Energy-delay estimation technique for high-performance microprocessor VLSI adders
元器件考题分析

第一章何谓电容器? 描述电容器的主要参数有哪些?由介质隔开的两块金属极板构成的电子元件,广泛用于储能和传递信息。
1. 标称容量和允许误差2. 额定电压3. 绝缘电阻4. 电容器的损耗5. 其他参数(频率特性,温度系数,稳定性,可靠性)有哪些类型的电容器? 各有何特点?无机介质电容器:介电常数高,介质损耗角正切小,电容温度系数范围宽,可靠性高,寿命长.有机介质电容器:电容量范围大,绝缘电阻(时间常数)大,工作电压高(范围宽),温度系数互为偿,损耗角正切值小,适合自动化生产.热稳定性不如无机介质电容器,化学稳定性差, 易老化,具有不同程度的吸湿性电解电容器:比电容大,体积小、质量轻,有自愈特性双电层电容器(超级电容器)何谓电阻器? 可采用哪些主要的参数描述电阻器的性能优劣?具有吸收电能作用的电子元件,可使电路中各元件按需要分配电能,稳定和调节电路中的电流和电压。
1. 标称阻值和允许误差2. 额定功率3. 额定电压 4. 噪声有哪些主要类型的电阻器? 分别采用什么符号表示这些类型的电阻器?1.薄膜电阻器2.合金型电阻器3无帽结构电阻器4高频电阻器5小片式电阻器何谓电感器? 描述电感器的主要参数有哪些?凡能产生电感作用的元件统称为电感器1. 电感量及其误差2. 线圈的品质因数3.固有电容4.额定电流5.稳定性有哪些主要类型的电感器?固定电感器,平面电感器,天线线圈,震荡线圈,阻流圈简述矩形片式厚膜电阻器的电极结构特点及其设计依据.简述MLC的结构特点.试述片式铝电解电容器的结构特点和制作工艺过程,并简要说明各工序的作用.结构模型:电容器芯子/密封料/电极端子/封装树脂/矩形端子板工艺过程:腐蚀,形成,切断,柳接,卷绕,浸渍,装配,卷边,组合装配,特性测试,包装试述片式钽电解电容器的结构特点和制作工艺过程,并简要说明各工序的作用.结构模型:钽/氧化钽/二氧化锰/胶状石墨层/导电涂料层/导电性粘接剂层工艺过程:掺合,引线埋人加压成型,干燥烧结,阳极氧化,粘附二氧化碳,中间形成,石墨层,涂敷固化,连接,模塑封装,引线成型,老化,检验,包装比较片式铝电解电容器和钽电解电容器的结构差别.简述片式薄膜电容器的结构特点.带基薄膜—蒸镀电极一喷镀薄膜一外部电极有哪三种片式电感器?与目前使用的片式电感器相比,编织型片式电感器有何特点?简述框式电感器的制作过程.I.框式:结构最简单,但电感量较小II.螺旋式:能够获得较大的电感量,但要从中间抽头出来比较困难III.叉指式:电感量介于上述两者之间,但高频损耗大编织型片式电感器特点:单位体积电感量比目前已使用的片式电感器有所提高,但使用频率不高,Q值低,尚未进入实用阶段,但这种工艺技术新颖.第二章:1.何谓微电子学?一种将电子产品微小型化的技术,他的核心产品是集成电路。
运动估计与运动补偿
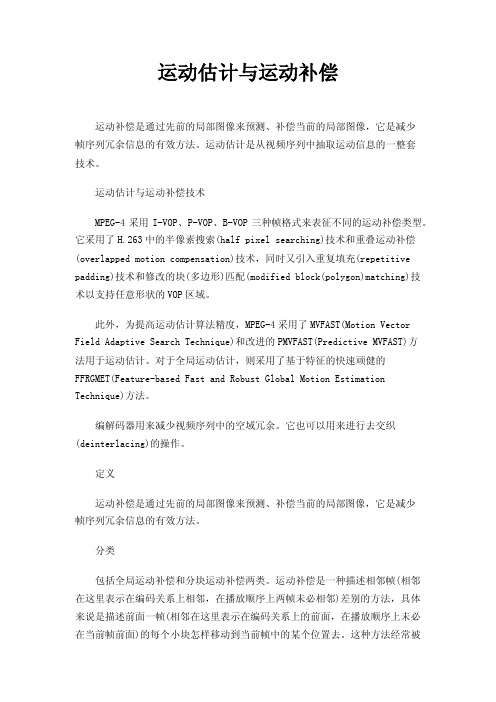
运动估计与运动补偿运动补偿是通过先前的局部图像来预测、补偿当前的局部图像,它是减少帧序列冗余信息的有效方法。
运动估计是从视频序列中抽取运动信息的一整套技术。
运动估计与运动补偿技术MPEG-4采用I-VOP、P-VOP、B-VOP三种帧格式来表征不同的运动补偿类型。
它采用了H.263中的半像素搜索(half pixel searching)技术和重叠运动补偿(overlapped motion compensation)技术,同时又引入重复填充(repetitive padding)技术和修改的块(多边形)匹配(modified block(polygon)matching)技术以支持任意形状的VOP区域。
此外,为提高运动估计算法精度,MPEG-4采用了MVFAST(Motion Vector Field Adaptive Search Technique)和改进的PMVFAST(Predictive MVFAST)方法用于运动估计。
对于全局运动估计,则采用了基于特征的快速顽健的FFRGMET(Feature-based Fast and Robust Global Motion Estimation Technique)方法。
编解码器用来减少视频序列中的空域冗余。
它也可以用来进行去交织(deinterlacing)的操作。
定义运动补偿是通过先前的局部图像来预测、补偿当前的局部图像,它是减少帧序列冗余信息的有效方法。
分类包括全局运动补偿和分块运动补偿两类。
运动补偿是一种描述相邻帧(相邻在这里表示在编码关系上相邻,在播放顺序上两帧未必相邻)差别的方法,具体来说是描述前面一帧(相邻在这里表示在编码关系上的前面,在播放顺序上未必在当前帧前面)的每个小块怎样移动到当前帧中的某个位置去。
这种方法经常被视频压缩/视频编解码器用来减少视频序列中的空域冗余。
它也可以用来进行去交织(deinterlacing)的操作。
电压闪变与波动的外文翻译
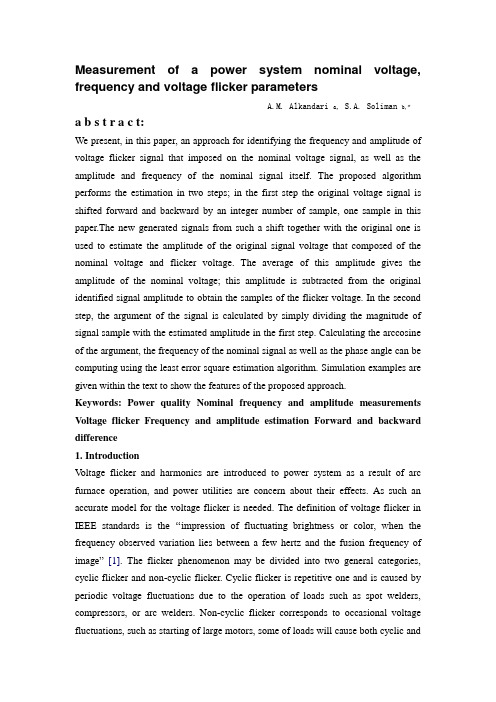
Measurement of a power system nominal voltage, frequency and voltage flicker parametersA.M. Alkandari a, S.A. Soliman b,*a b s t r a c t:We present, in this paper, an approach for identifying the frequency and amplitude of voltage flicker signal that imposed on the nominal voltage signal, as well as the amplitude and frequency of the nominal signal itself. The proposed algorithm performs the estimation in two steps; in the first step the original voltage signal is shifted forward and backward by an integer number of sample, one sample in this paper.The new generated signals from such a shift together with the original one is used to estimate the amplitude of the original signal voltage that composed of the nominal voltage and flicker voltage. The average of this amplitude gives the amplitude of the nominal voltage; this amplitude is subtracted from the original identified signal amplitude to obtain the samples of the flicker voltage. In the second step, the argument of the signal is calculated by simply dividing the magnitude of signal sample with the estimated amplitude in the first step. Calculating the arccosine of the argument, the frequency of the nominal signal as well as the phase angle can be computing using the least error square estimation algorithm. Simulation examples are given within the text to show the features of the proposed approach.Keywords: Power quality Nominal frequency and amplitude measurements Voltage flicker Frequency and amplitude estimation Forward and backward difference1.IntroductionV oltage flicker and harmonics are introduced to power system as a result of arc furnace operation, and power utilities are concern about their effects. As such an accurate model for the voltage flicker is needed. The definition of voltage flicker in IEEE standards is the ……impression of fluctuating brightness or color, when the frequency observed variation lies between a few hertz and the fusion frequency of image” [1]. The flicker phenomenon may be divided into two general categories, cyclic flicker and non-cyclic flicker. Cyclic flicker is repetitive one and is caused by periodic voltage fluctuations due to the operation of loads such as spot welders, compressors, or arc welders. Non-cyclic flicker corresponds to occasional voltage fluctuations, such as starting of large motors, some of loads will cause both cyclic andnon-cyclic flicker, such as arc furnace, welder, and ac choppers.Over the past three decades, many digital algorithms have been developed and tested to measure power system frequency and rate of change of frequency. Ref. [2] presents the application of the continuous Wavelet transform for power quality analysis. The transform appears to be reliable for detecting and measuring voltage sags, flicker and transients in power quality analysis. Ref. [3] pays attention to the fast Fourier transform and its pitfalls. A low pass.digital filter is used, and the effects of system voltage deviation on the voltage - flicker measurements by direct FFT are studied. The DC component leakage effect on the flicker components in the spectrum analysis of the effective value of the voltage and the windowing effect on the data acquisition of the voltage signal are discussed as well.A digital flicker meter is proposed in Ref. [6] based on forward and inverse FFT and on filtering, in the frequency domain, for the implementation of the functional blocks of simulation of lamp–eye–brain response. Refs. [5–7] propose a method based on Kalman filtering algorithms to measure the low frequency modulation of the 50/60 Hz signal. The method used, in these references, allows for random and deterministic variation of the modulation. The approach utilizes a combination of linear and non-linear Kalman filter modes.Ref. [8] presents a method for direct calculation of flicker level from digital measurements of voltage waveforms. The direct digital implementation uses Fast Fourier transform (FFT) as the first step in computation. A pruned FFT, customized for the flicker level computation, is also proposed. Presented in Ref. [9] is a static state estimation algorithm based on least absolute value error (LA V) for measurement of voltage flicker level. The waveform for the voltage signal is assumed to have, for simplicity, one flicker component. This algorithm estimates accurately the nominal voltage waveform and the voltage flicker component. An application of continuous wavelet transform (CWT) for analysis of voltage flicker-generated signals is proposed in Ref. [10] With the time-frequency localization characteristics embedded in the wavelets, the time and frequency information of a waveform can be integrally presentedRef. [11] presents an arc furnace model that implemented in the Simulink environment by using chaotic and deterministic elements. This model is obtained by solving the corresponding differential equation, which yields dynamic and multivalued v i characteristics of the arc furnace load. In order to evaluate the flicker in the simulated arc furnace voltage, the IEC flicker meter is implemented based on the IEC 1000-4-15 standard in Matlab environment.Ref. [12] presents an approach to estimate voltage flicker components magnitudes and frequencies, based on Lp norms (p = 1,2 and 1) and Taylor‟s‟ series linearization. It has been found that it is possible to design an Lp estimator to identify flicker frequency and amplitude from time series measurements. The Teager energy operator (TEO) and the Hilbert transform (HT) are introduced in Ref. [13] as effective approaches for tracking the voltage flicker levels. It has been found that TEO and HT are capable of tracking the amplitude variations of the voltage flicker and supply frequency in industrial systems with an average error 3%.Ref. [14] presents a control technique for flicker mitigation. This technique is based on the instantaneous tracking of the measured voltage envelope. The ADALINE (ADAptive LINear) neuron algorithm and the Recursive Least Square (RLS) algorithm are introduced for the flicker envelope tracking. In Ref. [15], an algorithm for tracking the voltage envelope based on calculating the energy operator of a sinusoidal waveform is presented. It is assumed that the frequency of the sinusoidal waveform is known and a lead-lag network with unity gain is used. Ref. [16] develops an enhanced method for estimating the voltage fluctuation (DV10) of the electric arc furnace (EAF). The method proposed considers the reactive power variation and also the active power variation in calculating DV10 value of ac and dc LEAFsControl and protection of power systems requires accurate measurement of system frequency. A system operates at nominal frequency, 50/60 Hz means a balance in the active power, i.e. The power generated equals the demand power plus losses. Imbalance in the active power causes the frequency to change. A frequency less than the nominal frequency means that the demand load plus losses are greater than the power generated, but a frequency greater than nominal frequency means that the system generation is greater than the load demand plus losses. As such, frequency can be used as a measure of system power balance.Ref. [17] presents a numerical differentiation-based algorithm for high-accuracy, wide-range frequency estimation of power systems. The signal, using this algorithm, includes up to 31st-order harmonic components. Ref. [18] presents a method for estimation of power frequency and its rate of change. The proposed methodaccommodates the inherent non-linearity of the frequency estimation problem. The estimator is based on a quadrature phase-look loop concept.An approach for designing a digital algorithm for local system frequency estimation is presented in Ref. [19]. The algorithm is derived using the maximum likelihood method. A recursive Newtontype algorithm suitable for various measurement applications in power system is developed in Ref. [20] and is used for power system frequency and spectra estimation. A precise digital algorithm based on discrete Fourier transforms (DFT) to estimate the frequency of a sinusoid with harmonics in real-time is proposed in Ref. [21]. This algorithm is called smart discrete Fourier transform (SDFT) that avoids the errors due to frequency deviation and keeps all the advantages of the DFT.Ref. [22] presents an algorithm for frequency estimation from distorted signals. The proposed algorithm is based on the extended complex Kalman filter, which uses discrete values of a three-phase voltage that are transformed into the well-known ab-transform Using such a transformation a non-linear state space formulation is obtained for the extended Kalman filter. This algorithm is iterative and complex and needs much computing time and uses the three-phase voltage measurements, to calculate the power system voltage frequency.Ref. [23] describes design, computational aspect, and implementation aspects of a digital signal processing technique for measuring the operating frequency of a power system. It is suggested this technique produces correct and noise-free estimates for near nominal, nominal and off-nominal frequencies in about 25 ms, and it requires modest computation. The proposed technique uses per-phase digitized voltage samples and applies orthogonal FIR digital filters with the least errors square (LES) algorithm to extract the system frequency.Ref. [24] presents an iterative technique for measuring power system frequency to a resolution of 0.01–0.02 Hz for near nominal, nominal and off-nominal frequencies in about 20 ms. The algorithm in this reference uses per-phase digitized voltage samples together with a FIR filter and the LES algorithm to extract iteratively the signal frequency.This algorithm has beneficial features including fixed sampling rate, fixed data window size and easy implementationRefs. [25,26] present a new pair of orthogonal filters for phasor computation; the technique proposed extracts accurately the fundamental component of fault voltageand current signal. Ref. [27] describes an algorithm for power system frequency estimation. The algorithm, applies orthogonal signal component obtained with use of two orthogonal FIR filters. The essential property of the algorithm proposed is outstanding immunity to both signals orthogonal component magnitudes and FIR filter gain variations. Again this algorithm uses the per-phase digitized voltage samples.Ref. [28] presents a method of measuring the power system frequency, based on digital filtering and Prony‟s estimation. The discrete Fourier transform with a variable data window is used to filter out the noise and harmonics associated with the signal. The results obtained using this algorithm are more accurate than when applying the method based on the measurement of angular velocity of the rotating voltage phasor. The response time of the proposed method equals to three to four periods of the fundamental components. This method uses also per phase digitized voltage samples to compute the system frequency from harmonics