细胞特异性调控元件预测
细胞类型特异性基因调控网络的重构与分析方法

细胞类型特异性基因调控网络的重构与分析方法细胞类型特异性基因调控网络的重构与分析方法是一项重要的研究工作,其旨在揭示细胞类型间基因表达差异的分子机制,并探索各种疾病的发生原因。
该方法通过整合多组学数据,如转录组、甲基化、组蛋白修饰等,从而重构细胞类型特异性的基因调控网络。
本文将介绍一种常用的细胞类型特异性基因调控网络的重构与分析方法,并探讨其在生物研究中的应用。
细胞类型特异性基因调控网络的重构可以通过多个步骤来实现。
首先,需要获取各种组学数据,如单细胞转录组数据或大规模组织样本的转录组数据。
其次,需要进行数据预处理,包括数据质控、归一化以及批次效应的去除等,以确保数据的可靠性和一致性。
接下来,可以利用一些算法和工具,如WGCNA (Weighted Gene Co-expression Network Analysis)、SCENIC (Single Cell Engineered Regulomes)和ChromVAR (Chromatin Variation Analysis)等,来从大规模基因表达数据中识别关键的调节基因和共表达模块。
这些共表达模块代表了一组在特定细胞类型中共同表达的基因,而关键的调节基因则可能是控制该模块的主要因素。
在细胞类型特异性基因调控网络的分析过程中,需要注重以下几个方面。
首先,需要对共表达模块和关键调节基因进行生物学功能注释,以了解其在特定细胞类型中的功能和作用。
这可以通过文献检索和数据库查询等手段来实现。
其次,需要对共表达模块进行进一步的验证与验证,使用独立的数据集或实验数据进行验证,以确保分析结果的可靠性和可重复性。
最后,可以通过建立基因调控网络模型来预测和验证某些基因的调控关系,并进一步研究其对细胞类型特异性功能的影响。
细胞类型特异性基因调控网络的重构与分析方法在生物医学研究中具有广泛的应用前景。
首先,通过这种方法可以揭示不同细胞类型之间的基因表达差异,从而深入了解细胞的特异性功能和发育过程。
a-box cis-acting regulatory element -回复

a-box cis-acting regulatory element -回复什么是Cis-Acting Regulatory Element?在基因组学中,核糖核酸(DNA和RNA)中的特定序列可以调控基因的表达。
这些序列被称为调控元件,它们处于基因所在的同一染色体上,因此被称为Cis-Acting Regulatory Element(cis-作用调控元件)。
Cis-作用调控元件能够通过与转录因子等调控蛋白相互作用,影响基因的转录和表达。
这一过程对于生物体正常功能的维持和发展至关重要。
Cis-作用调控元件的研究在揭示基因调控机制和开发基因治疗方面具有重要意义。
Cis-作用调控元件和基因调控过程之间的关系:在基因组中,与Cis-作用调控元件相对应的是转录因子结合位点(Trans-Acting Regulatory Element)。
Cis-作用调控元件位于基因的上游或下游区域,并且可以在基因的调控区域内。
其所在的位置在整个基因组内可能是特异性或普遍性的,它们对于控制基因的启动、沉默、重编程和调控等过程起着重要作用。
Cis-作用调控元件的分析方法:研究Cis-作用调控元件的最常用的方法是计算分析和实验验证相结合的策略。
在计算分析方面,研究人员可以使用生物信息学工具来预测和鉴定Cis-作用调控元件。
这些工具可以分析DNA序列的特定标记,如启动子序列、转录因子结合位点以及DNA甲基化位点等。
实验验证方面,研究人员可以利用CRISPR-Cas9技术来进行Cis-作用调控元件的编辑和功能验证。
Cis-作用调控元件的功能:Cis-作用调控元件可以具有多种功能。
首先,它们可以作为启动子(promoter)来驱动基因的转录。
在转录过程中,启动子通过结合转录因子和RNA聚合酶来实现基因的转录。
其次,Cis-作用调控元件还可以作为增强子(enhancer)和沉默子(silencer)。
增强子可以增强基因的转录活性,而沉默子则可以抑制基因的转录活性。
细胞信号传递的机理和调控
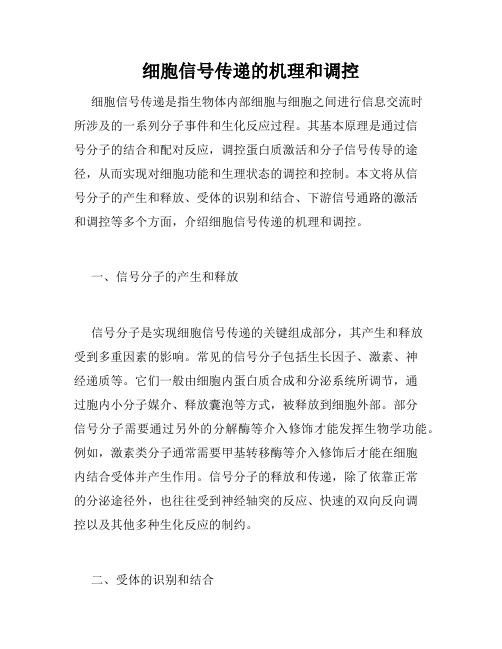
细胞信号传递的机理和调控细胞信号传递是指生物体内部细胞与细胞之间进行信息交流时所涉及的一系列分子事件和生化反应过程。
其基本原理是通过信号分子的结合和配对反应,调控蛋白质激活和分子信号传导的途径,从而实现对细胞功能和生理状态的调控和控制。
本文将从信号分子的产生和释放、受体的识别和结合、下游信号通路的激活和调控等多个方面,介绍细胞信号传递的机理和调控。
一、信号分子的产生和释放信号分子是实现细胞信号传递的关键组成部分,其产生和释放受到多重因素的影响。
常见的信号分子包括生长因子、激素、神经递质等。
它们一般由细胞内蛋白质合成和分泌系统所调节,通过胞内小分子媒介、释放囊泡等方式,被释放到细胞外部。
部分信号分子需要通过另外的分解酶等介入修饰才能发挥生物学功能。
例如,激素类分子通常需要甲基转移酶等介入修饰后才能在细胞内结合受体并产生作用。
信号分子的释放和传递,除了依靠正常的分泌途径外,也往往受到神经轴突的反应、快速的双向反向调控以及其他多种生化反应的制约。
二、受体的识别和结合在信号分子与靶细胞发生交互之前,它需要先与细胞上的受体结合,从而启动信号传递的下一步。
受体一般被细胞膜或内质网表面、细胞内蛋白质或其他有机物质所包裹,可与各种不同的信号分子相交互。
受体表面一般有特定结合位点,可以和信号分子中和配对,从而引发后继的反应。
受体与信号分子结合后,可处于激活、抑制、功能调控等多种状态。
受体与信号分子交互时的选择性往往决定于受体的特异性结构和信号分子的空间结构、亲和性匹配等特征。
对于一个特定的信号分子而言,要实现对靶细胞的选择性调控,则一定需要存在匹配的特异性受体。
三、下游信号通路的激活和调控信号分子经受体激活后,会通过下游信号转导通路,引发各种细胞功能和生理状态的改变,如蛋白质激活、基因表达、离子通道调控等。
下游信号通路包括多种传递和调控机制。
最重要的是多重酶催化和转移过程。
这些酶催化和转移过程包括级联反应、交联反应、反应速率调节、分解反应等多个方面,其中包括激酶、磷酸酶、蛋白激酶等多种不同类型的酶催化系统。
组织特异性基因表达调控机制研究
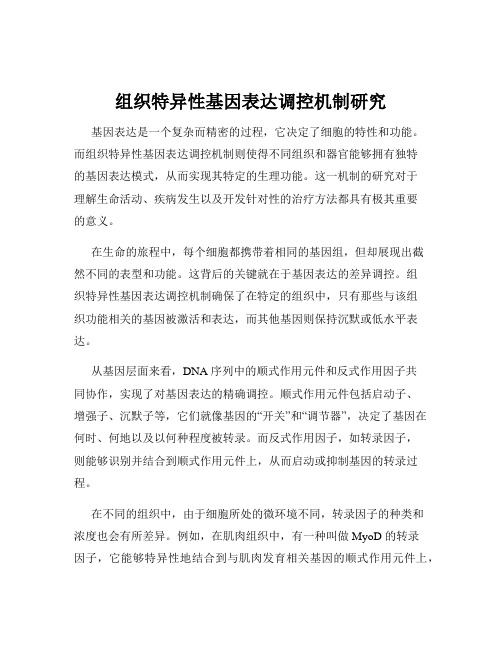
组织特异性基因表达调控机制研究基因表达是一个复杂而精密的过程,它决定了细胞的特性和功能。
而组织特异性基因表达调控机制则使得不同组织和器官能够拥有独特的基因表达模式,从而实现其特定的生理功能。
这一机制的研究对于理解生命活动、疾病发生以及开发针对性的治疗方法都具有极其重要的意义。
在生命的旅程中,每个细胞都携带着相同的基因组,但却展现出截然不同的表型和功能。
这背后的关键就在于基因表达的差异调控。
组织特异性基因表达调控机制确保了在特定的组织中,只有那些与该组织功能相关的基因被激活和表达,而其他基因则保持沉默或低水平表达。
从基因层面来看,DNA 序列中的顺式作用元件和反式作用因子共同协作,实现了对基因表达的精确调控。
顺式作用元件包括启动子、增强子、沉默子等,它们就像基因的“开关”和“调节器”,决定了基因在何时、何地以及以何种程度被转录。
而反式作用因子,如转录因子,则能够识别并结合到顺式作用元件上,从而启动或抑制基因的转录过程。
在不同的组织中,由于细胞所处的微环境不同,转录因子的种类和浓度也会有所差异。
例如,在肌肉组织中,有一种叫做 MyoD 的转录因子,它能够特异性地结合到与肌肉发育相关基因的顺式作用元件上,促进这些基因的表达,从而使得肌肉细胞能够合成特有的蛋白质,形成肌肉的结构和功能。
除了转录水平的调控,基因表达在转录后、翻译以及翻译后等多个层面也受到精细的调节。
在转录后阶段,RNA 的加工和修饰,如剪接、加帽和加尾等,会影响 mRNA 的稳定性和翻译效率。
不同组织中 RNA 加工酶的种类和活性不同,导致了相同的前体 mRNA 可以产生不同的成熟 mRNA 产物。
翻译水平的调控同样重要。
例如,某些组织中特有的 microRNA 可以与特定 mRNA 结合,抑制其翻译过程,从而降低相应蛋白质的合成量。
这种 microRNA 的表达模式在不同组织中存在差异,进一步增加了基因表达调控的复杂性和特异性。
在翻译后的修饰和加工过程中,蛋白质的折叠、磷酸化、甲基化、乙酰化等修饰方式也会影响其活性和功能。
《2024年人类基因组转录因子CTCF细胞特异性结合位点的预测》范文

《人类基因组转录因子CTCF细胞特异性结合位点的预测》篇一一、引言近年来,随着人类基因组学研究的深入发展,对于基因调控机制的研究已成为生物信息学领域的重要课题。
转录因子作为基因表达调控的关键因子,在细胞发育、分化以及疾病发生过程中发挥着重要作用。
CTCF(CCCTC结合因子)作为一种重要的转录因子,在多种细胞类型中具有广泛的调控作用。
本文旨在探讨人类基因组中CTCF细胞特异性结合位点的预测方法,为进一步研究其功能提供理论基础。
二、CTCF的概述CTCF是一种具有多种功能的转录因子,可与DNA上的特定序列结合,进而调控基因的表达。
其结合位点通常位于CCCTC重复序列周围,与多种生物学过程密切相关。
目前已有大量研究报道了CTCF在不同细胞类型中的表达模式及其与疾病的关系。
三、结合位点预测方法针对CTCF的细胞特异性结合位点预测,本文提出以下方法:1. 数据库资源利用:首先,收集并整合公开的转录因子数据库、基因组注释信息以及细胞特异性表达数据等资源。
这些资源为预测CTCF的结合位点提供了基础数据支持。
2. 序列分析:通过对已知的CTCF结合位点进行序列分析,找出其保守的序列特征,如CCCTC重复序列等。
同时,考虑不同细胞类型中CTCF的结合偏好,为后续预测提供依据。
3. 机器学习算法:采用机器学习算法对基因组序列进行训练和预测。
例如,利用支持向量机(SVM)等算法建立预测模型,将基因组序列作为输入特征,预测CTCF的结合位点。
4. 实验验证:对预测得到的结合位点进行实验验证,如ChIP-seq等实验技术,以确定其真实性。
通过实验验证的位点将用于进一步优化预测模型。
四、预测流程及结果分析1. 预测流程:首先,收集并整合相关数据库资源;然后,进行序列分析,提取保守序列特征和细胞特异性特征;接着,利用机器学习算法建立预测模型;最后,对预测结果进行实验验证和优化。
2. 结果分析:通过对比实验验证的位点与预测结果,评估预测模型的准确性和可靠性。
基因调控元件的识别和功能分析
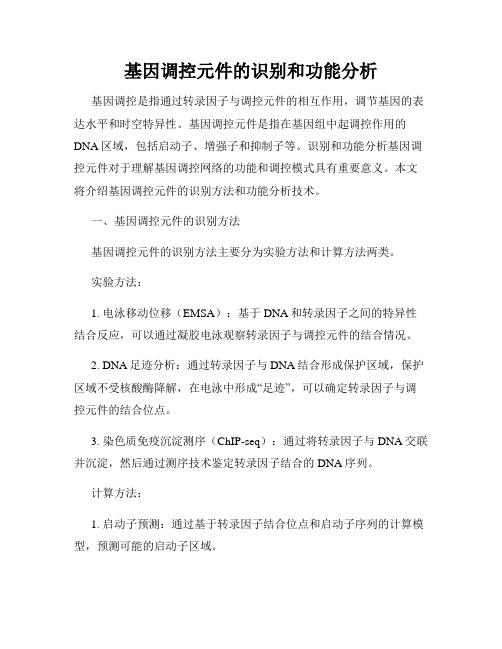
基因调控元件的识别和功能分析基因调控是指通过转录因子与调控元件的相互作用,调节基因的表达水平和时空特异性。
基因调控元件是指在基因组中起调控作用的DNA区域,包括启动子、增强子和抑制子等。
识别和功能分析基因调控元件对于理解基因调控网络的功能和调控模式具有重要意义。
本文将介绍基因调控元件的识别方法和功能分析技术。
一、基因调控元件的识别方法基因调控元件的识别方法主要分为实验方法和计算方法两类。
实验方法:1. 电泳移动位移(EMSA):基于DNA和转录因子之间的特异性结合反应,可以通过凝胶电泳观察转录因子与调控元件的结合情况。
2. DNA足迹分析:通过转录因子与DNA结合形成保护区域,保护区域不受核酸酶降解,在电泳中形成“足迹”,可以确定转录因子与调控元件的结合位点。
3. 染色质免疫沉淀测序(ChIP-seq):通过将转录因子与DNA交联并沉淀,然后通过测序技术鉴定转录因子结合的DNA序列。
计算方法:1. 启动子预测:通过基于转录因子结合位点和启动子序列的计算模型,预测可能的启动子区域。
2. DNA序列比对:通过比对不同物种或同一物种不同基因间的DNA序列,鉴定高度保守的区域,可能为调控元件。
3. 机器学习算法:利用大规模的实验数据和DNA序列特征,构建机器学习模型进行调控元件的预测和分类。
二、基因调控元件的功能分析基因调控元件的功能分析可以通过转录后修饰、突变和瞬时表达实验等方法进行。
1. 转录后修饰:通过测定某个调控元件的转录后修饰状态,如甲基化、乙酰化等,来评价其功能。
2. CRISPR/Cas9基因组编辑:利用CRISPR/Cas9技术对调控元件进行定点突变,观察突变对基因表达的影响,推断其功能。
3. 瞬时表达实验:构建含有调控元件启动子和荧光报告基因的重组质粒,转染细胞进行瞬时表达实验,观察报告基因的表达水平,以评价调控元件的功能。
以上是基因调控元件识别和功能分析的主要方法。
随着高通量测序技术和人工智能技术的不断发展,基因调控元件的识别和功能分析也在不断深入和完善。
组织特异性基因表达及其调控机制的研究

组织特异性基因表达及其调控机制的研究在生物学中,组织特异性基因表达是一个重要的研究领域。
基因的表达是指基因信息从DNA转录成RNA,再从RNA翻译成蛋白质的过程。
然而,不同细胞中基因的表达会有所不同,即基因表达呈现组织特异性。
研究组织特异性基因表达的机制可帮助我们更好地理解生物进化和发育的过程。
