Recommendation Based Discovery of Dynamic Virtual Communities
CCSA-基础词汇

CCSA-基础词汇CCSA基本词汇Aacceptance testing 验收测试access存取、访问access control访问控制accountability责任Accounting controls 会计控制achieve达到activity活动activity network diagram作业网络图activity-based costing(ABC)system 作业成本法activity-level objective作业层次目标Activity reports活动报告add value增加价值adequacy充分,足够;适当adequate controlAnalysis and Evaluation(IIAPreformance Standard 2320)分析和评价(IIA工作标准2320)Analysis audit procedures分析性审计程序analytical review分析性复核anonymity匿名,匿名者appearance表面application应用appraisal costs鉴定成本appreciation评价,鉴别appropriate适当的appropriation拨款,占用,盗用,挪用approve批准Approval批准Approval of risk-based plans (批准)以风险为导向的计划Approval of work programs (批准)工作方案artificial intelligence人工智能assess对??????进行评估,评价assessment评估Assessment control控制(评估)Assessment of control processes控制程序(评估)Assessment definition/timing of定义/时间安排(评估)Assessment of quality programs质量方案(评估)Assessment of risk management process风险管理过程(评估)Assets,control and ues of资产、控制和使用Assignment of authority and responsibility 权责划分assistance援助,帮助assistant辅助的,助理的assumption假设assurance确认、保证Assurance Services确认服务Assurance Services and consulting services确认服务和咨询服务Assurance Services nature of确认服务的性质Asymmetrical Digital Subscriber Line(ADSL) 非对称用户数字环线asynchronous异步attribute Standards属性标准attributes sampling属性抽样audience受众audit conclusion审计结论audit committees审计委员会communications with audit committees与审计委员会的沟通audit coverage审计覆盖面audit directors审计主管audit directors compliance with IIA’s attribute standards(审计主管)遵循IIA的属性标准duties of audit directors审计主管责任personnel responsibilities of audit directors人事责任planning by audit directors规划audit directors and policies/procedures 审计主管和政策/程序quality assurance role of audit directors 质量保障角色auditing control审计控制audit managers/supervisors审计经理/督导audit evidence审计证据audit finding审计发现audit methodology审计方法audit objectives审计目标auditors-in-charge主管审计师audit plans/planning审计计划audit procedures审计程序audit programs审计方案assessments to audit programs 评估审计方案audit recommendation审计建议audit reports审计报告audit resources审计资源audit risk审计风险audit scope审计范围audit team leaders 审计小组领导audit time budgets 审计时间预算audit trail审计踪迹audit work planning 审计工作计划audit work programs 审计工作方案auditee被审计单位authentication鉴别authority权威性,权限authorization授权avoid避免awareness意识Bbackup/restart procedure 备份/重启程序balance controls余额控制bar chart条形图,柱状图bar-code条码BBS电子公告牌benchmarking基准比较法biometric technology生物技术Board董事会(审计委员会)internal control responsibilities of board of directors(董事会的)内部控制责任bounded rationality有限理性brainstorming头脑风暴break-even盈亏平衡点bridge网桥browser浏览器bus network总线网business application systems业务应用系统see also internal control application development内部控制应用程序开发application system documentation control应用系统文本记录控制corrective controls纠正性控制data integrity controls数据完整性控制data origination/preparation/input controls 数据产生/编制/输入控制data output controls数据输出控制data processing controls数据处理控制detective controls检查性控制inventory of controls in存货控制operational application system controls操作应用系统控制preventive controls预防性控制spreadsheet controls电子数据表控制system-related file maintenance controls 系统相关文档维护控制business organizations经营组织business process reengineering业务流程再造business risk,audit risk vs.经营风险,审计风险Ccallback回拨capacity plan能力计划capital structure资本结构cause原因cause-and-effect diagrams因果图centralized processing集中处理certainty确定性certification authority(CA)证书认证中心Certified Internal Auditor(CIA) 国际注册内部审计师challenge挑战change control变更控制change management变革管理characteristics特性charter章程Check Sheet日常检查单checklists问题清单Chief Audit Executive(CAE) 首席审计执行官circumstantial evidence附属证据class类client委托人,客户,客户机client feedback mechanisms客户反馈机制closing conference结束会议cluster(block)sampling分块抽样CoCo model,see Criteria of control model 控制标准模式Code of Ethics职业道德规范monitoring compliance with 监控合规性coefficient of correlation相关系数coefficient of determination 决定系数cold site冷站collection收款combination controls合并控制comment观点,评论Committee委员会Committee of Sponsoring Organizations(COSO) 发起组织委员会Committee structure委员会结构communicating results沟通结果communication and internal control沟通和内部控制Compact Disc/Read-Only Memory(CD-ROM) 紧凑式只读光盘comparison比较comparison controls比较控制compensating controls 补偿性控制competency能力,胜任competent能胜任的,足够的competitive bid竞标compiler编译器complexity复杂性compliance遵循/合规性legal considerations in evaluating programs for compliance针对合规性评价方案的法律考虑事项compliance monitoring监控compliance audit合规性审计compliance test符合性测试computation controls计算控制computer-assisted audit technique计算机辅助审计技术computer controls计算机控制concerns关注点concise简洁conclusive evidence确证证据concurrency并发concurrent access controls 并行存取控制concurrent control并行控制condition条件conditional probability 条件概率confidence interval置信区间confidence level置信水平confidentiality保密confirmation函证conflicts of interest利益冲突、关注焦点consensus testing一致性测试consistent with与??????一致constructive建设性Consulting Services咨询服务contemporary management controls 现代管理控制contingency design权变理论contingency plan应急计划control控制self-assessment of control自我评估control activities控制活动control assessment控制评估control breakdowns控制崩溃control chart控制图control environment控制环境control files控制文档control report控制报告Control Self-assessment控制自我评价Control Self-assessment(CSA) model控制自我评估(CSA)模式control-based以控制为基础的controller会计部主任cooperation协作corporate governance公司治理corporate governance CoCo model控制标准(CoCo)模式corporate governance control self-assessment model控制自我评估模式corporate governance COCO’s internal control COCO的内部控制corporate governance separation of ownership from control源自控制的所有权分离corrective action纠正行动corrective controls纠正性控制corroborative evidence佐证证据COSO internal control modelCOSO内部控制模式cost of assurance保证成本cost of quality质量成本cost-volume-profit analysis 本-量-利分析credit committee信贷委员会criteria标准Critical Path Method(CPM) 关键路径法critical thinking关键思考cross-referencing交叉索引current当前、流动current ratio流动比率Cyclical Redundancy Checking(CRC) 循环冗余检验Ddata mining数据挖掘data processing controls数据处理控制data synthesis数据统计database数据库deadly embrace死锁debugger调试器decentralized processing 分散处理decision-tree analysis决策树分析decline谢绝,下降deduction扣除额;演绎;推论deficiency缺陷delegation授权Delphi techniques 德尔菲技术departmentalization 部门化depend upon取决于dependency check 相关性检验design设计detailed testing详细测试detect发掘,侦查detective control检查型控制diagnostic诊断difference estimation sampling 差额估计抽样digital certificate数字证书Digital Data Network(DDN) 数字数据网络digital signature数字签名direct cutover strategy直接转换策略direct evidence直接证据direct-access file直接存取文件directed sampling定向抽样directive control指导型控制disclosure披露discount折扣discovery sampling发现抽样discretionary access control 自主访问控制discriminate analysis 辨别分析disk磁盘disk utility磁盘工具distributed processing 分布处理distribution分发diversify分散,多样化division of labor劳动分工divisional structure分布型结构documental information 文件信息dollar-unit sampling货币单位抽样download下载dounsizing降型化draft草稿Due Professional Care 应有的职业审慎性dumb terminal哑终端Dynamic Link Libraries(DLL)动态链接库dynamic programming动态规划EE-commerce activities电子商务活动risk/control issues with E-commerce activities 电子商务活动风险/控制问题Economic Order Quantity(EOQ)经济订货量effect效果effectiveness有效性Electronic Data Interchange(EDI) 电子数据转换Electronic Data Process(EDP)电子数据处理Electronic Funds Transfer(EFT) 电子资金转账electronic voting电子投票embedded audit module嵌入式审计模块employees雇员responsibilities of employees雇员职责empowerment授权endorse背书end-user终端用户End-User Computing(EUC) 终端用户计算engagement审计业务engagement area审计业务范围engagement client审计业务客户engagement conclusion审计业务结论engagement information审计业务信息engagement observation审计业务观察结果engagement recommendation 审计业务建议engagement result审计业务结果enhance提高、加强environmental audits环境审计equal-weight加权平均ethics伦理/道德ethics monitoring compliance with code of conduct对行为规范遵循性的监控evaluate评估,评价evidence证据exception report例外报告existence check存在性检验exit conference退出会议expectation期望expected value期望值expert system专家系统expertise专长exponential smoothing 指数平滑exposure暴露extent延伸external assessment外部评估external information外部信息external-internal information 外-内信息Ffacilitated team workshops 推动型专题讨论会fail-soft protection故障弱化保护failure cost失败成本FDIC Improvement Act联邦存款保险公司改进法feasibility可行性feedback payments反馈控制feed-forward control前馈控制field字段final audit report最终审计报告final engagement communication 最终审计业务沟通financial control systems财务控制系统firewall防火墙fishbone diagram鱼骨图flat file平面文件flat organizations扁平化组织flexible budgeting弹性预算flowcharting流程图focus groups专题小组follow-up by internal auditors 后续审计follow -up后续follow -up review 跟踪检查format check格式检验Fragmentation 分割frame relay帧中继framework框架fraud舞弊fraud auditing 舞弊审计。
用密度函数理论和杜比宁方程研究活性炭纤维多段充填机理

密度函数理论和杜比宁方程可以用来研究活性炭纤维在多段充填过程中的吸附行为。
密度函数理论是一种分子统计力学理论,它建立在分子统计学和热力学的基础上,用来研究一种系统中分子的分布。
杜比宁方程是一种描述分子吸附行为的方程,它可以用来计算吸附层的厚度、吸附速率和吸附能量等参数。
在研究活性炭纤维多段充填过程中,可以使用密度函数理论和杜比宁方程来研究纤维表面的分子结构和吸附行为。
通过分析密度函数和杜比宁方程的解,可以得出纤维表面的分子结构以及纤维吸附的分子的种类、数量和能量。
这些信息有助于更好地理解活性炭纤维的多段充填机理。
在研究活性炭纤维的多段充填机理时,还可以使用其他理论和方法来帮助我们更好地了解这一过程。
例如,可以使用扫描电子显微镜(SEM)和透射电子显微镜(TEM)等技术来观察纤维表面的形貌和结构。
可以使用X射线衍射(XRD)和傅里叶变换红外光谱(FTIR)等技术来确定纤维表面的化学成分和结构。
还可以使用氮气吸附(BET)和旋转氧吸附(BJH)等技术来测量纤维表面的比表面积和孔结构。
通过综合运用密度函数理论、杜比宁方程和其他理论和方法,可以更全面地了解活性炭纤维的多段充填机理,从而更好地控制和优化多段充填的过程。
在研究活性炭纤维多段充填机理时,还可以使用温度敏感性测试方法来研究充填过程中纤维表面的动力学性质。
例如,可以使用动态氧吸附(DAC)或旋转杆氧吸附(ROTA)等技术来测量温度对纤维表面吸附性能的影响。
通过对比不同温度下纤维表面的吸附性能,可以更好地了解充填过程中纤维表面的动力学性质。
此外,还可以使用分子动力学模拟方法来研究纤维表面的吸附行为。
例如,可以使用拉曼光谱或红外光谱等技术来测量纤维表面的分子吸附构型。
然后,使用分子动力学模拟方法来模拟不同分子吸附构型下的纤维表面的动力学性质,帮助我们更好地了解活性炭纤维的多段充填机理。
SCItop区期刊表
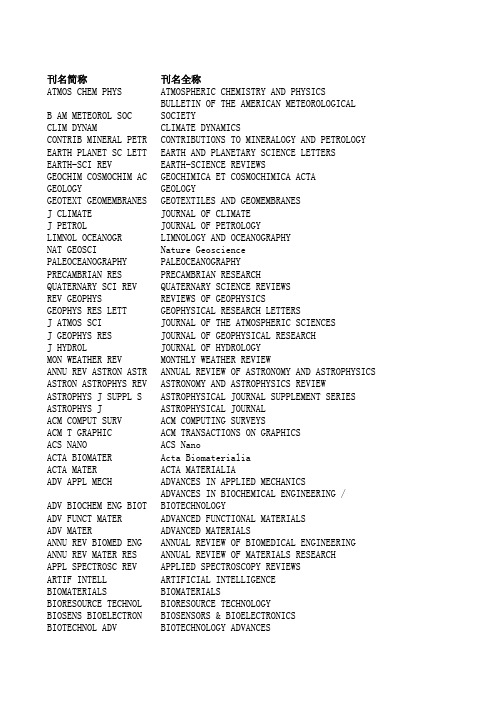
