FEATURE BASED REGISTRATION OF MULTISPECTRAL DATA-CUBES
人工智能领域中英文专有名词汇总
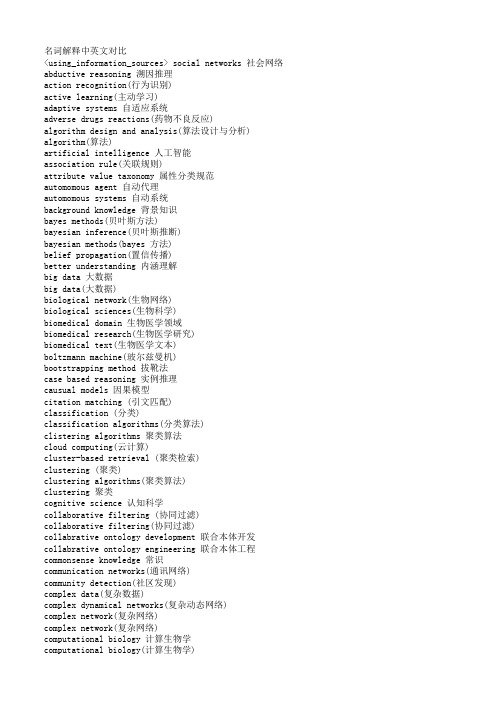
名词解释中英文对比<using_information_sources> social networks 社会网络abductive reasoning 溯因推理action recognition(行为识别)active learning(主动学习)adaptive systems 自适应系统adverse drugs reactions(药物不良反应)algorithm design and analysis(算法设计与分析) algorithm(算法)artificial intelligence 人工智能association rule(关联规则)attribute value taxonomy 属性分类规范automomous agent 自动代理automomous systems 自动系统background knowledge 背景知识bayes methods(贝叶斯方法)bayesian inference(贝叶斯推断)bayesian methods(bayes 方法)belief propagation(置信传播)better understanding 内涵理解big data 大数据big data(大数据)biological network(生物网络)biological sciences(生物科学)biomedical domain 生物医学领域biomedical research(生物医学研究)biomedical text(生物医学文本)boltzmann machine(玻尔兹曼机)bootstrapping method 拔靴法case based reasoning 实例推理causual models 因果模型citation matching (引文匹配)classification (分类)classification algorithms(分类算法)clistering algorithms 聚类算法cloud computing(云计算)cluster-based retrieval (聚类检索)clustering (聚类)clustering algorithms(聚类算法)clustering 聚类cognitive science 认知科学collaborative filtering (协同过滤)collaborative filtering(协同过滤)collabrative ontology development 联合本体开发collabrative ontology engineering 联合本体工程commonsense knowledge 常识communication networks(通讯网络)community detection(社区发现)complex data(复杂数据)complex dynamical networks(复杂动态网络)complex network(复杂网络)complex network(复杂网络)computational biology 计算生物学computational biology(计算生物学)computational complexity(计算复杂性) computational intelligence 智能计算computational modeling(计算模型)computer animation(计算机动画)computer networks(计算机网络)computer science 计算机科学concept clustering 概念聚类concept formation 概念形成concept learning 概念学习concept map 概念图concept model 概念模型concept modelling 概念模型conceptual model 概念模型conditional random field(条件随机场模型) conjunctive quries 合取查询constrained least squares (约束最小二乘) convex programming(凸规划)convolutional neural networks(卷积神经网络) customer relationship management(客户关系管理) data analysis(数据分析)data analysis(数据分析)data center(数据中心)data clustering (数据聚类)data compression(数据压缩)data envelopment analysis (数据包络分析)data fusion 数据融合data generation(数据生成)data handling(数据处理)data hierarchy (数据层次)data integration(数据整合)data integrity 数据完整性data intensive computing(数据密集型计算)data management 数据管理data management(数据管理)data management(数据管理)data miningdata mining 数据挖掘data model 数据模型data models(数据模型)data partitioning 数据划分data point(数据点)data privacy(数据隐私)data security(数据安全)data stream(数据流)data streams(数据流)data structure( 数据结构)data structure(数据结构)data visualisation(数据可视化)data visualization 数据可视化data visualization(数据可视化)data warehouse(数据仓库)data warehouses(数据仓库)data warehousing(数据仓库)database management systems(数据库管理系统)database management(数据库管理)date interlinking 日期互联date linking 日期链接Decision analysis(决策分析)decision maker 决策者decision making (决策)decision models 决策模型decision models 决策模型decision rule 决策规则decision support system 决策支持系统decision support systems (决策支持系统) decision tree(决策树)decission tree 决策树deep belief network(深度信念网络)deep learning(深度学习)defult reasoning 默认推理density estimation(密度估计)design methodology 设计方法论dimension reduction(降维) dimensionality reduction(降维)directed graph(有向图)disaster management 灾害管理disastrous event(灾难性事件)discovery(知识发现)dissimilarity (相异性)distributed databases 分布式数据库distributed databases(分布式数据库) distributed query 分布式查询document clustering (文档聚类)domain experts 领域专家domain knowledge 领域知识domain specific language 领域专用语言dynamic databases(动态数据库)dynamic logic 动态逻辑dynamic network(动态网络)dynamic system(动态系统)earth mover's distance(EMD 距离) education 教育efficient algorithm(有效算法)electric commerce 电子商务electronic health records(电子健康档案) entity disambiguation 实体消歧entity recognition 实体识别entity recognition(实体识别)entity resolution 实体解析event detection 事件检测event detection(事件检测)event extraction 事件抽取event identificaton 事件识别exhaustive indexing 完整索引expert system 专家系统expert systems(专家系统)explanation based learning 解释学习factor graph(因子图)feature extraction 特征提取feature extraction(特征提取)feature extraction(特征提取)feature selection (特征选择)feature selection 特征选择feature selection(特征选择)feature space 特征空间first order logic 一阶逻辑formal logic 形式逻辑formal meaning prepresentation 形式意义表示formal semantics 形式语义formal specification 形式描述frame based system 框为本的系统frequent itemsets(频繁项目集)frequent pattern(频繁模式)fuzzy clustering (模糊聚类)fuzzy clustering (模糊聚类)fuzzy clustering (模糊聚类)fuzzy data mining(模糊数据挖掘)fuzzy logic 模糊逻辑fuzzy set theory(模糊集合论)fuzzy set(模糊集)fuzzy sets 模糊集合fuzzy systems 模糊系统gaussian processes(高斯过程)gene expression data 基因表达数据gene expression(基因表达)generative model(生成模型)generative model(生成模型)genetic algorithm 遗传算法genome wide association study(全基因组关联分析) graph classification(图分类)graph classification(图分类)graph clustering(图聚类)graph data(图数据)graph data(图形数据)graph database 图数据库graph database(图数据库)graph mining(图挖掘)graph mining(图挖掘)graph partitioning 图划分graph query 图查询graph structure(图结构)graph theory(图论)graph theory(图论)graph theory(图论)graph theroy 图论graph visualization(图形可视化)graphical user interface 图形用户界面graphical user interfaces(图形用户界面)health care 卫生保健health care(卫生保健)heterogeneous data source 异构数据源heterogeneous data(异构数据)heterogeneous database 异构数据库heterogeneous information network(异构信息网络) heterogeneous network(异构网络)heterogenous ontology 异构本体heuristic rule 启发式规则hidden markov model(隐马尔可夫模型)hidden markov model(隐马尔可夫模型)hidden markov models(隐马尔可夫模型) hierarchical clustering (层次聚类) homogeneous network(同构网络)human centered computing 人机交互技术human computer interaction 人机交互human interaction 人机交互human robot interaction 人机交互image classification(图像分类)image clustering (图像聚类)image mining( 图像挖掘)image reconstruction(图像重建)image retrieval (图像检索)image segmentation(图像分割)inconsistent ontology 本体不一致incremental learning(增量学习)inductive learning (归纳学习)inference mechanisms 推理机制inference mechanisms(推理机制)inference rule 推理规则information cascades(信息追随)information diffusion(信息扩散)information extraction 信息提取information filtering(信息过滤)information filtering(信息过滤)information integration(信息集成)information network analysis(信息网络分析) information network mining(信息网络挖掘) information network(信息网络)information processing 信息处理information processing 信息处理information resource management (信息资源管理) information retrieval models(信息检索模型) information retrieval 信息检索information retrieval(信息检索)information retrieval(信息检索)information science 情报科学information sources 信息源information system( 信息系统)information system(信息系统)information technology(信息技术)information visualization(信息可视化)instance matching 实例匹配intelligent assistant 智能辅助intelligent systems 智能系统interaction network(交互网络)interactive visualization(交互式可视化)kernel function(核函数)kernel operator (核算子)keyword search(关键字检索)knowledege reuse 知识再利用knowledgeknowledgeknowledge acquisitionknowledge base 知识库knowledge based system 知识系统knowledge building 知识建构knowledge capture 知识获取knowledge construction 知识建构knowledge discovery(知识发现)knowledge extraction 知识提取knowledge fusion 知识融合knowledge integrationknowledge management systems 知识管理系统knowledge management 知识管理knowledge management(知识管理)knowledge model 知识模型knowledge reasoningknowledge representationknowledge representation(知识表达) knowledge sharing 知识共享knowledge storageknowledge technology 知识技术knowledge verification 知识验证language model(语言模型)language modeling approach(语言模型方法) large graph(大图)large graph(大图)learning(无监督学习)life science 生命科学linear programming(线性规划)link analysis (链接分析)link prediction(链接预测)link prediction(链接预测)link prediction(链接预测)linked data(关联数据)location based service(基于位置的服务) loclation based services(基于位置的服务) logic programming 逻辑编程logical implication 逻辑蕴涵logistic regression(logistic 回归)machine learning 机器学习machine translation(机器翻译)management system(管理系统)management( 知识管理)manifold learning(流形学习)markov chains 马尔可夫链markov processes(马尔可夫过程)matching function 匹配函数matrix decomposition(矩阵分解)matrix decomposition(矩阵分解)maximum likelihood estimation(最大似然估计)medical research(医学研究)mixture of gaussians(混合高斯模型)mobile computing(移动计算)multi agnet systems 多智能体系统multiagent systems 多智能体系统multimedia 多媒体natural language processing 自然语言处理natural language processing(自然语言处理) nearest neighbor (近邻)network analysis( 网络分析)network analysis(网络分析)network analysis(网络分析)network formation(组网)network structure(网络结构)network theory(网络理论)network topology(网络拓扑)network visualization(网络可视化)neural network(神经网络)neural networks (神经网络)neural networks(神经网络)nonlinear dynamics(非线性动力学)nonmonotonic reasoning 非单调推理nonnegative matrix factorization (非负矩阵分解) nonnegative matrix factorization(非负矩阵分解) object detection(目标检测)object oriented 面向对象object recognition(目标识别)object recognition(目标识别)online community(网络社区)online social network(在线社交网络)online social networks(在线社交网络)ontology alignment 本体映射ontology development 本体开发ontology engineering 本体工程ontology evolution 本体演化ontology extraction 本体抽取ontology interoperablity 互用性本体ontology language 本体语言ontology mapping 本体映射ontology matching 本体匹配ontology versioning 本体版本ontology 本体论open government data 政府公开数据opinion analysis(舆情分析)opinion mining(意见挖掘)opinion mining(意见挖掘)outlier detection(孤立点检测)parallel processing(并行处理)patient care(病人医疗护理)pattern classification(模式分类)pattern matching(模式匹配)pattern mining(模式挖掘)pattern recognition 模式识别pattern recognition(模式识别)pattern recognition(模式识别)personal data(个人数据)prediction algorithms(预测算法)predictive model 预测模型predictive models(预测模型)privacy preservation(隐私保护)probabilistic logic(概率逻辑)probabilistic logic(概率逻辑)probabilistic model(概率模型)probabilistic model(概率模型)probability distribution(概率分布)probability distribution(概率分布)project management(项目管理)pruning technique(修剪技术)quality management 质量管理query expansion(查询扩展)query language 查询语言query language(查询语言)query processing(查询处理)query rewrite 查询重写question answering system 问答系统random forest(随机森林)random graph(随机图)random processes(随机过程)random walk(随机游走)range