mnts on Efficacy and Labeling Issues for OTC Dr
Control of a solution copolymerization reactor using multi-model predictive control
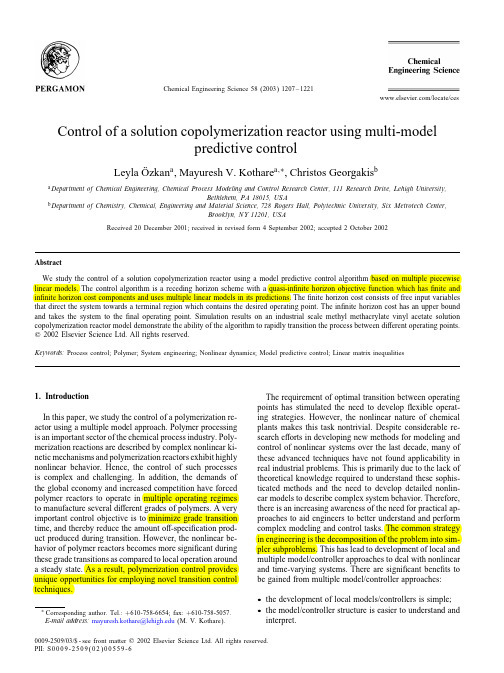
of Chemical Engineering, Chemical Process Modeling and Control Research Center, 111 Research Drive, Lehigh University, Bethlehem, PA 18015, USA b Department of Chemistry, Chemical, Engineering and Material Science, 728 Rogers Hall, Polytechnic University, Six Metrotech Center, Brooklyn, NY 11201, USA Received 20 December 2001; received in revised form 4 September 2002; accepted 2 October 2002
Corresponding author. Tel.: +610-758-6654; fax: +610-758-5057. E-mail address: mayuresh.kothare@ (M. V. Kothare).
0009-2509/03/$ - see front matter ? 2002 Elsevier Science Ltd. All rights reserved. PII: S 0 0 0 9 - 2 5 0 9 ( 0 2 ) 0 0 5 5 9 - 6
Keywords: Process control; Polymer; System engineering; Nonlinear dynamics; Model predictive control; Linear matrix inequalities
Driving forces behind the stagnancy of China’s energy-related CO2 emissions from 1996 to 1999 the r
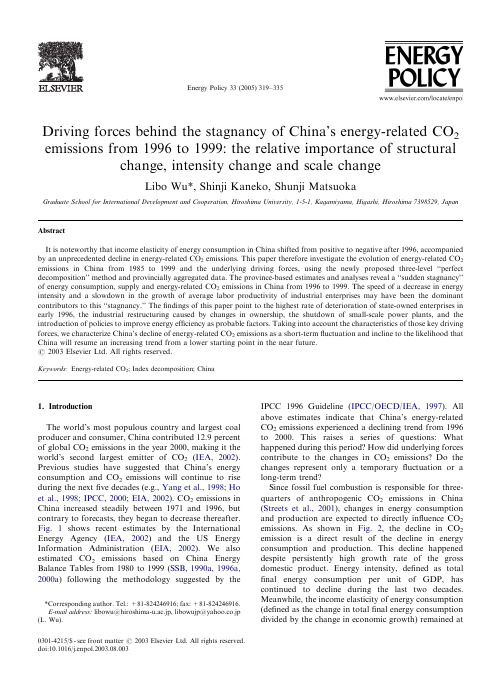
findings indicate that energy efficiency improvements in the industrial sector play the most important role in the evolution of China’s energy use; the structural shifts within the manufacturing sub-sectors or from primary to secondary or tertiary industry play only a nominal role. Such tendencies do not necessarily support continuity in the long run, however, and they do not definitely result in a sudden reversal in energy consumption trends (i.e., the decline in consumption) in the late 1990s.
Since fossil fuel combustion is responsible for threequarters of anthropogenic CO2 emissions in China (Streets et al., 2001), changes in energy consumption and production are expected to directly influence CO2 emissions. As shown in Fig. 2, the decline in CO2 emission is a direct result of the decline in energy consumption and production. This decline happened despite persistently high growth rate of the gross domestic product. Energy intensity, defined as total final energy consumption per unit of GDP, has continued to decline during the last two decades. Meanwhile, the income elasticity of energy consumption (defined as the change in total final energy consumption divided by the change in economic growth) remained at
The-impact-of-cross-border-mergers-and-acquisitions-on-the-acquirers-R-amp-D-Firm-level-evidence
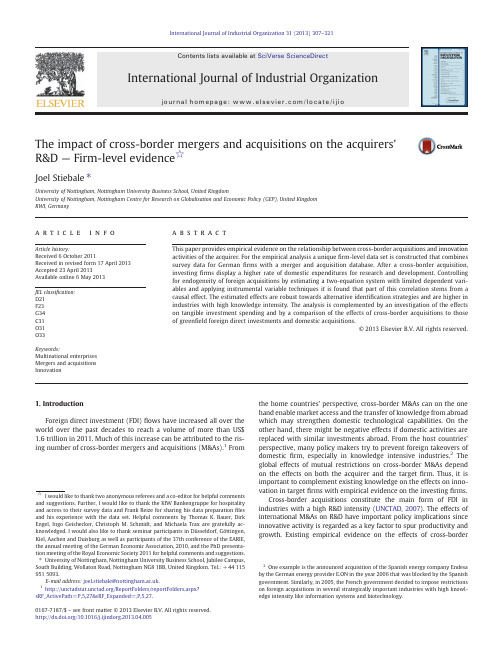
The impact of cross-border mergers and acquisitions on the acquirers'R&D —Firm-level evidence ☆Joel Stiebale ⁎University of Nottingham,Nottingham University Business School,United KingdomUniversity of Nottingham,Nottingham Centre for Research on Globalisation and Economic Policy (GEP),United Kingdom RWI,Germanya b s t r a c ta r t i c l e i n f o Article history:Received 6October 2011Received in revised form 17April 2013Accepted 23April 2013Available online 6May 2013JEL classi fication:D21F23G34C31O31O33Keywords:Multinational enterprises Mergers and acquisitions InnovationThis paper provides empirical evidence on the relationship between cross-border acquisitions and innovation activities of the acquirer.For the empirical analysis a unique firm-level data set is constructed that combines survey data for German firms with a merger and acquisition database.After a cross-border acquisition,investing firms display a higher rate of domestic expenditures for research and development.Controlling for endogeneity of foreign acquisitions by estimating a two-equation system with limited dependent vari-ables and applying instrumental variable techniques it is found that part of this correlation stems from a causal effect.The estimated effects are robust towards alternative identi fication strategies and are higher in industries with high knowledge intensity.The analysis is complemented by an investigation of the effects on tangible investment spending and by a comparison of the effects of cross-border acquisitions to those of green field foreign direct investments and domestic acquisitions.©2013Elsevier B.V.All rights reserved.1.IntroductionForeign direct investment (FDI)flows have increased all over the world over the past decades to reach a volume of more than US$1.6trillion in 2011.Much of this increase can be attributed to the ris-ing number of cross-border mergers and acquisitions (M&As).1Fromthe home countries'perspective,cross-border M&As can on the one hand enable market access and the transfer of knowledge from abroad which may strengthen domestic technological capabilities.On the other hand,there might be negative effects if domestic activities are replaced with similar investments abroad.From the host countries'perspective,many policy makers try to prevent foreign takeovers of domestic firm,especially in knowledge intensive industries.2The global effects of mutual restrictions on cross-border M&As depend on the effects on both the acquirer and the target firm.Thus,it is important to complement existing knowledge on the effects on inno-vation in target firms with empirical evidence on the investing firms.Cross-border acquisitions constitute the main form of FDI in industries with a high R&D intensity (UNCTAD,2007).The effects of international M&As on R&D have important policy implications since innovative activity is regarded as a key factor to spur productivity and growth.Existing empirical evidence on the effects of cross-borderInternational Journal of Industrial Organization 31(2013)307–321☆I would like to thank two anonymous referees and a co-editor for helpful comments and suggestions.Further,I would like to thank the KfW Bankengruppe for hospitality and access to their survey data and Frank Reize for sharing his data preparation files and his experience with the data set.Helpful comments by Thomas K.Bauer,Dirk Engel,Ingo Geishecker,Christoph M.Schmidt,and Michaela Trax are gratefully ac-knowledged.I would also like to thank seminar participants in Düsseldorf,Göttingen,Kiel,Aachen and Duisburg as well as participants of the 37th conference of the EARIE,the annual meeting of the German Economic Association,2010,and the PhD presenta-tion meeting of the Royal Economic Society 2011for helpful comments and suggestions.⁎University of Nottingham,Nottingham University Business School,Jubilee Campus,South Building,Wollaton Road,Nottingham NG81BB,United Kingdom.Tel.:+441159515093.E-mail address:joel.stiebale@ .1/ReportFolders/reportFolders.aspx?sRF_ActivePath=P,5,27&sRF_Expanded=,P,5,27.2One example is the announced acquisition of the Spanish energy company Endesa by the German energy provider E.ON in the year 2006that was blocked by the Spanish government.Similarly,in 2005,the French government decided to impose restrictions on foreign acquisitions in several strategically important industries with high knowl-edge intensity like information systems andbiotechnology.0167-7187/$–see front matter ©2013Elsevier B.V.All rights reserved./10.1016/j.ijindorg.2013.04.005Contents lists available at SciVerse ScienceDirectInternational Journal of Industrial Organizationj o u r n a l h om e p a g e :ww w.e l s e v i e r.c o m /l o c a t e /i j i oM&As is mostly limited to targetfirms,while little is known about the effects on the acquiringfirms.3Only recently,cross-border acquisitions as a type of FDI started to receive more attention in the international trade literature.Recent theoretical contributions analyze the role offirm heterogeneity and different motives that determine the choice of foreign market entry modes(Nocke and Yeaple,2007;Norbäck and Persson,2007).These models argue that international M&As are mainly driven by the desire to acquire complementary assets and technology while greenfield investments(newfirms or production units founded by foreign investors)do not provide direct access to foreign knowledge and are rather undertaken to exploit existingfirm-specific assets of the acquiringfirm or factor price differences across countries.If comple-mentarities between acquiring and targetfirm play a role for cross-border acquisitions and these involve innovative activities it is likely that the effects on domestic R&D are quite different from those of greenfield investments.Hence,it is not possible to derive conclusions about the effects of cross-border M&As from existing studies on greenfield investments or aggregate FDI.It is also likely that the effects of international acquisitions are different from those of domestic transactions since previous research argues that the motives and characteristics of cross-border M&As are different(see Shimizu et al.,2004,for instance).Theory suggests that the characteristics offirms that self-select into international acquisitions are quite different from those that engage in domestic acquisitions(see e.g.Nocke and Yeaple,2008).Market access–for instance via access to existing networks or market specific knowledge like marketing capabil-ities–might be a more important motive for international than for do-mestic M&As(see e.g.Nocke and Yeaple,2008;Guadalupe et al.,2012; Blonigen et al.,2012).Improved market access from the perspective of the acquiringfirm may increase the incentives to invest in cost reducing or quality enhancing innovations as these can be applied to a larger production output.Further,as efficiency differences within an industry are likely to be more pronounced across than within countries(Neary, 2007)it is likely that foreign and domestic acquisition targets have dif-ferent characteristics.This may result in different feedback effects on the investingfirm as well.The purpose of this paper is to investigate the impact of cross-border acquisitions on R&D activities of the investingfirm.This paper contributes to the existing literature in several aspects.First, empirical evidence on the effects of international acquisitions on innovation activities of the acquirer is sparse.4Further,I contribute to the industrial organization and the international economics litera-ture by comparing the effects of cross-border acquisitions to those of domestic acquisitions and greenfield foreign direct investments. Heterogeneous effects according to industries and target countries with different characteristics are provided.For this purpose a unique firm-level data set is constructed that combines survey data for Germanfirms with balance sheet data and an M&A database.The case of Germany is in particular interesting as it is one of the most technologically advanced countries in the world and is considerably engaged in FDI and global M&As.The empirical framework accounts for unobservedfirm heteroge-neity and the possible endogeneity of cross-border acquisitions.The main results are based on a non-linear two-equation model in which the decision to engage in an international acquisition as well as the decision of how much to spend on R&D is explained simulta-neously.Identification is achieved by exploiting unexpected shocks to foreign market growth rates and variation in the distance to foreign markets acrossfirms.The robustness of the results towards alternative empirical models and identifying assumptions is checked.This paper is organized as follows.In Section2,I summarize the related literature.Section3describes the empirical model and Section4provides a description of the data.Results of the empirical analysis are presented in Section5.Section6concludes.2.Cross-border acquisitions and R&DThis paper is related to several strands of theoretical and empirical literature that look at M&As from the perspective of industrial organi-zation(IO)economics,strategic management,or corporatefinance.5 As the M&A literature often does not distinguish explicitly between cross-border and domestic acquisitions or between effects on acquir-ingfirms and acquisition targets it is worth taking a look at the litera-ture on international trade and FDI as well.Cross-border acquisition can affect the investingfirm's innovation activities through a variety of channels.First,there might be direct effects via relocation of R&D activities.Second,acquisitions may have an impact on other determi-nants of R&D that have been identified in the theoretical and empirical innovation literature such as afirm's size,market share,competition, technological opportunities,external knowledge sources,market demand,andfinancial factors(see,for instance,Cohen and Levine, 1989or Hall and Mairesse,2006for an overview on the determinants of R&D).The main motives for M&As within the IO literature are the strengthening of market power(Kamien and Zang,1990)and the re-alization of efficiency gains(Röller et al.,2001).The effects on market power and efficiency also belong to the main channels through which M&As can affect R&D.M&As might be undertaken to gain access to targetfirms'assets such as production capabilities or intangible assets (e.g.Jovanovic and Rousseau,2008).Efficiency gains after an acquisi-tion may,for instance,stem from the diffusion of know-how within the merged entity(Röller et al.,2001)or the reallocation of technol-ogy to more efficient uses(Jovanovic and Rousseau,2008).Synergies resulting from M&As might entail an increase in the efficiency of R&D which might increase the incentives to innovate.Regarding the strategic aspect,a reduction in competition has a theoretically ambiguous effect on innovation incentives.This effect depends on market characteristics,the type of innovation,and the de-gree of R&D spillovers(see,for instance,Gilbert,2006;Vives,2008; Schmutzler,2010for a recent discussion).Reduced competition will increase afirm's residual demand–and thus the output to which cost reductions or quality improvements can be applied–but at the same time it tends to decrease the elasticity of demand and thus the impact of price reductions.However,if a merger solely reduces the number offirms in a market,it is likely that this induces a positive ef-fect on innovation incentives(Vives,2008).Further,the internaliza-tion of technology spillovers that have previously been captured by competitors can also increase the incentives for R&D(Kamien et al., 1992).Gilbert and Newbery(1982)argue thatfirms with monopoly power have additional incentives to engage in R&D due to the possibil-ity of preemptive patenting.Acquisitions that are motivated by strategic reasons also play a role in the international economics literature(e.g.Horn and Persson,2001; Neary,2007).Cost differences betweenfirms might be more pro-nounced across than within countries and this may increase the incen-tives for cross-border M&As(Bertrand and Zitouna,2006;Bjorvatn, 2004;Neary,2007).In Neary(2007),for instance,cross-border acqui-sitions are accompanied by a reallocation of production from less3The effects of cross-border M&As on targetfirms have received considerable atten-tion with respect to productivity(Arnold and Javorcik,2009;Benfratello and Sembenelli,2006)and employment(Almeida,2007).Recently,particular attention has been paid to the effects of foreign acquisitions on innovation activity(Bertrand, 2009;Bertrand et al.,2012;Guadalupe et al.,2012;Stiebale and Reize,2011).4Bertrand and Zuninga(2006)analyze effects of domestic and international M&As on R&D at the industry level.Firm-level studies that analyze differences between ef-fects of domestic and international acquisitions on the acquirers'innovation includeDesyllas and Hughes(2010),Cloodt et al.(2006)and Ahuja and Katila(2001),al-though analyzing effects of cross-border M&As is not at the core of their analysis.5The literature on cross-border M&As from the perspective of the management lit-erature is surveyed in Shimizu et al.(2004).308J.Stiebale/International Journal of Industrial Organization31(2013)307–321efficient acquisition targets to more efficient foreign investors.If M&As are primarily motivated by efficiency differences between firms across countries we would expect an increase in economic activ-ity in acquiringfirms at the expense of targetfirms.6The impact of cross-border acquisitions on R&D in acquiringfirms can be different from the effects on efficiency and the scale of produc-tion.Acquirers might relocate R&D facilities from targetfirms to the cor-porate headquarters,but keep production sites running(or vice versa). Manyfirms tend to cluster their R&D activities close to their headquar-ters or their main corporate production unit due to the aim of managers to keep track of these activities(Howell,1984).Sanna-Randaccio and Veugelers(2007)show in a theoretical model that centralizing R&D in the home country increases the appropriability of the results of R&D efforts as it prevents knowledge spillovers to foreign competitors in the host country.Centralizing R&D may also avoid costs of coordination and may allow a multinational enterprise to exploit economies of scale in R&D(Kumar,2001).Hence,it is well possible that relocation effects for R&D are more pronounced than for production activities.Cross-border acquisitions are a mode of FDI and thus might in addition be motivated by differences in production costs across countries,the desire to enter foreign markets,or the access to country specific assets.7In most theoretical trade models incorporatingfirm heterogeneity,market access is the most important motive for FDI (for instance,Helpman et al.,2004).This type of market-seeking FDI is usually referred to as horizontal investment.Horizontal FDI might reduce domestic production if it comes along with a substitution of exports.Contrarily,FDI might spur headquarter activities such as marketing activities and R&D as these investments can be applied to a larger production output after a foreign investment(Fors and Svensson,2002).This might in turn increase growth in the acquirers' home country.Vertical FDI in analogy to Head and Ries(2003)is motivated by differences in factor costs across countries.However,the motives for cross-border M&As might be quite different from greenfield investments(even in a monopolistic com-petition framework where they are not driven by strategic aspects). Theoretical trade models with heterogeneousfirms that differentiate between the modes of foreign market entry usually argue that green-field investments are chosen for FDI motivated by production cost differences(Nocke and Yeaple,2007,2008).In contrast,these models argue that cross-border M&As are aimed to achieve access to comple-mentaryfirm-specific assets of acquisition targets(Nocke and Yeaple, 2008),country-specific assets(Norbäck and Persson,2007),export networks(Blonigen et al.,2012),or capabilities that are non-mobile across countries(Nocke and Yeaple,2007).8If the exploitation of complementary assets entails innovation activities this might in-crease the returns to these activities and thus spur R&D expenditures.There are,however,also counterarguments regarding the effects of international M&As on acquiringfirms'R&D.Cross-border acquisi-tions might come along with a substitution of domestic by foreign activities.There might also be a reduction of duplicate R&D activities after a merger if the overlap between the research projects of acquirer and targetfirm is large(Veugelers,2006).Further,M&As may lead to a reduction in the competition in technology markets which may reduce the incentives of mergingfirms to engage in R&D activities further(Arrow,1962).There are also some counterarguments which can be derived from thefinancial economics and the manage-ment literature.M&As are oftenfinanced with a high amount of debt which might raise the costs for raising external funds for R&D and there is empirical evidence that especially after a leveraged buyout targets display declining expenditures for capital(Kaplan,1989) and R&D(Long and Ravenscraft,1993).Further,M&As might also arise out of a manager's utility maximization(Shleifer and Vishny, 1988)who wants a large empire under control and conducts M&As at the expense of other investment projects including R&D activities. Finally,M&As might reduce R&D due to increased organizational complexity and tighterfinancial controls(Hitt and Hoskisson,1990; Hitt et al.,1991)or due to a disruption of established routines (Ahuja and Katila,2001).Hence,from a theoretical point of view the relationship between foreign acquisitions and acquirers'R&D is unclear and thus boils down to an empirical matter.Empirical studies that deal with the effects of domestic M&As(or do not explicitly differentiate between domestic and international M&As)find in the majority negative effects(Cassiman et al.,2005).But the results seem to depend on product and technology market characteristics.Cassiman et al. (2005)argue that the impact of M&As on R&D in the merged entity depends on technological and market relatedness between acquirer and target.They suggest that M&As between rivalfirms lead to an overall reduction of R&D efforts,while they predict the opposite when the merged entities are technologically complementary. Studies that deal with the effects on innovation activities in foreign acquisition targets have so far yielded mixed results.For instance, Guadalupe et al.(2012)and Bertrand(2009)find positive effects of foreign acquisitions on innovation,while Stiebale and Reize(2011)find large negative effects once endogeneity and selection bias are taken into account,and Bertrand and Zuninga(2006)find no signifi-cant effect on average but some positive effects in industries with a medium technological intensity.Existing empirical studies that ana-lyze the impact of cross-border acquisitions on innovation activities at thefirm level are mostly limited to the evidence on the impact on targetfirms.9Marin and Alvarez(2009)find that acquisitions undertaken by foreign ownedfirms in Spain have a negative impact on the acquirers'innovation activities,in contrast to acquisitions by domestically ownedfirms,but they do not analyze the impact of cross-border acquisitions.Ahuja and Katila(2001)as well as Cloodt et al.(2006)analyze differences in a sample of mergingfirms according to cultural distance between acquirer and targetfirm. Desyllas and Hughes(2010)find that cross-border M&As have a more pronounced negative effect on the acquirer's R&D intensity than domestic M&As.3.Empirical strategyTwo main problems have to be addressed in the empirical analy-sis.First,structural zeros arise because a lot offirms report zero R&D expenditures.Second,endogeneity might arise from the fact that unobserved factors influencing R&D might also be correlated with a foreign acquisition.Thus,a model that accounts for both structural zeros and endogeneity is specified to evaluate the impact of international acquisitions on the acquirer's innovation.To evaluate the effect of outward cross-border acquisitions on domestic R&D expenditures,a two-equation model is specified:RDÃit¼x′itβ1þδIMA itþεitð1Þ6Stiebale and Trax(2011)provide evidence that acquirers'domestic sales and em-ployment tend to increase after international M&As.7See Helpman(2006)for an overview on the theoretical literature onfirms and FDI choices.8There are several further possible motives for cross-border acquisitions.In a model of Head and Ries(2008),cross-border acquisitions arise due to the possibility to shift ownership to a more efficient usage.Cross-border acquisitions(and FDI in general) may also be motivated by building an export platform in a tariff free block such as the European Union(Neary,2002).Cross-border and domestic acquisitions may also involve vertical integration.However,while cross-border M&As often take place acrossindustries they are rarely associated with input–output linkages(e.g.,Hijzen et al., 2008).9A detailed discussion about studies that analyze the relationship between foreign ownership and innovation can be found in Stiebale and Reize(2011).309J.Stiebale/International Journal of Industrial Organization31(2013)307–321IMA Ãit ¼x ′it β2þz it γþu it IMA it ¼(1;IMA Ãit >00;elseRD it ¼max RD Ãit ;0ðÞ:ð2ÞThe error terms of the two equations are assumed to be jointly normally distributed:εitu ite N 2ðÞ00 ;σερσερσε1where the variance of u it is normalized to one for identi fication.RD it denotes the domestic R&D to sales ratio,multiplied by 100,of firm i in period t .IMA it is a dummy variable that takes the value of one if a firm acquired a target in an international M&A between t −2and t .An acquisition is de fined as an increase in the ownership share from below to above 50%of equity —either directly or indirectly through a parent or a holding company.x it is a vector of firm-and industry-level variables that enters both equations.It contains variables that are usually used in innovation studies which are likely to affect both R&D expenditures and interna-tional acquisitions.10A firm's age is measured in years and serves as a proxy for experience and the stage of the product life cycle.Firm size enters the equations as the logarithm of the number of employees.Human capital intensity is approximated by the share of employees with a university degree.Capital intensity controls for past accumula-tion of tangible assets.The ability to raise equity for financing invest-ment is captured by a dummy variable that takes the value of one if the firm has financed part of its tangible investment by equity.Further,a dummy variable for incorporated enterprises is added to the model that captures differences in corporate governance and the ability to raise external finance.A dummy variable for Eastern Germany accounts for the transition process and regional differences.The model also includes a control variable for foreign ownership.Two dummy variables take the value of one if a firm cooperates with other firms or public scienti fic institutions,respectively.11Further,x it contains several variables that account for the competi-tive environment and market conditions.The firm's lagged domestic market share captures the potential to spread the gain from new or improved products and processes over production output.This variable also accounts for the selection of larger and more productive firms into foreign markets.The domestic market growth rate -measured at the two-digit level -controls for time-varying changes in market size at the industry level.To account for changes in competition,a further variable measures the net entry rate on the domestic market (see Aghion et al.,2009for an analysis on the effect of entry on innovation).It is also controlled for a firm's main regional market by a set of dummy variables that take the value of one if a firm's main market is international,national,or regional,respectively (for instance,Aw et al.,2007,2008analyze the role of exporting for R&D).Industry dummies at the two-digit level control for time invariant product and market characteristics and time dummies capture macroeconomic shocks.z it includes variables that are assumed to affect the propensity to engage in a cross-border acquisition but not domestic R&D expendi-tures.These variables are discussed in detail below.Endogeneity of IMA it ,in the two equation model,stems from a non-zero correlation between the two error terms (ρ≠0).A prerequi-site for logical consistency is that a recursive structure is imposed,i.e.RD it does not appear in Eq.(2)(see e.g.Maddala,1983).This prerequisite ismet in the chosen speci fication and seems reasonable,as an acquisition in the past on current R&D expenditures is evaluated.The model does not contain firm-fixed effects.The reason is that introducing fixed effects in non-linear models leads to inconsistent estimates of all parameters.12Estimation is carried out by full maximum likelihood.Full maximum likelihood is more demanding than a two-step control function approach as it requires specifying a joint distribution of the equation system,but it assures most ef ficient estimation if the model is correctly speci fied.13The robustness of the results towards the distributional as-sumptions is checked by using a linear instrumental variable estimator.Standard errors are clustered as some firms appear more than once in the sample.Irrespective of the estimation procedure,it is necessary for identi fication that there is at least one valid exclusion restriction,i.e.a variable that affects the probability to engage in a cross-border ac-quisition but not domestic R&D expenditures.In the context of the two equation model,this is a variable that enters z it but not x it .14Two exclusion restrictions are used in the empirical analysis.Score tests are computed to test the joint and individual validity of the two ex-clusion restrictions,and the results of these tests support the model's identifying assumptions.The first instrumental variable is the distance to foreign markets which is measured as the minimum distance to Western European countries.This variable captures the well known proximity –concentration tradeoff (see e.g.Brainard,1997):In models of horizontal FDI,firms face a trade-off between exporting on the one hand and producing locally via FDI on the other hand.The former re-quires them to pay higher transport costs of the goods shipped to the foreign market,but exporters can bene fit from concentrating produc-tion and thereby achieving scale economies.FDI,in contrast,involves paying higher sunk and fixed costs for the af filiate abroad but lower transport costs due to the proximity to consumers.15For this instrument to be valid,it is crucial that omitted regional fac-tors,that are correlated with distance to foreign markets,do not affect R&D expenditures.I argue that most of the systematic differences in inno-vativeness across regions are captured by the control variables,i.e.vari-ables in x it ,like industry dummies,external knowledge sources,and other firm-and industry-level variables.One might be concerned that firms choose a certain location because they plan to engage in cross-border acquisitions.However,only a few firms change their loca-tion after foundation,and the average firm age at the time of acquisition is more than 35years in our sample.Hence,it seems unlikely that M&As affect the location choice of firms.10See e.g.Cohen and Levine (1989)and Hall and Mairesse (2006)for an overview on empirical innovation studies.11The survey questions underlying these variables refer to cooperation with firms and institutions in general and not to cooperation on R&D as in CIS innovation surveys.Hence they do not imply but might affect R&D activities.12A further problem is that many firms in the data set only appear at most twice in the sample.However,some regressions in first differences and with controls for lagged values of the dependent variable on a reduced sample are presented to convey an im-pression about the importance of time-invariant unobserved firm heterogeneity.13Estimation was carried out in Stata®,version 10.1.The likelihood function of this model can be found in Appendix B available on the web,and the program code for estima-tion is available from the author upon request.Alternative models such as the instrumen-tal variable Tobit model developed by Smith and Blundell (1986)are not applicable as they do not allow for discrete endogenous regressors.Similarly,the fractional response es-timators suggested by Papke and Wooldridge (2008)cannot deal with binary endogenous regressors as well.Abadie (2003)proposes a semi-parametric estimator,but this estima-tor requires that there is a binary instrument variable available which is not the case in this application.Angrist (2001)proposes to use two-stage least squares,but this method is only consistent for censored outcome variables in special cases.Nonetheless,the robust-ness of the main results to using two stage least squares is checked in Section 5.3.14Due to nonlinearity the model is identi fied even without exclusion restrictions,but the results are not very reliable in this case as they critically hinge on distributional and functional form assumptions.15Nonetheless,the relationship between cross-border acquisitions and geographic distance is not unambiguous as this variable might capture other in fluences like cultur-al distance or vertical relations.Hijzen et al.(2008)find a negative relation between cross-border M&As and distance,measured at the industry-country level,which is more pronounced for non-horizontal M&As.However,a positive correlation between a firm's distance to the border and foreign acquisitions does not rule out a negative cor-relation between M&As and distance on a macroeconomic level.Firms may be induced to engage in cross-border acquisitions as opposed to serve a foreign market via exports by distance,but they may (conditional on this choice)choose a close-by target firm to minimize trade and transaction costs.310J.Stiebale /International Journal of Industrial Organization 31(2013)307–321。