刊名简称刊名全称ATMOS CHEM PHYS ATMOSPHERIC CHEMISTRY AND PHYSICSB AM METEOROL SOC BULLETIN OF THE AMERICAN METEOROLOGICAL SOCIETY CLIM DYNAM CLIMATE DYNAMICSCONTRIB MINERAL PETR CONTRIBUTIONS TO MINERALOGY AND PETROLOGY EARTH PLANET SC LETT EARTH AND PLANETARY SCIENCE LETTERSEARTH-SCI REV EARTH-SCIENCE REVIEWSGEOCHIM COSMOCHIM AC GEOCHIMICA ET COSMOCHIMICA ACTAGEOLOGY GEOLOGYGEOTEXT GEOMEMBRANES GEOTEXTILES AND GEOMEMBRANESJ CLIMATE JOURNAL OF CLIMATEJ PETROL JOURNAL OF PETROLOGYLIMNOL OCEANOGR LIMNOLOGY AND OCEANOGRAPHYNAT GEOSCI Nature GeosciencePALEOCEANOGRAPHY PALEOCEANOGRAPHYPRECAMBRIAN RES PRECAMBRIAN RESEARCHQUATERNARY SCI REV QUATERNARY SCIENCE REVIEWSREV GEOPHYS REVIEWS OF GEOPHYSICSGEOPHYS RES LETT GEOPHYSICAL RESEARCH LETTERSJ ATMOS SCI JOURNAL OF THE ATMOSPHERIC SCIENCESJ GEOPHYS RES JOURNAL OF GEOPHYSICAL RESEARCHJ HYDROL JOURNAL OF HYDROLOGYMON WEATHER REV MONTHLY WEATHER REVIEWANNU REV ASTRON ASTR ANNUAL REVIEW OF ASTRONOMY AND ASTROPHYSICS ASTRON ASTROPHYS REV ASTRONOMY AND ASTROPHYSICS REVIEWASTROPHYS J SUPPL S ASTROPHYSICAL JOURNAL SUPPLEMENT SERIES ASTROPHYS J ASTROPHYSICAL JOURNALACM COMPUT SURV ACM COMPUTING SURVEYSACM T GRAPHIC ACM TRANSACTIONS ON GRAPHICSACS NANO ACS NanoACTA BIOMATER Acta BiomaterialiaACTA MATER ACTA MATERIALIAADV APPL MECH ADVANCES IN APPLIED MECHANICSADV BIOCHEM ENG BIOT ADVANCES IN BIOCHEMICAL ENGINEERING / BIOTECHNOL ADV FUNCT MATER ADVANCED FUNCTIONAL MATERIALSADV MATER ADVANCED MATERIALSANNU REV BIOMED ENG ANNUAL REVIEW OF BIOMEDICAL ENGINEERINGANNU REV MATER RES ANNUAL REVIEW OF MATERIALS RESEARCHAPPL SPECTROSC REV APPLIED SPECTROSCOPY REVIEWSARTIF INTELL ARTIFICIAL INTELLIGENCEBIOMATERIALS BIOMATERIALSBIORESOURCE TECHNOL BIORESOURCE TECHNOLOGYBIOSENS BIOELECTRON BIOSENSORS & BIOELECTRONICSBIOTECHNOL ADV BIOTECHNOLOGY ADVANCESBIOTECHNOL BIOENG BIOTECHNOLOGY AND BIOENGINEERINGBIOTECHNOL BIOFUELS Biotechnology for BiofuelsCARBON CARBONCHEM MATER CHEMISTRY OF MATERIALSCOMPUT INTELL COMPUTATIONAL INTELLIGENCECRIT REV BIOTECHNOL CRITICAL REVIEWS IN BIOTECHNOLOGYCRIT REV FOOD SCI CRITICAL REVIEWS IN FOOD SCIENCE AND NUTRITION CURR OPIN BIOTECH CURRENT OPINION IN BIOTECHNOLOGY ELECTROCHEM COMMUN ELECTROCHEMISTRY COMMUNICATIONSHUM-COMPUT INTERACT HUMAN-COMPUTER INTERACTIONIEEE J SEL AREA COMM IEEE JOURNAL ON SELECTED AREAS IN COMMUNICATIONS IEEE SIGNAL PROC MAG IEEE SIGNAL PROCESSING MAGAZINEIEEE T EVOLUT COMPUT IEEE TRANSACTIONS ON EVOLUTIONARY COMPUTATION IEEE T IND ELECTRON IEEE TRANSACTIONS ON INDUSTRIAL ELECTRONICS IEEE T NEURAL NETWOR IEEE TRANSACTIONS ON NEURAL NETWORKSIEEE T PATTERN ANAL IEEE TRANSACTIONS ON PATTERN ANALYSIS AND MACHIN IEEE T SOFTWARE ENG IEEE TRANSACTIONS ON SOFTWARE ENGINEERINGINT J COMPUT VISION INTERNATIONAL JOURNAL OF COMPUTER VISIONINT J HYDROGEN ENERG INTERNATIONAL JOURNAL OF HYDROGEN ENERGYINT J NONLIN SCI NUM INTERNATIONAL JOURNAL OF NONLINEAR SCIENCES AND INT J PLASTICITY INTERNATIONAL JOURNAL OF PLASTICITYINT MATER REV INTERNATIONAL MATERIALS REVIEWSJ CATAL JOURNAL OF CATALYSISJ HAZARD MATER JOURNAL OF HAZARDOUS MATERIALSJ MATER CHEM JOURNAL OF MATERIALS CHEMISTRYJ MECH BEHAV BIOMED Journal of the Mechanical Behavior of Biomedical J NEURAL ENG Journal of Neural EngineeringJ POWER SOURCES JOURNAL OF POWER SOURCESJ WEB SEMANT Journal of Web SemanticsLAB CHIP LAB ON A CHIPMACROMOL RAPID COMM MACROMOLECULAR RAPID COMMUNICATIONS MACROMOLECULES MACROMOLECULESMAT SCI ENG R MATERIALS SCIENCE & ENGINEERING R-REPORTS MATER TODAY Materials TodayMED IMAGE ANAL MEDICAL IMAGE ANALYSISMETAB ENG METABOLIC ENGINEERINGMIS QUART MIS QUARTERLYMOL NUTR FOOD RES MOLECULAR NUTRITION & FOOD RESEARCHMRS BULL MRS BULLETINNANO LETT NANO LETTERSNANO TODAY Nano TodayNANOMED-NANOTECHNOL Nanomedicine-Nanotechnology Biology and Medicine NANOTECHNOLOGY NANOTECHNOLOGYNAT BIOTECHNOL NATURE BIOTECHNOLOGYNAT MATER NATURE MATERIALSNAT NANOTECHNOL Nature NanotechnologyORG ELECTRON ORGANIC ELECTRONICSP IEEE PROCEEDINGS OF THE IEEEPLASMONICS PlasmonicsPOLYM REV Polymer ReviewsPROG CRYST GROWTH CH PROGRESS IN CRYSTAL GROWTH AND CHARACTERIZATION PROG ENERG COMBUST PROGRESS IN ENERGY AND COMBUSTION SCIENCEPROG MATER SCI PROGRESS IN MATERIALS SCIENCEPROG PHOTOVOLTAICS PROGRESS IN PHOTOVOLTAICSPROG QUANT ELECTRON PROGRESS IN QUANTUM ELECTRONICSPROG SURF SCI PROGRESS IN SURFACE SCIENCERENEW SUST ENERG REV RENEWABLE & SUSTAINABLE ENERGY REVIEWSSMALL SMALLSOFT MATTER Soft MatterTRENDS BIOTECHNOL TRENDS IN BIOTECHNOLOGYTRENDS FOOD SCI TECH TRENDS IN FOOD SCIENCE & TECHNOLOGYVLDB J VLDB JOURNALAICHE J AICHE JOURNALAPPL MICROBIOL BIOT APPLIED MICROBIOLOGY AND BIOTECHNOLOGYCHEM ENG SCI CHEMICAL ENGINEERING SCIENCEELECTROCHIM ACTA ELECTROCHIMICA ACTAFOOD CHEM FOOD CHEMISTRYIEEE T ANTENN PROPAG IEEE TRANSACTIONS ON ANTENNAS AND PROPAGATION IEEE T AUTOMAT CONTR IEEE TRANSACTIONS ON AUTOMATIC CONTROLIEEE T INFORM THEORY IEEE TRANSACTIONS ON INFORMATION THEORYIEEE T MICROW THEORY IEEE TRANSACTIONS ON MICROWAVE THEORY AND TECHNI IEEE T SIGNAL PROCES IEEE TRANSACTIONS ON SIGNAL PROCESSINGIND ENG CHEM RES INDUSTRIAL & ENGINEERING CHEMISTRY RESEARCHINT J HEAT MASS TRAN INTERNATIONAL JOURNAL OF HEAT AND MASS TRANSFER J AM CERAM SOC JOURNAL OF THE AMERICAN CERAMIC SOCIETYJ ELECTROCHEM SOC JOURNAL OF THE ELECTROCHEMICAL SOCIETYJ MEMBRANE SCI JOURNAL OF MEMBRANE SCIENCEMATER LETT MATERIALS LETTERSSCRIPTA MATER SCRIPTA MATERIALIASENSOR ACTUAT B-CHEM SENSORS AND ACTUATORS B-CHEMICALSURF COAT TECH SURFACE & COATINGS TECHNOLOGYSYNTHETIC MET SYNTHETIC METALSTHIN SOLID FILMS THIN SOLID FILMSJ OPER MANAG JOURNAL OF OPERATIONS MANAGEMENTMANAGE SCI MANAGEMENT SCIENCEOMEGA-INT J MANAGE S OMEGA-INTERNATIONAL JOURNAL OF MANAGEMENT SCIENC PROD OPER MANAG PRODUCTION AND OPERATIONS MANAGEMENTEUR J OPER RES EUROPEAN JOURNAL OF OPERATIONAL RESEARCH ACCOUNTS CHEM RES ACCOUNTS OF CHEMICAL RESEARCHACTA CRYSTALLOGR A ACTA CRYSTALLOGRAPHICA SECTION AALDRICHIM ACTA ALDRICHIMICA ACTAANGEW CHEM INT EDIT ANGEWANDTE CHEMIE-INTERNATIONAL EDITIONANNU REV PHYS CHEM ANNUAL REVIEW OF PHYSICAL CHEMISTRYCATAL REV CATALYSIS REVIEWS-SCIENCE AND ENGINEERINGCHEM REV CHEMICAL REVIEWSCHEM SOC REV CHEMICAL SOCIETY REVIEWSCOORDIN CHEM REV COORDINATION CHEMISTRY REVIEWSENERG ENVIRON SCI Energy & Environmental ScienceINT REV PHYS CHEM INTERNATIONAL REVIEWS IN PHYSICAL CHEMISTRYJ AM CHEM SOC JOURNAL OF THE AMERICAN CHEMICAL SOCIETYJ PHOTOCH PHOTOBIO C JOURNAL OF PHOTOCHEMISTRY AND PHOTOBIOLOGY C-PHO NAT PROD REP NATURAL PRODUCT REPORTSPROG POLYM SCI PROGRESS IN POLYMER SCIENCESURF SCI REP SURFACE SCIENCE REPORTSTRAC-TREND ANAL CHEM TRAC-TRENDS IN ANALYTICAL CHEMISTRYANAL CHEM ANALYTICAL CHEMISTRYJ ORG CHEM JOURNAL OF ORGANIC CHEMISTRYJ PHYS CHEM B JOURNAL OF PHYSICAL CHEMISTRY BLANGMUIR LANGMUIRAPPL CATAL B-ENVIRON APPLIED CATALYSIS B-ENVIRONMENTALB AM MUS NAT HIST BULLETIN OF THE AMERICAN MUSEUM OF NATURAL HISTO CRIT REV ENV SCI TEC CRITICAL REVIEWS IN ENVIRONMENTAL SCIENCE AND TE ECOL LETT ECOLOGY LETTERSECOL MONOGR ECOLOGICAL MONOGRAPHSECOLOGY ECOLOGYENVIRON HEALTH PERSP ENVIRONMENTAL HEALTH PERSPECTIVESENVIRON MICROBIOL ENVIRONMENTAL MICROBIOLOGYEVOLUTION EVOLUTIONFRONT ECOL ENVIRON FRONTIERS IN ECOLOGY AND THE ENVIRONMENT GLOBAL CHANGE BIOL GLOBAL CHANGE BIOLOGYGLOBAL ECOL BIOGEOGR GLOBAL ECOLOGY AND BIOGEOGRAPHYISME J ISME JournalATMOS ENVIRON ATMOSPHERIC ENVIRONMENTCHEMOSPHERE CHEMOSPHEREENVIRON SCI TECHNOL ENVIRONMENTAL SCIENCE & TECHNOLOGYWATER RES WATER RESEARCHADV AGRON ADVANCES IN AGRONOMYAGR FOREST METEOROL AGRICULTURAL AND FOREST METEOROLOGYATLA-ALTERN LAB ANIM ATLA-ALTERNATIVES TO LABORATORY ANIMALSEUR J SOIL SCI EUROPEAN JOURNAL OF SOIL SCIENCEFISH FISH FISH AND FISHERIESFISH OCEANOGR FISHERIES OCEANOGRAPHYFISH SHELLFISH IMMUN FISH & SHELLFISH IMMUNOLOGYFOOD BIOPROCESS TECH Food and Bioprocess TechnologyGEODERMA GEODERMAILAR J ILAR JOURNALJ AGR FOOD CHEM JOURNAL OF AGRICULTURAL AND FOOD CHEMISTRYJ ANIM SCI JOURNAL OF ANIMAL SCIENCEJ DAIRY SCI JOURNAL OF DAIRY SCIENCEMOL BREEDING MOLECULAR BREEDINGREV FISH BIOL FISHER REVIEWS IN FISH BIOLOGY AND FISHERIESSOIL BIOL BIOCHEM SOIL BIOLOGY & BIOCHEMISTRYSOIL SCI SOC AM J SOIL SCIENCE SOCIETY OF AMERICA JOURNALTREE PHYSIOL TREE PHYSIOLOGYVET MICROBIOL VETERINARY MICROBIOLOGYVET RES VETERINARY RESEARCHAQUACULTURE AQUACULTURECAN J FISH AQUAT SCI CANADIAN JOURNAL OF FISHERIES AND AQUATIC SCIENC FOREST ECOL MANAG FOREST ECOLOGY AND MANAGEMENTJAVMA-J AM VET MED A JAVMA-JOURNAL OF THE AMERICAN VETERINARY MEDICAL PLANT SOIL PLANT AND SOILAM J BIOETHICS AMERICAN JOURNAL OF BIOETHICSECONOMETRICA ECONOMETRICAJ ECONOMETRICS JOURNAL OF ECONOMETRICSAM J HUM GENET AMERICAN JOURNAL OF HUMAN GENETICSANNU REV BIOCHEM ANNUAL REVIEW OF BIOCHEMISTRYANNU REV BIOPH BIOM ANNUAL REVIEW OF BIOPHYSICS AND BIOMOLECULAR STR ANNU REV BIOPHYS Annual Review of BiophysicsANNU REV CELL DEV BI ANNUAL REVIEW OF CELL AND DEVELOPMENTAL BIOLOGY ANNU REV ECOL EVOL S ANNUAL REVIEW OF ECOLOGY EVOLUTION AND SYSTEMATI ANNU REV ENTOMOL ANNUAL REVIEW OF ENTOMOLOGYANNU REV GENET ANNUAL REVIEW OF GENETICSANNU REV GENOM HUM G ANNUAL REVIEW OF GENOMICS AND HUMAN GENETICS ANNU REV MICROBIOL ANNUAL REVIEW OF MICROBIOLOGYANNU REV PHYTOPATHOL ANNUAL REVIEW OF PHYTOPATHOLOGYANNU REV PLANT BIOL ANNUAL REVIEW OF PLANT BIOLOGYCELL CELLCELL HOST MICROBE Cell Host & MicrobeCELL METAB Cell MetabolismCELL STEM CELL Cell Stem CellCRIT REV BIOCHEM MOL CRITICAL REVIEWS IN BIOCHEMISTRY AND MOLECULAR B CURR BIOL CURRENT BIOLOGYCURR OPIN CELL BIOL CURRENT OPINION IN CELL BIOLOGYCURR OPIN GENET DEV CURRENT OPINION IN GENETICS & DEVELOPMENTCURR OPIN PLANT BIOL CURRENT OPINION IN PLANT BIOLOGYCURR OPIN STRUC BIOL CURRENT OPINION IN STRUCTURAL BIOLOGYDEV CELL DEVELOPMENTAL CELLGENE DEV GENES & DEVELOPMENTGENOME RES GENOME RESEARCHJ CELL BIOL JOURNAL OF CELL BIOLOGYMICROBIOL MOL BIOL R MICROBIOLOGY AND MOLECULAR BIOLOGY REVIEWSMOL CELL MOLECULAR CELLMOL SYST BIOL Molecular Systems BiologyNAT CELL BIOL NATURE CELL BIOLOGYNAT CHEM BIOL Nature Chemical BiologyNAT GENET NATURE GENETICSNAT METHODS NATURE METHODSNAT REV GENET NATURE REVIEWS GENETICSNAT REV MICROBIOL NATURE REVIEWS MICROBIOLOGYNAT REV MOL CELL BIO NATURE REVIEWS MOLECULAR CELL BIOLOGYNAT STRUCT MOL BIOL NATURE STRUCTURAL & MOLECULAR BIOLOGYPLANT CELL PLANT CELLPLOS BIOL PLOS BIOLOGYPLOS GENET PLoS GeneticsPLOS PATHOG PLoS PathogensPROG LIPID RES PROGRESS IN LIPID RESEARCHQ REV BIOPHYS QUARTERLY REVIEWS OF BIOPHYSICSTRENDS BIOCHEM SCI TRENDS IN BIOCHEMICAL SCIENCESTRENDS CELL BIOL TRENDS IN CELL BIOLOGYTRENDS ECOL EVOL TRENDS IN ECOLOGY & EVOLUTIONTRENDS GENET TRENDS IN GENETICSTRENDS PLANT SCI TRENDS IN PLANT SCIENCEAPPL ENVIRON MICROB APPLIED AND ENVIRONMENTAL MICROBIOLOGY BIOCHEM J BIOCHEMICAL JOURNALBIOPHYS J BIOPHYSICAL JOURNALDEVELOPMENT DEVELOPMENTEMBO J EMBO JOURNALJ BACTERIOL JOURNAL OF BACTERIOLOGYJ BIOL CHEM JOURNAL OF BIOLOGICAL CHEMISTRYJ MOL BIOL JOURNAL OF MOLECULAR BIOLOGYMOL CELL BIOL MOLECULAR AND CELLULAR BIOLOGYNUCLEIC ACIDS RES NUCLEIC ACIDS RESEARCHPLANT PHYSIOL PLANT PHYSIOLOGYACTA MATH-DJURSHOLM ACTA