query(范围查询)RDF database 资源描述框架数据库RDF query 资源描述框架查询RDF repository 资源描述框架存储库RDF storge 资源描述框架存储real time(实时)recommender system(推荐系统)recommender system(推荐系统)recommender systems 推荐系统recommender systems(推荐系统)record linkage 记录链接recurrent neural network(递归神经网络) regression(回归)reinforcement learning 强化学习reinforcement learning(强化学习)relation extraction 关系抽取relational database 关系数据库relational learning 关系学习relevance feedback (相关反馈)resource description framework 资源描述框架restricted boltzmann machines(受限玻尔兹曼机) retrieval models(检索模型)rough set theroy 粗糙集理论rough set 粗糙集rule based system 基于规则系统rule based 基于规则rule induction (规则归纳)rule learning (规则学习)rule learning 规则学习schema mapping 模式映射schema matching 模式匹配scientific domain 科学域search problems(搜索问题)semantic (web) technology 语义技术semantic analysis 语义分析semantic annotation 语义标注semantic computing 语义计算semantic integration 语义集成semantic interpretation 语义解释semantic model 语义模型semantic network 语义网络semantic relatedness 语义相关性semantic relation learning 语义关系学习semantic search 语义检索semantic similarity 语义相似度semantic similarity(语义相似度)semantic web rule language 语义网规则语言semantic web 语义网semantic web(语义网)semantic workflow 语义工作流semi supervised learning(半监督学习)sensor data(传感器数据)sensor networks(传感器网络)sentiment analysis(情感分析)sentiment analysis(情感分析)sequential pattern(序列模式)service oriented architecture 面向服务的体系结构shortest path(最短路径)similar kernel function(相似核函数)similarity measure(相似性度量)similarity relationship (相似关系)similarity search(相似搜索)similarity(相似性)situation aware 情境感知social behavior(社交行为)social influence(社会影响)social interaction(社交互动)social interaction(社交互动)social learning(社会学习)social life networks(社交生活网络)social machine 社交机器social media(社交媒体)social media(社交媒体)social media(社交媒体)social network analysis 社会网络分析social network analysis(社交网络分析)social network(社交网络)social network(社交网络)social science(社会科学)social tagging system(社交标签系统)social tagging(社交标签)social web(社交网页)sparse coding(稀疏编码)sparse matrices(稀疏矩阵)sparse representation(稀疏表示)spatial database(空间数据库)spatial reasoning 空间推理statistical analysis(统计分析)statistical model 统计模型string matching(串匹配)structural risk minimization (结构风险最小化) structured data 结构化数据subgraph matching 子图匹配subspace clustering(子空间聚类)supervised learning( 有support vector machine 支持向量机support vector machines(支持向量机)system dynamics(系统动力学)tag recommendation(标签推荐)taxonmy induction 感应规范temporal logic 时态逻辑temporal reasoning 时序推理text analysis(文本分析)text anaylsis 文本分析text classification (文本分类)text data(文本数据)text mining technique(文本挖掘技术)text mining 文本挖掘text mining(文本挖掘)text summarization(文本摘要)thesaurus alignment 同义对齐time frequency analysis(时频分析)time series analysis( 时time series data(时间序列数据)time series data(时间序列数据)time series(时间序列)topic model(主题模型)topic modeling(主题模型)transfer learning 迁移学习triple store 三元组存储uncertainty reasoning 不精确推理undirected graph(无向图)unified modeling language 统一建模语言unsupervisedupper bound(上界)user behavior(用户行为)user generated content(用户生成内容)utility mining(效用挖掘)visual analytics(可视化分析)visual content(视觉内容)visual representation(视觉表征)visualisation(可视化)visualization technique(可视化技术) visualization tool(可视化工具)web 2.0(网络2.0)web forum(web 论坛)web mining(网络挖掘)web of data 数据网web ontology lanuage 网络本体语言web pages(web 页面)web resource 网络资源web science 万维科学web search (网络检索)web usage mining(web 使用挖掘)wireless networks 无线网络world knowledge 世界知识world wide web 万维网world wide web(万维网)xml database 可扩展标志语言数据库附录 2 Data Mining 知识图谱(共包含二级节点15 个,三级节点93 个)间序列分析)监督学习)领域 二级分类 三级分类。
SPE参考文献:著录存在的问题与规范化表达
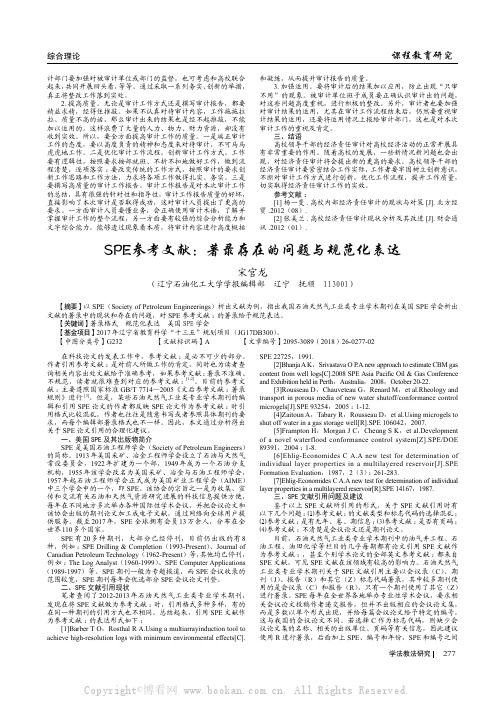
综合理论课程教育研究学法教法研究 277计部门要加强对被审计单位或部门的监督,也可考虑和高校联合起来,共同开展回头看,等等。
通过采取一系列务实、创新的举措,真正将整改工作落到实处。
2.提高质量。
无论是审计工作方式还是撰写审计报告,都要精益求精,经得住推敲。
如果不认真对待审计内容,工作拖拖拉拉、质量不高的话,那么审计出来的结果也是经不起推敲,不能加以运用的。
这样浪费了大量的人力、物力、财力资源,却没有收到实效。
所以,要全方面提高审计工作的质量。
一是端正审计工作的态度,要以高度负责的精神和态度来对待审计,不可马马虎虎地工作。
二是优化审计工作流程,创新审计工作方式。
工作要有逻辑性,按照要求按部就班、不折不扣地做好工作,做到流程清楚,逐项落实;要改变传统的工作方式,按照审计的要求创新工作思路和工作方法,力求将各项工作做得扎实、务实。
三是要撰写高质量的审计工作报告。
审计工作报告是对本次审计工作的总结,具有很强的针对性和指导性,审计工作报告质量的好坏,直接影响了本次审计是否取得成功,这对审计人员提出了更高的要求。
一方面审计人员要懂业务,会正确使用审计术语,了解并掌握审计工作的整个流程,另一方面要有较强的综合分析能力和文字综合能力,能够透过现象看本质,将审计内容进行高度概括和凝练,从而提升审计报告的质量。
3.加强运用。
要将审计后的结果加以应用,防止出现“只审不用”的现象。
被审计单位班子成员要正确认识审计出的问题,对这些问题高度重视,进行积极的整改。
另外,审计者也要加强对审计结果的运用,尤其在审计工作流程结束后,仍然要重视审计结果的运用,还要将运用情况上报给审计部门,这也是对本次审计工作的重视及肯定。
三、结语高校领导干部的经济责任审计对高校经济活动的正常开展具有非常重要的作用。
随着高校的发展,一些新情况新问题也会出现,对经济责任审计将会提出新的更高的要求。
高校领导干部的经济责任审计要紧密结合工作实际,工作者要牢固树立创新意识,不断对审计工作方式进行创新,优化工作流程,提升工作质量,切实取得经济责任审计工作的实效。
低频活动漂浮潜水船声探测系统(LFATS)说明书

LOW-FREQUENCY ACTIVE TOWED SONAR (LFATS)LFATS is a full-feature, long-range,low-frequency variable depth sonarDeveloped for active sonar operation against modern dieselelectric submarines, LFATS has demonstrated consistent detection performance in shallow and deep water. LFATS also provides a passive mode and includes a full set of passive tools and features.COMPACT SIZELFATS is a small, lightweight, air-transportable, ruggedized system designed specifically for easy installation on small vessels. CONFIGURABLELFATS can operate in a stand-alone configuration or be easily integrated into the ship’s combat system.TACTICAL BISTATIC AND MULTISTATIC CAPABILITYA robust infrastructure permits interoperability with the HELRAS helicopter dipping sonar and all key sonobuoys.HIGHLY MANEUVERABLEOwn-ship noise reduction processing algorithms, coupled with compact twin line receivers, enable short-scope towing for efficient maneuvering, fast deployment and unencumbered operation in shallow water.COMPACT WINCH AND HANDLING SYSTEMAn ultrastable structure assures safe, reliable operation in heavy seas and permits manual or console-controlled deployment, retrieval and depth-keeping. FULL 360° COVERAGEA dual parallel array configuration and advanced signal processing achieve instantaneous, unambiguous left/right target discrimination.SPACE-SAVING TRANSMITTERTOW-BODY CONFIGURATIONInnovative technology achievesomnidirectional, large aperture acousticperformance in a compact, sleek tow-body assembly.REVERBERATION SUPRESSIONThe unique transmitter design enablesforward, aft, port and starboarddirectional transmission. This capabilitydiverts energy concentration away fromshorelines and landmasses, minimizingreverb and optimizing target detection.SONAR PERFORMANCE PREDICTIONA key ingredient to mission planning,LFATS computes and displays systemdetection capability based on modeled ormeasured environmental data.Key Features>Wide-area search>Target detection, localization andclassification>T racking and attack>Embedded trainingSonar Processing>Active processing: State-of-the-art signal processing offers acomprehensive range of single- andmulti-pulse, FM and CW processingfor detection and tracking. Targetdetection, localization andclassification>P assive processing: LFATS featuresfull 100-to-2,000 Hz continuouswideband coverage. Broadband,DEMON and narrowband analyzers,torpedo alert and extendedtracking functions constitute asuite of passive tools to track andanalyze targets.>Playback mode: Playback isseamlessly integrated intopassive and active operation,enabling postanalysis of pre-recorded mission data and is a keycomponent to operator training.>Built-in test: Power-up, continuousbackground and operator-initiatedtest modes combine to boostsystem availability and accelerateoperational readiness.UNIQUE EXTENSION/RETRACTIONMECHANISM TRANSFORMS COMPACTTOW-BODY CONFIGURATION TO ALARGE-APERTURE MULTIDIRECTIONALTRANSMITTERDISPLAYS AND OPERATOR INTERFACES>State-of-the-art workstation-based operator machineinterface: Trackball, point-and-click control, pull-down menu function and parameter selection allows easy access to key information. >Displays: A strategic balance of multifunction displays,built on a modern OpenGL framework, offer flexible search, classification and geographic formats. Ground-stabilized, high-resolution color monitors capture details in the real-time processed sonar data. > B uilt-in operator aids: To simplify operation, LFATS provides recommended mode/parameter settings, automated range-of-day estimation and data history recall. >COTS hardware: LFATS incorporates a modular, expandable open architecture to accommodate future technology.L3Harrissellsht_LFATS© 2022 L3Harris Technologies, Inc. | 09/2022NON-EXPORT CONTROLLED - These item(s)/data have been reviewed in accordance with the InternationalTraffic in Arms Regulations (ITAR), 22 CFR part 120.33, and the Export Administration Regulations (EAR), 15 CFR 734(3)(b)(3), and may be released without export restrictions.L3Harris Technologies is an agile global aerospace and defense technology innovator, delivering end-to-endsolutions that meet customers’ mission-critical needs. The company provides advanced defense and commercial technologies across air, land, sea, space and cyber domains.t 818 367 0111 | f 818 364 2491 *******************WINCH AND HANDLINGSYSTEMSHIP ELECTRONICSTOWED SUBSYSTEMSONAR OPERATORCONSOLETRANSMIT POWERAMPLIFIER 1025 W. NASA Boulevard Melbourne, FL 32919SPECIFICATIONSOperating Modes Active, passive, test, playback, multi-staticSource Level 219 dB Omnidirectional, 222 dB Sector Steered Projector Elements 16 in 4 stavesTransmission Omnidirectional or by sector Operating Depth 15-to-300 m Survival Speed 30 knotsSize Winch & Handling Subsystem:180 in. x 138 in. x 84 in.(4.5 m x 3.5 m x 2.2 m)Sonar Operator Console:60 in. x 26 in. x 68 in.(1.52 m x 0.66 m x 1.73 m)Transmit Power Amplifier:42 in. x 28 in. x 68 in.(1.07 m x 0.71 m x 1.73 m)Weight Winch & Handling: 3,954 kg (8,717 lb.)Towed Subsystem: 678 kg (1,495 lb.)Ship Electronics: 928 kg (2,045 lb.)Platforms Frigates, corvettes, small patrol boats Receive ArrayConfiguration: Twin-lineNumber of channels: 48 per lineLength: 26.5 m (86.9 ft.)Array directivity: >18 dB @ 1,380 HzLFATS PROCESSINGActiveActive Band 1,200-to-1,00 HzProcessing CW, FM, wavetrain, multi-pulse matched filtering Pulse Lengths Range-dependent, .039 to 10 sec. max.FM Bandwidth 50, 100 and 300 HzTracking 20 auto and operator-initiated Displays PPI, bearing range, Doppler range, FM A-scan, geographic overlayRange Scale5, 10, 20, 40, and 80 kyd PassivePassive Band Continuous 100-to-2,000 HzProcessing Broadband, narrowband, ALI, DEMON and tracking Displays BTR, BFI, NALI, DEMON and LOFAR Tracking 20 auto and operator-initiatedCommonOwn-ship noise reduction, doppler nullification, directional audio。
