Real-Time Recurrent Regression Networks for Object Tracking." arXiv (2017). [paper] [code]DCFNet: Qiang Wang, Jin Gao, Junliang Xing, Mengdan Zhang, Weiming Hu. "Modeling and Propagating CNNs in a Tree Structure for Visual Tracking." arXiv (2017). [paper] [code]TCNN: Hyeonseob Nam, Mooyeol Baek, Bohyung Han. "Modeling and Propagating CNNs in a Tree Structure for Visual Tracking." arXiv (2016). [paper] [code]RDT: Janghoon Choi, Junseok Kwon, Kyoung Mu Lee. "Visual Tracking by Reinforced Decision Making." arXiv (2017). [paper]MSDAT: Xinyu Wang, Hanxi Li, Yi Li, Fumin Shen, Fatih Porikli . "Robust and Real-time Deep Tracking Via Multi-Scale DomainAdaptation." arXiv (2017). [paper]RLT: Da Zhang, Hamid Maei, Xin Wang, Yuan-Fang Wang. "Deep Reinforcement Learning for Visual Object Tracking in Videos." arXiv (2017). [paper]SCF: Wangmeng Zuo, Xiaohe Wu, Liang Lin, Lei Zhang, Ming-Hsuan Yang. "Learning Support Correlation Filters for Visual Tracking." arXiv (2016). [paper] [project]DMSRDCF: Susanna Gladh, Martin Danelljan, Fahad Shahbaz Khan, Michael Felsberg. "Deep Motion Features for Visual Tracking." ICPR Best Paper (2016). [paper]CRT: Kai Chen, Wenbing Tao. "Convolutional Regression for Visual Tracking." arXiv (2016). [paper]BMR: Kaihua Zhang, Qingshan Liu, and Ming-Hsuan Yang. "Visual Tracking via Boolean Map Representations." arXiv (2016). [paper]YCNN: Kai Chen, Wenbing Tao. "Once for All: a Two-flow Convolutional Neural Network for Visual Tracking." arXiv (2016). [paper]Learnet: Luca Bertinetto, Jo?o F. Henriques, Jack Valmadre, Philip H. S. Torr, Andrea Vedaldi. "Learning feed-forward one-shot learners." NIPS (2016). [paper]ROLO: Guanghan Ning, Zhi Zhang, Chen Huang, Zhihai He, Xiaobo Ren, Haohong Wang. "Spatially Supervised Recurrent Convolutional Neural Networks for Visual Object Tracking." arXiv (2016). [paper] [project] [github]Yao Sui, Ziming Zhang, Guanghui Wang, Yafei Tang, Li Zhang. "Real-Time Visual Tracking: Promoting the Robustness ofCorrelation Filter Learning." ECCV (2016). [paper] [project]Yao Sui, Guanghui Wang, Yafei Tang, Li Zhang. "Tracking Completion." ECCV (2016). [paper] [project]EBT: Gao Zhu, Fatih Porikli, and Hongdong Li. "Beyond Local Search: Tracking Objects Everywhere with Instance-Specific Proposals." CVPR (2016). [paper] [exe]RATM: Samira Ebrahimi Kahou, Vincent Michalski, Roland Memisevic. "RATM: Recurrent Attentive Tracking Model." arXiv (2015). [paper] [github]DAT: Horst Possegger, Thomas Mauthner, and Horst Bischof. "In Defense of Color-based Model-free Tracking." CVPR (2015). [paper] [project] [code]RAJSSC: Mengdan Zhang, Junliang Xing, Jin Gao, Xinchu Shi, Qiang Wang, Weiming Hu. "Joint Scale-Spatial Correlation Tracking with Adaptive Rotation Estimation." ICCV workshop (2015). [paper] [poster]SO-DLT: Naiyan Wang, Siyi Li, Abhinav Gupta, Dit-Yan Yeung. "Transferring Rich Feature Hierarchies for Robust Visual Tracking." arXiv (2015). [paper] [code]DLT: Naiyan Wang and Dit-Yan Yeung. "Learning A Deep Compact Image Representation for Visual Tracking." NIPS (2013). [paper] [project] [code]Naiyan Wang, Jianping Shi, Dit-Yan Yeung and Jiaya Jia. "Understanding and Diagnosing Visual Tracking Systems." ICCV (2015). [paper] [project] [code]Dataset-MoBe2016:Luka ?ehovin, Alan Luke?i?, Ale? Leonardis, Matej Kristan. "Beyond standard benchmarks: Parameterizing performance evaluation in visual object tracking." arXiv (2016). [paper]Dataset-UAV123: Matthias Mueller, Neil Smith and Bernard Ghanem. "A Benchmark and Simulator for UAV Tracking." ECCV (2016) [paper] [project] [dataset]Dataset-TColor-128: Pengpeng Liang, Erik Blasch, Haibin Ling. "Encoding color information for visual tracking: Algorithms and benchmark." TIP (2015) [paper] [project] [dataset]Dataset-NUS-PRO: Annan Li, Min Lin, Yi Wu, Ming-Hsuan Yang, and Shuicheng Yan. "NUS-PRO: A New Visual Tracking Challenge." PAMI (2015) [paper] [project] [Data_360(code:bf28)]?[Data_baidu]][View_360(code:515a)]?