MATHEMATICAANN MATH ANNALS OF MATHEMATICSANN STAT ANNALS OF STATISTICSB AM MATH SOC BULLETIN OF THE AMERICAN MATHEMATICAL SOCIETY COMMUN PUR APPL MATH COMMUNICATIONS ON PURE AND APPLIED MATHEMATICS FOUND COMPUT MATH FOUNDATIONS OF COMPUTATIONAL MATHEMATICS INVENT MATH INVENTIONES MATHEMATICAEINVERSE PROBL INVERSE PROBLEMSJ AM MATH SOC JOURNAL OF THE AMERICAN MATHEMATICAL SOCIETYJ AM STAT ASSOC JOURNAL OF THE AMERICAN STATISTICAL ASSOCIATION J R STAT SOC B JOURNAL OF THE ROYAL STATISTICAL SOCIETY SERIES MATH MOD METH APPL S MATHEMATICAL MODELS & METHODS IN APPLIED SCIENCE MATH PROGRAM MATHEMATICAL PROGRAMMINGMEM AM MATH SOC MEMOIRS OF THE AMERICAN MATHEMATICAL SOCIETY MULTISCALE MODEL SIM MULTISCALE MODELING & SIMULATIONMULTIVAR BEHAV RES MULTIVARIATE BEHAVIORAL RESEARCHNONLINEAR ANAL-REAL NONLINEAR ANALYSIS-REAL WORLD APPLICATIONSRISK ANAL RISK ANALYSISSIAM REV SIAM REVIEWSTAT SCI STATISTICAL SCIENCESTRUCT EQU MODELING STRUCTURAL EQUATION MODELING-A MULTIDISCIPLINARY FUZZY SET SYST FUZZY SETS AND SYSTEMSJ COMPUT APPL MATH JOURNAL OF COMPUTATIONAL AND APPLIED MATHEMATICS J DIFFER EQUATIONS JOURNAL OF DIFFERENTIAL EQUATIONSJ MATH ANAL APPL JOURNAL OF MATHEMATICAL ANALYSIS AND APPLICATIONNONLINEAR ANAL-THEOR NONLINEAR ANALYSIS-THEORY METHODS & APPLICATIONS SIAM J NUMER ANAL SIAM JOURNAL ON NUMERICAL ANALYSISSIAM J SCI COMPUT SIAM JOURNAL ON SCIENTIFIC COMPUTINGACTA CRYSTALLOGR A ACTA CRYSTALLOGRAPHICA SECTION AADV PHYS ADVANCES IN PHYSICSANNU REV FLUID MECH ANNUAL REVIEW OF FLUID MECHANICSANNU REV NUCL PART S ANNUAL REVIEW OF NUCLEAR AND PARTICLE SCIENCE CRIT REV SOLID STATE CRITICAL REVIEWS IN SOLID STATE AND MATERIALS SC J HIGH ENERGY PHYS JOURNAL OF HIGH ENERGY PHYSICSLASER PHOTONICS REV Laser & Photonics ReviewsLIVING REV RELATIV Living Reviews in RelativityMASS SPECTROM REV MASS SPECTROMETRY REVIEWSNAT PHOTONICS Nature PhotonicsNAT PHYS Nature PhysicsPHYS REP PHYSICS REPORTS-REVIEW SECTION OF PHYSICS LETTER PHYS REV LETT PHYSICAL REVIEW LETTERSPROG NUCL MAG RES SP PROGRESS IN NUCLEAR MAGNETIC RESONANCE SPECTROSC REP PROG PHYS REPORTS ON PROGRESS IN PHYSICSREV MOD PHYS REVIEWS OF MODERN PHYSICSAPPL PHYS LETT APPLIED PHYSICS LETTERSJ CHEM PHYS JOURNAL OF CHEMICAL PHYSICSPHYS REV B PHYSICAL REVIEW BPHYS REV D PHYSICAL REVIEW DADV DRUG DELIVER REV ADVANCED DRUG DELIVERY REVIEWSADV IMMUNOL ADVANCES IN IMMUNOLOGYAM J CLIN NUTR AMERICAN JOURNAL OF CLINICAL NUTRITIONAM J GASTROENTEROL AMERICAN JOURNAL OF GASTROENTEROLOGYAM J PSYCHIAT AMERICAN JOURNAL OF PSYCHIATRYAM J RESP CRIT CARE AMERICAN JOURNAL OF RESPIRATORY AND CRITICAL CAR AM J TRANSPLANT AMERICAN JOURNAL OF TRANSPLANTATIONANN INTERN MED ANNALS OF INTERNAL MEDICINEANN NEUROL ANNALS OF NEUROLOGYANN RHEUM DIS ANNALS OF THE RHEUMATIC DISEASESANN SURG ANNALS OF SURGERYANNU REV IMMUNOL ANNUAL REVIEW OF IMMUNOLOGYANNU REV MED ANNUAL REVIEW OF MEDICINEANNU REV NEUROSCI ANNUAL REVIEW OF NEUROSCIENCEANNU REV NUTR ANNUAL REVIEW OF NUTRITIONANNU REV PATHOL-MECH Annual Review of Pathology-Mechanisms of Disease ANNU REV PHARMACOL ANNUAL REVIEW OF PHARMACOLOGY AND TOXICOLOGY ANNU REV PHYSIOL ANNUAL REVIEW OF PHYSIOLOGYANNU REV PSYCHOL ANNUAL REVIEW OF PSYCHOLOGYANNU REV PUBL HEALTH ANNUAL REVIEW OF PUBLIC HEALTHARCH GEN PSYCHIAT ARCHIVES OF GENERAL PSYCHIATRYARCH INTERN MED ARCHIVES OF INTERNAL MEDICINEARTERIOSCL THROM VAS ARTERIOSCLEROSIS THROMBOSIS AND VASCULAR BIOLOGY ARTHRITIS RHEUM-US ARTHRITIS AND RHEUMATISMBBA-REV CANCER BIOCHIMICA ET BIOPHYSICA ACTA-REVIEWS ON CANCER BEHAV BRAIN SCI BEHAVIORAL AND BRAIN SCIENCESBIOL PSYCHIAT BIOLOGICAL PSYCHIATRYBLOOD BLOODBLOOD REV BLOOD REVIEWSBRAIN BRAINBRAIN RES REV BRAIN RESEARCH REVIEWSBRIT MED J BRITISH MEDICAL JOURNALCA-CANCER J CLIN CA-A CANCER JOURNAL FOR CLINICIANSCAN MED ASSOC J CANADIAN MEDICAL ASSOCIATION JOURNALCANCER CELL CANCER CELLCANCER METAST REV CANCER AND METASTASIS REVIEWSCANCER RES CANCER RESEARCHCEREB CORTEX CEREBRAL CORTEXCIRC RES CIRCULATION RESEARCHCIRCULATION CIRCULATIONCLIN CANCER RES CLINICAL CANCER RESEARCHCLIN INFECT DIS CLINICAL INFECTIOUS DISEASESCLIN MICROBIOL REV CLINICAL MICROBIOLOGY REVIEWSCLIN PHARMACOL THER CLINICAL PHARMACOLOGY & THERAPEUTICSCRIT CARE MED CRITICAL CARE MEDICINECURR OPIN IMMUNOL CURRENT OPINION IN IMMUNOLOGYCURR OPIN LIPIDOL CURRENT OPINION IN LIPIDOLOGYCURR OPIN NEUROBIOL CURRENT OPINION IN NEUROBIOLOGYCURR OPIN PHARMACOL CURRENT OPINION IN PHARMACOLOGYDIABETES DIABETESDIABETES CARE DIABETES CAREDIABETOLOGIA DIABETOLOGIADRUG DISCOV TODAY DRUG DISCOVERY TODAYDRUG RESIST UPDATE DRUG RESISTANCE UPDATESEMERG INFECT DIS EMERGING INFECTIOUS DISEASESENDOCR REV ENDOCRINE REVIEWSEPIDEMIOL REV EPIDEMIOLOGIC REVIEWSEUR HEART J EUROPEAN HEART JOURNALEUR UROL EUROPEAN UROLOGYFRONT NEUROENDOCRIN FRONTIERS IN NEUROENDOCRINOLOGY GASTROENTEROLOGY GASTROENTEROLOGYGASTROINTEST ENDOSC GASTROINTESTINAL ENDOSCOPYGUT GUTHEPATOLOGY HEPATOLOGYHUM MUTAT HUMAN MUTATIONHUM REPROD UPDATE HUMAN REPRODUCTION UPDATEHYPERTENSION HYPERTENSIONIMMUNITY IMMUNITYIMMUNOL REV IMMUNOLOGICAL REVIEWSJ ALLERGY CLIN IMMUN JOURNAL OF ALLERGY AND CLINICAL IMMUNOLOGYJ AM COLL CARDIOL JOURNAL OF THE AMERICAN COLLEGE OF CARDIOLOGYJ AM SOC NEPHROL JOURNAL OF THE AMERICAN SOCIETY OF NEPHROLOGYJ AUTOIMMUN JOURNAL OF AUTOIMMUNITYJ BONE MINER RES JOURNAL OF BONE AND MINERAL RESEARCHJ CLIN INVEST JOURNAL OF CLINICAL INVESTIGATIONJ CLIN ONCOL JOURNAL OF CLINICAL ONCOLOGYJ EXP MED JOURNAL OF EXPERIMENTAL MEDICINEJ HEPATOL JOURNAL OF HEPATOLOGYJ NATL CANCER I JOURNAL OF THE NATIONAL CANCER INSTITUTEJ NEUROSCI JOURNAL OF NEUROSCIENCEJ NUCL MED JOURNAL OF NUCLEAR MEDICINEJAMA-J AM MED ASSOC JAMA-JOURNAL OF THE AMERICAN MEDICAL ASSOCIATION LANCET LANCETLANCET INFECT DIS LANCET INFECTIOUS DISEASESLANCET NEUROL LANCET NEUROLOGYLANCET ONCOL LANCET ONCOLOGYLEUKEMIA LEUKEMIAMED RES REV MEDICINAL RESEARCH REVIEWSMOL ASPECTS MED MOLECULAR ASPECTS OF MEDICINEMOL PSYCHIATR MOLECULAR PSYCHIATRYNAT CLIN PRACT ONCOL Nature Clinical Practice OncologyNAT IMMUNOL NATURE IMMUNOLOGYNAT MED NATURE MEDICINENAT NEUROSCI NATURE NEUROSCIENCENAT REV CANCER NATURE REVIEWS CANCERNAT REV DRUG DISCOV NATURE REVIEWS DRUG DISCOVERYNAT REV IMMUNOL NATURE REVIEWS IMMUNOLOGYNAT REV NEUROSCI NATURE REVIEWS NEUROSCIENCENEUROLOGY NEUROLOGYNEURON NEURONNEUROPSYCHOPHARMACOL NEUROPSYCHOPHARMACOLOGYNEUROSCI BIOBEHAV R NEUROSCIENCE AND BIOBEHAVIORAL REVIEWSNEW ENGL J MED NEW ENGLAND JOURNAL OF MEDICINEOBES REV Obesity ReviewsONCOGENE ONCOGENEPHARMACOL REV PHARMACOLOGICAL REVIEWSPHARMACOL THERAPEUT PHARMACOLOGY & THERAPEUTICSPHYSIOL REV PHYSIOLOGICAL REVIEWSPHYSIOLOGY PHYSIOLOGYPLOS MED PLOS MEDICINEPROG NEUROBIOL PROGRESS IN NEUROBIOLOGYPROG RETIN EYE RES PROGRESS IN RETINAL AND EYE RESEARCHPSYCHOL BULL PSYCHOLOGICAL BULLETINPSYCHOL REV PSYCHOLOGICAL REVIEWREV MED VIROL REVIEWS IN MEDICAL VIROLOGYSCHIZOPHRENIA BULL SCHIZOPHRENIA BULLETINSEMIN CANCER BIOL SEMINARS IN CANCER BIOLOGYSEMIN IMMUNOL SEMINARS IN IMMUNOLOGYSTEM CELLS STEM CELLSSTROKE STROKETHORAX THORAXTRENDS COGN SCI TRENDS IN COGNITIVE SCIENCESTRENDS ENDOCRIN MET TRENDS IN ENDOCRINOLOGY AND METABOLISMTRENDS IMMUNOL TRENDS IN IMMUNOLOGYTRENDS MOL MED TRENDS IN MOLECULAR MEDICINETRENDS NEUROSCI TRENDS IN NEUROSCIENCESTRENDS PHARMACOL SCI TRENDS IN PHARMACOLOGICAL SCIENCESWHO TECH REP SER WHO TECHNICAL REPORT SERIESAM J CARDIOL AMERICAN JOURNAL OF CARDIOLOGYAM J EPIDEMIOL AMERICAN JOURNAL OF EPIDEMIOLOGYAM J PATHOL AMERICAN JOURNAL OF PATHOLOGYAM J PHYSIOL-HEART C AMERICAN JOURNAL OF PHYSIOLOGY-HEART AND CIRCULA ANTIMICROB AGENTS CH ANTIMICROBIAL AGENTS AND CHEMOTHERAPYBRIT J CANCER BRITISH JOURNAL OF CANCERCANCER-AM CANCER SOC CANCERCHEST CHESTCNS NEUROL DISORD-DR CNS & Neurological Disorders-Drug Targets ENDOCRINOLOGY ENDOCRINOLOGYEUR J NEUROSCI EUROPEAN JOURNAL OF NEUROSCIENCEFREE RADICAL BIO MED FREE RADICAL BIOLOGY AND MEDICINEINFECT IMMUN INFECTION AND IMMUNITYINT J CANCER INTERNATIONAL JOURNAL OF CANCERINT J RADIAT ONCOL INTERNATIONAL JOURNAL OF RADIATION ONCOLOGY BIOL INVEST OPHTH VIS SCI INVESTIGATIVE OPHTHALMOLOGY & VISUAL SCIENCEJ APPL PHYSIOL JOURNAL OF APPLIED PHYSIOLOGYJ CLIN ENDOCR METAB JOURNAL OF CLINICAL ENDOCRINOLOGY AND METABOLISM J CLIN MICROBIOL JOURNAL OF CLINICAL MICROBIOLOGYJ COMP NEUROL JOURNAL OF COMPARATIVE NEUROLOGYJ IMMUNOL JOURNAL OF IMMUNOLOGYJ INFECT DIS JOURNAL OF INFECTIOUS DISEASESJ MED CHEM JOURNAL OF MEDICINAL CHEMISTRYJ NEUROCHEM JOURNAL OF NEUROCHEMISTRYJ NEUROPHYSIOL JOURNAL OF NEUROPHYSIOLOGYJ NUTR JOURNAL OF NUTRITIONJ PHARMACOL EXP THER JOURNAL OF PHARMACOLOGY AND EXPERIMENTAL THERAPE J PHYSIOL-LONDON JOURNAL OF PHYSIOLOGY-LONDONJ UROLOGY JOURNAL OF UROLOGYJ VIROL JOURNAL OF VIROLOGYKIDNEY INT KIDNEY INTERNATIONALNEUROIMAGE NEUROIMAGENEUROSCIENCE NEUROSCIENCEPEDIATRICS PEDIATRICSRADIOLOGY RADIOLOGYTRANSPLANTATION TRANSPLANTATIONVIROLOGY VIROLOGYNATURE NATUREP NATL ACAD SCI USA PROCEEDINGS OF THE NATIONAL ACADEMY OF SCIENCES SCIENCE SCIENCEANN NY ACAD SCI ANNALS OF THE NEW YORK ACADEMY OF SCIENCESISSN大类名称复分大类分区是否top期刊2009年影响因子1680-7316地学1Y 4.881 0003-0007地学1Y 6.123 0930-7575地学1Y 3.917 0010-7999地学1Y 3.497 0012-821X地学1Y 4.062 0012-8252地学1Y 6.942 0016-7037地学1Y 4.385 0091-7613地学1Y 4.368 0266-1144地学1Y 4.039 0894-8755地学1Y 3.363 0022-3530地学1Y 3.738 0024-3590地学1Y 3.545 1752-0894地学1Y8.108 0883-8305地学1Y 3.644 0301-9268地学1Y 3.574 0277-3791地学1Y 4.245 8755-1209地学1Y8.021 0094-8276地学2Y 3.204 0022-4928地学2Y 2.911 0148-0227地学2Y 3.082 0022-1694地学2Y 2.433 0027-0644地学2Y 2.238 0066-4146地学天文1Y25.640 0935-4956地学天文1Y11.857 0067-0049地学天文1Y12.771 0004-637X地学天文2Y7.364 0360-0300工程技术1Y7.667 0730-0301工程技术1Y 3.619 1936-0851工程技术1Y7.493 1742-7061工程技术1Y 3.975 1359-6454工程技术1Y 3.760 0065-2156工程技术1Y 5.500 0724-6145工程技术1Y 4.165 1616-301X工程技术1Y 6.990 0935-9648工程技术1Y8.379 1523-9829工程技术1Y11.235 1531-7331工程技术1Y7.911 0570-4928工程技术1Y 3.243 0004-3702工程技术1Y 3.036 0142-9612工程技术1Y7.365 0960-8524工程技术1Y 4.253 0956-5663工程技术1Y 5.429 0734-9750工程技术1Y8.250 0006-3592工程技术1Y 3.377 1754-6834工程技术1Y 4.118 0008-6223工程技术1Y 4.5040897-4756工程技术1Y 5.368 0824-7935工程技术1Y 5.378 0738-8551工程技术1Y 3.567 1040-8398工程技术1Y 3.725 0958-1669工程技术1Y7.820 1388-2481工程技术1Y 4.243 0737-0024工程技术1Y 6.190 0733-8716工程技术1Y 