Feature extraction_rule-based-for ENVI EX
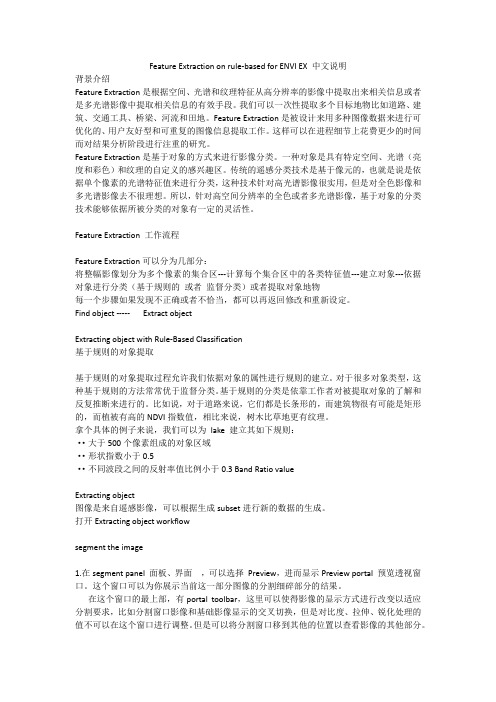
Feature Extraction on rule-based for ENVI EX 中文说明背景介绍Feature Extraction是根据空间、光谱和纹理特征从高分辨率的影像中提取出来相关信息或者是多光谱影像中提取相关信息的有效手段。
我们可以一次性提取多个目标地物比如道路、建筑、交通工具、桥梁、河流和田地。
Feature Extraction是被设计来用多种图像数据来进行可优化的、用户友好型和可重复的图像信息提取工作。
这样可以在进程细节上花费更少的时间而对结果分析阶段进行注重的研究。
Feature Extraction是基于对象的方式来进行影像分类。
一种对象是具有特定空间、光谱(亮度和彩色)和纹理的自定义的感兴趣区。
传统的遥感分类技术是基于像元的,也就是说是依据单个像素的光谱特征值来进行分类,这种技术针对高光谱影像很实用,但是对全色影像和多光谱影像去不很理想。
所以,针对高空间分辨率的全色或者多光谱影像,基于对象的分类技术能够依据所被分类的对象有一定的灵活性。
Feature Extraction 工作流程Feature Extraction可以分为几部分:将整幅影像划分为多个像素的集合区---计算每个集合区中的各类特征值---建立对象---依据对象进行分类(基于规则的或者监督分类)或者提取对象地物每一个步骤如果发现不正确或者不恰当,都可以再返回修改和重新设定。
Find object ----- Extract objectExtracting object with Rule-Based Classification基于规则的对象提取基于规则的对象提取过程允许我们依据对象的属性进行规则的建立。
对于很多对象类型,这种基于规则的方法常常优于监督分类。
基于规则的分类是依靠工作者对被提取对象的了解和反复推断来进行的。
比如说,对于道路来说,它们都是长条形的,而建筑物很有可能是矩形的,而植被有高的NDVI指数值,相比来说,树木比草地更有纹理。
基于改进小波包与样本熵的表面肌电信号特征提取
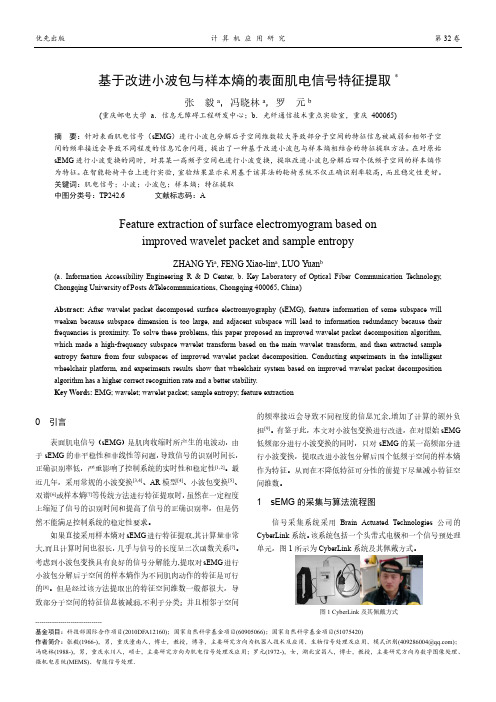
优先出版 计 算 机 应 用 研 究 第32卷--------------------------------基金项目:科技部国际合作项目(2010DFA12160);国家自然科学基金项目(60905066);国家自然科学基金项目(51075420)作者简介:张毅(1966-),男,重庆潼南人,博士,教授,博导,主要研究方向为机器人技术及应用、生物信号处理及应用、模式识别(409286004@);冯晓林(1988-),男,重庆永川人,硕士,主要研究方向为肌电信号处理及应用;罗元(1972-),女,湖北宜昌人,博士,教授,主要研究方向为数字图像处理、微机电系统(MEMS)、智能信号处理.基于改进小波包与样本熵的表面肌电信号特征提取 *张 毅a ,冯晓林a ,罗 元b(重庆邮电大学 a .信息无障碍工程研发中心;b .光纤通信技术重点实验室,重庆 400065)摘 要:针对表面肌电信号(sEMG )进行小波包分解后子空间维数较大导致部分子空间的特征信息被减弱和相邻子空间的频率接近会导致不同程度的信息冗余问题,提出了一种基于改进小波包与样本熵相结合的特征提取方法。
在对原始sEMG 进行小波变换的同时,对其某一高频子空间也进行小波变换,提取改进小波包分解后四个低频子空间的样本熵作为特征。
在智能轮椅平台上进行实验,室验结果显示采用基于该算法的轮椅系统不仅正确识别率较高,而且稳定性更好。
关键词:肌电信号;小波;小波包;样本熵;特征提取 中图分类号:TP242.6 文献标志码:AFeature extraction of surface electromyogram based onimproved wavelet packet and sample entropyZHANG Yi a , FENG Xiao-lin a , LUO Yuan b(a. Information Accessibility Engineering R & D Center, b. Key Laboratory of Optical Fiber Communication Technology, Chongqing University of Posts &Telecommunications, Chongqing 400065, China)Abstract: After wavelet packet decomposed surface electromyography (sEMG), feature information of some subspace will weaken because subspace dimension is too large, and adjacent subspace will lead to information redundancy because their frequencies is proximity. To solve these problems, this paper proposed an improved wavelet packet decomposition algorithm, which made a high-frequency subspace wavelet transform based on the main wavelet transform, and then extracted sample entropy feature from four subspaces of improved wavelet packet decomposition. Conducting experiments in the intelligent wheelchair platform, and experiments results show that wheelchair system based on improved wavelet packet decomposition algorithm has a higher correct recognition rate and a better stability.Key Words: EMG; wavelet; wavelet packet; sample entropy; feature extraction0 引言表面肌电信号(sEMG )是肌肉收缩时所产生的电波动,由于sEMG 的非平稳性和非线性等问题,导致信号的识别时间长,正确识别率低,严重影响了控制系统的实时性和稳定性[1,2]。
基于正则化稠密连接特征金字塔的实例显著性检测方法[发明专利]
![基于正则化稠密连接特征金字塔的实例显著性检测方法[发明专利]](https://img.taocdn.com/s3/m/9f390a1e2bf90242a8956bec0975f46527d3a73a.png)
(19)中华人民共和国国家知识产权局(12)发明专利申请(10)申请公布号 (43)申请公布日 (21)申请号 202010328784.6(22)申请日 2020.04.23(71)申请人 南开大学地址 300071 天津市南开区卫津路94号(72)发明人 吴宇寰 刘云 程明明 (74)专利代理机构 天津耀达律师事务所 12223代理人 侯力(51)Int.Cl.G06T 7/00(2017.01)G06T 7/11(2017.01)G06K 9/46(2006.01)(54)发明名称基于正则化稠密连接特征金字塔的实例显著性检测方法(57)摘要一种基于正则化稠密连接特征金字塔的实例显著性检测的方法。
该方法的目的是构造稠密连接的特征金字塔来进行图像实例级显著性检测。
该方法设计了一种新的卷积神经网络模型,这种新的卷积神经网络模型通过构建该发明提出的正则化的稠密连接在原有特征金字塔的基础上构造一个新的特征金字塔。
稠密连接为构建新特征金字塔提供了更多的低层级特征,而正则化操作在稠密连接提供更多低层级特征的情况内更好地提取其中的有用信息而刨除无用信息。
此外,利用多级RoIAlign方法进行特征聚合可以更精确地对实例进行分割。
使用该方法检测出的显著性实例,可以用于重要目标分割、自适应图像压缩、图像检索等应用领域。
权利要求书1页 说明书5页 附图3页CN 111598841 A 2020.08.28C N 111598841A1.基于正则化稠密连接(regularized dense connections)特征金字塔的实例显著性检测方法,其特征在于,该方法包含如下步骤:a.设计一个新的卷积神经网络模型,该模型分为三大部分,第一个是特征提取部分,第二个是侧向物体框预测分支部分,第三个是物体像素级分割分支部分;b.用户向所述卷积神经网络模型中输入一张任意大小的图片到模型的特征提取部分,即一个带有特征金字塔增强的卷积神经网络中,并输出一个特征金字塔;所述的特征金字塔增强是先利用“FCOS:Fully Convolutional One -Stage Object Detection ”中的特征金字塔增强方法生成增强的特征金字塔,再在该基础上使用正则化稠密连接构建新的特征金字塔;c.步骤b中生成的特征金字塔的每个特征层级都连接着所述卷积神经网络模型的第二部分即侧向物体框预测分支,得到预测到的物体框级别的显著性实例;d.利用步骤c中预测出的物体框级别的显著性实例,在“Mask R -CNN ”中提出的RoIAlign方法基础上,对步骤b中生成的特征金字塔进行特征复用,即使用多级RoIAlign方法,得到每个预测到的显著性实例的感兴趣区域(Region ofInterest)特征金字塔,再利用模型的第三部分即物体像素级分割分支部分得到每个实例的像素级分割预测结果,最后将这些预测结果对应到原图的各个位置,得到最后实例级的显著性物体检测结果。
动画专业英语

动画专业英语action['ækʃən] 动作animator:['æni,meitə] 原画者,动画设计assistant:[ə'sistənt] 动画者antic :['æntik] 预备动作air brushing:['brʌʃiŋ] 喷效angle:['æŋgl] 角度animated zoom['æni,meitid] 画面扩大或缩小animation film:[,æni'meiʃən] 动画片animation puter:[kəm'pju:tə] 电脑控制动画摄影atmosphere sketch:['ætməsfiə] [sketʃ] 气氛草图B.P.(bot pegs)下定位BG(background):['bækgraund]背景blurs:[blə:]模糊BLK(blink):[bliŋk]眨眼BRK DN(B.D.)(break-down)中割BG layout:['leiaut] 背景设计稿background keys 背景样本background hookup:[huk]衔接背景background pan 长背景background still:[stil] 短背景bar sheets :[ʃi:t]音节表beat:[bi:t]节拍blank :[blæŋk]空白bloom:[blu:m]闪光blow up :[bləu] 放大camera notes:['kæmərə] :[nəut] 摄影注意事项C.U.(close-up)特写clean up:[kli:n]清稿,修形,作监cut:[kʌt]镜头结束CEL=celluloid:['selju,lɔid]化学板cycle:['saikl]循环CW(clock-wise):[klɔk][waiz] 顺时针转动CCW(counter clock-wise):['kauntə] 逆时针转动continue(cont,con‘d):[kən'tinju:] 继续CAM(camera):['kæmərə] 摄影机CUSH(cushion):['kuʃən] 缓冲C=center:['sentə]中心点camera shake:[ʃeik] 镜头振动checker:['tʃekə]检查员constant :['kɔnstənt]等速持续color keys=color mark-ups:[mɑ:k]色指定color model:['mɔdl]彩色造型color flash(paint flash):[peint] 跳色camera animation动画摄影机cellevel:['levl]化学板层次character:['kæriktə]人物造型DIALOG(dialogue):['daiəlɔ:g] 对白及口形duble exposure:[iks'pəuʒə]双重曝光multiruns多重曝光1st run第一次曝光2nd run地二次曝光dry brushing::[drai]['brʌʃiŋ] 干刷DIAG PAN(diagonal):[dai'ægənl]斜移DWF(drawing):['drɔ:iŋ]画,动画纸double image :['imidʒ]双重影像dailies(RUSHES)样片director:[di'rektə]导演dissolve(X.D):[di'zɔlv] 溶景,叠化distortion:[dis'tɔ:ʃən] 变形double frame :[freim] 双(画)格drawing disc :[disk] 动画圆盘E.C.U = extreme close up:[iks'tri:m] 大特写EXT(exterior):[eks'tiəriə] 外面;室外景EFT(effect):[i'fekt]特效editing:['edit] 剪辑exit(moves out, o.s.):['eksit] 出去enter(in):['entə]入画ease-in:[i:z] 渐快ease-out:[aut]渐慢editor:['editə]剪辑师episode:['episəud]片集field(FLD):[fi:ld]安全框fade(in/on):[feid]画面淡入fade(out/off)画面淡出FIN(finish):['finiʃ]完成FOLOS(follows):['fɔləu]跟随,跟着fast;quickly:[fɑ:st] ['kwikli] 快速field guide:[gaid]安全框指示final check:['fainl] [tʃek]总检footage:['futidʒ]尺数(英尺)F.G.(foreground):['fɔ:graund]前景focallength :['fəukəl]:[leŋθ] 焦距frame:[freim]格数freeze frame:[freim]停格gain in:[gein]移入head up:[hed]抬头hook up:[huk] 接景;衔接hold:[həuld] 画面停格halo:['heiləu] 光圈INT(interior):[in'tiəriə] 里面;室内景INB(in between):[bi'twi:n]动画in-betweener动画员I&P(ink & paint):[iŋk] :[peint]描线和着色inking:[kiŋ]描线in sync:[siŋk] 同步intermittent:[,intə'mitənt] 间歇iris out :['airis] 画面旋逝jiggle:['dʒigl] 摇动jump:[dʒʌmp] 跳jitter:['dʒitə] 跳动LIP SYNC(synchronization)[lip] [,siŋkrənai'zeiʃən ] 口形level:['levl]层look:[luk]看listen:['lisn]听layout:['leiaut] 设计稿;构图laughs(LAFFS):[lɑ:f]笑L/S(light source):[lait] :[sɔ:s]光源linetest(pencil test):[lain]铅笔稿试拍;线拍M.S.(medium shot):['mi:diəm] :[ʃɔt]中景M.C.U.(medium close up)['mi:diəm]近景movesout(exit;o.s.):[mu:v] :['eksit]出去moves in[mu:v]进入match line:[mætʃ] :[lain]组合线multi runs :['mʌlti]:[rʌnz]多重拍摄mouth:[mauθ]嘴mouth charts:[tʃɑ:t]口形图MAG TRACK(magnetic sound track):[mæg'netik]音轨multi cellevels:['mʌlti] :['levl]多层次化学板multiplane:[plein]多层设计n/spegs:[peg]南北定位器N.G.(no good):[gud]不好的,作废narration:[næ'reiʃən]旁白叙述OL(overlay):['əuvə'lei]前层景Out of scene:[si:n]到画外面O.S.(off stage off scene):[steidʒ]出景off model:['mɔdl]走型OL/UL(underlay):['ʌndəlei]前层与中层间的景overlap action:['əuvə'læp] :['ækʃən]重叠动作ones:[wʌn]一格;单格pose:[pəuz] 姿势POS(position):[pə'ziʃən]位置;定点pan移动pops in/on突然出现pause:[pɔ:z]停顿;暂停perspective:[pə'spektiv]透视peg bar定位尺P.T.(painting):['peintiŋ] 着色paint flashes(color flashes)跳色papercut剪纸片pencil test:['pensl] 铅笔稿试拍persistence of vision:[pə'sistəns] :['viʒən] 视觉暂留post-synchronized sound:[saund]后期同步录音puppet:['pʌpit]木偶片ripple glass:['ripl] :[glɑ:s]水纹玻璃re-peg :[peg]重新定位RUFF(rough-drawing):[rʌf]:['drɔ:iŋ] 草稿run:[rʌn]跑REG(register):['redʒistə]组合RPT(repeat):[ri'pi:t]重复retakes: teik]重拍;修改registration pegs:[,redʒi'streiʃən]定位器registration holes:[həul]定位洞silhouette(SILO):[,silu'et]剪影speed line:[spi:d][lain]流线storm out:[stɔ:m]速转出sparkle:['spɑ:kl]火花;闪光shadow:['ʃædəu]阴影smile:[smail]微笑smoke:[sməuk]烟stop:[stɔp]停止slow:[sləu]慢慢的SC(scene):[si:n]镜号S/A(same as):[seim]兼用S.S(screen shake):[skri:n] :[ʃeik]画面振动size parison:[saiz] :[kəm'pærisn]大小比例storyboard(SAB):['stɔ:ri,bɔ:d]分镜头台本SFX(sound effect):[saund] :[i'fekt]声效;音效settle:['setl]定姿;定置self-line(self-trace line):[self] [treis] [lain]色线sound chart(bar sheets):[tʃɑ:t]:[ʃi:t]音节表special effect:['speʃəl] :[i'fekt]特效spin:[spin]旋转T.A.(top aux):auxiliary[ɔ:g'ziljəri]上辅助定位T.P.(top pegs):[peg]上定位track:[træk]声带turns:[tə:n]转向take:[teik]拍摄(一般指拍摄顺序)truck in:[trʌk]镜头推人truck out:[aut]镜头拉出tr(trace):[treis]同描tapers:['teipə]渐taper-up:[ʌp]渐快taper-down:[daun]渐慢tight field:[tait] :[fi:ld]小安全框tap(beat):[tæp]:[bi:t]节拍title:['taitl]片名;字幕Ul(underlay):['ʌndəlei]中景;后景up:[ʌp]上面use:[ju:z]用vert up:[və:t]垂直上移V.O.