Stochastic language generation for spoken dialogue systems
社会语言学变异研究经典文献 读书笔记
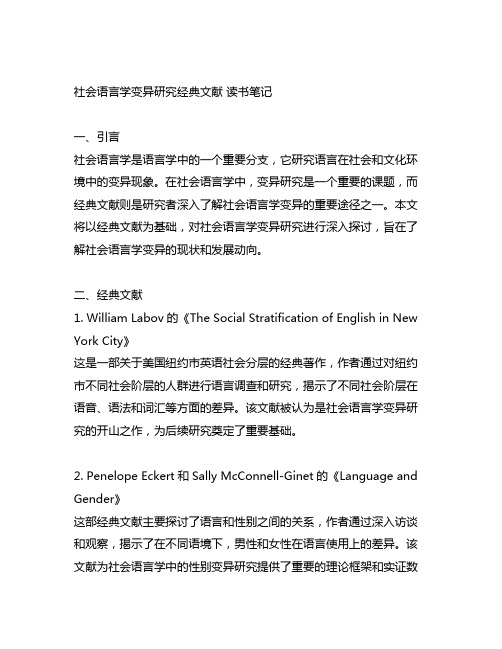
社会语言学变异研究经典文献读书笔记一、引言社会语言学是语言学中的一个重要分支,它研究语言在社会和文化环境中的变异现象。
在社会语言学中,变异研究是一个重要的课题,而经典文献则是研究者深入了解社会语言学变异的重要途径之一。
本文将以经典文献为基础,对社会语言学变异研究进行深入探讨,旨在了解社会语言学变异的现状和发展动向。
二、经典文献1. William Labov的《The Social Stratification of English in New York City》这是一部关于美国纽约市英语社会分层的经典著作,作者通过对纽约市不同社会阶层的人群进行语言调查和研究,揭示了不同社会阶层在语音、语法和词汇等方面的差异。
该文献被认为是社会语言学变异研究的开山之作,为后续研究奠定了重要基础。
2. Penelope Eckert和Sally McConnell-Ginet的《Language and Gender》这部经典文献主要探讨了语言和性别之间的关系,作者通过深入访谈和观察,揭示了在不同语境下,男性和女性在语言使用上的差异。
该文献为社会语言学中的性别变异研究提供了重要的理论框架和实证数据。
3. John Baugh的《Linguistic Profiling as a Challenge to the Assumptions of the Critical Period Hypothesis》这篇文章主要探讨了语言在种族和社会地位方面的变异。
作者通过对不同种族和社会地位的人群进行语言调查和研究,揭示了在不同社会语境下语言的歧视和分化现象。
该文献为社会语言学变异研究提供了新的视角和理论支持。
三、研究内容了解社会语言学变异的经典文献对于当前社会语言学变异研究具有重要意义。
通过分析经典文献可以深入了解社会语言学变异的理论基础、研究方法和实证数据,有助于我们对社会语言学变异现象的本质和特点有更加全面和深入的认识。
社会语言学的基本概念
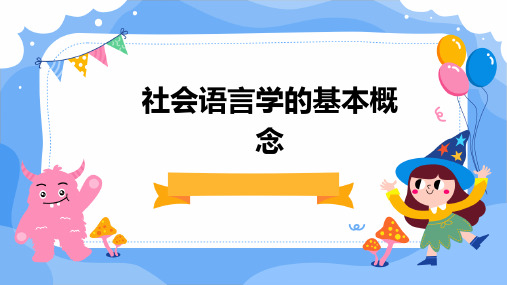
社会语言学为语言教育课程设置和教 材编写提供理论指导,确保教学内容 的实用性和针对性。
教师培训与教学评估
社会语言学有助于教师培训和教学评 估,提高教师的教学水平和评估能力 。
06
社会语言学的未来展望
语言与人工智能的结合
自然语言处理
利用人工智能技术,实现机器对人类语言的自动识别、理解和生 成,提高人机交互的效率和准确性。
语言规划实施
社会语言学关注语言规划的实施过程, 分析实施效果,提出改进建议,促进 语言规划的可持续发展。
跨文化交际
文化差异识别
社会语言学帮助人们识别不同文化间的语言 差异,促进跨文化交流的顺利进行。
交际能力培养
社会语言学强调培养交际能力,包括语言使 用得体、文化适应等,提高跨文化交际的效 果。
语言教育
语言与身份认同研究
关注个体和群体如何通过语言 表达和建构身份认同。
语言与文化研究
研究语言与文化之间的相互关 系,包括文化传承、文化交流 和文化冲突等方面。
语言政策与规划研究
关注政府和机构如何制定和实 施语言政策,以及这些政策对
社会的影响。
03
社会语言学的主要研究内 容
社会语境中的语言使用
语言使用与社会环境
社会语言学的起源与当时的社会文化背景密切 相关,随着社会的发展和人口迁移的增加,语 言接触和变异现象引起了学者们的关注。
社会语言学的起源也与语言学、社会学、人类 学等学科的交叉研究有关,这些学科的理论和 方法为社会语言学的发展提供了基础。
发展历程
20世纪60年代
社会语言学兴起,研究重点在于语言变异和 语言接触。
语言变化研究
社会语言学关注语言随时间和社会变迁而产生的变化,包括语音、词 汇、语法等方面的变化,探究这些变化的动因和规律。
社会语言学引论-概述说明以及解释
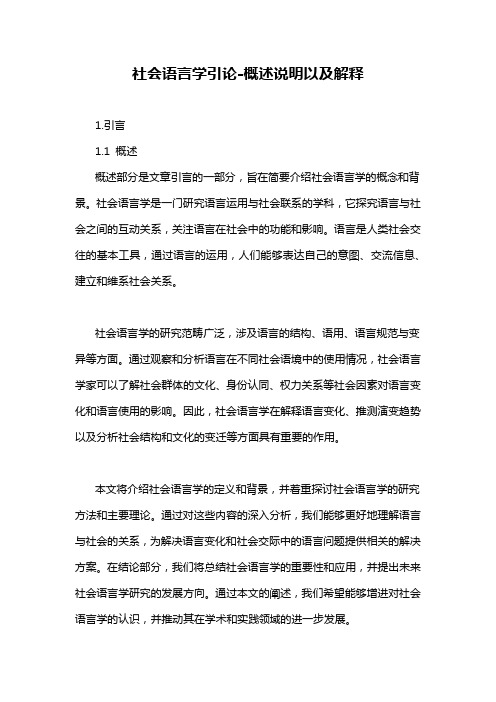
社会语言学引论-概述说明以及解释1.引言1.1 概述概述部分是文章引言的一部分,旨在简要介绍社会语言学的概念和背景。
社会语言学是一门研究语言运用与社会联系的学科,它探究语言与社会之间的互动关系,关注语言在社会中的功能和影响。
语言是人类社会交往的基本工具,通过语言的运用,人们能够表达自己的意图、交流信息、建立和维系社会关系。
社会语言学的研究范畴广泛,涉及语言的结构、语用、语言规范与变异等方面。
通过观察和分析语言在不同社会语境中的使用情况,社会语言学家可以了解社会群体的文化、身份认同、权力关系等社会因素对语言变化和语言使用的影响。
因此,社会语言学在解释语言变化、推测演变趋势以及分析社会结构和文化的变迁等方面具有重要的作用。
本文将介绍社会语言学的定义和背景,并着重探讨社会语言学的研究方法和主要理论。
通过对这些内容的深入分析,我们能够更好地理解语言与社会的关系,为解决语言变化和社会交际中的语言问题提供相关的解决方案。
在结论部分,我们将总结社会语言学的重要性和应用,并提出未来社会语言学研究的发展方向。
通过本文的阐述,我们希望能够增进对社会语言学的认识,并推动其在学术和实践领域的进一步发展。