每个细胞都包含相同的DNA序列,但是不同基因的表达程度因细胞类型而异。
这个差异可以通过用高通量基序中分析方法如RNA测序来衡量。
对千名人类基因组计划(ENCODE)的研究表明,人类基因组中大约80%的区域可以被转录成RNA。
此外,超过90%的转录本并未对蛋白质翻译产生影响,而是在细胞中扮演一些非编码RNA(ncRNA)以上的功能。
组织特异性基因表达的产生是由于不同组织中不同的启动子和转录因子间存在的特异性调控区域。
启动子是存在于基因上游的DNA序列和靠近基因和内部区域的增强子,它们是决定基因表达的主要元素。
这些调节元件中的特定顺序和组合可以帮助细胞鉴别不同的生理和 / 或发育状态,并确定基因在这些状态下的表达模式。
转录因子是在特定细胞类型中表达的蛋白质,它们可以结合到基因组DNA上的启动子和增强子上,影响基因的转录。
它们在组织特异性基因表达中起着举足轻重的作用。
例如,发现AUG(ATF)家族的转录因子家族在不同的组织中有不同的表达模式,而它们的靶向基因群也不同。
此外,表观遗传调控也是组织特异性基因表达的重要机制。
表观遗传是影响基因表达的不同方式,而不影响基因序列本身。
它包括DNA甲基化,组蛋白乙酰化等化学修饰方式。
这些修饰过程可以影响染色质结构的可及性,进而影响基因的转录。
例如,甲基化模式的改变可能导致一些基因在某些细胞类型中沉默。
总之,组织特异性基因表达和其调控机制是生物学研究的关键问题和挑战。
随着基因表达分析and生物信息学技术的发展,我们可以更好地理解不同细胞类型之间基因的表达差异,从而为今后生物学研究提供更加有力的工具和研究方向。
细胞周期调控的分子机制及其在疾病中的作用
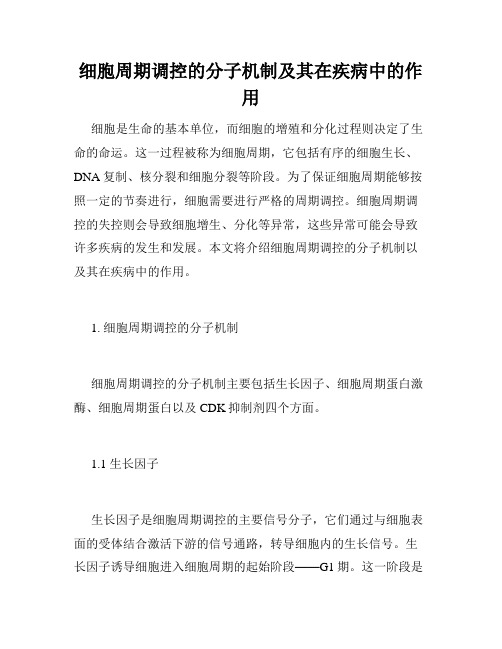
细胞周期调控的分子机制及其在疾病中的作用细胞是生命的基本单位,而细胞的增殖和分化过程则决定了生命的命运。
这一过程被称为细胞周期,它包括有序的细胞生长、DNA复制、核分裂和细胞分裂等阶段。
为了保证细胞周期能够按照一定的节奏进行,细胞需要进行严格的周期调控。
细胞周期调控的失控则会导致细胞增生、分化等异常,这些异常可能会导致许多疾病的发生和发展。
本文将介绍细胞周期调控的分子机制以及其在疾病中的作用。
1. 细胞周期调控的分子机制细胞周期调控的分子机制主要包括生长因子、细胞周期蛋白激酶、细胞周期蛋白以及CDK抑制剂四个方面。
1.1 生长因子生长因子是细胞周期调控的主要信号分子,它们通过与细胞表面的受体结合激活下游的信号通路,转导细胞内的生长信号。
生长因子诱导细胞进入细胞周期的起始阶段——G1期。
这一阶段是细胞生长和代谢发生变化的时期,细胞会对环境中的外界信号产生反应,准备进行DNA复制和分裂的后续步骤。
1.2 细胞周期蛋白激酶细胞周期蛋白激酶(Cyclin-dependent kinase, CDK)是细胞周期调控中的核心分子。
CDK主要由两个组成部分组成,一个是具有激酶活性的酶因子,一个是调控酶因子。
这两个组成部分可以通过与细胞周期蛋白(Cyclin)结合而激活其活性。
细胞内不同的Cyclin和CDK组合会对不同的细胞周期阶段进行调控,从而保证整个细胞周期的有序进行。
1.3 CDK抑制剂CDK抑制剂主要包括Cip/Kip家族和INK4家族两个家族。
它们通过结合CDK/Cyclin复合物的酶因子部分,抑制其酶活性,从而调控了细胞周期的进行。
不同成员的CDK抑制剂对不同的细胞周期蛋白复合物起着不同的作用,从而形成一个复杂的细胞周期调控网。
细胞周期的调控可以是正常的,也可以是异常的。
这一异常的调控过程被称为“细胞周期失控”。
接下来我们将探讨细胞周期失控在疾病中的作用。
2. 细胞周期失控在疾病中的作用细胞周期失控是导致细胞增殖、分化、肿瘤、衰老、免疫功能低下等多种病理过程的主要原因之一。
生物大数据技术如何分析细胞周期和细胞分化的调控网络
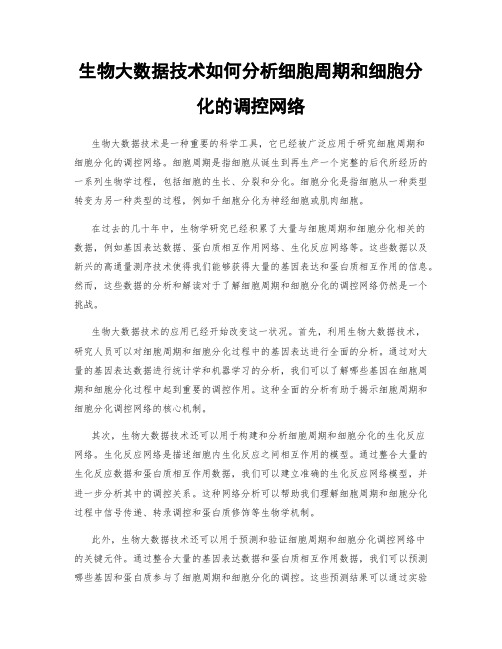
生物大数据技术如何分析细胞周期和细胞分化的调控网络生物大数据技术是一种重要的科学工具,它已经被广泛应用于研究细胞周期和细胞分化的调控网络。
细胞周期是指细胞从诞生到再生产一个完整的后代所经历的一系列生物学过程,包括细胞的生长、分裂和分化。
细胞分化是指细胞从一种类型转变为另一种类型的过程,例如干细胞分化为神经细胞或肌肉细胞。
在过去的几十年中,生物学研究已经积累了大量与细胞周期和细胞分化相关的数据,例如基因表达数据、蛋白质相互作用网络、生化反应网络等。
这些数据以及新兴的高通量测序技术使得我们能够获得大量的基因表达和蛋白质相互作用的信息。
然而,这些数据的分析和解读对于了解细胞周期和细胞分化的调控网络仍然是一个挑战。
生物大数据技术的应用已经开始改变这一状况。
首先,利用生物大数据技术,研究人员可以对细胞周期和细胞分化过程中的基因表达进行全面的分析。
通过对大量的基因表达数据进行统计学和机器学习的分析,我们可以了解哪些基因在细胞周期和细胞分化过程中起到重要的调控作用。
这种全面的分析有助于揭示细胞周期和细胞分化调控网络的核心机制。
其次,生物大数据技术还可以用于构建和分析细胞周期和细胞分化的生化反应网络。
生化反应网络是描述细胞内生化反应之间相互作用的模型。
通过整合大量的生化反应数据和蛋白质相互作用数据,我们可以建立准确的生化反应网络模型,并进一步分析其中的调控关系。
这种网络分析可以帮助我们理解细胞周期和细胞分化过程中信号传递、转录调控和蛋白质修饰等生物学机制。
此外,生物大数据技术还可以用于预测和验证细胞周期和细胞分化调控网络中的关键元件。
通过整合大量的基因表达数据和蛋白质相互作用数据,我们可以预测哪些基因和蛋白质参与了细胞周期和细胞分化的调控。
这些预测结果可以通过实验验证,并进一步优化调控网络模型。
这种预测和验证的策略可以加速我们对细胞周期和细胞分化调控网络的理解。
最后需要注意的是,尽管生物大数据技术为研究细胞周期和细胞分化调控网络提供了强大的工具,但仍然存在一些挑战。
组织特异性基因表达的分子机制研究
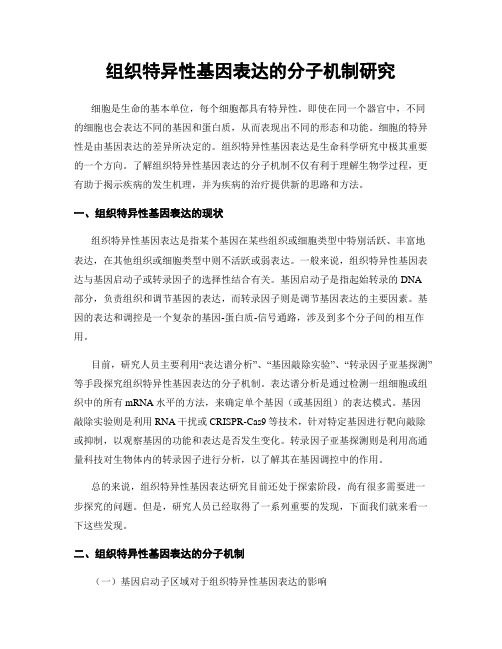
组织特异性基因表达的分子机制研究细胞是生命的基本单位,每个细胞都具有特异性。
即使在同一个器官中,不同的细胞也会表达不同的基因和蛋白质,从而表现出不同的形态和功能。
细胞的特异性是由基因表达的差异所决定的。
组织特异性基因表达是生命科学研究中极其重要的一个方向。
了解组织特异性基因表达的分子机制不仅有利于理解生物学过程,更有助于揭示疾病的发生机理,并为疾病的治疗提供新的思路和方法。
一、组织特异性基因表达的现状组织特异性基因表达是指某个基因在某些组织或细胞类型中特别活跃、丰富地表达,在其他组织或细胞类型中则不活跃或弱表达。
一般来说,组织特异性基因表达与基因启动子或转录因子的选择性结合有关。
基因启动子是指起始转录的DNA 部分,负责组织和调节基因的表达,而转录因子则是调节基因表达的主要因素。
基因的表达和调控是一个复杂的基因-蛋白质-信号通路,涉及到多个分子间的相互作用。
目前,研究人员主要利用“表达谱分析”、“基因敲除实验”、“转录因子亚基探测”等手段探究组织特异性基因表达的分子机制。
表达谱分析是通过检测一组细胞或组织中的所有mRNA水平的方法,来确定单个基因(或基因组)的表达模式。
基因敲除实验则是利用RNA干扰或CRISPR-Cas9等技术,针对特定基因进行靶向敲除或抑制,以观察基因的功能和表达是否发生变化。
转录因子亚基探测则是利用高通量科技对生物体内的转录因子进行分析,以了解其在基因调控中的作用。
总的来说,组织特异性基因表达研究目前还处于探索阶段,尚有很多需要进一步探究的问题。
但是,研究人员已经取得了一系列重要的发现,下面我们就来看一下这些发现。
二、组织特异性基因表达的分子机制(一)基因启动子区域对于组织特异性基因表达的影响基因启动子区域是启动转录的关键区域,通常包括转录起始位点(TSS)和调控元件(enhancer、silencer等)。
研究表明,基因启动子区域对于组织特异性基因表达的影响非常大。
其中,调控元件在组织特异性基因表达中发挥着重要作用。
生物学中的基因调控
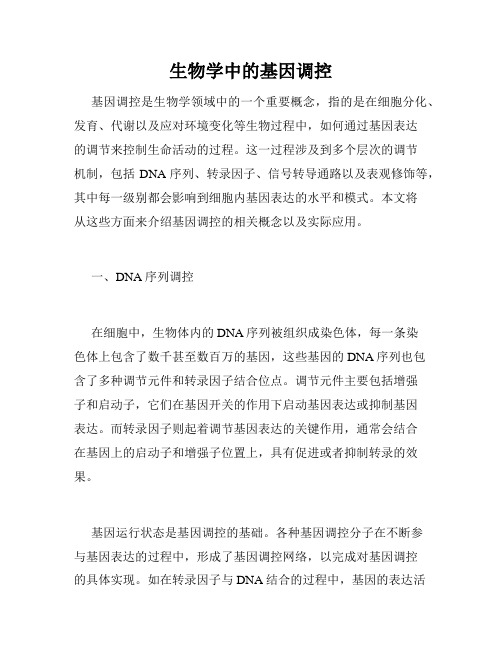
生物学中的基因调控基因调控是生物学领域中的一个重要概念,指的是在细胞分化、发育、代谢以及应对环境变化等生物过程中,如何通过基因表达的调节来控制生命活动的过程。
这一过程涉及到多个层次的调节机制,包括DNA序列、转录因子、信号转导通路以及表观修饰等,其中每一级别都会影响到细胞内基因表达的水平和模式。
本文将从这些方面来介绍基因调控的相关概念以及实际应用。
一、DNA序列调控在细胞中,生物体内的DNA序列被组织成染色体,每一条染色体上包含了数千甚至数百万的基因,这些基因的DNA序列也包含了多种调节元件和转录因子结合位点。
调节元件主要包括增强子和启动子,它们在基因开关的作用下启动基因表达或抑制基因表达。
而转录因子则起着调节基因表达的关键作用,通常会结合在基因上的启动子和增强子位置上,具有促进或者抑制转录的效果。
基因运行状态是基因调控的基础。
各种基因调控分子在不断参与基因表达的过程中,形成了基因调控网络,以完成对基因调控的具体实现。
如在转录因子与DNA结合的过程中,基因的表达活性等情况,都会影响到转录因子的结合效率。
而在序列区块的特定位置上的变异,也能够影响这一过程,以致对基因表达产生影响。
二、转录因子调控转录因子在基因调控中起着至关重要的作用。
不同的转录因子可以结合在基因启动子、增强子和靶基因等多个位置,通过控制基因转录而影响宿主细胞的命运。
从宏观的角度来看,不同种类的细胞具有不同的基因表达模式,这是因为不同类型的细胞在调节基因表达过程中,可结合的转录因子数量呈现巨大的差异。
这种现象被称为细胞类型特异性。
举个例子,人类胰岛素基因的转录因子是凝集素样转录因子-1(PDX1),PDX1在胰腺中高表达,在此多次与其启动子、增强子结合,并将胰岛素基因启动转录。
在转录过程中,PDX1还会参与到胰岛素分泌完全不同的销亡过程中,完成对胰腺特异调控的作用。
三、信号转导通路调控信号转导通路是指细胞收到外部信号后,通过不同的通路传递到核内,影响到基因表达的过程。
细胞通过空间和时间上的调控来实现蛋白质表达平衡如信号转导调控元件和蛋白质蛋白质相互作用等
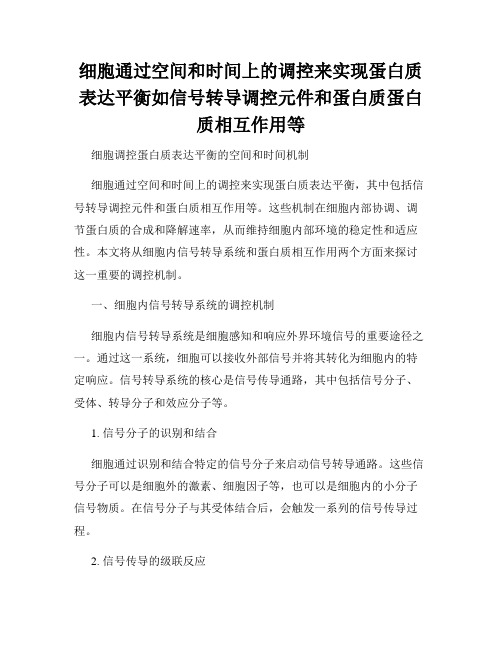
细胞通过空间和时间上的调控来实现蛋白质表达平衡如信号转导调控元件和蛋白质蛋白质相互作用等细胞调控蛋白质表达平衡的空间和时间机制细胞通过空间和时间上的调控来实现蛋白质表达平衡,其中包括信号转导调控元件和蛋白质相互作用等。
这些机制在细胞内部协调、调节蛋白质的合成和降解速率,从而维持细胞内部环境的稳定性和适应性。
本文将从细胞内信号转导系统和蛋白质相互作用两个方面来探讨这一重要的调控机制。
一、细胞内信号转导系统的调控机制细胞内信号转导系统是细胞感知和响应外界环境信号的重要途径之一。
通过这一系统,细胞可以接收外部信号并将其转化为细胞内的特定响应。
信号转导系统的核心是信号传导通路,其中包括信号分子、受体、转导分子和效应分子等。
1. 信号分子的识别和结合细胞通过识别和结合特定的信号分子来启动信号转导通路。
这些信号分子可以是细胞外的激素、细胞因子等,也可以是细胞内的小分子信号物质。
在信号分子与其受体结合后,会触发一系列的信号传导过程。
2. 信号传导的级联反应信号分子与受体的结合引发了一系列级联反应,通过不同的酶、激酶和磷酸酶的激活或抑制来调控下游信号分子的活性。
这些级联反应可以通过磷酸化、去磷酸化等方式来传递信号,最终将信号传递到效应分子上。
3. 信号转导调控元件的作用细胞内信号转导通路中存在着大量的调控元件,如激酶、磷酸酶、蛋白激酶等。
这些调控元件的活性受到多种因素的影响,如细胞内钙离子浓度、 pH 值、温度等。
通过调控这些元件的活性,细胞可以精确控制信号转导通路的开关和强度,从而实现蛋白质表达平衡的调控。
二、蛋白质相互作用的调控机制除了信号转导系统,蛋白质相互作用也是细胞调控蛋白质表达平衡的重要机制之一。
通过相互作用,蛋白质可以形成复合物,并参与到细胞的各种生物学过程中。
1. 蛋白质的组装和分解细胞中的蛋白质不仅可以通过合成来实现表达平衡,还可以通过组装和分解的方式进行调节。
蛋白质组装包括同类型蛋白质的组合以及与其他类型蛋白质的结合形成复合物。
细胞特异性基因表达调控机制

细胞特异性基因表达调控机制——解码生命进化密码在人们的常规认知中,同一个个体的所有细胞都拥有相同的基因,基因会发挥各种作用,从而控制着细胞的生命活动。
但事实上,不同细胞在同一基因的表达上存在着极大差异,也即某些基因只在特定类型的细胞中表达,而在其他细胞则处于沉默状态。
这就引出了一个极为重要的问题:。
一、基因表达调控的重要性基因表达是生命过程中的一个极其重要的环节,它决定了细胞的命运、个体特征的形成、以及物种进化的方向。
基因在细胞内以DNA形式存在,而细胞内许多物质都参与在基因表达的调控过程中,从而决定其中哪些基因会被激活,哪些会被沉默。