APPLICATION OF SYMMETRY ANALYSIS TO A PDE ARISING IN THE CAR WINDSHIELD DESIGN

APPLICATION OF SYMMETRY ANALYSIS TO APDE ARISING IN THE CAR WINDSHIELD DESIGN ∗NICOLETA B ˆIL ˘A†SIAM J.A PPL.M ATH .c2004Society for Industrial and Applied Mathematics Vol.65,No.1,pp.113–130Abstract.A new approach to parameter identification problems from the point of view of symmetry analysis theory is given.A mathematical model that arises in the design of car windshield represented by a linear second order mixed type PDE is considered.Following a particular case of the direct method (due to Clarkson and Kruskal),we introduce a method to study the group invariance between the parameter and the data.The equivalence transformations associated with this inverse problem are also found.As a consequence,the symmetry reductions relate the inverse and the direct problem and lead us to a reduced order model.Key words.symmetry reductions,parameter identification problemsAMS subject classifications.58J70,70G65,35R30,35R35DOI.10.1137/S00361399034340311.Introduction.Symmetry analysis theory links differential geometry to PDEs theory [18],symbolic computation [9],and,more recently,to numerical analysis theory[3],[6].The notion of continuous transformation groups was introduced by Sophus Lie [14],who also applied them to differential equations.Over the years,Lie’s method has been proven to be a powerful tool for studying a remarkable number of PDEs arising in mathematical physics (more details can be found for example in [2],[10],and [21]).In the last several years a variety of methods have been developed in order to find special classes of solutions of PDEs,which cannot be determined by applying the classical Lie method.Olver and Rosenau [20]showed that the common theme of all these methods has been the appearance of some form of group invariance.On the other hand,parameter identification problems arising in the inverse problems theory are concerned with the identification of physical parameters from observations of the evolution of a system.In general,these are ill-posed problems,in the sense that they do not fulfill Hadamard’s postulates for all admissible data:a solution exists,the solution is unique,and the solution depends continuously on the given data.Arbitrary small changes in data may lead to arbitrary large changes in the solution.The iterative approach of studying parameter identification problems is a functional-analytic setup with a special emphasis on iterative regularization methods [8].The aim of this paper is to show how parameter identification problems can be analyzed with the tools of group analysis theory.This is a new direction of research in the theory of inverse problems,although the symmetry analysis theory is a com-mon approach for studying PDEs.We restrict ourselves to the case of a parameter identification problem modeled by a PDE of the formF (x,w (m ),E (n ))=0,(1.1)where the unknown function E =E (x )is called parameter ,and,respectively,the arbitrary function w =w (x )is called data ,with x =(x 1,...,x p )∈Ω⊂R p a given∗Receivedby the editors September 4,2003;accepted for publication (in revised form)May 4,2004;published electronically September 24,2004.This work was supported by the Austrian Science Foundation FWF,Project SFB 1308“Large Scale Inverse Problems.”/journals/siap/65-1/43403.html †Institute for Industrial Mathematics,Johannes Kepler University,69Altenbergerstrasse,Linz,A-4040,Austria (bila@indmath.uni-linz.ac.at).113114NICOLETA BˆIL˘Adomain(here w(m)denotes the function w together with its partial derivatives up to order m).Assume that the parameters and the data are analytical functions. The PDE(1.1)sometimes augmented with certain boundary conditions is called the inverse problem associated with a direct problem.The direct problem is the same equation but the unknown function is the data,for which certain boundary conditions are required.The classical Lie method allows us tofind the symmetry group related to a PDE. This is a(local)Lie group of transformations acting on the space of the independent variables and the space of the dependent variables of the equation with the property that it leaves the set of all analytical solutions invariant.Knowledge of these classi-cal symmetries allows us to reduce the order of the studied PDE and to determine group-invariant solutions(or similarity solutions)which are invariant under certain subgroups of the full symmetry group(for more details see[18]).Bluman and Cole[1] introduced the nonclassical method that allows one tofind the conditional symmetries (also called nonclassical symmetries)associated with a PDE.These are transforma-tions that leave only a subset of the set of all analytical solutions invariant.Note that any classical symmetry is a nonclassical symmetry but not conversely.Another procedure forfinding symmetry reductions is the direct method(due to Clarkson and Kruskal[5]).The relation between these last two methods has been studied by Olver[19].Moreover,for a PDE with coefficients depending on an arbitrary function, Ovsiannikov[21]introduced the notion of equivalence transformations,which are(lo-cal)Lie group of transformations acting on the space of the independent variables, the space of the dependent variables and the space of the arbitrary functions that leave the equation unchanged.Notice that these techniques based on group theory do not take into account the boundary conditions attached to a PDE.Tofind symmetry reductions associated with the parameter identification problem (1.1)one can seek classical and nonclassical symmetries related to this equation.Two cases can occur when applying the classical Lie method or the nonclassical method, depending if the data w is known or not.From the symbolic computation point of view,the task offinding symmetry reductions for a PDE depending on an arbi-trary function might be a difficult one,due to the lack of the symbolic manipulation programs that can handle these kind of equations.Another method to determine symmetry reductions for(1.1)might be a particular case of the direct method,which has been applied by Zhdanov[24]to certain multidimensional PDEs arising in mathe-matical physics.Based on this method and taking into account that(1.1)depends on an arbitrary function,we introduce a procedure tofind the relation between the data and the parameter in terms of a similarity variable(see section2).As a consequence, the equivalence transformations related to(1.1)must be considered as well.These final symmetry reductions are found by using any symbolic manipulation program de-signed to determine classical symmetries for a PDE system—now both the data and the parameter are unknown functions in(1.1).The equivalence transformations relate the direct problem and the inverse problem.Moreover,one canfind special classes of data and parameters,respectively,written in terms of the invariants of the group action,the order of the studied PDE can be reduced at least by one,and analytical solutions of(1.1)can be found.At thefirst step,the group approach of the free boundary problem related to (1.1)can be considered and,afterwards,the invariance of the boundary conditions under particular group actions has to be analyzed(see[2]).In the case of parameter identification problems we sometimes have to deal with two pairs of boundary condi-tions,for data and the parameter as well,otherwise we might only know the boundarySYMMETRY ANALYSIS AND PARAMETER IDENTIFICATION PROBLEMS 115conditions for the data.Thus,the problem of finding symmetry reductions for a given data can be more complicated.At least by finding the equivalence transformations related to the problem,the invariants of the group actions can be used to establish suitable domains Ωon which the order of the model can be reduced.In this paper we consider a mathematical model arising in the car windshield design.Let us briefly explain the gravity sag bending process ,one of the main industrial processes used in the manufacture of car windshields.A piece of glass is placed over a rigid frame,with the desired edge curvature and heated from below.The glass becomes viscous due to the temperature rise and sags under its own weight.The final shape depends on the viscosity distribution of the glass obtained from varying the temperature.It has been shown that the sag bending process can also be controlled (in a first approximation)in the terms of Young’s modulus E ,a spatially varying glass material parameter,and the displacement of the glass w can be described by the thin linear elastic plate theory (see [11],[16],and [17]and references from there).The model is based on the linear plate equation(E (w xx +νw yy ))xx +2(1−ν)(Ew xy )xy +(E (w yy +νw xx ))yy =12(1−ν2)fh 3on Ω,(1.2)where w =w (x,y )represents the displacement of the glass sheet (the target shape)occupying a domain Ω⊂R 2,E =E (x,y )is Young’s modulus,a positive function that can be influenced by adjusting the temperature in the process of heating the glass,f is the gravitational force,ν∈ 0,12 is the glass Poisson ratio,and h is thickness of the plate.The direct problem (or the forward problem )is the following:for a given Young modulus E ,find the displacement w of a glass sheet occupying a domain Ωbefore the heating process.Note that the PDE (1.2)is an elliptic fourth order linear PDE for the function w .Until now,two problems related to (1.2)have been studied:the clamped plate case and the simply supported plate case (more details can be found for example in [15]).In this paper we consider the clamped case,in which the following boundary conditions are required:the plate is placed over a rigid frame,i.e.,w (x,y )|∂Ω=0,(1.3)and,respectively,∂w ∂n |∂Ω=0,(1.4)which means the (outward)normal derivative of w must be zero,i.e.,the sheet of glass is not allowed to freely rotate around the tangent to ∂Ω.The associated inverse problem consists of finding Young’s modulus E for a given data w in (1.2).This is a linear second order PDE for Young’s modulus that can be written as(1.5)(w xx +νw yy )E xx +2(1−ν)w xy E xy +(w yy +νw xx )E yy+2(∆w )x E x +2(∆w )y E y +(∆2w )E =1after the scaling transformations w →1k w or E →1k E ,with k =12(1−ν2)f h 3.In (1.5),∆denotes the Laplace operator.The main problem in the car windshield design is that the prescribed target shape w is frequent such that the discriminantD =(1−ν)2w 2xy −(w xx +νw yy )(w yy +νw xx )116NICOLETA BˆIL˘Aof(1.5)changes sign in the domainΩ,so that we get a mixed type PDE.This is one of the reasons for which optical defects might occur during the process.Note that (1.5)would naturally call for boundaries conditions for E on∂Ωin the purely elliptic case(when D<0),and Cauchy data on a suitable(noncharacteristic part)Γ⊂∂Ωin the purely hyperbolic part(for D>0).There is a recent interest in studying this inverse problem(see,e.g.,[13]).It is known[15]that a constant Young’s modulus corresponds to a data which satisfies the nonhomogeneous biharmonic equation(2.29).A survey on this subject can be found in[23].Salazar and Westbrook[22]studied the case when the data and the parameter are given by radial functions;K¨u gler[12] used a derivative free iterative regularization method for analyzing the problem on rectangular frames;and a simplified model for the inverse problem on circular domains was considered by Engl and K¨u gler[7].So far it is not obvious which shapes can be made by using this technique.Hence, we try to answer this question byfinding out the symmetry reductions related to the PDE(1.5)hidden by the nonlinearity that occurs between the data and the parameter. In this sense,we determine(see section3)the group of transformations that leave the equation unchanged,and so,its mixed type form.Knowledge of the invariants of these group actions allows us to write the target shape and the parameter in terms of them, and,therefore,to reduce the order of the studied equation.Wefind again the obvious result that a Young’s modulus constant corresponds to data which is a solution of a nonhomogeneous biharmonic equation.The circular case problem considered by Salazar and Westbrook is,in fact,a particular case of our study.We show that other target shapes which are not radial functions can be considered.We prove that(1.5) is invariant under scaling transformations.It follows that target shapes modeled by homogeneous functions can be analyzed as well.In particular,we are interested in target shapes modeled by homogeneous polynomials defined on elliptical domains or square domains with rounded corners.The paper is structured as follows.To reduce the order of the PDE(1.5)we propose in section2a method for studying the relation between the data and the pa-rameter in terms of the similarity variables.The equivalence transformations related to this equation are given in section3.The symbolic manipulation program DESOLV, authors Carminati and Vu[4]has been used for this purpose.Table1contains a com-plete classification of these symmetry reductions.In the last section,we discuss the PDE(1.5)augmented with the boundary conditions(1.3)and(1.4),namely,how to use the invariants of the group actions(on suitable bounded domainsΩ)in order to incorporate the boundary conditions.In this sense,certain examples of exact and of numerical solutions of the reduced ODEs are given.2.Conditional symmetries.The direct method approach to a second order PDEF(x,y,E(2))=0consists of seeking solutions written in the form(2.1)E(x,y)=Φ(x,y,F(z)),where z=z(x,y),(x,y)∈Ω.In this case the function z is called similarity variable and its level sets{z=k}are named similarity curves.After substituting(2.1)into the studied second order PDE, we require that the result to be an ODE for the arbitrary function F=F(z).Hence, certain conditions are imposed upon the functionsΦ,z and their partial derivatives.SYMMETRY ANALYSIS AND PARAMETER IDENTIFICATION PROBLEMS 117The particular caseE (x,y )=F (z (x,y ))(2.2)consists of looking for solutions depending only on the similarity variable z .If z is an invariant of the group action then the solutions of the form (2.2)are as well.Assume that the similarity variable is such that ∇z =0on ¯Ω.In this section we apply this particular approach to (1.5)in order to study if the parameter and the data are functionally independent,which means whether or not they can depend on the same similarity variable.Assume that Young’s modulus takes the form (2.2).In this case we get the relation(2.3)F (z ) z 2x (w xx +νw yy )+2z x z y (1−ν)w xy +z 2y (w yy +νw xx )+F (z )[z xx (w xx +νw yy )+2(1−ν)z xy w xy ++z yy (w yy +νw xx )+2z x (∆w )x +2z y (∆w )y ]+F (z )(∆2w )=1,which must be an ODE for the unknown function F =F (z ).This condition is satisfied if the coefficients of the partial derivatives of F are function of z only (note that these coefficients are also invariant under the same group action).Denote them byΓ1(z )=z 2x (w xx +νw yy )+2z x z y (1−ν)w xy +z 2y(w yy +νw xx ),Γ2(z )=z xx (w xx +νw yy )+2(1−ν)z xy w xy +z yy (w yy +νw xx )+2z x (∆w )x +2z y (∆w )y ,Γ3(z )=∆2w.(2.4)If these relations hold,then the PDE (1.5)is reduced to the second order linear ODE Γ1(z )F (z )+Γ2(z )F (z )+Γ3(z )F (z )=1.(2.5)2.1.Data and parameter invariant under the same group.If the target shape is invariant under the same group action as Young’s modulus,thenw (x,y )=G (z (x,y )),(2.6)where G =G (z ).Substituting (2.6)into the relations (2.4)we get Γ1=G (z 2x +z 2y )2+G (z 2x +νz 2y )z xx +2(1−ν)z x z y z xy +(z 2y +νz 2x )z yy ,Γ2=2G (z 2x +z 2y )2+G [7z 2x +(ν+2)z 2y ]z xx +2(5−ν)z x z y z xy +[7z 2y +(ν+2)z 2x ]z yy +G (∆z )2+2(1−ν)(z 2xy −z xx z yy )+2[z x (∆z )x +z y (∆z )y ]},Γ3=G (z 2x +z 2y )2+2G (3z 2x +z 2y )z xx +4z x z y z xy +(z 2x +3z 2y )z yy +G 3(∆z )2+4(z 2xy −z xx z yy )+4[z x (∆z )x +z y (∆z )y ] +G ∆2z.(2.7)Next,the coefficients of the partial derivatives of the function G ,denoted by Γi ,must depend only on z ,i.e.,Γ1=α4G +a 1G ,Γ2=2α4G +a 2G +a 3G ,Γ3=α4G +2a 4G +a 5G +a 6G ,118NICOLETA B ˆIL ˘Awhereα2(z )=z 2x +z 2y ,a 1(z )=(z 2x +νz 2y )z xx +2(1−ν)z x z y z xy +(z 2y +νz 2x )z yy ,a 2(z )= 7z 2x +(ν+2)z 2y z xx +2(5−ν)z x z y z xy + 7z 2y +(ν+2)z 2xz yy ,a 3(z )=(∆z )2+2(1−ν)(z 2xy −z xx z yy )+2[z x (∆z )x +z y (∆z )y ],a 4(z )=(3z 2x +z 2y )z xx +4z x z y z xy +(z 2x +3z 2y )z yy ,a 5(z )=3(∆z )2+4(z 2xy −z xx z yy )+4[z x (∆z )x +z y (∆z )y ],a 6(z )=∆2z.(2.8)The first relation in (2.8)is a two-dimensional (2D)eikonal equation.From this we getz 2xz xx +2z x z y z xy +z 2y z yy =α3(z )α (z ),z xx =α(z )α (z )−z y z x z xy ,z yy =α(z )α (z )−z x z y z xy .The last two equations implyz 2y z xx −2z x z y z xy +z 2x z yy =α3(z )α (z )−α4(z )z xy z x z y.(2.9)Assume that there is a function β=β(z )such thatz xy =β(z )z x z y .(2.10)Indeed,since the left-hand side in (2.9)depends only on z ,one can easily check if z satisfies both the 2D eikonal equation in (2.8)and (2.10),then all the functions a i =a i (z )defined by (2.8)are written in terms of αand β.Therefore,the problem of finding the similarity variable z is reduced to that of integrating the 2D eikonal equation and the PDE system⎧⎪⎪⎨⎪⎪⎩z xx =αα −βz 2y ,z xy =βz x z y ,z yy =αα −βz 2x .(2.11)The system (2.11)is compatible if the following relation holds:αα +α 2−3βαα +α2 β2−β =0.Denote µ=12α2.In this case,the above compatibility condition can be written asµ −3βµ +2µ β2−β =0.(2.12)On the other hand,if the function βis given byβ(z )=−λ (z ) ,(2.13)SYMMETRY ANALYSIS AND PARAMETER IDENTIFICATION PROBLEMS119 whereλis a nonconstant function,then(2.10)turns into(λ(z))xy=0.The general solution of this equation is given byλ(z(x,y))=a(x)+b(y),(2.14)with a and b being arbitrary functions.Substitutingβfrom(2.13)into the compati-bility condition(2.12)and after integrating once,we getµ λ +2µλ =k,(2.15)where k is an arbitrary constant.Case1.If k=0,then after integrating(2.15)and substituting backµ=12α2,wegetα2(z)=2kλ(z)+C1λ 2(z).(2.16)The relation(2.14)impliesλ (z)z x=a (x),andλ (z)z y=b (y).We substitute these relations,(2.14)and(2.16),into the2D eikonal equation(see(2.8)).It follows that the functions a=a(x)and b=b(y)are solutions of the following respective ODEs:a 2(x)−2ka(x)=C2andb 2(y)−2kb(y)=C3,with C2+C3=C1(here C i are real constants).The above ODEs admit the noncon-stant solutionsa(x)=12kk2(x−C4)2−C2and b(y)=12kk2(y−C5)2−C3,and so(2.14)takes the formλ(z(x,y))=k2(x−C4)2+(y−C5)2−C12k.(2.17)Notice that1k1λorλ+k2defines the same functionβas the functionλdoes.Moreover,since the PDE(1.5)is invariant under translations in the(x,y)-space,we can considerλ(z(x,y))=x2+y2.(2.18)If √λis a bijective function on a suitable interval,and if we denote byΦ=(√λ)−1its inverse function,then the similarity variable written in the polar coordinates(r,θ) (where x=r cos(θ),y=r sin(θ))is given byz(x,y)=Φ(r).(2.19)For simplicity,we considerΦ=Id,and from that we getE=F(r)and w=G(r),where z(x,y)=r.(2.20)Hence,the ODE(2.5)turns into(2.21)G +νrGF +2G +ν+2rG −1r2GF+G +2rG −1r2G +1r3GF=1,120NICOLETA B ˆIL ˘Awhich can be reduced to the first order ODEG +νG F + G +1G −1G F =r 2−r 20+γ,(2.22)where r 0∈[0,1]with the property that γ= (rG +νG )F + rG +G −1rG F |r =r 0is finite.The smoothness condition G (0)=0implies that (2.22)can be written as [15] G +νrG F + G +1r G −1r 2G F =r 2.(2.23)Case 2.If k =0,similarly we getz (x,y )=Φ(k 1x +k 2y ),(2.24)where k 1and k 2are real constants such that k 21+k 22>0.In this case,for Φ=Id,the parameter and the data are written asE =F (z )and w =G (z ),where z (x,y )=k 1x +k 2y,(2.25)and the ODE (2.5)turns into G (z )F (z )+2G (z )F (z )+G (z )F (z )=1(k 21+k 22)2,(2.26)with {z |G (z )=0}the associated set of singularities.Integrating the above ODE on the set {z |G (z )=0}we obtain that Young’s modulus is given byE (x,y )=(k 1x +k 2y )2+C 1(k 1x +k 2y )+C 22(k 21+k 22)2G (k 1x +k 2y ),where C i are arbitrary constants.2.2.Data and parameter invariant under different groups.Consider two functionally independent functions on Ω,say,z =z (x,y )and v =v (x,y ),and let w =H (v (x,y ))(2.27)be the target shape.In this case,the data and the parameter do not share the same invariance.Similar to the above,substituting (2.27)into the relations (2.4)we get Γ1=H (z x v x +z y v y )2+ν(z y v x −z x v y )2+H z 2x v xx +2z x z y v xy +z 2y v yy +ν z 2x v yy −2z x z y v xy +z 2y v xx ,Γ2=H (v 2x +v 2y )(z x v x +z y v y )+H v 2x z xx +2v x v y z xy +v 2y z yy +ν v 2y z xx −2v x v y z xy +v 2x z yy +2z x v x v xx +2(z x v y +z y v x )v xy +2z y v y v yy+(z x v x +z y v y )(∆v )]+H [z xx v xx +2z xy v xy +z yy v yy +ν(z xx v yy −2z xy v xy +z yy v xx )+z x (∆v )x +z y (∆v )y ],Γ3=H (v 2x +v 2y )2+2H (3v 2x +v 2y )v xx +4v x v y v xy +(v 2x +3v 2y )v yy +H 3v 2xx +4v 2xy +3v 2yy +2v xx v yy +4v x (∆v )x +4v y (∆v )y +H ∆2v.(2.28)SYMMETRY ANALYSIS AND PARAMETER IDENTIFICATION PROBLEMS121 Recall thatΓi’s are functions of z=z(x,y)only.Since each right-hand side in the above relations contains the function H=H(v)and its derivatives,we require that the coefficients of the derivatives of H to be functions of v.It follows thatΓi must be constant and denote them byγi.Therefore,the last condition in(2.28)becomes∆2(w)=γ3,(2.29)which is the biharmonic equation.According to the above assumption,we seek solu-tions of(2.29)that are functions of v only.Similar to section2.1,we getv(x,y)=Ψ(r),or v(x,y)=Ψ(k1x+k2y),(2.30)and thus,forΨ=Id,the target shape is written asw(x,y)=H(r),or w(x,y)=H(k1x+k2y).(2.31)Since z=z(x,y)and v=v(x,y)are functionally independent,we getz(x,y)=k1x+k2y,v(x,y)=x2+y2(2.32)orz(x,y)=x2+y2,v(x,y)=k1x+k2y.(2.33)One can prove that if the coefficientsγi are constant,and if z and v are given by (2.32)or(2.33),respectively,thenγ1=γ2=0,andγ3=0.On the other hand,the solutions of the biharmonic equation(2.29)of the form(2.31)are the following:w(x,y)=γ364z4+C1z2+C2ln(z)+C3z2ln(z)+C4for z=x2+y2,and,respectively,w(x,y)=γ324(k21+k22)2v4+C1v3+C2v2+C3v+C4for v=k1x+k2y,and these correspond to the constant Young’s modulusE(x,y)=1γ3.(2.34)Notice that only particular solutions of the biharmonic equation have been found in this case(i.e.,solutions invariant under rotations and translations).Since this PDE is also invariant under scaling transformations,which act not only on the space of the independent variables but on the data space as well,it is obvious to extend our study and to seek other types of symmetry reductions.3.Equivalence transformations.Consider a one-parameter Lie group of trans-formations acting on an open set D⊂Ω×W×E,where W is the space of the data functions,and E is the space of the parameter functions,given by⎧⎪⎪⎪⎪⎪⎨⎪⎪⎪⎪⎪⎩x∗=x+εζ(x,y,w,E)+O(ε2), y∗=y+εη(x,y,w,E)+O(ε2), w∗=w+εφ(x,y,w,E)+O(ε2), E∗=E+εψ(x,y,w,E)+O(ε2),(3.1)122NICOLETA BˆIL˘Awhereεis the group parameter.LetV=ζ(x,y,w,E)∂x+η(x,y,w,E)∂y+φ(x,y,w,E)∂w+ψ(x,y,w,E)∂E (3.2)be its associated general infinitesimal generator.The group of transformations(3.1) is called an equivalence transformation associated to the PDE(1.5)if this leaves the equation invariant.This means that the form of the equation in the new coordinates remains unchanged and the set of the analytical solutions is invariant under this trans-formation.The equivalence transformations can be found by applying the classical Lie method to(1.5),with E and w both considered as unknown functions(for more details see[10]and[21]).Following this method we obtain⎧⎪⎪⎪⎪⎪⎨⎪⎪⎪⎪⎪⎩ζ(x,y,w,E)=k1+k5x−k4y,η(x,y,w,E)=k2+k4x+k5y,φ(x,y,w,E)=k3+k7x+k6y+(4k5−k8)w,ψ(x,y,w,E)=k8E,(3.3)where k i are real constants.The vectorfield(3.2)is written as V= 8i=1k i V i,whereV1=∂x,V2=∂y,V3=∂w,V4=−y∂x+x∂y,V5=x∂x+y∂y+4w∂w,V6=y∂w,V7=x∂w,V8=−w∂w+E∂E.(3.4)Proposition3.1.The equivalence transformations related to the PDE(1.5)are generated by the infinitesimal generators(3.4).Thus,the equation is invariant under translations in the x-space,y-space,w-space,rotations in the space of the independent variables(x,y),scaling transformations in the(x,y,w)-space,Galilean transforma-tions in the(y,w)and(x,w)spaces,and scaling transformations in the(w,E)-space, respectively.Notice that the conditional symmetries found in section2represent particular cases of the equivalence transformations.Since each one-parameter group of trans-formations generated by V i is a symmetry group,if(w=G(x,y),E=F(x,y))is a pair of known solutions of(1.5),so are the following:w(1)=G(x−ε1,y),E(1)=F(x−ε1,y),w(2)=G(x,y−ε2),E(2)=F(x,y−ε2),w(3)=G(x,y)+ε3,E(3)=F(x,y),w(4)=G(˜x,˜y),E(4)=F(˜x,˜y),w(5)=e4ε5G(e−ε5x,e−ε5y),E(5)=F(e−ε5x,e−ε5y),w(6)=G(x,y)+ε6y,E(6)=F(x,y),w(7)=G(x,y)+ε7x,E(7)=F(x,y),w(8)=e−ε8G(x,y),E(8)=eε8F(x,y),(3.5)SYMMETRY ANALYSIS AND PARAMETER IDENTIFICATION PROBLEMS123where ˜x =x cos(ε4)+y sin(ε4),˜y=−x sin(ε4)+y cos(ε4),and εi are real constants.Moreover,the general solution of (1.5)constructed from a known one is given by w (x,y )=e 4ε5−ε8G (e −ε5(˜x −˜k 1),e −ε5(˜y −˜k 2))+e 4ε5−ε8ε6y +e 4ε5−ε8ε7x +e 4ε5−ε8ε3,E (x,y )=e ε8F (e −ε5(˜x −˜k 1),e −ε5(˜y −˜k 2)),where ˜k1=ε1cos(ε4)+ε2sin(ε4),and ˜k 2=ε1sin(ε4)−ε2cos(ε4).The equivalence transformations form a Lie group G with an eight-dimensional associated Lie algebra A .Using the adjoint representation of G ,one can find the optimal system of one-dimensional subalgebras of A (more details can be found in [18,pp.203–209]).This optimal system is spanned by the vector fields given in Table 1.Denote by z ,I ,and J the invariants related to the one-parameter group of transformations generated by each vector field V i .Here F and G are arbitrary functions,(r,θ)are the polar coordinates,and a,b,c are nonzero constants.To reduce the order of the PDE (1.5)one can also integrate the first order PDE systemζ(x,y,w,E )w x +η(x,y,w,E )w y =φ(x,y,w,E ),ζ(x,y,w,E )E x +η(x,y,w,E )E y =ψ(x,y,w,E ),(3.6)which defines the characteristics of the vector field (3.2).In Table 1,the associated reduced ODEs are listed.The invariance of (1.5)under the one-parameter groups of transformations generated by V 1,V 2,V 1+cV 6,and V 2+cV 7,respectively,leads us to the same ODE,F (z )G (z )+2F (z )G (z )+F (z )G (z )=1,(3.7)with the general solution F (z )=z 2+C 1z +C 22G (z )(3.8)on the set {z |G (z )=0}.The invariance under the scaling transformation generated by the vector field V 5yields the reduced ODEG z 2+1 2−6z (z 2+1)G +12(z 2+ν)G F +2z 2+1 2G−5z (z 2+1)G +3(4z 2+ν+1)G−12zG F+z 2+1 2G−4z (z 2+1)G +4(3z 2+1)G −24zG+24G F =1.(3.9)The ODEz 2+1 2G+2(c −3)z (z 2+1)G +(c −3)(c −4)(z 2+ν)G F+ 2 z 2+1 2G +2(2c −5)z (z 2+1)G +2(c −3)[z 2(c −4)+ν(c −1)−1]G −2(c −3)(c −4)zG }F+z 2+1 2G +2(c −2)z (z 2+1)G +(c −3)(c −4)z 2−2(c −2)+νc (c −1)]G −2(c −4)(c −3)zG +2(c −4)(c −3)G }F =1(3.10)124NICOLETA BˆIL˘ATable1Infintesimal generator Invariants w=w(x,y)E=E(x,y)ODE1.V1z=y w=G(z)E=F(z)(3.7)I=wJ=E2.V2z=x w=G(z)E=F(z)(3.7)I=wJ=E3.V4z=r w=G(z)E=F(z)(2.21)I=wJ=E4.V5z=yx w=x4G(z)E=F(z)(3.9)I=x−4wJ=E5.cV3+V4z=r w=cθ+G(z)E=F(z)(2.21)I=w−cθJ=E6.V5+cV8z=yx w=x4−c G(z)E=x c F(z)(3.10)I=x c−4wJ=x−c E7.V4+cV8z=r w=e−cθG(z)E=e cθF(z)(3.11)I=e cθwJ=e−cθE8.V4+cV5z=re−cθw=r4G(z)E=F(z)(3.13)I=r−4wJ=E9.V4+cX5+bV8z=re−cθw=r4−b c G(z)E=r b c F(z)(3.14)I=r b c−4wJ=r−b c E10.V1+cV6z=y w=cxy+G(z)E=F(z)(3.7)I=w−cxyJ=E11.V2+cV7z=x w=cxy+G(z)E=F(z)(3.7)I=w−cxyJ=E12.V1+cV8z=y w=e−cx G(z)E=e cx F(z)(3.15)I=e cx wJ=e−cx E13.V2+cV8z=x w=e−cy G(z)E=e cy F(z)(3.15)I=e cy wJ=e−cy Eis obtained in case6of Table1.The reduced equationG +νrG +νc2r2GF +2G +ν+2rG +2νc2−1r2G −c2(1+2ν)r3GF(3.11)+G +2rG +c2ν−1r2G +1−c2(2ν+1)r3G +2c2(ν+1)r4GF=1。
SUBMITTED TO IEEE TRANSACTIONS ON IMAGE PROCESSING 1 Group Testing for Image Compression Us
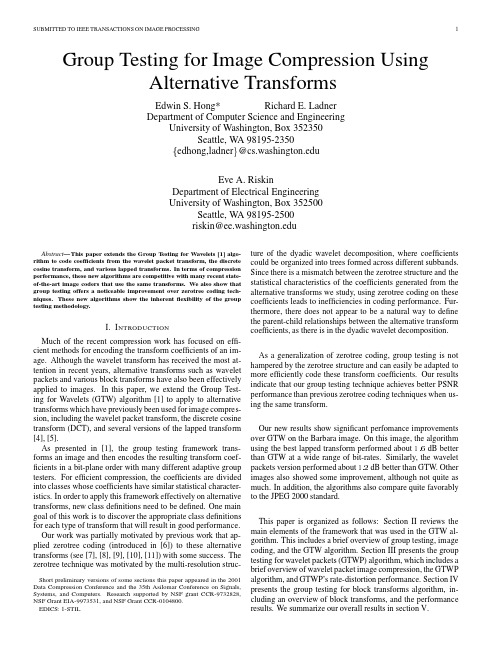
