2019年外研社教学之星大赛教学设计方案
外研社教学之星大赛PPT
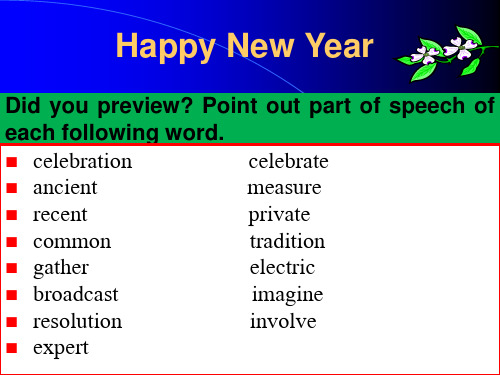
cheers from the audience. 6. The good news got __________
Happy New Year
Did you preview? Please complete each following sentence using words given.
cheer tradition
Happy New Year
Did you preview? Please complete each following sentence using words given.
cheer tradition
resolution celebrate
electric measure
involve private
Happy New Year
Question: How do Chinese spend the Spring Festival? useful words and phrases for reference
过年 spend Chinese New Year/the Spring Festival
Happy New Year
Holidays at home and abroad.
7月初七 Double-Seven’s Day is on _______. 11月1日 All Saints’ Day is on _______. The Fourth Thur in Nov Thanksgiving Day is on __________________
2019年外研社教学之星大赛(高职组)教学设计方案
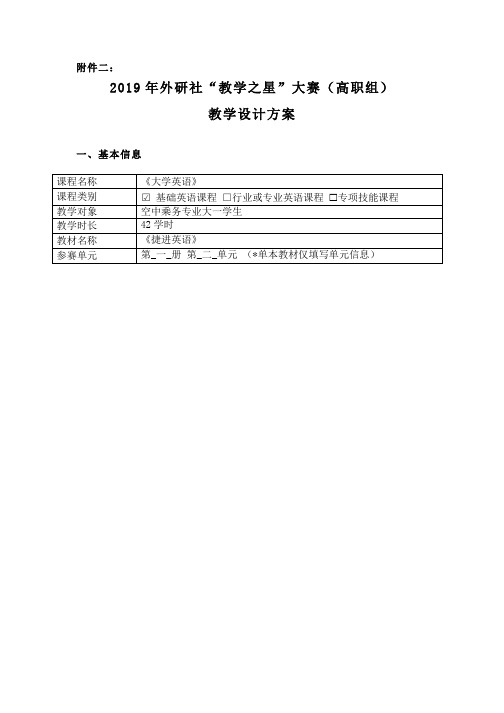
3、教学内容
“育人有温度,润物细无声”,为全面落实以德树人根本任务,本课程设计融合课程 思政理念,对教学内容进行智慧整合,将《捷进英语》第一册划分为以下七大模块:
1. 践行社会主义核心价值观,提高综合文化素养。 2. 培养岗位服务意识和社会责任意识,具备基本职业道德和岗位素质。 3. 培养批判性思维,具有较强的创新精神和思辨能力。 4. 培养跨文化交际意识和岗位交际能力,树立文化自信。 5. 培养团队协作能力,自主学习和终身学习能力。 6. 培养新时代下的信息化素养。
ü 助人为乐的美德教育:小人物大光辉
ü 精读:1.指示代词的指代对象
2.长难句分析
ü 职场思辨:空姐是否有义务帮乘客放行李? Pre-class ü 观看视频 how to offer help 学习目标句型
ü 利用 iwrite 自学感谢信的格式,写一封感谢信
Speaking 1: Help or not ü 职场小辩论 ü Offering help 句型四维梳理
人才培养定位:专业人才培养方案和行业标准对于空乘专业学生的外语语言能力和 交际水平要求较高。《大学英语》课程是我院学生必修的一门公共基础课程,本课程不仅 有助于打好坚实的语言基础,注重培养实际应用语言的技能,更要提高综合文化素质、 良好的职业品质,为提升学生的就业竞争力及未来的可持续发展打下必要的基础,从而 培养出服务交通运输行业及相关产业生产、建设、管理和服务所需的高素质技能人才。
