柔性蛇形机器人
关于蛇形机器人结构运动及控制的研究
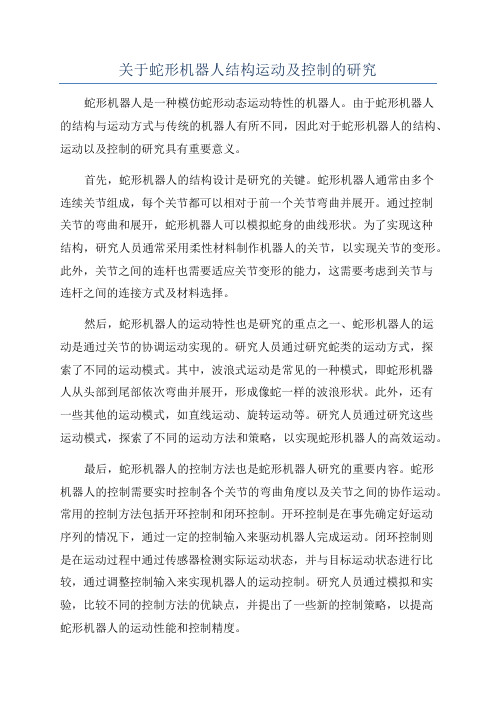
关于蛇形机器人结构运动及控制的研究蛇形机器人是一种模仿蛇形动态运动特性的机器人。
由于蛇形机器人的结构与运动方式与传统的机器人有所不同,因此对于蛇形机器人的结构、运动以及控制的研究具有重要意义。
首先,蛇形机器人的结构设计是研究的关键。
蛇形机器人通常由多个连续关节组成,每个关节都可以相对于前一个关节弯曲并展开。
通过控制关节的弯曲和展开,蛇形机器人可以模拟蛇身的曲线形状。
为了实现这种结构,研究人员通常采用柔性材料制作机器人的关节,以实现关节的变形。
此外,关节之间的连杆也需要适应关节变形的能力,这需要考虑到关节与连杆之间的连接方式及材料选择。
然后,蛇形机器人的运动特性也是研究的重点之一、蛇形机器人的运动是通过关节的协调运动实现的。
研究人员通过研究蛇类的运动方式,探索了不同的运动模式。
其中,波浪式运动是常见的一种模式,即蛇形机器人从头部到尾部依次弯曲并展开,形成像蛇一样的波浪形状。
此外,还有一些其他的运动模式,如直线运动、旋转运动等。
研究人员通过研究这些运动模式,探索了不同的运动方法和策略,以实现蛇形机器人的高效运动。
最后,蛇形机器人的控制方法也是蛇形机器人研究的重要内容。
蛇形机器人的控制需要实时控制各个关节的弯曲角度以及关节之间的协作运动。
常用的控制方法包括开环控制和闭环控制。
开环控制是在事先确定好运动序列的情况下,通过一定的控制输入来驱动机器人完成运动。
闭环控制则是在运动过程中通过传感器检测实际运动状态,并与目标运动状态进行比较,通过调整控制输入来实现机器人的运动控制。
研究人员通过模拟和实验,比较不同的控制方法的优缺点,并提出了一些新的控制策略,以提高蛇形机器人的运动性能和控制精度。
综上所述,关于蛇形机器人的结构、运动及控制的研究是一项具有重要意义的研究工作。
通过对蛇形机器人的结构、运动及控制的研究,可以为机器人的设计和应用提供一定的理论基础和实践经验,推动机器人技术的发展和应用。
同时,蛇形机器人的研究还可以为生物学、医学等领域提供一定的借鉴和启示,促进不同学科之间的跨界合作。
蛇形机器人研究综述及在核电中的应用展望

蛇形机器人研究综述及在核电中的应用展望随着科技的飞速发展,机器人已经从最初的简单机械手臂逐渐演变成了具有高度智能化和灵活性的现代工具。
其中,蛇形机器人以其独特的运动方式和适应性引起了广泛关注。
它们像蜿蜒前行的灵蛇,穿梭于狭窄或复杂的环境之中,展现出惊人的机动性和灵活性。
在众多应用领域中,核电行业因其特殊性和对安全性的高要求而成为蛇形机器人展示其才华的舞台。
核电站内部结构复杂,管道密集,常规机器人往往难以进入某些特定区域进行检修或监测。
这时,蛇形机器人的优势便凸显出来:它们能够轻松地穿过狭窄的缝隙,绕过障碍物,到达人员难以直接操作的位置。
然而,蛇形机器人的研究并非一帆风顺。
它们的设计需要考虑到如何在保持灵活性的同时提高负载能力,如何在复杂的三维空间中精确控制运动轨迹,以及如何确保在极端环境下的稳定性和可靠性。
这些问题如同悬在研究者头顶的达摩克利斯之剑,时刻提醒着他们不断探索和完善。
目前,蛇形机器人在核电领域的应用主要集中在设备巡检、故障诊断和维护等方面。
它们装备有高清摄像头和各种传感器,能够捕捉到微小的异常信号,及时发现潜在的安全隐患。
此外,一些蛇形机器人还具备简单的操作功能,可以在不中断核电站运行的情况下完成一些紧急维修任务。
展望未来,随着人工智能技术的进一步发展和材料科学的突破,我们有理由相信蛇形机器人将在核电行业中扮演更加重要的角色。
它们或许能够实现完全自主的操作,甚至与其他机器人或人类工作人员协同作业;它们的感知能力将更加敏锐,能够在第一时间内识别并响应各种复杂情况;它们的结构将更加坚固耐用,能够在高温、高压、强辐射等恶劣条件下长期稳定工作。
当然,这一切的实现都需要我们持续投入研究和创新。
我们需要更深入地理解蛇形机器人的运动机理和控制策略,开发更加高效和智能的算法来指导它们的行动;我们需要探索新型材料和制造工艺来提升它们的性能和降低成本;我们还需要加强跨学科的合作与交流,将不同领域的最新成果融合到蛇形机器人的设计和应用中去。
一种新型蛇形机器人的机构研究与设计
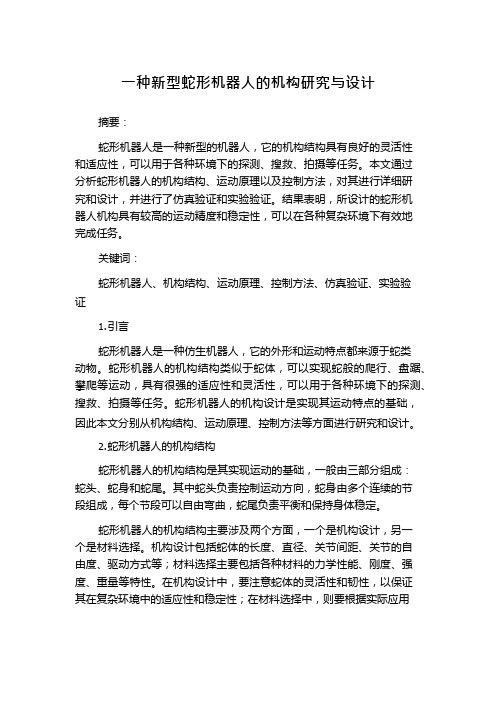
一种新型蛇形机器人的机构研究与设计摘要:蛇形机器人是一种新型的机器人,它的机构结构具有良好的灵活性和适应性,可以用于各种环境下的探测、搜救、拍摄等任务。
本文通过分析蛇形机器人的机构结构、运动原理以及控制方法,对其进行详细研究和设计,并进行了仿真验证和实验验证。
结果表明,所设计的蛇形机器人机构具有较高的运动精度和稳定性,可以在各种复杂环境下有效地完成任务。
关键词:蛇形机器人、机构结构、运动原理、控制方法、仿真验证、实验验证1.引言蛇形机器人是一种仿生机器人,它的外形和运动特点都来源于蛇类动物。