1.2 文章结构文章结构部分的内容如下:文章结构:本文将按照以下结构进行论述。
首先,在引言部分,将对社会语言学进行概述,介绍文章的结构和目的。
然后,在正文部分,将详细讨论社会语言学的定义和背景,社会语言学的研究方法以及社会语言学的主要理论。
最后,在结论部分,对社会语言学的重要性和应用进行总结,并提出未来社会语言学研究的发展方向。
最后,给出一个简短的结束语。
通过以上结构,将全面介绍社会语言学的相关内容。
首先,引言部分概述了本文的主题,同时也引导读者对社会语言学产生兴趣。
其次,正文部分分为三个小节,分别解释了社会语言学的定义和背景、研究方法以及主要理论,从不同的角度深入探讨社会语言学的内涵。
最后,结论部分对整篇文章进行总结,强调了社会语言学的重要性和应用,并提出了未来的研究方向,为读者提供了思考和进一步研究的方向。
语言顺应论的发展历史

语言顺应论的发展历史语言顺应论(Linguistic Accommodation Theory)是一种社会语言学理论,通过研究社会与心理的因素,解释人们在交流中为了获得互动效果,而调整他们的语言使用方式。
其发展历史可以追溯到20世纪初期的语言间关系(Language Contact)与社会语言学(Sociolinguistics)。
20世纪30年代,美国社会学家西奥多·新科姆(Theodore Newcomb)开始研究在跨文化交流中,语言以及文化的影响。
他认为语言是人群之间确定身份,交流知识,和建立人际关系的重要因素。
同时,他认为语言不仅是交流信息的工具,也是身份,归属感和文化认同的一种表现形式。
20世纪50年代至60年代,社会语言学取得了重大突破。
美国社会学家威廉·拉波夫(William Labov)对美国纽约的语言现象进行研究,发现了社会因素对语言使用的影响,如地区、家庭背景、职业、年龄等因素。
拉波夫强调了语言在社会中的作用,认为语言能够反映出社会现象,并能够成为社会现象的因素之一。
20世纪60年代至70年代,语言顺应论的概念被提出。
美国社会学家霍华德·加尔菲尔德(Howard Giles)认为,语言顺应是一种情境因素的调整和符合他人要求的一种策略。
即人们在交流中,为了获得交流的效果,会调整自己的语言使用方式。
此外,加尔菲尔德还提出了交叉路口(Intersection)理论,指出交流是互动的过程,其强度和影响因素取决于文化背景,性别,年龄和社会地位等因素。
20世纪80年代至90年代,语言顺应论的研究得到了进一步的发展。
加尔菲尔德与他的研究小组研究了不同文化背景下的语言使用情况,并提出了多元一体性(Divergent-In-Convergent)理论,认为多种文化背景的语言使用在某些方面是分离的,但在某些方面又是相似的。
同时,他还提出了社会标记(Social Markers)理论,指出社会标记是语言顺应识别的营养基质,是语言顺应的整合设计。
语言学流派
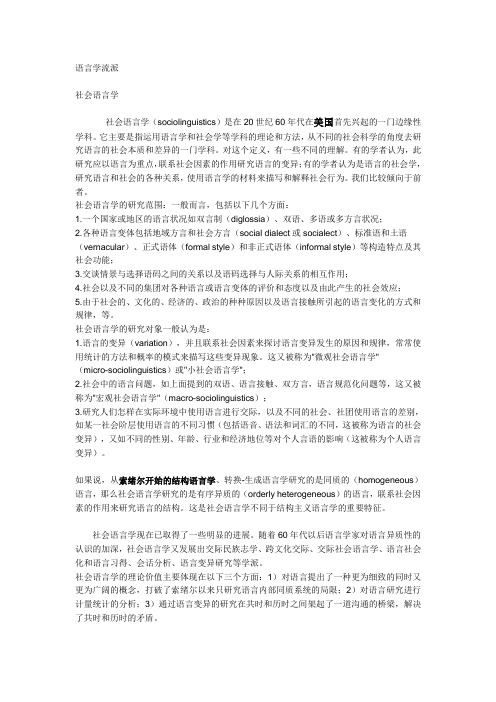
语言学流派社会语言学社会语言学(sociolinguistics)是在20世纪60年代在美国首先兴起的一门边缘性学科。
它主要是指运用语言学和社会学等学科的理论和方法,从不同的社会科学的角度去研究语言的社会本质和差异的一门学科。
对这个定义,有一些不同的理解。
有的学者认为,此研究应以语言为重点,联系社会因素的作用研究语言的变异;有的学者认为是语言的社会学,研究语言和社会的各种关系,使用语言学的材料来描写和解释社会行为。
我们比较倾向于前者。
社会语言学的研究范围:一般而言,包括以下几个方面:1.一个国家或地区的语言状况如双言制(diglossia)、双语、多语或多方言状况;2.各种语言变体包括地域方言和社会方言(social dialect或socialect)、标准语和土语(vernacular)、正式语体(formal style)和非正式语体(informal style)等构造特点及其社会功能;3.交谈情景与选择语码之间的关系以及语码选择与人际关系的相互作用;4.社会以及不同的集团对各种语言或语言变体的评价和态度以及由此产生的社会效应;5.由于社会的、文化的、经济的、政治的种种原因以及语言接触所引起的语言变化的方式和规律,等。
社会语言学的研究对象一般认为是:1.语言的变异(variation),并且联系社会因素来探讨语言变异发生的原因和规律,常常使用统计的方法和概率的模式来描写这些变异现象。
这又被称为"微观社会语言学"(micro-sociolinguistics)或"小社会语言学";2.社会中的语言问题,如上面提到的双语、语言接触、双方言,语言规范化问题等,这又被称为"宏观社会语言学"(macro-sociolinguistics);3.研究人们怎样在实际环境中使用语言进行交际,以及不同的社会、社团使用语言的差别,如某一社会阶层使用语言的不同习惯(包括语音、语法和词汇的不同,这被称为语言的社会变异),又如不同的性别、年龄、行业和经济地位等对个人言语的影响(这被称为个人语言变异)。
语言相对论的产生和发展
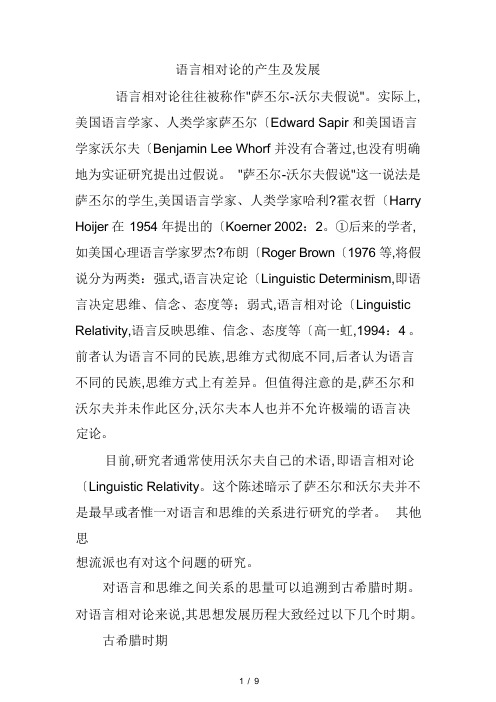
语言相对论的产生及发展语言相对论往往被称作"萨丕尔-沃尔夫假说"。