[View_baidu]]Dataset-PTB: Shuran Song and Jianxiong Xiao. "Tracking Revisited using RGBD Camera: Unified Benchmark and Baselines." ICCV (2013) [paper] [project] [5 validation] [95 evaluation]Dataset-ALOV300+: Arnold W. M. Smeulders, Dung M. Chu, Rita Cucchiara, Simone Calderara, Afshin Dehghan, Mubarak Shah. "Visual Tracking: An Experimental Survey." PAMI (2014) [paper] [project]?Mirror Link:ALOV300++ Dataset?Mirror Link:ALOV300++ GroundtruthDataset-DTB70: Siyi Li, Dit-Yan Yeung. "Visual Object Tracking for Unmanned Aerial Vehicles: A Benchmark andNew Motion Models." AAAI (2017) [paper] [project] [dataset]Dataset-VOT: [project][VOT13_paper_ICCV]The Visual Object Tracking VOT2013 challenge results[VOT14_paper_ECCV]The Visual Object Tracking VOT2014 challenge results[VOT15_paper_ICCV]The Visual Object Tracking VOT2015 challenge results[VOT16_paper_ECCV]The Visual Object Tracking VOT2016 challenge results深度学习方法(Deep Learning Method)由于其独有的优越性成为当前研究的热点,各种框架和算法层出不穷,这在前文的目标检测部分都有较为详细的介绍。
高级检索增强生成技术全面指南

高级检索增强生成技术全面指南引言高级检索增强生成技术是一项前沿的人工智能技术,通过有效的检索和生成算法,为用户提供更准确、更个性化的信息服务。
本文将全面介绍高级检索增强生成技术的原理、应用以及未来发展方向,以帮助读者深入了解该领域的最新研究进展。
1. 高级检索增强技术的概述高级检索增强技术是一种基于自然语言处理和机器学习的技术,旨在提高信息检索的准确性和个性化程度。
通过分析用户的查询意图和上下文信息,系统能够更好地理解用户需求,并提供更有针对性的搜索结果。
2. 高级检索增强技术的原理高级检索增强技术主要基于以下原理实现:a. 自然语言处理:通过对自然语言进行分词、词性标注、句法分析等处理,系统能够更好地理解用户的查询意图和文本语义。
b. 机器学习:通过构建训练集和使用机器学习算法,系统能够学习用户的偏好和上下文信息,从而提供更加个性化的搜索结果。
c. 深度学习:利用深度神经网络模型,系统能够进行更精确的语义理解和信息匹配,提升搜索结果的准确性。
3. 高级检索增强技术的应用领域高级检索增强技术在各个领域都有广泛的应用,包括但不限于以下几个方面:a. 互联网搜索引擎:通过提供更加准确的搜索结果,满足用户对信息的个性化需求。
b. 电子商务平台:通过分析用户的购物行为和历史数据,为用户推荐更符合其偏好的商品。
c. 在线教育平台:根据学生的学习习惯和知识水平,为其提供更加精准的学习资源。
d. 金融行业:通过分析用户的投资偏好和市场信息,为其提供更好的投资建议。
4. 高级检索增强技术的未来发展方向高级检索增强技术在未来有着广阔的发展前景,以下几个方向值得关注:a. 多模态信息检索:结合语音、图像等多种形式的信息,提供更加全面的检索服务。
b. 个性化推荐系统:通过分析用户的兴趣和行为,为其提供更加个性化的推荐服务。
c. 自动问答系统:利用自然语言处理和知识图谱等技术,实现智能问答功能。
d. 情感分析与情感生成:通过对用户情感的理解和生成,提供更加人性化的搜索结果和回答。
S t e r e o M a t c h i n g 文 献 笔 记

立体匹配综述阅读心得之Classification and evaluation of cost aggregation methods for stereo correspondence学习笔记之基于代价聚合算法的分类,主要针对cost aggregration 分类,20081.?Introduction经典的全局算法有:本文主要内容有:从精度的角度对比各个算法,主要基于文献【23】给出的评估方法,同时也在计算复杂度上进行了比较,最后综合这两方面提出一个trade-off的比较。
2?Classification?of?cost?aggregation?strategies?主要分为两种:1)The?former?generalizes?the?concept?of?variable?support?by? allowing?the?support?to?have?any?shape?instead?of?being?built?u pon?rectangular?windows?only.2)The?latter?assigns?adaptive?-?rather?than?fixed?-?weights?to?th e?points?belonging?to?the?support.大部分的代价聚合都是采用symmetric方案,也就是综合两幅图的信息。
(实际上在后面的博客中也可以发现,不一定要采用symmetric的形式,而可以采用asymmetric+TAC的形式,效果反而更好)。
采用的匹配函数为(matching?(or?error)?function?):Lp distance between two vectors包括SAD、Truncated SAD [30,25]、SSD、M-estimator [12]、similarity?function?based?on?point?distinctiveness[32] 最后要指出的是,本文基于平行平面(fronto-parallel)support。
- 1、下载文档前请自行甄别文档内容的完整性,平台不提供额外的编辑、内容补充、找答案等附加服务。
- 2、"仅部分预览"的文档,不可在线预览部分如存在完整性等问题,可反馈申请退款(可完整预览的文档不适用该条件!)。
- 3、如文档侵犯您的权益,请联系客服反馈,我们会尽快为您处理(人工客服工作时间:9:00-18:30)。
Taxonomy-based Adaptive Web Search MethodSaid Mirza Pahlevi and Hiroyuki KitagawaUniversity of TsukubaTsukuba,Ibaraki305-8573,Japanmirza,kitagawa@kde.is.tsukuba.ac.jpAbstractCurrent crawler-based search engines usually return a long list of search results containing a lot of noise docu-ments.By indexing collected documents on topic path in taxonomy,taxonomy-based search engines can improve the search result qualities.However,the searches are limited to the locally compiled databases.In this paper,we propose an adaptive web search method to improve the search result qualities enabling the users to search in many databases existing in the web space.The method has a characteris-tic that combines the taxonomy-based search engines and a machine learning technique.More specifically,we con-struct a rule-based classifier using pre-classified documents provided by a taxonomy-based search engine based on a se-lected context category on its taxonomy,and then use it to modify the user query.The resulting modified query will be sent to the crawler-based search engines and the returned results will be presented to the user.We evaluate the effec-tiveness of our method by showing that the returned results from the modified query almost contain documents that will be categorized into the selected context category.1.IntroductionIt is well-known that the crawler-based search engines have a good coverage of the web.It is because they crawl web pages automatically with only