蛇形机器人的机构结构类似于蛇体,可以实现蛇般的爬行、盘踞、攀爬等运动,具有很强的适应性和灵活性,可以用于各种环境下的探测、搜救、拍摄等任务。
蛇形机器人的机构设计是实现其运动特点的基础,因此本文分别从机构结构、运动原理、控制方法等方面进行研究和设计。
2.蛇形机器人的机构结构蛇形机器人的机构结构是其实现运动的基础,一般由三部分组成:蛇头、蛇身和蛇尾。
其中蛇头负责控制运动方向,蛇身由多个连续的节段组成,每个节段可以自由弯曲,蛇尾负责平衡和保持身体稳定。
蛇形机器人的机构结构主要涉及两个方面,一个是机构设计,另一个是材料选择。
机构设计包括蛇体的长度、直径、关节间距、关节的自由度、驱动方式等;材料选择主要包括各种材料的力学性能、刚度、强度、重量等特性。
在机构设计中,要注意蛇体的灵活性和韧性,以保证其在复杂环境中的适应性和稳定性;在材料选择中,则要根据实际应用的需要进行选择,比如在高温、腐蚀等极端环境中要选择具有耐高温、耐腐蚀性能的材料。
3.蛇形机器人的运动原理蛇形机器人运动的机理来源于蛇类动物的生物学特性,主要包括以下几个方面。
(1)鳞片滑跳:蛇类动物的身体表面被覆盖着很多光滑的鳞片,在运动时可以充当滑行的媒介。
这种滑行方式可以使蛇形机器人在各种环境下灵活运动,如在水中游动、在陡峭的斜面上攀爬等。
(2)节段结构:蛇类动物的身体由多个节段构成,每个节段可以自由弯曲,因此蛇形机器人可以实现蛇般的摆动和弯曲。
自主柔性变形蛇形机器人控制系统设计

r o b o t i s d e s i g n e d b a s e d O n ARM mi c r o p r o c e s s o r S T M3 2 a s c o r e wi t h mu l t i — s e n s o r f u s i o n, a c h i e v i n g r e mo t e
( 1 . B e i j i n g Ke y L a b o r a t o r y o f H i g h D y n a mi c N a v i g a t i o n T e c h n o l o g y , U n i v e r s i t y o f B e i j i n g I n f o r ma t i o n S c i e n c e& T e c h n o l o y, g B e i j i n g 1 0 0 1 0 1 , C h i n a ;2 . S c h o o l o f C o mp u t e r a n d I n f o r ma t i o n E n g i n e e r i n g , B e i j i n g T e c h n o l o g y a n d B u s i n e s s U n i v e r s i t y , B e i j i n g 1 0 0 o 4 8 , C h i n a ;3 . S c h o o l o f A u t o ma t i o n , B e i j i n g I n s t i t u t e o f T e c h n o l o y, g B e i j i n g 1 0 0 0 8 4 , C h i n a )
微处理器 S T M3 2为核心 、 多传感器融合的 自主柔性 变形蛇形 机器人 控制 系统 , 实现 了机 器人 的远程监 控
蛇形机器人的工作原理

蛇形机器人的工作原理
蛇形机器人是一种模仿蛇类运动的机器人,它的工作原理可以分为硬件和软件两个方面:
硬件方面:
1. 结构设计:蛇形机器人的身体由一系列连续的关节单元和连接杆组成,每个关节单元都有自己的驱动器和传感器,可以自由弯曲和转动。
2. 驱动系统:每个关节单元都有一个电机或压电驱动器,用于控制关节的运动。
这些驱动器可以根据软件控制发送恰当的指令以实现不同的运动方式。
3. 传感器系统:蛇形机器人通常具有各种传感器,如陀螺仪、加速度计、压力传感器等,用于感知自身的姿态、环境条件以及与外部物体的接触等信息。
4. 电源系统:蛇形机器人需要电源来供电驱动器和其他电子设备。
软件方面:
1. 运动规划:通过计算机算法,根据机器人当前位置和目标位置,确定每个关节单元的驱动器运动方式,以生成合适的运动序列和控制指令。
2. 执行控制:将计算出的控制指令发送给各个关节的驱动器,使得机器人按照预定的运动轨迹和姿态进行运动。
3. 感知与决策:机器人通过传感器系统获取环境信息,并利用算法对这些信息进行处理和分析,根据需要作出相应的决策,调整机器人的运动方式和姿态。
根据蛇形机器人的工作原理,在软硬件的协同作用下,它可以实现各种复杂的身
体姿态和运动方式,如爬行、攀爬、游泳等,以适应不同的环境和任务需求。
蛇形机器人使用说明书
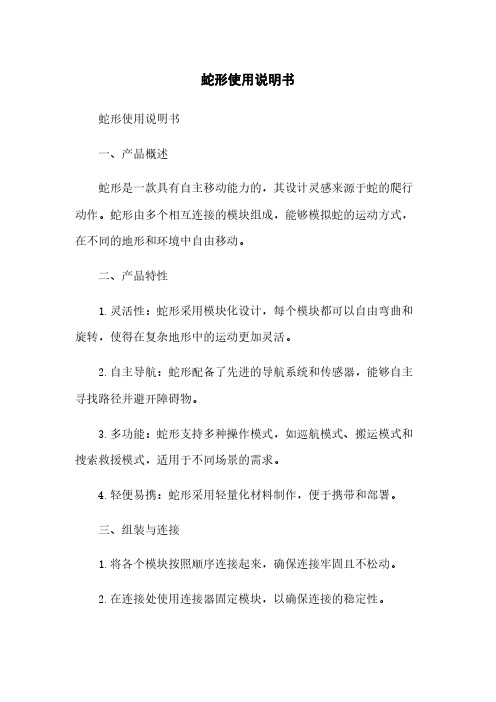
蛇形使用说明书蛇形使用说明书一、产品概述蛇形是一款具有自主移动能力的,其设计灵感来源于蛇的爬行动作。
