英文版greene 计量经济学Ch5
计量经济学课件英文版 伍德里奇
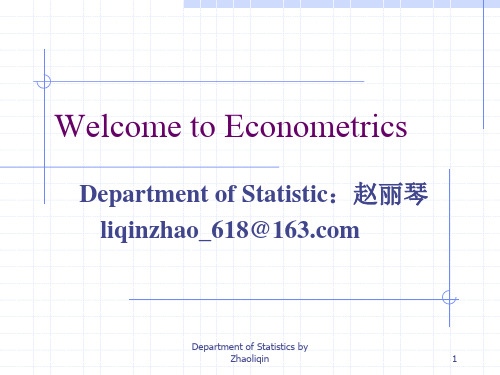
20
1.3 The Structure of Economic Data
Cross Sectional Time Series Panel
Department of Statistics by Zhaoliqin
21
Types of Data – Cross Sectional
Department of Statistics by Zhaoliqin 10
Why study Econometrics?
An empirical analysis uses data to test a theory or to estimate a relationship
A formal economic model can be tested
Theory may be ambiguous as to the effect of some policy change – can use econometrics to evaluate the program
Department of Statistics by Zhaoliqin 11
1.2 steps in empirical economic analysis
Welcome to Econometrics
Department of Statistic:赵丽琴 liqinzhao_618@
Department of Statistics by Zhaoliqin
1
About this Course
Textbook: Jeffrey M. Wooldridge, Introductory Econometrics—A Modern Approach. Main Software: Eviews. Sample data can be acquired from internet. If time permitted, R commands will be introduced.
高级经济学书籍
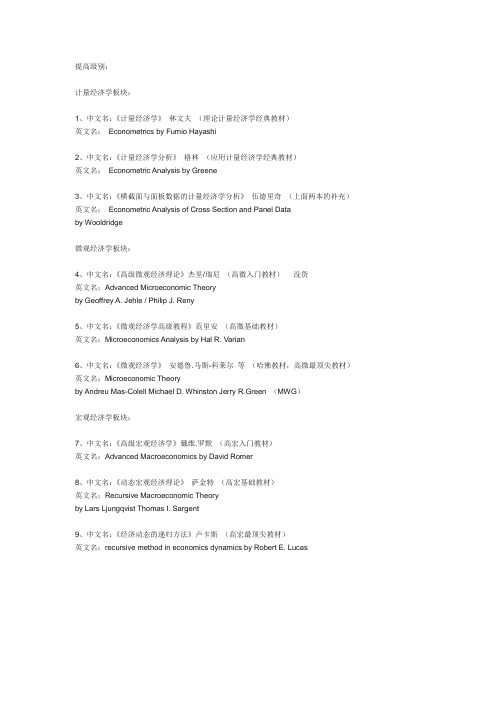
提高级别:计量经济学板块:1、中文名:《计量经济学》林文夫(理论计量经济学经典教材)英文名:Econometrics by Fumio Hayashi2、中文名:《计量经济学分析》格林(应用计量经济学经典教材)英文名:Econometric Analysis by Greene3、中文名:《横截面与面板数据的计量经济学分析》伍德里奇(上面两本的补充)英文名:Econometric Analysis of Cross Section and Panel Databy Wooldridge微观经济学板块:4、中文名:《高级微观经济理论》杰里/瑞尼(高微入门教材)没货英文名:Advanced Microeconomic Theoryby Geoffrey A. Jehle / Philip J. Reny5、中文名:《微观经济学高级教程》范里安(高微基础教材)英文名:Microeconomics Analysis by Hal R. Varian6、中文名:《微观经济学》安德鲁.马斯-科莱尔等(哈佛教材,高微最顶尖教材)英文名:Microeconomic Theoryby Andreu Mas-Colell Michael D. Whinston Jerry R.Green (MWG)宏观经济学板块:7、中文名:《高级宏观经济学》戴维.罗默(高宏入门教材)英文名:Advanced Macroeconomics by David Romer8、中文名:《动态宏观经济理论》萨金特(高宏基础教材)英文名:Recursive Macroeconomic Theoryby Lars Ljungqvist Thomas I. Sargent9、中文名:《经济动态的递归方法》卢卡斯(高宏最顶尖教材)英文名:recursive method in economics dynamics by Robert E. Lucas。
伍德里奇《计量经济学》chap5
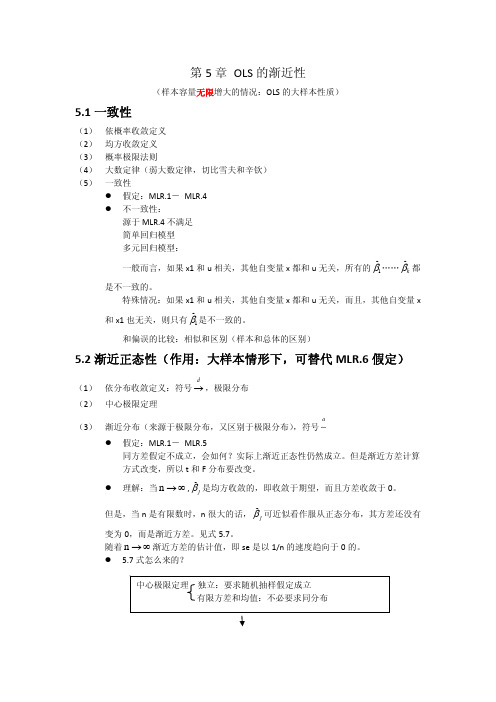
第5章 OLS 的渐近性(样本容量无限增大的情况:OLS 的大样本性质)5.1一致性(1) 依概率收敛定义 (2) 均方收敛定义 (3) 概率极限法则(4) 大数定律(弱大数定律,切比雪夫和辛钦) (5)一致性z 假定:MLR.1- MLR.4 z 不一致性:源于MLR.4不满足 简单回归模型 多元回归模型:一般而言,如果x1和u 相关,其他自变量x 都和u 无关,所有的1ˆβ……ˆkβ都是不一致的。
特殊情况:如果x1和u 相关,其他自变量x 都和u 无关,而且,其他自变量x和x1也无关,则只有1ˆβ是不一致的。
和偏误的比较:相似和区别(样本和总体的区别)5.2渐近正态性(作用:大样本情形下,可替代MLR.6假定)(1) 依分布收敛定义:符号d→,极限分布 (2) 中心极限定理(3) 渐近分布(来源于极限分布,又区别于极限分布),符号a∼z 假定:MLR.1- MLR.5同方差假定不成立,会如何?实际上渐近正态性仍然成立。
但是渐近方差计算方式改变,所以t 和F 分布要改变。
z 理解:当n →∞,ˆj β是均方收敛的,即收敛于期望,而且方差收敛于0。
但是,当n 是有限数时,n 很大的话,ˆjβ可近似看作服从正态分布,其方差还没有变为0,而是渐近方差。
见式5.7。
随着n →∞渐近方差的估计值,即se 是以1/n 的速度趋向于0的。
z 5.7式怎么来的?)12ˆijr −∑来5.3渐近有效性z 渐近有效性定义:“致,且渐近正态”的估计量,其渐近协方差阵小于等于任何一个一致且渐近正态的估计量的协方差阵,则它是渐近有效的。
z 格林P76:我们还没有在大样本中证明OLS 按照“任何”一种标准都是最优的。
定理5.3也不过是告诉我们:在某一类估计量中,OLS 是最优的,即渐近有效的。