(voice over):[vɔis] :['əuvə]旁白;画外音value:['vælju:]明暗度wipe:[waip]转(换)景方式work print:[wə:k][print]工作样片X(x-diss)(x.d.):[dis]两景交融xerox down缩小xerox up(xerox paste-ups):[peist]放大x-sheet:[ʃi:t]摄影表zoom out:[zu:m]拉出zoon chart::['zəuɔn][tʃɑ:t]镜头推拉轨迹zoom in推进zoom lens:[lenz]变焦距镜头Good afternoon teacherIt is really my honor to have this opportunity for introduce myselfI hope I can make a good performance today.I'm confident that I can succeed Now I will introduce myself brieflyMy name is BLUE I was born in Handan is south of Hubei.Handan is not a big city but it has long history, it can date back to QIN dynastyIt's really a fantasy place too.People there are very friendly and helpful.There're also some places of interests in my hometown, I love it and hope that you can visited it someday.There’re 5 people in my family.My parents one sister one brother and I.I’mthe youngest in my family.We love each other and livea happy life.I usually love basketball.But I’m not good at it, because I’m not enough tall and powerMy major is animation design, I love animation very much.I want to be a master of animation.Hayao Miyazaki is my idol, he is a Japanese mange artist and prominent film director and h animator of many popular anime feature films.He has been named one of the most influential people by Time Magazine.So I want to go to Japan to continue my education I has already to learn Japanese for one year.As a school animation student I am serious and responsible, good workIn a lot of study and practice, my ability to learn, work, innovations have been greatly improved;but I know that not enough, in the future still need to create, I will do better.I believe myselfSo, you see, I’m really an easygoing gal.That's all, thank you分享版本,希望大家珍惜这份词汇表字母音标班附表美语音标共51个,其中元音(韵母)22个,辅音(声母)29个。
多特征检测耦合混沌映射的红外图像加密算法
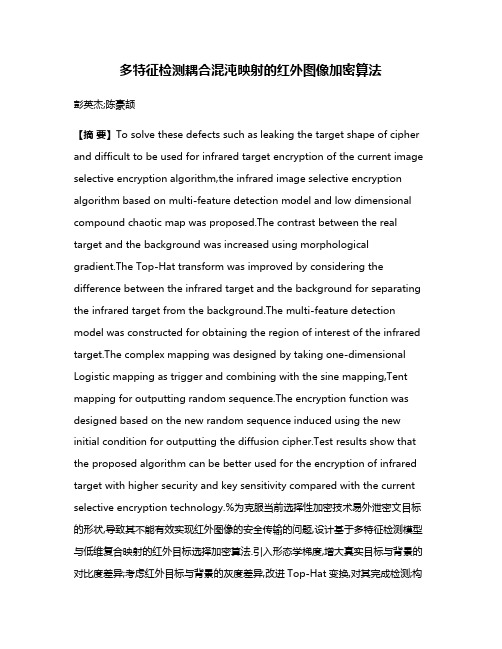
多特征检测耦合混沌映射的红外图像加密算法彭英杰;陈豪颉【摘要】To solve these defects such as leaking the target shape of cipher and difficult to be used for infrared target encryption of the current image selective encryption algorithm,the infrared image selective encryption algorithm based on multi-feature detection model and low dimensional compound chaotic map was proposed.The contrast between the real target and the background was increased using morphological gradient.The Top-Hat transform was improved by considering the difference between the infrared target and the background for separating the infrared target from the background.The multi-feature detection model was constructed for obtaining the region of interest of the infrared target.The complex mapping was designed by taking one-dimensional Logistic mapping as trigger and combining with the sine mapping,Tent mapping for outputting random sequence.The encryption function was designed based on the new random sequence induced using the new initial condition for outputting the diffusion cipher.Test results show that the proposed algorithm can be better used for the encryption of infrared target with higher security and key sensitivity compared with the current selective encryption technology.%为克服当前选择性加密技术易外泄密文目标的形状,导致其不能有效实现红外图像的安全传输的问题,设计基于多特征检测模型与低维复合映射的红外目标选择加密算法.引入形态学梯度,增大真实目标与背景的对比度差异;考虑红外目标与背景的灰度差异,改进Top-Hat变换,对其完成检测;构建多特征检测模型,获取包含红外目标的感兴趣区域;将一雏Logistic映射作为触发器,联合sine映射、Tent映射,设计复合映射,改变感兴趣区域内的像素位置;改变复合映射的初值,输出新的混沌数组,设计加密函数,输出扩散密文.测试结果表明,与当前选择性加密技术相比,该算法能够更好地用于红外目标的加密,且其安全性更高.【期刊名称】《计算机工程与设计》【年(卷),期】2017(038)011【总页数】7页(P3099-3105)【关键词】红外图像;选择性加密;低维复合映射;Top-Hat变换;多特征检测模型;感兴趣区域;形态学梯度【作者】彭英杰;陈豪颉【作者单位】青海民族大学计算机学院,青海西宁810007;兰州理工大学计算机与通信学院,甘肃兰州730050【正文语种】中文【中图分类】TP391.4传统的数据保密技术没有考虑图像的大数据容量与高的冗余度等特性,导致其难以确保加密图像进行安全传输[1-5]。
民间文学文本命名实体识别方法

第 22卷第 10期2023年 10月Vol.22 No.10Oct.2023软件导刊Software Guide民间文学文本命名实体识别方法黄健钰1,2,王笳辉1,2,段亮1,2,冉苒3(1.云南大学信息学院;2.云南省智能系统与计算重点实验室;3.云南大学文学院,云南昆明 650500)摘要:民间文学文本命名实体识别任务旨在从民间文学文本中判别实体并将其划分到预定义的语义类别,为民间文学的保存与传播奠定基础。
民间文学区别于一般中文语料,其文本存在一词多义情况突出与领域名词众多的问题,导致常规命名实体识别方法难以准确充分地识别出民间文学文本中存在的实体及其类别。
针对该问题,提出一种基于BERT的民间文学文本命名实体识别模型TBERT。
该模型首先在通用中文BERT模型的基础上融合民间文学文本语料特征与实体类型特征;然后利用BiLSTM模型进一步提取序列依赖特征;最后结合CRF模型获取的标签约束信息输出全局最优结果。
实验结果表明,该方法在民间文学文本数据集上具有良好表现。
关键词:民间文学文本;命名实体识别;Fine-Tune;TBERT-BiLSTM-CRF;特征融合DOI:10.11907/rjdk.222235开放科学(资源服务)标识码(OSID):中图分类号:TP391.1 文献标识码:A文章编号:1672-7800(2023)010-0065-08Named Entity Recognition Method for Folk Literature TextsHUANG Jianyu1,2, WANG Jiahui1,2, DUAN Liang1,2, RAN Ran3(1.School of Information Science and Engineering, Yunnan University;2.Yunnan Key Laboratory of Intelligent Systems and Com⁃puting;3.School of Chinese Language and Literature, Yunnan University, Kunming 650500, China)Abstract:The task of identifying named entities in folklore texts aims to identify entities from folklore texts and classify them into predefined semantic categories, laying the foundation for the preservation and dissemination of folklore. Folk literature is different from general Chinese corpus in that its text has prominent polysemy and numerous domain nouns, which makes it difficult for conventional named entity recognition methods to accurately and fully identify the entities and their categories present in folk literature texts. To address this issue, a folk literature text named entity recognition model TBERT based on BERT is proposed. This model first integrates the corpus features and entity type features of folk literature texts on the basis of the universal Chinese BERT model; Then, the BiLSTM model is used to further extract sequence depen⁃dent features; Finally, combine the label constraint information obtained from the CRF model to output the global optimal result. The experi⁃mental results show that this method performs well on the dataset of folk literature texts.Key Words:folk literature texts; named entity recognition; Fine-Tune; TBERT-BiLSTM-CRF; feature fusion0 引言民间文学是由人民群众以口头方式创作并传播,且经过不断集体修改与加工的文学,常以民间传说、民间故事、神话诗歌等形式存在。
混合词汇特征和lda的语义相关度计算方法
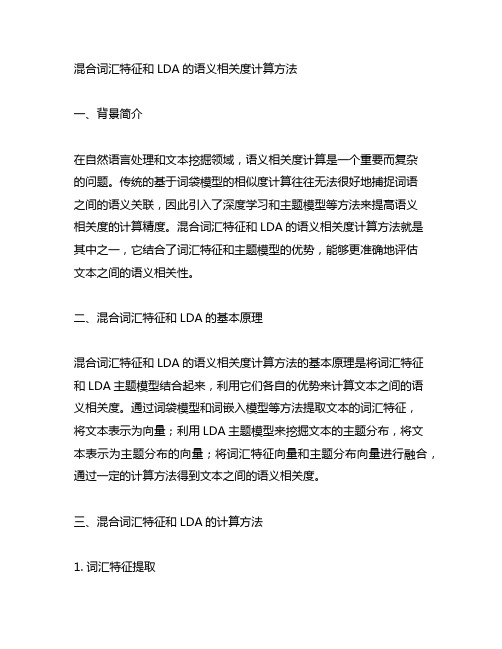
混合词汇特征和LDA的语义相关度计算方法一、背景简介在自然语言处理和文本挖掘领域,语义相关度计算是一个重要而复杂的问题。
传统的基于词袋模型的相似度计算往往无法很好地捕捉词语之间的语义关联,因此引入了深度学习和主题模型等方法来提高语义相关度的计算精度。
混合词汇特征和LDA的语义相关度计算方法就是其中之一,它结合了词汇特征和主题模型的优势,能够更准确地评估文本之间的语义相关性。
二、混合词汇特征和LDA的基本原理混合词汇特征和LDA的语义相关度计算方法的基本原理是将词汇特征和LDA主题模型结合起来,利用它们各自的优势来计算文本之间的语义相关度。
通过词袋模型和词嵌入模型等方法提取文本的词汇特征,将文本表示为向量;利用LDA主题模型来挖掘文本的主题分布,将文本表示为主题分布的向量;将词汇特征向量和主题分布向量进行融合,通过一定的计算方法得到文本之间的语义相关度。
三、混合词汇特征和LDA的计算方法1. 词汇特征提取词汇特征提取是语义相关度计算的基础,包括词袋模型、TF-IDF、词嵌入等方法。
在混合词汇特征和LDA的计算方法中,可以使用词袋模型将文本表示为词频向量,也可以利用词嵌入模型将词语转换为稠密的向量表示。
这些词汇特征能够捕捉文本中词语的语义信息,为后续的语义相关度计算奠定了基础。
2. LDA主题模型LDA主题模型是一种用于挖掘文本主题分布的概率生成模型,能够将文本表示为主题分布的向量。
在混合词汇特征和LDA的计算方法中,利用LDA主题模型可以发现文本隐含的语义主题,从而更好地表征文本的语义信息。
3. 混合计算方法混合词汇特征和LDA的计算方法采用了词汇特征向量和主题分布向量的融合策略,常见的计算方法包括余弦相似度、欧氏距离等。
这些方法能够将词汇特征和主题信息进行有效地整合,得到文本之间的语义相关度。
四、实际应用与案例分析混合词汇特征和LDA的语义相关度计算方法在文本相似度计算、信息检索、推荐系统等领域有着广泛的应用。
基于多级全局信息传递模型的视觉显著性检测
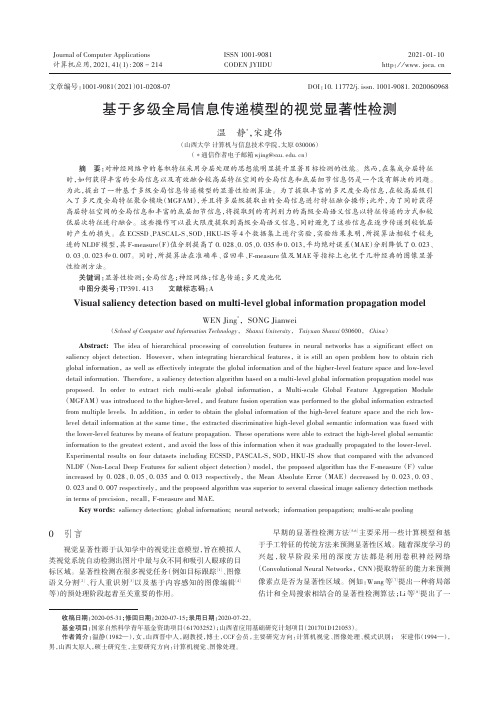
2021⁃01⁃10计算机应用,Journal of Computer Applications 2021,41(1):208-214ISSN 1001⁃9081CODEN JYIIDU http ://基于多级全局信息传递模型的视觉显著性检测温静*,宋建伟(山西大学计算机与信息技术学院,太原030006)(∗通信作者电子邮箱wjing@ )摘要:对神经网络中的卷积特征采用分层处理的思想能明显提升显著目标检测的性能。
然而,在集成分层特征时,如何获得丰富的全局信息以及有效融合较高层特征空间的全局信息和底层细节信息仍是一个没有解决的问题。
为此,提出了一种基于多级全局信息传递模型的显著性检测算法。
为了提取丰富的多尺度全局信息,在较高层级引入了多尺度全局特征聚合模块(MGFAM ),并且将多层级提取出的全局信息进行特征融合操作;此外,为了同时获得高层特征空间的全局信息和丰富的底层细节信息,将提取到的有判别力的高级全局语义信息以特征传递的方式和较低层次特征进行融合。
这些操作可以最大限度提取到高级全局语义信息,同时避免了这些信息在逐步传递到较低层时产生的损失。
在ECSSD 、PASCAL -S 、SOD 、HKU -IS 等4个数据集上进行实验,实验结果表明,所提算法相较于较先进的NLDF 模型,其F -measure (F )值分别提高了0.028、0.05、0.035和0.013,平均绝对误差(MAE )分别降低了0.023、0.03、0.023和0.007。
同时,所提算法在准确率、召回率、F -measure 值及MAE 等指标上也优于几种经典的图像显著性检测方法。
关键词:显著性检测;全局信息;神经网络;信息传递;多尺度池化中图分类号:TP391.413文献标志码:AVisual saliency detection based on multi -level global information propagation modelWEN Jing *,SONG Jianwei(School of Computer and Information Technology ,Shanxi University ,Taiyuan Shanxi 030600,China )Abstract:The idea of hierarchical processing of convolution features in neural networks has a significant effect onsaliency object detection.However ,when integrating hierarchical features ,it is still an open problem how to obtain rich global information ,as well as effectively integrate the global information and of the higher -level feature space and low -leveldetail information.Therefore ,a saliency detection algorithm based on a multi -level global information propagation model was proposed.In order to extract rich multi -scale global information ,a Multi -scale Global Feature Aggregation Module(MGFAM )was introduced to the higher -level ,and feature fusion operation was performed to the global information extracted from multiple levels.In addition ,in order to obtain the global information of the high -level feature space and the rich low -level detail information at the same time ,the extracted discriminative high -level global semantic information was fused with the lower -level features by means of feature propagation.These operations were able to extract the high -level global semantic information to the greatest extent ,and avoid the loss of this information when it was gradually propagated to the lower -level.Experimental results on four datasets including ECSSD ,PASCAL -S ,SOD ,HKU -IS show that compared with the advanced NLDF (Non -Local Deep Features for salient object detection )model ,the proposed algorithm has the F -measure (F )valueincreased by 0.028、0.05、0.035and 0.013respectively ,the Mean Absolute Error (MAE )decreased by 0.023、0.03、0.023and 0.007respectively ,and the proposed algorithm was superior to several classical image saliency detection methods in terms of precision ,recall ,F -measure and MAE.Key words:saliency detection;global information;neural network;information propagation;multi -scale pooling引言视觉显著性源于认知学中的视觉注意模型,旨在模拟人类视觉系统自动检测出图片中最与众不同和吸引人眼球的目标区域。
特征更新的动态图卷积表面损伤点云分割方法