Group Testing for Image Compression Using Alternative TransformsEdwin S.Hong*Richard dnerDepartment of Computer Science and EngineeringUniversity of Washington,Box352350Seattle,W A98195-2350edhong,ladner@Eve A.RiskinDepartment of Electrical EngineeringUniversity of Washington,Box352500Seattle,W A98195-2500riskin@Abstract—This paper extends the Group Testing for Wavelets[1]algo-rithm to code coefficients from the wavelet packet transform,the discrete cosine transform,and various lapped transforms.In terms of compression performance,these new algorithms are competitive with many recent state-of-the-art image coders that use the same transforms.We also show that group testing offers a noticeable improvement over zerotree coding tech-niques.These new algorithms show the inherentflexibility of the group testing methodology.I.I NTRODUCTIONMuch of the recent compression work has focused on effi-cient methods for encoding the transform coefficients of an im-age.Although the wavelet transform has received the most at-tention in recent years,alternative transforms such as wavelet packets and various block transforms have also been effectively applied to images.In this paper,we extend the Group Test-ing for Wavelets(GTW)algorithm[1]to apply to alternative transforms which have previously been used for image compres-sion,including the wavelet packet transform,the discrete cosine transform(DCT),and several versions of the lapped transform [4],[5].As presented in[1],the group testing framework trans-forms an image and then encodes the resulting transform coef-ficients in a bit-plane order with many different adaptive group testers.For efficient compression,the coefficients are divided into classes whose coefficients have similar statistical character-istics.In order to apply this framework effectively on alternative transforms,new class definitions need to be defined.One main goal of this work is to discover the appropriate class definitions for each type of transform that will result in good performance. Our work was partially motivated by previous work that ap-plied zerotree coding(introduced in[6])to these alternative transforms(see[7],[8],[9],[10],[11])with some success.The zerotree technique was motivated by the multi-resolution struc-Short preliminary versions of some sections this paper appeared in the2001 Data Compression Conference and the35th Asilomar Conference on Signals, Systems,and Computers.Research supported by NSF grant CCR-9732828, NSF Grant EIA-9973531,and NSF Grant CCR-0104800.EDICS:1-STIL ture of the dyadic wavelet decomposition,where coefficients could be organized into trees formed across different subbands. Since there is a mismatch between the zerotree structure and the statistical characteristics of the coefficients generated from the alternative transforms we study,using zerotree coding on these coefficients leads to inefficiencies in coding performance.Fur-thermore,there does not appear to be a natural way to define the parent-child relationships between the alternative transform coefficients,as there is in the dyadic wavelet decomposition. As a generalization of zerotree coding,group testing is not hampered by the zerotree structure and can easily be adapted to more efficiently code these transform coefficients.Our results indicate that our group testing technique achieves better PSNR performance than previous zerotree coding techniques when us-ing the same transform.Our new results show significant perfomance improvements over GTW on the Barbara image.On this image,the algorithm using the best lapped transform performed about dB better than GTW at a wide range of bit-rates.Similarly,the wavelet packets version performed about dB better than GTW.Other images also showed some improvement,although not quite as much.In addition,the algorithms also compare quite favorably to the JPEG2000standard.This paper is organized as follows:Section II reviews the main elements of the framework that was used in the GTW al-gorithm.This includes a brief overview of group testing,image coding,and the GTW algorithm.Section III presents the group testing for wavelet packets(GTWP)algorithm,which includes a brief overview of wavelet packet image compression,the GTWP algorithm,and GTWP’s rate-distortion performance.Section IV presents the group testing for block transforms algorithm,in-cluding an overview of block transforms,and the performance results.We summarize our overall results in section V.II.G ROUP T ESTING FOR I MAGE C OMPRESSIONA.IntroductionGroup testing is a technique used for identifying a few sig-nificant items out of a large pool of items.In this framework, the significant items can be identified only through a series of group tests.A group test consists of picking a subset of items and testing them together.There are two possible outcomes of a group test on set:either is insignificant(meaning all items in are insignificant),or is significant(meaning there is at least one significant item in).The goal is to minimize the number of group tests required to identify all the significant items.In this paradigm,the cost of testing any set of items for significance is the same as the cost of testing a single item.As shown in[1],group testing can be viewed as a generalized form of zerotree coding,where the groups tested together do not have to be coefficients organized strictly into trees.The encoded output would simply be a series of bits representing the group test results;this is exactly like using bits to represent whether a tree of coefficients is significant in zerotree coding.Group test-ing for image compression replaces the zerotree coding process of a typical embedded zerotree coder with a technique based on group testing.B.Group Testing Framework OverviewIn our group testing framework,we follow the standard prac-tice of applying a linear transform to the image data,and then coding the transform coefficients.The transform coefficients are coded in a bit-plane by bit-plane fashion,with each bit-plane coded by two passes:a significance pass that identifies newly significant coefficients in the current bit-plane,and a refinement pass that gives an additional bit of precision to already signifi-cant coefficients.The significance pass uses an adaptive form of group testing based on group iterations(described in section II-C.1).Since this adaptive method is known to work well on i.i.d.sources, we try to ensure that the coefficients we code are approxi-mately i.i.d.We accomplish this by dividing the coefficients into classes,where each class is coded by a different adaptive group tester.The classes are designed so that coefficients within one class are well approximated by an i.i.d.source.Note that divid-ing the coefficients into classes is similar to choosing a different methods of coding coefficents based on its context.Since the statistical characteristics of the transform coeffi-cients depend on the transform used,the classes should be de-signed separately for each transform.In[1],the GTW classes were designed for the dyadic wavelet decomposition of an im-age.In this work,we design new classes for the alternative transforms that we use.We present several different definitions of classes in sections III-D.1III-D.2,and IV-E.1.For the purposes of obtaining good embedded performance, we code the classes in order of the probability of significance of their coefficients.Classes with coefficients that have a higher probability of being significant should be encodedfirst.Since the probability of significance of the coefficients in any class depends on the class definition,we must choose a method of ordering the classes that depends upon the class definition.C.Some Significance Pass DetailsWefirst describe group iterations,the method by which our significance pass is encoded.We then describe our adaptive group testing strategy.Finally,we then end this subsection with a description of how the group testing framework encodes the different classes using adaptive group testing.This section will only present an overview of our significance pass;for full de-tails,see[1].C.1Group IterationsA group iteration is a simple procedure that is given a set of items,and uses group tests to identify up to1significant item, and up to insignificant items.At the end of a group iteration, there may be some unidentified items in that must be tested in a future group iteration.If the set contains a significant item,the group iteration will use group tests in a recursive,binary search-like process to identify one significant item;otherwise it will use exactly one group test to identify set as containing only insignificant items.C.2Adaptive Group TestingWe adaptively pick the group iteration size depending upon the statistical characteristics of the items being encoded.We start out initially in a doubling phase,with group iteration size 1,and double the size of each successive group iteration as long as no significant items have yet been found.Once a significant item has been found,we move to the steady-state estimation phase,where we choose a group iteration size that results in op-timal coding performance based on our estimate of the probabil-ity of significance.Our estimate is calculated as the percentage of significant items seen so far.C.3Significance Pass AlgorithmAs previously described,our method divides the coefficients of one bit-plane into classes,and uses the previously described adaptive group testing technique to code each class.Given the class ordering and the definition of classes,the algorithm for encoding the significance pass is conceptually very simple: Pick thefirst class(according to the class ordering)that con-tains enough coefficients.Then perform a group iteration of size on that class,where is chosen according to the statistics in the adaptive group tester for that class.Then update the coeffi-cients as necessary with the information learned from the group tests(coefficients could change classes at this point).Finally, repeat this entire procedure until all coefficients are coded.III.G ROUP T ESTING FOR W AVELET P ACKETSA.Wavelet Packets BackgroundAs described in[12],wavelet packets are a generalization of the standard dyadic wavelet decomposition of a signal.The standard dyadic wavelet transform decomposes the signal by applying a series of successivefilters to the lowest frequency subband.Wavelet packets are a generalization of this where the successivefilters can be applied to any subband of any orienta-tion,not just the lowest frequency LL subband.Any one partic-ular choice of subbands to decompose is known as a basis;theHONG,LADNER,AND RISKIN:GROUP TESTING3 choice of exactly which basis to use depends on the characteris-tics of the input.Figure1shows the subbands after transformingan image with the wavelet packet transform using one particularbasis.Fig.1.Sample subbands of a wavelet packet-transformed image.A basis that adapts well to the input signal can be chosenvia Coifman and Wickerhauser’s entropy-based technique[13]or by Ramchandran and Vetterli’s rate-distortion optimizationtechnique[14].These methods work by fully decomposingall subbands to a predefined maximum depth,thus forming adecomposition tree where each decomposed subband is repre-sented in the tree by a parent node with four child nodes.Thenthe best basis is found by pruning this decomposition tree in a re-cursive bottom-up fashion.The entropy-based technique prunesthe tree to minimize the overall estimated entropy of the waveletpacket structure.The rate-distortion method is given a particulartarget bit rate for the image and prunes the tree to minimize thedistortion of the image.Xiong et al.[15]first explored the combination of a waveletpacket decomposition of an image with the space-frequencyquantization(SFQ)coder,a coder that uses zerotree quantiza-tion techniques.The difficulty in applying zerotree quantizationto wavelet packets is that it is no longer clear how to define theparent-child relationships in the trees.As noted by Rajpoot etal.[7],there is a parenting conflict,where some child coeffi-cients could have multiple parents.This problem has typicallybeen solved by limiting the space of possible wavelet packet de-compositions so that no parenting conflict occurs,or by assign-ing the parent-child relationships in a somewhat ad hoc manner(see[15],[7],[8]).B.Group Testing for Wavelet PacketsWe propose a new coder,Group Testing for Wavelet Pack-ets(GTWP),that applies our group testing framework to the wavelet packet transform.Thefirst step is tofind the best ba-sis for the input image,and encode the structure of this basis in thefirst bits of our compressed image.Then we define the GTWP classes based on thecharacteristics of the wavelet packet decomposition of the image,so that the classes are encoded ef-ficiently.Along with the class definition,we also specify the order in which we will code the classes.Once both the GTWP classes and the ordering between them are defined,then we can code each class with a different group tester,and proceed as de-scribed in the group testing framework for image compression. Wefirst describe how we choose the best basis and encode it; then we describe two different methods for defining the GTWP classes with their associated orderings.C.Best BasisWe investigated using both the entropy-based technique and the rate-distortion technique for computing the best wavelet packet basis.For the entropy-based technique,we explored many different metrics for calculating the entropy of a partic-ular subband.Let represent the value of the coefficients of a subband.Then the entropy metrics we tried are as follows: log energy metric:.Shannon metric(used in[13]):.L-norm metric:.threshold metric:Given a threshold value,calculate.first-order entropy metric(used in[8]):Given a quantization step size,divide the coefficients into quantization bins,and estimate the probability of a bin occurring by,where is the number of coefficients in that bin,and is the total number of coefficients.Calculate.We also tried the rate-distortion optimization technique,opti-mizing for a wide variety of bit-rates for various different possi-ble scalar quantizers.Note that this technique is not well suited to our problem because it forces us to pick artificial parameters, namely,thefinal bit-rate for which to optimize and the quantizer step sizes to consider.Since GTWP is an embedded coder,the final bit-rate we choose for the purpose of obtaining the best ba-sis does not correspond to the actualfinal bit-rate to which we encode the image.Furthermore,since GTWP codes the trans-form coefficients bit-plane by bit-plane,it cannot choose to code a subband with a particular quantizer step size;the step size it ends up using may not have any relation to the quantizer step size parameters that we chose to run the rate-distortion optimiza-tion technique.It is interesting to note that for the Barbara image,the optimal calculated quantizer step size for all the subbands under the rate-distortion technique differed from each other by no more than a factor of.In the bit-plane encoding technique,if we stop coding in the middle of a bit-plane,then the coefficients that have not yet been coded in the current bit-plane are quantized with a step size of times the step size of those coefficients that have been coded.This suggests that GTWP’s bit-plane encoding technique may be a good approximation to the quantization step sizes that the rate-distortion optimization best basis produces.Fig.2.Illustration of the best basis for the Barbara image.4SUBMITTED TO IEEE TRANSACTIONS ON IMAGE PROCESSINGThe log energy,Shannon,and L-norm metrics are the sim-plest in that they do not require additional parameters(such as threshold value or quantization step size)to compute.The top performers for our algorithm are the log energy metric and the rate-distortion optimization metric.Seeing that the log-energy metric was simpler and did not require selecting artificial pa-rameters,we used it exclusively.As an example,we show the best basis chosen by the log energy metric on the Barbara image infigure2.For simplicity,we show only levels of decomposi-tion even though our algorithm uses a maximum of levels. To encode the decomposition tree,we simply perform a depth-first traversal of the tree,and encode a when that par-ticular node is split into children,and a when the node is a leaf.D.GTWP ClassesTo illustrate theflexibility of the group testing methodology, we implemented two different ways of choosing the GTWP classes:GTWP-S and GTWP-J.Thefirst method is a simpli-fication of the definition GTW classes(S for Simple),and the second method is based on the contexts used in the JPEG-2000 image coder(J for JPEG-2000).D.1GTWP-SThe GTWP-S classes are a simplification of GTW classes and are defined by two different characteristics:the subband level and the significant neighbor metric.D.1.a Subband Level.The lowest frequency subband repre-senting the average of the entire image counts as subband level one.There is one additional subband level for each level of the wavelet transform.Figure3shows the subband levels when levels of the wavelet transform are performed.Note that because we are using wavelet packets,each subband level may contain more than actual subbands.Fig.3.Subband levels of a wavelet-packet transformed image in GTWP-S.Solid lines separate subband levels;dotted lines separate subbands.All shaded coefficients are in subband level.Subband levels,,and have ,,and subbands,respectively.D.1.b Significant Neighbor Metric.Tofinesse the problem of defining parent-child relationships in the wavelet packet trans-form,we restrict the neighbors of a coefficient to be one of up to spatially adjacent coefficients in the same subband.Like GTW,there are values in the significant neighbor metric,0,1, 2,and3+,depending on whether,,,or more than neigh-bors are significant.Because there are only up to neighbors, the maximum neighbor count is.Overall,there are subband levels and significant neighbor types resulting in classes total.Note that the subband level and significant neighbor metric are similar to the correspond-ing characteristics of the original GTW classes.Our new def-inition omits the pattern type characteristic found in the GTW classes,because using it did not produce significantly better re-sults.These classes are ordered according to the same ordering as the GTW classes,namely,with significant neighbor metric rated as more important than the subband level characteristic.D.2GTWP-JThe GTWP-J classes are based on the contexts in Taubman’s EBCOT coder[16],and are also found in the JPEG2000coder. In this class definition,there are only two characteristics that define the classes:the orientation type and the neighborhood significance label.D.2.a Orientation Type.The orientation type of subband contained in subband level is based on the orientation of the largest parent subband in subband level that contains. Here,the subband levels are defined as specified in the GTWP-S classes.There are only orientation types,LH(vertically high-pass),HL(horizontally high-pass),and HH subbands.The subband at subband level(the LL subband)is considered to have orientation type LH.The orientation type of a coefficient in subband is the orientation type of subband.This is illus-trated infigure4.Fig.4.Illustration of orientation type of a wavelet-packet transformed image.All shaded coefficients have orientation type HL.D.2.b Neighborhood Significance Label.Let,,and rep-resent the number of significant neighbors that a coefficient has which are adjacent to it horizontally,vertically,and diagonally, respectively.Thus,and both have a value of up to,whereas has a maximum value of.The neighborhood significance la-bel is assigned according to table I.Note that the labeling is dependent on the orientation type of the coefficient.This label is taken from the context classifier in the EBCOT coder.With orientation types and significant neighbor labels for each orientation type,there are a total of classes.The classes are ordered according to the group iteration size.Classes with smaller group iteration size are codedfirst,since they are more likely to be significant.Ties are broken arbitrarily.E.ResultsHere we present our results on some standard-bit monochrome images:the images Barbara,Goldhill,HONG,LADNER,AND RISKIN:GROUP TESTING 5TABLE IN EIGHBORHOOD SIGNIFICANCE LABEL .Assigned Labeland Lena (available from [17]);and a fingerprint im-age from the FBI’s fingerprint compression standard [18].We present results for several different algorithms,including GTW,GTWP-S,GTWP-J,JPEG 2000and SFQ-WP [19].All algo-rithms use the Daubechies /-tap filters [20].JPEG 2000re-sults were produced with a beta version of a codec [21]for the JPEG 2000image compression standard.SFQ-WP represents the practical version of Xiong et al.’s SFQ algorithm applied with wavelet packets;results are taken from [19].To our knowl-edge,SFQ-WP is the current state-of-the-art method for image compression with wavelet packets.paring performance of GTW,GTWP,and JPEG-2000.Figure 5compares the PSNR curves for GTW,GTWP-S,GTWP-J,and JPEG 2000on the Barbara image.As can be seen,there is little difference between the two GTWP variants,and using the wavelet packets increases the PSNR by about dB over GTW.Table II lists PSNR results for all four images on the different algorithms.The table shows that the amount of improvement for using wavelet packets instead of the dyadic wavelet decomposition is highly dependent on the type of image.Some images (like Bar-bara)benefit significantly from the wavelet packet decomposi-tion;some images (like Goldhill)benefit slightly;and some (like Lena)do not benefit at all.In fact,the best wavelet packet ba-sis for the Lena image was calculated to be the standard dyadic wavelet decomposition with one additional decomposition of a highest frequency subband.Thus,as expected,the results for GTWP on Lena are roughly the same as that for GTW.The slight performance differences are due mostly to differing significant neighbor metrics.If we compare our results with the published results of previ-ous zerotree coding techniques on wavelet packets,we see that outperform Rajpoot et al.’s technique by over dB on the image,and we outperform Khalil et al.’s techniqueaboutdB on the Barbara image.is interesting to note that there was little difference between and GTWP-J.It appears that as long as something rea-is chosen,the exact method of classifying coefficients neighbors are significant does not matter that much.In we also tested GTW-S,a version of GTW simplified so that significant neighbor metric did not include spatially identi-neighbors in different subbands (making GTW-S similar to There was also little difference between GTW-S and It appears that the significance of a coefficient depends al-entirely on its immediately adjacent neighbors,and very on the parents and other neighbors in different subbands.agrees with the findings in [16].can be seen in the table,GTWP’s performance is often than JPEG 2000,and never significantly worse.Further-GTWP’s performance is not too far from that of SFQ-WP.GTWP is worse,GTWP is an embedded coder,while SFQ-WP is not.Furthermore,GTWP is much simpler than SFQ in that it does not use arithmetic coding,and does not perform rate-distortion optimization.IV.G ROUP T ESTING FOR B LOCK T RANSFORMSIn this section,we show the results of applying our group testing framework to some standard block transforms.We first overview the use of block transforms for image compression.We then define the classes that we use for the block transforms,and conclude with a discussion of our results.6SUBMITTED TO IEEE TRANSACTIONS ON IMAGE PROCESSINGTABLE IIC OMPARING PSNR RESULTS OF VARIOUS ALGORITHMS,WITH BEST RESULTS IN BOLDFACE.GTW IS USED AS THE BASELINE,SO THAT FOR ALGORITHM,GTWRate(Bits/pixel)AlgorithmGTWJPEG2000GTWP-SGTWP-JSFQ-WPLenaNAGTWJPEG2000GTWP-SGTWP-JfingerprintA.Block Transform OverviewA.1Block Transform BackgroundWhen applying standard block transforms such as the DCT to images,the input pixels are divided into into blocks, and each block is separately transformed into an output block of size.The coefficient at position in each output block is known as the DC coefficient,and all other coefficients are known as AC coefficients.Note that the DC coefficient of a particular block represents the average of the value of the pixels in the corresponding input block.A lapped transform[4]is a generalization of the standard block transform where the input is divided into overlapping blocks of length,with each block transformed into an out-put block of size;we call this an lapped transform. An lapped transform can be computed by multiplying the input row vector of length with an size matrix repre-senting the transform,resulting in a output block of length.In a typical example where,each input data point is used in two adjacent output blocks.In this case,the inverse transform to recover one original block of input data points is computed by taking two adjacent output blocks of coefficients(coef-ficients total)and multiplying it with another matrix representing the inverse transform.In the two-dimensional case, we can view a lapped transform as mapping overlapping input blocks of size into output blocks of size. Unlike non-lapped block transforms,lapped transforms can take correlation between adjacent blocks into account;this makes it more efficient at decorrelating pped trans-forms can also reduce blocking artifacts because their basis functions decay smoothly to near zero at the boundaries.Both lapped orthogonal transforms(LOT)and lapped biorthogonal transforms(LBT)have been studied.LBT’s have more degrees of freedom than LOT’s since the biorthogonality condition is weaker than the orthogonality condition.Aase and Ramstad [22]have shown that these extra degrees of freedom can be used to design better lapped transforms for image coding.anization into SubbandsThe block-transform coefficients of an image are typically stored in a block-by-block fashion,so that the output of a block transform that uses blocks consists of angrid of blocks,where each block represents anblock of the original input image.However,we can conceptu-ally reorder the transform coefficients into a grid of subbands,each of size.This reordering puts all the DC coefficients into one subband,ordered so that the DC coefficient in block is at position in the DC subband.Simi-larly,there will be a separate subband for each AC coefficient; subband will contain AC coefficients from position within their block,ordered so that the AC coefficient at position in block will be located at position in subband .Figure6illustrates this reorganization when and.In this reorganized picture,each of the subbands repre-sents the entire original image at a different frequency decompo-sition.Note that with this organization,these subbands are simi-lar to the subbands from a dyadic wavelet decomposition in that coefficients in a subband represent the same frequency decom-position of an image over differing spatial locations.Further-more,the upper-left block of DC coefficients(seefigure6)rep-resents a postage-stamp size overview of the entire image,muchHONG,LADNER,AND RISKIN:GROUP TESTING7Block View Subband ViewFig.6.Block transform coefficients on the left are reorganized into subbands on the right.The DC coefficients are represented as circles and end up together in one subband;black coefficients from one block are scattered out to all subbands.like the lowest frequency subband in a dyadic wavelet decom-position.The principal difference between the dyadic wavelet decomposition and this reorganized block transform picture is that all the subbands from block-transforms are the same size, whereas in the wavelet transform,the subbands’sizes decrease by a factor of with every additional level of the DWT per-formed.In other words,block transforms offer a uniform-band frequency partitioning of the input,in contrast to the octave-band frequency partitioning of the wavelet transform(see[11]). For the DCT transform,the DC coefficient of an output block represents an average of the input block.Since adjacent image blocks often are similar,adjacent coefficients in the DC subband will be correlated.For the lapped transforms,each tra-ditional output block is computed from an input block of the original image.Most of the energy in the DC coef-ficient of the lapped transform is from the average of the entire block.Since blocks are overlapping,some image pixels are used in more than one average and contribute their energy to adjacent coefficients in the DC subband.C.Relation to the Wavelet TransformWith the subband organization,it becomes clear that we can also perform several levels of block transforms by recursively reapplying the block transform to the DC subband.We use the term hierarchical block transform to refer to any block trans-form scheme that decorrelates its DC subband by applying an-other transform.Note that hierarchical block transforms are similar to the levels of the DWT in a dyadic wavelet decomposi-tion.Since the DC subband represents a small low-resolution overview of the entire image,we expect there to be signifi-cant correlation in the DC subband.Hierarchically reapplying a block transform to the DC subband should decorrelate it further and enable better compression performance.We could continue to perform levels of the block transform as long as the lowest-frequency DC subband is not too small.Note that after every block transform step,we always reorganize the transform co-efficients so that a DC subband is always present.Also note that in principle,any transform could be used to decorrelate the DC subbands;in addition to the lapped transforms and the DCT, even a DWT could be used to decorrelate the DC subband.Another relationship between lapped transforms and the DWT is that a lapped transform can be thought of as a gener-alization of one level of the DWT.Recall that the output coef-ficients of a wavelet transform can be computed via convolu-tion.For a-tap wavelet transform,any one output coefficient depends on at most consecutive input coefficients.Thus,anlapped transform can use the overlap of data points on the input to compute the convolution of the input with the waveletfilter coefficients as would be done by the DWT.In other words,the DWT can be implemented as a lapped trans-form.Furthermore,hierarchical lapped transforms can com-pletely implement the DWTs that use many levels.In its full generality,hierarchical block transforms have the potential to perform better than the DWT.D.Previous Zerotree CodersThe most widespread image compression format using DCT is the standard JPEG[23]format.It uses DCT blocks. Xiong et al.’s Embedded Zerotree DCT algorithm(EZ-DCT) [9]applied the zerotree technique to the DCT-transformed coef-ficients of an image.Although the coefficients of a DCT trans-form are not naturally tree-structured,this coder showed that by imposing a somewhat arbitrary tree structure on the coefficients, reasonable performance could be achieved,certainly better than JPEG.Malvar applied the zerotree technique to lapped transform co-efficients[9],[10].He basically used the same method as EZ-DCT,but replaced the DCT transform with lapped transforms. He defined an LOT transform as well as a LBT transform that were optimized for both image compression ef-ficiency and low computational requirements.We use EZ-LOT (EZ-LBT)to refer to Xiong et al.’s embedded zerotree technique when applied to Malvar’s fast version of the LOT(LBT)trans-forms.Tran et al.[9],[10],[11]focused on designing the best lapped transforms for image compression,and did not consider the speed of computation to be a crucial factor.They designed sev-eral lapped transforms,including the generalized LBT (GLBT).This transform was optimized solely for good coding performance on images.Tran et ed a hierarchical coder。
fast approximate energy minimization via graph cuts
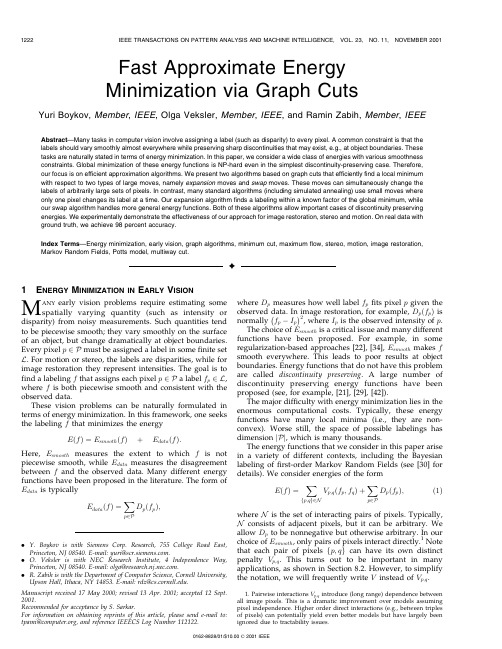
æ
1
ANY early vision problems require estimating some spatially varying quantity (such as intensity or disparity) from noisy measurements. Such quantities tend to be piecewise smooth; they vary smoothly on the surface of an object, but change dramatically at object boundaries. Every pixel p P must be assigned a label in some finite set v. For motion or stereo, the labels are disparities, while for image restoration they represent intensities. The goal is to find a labeling f that assigns each pixel p P a label fp P v, where f is both piecewise smooth and consistent with the observed data. These vision problems can be naturally formulated in terms of energy minimization. In this framework, one seeks the labeling f that minimizes the energy
AbstractÐMany tasks in computer vision involve assigning a label (such as disparity) to every pixel. A common constraint is that the labels should vary smoothly almost everywhere while preserving sharp discontinuities that may exist, e.g., at object boundaries. These tasks are naturally stated in terms of energy minimization. In this paper, we consider a wide class of energies with various smoothness constraints. Global minimization of these energy functions is NP-hard even in the simplest discontinuity-preserving case. Therefore, our focus is on efficient approximation algorithms. We present two algorithms based on graph cuts that efficiently find a local minimum with respect to two types of large moves, namely expansion moves and swap moves. These moves can simultaneously change the labels of arbitrarily large sets of pixels. In contrast, many standard algorithms (including simulated annealing) use small moves where only one pixel changes its label at a time. Our expansion algorithm finds a labeling within a known factor of the global minimum, while our swap algorithm handles more general energy functions. Both of these algorithms allow important cases of discontinuity preserving energies. We experimentally demonstrate the effectiveness of our approach for image restoration, stereo and motion. On real data with ground truth, we achieve 98 percent accuracy. Index TermsÐEnergy minimization, early vision, graph algorithms, minimum cut, maximum flow, stereo, motion, image restoration, Markov Random Fields, Potts model, multiway cut.