实际上, 美国语言学家、人类学家萨丕尔〔Edward Sapir 和美国语言学家沃尔夫〔Benjamin Lee Whorf 并没有合著过,也没有明确地为实证研究提出过假说。
"萨丕尔-沃尔夫假说"这一说法是萨丕尔的学生,美国语言学家、人类学家哈利?霍衣哲〔Harry Hoijer 在1954 年提出的〔Koerner 2002:2。
①后来的学者, 如美国心理语言学家罗杰?布朗〔Roger Brown〔1976 等,将假说分为两类:强式,语言决定论〔Linguistic Determinism,即语言决定思维、信念、态度等;弱式,语言相对论〔Linguistic Relativity,语言反映思维、信念、态度等〔高一虹,1994:4 。
前者认为语言不同的民族,思维方式彻底不同,后者认为语言不同的民族,思维方式上有差异。
但值得注意的是,萨丕尔和沃尔夫并未作此区分,沃尔夫本人也并不允许极端的语言决定论。
目前,研究者通常使用沃尔夫自己的术语, 即语言相对论〔Linguistic Relativity。
这个陈述暗示了萨丕尔和沃尔夫并不是最早或者惟一对语言和思维的关系进行研究的学者。
其他思想流派也有对这个问题的研究。
对语言和思维之间关系的思量可以追溯到古希腊时期。
对语言相对论来说,其思想发展历程大致经过以下几个时期。
古希腊时期古希腊哲学家柏拉图认为,世界存在于预设的外部理念, 语言若要存在下去,就必须竭力正确地反映这些理念。
"除了我们把思维准确地称作由心灵与它自身进行的无声的对话之外,思维和言谈是一回事。
""从心中发出通过嘴唇流出来的声音之流称作言谈。
"②持该种观点的人认为,语言的暗地里是普遍的理性本质,为天下人共有,至少为所有思想家共有。
词语无非是这种深层精华的表达媒介,语言是反映内在思想活动的"标签",是体验世界的工具,还没有考虑到语言对思想的作用。
社会语言学的兴起

拉波夫主张把语言放到社会中去研究
拉波夫是一个深入现实的社会语言学家。 1)拉波夫用语言变异的研究成果向美
国社会宣布,黑人英语并不是一种低级 的、残缺的语言。 2)拉波夫曾经三次以社会语言学家的 身份出庭为被告辩护。
2、甘柏兹
2002年9月,互动社会语言学奠基人甘柏兹 应邀参加在北京语言文化大学召开的“首届 社会语言学国际学术研讨会”,并作专题发 言。 互动社会语言学就是“交际社会语言学” 。
4、总的倾向
语言不可能存在于真空中。越来越多的语言 学家希望为语言学的理论研究找到更多经验 性的实实在在的证据,而不是从一个理论假 设出发去寻找抽象的普遍语法或是语言习得 机制,他们反对脱离社会环境来研究语言。 大家相信,影响语言使用的诸多社会因素如 阶级出身、家庭背景、社会地位、受教育程 度、年龄、性别、职业等,应该成为语言学 研究的内容,通过这种研究可以进一步解释 语言的本质和规律,加深对语言的认识,从 而找到语言学理论的坚实基础。
社会语言学的兴起
学科的诞生 外部的原因 内部的原因 语言研究的价值取向 面向社会的语言学
一、学科的诞生
1、术语的提出
2、学科的独立
3、研究的重点
二、学科兴起的外部原因
1、社会历史问题
2、社会现实问题
3、科技发展推动
三、学科兴起的内部原因
1、概述
2、“约定俗称”论
1、社会历史问题
急需解决的问题,比如:在世界范围内,新 独立国家官方语言或通用语言的确立问题, 民族划分中的语言识别问题,社会发展和文 化教育中的语言使用问题等,这一系列与语 言的实际使用情况密切相关的社会需求,成 为催生社会语言学的主要外部条件。
西方哲学的发展对英语词汇扩充的影响
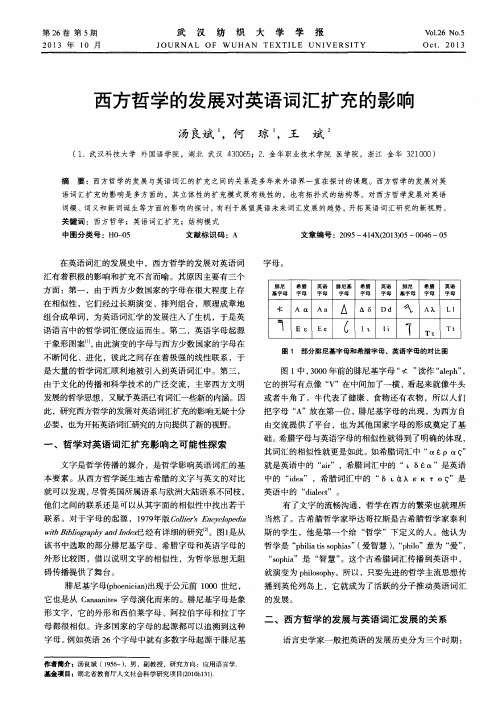
有 了文字 的流畅 沟通 ,哲学 在 西方 的繁 荣也 就理 所 当 然 了。古 希腊 哲 学 家毕 达哥 拉斯 是 古希 腊 哲学 家 泰利 斯 的学 生 ,他 是第 一个 给 “ 哲 学 ”下 定义 的人 。他认 为 哲学 是 “ p h i l i a t i s s o p h i a s ” ( 爱智 慧 ) ,“ p h i l o ”意为 “ 爱” ,
汤 良斌 ,何 琼 ,王 斌
( 1 .武 汉科技大 学 外国语学院 ,湖北 武汉 4 3 0 0 6 5 ;2 .金华职业技术学 院 医学院,浙江 金华 3 2 1 0 0 0 )
摘
要 :西方哲 学的发展 与英语词 汇的扩充之 问的关系是 多年来 外语界 一直在探讨 的课题。西方哲学的发展对 英
“
外形 比较 图,借以说明文字的相似性 ,为哲学思想无阻
s o p h i a ”是 “ 智慧” 。这个古希腊词汇传播到英语 中,
碍传播提供了舞台。
腓 尼基 字母 ( p h o e n i c i a n ) h  ̄ 现于 公元 前 1 0 0 0世 纪 ,
就 演变 为 p h i l o s o p h y 。所 以 , 只要 先进 的哲 学 主流思 想传
播到英伦列 岛上 ,它就成为了活跃的分子推动英语词汇
的发展 。
它也是从 C a n a a n i t e s字母演化而来的。腓尼基字母是象 形文字 ,它的外形和西伯莱字母 、阿拉伯字母和拉丁字 母都很相似。许多国家的字母的起源都可以追溯到这种
字母 。 例 如英 语 2 6 个 字母 中就有 多数 字母 起源 于腓 尼基