第41卷 第4期吉林大学学报(信息科学版)Vol.41 No.42023年7月Journal of Jilin University (Information Science Edition)July 2023文章编号:1671⁃5896(2023)04⁃0621⁃10特征更新的动态图卷积表面损伤点云分割方法收稿日期:2022⁃09⁃21基金项目:国家自然科学基金资助项目(61573185)作者简介:张闻锐(1998 ),男,江苏扬州人,南京航空航天大学硕士研究生,主要从事点云分割研究,(Tel)86⁃188****8397(E⁃mail)839357306@;王从庆(1960 ),男,南京人,南京航空航天大学教授,博士生导师,主要从事模式识别与智能系统研究,(Tel)86⁃130****6390(E⁃mail)cqwang@㊂张闻锐,王从庆(南京航空航天大学自动化学院,南京210016)摘要:针对金属部件表面损伤点云数据对分割网络局部特征分析能力要求高,局部特征分析能力较弱的传统算法对某些数据集无法达到理想的分割效果问题,选择采用相对损伤体积等特征进行损伤分类,将金属表面损伤分为6类,提出一种包含空间尺度区域信息的三维图注意力特征提取方法㊂将得到的空间尺度区域特征用于特征更新网络模块的设计,基于特征更新模块构建出了一种特征更新的动态图卷积网络(Feature Adaptive Shifting⁃Dynamic Graph Convolutional Neural Networks)用于点云语义分割㊂实验结果表明,该方法有助于更有效地进行点云分割,并提取点云局部特征㊂在金属表面损伤分割上,该方法的精度优于PointNet ++㊁DGCNN(Dynamic Graph Convolutional Neural Networks)等方法,提高了分割结果的精度与有效性㊂关键词:点云分割;动态图卷积;特征更新;损伤分类中图分类号:TP391.41文献标志码:A Cloud Segmentation Method of Surface Damage Point Based on Feature Adaptive Shifting⁃DGCNNZHANG Wenrui,WANG Congqing(School of Automation,Nanjing University of Aeronautics and Astronautics,Nanjing 210016,China)Abstract :The cloud data of metal part surface damage point requires high local feature analysis ability of the segmentation network,and the traditional algorithm with weak local feature analysis ability can not achieve the ideal segmentation effect for the data set.The relative damage volume and other features are selected to classify the metal surface damage,and the damage is divided into six categories.This paper proposes a method to extract the attention feature of 3D map containing spatial scale area information.The obtained spatial scale area feature is used in the design of feature update network module.Based on the feature update module,a feature updated dynamic graph convolution network is constructed for point cloud semantic segmentation.The experimental results show that the proposed method is helpful for more effective point cloud segmentation to extract the local features of point cloud.In metal surface damage segmentation,the accuracy of this method is better than pointnet++,DGCNN(Dynamic Graph Convolutional Neural Networks)and other methods,which improves the accuracy and effectiveness of segmentation results.Key words :point cloud segmentation;dynamic graph convolution;feature adaptive shifting;damage classification 0 引 言基于深度学习的图像分割技术在人脸㊁车牌识别和卫星图像分析领域已经趋近成熟,为获取物体更226吉林大学学报(信息科学版)第41卷完整的三维信息,就需要利用三维点云数据进一步完善语义分割㊂三维点云数据具有稀疏性和无序性,其独特的几何特征分布和三维属性使点云语义分割在许多领域的应用都遇到困难㊂如在机器人与计算机视觉领域使用三维点云进行目标检测与跟踪以及重建;在建筑学上使用点云提取与识别建筑物和土地三维几何信息;在自动驾驶方面提供路面交通对象㊁道路㊁地图的采集㊁检测和分割功能㊂2017年,Lawin等[1]将点云投影到多个视图上分割再返回点云,在原始点云上对投影分割结果进行分析,实现对点云的分割㊂最早的体素深度学习网络产生于2015年,由Maturana等[2]创建的VOXNET (Voxel Partition Network)网络结构,建立在三维点云的体素表示(Volumetric Representation)上,从三维体素形状中学习点的分布㊂结合Le等[3]提出的点云网格化表示,出现了类似PointGrid的新型深度网络,集成了点与网格的混合高效化网络,但体素化的点云面对大量点数的点云文件时表现不佳㊂在不规则的点云向规则的投影和体素等过渡态转换过程中,会出现很多空间信息损失㊂为将点云自身的数据特征发挥完善,直接输入点云的基础网络模型被逐渐提出㊂2017年,Qi等[4]利用点云文件的特性,开发了直接针对原始点云进行特征学习的PointNet网络㊂随后Qi等[5]又提出了PointNet++,针对PointNet在表示点与点直接的关联性上做出改进㊂Hu等[6]提出SENET(Squeeze⁃and⁃Excitation Networks)通过校准通道响应,为三维点云深度学习引入通道注意力网络㊂2018年,Li等[7]提出了PointCNN,设计了一种X⁃Conv模块,在不显著增加参数数量的情况下耦合较远距离信息㊂图卷积网络[8](Graph Convolutional Network)是依靠图之间的节点进行信息传递,获得图之间的信息关联的深度神经网络㊂图可以视为顶点和边的集合,使每个点都成为顶点,消耗的运算量是无法估量的,需要采用K临近点计算方式[9]产生的边缘卷积层(EdgeConv)㊂利用中心点与其邻域点作为边特征,提取边特征㊂图卷积网络作为一种点云深度学习的新框架弥补了Pointnet等网络的部分缺陷[10]㊂针对非规律的表面损伤这种特征缺失类点云分割,人们已经利用各种二维图像采集数据与卷积神经网络对风扇叶片㊁建筑和交通工具等进行损伤检测[11],损伤主要类别是裂痕㊁表面漆脱落等㊂但二维图像分割涉及的损伤种类不够充分,可能受物体表面污染㊁光线等因素影响,将凹陷㊁凸起等损伤忽视,或因光照不均匀判断为脱漆㊂笔者提出一种基于特征更新的动态图卷积网络,主要针对三维点云分割,设计了一种新型的特征更新模块㊂利用三维点云独特的空间结构特征,对传统K邻域内权重相近的邻域点采用空间尺度进行区分,并应用于对金属部件表面损伤分割的有用与无用信息混杂的问题研究㊂对邻域点进行空间尺度划分,将注意力权重分组,组内进行特征更新㊂在有效鉴别外邻域干扰特征造成的误差前提下,增大特征提取面以提高局部区域特征有用性㊂1 深度卷积网络计算方法1.1 包含空间尺度区域信息的三维图注意力特征提取方法由迭代最远点采集算法将整片点云分割为n个点集:{M1,M2,M3, ,M n},每个点集包含k个点:{P1, P2,P3, ,P k},根据点集内的空间尺度关系,将局部区域划分为不同的空间区域㊂在每个区域内,结合局部特征与空间尺度特征,进一步获得更有区分度的特征信息㊂根据注意力机制,为K邻域内的点分配不同的权重信息,特征信息包括空间区域内点的分布和区域特性㊂将这些特征信息加权计算,得到点集的卷积结果㊂使用空间尺度区域信息的三维图注意力特征提取方式,需要设定合适的K邻域参数K和空间划分层数R㊂如果K太小,则会导致弱分割,因不能完全利用局部特征而影响结果准确性;如果K太大,会增加计算时间与数据量㊂图1为缺损损伤在不同参数K下的分割结果图㊂由图1可知,在K=30或50时,分割结果效果较好,K=30时计算量较小㊂笔者选择K=30作为实验参数㊂在分析确定空间划分层数R之前,简要分析空间层数划分所应对的问题㊂三维点云所具有的稀疏性㊁无序性以及损伤点云自身噪声和边角点多的特性,导致了点云处理中可能出现的共同缺点,即将离群值点云选为邻域内采样点㊂由于损伤表面多为一个面,被分割出的损伤点云应在该面上分布,而噪声点则被分布在整个面的两侧,甚至有部分位于损伤内部㊂由于点云噪声这种立体分布的特征,导致了离群值被选入邻域内作为采样点存在㊂根据采用DGCNN(Dynamic Graph Convolutional Neural Networks)分割网络抽样实验结果,位于切面附近以及损伤内部的离群值点对点云分割结果造成的影响最大,被错误分割为特征点的几率最大,在后续预处理过程中需要对这种噪声点进行优先处理㊂图1 缺损损伤在不同参数K 下的分割结果图Fig.1 Segmentation results of defect damage under different parameters K 基于上述实验结果,在参数K =30情况下,选择空间划分层数R ㊂缺损损伤在不同参数R 下的分割结果如图2所示㊂图2b 的结果与测试集标签分割结果更为相似,更能体现损伤的特征,同时屏蔽了大部分噪声㊂因此,选择R =4作为实验参数㊂图2 缺损损伤在不同参数R 下的分割结果图Fig.2 Segmentation results of defect damage under different parameters R 在一个K 邻域内,邻域点与中心点的空间关系和特征差异最能表现邻域点的权重㊂空间特征系数表示邻域点对中心点所在点集的重要性㊂同时,为更好区分图内邻域点的权重,需要将整个邻域细分㊂以空间尺度进行细分是较为合适的分类方式㊂中心点的K 邻域可视为一个局部空间,将其划分为r 个不同的尺度区域㊂再运算空间注意力机制,为这r 个不同区域的权重系数赋值㊂按照空间尺度多层次划分,不仅没有损失核心的邻域点特征,还能有效抑制无意义的㊁有干扰性的特征㊂从而提高了深度学习网络对点云的局部空间特征的学习能力,降低相邻邻域之间的互相影响㊂空间注意力机制如图3所示,计算步骤如下㊂第1步,计算特征系数e mk ㊂该值表示每个中心点m 的第k 个邻域点对其中心点的权重㊂分别用Δp mk 和Δf mk 表示三维空间关系和局部特征差异,M 表示MLP(Multi⁃Layer Perceptrons)操作,C 表示concat 函数,其中Δp mk =p mk -p m ,Δf mk =M (f mk )-M (f m )㊂将两者合并后输入多层感知机进行计算,得到计算特征系数326第4期张闻锐,等:特征更新的动态图卷积表面损伤点云分割方法图3 空间尺度区域信息注意力特征提取方法示意图Fig.3 Schematic diagram of attention feature extraction method for spatial scale regional information e mk =M [C (Δp mk ‖Δf mk )]㊂(1) 第2步,计算图权重系数a mk ㊂该值表示每个中心点m 的第k 个邻域点对其中心点的权重包含比㊂其中k ∈{1,2,3, ,K },K 表示每个邻域所包含点数㊂需要对特征系数e mk 进行归一化,使用归一化指数函数S (Softmax)得到权重多分类的结果,即计算图权重系数a mk =S (e mk )=exp(e mk )/∑K g =1exp(e mg )㊂(2) 第3步,用空间尺度区域特征s mr 表示中心点m 的第r 个空间尺度区域的特征㊂其中k r ∈{1,2,3, ,K r },K r 表示第r 个空间尺度区域所包含的邻域点数,并在其中加入特征偏置项b r ,避免权重化计算的特征在动态图中累计单面误差指向,空间尺度区域特征s mr =∑K r k r =1[a mk r M (f mk r )]+b r ㊂(3) 在r 个空间尺度区域上进行计算,就可得到点m 在整个局部区域的全部空间尺度区域特征s m ={s m 1,s m 2,s m 3, ,s mr },其中r ∈{1,2,3, ,R }㊂1.2 基于特征更新的动态图卷积网络动态图卷积网络是一种能直接处理原始三维点云数据输入的深度学习网络㊂其特点是将PointNet 网络中的复合特征转换模块(Feature Transform),改进为由K 邻近点计算(K ⁃Near Neighbor)和多层感知机构成的边缘卷积层[12]㊂边缘卷积层功能强大,其提取的特征不仅包含全局特征,还拥有由中心点与邻域点的空间位置关系构成的局部特征㊂在动态图卷积网络中,每个邻域都视为一个点集㊂增强对其中心点的特征学习能力,就会增强网络整体的效果[13]㊂对一个邻域点集,对中心点贡献最小的有效局部特征的边缘点,可以视为异常噪声点或低权重点,可能会给整体分割带来边缘溢出㊂点云相比二维图像是一种信息稀疏并且噪声含量更大的载体㊂处理一个局域内的噪声点,将其直接剔除或简单采纳会降低特征提取效果,笔者对其进行低权重划分,并进行区域内特征更新,增强抗噪性能,也避免点云信息丢失㊂在空间尺度区域中,在区域T 内有s 个点x 被归为低权重系数组,该点集的空间信息集为P ∈R N s ×3㊂点集的局部特征集为F ∈R N s ×D f [14],其中D f 表示特征的维度空间,N s 表示s 个域内点的集合㊂设p i 以及f i 为点x i 的空间信息和特征信息㊂在点集内,对点x i 进行小范围内的N 邻域搜索,搜索其邻域点㊂则点x i 的邻域点{x i ,1,x i ,2, ,x i ,N }∈N (x i ),其特征集合为{f i ,1,f i ,2, ,f i ,N }∈F ㊂在利用空间尺度进行区域划分后,对空间尺度区域特征s mt 较低的区域进行区域内特征更新,通过聚合函数对权重最低的邻域点在图中的局部特征进行改写㊂已知中心点m ,点x i 的特征f mx i 和空间尺度区域特征s mt ,目的是求出f ′mx i ,即中心点m 的低权重邻域点x i 在进行邻域特征更新后得到的新特征㊂对区域T 内的点x i ,∀x i ,j ∈H (x i ),x i 与其邻域H 内的邻域点的特征相似性域为R (x i ,x i ,j )=S [C (f i ,j )T C (f i ,j )/D o ],(4)其中C 表示由输入至输出维度的一维卷积,D o 表示输出维度值,T 表示转置㊂从而获得更新后的x i 的426吉林大学学报(信息科学版)第41卷特征㊂对R (x i ,x i ,j )进行聚合,并将特征f mx i 维度变换为输出维度f ′mx i =∑[R (x i ,x i ,j )S (s mt f mx i )]㊂(5) 图4为特征更新网络模块示意图,展示了上述特征更新的计算过程㊂图5为特征更新的动态图卷积网络示意图㊂图4 特征更新网络模块示意图Fig.4 Schematic diagram of feature update network module 图5 特征更新的动态图卷积网络示意图Fig.5 Flow chart of dynamic graph convolution network with feature update 动态图卷积网络(DGCNN)利用自创的边缘卷积层模块,逐层进行边卷积[15]㊂其前一层的输出都会动态地产生新的特征空间和局部区域,新一层从前一层学习特征(见图5)㊂在每层的边卷积模块中,笔者在边卷积和池化后加入了空间尺度区域注意力特征,捕捉特定空间区域T 内的邻域点,用于特征更新㊂特征更新会降低局域异常值点对局部特征的污染㊂网络相比传统图卷积神经网络能获得更多的特征信息,并且在面对拥有较多噪声值的点云数据时,具有更好的抗干扰性[16],在对性质不稳定㊁不平滑并含有需采集分割的突出中心的点云数据时,会有更好的抗干扰效果㊂相比于传统预处理方式,其稳定性更强,不会发生将突出部分误分割或漏分割的现象[17]㊂2 实验结果与分析点云分割的精度评估指标主要由两组数据构成[18],即平均交并比和总体准确率㊂平均交并比U (MIoU:Mean Intersection over Union)代表真实值和预测值合集的交并化率的平均值,其计算式为526第4期张闻锐,等:特征更新的动态图卷积表面损伤点云分割方法U =1T +1∑Ta =0p aa ∑Tb =0p ab +∑T b =0p ba -p aa ,(6)其中T 表示类别,a 表示真实值,b 表示预测值,p ab 表示将a 预测为b ㊂总体准确率A (OA:Overall Accuracy)表示所有正确预测点p c 占点云模型总体数量p all 的比,其计算式为A =P c /P all ,(7)其中U 与A 数值越大,表明点云分割网络越精准,且有U ≤A ㊂2.1 实验准备与数据预处理实验使用Kinect V2,采用Depth Basics⁃WPF 模块拍摄金属部件损伤表面获得深度图,将获得的深度图进行SDK(Software Development Kit)转化,得到pcd 格式的点云数据㊂Kinect V2采集的深度图像分辨率固定为512×424像素,为获得更清晰的数据图像,需尽可能近地采集数据㊂选择0.6~1.2m 作为采集距离范围,从0.6m 开始每次增加0.2m,获得多组采量数据㊂点云中分布着噪声,如果不对点云数据进行过滤会对后续处理产生不利影响㊂根据统计原理对点云中每个点的邻域进行分析,再建立一个特别设立的标准差㊂然后将实际点云的分布与假设的高斯分布进行对比,实际点云中误差超出了标准差的点即被认为是噪声点[19]㊂由于点云数据量庞大,为提高效率,选择采用如下改进方法㊂计算点云中每个点与其首个邻域点的空间距离L 1和与其第k 个邻域点的空间距离L k ㊂比较每个点之间L 1与L k 的差,将其中差值最大的1/K 视为可能噪声点[20]㊂计算可能噪声点到其K 个邻域点的平均值,平均值高出标准差的被视为噪声点,将离群噪声点剔除后完成对点云的滤波㊂2.2 金属表面损伤点云关键信息提取分割方法对点云损伤分割,在制作点云数据训练集时,如果只是单一地将所有损伤进行统一标记,不仅不方便进行结果分析和应用,而且也会降低特征分割的效果㊂为方便分析和控制分割效果,需要使用ArcGIS 将点云模型转化为不规则三角网TIN(Triangulated Irregular Network)㊂为精确地分类损伤,利用图6 不规则三角网模型示意图Fig.6 Schematic diagram of triangulated irregular networkTIN 的表面轮廓性质,获得训练数据损伤点云的损伤内(外)体积,损伤表面轮廓面积等㊂如图6所示㊂选择损伤体积指标分为相对损伤体积V (RDV:Relative Damege Volume)和邻域内相对损伤体积比N (NRDVR:Neighborhood Relative Damege Volume Ratio)㊂计算相对平均深度平面与点云深度网格化平面之间的部分,得出相对损伤体积㊂利用TIN 邻域网格可获取某损伤在邻域内的相对深度占比,有效解决制作测试集时,将因弧度或是形状造成的相对深度判断为损伤的问题㊂两种指标如下:V =∑P d k =1h k /P d -∑P k =1h k /()P S d ,(8)N =P n ∑P d k =1h k S d /P d ∑P n k =1h k S ()n -()1×100%,(9)其中P 表示所有点云数,P d 表示所有被标记为损伤的点云数,P n 表示所有被认定为损伤邻域内的点云数;h k 表示点k 的深度值;S d 表示损伤平面面积,S n 表示损伤邻域平面面积㊂在获取TIN 标准包络网视图后,可以更加清晰地描绘损伤情况,同时有助于量化损伤严重程度㊂笔者将损伤分为6种类型,并利用计算得出的TIN 指标进行损伤分类㊂同时,根据损伤部分体积与非损伤部分体积的关系,制定指标损伤体积(SDV:Standard Damege Volume)区分损伤类别㊂随机抽选5个测试组共50张图作为样本㊂统计非穿透损伤的RDV 绝对值,其中最大的30%标记为凹陷或凸起,其余626吉林大学学报(信息科学版)第41卷标记为表面损伤,并将样本分类的标准分界值设为SDV㊂在设立以上标准后,对凹陷㊁凸起㊁穿孔㊁表面损伤㊁破损和缺损6种金属表面损伤进行分类,金属表面损伤示意图如图7所示㊂首先,根据损伤是否产生洞穿,将损伤分为两大类㊂非贯通伤包括凹陷㊁凸起和表面损伤,贯通伤包括穿孔㊁破损和缺损㊂在非贯通伤中,凹陷和凸起分别采用相反数的SDV 作为标准,在这之间的被分类为表面损伤㊂贯通伤中,以损伤部分平面面积作为参照,较小的分类为穿孔,较大的分类为破损,而在边缘处因腐蚀㊁碰撞等原因缺角㊁内损的分类为缺损㊂分类参照如表1所示㊂图7 金属表面损伤示意图Fig.7 Schematic diagram of metal surface damage表1 损伤类别分类Tab.1 Damage classification 损伤类别凹陷凸起穿孔表面损伤破损缺损是否形成洞穿××√×√√RDV 绝对值是否达到SDV √√\×\\S d 是否达到标准\\×\√\2.3 实验结果分析为验证改进的图卷积深度神经网络在点云语义分割上的有效性,笔者采用TensorFlow 神经网络框架进行模型测试㊂为验证深度网络对损伤分割的识别准确率,采集了带有损伤特征的金属部件损伤表面点云,对点云进行预处理㊂对若干金属部件上的多个样本金属面的点云数据进行筛选,删除损伤占比低于5%或高于60%的数据后,划分并装包制作为点云数据集㊂采用CloudCompare 