3.758 1053-5888工程技术1Y 4.914 1089-778X工程技术1Y 4.589 0278-0046工程技术1Y 4.678 1045-9227工程技术1Y 2.889 0162-8828工程技术1Y 4.378 0098-5589工程技术1Y 3.750 0920-5691工程技术1Y 3.508 0360-3199工程技术1Y 3.945 1565-1339工程技术1Y 5.276 0749-6419工程技术1Y 4.791 0950-6608工程技术1Y 4.857 0021-9517工程技术1Y 5.288 0304-3894工程技术1Y 4.144 0959-9428工程技术1Y 4.795 1751-6161工程技术1Y 3.176 1741-2560工程技术1Y 3.739 0378-7753工程技术1Y 3.792 1570-8268工程技术1Y 3.412 1473-0197工程技术1Y 6.306 1022-1336工程技术1Y 4.263 0024-9297工程技术1Y 4.539 0927-796X工程技术1Y12.217 1369-7021工程技术1Y11.452 1361-8415工程技术1Y 3.093 1096-7176工程技术1Y 4.725 0276-7783工程技术1Y 4.485 1613-4125工程技术1Y 4.356 0883-7694工程技术1Y 6.330 1530-6984工程技术1Y9.991 1748-0132工程技术1Y13.237 1549-9634工程技术1Y 5.440 0957-4484工程技术1Y 3.137 1087-0156工程技术1Y29.495 1476-1122工程技术1Y29.504 1748-3387工程技术1Y26.309 1566-1199工程技术1Y 3.262 0018-9219工程技术1Y 4.878 1557-1955工程技术1Y 3.723 1558-3724工程技术1Y 5.6120960-8974工程技术1Y9.250 0360-1285工程技术1Y11.024 0079-6425工程技术1Y15.769 1062-7995工程技术1Y 4.702 0079-6727工程技术1Y 4.091 0079-6816工程技术1Y7.913 1364-0321工程技术1Y 4.842 1613-6810工程技术1Y 6.171 1744-683X工程技术1Y 4.869 0167-7799工程技术1Y 6.909 0924-2244工程技术1Y 4.051 1066-8888工程技术1Y 4.517 0001-1541工程技术2Y 1.955 0175-7598工程技术2Y 2.896 0009-2509工程技术2Y 2.136 0013-4686工程技术2Y 3.325 0308-8146工程技术2Y 3.146 0018-926X工程技术2Y 2.011 0018-9286工程技术2Y 2.556 0018-9448工程技术2Y 2.357 0018-9480工程技术2Y 2.076 1053-587X工程技术2Y 2.212 0888-5885工程技术2Y 1.758 0017-9310工程技术2Y 1.947 0002-7820工程技术2Y 1.944 0013-4651工程技术2Y 2.241 0376-7388工程技术2Y 3.203 0167-577X工程技术2Y 1.940 1359-6462工程技术2Y 2.949 0925-4005工程技术2Y 3.083 0257-8972工程技术2Y 1.793 0379-6779工程技术2Y 1.901 0040-6090工程技术2Y 1.727 0272-6963管理科学1Y 3.238 0025-1909管理科学1Y 2.227 0305-0483管理科学1Y 3.101 1059-1478管理科学1Y 2.080 0377-2217管理科学2Y 2.093 0001-4842化学1Y18.203 0108-7673化学化学-晶体1Y49.926 0002-5100化学1Y18.688 1433-7851化学1Y11.829 0066-426X化学1Y17.464 0161-4940化学1Y7.765 0009-2665化学1Y35.957 0306-0012化学1Y20.086 0010-8545化学1Y11.2251754-5692化学1Y8.500 0144-235X化学1Y 5.000 0002-7863化学1Y8.580 1389-5567化学1Y7.952 0265-0568化学1Y9.202 0079-6700化学1Y23.753 0167-5729化学1Y13.462 0165-9936化学1Y 6.546 0003-2700化学2Y 5.214 0022-3263化学2Y 4.219 1520-6106化学2Y 3.471 0743-7463化学2Y 3.898 0926-3373环境科学1Y 5.252 0003-0090环境科学1Y 4.133 1064-3389环境科学1Y7.091 1461-023X环境科学1Y10.318 0012-9615环境科学1Y 4.862 0012-9658环境科学1Y 4.411 0091-6765环境科学1Y 6.191 1462-2912环境科学1Y 4.909 0014-3820环境科学1Y 5.429 1540-9295环境科学1Y 6.922 1354-1013环境科学1Y 5.561 1466-822X环境科学1Y 5.913 1751-7362环境科学1Y 6.397 1352-2310环境科学2Y 3.139 0045-6535环境科学2Y 3.253 0013-936X环境科学2Y 4.630 0043-1354环境科学2Y 4.355 0065-2113农林科学1Y 3.800 0168-1923农林科学1Y 3.197 0261-1929农林科学1Y 1.580 1351-0754农林科学1Y 2.131 1467-2960农林科学1Y 4.489 1054-6006农林科学1Y 2.427 1050-4648农林科学1Y 2.892 1935-5130农林科学1Y 2.238 0016-7061农林科学1Y 2.461 1084-2020农林科学1Y 2.806 0021-8561农林科学1Y 2.469 0021-8812农林科学1Y 2.466 0022-0302农林科学1Y 2.463 1380-3743农林科学1Y 2.272 0960-3166农林科学1Y 2.161 0038-0717农林科学1Y 2.978 0361-5995农林科学1Y 2.179 0829-318X农林科学1Y 2.2920378-1135农林科学1Y 2.874 0928-4249农林科学1Y 3.579 0044-8486农林科学2Y 1.925 0706-652X农林科学2Y 1.951 0378-1127农林科学2Y 1.950 0003-1488农林科学2Y 1.714 0032-079X农林科学2Y 2.517 1526-5161社会科学1Y 4.000 0012-9682社会科学1Y 4.000 0304-4076社会科学2Y 1.902 0002-9297生物1Y12.303 0066-4154生物1Y29.875 1056-8700生物1Y18.955 1936-122X生物1Y19.304 1081-0706生物1Y19.571 1543-592X生物1Y8.190 0066-4170生物1Y11.271 0066-4197生物1Y13.235 1527-8204生物1Y11.568 0066-4227生物1Y12.804 0066-4286生物1Y11.212 1543-5008生物1Y23.460 0092-8674生物1Y31.152 1931-3128生物1Y13.021 1550-4131生物1Y17.350 1934-5909生物1Y23.563 1040-9238生物1Y10.216 0960-9822生物1Y10.992 0955-0674生物1Y14.153 0959-437X生物1Y8.987 1369-5266生物1Y10.333 0959-440X生物1Y9.344 1534-5807生物1Y13.363 0890-9369生物1Y12.075 1088-9051生物1Y11.342 0021-9525生物1Y9.575 1092-2172生物1Y12.585 1097-2765生物1Y14.608 1744-4292生物1Y12.125 1465-7392生物1Y19.527 1552-4450生物1Y16.058 1061-4036生物1Y34.284 1548-7091生物1Y16.874 1471-0056生物1Y27.822 1740-1526生物1Y17.644 1471-0072生物1Y42.198 1545-9985生物1Y12.2731040-4651生物1Y9.293 1544-9173生物1Y12.916 1553-7390生物1Y9.532 1553-7366生物1Y8.978 0163-7827生物1Y8.167 0033-5835生物1Y10.200 0968-0004生物1Y11.572 0962-8924生物1Y12.115 0169-5347生物1Y11.564 0168-9525生物1Y8.689 1360-1385生物1Y9.883 0099-2240生物2Y 3.686 0264-6021生物2Y 5.155 0006-3495生物2Y 4.390 0950-1991生物2Y7.194 0261-4189生物2Y8.993 0021-9193生物2Y 3.940 0021-9258生物2Y 5.328 0022-2836生物2Y 3.871 0270-7306生物2Y 6.057 0305-1048生物2Y7.479 0032-0889生物2Y 6.235 0001-5962数学1Y 2.619 0003-486X数学1Y 4.174 0090-5364数学1Y 3.185 0273-0979数学1Y 3.294 0010-3640数学1Y 2.657 1615-3375数学1Y 1.905 0020-9910数学1Y 2.794 0266-5611数学1Y 1.900 0894-0347数学1Y 3.411 0162-1459数学1Y 2.322 1369-7412数学1Y 3.473 0218-2025数学1Y 2.095 0025-5610数学1Y 2.048 0065-9266数学1Y 2.240 1540-3459数学1Y 2.198 0027-3171数学1Y 2.328 1468-1218数学1Y 2.381 0272-4332数学1Y 1.953 0036-1445数学1Y 3.391 0883-4237数学1Y 3.523 1070-5511数学1Y 3.153 0165-0114数学2Y 2.138 0377-0427数学2Y 1.292 0022-0396数学2Y 1.426 0022-247X数学2Y 1.2250362-546X数学2Y 1.487 0036-1429数学2Y 1.840 1064-8275数学2Y 1.595 0108-7673物理物理-晶体1Y49.926 0001-8732物理1Y19.632 0066-4189物理1Y9.353 0163-8998物理1Y11.964 1040-8436物理1Y 5.167 1126-6708物理1Y 6.019 1863-8880物理1Y 5.814 1433-8351物理1Y10.600 0277-7037物理1Y10.623 1749-4885物理1Y22.869 1745-2473物理1Y15.491 0370-1573物理1Y17.752 0031-9007物理1Y7.328 0079-6565物理1Y 6.742 0034-4885物理1Y11.444 0034-6861物理1Y33.145 0003-6951物理2Y 3.554 0021-9606物理2Y 3.093 1098-0121物理2Y 3.475 1550-7998物理2Y 4.922 0169-409X医学1Y11.957 0065-2776医学1Y7.725 0002-9165医学1Y 6.307 0002-9270医学1Y 6.012 0002-953X医学1Y12.522 1073-449X医学1Y10.689 1600-6135医学1Y 6.433 0003-4819医学1Y16.225 0364-5134医学1Y9.317 0003-4967医学1Y8.111 0003-4932医学1Y7.900 0732-0582医学1Y37.902 0066-4219医学1Y9.940 0147-006X医学1Y24.822 0199-9885医学1Y8.783 1553-4006医学1Y13.500 0362-1642医学1Y22.468 0066-4278医学1Y18.170 0066-4308医学1Y22.750 0163-7525医学1Y7.915 0003-990X医学1Y12.257 0003-9926医学1Y9.813 1079-5642医学1Y7.235 0004-3591医学1Y7.332。
通信信号调制方式识别方法综述

通信信号调制方式识别方法综述曾创展;贾鑫;朱卫纲【摘要】对通信信号调制方式的识别进行了深入研究,对通信信号常用的数字调制技术和调制识别预处理技术、理想高斯白噪声条件下基于决策论和基于统计模式的识别法、非理想信道条件下的调制识别法以及对共信道多信号调制方式的识别等进行了总结.在简要介绍各种方法的来源、理论基础和发展基础上讨论了各自的优缺点,并提出了调制识别研究领域的进一步发展方向.【期刊名称】《通信技术》【年(卷),期】2015(048)003【总页数】6页(P252-257)【关键词】通信信号调制识别;基于决策论;基于统计模式;非理想信道条件下;共信道多信号【作者】曾创展;贾鑫;朱卫纲【作者单位】装备学院研究生管理大队,北京101416;装备学院光电装备系,北京101416;装备学院光电装备系,北京101416【正文语种】中文【中图分类】TN76;TN911调制识别通常位于接收机的前端,在信号检测和信号解调之间,接收方要根据信号的调制方式进行解调才能继续进行下一步操作直至最终获取信号携带的信息。
而在诸如无线电检测、侦察、对抗等应用中,侦察方通常缺乏足够的先验知识,如信号的调制参数、方式等,而为了达到区分信号来源、性质、内容等目的,就需要侦察方对信号的调制方式进行正确识别分类。
当前,制电磁权已日益成为重要的作战要素,战场电磁环境中存在着大量未知信号,此时人工识别已无法满足信号识别的实时性要求,因而,人们开始研究自动调制识别方法,1969年,C.S.Weaver等人就发表了第一篇关于自动调制识别方法研究的论文[1],根据信号频谱的差异完成了自动识别。
随着通信信号从模拟调制发展为数字调制,调制方式更加复杂多样,调制识别算法的研究成果也越来越多,涉及方法体系也十分广泛。
本文从AWGN 条件下的调制识别、非理想信道条件下的调制识别以及共信道多信号的调制识别三方面概述了多种识别方法,在对各方法简要介绍的基础上对比讨论了各自的优缺点,展望了调制识别研究领域的进一步发展方向。
接缝滑移定负荷法 英语

接缝滑移定负荷法英语The Present Situation of Seam Slippage Load-Deflection MethodSeam slippage load-deflection method is a commonly employed technique in the field of textile engineering.This method is used to measure the strength and flexibility of the seam in fabrics.In recent years,researchers have been actively studying and improving this method,aiming to enhance its accuracy and efficiency.To begin with,the seam slippage load-deflection method is based on the principle that the seam experiences deformation under the application of an external load.By measuring the load and corresponding deflection of the seam, one can evaluate its mechanical properties,such as strength, elasticity,and slip resistance.This method is widely usedin the textile industry to assess the quality of sewn products and ensure their durability.Several advancements have been made in recent years to improve the accuracy of the seam slippage load-deflection method.One significant improvement is the development of high-precision testing equipment.These advanced devices can precisely measure the load and deflection in real-time, providing more reliable data for analysis.Moreover,the introduction of automated data analysis software has further enhanced the efficiency of this method.Researchers can now easily process the collected experimental data and obtain accurate and meaningful results.In addition to its accuracy and efficiency,the seam slippage load-deflection method also offers severaladvantages over other testing techniques.Firstly,it is a non-destructive testing method,meaning that the samplefabric can be reused for further analysis or evaluation.This significantly reduces material waste and testing costs. Secondly,this method can be applied to various types of fabrics,including wovens,knits,and nonwovens,making it versatile and widely applicable in the textile industry. Furthermore,the test results obtained from the seam slippage load-deflection method correlate well with the actual performance of sewn products in real-life scenarios. Therefore,this method can effectively predict the durability and reliability of fabrics,ensuring their suitability for specific applications.In conclusion,the seam slippage load-deflection method plays a crucial role in evaluating the mechanical properties of fabrics in the textile industry.With constant advancements in testing equipment and data analysis software, this method has become more accurate and efficient.Its non-destructive nature,versatility,and predictive ability make it a preferred choice for assessing the strength andflexibility of seams.As researchers continue to refine and optimize this method,it is expected to contribute significantly to the improvement of textile products andtheir overall quality.。
不同管电压下胸部数字X线摄影影像效果与其对患者影响的对比
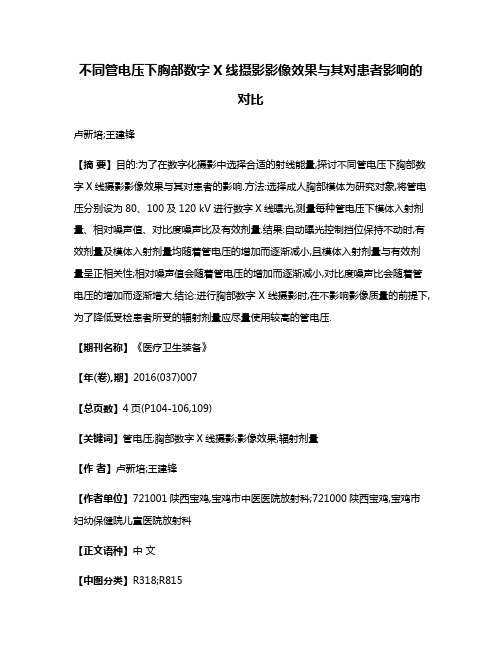
不同管电压下胸部数字X线摄影影像效果与其对患者影响的对比卢新培;王建锋【摘要】目的:为了在数字化摄影中选择合适的射线能量,探讨不同管电压下胸部数字X线摄影影像效果与其对患者的影响.方法:选择成人胸部模体为研究对象,将管电压分别设为80、100及120 kV进行数字X线曝光,测量每种管电压下模体入射剂量、相对噪声值、对比度噪声比及有效剂量.结果:自动曝光控制挡位保持不动时,有效剂量及模体入射剂量均随着管电压的增加而逐渐减小,且模体入射剂量与有效剂量呈正相关性;相对噪声值会随着管电压的增加而逐渐减小,对比度噪声比会随着管电压的增加而逐渐增大.结论:进行胸部数字X线摄影时,在不影响影像质量的前提下,为了降低受检患者所受的辐射剂量应尽量使用较高的管电压.【期刊名称】《医疗卫生装备》【年(卷),期】2016(037)007【总页数】4页(P104-106,109)【关键词】管电压;胸部数字X线摄影;影像效果;辐射剂量【作者】卢新培;王建锋【作者单位】721001陕西宝鸡,宝鸡市中医医院放射科;721000陕西宝鸡,宝鸡市妇幼保健院儿童医院放射科【正文语种】中文【中图分类】R318;R815胸部X线摄影在医学中是一种常见且具有挑战性的辅助性诊断工具,具有应用频率高、范围广等优点[1]。
20世纪70年代,计算机技术迅速发展起来,并在医学领域中有了一定的应用,医学影像学的数字时代在此时开辟[2]。