这个过程涉及多个调控层次的协调和交互,所以是一个极其复杂的系统。
二、基因表达调控机制的分级无论对哪种生物的基因表达调控系统,它都可以被分为三个层次的调控机制:1、转录前控制:指得是影响转录因子(TF)结合活性的模式,从而影响基因的转录水平。
2、转录过程调节:包括RNA剪切、RNA编辑以及RNA稳定性的控制等方面。
因为RNA是蛋白质的前体,所以对它的调控直接影响到蛋白质的表达水平。
3、转化后调控:因为不同组织、不同发育阶段以及不同时代的环境下,生物体对各种蛋白质的需求量是不同的,所以这个调控层次体现的是一种交互作用,以后天信号分子为主导,通过调控翻译后蛋白质的稳定性、修饰、分泌等来控制细胞内的蛋白质水平。
三、特异性基因表达的调控机制特异性基因表达是指某些基因只在特定类型的细胞中表达,而在其他细胞则处于沉默状态。
这种细胞特异性基因表达是生物分化、而非细胞分裂过程的结果。
含有同样基因的细胞。
与另外一种细胞不同的是,它们会利用关于局部控制信号的消息来决定哪些基因需要通过转录而表达。
这些消息的组合可以在不同细胞中产生不同的基因表达模式。
对于调控特异性家基因表达,影响因子主要来自两方面。
1、转录因子复合物的特异性一个转录因子复合物合成中包括的蛋白质种类、数量以及它们的相互作用不同。
细胞迁移调控因子的研究
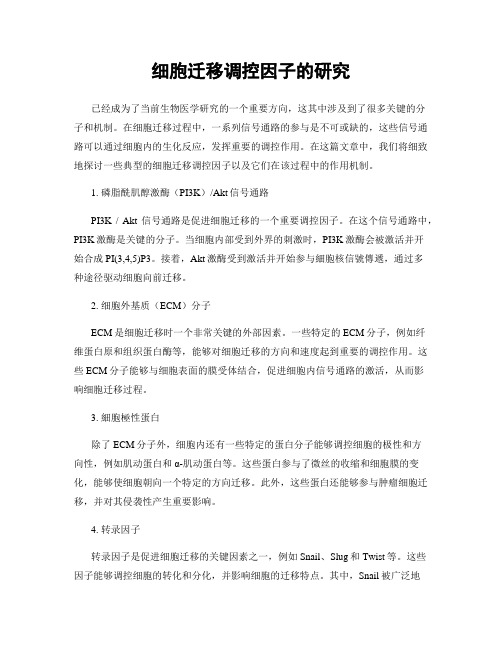
细胞迁移调控因子的研究已经成为了当前生物医学研究的一个重要方向,这其中涉及到了很多关键的分子和机制。
在细胞迁移过程中,一系列信号通路的参与是不可或缺的,这些信号通路可以通过细胞内的生化反应,发挥重要的调控作用。
在这篇文章中,我们将细致地探讨一些典型的细胞迁移调控因子以及它们在该过程中的作用机制。
1. 磷脂酰肌醇激酶(PI3K)/Akt信号通路PI3K / Akt信号通路是促进细胞迁移的一个重要调控因子。
在这个信号通路中,PI3K激酶是关键的分子。
当细胞内部受到外界的刺激时,PI3K激酶会被激活并开始合成PI(3,4,5)P3。
接着,Akt激酶受到激活并开始参与細胞核信號傳遞,通过多种途径驱动细胞向前迁移。
2. 细胞外基质(ECM)分子ECM是细胞迁移时一个非常关键的外部因素。
一些特定的ECM分子,例如纤维蛋白原和组织蛋白酶等,能够对细胞迁移的方向和速度起到重要的调控作用。
这些ECM分子能够与细胞表面的膜受体结合,促进细胞内信号通路的激活,从而影响细胞迁移过程。
3. 細胞極性蛋白除了ECM分子外,细胞内还有一些特定的蛋白分子能够调控细胞的极性和方向性,例如肌动蛋白和α-肌动蛋白等。
这些蛋白参与了微丝的收缩和细胞膜的变化,能够使细胞朝向一个特定的方向迁移。
此外,这些蛋白还能够参与肿瘤细胞迁移,并对其侵袭性产生重要影响。
4. 转录因子转录因子是促进细胞迁移的关键因素之一,例如Snail、Slug和Twist等。
这些因子能够调控细胞的转化和分化,并影响细胞的迁移特点。
其中,Snail被广泛地认为是肿瘤细胞侵袭的一个关键因素,它能够抑制E-cadherin的表达,进而促进细胞间转化和细胞间迁移。
5. 细胞间连接分子细胞间连接分子是参与细胞迁移的关键因素之一,包括E-cadherin、N-cadherin 和integrin等。
这些分子能够将细胞黏附在一起,从而影响细胞的迁移路线和速度。
一些研究表明,E-cadherin与其它进程存在着比较大的关系,如细胞的侵袭和瘤胚发生等。
分子生物学名词解释
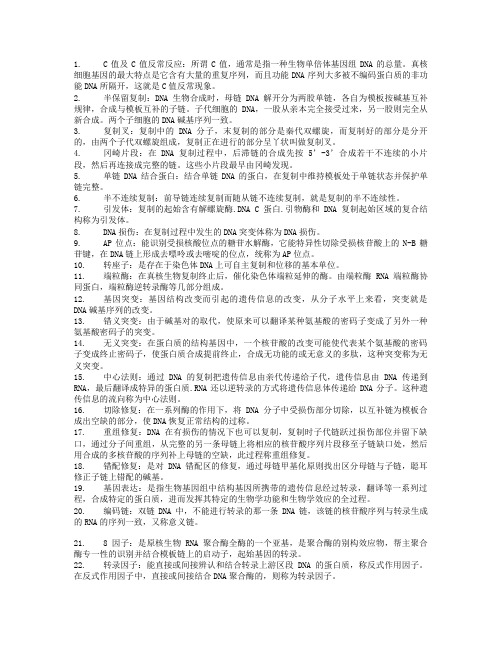
1. C值及C值反常反应:所谓C值,通常是指一种生物单倍体基因组DNA的总量。
真核细胞基因的最大特点是它含有大量的重复序列,而且功能DNA序列大多被不编码蛋白质的非功能DNA所隔开,这就是C值反常现象。
2. 半保留复制:DNA生物合成时,母链DNA解开分为两股单链,各自为模板按碱基互补规律,合成与模板互补的子链。
子代细胞的DNA,一股从亲本完全接受过来,另一股则完全从新合成。
两个子细胞的DNA碱基序列一致。
3. 复制叉:复制中的DNA分子,末复制的部分是秦代双螺旋,而复制好的部分是分开的,由两个子代双螺旋组成,复制正在进行的部分呈丫状叫做复制叉。
4. 冈崎片段:在DNA复制过程中,后滞链的合成先按5’-3’合成若干不连续的小片段,然后再连接成完整的链。
这些小片段最早由冈崎发现。
5. 单链DNA结合蛋白:结合单链DNA的蛋白,在复制中维持模板处于单链状态并保护单链完整。
6. 半不连续复制:前导链连续复制而随从链不连续复制,就是复制的半不连续性。
7. 引发体:复制的起始含有解螺旋酶.DNA C蛋白.引物酶和DNA复制起始区域的复合结构称为引发体。
8. DNA损伤:在复制过程中发生的DNA突变体称为DNA损伤。
9. AP位点:能识别受损核酸位点的糖苷水解酶,它能特异性切除受损核苷酸上的N-B糖苷键,在DNA链上形成去嘌呤或去嘧啶的位点,统称为AP位点。
10. 转座子:是存在于染色体DNA上可自主复制和位移的基本单位。
11. 端粒酶:在真核生物复制终止后,催化染色体端粒延伸的酶。
由端粒酶RNA端粒酶协同蛋白,端粒酶逆转录酶等几部分组成。
12. 基因突变:基因结构改变而引起的遗传信息的改变,从分子水平上来看,突变就是DNA碱基序列的改变。
13. 错义突变:由于碱基对的取代,使原来可以翻译某种氨基酸的密码子变成了另外一种氨基酸密码子的突变。
14. 无义突变:在蛋白质的结构基因中,一个核苷酸的改变可能使代表某个氨基酸的密码子变成终止密码子,使蛋白质合成提前终止,合成无功能的或无意义的多肽,这种突变称为无义突变。
高效的红细胞特异性表达系统的建立和优化

以红细胞特异性表达载体介导的携带蛋 白类药物的红细胞治疗以及非血液病基 因治疗奠定临床前的研究基础。 [ 关键词 ]慢病毒载体 ; 人凝 血 子I X; 红细胞特异性 ; 基因调控 [ 中图分 类号 ]Q 7 8 [ 文献标 志码 ]A [ 文章编号 ]2 0 9 5 — 3 0 9 7 ( 2 0 1 3 ) 0 来自 - 0 0 0 4 - 0 7
・
4・
£ 、
《 转化 医学杂志} 2 0 1 3年 2月 第 2卷 第 1 期
T r a n s l a t i o n a l Me d i c i n e J o u r n a l , V o 1 . 2 N o . 1 , F e b 2 0 1 3
喀
基础 研究
正常水平的 3 . 8 %( 1 9 1 n g / m 1 ) 。结论
通过适 当减少调控元件优化 慢病毒载体 不仅有助 于提 高携带 的外源
基 因片 段 长 度 、 增 加 载体 制 备 的有 效 性 、 实 现 温 和 提 高 目的 基 因 产 物 达 到 治疗 水 平 需求 量 的 目的 , 而 且 为 开 展
Ca n c e r I n s t i t u t e o f Yu n n a n P r o v i n c e , Yu n n a n K u n mi n g 6 5 0 1 l 8. C h i n a )
S ha n g h a i 2 0 0 43 3, Ch i n a;2. Ca n c e r I n s t i t u t e, t h e 3 r d Ho s p i t a l o f Ku n mi n g Me di c a l Co l l e g e.
基因转录调控中启动子元件的识别和预测
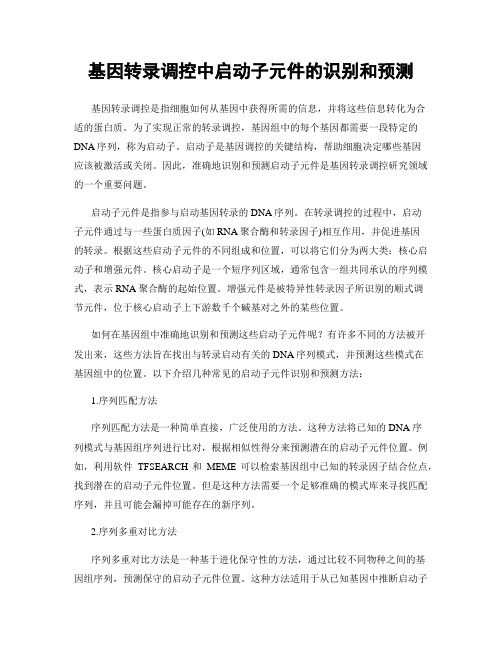
基因转录调控中启动子元件的识别和预测基因转录调控是指细胞如何从基因中获得所需的信息,并将这些信息转化为合适的蛋白质。
为了实现正常的转录调控,基因组中的每个基因都需要一段特定的DNA序列,称为启动子。
启动子是基因调控的关键结构,帮助细胞决定哪些基因应该被激活或关闭。
因此,准确地识别和预测启动子元件是基因转录调控研究领域的一个重要问题。
启动子元件是指参与启动基因转录的DNA序列。
在转录调控的过程中,启动子元件通过与一些蛋白质因子(如RNA聚合酶和转录因子)相互作用,并促进基因的转录。
根据这些启动子元件的不同组成和位置,可以将它们分为两大类:核心启动子和增强元件。
核心启动子是一个短序列区域,通常包含一组共同承认的序列模式,表示RNA聚合酶的起始位置。
增强元件是被特异性转录因子所识别的顺式调节元件,位于核心启动子上下游数千个碱基对之外的某些位置。
如何在基因组中准确地识别和预测这些启动子元件呢?有许多不同的方法被开发出来,这些方法旨在找出与转录启动有关的DNA序列模式,并预测这些模式在基因组中的位置。
以下介绍几种常见的启动子元件识别和预测方法:1.序列匹配方法序列匹配方法是一种简单直接,广泛使用的方法。
这种方法将已知的 DNA 序列模式与基因组序列进行比对,根据相似性得分来预测潜在的启动子元件位置。
例如,利用软件TFSEARCH和MEME可以检索基因组中已知的转录因子结合位点,找到潜在的启动子元件位置。
但是这种方法需要一个足够准确的模式库来寻找匹配序列,并且可能会漏掉可能存在的新序列。
2.序列多重对比方法序列多重对比方法是一种基于进化保守性的方法,通过比较不同物种之间的基因组序列,预测保守的启动子元件位置。
这种方法适用于从已知基因中推断启动子元件,但在新基因的情况下可能不太可行。
此外,该方法还需要完整基因组序列来进行多序列比对,鉴于基因组序列技术的限制,目前还无法得到许多物种的完整基因组序列。
3.机器学习方法机器学习方法是一种基于统计模型的方法,能够识别和预测未知的启动子元件。
组织特异性基因表达和调控

组织特异性基因表达和调控细胞是生命的基本单位,而基因则是细胞内最基本的遗传物质。
每一个细胞中都含有同样的遗传信息,但是在特定条件下,细胞会选择性地表达某些基因而抑制其他基因的表达。
这种组织特异性基因表达是由细胞内基因调控网络所控制的,其中包括DNA甲基化、染色质重构、转录调节因子和环境因素等因素。
一、DNA甲基化DNA甲基化是通过在DNA分子上添加甲基基团来调控基因表达的过程。
它主要是通过甲基化CpG位点的方式进行,这些位点通常位于CpG岛中。
CpG岛是一段长度为0.5-2kb的DNA序列,其中CpG的含量高于全基因组平均水平。
甲基化CpG位点通常与基因沉默相关,因为它们可以阻止转录因子结合到相应的顺式作用元件上。
尽管DNA甲基化功能多样,但它对于调控组织特异性基因表达的作用尤为重要。
举例而言,鳄鱼男性性决定基因DMRT1的启动子区域正常情况下都是甲基化的,这阻碍了该基因的转录。
而在鳄鱼雄性个体中,DMRT1启动子区域的甲基化程度下降,从而使得该基因获得了活性。
二、染色质重构染色体并不是一段静止不动的DNA序列,它们会通过染色质重构过程调控基因表达。
染色质的组分包括DNA、组蛋白以及非编码RNA等物质。
组蛋白是细胞质DNA包裹的主要蛋白质。
组蛋白代表性的修饰包括乙酰化、甲基化、磷酸化等。
组蛋白的修饰状态能够影响染色体的紧密程度,进而影响转录复合物的访问。
比如说,H3K27me3是一种组蛋白修饰,通常与基因沉默相关。
在小鼠胚胎干细胞中,大量的基因会产生这种修饰。
然而,当细胞开始分化成血细胞时,这个修饰状态逐渐减弱,导致被H3K27me3抑制的基因重新获得了转录能力。
三、转录调节因子转录调节因子是一类能够识别DNA序列并调控转录的蛋白质。
它们能够结合到DNA上,因而影响相应的基因表达。
转录调节因子和它们结合的顺义作用元件在细胞类型之间存在差异,因此它们能够控制组织特异性基因表达。
一个经典的例子是MYOD1基因在肌细胞中的表达。
细胞启动子及调控因子的研究
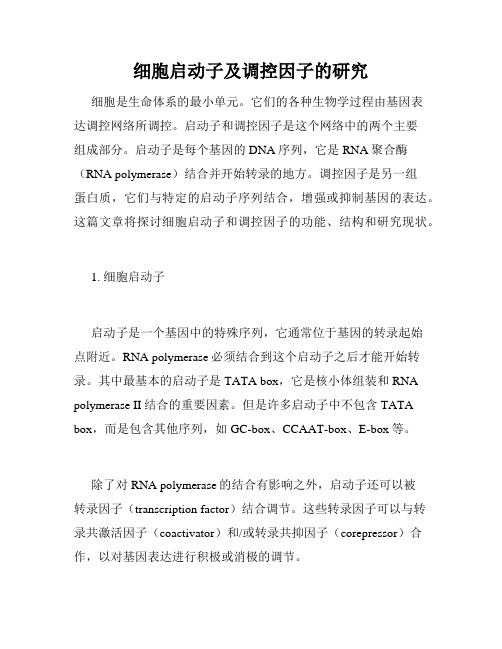
细胞启动子及调控因子的研究细胞是生命体系的最小单元。
它们的各种生物学过程由基因表达调控网络所调控。
启动子和调控因子是这个网络中的两个主要组成部分。
启动子是每个基因的DNA序列,它是RNA聚合酶(RNA polymerase)结合并开始转录的地方。
调控因子是另一组蛋白质,它们与特定的启动子序列结合,增强或抑制基因的表达。
这篇文章将探讨细胞启动子和调控因子的功能、结构和研究现状。
1. 细胞启动子启动子是一个基因中的特殊序列,它通常位于基因的转录起始点附近。
RNA polymerase必须结合到这个启动子之后才能开始转录。
其中最基本的启动子是TATA box,它是核小体组装和RNA polymerase II结合的重要因素。
但是许多启动子中不包含TATA box,而是包含其他序列,如GC-box、CCAAT-box、E-box等。
除了对RNA polymerase的结合有影响之外,启动子还可以被转录因子(transcription factor)结合调节。
这些转录因子可以与转录共激活因子(coactivator)和/或转录共抑因子(corepressor)合作,以对基因表达进行积极或消极的调节。
2. 细胞调控因子除了启动子外,调控因子也是影响基因表达的重要组成部分。