软件对样本金属上的损伤部分进行分类标记,共分为6种如上所述损伤㊂部件损伤的数据集制作参考点云深度学习领域广泛应用的公开数据集ModelNet40part㊂分割数据集包含了多种类型的金属部件损伤数据,这些损伤数据显示在510张总点云图像数据中㊂点云图像种类丰富,由各种包含损伤的金属表面构成,例如金属门,金属蒙皮,机械构件外表面等㊂用ArcGIS 内相关工具将总图进行随机点拆分,根据数据集ModelNet40part 的规格,每个独立的点云数据组含有1024个点,将所有总图拆分为510×128个单元点云㊂将样本分为400个训练集与110个测试集,采用交叉验证方法以保证测试的充分性[20],对多种方法进行评估测试,实验结果由单元点云按原点位置重新组合而成,并带有拆分后对单元点云进行的分割标记㊂分割结果比较如图8所示㊂726第4期张闻锐,等:特征更新的动态图卷积表面损伤点云分割方法图8 分割结果比较图Fig.8 Comparison of segmentation results在部件损伤分割的实验中,将不同网络与笔者网络(FAS⁃DGCNN:Feature Adaptive Shifting⁃Dynamic Graph Convolutional Neural Networks)进行对比㊂除了采用不同的分割网络外,其余实验均采用与改进的图卷积深度神经网络方法相同的实验设置㊂实验结果由单一损伤交并比(IoU:Intersection over Union),平均损伤交并比(MIoU),单一损伤准确率(Accuracy)和总体损伤准确率(OA)进行评价,结果如表2~表4所示㊂将6种不同损伤类别的Accuracy 与IoU 进行对比分析,可得出结论:相比于基准实验网络Pointet++,笔者在OA 和MioU 方面分别在贯通伤和非贯通伤上有10%和20%左右的提升,在整体分割指标上,OA 能达到90.8%㊂对拥有更多点数支撑,含有较多点云特征的非贯通伤,几种点云分割网络整体性能均能达到90%左右的效果㊂而不具有局部特征识别能力的PointNet 在贯通伤上的表现较差,不具备有效的分辨能力,导致分割效果相对于其他损伤较差㊂表2 损伤部件分割准确率性能对比 Tab.2 Performance comparison of segmentation accuracy of damaged parts %实验方法准确率凹陷⁃1凸起⁃2穿孔⁃3表面损伤⁃4破损⁃5缺损⁃6Ponitnet 82.785.073.880.971.670.1Pointnet++88.786.982.783.486.382.9DGCNN 90.488.891.788.788.687.1FAS⁃DGCNN 92.588.892.191.490.188.6826吉林大学学报(信息科学版)第41卷表3 损伤部件分割交并比性能对比 Tab.3 Performance comparison of segmentation intersection ratio of damaged parts %IoU 准确率凹陷⁃1凸起⁃2穿孔⁃3表面损伤⁃4破损⁃5缺损⁃6PonitNet80.582.770.876.667.366.9PointNet++86.384.580.481.184.280.9DGCNN 88.786.589.986.486.284.7FAS⁃DGCNN89.986.590.388.187.385.7表4 损伤分割的整体性能对比分析 出,动态卷积图特征以及有效的邻域特征更新与多尺度注意力给分割网络带来了更优秀的局部邻域分割能力,更加适应表面损伤分割的任务要求㊂3 结 语笔者利用三维点云独特的空间结构特征,将传统K 邻域内权重相近的邻域点采用空间尺度进行区分,并将空间尺度划分运用于邻域内权重分配上,提出了一种能将邻域内噪声点降权筛除的特征更新模块㊂采用此模块的动态图卷积网络在分割上表现出色㊂利用特征更新的动态图卷积网络(FAS⁃DGCNN)能有效实现金属表面损伤的分割㊂与其他网络相比,笔者方法在点云语义分割方面表现出更高的可靠性,可见在包含空间尺度区域信息的注意力和局域点云特征更新下,笔者提出的基于特征更新的动态图卷积网络能发挥更优秀的作用,而且相比缺乏局部特征提取能力的分割网络,其对于点云稀疏㊁特征不明显的非贯通伤有更优的效果㊂参考文献:[1]LAWIN F J,DANELLJAN M,TOSTEBERG P,et al.Deep Projective 3D Semantic Segmentation [C]∥InternationalConference on Computer Analysis of Images and Patterns.Ystad,Sweden:Springer,2017:95⁃107.[2]MATURANA D,SCHERER S.VoxNet:A 3D Convolutional Neural Network for Real⁃Time Object Recognition [C]∥Proceedings of IEEE /RSJ International Conference on Intelligent Robots and Systems.Hamburg,Germany:IEEE,2015:922⁃928.[3]LE T,DUAN Y.PointGrid:A Deep Network for 3D Shape Understanding [C]∥2018IEEE /CVF Conference on ComputerVision and Pattern Recognition (CVPR).Salt Lake City,USA:IEEE,2018:9204⁃9214.[4]QI C R,SU H,MO K,et al.PointNet:Deep Learning on Point Sets for 3D Classification and Segmentation [C]∥IEEEConference on Computer Vision and Pattern Recognition (CVPR).Hawaii,USA:IEEE,2017:652⁃660.[5]QI C R,SU H,MO K,et al,PointNet ++:Deep Hierarchical Feature Learning on Point Sets in a Metric Space [C]∥Advances in Neural Information Processing Systems.California,USA:SpringerLink,2017:5099⁃5108.[6]HU J,SHEN L,SUN G,Squeeze⁃and⁃Excitation Networks [C ]∥IEEE Conference on Computer Vision and PatternRecognition.Vancouver,Canada:IEEE,2018:7132⁃7141.[7]LI Y,BU R,SUN M,et al.PointCNN:Convolution on X⁃Transformed Points [C]∥Advances in Neural InformationProcessing Systems.Montreal,Canada:NeurIPS,2018:820⁃830.[8]ANH VIET PHAN,MINH LE NGUYEN,YEN LAM HOANG NGUYEN,et al.DGCNN:A Convolutional Neural Networkover Large⁃Scale Labeled Graphs [J].Neural Networks,2018,108(10):533⁃543.[9]任伟建,高梦宇,高铭泽,等.基于混合算法的点云配准方法研究[J].吉林大学学报(信息科学版),2019,37(4):408⁃416.926第4期张闻锐,等:特征更新的动态图卷积表面损伤点云分割方法036吉林大学学报(信息科学版)第41卷REN W J,GAO M Y,GAO M Z,et al.Research on Point Cloud Registration Method Based on Hybrid Algorithm[J]. Journal of Jilin University(Information Science Edition),2019,37(4):408⁃416.[10]ZHANG K,HAO M,WANG J,et al.Linked Dynamic Graph CNN:Learning on Point Cloud via Linking Hierarchical Features[EB/OL].[2022⁃03⁃15].https:∥/stamp/stamp.jsp?tp=&arnumber=9665104. [11]林少丹,冯晨,陈志德,等.一种高效的车体表面损伤检测分割算法[J].数据采集与处理,2021,36(2):260⁃269. LIN S D,FENG C,CHEN Z D,et al.An Efficient Segmentation Algorithm for Vehicle Body Surface Damage Detection[J]. Journal of Data Acquisition and Processing,2021,36(2):260⁃269.[12]ZHANG L P,ZHANG Y,CHEN Z Z,et al.Splitting and Merging Based Multi⁃Model Fitting for Point Cloud Segmentation [J].Journal of Geodesy and Geoinformation Science,2019,2(2):78⁃79.[13]XING Z Z,ZHAO S F,GUO W,et al.Processing Laser Point Cloud in Fully Mechanized Mining Face Based on DGCNN[J]. ISPRS International Journal of Geo⁃Information,2021,10(7):482⁃482.[14]杨军,党吉圣.基于上下文注意力CNN的三维点云语义分割[J].通信学报,2020,41(7):195⁃203. YANG J,DANG J S.Semantic Segmentation of3D Point Cloud Based on Contextual Attention CNN[J].Journal on Communications,2020,41(7):195⁃203.[15]陈玲,王浩云,肖海鸿,等.利用FL⁃DGCNN模型估测绿萝叶片外部表型参数[J].农业工程学报,2021,37(13): 172⁃179.CHEN L,WANG H Y,XIAO H H,et al.Estimation of External Phenotypic Parameters of Bunting Leaves Using FL⁃DGCNN Model[J].Transactions of the Chinese Society of Agricultural Engineering,2021,37(13):172⁃179.[16]柴玉晶,马杰,刘红.用于点云语义分割的深度图注意力卷积网络[J].激光与光电子学进展,2021,58(12):35⁃60. CHAI Y J,MA J,LIU H.Deep Graph Attention Convolution Network for Point Cloud Semantic Segmentation[J].Laser and Optoelectronics Progress,2021,58(12):35⁃60.[17]张学典,方慧.BTDGCNN:面向三维点云拓扑结构的BallTree动态图卷积神经网络[J].小型微型计算机系统,2021, 42(11):32⁃40.ZHANG X D,FANG H.BTDGCNN:BallTree Dynamic Graph Convolution Neural Network for3D Point Cloud Topology[J]. Journal of Chinese Computer Systems,2021,42(11):32⁃40.[18]张佳颖,赵晓丽,陈正.基于深度学习的点云语义分割综述[J].激光与光电子学,2020,57(4):28⁃46. ZHANG J Y,ZHAO X L,CHEN Z.A Survey of Point Cloud Semantic Segmentation Based on Deep Learning[J].Lasers and Photonics,2020,57(4):28⁃46.[19]SUN Y,ZHANG S H,WANG T Q,et al.An Improved Spatial Point Cloud Simplification Algorithm[J].Neural Computing and Applications,2021,34(15):12345⁃12359.[20]高福顺,张鼎林,梁学章.由点云数据生成三角网络曲面的区域增长算法[J].吉林大学学报(理学版),2008,46 (3):413⁃417.GAO F S,ZHANG D L,LIANG X Z.A Region Growing Algorithm for Triangular Network Surface Generation from Point Cloud Data[J].Journal of Jilin University(Science Edition),2008,46(3):413⁃417.(责任编辑:刘俏亮)。
多光谱语义分割数据集

多光谱语义分割数据集
多光谱语义分割数据集是指包含多个波段的遥感图像数据集,该数据集用于进行语义分割任务,即将不同类别的物体从图像中分割出来。
多光谱数据集通常由各种遥感传感器获取,可以包含可见光、红外线、热红外线等多个波段的图像。
这种数据集广泛应用于农业、林业、环境监测、城市规划等领域。
例如,在农业领域中,可以利用多光谱数据集进行作物的生长监测和病虫害检测;在城市规划领域中,可以利用多光谱数据集进行建筑物和道路的提取。
常用的多光谱语义分割数据集包括PASCAL VOC、COCO和ISPRS等。
这些数据集提供了大量的多光谱遥感图像,并标注了不同类别的物体。
研究人员可以利用这些数据集来训练和评估语义分割模型的性能。
ANSYS Maxwell和Simplorer新功能介绍
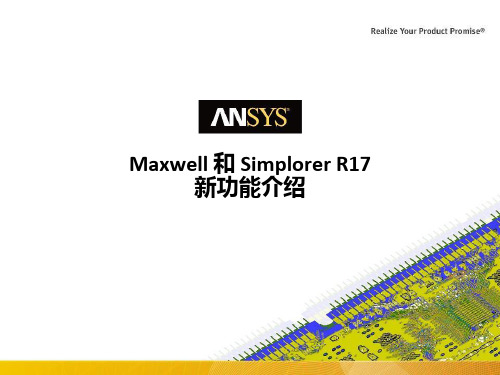
26
二维和三维涡流场求解器支持绕组设置
Winding setup is the same as transient except additional phase input
New Winding Definition
27
二维和三维涡流场求解器支持绕组设置
• R16 and before: only current sources were possible • R17: voltage source, circuit coupling available
30
Enhanced flow for Magnetization and Demagnetization
改进的充磁和退磁分析流程
Patent Pending
8
TDM 介绍
• 新方法: 沿着时间轴上的区域分解法,可以让用户不用再 一个一个时间点按序求解,而是同时求解多个时间点
t0
t1
t2
t3
t4
…
t_end
• 用户可以使用:
单个计 算单元
多个计 算单元
cluster
9
虚拟云
TDM 介绍
TDM可以用于分析2D和3D的任意瞬态磁场问题(旋转电机、变 压器等),包括:
更方便的设置初始网格中曲面曲线剖分密度用户可以使用手动设置也可以使用拖动工具条设置由于新的facetter模型处理算法r16和r17中曲面曲设置中的相同的surfacedeviationnormaldeviation和aspectratio可能会有不同的结果42新的rotationallayer网格剖分算法?应用于旋转电机?更有效计算导条里的涡流43新的cutedge剖分算法?该类型剖分的首次发布以后还会发布更多?在xy平面上增加一层网格单元44maxwell其他改进45二维瞬态分析支持multisliceapproachtosolveamachinewithskewedrotorusingseveralslicesinmaxwell2d
看明白新药如何开发溶出方法,才能做好仿制药
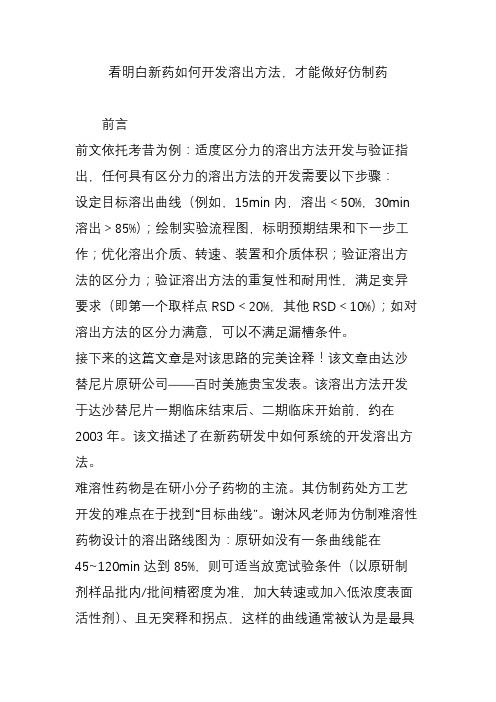
看明白新药如何开发溶出方法,才能做好仿制药前言前文依托考昔为例:适度区分力的溶出方法开发与验证指出,任何具有区分力的溶出方法的开发需要以下步骤:设定目标溶出曲线(例如,15min内,溶出<50%,30min溶出>85%);绘制实验流程图,标明预期结果和下一步工作;优化溶出介质、转速、装置和介质体积;验证溶出方法的区分力;验证溶出方法的重复性和耐用性,满足变异要求(即第一个取样点RSD<20%,其他RSD<10%);如对溶出方法的区分力满意,可以不满足漏槽条件。
接下来的这篇文章是对该思路的完美诠释!该文章由达沙替尼片原研公司——百时美施贵宝发表。
该溶出方法开发于达沙替尼片一期临床结束后、二期临床开始前,约在2003年。
该文描述了在新药研发中如何系统的开发溶出方法。
难溶性药物是在研小分子药物的主流。
其仿制药处方工艺开发的难点在于找到“目标曲线”。
谢沐风老师为仿制难溶性药物设计的溶出路线图为:原研如没有一条曲线能在45~120min达到85%,则可适当放宽试验条件(以原研制剂样品批内/批间精密度为准,加大转速或加入低浓度表面活性剂)、且无突释和拐点,这样的曲线通常被认为是最具体内外相关性,也是最理想的曲线。
这是剖析原研,找到原研的那一根“筋”,指导仿制药处方工艺的开发。
达沙替尼(Dasatanib, BMS-354825)是一种强效的酪氨酸激酶多靶点抑制剂。
为继尼洛替尼之后另一个用于伊马替尼耐药和不耐受的慢性粒细胞白血病(CML)慢性期的药物。
与针对Bcr-Abl融合蛋白单靶点的伊马替尼和尼洛替尼相比,达沙替尼属于多靶点药物,对5种关键性致癌酪氨酸蛋白激酶,即BCR-ABL、SRC、c-KIT、PDGFR和Ephrin(EPH)均有作用。
该产品最早由百时美施贵宝公司研发,2006年6月在美国获准上市,11月在欧盟上市。
我国已进口,商品名施达赛?。
国产药品为正大天晴,于2013年上市。
达沙替尼为BCSⅡ类。
SPE网络数据库使用指南
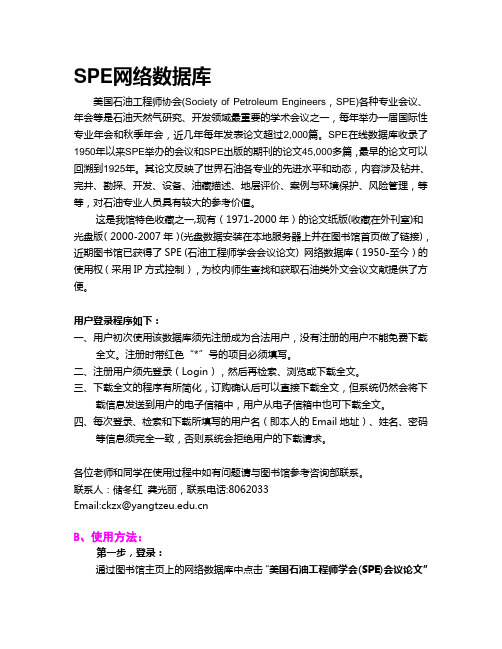
美国《石油文摘》(Petroleum Abstracts)由美国塔尔萨大学编辑加工出版,该文摘创刊于1961年。目前网络版检索系统收录了世界上28个语种(包括中文主要石油刊物)的石油专业期刊约700种,报道世界范围内关于石油、天然气等科技方面的期刊、论文集、会议录、政府报告、学位论文、专利公报出版物有参考价值的文献,主要内容涉及地质学、地球化学、地球物理、钻探、钻井、完井与维护、储运工程与回收方法、管道铺设、运输与储存、补充技术等。《石油文摘》网络版数据库现已成为石油行业中石油勘探及生产方面科技知识的全面、权威信息资源。通过《石油文摘》可以了解世界各国石油行业的专业水平和动态。
通过图书馆主页上的网络数据库中点击“美国石油工程师学会(SPE)会议论文”后面的“进入”按钮,或在浏览器的地址栏中直接输入网址:/elibrary/app/newSearch.do直接进入到检索界面
第二步,注册(Registration)和认证(Login):
登录后就成为合法用户,可以进行检索、浏览和下载全文等操作。
用户登录程序如下:
一、用户初次使用该数据库须先注册成为合法用户,没有注册的用户不能免费下载全文。注册时带红色“*”号的项目必须填写。
二、注册用户须先登录(Login),然后再检索、浏览或下载全文。
三、下载全文的程序有所简化,订购确认后可以直接下载全文,但系统仍然会将下载信息发送到用户的电子信箱中,用户从电子信箱中也可下载全文。
SPE网络数据库
美国石油工程师协会(Society of Petroleum Engineers,SPE)各种专业会议、年会等是石油天然气研究、开发领域最重要的学术会议之一,每年举办一届国际性专业年会和秋季年会,近几年每年发表论文超过2,000篇。SPE在线数据库收录了1950年以来SPE举办的会议和SPE出版的期刊的论文45,000多篇,最早的论文可以回溯到1925年。其论文反映了世界石油各专业的先进水平和动态,内容涉及钻井、完井、勘探、开发、设备、油藏描述、地层评价、案例与环境保护、风险管理,等等,对石油专业人员具有较大的参考价值。
211078226_基于多源数据与K-means聚类的地域特征关联纸质包装色彩应用研究
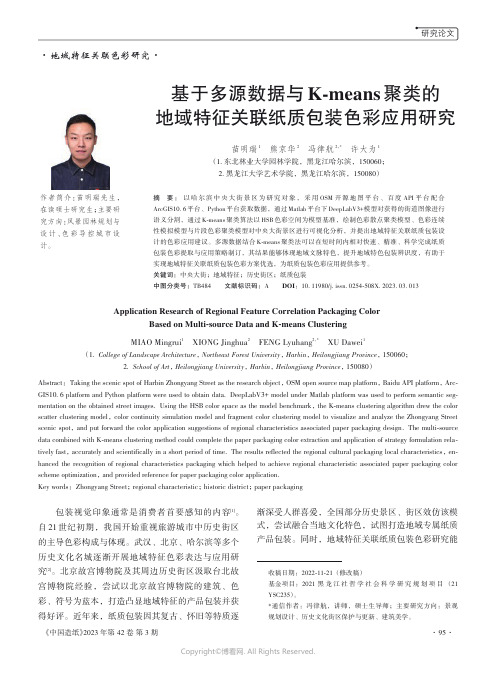
·地域特征关联色彩研究·基于多源数据与K⁃means 聚类的地域特征关联纸质包装色彩应用研究苗明瑞1 熊京华2 冯律航2,*许大为1(1. 东北林业大学园林学院,黑龙江哈尔滨,150060;2. 黑龙江大学艺术学院,黑龙江哈尔滨,150080)摘要: 以哈尔滨中央大街景区为研究对象,采用OSM 开源地图平台、百度API 平台配合ArcGIS10.6平台、Python 平台获取数据,通过Matlab 平台下DeepLabV3+模型对获得的街道图像进行语义分割,通过K -means 聚类算法以HSB 色彩空间为模型基准,绘制色彩散点聚类模型、色彩连续性模拟模型与片段色彩聚类模型对中央大街景区进行可视化分析,并提出地域特征关联纸质包装设计的色彩应用建议。
多源数据结合K -means 聚类法可以在短时间内相对快速、精准、科学完成纸质包装色彩提取与应用策略制订,其结果能够体现地域文脉特色,提升地域特色包装辨识度,有助于实现地域特征关联纸质包装色彩方案优选,为纸质包装色彩应用提供参考。