恩格列净使用剂量对2_型糖尿病治疗效果和不良反应的影响研究
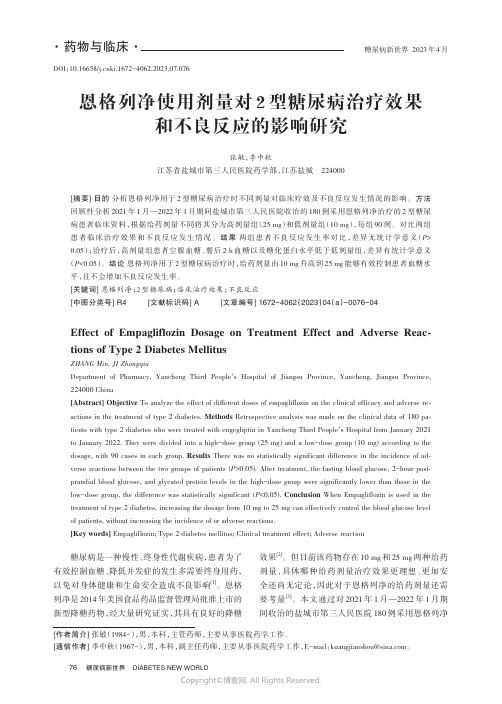
恩格列净使用剂量对2型糖尿病治疗效果和不良反应的影响研究张敏,季中秋江苏省盐城市第三人民医院药学部,江苏盐城224000[摘要]目的分析恩格列净用于2型糖尿病治疗时不同剂量对临床疗效及不良反应发生情况的影响。
方法回顾性分析2021年1月—2022年1月期间盐城市第三人民医院收治的180例采用恩格列净治疗的2型糖尿病患者临床资料,根据给药剂量不同将其分为高剂量组(25 mg)和低剂量组(10 mg),每组90例。
对比两组患者临床治疗效果和不良反应发生情况。
结果两组患者不良反应发生率对比,差异无统计学意义(P> 0.05);治疗后,高剂量组患者空腹血糖、餐后2 h血糖以及糖化蛋白水平低于低剂量组,差异有统计学意义(P<0.05)。
结论恩格列净用于2型糖尿病治疗时,给药剂量由10 mg升高到25 mg能够有效控制患者血糖水平,且不会增加不良反应发生率。
[关键词] 恩格列净;2型糖尿病;临床治疗效果;不良反应[中图分类号] R4 [文献标识码] A [文章编号] 1672-4062(2023)04(a)-0076-04Effect of Empagliflozin Dosage on Treatment Effect and Adverse Reac⁃tions of Type 2 Diabetes MellitusZHANG Min, JI ZhongqiuDepartment of Pharmacy, Yancheng Third People's Hospital of Jiangsu Province, Yancheng, Jiangsu Province, 224000 China[Abstract] Objective To analyze the effect of different doses of empagliflozin on the clinical efficacy and adverse re⁃actions in the treatment of type 2 diabetes. Methods Retrospective analysis was made on the clinical data of 180 pa⁃tients with type 2 diabetes who were treated with engegliptin in Yancheng Third People's Hospital from January 2021 to January 2022. They were divided into a high-dose group (25 mg) and a low-dose group (10 mg) according to the dosage, with 90 cases in each group. Results There was no statistically significant difference in the incidence of ad⁃verse reactions between the two groups of patients (P>0.05). After treatment, the fasting blood glucose, 2-hour post⁃prandial blood glucose, and glycated protein levels in the high-dose group were significantly lower than those in the low-dose group, the difference was statistically significant (P<0.05). Conclusion When Empagliflozin is used in the treatment of type 2 diabetes, increasing the dosage from 10 mg to 25 mg can effectively control the blood glucose level of patients, without increasing the incidence of or adverse reactions.[Key words] Empagliflozin; Type 2 diabetes mellitus; Clinical treatment effect; Adverse reaction糖尿病是一种慢性、终身性代谢疾病,患者为了有效控制血糖、降低并发症的发生多需要终身用药,以免对身体健康和生命安全造成不良影响[1]。
跳过序列:在调查问卷中的一个重要问题
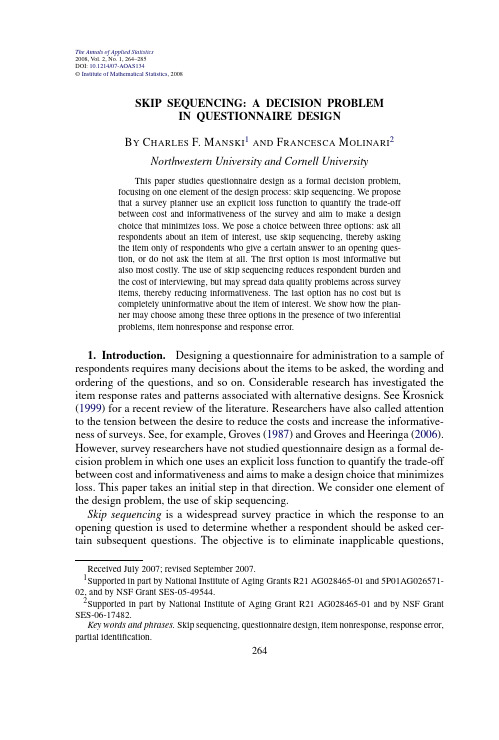
The Annals of Applied Statistics2008,V ol.2,No.1,264–285DOI:10.1214/07-AOAS134©Institute of Mathematical Statistics,2008SKIP SEQUENCING:A DECISION PROBLEMIN QUESTIONNAIRE DESIGNB YC HARLES F.M ANSKI1AND F RANCESCA M OLINARI2Northwestern University and Cornell UniversityThis paper studies questionnaire design as a formal decision problem, focusing on one element of the design process:skip sequencing.We proposethat a survey planner use an explicit loss function to quantify the trade-offbetween cost and informativeness of the survey and aim to make a designchoice that minimizes loss.We pose a choice between three options:ask allrespondents about an item of interest,use skip sequencing,thereby askingthe item only of respondents who give a certain answer to an opening ques-tion,or do not ask the item at all.Thefirst option is most informative butalso most costly.The use of skip sequencing reduces respondent burden andthe cost of interviewing,but may spread data quality problems across surveyitems,thereby reducing informativeness.The last option has no cost but iscompletely uninformative about the item of interest.We show how the plan-ner may choose among these three options in the presence of two inferentialproblems,item nonresponse and response error.1.Introduction.Designing a questionnaire for administration to a sample of respondents requires many decisions about the items to be asked,the wording and ordering of the questions,and so on.Considerable research has investigated the item response rates and patterns associated with alternative designs.See Krosnick (1999)for a recent review of the literature.Researchers have also called attention to the tension between the desire to reduce the costs and increase the informative-ness of surveys.See,for example,Groves(1987)and Groves and Heeringa(2006). However,survey researchers have not studied questionnaire design as a formal de-cision problem in which one uses an explicit loss function to quantify the trade-off between cost and informativeness and aims to make a design choice that minimizes loss.This paper takes an initial step in that direction.We consider one element of the design problem,the use of skip sequencing.Skip sequencing is a widespread survey practice in which the response to an opening question is used to determine whether a respondent should be asked cer-tain subsequent questions.The objective is to eliminate inapplicable questions, Received July2007;revised September2007.1Supported in part by National Institute of Aging Grants R21AG028465-01and5P01AG026571-02,and by NSF Grant SES-05-49544.2Supported in part by National Institute of Aging Grant R21AG028465-01and by NSF Grant SES-06-17482.Key words and phrases.Skip sequencing,questionnaire design,item nonresponse,response error, partial identification.264SKIP SEQUENCING265 thereby reducing respondent burden and the cost of interviewing.However,skip sequencing can amplify data quality problems.In particular,skip sequencing ex-acerbates the identification problems caused by item nonresponse and response errors.A respondent may not answer the opening question.When this happens,a com-mon practice is to label the subsequent questions as inapplicable.However,they may be applicable,in which case the item nonresponse problem is amplified.An-other practice is to impute the answer to the opening question and,if the imputation is positive,to also impute answers to the subsequent questions.Some of these im-putations will inevitably be incorrect.A particularly odd situation occurs when the answer to the opening question should be negative but the imputation is positive. Then answers are imputed to subsequent questions that actually are inapplicable.A respondent may answer the opening question with error.An error may cause subsequent questions to be skipped,when they should be asked,or vice versa. An error of thefirst type induces nonresponse to the subsequent questions.The consequences of an error of the second type depend on how the respondent answers the subsequent questions,having answered the opening one incorrectly.I LLUSTRATION1.The2006wave of the Health and Retirement Study(HRS) asked current Social Security recipients about their expectations for the future of the Social Security system.An opening question asked broadly:“Thinking of the Social Security program in general and not just your own Social Security benefits: On a scale from0to100(where0means no chance and100means absolutely certain),what is the percent chance that Congress will change Social Security sometime in the next10years,so that it becomes less generous than now?”If the answer was a number greater than zero,a follow-up question asked“We just asked you about changes to Social Security in general.Now we would like to know whether you think these Social Security changes might affect your own benefits. On a scale from0to100,what do you think is the percent chance that the benefits you yourself are receiving from Social Security will be cut some time over the next 10years?”If a person did not respond to the opening question or gave an answer of0,the follow-up question was not asked.I LLUSTRATION2.The1990wave of the National Longitudinal Survey of Older Men(NLSOM)queried respondents about their limitations in activities of daily living(ADLs).An opening question asked broadly:“Because of a health or physical problem,do you ever need help from anyone in looking after personal care such as dressing,bathing,eating,going to the bathroom,or other such daily activities?”If the answer was positive,the respondent was then asked if he/she re-ceives help from another person in each of six specific ADLs(bathing/showering, dressing,eating,getting in or out of a chair or bed,walking,using the toilet).If the answer was negative or missing,the subsequent questions were skipped out.266 C.F.MANSKI AND F.MOLINARIThese illustrative uses of skip sequencing save survey costs by asking a broad questionfirst and by following up with a more specific question only when the answer to the broad question meets specified criteria.However,nonresponse or response error to the opening question may compromise the quality of the data obtained.This paper studies skip sequencing as a decision problem in questionnaire de-sign.We suppose that a survey planner is considering whether and how to ask about an item of interest.Three design options follow:Option All(A):ask all respondents the question.Option Skip(S):ask only those respondents who respond positively to an opening question.Option None(N):do not ask the question at all.These options vary in the cost of administering the questions and in the informa-tiveness of the data they yield.Option(A)is most costly and is potentially most informative.Option(S)is less costly but may be less informative if the opening question has nonresponse or response errors.Option(N)has no cost but is un-informative about the item of interest.We suppose that the planner must choose among these options,weighing cost and informativeness as he deems appropriate. We suggest an approach to this decision problem and give illustrative applications.The paper is organized as follows.As a prelude,Section2summarizes the few precedent studies that consider the data quality aspects of skip sequencing.These studies do not analyze skip sequencing as a decision problem.Section3formalizes the problem of choice among design options.We assume that the survey planner wants to minimize a loss function whose value depends on the cost of a design option and its informativeness.Thus,evaluation of the design options requires that the planner measure their cost and informativeness.Suppose that a planner wants to combine sample data on an item with specified assumptions in order to learn about a population parameter of interest.When the sample size is large,we propose that informativeness be measured by the size of the identification region that a design option yields for this parameter.As explained in Manski(2003),the identification region for the parameter is the set of values that remain feasible when unlimited observations from the sampling process are com-bined with the maintained assumptions.The parameter is point-identified when this set contains a single value and is partially identified when the set is smaller than the parameter’s logical range,but is not a single point.In survey settings with large samples of respondents,where identification rather than statistical inference is the dominant inferential problem,we think it natural to measure informativeness by the size of the identification region.The smaller the identification region,the better.Section6discusses measurement of informativeness when the sample size is small.Then confidence intervals for the partially identified parameter may be used to measure informativeness.SKIP SEQUENCING267 Sections4and5apply the general ideas of Section3in two polar settings hav-ing distinct inferential problems.Section4studies cases in which there may be nonresponse to the questions posed but it is assumed that there are no response errors.Wefirst derive the identification regions under options A,S and N.We then show the circumstances in which a survey planner should choose each option. To illustrate,we consider choice among options for querying respondents about their expectations for future personal Social Security benefits.The HRS2006used skip sequencing,as described in Illustration1.Another option would be to ask all respondents both the broad and the personal question.A third option would be to ask only the broad question,omitting the one about future personal benefits.Section5studies the other polar setting in which there is full response but there may be response errors.Again,wefirst derive the identification regions under the three design options and then show when a survey planner should choose each option.To illustrate,we consider choice among options for querying respondents about limitations in ADLs.The NLSOM used skip sequencing,as described in Illustration2.Another survey,the1993wave of the Assets and Health Dynamics Among the Oldest Old(AHEAD)asked all respondents about a set of specific ADLs.A third option would be to not ask about specific ADLs at all.Section6concludes by calling for further analysis of questionnaire design as a decision problem.2.Previous studies of skip sequencing.As far as we are aware,there has been no precedent research studying skip sequencing as a decision problem in questionnaire design.Messmer and Seymour(1982)and Hill(1991,1993)are the only precedent studies recognizing that skip sequencing may amplify data quality problems.Messmer and Seymour studied the effect of skip sequencing on item nonre-sponse in a large scale mail survey.Their analysis asked whether the difficult structure of the survey,particularly the fact that respondents were instructed to skip to other questions perhaps several pages away in the questionnaire,increased the number of unanswered questions.Their analysis indicates that branching in-structions significantly increased the rate of item nonresponse for questions fol-lowing a branch,and that this effect was higher for older individuals.This work is interesting but it does not have direct implications for modern surveys,where skip sequencing is automated rather than performed manually.Hill used data fromfive interview/reinterview sequence pairs in the1984Survey of Income and Program Participation(SIPP)Reinterview Program.He examined data errors that manifest themselves through a discrepancy between the responses given in the two interviews,and categorized these discrepancies in three groups.In his terminology,a response discrepancy occurs when a different answer is recorded for an opening question in the interview and in the reinterview.A response induced sequencing discrepancy occurs when,as a consequence of different answers to the268 C.F.MANSKI AND F.MOLINARIopening question,a subsequent question is asked in only one of the two inter-views.A procedurally induced sequencing discrepancy occurs when,in one of the two interviews but not both,an opening question is not asked and,therefore,the subsequent question is not asked either.Hill used a discrete contagious regression model to assess the relative impor-tance of these errors in reducing data quality.The contagion process was used to express the idea that error spreads from one question to the next via skip sequenc-ing.Within this model,the“conditional population at risk of contagion”expresses the idea that the number of remaining questions in the sequence at the point where the initiating error occurs gives an upper bound on the number of errors that can be induced.Hill’s results suggest that the losses of data reliability caused by in-duced sequencing errors are at least as large as those induced by response errors. Moreover,the relative importance of sequencing errors strongly increases with the sequence length.This suggests that the reliability of individual items will be lower, all else equal,the later they appear in the sequence.3.A formal design problem.3.1.The choice setting.We pose here a formal questionnaire design problem that highlights how skip sequencing may affect data quality.To focus on this mat-ter,wefind it helpful to simplify the choice setting in three major respects.First,we suppose that a large random sample of respondents is drawn from a much larger population.This brings identification to the fore as the dominant inferential problem,the statistical precision of sample estimates receding into the background as a minor concern.We also suppose that all sample members agree to be interviewed.Hence,inferential problems arise only from item nonresponse and response errors,not from interview nonresponse.Second,we perform a“marginalist”analysis that supposes the entire design of the questionnaire has been set except for one item.The only decision is whether and how to ask about this item.Marginalist analysis enormously simplifies the de-cision problem.In practice,a survey planner must choose the entire structure of the questionnaire,and the choice made about one item may interact with choices made about others.We recognize this but,nevertheless,find it useful for exposi-tion to focus on a single aspect of the global design problem,holdingfixed the remainder of the questionnaire.Third,we assume that the design chosen for the specific item in our marginalist analysis affects only the informativeness of that item.In practice,the choice of how to ask a specific item affects the length of the entire survey,which may influence respondents’willingness or ability to provide reliable responses to other items. We recognize this but,nevertheless,find it useful for exposition to suppose that the effect on other items is negligible.Let y denote the item under consideration.As indicated in the Introduction,the design options are as follows:SKIP SEQUENCING269 A:ask all respondents to report y.S:ask only those respondents who respond positively to an opening ques-tion.N:do not ask about y at all.The population parameter of interest is labeledτ[P(y)],where P is the pop-ulation distribution of y.For example,τ[P(y)]might be the population mean ormedian value of y.3.2.Measuring the cost,informativeness,and loss of the design options.Thedesign options differ in their costs and in their informativeness aboutτ[P(y)].Abstractly,let c k denote the cost of option k,let d k denote its informativeness,andlet L k=L(c k,d k)be the loss that the survey planner associates with option k.We suppose that the planner wants to choose a design option that minimizes L(c k,d k)over k∈(A,S,N).To operationalize this abstract optimization problem,a survey planner must de-cide how to measure loss,cost,and informativeness.Loss presumably increaseswith cost and decreases with informativeness.We will not be more specific aboutthe form of the loss function here.We will,for simplicity,use a linear form in ourapplications.Cost presumably increases with the fraction of respondents who are asked theitem.In some settings,cost may be proportional to this fraction.Then c k=γf k, whereγ>0is the cost per respondent of data collection and f k is the fraction of respondents asked the item under option k.It is the case that1=f A≥f S≥f N= 0.Hence,c A=γ,c S=γf S,c N=0.As indicated in the Introduction,we propose measurement of the informative-ness of a design option by the size of the identification region obtained for theparameter of interest.In general,the size of an identification region depends onthe specified parameter,the data produced by a design option,and the assumptionsthat the planner is willing to maintain.Sections4and5show how in some leadingcases.4.Question design with nonresponse.This section examines how nonre-sponse affects choice among the three design options.To focus attention on the in-ferential problem created by nonresponse,we assume that when sample members do respond,all answers are accurate.Section4.1considers identification of the parameterτ[P(y)].Section4.2shows how to use thefindings to choose a design. Section4.3uses questions on future generosity of Social Security to illustrate.4.1.Identification with nonresponse.It has been common in survey researchto impute missing values and to use these imputations as if they are real data.Stan-dard imputation methods presume that data are missing at random(MAR),condi-tional on specified observable covariates;see Little and Rubin(1987).If the main-tained MAR assumptions are correct,then parameterτ[P(y)]is point-identified270 C.F.MANSKI AND F.MOLINARIunder both of design options A and S.Option S is less costly,so there is no reasonto contemplate option A from the perspective of identification.If option A is usedin practice,the reason must be to provide a larger sample of observations in orderto improve statistical inference.Identification becomes the dominant concern when,as is often the case,a surveyplanner has only a weak understanding of the distribution of missing data.Wefocus here on the worst-case setting,in which the planner knows nothing at allabout the missing data.It is straightforward to determine the identification regionforτ[P(y)]under design options A and S.We draw on Manski[(2003),Chapter1]to show how.Option A.To formalize the identification problem created by nonresponse,let each member j of a population J have an outcome y j in a space Y≡[0,s].Heres can befinite or can equal∞,in which case Y is the nonnegative part of theextended real line.The assumption that y is nonnegative is not crucial for ouranalysis,but it simplifies the exposition and notation.The population is a probability space and y:J→Y is a random variable withdistribution P(y).Let a sampling process draw persons at random from J.How-ever,not all realizations of y are observable.Let the realization of a binary randomvariable z A y indicate observability;y is observable if z A y=1and not observable if z A y=0.The superscript A shows the dependence of observability of y on design option A.By the Law of Total Probability,(1)P(y)=P(y|z A y=1)P(z A y=1)+P(y|z A y=0)P(z A y=0).The sampling process reveals P(y|z A y=1)and P(z A y),but it is uninformative regarding P(y|z A y=0).Hence,the sampling process partially identifies P(y).In particular,it reveals that P(y)lies in the identification region(2)H A[P(y)]≡[P(y|z A y=1)P(z A y=1)+ψP(z A y=0),ψ∈ Y].Here Y is the space of all probability distributions on Y and the superscript A onH shows the dependence of the identification region on the design option.The identification region for a parameter of P(y)follows immediately fromH A[P(y)].Consider inference on the parameterτ[P(y)].The identification region consists of all possible values of the parameter.Thus,(3)H A{τ[P(y)]}≡{τ(η),η∈H A[P(y)]}.Result(3)is simple but is too abstract to be useful as stated.Research on par-tial identification has sought to characterize H A{τ[P(y)]}for different parame-ters.Manski(1989)does this for means of bounded functions of y,Manski(1994) for quantiles,and Manski[(2003),Chapter1]for all parameters that respectfirst-order stochastic dominance.Blundell et al.(2007)and Stoye(2005)characterizeSKIP SEQUENCING271 the identification regions for spread parameters such as the variance,interquartile range and the Gini coefficient.The results for means of bounded functions are easy to derive and instructive, so we focus on these parameters here.To further simplify the exposition,we re-strict attention to monotone functions.Let be the extended real line.Let g(·)be a monotone function that maps Y into and that attainsfinite lower and upper bounds g0≡min y∈Y g(y)=g(0)and g1≡max y∈Y g(y).Without loss of gener-ality,by a normalization,we set g0=0and g1=1.The problem of interest is toinfer E[g(y)].The Law of Iterated Expectations givesE[g(y)]=E[g(y)|z A y=1]P(z A y=1)+E[g(y)|z A y=0]P(z A y=0). (4)The sampling process reveals E[g(y)|z A y=1]and P(z A y),but it is uninformative regarding E[g(y)|z A y=0],which can take any value in the interval[0,1].Hence, the identification region for E[g(y)]is the closed intervalH A{E[g(y)]}=E[g(y)|z A y=1]P(z A y=1),(5)E[g(y)|z A y=1]P(z A y=1)+P(z A y=0) .H A{E[g(y)]}is a proper subset of[0,1]whenever P(z A y=0)is less than one.The width of the region is P(z A y=0).Thus,the severity of the identification problem varies directly with the prevalence of missing data.Option S.There are two sources of nonresponse under option S.First,a sam-ple member may not respond to the opening question,in which case she is not asked about item y.Second,a sample member may respond to the opening ques-tion but not to the subsequent question about item y.Let x denote the item whose value is sought in the opening question.As in Il-lustrations1and2,we suppose that x is a broad item and that y is a more specific one.For simplicity,we suppose here that x∈{0,1}and that x=0 ⇒y=0.A respondent is asked about y only if she answers the opening question and re-ports x=1.For example,consider Illustration2discussed in the Introduction.If a respondent does not have any limitation in ADLs(x=0),then clearly the respon-dent does not have a limitation in bathing/showering(y=0).Hence,the NLSOM asks about y only when a respondent reports x=1.To formalize the identification problem,we need two response indicators,z S x and z S y,the superscript S showing the dependence of nonresponse on design op-tion S.Let z S x=1if a respondent answers the opening question and let z S x=0 otherwise.Let z S y=1if a respondent who is asked the follow-up question gives a response,with z S y=0otherwise.Hence,z S y=1 ⇒z S x=1.This and the Law of272 C.F.MANSKI AND F.MOLINARIIterated Expectations and the fact that g(0)=0giveE [g(y)]=E [g(y)|x =1]P (x =1)+E [g(y)|x =0]P (x =0)=E [g(y)|x =1,z S y=1]P (z S y =1,x =1)+E [g(y)|x =1,z S x=1,z S y =0]P (z S x =1,z S y =0,x =1)+E [g(y)|x =1,z S x=0]P (z S x =0,x =1).The sampling process reveals E [g(y)|x =1,z S y =1],P (z S x =1,z S y =0,x =1),and P (z S y =1)=P (z S y =1,x =1),where the last equality holds because z S y =1 ⇒x =1.The data are uninformative about E [g(y)|x =1,z S x =1,z S y =0]and E [g(y)|x =1,z S x =0],which can take any values in [0,1].The data are partially informative about P (z S x =0,x =1),which can take any value in [0,P (z S x =0)].It follows that the identification region for E [g(y)]is the closed intervalH S {E [g(y)]}=E [g(y)|z S y =1]P (z S y =1),E [g(y)|z S y=1]P (z S y =1)(6)+P (z S x =1,z S y =0,x =1)+P (z S x =0).Thus,the severity of the identification problem varies directly with the prevalence of nonresponse to the opening question and to the follow-up question in the sub-population in which it is asked.4.2.Choosing a design.Now consider choice among the three design options (A,S,N).The widths of the identification regions for E [g(y)]under these options are as follows:d A =P (z A y =0),d S =P (z S x =1,z S y =0,x =1)+P (z S x =0),d N =1.For specificity,let the loss function have the linear form L k =γf k +d k .The first component measures survey cost and the second measures the informativeness of the design option.We set the coefficient on d k equal to one as a normalization of scale.The parameter γmeasures the importance that the survey planner gives to cost relative to informativeness.There is no universally “correct”value of this parameter.Its value is something that the survey planner must specify,depending on the survey context and the nature of item y .It follows from the above and from the derivations of Section 4.1that the losses associated with the three design options are as follows:L A =γ+P (z A y=0),L S =γP (z S x =1,x =1)+P (z S x =1,z S y =0,x =1)+P (z S x =0),L N =1.SKIP SEQUENCING273 Thus,it is optimal to administer item y to all sample members ifγ+P(z A y=0)≤min{1,γP(z S x=1,x=1)+P(z S x=1,z S y=0,x=1)+P(z S x=0)}.Skip sequencing is optimal ifγP(z S x=1,x=1)+P(z S x=1,z S y=0,x=1)+P(z S x=0)≤min{1,γ+P(z A