观摩第二届外研社“教学之星”大赛的几点感受
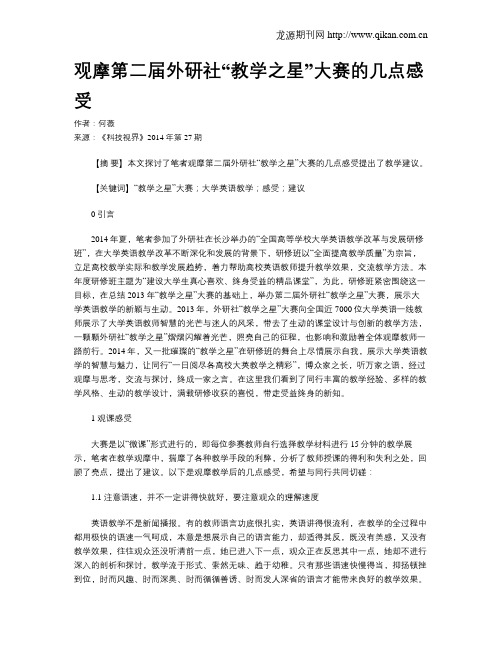
观摩第二届外研社“教学之星”大赛的几点感受
作者:何薇
来源:《科技视界》2014年第27期
【摘要】本文探讨了笔者观摩第二届外研社“教学之星”大赛的几点感受提出了教学建议。
【关键词】“教学之星”大赛;大学英语教学;感受;建议
0 引言
2014年夏,笔者参加了外研社在长沙举办的“全国高等学校大学英语教学改革与发展研修班”,在大学英语教学改革不断深化和发展的背景下,研修班以“全面提高教学质量”为宗旨,立足高校教学实际和教学发展趋势,着力帮助高校英语教师提升教学效果,交流教学方法。本年度研修班主题为“建设大学生真心喜欢、终身受益的精品课堂”,为此,研修班紧密围绕这一目标,在总结2013年“教学之星”大赛的基础上,举办第二届外研社“教学之星”大赛,展示大学英语教学的新颖与生动。2013年,外研社“教学之星”大赛向全国近7000位大学英语一线教师展示了大学英语教师智慧的光芒与迷人的风采,带去了生动的课堂设计与创新的教学方法,一颗颗外研社“教学之星”熠熠闪耀着光芒,照亮自己的征程,也影响和激励着全体观摩教师一路前行。2014年,又一批璀璨的“教学之星”在研修班的舞台上尽情展示自我,展示大学英语教学的智慧与魅力,让同行“一日阅尽各高校大英教学之精彩”,博众家之长,听万家之语,经过观摩与思考,交流与探讨,终成一家之言。在这里我们看到了同行丰富的教学经验、多样的教学风格、生动的教学设计,满载研修收获的喜悦,带走受益终身的新知。
1 观课感受
大赛是以“微课”形式进行的,即每位参赛教师自行选择教学材料进行15分钟的教学展示,笔者在教学观摩中,揣摩了各种教学手段的利弊,分析了教师授课的得利和失利之处,回顾了亮点,提出了建议。以下是观摩教学后的几点感受,希望与同行共同切磋:
2023“外研之星”全国中小学英语教学展评活动通知
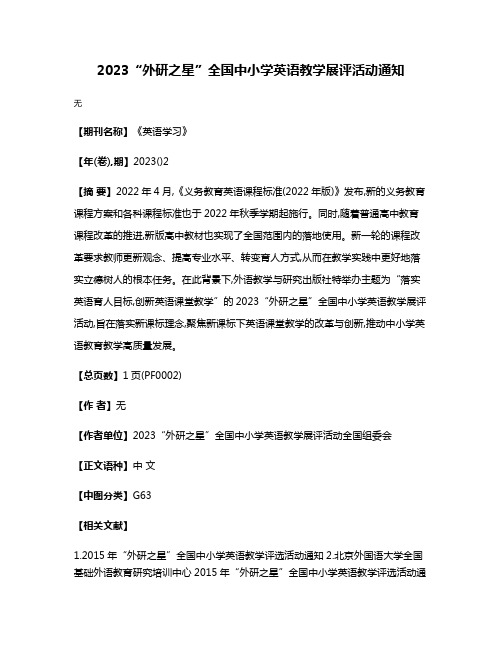
2023“外研之星”全国中小学英语教学展评活动通知
无
【期刊名称】《英语学习》
【年(卷),期】2023()2
【摘要】2022年4月,《义务教育英语课程标准(2022年版)》发布,新的义务教育课程方案和各科课程标准也于2022年秋季学期起施行。同时,随着普通高中教育课程改革的推进,新版高中教材也实现了全国范围内的落地使用。新一轮的课程改革要求教师更新观念、提高专业水平、转变育人方式,从而在教学实践中更好地落实立德树人的根本任务。在此背景下,外语教学与研究出版社特举办主题为“落实英语育人目标,创新英语课堂教学”的2023“外研之星”全国中小学英语教学展评活动,旨在落实新课标理念,聚焦新课标下英语课堂教学的改革与创新,推动中小学英语教育教学高质量发展。
【总页数】1页(PF0002)
【作者】无
【作者单位】2023“外研之星”全国中小学英语教学展评活动全国组委会