a bit of human inter-vention.However,since the engines store each crawled page/document as a bunch of keywords–no topic orga-nization of the pages is made,they typically only sup-port keyword-based search,which makes the returned re-sults generally contain a lot of noise documents.On the other hand,taxonomy(directory)-based search engines like Open Directory Project/ODP()have good precision of the search results.It is because they manage web pages by using taxonomy.Pages with similar topics are(logically)stored/grouped in the same category,which makes the user easilyfind the information sought.How-ever,since the classification is done manually,the engines can only cover a small fraction of the web.There are many attempts to classify the web content au-tomatically into a taxonomy[2][4].The main goal of those systems is to deal with the exponential growth in the vol-ume of the online text databases.They start with a small sample of corpus that is classified by hand to build a hierar-chical classifier.At run time,each web page retrieved will be classified automatically by the classifier into an appro-priate category.However,this approach has the following disadvantages.It is very hard to build a good and large hierarchical classifier that can deal with a wide variety of topics like ODP.Most of the classifiers cannot deal with modification of category hierarchies,for instance deletion and addi-tion of category nodes and their associated documents, which is important in the dynamic web environment.In this paper,we propose an adaptive web search method combining existing taxonomy-based search engines and a machine learning technique.More specifically,we modify the user query by using a rule-based classifier constructed from a document collection provided by a taxonomy-based search engine and send the modified query to the crawler-based search engines.The query is modified such that the results returned by the crawler-based search engines will almost contain documents that will be categorized into a selected category on the taxonomy of the taxonomy-based search engine.The modification process is adaptive–the classifier constructed is different depending on both the se-lected category and the query given by the user.The most important characteristic of our method is that it can be ap-plied to the query-based search of any databases compiled independently from the given taxonomy as long as they sup-port Boolean search.The paper is organized as follows.Section2elaborates our motivation.Section3describes the proposed method. Section4presents the experiments and results.Section5reviews related work.Section6gives our conclusions and suggests future work.2.MotivationOne of the main reasons why the crawler-based search engines generally return results containing a lot of noise documents is the ambiguity of terms used in the user query.This ambiguity originates from the use of very short queries,which is usual in the web environment[6].By al-lowing the user to select an appropriate category in a taxon-omy besides providing search terms,the taxonomy-based search engines can solve the search term ambiguity prob-lem.It is because the searches can now be restricted to doc-uments in the specified category.However,the searches can only be done against the manually compiled local databases, and cannot be expected to give many useful results.The idea here is instead of providing the search results from the taxonomy-based search engine directly to the user, it is better to get some useful keywordsfirst by”learning”the search results,then use the keywords to modify the user query andfinally send the modified query to the crawler-based search engines.3.Proposed methodOur challenge is to make the best of the existing taxonomy-based search engines to facilitate web searches. One way to do this is to extract some useful information from the taxonomy-based search engines and use the infor-mation to enrich the user query.Another challenge is to pre-serve the enriched user query so that it is still in a Boolean form.By doing this,we can get many useful information from many search engines available in the web space since they typically support Boolean query.In this paper,we assume that a crawler-based search engine and a taxonomy-based search engine are available and they can process queries in a Boolean form.We fur-ther assume that the taxonomy-based search engine allows search based on all categories existing in the taxonomy and provides additional information about the category of each matched document.