最初的数字医学影像学是利用一种成像板作为探测器的计算机X线摄影(computed radiography,CR),而数字化X线摄影(digital radiogr aphy,DR)是一种利用活跃的无定型硒或硅矩阵作为探测器直接将无形的射线能量转化为可视的数字信号[3]。
DR技术在影像的显示、传输与贮存等各个环节均有了很大的进步,这不仅使医学诊断的质量有了显著的提高,而且使采集、存储、显示、处理及传输等医学图像的处理方法有了根本的改变,还使远程放射学与远程医学通过网络得以实现[4]。
1、循证医学数据库DynaMed
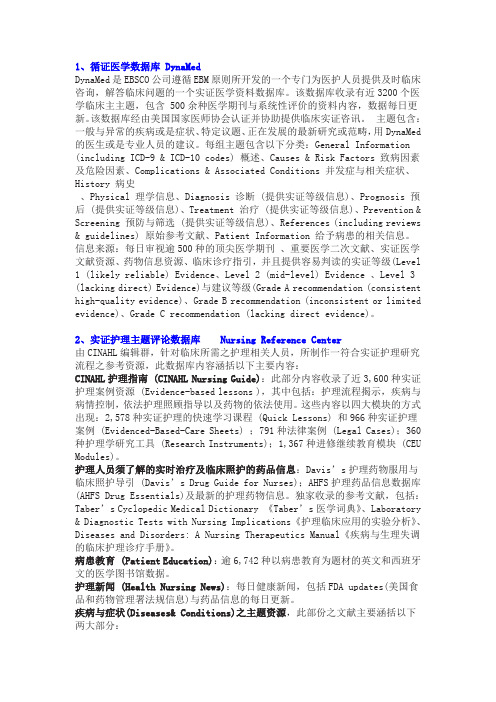
1、循证医学数据库 DynaMedDynaMed是EBSCO公司遵循EBM原则所开发的一个专门为医护人员提供及时临床咨询,解答临床问题的一个实证医学资料数据库。
该数据库收录有近3200个医学临床主主题,包含 500余种医学期刊与系统性评价的资料内容,数据每日更新。
该数据库经由美国国家医师协会认证并协助提供临床实证咨讯。
主题包含:一般与异常的疾病或是症状、特定议题、正在发展的最新研究或范畴,用DynaMed 的医生或是专业人员的建议。
每组主题包含以下分类:General Information (including ICD-9 & ICD-10 codes) 概述、Causes & Risk Factors 致病因素及危险因素、Complications & Associated Conditions 并发症与相关症状、History 病史、Physical 理学信息、Diagnosis 诊断 (提供实证等级信息)、Prognosis 预后 (提供实证等级信息)、Treatment 治疗 (提供实证等级信息)、Prevention & Screening 预防与筛选 (提供实证等级信息)、References (including reviews & guidelines) 原始参考文献、Patient Information 给予病患的相关信息。
信息来源:每日审视逾500种的顶尖医学期刊、重要医学二次文献、实证医学文献资源、药物信息资源、临床诊疗指引,并且提供容易判读的实证等级(Level 1 (likely reliable) Evidence、Level 2 (mid-level) Evidence 、Level 3 (lacking direct) Evidence)与建议等级(Grade A recommendation (consistent high-quality evidence)、Grade B recommendation (inconsistent or limited evidence)、Grade C recommendation (lacking direct evidence)。
Teece(蒂斯)——动态能力与战略管理中译版

动态能力与战略管理*大卫·蒂斯加里·皮萨诺Amy Shuen【作者简介】大卫·蒂斯(David J. Teece), 坎特伯雷大学文学学士,宾夕法尼亚大学文学硕士,宾夕法尼亚大学经济学博士。
现为加利福尼亚大学伯克利分校Haas商学院Thomas W. Tusher 全球商务讲座教授,管理、创新与组织研究所所长。
·蒂斯教授的主要研究领域包括:公司战略与公共政策,技术创新,知识管理与知识产权,规制与反托拉斯经济学以及电信、计算机与能源问题等。
·蒂斯教授累计出版和发表的论文与著作超过200篇(部),根据Science Watch杂志的统计,他位列1995到2005十年中经济学和管理学领域文章引用率最高的前十位学者。
他还是Industrial and Corporate Change杂志的编辑与创立者之一。
加里·皮萨诺(Gary Pisano),耶鲁大学文学学士,加利福尼亚大学伯克利分校博士。
现为哈佛大学商学院Harry E. Figgie, Jr.工商管理讲座教授,技术与运作管理系系主任。
皮萨诺教授的研究兴趣主要为生物技术与制药产业的技术战略,产品与过程开发管理,组织学习,垂直整合与外包战略等问题。
皮萨诺教授在国际一流期刊中发表了超过25篇学术论文,已出版的著作有:《研发工厂》,《运作、战略与技术》和《科技企业:生物技术的前景,现实与未来》。
Amy Shuen,耶鲁大学理学学士,哈佛大学MBA,加利福尼亚大学伯克利分校博士。
现为Silicon Valley Strategy Group总裁和CEO。
在此之前,她曾任教于宾夕法尼亚大学沃顿商学院和加利福尼亚大学伯克利分校Haas商学院。
【摘要】动态能力框架分析了在高速技术变迁环境中,企业获取财富和创造财富的根源与方法。
本文认为,这些企业的竞争优势由一些独特的过程(协调和组织方式)所确定。
这些独特的过程又是由企业特定的资产状况(如企业不可交易的知识资产与互补性资产的组合方式)和其选择或继承的演化路径所确定。
多参数监护仪临床警报管理实践指南(2020版)简版
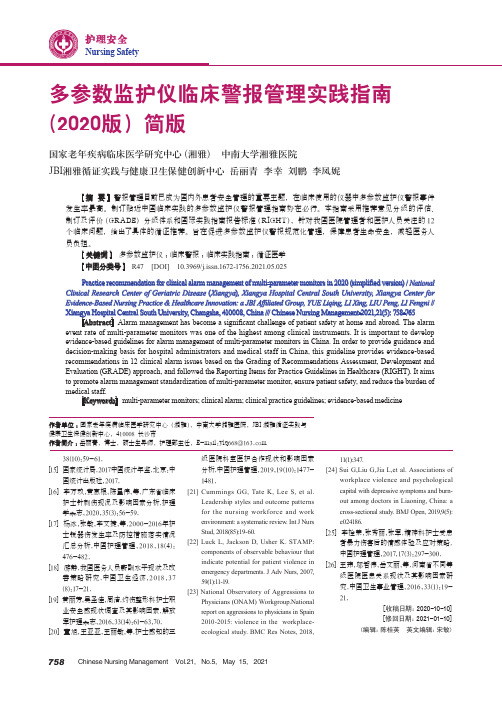
38(10):59-61.[15] 国家统计局.2017中国统计年鉴.北京:中国统计出版社,2017.[16] 李万玖,黄惠根,陈星伟,等.广东省临床护士针刺伤现况及影响因素分析.护理学杂志,2020,35(3):56-59.[17] 杨冰,张敏,李文捷,等.2000-2016年护士锐器伤发生率及防控措施落实情况汇总分析.中国护理管理,2018,18(4): 476-482.[18] 游静.我国医务人员薪酬水平现状及改善策略研究.中国卫生经济,2018,37 (8):17-21.[19] 黄丽芳,吴圣佳,周洁.灼伤整形科护士职业安全感现状调查及其影响因素.解放军护理杂志,2016,33(14):61-63,70.[20] 董旭,王亚亚,王丽敏,等.护士感知的三级医院科室医护合作现状和影响因素分析.中国护理管理,2019,19(10):1477- 1481.[21] Cummings GG, Tate K, Lee S, et al.Leadership styles and outcome patterns for the nursing workforce and work environment: a systematic review. Int J Nurs Stud, 2018(85):19-60.[22] Luck L, Jackson D, Usher K. STAMP:components of observable behaviour that indicate potential for patient violence in emergency departments. J Adv Nurs, 2007, 59(1):11-19.[23] National Observatory of Aggressions toPhysicians (ONAM) Workgroup.National report on aggressions to physicians in Spain 2010-2015: violence in the workplace-ecological study. BMC Res Notes, 2018,11(1):347.[24] Sui G,Liu G,Jia L,et al. Associations ofworkplace violence and psychological capital with depressive symptoms and burn-out among doctors in Liaoning, China: a cross-sectional study. BMJ Open, 2019,9(5): e024186.[25] 李拴荣,张秀丽,张军.精神科护士受患者暴力伤害后的情感体验及应对策略. 中国护理管理,2017,17(3):297-300.[26] 王玮,邬智伟,岳文丽,等.河南省不同等级医院医患关系现状及其影响因素研究.中国卫生事业管理,2016,33(1):19-21.[收稿日期:2020-10-10][修回日期:2021-01-10](编辑:陈桂英 英文编辑:宋敏)作者单位:国家老年疾病临床医学研究中心(湘雅),中南大学湘雅医院,JBI 湘雅循证实践与健康卫生保健创新中心,410008 长沙市作者简介:岳丽青,博士,硕士生导师,护理部主任,E-mail:**************[摘 要] 警报管理目前已成为国内外患者安全管理的重要主题,在临床使用的仪器中多参数监护仪警报事件发生率最高。
Evaluation of Objective Measures for Speech Enhancement

In our objective evaluations, we considered distortions introduced by speech enhancement algorithms and background noise. The list of speech enhancement algorithms considered in our study can be found in [4]. Noise was artificially added to the speech signal as follows. The Intermediate Reference System (IRS) filter used in ITU-T P.862 [5] for evaluation of the PESQ measures was independently applied to the clean and noise signals. The active speech level of the filtered clean speech signal was first determined using method B of ITU-T P.56 [6]. A noise segment of the same length as the speech signal was randomly cut out of the noise recordings taken from the AURORA database [7], appropriately scaled to reach the desired SNR level and finally added to the filtered clean speech signal. A total of 16 sentences corrupted in four background noise environments (car, street, babble and train) at two SNR levels (5dB and 10dB) were processed by the 13 speech enhancement algorithms. These sentences were produced by two male and two female speakers.
第一届世界青年地球科学家大会

Building
3. Contact Details
Xinhua Lei
Meeting Unit
Chuangheng Zhang
Guang Yu
Yong Liang Reception Unit
Dahu Meng
Info.Unit
Hui Yao
13701021778 13701326062 13501201226 13683344330 13321187200
Opening Ceremony: 9:00 25th Oct 2009 Spot of Opening Ceremony: Academic Exchange Center Of China University Of Geosciences (Beijing) Academic Report: 25th -28th Oct 2009 Spot of Theme Topic Report: Conference Hall Of Academic Exchange Center Of China University Of Geosciences (Beijing) Spot of Special Topic Report: Conference Rooms At Yifu Building Of China University Of Geosciences (Beijing) Closing Ceremony: 14:00 28th Oct 2009 Spot of Closing Ceremony: Academic Exchange Center Of China University Of Geosciences (Beijing) Dining Time and Place (Start From Evening 24th Oct) Breakfast: 06: 50 – 07:30 Place: Dining Hall Of Accommodated Hotel Lunch: 12:00 – 13:00 Place: The Forth Floor, Catering Center Of China University Of Geosciences (Beijing) Dinner: 18:00 – 19:00 Place: The Forth Floor, Catering Center Of China University Of Geosciences (Beijing)
From Data Mining to Knowledge Discovery in Databases
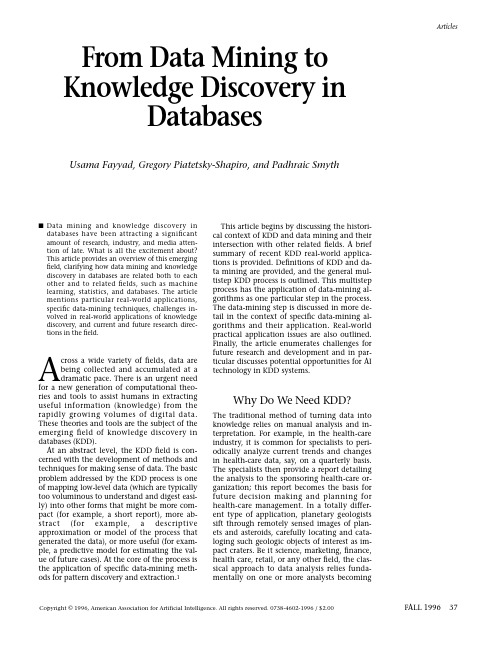
s Data mining and knowledge discovery in databases have been attracting a significant amount of research, industry, and media atten-tion of late. What is all the excitement about?This article provides an overview of this emerging field, clarifying how data mining and knowledge discovery in databases are related both to each other and to related fields, such as machine learning, statistics, and databases. The article mentions particular real-world applications, specific data-mining techniques, challenges in-volved in real-world applications of knowledge discovery, and current and future research direc-tions in the field.A cross a wide variety of fields, data arebeing collected and accumulated at adramatic pace. There is an urgent need for a new generation of computational theo-ries and tools to assist humans in extracting useful information (knowledge) from the rapidly growing volumes of digital data. These theories and tools are the subject of the emerging field of knowledge discovery in databases (KDD).At an abstract level, the KDD field is con-cerned with the development of methods and techniques for making sense of data. The basic problem addressed by the KDD process is one of mapping low-level data (which are typically too voluminous to understand and digest easi-ly) into other forms that might be more com-pact (for example, a short report), more ab-stract (for example, a descriptive approximation or model of the process that generated the data), or more useful (for exam-ple, a predictive model for estimating the val-ue of future cases). At the core of the process is the application of specific data-mining meth-ods for pattern discovery and extraction.1This article begins by discussing the histori-cal context of KDD and data mining and theirintersection with other related fields. A briefsummary of recent KDD real-world applica-tions is provided. Definitions of KDD and da-ta mining are provided, and the general mul-tistep KDD process is outlined. This multistepprocess has the application of data-mining al-gorithms as one particular step in the process.The data-mining step is discussed in more de-tail in the context of specific data-mining al-gorithms and their application. Real-worldpractical application issues are also outlined.Finally, the article enumerates challenges forfuture research and development and in par-ticular discusses potential opportunities for AItechnology in KDD systems.Why Do We Need KDD?The traditional method of turning data intoknowledge relies on manual analysis and in-terpretation. For example, in the health-careindustry, it is common for specialists to peri-odically analyze current trends and changesin health-care data, say, on a quarterly basis.The specialists then provide a report detailingthe analysis to the sponsoring health-care or-ganization; this report becomes the basis forfuture decision making and planning forhealth-care management. In a totally differ-ent type of application, planetary geologistssift through remotely sensed images of plan-ets and asteroids, carefully locating and cata-loging such geologic objects of interest as im-pact craters. Be it science, marketing, finance,health care, retail, or any other field, the clas-sical approach to data analysis relies funda-mentally on one or more analysts becomingArticlesFALL 1996 37From Data Mining to Knowledge Discovery inDatabasesUsama Fayyad, Gregory Piatetsky-Shapiro, and Padhraic Smyth Copyright © 1996, American Association for Artificial Intelligence. All rights reserved. 