polluted voltage signal. Ref. [29] implements a digital technique for the evaluation of power system frequency. The algorithm is suitable for microprocessor implementation and uses only standard hardware. The algorithm works with any relative phase of the input signal and produces a new frequency estimate for every new input sample. This algorithm uses the orthogonal sine and cosine- filtering algorithm..A frequency relay, which is capable of under/over frequency and rate of change of frequency measurements using an instantaneous frequency-measuring algorithm, is presented in Ref. [30]. It has been shown that filtering the relay input signal could adversely affect the dynamic frequency evaluation response. Misleading frequency behavior is observed in this method, and an algorithm has been developed to improve this behavior. The under/over frequency function of the relay will cause it to operate within 30 ms.Digital state estimation is implemented to estimate the power system voltage amplitude and normal frequency and its rate of change. The techniques employed for static state estimation are least errors square technique [31–33], least absolute value technique [34–36]. While linear and non-linear Kalman filtering algorithms are implemented for tracking the system operating frequency, rate of change of frequency and power system voltage magnitude from a harmonic polluted environment of the system voltage at the relay location. Most of these techniques use the per-phasedigitized voltage samples, and assume that the three-phase voltages are balanced and contain the same noise and harmonics, which is not the case in real-time, especially in the distribution systems, where different single phase loads are supplied from different phases.An approach for identifying the frequency and amplitude of flicker signal that imposed on the nominal voltage signal, as well as the amplitude and frequency of the nominal signal itself is presented in this text. The proposed algorithm performs the estimation in two steps. While, in the first step the original signal is shifted forward and backward by an integer number of sample, one sample in this paper. The generated signals from such shift together with the original one are used to estimate the amplitude of the original voltage signal that composed of the nominal voltage and the flicker voltage, the average of this amplitude gives the amplitude of the nominal voltage. This amplitude is subtracted from the original identified amplitude to obtain the samples of the flicker voltage. In the second step, the argument of the signal is calculated by simply dividing the magnitude of signal sample with the estimated amplitude in step one. Computing the arccosine of the argument, the frequency of the nominal signal as well as the phase angle can be calculated using the least error square estimation algorithm. Simulation examples are given within the text to show the features of the proposed approach.2. Flicker voltage identificationGenerally speaking, the voltage during the time of flicker can be expressed as [2]:where AO is the amplitude of the nominal power system voltage, xO is the nominal power frequency, and /O is the nominal phase angle. Furthermore, Ai is the amplitude of the flicker voltage, xfi its frequency, and /fi its phase angle and M is the expected number of flicker voltage signal in the voltage waveform. This type of voltage signal is called amplitude modulated (AM) signal.2.1. Signal amplitude measurement::The first bracket in Eq. (1) is the amplitude of the signal, A(t) which can be written as:As such Eq. (1) can be rewritten asAssume that the signal is given forward and backward shift by an angle equals an integral number of the sampling angle. Then Eq. (3) can be written in the forward direction as:While for the backward direction, it can be written as:where h is the shift angle and is given byN is the number of samples required for the shift, fO is the signal frequency and m is the total number of samples over the data window size. Using Eqs. (4)–(6), one obtainsThe recursive equation for the amplitude A(k) is given by:Having identified the amplitude A(k), the amplitude of the nominal voltage signal of frequency x O can be calculated, just by taking the average over complete data window size as:Having identified the power signal amplitude AO, then the flicker voltage components can be determined by;This voltage flicker signal can be written as;where DT is the sampling time that is the reciprocal of the sampling frequency.2.2. Measurement of flicker frequencyWithout loss of generality, we assume that the voltage flicker signal has only one component given by, i = 1To determine the flicker amplitude Vf1(k) and the frequency xf1 from the available m samples, we may use the algorithm explained in Ref. [9]. The frequency is calculated fromWhile the amplitude can be calculated as:In the above equations v0 are the fist and second derivative of the flicker signal, they can be calculated, using the central forward and backward difference [9] as:2.3. Nominal voltage signal frequency and phase angleThe signal argument can be calculated fromwhere AR(k) is given byIn the above equation W0, u are the two parameters to be estimated from the available m samples of the argument AR(k). At least two samples are required for such a linear estimation.Eq. (17) can be written, for m samples, asIn vector form, Eq. (19) can be written as:where Z is m 1 measurements vector for the argument samples, H is m 2 measurements matrix the element of this matrix depend on the sampling time, sampling frequency, X is the 2 1 parameters vector to be estimated and f is m 1 error vector to be minimized. The minimum of f based on least error squares occurs when:The above two equations give directly the frequency and phase angle, in closed forms, for the signal under study. To have a practical approach those formulas should not be sensitive to noise and harmonics. One way to reduce those sensitivities is to use of least error squares algorithm, as we explained in Eq. (21), for the frequency estimation in the paper. In the following section we offer examples from the area of power system voltage flickers that can be considered as amplitude modulated signals.puter experimentsThe above algorithm is tested using amplitude modulated signal with one voltage flicker signal given by;The signal is sampled at 10000 Hz and is giving a forward shift and backward shift by one sample, h = 7.2 and 1000 samples are used. The power system voltage, 50 Hz signal, amplitude is estimated using the algorithm explained earlier, using Eqs. (8) and (9), and it has been found to that the proposed algorithm is succeeded in estimating this amplitude with great accuracy and is found be AO = 1.0. Fig. 1 gives the actualvoltage signal, the tracked signal and the voltage signal amplitude. Examining this figure reveals the following:The power voltage signal amplitude, 50 Hz, is almost 1 p.u., the average value of A(t), as that calculated using Eq. (9).The proposed technique tracked the actual signal exactly.The flicker signal frequency is estimated using 200 samples only with Eq. (13). Fig. 2 gives the estimated flicker voltage frequency at each sampling step. Examining this figure reveals that the proposed algorithm estimates the flicker frequency with great accuracy. The spikes, in these curves, are due to the value of the voltage flicker signal at this time of sampling which is very small, and looking to Eq. (13) one can notice that to calculate the frequency we divide by this value ceeded to estimate the flicker amplitude, except at the points of spikes, as we explained earlier in the frequency estimation.Another example has been solved, where the voltage signal has two flicker signals with different amplitude and frequency. The voltage signal in this case is given by The signal is sampled at 500 Hz and is giving a forward shift and backward shift by one sample, h = 7.2 and 500 samples are used. The voltage nominal amplitude is estimated using the technique explained earlier and has found to be one per unit, and the tracking voltage, using this technique, tracks the signal exactly as shown in Fig. 4.4.ConclusionsAn approach for identifying the frequency and amplitude of flicker signal that imposed on the nominal voltage signal, as well as the amplitude and frequency of the nominal signal itself is presented in this paper. The proposed algorithm performs the estimation in two steps; in the first step the original signal is shifted forward and backward by an integer number of sample, at least one sample. The new generated signals from such a shift together with the original one is used to estimate the amplitude of the original voltage signal that composed of the nominal voltage and theflicker voltage. The average of this amplitude gives the amplitude of the nominal voltage; this amplitude is subtracted from the original identified amplitude to obtain the samples of the flicker voltage. The frequency of the flicker voltage is calculated using the forward and backward difference for the first and second derivatives for the voltage flicker signal.In the second step, the argument of the signal is calculated by simply dividing the magnitude of signal sample with the estimated amplitude in step one. Calculating the arccosine of the argument, the frequency of the nominal signal as well as the phase angle can be calculated using the least error square estimation algorithm. Simulation examples are given. It has been shown that the proposed algorithm is succeeded in estimating the voltage flicker frequency and amplitude as well as the amplitude and frequency of the power voltage signal.The proposed algorithm can be used off-line as well as on-line. In the on-line mode we recommend the usage of a digital lead- lag circuit. Wile in the off-line mode; just shift the registration counter on sample in the backward direction and another on in the forward direction to obtain the required sample of the data window size.电力系统的额定电压,频率和电压闪变参数的测量摘要我们提出,在本文中,用于识别施加于标称电压信号电压闪变信号,以及标称信号本身的振幅和频率的频率和幅度的方法。
动力学参数辨识英文

动力学参数辨识英文Dynamics Parameter Identification.Dynamics parameter identification is a crucial processin various engineering applications, ranging from aerospace engineering to civil engineering. It involves theestimation of unknown parameters in dynamic systems basedon experimental data or system observations. These parameters are essential for understanding the behavior of the system and predicting its response to external excitations.The process of dynamics parameter identificationtypically involves several steps, including data collection, model development, parameter estimation, and validation. Each step requires careful consideration and may involve complex mathematical techniques and computational algorithms.1. Data Collection.The first step in dynamics parameter identification is to collect relevant data from the system. This data can be obtained through experiments, simulations, or real-time monitoring of the system's behavior. The quality and quantity of data collected directly impact the accuracy and reliability of the parameter identification results. Therefore, it is crucial to ensure that the data is representative of the system's dynamics and covers a wide range of operating conditions.2. Model Development.Once the data is collected, the next step is to develop a mathematical model that describes the system's dynamics. This model should capture the essential features of the system and be capable of predicting its response to external excitations. The model may be a simple linear model or a complex nonlinear model, depending on the nature of the system and the level of detail required for parameter identification.3. Parameter Estimation.The next step is to estimate the unknown parameters in the developed model using the collected data. This step involves solving a set of equations or optimization problems to find the parameter values that best fit the observed data. Various estimation techniques can be employed, including least squares methods, maximum likelihood estimation, and Bayesian inference. The choice of estimation technique depends on the nature of the model, the type of data available, and the desired accuracy of the parameter estimates.4. Validation.After estimating the parameters, it is crucial to validate the identified model against the original data or additional experimental data. This validation step helps assess the accuracy and reliability of the identified parameters and ensures that the model can indeed predict the system's behavior. If the validation results are satisfactory, the identified parameters can be used forfurther analysis, design, or control of the system.Challenges and Considerations in Dynamics Parameter Identification.Dynamics parameter identification, while a crucial process, also faces several challenges and considerations.1. Noise and Uncertainty.Real-world data often contains noise and uncertainty, which can affect the accuracy of parameter estimation. To address these issues, it is necessary to employ robust estimation techniques that can handle noisy and uncertain data effectively. This may involve the