y=0)}.If neither of these inequalities hold,it is optimal not to ask the item at all.Determination of the optimal design option requires knowledge of the response rates that would occur under options A and S.This is where the body of survey research reviewed by Krosnick(1999)has a potentially important role to play. Through the use of randomized experiments embedded in surveys,researchers have developed considerable knowledge of the response rates that occur when var-ious types of questions are posed to diverse populations.In many cases,this body of knowledge can be brought to bear to provide credible values for the response rates that determine loss under options A and S.When the literature does not provide credible values for these response rates,a survey planner may want to perform his own pretest,randomly assigning sample members to options A and S.The size of the pretest sample only needs to be large enough to determine with reasonable confidence which design option is best.It does not need to be large enough to give precise estimates of the response rates.4.3.Questioning about expectations on the generosity of social security.Con-sider the questions on expectations for the future generosity of the Social Security program cited in Illustration1.The opening question was posed to10,748re-spondents to the2006HRS who currently receive social security benefits,and the follow-up was asked to the sub-sample of9356persons who answered the opening question and gave a response greater than zero.We assume here that the only data problem is nonresponse.The nonresponse rate to the opening question was7.23%. The nonresponse rate to the follow-up question,for the subsample asked this ques-tion,was2.27%.It is plausible that someone may not be willing to respond to the first question and yet be willing to respond to the second one.In particular,this would happen if a person does not want to speculate on what Congress will do but, nevertheless,is sure that if Congress does act,it would only change benefits for fu-ture retirees,not for those already in the system.The HRS use of skip sequencing prevents observation of y in such cases.To cast this application into the notation of the previous section,we let x=1if a respondent places a positive probability on Congress acting,with x=0otherwise. The rest of the notation is the same as above.An early release of the HRS data provide these empirical values for the quan-tities that determine the identification region for E[g(y)]and loss under design option S:P(z S x=1,z S y=0,x=1)=0.0197,P(z S x=1,x=1)=0.8705,P(z S y=1)=0.8508,P(z S x=0)=0.0723,E[g(y)|z S y=1]=0.4039,where g(y)≡y100.Hence,the identification region for E[g(y)]under option S isH S{E[g(y)]}=[0.3436,0.4356]and loss is L S=0.8705γ+0.0920.The HRS data do not reveal the quantities that determine the identification re-gion for E[g(y)]and loss under design option A.For this illustration,we con-jecture that the mean response to item y that would be obtained under option A equals the mean response that is observed under option S.Thus,E[g(y)|z A y= 1]=0.4039.We suppose further that the nonresponse probability would be P(z A y=0)=0.08.Then the identification region for E[g(y)]under option A is H A{E[g(y)]}=[0.3716,0.4516]and loss is L A=γ+0.08.It follows from the above that it is optimal to administer item y to all sample members ifγ≤0.0927.Skip sequencing is optimal if0.0927≤γ≤1.0431.If neither of these inequalities hold,it is optimal not to ask the item at all.5.Question design with data errors.This section examines how response errors affect choice among the three design options.To focus attention on the infer-ential problem created by such errors,we assume that all sample members respond to the questions posed.Section5.1considers identification.Section5.2shows how to use thefindings to choose a design.Section5.3uses questions on limitations in ADLs to illustrate.5.1.Identification with response errors.Section4showed that assumptions about the distribution of missing data are unnecessary for partially informative inference in the presence of nonresponse.In contrast,assumptions on the nature or prevalence of response errors are a prerequisite for inference.In cases where y is discrete,it is natural to think of data errors as classification errors.We con-ceptualize response error here through a misclassification model previously used。
Do Multinational Enterprises Relocate Employment to Low Wage Regions
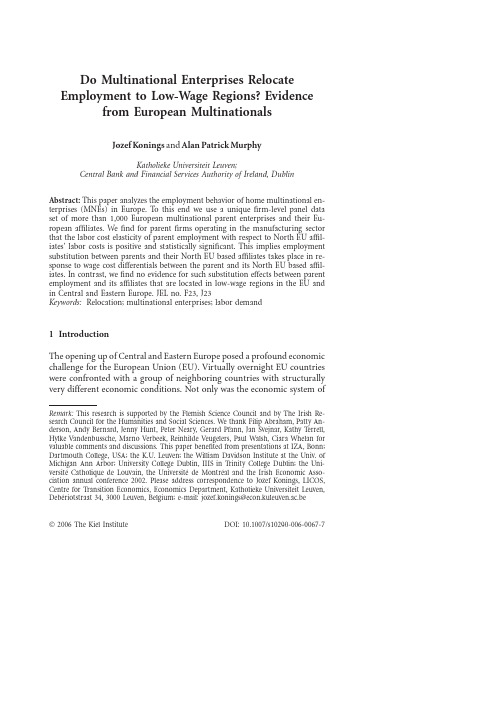
Do Multinational Enterprises Relocate Employment to Low-Wage Regions?Evidence from European MultinationalsJozef Konings and Alan Patrick MurphyKatholieke Universiteit Leuven;Central Bank and Financial Services Authority of Ireland,Dublin Abstract:This paper analyzes the employment behavior of home multinational en-terprises(MNEs)in Europe.T o this end we use a uniquefirm-level panel data set of more than1,000European multinational parent enterprises and their Eu-ropean affiliates.Wefind for parentfirms operating in the manufacturing sector that the labor cost elasticity of parent employment with respect to North EU affil-iates’labor costs is positive and statistically significant.This implies employment substitution between parents and their North EU based affiliates takes place in re-sponse to wage cost differentials between the parent and its North EU based affil-iates.In contrast,wefind no evidence for such substitution effects between parent employment and its affiliates that are located in low-wage regions in the EU and in Central and Eastern Europe.JEL no.F23,J23Keywords:Relocation;multinational enterprises;labor demand1IntroductionThe opening up of Central and Eastern Europe posed a profound economic challenge for the European Union(EU).Virtually overnight EU countries were confronted with a group of neighboring countries with structurally very different economic conditions.Not only was the economic system of Remark:This research is supported by the Flemish Science Council and by The Irish Re-search Council for the Humanities and Social Sciences.We thank Filip Abraham,Patty An-derson,Andy Bernard,Jenny Hunt,Peter Neary,Gerard Pfann,Jan Svejnar,Kathy T errell, Hylke Vandenbussche,Marno Verbeek,Reinhilde Veugelers,Paul Walsh,Ciara Whelan for valuable comments and discussions.This paper benefited from presentations at IZA,Bonn; Dartmouth College,USA;the K.U.Leuven;the William Davidson Institute at the Univ.of Michigan Ann Arbor;University College Dublin,IIIS in Trinity College Dublin;the Uni-versitéCatholique de Louvain,the Universitéde Montréal and the Irish Economic Asso-ciation annual conference2002.Please address correspondence to Jozef Konings,LICOS, Centre for Transition Economics,Economics Department,Katholieke Universiteit Leuven, Debériotstraat34,3000Leuven,Belgium;e-mail:jozef.konings@econ.kuleuven.ac.be©2006The Kiel Institute DOI:10.1007/s10290-006-0067-7268Review of World Economics2006,Vol.142(2)the Central and Eastern European Countries(CEEC)built on nearly50 years of centrally based planning.Even more importantly from the EU’s perspective was the huge gap in income,wages,and productivity between the two regions.The demise of the Communist legacy represented an abrupt shock,especially when compared to the gradual process that characterizes post-war West European integration.Most of the policy concerns relate to employment,because Eastern Europe represents a large reservoir of low-wage labor in the EU’s backyard.In light of the above,one concern is that low-wage import competition from the CEEC may result in job losses in EU member states.Alternatively, EU companies may just move some of their operations to the CEEC.One of the most obvious channels through which home(EU)jobs may be affected by this increased economic integration is through the employment(re)-allocation decisions of multinational enterprises(MNEs).Indeed,it is often argued that MNEs are footloose(Caves1996;Görg and Strobl2003).In this paper we study the effect of foreign wages on the demand for labor by EU MNEs.1We usefirm-level data of1,067medium and large sized parent MNEs matched with their2,078affiliates located in the EU and/or CEEC.Therefore,we can analyze how labor demand in parent and affiliate enterprises is associated with changes in affiliate wages relative to parent wages.2We define a parent as afirm located in country i holding a direct ownership share of at least50percent in one or morefirms located in another country j=i and refer to thesefirms as affiliates.Thus we only consider this direct relationship and do not consider indirect holding structures.The fact that we have a panel of matched parentfirms with their affiliates allows us to control forfirm-specific technology that may affect labor allo-cation across different regions.This enables us to focus on the employment substitution effects between parentfirms(or home parent employment) and their affiliates.Substitution effects may exist in response to changing wage conditions in different countries,taking as given global output of the MNE.This paper is not about the actual investment decision and its impact on employment in MNEs,rather we take locations as given.What we con-1Wages refer to total labor costs including social security contribution and payroll tax. 2A related literature is concerned with outsourcing by multinationalfirms in reducing de-mand for unskilled labor in the home country(e.g.Slaughter2000;Feenstra and Hanson 1996).However,we have no information on the skill composition of the workers in our firm-level data,so we are not able to focus on this type of demand shifts.Konings/Murphy:Do Multinational Enterprises Relocate Employment269 sider is how MNEs reshuffle jobs between the parent and their affiliates in response to wage differentials that may exist between these operations.Our data do not provide any information on the actual timing of the investment decision,so that we cannot evaluate the effects on employment in response to the actual investment/location decision.Of course,relative wage costs in various countries may play a role in the location decision of a MNE,which may have implications for employment responses.However, strategic reasons related to market penetration and market expansion are often found as the main driving forces for foreign direct investment,rather than labor cost differentials(e.g.,Lankes and Venables1996;Abraham and Konings1999).It is only recently that matched parent-affiliate data sets have become available and have been used to address similar questions.Brainard and Riker(1997)usefirm-level data of US MNEs in the1980s,butfind very low substitution effects between home parent employment and their foreign affiliates.In contrast,Blomström et al.(1997)also usingfirm-level data on US and Swedish MNEsfind evidence that US parentfirms have allocated some of their more labor-intensive operations to affiliates in developing countries.In addition,theyfind no evidence that Swedish MNEs relocate employment between the parent and its low-wage foreign affiliates.Like-wise,Bruno and Falzoni(2000)find strong employment relocation effects between US parentfirms and their affiliates in developing countries.In-terestingly,Hatzius(1998)and Braconier and Ekholm(2000)use Swedish firm-level panel data,collected through surveys,andfind that employment relocation is taking place between the Swedish headquarters and their af-filiates in other high-income locations.Hanson et al.(2001)find for US multinationals that affiliate wages have an effect on parent employment, but also tax rates seem to matter.The data that we use in this paper offer a number of advantages.First, in contrast to earlier studies,this paper uses a largefirm-level panel data set of medium and large sized MNEs with parents located in various EU coun-tries.Second,our data include both manufacturing and nonmanufacturing parentfirms and their affiliates.This allows us to make a distinction be-tween MNEs with affiliates operating in the same sector or different sectors compared to their parents,which may shed some light on the strategies that MNEs are pursuing.A third advantage of the current work and data is that we are able to differentiate on the basis of wage costs across the European regions.In our analysis we can distinguish between“very low”-wage loca-tions(CEEC),“low”-wage locations(South EU)and“high”-wage locations270Review of World Economics2006,Vol.142(2)(North EU).This allows us to assert whether low-wage competition may potentially be important for“footloose”multinationals,enabling them to reshuffle expensive jobs to cheaper ones in the low-wage locations within and outside the EU.Our mainfinding is that wefind very small substitution effects between parent and affiliate employment.Especially wages in affiliates located in Central Europe seem to have no effect on parent employment.The structure of this paper is as follows.In the next section we have afirst look at the data that we use.Section3sets up the econometric framework and reports the main results.Section4reports some robustness checks, while Section5gives the conclusion.2Data and Preliminary FactsWe make use of a commercial database of company accounts,comparable to other company account data sets such as the Compustat database in the United States or the Exstat database in the United Kingdom.The data are commercialized under the name“Amadeus”by Bureau Van Dijk(BvD) and have been used in recent years to analyze various economic issues in a growing number of academic papers.3Amadeus data include information from the Balance Sheets and Income and Loss Statements of medium and large sized companies in the EU and in CEEC(see also data appendix).In most European countries medium and large sized enterprises are required by law to submit company accounts to their Central Bank or National Statistical Offices.All these company accounts went through a formal external auditing process,so we have no reasons to believe that the reported information in the Balance Sheet and Income and Loss Statement is incorrect.Apart from the standard data provided in company accounts,the data also include information on the ownership structure offirms.The company records include information on whether the company has an ownership stake in a foreign affiliate,and identify affiliates by name and an identifi-cation number.For some countries(e.g.,Belgium)companies are required by law to report their affiliates,while for some other countries(e.g.,the 3Budina et al.(2000)investigate liquidity constraints in Bulgarianfirms,Konings et al. (2001)study price-cost margins in Belgian and Dutchfirms,Budd et al.(2005)analyze in-ternational rent-sharing in European multinationalfirms,Checchi et al.(2003)investigate how labor demand adjusts in foreign versus domestic Europeanfirms.Konings/Murphy:Do Multinational Enterprises Relocate Employment271 Netherlands)companies can voluntarily choose whether or not to report their affiliates.Financial and operational information is available for1993through 1998,and we retrieved all companies for which unconsolidated accounts were available separately for the parent and its affiliates.Due to variation in national reporting requirements,all companies in some countries—in particular Greece and Finland—lack basic information(e.g.,wage bills)that are essential for our analysis.Otherwise,we include companies in the data set simply on the basis of data availability and the ability to link parents with foreign affipanies in all industries are included,with primary industry for each parent and affiliate reported at the2-digit level of the NACE system.The available ownership information refers to the year1998,and we assume that the parent-affiliate ownership structure for1998applies to the earlier years.While we cannot trace ownership changes during the sample period,we do not believe that this is a serious problem.T o the extent that we are potentially including a few affiliates who were not affiliated in earlier years,we are introducing measurement error that may bias our results toward zero.Our eventual data set covers the period1993–1998and is an unbalanced panel of1,067parent companies located in the EU,with2,078affiliates located in the EU or CEEC or both.4We only take into account direct ownership links and furthermore there is no affiliate that also appears as a parent in our data set.5Table1shows the distribution of parentfirms and their affiliates across the various European countries.Germany,France,and Belgium host almost60percent of the parentfirms in our sample.France, Italy,Spain,and the United Kingdom contain many of the affiliates in our sample,with only5.34percent located in Central and Eastern Europe.Table1also shows the distribution of parent affiliates across the two broad classes of sectors,manufacturing versus nonmanufacturing.In our sample nearly half(48percent)of manufacturing parentfirms have affil-iates solely in the manufacturing sector.Almost one third(32.19percent) of manufacturing parents have affiliates in nonmanufacturing only,while onefifth(19.72percent)have affiliates both in manufacturing and non-4Amadeus does not reportfinancial information on companies that are located in the United States,Africa,Asia,so our analysis is restricted to Europe.Given that wage cost dif-ferentials are already substantial within Europe we believe we are already picking up some basic patterns,which would persist if we included additional low-wage regions.5Information on indirect ownership structures was often lacking from the data.272Review of World Economics2006,Vol.142(2)Table1:Distribution of Multinational Firms by Country and Sector,1993–1998(percent)Frequency of parentfirms Frequency of affiliatefirms Austria 2.08 1.89Belgium13.548.45 Denmark 3.650.68Ireland0.220.89France27.1622.62 Germany20.83 2.27Italy14.1411.37 Luxemburg0.300.83 Netherlands 2.23 2.12Portugal0.15 3.18Spain 5.3620.58Sweden N.A. 3.83United Kingdom10.3415.95Central and Eastern Europe N.A. 5.34Sector distribution of parent and affiliatefirmsAffiliateManufacturing Nonmanufacturing Both ParentManufacturing48.0932.1919.72Nonmanufacturing24.6658.4716.87 manufacturing sectors.Typically,manufacturing parentfirms in these last two categories have over80percent of their affiliates in the wholesale and retail distribution sectors.It is therefore unlikely,for this category offirms, that relocation of employment in response to wage cost differentials is im-portant.This is because the main activity of the foreign affiliate is related to distribution rather than production within the multinational group.Turning to the nonmanufacturing parentfirms we note that most (58.47percent)of them control affiliates only in the nonmanufacturing sec-tors,with a substantial fraction(24.66percent)having affiliates in manufac-turing only.This latter fraction could reflect cases in which the production is“outsourced”to the affiliates,while the“administration”is done in the home parentfirm.Our analysis will exploit some of these dimensions.Looking at Table2we can see the evolution of total affiliate employment as a fraction of total MNE employment,i.e.,the sum of total affiliate andKonings/Murphy:Do Multinational Enterprises Relocate Employment273 Table2:Evolution of Employment in EU Multinational Firms,1993–1998(percent)(1)(2)(3)(4)(5)(6)Parent Affiliate EU CEEC South EU North EUfirmfirm affiliates affiliates affiliates affiliates 199382.1917.8116.80 1.00 3.5513.25 199477.0122.9919.59 3.41 6.2413.34 199576.7723.2320.57 2.66 6.2514.32 199676.7623.2419.95 3.29 6.7113.24 199771.6428.3625.36 3.017.5517.81 199871.3128.6925.65 3.057.1118.54 parent employment.In columns1and2we can see that the employment share of parent MNEs has declined from85percent to71percent between 1993and1998,while the employment share of its affiliates has steadily increased from18percent to29percent in this period.This suggests that some reshuffling of jobs between parentfirms and their affiliates took place in this relatively short time period.Columns3–6shed some more light on this reshuffling.Looking at columns3and4we note that it is especially the affiliates located in the EU that have gained in relative employment, while the employment shares of the affiliates in CEEC remained relatively stable.Finally,columns5and6make an additional distinction between affiliates located in South EU and North EU.We defined the South EU as the low-wage countries in the EU,i.e.,Spain,Italy,Portugal,and Ireland. We can see that the increased fraction of affiliate EU employment is mainly driven by an increased fraction of employment in affiliates located in the North EU.These patterns suggest that most of the job relocation took place between EU parentfirms and their affiliates located in the North EU.We will test this hypothesis in a more rigorous framework in Section3.Table3shows summary statistics on the data that we are using.We proxy output by the total value added of the MNE using a weighted sum of the value added of the parent and of its affiliates.As we can see from Table3,parent companies in our sample employ on average1,873persons,while their affiliates employ less workers on average.The typical EU affiliate employs 243workers on average,while the typical affiliate in CEEC employs almost twice as many workers,460.This is not surprising since unit labor costs are much lower in the latter region.The average labor cost per worker per year is US$52,000in parentfirms,while this is only US$7,000in the typical affiliate in CEEC.Although the labor cost in CEEC is much lower than in274Review of World Economics 2006,Vol.142(2)Table 3:Summary StatisticsMeanStandard error Parent employment1,8734,444Affiliate employment257409of which:in the EU243390in CEEC460577in South EU225354in North EU252407Parent wage cost per worker (US $)52,00018.38Affiliate wage cost per worker (US $)of which:in the EU45,00017in CEEC7,0007in South EU41,00015in North EU47,00017Parent Value added per worker (US $)104,00079Affiliate value added per worker (US $)of which:in the EU82,30071in CEEC22,00036in South EU81,00062in North EU83,00076Number of affiliates 1.65 2.44Europe,also the average labor productivity is much lower.In our sample value added per worker in the North EU is US $83,000and is US $81,000in the South EU,but only US $22,000in the Central and Eastern Europe region on average.3Econometric Framework and Results3.1Econometric SpecificationConsider a MNE that produces global output,Y ,using the following produc-tion function,which depends only on labor input in the various locations:Y =F L P ,L A NEU ,L A SEU ,L A CEEC ,(1)where Y =total output of the multinationals (i.e.,the sum of output in the parent and all its affiliates),F is the production function,L P =parentKonings/Murphy:Do Multinational Enterprises Relocate Employment 275employment,L A l =affiliate employment in location l (l =NEU,i.e.North EU,SEU,i.e.South EU,CEEC).T otal cost minimization under constraint (1)yields the conditional demand for parent employmentL P =h P (W P ,W A NEU,W A SEU ,W A CEEC ,,Y ),(2)−++++where W P stands for the parent wage cost per worker,W A l stands for the wage cost per worker of the affiliate located in l (l =NEU,SEU,CEEC).We expect the following partial derivatives:•The own wage to be negatively related to home parent labor demand;δh P /δW P <0.•If there are substitution effects between parent and affiliate employment;δh P /δW A l >0,with l =NEU,SEU,CEEC.