机器学习个人笔记完整版v5.3.pdf
NUC130中文手册
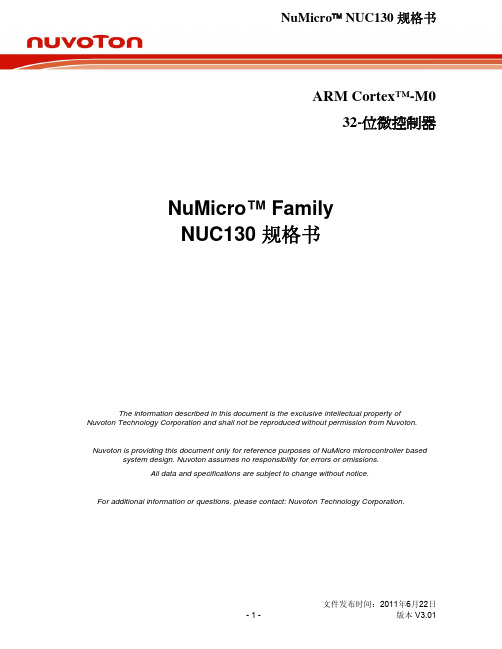
3
编号信息列表及管脚名称定义 ................................................................................................... 12
3.1 NuMicro™ NUC130产品选型指南 ................................................................................. 12
3.2.1 NuMicro™ NUC130管脚图 ..............................................................................................13
3.3 管脚功能描述 ................................................................................................................ 16
5.5 I2C 串行接口控制器 (Master/Slave) (I2C)...................................................................... 44
5.5.1 概述.................................................................................................................................44 5.5.2 特征.................................................................................................................................45
激光切割机器人分拣

激光切割工业机器人分拣系统1 / 111目录摘要: (4)关键词: (4)2概述 (5)2.1激光切割简述 (5)2.1.1定义 (5)2.1.2简介 (5)2.1.3原理 (5)2.1.4分类 (5)2.1.5特点 (6)2.2机器视觉引导与定位 (7)2.2.1什么是机器视觉 (7)2.2.2什么是视觉引导 (7)2.2.3定位 (7)3机器人分拣系统在激光切割设备上的应用 (8)3.1自动化系统的组成 (8)3.1.1工业机器人 (8)3.1.2相机 (8)3.1.3磁铁矩阵夹爪 (9)3.2自动化系统的工作原理 (10)3.2.13D扫描相机的工作原理 (10)3.2.2机器人的工作原理 (10)3.3工业机器人在该系统中的调试技巧 (11)3.3.1工具中心点的设定(tool center point) (11)3.3.2机器人路径规划 (11)2 / 113.3.3机器人IO设定 (11)3.4总结 (11)3 / 11摘要:中国劳动力价格优势已经逐渐失去,劳动密集型企业升级迫在眉睫。
随着技术改革步伐的加快,自动化的水平不断提到,机器人换人的呼声也越来越高。
激光切割在金属板材切割领域受到了越来越广泛的关注,是一种利用激光束聚焦后的高能量密度实现板材切割的加工方式,在电气制造、汽车、仪表开关、纺织机械、输机械、家电制造、电梯设备制造、食品工业等多个领域都具有较大的市场需求。
高能量密度的激光束使得板材迅速汽化蒸发形成孔洞、随着光束与材料相对线性移动、使孔洞连续形成宽度很窄的切缝,具备热变形小、切口整齐、加工精度高等特点。
通过计算机编程,激光切割专机或机器人能够实现复杂曲线的数控切割,具备较高的精确度和柔性,且切割过程自动化程度高,通过计算机程序的优化,能够对复杂切割图形进行排版,尽可能利用材料,节约成本。
关键词:激光切割、机器人换人、自动化生产线4 / 112概述2.1激光切割简述2.1.1定义利用高功率密度激光束照射被切割材料,使材料很快被加热至汽化温度,蒸发形成孔洞,随着光束对材料的移动,孔洞连续形成宽度很窄的(如0.1mm左右)切缝,完成对材料的切割。
TICRA 系列软件介绍_未尔科技
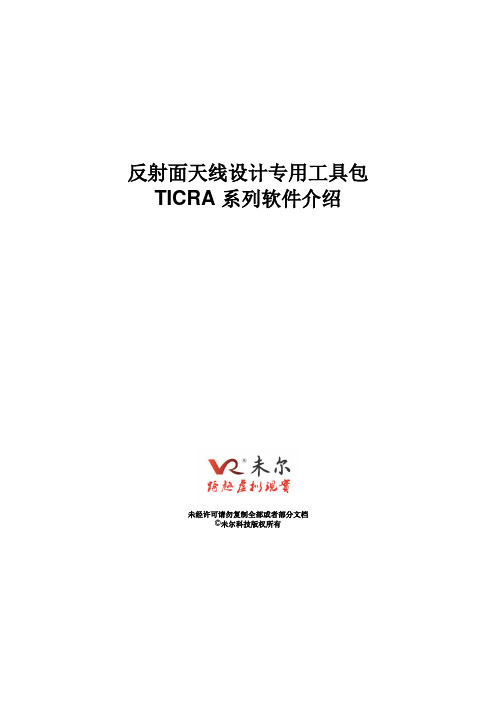
通用反射面天线软件包 GRASP 介绍 ....................................................................................... 3 2.1 产品概述 ........................................................................................................................... 3 2.2 功能应用 ........................................................................................................................... 3 功能概述 ..................................................................................................................... 3 2.2.1 2.2.2 参考案例 ..................................................................................................................... 3 2.3 功能特点 ........................................................................................................................... 6 2.3.1 适用于复杂结构体分析 ............................................................................................... 6 2.3.2 可视化图形界面 .......................................................................................................... 6 2.3.3 支持多种反射面类型 ................................................................................................... 7 2.3.4 独立的反射体边缘定义 ............................................................................................... 8 2.3.5 丰富的内置馈源模型 ................................................................................................... 8 2.3.6 支持多种表面材料特性 ............................................................................................... 8 2.3.7 支持支架对天线性能影响的分析 ................................................................................. 9 2.3.8 灵活的分析模式 .......................................................................................................... 9 2.3.9 友好的用户界面 .......................................................................................................... 9 2.4 附加模块 ......................................................................................................................... 10 2.4.1 Multi-Reflector GTD ................................................................................................. 10 2.4.2 COUPLING .............................................................................................................. 10 2.4.3 MOM ........................................................................................................................ 11 2.4.4 QUAST ..................................................................................................................... 11 2.4.5 MPI........................................................................................................................... 13
先进的PID控制

北京化工大学本科毕业论文题目:基于遗传算法整定的PID控制院系:专业:电气工程及其自动化班级:________ ____ _ _ _____ 学生姓名:____________ ________ _____ 执导老师:___________ ______________ ______论文提交日期:年月日论文答辩日期:年月日摘要PID控制器是在工业过程控制中常见的一种控制器,因此,PID参数整定与优化一直是自动控制领域研究的重要问题。
遗传算法是一种具有极高鲁棒性的全局优化方法,在自控领域得到广泛的应用。
针对传统PID 参数整定的困难性,本文提出了把遗传算法运用于PID参数整定中。
本文首先对PID控制的原理和PID参数整定的方法做了简要的介绍。
其次介绍了遗传算法的原理、特点和应用。
再次,本文结合实例阐述了基于遗传算法的PID参数优化方法,采用误差绝对值时间积分性能指标作为参数选择的最小目标函数,利用遗传算法的全局搜索能力,使得在无须先验知识的情况下实现对全局最优解的寻优,以降低PID参数整定的难度,达到总体上提高系统的控制精度和鲁棒性的目的。
最后,本文针对遗传算法收敛速度慢、易早熟等缺点,将传统的赌盘选择法与最优保存策略结合起来,并采用改进的自适应交叉算子和自适应变异算子对PID参数进行迭代寻优整定。
采用MATLAB对上述算法进行仿真验证,仿真结果表明了遗传算法对PID参数整定的有效性。
关键词:PID;参数控制;遗传算法;MATLABAbstractPID controller is a kind of controller that is usual in industrial process control. Therefore, tuning and optimization of PID parameters are important researchable problems in the automatic control field, where Genetic algorithm is widely used because of the highly robust global optimization ability of it. Aiming at the difficulty of traditional tuning of PID parameter, this paper puts forward a method that genetic algorithm is applied to the tuning of PID parameters.Firstly, the principle of PID control and the methods of tuning of PID parameters are introduced briefly. Secondly, this paper introduces the principle, characteristics and application of genetic algorithm. Thirdly, this article expounds on the methods of tuning of PID parameters based on genetic algorithm with an example. In this paper, the performance index of time integral of absolute error serves as the minimum objective function in the tuning of PID parameters, and the global search ability of genetic algorithm is used, so the global optimal solution is obtained without prior knowledge, and the difficulty of tuning of PID parameter is reduced, so the goal is achieved which is improving the control accuracy and robustness of the system overall. Finally, aiming at the weakness of genetic algorithm, such as the slow convergence of prematurity and precocious, the traditional gambling