【正文语种】中文
【中图分类】G63
【相关文献】
1.2015年“外研之星”全国中小学英语教学评选活动通知
2.北京外国语大学全国基础外语教育研究培训中心2015年“外研之星”全国中小学英语教学评选活动通
知3.2015“外研之星”全国中小学英语教学设计评选初评阶段圆满结束
4.2022“外研之星”全国中小学英语教学展评活动通知
5.2022“外研之星”全国中小学英语教学展评活动通知
因版权原因,仅展示原文概要,查看原文内容请购买
基于POA产出导向法的大学英语教学设计与探析
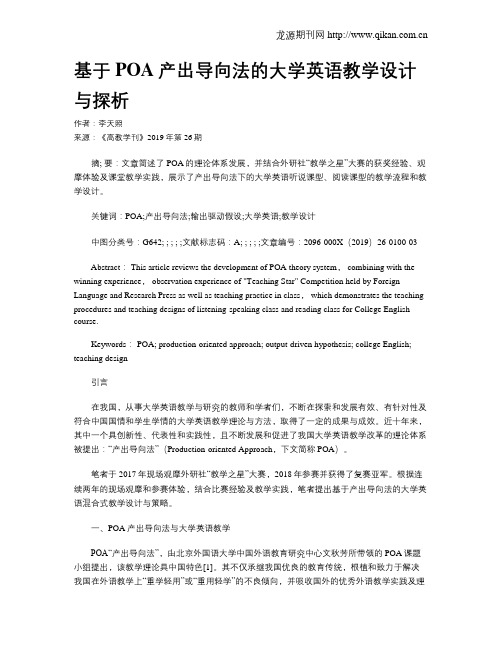
基于POA产出导向法的大学英语教学设计与探析
作者:李天照
来源:《高教学刊》2019年第26期
摘; 要:文章简述了POA的理论体系发展,并结合外研社“教学之星”大赛的获奖经验、观摩体验及课堂教学实践,展示了产出导向法下的大学英语听说课型、阅读课型的教学流程和教学设计。
关键词:POA;产出导向法;输出驱动假设;大学英语;教学设计
中图分类号:G642; ; ; ; ;文献标志码:A; ; ; ; ;文章编号:2096-000X(2019)26-0100-03
Abstract: This article reviews the development of POA theory system, combining with the winning experience, observation experience of "Teaching Star" Competition held by Foreign Language and Research Press as well as teaching practice in class, which demonstrates the teaching procedures and teaching designs of listening-speaking class and reading class for College English course.
Keywords: POA; production-oriented approach; output-driven hypothesis; college English; teaching design
第二赛场获奖名单 第二届外研社“教学之星”大赛
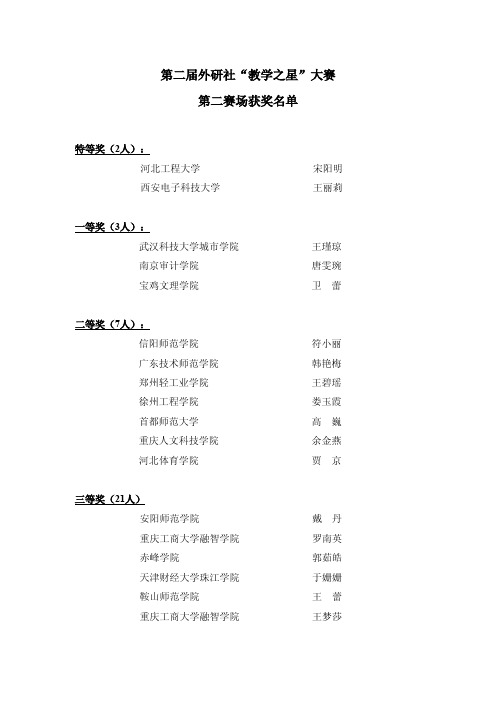
新乡医学院三全学院宋爽辽宁工程技术大学曹静北京警察学院王晨颖云南警官学院李伟西南石油大学王娜贵州师范大学王湘霁泸州医学院邓家齐阜阳师范学院靳宁东北林业大学吴晶信阳农林学院朱凌奕安徽工业大学工商学院项秀珍华东交通大学理工学院李婷长沙医学院谭立东北农业大学高延宏安徽建筑大学戴媛
基于poa产出导向法的大学英语教学设计与探析
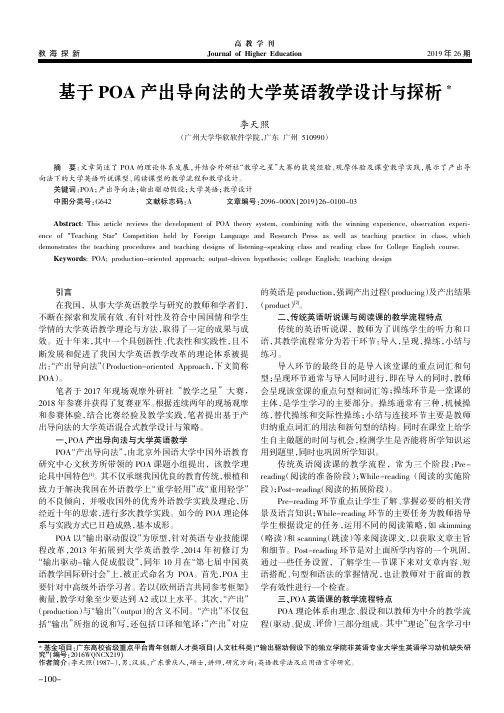
2019年26期教海探新高教学刊
基于POA产出导向法的大学英语教学设计与探析*
李天照
(广州大学华软软件学院,广东广州510990)