蛇形由多个相互连接的模块组成,能够模拟蛇的运动方式,在不同的地形和环境中自由移动。
二、产品特性1.灵活性:蛇形采用模块化设计,每个模块都可以自由弯曲和旋转,使得在复杂地形中的运动更加灵活。
2.自主导航:蛇形配备了先进的导航系统和传感器,能够自主寻找路径并避开障碍物。
3.多功能:蛇形支持多种操作模式,如巡航模式、搬运模式和搜索救援模式,适用于不同场景的需求。
4.轻便易携:蛇形采用轻量化材料制作,便于携带和部署。
三、组装与连接1.将各个模块按照顺序连接起来,确保连接牢固且不松动。
2.在连接处使用连接器固定模块,以确保连接的稳定性。
3.在连接模块时,请确认每个连接器的方向和位置正确,以免造成连接错误。
4.连接完成后,进行功能测试,确保能够正常工作。
四、使用方法1.开机和关机操作:按下背部的电源开关按钮,即可启动或关闭。
2.操作模式选择:支持多种模式选择,通过面板上的模式选择按钮进行切换。
3.移动控制:使用遥控器或者智能方式APP,控制的运动方向和速度。
4.使用注意事项:在使用蛇形的过程中,需要注意周围环境,避免碰撞或损坏。
五、常见问题解答Q: 无法启动怎么办?A: 请检查的电源是否连接正常,是否有足够的电量。
Q: 移动困难怎么办?A: 请检查的连接是否牢固,模块之间是否有松动。
Q: 无法避开障碍物怎么办?A: 请检查的传感器是否正常工作,是否有物体遮挡传感器。
六、维护与保养1.定期清洁的外表面和连接器,保持的正常运行。
2.在使用中发现故障或异常情况时,及时联系售后服务中心进行维修。
附件:本文档无附件。
法律名词及注释:1.模块化设计:将产品拆分成多个相互独立的模块,使每个模块具备独立的功能和特点。
蛇形机器人的原理
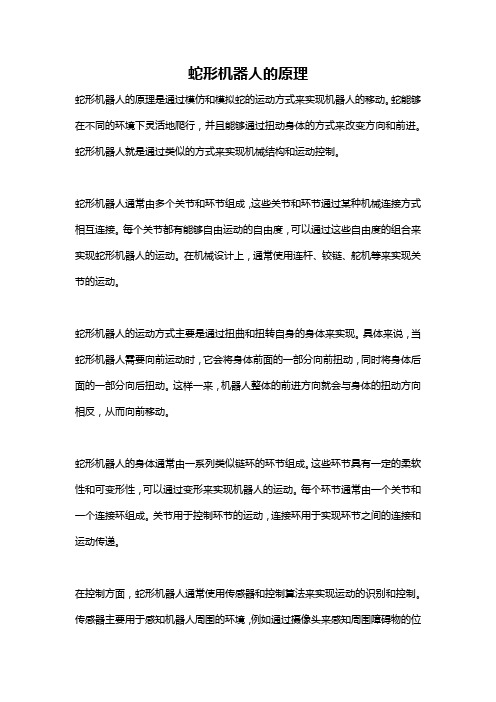
蛇形机器人的原理蛇形机器人的原理是通过模仿和模拟蛇的运动方式来实现机器人的移动。
蛇能够在不同的环境下灵活地爬行,并且能够通过扭动身体的方式来改变方向和前进。
蛇形机器人就是通过类似的方式来实现机械结构和运动控制。
蛇形机器人通常由多个关节和环节组成,这些关节和环节通过某种机械连接方式相互连接。
每个关节都有能够自由运动的自由度,可以通过这些自由度的组合来实现蛇形机器人的运动。
在机械设计上,通常使用连杆、铰链、舵机等来实现关节的运动。
蛇形机器人的运动方式主要是通过扭曲和扭转自身的身体来实现。
具体来说,当蛇形机器人需要向前运动时,它会将身体前面的一部分向前扭动,同时将身体后面的一部分向后扭动。
这样一来,机器人整体的前进方向就会与身体的扭动方向相反,从而向前移动。
蛇形机器人的身体通常由一系列类似链环的环节组成。
这些环节具有一定的柔软性和可变形性,可以通过变形来实现机器人的运动。
每个环节通常由一个关节和一个连接环组成。
关节用于控制环节的运动,连接环用于实现环节之间的连接和运动传递。
在控制方面,蛇形机器人通常使用传感器和控制算法来实现运动的识别和控制。
传感器主要用于感知机器人周围的环境,例如通过摄像头来感知周围障碍物的位置和距离。
控制算法则负责根据传感器的数据来计算机器人的运动轨迹和关节的运动方式。
在运动控制方面,蛇形机器人的目标是通过对每个关节的运动控制来实现机器人整体的运动。
通常,每个关节都由一个电机或舵机驱动,通过改变电机或舵机的转动角度来实现关节的运动。
控制算法根据机器人的运动目标和当前环境的信息,计算每个关节应该运动的角度和方向,然后发送控制信号给相应的电机或舵机。
总结起来,蛇形机器人的原理是通过模仿和模拟蛇的运动方式来实现机器人的移动。
它由多个关节和环节组成,通过某种机械连接方式相互连接,并且通过扭曲和扭转身体来实现运动。
蛇形机器人通过传感器和控制算法来感知环境和控制运动,以实现机器人整体的运动和导航。
一种仿生蛇形机器人的结构设计
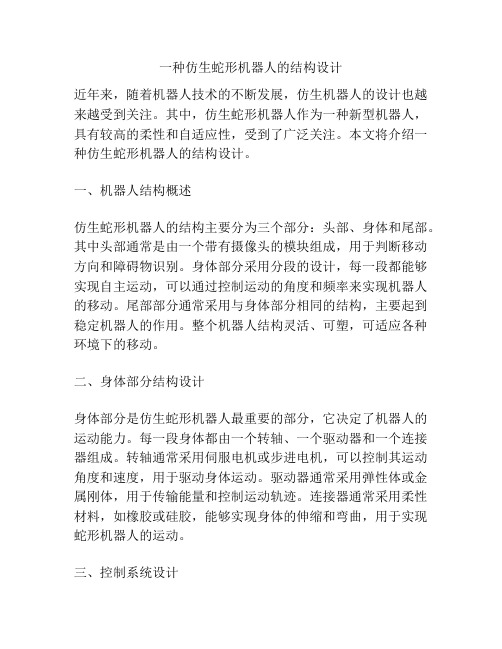
一种仿生蛇形机器人的结构设计近年来,随着机器人技术的不断发展,仿生机器人的设计也越来越受到关注。
其中,仿生蛇形机器人作为一种新型机器人,具有较高的柔性和自适应性,受到了广泛关注。
本文将介绍一种仿生蛇形机器人的结构设计。
一、机器人结构概述仿生蛇形机器人的结构主要分为三个部分:头部、身体和尾部。
其中头部通常是由一个带有摄像头的模块组成,用于判断移动方向和障碍物识别。
身体部分采用分段的设计,每一段都能够实现自主运动,可以通过控制运动的角度和频率来实现机器人的移动。
尾部部分通常采用与身体部分相同的结构,主要起到稳定机器人的作用。
整个机器人结构灵活、可塑,可适应各种环境下的移动。
二、身体部分结构设计身体部分是仿生蛇形机器人最重要的部分,它决定了机器人的运动能力。
每一段身体都由一个转轴、一个驱动器和一个连接器组成。
转轴通常采用伺服电机或步进电机,可以控制其运动角度和速度,用于驱动身体运动。
驱动器通常采用弹性体或金属刚体,用于传输能量和控制运动轨迹。
连接器通常采用柔性材料,如橡胶或硅胶,能够实现身体的伸缩和弯曲,用于实现蛇形机器人的运动。