0738-4602-1996 / $2.00areas is astronomy. Here, a notable success was achieved by SKICAT ,a system used by as-tronomers to perform image analysis,classification, and cataloging of sky objects from sky-survey images (Fayyad, Djorgovski,and Weir 1996). In its first application, the system was used to process the 3 terabytes (1012bytes) of image data resulting from the Second Palomar Observatory Sky Survey,where it is estimated that on the order of 109sky objects are detectable. SKICAT can outper-form humans and traditional computational techniques in classifying faint sky objects. See Fayyad, Haussler, and Stolorz (1996) for a sur-vey of scientific applications.In business, main KDD application areas includes marketing, finance (especially in-vestment), fraud detection, manufacturing,telecommunications, and Internet agents.Marketing:In marketing, the primary ap-plication is database marketing systems,which analyze customer databases to identify different customer groups and forecast their behavior. Business Week (Berry 1994) estimat-ed that over half of all retailers are using or planning to use database marketing, and those who do use it have good results; for ex-ample, American Express reports a 10- to 15-percent increase in credit-card use. Another notable marketing application is market-bas-ket analysis (Agrawal et al. 1996) systems,which find patterns such as, “If customer bought X, he/she is also likely to buy Y and Z.” Such patterns are valuable to retailers.Investment: Numerous companies use da-ta mining for investment, but most do not describe their systems. One exception is LBS Capital Management. Its system uses expert systems, neural nets, and genetic algorithms to manage portfolios totaling $600 million;since its start in 1993, the system has outper-formed the broad stock market (Hall, Mani,and Barr 1996).Fraud detection: HNC Falcon and Nestor PRISM systems are used for monitoring credit-card fraud, watching over millions of ac-counts. The FAIS system (Senator et al. 1995),from the U.S. Treasury Financial Crimes En-forcement Network, is used to identify finan-cial transactions that might indicate money-laundering activity.Manufacturing: The CASSIOPEE trou-bleshooting system, developed as part of a joint venture between General Electric and SNECMA, was applied by three major Euro-pean airlines to diagnose and predict prob-lems for the Boeing 737. To derive families of faults, clustering methods are used. CASSIOPEE received the European first prize for innova-intimately familiar with the data and serving as an interface between the data and the users and products.For these (and many other) applications,this form of manual probing of a data set is slow, expensive, and highly subjective. In fact, as data volumes grow dramatically, this type of manual data analysis is becoming completely impractical in many domains.Databases are increasing in size in two ways:(1) the number N of records or objects in the database and (2) the number d of fields or at-tributes to an object. Databases containing on the order of N = 109objects are becoming in-creasingly common, for example, in the as-tronomical sciences. Similarly, the number of fields d can easily be on the order of 102or even 103, for example, in medical diagnostic applications. Who could be expected to di-gest millions of records, each having tens or hundreds of fields? We believe that this job is certainly not one for humans; hence, analysis work needs to be automated, at least partially.The need to scale up human analysis capa-bilities to handling the large number of bytes that we can collect is both economic and sci-entific. Businesses use data to gain competi-tive advantage, increase efficiency, and pro-vide more valuable services to customers.Data we capture about our environment are the basic evidence we use to build theories and models of the universe we live in. Be-cause computers have enabled humans to gather more data than we can digest, it is on-ly natural to turn to computational tech-niques to help us unearth meaningful pat-terns and structures from the massive volumes of data. Hence, KDD is an attempt to address a problem that the digital informa-tion era made a fact of life for all of us: data overload.Data Mining and Knowledge Discovery in the Real WorldA large degree of the current interest in KDD is the result of the media interest surrounding successful KDD applications, for example, the focus articles within the last two years in Business Week , Newsweek , Byte , PC Week , and other large-circulation periodicals. Unfortu-nately, it is not always easy to separate fact from media hype. Nonetheless, several well-documented examples of successful systems can rightly be referred to as KDD applications and have been deployed in operational use on large-scale real-world problems in science and in business.In science, one of the primary applicationThere is an urgent need for a new generation of computation-al theories and tools toassist humans in extractinguseful information (knowledge)from the rapidly growing volumes ofdigital data.Articles38AI MAGAZINEtive applications (Manago and Auriol 1996).Telecommunications: The telecommuni-cations alarm-sequence analyzer (TASA) wasbuilt in cooperation with a manufacturer oftelecommunications equipment and threetelephone networks (Mannila, Toivonen, andVerkamo 1995). The system uses a novelframework for locating frequently occurringalarm episodes from the alarm stream andpresenting them as rules. Large sets of discov-ered rules can be explored with flexible infor-mation-retrieval tools supporting interactivityand iteration. In this way, TASA offers pruning,grouping, and ordering tools to refine the re-sults of a basic brute-force search for rules.Data cleaning: The MERGE-PURGE systemwas applied to the identification of duplicatewelfare claims (Hernandez and Stolfo 1995).It was used successfully on data from the Wel-fare Department of the State of Washington.In other areas, a well-publicized system isIBM’s ADVANCED SCOUT,a specialized data-min-ing system that helps National Basketball As-sociation (NBA) coaches organize and inter-pret data from NBA games (U.S. News 1995). ADVANCED SCOUT was used by several of the NBA teams in 1996, including the Seattle Su-personics, which reached the NBA finals.Finally, a novel and increasingly importanttype of discovery is one based on the use of in-telligent agents to navigate through an infor-mation-rich environment. Although the ideaof active triggers has long been analyzed in thedatabase field, really successful applications ofthis idea appeared only with the advent of theInternet. These systems ask the user to specifya profile of interest and search for related in-formation among a wide variety of public-do-main and proprietary sources. For example, FIREFLY is a personal music-recommendation agent: It asks a user his/her opinion of several music pieces and then suggests other music that the user might like (<http:// www.ffl/>). CRAYON(/>) allows users to create their own free newspaper (supported by ads); NEWSHOUND(<http://www. /hound/>) from the San Jose Mercury News and FARCAST(</> automatically search information from a wide variety of sources, including newspapers and wire services, and e-mail rele-vant documents directly to the user.These are just a few of the numerous suchsystems that use KDD techniques to automat-ically produce useful information from largemasses of raw data. See Piatetsky-Shapiro etal. (1996) for an overview of issues in devel-oping industrial KDD applications.Data Mining and KDDHistorically, the notion of finding useful pat-terns in data has been given a variety ofnames, including data mining, knowledge ex-traction, information discovery, informationharvesting, data archaeology, and data patternprocessing. The term data mining has mostlybeen used by statisticians, data analysts, andthe management information systems (MIS)communities. It has also gained popularity inthe database field. The phrase knowledge dis-covery in databases was coined at the first KDDworkshop in 1989 (Piatetsky-Shapiro 1991) toemphasize that knowledge is the end productof a data-driven discovery. It has been popular-ized in the AI and machine-learning fields.In our view, KDD refers to the overall pro-cess of discovering useful knowledge from da-ta, and data mining refers to a particular stepin this process. Data mining is the applicationof specific algorithms for extracting patternsfrom data. The distinction between the KDDprocess and the data-mining step (within theprocess) is a central point of this article. Theadditional steps in the KDD process, such asdata preparation, data selection, data cleaning,incorporation of appropriate prior knowledge,and proper interpretation of the results ofmining, are essential to ensure that usefulknowledge is derived from the data. Blind ap-plication of data-mining methods (rightly crit-icized as data dredging in the statistical litera-ture) can be a dangerous activity, easilyleading to the discovery of meaningless andinvalid patterns.The Interdisciplinary Nature of KDDKDD has evolved, and continues to evolve,from the intersection of research fields such asmachine learning, pattern recognition,databases, statistics, AI, knowledge acquisitionfor expert systems, data visualization, andhigh-performance computing. The unifyinggoal is extracting high-level knowledge fromlow-level data in the context of large data sets.The data-mining component of KDD cur-rently relies heavily on known techniquesfrom machine learning, pattern recognition,and statistics to find patterns from data in thedata-mining step of the KDD process. A natu-ral question is, How is KDD different from pat-tern recognition or machine learning (and re-lated fields)? The answer is that these fieldsprovide some of the data-mining methodsthat are used in the data-mining step of theKDD process. KDD focuses on the overall pro-cess of knowledge discovery from data, includ-ing how the data are stored and accessed, howalgorithms can be scaled to massive data setsThe basicproblemaddressed bythe KDDprocess isone ofmappinglow-leveldata intoother formsthat might bemorecompact,moreabstract,or moreuseful.ArticlesFALL 1996 39A driving force behind KDD is the database field (the second D in KDD). Indeed, the problem of effective data manipulation when data cannot fit in the main memory is of fun-damental importance to KDD. Database tech-niques for gaining efficient data access,grouping and ordering operations when ac-cessing data, and optimizing queries consti-tute the basics for scaling algorithms to larger data