引言
在我国,从事大学英语教学与研究的教师和学者们,不断在探索和发展有效、有针对性及符合中国国情和学生学情的大学英语教学理论与方法,取得了一定的成果与成效。近十年来,其中一个具创新性、代表性和实践性,且不断发展和促进了我国大学英语教学改革的理论体系被提出:“产出导向法”(Production-oriented Approach,下文简称
POA)。
笔者于2017年现场观摩外研社“教学之星”大赛,2018年参赛并获得了复赛亚军。根据连续两年的现场观摩和参赛体验,结合比赛经验及教学实践,笔者提出基于产出导向法的大学英语混合式教学设计与策略。
一、POA产出导向法与大学英语教学
POA“产出导向法”,由北京外国语大学中国外语教育研究中心文秋芳所带领的POA课题小组提出,该教学理论具中国特色[1]。其不仅承继我国优良的教育传统,根植和致力于解决我国在外语教学上“重学轻用”或“重用轻学”的不良倾向,并吸收国外的优秀外语教学实践及理论,历经近十年的思索,进行多次教学实践。如今的POA理论体系与实践方式已日趋成熟,基本成形。
POA以“输出驱动假设”为原型,针对英语专业技能课程改革,2013年拓展到大学英语教学,2014年初修订为“输出驱动-输入促成假设”,同年10月在“第七届中国英语教学国际研讨会”上,被正式命名为POA。首先,POA主要针对中高级外语学习者。若以《欧州语言共同参考框架》衡量,教学对象至少要达到A2或以上水平。其次,“产出”(production)与“输出”(output)的含义不同。“产出”不仅包括“输出”所指的说和写,还包括口译和笔译;“产出”对应
外语课程教学比赛方案模板
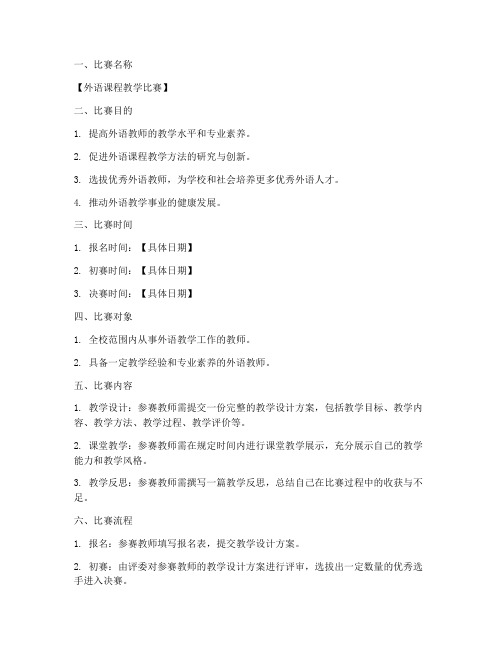
一、比赛名称
【外语课程教学比赛】
二、比赛目的
1. 提高外语教师的教学水平和专业素养。
2. 促进外语课程教学方法的研究与创新。
3. 选拔优秀外语教师,为学校和社会培养更多优秀外语人才。
4. 推动外语教学事业的健康发展。
三、比赛时间
1. 报名时间:【具体日期】
2. 初赛时间:【具体日期】
3. 决赛时间:【具体日期】
四、比赛对象
1. 全校范围内从事外语教学工作的教师。
2. 具备一定教学经验和专业素养的外语教师。
五、比赛内容
1. 教学设计:参赛教师需提交一份完整的教学设计方案,包括教学目标、教学内容、教学方法、教学过程、教学评价等。
2. 课堂教学:参赛教师需在规定时间内进行课堂教学展示,充分展示自己的教学能力和教学风格。
3. 教学反思:参赛教师需撰写一篇教学反思,总结自己在比赛过程中的收获与不足。
六、比赛流程
1. 报名:参赛教师填写报名表,提交教学设计方案。
2. 初赛:由评委对参赛教师的教学设计方案进行评审,选拔出一定数量的优秀选手进入决赛。
3. 决赛:决赛分为课堂教学展示和教学反思答辩两个环节。
4. 评审:决赛评委根据参赛教师的教学设计、课堂教学展示和教学反思进行综合评分。
5. 颁奖:对获奖选手进行表彰,颁发荣誉证书和奖品。
七、评分标准
1. 教学设计:内容完整、目标明确、方法合理、过程清晰、评价科学。