use of regularization techniques, noise models, or advanced optimization algorithms.2. Model Complexity.The complexity of the system model can significantly affect the parameter identification process. Simple modelsmay not capture all the essential features of the system, leading to inaccurate parameter estimates. On the other hand, overly complex models can make the estimation process computationally intractable. Therefore, it is crucial to strike a balance between model complexity and accuracy.3. Experimental Design.The design of experiments for data collection is also crucial for successful parameter identification. The experiments should be designed to excite the system in a way that allows for accurate estimation of the parameters. This may involve careful consideration of the excitation signals, measurement setup, and operating conditions.4. Identification of Multiple Parameters.In some cases, there may be multiple unknown parameters in the system model. Estimating these parameters simultaneously can be a challenging task, as it may require solving highly nonlinear and coupled equations. Advanced estimation techniques, such as multi-objective optimizationor genetic algorithms, may be required to handle such complex estimation problems.Conclusion.Dynamics parameter identification is a fundamental task in engineering analysis and design. It involves estimating unknown parameters in dynamic systems based on experimental data or system observations. The process involves data collection, model development, parameter estimation, and validation. Successful parameter identification requires careful consideration of various challenges and considerations, such as noise and uncertainty, model complexity, experimental design, and identification of multiple parameters. By addressing these challenges and employing appropriate estimation techniques, engineers can obtain accurate and reliable parameter estimates that support effective system analysis, design, and control.。
美国材料与试验协会(ASTM)氢能及燃料电池标准汇总

通过气相色谱/ 质谱法测定氢 燃料中总有机 卤化物,总非甲 烷碳氢化合物 和甲醛的标准
测试方法
ASTM
Standard Test Method
使用连续波腔
28 D7941/D7941M-1 for Hydrogen Purity
衰荡光谱分析
Standard Test Method for Visualizing Particulate Sizes and Morphology of
Particles Contained in Hydrogen Fuel by
Microscopy
通过显微镜观 察氢燃料中所 含颗粒的颗粒 尺寸和形态的 标准测试方法
理的标准规范
of Hydrogen Embrittlement
Standard Guide for
钢的后涂层处
ASTM
Post-Coating Treatments of 理以减少氢渗
4
B850-98(2015) Steel for Reducing the Risk 透风险的标准
of Hydrogen Embrittlement
的标准规范
27 ASTM D7892-15
Standard Test Method for Determination of Total Organic Halides, Total Non-Methane Hydrocarbons,
and Formaldehyde in Hydrogen Fuel by Gas
测定煤分析样
Determination of
品中碳,氢和氮
Carbon, Hydrogen and
声源定位中广义互相关时延估计算法的研究
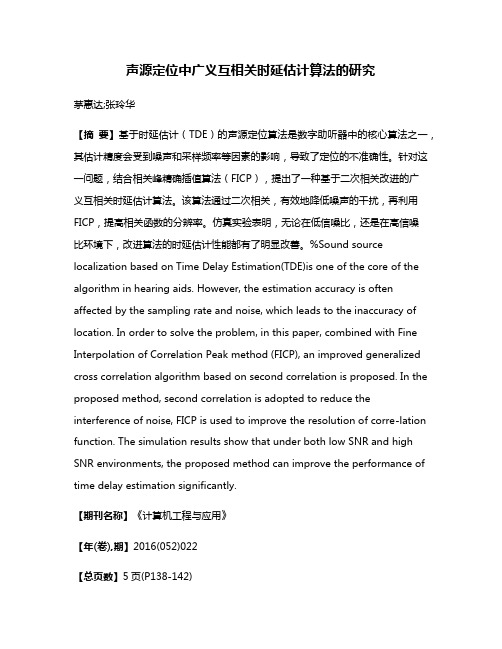
声源定位中广义互相关时延估计算法的研究茅惠达;张玲华【摘要】基于时延估计(TDE)的声源定位算法是数字助听器中的核心算法之一,其估计精度会受到噪声和采样频率等因素的影响,导致了定位的不准确性。
针对这一问题,结合相关峰精确插值算法(FICP),提出了一种基于二次相关改进的广义互相关时延估计算法。
该算法通过二次相关,有效地降低噪声的干扰,再利用FICP,提高相关函数的分辨率。
仿真实验表明,无论在低信噪比,还是在高信噪比环境下,改进算法的时延估计性能都有了明显改善。
%Sound source localization based on Time Delay Estimation(TDE)is one of the core of the algorithm in hearing aids. However, the estimation accuracy is often affected by the sampling rate and noise, which leads to the inaccuracy of location. In order to solve the problem, in this paper, combined with Fine Interpolation of Correlation Peak method (FICP), an improved generalized cross correlation algorithm based on second correlation is proposed. In the proposed method, second correlation is adopted to reduce the interference of noise, FICP is used to improve the resolution of corre-lation function. The simulation results show that under both low SNR and high SNR environments, the proposed method can improve the performance of time delay estimation significantly.【期刊名称】《计算机工程与应用》【年(卷),期】2016(052)022【总页数】5页(P138-142)【关键词】时延估计;广义互相关;二次相关;相关峰精确插值【作者】茅惠达;张玲华【作者单位】南京邮电大学通信与信息工程学院,南京 210003;南京邮电大学通信与信息工程学院,南京 210003【正文语种】中文【中图分类】TP391MAO Huida,ZHANG Linghua.Computer Engineering andApplications,2016,52(22):138-142.声源定位技术是数字助听器中的一项关键技术,它利用麦克风阵列对声音信号进行采集,并通过对采集到的信号进行处理从而得到声源位置。
IEEETransactionsonSmartGrid
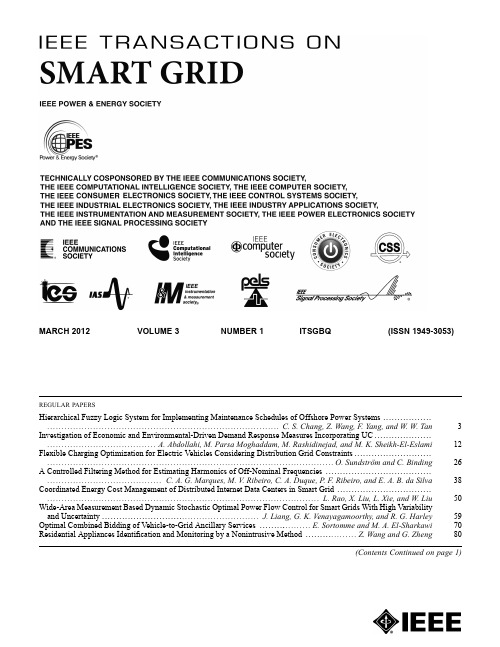
MARCH2012VOLUME3NUMBER1ITSGBQ(ISSN1949-3053)REGULAR PAPERSHierarchical Fuzzy Logic System for Implementing Maintenance Schedules of Offshore Power Systems................. .................................................................................C.S.Chang,Z.Wang,F.Yang,and W.W.Tan3 Investigation of Economic and Environmental-Driven Demand Response Measures Incorporating UC.................... ......................................A.Abdollahi,M.Parsa Moghaddam,M.Rashidinejad,and M.K.Sheikh-El-Eslami12 Flexible Charging Optimization for Electric Vehicles Considering Distribution Grid Constraints........................... ....................................................................................................O.Sundström and C.Binding26 A Controlled Filtering Method for Estimating Harmonics of Off-Nominal Frequencies..................................... ........................................ C.A.G.Marques,M.V.Ribeiro,C.A.Duque,P.F.Ribeiro,and E.A.B.da Silva38 Coordinated Energy Cost Management of Distributed Internet Data Centers in Smart Grid................................. ...............................................................................................L.Rao,X.Liu,L.Xie,and W.Liu50 Wide-Area Measurement Based Dynamic Stochastic Optimal Power Flow Control for Smart Grids With High Variabilityand Uncertainty.......................................................J.Liang,G.K.Venayagamoorthy,and R.G.Harley59 Optimal Combined Bidding of Vehicle-to-Grid Ancillary Services...................E.Sortomme and M.A.El-Sharkawi70 Residential Appliances Identification and Monitoring by a Nonintrusive Method..................Z.Wang and G.Zheng80(Contents Continued on page1)(Contents Continued from Front Cover)Modes of Operation and System-Level Control of Single-Phase Bidirectional PWM Converter for Microgrid Systems.. ...................................D.Dong,T.Thacker,I.Cvetkovic,R.Burgos,D.Boroyevich,F.F.Wang,and G.Skutt93 Generation-Load Mismatch Detection and Analysis...............................................R.M.Gardner and Y.Liu105 A Fault Location Technique for Two-Terminal Multisection Compound Transmission Lines Using Synchronized Phasor Measurements................................................................C.-W.Liu,T.-C.Lin,C.-S.Yu,and J.-Z.Yang113 Modeling and Control System Design of a Grid Connected VSC Considering the Effect of the Interface Transformer Type.................................................................................................H.Mahmood and J.Jiang122 Profile of Charging Load on the Grid Due to Plug-in Vehicles................S.Shahidinejad,S.Filizadeh,and E.Bibeau135 Sizing of Energy Storage for Microgrids...........................................S.X.Chen,H.B.Gooi,and M.Q.Wang142 On the Accuracy Versus Transparency Trade-Off of Data-Mining Models for Fast-Response PMU-Based Catastrophe Predictors.........................................................................I.Kamwa,S.R.Samantaray,and G.Joós152 Optimal Power Allocation Under Communication Network Externalities..................................................... ..........................................................................M.G.Kallitsis,G.Michailidis,and M.Devetsikiotis162 Optimal PMU Placement by an Equivalent Linear Formulation for Exhaustive Search...................................... ......................................................S.Azizi,A.S.Dobakhshari,S.A.Nezam Sarmadi,and A.M.Ranjbar174 Towards Optimal Electric Demand Management for Internet Data Centers................J.Li,Z.Li,K.Ren,and X.Liu183 High Level Event Ontology for Multiarea Power System....................Y.Pradeep,S.A.Khaparde,and R.K.Joshi193 Linear Active Stabilization of Converter-Dominated DC Microgrids..........A.A.A.Radwan and Y.A.-R.I.Mohamed203 Analysis and Methodology to Segregate Residential Electricity Consumption in Different Taxonomies................... ...............................................................................J.D.Hobby,A.Shoshitaishvili,and G.H.Tucci217 Quality of Optical Channels in Wireless SCADA for Offshore Wind Farms..........................................X.Liu225 Calculating Frequency at Loads in Simulations of Electro-Mechanical Transients........J.Nutaro and V.Protopopescu233 Smart“Stick-on”Sensors for the Smart Grid........................................R.Moghe,mbert,and D.Divan241 The Load as an Energy Asset in a Distributed DC SmartGrid Architecture....R.S.Balog,W.W.Weaver,and P.T.Krein253 A Network Decoupling Transform for Phasor Data Based V oltage Stability Analysis and Monitoring..................... .............................................................................W.Xu,I.R.Pordanjani,Y.Wang,and E.Vaahedi261 A Two Ways Communication-Based Distributed Control for V oltage Regulation in Smart Distribution Feeders.......... ........................................................................H.E.Z.Farag,E.F.El-Saadany,and R.Seethapathy271 Investigation of Domestic Load Control to Provide Primary Frequency Response Using Smart Meters.................... .................................................................................K.Samarakoon,J.Ekanayake,and N.Jenkins282 SPECIAL SECTION ON TRANSPORTATION ELECTRIFICATION AND VEHICLE-TO-GRID APPLICATIONS GUEST EDITORIALSpecial Section on Transportation Electrification and Vehicle-to-Grid Applications................................A.Emadi295SPECIAL SECTION PAPERSA Novel Integrated Magnetic Structure Based DC/DC Converter for Hybrid Battery/Ultracapacitor Energy Storage Systems.............................................................................................O.C.Onar and A.Khaligh296 Performance Evaluation of an EDA-Based Large-Scale Plug-In Hybrid Electric Vehicle Charging Algorithm............ ............................................................................................................W.Su and M.-Y.Chow308 Source-to-Wheel(STW)Analysis of Plug-in Hybrid Electric Vehicles....S.G.Wirasingha,R.Gremban,and A.Emadi316 Prototype Design and Controller Implementation for a Battery-Ultracapacitor Hybrid Electric Vehicle Energy Storage System..........................................................................................Z.Amjadi and S.S.Williamson332 PEV Charging Profile Prediction and Analysis Based on Vehicle Usage Data................................................ ......................................................................A.Ashtari,E.Bibeau,S.Shahidinejad,and T.Molinski341 Optimal Scheduling of Vehicle-to-Grid Energy and Ancillary Services..............E.Sortomme and M.A.El-Sharkawi351 Online Estimation of State of Charge in Li-Ion Batteries Using Impulse Response Concept................................ .......................................................................A.H.Ranjbar,A.Banaei,A.Khoobroo,and B.Fahimi360 Load Scheduling and Dispatch for Aggregators of Plug-In Electric Vehicles........D.Wu,D.C.Aliprantis,and L.Ying368 Catenary V oltage Support:Adopting Modern Locomotives With Active Line-Side Converters............................. ........................................................................................B.Bahrani,A.Rufer,and M.Aeberhard377 An Optimized EV Charging Model Considering TOU Price and SOC Curve................................................. ..................................................................Y.Cao,S.Tang,C.Li,P.Zhang,Y.Tan,Z.Zhang,and J.Li388(Contents Continued on page2)(Contents Continued from page1)Spatial and Temporal Model of Electric Vehicle Charging Demand...............................S.Bae and A.Kwasinski394 Study of PEV Charging on Residential Distribution Transformer Life......................................................... ......................................................................Q.Gong,S.Midlam-Mohler,V.Marano,and G.Rizzoni404 Evaluation and Efficiency Comparison of Front End AC-DC Plug-in Hybrid Charger Topologies.......................... .......................................................................F.Musavi,M.Edington,W.Eberle,and W.G.Dunford413 Design of a Novel Wavelet Based Transient Detection Unit for In-Vehicle Fault Determination and Hybrid Energy Storage Utilization.........................................................C.Sen,ama,T.Carciumaru,X.Lu,and N.C.Kar422 Vehicle-to-Aggregator Interaction Game..........................................C.Wu,H.Mohsenian-Rad,and J.Huang434 Optimized Bidding of a EV Aggregation Agent in the Electricity Market..................................................... .....................................................................R.J.Bessa,M.A.Matos,F.J.Soares,and J.A.P.Lopes443 Coordinating Vehicle-to-Grid Services With Energy Trading..............................A.T.Al-Awami and E.Sortomme453 Energy Management Optimization in a Battery/Supercapacitor Hybrid Energy Storage System............................ ...........................................................................................M.-E.Choi,S.-W.Kim,and S.