调控因子是一些蛋白质,它们与DNA序列特异性结合,并增强或抑制RNA polymerase II的转录活性。
它们通常分为激活因子和抑制因子。
激活因子能够绑定到特定的启动子区域,并激活RNA polymerase II的转录活性。
大多数激活因子具有DNA结合域和活化域。
DNA结合域与DNA序列的特定部分结合,而活化域与调控因子、转录共激活因子合作,增强RNA polymerase II的转录活性。
抑制因子则与激活因子相反,它们抑制RNA polymerase II的转录活性。
大多数抑制因子具有DNA结合域和抑制域。
DNA结合域与DNA序列的特定部分结合,而抑制域与调控因子、转录共抑因子合作,抑制RNA polymerase II的转录活性。
- 1、下载文档前请自行甄别文档内容的完整性,平台不提供额外的编辑、内容补充、找答案等附加服务。
- 2、"仅部分预览"的文档,不可在线预览部分如存在完整性等问题,可反馈申请退款(可完整预览的文档不适用该条件!)。
- 3、如文档侵犯您的权益,请联系客服反馈,我们会尽快为您处理(人工客服工作时间:9:00-18:30)。
Discovery of cell-type specific regulatory elements in the human genome using differential chromatin modification analysisChen Chen,Shihua Zhang*and Xiang-Sun ZhangNational Center for Mathematics and Interdisciplinary Sciences,Academy of Mathematics and Systems Science,Chinese Academy of Sciences,Beijing 100190,ChinaReceived January 4,2013;Revised July 18,2013;Accepted July 22,2013ABSTRACTChromatin modifications have been comprehen-sively illustrated to play important roles in gene regulation and cell diversity in recent years.Given the rapid accumulation of genome-wide chromatin modification maps across multiple cell types,there is an urgent need for computational methods to analyze multiple maps to reveal combinatorial modi-fication patterns and define functional DNA elements,especially those are specific to cell types or tissues.In this current study,we developed a computational method using differential chroma-tin modification analysis (dCMA)to identify cell-type-specific genomic regions with distinctive chromatin modifications.We then apply this method to a public data set with modification profiles of nine marks for nine cell types to evaluate its effectiveness.We found cell-type-specific elements unique to each cell type investigated.These unique features show signifi-cant cell-type-specific biological relevance and tend to be located within functional regulatory elements.These results demonstrate the power of a differential comparative epigenomic strategy in deciphering the human genome and characterizing cell specificity.INTRODUCTIONAll human cells share the same genetic information encoded by genomic DNA sequences,regardless of the cells’type.However,cells exhibit dramatically diverse phenotypes (1).In eukaryotic cells,genomic DNA is modulated by numerous chemical modifications,thus adding an extra layer of information to the genome sequence.These modifications enable genomic DNA to encode a vast and complex program of gene regulation (2–4),giving rise to diverse protein expression patterns and subsequent tissue-specific phenotypic diversity (5).Discovering functional elements and understanding how diverse modifications regulate these elements are central challenges to elucidate global gene regulation in humans.Cooperative binding of chromatin modifications,including histone modifications,DNA methylation and regulatory proteins shape the macro-environment of DNA and affect context-dependent interpretation of it.With the advent of chromatin immunoprecipitation coupled with tilling arrays (ChIP-chip)or parallel DNA sequencing (ChIP-seq),people have come genome-wide profiling of binding sites (6).More and more data sets are being generated for various chromatin features in multiple cell types,providing abundant resources for decoding chromatin modification patterns.One popular approach used to interpret epigenomic data is to identify and functionally characterize combina-torial patterns and systematically define DNA regulatory elements.For example,methylation of both lysine 4and lysine 27on histone H3is an epigenetic signature charac-teristic of embryonic stem cells,which keeps silenced developmental genes poised for activation (7,8).Another example is,by applying supervised regression framework,histone modification intensities around promoters were shown to be predictive for gene expression (3,9).Clustering approaches,used in early signature detection work,grouped well-annotated promoters on the basis of specific histone modification patterns (10).This research has recently been adopted into two data analysis plat-forms:seqMINER (11)and Cistrome (12).Hon et al.(13)developed a probabilistic method,ChromaSig,to identify histone modification signatures de novo that are repeated across the genome,without using any existing annotations.Jascheck and Tanay (14)proposed a spatial clustering algorithm to identify sets of common combina-torial modification patterns,defined over contiguous genomic regions.More recently,the hidden Markov*To whom correspondence should be addressed.Tel/Fax:+860162616670;Email:zsh@The authors wish it to be known that,in their opinion,the first two authors should be regarded as joint First Authors.Nucleic Acids Research,2013,1–13doi:10.1093/nar/gkt712ßThe Author(s)2013.Published by Oxford University Press.This is an Open Access article distributed under the terms of the Creative Commons Attribution Non-Commercial License (/licenses/by-nc/3.0/),which permits non-commercial re-use,distribution,and reproduction in any medium,provided the original work is properly cited.For commercial re-use,please contact journals.permissions@Nucleic Acids Research Advance Access published August 14, 2013 by guest on January 4, 2014/Downloaded frommodel (HMM)and Bayesian network approaches have been used to uncover recurrent chromatin states by seg-menting the epigenome into regions defined by character-istic histone mark combinations (15,16).In contrast to these clustering-type methods,Ucar et al.