2. 课堂教学:教学过程流畅、教学手段丰富、课堂氛围活跃、学生参与度高、教学效果显著。
3. 教学反思:反思深刻、内容充实、结构完整、语言表达准确。
八、奖励措施
1. 一等奖:1名,颁发荣誉证书和奖品。
外研社教学之星江苏省特等奖比赛文稿
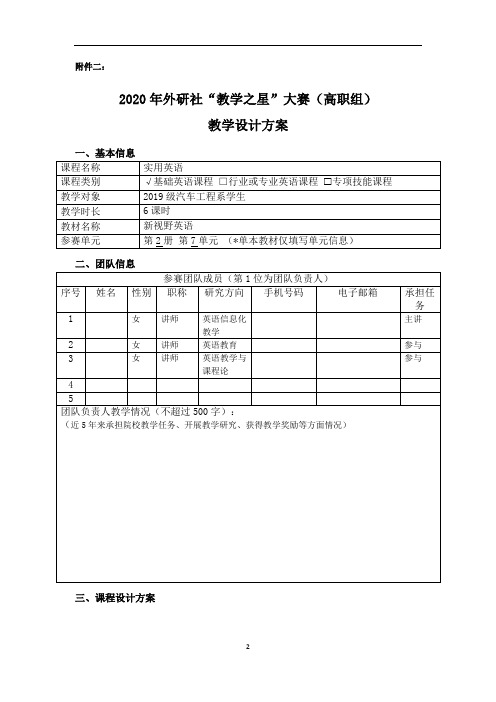
附件二:
2020年外研社“教学之星”大赛(高职组)
教学设计方案
一、基本信息
二、团队信息
三、课程设计方案
1、课程定位(基于院校特色与教学对象特点,介绍本课程的人才培养定位与设计理念)
1.1院校特色及其教学对象特点:
我校作为省22所高水平高职院建设单位,是江苏省高技能人才培养示范基地。学院历来重视对外交流与合作,积极引入国际先进职业教育理念和经验,与加拿大圣克莱尔学院合作开办“建筑装饰工程技术”和“会计”专业,加入了“海外本科直通车”项目,英国考文垂大学“3+1+1”升本升硕、马来西亚马拉工业大学“3+2”升本项目等,故对公共基础课程英语有着较高的定位和教学目标要求。学生在学习本课程之前,已经具备较高水平的英语综合语言技能,并完成《新视野英语教程 1》课程的学习,具有较扎实的英语语言文化背景知识,听说读写译能力得到有效的提升。
1.2 人才培养定位与设计理念
为了积极配合学院“培养应用型人才”的定位,本课程设计旨在进一步提升学生语言综合运用能力,尤其是语言的交际能力,同时在语言教学的过程中融入西方文化,时政新闻,社会百态等语言文化素养的题材,引导学生对西方文化有着正确的理解和认知,培养学生家国天下的国际视野,提升文化品格基调。
2、课程目标(介绍课程时长及总体目标,目标应包括语言目标与育人目标)
授课总学时:第二学期为64课时
3、课程内容(介绍课程主要内容,特别说明如何实现语言与育人的融合)
《新视野英语》课程体裁多样,贴近学生生活,关注时代热点,体现职业特色,注重信息性、实用性和趣味性。该教材具有纯正、地道、规范,内容新颖,编排合理的特点,涉及文化习俗,信息技术,社会焦点等多种学科的基本概念与相关信息。课文材料全部出自英美等国的多种刊物和杂志,与时俱进,具有较强的人文现代感。课后练习具有针对性,既注重各项能力之间的衔接和互补,也注重篇章层次上的一体化技能训练,从而更加有效地提高学生的语言应用能力。
智慧教学设计方案(smartdesign)
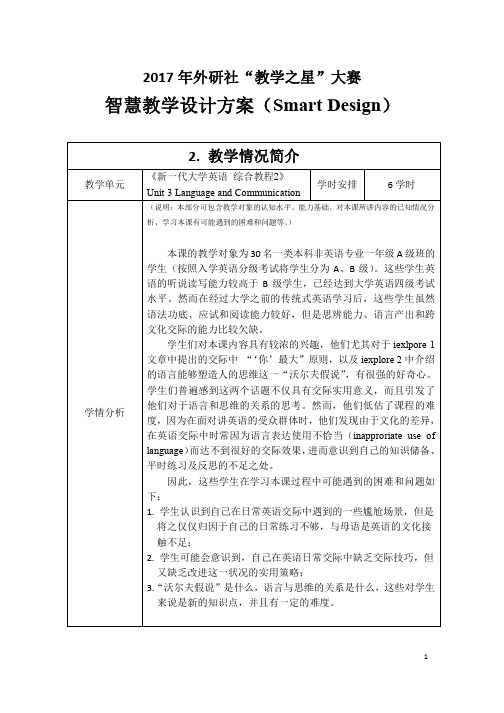
2017年外研社“教学之星”大赛
智慧教学设计方案(Smart Design)
2. 教学情况简介
教学单元《新一代大学英语 综合教程2》
Unit 3 Language and Communication
学时安排6学时
学情分析(说明:本部分可包含教学对象的认知水平、能力基础、对本课所讲内容的已知情况分析、学习本课有可能遇到的困难和问题等。)
本课的教学对象为30名一类本科非英语专业一年级A级班的学生(按照入学英语分级考试将学生分为A、B级)。这些学生英语的听说读写能力较高于B级学生,已经达到大学英语四级考试水平。然而在经过大学之前的传统式英语学习后,这些学生虽然语法功底、应试和阅读能力较好,但是思辨能力、语言产出和跨文化交际的能力比较欠缺。