•If there are no substitution effects between parent and affiliate employ-ment;δh P /δW A l ≤0,with l =NEU,SEU,CEEC.The substitution effect or employment relocation effect gives an indi-cation of the technological substitution possibilities between parent and affiliate employment,for a given production of a global output level.It represents the technological possibilities to move along the same isoquant.Equation (2)will form the basis of our empirical specifications.In particular we will estimate (2)by assuming a log-linear approximation orln L P it =αP i +α1ln W P it +α2ln W A iNEUt +α3ln W A iSEUt(3)+α4ln W A iCEECt +α5ln Y it +εit ,where i =firm i ,t =year,and εit =white noise.We include αP i ,which is a firm-level fixed effect that is not observable.This may include distance between the parent and affiliate company,in general it refers to unobserved heterogeneity.T o take into account that not all parent firms have affiliates in all locations (NEU,SEU,CEEC)we will estimate (3)including location dummies.6Furthermore we include in (3)year dummies to control for unobserved aggregate shocks,which are common to all parent firms.6T echnically the firm-level fixed effects control for these location dummies as they are perfectly collinear with the firm-level fixed effect.276Review of World Economics2006,Vol.142(2)The above framework does not take into account potential employ-ment adjustment costs in response to shocks,which would imply a dynamic specification.T o theoretically model adjustment costs for multinational en-terprises is not straightforward as these costs may be different for the parent company and its affiliates,depending on the local institutional constraints. Studies that assume symmetric quadratic costs of adjustment suggest that the speed of adjustment varies in different countries.For instance Anderson (1993)finds for American retail establishments that most of the adjustment is completed in one quarter.Likewise,Mairesse and Dormont(1985)find that for American manufacturingfirms nearlyfive-sixths of the response is completed within a year,while for French and German manufacturing firms theyfind a very slow adjustment.Also Nickell and Wadhwani(1991)find for British manufacturingfirms that only20percent of the adjustment to a shock is made up in one year.Hamermesh and Pfann(1996)suggest the assumption of symmetric quadratic adjustment costs is one of the reasons tofind differences in the speed of adjustment and suggest some alternatives.It is not our purpose to model such an adjustment process for the allocation decision of employment for rmation on the opening and closing of affiliates is likely to be important for this,however,this is not given in our data.Instead we will conduct a number of robustness checks by estimating a simple dynamic employment equation,without deriving this theoretically.3.2ResultsTable4showsfirm-levelfixed effects estimates for equation(3).Column1 gives the results for the overall sample,while columns2and3give the results for parentfirms operating in the manufacturing and nonmanufacturing, respectively.Thefirst point worth noting is that the own wage elasticity(i.e., the effect of W P)is estimated at−0.89,this is well within the range of esti-mated labor demand elasticities reported in the literature(e.g.,Hamermesh 1993).The substitution elasticities give an indication about the responsive-ness of parent employment to wage changes in affiliates.These elasticities are given by the coefficients that are associated with W NEU,W SEU,W CEEC, reflecting the effect of wage changes in affiliates located in North EU,South EU,and CEEC,respectively.All three are estimated positively,however,only the wage effect on parent employment of affiliates located in North EU is estimated positive and statistically significant,with a coefficient of0.018.Table4:Parent Employment and Wages in North EU,South EU,and CEEC(FixedEffects Estimates)(1)(2)(3)Whole sample Manufacturing NonmanufacturingW P −0.89∗∗∗−1.03∗∗∗−0.69∗∗∗(0.032)(0.041)(0.050)W A NEU0.018∗∗0.032∗∗−0.02 (0.01)(0.011)(0.017)W A SEU0.0020.009−0.013 (0.01)(0.012)(0.02)W A CEEC0.0240.0150.04 (0.021)(0.028)(0.03)Y0.48∗∗0.57∗∗∗0.33∗∗∗(0.015)(0.02)(0.024)Number of437528171558 observationsR2within0.350.420.26R2between0.620.640.59R2overall0.690.720.64∗∗∗,∗∗,∗denote significance at the1,5,and10percent level,respectively.Note:All equations include year dummies.Robust standard errors in parentheses.This suggests that a reduction of say10percent in affiliate wages located in the North EU is associated with a reduction in home(parent)employment of0.18percent on average.Wefind no statistically significant effect of a reduction in wages of affiliates located in the South EU and in CEEC. This suggests that employment substitution or relocation in response to relative wage changes only takes place between parentfirms(which are mainly located in the North EU)and their affiliates that are also located in the North EU.This result comes as a bit of a surprise and suggests that competition from low-wage locations(on average)does not constitute a threat to par-ent employment.Braconier and Ekholm(2000)report similar results for Swedish MNEs.A potential explanation for thisfinding is the proximity hypothesis put forward by Brainard(1997).Brainard shows that it is more likely that substitution between parent and affiliate employment takes place in response to wage cost differentials when the proximity to thefinal mar-ket is important.In this case transport or trade costs are assumed to be negligible.Such substitution effects are also more likely when the initialfactor endowments are similar across locations.This is the case for North EU affiliate and(mostly North EU based)parentfirms in our sample.In the second and third columns of Table4we report results for the subsamples of parent companies operating in the manufacturing versus the nonmanufacturing sector.We can see that the relocation effect,estimated by the coefficient on W NEU,is driven mainly by the subsample of parent firms operating in the manufacturing sector.From column2we note that this estimated effect is now twice as high,at0.032,compared to the estimate based on the whole sample in column1.Moreover,wefind no statistically significant substitution elasticities for our subsample of parentfirms oper-ating in the nonmanufacturing sector as shown in column3.One potential reason why wefind no substitution effects in the nonmanufacturing sector could be due to the nature of these activities,in that it is believed that there are more nontradables in nonmanufacturing.4Robustness ChecksIn Table5we report some robustness checks.As discussed earlier it is well known that adjustment costs in employment are potentially important, which may imply a dynamic employment specification.In Table5we report a simple dynamic model in which we include the lagged dependent variable. The introduction of a lagged dependent variable in afixed effects model introduces an endogeneity bias.We therefore estimated this model infirst differences to control for the unobservedfirm-levelfixed effects and applied the Arellano and Bond(1991)IV GMM estimator.This means that we used all available moment restrictions on employment dated from t−2 and before.Furthermore,we also instrumented output using all available moment restrictions from t−2and before.Additional instruments included parent country dummies,which may capture institutional differences such as minimum wage laws,employ-ment protection legislation,etc.between countries.The Sargan test(Chi2-distribution)and the second order serial correlation test(normal distribu-tion)suggest that the instruments and model specification are valid.Our basic results remain robust.Wefind that the parent own short-and long-run wage elasticity is estimated at−0.65and−1.0,respectively,while the short-and long-run substitution elasticity between parent employment and North EU affiliate employment is estimated0.03and0.05,respectively.Thus,as before employment relocation seems to take place,but only between NorthTable5:Parent Employment and Wages in North EU,South EU,and CEEC(Arellano and Bond GMM IV Estimates)(1)(2)(3)Whole sample Manufacturing NonmanufacturingL P t−10.40∗∗∗0.20∗∗∗0.46∗∗∗(0.05)(0.067)(0.10)W P −0.65∗∗∗−0.82∗∗∗−0.57∗∗∗(0.11)(0.15)(0.11)W A NEU0.03∗0.06∗∗∗0.022 (0.017)(0.022)(0.016)W A SEU0.0150.03−0.017 (0.015)(0.02)(0.014)W A CEEC0.012−0.010.038∗(0.016)(0.02)(0.022)Y0.57∗∗∗0.72∗∗∗0.33∗∗∗(0.086)(0.11)(0.10)Number of observations157********Sargan test(Prob>Chi2)0.740.540.99T est of second order serial−0.220.170.93 correlation(z-value)∗∗∗,∗∗,∗denote significance at the1,5,and10percent level,respectively.Note:All equations include year dummies.Robust one step standard errors in parentheses. The lagged dependent variable and total output are instrumented using all available moment restrictions.Parent country dummies are included as additional instruments.EU parent employment and North EU affiliate employment.Again,this result is driven by the substitution possibilities in the manufacturing sector, where estimated short-and long-run elasticity of substitution is0.06and 0.08,respectively.One of the empirical regularities characterizing MNEs is that they mostly operate in sectors that are R&D intensive and are often characterized by high levels of intangible assets,which is often reflected in the skill composition of their workforce.The data that we use have no information on the skill composition of the workforce,so we treated labor as homogeneous.Slaugh-ter(2000)has shown for the United States,that this may not be too much of a problem.Hefinds that MNE transfer to low-wage countries has oc-curred,however,hefinds no evidence that this has contributed to shifts in the relative demand for fewer unskilled workers in the United States.As an extra robustness check we include as extra controls in our equation proxies for R&D intensity at the parentfirm.As a proxy for R&D we use intangible assets as a percentage of total assets in the parentfirm.A second。
Announcements
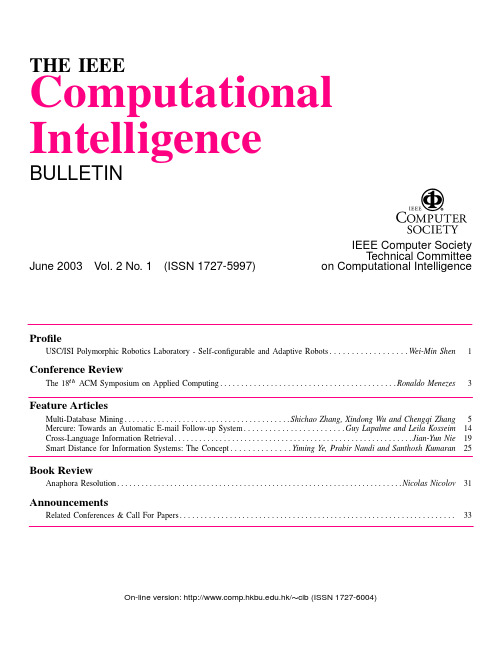
Computational Intelligence
BULLETIN
June 2003 Vol. 2 No. 1 (ISSN 1727-5997) IEEE Computer Society Technical Committee on Computational Intelligence
Profile
USC/ISI Polymorphic Robotics Laboratory - Self-configurable and Adaptive Robots . . . . . . . . . . . . . . . . . . Wei-Min Shen 1
Conference Review ¡
Announcements
Related Conferences & Call For Papers . . . . . . . . . . . . . . . . . . . . . . . . . . . . . . . . . . . . . . . . . . . . . . . . . . . . . . . . . . . . . . . . . . 33
Publisher: The IEEE Computer Society Technical Committee on Computational Intelligence Address: Department of Computer Science, Hong Kong Baptist University, Kowloon Tong, Hong Kong (Attention: Dr. Jiming Liu; Email: jiming@.hk) ISSN Number: 1727-5997 (printed) 1727-6004 (on-line) Abstracting and Indexing: All the published articles will be submitted to the following on-line search engines and bibliographies databases for indexing — Google (), The ResearchIndex (), The Collection of Computer Science Bibliographies (a.de/bibliography/index.html), and DBLP Computer Science Bibliography (rmatik.uni-trier.de/ ley/db/index.html). c 2003 IEEE. Personal use of this material is permitted. However, permission to reprint/republish this material for advertising or promotional purposes or for creating new collective works for resale or redistribution to servers or lists, or to reuse any copyrighted component of this work in other works must be obtained from the IEEE.
Bearing capacity of spatially random soil the undrained clay Prandtl
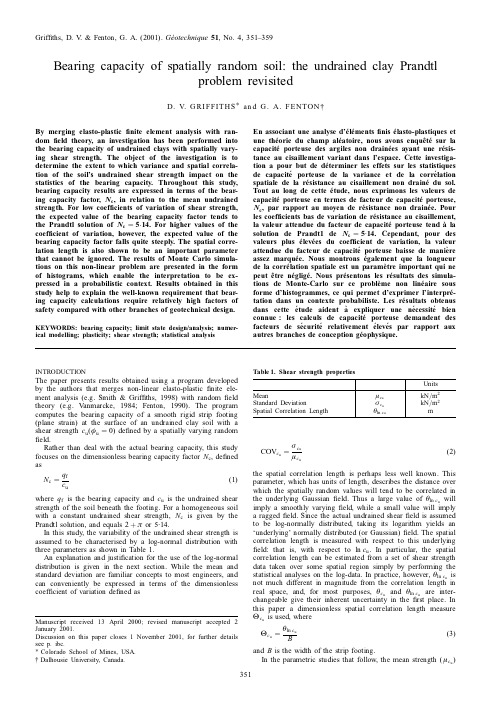
Bearing capacity of spatially random soil:the undrained clay Prandtlproblem revisitedD.V.GRIFFITHSÃand G.A.FENTON{By merging elasto-plastic®nite element analysis with ran-dom®eld theory,an investigation has been performed into the bearing capacity of undrained clays with spatially vary-ing shear strength.The object of the investigation is to determine the extent to which variance and spatial correla-tion of the soil's undrained shear strength impact on the statistics of the bearing capacity.Throughout this study, bearing capacity results are expressed in terms of the bear-ing capacity factor,N c,in relation to the mean undrained strength.For low coef®cients of variation of shear strength, the expected value of the bearing capacity factor tends to the Prandtl solution of N c 5X14.For higher values of the coef®cient of variation,however,the expected value of the bearing capacity factor falls quite steeply.The spatial corre-lation length is also shown to be an important parameter that cannot be ignored.The results of Monte Carlo simula-tions on this non-linear problem are presented in the form of histograms,which enable the interpretation to be ex-pressed in a probabilistic context.Results obtained in this study help to explain the well-known requirement that bear-ing capacity calculations require relatively high factors of safety compared with other branches of geotechnical design.KEYWORDS:bearing capacity;limit state design/analysis;numer-ical modelling;plasticity;shear strength;statistical analysis En associant une analyse d'eÂleÂments®nis eÂlasto-plastiques et une theÂorie du champ aleÂatoire,nous avons enqueÃteÂsur la capaciteÂporteuse des argiles non draineÂes ayant une reÂsis-tance au cisaillement variant dans l'espace.Cette investiga-tion a pour but de deÂterminer les effets sur les statistiques de capaciteÂporteuse de la variance et de la correÂlation spatiale de la reÂsistance au cisaillement non draineÂdu sol. Tout au long de cette eÂtude,nous exprimons les valeurs de capaciteÂporteuse en termes de facteur de capaciteÂporteuse, N c,par rapport au moyen de reÂsistance non draineÂe.Pour les coef®cients bas de variation de reÂsistance au cisaillement, la valeur attendue du facteur de capaciteÂporteuse tend aÁla solution de Prandt1de N c 5X14.Cependant,pour des valeurs plus eÂleveÂes du coef®cient de variation,la valeur attendue du facteur de capaciteÂporteuse baisse de manieÁre assez marqueÂe.Nous montrons eÂgalement que la longueur de la correÂlation spatiale est un parameÁtre important qui ne peut eÃtre neÂgligeÂ.Nous preÂsentons les reÂsultats des simula-tions de Monte-Carlo sur ce probleÁme non lineÂaire sous forme d'histogrammes,ce qui permet d'exprimer l'interpreÂ-tation dans un contexte probabiliste.Les reÂsultats obtenus dans cette eÂtude aident aÁexpliquer une neÂcessiteÂbien connue:les calculs de capaciteÂporteuse demandent des facteurs de seÂcuriteÂrelativement eÂleveÂs par rapport aux autres branches de conception geÂophysique.INTRODUCTIONThe paper presents results obtained using a program developed by the authors that merges non-linear elasto-plastic®nite ele-ment analysis(e.g.Smith&Grif®ths,1998)with random®eld theory(e.g.Vanmarcke,1984;Fenton,1990).The program computes the bearing capacity of a smooth rigid strip footing (plane strain)at the surface of an undrained clay soil with a shear strength c u(öu 0)de®ned by a spatially varying random ®eld.Rather than deal with the actual bearing capacity,this study focuses on the dimensionless bearing capacity factor N c,de®nedasN c q fu(1)where q f is the bearing capacity and c u is the undrained shear strength of the soil beneath the footing.For a homogeneous soil with a constant undrained shear strength,N c is given by the Prandtl solution,and equals2 ðor5´14.In this study,the variability of the undrained shear strength is assumed to be characterised by a log-normal distribution with three parameters as shown in Table1.An explanation and justi®cation for the use of the log-normal distribution is given in the next section.While the mean and standard deviation are familiar concepts to most engineers,and can conveniently be expressed in terms of the dimensionless coef®cient of variation de®ned asCOV cuócuìcu(2)the spatial correlation length is perhaps less well known.This parameter,which has units of length,describes the distance over which the spatially random values will tend to be correlated in the underlying Gaussian®eld.Thus a large value ofèln cuwill imply a smoothly varying®eld,while a small value will implya ragged®eld.Since the actual undrained shear®eld is assumedto be log-normally distributed,taking its logarithm yields an `underlying'normally distributed(or Gaussian)®eld.The spatial correlation length is measured with respect to this underlying ®eld:that is,with respect to ln c u.In particular,the spatial correlation length can be estimated from a set of shear strength data taken over some spatial region simply by performing the statistical analyses on the log-data.In practice,however,èln cuis not much different in magnitude from the correlation length in real space,and,for most purposes,ècuandèln cuare inter-changeable give their inherent uncertainty in the®rst place.In this paper a dimensionless spatial correlation length measure Ècuis used,whereÈcuèln cuB(3)and B is the width of the strip footing.In the parametric studies that follow,the mean strength(ìcu) 351Grif®ths,D.V.&Fenton,G.A.(2001).GeÂotechnique51,No.4,351±359Manuscript received13April2000;revised manuscript accepted2 January2001.Discussion on this paper closes1November2001,for further details see p.ibc.ÃColorado School of Mines,USA.{Dalhousie University,Canada.Table1.Shear strength propertiesUnits MeanìcukN a m2 Standard DeviationócukN a m2 Spatial Correlation Lengthèln cumhas been held constant at 100kN a m 2,while the standard deviation (óc u )and spatial correlation length (Èc u )are varied systematically.It has been suggested (e.g.Lee et al .,1983;Kulhawy et al .,1991;Duncan,2000)that typical COV c u values for the un-drained shear strength lie in the range 0´1±0´5;however,the spatial correlation length is less well documented,especially in the horizontal direction,and may well exhibit anisotropy.While the analysis tools used in this study are capable of modelling an anistropic spatial correlation ®eld,all the results presented in this paper assume that Èc u is isotropic.For each set of assumed statistical properties given by COV c u and Èc u ,Monte Carlo simulations have been performed invol-ving n sim repetitions or `realisations'of the shear strength random ®eld and the subsequent ®nite-element analysis of bearing capacity.This means that each realisation,while having the same underlying statistics,leads to a quite different spatial pattern of shear strength values beneath the footing.Each realisation therefore leads to a different value of the bearing capacity and,after normalisation by the mean undrained shear strength,a different value of the bearing capacity factor,N c i q fi ìc u i 1,2,F F F ,n sim (4)In this study n sim 1000,and once the bearing capacity factors from all the realisations have been accumulated,they in turn can be subjected to statistical analysis.Estimated (sample)mean bearing capacities will have a standard error (Æone standard deviation)equal to the sample standard deviation times 1a p n sim 1a p 1000 0X 032,or about 3%of the sample stan-dard deviation.Similarly,the estimated variance will have a standard error equal to the sample variance times p(2a (n sim À1)) p (2a 99) 0X 045,or about 4%of the sample variance.This means that estimated quantities will generally be within about 5%of the true quantities,statistically speaking.Of particular interest in the present study is the probability that the actual bearing capacity factor,N c ,as de®ned in equa-tion (4),will be less than the Prandtl value of 5´14that would be obtained assuming a homogeneous soil with undrained shear strength everywhere equal to the mean value ìc u .REVIEW OF THE LOG-NORMAL DISTRIBUTIONA log-normal distribution for the undrained shear strength,c u ,has been adopted in this study,meaning that ln c u is normally distributed.If the mean and standard deviation of the undrained shear strength are ìc u and óc u respectively,then the standard deviation and mean of the underlying normal distribu-tion of ln c u are given byóln c u r ln 1óc uìc u245@A(5)ìln c u ln ìc u À12ó2ln c u(6)and the probability density function of the lognormal distribu-tion is given byf (c u ) 1c u óln c u 2ðp exp À12ln c u Àìln c uln c u 245(7)In terms of the properties of the underlying normal distribution,the properties of the log-normal distribution can therefore be summarised as follows:ìc u exp ìln c u 12ó2ln c u(8)óc u ìc u p[exp(ó2ln c u )À1](9)Median exp(ìln c u )(10)Mode exp(ìln c u Àó2ln c u )(11)Use of the log-normal distribution,as opposed to the more familiar normal distribution,or even some other more complex distribution,is based on the following arguments:First,there is a lack of exhaustive ®eld data that would be necessary to conclusively support one kind of distribution over another.However,there is some evidence from the ®eld to support the log-normal distribution for some soil properties (e.g.Hoeksema &Kitanidis,1985;Sudicky,1986).Use of the log-normal distribution is also based on the simplicity and familiarity of its two-parameters description.Second,and perhaps more impor-tantly from a physical standpoint,the log-normal distribution is strictly non-negative,unlike the normal distribution,and so there is no possibility of generating properties with meaningless negative values,particularly in the extremes of the distribution (which may be important from a reliabilty standpoint).It might also be noted that a log-normal distribution looks quite similar to a normal distribution for low values of the COV .Lee et al .(1983)comment that the `normal or log-normal distributions are adequate for the large majority of geotechnical data';however,Harr (1987)®nds the unbounded nature of the upper end of the log-normal distribution objectionable.The potential for the log-normal distribution to generate very high property values (albeit with a low probability)is not considered a serious ¯aw,especially in a study involving the shear strength of heterogeneous soil that is spatially distributed (what is the shear strength of a point that happens to fall inside a boulder of granite?).It is certainly possible that a soil deposit will contain occasional inclusions of very strongly cemented material.A typical log-normal distribution based on equation (7)with mean ìc u 100kN a m 2and standard deviation óc u 50kN a m 2(COV c u 0X 5)is shown in Fig.1.From equations (5)and (6)it is easily shown that the underlying `normal'statistics are given by óln c u 0X 472and ìln c u 4X 494.High-lighted also on the ®gure are the median and mode of the distribution,which can be shown from equations (10)and (11)to equal,respectively,89X 4kN a m 2and 71X 6kN a m 2.The skewed nature of the log-normal distribution always results in the mode,median and mean being in the sequence indicated.In a log-normal distribution the median is always smaller than the mean,and this will have implications for the probabilistic interpretation of the bearing capacity results described later in the paper.12108642050100150200300250P r o b a b i l i t y d e n s i t y f u n c t i o n : × 10–3M ode = 71·6M edian = 89·4M ean = 100·0c uFig.1.Typical log-normal distribution of undrained shear strength with a mean of 100and standard deviation of 50(COV c u 0X 5).All units are in kN a m 2352GRIFFITHS AND FENTONBRIEF DESCRIPTION OF THE FE METHOD USEDThe bearing capacity analyses use an elastic-perfectly plastic stress±strain law with a Tresca failure criterion.Plastic stress redistribution is accomplished using a viscoplastic algorithm.The program uses 8-node quadrilateral elements and reduced Gaussian integration in both the stiffness and stress redistribu-tion parts of the algorithm.The theoretical basis of the method is described more fully in Chapter 6of the text by Smith &Grif®ths (1998).The ®nite element model incorporates three parameters:Y oung's modulus (E ),Poisson's ration (í),and the undrained shear strength (c u ).The methodology allows for random dis-tributions of all three parameters;however,in the present study E and íare held constant while c u is randomised.A mesh is shown in Fig.2consisting of 1000elements,with 50columns and 20rows.Each element is square,and the strip footing has a width of 10elements.At the i th realisation of the Monte Carlo process,the footing is incrementally displaced vertically (äv )into the soil,and the sum of the nodal reactions (Q i )is back-®gured from the converged stress state.When the sum of the nodal reactions levels out to within a quite strict tolerance,`failure'is said to have occurred,and the sum of the nodal reactions divided by the footing area is the `bearing capacity'(q f i Q f i a B )of that particular realisation.A BRIEF DESCRIPTION OF THE RANDOM FIELD MODELThe undrained shear strength is obtained through the trans-formationc u i exp(ìln c u óln c u g i )(12)in which c u i is the undrained shear strength assigned to the i th element,g i is the local average of a standard Gaussian random ®eld,g ,over the domain of the i th element,and ìln c u and óln c u are the mean and standard deviation of the logarithm of c u (obtained from the `point'mean and standard deviation ìc u and óc u after local averaging).The LAS technique (Fenton,1990;Fenton &Vanmarcke,1990)generates realisations of the local averages,g i ,that are derived from the random ®eld g having zero mean,unit vari-ance,and a spatial correlation length èln c u .As the spatial correlation length tends to in®nity,g i becomes equal to g j for all elements i and j :that is,the ®eld of shear strengths tends tobecome uniform for each realisation.At the other extreme,as the spatial correlation length tends to zero,g i and g j become independent for all i j :the soil's undrained shear strength changes rapidly from point to point.In the present study,a Markovian spatial correlation function was used,of the formr (j ôj ) exp À2èln c u j ôj(13)where r is the correlation coef®cient between the logarithm of the undrained strength values at any two points separated by a distance ôin a random ®eld with spatial correlation length èln c u .In the two-dimensional analysis presented in this paper,the spatial correlation lengths in the vertical and horizontal direc-tions are taken to be equal (isotropic)for simplicity.Fenton (1999)examined CPT data in relation to random ®eld model-ing;however,the actual spatial correlation structure of soil deposits is not usually well known,especially in the horizontal direction (e.g.Asaoka &Grivas,1982;de Marsily,1985;DeGroot &Baecher,1993).In this paper therefore,a parametric approach has been employed to study the in¯uence of èln c u .The plane strain model used herein implies that the out-of-plane spatial correlation length is in®nite:thus soil properties are constant in this direction.This is clearly a de®ciency.However,previous studies by the authors (Grif®ths &Fenton,1997)involving seepage through two-and three-dimensional random ®elds have indicated that the difference may not be very great.The role of the third dimension is an area of ongoing research by the authors.A local averaging process has been included in the formula-tion to take full account of the level of mesh discretisation,and the size of the ®nite elements onto which the random ®eld is to be mapped.Local averaging preserves the mean,but reduces the standard deviation of the underlying normal ®eld to a `target'value.The amount by which the standard deviation is reduced depends on the size of the elements and the nature of the spatial correlation function governing the ®eld.More speci-®cally,there is a function called the `variance function',which can be derived from the correlation function,and which governs the rate at which the standard deviation drops as the averaging domain grows larger.The interested reader is referred to Vanmarcke (1984)for a detailed description of this formulation.Although the mean of the underlying Gaussian ®eld isB Q5B2BFixed R o l l e r sR o l l e r sq = Q /BFig.2.Mesh used in probabilistic bearing capacity analysesBEARING CAPACITY OF SPATIALL Y RANDOM SOIL353unaltered by local averaging,equations (8)and (9)indicate that since both the mean and standard deviation of the log-normal ®eld are functions of óln c u they will both be reduced by the local averaging process.Thus the coarser the mesh,the greater the reduction in the `target'statistics from their nominal `point'values.This local averaging approach is fully implemented in this study,and removes any `mesh effects'that might otherwise be present.It might also be noted that this approach is quite consistent with the philosophy of the ®nite element method,in which ®ner meshes resolve the ®ner variations in the stress and material property ®elds.PARAMETRIC STUDIESAnalyses were performed using the mesh of Fig.2with the input parameters in the following ranges:0X 125<Èc u ,I (14)0X 125<COV c u <4To indicate the nature of the different solutions obtained at each realisation of the Monte Carlo process,load/deformation results for ten typical realisations of the footing analysis are shown in Fig.3for the case when Èc u 1and COV c u 1.The average stress,q ,under the footing has been non-dimensionalised by dividing it by the mean undrained shear strength,ìc u .The reader should bear in mind the Prandtl solution of 5´14when viewing this ®gure.It is clear that a majority of the curves ¯atten out at bearing capacity values below the Prandtl solution.This trend will be con®rmed in all the results shown in this paper.Figure 4shows a typical deformed mesh at failure with a superimposed greyscale corresponding to Èc u 1,in which lighter regions indicated stronger soil and darker regions in-dicated weaker soil.In this case the dark zones and the light zones are roughly the width of the footing itself,and it appears that the weak (dark)region near the ground surface to the right of the footing has triggered a quite non-symmetric failure mechanism.The shape of the non-symmetric mechanism is emphasised further by the plot of displacement vectors for the same realisation,shown in Fig.5.For each combination of Èc u and COV c u ,n sim 1000rea-lisations of the Monte Carlo process were performed,and the estimated mean (m N c )and standard deviation (s N c )of the resulting 1000bearing capacity factors from equation (4)were computed.Figure 6(a)shows how the estimated mean bearing capacity factor,m N c ,varies with Èc u and COV c u .The plot con®rms that,for low values of COV c u ,m N c tends to the deterministic Prandtl value of 5´14.For higher values of COV c u ,however,the mean bearing capacity factor falls steeply,especially for lower values of Èc u .For example,in a highly variable case where Èc u 0X 5and COV c u 4,the predicted m N c value is less than unityÐover ®ve times smaller than the Prandtl value!For the recom-mended upper limit of COV c u 0X 5suggested by Lee et al .