(17)developed a biclustering algorithm,CoSBI,to search combinatorial patterns involving only subsets of histone marks.Teng and Tan (18)further described a semi-supervised version of the CoSBI algorithm to incorporate existing knowledge of combinatorial patterns into the mining procedure.Although these methods facilitated the identification and characterization of chromatin modification patterns and functional genomic elements in the human genome,we are far from understanding the underlying modifica-tion patterns and biological mechanisms that dictate phenotypes of cells and tissues.In a recent pioneering study,Ernst et al.(19)applied the HMM method (15)to define chromatin states of nine cell types.Furthermore,cell-type-specific changes,which go toward elucidating cell-type specificities and predict regulatory mechanisms that drive gene regulation,were investigated.To date,cell-type-specific functional regions of the genome,which have key roles in cell diversity,have not been analyzed or defined directly.In this article,we aim to identify cell-type-specific regu-latory elements (CSREs)in the human genome,by d ifferential C hromatin M odification A nalysis (dCMA).To this end,we developed a computational framework capable of identifying genomic regions,containing dis-tinctive chromatin modifications that are unique to each cell type.We applied this method to the public ChIP-seq data set used in (19)to demonstrate its effectiveness.We identified CSREs for each cell type related to various genomic features,which showed significant,cell-type-spe-cific biological relevance and tended to be regulatory elements.Moreover,a large majority of CSREs are located in non-coding regions lacking annotations.These results can shed light on experimental human genome in-vestigations.The proposed framework demonstrates the power of a differential comparative epigenomics strategy in deciphering aspects of the human genome and characterizing cell specificity.MATERIALS AND METHODS MaterialsGenome-wide maps of nine chromatin marks and a control case in nine cell types have been generated using ChIP-seq in a recent study (19).These nine cell types include embryonic stem cells (H1ES or H1),erythrocytic leukemia cells (K562),B-lymphoblastoid cells (GM12878),hepatocellular carcinoma cells (HepG2),umbilical vein endothelial cells (HUVEC),skeletal muscle myoblasts (HSMM),normal lung fibroblasts (NHLF),normal epi-dermal keratinocytes (NHEK)and mammary epithelial cells (HMEC).In each cell type,nine chromatin marks consisting of CCCTC-binding factor (CTCF),H3K27me3,H3K36me3,H4K20me1,H3K4me1,H3K4me2,H3K4me3,H3K27ac and H3K9ac were profiled,and a whole-cell extract (WCE)sequencing wasconducted as control.RNA expression profiles of the nine cell types were also generated using Affymetrix GeneChip arrays.In this article,we downloaded these data for the following studies.The raw ChIP-seq data were preprocessed as previously described in (19).Whole-genome data were divided into non-overlapping 200bp bins.All sequencing reads were extended by 200bp in the 30direction,to capture actual binding sites and then assigned a unique 200bp bin accord-ing to their middle points.We had an integer for each bin,which summaries neighboring read counts.Next,the integers were binarized using a Poisson null model.Specifically,the null hypothesis of a ChIP-seq experiment is that all reads distribute uniformly across the genome;therefore,the mean of the Poisson distribution can be calculated by dividing the total read counts by the number of 200bp bins in the human genome.A threshold of 10À4was chosen to transform the integers into binary values.After binarization,we observed that many 200bp bins have no signals in all cell types,which may consist of sequences with low ‘mappability’(20).Those consecutive regions with length >10kb were removed from the genome (20.7%of the whole genome)in the analyses.Finally,we obtained nine binary matrices of size 10(nine chromatin marks and WCE)by N (the number of 200bp bins in human genome).Cytosolic RNA was isolated and quantified using Affymetrix GeneChip arrays (19).The raw data stored in CEL files were processed with RMA,and replicate expression values were averaged.The result-ing expression profiles were processed using quantile normalization (21)across the nine cell types.MethodsAfter data preprocessing,we obtained binary modification profiles for all cell types (Figure 1A).We introduce the following steps to identify CSREs.We have implemented this method and made a package called dCMA,which can be easily used for other researchers (Supplementary Methods ).Step 1:Calculation of the differential modification score.For each genomic position (a 200bp bin),hamming distance was used to measure differences of the modifica-tion characteristics between each pair of cell types (Figure 1B).Suppose there were K cell types and M chro-matin marks and the human genome was divided into N 200bp bins.Let b i ðc Þdenote the mark occurrence profile at genomic bin coordinate c in cell type i (a binary vector with M components).Then,the Differential Modification Score (DMS)for a particular cell type t at genomic coord-inate c was the summation of hamming distances between this cell type and others,which can be represented as DMS ðt ,c Þ¼P Ki ¼1HD ðb t ðc Þ,b i ðc ÞÞ,where HD represents the hamming distance operator.After these calculations,we had a DMS profile across the human genome in each cell type.Step 2:Normalization and correction of the DMSs .First,we normalized the sum of squares of each cell type’s DMS profile to a constant.We further calculated the Z -score of DMSs for each bin among all cell types:z ðt ,c Þ¼DMS ðt ,c ÞÀ ðc Þðc Þ,where ðc Þand ðc Þare the mean and 2Nucleic Acids Research,2013by guest on January 4, 2014/Downloaded fromthe standard deviation of the DMS at genomic position c ,respectively.The normalized DMS were then multiplied by the corresponding Z -scores to get the corrected scores.DS ðt ,c Þ¼DMS ðt ,c ÞÂz ðt ,c Þ(Figure 1C).The corrected scores reflect both the scale and relative size of the original scores.Step 3:Wavelet smoothing and CSRE extraction .The wavelet transform is a widely used filtering and smoothing technique,which has been comprehensively applied in computational biology (Figure 1D).It has been used in applications such as noise removal from microarray data (22)and normalization of diverse data types to a common scale in integrative analysis (23).We applied the maximal overlap discrete wavelet transform (24),a modification of discrete wavelet transform,to the corrected DMS profiles to further reduce noises and enhance the signal-to-noise ratio (Figure 1D).The genomic regions corresponding to smoothed DSs peaks in each cell type are defined as CSREs and can be directly extracted with given height and length parameters.Finally,the results with height =1.5and length =12were used to illustrate the strong biological relevance of CSREs.As to the selection of these two parameters,we performed enrichmentanalysis on CSREs identified from four other groups of parameter settings to show their robustness (Supplementary Methods ).Step 4:Measuring the statistical significance of CSREs.The null hypothesis in defining CSREs is chromatin marks occupy the 200bp bins with equal probability in all nine cell types.We estimated the probability of occurrence for the marks in each bin and simulate data under null hypothesis,according to the estimated probabilities.The summation of the DMS,within the CSRE,was chosen as the testing statistic,and then the right-sided probability in the null distribution (fitted by a Gaussian distribution)was computed as P -value.P -values were corrected for multiple hypothesis testing by Benjamini–Hochberg correction (25).Mapping CSRE bins to various genomic featuresThe CSREs were identified in a comparative manner,and we examined their functional potential roles by mapping them to known genomic features.All base pairs of the human genome were categorized into six classes:promoter,50UTR,exon,intron,30UTR andintergenic.A C BFigure 1.Illustration of the framework used in identifying CSREs.(A )The data profiles of nine cell types as characterized by nine marks and one control.The raw ChIP-seq reads were mapped to 200bp bins and the signals were binarized using a Poisson null model.(B )For each bin,the dissimilarity of the resulting binary vectors between two different cell types was measured using hamming distance.For each cell type,the DMS of a bin was the summation of pairwise hamming distance (sPHD)computed between it and other cell types.(C )The DMS profile of each cell type was normalized across the genome.Then,each column of the matrix was multiplied by the corresponding Z -scores to consider the variance in the column.(D )Wavelets smoothing strategy was adopted to smooth the resulting differential profile of each cell type.CSREs were extracted by selecting suitable height and length parameters.Statistical significance (P -value)of each CSRE was calculated by a non-parametric test.Nucleic Acids Research,20133by guest on January 4, 2014/Downloaded fromSpecifically,base pairs within 2kb from a known RefSeq transcription start site (TSS)were labeled as promoter,and those located further than 2kb from any RefSeq gene were labeled as intergenic.We purposefully defined intergenic regions in this loose fashion to allow us to cover the majority of the human genome using these six categories.The CSRE bins were then classified into six feature categories according to their bins’feature annota-tions,as described earlier in the text.The CSREs bins were classified in a hierarchical fashion,i.e.if the bins of a CSRE belong to more than one features,it was processed according to the order:promoter >50UTR >30UTR >exon >intron >intergenic.For each kind of genomic region,the corresponding proportion in CSREs was divided by the genome-wide proportion to determine the fold enrichment.The statistical significance was evaluated with Fisher’s exact test.Overlap analysis of the CSREs between cell types To test whether the CSREs of each cell type are cell-type specific,we calculate the overlap of CSREs between any two cell types.Two CSREs A and B are defined to beoverlapped if length ðA \B Þmin ðlength ðA Þ,length ðB ÞÞ>0:1,where length (X )rep-resents the number of bins in fragment X .Then,for each pair of cell types,namely,i and j ,we can determine the number of CSREs in cell type i overlapped by those in cell type j .To test the statistical significance of the overlap,Fisher’s exact tests were applied (left-sided and right-sided P -values for significant less and more overlaps,respectively).Enrichment analysisTo investigate the biological relevance of CSREs,we conducted housekeeping gene,biological function and network enrichment analysis.CSREs were mapped to RefSeq genes first (version hg18,downloaded from UCSC Genome Browser).Specifically,a CSRE was mapped to a gene when it overlaps the gene region.Here,the gene region starts from the TSS and ends at the transcription end site.We call the overlapping gene as the neighboring gene of this CSRE.To assess whether the CSREs are cell-type-specific regu-latory elements,we calculated the overlap between CSRE neighboring genes and