三、控制系统设计仿生蛇形机器人的控制系统包括硬件和软件两部分。
硬件方面,需要运用传感器、电机控制器、信号处理器等设备。
具体来说,需要安装陀螺仪、加速器、振动器等传感器,用于检测机器人的角度、速度和加速度。
电机控制器负责驱动电机,控制机器人的运动。
信号处理器用于处理控制指令和传感器数据,控制机器人的移动。
在软件方面,主要有机器人控制程序和运动学算法两部分。
控制程序负责接收用户指令,解析传感器数据,并将控制命令转换成电机控制信号。
运动学算法主要用于定位机器人的位置、速度和运动轨迹。
四、应用场景仿生蛇形机器人可应用于海底探测、医疗器械、安防巡逻、排爆等领域。
例如,用于水下探测可以采用防水材料,实现机器人在水中的自由运动。
医疗器械方面,仿生蛇形机器人可以用于手术,实现微创手术、准确治疗等。
基于多模态CPG模型的蛇形机器人仿生控制研究的开题报告
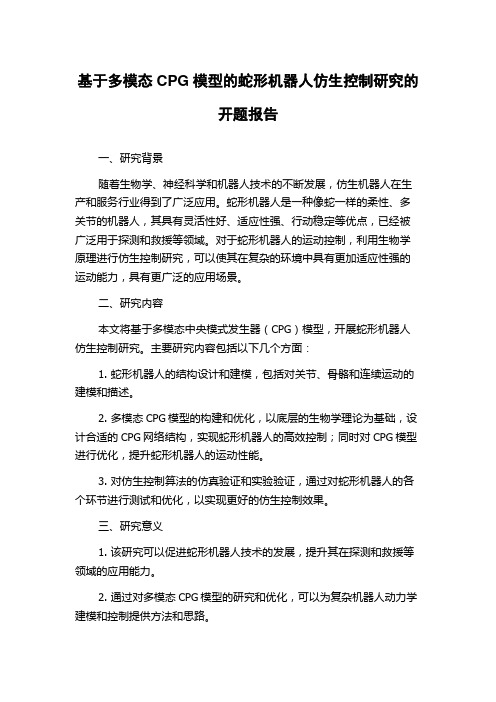
基于多模态CPG模型的蛇形机器人仿生控制研究的开题报告一、研究背景随着生物学、神经科学和机器人技术的不断发展,仿生机器人在生产和服务行业得到了广泛应用。
蛇形机器人是一种像蛇一样的柔性、多关节的机器人,其具有灵活性好、适应性强、行动稳定等优点,已经被广泛用于探测和救援等领域。
对于蛇形机器人的运动控制,利用生物学原理进行仿生控制研究,可以使其在复杂的环境中具有更加适应性强的运动能力,具有更广泛的应用场景。
二、研究内容本文将基于多模态中央模式发生器(CPG)模型,开展蛇形机器人仿生控制研究。
主要研究内容包括以下几个方面:1. 蛇形机器人的结构设计和建模,包括对关节、骨骼和连续运动的建模和描述。
2. 多模态CPG模型的构建和优化,以底层的生物学理论为基础,设计合适的CPG网络结构,实现蛇形机器人的高效控制;同时对CPG模型进行优化,提升蛇形机器人的运动性能。
3. 对仿生控制算法的仿真验证和实验验证,通过对蛇形机器人的各个环节进行测试和优化,以实现更好的仿生控制效果。
三、研究意义1. 该研究可以促进蛇形机器人技术的发展,提升其在探测和救援等领域的应用能力。
2. 通过对多模态CPG模型的研究和优化,可以为复杂机器人动力学建模和控制提供方法和思路。
3. 该研究对于探索生物学运动控制原理,并揭示其普适性和应用价值,也具有一定的理论意义。
四、研究方法本研究将采用多模态CPG模型,通过对蛇形机器人的关节、骨骼和动力学特征进行建模,确定适合的神经网络结构,构建并优化CPG模型。
根据仿真角度和实验角度的不同,本研究将应用不同的研究方法,包括仿真模拟分析、控制参数优化、实验验证和运动学分析等方法。
五、预期结果与成果通过本研究将获得以下预期结果:1. 提出一种基于多模态CPG模型的蛇形机器人仿生控制算法,适用于不同复杂环境下的机器人运动控制。
2. 构建了蛇形机器人的神经网络结构和动力学模型,并对CPG模型进行优化和改进,提升机器人的运动控制能力。
2.2、蛇形机器人结构简介
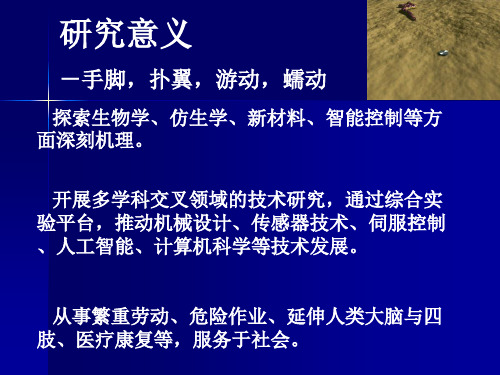
蛇形机器人运动示意图
平面蜿蜒运动 平面蜿蜒运动是通过控制关节模块水平轴的关节保持直线,而垂直轴的关节呈正弦变化产生;采用的算法公式为:
(b)侧向运动 侧向运动是控制关节模块的垂直轴(yaw轴)的关节和水平轴(pitch轴)的关节都呈正弦变化,两个曲线之间有一个相位差;采用的算法公式为:
定义
发展趋势 仿生 多样 应用
日本京都大学研制的 蛇形搜救机器人“莫伊拉”
仿生机构
日本东京工业大学研制的 废墟搜索机器人
(1) 蛇形机器人机构研究
生物蛇研究
蛇的肌肉骨骼 简化模型
蛇的肌肉骨骼 结构
生物蛇
蛇形机器人单自由度关节模块
关节模块设计
相邻关节模块连接示意图
(3)分布式控制系统和微型嵌入式控制器的研究
蛇形机器人原理样机控制系系统结构
主控单元
执行 单元
CAN总线
执行 单元
执行 单元
执行 单元
执行 单元
执行 单元
监控系统
机器人 控制系统
(b)分布式控制系统结构
已应用在: 拟人机器人 星球探测机器人
蛇形机器人机构的构成
(2)蛇形机器人运动控制方法研究 蛇可以做多种动作来适应它所处的环境,但大体上可分为四种步态: 蜿蜒运动 直线运动 伸缩运动 侧向运动
蜿蜒运动
生物蛇的蜿蜒运动
直线运动
生物蛇的直线运动
伸缩运动
生物蛇的伸缩运动
侧向运动
(c)微型嵌入式控制器的研究
特点:模块化 体积小 功能全 功耗低 现场可编程
(4)地面特征识别技术的研究
传感器
压杆
传感装置
关节模块
灵活的软体蛇形机器人
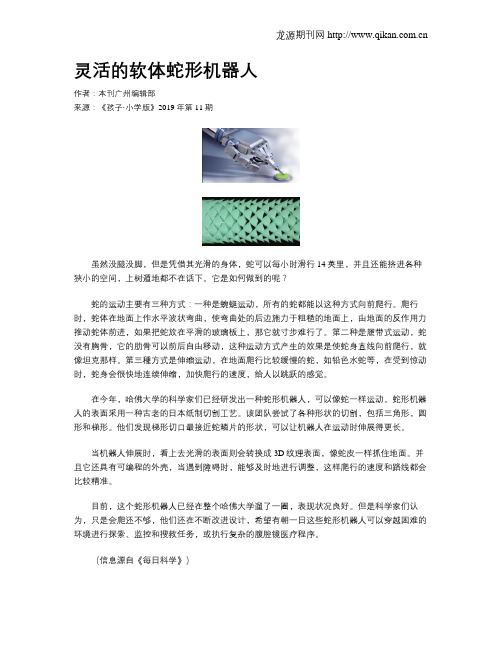
龙源期刊网
灵活的软体蛇形机器人
作者:本刊广州编辑部
来源:《孩子·小学版》2019年第11期
虽然没腿没脚,但是凭借其光滑的身体,蛇可以每小时滑行14英里,并且还能挤进各种狭小的空间,上树遁地都不在话下。
它是如何做到的呢?