(1983)and others,the m N c value is closer to 4,corresponding to a more modest reduction of 20%.What this implies from a design standpoint is that the bearing capacity of a heterogeneous soil will on average be less than the Prandtl solution that would be predicted assuming the soil is homogeneous with its strength given by the mean value.The in¯uence of Èc u is also pronounced with the greatest reduction from the Prandtl solu-tion being observed with values around Èc u %0X 5.As the value of Èc u is reduced further towards zero,there is evidence of a gradual increase in the value of m N c ,as shown in Fig.6(b).From a theoretical point of view,it could be speculated that,as5101520012345678Prandtl, 5·14δv /B : × 10–3q /µcuFig.3.Typical load/deformation curves corresponding to different realisations in the bearing capacity analysis of an undrained clay with Èc u 1and COV c uFig.4.Typical deformed mesh and greyscale at failure with Èc u The darker regions indicate weaker soil354GRIFFITHS AND FENTONÈc u becomes vanishingly small,the mean bearing capacity factor will continue to increase towards the deterministic Prandtl solution of 5´14.The explanation lies in the fact that as the spatial correlation length decreases,the weakest path becomes increasingly tortuous and its length correspondingly longer.As a result,the weakest path starts to look for shorter routes cutting through higher-strength material.In the limit,as Èc u 30,it is expected that the optimum failure path will be the same as in a uniform material with strength equal to the mean value,hence returning to the deterministic Prandtl solu-tion.Also included in Fig.6(a)is a horizontal line corresponding to the analytical solution that would be obtained for Èc u I .This hypothetical case implies that each realisation of the Monte Carlo process involves an essentially homogeneous soil,albeit with strength varying only from one realisation to thenext.In this case,the distribution of q f will be statistically similar to the underlying distribution of c u but magni®ed by 5´14.The mean bearing capacity will therefore be given by ìq f 5X 14ìc u (15)hence m N c 5X 14for all COV c u .Figure 7shows the in¯uence of Èc u and COV c u on the estimated coef®cient of variation of the bearing capacity factor,COV N c s N c a m N c .The plots indicate that COV N c is positively correlated with both COV c u and Èc u .This ®gure also indicates that the correlation length,Èc u ,has a signi®cant in¯uence on COV N c .For small correlation lengths COV N c is small and rather insensitive to COV c u ;however,for higher correlation lengths COV N c increases quite consistently until it reaches the limiting maximum value corresponding to Èc u I ,de®ned by the straight line where COV N c COV c u.Fig.5.Displacement vectors at failure for the same case shown in Fig.4.The non-symmetric shape of the failure mechanism is clearly visibleFig.6.(a)Estimated mean bearing capacity factor,m N c ,as a function of undrained shear strength statistics,Èc u and COV N c .(b)More clearly shows the increase in m N c as Èc u 305·55·04·54·03·53·02·52·01·50·51·010–11Prandtl, 5·14COV cu (a )102345678923456789m NcΘc u= 0·5Θc u= 1·0Θc u= 2·0Θc u= 4·0Θc u= 8·0Θc u= ∞54321012340·51·52·53·5Prandtl, 5·14(b)m NcCOV c u= 0·125COV c u= 0·25COV c u= 0·5COV c u= 1COV c u= 2COV c u= 4ΘcuBEARING CAPACITY OF SPATIALL Y RANDOM SOIL 355PROBABILISTIC INTERPRETATIONFollowing Monte Carlo simulation for each parametric com-bination of input parameters (Èc u and COV c u ),the suite of computed bearing capacity factor values from equation (4)was plotted in the form of a histogram,and a `best-®t'log-normal distribution superimposed.An example of such a plot is shown in Fig.8for the case where Èc u 2and COV c u 1.Since the log-normal ®t has been normalised to enclose an area of unity,areas under the curve can be directly related to probabilities.From a practical viewpoint it would be of interest to estimate the probability of `design failure',de®ned here as occurring when the computed bearing capacity is less than the Prandtl value based on the mean strength.That is:`Design failure'if q f ,5X 14ìc u (16)Let this probability be p (N c ,5X 14):hence from the properties of the underlying normal distribution we getp (N c ,5X 14) Öln 5X 14Àm ln N cs ln N c (17)where Öis the cumulative normal function.For the particular case shown in Fig.8,the ®tted log-normal distribution has the properties m N c 3X 31and s N c 2X 08:hence from equations (5)and (6)the underlying normal dis-tribution is de®ned by m ln N c 1X 03and s ln N c 0X 58.Equation (17)therefore gives p (N c ,5X 14) 0X 85,indicating an 85%probability that the actual bearing capacity will be less than the Prandtl value.Figure 9gives a summary of p (N c ,5X 14)for a range of values of Èc u and COV c u .The ®gure indicates a wide spread of probability values with respect to Èc u ,with the highest prob-abilities corresponding to the lowest values of Èc u .For exam-ple,a soil with COV c u 0X 5exhibits a range of 0X 59,p (N c ,5X 14),0X 95,with the low and high values corre-sponding to Èc u I and Èc u 0X 5respectively.The in¯uence of COV c u on the probability is also signi®cant.Theoretically,as COV c u 30,the probability p (N c ,5X 14)30X 5,irrespective of the value of Èc u .The results in Fig.9indicate that this convergence occurs faster for higher values of Èc u than for lower values.It would appear that low values of Èc u permit such widely scattered weak elements that the prob-ability of the actual bearing capacity lying below the Prandtl value remains high,even for low COV c u values.This general trend is to be expected,however,because for low COV c u values the distribution of bearing capacity factors becomes `bunched up'and `centred'on 5´14,giving an almost equal chance of the computed bearing capacity factor lying on either side of the Prandtl solution.As COV c u is increased,the probability p (N c ,5X 14)also increases.For example,when Èc u 0X 5and COV c u 0X 5,p (N c ,5X 14) 0X 95,indicating a 95%probabilty that the ac-tual bearing capacity will be lower than the Prandtl solution.The result corresponding to the limiting cases of Èc u I is also indicated in Fig.9.As dicussed previously,the distribution of q f in this case is statistically similar to the underlying distribution of c u ,and the required probability,p (N c ,5X 14),simply equals the area under the probability density function to the left of the mean.For a log-normal distribution this prob-ability is always greater than 0´5,and is given by p (N c ,5X 14) Ö(0X 5óln c u )(18)Thus from equation (5):p (N c ,5X 14) Ö(0X 5p[ln(1 COV 2c u )](19)Figure 9indicates that the expected bearing capacity of a strip footing on an undrained clay with variable shear strength de®ned by a log-normal distribution will always be lower than the Prandtl value based on the mean strength.It could be argued,however,that this interpretation gives an over-pessimis-tic impression of the role of soil strength variability by not taking account of the variance of the bearing capacity.Even an essentially deterministic analysis with a very small shear01234512345COV cuC O V NcΘc u= 0·5Θc u= 0·25Θc u= 0·125Θc u= 1·0Θc u= 2·0Θc u= 4·0Θc u= 8·0Θc u= ∞Fig.7.Estimated coef®cient of variation of the bearing capacityfactor COV N c s N c a m N c as a function of undrained shear strength statistics,Èc u COV c u00·10·20·30·42468101214Prandtl, 5·14f (N c )N cFig.8.Histogram and log-normal ®t for the computed bearing capacity factors when Èc u 2and COV c u The log-normal function has the properties N c 3X 31and s N c 2X 0800·10·20·30·40·50·60·70·80·91·010–11COV cu102345678923456789p (N c < 5·14)Θc u= 0·5Θc u= 1·0Θc u= 2·0Θc u= 4·0Θc u= ∞Fig.9.Graph showing the probability p (N c ,5X 14)that the bearing capacity factor will be lower than the Prandtl solution based on the mean strength356GRIFFITHS AND FENTON。
夸克流1
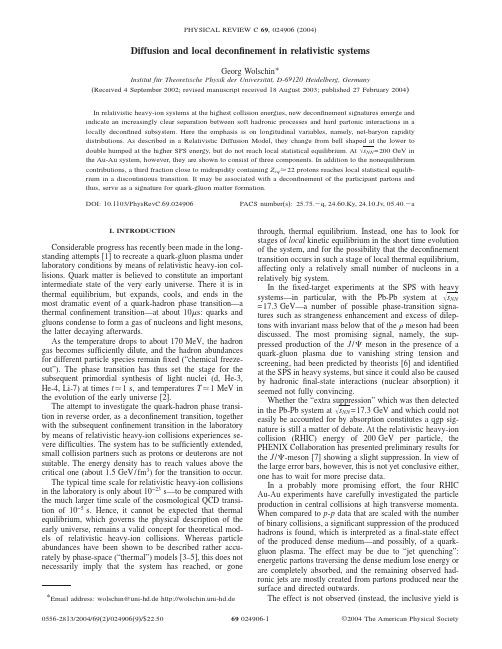
*Email address: wolschin@uni-hd.de http://wolschin.uni-hd.de
0556-2813/2004/69(2)/024906(9)/$22.50
through, thermal equilibrium. Instead, one has to look for stages of local kinetic equilibrium in the short time evolution of the system, and for the possibility that the deconfinement transition occurs in such a stage of local thermal equilibrium, affecting only a relatively small number of nucleons in a relatively big system. In the fixed-target experiments at the SPS with heavy systems—in particular, with the Pb-Pb system at ͱsNN = 17.3 GeV—a number of possible phase-transition signatures such as strangeness enhancement and excess of dileptons with invariant mass below that of the meson had been discussed. The most promising signal, namely, the suppressed production of the J / ⌿ meson in the presence of a quark-gluon plasma due to vanishing string tension and screening, had been predicted by theorists [6] and identified at the SPS in heavy systems, but since it could also be caused by hadronic final-state interactions (nuclear absorption) it seemed not fully convincing. Whether the “extra suppression” which was then detected in the Pb-Pb system at ͱsNN = 17.3 GeV and which could not easily be accounted for by absorption constitutes a qgp signature is still a matter of debate. At the relativistic heavy-ion collision (RHIC) energy of 200 GeV per particle, the PHENIX Collaboration has presented preliminary results for the J / ⌿-meson [7] showing a slight suppression. In view of the large error bars, however, this is not yet conclusive either, one has to wait for more precise data. In a probably more promising effort, the four RHIC Au-Au experiments have carefully investigated the particle production in central collisions at high transverse momenta. When compared to p- p data that are scaled with the number of binary collisions, a significant suppression of the produced hadrons is found, which is interpreted as a final-state effect of the produced dense medium—and possibly, of a quarkgluon plasma. The effect may be due to “jet quenching”: energetic partons traversing the dense medium lose energy or are completely absorbed, and the remaining observed hadronic jets are mostly created from partons produced near the surface and directed outwards. The effect is not observed (instead, the inclusive yield is
Mycoplasma Mastitis Causes,Transmission,and Control
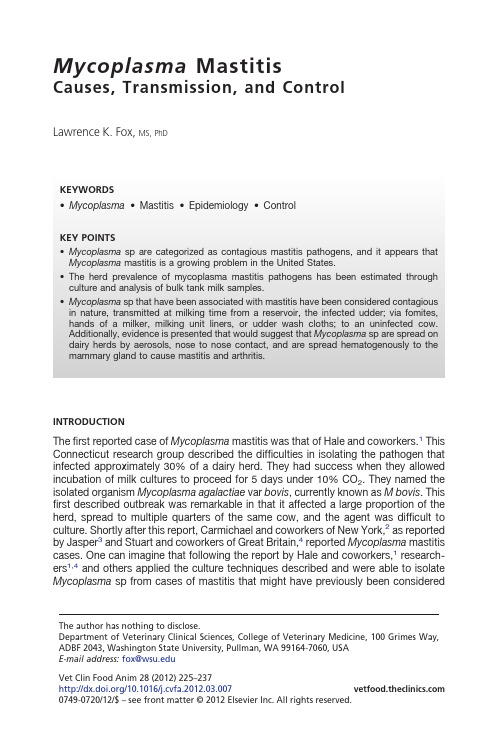
Mycoplasma MastitisCauses,Transmission,and Control Lawrence K.Fox,MS,PhDINTRODUCTIONThe first reported case of Mycoplasma mastitis was that of Hale and coworkers.1This Connecticut research group described the difficulties in isolating the pathogen that infected approximately 30%of a dairy herd.They had success when they allowed incubation of milk cultures to proceed for 5days under 10%CO 2.They named the isolated organism Mycoplasma agalactiae var bovis ,currently known as M bovis .This first described outbreak was remarkable in that it affected a large proportion of the herd,spread to multiple quarters of the same cow,and the agent was difficult to culture.Shortly after this report,Carmichael and coworkers of New York,2as reported by Jasper 3and Stuart and coworkers of Great Britain,4reported Mycoplasma mastitis cases.One can imagine that following the report by Hale and coworkers,1research-ers 1,4and others applied the culture techniques described and were able to isolate Mycoplasma sp from cases of mastitis that might have previously been considered The author has nothing to disclose.Department of Veterinary Clinical Sciences,College of Veterinary Medicine,100Grimes Way,ADBF 2043,Washington State University,Pullman,WA 99164-7060,USA E-mail address:fox@Vet Clin Food Anim 28(2012)225–237/10.1016/ 0749-0720/12/$–see front matter ©2012Elsevier Inc.All rights reserved.KEYWORDS•Mycoplasma •Mastitis •Epidemiology •ControlKEY POINTS•Mycoplasma sp are categorized as contagious mastitis pathogens,and it appears that Mycoplasma mastitis is a growing problem in the United States.•The herd prevalence of mycoplasma mastitis pathogens has been estimated through culture and analysis of bulk tank milk samples.•Mycoplasma sp that have been associated with mastitis have been considered contagious in nature,transmitted at milking time from a reservoir,the infected udder;via fomites,hands of a milker,milking unit liners,or udder wash cloths;to an uninfected cow.Additionally,evidence is presented that would suggest that Mycoplasma sp are spread on dairy herds by aerosols,nose to nose contact,and are spread hematogenously to the mammary gland to cause mastitis and arthritis.226Foxidiopathic.Thus,50years ago it was apparent that Mycoplasma mastitis was a problem,perhaps an emerging problem.Today it is recognized that Mycoplasma mastitis affects cattle around the world.5,6 Mycoplasma sp are categorized as contagious mastitis pathogens7and it appears that Mycoplasma mastitis is a growing problem in the United States.3,8–10Moreover, given the difficulty in culturing the pathogen that wasfirst noted50years ago,there is reason to suspect that cases of Mycoplasma mastitis are underreported.11In this review the epidemiology of Mycoplasma mastitis will be discussed,followed by a discussion of the host–pathogen interaction and elements associated with control of the disease.A focus of this article will be the presentation of recentfindings that would explain why Mycoplasma may be an emerging mastitis pathogen.EPIDEMIOLOGYMycoplasma sp are pathogens associated with several cattle diseases,primarily otitis media,inflammation of the urogenital tract,arthritis,pneumonia,and mastitis.12,13 The most prevalent species causing these diseases is M bovis.5,14With respect to Mycoplasma mastitis,M bovis is the predominant causative agent and M californicum and M bovigenitalium appear to the next most common(Table1).Jasper15summarized the agents associated with cases of clinical Mycoplasma mastitis during a14-year period and found that M bovis and californicum were the most common.The third most common was M alkalescens,which comprised approximately12%of intramammary infections,followed by M bovigenitalium at5% (see Table1).Kirk and coworkers16surveyed bulk tank milk from a cooperative of267 dairies in CA monthly for6years.The annual prevalence of tanks with Mycoplasma sp known to be mastitis agents ranged from1.2%to3.1%of tank samples.They reported that M bovis,californicum,and bovigenitalium were the most consistently the Mycoplasma mastitis agents isolated.Boonyayatra and colleagues17examined milk samples from248cases of clinical mastitis from a variety of sources over several years and reported85%were M bovis,5%were M californicum,and only1%were M bovigenitalium.In the surveys reported in Table1,it is clear that M bovis and M californicum appear to be the2most prevalent Mycoplasma mastitis pathogens.Other species that have been noted as causes of Mycoplasma mastitis include M arginini, bovirhinis,canadense,dispar,bovine group7,and F-38.18PrevalencePrevalence of contagious mastitis pathogens estimates have been made through culture and analysis of bulk tank milk samples.9,19The major contagious mastitis pathogens identified this way in the United States are Staphylococcus aureus, Streptococcus agalactiae,and Mycoplasma sp,with herd level prevalence of43.0%,2.6%,and3.2%.9In this survey,9the herd size affected the prevalence of onlyMycoplasma mastitis,with the prevalence of other contagious mastitis pathogensunaltered by the number of cows per herd.In large herds(Ͼ500cows),the prevalence of Mycoplasma mastitis was14.4%.Results from a previous study were similar as it was reported that the percentages of Mycoplasma positive bulk tanks from herds with less than100,100to499,and more than500cows was2.1%,3.9%,and21.7%.8In the later survey,regional differences were noted with9.4%of the operations in the West having one positive Mycoplasma bulk tank culture,with operations in the Northeast and Midwest with less than3%and the Southeast having6.6%.Presum-ably,the regional differences are a function of herd size as herds in the West tend to have the most cows and herds in the Northeast and Midwest tend to have the fewest number of cows.20Based on bulk tank surveys,the prevalence of Mycoplasma mastitis varies across the globe.In the European Union countries of Belgium,France,and Greece,the range in prevalence was less than1%to5.4%of herds.21–23Yet surveys done in Mexico,24 Iran,25and Australia26indicate prevalence estimates as high as55%to100%of herds.In New Zealand,McDonald and coworkers27surveyed244herds and could not detect Mycoplasma sp in any bulk tank samples,suggesting a very low prevalence.The wide variation in global prevalence may be a function of exposure to these agents.Importation and mixing of cattle have been reported to lead to outbreaks of Mycoplasma diseases.For example,thefirst reported case of Myco-plasma cattle disease in Ireland occurred in1993and was attributed to the relaxation of import controls within the European Union.28Exposure of naïve cattle to this agent led to the appearance and then a significant increase in bovine Mycoplasma diseases.28Herd replacement cattle exposed to cattle outside the herd,either imported or reared off-site,increased with increasing herd size,a biosecurity risk factor.29It was found that herd size10,30and culling30were risk factors for increased herd prevalence of Mycoplasma mastitis.Presumably this is a result of herd expansion,the entrance of new cattle with symptomatic,or asymptomatic carriage of new strains of Mycoplasma sp into the herd.Thus,the elevated prevalence of Mycoplasma mastitis in herds,and herds of some countries,where cattle movement into and out of a herd is common,could explain the increased prevalence of this disease.Cow-level prevalence is more difficult to estimate.It has been reported that in Great Britain,less than1%of cows are affected by Mycoplasma mastitis.31Mycoplasma mastitis has most often been reported as a clinical disease.A survey of clinical mastitis in New York indicates that Mycoplasma sp are the cause of1.5%of cases.32 TransmissionMycoplasma sp that have been associated with mastitis have been considered contagious in nature,transmitted mostly at milking time from a reservoir,the infected udder;via fomites,hands of a milker,milking unit liners,or udder wash cloths;to an uninfected cow.7Strict milking time hygiene practices of disinfectant of udders before milking using single service towels,use of gloves by milkers,post-milking unit disinfection,and disinfection of teats post-milking were very effective in controlling the traditional contagious mastitis pathogens of S aureus and S agalactiae.33It has been assumed,but not tested,that such practices would be effective in the control of Mycoplasma mastitis.Mycoplasma sp can spread from one bovine body site to another presumably via lymph or peripheral blood systems.Mycoplasma sp associated with mastitis have been isolated from the blood of cattle.34–36In outbreaks with Mycoplasma mastitis,it is not unusual tofind cases of Mycoplasma arthritis.37–41Similarly,afield outbreak of Mycoplasma-associated bovine respiratory disease was associated with outbreaks of 227Mycoplasma Mastitis Causes,Transmission,and Control228Foxarthritis.41The link between arthritic Mycoplasma disease events and mastitis or pneumonia is indicative that internal somatic spread of this agent is not uncommon.Often multiple organ sites of cattle can be colonized and it is clear that the strain causing the disease is most often the same strain that is widely disseminated throughout the body.35This is also been shown by Jain and colleagues,42who experimentally induced intramammary infections with Mycoplasma sp in lactating cows and found that the apparent strain inoculated was shed at the mucosal surfaces of the eyes,nose,vagina,and rectum,within hours to days after inoculation.With this experiment,they also demonstrated vertical transfer of the agent as a calf,born during the trial from one experimentally infected cow,became colonized by the agent.42Moreover,in an outbreak of Mycoplasma mastitis,the agent was found colonizing the nares of cattle,both cows and/or calves.43,44The strain causing mastitis was found from nasal swab samples collected from cows and calves.40Thus, transmission of Mycoplasma sp associated with bovine mastitis may occur within the cow internally,from one infected organ site to the udder or reverse;and between cows from indirect udder to udder contact at milking time;or perhaps by shedding of the pathogen through external mucosal surfaces of an infected or colonized animal toa naïve animal.Transmission of Mycoplasma sp from environmental sources to the udder has been discussed.18In this review,the authors report on2studies,1in Italy and1in Germany,where it was found that M bovis survived in and on multiple surfaces at various temperatures for up to8months.Materials studied were those that could be typically found on dairies including sponges,stainless steel,wood,rubber,glass,and water.Justice-Allen and coworkers45in Utah discovered that Mycoplasma could live for up to8months in a sand pile.The sand originated from a herd with an outbreak of Mycoplasma mastitis.Mycoplasma was also isolated in sand from2other dairies.The authors45suggested that sand could be a reservoir for Mycoplasma mastitis.However,in a separate investigation where there appeared to be a link between sand bedding and a clinical mastitis outbreak,it was found that the strains of Mycoplasma sp in the bedding had a completely different DNAfingerprint than those causing mastitis(Fox and Corbett,unpublished data,2008).Utah researchers46investigated the possible transmission of M bovis from sand to naïve dairy calves during a105-day trial.Although calves housed on sand bedding with M bovis carried this agent for periods of time during the trial,there was no evidence of carriage beyond transient colonization and no specific antibody titers formed against the agent.The authors concluded that there was no evidence that the contaminated bedding would serve asa source of M bovis disease transmission to naïve dairy calves.Thus,although it isclear that environmental sources could serve as a reservoir for Mycoplasma mastitis, there is no evidence to support that M bovis transmission from the environment to a cow is a likely mechanism involved in Mycoplasma mastitis.CarriageMost cases of mastitis are subclinical and the greatest loss to a dairy is a result of the subclinical nature of the disease.47Jasper48indicates that a significant number of cows might be shedding Mycoplasma pathogens in their milk without symptoms.Perhaps given the difficulty,expense,and the historically low prevalence of the Mycoplasma mastitis,a good estimate of the prevalence of subclinical Mycoplasma mastitis infections has not been reported.It is well established that Mycoplasma sp can be isolated from mucosal surfaces of clinically normal calves and cows.40,49The prevalence of calves shedding M bovis at the nares was34%in herds with noted Mycoplasma mastitis and only6%in herdsapparently free of disease.49The prevalence of mucosal surface shedding by asymptomatic carriers with the same clone of M bovis causing a Mycoplasma-associated disease outbreak may be as high as21%to47%of cattle in a dairy herd.40Thesefindings indicate that Mycoplasma shedding by ostensibly healthy cattle is not uncommon but may be far more likely in herds experiencing a current outbreak.The role of the asymptomatic Mycoplasma carrier animal in an outbreak of mastitis is not clear.It is known that M bovis carriage in the lungs of beef cattle calves is approximately that of dairy calves in situations without apparent Mycoplasma disease.Carriage increases when cattle are stressed,such as when they are moved from their place of rearing and then comingled in different locations as in feedlots.12 Climatic stresses and Mycoplasma disease outbreaks have also been documented. Episodes of Mycoplasma pneumonia were observed in a closed beef herd where a number of calves became diseased after a spring storm.50Only1strain of M bovis was identified from pulmonary samples.Given the herd50was closed,it could be suspected that the strain identified was asymptomatically carried by cattle of this herd,and with climatic stresses and potentially associated compromised hosts,the M bovis strain was able to transform cattle from symptomless to diseased.Thus,a change in the environment of the calf,a move away from their accustomed setting,a change in climate,and/or the exposure to potential new strains of Mycoplasma sp can increase the prevalence of carriage of these agents and such carriage might be associated with subclinical or clinical disease.A dairy herd will generally increase the exposure of its herd,to outside animals, through the purchase of replacements and via off-site rearing of calves.The University of Idaho dairy with approximately90to100lactating cows was historically free of Mycoplasma mastitis,and ostensibly other Mycoplasma diseases were rare or nonexistent.An outbreak of Mycoplasma-associated diseases at the University of Idaho dairy began shortly after a state institutional herd contracted with the dairy to raise their calves.The institutional herd also leased their primparae to the university dairy.40Within2months of initiating the contract,several cases of Mycoplasma diseases in calves and mastitis in cows developed.Diseased animals were culled from the herd,and during the third month of the initial outbreak,samples of mucosal surfaces of all animals were collected.Nearly25%of all animals were shedding the same clone of M bovis from the mucosal surfaces as that causing disease.Yet,within 6months only1cow and1calf were shedding the clone.During the course of the next year,the outbreak strain was infrequently detected.However,the outbreak clone was the only cause of Mycoplasma mastitis,with4cases occurring in total.One case spontaneously cured,and the other3cases were removed from the herd.New strains of Mycoplasma sp were detected,and these strains appeared to be very similar to the outbreak clone.None of these similar strains caused disease.Thesefindings suggest an outbreak strain may be widely disseminated within a herd initially,with a few cases of disease,but concomitant with the dissolution of the outbreak is the reduction of shedding of the agent from mucosal surfaces.Additionally,the authors40concluded that the outbreak strain originated with the animals exposed to the institutional dairy herd and thus was imported into the herd.Punyapornwithaya and colleagues41also reported on an outbreak of Mycoplasma mastitis that appeared to originate with an imported heifer.The M bovis clone that caused mastitis in the original