关键词:中央大街;地域特征;历史街区;纸质包装中图分类号:TB484 文献标识码:A DOI :10.11980/j.issn.0254-508X.2023.03.013Application Research of Regional Feature Correlation Packaging ColorBased on Multi -source Data and K -means ClusteringMIAO Mingrui 1 XIONG Jinghua 2 FENG Lyuhang 2,*XU Dawei 1(1. College of Landscape Architecture , Northeast Forest University , Harbin , Heilongjiang Province , 150060;2. School of Art , Heilongjiang University , Harbin , Heilongjiang Province , 150080)Abstract : Taking the scenic spot of Harbin Zhongyang Street as the research object , OSM open source map platform , Baidu API platform , Arc⁃GIS10.6 platform and Python platform were used to obtain data. DeepLabV3+ model under Matlab platform was used to perform semantic seg⁃mentation on the obtained street images. Using the HSB color space as the model benchmark , the K -means clustering algorithm drew the color scatter clustering model , color continuity simulation model and fragment color clustering model to visualize and analyze the Zhongyang Street scenic spot , and put forward the color application suggestions of regional characteristics associated paper packaging design. The multi -source data combined with K -means clustering method could complete the paper packaging color extraction and application of strategy formulation rela⁃tively fast , accurately and scientifically in a short period of time. The results reflected the regional cultural packaging local characteristics , en⁃hanced the recognition of regional characteristics packaging which helped to achieve regional characteristic associated paper packaging color scheme optimization , and provided reference for paper packaging color application.Key words : Zhongyang Street ; regional characteristic ; historic district ; paper packaging包装视觉印象通常是消费者首要感知的内容[1]。
mmsegmentation多光谱
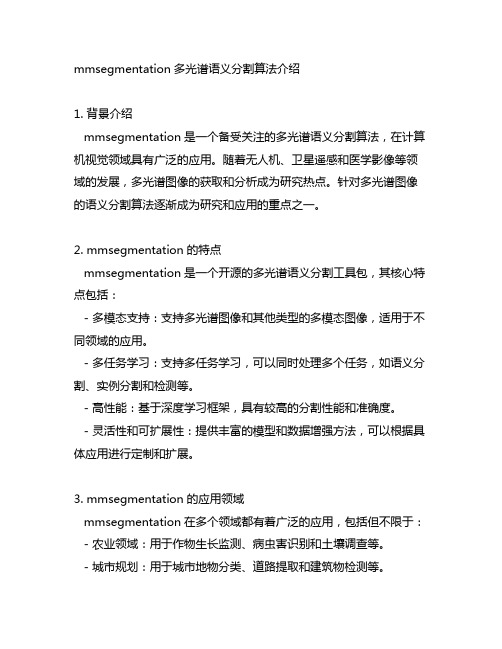
mmsegmentation多光谱语义分割算法介绍1. 背景介绍mmsegmentation是一个备受关注的多光谱语义分割算法,在计算机视觉领域具有广泛的应用。
随着无人机、卫星遥感和医学影像等领域的发展,多光谱图像的获取和分析成为研究热点。
针对多光谱图像的语义分割算法逐渐成为研究和应用的重点之一。
2. mmsegmentation的特点mmsegmentation是一个开源的多光谱语义分割工具包,其核心特点包括:- 多模态支持:支持多光谱图像和其他类型的多模态图像,适用于不同领域的应用。
- 多任务学习:支持多任务学习,可以同时处理多个任务,如语义分割、实例分割和检测等。
- 高性能:基于深度学习框架,具有较高的分割性能和准确度。
- 灵活性和可扩展性:提供丰富的模型和数据增强方法,可以根据具体应用进行定制和扩展。
3. mmsegmentation的应用领域mmsegmentation在多个领域都有着广泛的应用,包括但不限于: - 农业领域:用于作物生长监测、病虫害识别和土壤调查等。
- 城市规划:用于城市地物分类、道路提取和建筑物检测等。
- 医学影像:用于医学图像分割、病变检测和器官定位等。
- 环境监测:用于植被覆盖度评价、水体监测和土地利用分类等。
4. mmsegmentation的未来发展随着深度学习和人工智能技术的不断进步,mmsegmentation在多光谱语义分割领域仍然具有广阔的发展前景。
未来,mmsegmentation有望在以下方面取得进一步突破:- 模型优化:进一步优化算法模型,提高分割性能和鲁棒性。
- 多模态融合:研究多模态数据融合的方法,实现更全面和准确的信息提取。
- 实时应用:探索在实时场景下的应用,如自动驾驶、智能农业等。
- 应用拓展:拓展到更多新兴领域,如海洋监测、气象预测等。
总结:mmsegmentation作为一款多光谱语义分割算法,具有多模态支持、多任务学习、高性能、灵活性和可扩展性等特点。
- 1、下载文档前请自行甄别文档内容的完整性,平台不提供额外的编辑、内容补充、找答案等附加服务。
- 2、"仅部分预览"的文档,不可在线预览部分如存在完整性等问题,可反馈申请退款(可完整预览的文档不适用该条件!)。
- 3、如文档侵犯您的权益,请联系客服反馈,我们会尽快为您处理(人工客服工作时间:9:00-18:30)。
FEATURE BASED REGISTRATION OF MULTISPECTRAL DATA-CUBESA.Broersen and R.van Liere Visualization and 3D InterfacesCWIKruislaan 413,1098SJ Amsterdam,The Netherlands {a.broersen,robert.van.liere }@cwi.nlABSTRACTImaging spectroscopy can be used to scan the structure of chemical elements on the surface materials.Multiple three-dimensional datasets are created that contain the chemi-cal properties on each location of the scanned surface.A manual pixel-based registration method was used to align the data-cubes to create one large mosaic of data-cubes.In this paper,we present a feature-based method for the registration of multispectral data-cubes.A feature is de-fined as a combination of important correlated chemical el-ements in the spectral distribution.One advantage of our feature-based registration is that the presence of noise in a data-cube is greatly reduced and,therefore,will result in a more robust registration.Another advantage is that mul-tiple attempts for registration can be performed using sev-eral extracted features to improve robustness.This paper describes our registration method and the conditions to in-dicate if extracted features contain sufficient information.We also compare the performance of our method with a pixel-based registration method.KEY WORDSRegistration,PCA,and multispectral data-cubes1IntroductionAn imaging mass spectroscope is a device that can acquire spectral distributions of chemical elements on the surface of a material.The output of the mass spectroscope is a multispectral data-cube;a three-dimensional dataset with spatial properties of the surface in the xy-dimension and the spectral distribution of a combination of chemical ele-ments in the z-dimension.In contrast to a volume consist-ing of 3D points of scalar values,a spectral dataset consists of two spatial dimensions and mass-to-charge ratio in the third dimension.Each scalar value in the volume is inter-preted as the intensity on a mass-to-charge ratio at a 2D position on the surface of a material.Material scientists of-ten refer to a spectral volumetric dataset as a multispectral data-cube .Compared to a high resolution microscopic image (see Figure 1a),a spectroscopic Total Ion Count -image (TIC-image)mosaic discloses the chemical composition of surface material on each location.This TIC-image in Fig-(a)(b)Figure 1.(a)Microscopic image and (b)spectrometric TIC-image mosaic of a droplet-deposition on a silicon substrateure 1b is created by taking the sum of all spectral intensities on a single two-dimensional location.Instead of the black background in between the crystallized droplets in the mi-croscopic image,the spectroscopic image provides spectra of the material in between the crystals as false color infor-mation in the TIC-image.Recently,more advanced tech-niques for data acquisition are able to sample a larger sur-face area by capturing a number of data-cubes on a higher resolution.State-of-the-art data-cube registration methods use TIC-images to create one large mosaic of data-cubes.These methods are very similar to pixel-based registration techniques found in many image processing handbooks,for instance [1].In this paper,we present a feature-based method for the registration of multispectral data-cubes.A feature is de-fined to be one of the many chemical elements in the spec-tral distribution.Since chemical elements have a unique and known spectral profile,scientists can use spectroscopy to investigate which elements are present on the surface of a material provided their spectral profile can be extracted from the data-cube.In a previous paper,we have shown how Principle Component Analysis (PCA)can be used for extracting specific spectral profiles from multispectral data-cubes [2].In the next section,we describe the methods for se-lecting features from a data-cube,registering these features,adding additional weights to areas of high entropy,and the complete algorithm.Section 3compares our feature reg-istration method with the pixel-based method on two test collections.Finally,we conclude with a discussion of the541-148543pros and cons of our method.2MethodOur registration method can be divided into three parts.In thefirst part,the data is reduced by selecting and extract-ing the most important features from the data-cubes using PCA.Then,by applying the mean squares metric to corre-sponding features in adjacent cubes,a minimalization land-scape is constructed.This landscape represents the’fit’of the feature when two adjacent data-cubes are aligned with each other.The minima provide the regions with the most similarities.Under various conditions,however,the land-scape does not provide sufficient information to robustly assume that the lowest value in the landscape is indeed the desired solution.These conditions can be described in the third step by analyzing the entropy of the landscape and by adding a weight to all of the possible solutions.The result-ing algorithm in subsection2.4gives an overview of how a solution is found in thefinal search space.2.1Principal Component AnalysisFirst,PCA is applied to the data-cubes to reduce the data-cubes by preserving the most important features into a number of spectral and their corresponding image compo-nents.There are many methods for decomposing multidi-mensional data or dimension reduction(see[3,4,5]),but these are better suited for decomposing multi-dimensional data in many independent features instead of in only a few components that describe most of the information.PCA can be used to compress the thousands of image planes into a few image components.It selects correlated spectral and spatial features in the data-cubes which results in different components.When sorted,thefirst few components de-scribe the most variance in the data and therefore provide the most contrast in the image components.PCA is used by unfolding a non-preprocessedλby x by y data-cube in such a way that a2Dλby x×y matrix X is constructed. The standard PCA model is used to compute a sorted list of principle components in an orthonormal matrix P(see Equation1)using eigenvector decomposition.Y=P·X T(1) Thefirst principle components in P describe those spatial regions in the data-cube with the greatest spectral variance.The original data-cube is projected using the principle components as bases resulting in a matrix Y with the spatial(Y images)or spectral(Y spectra)score vectors. Both these matrices are extracted and combined to be able to select similar spectral components from two data-cubes. Once two similar spectral components can be found in both pieces of the same collection,the corresponding image components(see Figure2)can be used for registration.For example,Figure2a shows an area in yellow at the bottom left corner which contains some high intensity values.A(a)(b)Figure2.