-W.Seo463 BEVs/PHEVs as Dispersed Energy Storage for V2B Uses in the Smart Grid......C.Pang,P.Dutta,and M.Kezunovic473 An Evaluation of State-of-Charge Limitations and Actuation Signal Energy Content on Plug-in Hybrid Electric Vehicle, Vehicle-to-Grid Reliability,and Economics...................................C.Quinn,D.Zimmerle,and T.H.Bradley483 Modeling of Plug-in Hybrid Electric Vehicle Charging Demand in Probabilistic Power Flow Calculations................ ............................................................................................................G.Li and X.-P.Zhang492 The Evolution of Plug-In Electric Vehicle-Grid Interactions......................................D.P.Tuttle and R.Baldick500 Methodology to Analyze the Economic Effects of Electric Cars as Energy Storages......................................... ssila,J.Haakana,V.Tikka,and J.Partanen506 An Economic Analysis of Used Electric Vehicle Batteries Integrated Into Commercial Building Microgrids............. .............................................S.Beer,T.Gómez,D.Dallinger,I.Momber,C.Marnay,M.Stadler,and i517 Transport-Based Load Modeling and Sliding Mode Control of Plug-In Electric Vehicles for Robust Renewable Power Tracking............................................................................................S.Bashash and H.K.Fathy526 Intelligent Energy Resource Management Considering Vehicle-to-Grid:A Simulated Annealing Approach.............. ..........................................................................T.Sousa,H.Morais,Z.Vale,P.Faria,and J.Soares535 Grid Integration of Electric Vehicles and Demand Response With Customer Choice........................................ ...............................................................................S.Shao,M.Pipattanasomporn,and S.Rahman543 Analysis of the Filters Installed in the Interconnection Points Between Different Railway Supply Systems............... ......................................................................................................M.Brenna and F.Foiadelli551 Autonomous Distributed V2G(Vehicle-to-Grid)Satisfying Scheduled Charging............................................. ............................................Y.Ota,H.Taniguchi,T.Nakajima,K.M.Liyanage,J.Baba,and A.Yokoyama559 Implementation of Vehicle to Grid Infrastructure Using Fuzzy Logic Controller.........M.Singh,P.Kumar,and I.Kar565。
声源定位相关算法
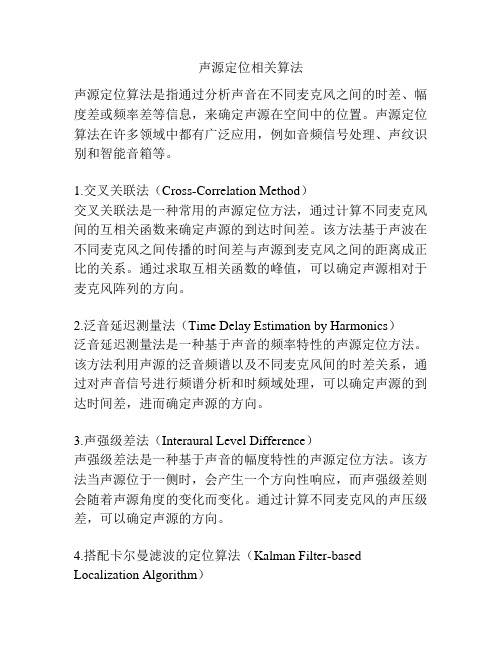
声源定位相关算法声源定位算法是指通过分析声音在不同麦克风之间的时差、幅度差或频率差等信息,来确定声源在空间中的位置。
声源定位算法在许多领域中都有广泛应用,例如音频信号处理、声纹识别和智能音箱等。
1.交叉关联法(Cross-Correlation Method)交叉关联法是一种常用的声源定位方法,通过计算不同麦克风间的互相关函数来确定声源的到达时间差。
该方法基于声波在不同麦克风之间传播的时间差与声源到麦克风之间的距离成正比的关系。
通过求取互相关函数的峰值,可以确定声源相对于麦克风阵列的方向。
2.泛音延迟测量法(Time Delay Estimation by Harmonics)泛音延迟测量法是一种基于声音的频率特性的声源定位方法。
该方法利用声源的泛音频谱以及不同麦克风间的时差关系,通过对声音信号进行频谱分析和时频域处理,可以确定声源的到达时间差,进而确定声源的方向。
3.声强级差法(Interaural Level Difference)声强级差法是一种基于声音的幅度特性的声源定位方法。
该方法当声源位于一侧时,会产生一个方向性响应,而声强级差则会随着声源角度的变化而变化。
通过计算不同麦克风的声压级差,可以确定声源的方向。
4.搭配卡尔曼滤波的定位算法(Kalman Filter-based Localization Algorithm)搭配卡尔曼滤波的定位算法是一种基于状态估计的声源定位方法,可以用来估计声源的位置和速度。
该方法结合了声音传播模型和测量模型,通过初始位置和速度的估计以及麦克风阵列的测量信息,通过递推的方式对声源的位置和速度进行估计。
5.分束技术(Beamforming Technique)分束技术是一种基于声音波前的声源定位方法。
该方法利用多个麦克风的信号相位差,通过调整麦克风阵列的权重系数,可以实现声源的定向接收和抑制噪声的目的。
分束技术可以用于提高声源定位的准确性和鲁棒性。
除了上述几种常见的声源定位算法,还有一些其他的方法和改进技术,例如多麦克风阵列的布置优化、噪声环境下的声源定位方法、深度学习在声源定位中的应用等。
气科院大气物理面试英语专业词汇[1]
![气科院大气物理面试英语专业词汇[1]](https://img.taocdn.com/s3/m/dc738b36dcccda38376baf1ffc4ffe473368fdf6.png)
大气科学系微机应用基础Primer of microcomputer applicationFORTRAN77程序设计FORTRAN77 Program Design大气科学概论An Introduction to Atmospheric Science大气探测学基础Atmospheric Sounding流体力学Fluid Dynamics天气学Synoptic Meteorology天气分析预报实验Forecast and Synoptic analysis生产实习Daily weather forecasting现代气候学基础An introduction to modern climatology卫星气象学Satellite meteorologyC语言程序设计 C Programming大气探测实验Experiment on Atmospheric Detective Technique云雾物理学Physics of Clouds and fogs动力气象学Dynamic Meteorology计算方法Calculation Method诊断分析Diagnostic Analysis中尺度气象学Meso-Microscale Synoptic Meteorology边界层气象学Boundary Layer Meteorology雷达气象学Radar Meteorology数值天气预报Numerical Weather Prediction气象统计预报Meteorological Statical Prediction大气科学中的数学方法Mathematical Methods in Atmospheric Sciences专题讲座Seminar专业英语English for Meteorological Field of Study计算机图形基础Basic of computer graphics气象业务自动化Automatic Weather Service空气污染预测与防治Prediction and Control for Air Pollution现代大气探测Advanced Atmospheric Sounding数字电子技术基础Basic of Digital Electronic Techniqul大气遥感Remote Sensing of Atmosphere模拟电子技术基础Analog Electron Technical Base大气化学Atmospheric Chemistry航空气象学Areameteorology计算机程序设计Computer Program Design数值预报模式与数值模拟Numerical Model and Numerical Simulation接口技术在大气科学中的应用Technology of Interface in Atmosphere Sciences Application海洋气象学Oceanic Meteorology现代实时天气预报技术(MICAPS系统)Advanced Short-range Weather Forecasting Technique(MICAPS system)1) atmospheric precipitation大气降水2) atmosphere science大气科学3) atmosphere大气1.The monitoring and study of atmosphere characteristics in near space as an environment forspace weapon equipments and system have been regarded more important for battle support.随着临近空间飞行器的不断发展和运用,作为武器装备和系统环境的临近空间大气特性成为作战保障的重要条件。
局部心肌功能评价的新方法-斑点追踪技术和速度向量显像
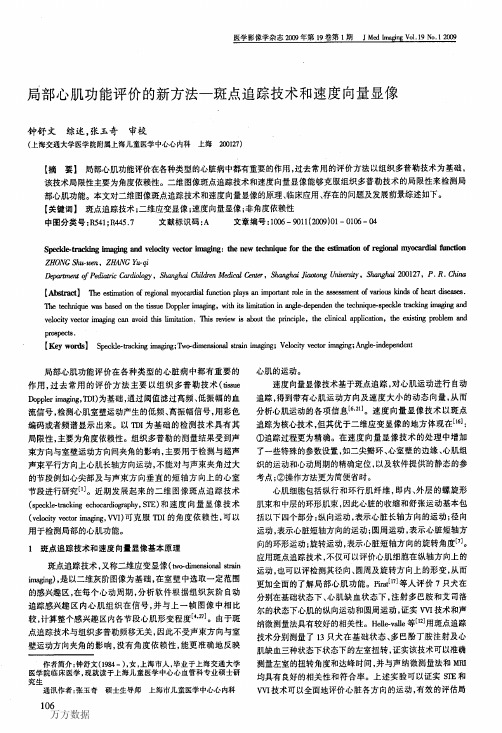
值差异有统计学意义。wI的优势体现在除了纵向,还可以
有效的评价径向和环向的心肌运动的协调性【21,22 J。心肌病 伴发的收缩功能异常中最普遍是心肌机械运动的不协调,
Friedberg等¨9J人用、M来检测正常对照组和心肌病患儿心
肌运动的协调性,结果发现两组左室室壁运动延迟时间有明 显差异(分别为51±41ms、20±20ms,P<0.01)。 心脏再同步化治疗(cardiac
2.1评价局部心肌节段的功能 局部心肌节段功能是一直是众多学者研究的热点。根 据美国超声心动图学会的推荐【25J,二维超声心动图将左室
分为16个节段,评价左心室内膜运动和左室容积时将左室
分为17个节段。vⅥ软件进行处理时,进入SR界面,将取样
点置于局部心肌的各个节段,可以得到相应的速度,应变和
应变率曲线。右心室几何结构的不规则,因此一般的超声技 术手段难以对其进行测量,在斑点追踪技术出现前,主要是 TDI对其进行评估【2,5】。斑点追踪技术不依赖角度。不仅可 以对左室的长轴和短轴【1'7J进行评估,而且可以有效的评价
左室基底部顺时针旋转,心尖部逆时针旋转,扭转定义为心
尖部相对于基底部的旋转,即心尖部和基底部的旋转角度绝 对值之和。左室的解旋主要发生在等容舒张期,其迅速的弹 性回缩释放了扭转时储存的弹性势能,使舒张期心室内的压 力梯度和心房心室问的压力梯度增加,造成抽吸作用,从而 引起左室早期充盈【13 J。 超声二维斑点追踪技术可以分别测量扭心尖部和基底 部旋转的角度和速度。采集图像,进入二维应变工作系统 后,可根据左室基底部和心尖部短轴平面的旋转角度一时问 和旋转速度时间曲线,根据其平均值绘制左室整体扭转角度 一时间、扭转速度一时间曲线,测定扭转峰值、等容舒张末 期,即二尖瓣开放时扭转角度、扭转速度峰值和达峰时间。
(项目管理)项目管理计划模板(英文)

<Project Name>Project Management PlanVersion <x.x> [Note: The following template is provided for use in Xavor projects. Text enclosed in square brackets and displayed in blue italics (style=InfoBlue) is included to provide guidance to the author and should be deleted before publishing the document.]Revision History[For every revision of this document, provide the revision history that should include the date of revision, version number, description of the changes in the document, and author of the document for that particular version.]Distribution List[State the persons/teams/groups to whom this document should be distributed whenever the document is revised. Also state the name of their parent organization.]Table of Contents1.Introduction 51.1Purpose 51.2Scope 51.3Definitions, Acronyms and Abbreviations 51.4References 51.5Overview 52.Project Overview 62.1Project Name, Code and Leader 62.2Project Purpose, Scope and Objectives 62.3Assumptions and Constraints 62.3.1Critical Assumptions and Constraints 62.3.2Non-Critical Assumptions and Constraints 62.4Project Milestones 62.5Project Deliverables 62.6Tailoring Guidelines 72.7Software Development Life Cycle 73.Project Organization 83.1Organizational Structure 83.2External Interfaces 103.3Roles and Responsibilities 103.3.1<Organizational Unit Name> 104.Management Process 114.1Work Breakdown Structure (WBS) 114.2Project Estimates 114.2.1Estimation Technique 114.2.2Size 114.2.3Effort 114.3Project Schedule 114.3.1Pre-Development Schedule 114.3.2Development Schedule 114.4Project Phases, Iterations and Releases 114.4.1Project Phases 114.4.2Project Iterations 114.4.3Releases 114.5Project Resourcing 114.5.1Staffing 114.5.2Resource Acquisition 124.5.3Training 124.6Project Budget 124.7Project Monitoring and Control 124.7.1Schedule Control 124.7.2Budget Control 124.7.3Measurements 124.8Risk Management Plan 124.9Project Closure 125.Technical Process Plans 135.1User Experience Design 135.2Requirements Management 135.3Analysis and Design 135.4Development Plan 135.5Peer Review Plan 135.6Project Maintenance 135.7Test Plan 135.8Tools, Techniques and Standards 135.8.1Tools 135.8.2Techniques and Standards 145.9Infrastructure 145.10Facilities 145.11Security Plan 146.Supporting Process Plans 156.1Configuration Management Plan 156.2Documentation 156.3Software Quality Assurance Plan 156.4Intergroup Coordination 156.5Communication 156.6Problem Resolution 156.7Subcontractor Management 157.Additional plans 168.Appendices 16Project Management Plan1. Introduction[The introduction of the Project Management Plan should provide an overview of the entiredocument. It should include the purpose, scope, definitions, acronyms, abbreviations, references and overview of this Project Management Plan.]1.1 Purpose[Specify the purpose of this Project Management Plan.]1.2 Scope[A brief description of the scope of this Project Management Plan; what Project(s) it isassociated with, and anything else that is affected or influenced by this document.]1.3 Definitions, Acronyms and Abbreviations[This subsection should provide the definitions of all terms, acronyms, and abbreviationsrequired to interpret properly the Project Management Plan. This information may be provided by reference to the project Glossary.]1.4 References[This subsection should provide a complete list of all documents referenced elsewhere in theProject Management Plan. Each document should be identified by title, report number (ifapplicable), date, and publishing organization. Specify the sources from which the referencescan be obtained. This information may be provided by reference to an appendix or to anotherdocument. For the Project Management Plan, the list of referenced artifacts may include:•Risk Management Plan•User Interfaces Guidelines•Configuration Management Plan•Software Quality Assurance Plan, etc.]1.5 Overview[This subsection should describe what the rest of the Project Management Plan contains andexplain how the document is organized.]2. Project Overview2.1 Project Name, Code and Leader[Specify the project name, project code and project leader (project manager).]Project Name: <Project Name>Project Code: <xxx-xxx>Project Leader: <Name>2.2 Project Purpose, Scope and Objectives[A brief description of the purpose and objectives of this project, and a brief description of whatdeliverables the project is expected to deliver.]2.3 Assumptions and Constraints[A list of assumptions that this plan is based on, and any constraints (e.g. budget, staff, equipment,schedule, etc.) that apply to the project. Make a distinction between critical and non-criticalfactors.]2.3.1 Critical Assumptions and Constraints[State the critical assumptions and constraints affecting the project.]2.3.2 Non-Critical Assumptions and Constraints[State the non-critical assumptions and constraints affecting the project.]2.4 Project Milestones[Tabular list of major milestones to be achieved during the project, with target dates.]2.5 Project Deliverables[Tabular list of the artifacts to be created during the project, with target delivery dates.]2.6 Tailoring Guidelines[Specify the tailoring guidelines for the project.]2.7 Software Development Life Cycle[Specify the Software Development Life Cycle that is to be followed in the project.]3. Project Organization3.1 Organizational Structure[Describe the organizational structure of the project team, including management and otherreview authorities. This should include identification of all project organizational units and adescription of their function and responsibility. A diagram of the organizational structure should also be attached for further illustration.Examples of project organizational units are:•Project Implementation Committee•Project Steering Committee•Project Management Team•Architecture Group•User Experience Design Team•Requirements Team•Analysis and Design Team•Implementation Group•Development Team•Database Management Team•Testing Team•Infrastructure Team•Configuration Management Team•Software Quality Assurance Team, etc.]3.2 External Interfaces[Describe how the project interfaces with external groups. For each external group, identify the internal/external contact names.]3.3 Roles and Responsibilities[Specify the roles, responsibilities and role holders within each organizational unit of the project.] 