sets. Most data-mining algorithms from statistics, pattern recognition, and machine learning assume data are in the main memo-ry and pay no attention to how the algorithm breaks down if only limited views of the data are possible.A related field evolving from databases is data warehousing,which refers to the popular business trend of collecting and cleaning transactional data to make them available for online analysis and decision support. Data warehousing helps set the stage for KDD in two important ways: (1) data cleaning and (2)data access.Data cleaning: As organizations are forced to think about a unified logical view of the wide variety of data and databases they pos-sess, they have to address the issues of map-ping data to a single naming convention,uniformly representing and handling missing data, and handling noise and errors when possible.Data access: Uniform and well-defined methods must be created for accessing the da-ta and providing access paths to data that were historically difficult to get to (for exam-ple, stored offline).Once organizations and individuals have solved the problem of how to store and ac-cess their data, the natural next step is the question, What else do we do with all the da-ta? This is where opportunities for KDD natu-rally arise.A popular approach for analysis of data warehouses is called online analytical processing (OLAP), named for a set of principles pro-posed by Codd (1993). OLAP tools focus on providing multidimensional data analysis,which is superior to SQL in computing sum-maries and breakdowns along many dimen-sions. OLAP tools are targeted toward simpli-fying and supporting interactive data analysis,but the goal of KDD tools is to automate as much of the process as possible. Thus, KDD is a step beyond what is currently supported by most standard database systems.Basic DefinitionsKDD is the nontrivial process of identifying valid, novel, potentially useful, and ultimate-and still run efficiently, how results can be in-terpreted and visualized, and how the overall man-machine interaction can usefully be modeled and supported. The KDD process can be viewed as a multidisciplinary activity that encompasses techniques beyond the scope of any one particular discipline such as machine learning. In this context, there are clear opportunities for other fields of AI (be-sides machine learning) to contribute to KDD. KDD places a special emphasis on find-ing understandable patterns that can be inter-preted as useful or interesting knowledge.Thus, for example, neural networks, although a powerful modeling tool, are relatively difficult to understand compared to decision trees. KDD also emphasizes scaling and ro-bustness properties of modeling algorithms for large noisy data sets.Related AI research fields include machine discovery, which targets the discovery of em-pirical laws from observation and experimen-tation (Shrager and Langley 1990) (see Kloes-gen and Zytkow [1996] for a glossary of terms common to KDD and machine discovery),and causal modeling for the inference of causal models from data (Spirtes, Glymour,and Scheines 1993). Statistics in particular has much in common with KDD (see Elder and Pregibon [1996] and Glymour et al.[1996] for a more detailed discussion of this synergy). Knowledge discovery from data is fundamentally a statistical endeavor. Statistics provides a language and framework for quan-tifying the uncertainty that results when one tries to infer general patterns from a particu-lar sample of an overall population. As men-tioned earlier, the term data mining has had negative connotations in statistics since the 1960s when computer-based data analysis techniques were first introduced. The concern arose because if one searches long enough in any data set (even randomly generated data),one can find patterns that appear to be statis-tically significant but, in fact, are not. Clearly,this issue is of fundamental importance to KDD. Substantial progress has been made in recent years in understanding such issues in statistics. Much of this work is of direct rele-vance to KDD. Thus, data mining is a legiti-mate activity as long as one understands how to do it correctly; data mining carried out poorly (without regard to the statistical as-pects of the problem) is to be avoided. KDD can also be viewed as encompassing a broader view of modeling than statistics. KDD aims to provide tools to automate (to the degree pos-sible) the entire process of data analysis and the statistician’s “art” of hypothesis selection.Data mining is a step in the KDD process that consists of ap-plying data analysis and discovery al-gorithms that produce a par-ticular enu-meration ofpatterns (or models)over the data.Articles40AI MAGAZINEly understandable patterns in data (Fayyad, Piatetsky-Shapiro, and Smyth 1996).Here, data are a set of facts (for example, cases in a database), and pattern is an expres-sion in some language describing a subset of the data or a model applicable to the subset. Hence, in our usage here, extracting a pattern also designates fitting a model to data; find-ing structure from data; or, in general, mak-ing any high-level description of a set of data. The term process implies that KDD comprises many steps, which involve data preparation, search for patterns, knowledge evaluation, and refinement, all repeated in multiple itera-tions. By nontrivial, we mean that some search or inference is involved; that is, it is not a straightforward computation of predefined quantities like computing the av-erage value of a set of numbers.The discovered patterns should be valid on new data with some degree of certainty. We also want patterns to be novel (at least to the system and preferably to the user) and poten-tially useful, that is, lead to some benefit to the user or task. Finally, the patterns should be understandable, if not immediately then after some postprocessing.The previous discussion implies that we can define quantitative measures for evaluating extracted patterns. In many cases, it is possi-ble to define measures of certainty (for exam-ple, estimated prediction accuracy on new data) or utility (for example, gain, perhaps indollars saved because of better predictions orspeedup in response time of a system). No-tions such as novelty and understandabilityare much more subjective. In certain contexts,understandability can be estimated by sim-plicity (for example, the number of bits to de-scribe a pattern). An important notion, calledinterestingness(for example, see Silberschatzand Tuzhilin [1995] and Piatetsky-Shapiro andMatheus [1994]), is usually taken as an overallmeasure of pattern value, combining validity,novelty, usefulness, and simplicity. Interest-ingness functions can be defined explicitly orcan be manifested implicitly through an or-dering placed by the KDD system on the dis-covered patterns or models.Given these notions, we can consider apattern to be knowledge if it exceeds some in-terestingness threshold, which is by nomeans an attempt to define knowledge in thephilosophical or even the popular view. As amatter of fact, knowledge in this definition ispurely user oriented and domain specific andis determined by whatever functions andthresholds the user chooses.Data mining is a step in the KDD processthat consists of applying data analysis anddiscovery algorithms that, under acceptablecomputational efficiency limitations, pro-duce a particular enumeration of patterns (ormodels) over the data. Note that the space ofArticlesFALL 1996 41Figure 1. An Overview of the Steps That Compose the KDD Process.methods, the effective number of variables under consideration can be reduced, or in-variant representations for the data can be found.Fifth is matching the goals of the KDD pro-cess (step 1) to a particular data-mining method. For example, summarization, clas-sification, regression, clustering, and so on,are described later as well as in Fayyad, Piatet-sky-Shapiro, and Smyth (1996).Sixth is exploratory analysis and model and hypothesis selection: choosing the data-mining algorithm(s) and selecting method(s)to be used for searching for data patterns.This process includes deciding which models and parameters might be appropriate (for ex-ample, models of categorical data are differ-ent than models of vectors over the reals) and matching a particular data-mining method with the overall criteria of the KDD process (for example, the end user might be more in-terested in understanding the model than its predictive capabilities).Seventh is data mining: searching for pat-terns of interest in a particular representa-tional form or a set of such representations,including classification rules or trees, regres-sion, and clustering. The user can significant-ly aid the data-mining method by correctly performing the preceding steps.Eighth is interpreting mined patterns, pos-sibly returning to any of steps 1 through 7 for further iteration. This step can also involve visualization of the extracted patterns and models or visualization of the data given the extracted models.Ninth is acting on the discovered knowl-edge: using the knowledge directly, incorpo-rating the knowledge into another system for further action, or simply documenting it and reporting it to interested parties. This process also includes checking for and resolving po-tential conflicts with previously believed (or extracted) knowledge.The KDD process can involve significant iteration and can contain loops between any two steps. The basic flow of steps (al-though not the potential multitude of itera-tions and loops) is illustrated in figure 1.Most previous work on KDD has focused on step 7, the data mining. However, the other steps are as important (and probably more so) for the successful application of KDD in practice. Having defined the basic notions and introduced the KDD process, we now focus on the data-mining component,which has, by far, received the most atten-tion in the literature.patterns is often infinite, and the enumera-tion of patterns involves some