(Most of major taxonomy-based search engines support this.)3.1.Query formulation and context category selec-tionThe query formulation process is the same as the search process that is usually used in taxonomy-based search en-gines.Tofind relevant information,first the user navigates the taxonomy provided by a taxonomy-based search en-gine.After the user has found a category related to the topic sought,he/she then constructs a keyword-based query1that will be sent to the engine.We call the category selected by the user as a context category.The user may choose the context category after browsing some documents under the category or seeing the category description.3.2.Separation of relevant and non-relevant docu-mentsThe system sends the given query condition to the taxonomy-based search engine without specifying a specific category.After the system receives the query results from the engine,it separates the relevant and non-relevant docu-ments based on the context category as follows.Documents that are classified into the context cate-gory(and subcategories under the context category)2 are considered to be relevant to the user query.This conforms to the method used by the taxonomy-based search engines to catch the user intent.Otherwise they are considered to be non-relevant to the query.Based on this procedure,a relevant document is a docu-ment that matches the user query condition and is classified into the context category.3.3.Query modification and executionAfter the relevant and non-relevant documents have been found,next the system modifies the user query and sends it to the crawler-based search engines.In this work,we use a rule-based classifier to modify a Boolean query.First,we construct a classifier for two new categories:relevant and non-relevant categories.The relevant category is a cate-gory for the relevant documents while the non-relevant cat-egory is for the non-relevant documents.The classifier is constructed by setting the relevant and non-relevant docu-ments as positive and negative examples,respectively.The resulting classifier is a set of rules in the form ofwhere is a conjunction of terms and is Relevant or Non-relevant category.Construction of such rule-based classi-fiers has been intensively studied in the area of machine learning[3][1].In our experiment explained in Section4, we use RIPPER[3]for constructing the classifier.Next we modify the initial user query with the rule set for the relevant category as follows.1.Let be the rule set for the relevantcategory,where.Note that isa conjunction of terms.Figure1.Experiment1.Figure2.Experiment2.2.Let be OR OR.3.Finally,we modify by AND-ing it with,that is,AND is the query condition of the modified query.It is important to note that,our method dynamically con-structs the classifier used to modify the user query.It is be-cause the classifier is constructed based on documents that match the query where the documents should be different from query to query.This characteristic is important be-cause each query usually has different meaning even for the same context category.The classifier is used to tell whether a document that matches the initial query condition will be classified to the context category.In other words,we use the classifier to eliminate the term ambiguity problem that may occur when conducting a search in the crawler-based search engines. Hence,by”sending”the classifier with the initial query to the crawler-based search engine(i.e.,transforming it to a Boolean condition and modifying the initial query),it seems that the returned results from the search engine will almost contain documents that are related to the user intent.4.Experiments and resultsTo evaluate the effectiveness of the proposed method,we compare the precision and recall of the modified query with