作者简介 :汤良斌 ( 1 9 5 6 一) ,男,副教授,研究方向:应用语言学 基 金 项 目 :湖 北省 教 育厅人 文社 会科 学研 究项 1  ̄( 2 0 1 0 b 1 3 1 )
语言顺应论的发展历史
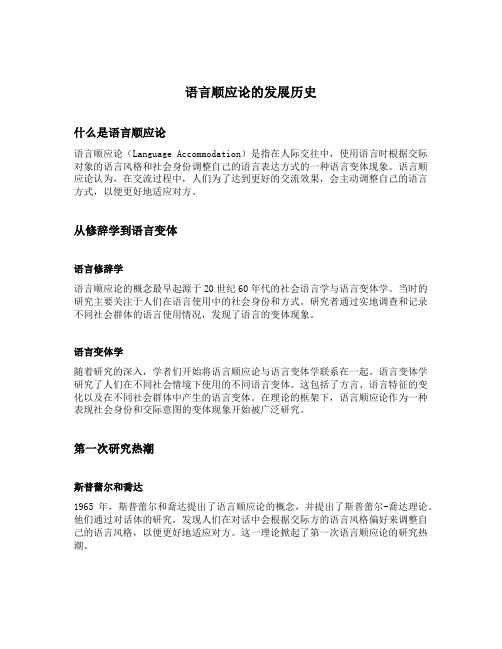
语言顺应论的发展历史什么是语言顺应论语言顺应论(Language Accommodation)是指在人际交往中,使用语言时根据交际对象的语言风格和社会身份调整自己的语言表达方式的一种语言变体现象。
语言顺应论认为,在交流过程中,人们为了达到更好的交流效果,会主动调整自己的语言方式,以便更好地适应对方。
从修辞学到语言变体语言修辞学语言顺应论的概念最早起源于20世纪60年代的社会语言学与语言变体学。
当时的研究主要关注于人们在语言使用中的社会身份和方式。
研究者通过实地调查和记录不同社会群体的语言使用情况,发现了语言的变体现象。
语言变体学随着研究的深入,学者们开始将语言顺应论与语言变体学联系在一起。
语言变体学研究了人们在不同社会情境下使用的不同语言变体。
这包括了方言、语言特征的变化以及在不同社会群体中产生的语言变体。
在理论的框架下,语言顺应论作为一种表现社会身份和交际意图的变体现象开始被广泛研究。
第一次研究热潮斯普蕾尔和喬达1965年,斯普蕾尔和喬达提出了语言顺应论的概念,并提出了斯普蕾尔-喬达理论。
他们通过对话体的研究,发现人们在对话中会根据交际方的语言风格偏好来调整自己的语言风格,以便更好地适应对方。
这一理论掀起了第一次语言顺应论的研究热潮。
语言变体与社会身份在斯普蕾尔-喬达理论的影响下,学者们开始研究语言变体与社会身份之间的关系。
他们发现,人们在语言使用中会根据自己的社会身份、地区特点以及交际对象的身份来选择不同的语言变体。
社会身份对于语言使用的影响成为了研究的热点。
第二次研究热潮社会认同与语言变体20世纪80年代,语言顺应论再度引起学者们的关注。
这一次,学者们开始将语言顺应论与社会认同联系在一起,提出了社会认同理论。
社会认同理论认为,人们在语言使用中会调整自己的语言方式,以展现自己所属的社会群体和身份。
他们的研究表明,语言顺应不仅仅是为了更好地沟通,同时也是一种身份展示的方式。
社会语言学的兴起在第二次研究热潮中,语言顺应论进一步与社会语言学结合起来。
社会语言学引论
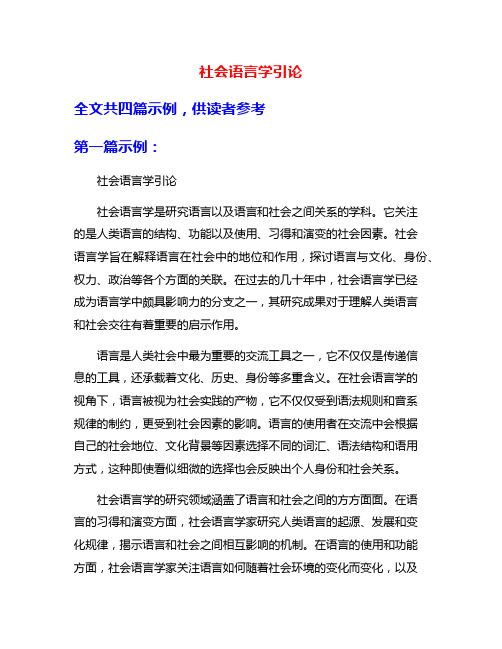
社会语言学引论全文共四篇示例,供读者参考第一篇示例:社会语言学引论社会语言学是研究语言以及语言和社会之间关系的学科。
它关注的是人类语言的结构、功能以及使用、习得和演变的社会因素。
社会语言学旨在解释语言在社会中的地位和作用,探讨语言与文化、身份、权力、政治等各个方面的关联。
在过去的几十年中,社会语言学已经成为语言学中颇具影响力的分支之一,其研究成果对于理解人类语言和社会交往有着重要的启示作用。
语言是人类社会中最为重要的交流工具之一,它不仅仅是传递信息的工具,还承载着文化、历史、身份等多重含义。
在社会语言学的视角下,语言被视为社会实践的产物,它不仅仅受到语法规则和音系规律的制约,更受到社会因素的影响。
语言的使用者在交流中会根据自己的社会地位、文化背景等因素选择不同的词汇、语法结构和语用方式,这种即使看似细微的选择也会反映出个人身份和社会关系。
社会语言学的研究领域涵盖了语言和社会之间的方方面面。
在语言的习得和演变方面,社会语言学家研究人类语言的起源、发展和变化规律,揭示语言和社会之间相互影响的机制。
在语言的使用和功能方面,社会语言学家关注语言如何随着社会环境的变化而变化,以及语言如何在不同社会群体之间传递信息、构建身份和权力关系等。
社会语言学还关注语言政策、语言权利、语言规范等问题,探讨语言对社会发展和文化传承的影响。
社会语言学的研究方法多样,包括问卷调查、实地观察、语料库分析、访谈调查等。
社会语言学家通过这些方法来收集大量的语言数据,以揭示语言使用的规律和特点。
通过对语言数据的分析和解释,社会语言学家可以发现语言与社会之间的互动关系,识别语言中隐藏的社会意义,并为语言教学、语言政策制定等提供参考和建议。
社会语言学是一门既深刻又广泛的学科,它揭示了语言作为社会现象的独特性和复杂性。
通过社会语言学的研究,我们可以更好地理解语言与社会之间的关系,促进语言教育和跨文化交流,促进多元文化的共存与发展。
社会语言学引论为我们提供了一个全面、系统地认识语言与社会互动关系的基础,帮助我们更深入地探索语言这一重要的社会现象。
slooss争论名词解释

slooss争论名词解释
Sloss 争论是指关于语言习得的一种理论观点之间的争论。
该争论源于美国语言学家诺姆·乔姆斯基(Noam Chomsky)和语言学家威廉·史洛斯(William Sloos)之间的一场辩论。
乔姆斯基提出了“天赋论”,认为人类天生具有语言习得的能力,而这种能力是通过基因传递的。
他认为,儿童在接触语言输入之前就已
经具备了一种语言生成机制,这种机制可以帮助他们理解和生成语言。
而史洛斯则提出了“经验论”,认为语言习得是通过经验和学习获得的。
他认为,儿童通过接触语言输入和与他人互动来学习语言,而不
是通过天赋的机制。
这场争论在语言学界引起了广泛的关注和讨论,并对语言习得的研究产生了深远的影响。