ch5

ˆ ˆ ˆ Yt = β 0 + β 1 X t
若回归函数在两个时刻t1,t2发生结构变 若回归函数在两个时刻 化,可定义两个虚拟变量
1, t ≥ t 2 D1 = 0, t < t2 1, t ≥ t1 Dt = 0 , t < t1
相应的回归模型 Yt = β 0 + β1 X t + β 2 ( X t − X t ) Dt + β 3 ( X t − X t ) D2 + µt
Yi = β 0 + β1 X i + β 2 D1 + β 3 D2 + µ i (i = 1,2, L , n )
其中: 为企业职工的薪金, 其中:Yi 为企业职工的薪金,Xi 为工龄
1 (男性) D1 = 0 (女性)
1 (本科及以上) D2 = 0 (本科以下)
二、虚拟变量的引入
用OLS法可得样本回归函数 法可得样本回归函数
ˆ ˆ ˆ ˆ Yt = β 0 + β1 X t + β 2 ( X t − X t* ) Dt
ˆ ˆ ˆ ˆ ˆ 当t ≥ t*时,Dt=1, Yt = ( β 0 − β 2 X t* ) + ( β1 + β 2 ) X t 时
当t < t*时,Dt=0, 时
其矩阵形式为: 其矩阵形式为:
Y = (X
β D ) + µ α
如果取六个观测值,其中春季与夏季取了两次, 如果取六个观测值,其中春季与夏季取了两次,秋、冬各 取到一次观测值,则解释变量矩阵: 取到一次观测值,则解释变量矩阵:
1 1 1 D) = 1 1 1
1, , D= 0, , 女性 男性
计量经济学(英文PPT)Chapter 5 Interval Estimation and Hypothesis Testing

relying on the point estimate alone, we may construct a interval around the point estimate, such that this interval has a certain probability of the true parameter value. This is the idea behind the interval estimation.
ˆ2 t / 2se(ˆ2 )
Example: P123
Second , confidence interval for 1
By the virtue of E(ˆ1 ) 1 and
2 ˆ1 n
Xi2 xi 2
2
,we
can
get
the
equations
as
Equation(5.2.1)shows that the interval has a probability 1 of including the
true 2. The interval estimator thus give a ranger of values within which the true
§5.3confidence intervals for regression
Coefficients 1 and 2
First, confidence interval for 2
It is shown before that the OLS estimator ˆ1 and ˆ2 are themselves normally
英文版greene 计量经济学Ch5
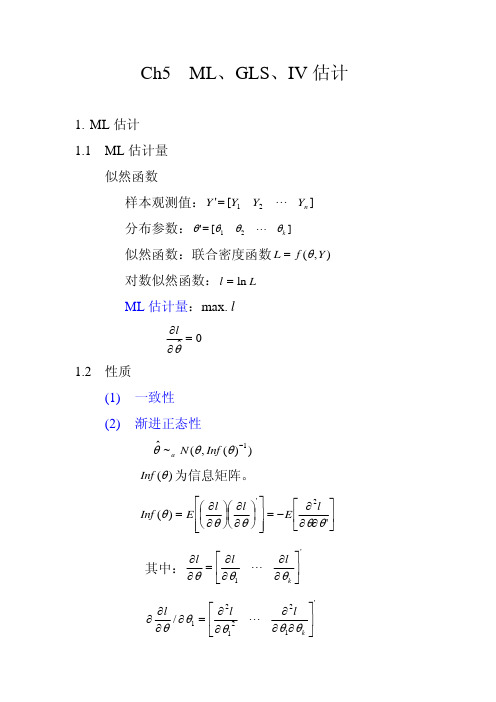
0⎤
2σ~
2
⎥ ⎥
n⎦
统计量:
LM = s'(θ~)Inf (θ~)s(θ~)
=
n
u~'
X
(
X ' X )−1 u~'u~
X
'u~
= nRu~2→X
特点:只需估计受约束方程。
等价表述:
LM = n(uˆ'uˆR − uˆ'uˆUR ) uˆ ' uˆ R
三个检验统计量之间的关系:
W ≥ LR ≥ LM
−
1 2σ 2
(Y
−
Xβ )' Ω −1(Y
−
Xβ )
解析式:
∂l ∂βˆ
=
0
∂l ∂σˆ 2
=
0
估计量:
βˆ = ( X ' Ω −1 X )−1 X ' Ω −1Y σˆ 2 = (Y − Xβˆ)' Ω −1(Y − Xβˆ) / n 4.2 GLS 估计 设非奇异矩阵:
P' P = Ω −1 PY = PXβ + Pu
5.2 2SLS 估计 解释变量对工具变量回归
Xˆ = Z (Z ' X )−1 Z ' X
Y 对 Xˆ 回归:
βˆ2SLS = βˆIV
5.3 IV 估计量与工具变量个数 工具变量个数增加,方差减小,偏误增大。
工具变量个数为 n 时, βˆIV = βˆOLS 。
5.4 线性约束检验
(1) Y 对 Xˆ 分别进行受约束和无约束回归 (2) 计算残差:
var(Pu) = E(Puu' P' ) = σ 2I GLS 估计量:
《计量经济学》ch_02_wooldridge_5e_ppt.ppt

as long as
By how much does the dependent variable change if the independent variable is increased by one unit?
Interpretation only correct if all other things remain equal when the independent variable is increased by one unit
Labor force experience, tenure with current employer, work ethic, intelligence …
Chapter End © 2013 Cengage Learning. All Rights Reserved. May not be scanned, copied or duplicated, or posted to a publicly accessible website, in whole or in part.