those of the initial query.However,in order to calculate the precision and recall,we have to know the”true”answer of each query with respect to the selected context category. One way to do this is to check whether each document re-turned by the crawler-based search engine is relevant or not to the query.However,this approach requires too much ef-fort since the returned result size is usually very large.4.1.Experiment1To make relevance judgment easy,we simulate the crawler-based search engine with a taxonomy-based one. This can be done by having the search carried out against documents in all categories of the taxonomy-based search engine.That is,the search is not done against a particular category as usual.The”true”answer of a query from the simulated crawler-based search engine is the subset of docu-ments that match the query condition and that are classified into the query’s context category.(Note that the returned documents are associated with their categories.) The detail of Experiment1is shown in Figure1.The taxonomy-based search engine has two functions:it is used to catch the user intent by the proposed method and used as the simulated crawler-based search engine.Theflow of the experiment is as follows.First,we define an initial query and select an appropriate context category for the query from the taxonomy.After the query is submitted to the taxonomy-based search engine without specifying a specific category,we get the initial query result set.The result set is then divided into training set and test set(),which in turn are divided into relevant and non-relevant document sets based on the selected context category.The relevant and non-relevant documents in the training set are used to construct the classifier,which in turn is used to modify the initial query.The resulting modified query is then sent to the simulated crawler-based search engine(in this case the taxonomy-based search engine itself)and the precision and recall of the returned results are calculated based on.Table 1.Queries and their context categories.oil product for oil product in /Business/Industri q4:oil /Business/Financial_Services/Banking_Ser ATM health/Shopping/Health/Beauty/c4.2'/Shopping/c4.2industries /Business/Industries/Energy/Oil_and_Gas/c4.1'es/c4.1AND productapple cooking /Home/Cooking/Fruits_and_Vegetables/c3.2'/Home/Cooking/c3.2Apple computer /Computers/Systems/Apple/c3.1'/Computers/c3.1q3:applesalsa sauce /Shopping/Food/Condiments/c2.2'/Shopping/c2.2salsa art /Arts/Performing_Arts/Dance/Latin/Salsa/c2.1'/Arts/c2.1q2:salsaATM banks vices/Automatic_Teller_Machines/c1.2'/Business/c1.2networks /Computers/Data_Communications/c1.1'/Computers/c1.1q1:ATMContext categoryNotation Context category Notation Basic meaning Narrow context categoryBroad context category QueryThe precision and recall of the modified query is calcu-lated as follows.Let be a set of documents included in the result set of the modified query and in .Let be the set of relevant documents in ,namely,documents that meet the initial query condition and are classified intothe context category.Similarly,let be the set of rel-evant documents in .In this experiment,is the ”true”answer of the initial query because it is a relevant document set and it is not involved in constructing classi-fier of the proposed method.We calculate the precision and recall of the modified query using the following equation.Note that the recall of the initial query is always 1,while the precision is .We conduct the evaluation process with 3-fold cross validation and use Open Directory Project/ODP as the taxonomy-based search engine.The search results from ODP are lists of site entries,each of which consists of a title,description,address and category name.In the experi-ments,each site entry is regarded as a document.There are 4queries with 16different meanings used in the experiment.We select two context categories for each query such that the meaning of the query at each context category is different.For example,the meaning of query ”apple”at context category ”/Computers/”is completely different from the same query at different context category ”/Home/Cooking/”.We also do evaluation when