site selection method and elitist model are united in this paper, and the paper alsoadopted adaptive crossover operator and adaptive mutation operator to optimize PID parameters iteratively.Use MATLAB to simulate these algorithms, and the simulation results show that PID controller tuning based on genetic algorithm is effective.Keywords: Genetic algorithm; PID control; optimum; MATLAB目录第一章引言 (1)1.1 课题研究的背景及意义 (1)1.2 PID控制的发展与现状 (1)1.3 本文研究的内容 (2)第二章PID控制 (4)2.1 PID控制原理 (4)2.2 常规PID参数整定方法 (6)2.2.1 Ziegler-Nichols整定方法 (6)2.2.2 改进的Ziegler-Nichols整定方法 (8)2.2.3 ISTE最优设定方法的经验公式 (9)2.2.4 Haalman法的计算公式 (10)2.2.5 KT整定法 (11)第三章基于遗传算法整定的PID控制 (13)3.1 遗传算法基本原理 (13)3.1.1 遗传算法概要 (13)3.1.2 遗传算法的应用步骤 (14)3.2 遗传算法的实现 (15)3.2.1 编码方法 (15)3.2.2 适应度函数 (16)3.2.3 选择算子 (17)3.2.4 交叉算子 (17)3.2.5 变异算子 (18)3.2.6 遗传算法控制参数选取 (19)3.3 遗传算法的仿真验证 (20)3.2.6遗传算法中关键参数的确定 (23)3.3 遗传算法的主要步骤 (23)3.3.1 准备工作 (23)3.3.2 基本遗传算法的步骤 (24)3.4遗传算法PID参数整定的编程实现 (24)3.4.1初始群体 (24)3.4.2 编码 (25)3.4.3 基本操作算子 (26)3.4.4 目标函数 (29)3.4.5 画图 (29)第四章PID整定方法的仿真应用 (31)4.1 一阶对象 (31)4.2 二阶对象 (32)4.3 三阶对象 (34)第五章结论 (37)参考文献 (38)致谢 (40)第一章引言1.1 课题研究的背景及意义PID(p一proportion,I一Integral,D一Differentia)控制是比例、积分、微分控制的简称PID[l]。
[鱼书笔记]深度学习入门:基于Python的理论与实现个人笔记分享
![[鱼书笔记]深度学习入门:基于Python的理论与实现个人笔记分享](https://img.taocdn.com/s3/m/0157d41d77c66137ee06eff9aef8941ea76e4be7.png)
[鱼书笔记]深度学习⼊门:基于Python的理论与实现个⼈笔记分享为了完成毕设, 最近开始⼊门深度学习.在此和⼤家分享⼀下本⼈阅读鱼书时的笔记,若有遗漏,欢迎斧正!若转载请注明出处!⼀、感知机感知机(perceptron)接收多个输⼊信号,输出⼀个信号。
如图感知机,其接受两个输⼊信号。
其中θ为阈值,超过阈值神经元就会被激活。
感知机的局限性在于,它只能表⽰由⼀条直线分割的空间,即线性空间。
多层感知机可以实现复杂功能。
⼆、神经⽹络神经⽹络由三部分组成:输⼊层、隐藏层、输出层1. 激活函数激活函数将输⼊信号的总和转换为输出信号,相当于对计算结果进⾏简单筛选和处理。
如图所⽰的激活函数为阶跃函数。
1) sigmoid 函数sigmoid函数是常⽤的神经⽹络激活函数。
其公式为:h(x)=11+e−x如图所⽰,其输出值在 0到 1 之间。
2) ReLU 函数ReLU(Rectified Linear Unit)函数是最近常⽤的激活函数。
3) tanh 函数2. 三层神经⽹络的实现该神经⽹络包括:输⼊层、2 个隐藏层和输出层。
def forward(network, x): # x为输⼊数据# 第1个隐藏层的处理,点乘加上偏置后传⾄激活函数a1 = np.dot(x, W1) + b1z1 = sigmoid(a1)# 第2个隐藏层的处理a2 = np.dot(z1, W2) + b2z2 = sigmoid(a2)#输出层处理 identidy_function原模原样输出a3a3 = np.dot(z2, W3) + b3y = identify_function(a3)return y # y为最终结果3. 输出层激活函数⼀般来说,回归问题选择恒等函数,分类问题选择softmax函数。
softmax函数的公式:y k=e a k ∑n i=1e a i假设输出层有n个神经元,计算第k个神经元的输出y k。
Enfocus_PitStop_pro_11_中文版用户指南
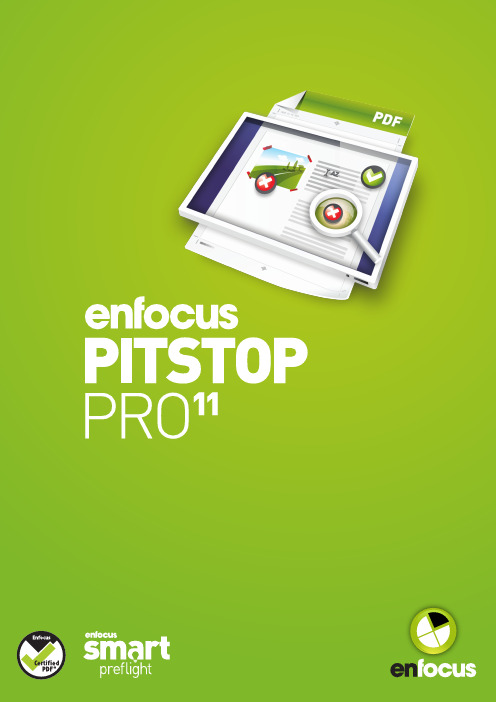
2.2 设置Enfocus PitStop Pro首选项..........................................................................14 PitStop Pro和StatusCheck首选项..................................................................14 共享首选项......................................................................................................14 访问Enfocus首选项........................................................................................14 首选项 > Enfocus PitStop Pro首选项 > 常规..................................................15 首选项 > Enfocus PitStop Pro首选项 > 编辑..................................................16 首选项 > Enfocus PitStop Pro首选项 > 色彩..................................................19 首选项 > Enfocus PitStop Pro首选项 > 语言..................................................20 首选项 > Enfocus PitStop Pro首选项 > 色彩管理...........................................22 首选项 > Enfocus PitStop Pro首选项 > 变量集..............................................23 首选项 > Enfocus PitStop Pro首选项 > 单位和参考线...................................24 首选项 > Enfocus PitStop Pro首选项 > 窗口..................................................24 首选项 > Enfocus PitStop Pro首选项 > 警告..................................................24 首选项 > Enfocus PitStop Pro首选项 > 预设数据库.......................................25 首选项 > Enfocus PitStop Pro首选项 > 帮助..................................................25 首选项 > Enfocus PitStop Pro首选项 > 许可..................................................25 首选项 > Enfocus PitStop Pro首选项 > 更新..................................................25 首选项 > Enfocus StatusCheck首选项 > 常规................................................26 首选项 > Enfocus StatusCheck首选项 > 语言................................................26 首选项 > Enfocus StatusCheck首选项 > 个人信息.........................................27 首选项 > Enfocus StatusCheck首选项 > ............................27 首选项 > Enfocus StatusCheck首选项 > 数据库............................................27 首选项 > Enfocus StatusCheck首选项 > 自动................................................27
RSLINX CLASSIC 说明书

1 • 欢迎使用 RSLinx Classic
3
什么是 RSLinx Classic? .............................................................................................................. 3 不同类型 RSLinx Classic 的区别................................................................................................ 3
RSLinx Classic for FactoryTalk View .................................................................................. 5
快速入门.......................................................................................................................................... 6 步骤 1 - 配置驱动程序 ........................................................................................................ 6 步骤 2 - 配置主题 ................................................................................................................. 7 步骤 3 - 复制链接到剪贴板............................................................................................... 8 步骤 4 - 从剪贴板粘贴链接............................................................................................... 9
LTE系统的MIMO信道建模与仿真
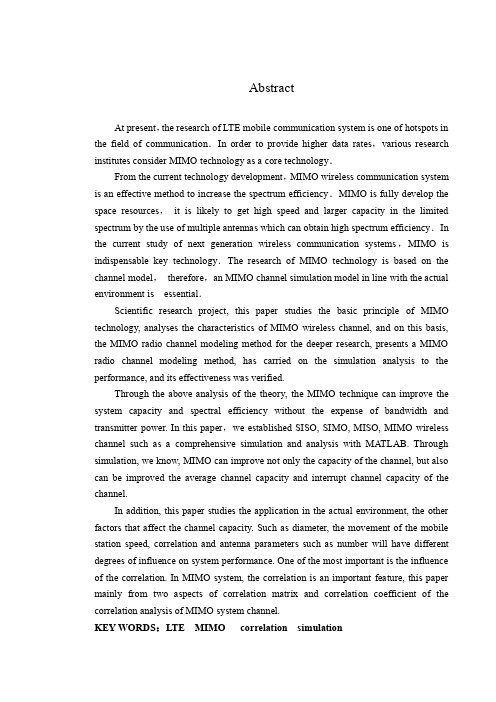
indispensable key technology.The research of MIMO technology is based on the
mainly from two aspects of correlation matrix and correlation coefficient of the
correlation analysis of MIMO system channel.
KEY WORDS:LTE
MIMO
correlation simulation
radio channel modeling method, has carried on the simulation analysis to the
performance, and its effectiveness was verified.
Through the above analysis of the theory, the MIMO technique can improve the
3.3 信道衰落 ....................................................................................................... 14
3.3.1 小尺度衰落特性 ............................................................................... 14
can be improved the average channel capacity and interrupt channel capacity of the
ETSI EN 300 019-1-2-2003[1]
![ETSI EN 300 019-1-2-2003[1]](https://img.taocdn.com/s3/m/84dcd923482fb4daa58d4b83.png)
Foreword.............................................................................................................................................................4
If you find errors in the present document, send your comment to: editor@
Copyright Notification No part may be reproduced except as authorized by written permission. The copyright and the foregoing restriction extend to reproduction in all media.