学生们对本课内容具有较浓的兴趣,他们尤其对于iexlpore 1文章中提出的交际中“‘你’最大”原则,以及iexplore 2中介绍的语言能够塑造人的思维这一“沃尔夫假说”,有很强的好奇心。学生们普遍感到这两个话题不仅具有交际实用意义,而且引发了他们对于语言和思维的关系的思考。然而,他们低估了课程的难度,因为在面对讲英语的受众群体时,他们发现由于文化的差异,在英语交际中时常因为语言表达使用不恰当(inapproriate use of language)而达不到很好的交际效果,进而意识到自己的知识储备、平时练习及反思的不足之处。
因此,这些学生在学习本课过程中可能遇到的困难和问题如下:
1. 学生认识到自己在日常英语交际中遇到的一些尴尬场景,但是将之仅仅归因于自己的日常练习不够,与母语是英语的文化接触不足;
教学设计大赛实施方案
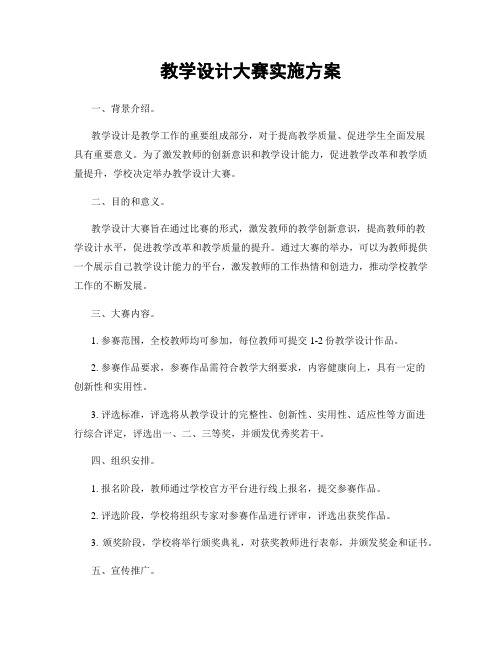
教学设计大赛实施方案
一、背景介绍。
教学设计是教学工作的重要组成部分,对于提高教学质量、促进学生全面发展
具有重要意义。为了激发教师的创新意识和教学设计能力,促进教学改革和教学质量提升,学校决定举办教学设计大赛。
二、目的和意义。
教学设计大赛旨在通过比赛的形式,激发教师的教学创新意识,提高教师的教
学设计水平,促进教学改革和教学质量的提升。通过大赛的举办,可以为教师提供一个展示自己教学设计能力的平台,激发教师的工作热情和创造力,推动学校教学工作的不断发展。
三、大赛内容。
1. 参赛范围,全校教师均可参加,每位教师可提交1-2份教学设计作品。
2. 参赛作品要求,参赛作品需符合教学大纲要求,内容健康向上,具有一定的
创新性和实用性。
3. 评选标准,评选将从教学设计的完整性、创新性、实用性、适应性等方面进
行综合评定,评选出一、二、三等奖,并颁发优秀奖若干。
四、组织安排。
1. 报名阶段,教师通过学校官方平台进行线上报名,提交参赛作品。
2. 评选阶段,学校将组织专家对参赛作品进行评审,评选出获奖作品。
3. 颁奖阶段,学校将举行颁奖典礼,对获奖教师进行表彰,并颁发奖金和证书。
五、宣传推广。
学校将通过校园公告、微信公众号、学校网站等渠道,广泛宣传教学设计大赛的相关信息,鼓励更多的教师积极参与,共同促进教学改革和提高教学质量。
六、注意事项。
1. 参赛教师需严格按照规定时间提交参赛作品,逾期作品将不予受理。
2. 参赛作品需保证原创性,不得抄袭他人作品,一经发现将取消参赛资格。
3. 参赛教师需严格遵守比赛规定,如有违规行为将取消参赛资格。
2019年外研社教学之星大赛教学设计方案
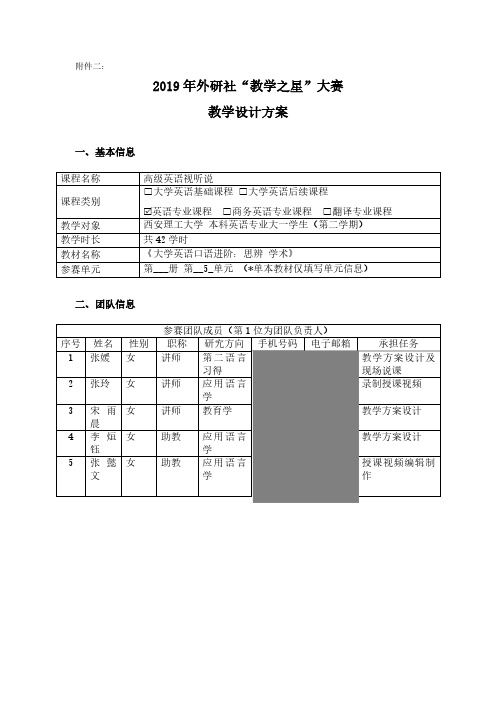
附件二:
2019年外研社“教学之星”大赛
教学设计方案
一、基本信息
二、团队信息
三、课程设计方案
1)教学准备及课前自主学习:根据每个单元的话题,教师设计出突出交际价值的教学任务和课堂输
入材料为课堂教学做准备,包括线上视频,微课,MOOC,TED视频,线下PPT,文本及听说素材;
学生按照教师的要求完成课前自主学习任务。