the con-text categories are shifted to narrower concepts (i.e.,shift-ing them to subcategories).By shifting the context categoryof each query to a narrower one,we can shift the meaning of the query to a more specific topic.It is because the meaning of the query depends on its context category.For example,the meaning of query ”apple”at context category ”/Com-puters/”is to find pages related to Apple computers such as companies,hardware,software etc.,while the meaning of the query at context category ”/Computers/System/Apple/”is to find pages specially related to Apple computer system 3.Table 1shows the queries and their basic meanings at the selected context categories.Figures 3through 6show the experiment results.Prefix I and M denote the initial query and modified query,respectively.The recall of the initial query is omitted,because it is always 1.As can be seen,at broad context categories our proposed method can significantly increase the precision of the initial query with a low decrease in recall.At narrow context categories,the increase of the precision is slightly change but the decrease of recall is somewhat larger.The performance at the broad context categories is bet-ter than that at narrow context categories because the more specific the context categories are,the harder for the clas-sifiers to recognize documents belonging to the categories.This is also true in a real world situation.For example,it is generally easier to decide whether a document belongs to category ”/Health/”or ”/Art/”rather than to decide whether a document belongs to category ”/Health/Medicine/”or ”/Health/Pharmacy/”assuming the document has been clas-sified into the ”/Health/”category.Figure 3.Precision at broadcontextcategories.Figure 4.Recall at broad con-text categories.Figure 5.Precision at narrow contextcategories.Figure 6.Recall at narrow contextcategories.Figure 7.Precision at North-ernlight.Figure 8.Recall at Northern-light.4.2.Experiment 2We use a real crawler-based search engine in this ex-periment.To make relevance judgment easy,the (ini-tial/modified)query is sent to a crawler-based search engine that clusters its search results.Then,we identify clusters whose documents will most likely be classified into the se-lected context category and treat documents in the clusters as the ”true”answer for the query.We denote the selected and remaining clusters as relevant and non-relevant clus-ters ,respectively.The relevant clusters are picked by in-specting the cluster names and several documents existing in the clusters.However,since it is difficult to match the clusters with a context category having a very specific topic,we only take broad categories as the context categories in the experiment.(Note that queries used in the experiment are the same as those of Experiment 1.)Figure 2shows the flow of Experiment 2.As shown in the figure,we reuse the modified queries derived from Experiment 1.Let be a set of docu-ments from relevant clusters (non-relevant clusters)and letbe a set of documents from the modifiedquery results that are in .Note that,since we modified the initial query by AND-ing it with a Boolean condition,the returned results of the modified query should be a subset of those of the initial query.The recall and pre-cision of the modified query is shown below.Similar to Experiment 1,the recall of the initial query is always 1and the precision is shown below.4.3.SummaryFrom the experiments,it is clear that our method can re-trieve documents based on a selected context category with high precision regardless of the context category position in the taxonomy,while the recall change depending on the position.This indicates that our method is suitable for the searches in the web space that require high precision.5.Related workThe most closely related to our work is the Inquirus2 [5].They proposed an automated method for learning query modifications to locate pages within specified categories us-ing web search engines.Our work is different from theirs in that we use the existing taxonomy to catch the user in-tent.By using the existing taxonomy,we can make best of it as a useful information source.On the other hand,they