Chapter 2 The Simple Regression Model
2.1 Definition of the Simple Regression Model (1/6)
„Explains variable in terSlope parameter
Chapter 2 The Simple Regression Model
2.1 Definition of the Simple Regression Model 2.2 Deriving the Ordinary Least Squares Estimates 2.3 Algebraic Properties of OLS on Any Sample of Data 2.4 Units of Measurement and Functional Form 2.5 Expected Values and Variances of the OLS Estimators 2.6 Regression through the Origin and Regression on a Constant Introduction to Eviews Assignments: Problems 6-10, Computer Exercises C2, C4, C6
计量经济学-chapter 5
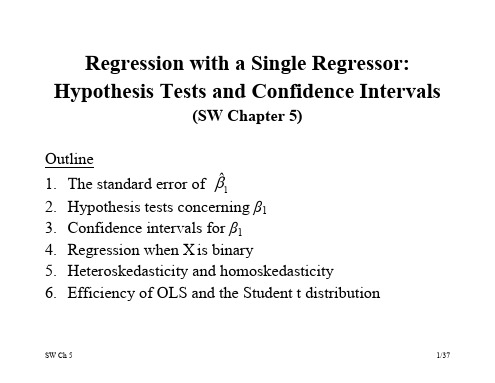
ˆ variance of the sampling distribution of 1
SW Ch 5
5/37
ˆ) Formula for SE( 1
ˆ (large n): Recall the expression for the variance of 1
2 var[( X ) u ] i x i v ˆ)= var( = , where vi = (Xi – X)ui. 1 2 2 2 2 n( X ) n( X ) ˆ replaces the unknown The estimator of the variance of
SW Ch 5
7/37
Example: Test Scores and STR, California data Estimated regression line: TestScore = 698.9 – 2.28STR Regression software reports the standard errors:
0 1
distributions in large samples Testing: H0: 1 = 1,0 v. 1 1,0 (1,0 is the value of 1 under H0) ˆ – 1,0)/SE( ˆ) t = (
, where vi = (Xi – X)ui
SW Ch 5
3/37
ˆ Hypothesis Testing and the Standard Error of 1 (Section 5.1)
The objective is to test a hypothesis, like 1 = 0, using data – to reach a tentative conclusion whether the (null) hypothesis is correct or incorrect. General setup Null hypothesis and two-sided alternative: H0: 1 = 1,0 vs. H1: 1 1,0 where 1,0 is the hypothesized value under the null. Null hypothesis and one-sided alternative: H0: 1 = 1,0 vs. H1: 1 < 1,0
伍德里奇计量经济学英文版各章总结
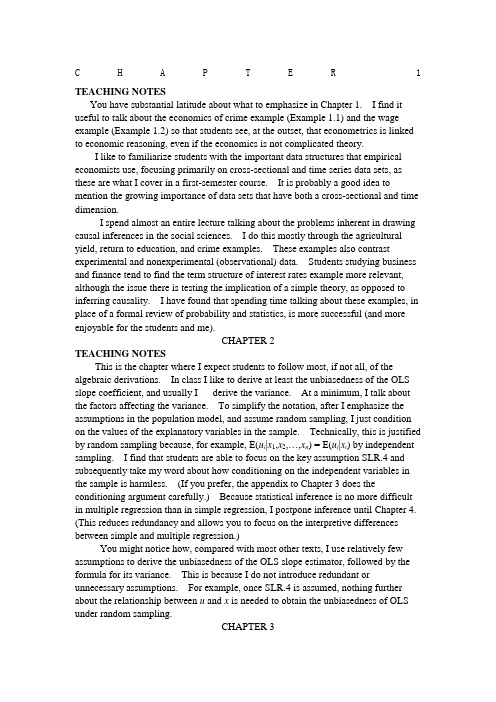
C H A P T E R 1 TEACHING NOTESYou have substantial latitude about what to emphasize in Chapter 1. I find it useful to talk about the economics of crime example (Example 1.1) and the wage example (Example 1.2) so that students see, at the outset, that econometrics is linked to economic reasoning, even if the economics is not complicated theory.I like to familiarize students with the important data structures that empirical economists use, focusing primarily on cross-sectional and time series data sets, as these are what I cover in a first-semester course. It is probably a good idea to mention the growing importance of data sets that have both a cross-sectional and time dimension.I spend almost an entire lecture talking about the problems inherent in drawing causal inferences in the social sciences. I do this mostly through the agricultural yield, return to education, and crime examples. These examples also contrast experimental and nonexperimental (observational) data. Students studying business and finance tend to find the term structure of interest rates example more relevant, although the issue there is testing the implication of a simple theory, as opposed to inferring causality. I have found that spending time talking about these examples, in place of a formal review of probability and statistics, is more successful (and more enjoyable for the students and me).CHAPTER 2TEACHING NOTESThis is the chapter where I expect students to follow most, if not all, of the algebraic derivations. In class I like to derive at least the unbiasedness of the OLS slope coefficient, and usually I derive the variance. At a minimum, I talk about the factors affecting the variance. To simplify the notation, after I emphasize the assumptions in the population model, and assume random sampling, I just condition on the values of the explanatory variables in the sample. Technically, this is justified by random sampling because, for example, E(u i|x1,x2,…,x n) = E(u i|x i) by independent sampling. I find that students are able to focus on the key assumption SLR.4 and subsequently take my word about how conditioning on the independent variables in the sample is harmless. (If you prefer, the appendix to Chapter 3 does the conditioning argument carefully.) Because statistical inference is no more difficultin multiple regression than in simple regression, I postpone inference until Chapter 4. (This reduces redundancy and allows you to focus on the interpretive differences between simple and multiple regression.)You might notice how, compared with most other texts, I use relatively few assumptions to derive the unbiasedness of the OLS slope estimator, followed by the formula for its variance. This is because I do not introduce redundant or unnecessary assumptions. For example, once SLR.4 is assumed, nothing further about the relationship between u and x is needed to obtain the unbiasedness of OLS under random sampling.CHAPTER 3TEACHING NOTESFor undergraduates, I do not work through most of the derivations in this chapter, at least not in detail. Rather, I focus on interpreting the assumptions, which mostly concern the population. Other than random sampling, the only assumption that involves more than population considerations is the assumption about no perfect collinearity, where the possibility of perfect collinearity in the sample (even if it does not occur in the population) should be touched on. The more important issue is perfect collinearity in the population, but this is fairly easy to dispense with via examples. These come from my experiences with the kinds of model specification issues that beginners have trouble with.The comparison of simple and multiple regression estimates – based on the particular sample at hand, as opposed to their statistical properties – usually makes a strong impression. Sometimes I do not bother with the “partialling out” interpretation of multiple regression.As far as statistical properties, notice how I treat the problem of including an irrelevant variable: no separate derivation is needed, as the result follows form Theorem 3.1.I do like to derive the omitted variable bias in the simple case. This is not much more difficult than showing unbiasedness of OLS in the simple regression case under the first four Gauss-Markov assumptions. It is important to get the students thinking about this problem early on, and before too many additional (unnecessary) assumptions have been introduced.I have intentionally kept the discussion of multicollinearity to a minimum. This partly indicates my bias, but it also reflects reality. It is, of course, very important for students to understand the potential consequences of having highly correlated independent variables. But this is often beyond our control, except that we can ask less of our multiple regression analysis. If two or more explanatory variables are highly correlated in the sample, we should not expect to precisely estimate their ceteris paribus effects in the population.I find extensive treatments of multicollinearity, where one “tests” or somehow “solves” the multicollinearity problem, to be misleading, at best. Even the organization of some texts gives the impression that imperfect multicollinearity is somehow a violation of the Gauss-Markov assumptions: they include multicollinearity in a chapter or part of the book devoted to “violation of the basic assumptions,” or something like that. I have noticed that master’s students who have had some undergraduate econometrics are often confused on the multicollinearity issue. It is very important that students not confuse multicollinearity among the included explanatory variables in a regression model with the bias caused by omitting an important variable.I do not prove the Gauss-Markov theorem. Instead, I emphasize its implications. Sometimes, and certainly for advanced beginners, I put a special case of Problem 3.12 on a midterm exam, where I make a particular choice for the function g(x). Rather than have the students directly compare the variances, they shouldappeal to the Gauss-Markov theorem for the superiority of OLS over any other linear, unbiased estimator.CHAPTER 