3.3.1 <Organizational Unit Name>4. Management Process4.1 Work Breakdown Structure (WBS)[List the activities necessary for completing the project.]4.2 Project Estimates4.2.1 Estimation Technique[Specify the estimation method and the reason for its choice. Provide the estimated cost as well as the basis for those estimates, and the points/circumstances in the project when re-estimation will occur.]4.2.2 Size[State the size of each activity as calculated according to the estimation technique. Units of size may be in LOC, FP, etc.]4.2.3 Effort[Specify the amount of effort required to perform each activity on the basis of the size estimation.Units may be man-hours, man-days, etc.]4.3 Project Schedule[Diagrams/tables showing target dates for completion of iterations and phases, release points,demos, and other milestones. Critical path must be specified. Usually enclosed by reference to MS Project file.]4.3.1 Pre-Development Schedule[This schedule will cater for project planning, requirements, analysis and design activities.]4.3.2 Development Schedule[This schedule will cater coding, testing and deployment activities.]4.4 Project Phases, Iterations and Releases4.4.1 Project Phases[Identify phases and major milestones with their achievement criteria.]4.4.2 Project Iterations[Specify the number of iterations and list the objectives to be accomplished for each of theiterations.]4.4.3 Releases[Brief descriptions of each software release, whether demo, beta, etc.]4.5 Project Resourcing4.5.1 Staffing[Identify here the numbers and type of staff required (including and special skills or experience), scheduled by project phase or iteration. State what resources are critical.]4.5.2 Resource Acquisition[Describe how you will approach finding and acquiring the staff needed for the project.]4.5.3 Training[List any special training project team members will require, with target dates for when thistraining should be completed.]4.6 Project Budget[Allocation of costs against the WBS and the project phases.]4.7 Project Monitoring and Control4.7.1 Schedule Control[Describes the approach to be taken to monitor progress against the planned schedule and how to take corrective action when required.]4.7.2 Budget Control[Describes the approach to be taken to monitor spending against the project budget and how to take corrective action when required.]4.7.3 Measurements[Describe the types of measurements to be taken, their frequency, and responsibleworkers/entities for this purpose.]4.8 Risk Management Plan[Enclosed by reference]4.9 Project Closure[Describe the activities for the orderly completion of the project, including staff reassignment, archiving of project materials, post-mortem debriefings and reports etc.]5. Technical Process Plans5.1 User Experience Design[Describe the approach that will be adopted with details of processes, procedures, and guidelines to be followed.]5.2 Requirements[Describe the approach that will be adopted with details of processes, procedures, and guidelines to be followed.]5.3 Analysis and Design[Describe the approach that will be adopted with details of processes, procedures, and guidelines to be followed.]5.4 Development Plan[Enclosed by reference]5.5 Peer Review Plan[Specify the work products to be peer reviewed, type of peer review, their frequency, etc.]5.6 Maintenance[Describe details of any software maintenance for the warranty period of the project.]5.7 Test Plan[Enclosed by reference]5.8 Tools, Techniques and Standards5.8.1 Tools5.8.1.1 Project Management Tools[Specify the project management tools that are to be used in the project and the reasons for their selection. Examples of areas to be covered are project planning, project scheduling, projectmonitoring, status reporting, measurements, etc. Examples of these tools are MS Project, etc.]5.8.1.2 Requirements Management Tools[Specify the requirements management tools that are to be used in the project and the reasons for their selection. Examples of areas to be covered are requirements gathering, requirement issueresolution, requirement change management, measurements, etc. Examples of these tools areRational Requisite Pro, EINS, etc.]5.8.1.3 System Analysis & Design Tools[Specify the system analysis and design tools that are to be used in the project and the reasonsfor their selection. Examples of tools in this area are Visio, Rational Rose, Power Designer etc.]5.8.1.4 Languages[Specify the languages that are to be used for software development in the project and thereasons for their selection. Examples of languages are HTML, Java, etc.]5.8.1.5 User-Interface Development Tools[Specify the tools that are to be used for UI development in the project and the reasons for their selection. Examples of these tools can be Dreamweaver, Flash, etc.]5.8.1.6 Database Management System Software[Specify the database management system software that is to be used in the project and thereasons for their selection. Examples of these tools are Oracle, SQL Server, etc.]5.8.1.7 Third Party Software[Specify any third party software that is to be used in the project and the reasons for theirselection. Examples are Inktomi, Infranet, etc.]5.8.1.8 Software Testing Tools[Specify the software testing tools that are to be used in the project and the reasons for theirselection. Examples of these tools are WinRunner, LoadRunner, etc.]5.8.1.9 Defect and Change Management Tools[Specify the defect and change management tools that are to be used in the project and thereasons for their selection. Examples of these tools are ClearQuest, etc.]5.8.1.10 Configuration Management Tools[Specify the configuration management tools that are to be used in the project and the reasonsfor their selection. Examples of these tools are ClearCase, etc.]5.8.1.11 Integrated Development Environment[Specify the operating systems (platforms), web servers, application servers, development servers that are to be used in the project and the reasons for their selection. Examples of these tools are Sun Solaris, iPlanet, JBuilder, WebSphere, etc.]5.8.2 Techniques and Standards[Lists the documented project technical standards etc by reference. Examples may be:User-Interface GuidelinesProgramming GuidelinesTest Guidelines, etc.]5.9 Infrastructure[Specify hardware, network connectivity, bandwidth, etc., required in this project. Make a clear distinction about what factors are critical.]5.10 Facilities[Describe the facilities required for the execution of the project. This will cover physicalworkspace, buildings, etc.]5.11 Security Plan[List down the security consideration e.g. of security can be operating system, access controls to site/product, physical security considerations.]6. Supporting Process Plans6.1 Configuration Management Plan[Enclosed by reference]6.2 Documentation[Specify the documents that will be produced in the project, what document templates will beused, and any other information pertaining to documentation.]6.3 Software Quality Assurance Plan[Enclosed by reference]6.4 Intergroup Coordination[Describe how different project groups will communicate with one another; specify dependencies, and commitments.]6.5 Communication[Specify how various workers/units/entities, both within and outside the project team, willcommunicate with each other.]6.6 Problem Resolution[Describe the approach for resolving issues in the project, escalation procedures, etc.]6.7 Subcontractor Management[If subcontractors are involved in the project give details of what kind of contractors are required for various tasks, the duration for which they are required and how they will be managed.]7. Additional plans[Additional plans if required by contract or regulations.] 8. Appendices[Attach any supplementary information.]。
电池soc-ocv的测定方法英文
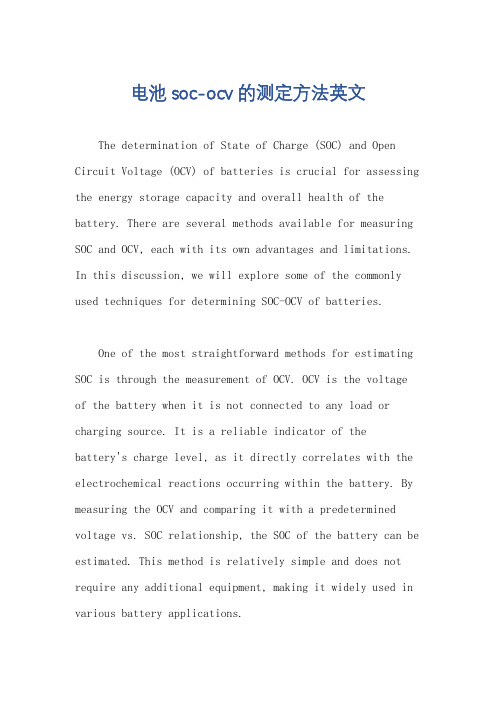
电池soc-ocv的测定方法英文The determination of State of Charge (SOC) and Open Circuit Voltage (OCV) of batteries is crucial for assessing the energy storage capacity and overall health of the battery. There are several methods available for measuring SOC and OCV, each with its own advantages and limitations. In this discussion, we will explore some of the commonly used techniques for determining SOC-OCV of batteries.One of the most straightforward methods for estimating SOC is through the measurement of OCV. OCV is the voltage of the battery when it is not connected to any load or charging source. It is a reliable indicator of thebattery's charge level, as it directly correlates with the electrochemical reactions occurring within the battery. By measuring the OCV and comparing it with a predetermined voltage vs. SOC relationship, the SOC of the battery can be estimated. This method is relatively simple and does not require any additional equipment, making it widely used in various battery applications.However, the OCV method alone may not provide accurate SOC estimation due to the influence of factors such as temperature, aging, and load history. To overcome these limitations, more sophisticated methods are employed. One such approach is the coulomb counting method, which determines SOC by integrating the current flowing in and out of the battery over time. By measuring the charge or discharge current accurately and accounting for losses, the SOC can be calculated. This method provides more accurate results compared to the OCV method, but it requires precise current measurement and can be affected by errors in the current integration.Another commonly used technique for SOC-OCV determination is based on impedance spectroscopy. This method involves applying a small AC signal to the battery and measuring the impedance response at different frequencies. The impedance of the battery is influenced by various factors, including SOC, electrode kinetics, and electrolyte properties. By analyzing the impedance data using mathematical models or equivalent circuit models, theSOC can be estimated. Impedance spectroscopy offers a non-destructive and real-time measurement of SOC, making it suitable for online monitoring of battery systems.In addition to the above methods, there are other indirect techniques for SOC-OCV determination. These include using voltage response during load changes, analyzing the battery's internal resistance, and employing advanced algorithms based on machine learning andartificial intelligence. These methods utilize the dynamic behavior of the battery under different operating conditions to estimate SOC and OCV accurately. However, they may require more complex instrumentation and computational algorithms, making them less commonly used in practical applications.It is worth mentioning that the accuracy of SOC-OCV determination depends on several factors, including battery chemistry, temperature, and aging effects. Therefore, it is essential to calibrate and validate the chosen method for specific battery types and operating conditions. Additionally, combining multiple methods or using hybridapproaches can enhance the accuracy and reliability of SOC-OCV estimation.In conclusion, the determination of battery SOC-OCV is crucial for assessing the energy storage capacity and overall health of the battery. Various methods, such as OCV measurement, coulomb counting, impedance spectroscopy, and indirect techniques, can be employed for SOC-OCV determination. Each method has its own advantages and limitations, and the choice depends on factors such as accuracy requirements, cost, and practicality. Calibration and validation of the chosen method are essential to ensure accurate SOC-OCV estimation.。
基于ANFIS和减法聚类的动力电池放电峰值功率预测

基于ANFIS和减法聚类的动力电池放电峰值功率预测孙丙香;高科;姜久春;罗敏;何婷婷;郑方丹;郭宏榆【摘要】动力电池的短时峰值功率预测对于实际使用来说至关重要.本文采用基于一阶Sugeno模糊推理系统的自适应神经模糊推理系统(ANFIS)模型估计放电峰值功率.选取温度、SOC和欧姆内阻为模型输入量,10s脉冲放电峰值功率为输出变量.基于实测和曲线拟合相结合的方法得到训练数据组,采用305组数据组模型进行训练,采用网格生成法和减法聚类法分别生成模糊集合,并采用单一BP神经网络方法和混合训练方法分别进行模型训练.发现采用减法聚类法生成模糊结构,能大幅减少模糊规则的数目,并提高收敛速度,在满足预测准确度的前提下降低了模型的复杂程度;采用混合训练方法进行网络学习能够加强模型的收敛能力并克服单一BP算法的局部最优问题,准确度更高.最后,采用125组数据组模型进行验证,预测误差在10%以内,基于ANFIS的模型能够很好地估计电池的脉冲峰值功率.【期刊名称】《电工技术学报》【年(卷),期】2015(030)004【总页数】9页(P272-280)【关键词】动力电池;峰值功率;ANFIS;减法聚类;混合训练【作者】孙丙香;高科;姜久春;罗敏;何婷婷;郑方丹;郭宏榆【作者单位】北京交通大学国家能源主动配电网技术研发中心北京 100044;北京交通大学国家能源主动配电网技术研发中心北京 100044;北京交通大学国家能源主动配电网技术研发中心北京 100044;广东电网公司电力科学研究院广州510080;北京交通大学国家能源主动配电网技术研发中心北京 100044;北京交通大学国家能源主动配电网技术研发中心北京 100044;惠州市亿能电子有限公司惠州 516006【正文语种】中文【中图分类】TM9111 引言能源危机和环境保护的双重压力,助推了电动汽车和电力储能的大力发展。
动力电池作为主要能量源,其短时峰值功率预测的准确性直接关系到使用中的控制策略和可靠性。
自适应阵列抗干扰性能的解析定量分析
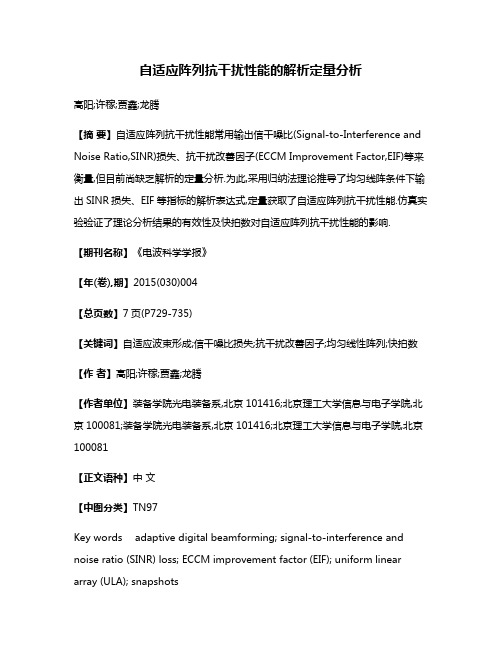