2 References ................................................................................................................................................5
3 Definitions................................................................................................................................................5
5.3
Chemically active substances .............................................................................................................................8
第五章 机器学习

谋杀事件语义网络
谋杀事件的具体化链表示
14
(2) 解释转 换运用 类比解 题
先例
15
解释样板
16
练习2 Domination (支配) 这是个关于一位贵族与一位飞扬跋扈的和贪婪的 女人的练习。该贵族与该女人结了婚。试说明该贵族 很可能想当皇帝。
琳达是女人,而迪克是男人。迪克与琳达结了婚。迪克 因琳达的盛气凌人而厌倦。迪克因厌倦而软弱。
第五章 机器学习
5.1 机器学习的定义、研究意义与发展历史 5.2 机器学习的主要策略与基本结构 5.3 几种常用的学习方法
2
5.1 机器学习的定义和发展历史
5.1.1 机器学习的定义
机器学习的定义 顾名思义,机器学习是研究如何使用机 器来模拟人类学习活动的一门学科。 稍为严格的提法是:机器学习是一门 研究机器获取新知识和新技 能,并识别现 有知识的学问。
18
5.5 归纳学习(基于概念、决策树的学习)
归纳学习(induction learning)是应用归纳推 理进行学习的一种方法。根据归纳学习有无教师 指导,可把它分为示例学习和观察与发现学习。 5.5.1 归纳学习的模式和规则 归纳学习的模式
解释过程 实例空间 规则空间
规划过程
19
5.6 类比学习
9
例:一个决定受损汽车修理费用的汽 车保险程序
这个程序的输入是被损坏的汽车的描述,包括制造厂家、 生产年代、汽车的种类以及记录汽车被损坏部位和损坏 程度的一个表; 程序的输出是保险公司应付的修理费用。 这个系统是个机械记忆系统。为了估算损坏汽车的修理 费用,程序系统必须在存储器中查找同一厂家、同一生 产年代、损坏的部位和程度相同的汽车,然后把对应的 费用提交给用户。 如果系统没有发现这样的汽车,则它使用保险公司公布 的赔偿规则估算出一个修理费用,然后把厂家、生产日 期和损坏情况等特征与估算出的费用保存起来,以便将 来查找使用。
东北大学本科毕业设计论文《基于支持向量机算法的电网故障诊断方法研究》
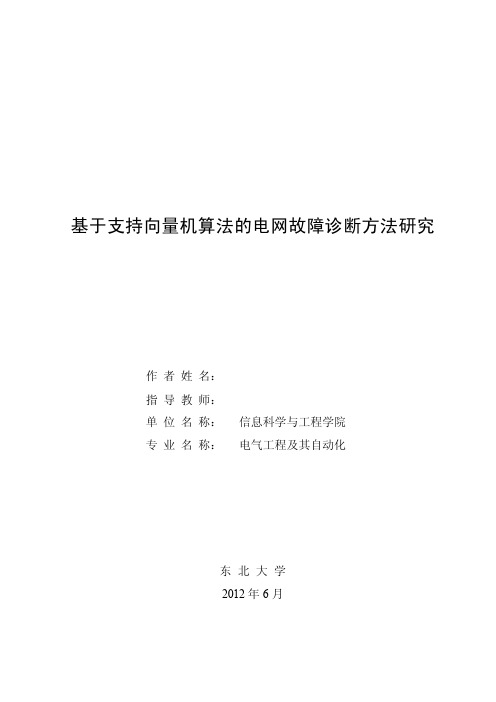
ABSTRACT
With electricity demand growth and technology progress, power grid has become larger and more complex. Due to the formation of large power grids, the quality of electricity supply and electric security improves, also, resources complementary has been strengthened. Once fault occurs, however, it will spread to a wider area with a faster speed. For these merits, this study focuses on the fault diagnosis for power network based on support vector machine. By analyzing relative literatures and building a simulation model, this thesis finishes the analyzing of fault waveforms and harmonic distribution, and studies fault characteristics from the perspective of signal synthesis. To extract fault features submerged in original fault data, this thesis deeply studies the fuzzy processing method, the value detection of instantaneous current and the common fault feature extraction method based on wavelet singular entropy. For the error-prone of instantaneous current detection, fuzzing set ideas is drew to optimize the training samples and by modifying diagnostic strategies, the shortcoming is overcame. To reduce the elapsed time of the common fault feature extraction method based on wavelet singular entropy, a new fault feature combination is proposed by comparing the method with instantaneous current detection. This new combination can inspect faults rapidly when current has a sharp rise such as no- load line closing serious short circuit and improve the diagnostic accuracy when fault current rise is more gentle by taking advantage of wavelet transform which has a wealth of information. Under the condition that the fault features are extracted entirely, artifirt vector machine are used to diagnose power network faults. On one hand, a comparison of the two methods and a study on kernels, multi-class classification methods and SVM training algorithms are carried out. On the other hand, for a figurative expression of the diagnostic results, two dimensions are constructed from the training samples and a twodimensional optimal hyperplane is established by analyzing simulation system structure and data characteristics. Finally, by analyzing the spatial distribution of sample points, the three-dimensional optimal hyperplane is explored. -III-
python 300本电子书合集
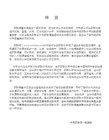
Rapid+GUI+Programming+with+Python+and+Qt.pdf
quantsp研究计划书.pdf
Qt5_Python_GUI_Programming_Cookbook.pdf
PYTHON自然语言处理中文翻译 NLTK 中文版.pdf
Python编程导论第2版_2018(#).pdf
Python编程初学者指南.pdf
Python编程:从入门到实践.pdf
Python_文本处理指南[经典].pdf
Python_Web开发实战.pdf
Python_Web开发:测试驱动方法.pdf
Python_Testing_Cookbook.pdf
Python机器学习实践指南(中文版带书签)、原书代码、数据集
python官方文档
Python编程(第4版 套装上下册)
linux
征服PYTHON-语言基础与典型应用.pdf
与孩子一起学编程_中文版_详细书签.pdf
用Python做科学计算.pdf
用Python写网络爬虫.pdf
用Python进行自然语言处理(中文翻译NLTK).pdf
面向对象的思考过程.pdf
码农 第8期.pdf
码农 第7期.pdf
码农 第6期.pdf
码农 第5期.pdf
流畅的python.pdf
零基础学python.pdf
量化投资以Python为工具.pdf
利用Python进行数据分析(###).pdf
可爱的Python(哲思社区.插图版_文字版).pdf
需求规格说明书-范本
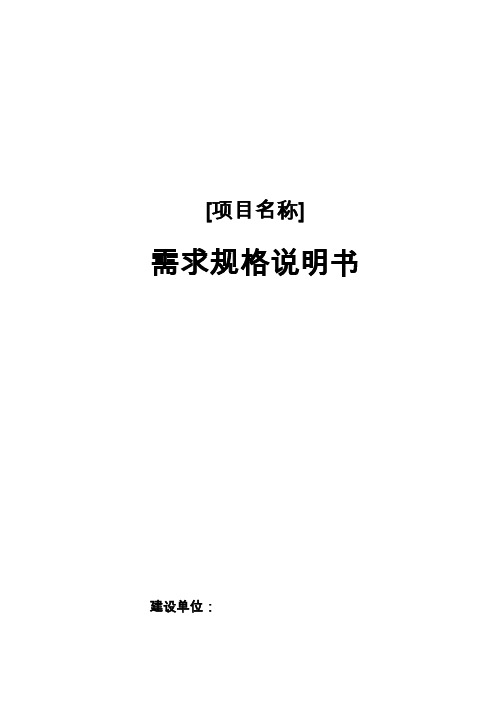
3.1
功能需求概述 .............................................................................2
3.2
用户角色 ....................................................................................2
3.5
模块二 .......................................................................................4
第 4 章 用户界面需求 ............................................................. 4
简要描述本章节业务需求,如果业务流程章节省略,本章节须与上一章节合并。
2.3.2 业务流程
可选章节,结合业务流程图对业务流程描述和所需的业务表单进行说明,如果业务流程比较简 单,可以整体描述,如果业务流程比较复杂,须分节点描述。
2.4 业务需求二
第 3 章 功能需求
3.1 功能需求概述
对系统功能进行概述,画出系统功能结构图并对其进行说明,注意一定要包含后台维护性功能 与统计性功能的说明。
4. 功能操作说明 输入 详细描述该用户界面的输入数据,如:输入源、数量或有效范围、度量单位、时间设定。 业务处理 说明该功能的业务处理过程,一般包含: 输入数据的有效性检查; 业务操作顺序(包括事件的时间设定); 异常响应处理,例如,溢出、通信故障、错误处理等; 受操作影响的参数; 输出数据的有效性检查。 输出 详细描述该功能所有输出数据,包含输出目的地、数量或有效范围、度量单位、时间关系、出 错信息描述;
Python机器学习(PythonMachineLearning中文版PDF)
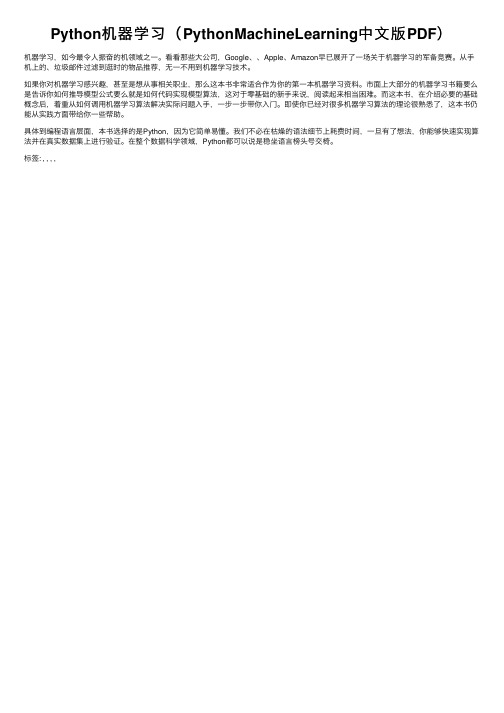
Python机器学习(PythonMachineLearning中⽂版PDF)
机器学习,如今最令⼈振奋的机领域之⼀。
看看那些⼤公司,Google、、Apple、Amazon早已展开了⼀场关于机器学习的军备竞赛。
从⼿机上的、垃圾邮件过滤到逛时的物品推荐,⽆⼀不⽤到机器学习技术。
如果你对机器学习感兴趣,甚⾄是想从事相关职业,那么这本书⾮常适合作为你的第⼀本机器学习资料。
市⾯上⼤部分的机器学习书籍要么是告诉你如何推导模型公式要么就是如何代码实现模型算法,这对于零基础的新⼿来说,阅读起来相当困难。
⽽这本书,在介绍必要的基础概念后,着重从如何调⽤机器学习算法解决实际问题⼊⼿,⼀步⼀步带你⼊门。
即使你已经对很多机器学习算法的理论很熟悉了,这本书仍能从实践⽅⾯带给你⼀些帮助。
具体到编程语⾔层⾯,本书选择的是Python,因为它简单易懂。
我们不必在枯燥的语法细节上耗费时间,⼀旦有了想法,你能够快速实现算法并在真实数据集上进⾏验证。
在整个数据科学领域,Python都可以说是稳坐语⾔榜头号交椅。
标签: , , , ,。
Python机器学习(原书第3版)

15.5本章小 结
16.1序列数据 1
介绍
16.2循环神经 2
网络序列建模
3
16.3用 TensorFlow
实现循环神经
网络序列建模
4 16.4用转换器
模型理解语言
5
16.5本章小结
17.2从零开始实现 GAN
17.1生成对抗网络 介绍
17.3用卷积和 Wasserstein GAN 提高合成图像的质
回归模型的性 能
10.6用正则化 2
方法进行回归
3
10.7将线性回 归模型转换为
曲线——多项
式回归
4 10.8用随机森
林处理非线性
关系
5
10.9本章小结
11.1用k-均值进行 相似性分组
11.2把集群组织成 层次树
11.3通过DBSCAN定 位高密度区域
11.4本章小结
12.1用人工神经网 络建立复杂函数模型
量
17.4其他的 GAN应用
17.5本章小 结
18.1概述—— 1
从经验中学习
18.2 RL的理 2
论基础
3 18.3强化学习
算法
4 18.4实现第一
个RL算法
5
18.5本章小结
作者介绍
这是《Python机器学习(原书第3版)》的读书笔记模板,暂无该书作者的介绍。
精彩摘录
这是《Python机器学习(原书第3版)》的读书笔记模板,可以替换为自己的精彩内容摘录。
谢谢观看
GradientTap
e计算梯度
5
14.5通过 Keras API简
化通用体系结
构的实现
14.6 TensorFlow
机器学习读书笔记
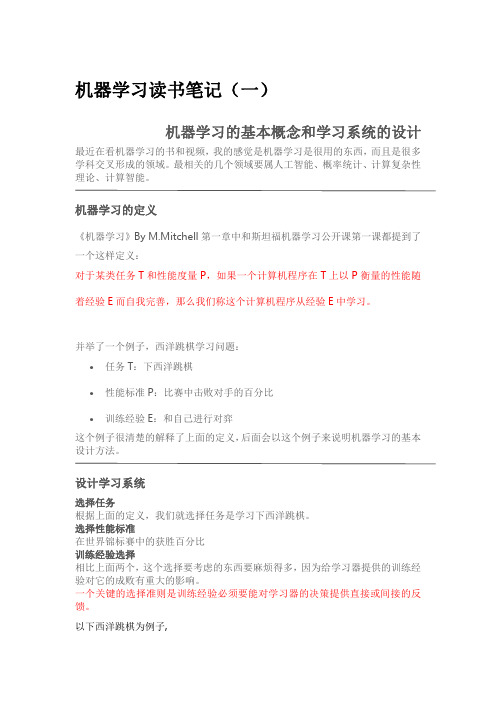
机器学习的基本概念和学习系统的设计
最近在看机器学习的书和视频,我的感觉是机器学习是很用的东西,而且是很多 学科交叉形成的领域。最相关的几个领域要属人工智能、概率统计、计算复tchell 第一章中和斯坦福机器学习公开课第一课都提到了 一个这样定义: 对于某类任务 T 和性能度量 P,如果一个计算机程序在 T 上以 P 衡量的性能随
概念学习
给定一样例集合以及每个样例是否属于某一概念的标注,怎样自动推断出该概念 的一般定义。这一问题被称为概念学习。 一个更准确的定义: 概念学习是指从有关某个布尔函数的输入输出训练样例中推断出该布尔函数。注 意,在前面一篇文章《机器学习的基本概念和学习系统的设计》中提到,机器学 习中要学习的知识的确切类型通常是一个函数,在概念学习里面,这个函数被限 定为是一个布尔函数,也就是它的输出只有{0,1}(0代表 false,1(代表 true)), 也就是说目标函数的形式如下:
x1:棋盘上黑子的数量
x2:棋盘上白子的数量
x3:棋盘上黑王的数量 x4:棋盘上红王的数量 x5:被红字威胁的黑子数量(即会在下一次被红子吃掉的黑子数量) x6:被黑子威胁的红子的数量 于是学习程序把 V’(b)表示为一个线性函数 V’(b)=w0 + w1x1 + w2x2 + w3x3 + w4x4 + w5x5 + w6x6 其中,w0到 w6为数字系数,或叫权,由学习算法来选择。在决定某一个棋盘状 态值,权 w1到 w6决定了不同的棋盘特征的相对重要性,而权 w0为一个附加的 棋盘状态值常量。 好了,现在我们把学习西洋跳棋战略的问题转化为学习目标函数表示中系数 w0 到 w6值的问题,也即选择函数逼近算法。 选择函数逼近算法 为了学习 V’(b),我们需要一系列训练样例,它的形式为<b, Vtrain(b)>其中,b 是由 x1-x6参数描述的棋盘状态,Vtrain(b)是 b 的训练值。 举例来说, <<x1=3,x2=0,x3=1,x4=0,x5=0,x6=0>, +100>;描述了一个黑棋取胜的棋盘 状态 b,因为 x2=0表示红旗已经没有子了。 上面的训练样例表示仍然有一个问题:虽然对弈结束时最终状态的棋盘的评分 Vtrain(b)很好确定,但是大量中间状态(未分出胜负)的棋盘如何评分呢? 于是这里需要一个训练值估计法则: Vtrain(b) <- V’(Successor(b)) Successor(b)表示 b 之后再轮到程序走棋时的棋盘状态(也就是程序走了一步 和对手回应了一步以后的棋局)。 这个看起来有点难理解,我们用当前的 V’来估计训练值,又用这一训练值来更 新 V’。当然,我们使用后续棋局 Successor(b)的估计值来估计棋局 b 的值。直
机器学习王衡军考试复习资料
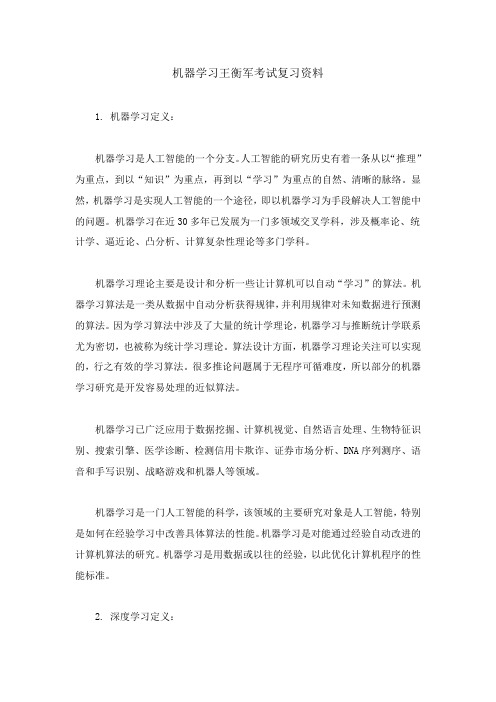
机器学习王衡军考试复习资料1. 机器学习定义:机器学习是人工智能的一个分支。
人工智能的研究历史有着一条从以“推理”为重点,到以“知识”为重点,再到以“学习”为重点的自然、清晰的脉络。
显然,机器学习是实现人工智能的一个途径,即以机器学习为手段解决人工智能中的问题。
机器学习在近30多年已发展为一门多领域交叉学科,涉及概率论、统计学、逼近论、凸分析、计算复杂性理论等多门学科。
机器学习理论主要是设计和分析一些让计算机可以自动“学习”的算法。
机器学习算法是一类从数据中自动分析获得规律,并利用规律对未知数据进行预测的算法。
因为学习算法中涉及了大量的统计学理论,机器学习与推断统计学联系尤为密切,也被称为统计学习理论。
算法设计方面,机器学习理论关注可以实现的,行之有效的学习算法。
很多推论问题属于无程序可循难度,所以部分的机器学习研究是开发容易处理的近似算法。
机器学习已广泛应用于数据挖掘、计算机视觉、自然语言处理、生物特征识别、搜索引擎、医学诊断、检测信用卡欺诈、证券市场分析、DNA序列测序、语音和手写识别、战略游戏和机器人等领域。
机器学习是一门人工智能的科学,该领域的主要研究对象是人工智能,特别是如何在经验学习中改善具体算法的性能。
机器学习是对能通过经验自动改进的计算机算法的研究。
机器学习是用数据或以往的经验,以此优化计算机程序的性能标准。
2. 深度学习定义:深度学习(英语:deep learning)是机器学习的分支,是一种以人工神经网络为架构,对资料进行表征学习的算法。
深度学习是机器学习中一种基于对数据进行表征学习的算法。
观测值(例如一幅图像)可以使用多种方式来表示,如每个像素强度值的向量,或者更抽象地表示成一系列边、特定形状的区域等。
而使用某些特定的表示方法更容易从实例中学习任务(例如,人脸识别或面部表情识别)。
深度学习的好处是用非监督式或半监督式的特征学习和分层特征提取高效算法来替代手工获取特征。
表征学习的目标是寻求更好的表示方法并创建更好的模型来从大规模未标记数据中学习这些表示方法。
收藏复旦大学机器学习、深度学习公开课,附PDF课件下载
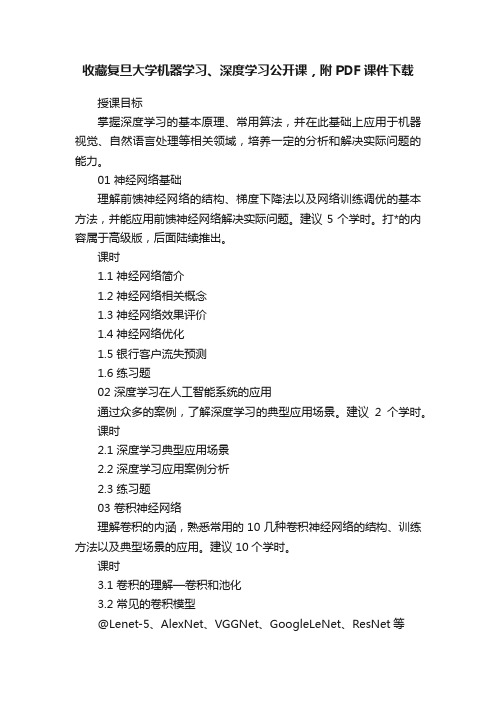
收藏复旦大学机器学习、深度学习公开课,附PDF课件下载授课目标掌握深度学习的基本原理、常用算法,并在此基础上应用于机器视觉、自然语言处理等相关领域,培养一定的分析和解决实际问题的能力。
01 神经网络基础理解前馈神经网络的结构、梯度下降法以及网络训练调优的基本方法,并能应用前馈神经网络解决实际问题。
建议5个学时。
打*的内容属于高级版,后面陆续推出。
课时1.1 神经网络简介1.2 神经网络相关概念1.3 神经网络效果评价1.4 神经网络优化1.5 银行客户流失预测1.6 练习题02 深度学习在人工智能系统的应用通过众多的案例,了解深度学习的典型应用场景。
建议2个学时。
课时2.1 深度学习典型应用场景2.2 深度学习应用案例分析2.3 练习题03 卷积神经网络理解卷积的内涵,熟悉常用的10几种卷积神经网络的结构、训练方法以及典型场景的应用。
建议10个学时。
课时3.1 卷积的理解—卷积和池化3.2 常见的卷积模型@Lenet-5、AlexNet、VGGNet、GoogleLeNet、ResNet等@Inception v2-v4、DarkNet、DenseNet、SSD等*@MobileNet,ShuffleNet*3.3 胶囊网络*3.4 CNN卷积神经网络应用案例3.5 目标检测常用算法@R-CNN、Fast RCNN、Faster RCNN、YOLOv1-v3等3.5 图像分类3.6 动物识别3.7 物体检测3.8 人脸表情年龄特征识别*3.9 练习题04 循环神经神经网络理解循环神经网络以及变种LSTM、GRU的结构、训练方法以及典型场景的应用。
建议6个学时。
课时4.1 RNN基本原理4.2 LSTM4.3 GRU4.4 CNN+LSTM模型4.5 Bi-LSTM双向循环神经网络结构4.6 Seq2seq模型4.7 注意力机制4.8 自注意力机制*4.9 ELMo、Transformer等*4.10 BERT、EPT、XLNet、ALBERT等*4.11 机器翻译4.12 练习题05 生成对抗网络理解生成对抗网络的结构、训练方法以及典型场景的应用。
机器学习实战源代码
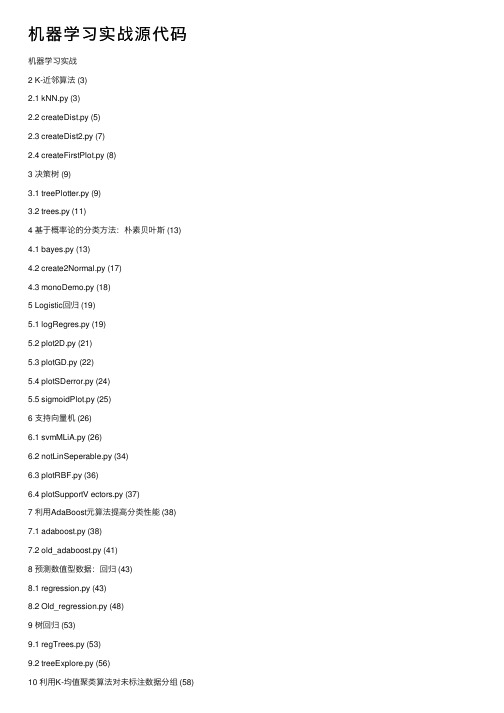
机器学习实战源代码机器学习实战2 K-近邻算法 (3)2.1 kNN.py (3)2.2 createDist.py (5)2.3 createDist2.py (7)2.4 createFirstPlot.py (8)3 决策树 (9)3.1 treePlotter.py (9)3.2 trees.py (11)4 基于概率论的分类⽅法:朴素贝叶斯 (13) 4.1 bayes.py (13)4.2 create2Normal.py (17)4.3 monoDemo.py (18)5 Logistic回归 (19)5.1 logRegres.py (19)5.2 plot2D.py (21)5.3 plotGD.py (22)5.4 plotSDerror.py (24)5.5 sigmoidPlot.py (25)6 ⽀持向量机 (26)6.1 svmMLiA.py (26)6.2 notLinSeperable.py (34)6.3 plotRBF.py (36)6.4 plotSupportV ectors.py (37)7 利⽤AdaBoost元算法提⾼分类性能 (38)7.1 adaboost.py (38)7.2 old_adaboost.py (41)8 预测数值型数据:回归 (43)8.1 regression.py (43)8.2 Old_regression.py (48)9 树回归 (53)9.1 regTrees.py (53)9.2 treeExplore.py (56)10 利⽤K-均值聚类算法对未标注数据分组 (58)10.1 kMeans.py (58)11 使⽤Apriori算法进⾏关联分析 (61)11.1 apriori.py (61)12 使⽤FP-growth算法来⾼效分析频繁项集 (65)12.1 fpGrowth.py (65)13 利⽤PCA来简化数据 (68)13.1 pca.py (68)13.2 createFig1.py (69)13.2 createFig2.py (70)13.3 createFig3 (70)13.4 createFig4.py (72)14 利⽤SVD简化数据 (72)14.1 svdRec.py (72)15 ⼤数据与MapReduce (75)15.1 mrMean.py (75)15.2 mrMeanMapper.py (76)15.3 mrMeanReducer.py (76)15.4 mrSVM.py (77)15.5 mrSVMkickStart.py (79)15.6 pegasos.py (79)15.7 proximalSVM.py (81)15.8 py27dbg.py (82)15.9 wc.py (83)2 K-近邻算法2.1 kNN.py'''Created on Sep 16, 2010kNN: k Nearest NeighborsInput: inX: vector to compare to existing dataset (1xN)dataSet: size m data set of known vectors (NxM)labels: data set labels (1xM vector)k: number of neighbors to use for comparison (should be an odd number) Output: the most popular class label@author: pbharrin'''from numpy import *import operatorfrom os import listdirdef classify0(inX, dataSet, labels, k):dataSetSize = dataSet.shape[0]diffMat = tile(inX, (dataSetSize,1)) - dataSetsqDiffMat = diffMat**2sqDistances = sqDiffMat.sum(axis=1)distances = sqDistances**0.5sortedDistIndicies = distances.argsort()classCount={}for i in range(k):voteIlabel = labels[sortedDistIndicies[i]]classCount[voteIlabel] = classCount.get(voteIlabel,0) + 1sortedClassCount = sorted(classCount.iteritems(), key=operator.itemgetter(1), reverse=True) return sortedClassCount[0][0] def createDataSet():group = array([[1.0,1.1],[1.0,1.0],[0,0],[0,0.1]])labels = ['A','A','B','B']return group, labelsdef file2matrix(filename):fr = open(filename)numberOfLines = len(fr.readlines()) #get the number of lines in the filereturnMat = zeros((numberOfLines,3)) #prepare matrix to returnclassLabelV ector = [] #prepare labels returnfr = open(filename)index = 0for line in fr.readlines():line = line.strip()listFromLine = line.split('\t')returnMat[index,:] = listFromLine[0:3]classLabelV ector.append(int(listFromLine[-1]))index += 1return returnMat,classLabelV ectordef autoNorm(dataSet):minV als = dataSet.min(0)maxV als = dataSet.max(0)ranges = maxV als - minV alsnormDataSet = zeros(shape(dataSet))m = dataSet.shape[0]normDataSet = dataSet - tile(minV als, (m,1))normDataSet = normDataSet/tile(ranges, (m,1)) #element wise dividereturn normDataSet, ranges, minV alsdef datingClassTest():hoRatio = 0.50 #hold out 10%datingDataMat,datingLabels = file2matrix('datingTestSet2.txt') #load data setfrom filenormMat, ranges, minV als = autoNorm(datingDataMat)m = normMat.shape[0]numTestVecs = int(m*hoRatio)errorCount = 0.0for i in range(numTestV ecs):classifierResult = classify0(normMat[i,:],normMat[numTestVecs:m,:],datingLabels[numTestV ecs:m],3) print "the classifier came back with: %d, the real answer is: %d" % (classifierResult, datingLabels[i])if (classifierResult != datingLabels[i]): errorCount += 1.0print "the total error rate is: %f" % (errorCount/float(numTestV ecs))print errorCountdef img2vector(filename):returnV ect = zeros((1,1024))fr = open(filename)for i in range(32):lineStr = fr.readline()for j in range(32):returnV ect[0,32*i+j] = int(lineStr[j])return returnV ectdef handwritingClassTest():hwLabels = []trainingFileList = listdir('trainingDigits') #load the training setm = len(trainingFileList)trainingMat = zeros((m,1024))for i in range(m):fileNameStr = trainingFileList[i]fileStr = fileNameStr.split('.')[0] #take off .txtclassNumStr = int(fileStr.split('_')[0])hwLabels.append(classNumStr)trainingMat[i,:] = img2vector('trainingDigits/%s' % fileNameStr)testFileList = listdir('testDigits') #iterate through the test seterrorCount = 0.0mTest = len(testFileList)for i in range(mTest):fileNameStr = testFileList[i]fileStr = fileNameStr.split('.')