heifer at parturition also caused mastitis,pneumonia,and arthritis in the home herd of lactating cows.The strain then“ran its course”and disappeared after4months.A similar outbreak of M bovis disease was reported to start with mastitis.44Here an imported heifer developed mastitis at parturition,and within a few weeks several of the 229Mycoplasma Mastitis Causes,Transmission,and Control230Foxhomebred cows developed M bovis mastitis,1cow developed arthritis,and several calves developed pneumonia.These reports40,41,44demonstrate that in an outbreak, there is the potential for multiple animals to become infected with several forms of Mycoplasma disease.In aggregate,these studies40,41indicate that a single clone of M bovis can readily transmit through the herd,but only a small proportion of cows become infected,and both asymptomatic carrier(s)or diseased animals can be the nidus of the outbreak.The nature of transmission might have been during milking time in one herd41but in the other40it was concluded by the authors that nose-to-nose contact was the most likely means of transmission.Pulmonary transmission would account for the rapid spread,the involvement of both lactating and nonlactating animals,and the involvement of both respiratory and joint diseases.Both Bicknell and colleagues51and Jasper49discuss the role of the asymptomatic carrier in Myco-plasma mastitis disease outbreaks.Both warn that asymptomatic carriers may be reservoirs of disease,although neither author presents evidence that such an outcome is likely or unlikely.Jasper49indicates that some dairy managers will cull asymptomatic Mycoplasma carriers and some will isolate carriers until shedding subsides;successful control can be achieved with either method.The odds of an asymptomatic carrier causing an outbreak is unknown.Additionally,preferential culling or isolation of carrier animals was not apparently necessary to control Mycoplasma mastitis,and no animal appeared to be an asymptomatic carrier prior to the appearance of Mycoplasma mastitis.40Additionally,a cow or cows with Mycoplasma mastitis may not pose a risk to the development of an outbreak and may not need to be preferentially culled to control transmission.52It appears that asymptomatic carriage of Mycoplasma sp is involved in a Mycoplasma mastitis outbreaks.However,the definitive role carrier animals play in the outbreak and how they should be controlled are unclear.If culling asymptomatic carriers is chosen as a Mycoplasma mastitis control strategy,then it should be used judiciously while considering the number of potential culls and their proximity to susceptible animals.Isolation of affected animals and monitoring new carrier and infected animals might be effective tools of control of Mycoplasma mastitis.CHARACTERISTICS OF PATHOGENIC MYCOPLASMA SPRazin and Hayflick52have recently reviewed the research on Mycoplasma sp.They report that the Mycoplasma sp evolved from gram-positive bacteria in a degenerative evolution where these simple organisms lost the ability to produce a cell wall,one manifestation of the diminution of the genome.Razin and Hayflick53wrote that Mycoplasma cells have essentially3organelles:cell membrane,ribosomes,and densely packed circular DNA.The Mycoplasma cell is spherical about0.3to0.8m in diameter.The species have a significant requirement for fatty acids and sterols and intermediate metabolic pathways are often truncated.Mycoplasma sp are perhaps the smallest and most simple self-replicating bacteria.54Given their simple nature and fastidious growth requirements,theyfind ecological niches within their host.In cases of intramammary infections,Mycoplasma sp do not appear to often cause a significant,if any,febrile response,48,55,56which may be consistent with their nature to colonize cows asymptomatically.Mycoplasma sp lack a cell wall and thus are inherently resistant to beta-lactam antibiotics.The study of the pathogenicity of Mycoplasma organisms is diverse given that there are more than100Mycoplasma sp,with most of these pathogens specific for one or a few host species.Yet it appears that the pathogenic characteristics of Mycoplasm a sp in general are(1)adherence to host cells,(2)internalization into hostcells,(3)immunomodulatory characteristics,and(4)ability to colonize host tissue without causing fulminant disease.Several Mycoplasma sp including M bovis possess adhesion molecules as part of their cell membranes,which allow them to bind to host tissue cells.57M pneumonia, for example,possesses a protein complex(P1,P30,P116,HMW1-3,A,B,and C)that provides for structural and functional adherence to cells and enables gliding mobil-ity.58M bovis possesses variable surface lipoproteins(Vsps)that are involved in adherence to host cells.59–61These Vsps are part of a complex bacterial system that is notably most antigenically diverse and associated with much variation in gene expression.60,62,63Browning and colleagues64describe the high-frequency phase variation of the multigene families that encode surface proteins that are part of the Mycoplasma sp genome.They indicate that it has been generally accepted that the antigenic variation that results from the genetic phase variation is an immune evasion characteristic,although this hypothesis has not been tested.Adherence to mammary epithelial surfaces is a characteristic of contagious mastitis pathogens,and this adherence characteristic appears to differentiate the contagious from the noncontagious mastitis pathogens.65,66It would be logical to assume that since Mycoplasma sp are considered contagious mastitis pathogens and as Mycoplasma mastitis pathogens are likely to produce cytadhesins,they would also have the ability to adhere to mammary epithelial cells,although this has been untested.There may be other benefits to these adhesion proteins.The ability to adhere to host cell mucosal surfaces may enable the Mycoplasma sp to access nutrients including amino acids,nucleic acids,fatty acids,and sterols.67Mycoplasma sp tend to have truncated intermediate metabolic pathways53and thus have significant nutrient requirements,especially for sterols and fatty acids.Pathogens that have the ability to invade and survive within the host cell have the advantage of the protection that the host cell affords against the host’s own immune response and antimicrobial therapy.The mastitis pathogen Staphylococcus aureus has been described to possess this factor.68The ability to invade mammary epithelial cells may be a function of the virulence of the S aureus mastitis pathogen.69 Mycoplasma sp have the ability to invade eukaryotic host cells.70–72There is evidence to indicate that M bovis can invade peripheral blood mononuclear cells and erythro-cytes in vitro73and in both renal tubular epithelial cells and hepatocytes in clinically diseased bull calves determined at necropsy.74van de Merwe and colleagues73 acknowledge that what might have seemed to be M bovis–induced invasion might have been a phagocytic response by specific immunocytes.However,M bovis appeared to be internalized by lymphocytes and erythrocytes.Not only would such internalization afford the pathogen protection from the immune response and antibiotic treatment,but this characteristic would enable it to reach multiple organ systems,consistent with the ability of M bovis to spread to multiple body sites of diseased cattle.35,55M bovis has the ability to modulate the immune system.Findings by van der Merwe and colleagues73and Vanden Bush and Rosenbusch75indicate the pathogen secretes a peptide,a factor that can inhibit lymphocyte proliferation.This factor appears in the culture supernatant.73In addition,M bovis can cause immunomodu-lation of both the humoral and cell-mediated responses.Antibody titers may be reduced in M bovis–affected cattle,76and the ratio of IgG1to IgG2was reversed in some pneumonic calves.77,78An alteration in the T-helper cell response to M bovis lung infections was noted,79and there was evidence indicating that anti-inflammatory cytokine production was altered by an M bovis infection.231Mycoplasma Mastitis Causes,Transmission,and Control232FoxCONTROLHistorically,it has been thought that Mycoplasma mastitis might be best controlled bya test and slaughter program.Cows with Mycoplasma mastitis need to be identifiedand culled from the herd.6,18,80A critical component of this Mycoplasma mastitis control program is a monitoring system.First,a potential problem with Mycoplasma mastitis must be known and cows suspected of Mycoplasma mastitis must be identified and verified as diseased.Culture of bulk tank milk on a regular basis is a method to monitor a herd’s Mycoplasma mastitis status,10,16,81and such regular sampling and culture of bulk tank milk as a monitor of Mycoplasma mastitis in a herd have been advocated.52It is generally believed that the culture of Mycoplasma sp from bulk tank milk is indicative of at least one herd cow having Mycoplasma mastitis, although a negative culture does not necessarily indicate that the herd is free of this disease.82If a herd has zero tolerance of Mycoplasma mastitis,then a positive bulk tank culture must be followed by the identification of cows with mastitis.Generally, cows with recent or chronic cases of clinical mastitis would be identified and milk from infected mammary quarters cultured and tested for Mycoplasma sp.Addition-ally,cows with elevated milk somatic cell counts would be identified and milk cultured.Cows once identified with Mycoplasma mastitis would be culled from the herd.However,the process of collection of a sample,transport to the laboratory,and culture and identification of the agent can take at least4to7days,an interim period.Cows may be penned with other infirm cows without Mycoplasma mastitis during this interim period.The transmission of Mycoplasma mastitis within these hospital pens might be as much as100-fold more than in the cow’s home pen.41Thus,hospital pen cows must be managed carefully to control this disease such that Mycoplasma mastitis is not transmitted to the home pens,when cows falsely believed to be free of this disease are returned.The test and slaughter method of control might not be required.Some53,83,84 reported that control could be achieved without culling,although another report indicated success with specific removal of cows with Mycoplasma mastitis.85 Mycoplasma mastitis as a contagious mastitis pathogen should be controlled by full milking time hygiene practices that include disinfectant in the udder premilking wash, single service towels used to clean and dry udders premilking,use of clean gloved hands by milkers,milking unit backflush,and postmilking teat disinfection.52Bios-ecurity practices of isolation of all cattle before entry into a new herd,the testing of those cattle for carriage of Mycoplasma sp and elimination of those testing positive prior to entry into the herd,would in theory be an effective control strategy.Yet such a strategy does not appear to be a most common practice.52Quarantine of incoming animals requires considerable management as a practice.Quarantine as a control ofa disease like Mycoplasma mastitis,that is emerging but affects a minority of cattleand herds,may not be cost effective.Yet M bovis was believed to be asymptomat-ically carried from imported cows into a herd believed to have been free of Mycoplasma mastitis.40Such carriage resulted into an outbreak of Mycoplasma diseases,mastitis,arthritis,and pneumonia,in cows and replacements in this herd.Control of Mycoplasma mastitis via treatment is generally not viewed as a primary strategy.It is clear from previous discussion that the immune system will respond to Mycoplasma sp as a foreign agent.Yet it is also clear that Mycoplasma sp have the ability to evade the immune system by altering their surface proteins and inducing immunomodulatory effects.Perhaps the latter2characteristics would explain in part the heretofore lack of a successful development of mastitis vaccines against this agent.6,18An excellent review of Mycoplasma mastitis therapy can be found in。
Stata xtgee 回归分析工具包用户指南说明书
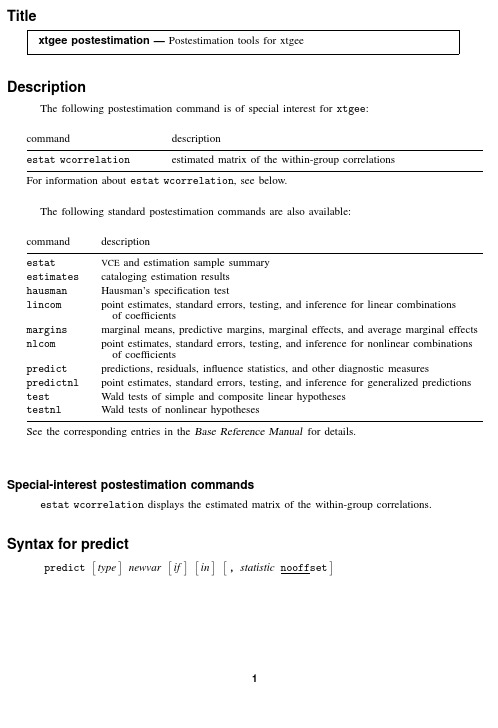
Titlextgee postestimation —Postestimation tools for xtgeeDescriptionThe following postestimation command is of special interest for xtgee :commanddescriptionestat wcorrelationestimated matrix of the within-group correlationsFor information about estat wcorrelation ,see below.The following standard postestimation commands are also available:command descriptionestatVCE and estimation sample summaryestimates cataloging estimation results hausman Hausman’s specification testlincom point estimates,standard errors,testing,and inference for linear combinations of coefficientsmargins marginal means,predictive margins,marginal effects,and average marginal effects nlcom point estimates,standard errors,testing,and inference for nonlinear combinations of coefficientspredict predictions,residuals,influence statistics,and other diagnostic measurespredictnl point estimates,standard errors,testing,and inference for generalized predictions test Wald tests of simple and composite linear hypotheses testnlWald tests of nonlinear hypothesesSee the corresponding entries in the Base Reference Manual for details.Special-interest postestimation commandsestat wcorrelation displays the estimated matrix of the within-group correlations.Syntax for predictpredicttypenewvarifin,statistic nooffset12xtgee postestimation—Postestimation tools for xtgeestatistic descriptionMainmu predicted value of depvar;considers the offset()or exposure();the default rate predicted value of depvarpr(n)probability Pr(y j=n)for family(poisson)link(log)pr(a,b)probability Pr(a≤y j≤b)for family(poisson)link(log)xb linear predictionstdp standard error of the linear predictionscorefirst derivative of the log likelihood with respect to x jβThese statistics are available both in and out of sample;type predict...if e(sample)...if wanted only for the estimation sample.MenuStatistics>Postestimation>Predictions,residuals,etc.Options for predict££Main mu,the default,and rate calculate the predicted value of depvar.mu takes into account the offset() or exposure()together with the denominator if the family is binomial;rate ignores those adjustments.mu and rate are equivalent if you did not specify offset()or exposure()when youfit the xtgee model and you did not specify family(binomial#)or family(binomial varname),meaning the binomial family and a denominator not equal to one.Thus mu and rate are the same for family(gaussian)link(identity).mu and rate are not equivalent for family(binomial pop)link(logit).Then mu would predict the number of positive outcomes and rate would predict the probability of a positive outcome.mu and rate are not equivalent for family(poisson)link(log)exposure(time).Then mu would predict the number of events given exposure time and rate would calculate the incidence rate—the number of events given an exposure time of1.pr(n)calculates the probability Pr(y j=n)for family(poisson)link(log),where n is a nonnegative integer that may be specified as a number or a variable.pr(a,b)calculates the probability Pr(a≤y j≤b)for family(poisson)link(log),where a andb are nonnegative integers that may be specified as numbers or variables;b missing(b≥.)means+∞;pr(20,.)calculates Pr(y j≥20);pr(20,b)calculates Pr(y j≥20)in observations for which b≥.and calculatesPr(20≤y j≤b)elsewhere.pr(.,b)produces a syntax error.A missing value in an observation of the variable a causes a missing value in that observation for pr(a,b).xb calculates the linear prediction.stdp calculates the standard error of the linear prediction.xtgee postestimation —Postestimation tools for xtgee 3score calculates the equation-level score,u j =∂ln L j (x j β)/∂(x j β).nooffset is relevant only if you specified offset(varname ),exposure(varname ),fam-ily(binomial #),or family(binomial varname )when you fit the model.It modifies the calculations made by predict so that they ignore the offset or exposure variable and the binomial denominator.Thus predict ...,mu nooffset produces the same results as predict ...,rate .Syntax for estat wcorrelationestat wcorrelation,compact format(%fmt )MenuStatistics>Postestimation>Reports and statisticsOptions for estat wcorrelationcompact specifies that only the parameters (alpha)of the estimated matrix of within-group correlations be displayed rather than the entire matrix.format(%fmt )overrides the display format;see [D ]format .RemarksExample 1xtgee can estimate rich correlation structures.In example 2of [XT ]xtgee ,we fit the model.use /data/r11/nlswork2(National Longitudinal Survey.Young Women 14-26years of age in 1968).xtgee ln_w grade age c.age#c.age (output omitted )After estimation,estat wcorrelation reports the working correlation matrix R :.estat wcorrelationEstimated within-idcode correlation matrix R:c1c2c3c4c5c6r11r2.48513561r3.4851356.48513561r4.4851356.4851356.48513561r5.4851356.4851356.4851356.48513561r6.4851356.4851356.4851356.4851356.48513561r7.4851356.4851356.4851356.4851356.4851356.4851356r8.4851356.4851356.4851356.4851356.4851356.4851356r9.4851356.4851356.4851356.4851356.4851356.4851356c7c8c9r71r8.48513561r9.4851356.485135614xtgee postestimation—Postestimation tools for xtgeeThe equal-correlation model corresponds to an exchangeable correlation structure,meaning that the correlation of observations within person is a constant.The working correlation estimated by xtgee is0.4851.(xtreg,re,by comparison,reports0.5140.)We constrained the model to have this simple correlation structure.What if we relaxed the constraint?To go to the other extreme, let’s place no constraints on the matrix(other than its being symmetric).We do this by specifying correlation(unstructured),although we can abbreviate the option..xtgee ln_w grade age c.age#c.age,corr(unstr)nologGEE population-averaged model Number of obs=16085Group and time vars:idcode year Number of groups=3913Link:identity Obs per group:min=1Family:Gaussian avg= 4.1Correlation:unstructured max=9Wald chi2(3)=2405.20 Scale parameter:.1418513Prob>chi2=0.0000ln_wage Coef.Std.Err.z P>|z|[95%Conf.Interval]grade.0720684.00215133.500.000.0678525.0762843age.1008095.008147112.370.000.0848416.1167775c.age#c.age-.0015104.0001617-9.340.000-.0018272-.0011936_cons-.8645484.1009488-8.560.000-1.062404-.6666923.estat wcorrelationEstimated within-idcode correlation matrix R:c1c2c3c4c5c6r11r2.43548381r3.4280248.55973291r4.3772342.5012129.54751131r5.4031433.5301403.502668.62162271r6.3663686.4519138.4783186.5685009.73060051r7.2819915.3605743.3918118.4012104.4642561.50219r8.3162028.3445668.4285424.4389241.4696792.5222537r9.2148737.3078491.3337292.3584013.4865802.4613128c7c8c9r71r8.64756541r9.5791417.73865951This correlation matrix looks different from the previously constrained one and shows,in particular, that the serial correlation of the residuals diminishes as the lag increases,although residuals separated by small lags are more correlated than,say,AR(1)would imply.Example2In example1of[XT]xtprobit,we showed a random-effects model of unionization using the union data described in[XT]xt.We performed the estimation using xtprobit but said that we could have used xtgee as well.Here wefit a population-averaged(equal correlation)model for comparison:xtgee postestimation—Postestimation tools for xtgee5 .use /data/r11/union(NLS Women14-24in1968).xtgee union age grade i.not_smsa south##c.year,family(binomial)link(probit)Iteration1:tolerance=.12544249Iteration2:tolerance=.0034686Iteration3:tolerance=.00017448Iteration4:tolerance=8.382e-06Iteration5:tolerance=3.997e-07GEE population-averaged model Number of obs=26200Group variable:idcode Number of groups=4434Link:probit Obs per group:min=1Family:binomial avg= 5.9Correlation:exchangeable max=12Wald chi2(6)=242.57 Scale parameter:1Prob>chi2=0.0000 union Coef.Std.Err.z P>|z|[95%Conf.Interval]age.0089699.0053208 1.690.092-.0014586.0193985grade.0333174.0062352 5.340.000.0210966.04553821.not_smsa-.0715717.027543-2.600.009-.1255551-.01758841.south-1.017368.207931-4.890.000-1.424905-.6098308year-.0062708.0055314-1.130.257-.0171122.0045706 south#c.year1.0086294.00258 3.340.001.0035727.013686_cons-.8670997.294771-2.940.003-1.44484-.2893592Let’s look at the correlation structure and then relax it:.estat wcorrelation,format(%8.4f)Estimated within-idcode correlation matrix R:c1c2c3c4c5c6c7 r1 1.0000r20.4615 1.0000r30.46150.4615 1.0000r40.46150.46150.4615 1.0000r50.46150.46150.46150.4615 1.0000r60.46150.46150.46150.46150.4615 1.0000r70.46150.46150.46150.46150.46150.4615 1.0000r80.46150.46150.46150.46150.46150.46150.4615r90.46150.46150.46150.46150.46150.46150.4615r100.46150.46150.46150.46150.46150.46150.4615r110.46150.46150.46150.46150.46150.46150.4615r120.46150.46150.46150.46150.46150.46150.4615c8c9c10c11c12r8 1.0000r90.4615 1.0000r100.46150.4615 1.0000r110.46150.46150.4615 1.0000r120.46150.46150.46150.4615 1.0000We estimate thefixed correlation between observations within person to be0.4615.We have many data(an average of5.9observations on4,434women),so estimating the full correlation matrix is feasible.Let’s do that and then examine the results:6xtgee postestimation—Postestimation tools for xtgee.xtgee union age grade i.not_smsa south##c.year,family(binomial)link(probit)>corr(unstr)nologGEE population-averaged model Number of obs=26200Group and time vars:idcode year Number of groups=4434Link:probit Obs per group:min=1Family:binomial avg= 5.9Correlation:unstructured max=12Wald chi2(6)=198.45 Scale parameter:1Prob>chi2=0.0000union Coef.Std.Err.z P>|z|[95%Conf.Interval]age.0096612.0053366 1.810.070-.0007984.0201208grade.0352762.0065621 5.380.000.0224148.04813771.not_smsa-.093073.0291971-3.190.001-.1502983-.03584781.south-1.028526.278802-3.690.000-1.574968-.4820839year-.0088187.005719-1.540.123-.0200278.0023904 south#c.year1.0089824.00348652.580.010.002149.0158158_cons-.7306192.316757-2.310.021-1.351451-.109787.estat wcorrelation,format(%8.4f)Estimated within-idcode correlation matrix R:c1c2c3c4c5c6c7 r1 1.0000r20.6667 1.0000r30.61510.6523 1.0000r40.52680.57170.6101 1.0000r50.33090.36690.40050.4783 1.0000r60.30000.37060.42370.45620.6426 1.0000r70.29950.35680.38510.42790.49310.6384 1.0000r80.27590.30210.32250.37510.46820.55970.7009r90.29890.29810.30210.38060.46050.50680.6090r100.22850.25970.27480.36370.39810.49090.5889r110.23250.22890.26960.32460.35510.44260.5103r120.23590.23510.25440.31340.34740.38220.4788c8c9c10c11c12r8 1.0000r90.6714 1.0000r100.59730.6325 1.0000r110.56250.57560.5738 1.0000r120.49990.54120.53290.6428 1.0000As before,wefind that the correlation of residuals decreases as the lag increases,but more slowly than an AR(1)process.Example3In this example,we examine injury incidents among20airlines in each of4years.The data are fictional,and,as a matter of fact,are really from a random-effects model.xtgee postestimation—Postestimation tools for xtgee7.use /data/r11/airacc.generate lnpm=ln(pmiles).xtgee i_cnt inprog,family(poisson)eform offset(lnpm)nologGEE population-averaged model Number of obs=80Group variable:airline Number of groups=20Link:log Obs per group:min=4Family:Poisson avg= 4.0Correlation:exchangeable max=4Wald chi2(1)= 5.27 Scale parameter:1Prob>chi2=0.0217 i_cnt IRR Std.Err.z P>|z|[95%Conf.Interval]inprog.9059936.0389528-2.300.022.8327758.9856487lnpm(offset).estat wcorrelationEstimated within-airline correlation matrix R:c1c2c3c4r11r2.46064061r3.4606406.46064061r4.4606406.4606406.46064061Now there are not really enough data here to reliably estimate the correlation without any constraints of structure,but here is what happens if we try:.xtgee i_cnt inprog,family(poisson)eform offset(lnpm)corr(unstr)nologGEE population-averaged model Number of obs=80Group and time vars:airline time Number of groups=20Link:log Obs per group:min=4Family:Poisson avg= 4.0Correlation:unstructured max=4Wald chi2(1)=0.36 Scale parameter:1Prob>chi2=0.5496 i_cnt IRR Std.Err.z P>|z|[95%Conf.Interval]inprog.9791082.0345486-0.600.550.9136826 1.049219lnpm(offset).estat wcorrelationEstimated within-airline correlation matrix R:c1c2c3c4r11r2.57002981r3.716356.41921261r4.2383264.3839863.35212871There is no sensible pattern to the correlations.We created this dataset from a random-effects Poisson model.We reran our data-creation program and this time had it create400airlines rather than20,still with4years of data each.Here are the equal-correlation model and estimated correlation structure8xtgee postestimation—Postestimation tools for xtgee.use /data/r11/airacc2,clear.xtgee i_cnt inprog,family(poisson)eform offset(lnpm)nologGEE population-averaged model Number of obs=1600Group variable:airline Number of groups=400Link:log Obs per group:min=4Family:Poisson avg= 4.0Correlation:exchangeable max=4Wald chi2(1)=111.80 Scale parameter:1Prob>chi2=0.0000 i_cnt IRR Std.Err.z P>|z|[95%Conf.Interval]inprog.8915304.0096807-10.570.000.8727571.9107076lnpm(offset).estat wcorrelationEstimated within-airline correlation matrix R:c1c2c3c4r11r2.52917071r3.5291707.52917071r4.5291707.5291707.52917071The following estimation results assume unstructured correlation:.xtgee i_cnt inprog,family(poisson)corr(unstr)eform offset(lnpm)nologGEE population-averaged model Number of obs=1600Group and time vars:airline time Number of groups=400Link:log Obs per group:min=4Family:Poisson avg= 4.0Correlation:unstructured max=4Wald chi2(1)=113.43 Scale parameter:1Prob>chi2=0.0000 i_cnt IRR Std.Err.z P>|z|[95%Conf.Interval]inprog.8914155.0096208-10.650.000.8727572.9104728lnpm(offset).estat wcorrelationEstimated within-airline correlation matrix R:c1c2c3c4r11r2.47331891r3.5240576.57488681r4.5139748.5048895.58407071The equal-correlation model estimated afixed correlation of0.5292,and above we have correlations ranging between0.4733and0.5841with little pattern in their structure.xtgee postestimation—Postestimation tools for xtgee9Methods and formulasAll postestimation commands listed above are implemented as ado-files.Also see[XT]xtgee—Fit population-averaged panel-data models by using GEE[U]20Estimation and postestimation commands。
Response to Intervention Understanding the Three-Tier Model干预反应的理解三层模型
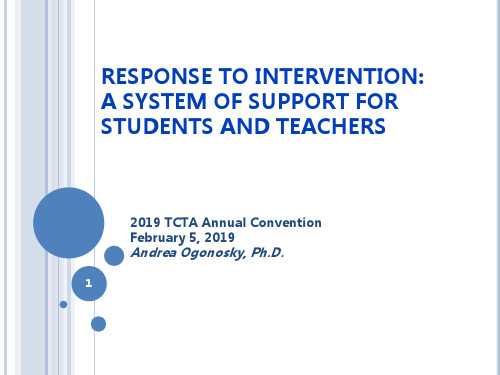
GUIDANCE DOCUMENT: DATA FOR SUFFICIENT
PROGRESS BASED ON RTI
Provision of targeted and supplemental services beyond what is provided for all students.
interventions Establishing a written plan of intervention which
includes detailing accountability Using progress monitoring (CBM) Comparing pre and post intervention da
§ 300.307 Specific learning disabilities.
(a) General. A State must adopt criteria for determining whether a
child has a specific learning disability…. the criteria adopted by the State— (2) May not require the use of a severe discrepancy between intellectual ability and achievement for determining whether a child has a specific learning disability as defined in § 300.8; [‘Discrepancy’ Model] (3) Must permit the use of a process that determines if the child responds to scientific, research-based intervention…