自适应阵列抗干扰性能的解析定量分析高阳;许稼;贾鑫;龙腾【摘要】自适应阵列抗干扰性能常用输出信干噪比(Signal-to-Interference and Noise Ratio,SINR)损失、抗干扰改善因子(ECCM Improvement Factor,EIF)等来衡量,但目前尚缺乏解析的定量分析.为此,采用归纳法理论推导了均匀线阵条件下输出SINR损失、EIF等指标的解析表达式,定量获取了自适应阵列抗干扰性能.仿真实验验证了理论分析结果的有效性及快拍数对自适应阵列抗干扰性能的影响.【期刊名称】《电波科学学报》【年(卷),期】2015(030)004【总页数】7页(P729-735)【关键词】自适应波束形成;信干噪比损失;抗干扰改善因子;均匀线性阵列;快拍数【作者】高阳;许稼;贾鑫;龙腾【作者单位】装备学院光电装备系,北京101416;北京理工大学信息与电子学院,北京100081;装备学院光电装备系,北京101416;北京理工大学信息与电子学院,北京100081【正文语种】中文【中图分类】TN97Key words adaptive digital beamforming; signal-to-interference and noise ratio (SINR) loss; ECCM improvement factor (EIF); uniform linear array (ULA); snapshots联系人:许稼E-mail:*************.cn种自适应算法,得到优化的天线响应[2-6].自适应阵列处理能够显著提高现代信息系统在复杂信号环境中的适应能力[1],被广泛应用于雷达、声呐、导航等诸多领域. 对该方面的研究最早开始于20世纪50年代末,Van Atta等提出了自适应天线的概念,Howells在此基础上提出了最初的旁瓣对消器形式. 随后,Applebaum将二元阵旁瓣对消器推广至多旁瓣对消器. 在此基础上,现代自适应阵列突破旁瓣对消器的结构框架,继承并发展了多在抗干扰应用中,自适应阵列根据优化准则计算最优权矢量,而实际阵列中的非理想因素往往导致抗干扰性能下降,例如,采样快拍数有限、阵列误差、通道幅相误差等[3,8-9]. 为分析自适应阵列抗干扰性能,目前已有信干噪比(Signal-to-Interference and Noise Ratio, SINR)损失[3]、抗干扰改善因子(ECCM Improvement Factor, EIF)[6-7]等性能指标,分别反映抗干扰措施对系统性能的影响和改善. 虽然这些指标有明确的物理含义,但通常缺乏解析分析,难以在设计和应用阶段定量分析. 为此,论文深入分析推导了理想条件下SINR损失、EIF解析表达式,定量获取自适应阵列处理的抗干扰性能. 最后,仿真实验结果验证了解析表达式的有效性和采样快拍数对抗干扰性能的影响,为自适应阵列工程应用提供了理论参考.假设均匀线阵阵元数为N,阵元间距d=λ/2,波长为λ,如图1所示.阵列接收信号可表示为X(t)+N(t).式中: X(t)为t时刻各阵元接收数据向量; A为阵列流形矩阵,A=[ad,ai],a为入射信号导向矢量,dsinθ, S=[sd, si]为期望和干扰信号向量,功率分别为为t时刻各阵元接收噪声向量,分别服从独立的高斯分布,方差为.对于常规波束形成(Common Beamforming, CBF),阵列权矢量可表示为wd.对于自适应波束形成(Adaptive Digital Beam-forming, ADBF),实际中只能利用有限的快拍数进行估计,论文采用采样矩阵求逆(Sample Matrix Inversion, SMI)算法进行权矢量估计,SMI是最小方差无失真响应(Minimum Variance Distortionless Response, MVDR)波束形成器的具体实现,对权矢量的约束条件满足即对期望信号方向增益为1,使期望信号无失真通过. SMI算法的自适应权矢量表示为式中表示估计的干扰噪声协方差矩阵,K表示快拍数,Xi+n表示干扰噪声数据.为评价自适应阵列的干扰抑制性能,SINR损失LSINR定义为抗干扰输出SINR与无干扰时CBF输出SNR之比[3],满足0≤LSINR≤1. 通常,当干扰被有效抑制且信号无增益损失时,LSINR≈1,其值越小,系统性能损失越大. 其具体定义如下:式中:下标“c”和“a”用以区分CBF和ADBF; Rs、Rn、Rin分别表示阵列期望信号、噪声和干扰噪声信号的协方差矩阵.理想条件下,将式(4)、(6)、(7)代入式(5),可获得理论的SINR损失为根据附录A的分析,SINR损失可解析表达为式中为输入干噪比λ. 根据式(9),分析几个特例如下:特例1θ1=2°(干扰位于主瓣内),N=16,可得B=N+1-212.93/N,有在干扰出现在主瓣时,干扰信号与期望信号入射角度间隔越大, LSINR趋近1,即SINR损失越小.特例2θ1=18°(干扰位于常规波束第二副瓣),N=16,可得,即SINR损失接近为0.特例3θ1=30°(干扰位于常规波束副瓣零点),随着γ和N变化,均有LSINR≈1,即SINR损失接近为0.根据上述特例分析知,SINR损失与干扰方向有关,主瓣干扰时SINR损失较大,对于副瓣干扰,SINR损失基本为零,与自适应阵列理论相符. 相比式(8),式(9)无需复杂的数值计算,直接给出自适应阵列的理论曲线,更简洁地反映自适应阵列抗干扰理论性能,具有直观性和可用性.EIF[6-7]是1974年美国学者Johnston提出的,用于衡量雷达的抗干扰能力,随后被IEEE采纳. 其定义为接收机抗干扰输出信干比与不进行抗干扰时输出信干比之比,其物理意义是抗干扰措施对信干比的改善程度.对于自适应阵列,EIF可定义为阵列ADBF输出信干比( RSIa)与CBF输出信干比(RSIc)之比:由阵列信号模型可知:自适应波束形成的EIF可表示为根据附录B的推导证明,EIF的理论表达式为ηADBF=(A·γ+N)2/N2=(B·γ+1)2.式中,A=N·B,其他变量含义同式(9).同理,相比于式(14),式(15)更简洁直观地反映了自适应阵列抗干扰性能,具有更好的直观性和可用性. 例如,由式(15)可直接推知EIF是随干扰偏离主瓣指向和干噪比递增而单调递增的.本节基于数值仿真验证表征SINR损失和EIF的解析表达式,即式(9)和式(15)的正确性. 仿真条件与阵列信号模型相同,令阵元数N=16,期望信号信噪比为0dB.4.1 SINR损失的性能验证不考虑阵列非理想因素影响,理论值由上述解析表达式获得,仿真值为仿真计算结果,噪声协方差矩阵用对角阵表示,期望信号、干扰信号的协方差矩阵分别由给定的导向矢量和信号功率获得.图2(a)中干扰信号角度18°不变,干噪比从-30~50 dB变化,可见,随干噪比增大,SINR损失在0 dB左右;图2(b)中干噪比为20 dB不变,干扰信号角度从-40°~40°变化,可见,当干扰信号角度在0°附近时,SINR损失严重,在2°时SINR损失为-6.36 dB. 显然,式(9)与仿真结果吻合,且较数值仿真更简洁直观反映出系统性能变化.4.2 EIF的性能验证仿真条件与4.1节相同.图3(a)中当干噪比较小时,EIF为0 dB,随着干噪比增大,EIF逐渐增大. 图3(b)中当干扰信号角度在0°附近(相当于主瓣干扰)时,EIF趋近于0. 显然,式(15)与仿真结果吻合,且更能简洁直观反映出系统性能变化.4.3 有限快拍数下的抗干扰性能验证随着自适应阵列处理的发展及硬件水平的提高,阵元误差的影响可以被减弱或校正,而有限的采样快拍数一直是影响阵列处理性能的重要因素. 在理想条件下,MVDR 波束形成器能够获得最佳的抗干扰性能,但在工程应用中,数据协方差矩阵往往只能由有限的采样数据来估计,且估计易出现偏差[8]. 针对此问题,重点分析了有限采样快拍数下自适应阵列的抗干扰性能,结合性能指标理论曲线,验证了采样快拍数对抗干扰性能的影响.图4(a)和(b)仿真了快拍数K=29、K=50时的SINR损失.快拍数越多性能损失越小. Reed等人[9]研究指出,为保证比最优情况时损失在3 dB内,快拍数应当满足K≥2N-3. 仿真中K=29时,SINR损失约为-3 dB,与理论分析一致.图5(a)和(b)显示了在不同快拍数下EIF变化情况.当K增大时,仿真曲线在趋势上更接近于理论曲线. 图5(b)中EIF随干扰入射角度变化规律与波束方向图有密切联系.图6(a)中干扰入射角为22.02°,为常规波束方向图的零点,阵列接收干扰功率较小,而自适应处理后方向图零陷变浅,进而导致EIF变小;图6(b)中干扰入射角为18°,为常规波束方向图的第二副瓣,而自适应方向图在该方向能够产生零陷,且快拍数越多,零陷越深,其对应的EIF也越大.可见,EIF受采样快拍数影响较大,因此,在有限快拍数下自适应阵列难以获得理想的EIF.为深入研究自适应阵列抗干扰性能,在阵列信号模型基础上,分析推导了均匀线性阵列SINR损失、EIF因子的解析表达式,更简洁有效地表示自适应阵列的抗干扰性能. 同时,仿真实验也验证了解析式的正确性,上述工作对自适应阵列工程应用的性能分析有一定的指导意义. 为进一步推广研究工作,对任意阵列流型、密集多干扰等条件下的抗干扰性能优化及指标分析是未来的研究方向.附录A: SINR损失解析表达式推导应用归纳法对SINR损失的解析表达式进行推导,不失一般性,假设期望信号角度θd=0°,干扰信号角度为θi,阵元接收到的期望信号、干扰信号和噪声的功率分别为,则有:,.式中表示通道间干扰信号的互相关系数,1≤m,n≤N,φ=2π dsinθi/λ..当N=2时, ,.当N=3时, ,.当N=4时,,.归纳可知,当阵元数N≥2时,.式中附录B: EIF解析表达式推导应用归纳法对EIF的解析表达式进行推导,条件与附录A相同,则有:当N=2时, ,,η2=[(2-ejφ-e-jφ)·γ+2]2/4.当N=3时,,η3=[(6-2ejφ-2e -jφ-ej2φ-e -j2φ)·γ+3]2/9.当N=4时, ,,η4=[(12-3ejφ-3e -jφ-2ej2φ-2e -j2φ-ej3φ-e -j3φ)·γ+4]2/16.归纳可知,当N≥2时, η=(γ+N)2/N2=(B·γ+1)2.式中N.[1]VAN TREESHL.Optimum Array Processing:IVofDetection,Estimation,andModulationTheory[M].NewYork:JohnWiley &SonsInc, 2002.[2] 陈希信, 韩彦明, 李景兰. 高频雷达自适应波束形成抗干扰研究 [J]. 电波科学学报, 2010, 25(6): 1169-1173.CHENXixin,HANYanming,LIJinglan.ResearchforinterferencesuppressioninHFradarbasedonADBF[J].ChineseJournalofRadioScience, 2010, 25(6): 1169-1173. ( inChinese)[3] 王永良, 丁前军, 李荣峰. 自适应阵列处理[M]. 北京: 清华大学出版社, 2009.[4] 吴铁平, 赵洪立, 保铮. 天波超视距雷达空域干扰抑制 [J]. 电波科学学报, 2005, 20(3): 347-352.WUTieping,ZHAOHongli,BAOZheng.Spaceinterferenceexcisioninover-the-horizonradar[J].ChineseJournalofRadioScience, 2005, 20(3): 347-352. (inChinese)[5] 周围, 周正中, 张德民. 相干多径环境下自适应阵列的性能改进 [J]. 电波科学学报, 2007, 22(3): 419-423,435.ZHOUWei,ZHOUZhengzhong,ZHANGDemin.Improvementofperformanceforadaptivearrayincoherentmultipathenvironment[J].ChineseJournalofRadioScience, 2007, 22(3): 419-423, 435. ( inChinese)[6]JOHNSTONSL.ECCMimprovementfactors (EIF)[J].Electronic WarfareMagazine, 1974, 6(3): 41-45.[7]IEEEStandardBoard.IEEEStd 686-1997:IEEEStandardRadarDefinitions[S].NewYork:TheInstituteofElectricalandElectronicsEngineers, 1998.[8] 高阳. 相控阵雷达自适应旁瓣相消器干扰技术研究 [D]. 北京: 装备学院, 2011.GAOYang.JammingTechniquetoAdaptiveSide-lobeCancellerofPhasedArrayRadar[D].Beijing:AcademyofEquipment, 2011. ( in Chinese)[9]REEDS,MALLETT JD,BRENNAN LE.Rapid convergencerateinadaptivearrays[J].TransonAerospaceandElectronicSystems, 1974, 10(6): 853-863.高阳 (1988-),男,河南人,解放军装备学院在读博士研究生,研究方向为雷达信号处理、雷达对抗.许稼 (1974-),男,安徽人,北京理工大学教授、博士生导师,研究方向包括雷达高分辨率成像、检测与估计、信息对抗等.贾鑫 (1958-),男,江苏人,解放军装备学院教授、博士生导师,研究方向为信号处理、电子对抗等.龙腾(1968-),男,河南人,北京理工大学教授、博士生导师,研究方向为实时信号处理、目标探测与识别等.樊高辉, 刘尚合, 刘卫东, 等. 基于高阶累积量和动态统计的放电信号时延算法[J]. 电波科学学报,2015,30(4):736-743. doi: 10.13443/j.cjors. 2014101001FAN Gaohui, LIU Shanghe, LIU Weidong, et al. Time-delay estimation algorithm of discharge signals based on higher-order cumulant and dynamic statistics[J]. Chinese Journal of Radio Science,2015,30(4):736-743. (in Chinese). doi: 10.13443/j.cjors. 2014101001。
奇异值分解和Teage r能量算子的电压暂降方法

奇异值分解和Teage r能量算子的电压暂降方法苏清梅【期刊名称】《广东电力》【年(卷),期】2014(000)010【摘要】针对噪声干扰严重影响电压暂降检测分析精度的问题,提出一种采用奇异值分解和 Teager 能量算子(Teager energy operator,TEO)进行电压暂降检测的改进算法,即采用 Hankel矩阵对电压暂降信号进行奇异值分解法(singular value decomposition,SVD),从而获得降噪后的供电点上电压暂降近似信号;利用Teager 能量算子跟踪该近似信号的瞬时幅值;最后通过仿真算例验证该方法的可行性和有效性。
%Aiming at problem of noise disturbance seriously affecting voltage sag detection analysis precision,an improved al-gorithm for detecting voltage sag based on singular value decomposition and Teager energy operator was proposed which was to use Hankel matrix to realize singular value decomposition for voltage sag signal and obtain approximate signal about volt-age sag at the point of power supply after denoising.By using Teager energy operator,it was able to trace instantaneous am-plitude of this approximate signal.By simulation verification,feasibility and effectiveness of this method was proved.【总页数】5页(P47-51)【作者】苏清梅【作者单位】福建省电力有限公司电力科学研究院,福建福州350008【正文语种】中文【中图分类】TM71【相关文献】1.结合小波变换和能量算子的电压暂降检测方法 [J], 鲁波涌;黄文清2.基于改进型能量算子的电压暂降快速检测方法 [J], 董娜;黄文清;周超;孙干辉3.利用Teager能量算子瞬时能量的模块化多电平换流器多端柔性直流电网保护方法 [J], 高淑萍;高悦;宋国兵;姜元月;段必聪4.基于小波能量熵的配电网电压暂降源定位方法 [J], 胡安平;姜玉洁;陶以彬;孙浩天;易皓5.阶次跟踪能量算子与奇异值分解结合的滚动轴承故障诊断 [J], 江志农;胡明辉;冯坤;贺雅因版权原因,仅展示原文概要,查看原文内容请购买。
基于恒压法结合变步长电导增量法的最大功率点跟踪
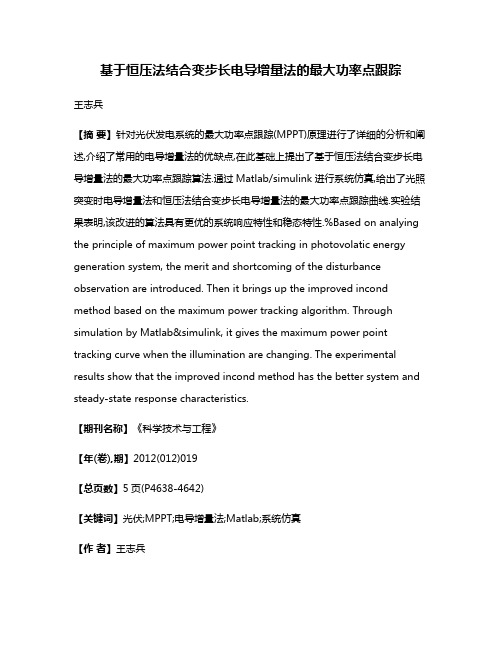
基于恒压法结合变步长电导增量法的最大功率点跟踪王志兵【摘要】针对光伏发电系统的最大功率点跟踪(MPPT)原理进行了详细的分析和阐述,介绍了常用的电导增量法的优缺点,在此基础上提出了基于恒压法结合变步长电导增量法的最大功率点跟踪算法.通过Matlab/simulink进行系统仿真,给出了光照突变时电导增量法和恒压法结合变步长电导增量法的最大功率点跟踪曲线.实验结果表明,该改进的算法具有更优的系统响应特性和稳态特性.%Based on analying the principle of maximum power point tracking in photovolatic energy generation system, the merit and shortcoming of the disturbance observation are introduced. Then it brings up the improved incond method based on the maximum power tracking algorithm. Through simulation by Matlab&simulink, it gives the maximum power point tracking curve when the illumination are changing. The experimental results show that the improved incond method has the better system and steady-state response characteristics.【期刊名称】《科学技术与工程》【年(卷),期】2012(012)019【总页数】5页(P4638-4642)【关键词】光伏;MPPT;电导增量法;Matlab;系统仿真【作者】王志兵【作者单位】扬州晶旭电源有限公司,扬州市太阳能光伏发电系统工程技术研究中心,扬州225127【正文语种】中文【中图分类】TK513.1能源危机已严重影响着人类经济的发展,光伏发电以其独特优势受到了人们的青睐[1,2]。
LTE系统中一种有效的速度估计算法

LTE系统中一种有效的速度估计算法在移动通信系统中,传统的速度移动用户估计方法一般分为时域相关法、频域相关法、经验函数法。
文章提出了一种联合频域相关法和经验函数法的速度估计算法,该算法通过把频域相关法和经验函数法的优点结合起来,使得估计的准确度有所提高。
文章还针对该算法的准确度效果,在LTE-FDD 系统的PUSCH 链路中进行了仿真验证。
【摘 要】【关键词】LTE-FDD 速度估计 频域相关 经验函数黎 广 武汉理工大学管 鲍 武汉虹信软件有限责任公司李平安 武汉理工大学王 俊 武汉虹信软件有限责任公司收稿日期:2012-08-21责任编辑:陈雍君********************1 引言在L T E 系统中,速度估计指的是在U E (U s e r Equipment)接收端(即基站)对UE的速度进行估计。
由于高速移动的UE会带来多普勒频偏,这种频移会破坏子载波的正交性,给信号解调带来困难,特别是当无线信道中存在多径传输时,接收机的高速移动会导致接收信号受到快速时变衰落的干扰[1],因此产生信号的严重衰变,带来OFDM(Orthogonal Frequency Division Multiplexing)系统性能的大幅下降。
因此,在LTE系统中,可以根据估计出来的UE速度值来判断出当前移动传播环境的好坏,从而进行自适应编码;且高层可以根据UE速度的大小决定UE配置在宏小区还是微小区,避免不停地切换。
传统的速度估计算法一般分为时域相关法[2-3]、频域相关法[4]、经验函数法[5],但是上述三种方法要么存在实现困难要么存在精确度不够的缺点。
在LTE系统中,由于在硬件实现时,是将接收端的数据统一变为频域数据后再进行处理的,所以时域相关法不能实现。
而频域相关法和经验函数法的准确度都不是很高,因此本文提出一种结合频域相关法和经验函数法的速度估计算法,将提高速度估计的准确度。
2 系统模型以两根发送天线和两根接收天线为例进行说明。
能量比函数法估算局部放电时延迟
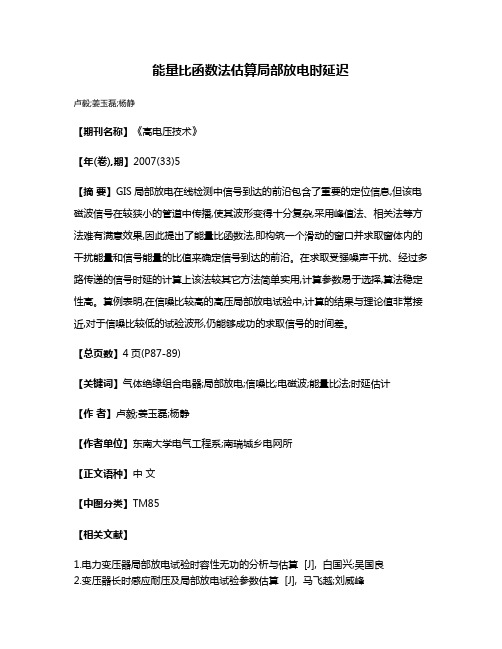
能量比函数法估算局部放电时延迟
卢毅;姜玉磊;杨静
【期刊名称】《高电压技术》
【年(卷),期】2007(33)5
【摘要】GIS局部放电在线检测中信号到达的前沿包含了重要的定位信息,但该电磁波信号在较狭小的管道中传播,使其波形变得十分复杂,采用峰值法、相关法等方法难有满意效果,因此提出了能量比函数法,即构筑一个滑动的窗口并求取窗体内的干扰能量和信号能量的比值来确定信号到达的前沿。
在求取受强噪声干扰、经过多路传递的信号时延的计算上该法较其它方法简单实用,计算参数易于选择,算法稳定性高。
算例表明,在信噪比较高的高压局部放电试验中,计算的结果与理论值非常接近,对于信噪比较低的试验波形,仍能够成功的求取信号的时间差。
【总页数】4页(P87-89)
【关键词】气体绝缘组合电器;局部放电;信噪比;电磁波;能量比法;时延估计
【作者】卢毅;姜玉磊;杨静
【作者单位】东南大学电气工程系;南瑞城乡电网所
【正文语种】中文
【中图分类】TM85
【相关文献】
1.电力变压器局部放电试验时容性无功的分析与估算 [J], 白国兴;吴国良
2.变压器长时感应耐压及局部放电试验参数估算 [J], 马飞越;刘威峰
3.基于AIC准则和时窗能量比的电缆局部放电在线检测与定位 [J], 孙抗;郭景蝶;杨延举;马星河
4.变压器感应耐压及局部放电试验时的功率估算 [J], 刘辉
5.采用Fisher线性判别法提取GIS内部局部放电信号最优能量特征 [J], 田宇; 罗沙; 李宾宾; 孙文
因版权原因,仅展示原文概要,查看原文内容请购买。
双电压激励法在电容层析成像系统中的应用
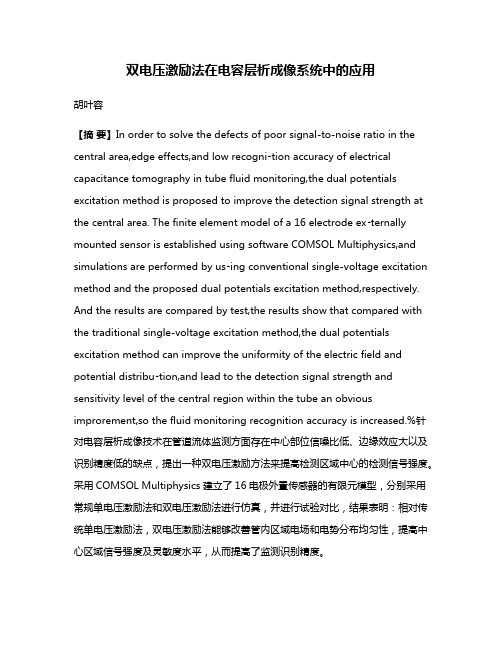
双电压激励法在电容层析成像系统中的应用胡叶容【摘要】In order to solve the defects of poor signal-to-noise ratio in the central area,edge effects,and low recogni⁃tion accuracy of electrical capacitance tomography in tube fluid monitoring,the dual potentials excitation method is proposed to improve the detection signal strength at the central area. The finite element model of a 16 electrode ex⁃ternally mounted sensor is established using software COMSOL Multiphysics,and simulations are performed by us⁃ing conventional single-voltage excitation method and the proposed dual potentials excitation method,respectively. And the results are compared by test,the results show that compared with the traditional single-voltage excitation method,the dual potentials excitation method can improve the uniformity of the electric field and potential distribu⁃tion,and lead to the detection signal strength and sensitivity level of the central region within the tube an obvious improrement,so the fluid monitoring recognition accuracy is increased.%针对电容层析成像技术在管道流体监测方面存在中心部位信噪比低、边缘效应大以及识别精度低的缺点,提出一种双电压激励方法来提高检测区域中心的检测信号强度。
- 1、下载文档前请自行甄别文档内容的完整性,平台不提供额外的编辑、内容补充、找答案等附加服务。
- 2、"仅部分预览"的文档,不可在线预览部分如存在完整性等问题,可反馈申请退款(可完整预览的文档不适用该条件!)。
- 3、如文档侵犯您的权益,请联系客服反馈,我们会尽快为您处理(人工客服工作时间:9:00-18:30)。
Energy-Delay Estimation Technique for High-Performance Microprocessor VLSI AddersVojin G. Oklobdzija1, Bart R. Zeydel1, Hoang Dao1, Sanu Mathew2, Ram Krishnamurthy2 2 1 Intel Corporation ACSEL Circuit Research Labs University of California Hillsboro, OR 97124 Davis, CA 95616 Sanu.k.Mathew@ /acselAbstractIn this paper, we motivate the concept of comparing VLSI adders based on their energy-delay trade-offs and present a technique for estimating the energy-delay space of various high-performance VLSI adder topologies. Further, we show that our estimates accurately represent tradeoffs in the energy-delay space for high-performance 32-bit and 64-bit processor adders in 0.13µm and 0.10µm CMOS technologies, with an accuracy of 8% in delay estimates and 20% in energy estimates, compared with simulated data. method of Logical Effort (LE) [3], which has been popularized by Harris [4]. Recently, we used Logical Effort to estimate the speed of various VLSI adders and we compared those results with those obtained using a more complex circuit simulation tool H-SPICE [5]. This comparison showed a good match and pointed to the right direction. However, the process of analysis was now time consuming and did not provide a comparison for various circuit sizing that could have been applied. This paper is organized as follows: the second section discusses speed estimation using more realistic measures such as logical effort, the third section introduces the energy effects and discusses the performance in the energy-delay space, the fourth section describes the estimation tool that was developed, the fifth section shows results applied to several well known adder topologies and compares them with simulated results in 0.13µm technology.1. IntroductionIn the course of VLSI processor design it is very important to choose the adder topology that would yield the desired performance. However, the performance of a chosen topology will be known only after the design is finished. Therefore a lingering question remains: could we have achieved a higher performance, or could we have had a better VLSI adder topology? The answers to those questions are generally not known. There is no consistent and realistic speed estimation method employed today by the computer arithmetic community. Most of the algorithms are based on out-dated methods of counting the number of logic gates in the critical path producing inaccurate and misleading results. The importance of loading and wire delay is not taken into account by most. Knowles has shown how different topologies may influence fan-out and wiring density thus influencing design decisions and yielding better area/power than known cases [1]. This work has further emphasized a disconnect existing between algorithms that are used to derive VLSI adder topologies and the final result. In previous work we have shown the importance of accounting for fan-in and fan-out on the critical path, not merely the number of logic levels [2]. This has led to theThis work has been supported by SRC Research Grant No. 931.001 and California MICRO 01-0632. Speed EstimationThe speed of a VLSI adder depends on many factors: the technology of implementation (and its own internal rules), circuit family used for the implementation, sizing of transistors, chosen topology of the VLSI adder, and many other second order effect parameters. There were no simple rules that could be applied when estimating VLSI adder speed. Skilled engineers are capable of fine-tuning the design by carefully selecting transistor sizes, obtaining the best performance and energy trade-off. Therefore it is very difficult, if not impossible, to predict which of the topologies developed by the computer arithmetic community is best, even if it is really useful.2.1 Logical EffortLogical Effort methodology takes into account the fact that the speed of a digital circuit block is dependent on its output load (fan-out) and its topology (fan-in). Further, LE introduces technology independence by normalizing the speed to that of a minimal size inverterwhich makes the comparisons of different topologies, implemented in different technologies, possible. For proper understanding and further reading of this paper the reader should be familiar with the LE methodology [3,4]. We will briefly describe some of the main features of LE in this sub-section. The delay expression of a logic block in LE is given as:Logical Effort tells us that the delay will be minimal when each stage bears equal effort given as:fˆ = g i h i = Fˆ D = Nf + P1 N(3)In such a case, delay of the path will be equal to: (4) In order to calculate optimal transistor sizes to achieve minimal delay, we start from the output and calculate Cin for each stage, which determines the sizing of each stage.d= f+p(1)where p = parasitic delay, f = effort or stage delay. Further f = gh where g is defined as logical effort and h as electrical effort. Thus:d = gh + pThis dependency is illustrated in Fig. 1.2.2. 