housekeeping genes.We down-loaded the housekeeping gene list generated in (26),and 3218genes were obtained after mapping to the RefSeq gene list (version hg18).Fold enrichments of the overlaps were obtained,and the corresponding statistical significance was evaluated using Fisher’s exact test.Gene Ontology (GO)terms enrichment analysis was performed using STEM (27)where Fisher’s exact test was used and the Bonferroni corrected q -values were reported.For each cell type,the top five enriched GO terms associating 5–500genes were selected (Figure 3E).A human protein–protein interaction network consisting of 13207proteins and 64549interactions was downloaded from the BioGRID website (28).For each cell type,a sub-network was determined by mapping CSRE neighboring genes to the protein–protein interaction,and we let m be the number of interactions observed therein.The expectednumber of interactions in the sub-network wasEI ¼n 2 M N2,where N and n are the numbers of nodes in the whole network and the sub-network,respect-ively,and M is the number of observed interactions in thewhole network.The fold enrichment was mEI .The statistical significance of the fold enrichment was calculated using right-sided Fisher’s exact test.Relationship between CSREs and disease-associated variantsThe NHGRI’s collection of trait/disease-associated SNPs from published genome-wide association studies were downloaded from UCSC genome browser (July 12,2012)(29,30).There were 7899SNPs associated with 575traits in total.For each cell type,we extract a subset of SNPs corresponding to CSREs.The traits of the set of SNPs are used to investigate their characteristics.We aimed to investigate the relevance between the selected SNPs’traits and each cell type,based on the identified CSREs.To this end,we tested whether SNPs associated with a trait are significantly located in CSREs of a cell type through the Fisher’s exact test.P -values were cor-rected for multiple hypotheses testing by Benjamini–Hochberg correction.Relationship between CSREs and DNase I hypersensitive and EP300binding sitesDNase I hypersensitive sites (DHSs)peaks in eight of the nine studied cell types (except HepG2)and EP300binding peaks for three cell types (H1ES,GM12878and HepG2)were obtained from the UCSC browser ().Cell-type specific peaks are defined by filtering out those peaks appearing in other cell types.Considering the chromatin modifications happened on nucleosomes,while the DHSs and transcrip-tion factor binding sites are usually located in nucleosome depleted regions,we extended these cell-type-specific peaks by 2.5kb in each direction to their upstream and downstream,respectively.(We have also tested this with a more stringent criterion and get similar results,see Supplementary Methods and Supplementary Figure S1.)CSREs that overlapped,by at least 1bp,the extended peaks were considered as DHS or EP300proximal.For genome-wide background,we randomly selected 1000sets of CSREs for each cell type with length and chromosome attributes reserved and calculated the corresponding number of DHS or EP300proximal ones.One-sample Wilcoxon test was used to evaluate the statistical signifi-cance of the real number.RESULTSWe have developed a multi-stage method to identify CSREs,which are likely to be cell-selective regulatory regions (Supplementary Table S1).We applied our method to the data set generated in (19)for nine cell types.Analysis of these data sets defined,on average,4Nucleic Acids Research,2013by guest on January 4, 2014/Downloaded from4110.8CSREs per cell type(ranging from1701in NHLF to6659in GM12878;Supplementary Figure S2A) spanning an average0:53%of the genome.On average, 92%of CSREs in each cell type are statistical significant with q<0.0001.The median lengths of CSREs across the nine cell types was similar($3Kb),except two of them (K562and GM12878)are slightly longer than the others (Supplementary Figure S2B).The total numbers of base pairs,found in cell-type specific CSREs,varies from$7.5 (NHLF)to28.5Mb(GM12878)(Supplementary Figure S2C).The number of genes near to CSREs also varies, from$1546(NHLF)to4907(GM12878)(Supplementary Figure S2D).These diverse distributions may imply the functional complexity of these cell types(Supplementary Figure S2).CSREs relate to various genomic featuresWe explored the relations between CSREs and various genomic features to illustrate their potential functional roles.The proportion of CSREs in different genomic regions varied across the cell types(Figure2A).The CSREs were significantly enriched at well-known regula-tory regions,such as promoters,50and30UTRs (P<0:0001,Fisher’s exact tests)(Figure2B).Exon and intron regions were also enriched in CSREs,suggesting that part of a gene body may serve as regulatory elements,such as enhancers,for its own expression(31). The strong enrichments of CSREs in promoter regions (P<0:0001,Fisher’s exact tests)demonstrates underlying modifications acting in promoter regions play critical roles in regulating gene expression.In particular,CSREs in the promoter regions of H1ES cells were substantially enriched when compared to those found in the other cell types.These results imply more promoter regions are under epigenetic regulation in embryonic stem cells.As discussed later in the text,many promoters in H1ES are marked by H3K27me3,a repressive chromatin modifica-tion,but these regions are tuned in poised status.These characteristics are consistent with the unique cellular context of pluripotent cells.Although CSREs were not enriched in intergenic regions,this group