[0] #take off .txtclassNumStr = int(fileStr.split('_')[0])vectorUnderTest = img2vector('testDigits/%s' % fileNameStr)classifierResult = classify0(vectorUnderTest, trainingMat, hwLabels, 3)print "the classifier came back with: %d, the real answer is: %d" % (classifierResult, classNumStr) if (classifierResult != classNumStr): errorCount += 1.0print "\nthe total number of errors is: %d" % errorCountprint "\nthe total error rate is: %f" % (errorCount/float(mTest))2.2 createDist.py'''Created on Oct 6, 2010@author: Peter'''from numpy import *import matplotlibimport matplotlib.pyplot as pltfrom matplotlib.patches import Rectanglen = 1000 #number of points to createxcord = zeros((n))ycord = zeros((n))markers =[]colors =[]fw = open('testSet.txt','w')for i in range(n):[r0,r1] = random.standard_normal(2)myClass = random.uniform(0,1)if (myClass <= 0.16):fFlyer = random.uniform(22000, 60000)tats = 3 + 1.6*r1markers.append(20)colors.append(2.1)classLabel = 1 #'didntLike'print ("%d, %f, class1") % (fFlyer, tats)elif ((myClass > 0.16) and (myClass <= 0.33)):fFlyer = 6000*r0 + 70000tats = 10 + 3*r1 + 2*r0markers.append(20)colors.append(1.1)classLabel = 1 #'didntLike'print ("%d, %f, class1") % (fFlyer, tats)elif ((myClass > 0.33) and (myClass <= 0.66)):fFlyer = 5000*r0 + 10000tats = 3 + 2.8*r1markers.append(30)colors.append(1.1)classLabel = 2 #'smallDoses'print ("%d, %f, class2") % (fFlyer, tats)else:fFlyer = 10000*r0 + 35000tats = 10 + 2.0*r1markers.append(50)colors.append(0.1)classLabel = 3 #'largeDoses'print ("%d, %f, class3") % (fFlyer, tats)if (tats < 0): tats =0if (fFlyer < 0): fFlyer =0xcord[i] = fFlyer; ycord[i]=tatsfw.write("%d\t%f\t%f\t%d\n" % (fFlyer, tats, random.uniform(0.0, 1.7), classLabel)) fw.close()fig = plt.figure()ax = fig.add_subplot(111)ax.scatter(xcord,ycord, c=colors, s=markers)type1 = ax.scatter([-10], [-10], s=20, c='red')type2 = ax.scatter([-10], [-15], s=30, c='green')type3 = ax.scatter([-10], [-20], s=50, c='blue')ax.legend([type1, type2, type3], ["Class 1", "Class 2", "Class 3"], loc=2)#ax.axis([-5000,100000,-2,25])plt.xlabel('Frequent Flyier Miles Earned Per Year')plt.ylabel('Percentage of Body Covered By Tatoos') plt.show()2.3 createDist2.py'''Created on Oct 6, 2010@author: Peter'''from numpy import *import matplotlibimport matplotlib.pyplot as pltfrom matplotlib.patches import Rectanglen = 1000 #number of points to createxcord1 = []; ycord1 = []xcord2 = []; ycord2 = []xcord3 = []; ycord3 = []markers =[]colors =[]fw = open('testSet.txt','w')for i in range(n):[r0,r1] = random.standard_normal(2)myClass = random.uniform(0,1)if (myClass <= 0.16):fFlyer = random.uniform(22000, 60000)tats = 3 + 1.6*r1markers.append(20)colors.append(2.1)classLabel = 1 #'didntLike'xcord1.append(fFlyer); ycord1.append(tats) elif ((myClass > 0.16) and (myClass <= 0.33)): fFlyer = 6000*r0 + 70000 tats = 10 + 3*r1 + 2*r0markers.append(20)colors.append(1.1)classLabel = 1 #'didntLike'if (tats < 0): tats =0if (fFlyer < 0): fFlyer =0xcord1.append(fFlyer); ycord1.append(tats) elif ((myClass > 0.33) and (myClass <= 0.66)): fFlyer = 5000*r0 + 10000 tats = 3 + 2.8*r1markers.append(30)colors.append(1.1)classLabel = 2 #'smallDoses'if (tats < 0): tats =0if (fFlyer < 0): fFlyer =0xcord2.append(fFlyer); ycord2.append(tats)else:fFlyer = 10000*r0 + 35000tats = 10 + 2.0*r1markers.append(50)colors.append(0.1)classLabel = 3 #'largeDoses'if (tats < 0): tats =0if (fFlyer < 0): fFlyer =0xcord3.append(fFlyer); ycord3.append(tats)fw.close()fig = plt.figure()ax = fig.add_subplot(111)#ax.scatter(xcord,ycord, c=colors, s=markers)type1 = ax.scatter(xcord1, ycord1, s=20, c='red')type2 = ax.scatter(xcord2, ycord2, s=30, c='green')type3 = ax.scatter(xcord3, ycord3, s=50, c='blue')ax.legend([type1, type2, type3], ["Did Not Like", "Liked in Small Doses", "Liked in Large Doses"], loc=2)ax.axis([-5000,100000,-2,25])plt.xlabel('Frequent Flyier Miles Earned Per Year')plt.ylabel('Percentage of Time Spent Playing Video Games')plt.show()2.4 createFirstPlot.py'''Created on Oct 27, 2010@author: Peter'''from numpy import *import kNNimport matplotlibimport matplotlib.pyplot as pltfig = plt.figure()ax = fig.add_subplot(111)datingDataMat,datingLabels = kNN.file2matrix('datingTestSet.txt')#ax.scatter(datingDataMat[:,1], datingDataMat[:,2])ax.scatter(datingDataMat[:,1], datingDataMat[:,2], 15.0*array(datingLabels), 15.0*array(datingLabels)) ax.axis([-2,25,-0.2,2.0])plt.xlabel('Percentage of Time Spent Playing Video Games')plt.ylabel('Liters of Ice Cream Consumed Per Week')plt.show()3 决策树3.1 treePlotter.py'''Created on Oct 14, 2010@author: Peter Harrington'''import matplotlib.pyplot as pltdecisionNode = dict(boxstyle="sawtooth", fc="0.8")leafNode = dict(boxstyle="round4", fc="0.8")arrow_args = dict(arrowstyle="<-")def getNumLeafs(myTree):numLeafs = 0firstStr = myTree.keys()[0]secondDict = myTree[firstStr]for key in secondDict.keys():if type(secondDict[key]).__name__=='dict':#test to see if the nodes are dictonaires, if not they are leaf nodes numLeafs += getNumLeafs(secondDict[key])else: numLeafs +=1return numLeafsdef getTreeDepth(myTree):maxDepth = 0firstStr = myTree.keys()[0]secondDict = myTree[firstStr]for key in secondDict.keys():if type(secondDict[key]).__name__=='dict':#test to see if the nodes are dictonaires, if not they are leaf nodesthisDepth = 1 + getTreeDepth(secondDict[key])else: thisDepth = 1if thisDepth > maxDepth: maxDepth = thisDepthreturn maxDepthdef plotNode(nodeTxt, centerPt, parentPt, nodeType):createPlot.ax1.annotate(nodeTxt, xy=parentPt, xycoords='axes fraction',xytext=centerPt, textcoords='axes fraction',va="center", ha="center", bbox=nodeType, arrowprops=arrow_args )def plotMidText(cntrPt, parentPt, txtString):xMid = (parentPt[0]-cntrPt[0])/2.0 + cntrPt[0]yMid = (parentPt[1]-cntrPt[1])/2.0 + cntrPt[1]createPlot.ax1.text(xMid, yMid, txtString, va="center", ha="center", rotation=30)def plotTree(myTree, parentPt, nodeTxt):#if the first key tells you what feat was split on numLeafs = getNumLeafs(myTree) #this determines the x width of this treedepth = getTreeDepth(myTree)firstStr = myTree.keys()[0] #the text label for this node should be thiscntrPt = (plotTree.xOff + (1.0 + float(numLeafs))/2.0/plotTree.totalW, plotTree.yOff)plotMidText(cntrPt, parentPt, nodeTxt)plotNode(firstStr, cntrPt, parentPt, decisionNode)secondDict = myTree[firstStr]plotTree.yOff = plotTree.yOff - 1.0/plotTree.totalDfor key in secondDict.keys():if type(secondDict[key]).