64-bit Adder Speed Comparison using Logical EffortWe used several representative topologies and performed critical path analysis using Logical Effort technique to compare performance. The adders that were examined were: (a) Static: Kogge-Stone (KS) radix-2 [6], Mux-based carry-select [7], and Han-Carlson (HC) radix2 [8,9] (b) Dynamic: KS radix-2, Ling Adder [10], HC radix-2 and CLA adder with 4-bit grouping.Delay (No. of FO4) 16 14 12 10 8 6(2)Static CM OS Logic ImplementationLogical Effort HSPICE Dynam ic CM OS ImplementationFig. 1. Delay expressed in terms of a minimal size inverter [3,4]4 2 0An important result of LE is that it provides a way of determining appropriate transistor sizing of the critical path to minimize delay. LE also provides an estimate of the critical path delay. Logical Effort results are summarized in Table 1.Table 1: Logical Effort EquationsKSMXAHCKSLingHCCLAFig. 2. Speed estimation of various VLSI adders using Logical Effort vs. H-SPICE resultsPath Logical Effort Path Effort: ElectricalG = ∏ giH = ∏ pi =b=Cout CinBranching EffortCoff − path + Con− path Con− pathPath Branching Effort: Path parasitic delay Path Effort:B = ∏ bi P = ∑ piF = GBHThe results obtained using Logical Effort were compared with the results obtained using H-SPICE simulation. The comparison results are shown in Fig. 2. Wire delays were accounted for by estimating the length of the wire and assigning appropriate delay to it, however, the portion of the wire delay was not significant (less than 10% of the total delay) due to the proximity of the cells. The first obvious observation is that there is a huge difference between Static CMOS and Dynamic CMOS implementations. This demonstrates the dependency on logic design style, which obscures any differences between different VLSI adders. This fact has been known by practitioners and rarely would we see a Static CMOS adder in places where high-speed is required. The prediction error is under 10% in most cases.We are still in the process of refining the LE calculation in order to gain better accuracy. However, our objective is to have a simple “back of the envelope” method for quick estimation and evaluation of different VLSI adder topologies without venturing into CAD tool complexity. Therefore, we compromised by using MS-Excel as a tool for comparison, because of its simplicity and ability to perform complex calculations. An example of the Excel tool used is shown in Table 3 (CM – represents CarryMerge cell, Dk1ND2 – represents a “footed” dynamic NAND). Before the analysis, it is necessary to characterize the technology used. This step needs to be done only once, but it improves the accuracy of the LE since the characteristics of the technology are taken into account.P re fix -2 Kogge -Stone (S ta tic) Bra nch Bit Effort S ta ge s Spa n (b i ) g0 (N AND2) C0 (O A I) C2 (A OI) C6 (O A I) C14 (A OI) C30 (OA I) C62 (A OI) S 63 (TGXORs) INV (INV) 0 2 4 8 16 32 0 0 0 2. 0 2. 2 2. 4 2. 8 3. 6 5. 2 1. 0 1. 0 3. 0Table 2: Normalized LE parameters0.10µm technology, FO4=19pS Parasitics Gate type LE (g) (p inv) Inverter Dyn. Nand Dyn. CM Dyn. CM-4N Static CM Mux XOR 1 0.6 0.6 1 1.48 1.68 1.69 1 1.34 1.62 3.71 2.53 2.93 2.97Table 3: Delay Comparison of Static and Dynamic implementation of Kogge-Stone Prefix-2 AdderLE (g i ) 1.11 1.55 1.52 1.55 1.52 1.55 1.52 1.56 1.00 P a ra sitic (p i ) 1.84 2.26 2.76 2.26 2.76 2.26 2.76 2.59 1.00 Tota l Bra nch (B) Tota l LE (G) P a th Effort (F) Fopt (f) Effort De la y (p s) P a ra sitic De la y (ps) W ire De la y (ps) Tota l De la y (ps) Tota l De la y (FO 4)1.66E +03 2.26E +01 3.76E +043.2210 6881420911.0P re fix -2 Kogge -S tone (Dyn a m ic) Bra nch LE Bit Effort S ta ge s S pa n (g i ) (b i ) g0 (D k1ND2) C0 (O A I) C2 (D AOI) C6 (O A I) C14 (DAOI) C30 (OA I) C62 (DAOI) S 63 (TGXORs) INV (INV ) 0 2 4 8 16 32 0 0 0 2 .0 2 .2 2 .4 2 .8 3 .6 5 .2 1 .0 1 .0 3 .0 1.02 1.36 0.68 1.36 0.68 1.36 0.68 1.56 1.00Pa ra sitic (p i ) 1.34 1.69 1.33 1.69 1.33 1.69 1.33 2.59 1.00Tota l Bra nch (B)Tota l LE (G)Pa th Effort (F)Fopt (f)E ffort P a ra sitic W ire De l a y D e la y De la y (p s) (ps) (ps)Tota l De la y (ps)Tota l De la y (FO4)1.66E+031.26E+ 00 2.09E +032.347760141518.0Characterization is performed using SPICE simulation of the gate delay for various output loads driving a copy of itself, according to the LE rules. This is repeated for each cell used in the logic library. Characterization of dynamic gates requires special attention due to the fact that only one transition is of interest. Obtained results are compared to that of an inverter and parameters such as parasitic delay (p) and effort (g) were normalized with respect to that of an inverter. Select results are shown in Table 2.This step preserves LE features, allowing delay results to be presented in terms of fan-out of 4 (FO4) delay, relatively independent of the technology of implementation. The LE-based delay estimation tool works on the logic stages in the critical path, assigning branch effort (bi), logical effort (gi) and parasitic effort (pi) to each gate (Table 3). In computing the branch effort,we take into consideration the worst-case interconnect at each stage. In a 64-bit Kogge-Stone adder, the worst-case interconnect in stage 6 (CM C30) spans 32 bit-slices. We make an assumption that the adder bit-pitch is 10um, which would result in a 320µm wire. To account for the propagation delay through a wire we incorporate an Elmore delay model in Table 3, which corresponds to the critical-path interconnect delay in the adder. A comparison of representative VLSI adders implemented in static and dynamic CMOS design style is presented in Table 4. It is interesting to see that there are indeed very small speed differences between the three fastest dynamic adders: KS, HC and Quarternary (QT). The advantage of KS is achieved by reduced parasitic delays resulting from fewer stages. It is also very difficult to determine the fastest VLSI adder from the results presented in Table 4.Table 4. Comparison of representative VLSI adders using Logical Effort (wire delay estimate included)Adders Static MXA Static KS Static HC Dynamic KS Dynamic HC Dynamic QT Dynamic LNG Dynamic CLAStages 15 9 10 9 10 10 10 14Total Branch (B) 11600 1660 1660 1660 1660 1540 1430 20600Total LE (G) 0.369 22.6 22.6 1.26 1.26 2.08 0.973 0.627Path Effort (F) 4280 37600 37600 2090 2090 3220 1400 12900fopt 1.75 3.22 2.87 2.34 2.15 2.24 2.06 1.97Effort Delay (pS) 96 106 105 77 79 82 76 101Parasitic Delay (pS) 93 88 92 60 64 68 70 81Wire Delay (pS) 14 14 14 14 14 8 15 12Total Delay (pS) (FO4) 203 209 212 151 157 158 161 195 10.7 11 11.1 8.0 8.26 8.3 8.47 10.26The differences between presented topologies are small and fall within the margin of error introduced by inaccuracy of the estimation method. This further emphasizes difficulties in comparing VLSI adder topologies and determining the best one.3. Energy-Delay TradeoffsComparing VLSI adders becomes more difficult when the notion of power or energy used for computation is introduced. Suppose that an adder A is compared with an adder B and that adder A is faster than adder B. Based on speed only, our inclination would be to use adder A in our design. However, this is not the complete picture. If the energy consumed is considered, and if adder B turns out to be using less energy, we may chose adder B, depending on the power requirements imposed on our design. However, power (energy) can be traded for speed and vice versa. Fig. 3 illustrates the hypothetical energydelay dependencies of adders A and B.Energy Adder Apoints A and B with respect to speed and disregard the energy aspect. As the curves show, adder B has more room for improvement and with further energy-delay optimization this adder would move to the point where better performance is obtained by using the adder topology B than topology A (Region 1). However, if lowenergy of operation is our objective, we see that adder topology A is better because it can achieve lower energy for the same delay (Region 2). Thus, Fig. 3 illustrates the importance of taking the energy into account, not just merely the speed of the adder.3.1. Energy EstimationLogical Effort method does include an estimation of energy. It only provides one point on the Energy-Delay curve corresponding to a sizing optimized for speed. Where this point lies on the Energy-Delay curve remains an unknown. In order to generate Energy-Delay estimates it is first necessary to include some way of estimating energy into the Logical Effort method. We start by characterizing each cell in terms of energy. The energy depends on at least two parameters: output load and cell size. Energy of a two-input NAND gate as a function of its size and fan-out load is shown in Fig. 410AB Adder B8EnergyRegion 1 Region 2642DelayFig. 3. Energy-Delay dependency038 4 5 6 7 8In this example, adder A would be chosen as a better adder topology if we were just to compare two designFanout Fig. 4. Energy dependency of a 2-input NAND cellSi z e20 16 12Thus, the energy estimate will depend on the sizing determined by logical effort as well as the fan-out load on the output of the cell. Each cell used in the design is characterized and parameters determining the energy dependency on the cell size and fan-out load were stored in the table from which dependency parameters were determined. The method of logical effort simplifies the energy calculations as it roughly equalizes the input and output slopes of each gate. This implies that for a given output load and gate size, which defines the output slope, there is only one input slope that is possible. Although it is true that parasitics and unequal stage effort due to wiring will result in some variations in the slopes, it provides enough accuracy for the analysis being performed. Optimal sizing for speed is determined for each adder by using a modified logical effort methodology. From the information about the size and topology of an adder, the energy consumption is determined. We report worst-case energy for the estimates, defined as the energy consumed when every internal node is switching.In order to stay on the same curve we vary the size of the inputs by assigning different values to the input capacitance Cin, or by assigning different values to H (electrical effort). This results in different sizes (all optimal in terms of speed) determined by LE methodology using different energies. The adders were implemented as regular domino and compound-domino. The single points obtained when using the same input size for each adder implementation are shown in Fig. 5. The difference between regular domino and compounddomino is in the Carry-Merge stage. Given that dynamic CMOS Domino logic is used for implementation of Carry-Merge blocks as in Fig. 6.VDD Clk VDD VDD Clk Gi Gi-1 Pi Clk Gi-2 Gi-3 Pi-2 VDD Pi-1 Pi Delayed Clk3.2. SizingIn general, producing various points on the EnergyDelay curve poses a sizing problem. Assuming that we start from some given size - e.g. minimal, Logical Effort should give us an answer to how the circuit blocks should be sized to achieve minimal delay. From the assigned sizes, we can calculate the energy that will be consumed by the adder for a given input activity. However, this is just one point on the Energy-Delay curve. From this point we can move in both directions; toward smaller and toward larger sizes. Such an Energy-Delay curve, produced for two 64-bit implementations of KS and HC adders is shown in Fig. 5.9 8Fig. 6. Carry-Merge: Regular Domino ImplementationIt has been realized that the inverter, which is necessary in the CMOS Domino logic block, can be replaced with a static AND-NOR gate, referred to as compound-domino. Thus, two domino blocks are merged into one with the advantage that an additional function is achieved by replacing the inverter. This is shown in Fig. 7.VD D ClkV DD ClkKS dominoStatic GateP i-1 Pi7Normalized Energy6G i-2 G i-3 P i-2 Clk GiV DD543KS compound-domino HC compound-domino HC dominoG i-1Pi21Fig. 7. Carry-Merge: Compound-Domino Implementation00.911.11.21.31.41.51.61.7Normalized DelayFig. 