目前,关于语言习得的理论观点仍然存在争议,但大多数语言学家认为,语言习得是一个复杂的过程,涉及到基因、环境和经验等多个因素的相互作用。
合法化语码理论对伯恩斯坦知识结构理论的传承与创新资料

合法化语码理论对伯恩斯坦知识结构理论的传承与创新资料合法化语码理论是法国文化学者罗兰·巴尔特(Roland Barthes)提出的一种理论,在对语言和符号系统研究的基础上,探讨了个体如何通过符号系统来建构并理解现实世界的问题。
与之相对应的,伯恩斯坦知识结构理论则是由美国社会学家彼得·伯恩斯坦(Peter Berger)在20世纪60年代提出的,是一种关于社会现象和知识构建的理论。
下面将对合法化语码理论对伯恩斯坦知识结构理论的传承和创新进行详细阐述。
首先,合法化语码理论强调个体在符号系统中的位置性。
巴尔特认为,个体通过符号系统来建构和理解现实世界,符号系统包括语言、文字、图片等。
他认为符号系统是社会意义的生产者,在其中个体被赋予了特定的角色和意义。
这与伯恩斯坦知识结构理论中的“外部性”概念相对应。
伯恩斯坦认为,个体的认知和行为是受到社会结构限制的,社会结构为个体提供了行动方向和环境。
合法化语码理论将这一概念延伸到符号系统的层面,强调了符号系统对个体的限制和塑造作用。
因此,合法化语码理论在这一方面对伯恩斯坦知识结构理论进行了传承和发展。
其次,合法化语码理论强调了社会语境和符号系统的互动关系。
巴尔特认为,符号系统不仅仅是个体的工具,还与社会结构相互作用,从而对个体进行建构和考察,而且符号系统在不同的社会语境中具有不同的意义。
这与伯恩斯坦知识结构理论中的“双重观念”相对应。
伯恩斯坦认为,社会现象既是被建构的,也是对个体建构的反馈。
合法化语码理论将这一观点延伸到符号系统的层面,将符号系统看作是社会现象的一种表现形式,并且认为符号系统在社会语境中具有特定的意义。
合法化语码理论通过强调符号系统和社会语境的互动关系,对伯恩斯坦知识结构理论进行了创新。
此外,合法化语码理论在方法论方面对伯恩斯坦知识结构理论进行了发展。
巴尔特认为,个体对现实的理解和建构是通过符号系统的参与和建立来实现的。
他强调了符号系统的多样性和灵活性,因此,他提出了对符号系统进行细致的分析和描述的方法。
合法化语码理论对伯恩斯坦知识结构理论的传承与创新资料
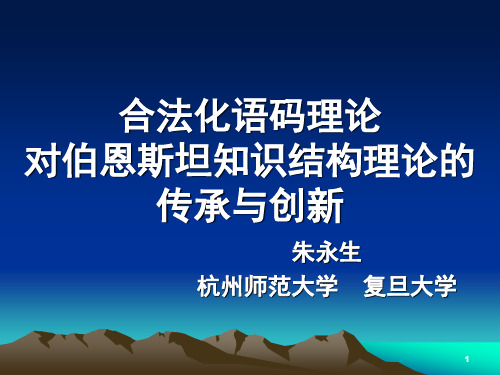
2 传承
Bernstein(1999,2000)发现,人类 所使用的话语并不是完全一致的,认 为可以把话语分为“水平话语” (horizontal discourse)和“垂直话语” (vertical discourse)两大类。
35
• 如图4(基于Maton 2012:30)所示,Maton 从专门性所包含的认识关系和社会关系两个 角度把这些要求分成知识语码(Knowledge codes)、知者语码(Knower codes)、精 英语码(Elite codes)和相对语码 (Relativist codes)等4类:
23
• 认知手段:能使知识领域得以维持、再生、 转换和变化的途径和方法
• 合法性语码:能通过认知手段的使用来表达 认知关系和社会关系的语言形式。
• 在Maton看来,认知手段十分重要。任何人 只要能控制认知手段,就会拥有设定符合自 身利益的场域结构和语法的方法,并表达和 实现对知识、地位和资源的诉求。
13
• 然而,Maton发现,Bernstein的研究成果只 看到不同场域使用不同的知识结构,却未能 提供产生这种现象的深层指导原则。 Bernstein 本 人 也 承 认 这 方 面 的 研 究 “ 非 常 薄弱”(Bernstein 2000: 124),但他在后 来的研究中并没有解决这个问题。正因为如 此,Maton才产生了丰富Bernstein知识结构 理论的想法,并把这个想法付诸实施,使他 创立的LCT在前人的研究基础上有所创新。
25
就知识结构而言,理科的知识结构通 常是水平的而不是垂直的;而文科的 知识结构通常是垂直的而不是水平的。 就知识语码而言,理科的知识语码通 常具有较弱的语义引力和较强的语义 密度;而文科的知识语码通常具有较 强的语义引力和较弱的语义密度。
社会语言学的语言观自然生理心理社会
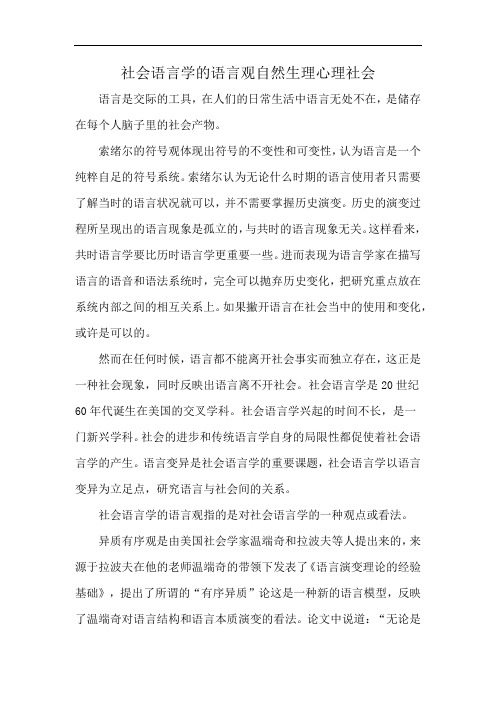
社会语言学的语言观自然生理心理社会语言是交际的工具,在人们的日常生活中语言无处不在,是储存在每个人脑子里的社会产物。
索绪尔的符号观体现出符号的不变性和可变性,认为语言是一个纯粹自足的符号系统。
索绪尔认为无论什么时期的语言使用者只需要了解当时的语言状况就可以,并不需要掌握历史演变。
历史的演变过程所呈现出的语言现象是孤立的,与共时的语言现象无关。
这样看来,共时语言学要比历时语言学更重要一些。
进而表现为语言学家在描写语言的语音和语法系统时,完全可以抛弃历史变化,把研究重点放在系统内部之间的相互关系上。
如果撇开语言在社会当中的使用和变化,或许是可以的。
然而在任何时候,语言都不能离开社会事实而独立存在,这正是一种社会现象,同时反映出语言离不开社会。
社会语言学是20世纪60年代诞生在美国的交叉学科。
社会语言学兴起的时间不长,是一门新兴学科。
社会的进步和传统语言学自身的局限性都促使着社会语言学的产生。
语言变异是社会语言学的重要课题,社会语言学以语言变异为立足点,研究语言与社会间的关系。