useflat categories that they have to construct and provide to users.Another difference is that their query modification is static,while ours changes dynamically depending on the query provided by the user.Another related work is WebSifter II,a semantic taxonomy-based personalizable meta-search engine agent system[7].The system captures the user intent by hav-ing users create personalized taxonomies expressing their queries via the proposed Weighted Semantic-Taxonomy Tree.The taxonomies are then transformed into Boolean queries processed by existing search engines.Although the system uses taxonomy,it does not employ classifiers.In addition,the system needs a new taxonomy for each query intent.6.Conclusions and future workWe have proposed an adaptive web search method com-bining existing taxonomy-based search engines and a ma-chine learning technique.The method is able to adaptively modify the user query based on a selected context category from taxonomy provided by the taxonomy-based search en-gines.The modification is done so that the results returned by the crawler-based search engines may contain many doc-uments that will be categorized into the selected context cat-egory.This enables the user to freely shift the broadness of his/her intent topics just by selecting an appropriate cate-gory from the taxonomy.Our method is adaptive in that the classifier constructed to modify the query is different depending on both the se-lected category and the query given by the user.In other words,our method dynamically constructs a small classi-fier corresponding to a small part of the taxonomy that is related to the current user query.Moreover,since the mod-ified query is still in a Boolean form,our method can be applied to any databases supporting Boolean search.This characteristic is very important because we can get more relevant information not only from the crawler-based search engines but also from many legacy databases or hidden web (i.e.the web that cannot be indexed by crawlers)that actu-ally occupy a large portion of the web.We are going to do detailed evaluation of the perfor-mance(response time)of the proposed method.The re-sponse time is the time between issuing the initial query and modifying the query.In the experiment,most of the time is occupied by the time to collect the positive and negative examples from the taxonomy-based search engine. (Note that the time needed to build the classifier in only a few seconds.)It is because the taxonomy-based search en-gine does not present the resulting documents in one page at a time,so that we have to fetch them by sending sev-eral HTTP requests.We can decrease the time by sending the requests concurrently using multi-thread process.Fur-thermore,since the documents are only used to build the classifier(not presented to the user),we can stop sending the requests after we get enough examples to build the clas-sifier.7.AcknowledgementThis research has been supported in part by the Grant-in-Aid for Scientific Research from Japan Agency for the Promotion of Science.References[1] C.Apte et al.Towards language independent automated learn-ing of text categorization models.In SIGIR Conference on Research and Development in Information Retrieval,pages 23–30,1994.[2]S.Chakrabarti et al.Scalable feature selection,classificationand signature generation for organizing large text databases into hierarchical topic taxonomies.The VLDB journal, 7(3):163–178,1998.[3]W.W.Cohen.Fast effective rule induction.InternationalConference on Machine Learning,pages115–123,1995. [4]S.Dumais and H.Chen.Hierarchical classification of webcontent.In SIGIR Conference on Research and Development in Information Retrieval,pages256–263,2000.[5] E.J.Glover et al.Improving category specific web searchby learning query modifications.Symposium on Applications and the Internet(SAINT),pages23–31,2001.[6] B.J.Jansen et al.Real life information retrieval:A study ofuser queries on the web.SIGIR Forum,32(1):5–18,1998. [7]L.Kerschberg et al.A semantic taxonomy-based personaliz-able meta-search agent.Proceedings of the second Interna-tional Conference on Web Information Systems Engineering (WISE),pages53–62,2001.。