2)任务驱动产出:教师给学生布置“产出性任务”,包括翻转课堂、小组抢答、个人竞答、全班游
戏等多种形式的互动,引导学生自主或合作分组学习,鼓励学生在完成产出任务的过程中发现问题,解决问题,为输入性学习做准备。
3) 课堂促成选择性学习:教师制订详细的产出任务,学生在教师的指导下完成“选择性学习”,与
此同时教师发挥脚手架作用,在学生互动交流的基础上,教师提供恰当的输入材料,并对学生的产出进行正确引导与检查。这些“专家引领”的材料能够有效拓展学生的思路和现有语言体系,将产出水平推向新高度。
4) 课后评价:评价学生学习和产出任务中的学习效果,为学生提供有针对性的反馈,帮助学生调整
学习方法,提高学习效率。
输入过程性评价产出过程性评价
四、单元设计方案
⚫用英语描述“某项科学技术或产品”
在课程综合表达部分,采用“任务驱动”教学策略以及“情景化”教学模式,围绕本单元主题、语言知识和技能,设计基于内容和主题的交际活动,即模拟大学生“创新创业大赛”的展示过程──要求学生站在产品开发者的视角,用英语展示自己的科技创新产品,促使学生在解决问题的过程中综合运用已有知识和在本单元中学习到的新知识与技能完成任务,达到有效提升英语应用能力的目的,集中展示小组的“科技创新产品”,最后学生将“任务达成卡”上传至线上互动平台完成组内和组间的互评。
2015年外研社“教学之星”大赛章程(高职高专组)
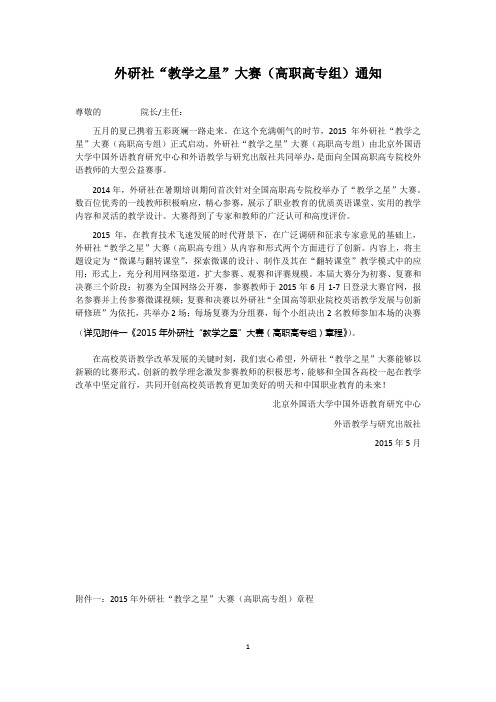
外研社“教学之星”大赛(高职高专组)通知
尊敬的院长/主任:
五月的夏已携着五彩斑斓一路走来。在这个充满朝气的时节,2015年外研社“教学之星”大赛(高职高专组)正式启动。外研社“教学之星”大赛(高职高专组)由北京外国语大学中国外语教育研究中心和外语教学与研究出版社共同举办,是面向全国高职高专院校外语教师的大型公益赛事。
2014年,外研社在暑期培训期间首次针对全国高职高专院校举办了“教学之星”大赛。数百位优秀的一线教师积极响应,精心参赛,展示了职业教育的优质英语课堂、实用的教学内容和灵活的教学设计。大赛得到了专家和教师的广泛认可和高度评价。
2015年,在教育技术飞速发展的时代背景下,在广泛调研和征求专家意见的基础上,外研社“教学之星”大赛(高职高专组)从内容和形式两个方面进行了创新。内容上,将主题设定为“微课与翻转课堂”,探索微课的设计、制作及其在“翻转课堂”教学模式中的应用;形式上,充分利用网络渠道,扩大参赛、观赛和评赛规模。本届大赛分为初赛、复赛和决赛三个阶段:初赛为全国网络公开赛,参赛教师于2015年6月1-7日登录大赛官网,报名参赛并上传参赛微课视频;复赛和决赛以外研社“全国高等职业院校英语教学发展与创新研修班”为依托,共举办2场;每场复赛为分组赛,每个小组决出2名教师参加本场的决赛
(详见附件一《2015年外研社“教学之星”大赛(高职高专组)章程》)。
在高校英语教学改革发展的关键时刻,我们衷心希望,外研社“教学之星”大赛能够以新颖的比赛形式、创新的教学理念激发参赛教师的积极思考,能够和全国各高校一起在教学改革中坚定前行,共同开创高校英语教育更加美好的明天和中国职业教育的未来!