社会语言学的语言观指的是对社会语言学的一种观点或看法。
异质有序观是由美国社会学家温端奇和拉波夫等人提出来的,来源于拉波夫在他的老师温端奇的带领下发表了《语言演变理论的经验基础》,提出了所谓的“有序异质”论这是一种新的语言模型,反映了温端奇对语言结构和语言本质演变的看法。
论文中说道:“无论是共时还是历时,语言都是有序异质的客体。
”“质”既可以看作事物的性质,也可以看作事物的本质。
索绪尔研究的是语言的本质,他认为言语活动是从语言和言语两种形式来对语言进行研究,认为语言是抽象的、稳定的,言语是具体的、变化的。
后来,乔姆斯基在其观点之上是把语言区分为语言能力和语言行为。
乔姆斯基用语言能力表示索绪尔语言,用语言行为表示索绪尔的言语。
而一些社会语言学家认为,语言能力和语言行为是一个整体,并没有把言语活动区分开来,认为语言是异质有序的。
社会语言学

社会语言学第三章语言变异与语言变体语言学的产生和发展一般是追随着这样一个目标:就是发现或总结出语言的规律性。
可是,伴随着语言学在总结规律性方面的成就,特别是语言的规律性和系统性被理论化以后,语言学家们就往往会产生过度忽视语言现实中的不规则现象的倾向。
早在1929年吗,萨丕尔就曾指出,尽管语言的规律性足以使语言的研究媲美自然科学,但语言学家不应该忘记语言学的完美整齐的框框条条不过是对千变万化的社会文化行为的一种概括性总结。
20世纪60年代社会语言学诞生以后,在语言学研究中一度被忽视的语言变异现象受到了重视,目前已经成为社会语言学的一个最重要的研究对象。
以美国的拉波夫为首的“变异学派”宣布,语言是一个“有序的异质体”。
拉波夫1966年对纽约市民的发音调查被认为是开创性的对语音变异的研究。
通过调查得出这样的结论:(r)发音时的变异是纽约口音的一个特征,但对纽约人来说讲话中的(r)的变异还具有社会象征意义。
地道的纽约人都具有根据谈话中的卷舌概率来解释其象征意义的能力。
以往,人们认为一个语言社团最重要的特点就是社会成员的语言行为的一致性。
拉波夫认为应该用讲话人的主观判断来作为语言社团的一致性基础标准,语言社团的一致性并不表现在语言行为的一致性上,而是表现在相同的语言态度上。
如,以纽约的卷舌变异为例,纽约人被认为是属于一个语言社团,不是因为他们讲话中都具有相同的卷舌现象,而是他们具有相同的在较正规场合提高卷舌比例的趋势。
他们是同一语言社团的成员不是因为他们讲起话来一摸一样,而是因为他们对于同样的语言现象有着大致相同的评价和基本相同的理解。
用讲话人的主观判断来作为语言社团的一致性基础是一项在语言社团定义研究方面的突破。
这样,既避免了脱离实际地强调语言社团的一致性,又奠定了在“有序的异质体”这一理论前提下开展语言社团内部结构研究的基础。
第一节语言变异,语言变体,语言变项语言是处在不断变化发展的过程中,其中与社会因素相关的语言变异就成为社会语言学的研究重点。
语言如何进化(英文)
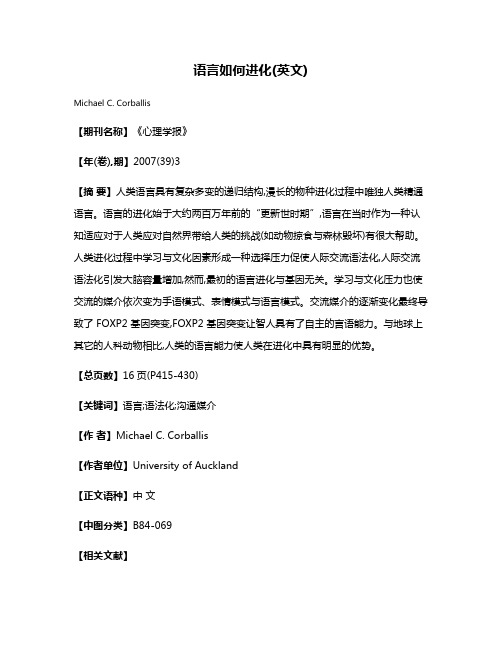
语言如何进化(英文)Michael C. Corballis【期刊名称】《心理学报》【年(卷),期】2007(39)3【摘要】人类语言具有复杂多变的递归结构,漫长的物种进化过程中唯独人类精通语言。
语言的进化始于大约两百万年前的“更新世时期”,语言在当时作为一种认知适应对于人类应对自然界带给人类的挑战(如动物掠食与森林毁坏)有很大帮助。
人类进化过程中学习与文化因素形成一种选择压力促使人际交流语法化,人际交流语法化引发大脑容量增加,然而,最初的语言进化与基因无关。
学习与文化压力也使交流的媒介依次变为手语模式、表情模式与语言模式。
交流媒介的逐渐变化最终导致了 FOXP2 基因突变,FOXP2 基因突变让智人具有了自主的言语能力。
与地球上其它的人科动物相比,人类的语言能力使人类在进化中具有明显的优势。
【总页数】16页(P415-430)【关键词】语言;语法化;沟通媒介【作者】Michael C. Corballis【作者单位】University of Auckland【正文语种】中文【中图分类】B84-069【相关文献】1.如何提高英文科技期刊的语言表达质量——《世界胃肠病学杂志(英文版)》语言把关的实践 [J], 程剑侠2.语言的进化与生物语言学进路诠疏——兼评《为什么只有我们:语言与进化》 [J], 赵永刚3.生物进化语境下的语言递归性——评乔姆斯基、杰肯道夫等人的语言机能进化观[J], 代天善4.语言本能——进化论里的语言本能说——史蒂芬·平克的《语言本能》介绍 [J], 唐再凤;范秀华5.语言进化研究动态一瞥——记第六届语言进化国际研讨会 [J], 楚行军因版权原因,仅展示原文概要,查看原文内容请购买。
知觉视点在翻译中的转换与等值效果
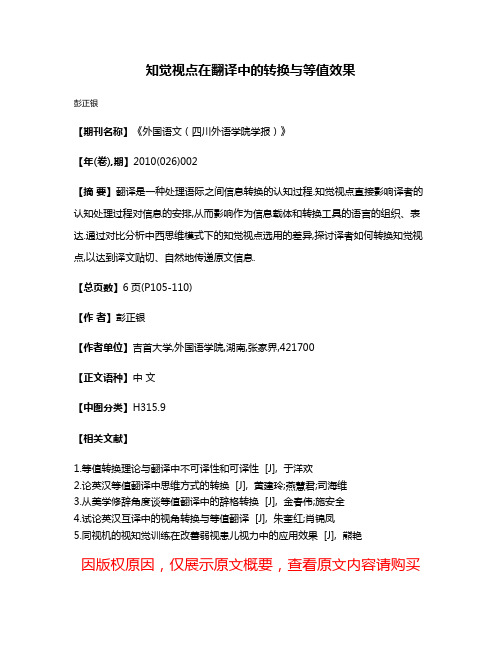
知觉视点在翻译中的转换与等值效果
彭正银
【期刊名称】《外国语文(四川外语学院学报)》
【年(卷),期】2010(026)002
【摘要】翻译是一种处理语际之间信息转换的认知过程.知觉视点直接影响译者的认知处理过程对信息的安排,从而影响作为信息载体和转换工具的语言的组织、表达.通过对比分析中西思维模式下的知觉视点选用的差异,探讨译者如何转换知觉视点,以达到译文贴切、自然地传递原文信息.