蛇的运动主要有三种方式:一种是蜿蜒运动,所有的蛇都能以这种方式向前爬行。
爬行时,蛇体在地面上作水平波状弯曲,使弯曲处的后边施力于粗糙的地面上,由地面的反作用力推动蛇体前进,如果把蛇放在平滑的玻璃板上,那它就寸步难行了。
第二种是履带式运动,蛇没有胸骨,它的肋骨可以前后自由移动,这种运动方式产生的效果是使蛇身直线向前爬行,就像坦克那样。
第三種方式是伸缩运动,在地面爬行比较缓慢的蛇,如铅色水蛇等,在受到惊动时,蛇身会很快地连续伸缩,加快爬行的速度,给人以跳跃的感觉。
在今年,哈佛大学的科学家们已经研发出一种蛇形机器人,可以像蛇一样运动。
蛇形机器人的表面采用一种古老的日本纸制切割工艺。
该团队尝试了各种形状的切割,包括三角形,圆形和梯形。
他们发现梯形切口最接近蛇鳞片的形状,可以让机器人在运动时伸展得更长。
当机器人伸展时,看上去光滑的表面则会转换成3D纹理表面,像蛇皮一样抓住地面。
并且它还具有可编程的外壳,当遇到障碍时,能够及时地进行调整,这样爬行的速度和路线都会比较精准。
目前,这个蛇形机器人已经在整个哈佛大学遛了一圈,表现状况良好。
但是科学家们认为,只是会爬还不够,他们还在不断改进设计,希望有朝一日这些蛇形机器人可以穿越困难的环境进行探索、监控和搜救任务,或执行复杂的腹腔镜医疗程序。
(信息源自《每日科学》)。
蛇形机器人研究现状
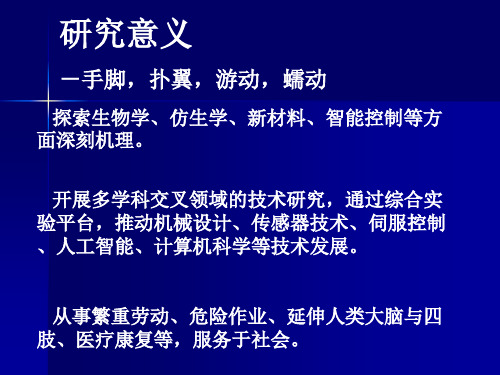
结束语
主要介绍了微小型仿生技术与机器人基 本内涵、研究意义、关键技术、发展趋势。
嵌入式芯片、微机电系统、新材料等方 面发展给微小型仿生技术研究带来了机遇。
智能仿生机器人是人类科学研究追求目 标,开展这方面研究不仅促进多学科发展, 而且广泛应用服务于社会。
谢谢大家
关键技术-挑战性研究 方向
• 理论方法问题,仿生静态与动态模型,复杂模型计算方法,基于 传感器变结构方法,往往是非线性、非定常问题,…… 多自由度灵巧机构,运动学与动力学,冗余与柔性,高效驱动装 置,…… 感知与模式识别技术,内部姿态,外部环境,距离、力觉、触觉 等,特别是视觉问题,…… 嵌入式控制技术:微小型硬件平台,高可靠性软件系统,高性能 实时学习技术,基于传感器的群体行为智能控制技术,…… 人机交互技术,通讯,时延,临境与虚拟现实,…… 微小型机电系统设计、加工、装配,…… 微、仿、多、遥、网
主要功能
该样机长1米左右,每分钟可爬行15.6 米,能做前进、后退、转弯、变速等动作
模块化、可重组机器人
主要功能
能组成蛇形构形
蛇形机器人
主要功能
可在普通路面、草 地等环境中爬行
蛇形机器人
主要功能
穿上“蛇皮”后还可在水中自由游动
模块化、可重组机器人
国内外机器蛇设计特点
美国宇航局(NASA) NASA于1999年开始 研究多关节的蛇形机器人
➢发展趋势 仿生 多样 应用
仿生机构
日本京都大学研制的 蛇形搜救机器人“莫伊拉”
日本东京工业大学研制的 废墟搜索机器人
北航 SOLIDSNAKE 蛇形机器人
概述
近几年来,仿生机器人学正 在机器人领域占有越来越重 要的位置。对于障碍物众多、 凸凹不平、以及狭窄地形等 环境,类似蛇形的机器人有 较大的运动优势,可以满足 多种用途。
仿生蛇说明书

目录一、简介..........................................................................................................错误!未定义书签。
二、设计初衷 (2)三、创新点分析................................................................................................错误!未定义书签。
四、方案设计....................................................................................................错误!未定义书签。
1、构件图: (4)2、主要元件的图片、功能表: (4)3、实现过程: (6)五、结构设计 (6)1、底盘机构: (6)2、升降机构 (7)3、生命探测系统 (7)4、整体结构 (7)六、程序设计 (8)1、总程序概览 (8)2、避障 (9)3、搜救 (10)七、实用可行性 (10)八、成本分析 (11)九、应用前景 (11)一、简介图一作品整体图本蛇形机器人的存在作用是用于在地震等灾害后,进入狭小空间搜寻幸存者。
本机器人使用履带驱动,携带生命检测装置,定位装置,遥控装置以及双重避障系统。
本机器人较为小的体型以及多节化的设计,可以让机器人进入许多常规机器人难以进入的环境,头部带有的举升装置可以有效提高机器人的通过性能。
生命检查装置发现幸存者后会发送定位信号给搜救人员。
自带的气囊及螺旋桨则可以保证机器人在遇到深水区无法通行时也能顺利通过。
具体操作为救援人员可以通过遥控来控制机器人进入狭小空间搜救,在遇到障碍物时,较小的可以通过举升装置来通过,生命检查装置发现幸存者后会自动上传定位信号,当遥控信号遇到干扰时,系统会开启自动避障功能,继续进行搜救任务。
- 1、下载文档前请自行甄别文档内容的完整性,平台不提供额外的编辑、内容补充、找答案等附加服务。
- 2、"仅部分预览"的文档,不可在线预览部分如存在完整性等问题,可反馈申请退款(可完整预览的文档不适用该条件!)。
- 3、如文档侵犯您的权益,请联系客服反馈,我们会尽快为您处理(人工客服工作时间:9:00-18:30)。
REACTIVE MOTION PLANNING: A MULTIAGENT APPROACHLARS OVERGAARD, HENRIK G. PETERSEN, andJOHN W. PERRAMLindù Center for Applied Mathematics, OdenseUniversity, DenmarkWe present an efficient approach to reactive robot motion planning and collision avoidance.Unlike the traditional methods, there is no centralized control; instead the links and the joints of the robot are autonomous agents. This is a completely new approach. A set of dynamic equa-tions of motion for an arbitrary robot is derived. Artificial forces are introduced to express and combine multiple, possibly conflicting objectives, such as avoiding obstacles while approach-ing a goal. The joint agents impose forces of constraint between the link agents, and these forces establish a flow of information among the agents. The emergent behavior of the multi-agent system gives an impression of surprisingly intelligent overall control. The developed method is used in actual industrial applications to control welding robot installations for ship building with up to 11 degrees of freedom (DOF). Experimental results from the simulation of a 25-DOF snakelike robot operating in a complex three-dimensional structure are given. It isdemonstrated that the time complexity is O(n 3) for branched n-DOF robots, while for serialrobots such as standard manipulators and the snake, it is O(n).Robot motion planning is the problem of finding a collision-free path for a robot with internal degrees of freedom in an environment with obstacles. It is a key component in autonom ous and intelligent robot systems, where robots are instructed in which problem to solve, rather than how to solve it.Motion planning has received much attention in the last 20 years by very different approaches. Some of the most important achievements are as follows.·theoretical work on the piano movers’ problem by Schwartz and Sharir (1983)·Donald’s (1987) complete algorithm for the full 6 DOF discretized movers’problem·path planning in the robot’s configuration space (C space), suggested by Lozano-Per…z (1981, 1983, 1987)·Brooks’ (1983a, 1983b ) development of Freeways and C space techniques ·the concept of artificial potentials presented by Krogh (1984) and Khatib (1986)·Barraquand and Latombe’s (1991) work on potential functions in a hierarchicalgrid representation of the robot work spaceApplied Artificial Intelligence, 10:35±51, 1996Copyright 1996 Taylor & Francis0883-9514/96 $10.00 + .0035Lars Overgaard was supported by the Danish Research Academy and Odense Steel Shipyard Ltd.Address correspondence to Lars Overgaard, Lindù Center for Applied Mathematics, Odense University,DK-5230 Odense M, Denmark.36 L. Overgaard et al.This article presents an approach to robot motion planning inspired by the artificial potential idea and the methods for obtaining dynamic equations of motion for mechanical systems presented by Perram and Petersen (1988a) and de Leeuw et al. (1990). Artificial potential methods use artificial forces to produce the motion of a dynamic model of the robot. The forces are chosen such that they encourage the robot to do what it is supposed to do and discourages it from doing what it should not do. Kearney (1992) combined the multiple objectives of prey and predator agents, such as fear, hunger, and thirst, in a similar way. The total sum of the forces is a comprom ise of all the various, sometimes conflicting, objectives of the robot. For example, the avoidance of one obstacle might bring the robot closer to another obstacle.One of the most important advantages of the artificial potential approach over the more traditional path planning methods is that it allows reactive robot behavior toward, e.g., unexpected events in the environm ent or other robots.The basic idea of our method is to distribute control of the robot to a set of agents. Each agent is part of a constrained dynam ical system. An agent controls a subpart of the robot, and an apparently intelligent motion emerges from the agents’s rather simple attempts to selfish utility optimization. Using this distribution scheme, a very general method is obtained. That is, the complicated control of an articulated robot is reduced to a much simpler control of each subpart, and when applying the method to robots with different topologies of the parts, the only change needed is to set up the proper communication scheme among the agents.Thus our method can easily be applied to a wide range of problem s, including all combinations of off-line robot program ming, robot systems with uncertainty, on-line control of sensor-based robots, highly redundant robots, multitool robots, and multirobot systems. For simplicity, however, we concentrate on a single robot in a static well-known three-dimensional environment.The presented algorithm is incomplete. That is, it may fail to find a solution to a motion planning problem, even if a solution exists. Nevertheless, numerous experiments and actual industrial application have shown that such failures are very rare.The following section describes the multiagent model we implement to achieve reactive robot motion planning. We then describe the various agents in the multi-agent system in detail and we finally report some interesting results of applying our method to various problems.The work described in this article is part of the autonomous multiple robot operation in structured environm ents (AMROSE) project, a joint research project between Odense University and Odense Steel Shipyard Ltd. The purpose of this project is to make a system able to control multilink robots of different types working together in a complex environm ent. A more detailed description of AMROSE is