constitute$36.4%(averaged across the nine cell types)of total CSREs.Moreover,CSREs in intronic regions made up$25.5%(averaged across the nine cell types)of the total CSREs.These results highlight the potential regulatory roles of non-coding regions and, also,the power of a comparative epigenetics strategy in investigating functional roles of the human genome. Intuitively,we hypothesized most of the CSREs target their neighboring genes.The distances are significantly shorter than those found in the genomic background, which,to some extent,verifies our assumption (Figure2C and Supplementary Figure S3).We further explored the distribution of CSREs in all23 chromosomes(1–22,X)in each cell type(Figure2D). Specifically,we calculated the normalized proportion of CSREs,with respect to chromosome length,for each cell type.We used the coefficient of variation(CV)to quantify the dispersion of the proportions and found that the CVs of two cancer cells(K562and HepG2)are apparently larger than those of the others(Figure2E).We observedsignificantly more CSREs in K562’s chromosome22andin HepG2’s chromosomes16,17and20.Interestingly,a reciprocal translocation between chromosome9and22in leukemia cells(32)has been well studied.The transloca-tion results in the oncogenic BCR-ABL gene fusion,whichis a highly sensitive marker for leukemia(33).For HepG2, chromosome translocations involving chromosomes16and17have been observed with spectral karyotyping. Moreover,chromosomes2,14and20were found to beamplified(34).These previous studies confirm the inform-ative nature of CSREs.In this case,it is non-uniform dis-tribution of CSREs,in K562and HepG2cells.These observations demonstrate that the CSREs defined by chromatin modifications may relate to the structural abnormity of chromatin,which contributes to cancer pro-gression,as well as other disorders.In total,we identified34721distinct CSREs in the human genome(collectively spanning 4.62%),most ofwhich(94.1%)were specific to a single cell type with only5.9%being found in two or more cell types(Figure3A). Next,we calculated the number of overlapping CSREs between cell types(Figure3B).As expected,most pairsof cell types exhibit significantly less overlaps(P<0:0001,left-sided Fisher’s exact tests).In contrast,significant overlaps(P<0:0001,Fisher’s exact tests)of CSREs occurred between NHEK and HMEC cell lines.This observation is not surprising,given NHEK are keratinizing epithelial cells,and their functions are more similar with HMEC than other cell types.We observed protein products of genes near to CSREstend to interact with each other in each cell type(P<0:0001,Fisher’s exact tests)(Figure3C and Supplementary Figure S4).This result suggests genesnear CSREs are more likely to work in a cooperative fashion to carry out biological functions.We also analyzed any overlaps between CSRE neighboring genesin each cell type and housekeeping genes.As expected,inseven cell types,CSRE neighboring genes tend not to be housekeeping genes(P<0:054)(Figure3D and Supplementary Figure S5).This observation implies genes near to CSREs are likely to execute cell-type-specific biological functions.CSREs are defined as genomic regions exhibiting dis-tinctive modification patterns,relative to those in othercell types.It is a reasonable assumption that these CSRE neighboring genes are involved in cell-type-specific biological processes.We mapped CSREs to RefSeq genes,and a gene set was obtained for each cell ing GO enrichment analysis,we found overrepresented GO termswere highly relevant to the functions of corresponding cell types,showing distinct cell-type specificity(Figure3E and Supplementary Tables S2–S5).For example,terms relatedto development such as‘brain development’and‘regula-tion of nervous system development’are enriched in embryonic stem cells(H1ES);terms related to immune response such as‘lymphocyte activation’and‘immune response-regulating signaling pathway’are enriched inB-lymphoblastoid cells(GM12878).As shown in the overlap analysis,NHEK and HMEC are highly relatedcell types;this is also seen in the functional enrichmentNucleic Acids Research,20135by guest on January 4, 2014/Downloaded fromanalysis data.Functional categories enriched in NHEK are likely to be enriched in HMEC.These results suggest that CSREs act in the regulation of cell-type-specific bio-logical processes,which highlights the role of chromatin modifications in controlling and maintaining differential cell-type gene expression patterns.Numerous genome-wide association studies have provided a plethora of links between commongeneticFigure 2.Relationship between CSREs and various genomic features.(A )The distribution of CSREs in six different genomic regions,including promoter,50UTR,30UTR,exon,intron and intergenic regions.(B )The fold enrichments of the CSREs in the six different genomic regions.(C )Box plot of the distance between the intergenic CSREs and the nearest TSSs,compared with those of randomly generated ones.For each intergenic CSRE,the random one was an arbitrarily selected genomic element from the same chromosome with the same length.Then,the distances between the random regions to their nearest TSS were computed.(D )The normalized proportion of CSREs in each chromosome (1–22,X)in all cell types.(The bar of chromosome 22in K562was truncated to 0.03for visualization,and its real number is 0.056)(E )Bar plot of the CV (defined as the ratio of the standard deviation to the mean)of normalized proportion of CSREs in each cell type.6Nucleic Acids Research,2013by guest on January 4, 2014/Downloaded from。