【总页数】6页(P105-110)
【作者】彭正银
【作者单位】吉首大学,外国语学院,湖南,张家界,421700
【正文语种】中文
【中图分类】H315.9
【相关文献】
1.等值转换理论与翻译中不可译性和可译性 [J], 于洋欢
2.论英汉等值翻译中思维方式的转换 [J], 黄建玲;燕慧君;司海维
3.从美学修辞角度谈等值翻译中的辞格转换 [J], 金春伟;施安全
4.试论英汉互译中的视角转换与等值翻译 [J], 朱奎红;肖锦凤
5.同视机的视知觉训练在改善弱视患儿视力中的应用效果 [J], 熊艳
因版权原因,仅展示原文概要,查看原文内容请购买。
- 1、下载文档前请自行甄别文档内容的完整性,平台不提供额外的编辑、内容补充、找答案等附加服务。
- 2、"仅部分预览"的文档,不可在线预览部分如存在完整性等问题,可反馈申请退款(可完整预览的文档不适用该条件!)。
- 3、如文档侵犯您的权益,请联系客服反馈,我们会尽快为您处理(人工客服工作时间:9:00-18:30)。
Stochastic Language Generation for Spoken Dialogue SystemsAlice H. Oh Carnegie Mellon University 5000 Forbes Ave.Pittsburgh, PA 15213aliceo+@Alexander I. Rudnicky Carnegie Mellon University 5000 Forbes Ave.Pittsburgh, PA 15213air+@AbstractThe two current approaches to language generation, template-based and rule-based (linguistic) NLG, have limitations when applied to spoken dialogue systems, in part because they were developed for text generation. In this paper, we propose a new corpus-based approach to natural language generation, specifically designed for spoken dialogue systems.IntroductionSeveral general-purpose rule-based generation systems have been developed, some of which are available publicly (cf. Elhadad, 1992). Unfortunately these systems, because of their generality, can be difficult to adapt to small, task-oriented applications. Bateman and Henschel (1999) have described a lower cost and more efficient generation system for a specific application using an automatically customized subgrammar. Busemann and Horacek (1998) describe a system that mixes templates and rule-based generation. This approach takes advantages of templates and rule-based generation as needed by specific sentences or utterances. Stent (1999) has proposed a similar approach for a spoken dialogue system. However, there is still the burden of writing and maintaining grammar rules, and processing time is probably too slow for sentences using grammar rules (only the average time for templates and rule-based sentences combined is reported in Busemann and Horacek, 1998), for use in spoken dialogue systems.Because comparatively less effort is needed, many current dialogue systems use template-based generation. But there is one obvious disadvantage: the quality of the output depends entirely on the set of templates. Even in a relatively simple domain, such as travel reservations, the number of templates necessary for reasonable quality can become quite large that maintenance becomes a serious problem. There is an unavoidable trade-off between the amount of time and effort in creating and maintaining templates and the variety and quality of the output utterances.Given these shortcomings of the above approaches, we developed a corpus-based generation system, in which we model language spoken by domain experts performing the task of interest, and use that model to stochastically generate system utterances. We have applied this technique to sentence realization and content planning, and have incorporated the resulting generation component into a working natural dialogue system (see Figure 1). In this paper, we describe the technique and report the results of two evaluations.We used two corpora in the travel reservations domain to build n-gram language models. One corpus (henceforth, the CMU corpus) consists of 39 dialogues between a travel agent and clients (Eskenazi, et al. 1999).Figure 1 : Overall ArchitectureFigure 2 : utterance classesFigure 3 : word classesAnother corpus (henceforth, the SRI corpus) consists of 68 dialogues between a travel agent and users in the SRI community (Kowtko and Price 1989).The utterances in the two corpora were tagged with utterance classes and word classes (see Figure 2 and Figure 3). The CMU corpus was manually tagged, and back-off trigram models built (using Clarkson and Rosenfeld, 1997). These language models were used to automatically tag the SRI corpus; the tags were manually checked.1Content PlanningIn content planning we decide which attributes (represented as word classes, see Figure 3) should be included in an utterance. In a task-oriented dialogue, the number of attributes generally increases during the course of the dialogue. Therefore, as the dialogue progresses, we need to decide which ones to include at each system turn. If we include all of them every time (indirect echoing, see Hayes and Reddy, 1983), the utterances become overly lengthy, but if we remove all unnecessary attributes, the user may get confused. With a fairly high recognition error rate, this becomes an even more important issue.The problem, then, is to find a compromise between the two. We compared two ways to systematically generate system utterances with only selected attributes, such that the user hears repetition of some of the constraints he/she has specified, at appropriate points in the dialogue, without sacrificing naturalness and efficiency. The specific problems, then, are deciding what should be repeated, and when. We first describe a simple heuristic of old versus new information. Then we present a statistical approach, based on bigram models.1.1First approach: old versus newAs a simple solution, we can use the previous dialogue history, by tagging the attribute-value pairs as old (previously said by the system) information or new (not said by the system yet) information. The generation module would select only new information to be included in the system utterances. Consequently, information given by the user is repeated only once in the dialogue, usually in the utterance immediately following the user utterance in which the new information was given1.Although this approach seems to work fairly well, echoing user’s constraints only once may not be the right thing to do. Looking at human-human dialogues, we observe that this is not very natural for a conversation; humans often repeat mutually known information, and they also often do not repeat some information at all. Also, this model does not capture the close relationship between two consecutive utterances within a dialogue. The second approach tries to address these issues.1.2Second approach: statistical model For this approach, we adopt the first of the two sub-maxims in (Oberlander, 1998) “Do the human thing”. Oberlander (1998) talks about generation of referring expressions, but it is universally valid, at least within natural language generation, to say the best we can do is 1 When the system utterance uses a template that does not contain the slots for the new information given in the previous user utterance, then that new information will be confirmed in the next available system utterance in which the template contains those slots.query_arrive_city inform_airportquery_arrive_time inform_confirm_utterance query_confirm inform_flightquery_depart_date inform_flight_another query_depart_time inform_flight_earlier query_pay_by_card nform_flight_earliest query_preferred_airport inform_flight_later query_return_date inform_flight_latest query_return_time inform_not_availhotel_car_info inform_num_flights hotel_hotel_chain inform_pricehotel_hotel_info otherairline depart_datearrive_airport depart_timearrive_city flight_numarrive_date hotel_cityarrive_time hotel_pricecar_company namecar_price num_flightsdepart_airport pmdepart_city priceto mimic human behavior. Hence, we built a two-stage statistical model of human-human dialogues using the CMU corpus. The model first predicts the number of attributes in the system utterance given the utterance class, then predicts the attributes given the attributes in the previous user utterance.1.2.1 The number of attributes modelThe first model will predict the number of attributes in a system utterance given the utterance class. The model is the probability distribution P(n k ) = P(n k |c k ), where n k is the number of attributes and c k is the utterance class for system utterance k .1.2.2 The bigram model of the attributesThis model will predict which attributes to use in a system utterance. Using a statistical model,what we need to do is find the set of attributes A * = {a 1, a 2, …, a n } such thatWe assume that the distributions of the a i ’s are dependent on the attributes in the previous utterances. As a simple model, we look only at the utterance immediately preceding the current utterance and build a bigram model of the attributes. In other words, A * = arg max P(A |B ),where B = {b 1, b 2, …, b m }, the set of m attributes in the preceding user utterance.If we took the above model and tried to apply it directly, we would run into a serious data sparseness problem, so we make two independence assumptions. The first assumption is that the attributes in the user utterance contribute independently to the probabilities of the attributes in the system utterance following it. Applying this assumption to the model above,we get the following:The second independence assumption is that the attributes in the system utterance are independent of each other. This gives the final model that we used for selecting the attributes.Although this independence assumption is an oversimplification, this simple model is a good starting point for our initial implementation of this approach.2 Stochastic Surface RealizationWe follow Busemann and Horacek (1998) in designing our generation engine with “different levels of granularity.” The different levels contribute to the specific needs of the various utterance classes. For example, at the beginning of the dialogue, a system greeting can be simply generated by a “canned” expression. Other short,simple utterances can be generated efficiently by templates. In Busemann and Horacek (1998), the remaining output is generated by grammar rules.We replace the generation grammar with a simple statistical language model to generate more complex utterances.There are four aspects to our stochastic surface realizer: building language models,generating candidate utterances, scoring the utterances, and filling in the slots. We explain each of these below.2.1 Building Language ModelsUsing the tagged utterances