found in the works by Jacobsen et al. (1990) and Larsen et al. (1991).Reactive Motion Planning 37 MULTIAGENT MOTION PLANNINGIn this section we specify the exact properties that we require from the motion planning method. The required properties lead us to identify the agents needed for the robot motion planning.Requirements to Motion PlanningThere are several requirements when planning the collision-free motion of an articulated robot. We will consider four basic requirements. It is required that the robot does not collide with objects in the environm ent and that the robot’s joints stay inside their legal range. These requirements are expressed more form ally by the following collision avoidance inequality constraints:1. The minimum distance between any link of the robot and any object in the environment must always be greater than zero.2. The value of any joint coordinate must always stay within its mechanical limits.In addition to avoiding collisions, the robot must perform some task. This is expressed through the following requirements to the end effector:3. It must reach a location at the beginning of the task.4. It must perform a specific high-precision motion from that location.We focus on these four basic requirements, although the presented m ethod can handle many other useful requirem ents. These include avoidance of collisions between different links in the same robot or between multiple robots, occlusion avoidance in vision-based robot systems, and limits on joint velocities and accelerations.The four requirements are constraints on the motion of the robot and must be taken into account during motion planning. Examples of distributed artificial intel-ligence and multiagent system approaches to constraint satisfaction in planning problem s are found in the works by Nagao and Hasida (1993) and Liu and Sycara (1993). For many problems, however, approaches outside the dom ain of traditional symbolic reasoning AI give good results. This article presents an example. Identifying the AgentsThe requirements described in the previous section lead us to view an articulated robot as a multiagent system. We identify four types of agents for the robot (see Figure 1):1. Each individual link of the robot is a self-contained link agent L with the aim of avoiding collisions with the environment.2. The aim of the joint agents J is to connect one link agent to another link agent (or to a task agent; agent type 4 below) and to avoid joint limit violations.3. End effector agents are special link agents L* with additional aims related to task perform ance.4. The high-precision task perform ance is taken care of by task agents T.This choice is a one-to-one reflection of the requirements stated in the previous section. Link agents seek to satisfy requirement 1, while joint agents take care of requirem ent 2. The end effector agents’ additional aims are to reach a location at the beginning of the task (requirement 3), and task agents force end effectors to perform high-precision motion (requirement 4). Notice that the link agents have an actual embodiment, while the joint and task agents are nonphy sical.Path FindingThe agents only respond to their local neighborhood. Therefore this method alone will not solve mazelike path finding problems of global character. We thus rely on assistance from a simple method that makes a kind of rough path findingFigure 1. The agents that control the robot are link agents L, joint agents J , and task agents T.The link agents have a physical representation; the other agents are artificial. The link and joint agents involved in task execution are marked with an asterisk.38 L. Overgaard etal.Reactive Motion Planning 39 and decomposes the global problem into a sequence of smaller motion planning problem s of local character.This ªpath finderº may be viewed as yet another agent with a global but rough view of the environm ent. The role of the path finder is to identify key points on the way to the goal and to communicate these key points to the end effector agent. Our method for local motion planning produc es the actual collision-free motion from one key point to the next.We will, however, not discuss the path finder in this article but will simply assume that it provide s the end effector agent with the necessary inform ation. More details about the path finder are given by Jacobsen et al. (1992).AGENT TYPESIn this section we describe the various types of agents in the robot motion planning method. This is the first presentation ever of this agent-based problem decomposition.Link AgentA link agent is responsible for avoiding collisions between one of the robot’s links and possible obstacles in its neighborhood. The link agent is the only type of agent that has a physical representation.Relating to the WorldThe agent must relate the link to the obstacles in the environment. In a computer simulation, the agent perform s the necessary geometric calculations based on a geometric (NURBS or polyhedron) model of the environm ent and the robot link. The closest points of the link and the environment are calculated, and the agent applies repulsive forces to the link acting in that point. The repulsive force expresses the link agent’s intention to avoid the closest obstacle point.According to Brooks (1991, p. 583), however, ªThe world is its own best model.º In systems with the proper sensors, the world model can be omitted. If the sensors provide inform ation about the required closest points, the environment model is not necessary.Choosing the Coordinate RepresentationTraditionally, a robot’s configuration is given in C space, where each dimension represents a joint coordinate. The structure of the obstacles and the description of the robot’s task, however, are given in three-dimensional Cartesian space. In order to relate the robot to the obstacles, it is necessary to either transform the robot’s configuration into Cartesian coordinates, or to transform the obstacles into C obstacles. Transformations of this kind are the main computational burden of many motion planning methods.We choose to represent the configuration of the i th link agent L i in Cartesian space by a vector r i , giving the position of the center of mass, and the three principal axis vectors u i 1, u i 2, u i 3. In this way, we remove the need for transformations to and from C space.ConstraintsThe 12 Cartesian coordinates in [r i , u i 1, u i 2, u i 3] are, of course, a redundant representation of the link’s 6 DOF. But principal axis vectors must be orthogonal unit vectors, and the redundancy is removed by the 6 constraints of orthonormality {presented by Perram and Petersen (1988b)}:u ie u if ± ef = 0 1 e f 3where ef is the Kronecker delta function.The constraints cause constraint forces to act on the dynamical system. A classic example of a system with constraint forces is the pendulum. The constraint is the constant length of the string, and the constraint force is the string force. The constraint force acts along the string and always has exactly the size necessary to keep the pendulum on a sphere with the center in the pendulum’s point of support.Likewise, each constraint on the link agents’ coordina tes causes constraint forces in the direction of the constraint.Equation of MotionFor the i th link agent L i , let q i = [r i , u i 1, u i 2, u i 3] be the vector of all the agent’s coordinates. The total force acting on the agent’s coordinates f, is the sum of theagent’s own intention forces f i and the constraint forces f c . These constraint forcesare found by solving a system of linear equations. The detailed procedure for this is described by de Leeuw et al. (1990).From the forces, we obtain the Newtonian equations of motion for the con-strained link agent coordinates:q .. = M ±1 (f i + f c )(1)where M is the diagonal mass matrix and q ..is the acceleration vector.Numerical integration of all the equations of motion will give us the motion of the multiagent system. Standard methods for num erical integration of differential