5. Estimated Energy-Delay dependency of 64-bit Prefix-2 KS and HC Adders for Domino and Compound-Domino implementationsWhen using compound-domino circuits for adders, it is important to note that the dynamic and the static outputs are potentially attached to long wires, while in domino only the inverter outputs are attached to long wires. Note that “footless domino”, i.e. a circuit where the bottom transistor is eliminated, is used. A 64-bit criticalpath contains 6 carry-merge stages; none of which contain a stack of more than two n or p transistors. A critical path in the HC adder is shown in Fig. 8 [9]. It contains one more stage in the critical path, but it eliminates approximately one half of the blocks in the carry-merge tree, thus bringing some potential energy advantages over a KS implementation.31 30 29 28 27 26 25 24 23 22 21 20 19 18 17 16 15 14 13 12 11 10 9 87 6 5 43 2 1 0clkPG 3N 3N 3N 3N 3N 3N 3N 3N 3N 3N 3N 3N 3N 3N 3N 3N 3N 3N 3N 3N 3N 3N 3N 3N 3N 3N 3N 3N 3N 3N 3N 3N Gen. Carry2P2P2P2P2P2P2P2P 2P 2N 2P 2N2P2P2P2P 2N 2P2P2P2P Merge1Carryclk2 2N2N2N 2P2N2N2N Merge2Carry Merge3 Carry Merge4 Carry Merge5clk32N 2P 2N4bit 2:1 Mux2N2P C23#4bit 2:1 Mux 4bit 2:1 Mux2P C19#4bit 2:1 MuxC27#C15#4bit 2:1 MuxC11#4bit 2:1 MuxC7#4bit 2:1 MuxC3#4bit 2:1 MuxCout Sum[31:28] Sum[27:24] Sum[23:20] Sum[19:16] Sum[15:12] Sum[11:8] Sum[7:4]Sum[3:0]Fig. 9. 32-bit Quaternary-tree Adder Core [12]Fig. 8. Critical Path in the 64-bit Han-Carlson adder [9]The energy-delay chart in Fig. 5 shows domino HC having an advantage over domino KS in terms of lower energy, but domino KS can stretch further in terms of lower delay at the expense of increased energy. When comparing compound-domino implementations, both HC and KS result in lower energy than the regular domino implementations. If one is concerned about energy, HC is better. However, as the speed becomes increasingly important the advantage moves in favor of KS. Both schemes suffer from high gate counts, large transistor sizes, and excessive wiring, resulting in large layout areas and high-energy consumption. In an attempt to arrive at a denser design, a Ladner-Fischer adder described in [1,11] trades off wiring complexity by exponentially growing the fan-outs of successive carry-merge gates to 1,2,4,8 and 16 respectively. However, this does not address the problem of high gate counts and large-transistor sizes. The idea of reducing energy by breaking the scheme into 4-bit blocks, which are conditionally added, was further developed by Intel [12,13]. It was realized that pruning down the main tree to obtain a sparse-tree that propagates P and G signals for 4-bit sections of the adder and combining this with a modest increase in hardware complexity due to the conditional sum technique, might be a good energy-delay trade-off. A 32-bit QuaternaryTree (QT) adder core as described in [12], consists of a sparse-tree that generates 1 in 4 carries (Fig. 9) and a parallel side-path of 4-bit conditional sum blocks, resulting in an 8-stage design (same number of stages as KS).Carries generated by the sparse-tree select the final sum using a 2:1 multiplexer. QT adder achieves energy reduction due to two factors: (a) reduced fan-outs and reduced wiring as compared to KS and HC structure (resulting in smaller transistor sizes) and (b) use of noncritical ripple-carry conditional sum blocks (with much smaller transistor sizes than main tree). In contrast to a Ladner-Fischer design, the QT adder tree has unit fanouts on all generate gates, except the 3 highlighted gates in the Carry-Merge3, Carry-Merge4 and Carry-Merge5 stages. These 3 gates have fan-outs of 2,3 and 4 respectively (In both cases, we ignore the presence of the buffering inverters at the LSBs). Parallel-prefix logic removed from the main-tree is performed in the conditional sum blocks using an energy-efficient ripplecarry scheme with smaller transistor sizes. The structure of conditional sum blocks is shown in Fig. 10.Pi+3,Gi+3 Pi+2 ,Gi+2 Pi+1 Gi+1 Pi Optimized 1st-level 1stcarry-merge carryCM CM XOR CM XOR XOR 2:1 Sumi+2 CMSumi ,1 Sumi ,0XORXORXOR2:1 2:1 Sumi+3 i+32:1 Sumi+12:1 SumiCarryFig. 10. Quaternary-tree Adder: Conditional Sum [12]A 64-bit QT adder (Fig. 11) has 2 levels of conditional carry generation with the main tree generating 1 in 16 carries, which select betwwen the 1 in 4 conditional carries generated by the intermediate generator. The 1 in 4 carries then choose the appropriate 4-bit conditonal sum. Such a design has 10 stages (same as HC).63 61 59 57bb bbC47Int. Carry Genr Int. Carry GenrC31Int. Carry GenrC15Int. Carry GenrC inenergy-delay space, we need to determine a method for estimating the energy of an adder. By determining the number of gates per stage and assuming the same energy for those gates as the corresponding energy of the critical path gate in the same stage, we were able to obtain reasonably accurate results (within 20%). This provided a simple method to apply to most parallel prefix designs, however for a design like QT where the number of gates on each path is different, more care must be taken in determining what energy the off-path circuits consume.3000C59 C554-bit SumGenrC5C47C43 C39 C35C31C27 C23 C19C15C11 C7C3HC Compound-Domino25004-bit SumGenr4-bit SumGenr4-bit SumGen r4-bit SumGenrSum[63:60]Sum[47:44]Sum[31:28]Sum[15:12]Sum[3:0]2000Fig. 11. 64-bit Quaternary-tree Adder CoreEnergy [fJ]15004. Estimation MethodThe goal of the estimation method is to provide a simple, yet accurate, method for comparing designs in the energy-delay space. Logical effort has been shown to yield reasonably accurate results for the parallel prefix adders that were tested, thus finding it suitable for delay estimation and sizing. One of the issues with Logical Effort is branching, specifically with regards to long internal wires and different number of stages in the off path. These effects are difficult to account for and accurate accounting makes LE complex, thus simplifications are required to make the analysis linear. A more detailed account of these issues would result in greater delay accuracy when applying LE to adders.HC Static1000KS Compound-Domino KS Static5000100120140160180200220240260280Delay [pS]Fig. 12. Energy Delay comparison of 64-bit KS and HC Compound-Domino and Static adders5. ResultsThe accuracy of our energy-delay estimation was tested on 32-bit KS and QT compound-domino adder implementations, with comparison to simulation results in 0.13µm technology [12]. Each of the energy-delay simulation points was obtained using a circuit optimizer. The energy reported was obtained by running the worstcase energy vector for each topology. Since the simulated data points were in 0.13µm technology, we needed to extrapolate the results and normalize them to the same technology as our estimates (0.10µm). We used a rule of thumb of 30% performance improvement per generation as well as a 50% energy improvement. The energy-delay estimates were obtained by varying the size of the input gate to the adder, as previously described. The estimated energy assumes an estimated worst-case switching activity associated with the topology of the adder and the logic design style. A more accurate method would require detailed modeling of the switching activity within each adder core, which is not possible prior to implementation. The comparison of the estimated energy-delay versus simulated is shown in Fig. 13. At iso-delay the simulations show 55% difference in energy at the knee of the curves, while estimation shows 35% difference. At iso-energy, the simulations show 21% delay improvement at the knee of the curves, while estimation shows 13%.4.1 Energy-Delay Curve EstimationAs previously described, the different points on the energy-delay curve are obtained by varying the size of the input gate for each adder. This provides a delay estimate and the sizing of the critical path, which in turn gives an energy estimate for the gates on the critical path. However, this estimation does not provide an energy estimate for the entire adder. The estimated energy for the critical paths is shown in Fig.12. From these estimates, it can be seen that the critical path of KS uses less energy for a given delay then HC. This is explained by the one extra stage that HC uses. However as mentioned previously, HC uses approximately half of the gates in the CM-section than KS. This must be accounted for in the total energy estimate for the adders. The critical path energy-delay estimate provides an idea of the minimal delay that can be achieved, which is shown by the vertical asymptote of the curves in Fig.12. Since we are interested in comparing adders not only by delay, but also in the60reduction in energy vs. HC compound-domino at the knee of the curve.KS Estimate506. Conclusion40Energy [pJ]3055%2035%QT Estimate10KS [9]QT [9]0 90 100 110 120 130 140 150 160Delay [pS]Fig. 13. Energy-Delay comparison of 32-bit QT and KS adders: estimated vs. simulation in 0.10µ m technology µAs the intent of this method is to compare the energy-delay characteristics of a given design, this comparison shows the estimation accurately represents tradeoffs in the energy-delay space for different architectures. To further explore the energy-delay design space we analyzed 64-bit KS, HC and QT compounddomino and static topologies, shown in Fig. 14.3We have shown that an LE based analysis of logical circuits is an effective tool for a quick exploration of the energy-delay space for comparing the performance of high-performance adders. Further, a tool was developed based on this technique to quickly estimate the energydelay space of 32/64-bit Kogge-Stone, Han-Carlson, and Quaternary-tree adders implemented in 0.13µm and 0.10µm CMOS technologies using static and dynamic circuit styles, thereby, accurately representing tradeoffs in the energy-delay space with an accuracy of 8% in delay estimates and 20% in energy estimates, compared with simulated data.References[1] S. Knowles, “A Family of Adders”, Proceedings of the 14th Symposium on Computer Arithmetic, Australia. April 1999. [2] V. G. Oklobdzija and E. R. Barnes, “On Implementing Addition in VLSI Technology,” IEEE Journal of Parallel and Distributed Computing, No. 5, 1988 pp. 716-728. [3] R. F. Sproull, and I. E. Sutherland, “Logical Effort: Designing for Speed on the Back of an Envelop,” IEEE Adv. Research in VLSI, C. Sequin (editor), MIT Press, 1991. [4] D. Harris, R.F. Sproull, and I.E. Sutherland, “Logical Effort Designing Fast CMOS Circuits,” Morgan Kaufmann Pub., 1999. [5] H.Q. Dao, V. G. Oklobdzija, “Application of Logical Effort Techniques for Speed Optimization and Analysis of Representative Adders,” 35th Annual Asilomar Conference on Signals, Systems and Computers, 2001. [6] P.M. Kogge and H.S. Stone, “A parallel algorithm for the efficient solution of a general class of recurrence equations”, IEEE Trans. on Comp. Vol. C-22, No.8, Aug. 1973, pp.786-793. [7] A. Farooqui, V. G. Oklobdzija, F. Chehrazi, “Multiplexer Based Adder for Media Signal Processing”, International Symp on VLSI Technology, Systems, and Applications, 1999 [8] T. Han, D. A. Carlson, and S. P. Levitan, “VLSI Design of High-Speed Low-Area Addition Circuitry,” Proceedings of the IEEE International Conference on Computer Design: VLSI in Computers and Processors,1987, pp.418-422. [9] S.K. Mathew et al, “Sub-500-ps 64-b ALUs in 0.18µm SOI/bulk CMOS: design and scaling trends,” IEEE Journal of Solid-State Circuits, vol.36, Nov. 2001, pp.1636-46. [10] H. Ling, “High Speed Binary Adder”, IBM Journal of Research and Development, Vol. 25, No 3, 1981, p.156-166. [11] R.E. Laddner and M.J. Fischer, “Parallel prefix computation”, Journal of ACM, Vol.27, No.4, 1980, pp.831-38. [12] S.K. Mathew et al, “A 4GHz 130nm Address Generation Unit with 32-bit Sparse-tree Adder Core,” 2002 Symposium on VLSI Circuits Digest of Technical Papers, pp.126-127. [13] J. Sklansky, “Conditional-sum addition logic.” IRE Transactions on Electronic Computers, vol.9, 1960, pp. 226-231.KS compound-domino2.5Normalized Energy2KS Static1.51HC compound-dominoHC StaticQT compound-domino0.5QT Static00.91.11.31.51.71.92.1Normalized DelayFig. 14. Energy-Delay comparison of 64-bit KS, HC and QT addersThe 64-bit QT implementation used has one extra stage than the KS, which accounts for the higher performance that can be achieved by KS for both compound-domino and static topologies. The difference in energy for iso-delay is more significant in the 64-bit design space. This is due to the increased number of stages over which the delay is evenly distributed, resulting in increased gate sizes, and increased number of gates, e.g. a 32-bit KS has 417 gates, while a 64-bit KS has 1025 gates. Thus in the 64-bit design space, energy efficient designs display greater savings, which explains why QT uses substantially less energy than either KS or HC. The QT compound-domino design achieves an 86%。