4TEACHING NOTESAt the start of this chapter is good time to remind students that a specific error distribution played no role in the results of Chapter 3. That is because only the first two moments were derived under the full set of Gauss-Markov assumptions. Nevertheless, normality is needed to obtain exact normal sampling distributions (conditional on the explanatory variables). I emphasize that the full set of CLM assumptions are used in this chapter, but that in Chapter 5 we relax the normality assumption and still perform approximately valid inference. One could argue that the classical linear model results could be skipped entirely, and that only large-sample analysis is needed. But, from a practical perspective, students still need to know where the t distribution comes from because virtually all regression packages report t statistics and obtain p -values off of the t distribution. I then find it very easy tocover Chapter 5 quickly, by just saying we can drop normality and still use t statistics and the associated p -values as being approximately valid. Besides, occasionally students will have to analyze smaller data sets, especially if they do their own small surveys for a term project.It is crucial to emphasize that we test hypotheses about unknown population parameters. I tell my students that they will be punished if they write something likeH 0:1ˆ = 0 on an exam or, even worse, H 0: .632 = 0. One useful feature of Chapter 4 is its illustration of how to rewrite a population model so that it contains the parameter of interest in testing a single restriction. I find this is easier, both theoretically and practically, than computing variances that can, in some cases, depend on numerous covariance terms. The example of testing equality of the return to two- and four-year colleges illustrates the basic method, and shows that the respecified model can have a useful interpretation. Of course, some statistical packages now provide a standard error for linear combinations of estimates with a simple command, and that should be taught, too.One can use an F test for single linear restrictions on multiple parameters, but this is less transparent than a t test and does not immediately produce the standard error needed for a confidence interval or for testing a one-sided alternative. The trick of rewriting the population model is useful in several instances, including obtaining confidence intervals for predictions in Chapter 6, as well as for obtaining confidence intervals for marginal effects in models with interactions (also in Chapter6).The major league baseball player salary example illustrates the differencebetween individual and joint significance when explanatory variables (rbisyr and hrunsyr in this case) are highly correlated. I tend to emphasize the R -squared form of the F statistic because, in practice, it is applicable a large percentage of the time, and it is much more readily computed. I do regret that this example is biased toward students in countries where baseball is played. Still, it is one of the better examplesof multicollinearity that I have come across, and students of all backgrounds seem to get the point.CHAPTER 5TEACHING NOTESChapter 5 is short, but it is conceptually more difficult than the earlier chapters, primarily because it requires some knowledge of asymptotic properties of estimators. In class, I give a brief, heuristic description of consistency and asymptotic normality before stating the consistency and asymptotic normality of OLS. (Conveniently, the same assumptions that work for finite sample analysis work for asymptotic analysis.) More advanced students can follow the proof of consistency of the slope coefficient in the bivariate regression case. Section E.4 contains a full matrix treatment of asymptotic analysis appropriate for a master’s level course.An explicit illustration of what happens to standard errors as the sample size grows emphasizes the importance of having a larger sample. I do not usually cover the LM statistic in a first-semester course, and I only briefly mention the asymptotic efficiency result. Without full use of matrix algebra combined with limit theorems for vectors and matrices, it is very difficult to prove asymptotic efficiency of OLS.I think the conclusions of this chapter are important for students to know, even though they may not fully grasp the details. On exams I usually include true-false type questions, with e xplanation, to test the students’ understanding of asymptotics. [For example: “In large samples we do not have to worry about omitted variable bias.” (False). Or “Even if the error term is not normally distributed, in large samples we can still compute approximately valid confidence intervals under the Gauss-Markov assumptions.” (True).]CHAPTER 6TEACHING NOTESI cover most of Chapter 6, but not all of the material in great detail. I use the example in Table 6.1 to quickly run through the effects of data scaling on the important OLS statistics. (Students should already have a feel for the effects of data scaling on the coefficients, fitting values, and R-squared because it is covered in Chapter 2.) At most, I briefly mention beta coefficients; if students have a need for them, they can read this subsection.The functional form material is important, and I spend some time on more complicated models involving logarithms, quadratics, and interactions. An important point for models with quadratics, and especially interactions, is that we need to evaluate the partial effect at interesting values of the explanatory variables. Often, zero is not an interesting value for an explanatory variable and is well outside the range in the sample. Using the methods from Chapter 4, it is easy to obtain confidence intervals for the effects at interesting x values.As far as goodness-of-fit, I only introduce the adjusted R-squared, as I think using a slew of goodness-of-fit measures to choose a model can be confusing to novices (and does not reflect empirical practice). It is important to discuss how, ifwe fixate on a high R-squared, we may wind up with a model that has no interesting ceteris paribus interpretation.I often have students and colleagues ask if there is a simple way to predict y when log(y) has been used as the dependent variable, and to obtain a goodness-of-fit measure for the log(y) model that can be compared with the usual R-squared obtained when y is the dependent variable. The methods described in Section 6.4 are easy to implement and, unlike other approaches, do not require normality.The section on prediction and residual analysis contains several important topics, including constructing prediction intervals. It is useful to see how much wider the prediction intervals are than the confidence interval for the conditional mean. I usually discuss some of the residual-analysis examples, as they have real-world applicability.CHAPTER 7TEACHING NOTESThis is a fairly standard chapter on using qualitative information in regression analysis, although I try to emphasize examples with policy relevance (and only cross-sectional applications are included.).In allowing for different slopes, it is important, as in Chapter 6, to appropriately interpret the parameters and to decide whether they are of direct interest. For example, in the wage equation where the return to education is allowed to depend on gender, the coefficient on the female dummy variable is the wage differential between women and men at zero years of education. It is not surprising that we cannot estimate this very well, nor should we want to. In this particular example we would drop the interaction term because it is insignificant, but the issue of interpreting the parameters can arise in models where the interaction term is significant.In discussing the Chow test, I think it is important to discuss testing for