equations can be found in the work by Press et al. (1988).Joint AgentThe responsibility of the joint agent is to ensure that its two link agents move as if they are connected by a mechanical joint and to ensure that the links do not violate the mechanical joint limits.40 L. Overgaard et al.The joint connection can be described by a set of geom etric constraints similar to the constraints described in the previous section. Without constraints, the two link agents have 6 DOF relative to each other. The joint agent limits this freedom to the 1 DOF of a revolute or prismatic joint by imposing five independent geometric constraints that relate [r i , u ie ] and [r j , u je ], the coordinates of the two joined agents.The constraints remove the redundancy of the Cartesian description of the system.The geometric constraints used to obtain a revolute joint are demands for constant distances between pairs of points fixed in the two links. If the points are chosen as shown in Figure 2, the only remaining freedom is rotation about the axis through the points p ij,0 and p ji,0. Let d ij,m be the distance between the two points p ij,m and p ji,0. Then the five constraints can be expressed asd ij,m ± l ij,m = 0 m = 0, 1, 2(2)d ij,m ± l ji,m = 0 m = 1, 2where the l ij,m are constants. This description of revolute joints has been given by Perram and Petersen (1988a).Something similar is done to obtain a prismatic joint. Now the five distances between the points must not be constant; we demand them to be equal. These four constraints leave one rotational and one translational degree of freedom. The rotation is prevented by demanding orthogonality of two vectors, one fixed in each link agent,as seen from Figure 3:Figure 2. The revolute joint agent J ij connects the link agents L i and L j by five distance constraints.Reactive Motion Planning41d ij,m ± d ij,0 = 0 m = 1, 2d ji,m ± d ij,0 = 0 m = 1, 2v ij v ji = 0Note that d ij,0 is not constant. The above constraints for prismatic joints were originally proposed by Overgaard (1991).Other types of joints are easily achieved. For example, Eq. (2) alone is a 3-DOF ball joint. On Figure 2 this corresponds to removing the two lines from p ji,1 and p ji,2to p ij,0.The link agents provide the joint agent with information about their positions,velocities, and intentions (forces). The joint agent then eventually adds an artificial joint limit repulsive force to the link agents’ forces. Furthermore, by communicating with other joint agents, the joint agents are able to find the proper forces due to the joint constraints. The constraint forces are also added to the link agents’ forces; they ensure that the joint constraints are exactly satisfied. Thus the flow of information communi-cated among the agents enables one agent to react on the intentions of any other agent in the multiagent system, e.g., repulsion on one link may cause all links to move.Summarizing, the role of a joint agent is to influence the intentions of its link agents in such a way that joint constraints and joint limits are not violated.Figure 3. A prismatic joint agent J ij connects the link agents L i and L j by five different geomet-ric constraints.42 L. Overgaard etal.Reactive Motion Planning 43End Effector AgentThe end effector is a link agent with additional aims. It has two different modes of behavior. During transfer movement between tasks, the end effector agent is aimed at reaching the starting point of the next task. To achieve this aim, the agent adds an attractive force directed toward the starting point.During task execution, the agent is a normal link agent without additional aims.A joint agent connects the end effector to a task agent, which guides the end effector along the task (see below).Task AgentThe task of welding, and most other tasks, requires high precision, more than can be achieved by using artificial forces. We obtain the required high-precision motion by introducing an imaginary task agent. The task agent is a moving frame of reference without any physical representation. The role of the task agent is to guide the end effector (which is passive during task execution) to execute the task.At the beginning of the task, a joint agent attaches the end effector to the task agent. W hen the task agent moves along the curve prescribed by the task specifica-tion, the joint agent forces the end effector to come along. The joint agent only imposes the constraints necessary for the specific task; the remaining freedom is left for the end effector agent.The process of welding norm ally requires control of only 5 out of the end effector’s 6 DOF; there is rotational symmetry about the torch. Therefore the end effector agent and the task agent are joined by a revolute joint about the torch.Details of the methods used to move the task agent can be found in the work by Larsen et al. (1994).EXPERIMENTAL RESULTSIn all applications, we place a level of centralized control on top of the described method. This level of control may be seen as a controller, giving input to the multiagent system. The controller assigns new tasks to end effector agents when they are ready for it. This approach has been shown to work well in several different cases.Welding ITo demonstrate the multiagent properties of our method as clearly as possible, we first show the very unusua l robot movem ent in the absence of the joint agents that norm ally connect the link agents. The link agents detect each other’s presence and react on it, but there is no other communication among the agents. Their actions44 L. Overgaard et al.are completely uncoordinated. The system’s behavior is governe d purely by the links’ selfish utility optimization.We consider a simple case, where a 5-DOF Hirobo WR-L80 robot, attached to a 3-DOF Cartesian gantry, welds two seams in a ship section. We include gravity in the artificial forces, so that all link agents tend to fall down toward the steel plates of the ship section. The link agents will try to avoid collisions with the steel plates. Furthermore, in this section’s experiment, the link agents avoid each other. Two weld tasks are assigned to the end effector.Figure 4 shows snapshots from the result of a num erical simulation of the multiagent system. The pictures show various stages of the scenery:1. The robot is in its initial configuration, and all velocities are zero.2. The robot disintegrates because of the link agents’ mutual repulsion. Because of gravity, the agents fall down toward the steel construction. The end effector falls faster than the other agents because of its additional attraction toward the corner.3. The end effector is close to the start of the first seam. At about the same time, the link agents stop falling because of their repulsion away from the steel plates.4. The end effector is attached to the task agent by a task joint agent, and the end effector starts to weld. The other agents float at safe distances from the steel plates and each other.5. The end effector has been released from the task agent and is now attracted toward another task starting in the opposite corner. During this process, a link agent is pushed away. In turn, this link agent pushes away other link agents.6. Finally, the end effector starts welding the second seam while being joined to the task agent. The link agents are repelled further away, to make more room for the end effector.This clearly demonstrates the agents’ autonomy.Welding IIIn this section, we do exactly the same as in the previous section with one extension only. We include the presence of the joint agents that connect neighboring links. The joint agents convey a flow of inform ation through the multiagent system that was absent in the previous experim ent.As before, the end effector must weld the two seams starting in the corners. The gantry has 3 DOF and the robot has 5, so the gantry/robot system is in fact an 8-DOF robot. The task agent controls 5 of the end effector’s DOF, so the system is kinematically redundant. Thus it has the freedom to avoid