- 1、下载文档前请自行甄别文档内容的完整性,平台不提供额外的编辑、内容补充、找答案等附加服务。
- 2、"仅部分预览"的文档,不可在线预览部分如存在完整性等问题,可反馈申请退款(可完整预览的文档不适用该条件!)。
- 3、如文档侵犯您的权益,请联系客服反馈,我们会尽快为您处理(人工客服工作时间:9:00-18:30)。
附件二:
2019年外研社“教学之星”大赛
教学设计方案
一、基本信息
二、团队信息
三、课程设计方案
1)教学准备及课前自主学习:根据每个单元的话题,教师设计出突出交际价值的教学任务和课堂输
入材料为课堂教学做准备,包括线上视频,微课,MOOC,TED视频,线下PPT,文本及听说素材;
学生按照教师的要求完成课前自主学习任务。
2)任务驱动产出:教师给学生布置“产出性任务”,包括翻转课堂、小组抢答、个人竞答、全班游
戏等多种形式的互动,引导学生自主或合作分组学习,鼓励学生在完成产出任务的过程中发现问题,解决问题,为输入性学习做准备。
3) 课堂促成选择性学习:教师制订详细的产出任务,学生在教师的指导下完成“选择性学习”,与
此同时教师发挥脚手架作用,在学生互动交流的基础上,教师提供恰当的输入材料,并对学生的产出进行正确引导与检查。这些“专家引领”的材料能够有效拓展学生的思路和现有语言体系,将产出水平推向新高度。
4) 课后评价:评价学生学习和产出任务中的学习效果,为学生提供有针对性的反馈,帮助学生调整
学习方法,提高学习效率。
输入过程性评价产出过程性评价
四、单元设计方案
⚫用英语描述“某项科学技术或产品”
在课程综合表达部分,采用“任务驱动”教学策略以及“情景化”教学模式,围绕本单元主题、语言知识和技能,设计基于内容和主题的交际活动,即模拟大学生“创新创业大赛”的展示过程──要求学生站在产品开发者的视角,用英语展示自己的科技创新产品,促使学生在解决问题的过程中综合运用已有知识和在本单元中学习到的新知识与技能完成任务,达到有效提升英语应用能力的目的,集中展示小组的“科技创新产品”,最后学生将“任务达成卡”上传至线上互动平台完成组内和组间的互评。
⚫用英语评价“某项科学技术及产品”,讨论优缺点
在用英语描述某项电子产品的基础上,引导学生从消费者和新产品投资者的视角评价某项创新产品,即客观分析产品的优缺点及研发价值。通过这种转换视角的方式,启发学生思考,发展学生的批判性思维。
2)设计理念与思路
课前导学,即线上自主学习阶段。教师为学生提供丰富高质量的自主学习材料,学生根据学习材料和教学目标完成对教学单元相关知识点的学习,并将学习情况反馈给老师,将宝贵的课堂面授时间留给更需要老师提供指导和帮助的任务上,满足学生进行个性化自主学习的需求,促使学生从“被动学习”向“主动学习”转变。体现以教师为主导、以学生为主体的教学理念,提高课堂教学效率。
课堂教学,即课堂应用实践阶段。课堂教学应是学生进行语言操练、展示学习成果的场所。因此,该阶段不仅需要教师讲解,也需要学生的积极参与,该阶段的重点放在需要教师提供有针对性指导和帮助的实践操练部分。实践操练主要针对知识重难点,知识运用和教学新内容而设定。教师需要根据学生的学习风格和特点,结合输入部分的知识,设计产出与输入紧密结合的课堂活动,使英语接受性知识转化为可用于实际英语交流的产出性知识,并培养“为用而学,以用促学,在用中学”的英语学习观念。课堂上充分利用各种教学信息技术,如Unipus、微信平台等网络平台,及时检测学生对单元
五、教学设计特色
注:本表请以“学校名称”命名,保存为PDF格式,与参赛授课录像(以“学校名称”命名)同于5月22日24:00前上传至大赛官网的相应参赛组别。
附件1:板书
附件2:
1). if条件句语法点总结
习题
2). Words and expressions in video
附件3
Advantages and disadvantages of driverless cars Advantages of Driverless Car
Accidents due to Software Error or Hacking
Driverless car drive with the help of software and we all know that software’s are not 100 percent error proof and that error in software can lead to big accidents and also people with bad intentions can hack the software and do bigger damage to both cars as well as people.
附件4
综合表达活动《任务达成表》
附件5:同伴互评表
附件6
TED Talk 文本资料
Part 1
The robot I’m holding in my hand is this one, and it’s been created by two students, Alex and Daniel. So this weighs a little more than a tenth of a pound. It consumes about 15 watts of power. And as you can see, it’s about eight inches in diameter.
[…] So why build robots like this? Well, robots like this have many applications. You can send them inside buildings like this, as first responders to look for intruders, maybe look for biochemical leaks, gaseous leaks. You can also use them for applications like construction. So here are robots carrying beams, columns and assembling cube-like structures. I’ll tell you a little bit more about this. The