__name__=='dict':#test to see if the nodes are dictonaires, if not they are leaf nodesplotTree(secondDict[key],cntrPt,str(key)) #recursionelse: #it's a leaf node print the leaf nodeplotTree.xOff = plotTree.xOff + 1.0/plotTree.totalWplotNode(secondDict[key], (plotTree.xOff, plotTree.yOff), cntrPt, leafNode)plotMidText((plotTree.xOff, plotTree.yOff), cntrPt, str(key))plotTree.yOff = plotTree.yOff + 1.0/plotTree.totalD#if you do get a dictonary you know it's a tree, and the first element will be another dictdef createPlot(inTree):fig = plt.figure(1, facecolor='white')fig.clf()axprops = dict(xticks=[], yticks=[])createPlot.ax1 = plt.subplot(111, frameon=False, **axprops) #no ticks#createPlot.ax1 = plt.subplot(111, frameon=False) #ticks for demo puropses plotTree.totalW = float(getNumLeafs(inTree))plotTree.totalD = float(getTreeDepth(inTree))plotTree.xOff = -0.5/plotTree.totalW; plotTree.yOff = 1.0;plotTree(inTree, (0.5,1.0), '')plt.show()#def createPlot():# fig = plt.figure(1, facecolor='white')# fig.clf()# createPlot.ax1 = plt.subplot(111, frameon=False) #ticks for demo puropses # plotNode('a decision node', (0.5, 0.1), (0.1, 0.5), decisionNode)# plotNode('a leaf node', (0.8, 0.1), (0.3, 0.8), leafNode)# plt.show()def retrieveTree(i):listOfTrees =[{'no surfacing': {0: 'no', 1: {'flippers': {0: 'no', 1: 'yes'}}}},{'no surfacing': {0: 'no', 1: {'flippers': {0: {'head': {0: 'no', 1: 'yes'}}, 1: 'no'}}}}]return listOfTrees[i]#createPlot(thisTree)3.2 trees.py'''Created on Oct 12, 2010Decision Tree Source Code for Machine Learning in Action Ch. 3@author: Peter Harrington'''from math import logimport operatordef createDataSet():dataSet = [[1, 1, 'yes'],[1, 0, 'no'],[0, 1, 'no'],[0, 1, 'no']]labels = ['no surfacing','flippers']#change to discrete valuesreturn dataSet, labelsdef calcShannonEnt(dataSet):numEntries = len(dataSet)labelCounts = {}for featV ec in dataSet: #the the number of unique elements and their occurance currentLabel = featVec[-1]if currentLabel not in labelCounts.keys(): labelCounts[currentLabel] = 0labelCounts[currentLabel] += 1shannonEnt = 0.0for key in labelCounts:prob = float(labelCounts[key])/numEntriesshannonEnt -= prob * log(prob,2) #log base 2return shannonEntdef splitDataSet(dataSet, axis, value):retDataSet = []for featV ec in dataSet:if featV ec[axis] == value:reducedFeatV ec = featV ec[:axis] #chop out axis used for splittingreducedFeatV ec.extend(featVec[axis+1:])retDataSet.append(reducedFeatVec)return retDataSetdef chooseBestFeatureToSplit(dataSet):numFeatures = len(dataSet[0]) - 1 #the last column is used for the labelsbaseEntropy = calcShannonEnt(dataSet)bestInfoGain = 0.0; bestFeature = -1for i in range(numFeatures): #iterate over all the featuresfeatList = [example[i] for example in dataSet]#create a list of all the examples of this feature uniqueV als = set(featList) #get a set of unique valuesnewEntropy = 0.0subDataSet = splitDataSet(dataSet, i, value)prob = len(subDataSet)/float(len(dataSet))newEntropy += prob * calcShannonEnt(subDataSet)infoGain = baseEntropy - newEntropy #calculate the info gain; ie reduction in entropyif (infoGain > bestInfoGain): #compare this to the best gain so farbestInfoGain = infoGain #if better than current best, set to bestbestFeature = ireturn bestFeature #returns an integerdef majorityCnt(classList):classCount={}for vote in classList:if vote not in classCount.keys(): classCount[vote] = 0classCount[vote] += 1sortedClassCount = sorted(classCount.iteritems(), key=operator.itemgetter(1), reverse=True) return sortedClassCount[0][0] def createTree(dataSet,labels):classList = [example[-1] for example in dataSet]if classList.count(classList[0]) == len(classList):return classList[0]#stop splitting when all of the classes are equalif len(dataSet[0]) == 1: #stop splitting when there are no more features in dataSet return majorityCnt(classList)bestFeat = chooseBestFeatureToSplit(dataSet)bestFeatLabel = labels[bestFeat]myTree = {bestFeatLabel:{}}del(labels[bestFeat])featV alues = [example[bestFeat] for example in dataSet]uniqueV als = set(featV alues)for value in uniqueV als:subLabels = labels[:] #copy all of labels, so trees don't mess up existing labelsmyTree[bestFeatLabel][value] = createTree(splitDataSet(dataSet, bestFeat, value),subLabels)return myTreedef classify(inputTree,featLabels,testV ec):firstStr = inputTree.keys()[0]secondDict = inputTree[firstStr]featIndex = featLabels.index(firstStr)key = testV ec[featIndex]if isinstance(valueOfFeat, dict):classLabel = classify(valueOfFeat, featLabels, testVec)else: classLabel = valueOfFeatreturn classLabeldef storeTree(inputTree,filename):import picklefw = open(filename,'w')pickle.dump(inputTree,fw)fw.close()def grabTree(filename):import picklefr = open(filename)return pickle.load(fr)4 基于概率论的分类⽅法:朴素贝叶斯4.1 bayes.py'''Created on Oct 19, 2010@author: Peter'''from numpy import *def loadDataSet():postingList=[['my', 'dog', 'has', 'flea', 'problems', 'help', 'please'],['maybe', 'not', 'take', 'him', 'to', 'dog', 'park', 'stupid'],['my', 'dalmation', 'is', 'so', 'cute', 'I', 'love', 'him'],['stop', 'posting', 'stupid', 'worthless', 'garbage'],['mr', 'licks', 'ate', 'my', 'steak', 'how', 'to', 'stop', 'him'],['quit', 'buying', 'worthless', 'dog', 'food', 'stupid']] classV ec = [0,1,0,1,0,1] #1 is abusive, 0 not return postingList,classVecdef createV ocabList(dataSet):vocabSet = set([]) #create empty setfor document in dataSet:vocabSet = vocabSet | set(document) #union of the two sets return list(vocabSet)def setOfWords2Vec(vocabList, inputSet):returnV ec = [0]*len(vocabList)if word in vocabList:returnV ec[vocabList.index(word)] = 1else: print "the word: %s is not in my V ocabulary!" % word return returnV ecdef trainNB0(trainMatrix,trainCategory):numTrainDocs = len(trainMatrix)numWords = len(trainMatrix[0])pAbusive = sum(trainCategory)/float(numTrainDocs)p0Num = ones(numWords); p1Num = ones(numWords) #change to ones() p0Denom = 2.0; p1Denom = 2.0 #change to 2.0 for i in range(numTrainDocs):if trainCategory[i] == 1:p1Num += trainMatrix[i]p1Denom += sum(trainMatrix[i])else:p0Num += trainMatrix[i]p0Denom += sum(trainMatrix[i])p1V ect = log(p1Num/p1Denom) #change to log()p0V ect = log(p0Num/p0Denom) #change to log()return p0Vect,p1V ect,pAbusivedef classifyNB(vec2Classify, p0Vec, p1Vec, pClass1):p1 = sum(vec2Classify * p1Vec) + log(pClass1) #element-wise multp0 = sum(vec2Classify * p0Vec) + log(1.0 - pClass1)if p1 > p0:return 1else:return 0def bagOfWords2V ecMN(vocabList, inputSet):returnV ec = [0]*len(vocabList)for word in inputSet:if word in vocabList:returnV ec[vocabList.index(word)] += 1return returnV ecdef testingNB():listOPosts,listClasses = loadDataSet()myV ocabList = createV ocabList(listOPosts)for postinDoc in listOPosts:trainMat.append(setOfWords2V ec(myV ocabList, postinDoc)) p0V,p1V,pAb = trainNB0(array(trainMat),array(listClasses)) testEntry = ['love', 'my', 'dalmation']thisDoc = array(setOfWords2V ec(myV ocabList, testEntry))print testEntry,'classified as: ',classifyNB(thisDoc,p0V,p1V,pAb) testEntry = ['stupid', 'garbage']thisDoc = array(setOfWords2V ec(myV ocabList, testEntry))print testEntry,'classified as: ',classifyNB(thisDoc,p0V,p1V,pAb)def textParse(bigString): #input is big string, #output is word list import relistOfTokens = re.split(r'\W*', bigString)return [tok.lower() for tok in listOfTokens if len(tok) > 2]def spamTest():docList=[]; classList = []; fullText =[]for i in range(1,26):wordList = textParse(open('email/spam/%d.txt' % i).read())docList.append(wordList)fullText.extend(wordList)classList.append(1)wordList = textParse(open('email/ham/%d.txt' % i).read())docList.append(wordList)fullText.extend(wordList)classList.append(0)vocabList = createV ocabList(docList)#create vocabularytrainingSet = range(50); testSet=[] #create test setfor i in range(10):randIndex = int(random.uniform(0,len(trainingSet)))testSet.append(trainingSet[randIndex])trainMat=[]; trainClasses = []for docIndex in trainingSet:#train the classifier (get probs) trainNB0trainMat.append(bagOfWords2VecMN(vocabList, docList[docIndex]))trainClasses.append(classList[docIndex])p0V,p1V,pSpam = trainNB0(array(trainMat),array(trainClasses))errorCount = 0for docIndex in testSet: #classify the remaining itemswordV ector = bagOfWords2V ecMN(vocabList, docList[docIndex])print "classification error",docList[docIndex]print 'the error rate is: ',float(errorCount)/len(testSet)#return vocabList,fullTextdef calcMostFreq(vocabList,fullText):import operatorfreqDict = {}for token in vocabList:freqDict[token]=fullText.count(token)sortedFreq = sorted(freqDict.iteritems(), key=operator.itemgetter(1), reverse=True) return sortedFreq[:30] def localWords(feed1,feed0):import feedparserdocList=[]; classList = []; fullText =[]minLen = min(len(feed1['entries']),len(feed0['entries']))for i in range(minLen):wordList = textParse(feed1['entries'][i]['summary'])docList.append(wordList)fullText.extend(wordList)classList.append(1) #NY is class 1wordList = textParse(feed0['entries'][i]['summary'])docList.append(wordList)fullText.extend(wordList)classList.append(0)vocabList = createV ocabList(docList)#create vocabularytop30Words = calcMostFreq(vocabList,fullText) #remove top 30 wordsfor pairW in top30Words:if pairW[0] in vocabList: vocabList.remove(pairW[0])trainingSet = range(2*minLen); testSet=[] #create test setfor i in range(20):randIndex = int(random.uniform(0,len(trainingSet)))testSet.append(trainingSet[randIndex])trainMat=[]; trainClasses = []for docIndex in trainingSet:#train the classifier (get probs) trainNB0trainMat.append(bagOfWords2VecMN(vocabList, docList[docIndex]))trainClasses.append(classList[docIndex])errorCount = 0for docIndex in testSet: #classify the remaining itemswordV ector = bagOfWords2V ecMN(vocabList, docList[docIndex])if classifyNB(array(wordV ector),p0V,p1V,pSpam) != classList[docIndex]: errorCount += 1print 'the error rate is: ',float(errorCount)/len(testSet)return vocabList,p0V,p1Vdef getTopWords(ny,sf):import operatorvocabList,p0V,p1V=localWords(ny,sf)topNY=[]; topSF=[]for i in range(len(p0V)):if p0V[i] > -6.0 : topSF.append((vocabList[i],p0V[i]))if p1V[i] > -6.0 : topNY.append((vocabList[i],p1V[i]))sortedSF = sorted(topSF, key=lambda pair: pair[1], reverse=True)print "SF**SF**SF**SF**SF**SF**SF**SF**SF**SF**SF**SF**SF**SF**SF**SF**" for item in sortedSF:print item[0]sortedNY = sorted(topNY, key=lambda pair: pair[1], reverse=True)print"NY**NY**NY**NY**NY**NY**NY**NY**NY**NY**NY**NY**NY**NY**NY**NY** " for item in sortedNY:print item[0]4.2 create2Normal.py'''Created on Oct 6, 2010@author: Peter'''from numpy import *import matplotlibimport matplotlib.pyplot as pltn = 1000 #number of points to createxcord0 = []ycord0 = []colors =[]fw = open('testSet.txt','w')for i in range(n):[r0,r1] = random.standard_normal(2)myClass = random.uniform(0,1)if (myClass <= 0.5):fFlyer = r0 + 9.0tats = 1.0*r1 + fFlyer - 9.0xcord0.append(fFlyer)ycord0.append(tats)else:fFlyer = r0 + 2.0tats = r1+fFlyer - 2.0xcord1.append(fFlyer)ycord1.append(tats)#fw.write("%f\t%f\t%d\n" % (fFlyer, tats, classLabel))fw.close()fig = plt.figure()ax = fig.add_subplot(111)#ax.scatter(xcord,ycord, c=colors, s=markers)ax.scatter(xcord0,ycord0, marker='^', s=90)ax.scatter(xcord1,ycord1, marker='o', s=50, c='red')plt.plot([0,1], label='going up')plt.show()4.3 monoDemo.py'''Created on Oct 6, 2010Shows montonocity of a function and the log of that function @author: Peter '''from numpy import *import matplotlibimport matplotlib.pyplot as pltt = arange(0.0, 0.5, 0.01)fig = plt.figure()ax = fig.add_subplot(211)ax.set_ylabel('f(x)')ax.set_xlabel('x')ax = fig.add_subplot(212)ax.plot(t,logS)ax.set_ylabel('ln(f(x))')ax.set_xlabel('x')plt.show()5 Logistic回归5.1 logRegres.py'''Created on Oct 27, 2010Logistic Regression Working Module@author: Peter'''from numpy import *def loadDataSet():dataMat = []; labelMat = []fr = open('testSet.txt')for line in fr.readlines():lineArr = line.strip().split()dataMat.append([1.0, float(lineArr[0]), float(lineArr[1])]) labelMat.append(int(lineArr[2]))return dataMat,labelMatdef sigmoid(inX):return 1.0/(1+exp(-inX))def gradAscent(dataMatIn, classLabels):dataMatrix = mat(dataMatIn) #convert to NumPy matrixlabelMat = mat(classLabels).transpose() #convert to NumPy matrix m,n = shape(dataMatrix)alpha = 0.001maxCycles = 500for k in range(maxCycles): #heavy on matrix operationsh = sigmoid(dataMatrix*weights) #matrix multerror = (labelMat - h) #vector subtractionweights = weights + alpha * dataMatrix.transpose()* error #matrix mult return weights def plotBestFit(weights):。
AbaqusUSDFLD使用教程
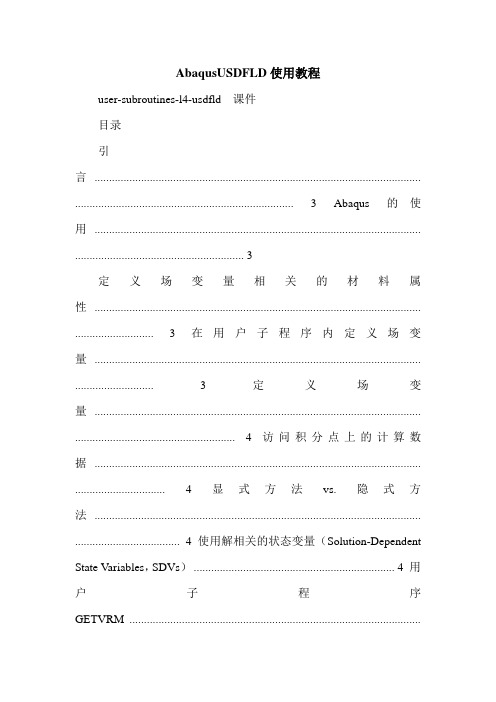
AbaqusUSDFLD使用教程user-subroutines-l4-usdfld 课件目录引言................................................................................................................ ........................................................................... 3 Abaqus的使用................................................................................................................ . (3)定义场变量相关的材料属性................................................................................................................ ........................... 3 在用户子程序内定义场变量................................................................................................................ ........................... 3 定义场变量................................................................................................................ ....................................................... 4 访问积分点上的计算数据................................................................................................................ ............................... 4 显式方法vs. 隐式方法................................................................................................................ .................................... 4 使用解相关的状态变量(Solution-Dependent State Variables,SDVs)..................................................................... 4 用户子程序GETVRM .................................................................................................... (4)GETVRM子程序界面................................................................................................................ ..................................... 5 提供给GETVRM的变量................................................................................................................ ................................ 5 GETVRM返回的变量................................................................................................................ ..................................... 5 GETVRM所支持的单元................................................................................................................ ................................. 