collisions, not only during the transfer movement between tasks but also when welding.The effect of the joint agents is clearly demonstrated by the snapshots from the simulation presented in Figure 5:Figure 4. Link agents move freely to perform their individual tasks. They all avoid collisions,and the end effector agent moves to two weld tasks. During welding, a task joint agent con-nects the end effector to the task agent.Reactive Motion Planning45Figure 5. Joint agents connect the link agents. The agents’ configuration space is now reduced to the one of the physical robot. The weld tasks are the same as in Figure 2.46 L. Overgaard etal.Reactive Motion Planning 471. The robot is in the same initial configuration as previously.2. The end effector is attracted toward the corner, and all the other agents have to follow it because of the constraint forces imposed by the joint agents.3. Now the end effector has pulled the robot close to the corner where the vertical seam begins. The comprom ise between attraction and repulsion yields a collision-free path.4. A snapshot of the welding process. The collision-free path to this configu-ration has never been planned but has simply emerged.5. After finishing the first task, the end effector is released and attracted toward the start of the next task in the other corner. The end effector and thus the other agents are repelled from the steel plates on the way to the other corner.6. The end effector has started to weld the horizontal seam. The task agent controls the process but not the end effector’s rotation about the torch.A comparison of Figure 4 and Figure 5 clearly shows the effect of the joint agents on the multiagent system.Snake SimulationWe have simulated a 25-DOF snakelike robot moving through a simple maze, using exactly the same method as in the two previous sections. The snake’s nose was the (active) end effector agent, while the other (passive) link agents were pulled through the maze without collisions. Figure 6 illustrates the result of the simulation. The time complexity of the simulation is given in the following section.A branched 25-DOF robot with two end effectors has also been simulated. Our method was immediately applicable. The only change was that the controller now had to coordinate the actions of the two end effector agents.Chirikjian and Burdick (1993) report on the actual construction of a 30-DOF planar snake robot and the method applied for obstacle avoidance. Computational C omplexityWe shall in this section find the complexities in the number of agents. Our approach has two main consum ers of computing time. One is the link agent’s computation for finding the closest obstacle. This cost is proportional to the num ber of agents in the robot. In situations where the link agents avoid each other, the cost of closest obstacle computation is quadratic in the number of agents.The other time consumer is the solution of linear equations for the constraint forces f c in Eq. (1). The matrix is irregular but sparse. Solving sets of m linear equations in general has the time complexity O(m3). The equation matrix for robots with a single chain of links is block tridiagonal, so the time complexity for a linear robot with n joints reduces from O(n3) to O(n). Figure 7 shows the total computational cost in ourFigure 6. A 25-DOF snakelike robot is maneuvering in a complex three-dimensional environment.48 L. Overgaard etal.simulations of snakes with different num bers of links. Notice that it would have been much more expensive to transform the snake’s environment (Figure 6) into C obstacles in the 25-dimensional C space and subsequently search for a feasible path.Thus the time complexity for robots with n joints varies between O (n ) for robotswith a serial chain of links to O (n 3) for very complex multitool robots, the worst casetopology.Emergent Intelligence?As stated by Brady (1985, p. 79), ªSince Robotics is the field concerned with the connection of perception to action, Artificial Intelligence must have a central role in Robotics if the connection is to be intelligent.º Although not quite up to date,Brady (1985) gives a very good review of AI and robotics.The apparently intelligent behavior of the robot in the presented experiments was achieved purely by a simple artificial force strategy. It looks as if the robot’s intelligence has appeared out of nothing. However, according to Brooks (1991,p. 585), ªIntelligence is in the eye of the observer.º The seat of intelligence is hard to identify, as the impression of intelligence is produce d by the interactions of all components, including the environment.In a recent paper, Werner (1994) presents a (rather inform al) theory called the ªcomplexity conservation principle.º Without actually defining the notion of com -plexity, Werner argues that a complex goal can only be achieved if either the agents or their environment has a complexity of matching stature.Thus, if our robot is intelligent, this intelligence is contained in the force strategy,in the structure of the robot and the environment, and in the inform ation defining the task.Figure 7. The time complexity is O (n ) for a serial robot with n joints.Reactive Motion Planning4950 L. Overgaard et al.CONC LUSIONThe multiagent model allows us to solve the motion planning problem as a distributed constraint satisfaction problem, where each agent is responsible for the satisfaction of its own partition of the constraints. It enables us to replace the traditional high-level planning approach by a low-level method with distributed local reactive control.Our approach is an artificial force method, where each agent expresses its intentions through repulsive, attractive, and constraint forces. Summarizing, the key characteristics of the presented method are as follows:Flexibility: Any robot can be controlled by this m ethod. There is no fundamental limit on the number of joints in the robot, in contrast to traditional meth-ods. The role of the individual agent is unchanged if, for example, the application is changed from one robot to another with a completely different topology.Efficiency: The obtained motion is not planned; instead it emerges because of the forces. No reasoning about future states is necessary, and thus immediate response is possible in nonstatic environments like sensor-based systems, systems with moving obstacles, and multirobot systems. The method is highly efficient for robots with many (n) joints, since the time complexity is as low as O(n) for the best case and O(n3) for the worst case. This is to be compared with the nonpolynom ial complexity of most traditional methods.Elegance: The distributed control gives rise to a very smooth and elegant movement of the articulated robot. All joints in the (possibly highly redundant) robot move simultaneously, and due to the dynamic model of the robot, there are no sudden accelerations or other discontinuities in the movement. This quality has an aesthetic value and also a practical one in the motion planning for high-speed robots.We have successfully applied the method to off-line robot progra mming at Odense Steel Shipyard Ltd. Since spring 1995, the system has been used to program 9-DOF welding robot installations in actual daily production. Later that year, a 11-DOF welding system was successfully tested. The backbone of the system is the motion planning technique described in this paper.The system is currently being extended to cover robot spray painting. The only changes are the description of the tasks and the behavior of the task agents. All other components of the system are general enough to be reused without changes. The next step is to investigate the effect of sensor feedback. Some very prom ising experiments in grasping moving objects have been perform ed. In such an on-line application, the fast response to changes provided by our reactived method is crucial.In future applications we will exploit the method’s obvious advantages in on-line control of multilink robots and multirobot systems.。