as described in the introduction, we built an unsmoothed n-gram language model for each utterance class. Tokens that belong in word classes (e.g., “U.S.Airways” in class “airline”) were replaced by the word classes before building the language models. We selected 5 as the n in n-gram to introduce some variability in the output utterances while preventing nonsense utterances.Note that language models are not used here in the same way as in speech recognition. In speech recognition, the language model probability acts as a ‘prior’ in determining the most probable sequence of words given the acoustics. In other words,W* = arg max P(W |A )= arg max P(A | W )Pr(W )where W is the string of words, w 1, …, w n , and A is the acoustic evidence (Jelinek 1998).Although we use the same statistical tool,we compute and use the language model probability directly to predict the next word. In other words, the most likely utterance is W* =∏=)..., , ,P(max arg n 21a a a *A )|)P(P(max arg m1k k k b A b *A ∑==∑∏===m k ni 11)|P()P(max arg k i k b a b A*arg max P(W|u), where u is the utterance class. We do not, however, look for the most likely hypothesis, but rather generate each word randomly according to the distribution, as illustrated in the next section.2.2Generating UtterancesThe input to NLG from the dialogue manager is a frame of attribute-value pairs. The first two attribute-value pairs specify the utterance class. The rest of the frame contains word classes and their values. Figure 4 is an example of an input frame to NLG.Figure 4 : an input frame to NLGThe generation engine uses the appropriate language model for the utterance class and generates word sequences randomly according to the language model distributions. As in speech recognition, the probability of a word using the n-gram language model isP(w i) = P(w i|w i-1, w i-2, … w i-(n-1), u)where u is the utterance class. Since we have built separate models for each of the utterance classes, we can ignore u, and say thatP(w i) = P(w i|w i-1, w i-2, … w i-(n-1))using the language model for u.Since we use unsmoothed 5-grams, we will not generate any unseen 5-grams (or smaller n-grams at the beginning and end of an utterance). This precludes generation of nonsense utterances, at least within the 5-word window. Using a smoothed n-gram would result in more randomness, but using the conventional back-off methods (Jelinek 1998), the probability mass assigned to unseen 5-grams would be very small, and those rare occurrences of unseen n-grams may not make sense anyway. There is the problem, as in speech recognition using n-gram language models, that long-distance dependency cannot be captured.2.3Scoring UtterancesFor each randomly generated utterance, we compute a penalty score. The score is based on the heuristics we’ve empirically selected. Various penalty scores are assigned for an utterance that 1. is too short or too long (determined by utterance-class dependent thresholds), 2. contains repetitions of any of the slots, 3. contains slots for which there is no valid value in the frame, or 4. does not have some required slots (see section 2 for deciding which slots are required).The generation engine generates a candidate utterance, scores it, keeping only the best-scored utterance up to that point. It stops and returns the best utterance when it finds an utterance with a zero penalty score, or runs out of time.2.4Filling SlotsThe last step is filling slots with the appropriate values. For example, the utterance “What time would you like to leave {depart_city}?”becomes “What time would you like to leave New York?”.3EvaluationIt is generally difficult to empirically evaluate a generation system. In the context of spoken dialogue systems, evaluation of NLG becomes an even more difficult problem. One reason is simply that there has been very little effort in building generation engines for spoken dialogue systems. Another reason is that it is hard to separate NLG from the rest of the system. It is especially hard to separate evaluation of language generation and speech synthesis.As a simple solution, we have conducted a comparative evaluation by running two identical systems varying only the generation component. In this section we present results from two preliminary evaluations of our generation algorithms described in the previous sections. 3.1Content Planning: ExperimentFor the content planning part of the generation system, we conducted a comparative evaluation of the two different generation algorithms: old/new and bigrams. Twelve subjects had two dialogues each, one with the old/new generation system, and another with the bigrams generation{act querycontent depart_timedepart_city New Yorkarrive_city San Francisco depart_date 19991117}system (in counterbalanced order); all other modules were held fixed. Afterwards, each subject answered seven questions on a usability survey. Immediately after, each subject was given transcribed logs of his/her dialogues and asked to rate each system utterance on a scale of 1 to 3 (1 = good; 2 = okay; 3 = bad).3.2Content Planning: ResultsFor the usability survey, the results seem to indicate subjects’ preference for the old/new system, but the difference is not statistically significant (p = 0.06). However, six out of the twelve subjects chose the bigram system to the question “During the session, which system’s responses were easier to understand?” compared to three subjects choosing the old/new system.3.3Surface Realization: Experiment For surface realization, we conducted a batch-mode evaluation. We picked six recent calls to our system and ran two generation algorithms (template-based generation and stochastic generation) on the input frames. We then presented to seven subjects the generated dialogues, consisting of decoder output of the user utterances and corresponding system responses, for each of the two generation algorithms. Subjects then selected the output utterance they would prefer, for each of the utterances that differ between the two systems. The results show a trend that subjects preferred stochastic generation over template-based generation, but a t-test shows no significant difference (p = 0.18). We are in the process of designing a larger evaluation.4ConclusionWe have presented a new approach to language generation for spoken dialogue systems. For content planning, we built a simple bigram model of attributes, and found that, in our first implementation, it performs as well as a heuristic of old vs. new information. For surface realization, we used an n-gram language model to stochastically generate each utterance and found that the stochastic system performs at least as well as the template-based system.Our stochastic generation system has several advantages. One of those, an important issue for spoken dialogue systems, is the response time. With stochastic surface realization, the average generation time for the longest utterance class (10 – 20 words long) is about 200 milliseconds, which is much faster than any rule-based systems. Another advantage is that by using a corpus-based approach, we are directly mimicking the language of a real domain expert, rather than attempting to model it by rule. Corpus collection is usually the first step in building a dialogue system, so we are leveraging the effort rather than creating more work. This also means adapting this approach to new domains and even new languages will be relatively simple.The approach we present does require some amount of knowledge engineering, though this appears to overlap with work needed for other parts of the dialogue system. First, defining the class of utterance and the attribute-value pairs requires care. Second, tagging the human-human corpus with the right classes and attributes requires effort. However, we believe the tagging effort is much less difficult than knowledge acquisition for most rule-based systems or even template-based systems. Finally, what may sound right for a human speaker may sound awkward for a computer, but we believe that mimicking a human, especially a domain expert, is the best we can do, at least for now. AcknowledgementsWe are thankful for significant contribution by other members of the CMU Communicator Project, especially Eric Thayer, Wei Xu, and Rande Shern. We would like to thank the subjects who participated in our evaluations. We also extend our thanks to two anonymous reviewers.ReferencesBateman, J. and Henschel, R. (1999) From full generation to ‘near-templates’ without losing generality. In Proceedings of the KI’99 workshop, "May I Speak Freely?"Busemann, S. and Horacek, H. (1998) A flexible shallow approach to text generation. In Proceedings of the International Natural Language Generation Workshop. Niagara-on-the-Lake, Canada.Clarkson, P. and Rosenfeld, R. (1997) Statistical Language Modeling using the CMU-Cambridge toolkit. In Proceedings of Eurospeech97. Elhadad, M. (1992) Using argumentation to control lexical choice: A functional unification-based approach. Ph.D. thesis, Computer Science Department, Columbia University.Eskenazi, M. Rudnicky, A. Gregory, K. Constantinides, P. Brennan, R. Bennett, C. and Allen, C. (1999) Data Collection and Processing in the Carnegie Mellon Communicator. In Proceedings of Eurospeech, 1999, 6, 2695-2698. Hayes, P.J. and Reddy, D.R. (1983) Steps toward graceful interaction in spoken and written man-machine communication. International Journal of Man-Machine Studies, v.19, p. 231-284. Jelinek, F. (1998) Statistical methods for speech recognition. The MIT Press. Cambridge, Massachusetts.Kowtko, J. and Price, P. (1989) Data collection and analysis in the air travel planning domain. In Proceedings of DARPA Speech and Natural Language Workshop, October 1989. Oberlander, J. (1998) Do the right thing... but expect the unexpected. In Computational Linguistics. Stent, D. (1999) Content planning and generation in continuous-speech spoken dialog systems. In Proceedings of the KI’99 workshop, "May I Speak Freely?"。