differences in slope coefficients after allowing for an intercept difference. In many applications, a significant Chow statistic simply indicates intercept differences. (See the example in Section 7.4 on student-athlete GPAs in the text.) From a practical perspective, it is important to know whether the partial effects differ across groups or whether a constant differential is sufficient.I admit that an unconventional feature of this chapter is its introduction of the linear probability model. I cover the LPM here for several reasons. First, the LPM is being used more and more because it is easier to interpret than probit or logit models. Plus, once the proper parameter scalings are done for probit and logit, the estimated effects are often similar to the LPM partial effects near the mean or median values of the explanatory variables. The theoretical drawbacks of the LPM are often of secondary importance in practice. Computer Exercise C7.9 is a good one to illustrate that, even with over 9,000 observations, the LPM can deliver fitted values strictly between zero and one for all observations.If the LPM is not covered, many students will never know about using econometrics to explain qualitative outcomes. This would be especially unfortunate for students who might need to read an article where an LPM is used, or who mightwant to estimate an LPM for a term paper or senior thesis. Once they are introduced to purpose and interpretation of the LPM, along with its shortcomings, they can tackle nonlinear models on their own or in a subsequent course.A useful modification of the LPM estimated in equation (7.29) is to drop kidsge6 (because it is not significant) and then define two dummy variables, one for kidslt6 equal to one and the other for kidslt6 at least two. These can be included in place of kidslt6 (with no young children being the base group). This allows a diminishing marginal effect in an LPM. I was a bit surprised when a diminishing effect did not materialize.CHAPTER 8TEACHING NOTESThis is a good place to remind students that homoskedasticity played no role in showing that OLS is unbiased for the parameters in the regression equation. In addition, you probably should mention that there is nothing wrong with the R-squared or adjusted R-squared as goodness-of-fit measures. The key is that these are estimates of the population R-squared, 1 – [Var(u)/Var(y)], where the variances are the unconditional variances in the population. The usual R-squared, and the adjusted version, consistently estimate the population R-squared whether or not Var(u|x) = Var(y|x) depends on x. Of course, heteroskedasticity causes the usual standard errors, t statistics, and F statistics to be invalid, even in large samples, with or without normality.By explicitly stating the homoskedasticity assumption as conditional on the explanatory variables that appear in the conditional mean, it is clear that only heteroskedasticity that depends on the explanatory variables in the model affects the validity of standard errors and test statistics. The version of the Breusch-Pagan test in the text, and the White test, are ideally suited for detecting forms of heteroskedasticity that invalidate inference obtained under homoskedasticity. If heteroskedasticity depends on an exogenous variable that does not also appear in the mean equation, this can be exploited in weighted least squares for efficiency, but only rarely is such a variable available. One case where such a variable is available is when an individual-level equation has been aggregated. I discuss this case in the text but I rarely have time to teach it.As I mention in the text, other traditional tests for heteroskedasticity, such as the Park and Glejser tests, do not directly test what we want, or add too many assumptions under the null. The Goldfeld-Quandt test only works when there is a natural way to order the data based on one independent variable. This is rare in practice, especially for cross-sectional applications.Some argue that weighted least squares estimation is a relic, and is no longer necessary given the availability of heteroskedasticity-robust standard errors and test statistics. While I am sympathetic to this argument, it presumes that we do not care much about efficiency. Even in large samples, the OLS estimates may not be precise enough to learn much about the population parameters. With substantial heteroskedasticity we might do better with weighted least squares, even if the weighting function is misspecified. As discussed in the text on pages 288-289, onecan, and probably should, compute robust standard errors after weighted least squares. For asymptotic efficiency comparisons, these would be directly comparable to the heteroskedasiticity-robust standard errors for OLS.Weighted least squares estimation of the LPM is a nice example of feasible GLS, at least when all fitted values are in the unit interval. Interestingly, in the LPM examples in the text and the LPM computer exercises, the heteroskedasticity-robust standard errors often differ by only small amounts from the usual standard errors. However, in a couple of cases the differences are notable, as in Computer ExerciseC8.7.CHAPTER 9TEACHING NOTESThe coverage of RESET in this chapter recognizes that it is a test for neglected nonlinearities, and it should not be expected to be more than that. (Formally, it can be shown that if an omitted variable has a conditional mean that is linear in the included explanatory variables, RESET has no ability to detect the omitted variable. Interested readers may consult my chapter in Companion to Theoretical Econometrics, 2001, edited by Badi Baltagi.) I just teach students the F statistic version of the test.The Davidson-MacKinnon test can be useful for detecting functional form misspecification, especially when one has in mind a specific alternative, nonnested model. It has the advantage of always being a one degree of freedom test.I think the proxy variable material is important, but the main points can be made with Examples 9.3 and 9.4. The first shows that controlling for IQ can substantially change the estimated return to education, and the omitted ability bias is in the expected direction. Interestingly, education and ability do not appear to have an interactive effect. Example 9.4 is a nice example of how controlling for a previous value of the dependent variable – something that is often possible with survey and nonsurvey data – can greatly affect a policy conclusion. Computer Exercise 9.3 is also a good illustration of this method.I rarely get to teach the measurement error material, although the attenuation bias result for classical errors-in-variables is worth mentioning.The result on exogenous sample selection is easy to discuss, with more details given in Chapter 17. The effects of outliers can be illustrated using the examples. I think the infant mortality example, Example 9.10, is useful for illustrating how a single influential observation can have a large effect on the OLS estimates.With the growing importance of least absolute deviations, it makes sense to at least discuss the merits of LAD, at least in more advanced courses. Computer Exercise 9.9 is a good example to show how mean and median effects can be very different, even though there may not be “outliers” in the usual sense.CHAPTER 10TEACHING NOTESBecause of its realism and its care in stating assumptions, this chapter puts a somewhat heavier burden on the instructor and student than traditional treatments oftime series regression. Nevertheless, I think it is worth it. It is important that students learn that there are potential pitfalls inherent in using regression with time series data that are not present for cross-sectional applications. Trends, seasonality, and high persistence are ubiquitous in time series data. By this