(a)One image component and(b)an other spectrally matching image componentsimilar area can be found in Figure2b in the top left corner. The similarity between both regions could be measured us-ing an appropriate similarity metric.2.2Mean SquaresAlthough many metrics and applications already exist for the registration of images[6,7,8,etc.],the most basic ap-proach is used in this method.In most cases,there are no clear defined edges or distinguishing regions present in the resulting image ndmarking or region-based registration could be problematic when applied on this type of datasets.Most of these approaches use a metric to mea-sure the difference between intensity values between two regions in two images and an optimizer to transform one of the images according to afitness value tofind a betterfit. One image is called thefixed image and the image that is being transformed the moving image.Because these spec-tral data-cubes do not contain many spatial features and could contain some noise,the complete search space has to be considered tofind a suitable minimum and a correct offset between the two images.An optimizer is no longer needed,but this is only possible when neither image is too large and-even more important-when the moving image does not need any rotational,scaling or warping transfor-mations.The most simple pixel-based similarity measure is the Mean Squares(MS)measure.The mean squared pixel-wise difference is calculated using Equation2in which A is a region in thefixed image,B a region in the moving image,N the number of pixels in these regions and i the pixel position.MS(A,B)=1Figure3.Shifting two image components of256x256pixels to create a MS landscape of511x511pixels of each overlapping re-gion tofind the correct minimum represented by the dark colored pointsresult in high values of the metric with well-defined minima and sharp peaks.The metric is sensitive to clutter,occlu-sion and non-linear changes in contrast.It is not used in this case,because it does not perform considerably better than the MS metric on these multispectral datasets.2.3EntropyLooking at the complete MS landscape on the top right in Figure3,there are still many-black-areas with low val-ues.This is mainly caused by the fact that the intensities in certain regions do not have enough contrast and/or a high amount of randomness and just simply do not contain enough information to accurately use the difference metric. If the subregions consist of only one pixel(at the corners of the MS landscape),it is practically impossible tofind an accurate metric for the similarity.Image characteristics can provide some statistical information about the information in an image(see[9]).They can give an indication offit-ness of a complete image of a region in an image relative to the other image regions.This characteristic can be used to provide a weight for the MS landscape and create a more realistic search space in order tofind the most appropriate minimum.Some commonly used image characteristics are con-trast,correlation,energy and homogeneity,but in this con-text the measure for entropy in[10]is the most suitable to act as a weight.It provides an indication of the’random-ness’of intensities in an image using its histogram.The entropy H of each region in one image component is cal-culated using Equation3,in which A is the region in an image component and A p the histogram values of A.H(A)=− p A·log p A(3) The entropy of each region of thefixed image com-ponent can be calculated and put into a landscape corre-sponding with the MS landscape from Figure3.The en-tropy landscape of the moving image component can be(a)(b)Figure4.(a)Entropy space of all regions in the image compo-nent of Figure2a and(b)the standard deviationfitted entropy of the same image componentcreated similarly and combined with thefixed entropy land-scape using the entry-by-entry product of both entropy val-ues.The resulting combined entropy landscape of the im-age components in Figure2can be found in Figure4a.This combined entropy landscape contains some outliers, mostly located at the corners where only a few pixels are being considered.An additionalfit on the histogram is made to remove unwanted outliers.This is done according to the normal distribution,by using only the mean values including the standard deviation in the histogram.The val-ues in the histogram left of this area are set to zero and the values to the right of this area are set to the remaining max-imum.The remaining landscape(see Figure4b)is applied as a weight for the MS landscape.The black regions in entropy spaces indicate that a minimum found in the corresponding regions of the MS landscape most likely will not contain enough information to be considered as a solution.Thefinal weighted search space can be created by dividing all values in the MS land-scape by thefitted entropy landscape.This results in a MSentropysearch spaces can be compared in order tofind the correct offset.1.PCA on two data-cubes from one collection of mea-surements which results in a decomposition of eachdata-cube with a number of corresponding spectraland image components.2.Select matching spectral components of both data-cubes by comparing of spectral peaks,starting fromthe components describing the most variance.3.Registration of the corresponding selected imagecomponents from both data-cubes by creating a mean 545(a)(b)Figure5.The relative locations of(a)four pieces of the crystal dataset and(b)five pieces of the kneecap datasetsquares landscape of all possible combinations of re-gions in the image components.4.Add a weight function to the MS landscape using anentropy image characteristic applied to each region of both the image components to exclude regions that contain not enough spatial information to be consid-ered as a solution.5.Select the lowest points in thefirstfive regions with lo-cal minima in the MSentropy metric which is used in the PCA-based approachto be able to quantitatively compare both approaches.The crystal dataset consists of four data-cubes resulting in six combinations,in which there is an overlap with every other data-cube.The kneecap dataset consists offive data-cubes resulting in ten combinations,in which seven combinations do have an overlap and three of them do not.The results of this registration can be found in the next subsection.All combinations in each dataset are being registered using this new PCA-based method.First,a PCA is con-ducted on each data-cube using the algorithm from subsec-tion2.4.Similar spectral components are being matched and each corresponding image component(see Figure2)is used for the registration.This results in several MS land-scapes(see Figure6)for each matching spectral component in which minima have to be found to get a most appropriate solution for a registration.The entropy space(see Figure4)of each component image is calculated and combined into the MSentropy search space of thefirst spec-trally matched components of two data-cubes.And Fig-ure7b shows the MS(a)(b)Figure6.Mean Squares landscape from comparing the(a)first and the(b)second principal component of C and E of the kneecap dataset in which the location of the correct solution for the regis-tration is marked(a)(b)Figure7.MSentropy search space could be considered for eachcombination of data-cubes.So the global minimum in the search space was used as a solution for a bestfit.ThereTIC-based array of crystalsfound foundglobalfit02-3 Table1.Summary of the results from the TIC-based approachPCA-based array of crystalsfound foundcorrectfit55-1-0 Table2.Summary of the results from the PCA-based approachwere only two correct offsets successfully found within the kneecap dataset using the global minimum in the MSentropysearch space was used and its global minimum was considered as a solution.The three com-binations in the kneecap dataset without any overlap were correctly found as indicated in the row with the’incorrect fit’.Two offsets that did exist could unfortunately not be found.This was caused by the lack of entropy in regions that should provide for a correctfit.The remaining four of six existing offsets were found and one was found,but was incorrect.This solution was found by comparing two regions without much intensity information.The joint en-tropy was not low enough to dismiss it as a possible solu-tion.4ConclusionThe results show that the PCA-based approach for the reg-istration of a collection of multispectral data-cubes is supe-rior to a traditional TIC-based method.Pixel-based regis-tration of selected image components using the MS metric with a complete coverage of the search space and an ad-ditional entropy weighting is able to correctly register two data-cubes if a solution exists.We canfind a more robust solution with the multiple minima from the landscapes of several extracted features instead of using only one pair of images.Some remarks can be made about the metrics used in this method with the possibility to improve and/or opti-mize the algorithm.4.1DiscussionThere was only one case in the kneecap dataset in which the solution found by the algorithm was incorrect.This547was mainly caused by the fact that the overlapping regions with the found minimum did not contain enough inten-sity information.Unfortunately,the entropy did not con-tribute enough as a weight to eliminate it as a solution in the MSentropy searchspace.The MS metric was not able tofind an unambiguous offset to register the TIC-images,while they can be found with the PCA-based approach.So even if some of the data-cubes do not have much overlap or useful spatial features in those overlapping regions,it is still possible to create a complete mosaic when the complete MS。