5 USDFLD子程序界面.................................................................................................................. (5)需定义的变量................................................................................................................ ................................................... 6 可能被定义的变量................................................................................................................ ........................................... 6 变量信息................................................................................................................ ........................................................... 6 USDFLD与自动时间增量................................................................................................................ ............................... 7 实例:层状复合板失效................................................................................................................ (7)材料模型................................................................................................................ ........................................................... 8 基体拉伸开裂................................................................................................................ ................................................... 9 基体压缩开裂................................................................................................................ ................................................... 9 纤维-基体剪切失效................................................................................................................ .......................................... 9 部分输入数据................................................................................................................ ................................................. 10 用户子程序................................................................................................................ ..................................................... 10 结果................................................................................................................ ................................................................. 10 备注................................................................................................................ ................................................................. 10 1.1.49 USDFLD: User subroutine to redefine field variables at a material point.. (11)参考文献......................................................................................................................................................................... 11 概述................................................................................................................ ................................................................. 11 明确的解依赖性................................................................................................................ ............................................. 11 定义场变量................................................................................................................ ..................................................... 11 访问材料点数据................................................................................................................ ............................................. 12 状态变量................................................................................................................ ......................................................... 12 用户子程序界面................................................................................................................ ............................................. 12 定义的变量................................................................................................................ ..................................................... 12 能够更新的变量................................................................................................................ ............................................. 12 传递信息的变量................................................................................................................ ............................................. 13 实例:损伤弹性模型................................................................................................................ ..................................... 14 2.1.6 Obtaining material point information in an Abaqus/Standardanalysis (15)参考文献................................................................................................................ ......................................................... 15 概述................................................................................................................ ................................................................. 16 界面................................................................................................................ ................................................................. 16 提供给实用程序的变量................................................................................................................ (16)从实用程序中返回的变量................................................................................................................ ............................. 16 可用的输出变量钥匙................................................................................................................ ..................................... 16 返回分量的顺序................................................................................................................ ............................................. 17 返回值的分析时间................................................................................................................ ......................................... 17 返回值的平衡状态................................................................................................................ ......................................... 17 实例................................................................................................................................................................................. 17 访问状态依赖变量................................................................................................................ ......................................... 17 不支持的单元类型、进程与输出变量钥匙.................................................................................................................17引言·通常使用用户子程序USDFLD,当需要对复杂材料行为建模与用户不想要使用UMAT子程序时——在ABAQUS/Standard中的大多数材料属性可以被定为场变量的函数——子程序USDFLD允许在单元的每个积分点定义——子程序可以访问计算结果数据,因此,材料属性可以是计算结果数据的函数·子程序USDFLD只能使用在具有*Material选项的材料属性的单元(详见p. L4.18页上GETVRM所支持的单元)Abaqus的使用·在模型中,与DLOAD和FILM子程序相比,包括USDFLD在内的子程序需要付出更多的努力·通常用户必须定义材料属性的依赖性,例如弹性模量或屈服应力,作为场变量的函数——这可以通过表格输入或额外用户子程序来完成·使用子程序USDFLD来在积分点上定义的值——在材料定义中包括了?USER DEFINED FIELD 选项,这表明对于使用材料定义的这些单元来说,USDFLD子程序可以使用——可以被定义为在积分点上有的计算结果数据的函数,例如应力、应变定义场变量相关的材料属性这里有两种方法,能够定义场变量相关的材料属性·对于Abaqus 内置的材料模型,使用表格定义方式·使用其他用户子程序来定义材料属性为的函数,例如蠕变CREEP表格定义·使用在材料选项上DEPENDENCIES选项来指定对于给定材料选项存在有多少不同场变量——弹性模量(E)是场变量#1(f1)的函数。