time, students should have a firm grasp of multiple regression mechanics and inference, and so you can focus on those features that make time series applications different fromcross-sectional ones.I think it is useful to discuss static and finite distributed lag models at the same time, as these at least have a shot at satisfying the Gauss-Markov assumptions.Many interesting examples have distributed lag dynamics. In discussing the time series versions of the CLM assumptions, I rely mostly on intuition. The notion of strict exogeneity is easy to discuss in terms of feedback. It is also pretty apparent that, in many applications, there are likely to be some explanatory variables that are not strictly exogenous. What the student should know is that, to conclude that OLS is unbiased – as opposed to consistent – we need to assume a very strong form of exogeneity of the regressors. Chapter 11 shows that only contemporaneous exogeneity is needed for consistency.Although the text is careful in stating the assumptions, in class, after discussing strict exogeneity, I leave the conditioning on X implicit, especially when I discuss the no serial correlation assumption. As this is a new assumption I spend some time on it. (I also discuss why we did not need it for random sampling.)Once the unbiasedness of OLS, the Gauss-Markov theorem, and the sampling distributions under the classical linear model assumptions have been covered – which can be done rather quickly – I focus on applications. Fortunately, the students already know about logarithms and dummy variables. I treat index numbers in this chapter because they arise in many time series examples.A novel feature of the text is the discussion of how to compute goodness-of-fit measures with a trending or seasonal dependent variable. While detrending or deseasonalizing y is hardly perfect (and does not work with integrated processes), it is better than simply reporting the very high R-squareds that often come with time series regressions with trending variables.CHAPTER 11TEACHING NOTESMuch of the material in this chapter is usually postponed, or not covered at all, in an introductory course. However, as Chapter 10 indicates, the set of time series applications that satisfy all of the classical linear model assumptions might be very small. In my experience, spurious time series regressions are the hallmark of many student projects that use time series data. Therefore, students need to be alerted to the dangers of using highly persistent processes in time series regression equations. (Spurious regression problem and the notion of cointegration are covered in detail in Chapter 18.)It is fairly easy to heuristically describe the difference between a weakly dependent process and an integrated process. Using the MA(1) and the stable AR(1) examples is usually sufficient.When the data are weakly dependent and the explanatory variables are contemporaneously exogenous, OLS is consistent. This result has many applications, including the stable AR(1) regression model. When we add the appropriate homoskedasticity and no serial correlation assumptions, the usual test statistics are asymptotically valid.The random walk process is a good example of a unit root (highly persistent) process. In a one-semester course, the issue comes down to whether or not to first difference the data before specifying the linear model. While unit root tests are covered in Chapter 18, just computing the first-order autocorrelation is often sufficient, perhaps after detrending. The examples in Section 11.3 illustrate how different first-difference results can be from estimating equations in levels.Section 11.4 is novel in an introductory text, and simply points out that, if a model is dynamically complete in a well-defined sense, it should not have serial correlation. Therefore, we need not worry about serial correlation when, say, we test the efficient market hypothesis. Section 11.5 further investigates the homoskedasticity assumption, and, in a time series context, emphasizes that what is contained in the explanatory variables determines what kind of heteroskedasticity is ruled out by the usual OLS inference. These two sections could be skipped without loss of continuity.CHAPTER 12TEACHING NOTESMost of this chapter deals with serial correlation, but it also explicitly considers heteroskedasticity in time series regressions. The first section allows a review of what assumptions were needed to obtain both finite sample and asymptotic results. Just as with heteroskedasticity, serial correlation itself does not invalidate R-squared. In fact, if the data are stationary and weakly dependent, R-squared and adjustedR-squared consistently estimate the population R-squared (which is well-defined under stationarity).Equation (12.4) is useful for explaining why the usual OLS standard errors are not generally valid with AR(1) serial correlation. It also provides a good starting point for discussing serial correlation-robust standard errors in Section 12.5. The subsection on serial correlation with lagged dependent variables is included to debunk the myth that OLS is always inconsistent with lagged dependent variables and serial correlation. I do not teach it to undergraduates, but I do to master’s students.Section 12.2 is somewhat untraditional in that it begins with an asymptotic t test for AR(1) serial correlation (under strict exogeneity of the regressors). It may seem heretical not to give the Durbin-Watson statistic its usual prominence, but I do believe the DW test is less useful than the t test. With nonstrictly exogenous regressors I cover only the regression form of Durbin’s test, as the h statistic is asymptotically equivalent and not always computable.。
计量经济学-ch_02_wooldridge_5e_ppt
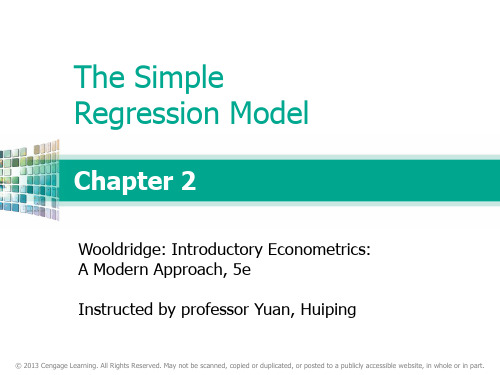
Chapter 2 The Simple Regression Model
2.1 Definition of the Simple Regression Model (6/6)
f(y|x)
y = b0 + b1x + u
E u x = 0
E(y|x3) E(y|x2) E(y|x1)
y, E(y|x)
This means that the average value of the dependent variable can be expressed as a linear function of the explanatory variable Chapter End © 2013 Cengage Learning. All Rights Reserved. May not be scanned, copied or duplicated, or posted to a publicly accessible website, in whole or in part.
Chapter 2 The Simple Regression Model
2.2 Deriving the Ordinary Least Squares Estimates (2/10)
Fit as good as possible a regression line through the data points:
Chapter 2 The Simple Regression Model
2.2 Deriving the Ordinary Least Squares Estimates (1/10)
In order to estimate the regression model one needs data A random sample of observations
《计量经济学》ch_02_wooldridge_5e_ppt
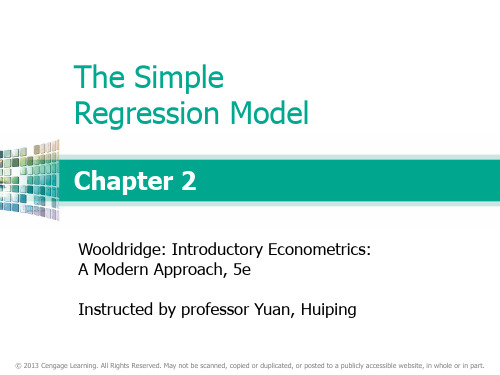
Chapter 2 The Simple Regression Model
2.1 Definition of the Simple Regression Model (3/6)
Example: Soybean yield and fertilizer
Measures the effect of fertilizer on yield, holding all other factors fixed
Example: wage equation
The explanatory variable must not contain information about the mean of the unobserved factors
e.g. intelligence …
The conditional mean independence assumption is unlikely to hold because individuals with more education will also be more intelligent on average.
For example, the i-th data point
Fitted regression line
Chapter End © 2013 Cengage Learning. All Rights Reserved. May not be scanned, copied or duplicated, or posted to a publicly accessible website, in whole or in part.
Chapter 2 The Simple Regression Model
《计量经济学》ch_01_wooldridge_5e_ppt
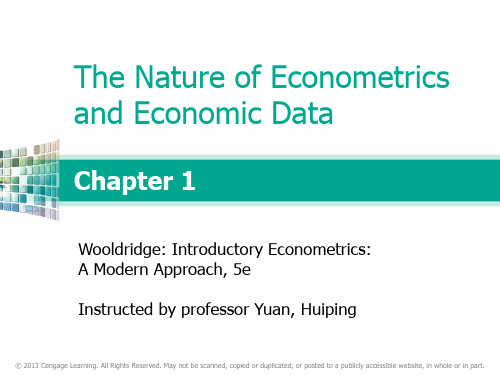
Economic models Maybe micro- or macromodels Often use optimizing behaviour, equilibrium modeling, … Establish relationships between economic variables Examples: demand equations, pricing equations, …
Chapter End © 2013 Cengage Learning. All Rights Reserved. May not be scanned, copied or duplicated, or posted to a publicly accessible website, in whole or in part.
Measure of criminal activity
Wage for legal employment
Other income
Frequency of prior arrests
Unobserved determinants of criminal activity
Frequency of conviction
Econometric methods depend on the nature of the data used Use of inappropriate methods may lead to misleading results
(完整word版)计量经济学(英文)重点知识点考试必备
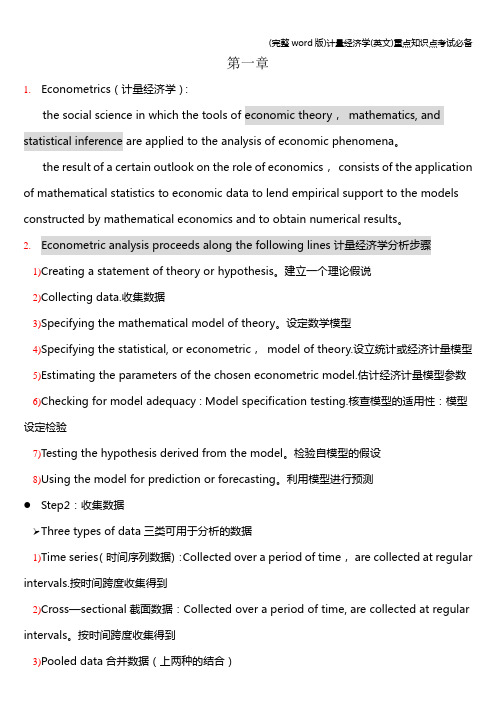
第一章1.Econometrics(计量经济学):the social science in which the tools of economic theory,mathematics, and statistical inference are applied to the analysis of economic phenomena。
the result of a certain outlook on the role of economics,consists of the application of mathematical statistics to economic data to lend empirical support to the models constructed by mathematical economics and to obtain numerical results。
2.Econometric analysis proceeds along the following lines计量经济学分析步骤1)Creating a statement of theory or hypothesis。
建立一个理论假说2)Collecting data.收集数据3)Specifying the mathematical model of theory。
设定数学模型4)Specifying the statistical, or econometric,model of theory.设立统计或经济计量模型5)Estimating the parameters of the chosen econometric model.估计经济计量模型参数6)Checking for model adequacy : Model specification testing.核查模型的适用性:模型设定检验7)Testing the hypothesis derived from the model。
伍德里奇《计量经济学导论》(第5版)笔记和课后习题详解-第15章工具变量估计与两阶段最小二乘法【圣

伍德里奇《计量经济学导论》(第5版)笔记和课后习题详解-第15章工具变量估计与两阶段最小二乘法【圣第15章工具变量估计与两阶段最小二乘法15.1复习笔记一、动机:简单回归模型中的遗漏变量1.面对可能发生的遗漏变量偏误(或无法观测异质性)的四种选择(1)忽略遗漏变量问题,承受有偏而又不一致估计量,若能把估计值与关键参数的偏误方向一同给出,则该方法便令人满意。
(2)试图为无法观测变量寻找并使用一个适宜的代理变量,该方法试图通过用代理变量取代无法观测变量来解决遗漏变量的问题,但并不是总可以找到一个好的代理。
(3)假定遗漏变量不随时间变化,运用固定效应或一阶差分方法。
(4)将无法观测变量留在误差项中,但不是用OLS 估计模型,而是运用一种承认存在遗漏变量的估计方法,工具变量法。
2.工具变量法简单回归模型01y x uββ=++其中x 与u 相关:()Cov 0,x u ≠(1)为了在x 和u 相关时得到0β和1β的一致估计量,需要有一个可观测到的变量z,z 满足两个假定:①z 与u 不相关,即Cov(z,u)=0;②z 与x 相关,即Cov(z,x)≠0。
满足这两个条件,则z 称为x 的工具变量,简称为x 的工具。
z 满足①式称为工具外生性条件,工具外生性意味着,z 应当对y 无偏效应(一旦x 和u 中的遗漏变量被控制),也不应当与其他影响y 的无法观测因素相关。
z 满足②式意味着z 必然与内生解释变量x 有着或正或负的关系。
这个条件被称为工具相关性。
(2)工具变量的两个要求之间的差别①Cov(z,u)是z 与无法观测误差u 的协方差,通常无法对它进行检验:在绝大多数情形中,必须借助于经济行为或反思来维持这一假定。
②给定一个来自总体的随机样本,z 与x(在总体中)相关的条件则可加以检验。
最容易的方法是估计一个x 与z 之间的简单回归。
在总体中,有01x z vππ=++从而,由于()()1Cov /ar V ,x z z π=所以式Cov(z,x)≠0中的假定当且仅当10π≠时成立。
- 1、下载文档前请自行甄别文档内容的完整性,平台不提供额外的编辑、内容补充、找答案等附加服务。
- 2、"仅部分预览"的文档,不可在线预览部分如存在完整性等问题,可反馈申请退款(可完整预览的文档不适用该条件!)。
- 3、如文档侵犯您的权益,请联系客服反馈,我们会尽快为您处理(人工客服工作时间:9:00-18:30)。
var(Pu) = E(Puu' P' ) = σ 2I GLS 估计量:
βˆ = ( X ' P' PX )−1 X ' P' PY = ( X ' Ω −1 X )−1 X ' Ω −1Y
var(βˆ ) = σ 2 ( X ' Ω −1 X )−1
σˆ 2 = (Y − Xβˆ)' Ω −1(Y − Xβˆ) /(n − k) 线性约束检验:
4. 扰动项的异方差与自相关
对模型: Y = Xβ + u
假定: u ~ N (0, Ωσ 2 )
4.1 ML 估计
L = f (u) = 2π σ −n/ 2 −n Ω −1/ 2 exp[− 1 u' (Ωσ 2 )−1u] 2
l
= − n ln(2π ) − 2
n ln σ 2 2
− 1 ln Ω 2
"
∂2l ⎤'
∂θ1∂θ k
⎥ ⎥
" % "
∂2l ⎥
∂θ 2 ∂θ # ∂ 2l
k
⎥ ⎥ ⎥ ⎥
∂θ
2 k
⎥⎦
(3) 渐进有效性
n (θˆ − θ ) →d
N (0,−E( 1 H )−1) n
(4) 不变性
如果 g(θ ) 是θ 的连续函数,则 g(θˆ) 是 g(θ ) 的 MLE。
(5)
定义 s(θ )
βˆIV
=β
+ (1 n
X ' Z (Z ' Z )−1 Z ' X )−1( 1 n
X ' Z (Z ' Z )−1 Z ' u)
p lim βˆIV = β
5.1 IV 估计的特例
如果工具变量个数与解释变量个数相等。
βˆIV = (Z ' X )−1 Z 'Y var(βˆ) = σ 2 ( X ' Z (Z ' Z )−1 Z ' X )−1
2
(Y
−
Xβ )' (Y
−
Xβ )
解析式:
∂l ∂βˆ
=
0
∂l ∂σˆ 2
=
0
估计量:
βˆ = ( X ' X )−1 X 'Y
σˆ 2 = (Y − Xβ )' (Y − Xβ ) / n
估计量的协方差矩阵:
H
=
⎢⎡− ⎢ ⎢−
X'X
σ2 X 'u
⎣ σ4
− n
X 'u ⎤
σ −
4
u
'
u
⎥ ⎥ ⎥
n (θˆ − θ ) = −H −1[ ns(θ )] − nH −1RT
2. 线性 MLE
n (θˆ − θ ) →d H −1[ ns(θ )]
n (θˆ − θ ) →d
N (0,−E( 1 H )−1) n
Y = Xβ + u 正态性假定
l
=
−
T 2
ln(2π )
−
T 2
ln σ
2
−
1 2σ
uˆR = Y − XˆβˆR
uˆUR = Y − XβˆUR
F
=
(uˆ'uˆR − uˆ'uˆUR ) / uˆ'uˆUR /(n − k )
q
~a
F(q, n
−
k)
说明:检验统计量渐进有效
H0 : Rβ = r
(Rβˆ-r)'[R
(
X uˆ'
' Ω −1 X )−1)R' ]−1 Ω −1uˆ(/ n − k)
(Rβˆ-r)
/
q
~F(q,n
−
k)
更一般型式:
u ~ N (0,V )
βˆ = ( X 'V −1 X )−1 X 'V −1Y
var(βˆ) = ( X 'V −1 X )−1
=
0
(1) 一致性
(2) 渐进正态性
θˆ ~a N (θ , Inf (θ )−1) Inf (θ ) 为信息矩阵。
Inf
(θ
)
=
E ⎢⎡⎜⎛ ⎢⎣⎝
∂l ∂θ
⎟⎞⎜⎛ ⎠⎝
∂l ∂θ
⎟⎞
'
⎤ ⎥
⎠ ⎥⎦
=
−
E
⎡ ⎢ ⎣
∂ 2l ∂θ∂θ
⎤
'
⎥ ⎦
其中:
∂l ∂θ
=
⎡ ⎢ ⎣
∂l ∂θ1
"
∂l ⎤'
2σ 4 σ 6 ⎦
⎡X'X
Inf
⎢ =⎢
σ2
⎢0 ⎣
0
⎤ ⎥
n
⎥ ⎥
2σ 2 ⎦
⎡σ 2 ( X ' X )−1 Inf −1 = ⎢
⎢0 ⎣
0⎤
2σ
2
⎥ ⎥
n⎦
注:系数和扰动项方差的估计量相互独立。
极大似然函数值:
max .l = cont − n ln(uˆ'u) 2
根据残差可以进行极大似然函数的迭代。
∂θ k
⎥ ⎦
∂
∂l ∂θ
/ ∂θ1
ቤተ መጻሕፍቲ ባይዱ
=
⎡ ∂2l
⎢ ⎣
∂θ12
"
∂2l ⎤'
∂θ1∂θ k
⎥ ⎦
海塞矩阵:
⎡ ∂2l
⎢ ⎢
∂θ12
H
=
∂ 2l ∂θ∂θ '
=
⎢ ∂2l
⎢ ⎢ ⎢ ⎢
∂θ 2 ∂θ1 # ∂ 2l
⎢⎣ ∂θk ∂θ1
∂ 2l
∂θ1∂θ 2 ∂ 2l
∂θ
2 2
#
∂ 2l
∂θ 2 ∂θ k
=
∂l ∂θ
,则 E(s)
=
0,
var(s)
=
Inf
(θ )
。
∫ ∫ " f (Y1,",Yn ,θ )dY1 " dY2 = 1
∫" ∫
∂f ∂θ
dY
=
0
E(s)
=
∫
"
∫
∂l ∂θ
fdY
=0
var(s) = E(ss' ) = −E(H ) = Inf (θ )
说明:信息矩阵与海塞矩阵的期望值:
∫
−
1 2σ 2
(Y
−
Xβ )' Ω −1(Y
−
Xβ )
解析式:
∂l ∂βˆ
=
0
∂l ∂σˆ 2
=
0
估计量:
βˆ = ( X ' Ω −1 X )−1 X ' Ω −1Y σˆ 2 = (Y − Xβˆ)' Ω −1(Y − Xβˆ) / n 4.2 GLS 估计 设非奇异矩阵:
P' P = Ω −1 PY = PXβ + Pu
"
∫
∂l ∂θ
fdY
=0
∫
"
∫
(
∂ 2l ∂θ∂θ
'
f
+ ∂l ∂θ
∂f )dY ∂θ '
=0
∂f ∂θ '
=
∂l ∂θ '
f
∫
"
∫
(
∂2l ∂θ∂θ
'
+
∂l ∂θ
∂l ) fdY ∂θ '
=0
E(ss' ) = −E(H )
MLE 的分布
s(θˆ) = 0
在 0 值邻域内进行二阶泰勒展开
s(θˆ) = s(θ ) + H (θˆ − θ ) + RT
3. LR、Wald、LM 检验统计量
线性约束: Rβ = r
其中:R 是 q × k 的常数矩阵,r 是 q ×1 的常数向量。
3.1 似然比检验
似然比: λ
=
LR LUR
大样本检验统计量:
LR = −2 ln λ = 2(lUR − lR ) = n[ln(uˆ'uˆ)R − ln(uˆ'uˆ)UR ]
LR ~a χ 2 (q)
特点:同时估计受约束和无约束模型
3.2 沃尔德检验
如果 H0 : Rβ = r 成立,对无约束估计量:
(Rβˆ − r) ~a N (0, RInf (β )−1 R' ) (多维)
[ ] (Rβˆ − r)' RInf (β )−1 R' −1(Rβˆ − r) ~a χ 2 (q)
如果约束成立,对受约束估计量:
LM = s'(θ~)Inf (θ~)s(θ~) ~a χ 2 (q) 。
s(θ )
=
⎡1 ⎢⎢σ 2
X 'u
⎢⎢⎣−
n 2σ 2
+
u'u 2σ 4
⎤ ⎥ ⎥ ⎥ ⎥⎦
,( σ~ 2
=
u~ ' u~
/
n
)
s(θ~)
=
⎡1 ⎢⎢σ 2
X 'u~⎥⎤ ⎥
⎣0
⎦
⎡σ~2 ( X ' X )−1 Inf −1 = ⎢
用σ 2 代替σˆ 2 ,渐进分布依然成立。
[ ] W
=
(Rβˆ
− r)'
R( X ' X )−1 R'