form of search in this space. Practical computational constraints place severe limits on the sub-space that can be explored by a data-mining algorithm.The KDD process involves using the database along with any required selection,preprocessing, subsampling, and transforma-tions of it; applying data-mining methods (algorithms) to enumerate patterns from it;and evaluating the products of data mining to identify the subset of the enumerated pat-terns deemed knowledge. The data-mining component of the KDD process is concerned with the algorithmic means by which pat-terns are extracted and enumerated from da-ta. The overall KDD process (figure 1) in-cludes the evaluation and possible interpretation of the mined patterns to de-termine which patterns can be considered new knowledge. The KDD process also in-cludes all the additional steps described in the next section.The notion of an overall user-driven pro-cess is not unique to KDD: analogous propos-als have been put forward both in statistics (Hand 1994) and in machine learning (Brod-ley and Smyth 1996).The KDD ProcessThe KDD process is interactive and iterative,involving numerous steps with many deci-sions made by the user. Brachman and Anand (1996) give a practical view of the KDD pro-cess, emphasizing the interactive nature of the process. Here, we broadly outline some of its basic steps:First is developing an understanding of the application domain and the relevant prior knowledge and identifying the goal of the KDD process from the customer’s viewpoint.Second is creating a target data set: select-ing a data set, or focusing on a subset of vari-ables or data samples, on which discovery is to be performed.Third is data cleaning and preprocessing.Basic operations include removing noise if appropriate, collecting the necessary informa-tion to model or account for noise, deciding on strategies for handling missing data fields,and accounting for time-sequence informa-tion and known changes.Fourth is data reduction and projection:finding useful features to represent the data depending on the goal of the task. With di-mensionality reduction or transformationArticles42AI MAGAZINEThe Data-Mining Stepof the KDD ProcessThe data-mining component of the KDD pro-cess often involves repeated iterative applica-tion of particular data-mining methods. This section presents an overview of the primary goals of data mining, a description of the methods used to address these goals, and a brief description of the data-mining algo-rithms that incorporate these methods.The knowledge discovery goals are defined by the intended use of the system. We can distinguish two types of goals: (1) verification and (2) discovery. With verification,the sys-tem is limited to verifying the user’s hypothe-sis. With discovery,the system autonomously finds new patterns. We further subdivide the discovery goal into prediction,where the sys-tem finds patterns for predicting the future behavior of some entities, and description, where the system finds patterns for presenta-tion to a user in a human-understandableform. In this article, we are primarily con-cerned with discovery-oriented data mining.Data mining involves fitting models to, or determining patterns from, observed data. The fitted models play the role of inferred knowledge: Whether the models reflect useful or interesting knowledge is part of the over-all, interactive KDD process where subjective human judgment is typically required. Two primary mathematical formalisms are used in model fitting: (1) statistical and (2) logical. The statistical approach allows for nondeter-ministic effects in the model, whereas a logi-cal model is purely deterministic. We focus primarily on the statistical approach to data mining, which tends to be the most widely used basis for practical data-mining applica-tions given the typical presence of uncertain-ty in real-world data-generating processes.Most data-mining methods are based on tried and tested techniques from machine learning, pattern recognition, and statistics: classification, clustering, regression, and so on. The array of different algorithms under each of these headings can often be bewilder-ing to both the novice and the experienced data analyst. It should be emphasized that of the many data-mining methods advertised in the literature, there are really only a few fun-damental techniques. The actual underlying model representation being used by a particu-lar method typically comes from a composi-tion of a small number of well-known op-tions: polynomials, splines, kernel and basis functions, threshold-Boolean functions, and so on. Thus, algorithms tend to differ primar-ily in the goodness-of-fit criterion used toevaluate model fit or in the search methodused to find a good fit.In our brief overview of data-mining meth-ods, we try in particular to convey the notionthat most (if not all) methods can be viewedas extensions or hybrids of a few basic tech-niques and principles. We first discuss the pri-mary methods of data mining and then showthat the data- mining methods can be viewedas consisting of three primary algorithmiccomponents: (1) model representation, (2)model evaluation, and (3) search. In the dis-cussion of KDD and data-mining methods,we use a simple example to make some of thenotions more concrete. Figure 2 shows a sim-ple two-dimensional artificial data set consist-ing of 23 cases. Each point on the graph rep-resents a person who has been given a loanby a particular bank at some time in the past.The horizontal axis represents the income ofthe person; the vertical axis represents the to-tal personal debt of the person (mortgage, carpayments, and so on). The data have beenclassified into two classes: (1) the x’s repre-sent persons who have defaulted on theirloans and (2) the o’s represent persons whoseloans are in good status with the bank. Thus,this simple artificial data set could represent ahistorical data set that can contain usefulknowledge from the point of view of thebank making the loans. Note that in actualKDD applications, there are typically manymore dimensions (as many as several hun-dreds) and many more data points (manythousands or even millions).ArticlesFALL 1996 43Figure 2. A Simple Data Set with Two Classes Used for Illustrative Purposes.。
Youngs Modulus Understanding Materials

Youngs Modulus Understanding Materials Young's modulus is a crucial concept in materials science and engineering, as it helps us understand how materials behave under stress and strain. It is a measure of a material's stiffness or elasticity, indicating how much it will deform under a given amount of force. This fundamental property is essential for designing and selecting materials for various applications, from building structures to manufacturing components for machinery.From a scientific perspective, Young's modulus is defined as the ratio of stress to strain within the elastic limit of a material. Stress refers to the force applied to a material per unit area, while strain is the resulting deformation or elongation. By measuring the stress and strain of a material, we can calculate its Young's modulus, which is expressed in pascals (Pa) or gigapascals (GPa). This allows us to compare the stiffness of different materials and predict how they will respond to external forces.In practical terms, understanding Young's modulus is crucial for engineers and designers when selecting materials for specific applications. For example, in civil engineering, the choice of materials for building structures depends on their ability to withstand the forces they will experience, such as compression, tension, or bending. By knowing the Young's modulus of different materials, engineers can select the most suitable ones to ensure the safety and longevity of the structures.Moreover, Young's modulus also plays a significant role in material testing and quality control. Manufacturers need to ensure that the materials they use meet the required standards and specifications for their intended use. By testing the Young's modulus of materials, they can verify their mechanical properties and identify any defects or inconsistencies that could affect their performance.On a more personal note, understanding Young's modulus can also foster a deeper appreciation for the materials that surround us in our daily lives. From the metal in our cars to the concrete in our buildings, every material has its own unique Young's modulus that determines its behavior under different conditions. This knowledge can lead to a greater understanding and respect for the engineering and science behind the materials we rely on.In conclusion, Young's modulus is a fundamental concept with far-reaching implications in materials science and engineering. Its significance spans from scientific principles to practical applications, influencing the design, selection, and testing of materials for various purposes. By grasping the concept of Young's modulus, we can gain a deeper understanding of the materials that shape the world around us and make informed decisions in their use and development.。
《信息检索》模拟考试试题
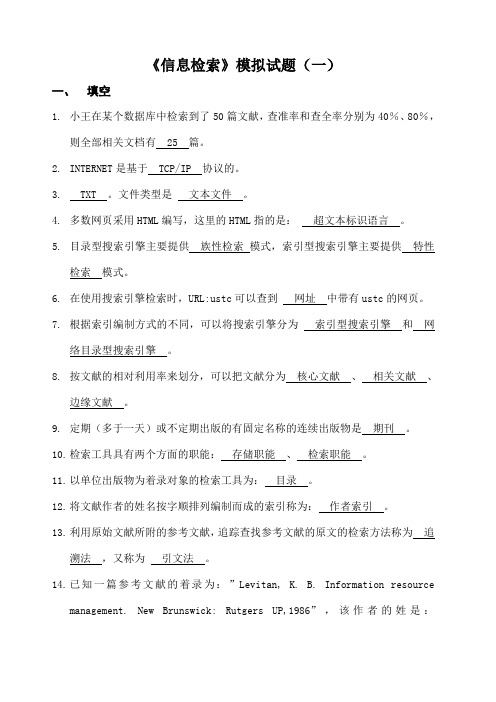
《信息检索》模拟试题(一)一、填空1. 小王在某个数据库中检索到了50篇文献,查准率和查全率分别为40%、80%,则全部相关文档有 25 篇。
2. INTERNET是基于 TCP/IP 协议的。
3. TXT 。
文件类型是文本文件。
4. 多数网页采用HTML编写,这里的HTML指的是:超文本标识语言。
5. 目录型搜索引擎主要提供族性检索模式,索引型搜索引擎主要提供特性检索模式。
6. 在使用搜索引擎检索时,URL:ustc可以查到网址中带有ustc的网页。
7. 根据索引编制方式的不同,可以将搜索引擎分为索引型搜索引擎和网络目录型搜索引擎。
8. 按文献的相对利用率来划分,可以把文献分为核心文献、相关文献、边缘文献。
9. 定期(多于一天)或不定期出版的有固定名称的连续出版物是期刊。
10. 检索工具具有两个方面的职能:存储职能、检索职能。
11. 以单位出版物为着录对象的检索工具为:目录。
12. 将文献作者的姓名按字顺排列编制而成的索引称为:作者索引。
13. 利用原始文献所附的参考文献,追踪查找参考文献的原文的检索方法称为追溯法,又称为引文法。
14. 已知一篇参考文献的着录为:”Levitan, K. B. Information resourcemanagement. New Brunswick: Rutgers UP,1986”,该作者的姓是:Levitan 。
15. 检索语言可分为两大类:分类语言、主题词语言。
16. LCC指的是美国国会图书馆分类法。
17. 当检索关键词具有多个同义词和近义词时,容易造成漏检,使得查全率较低。
18. 主题词的规范化指的是词和概念一一对应,一个词表达一个概念。
19. 国际上通常根据内容将数据库划分为:参考数据库、源数据库、混合数据库。
20. 查询关键词为短语"DATA OUTPUT",可以用位置算符(W)改写为: DATA (W)OUTPUT 。
21. 着录参考文献时,对于三个以上的着者,可以在第一着者后面加上 et al. ,代表"等人"的意思。
物理评论d级别
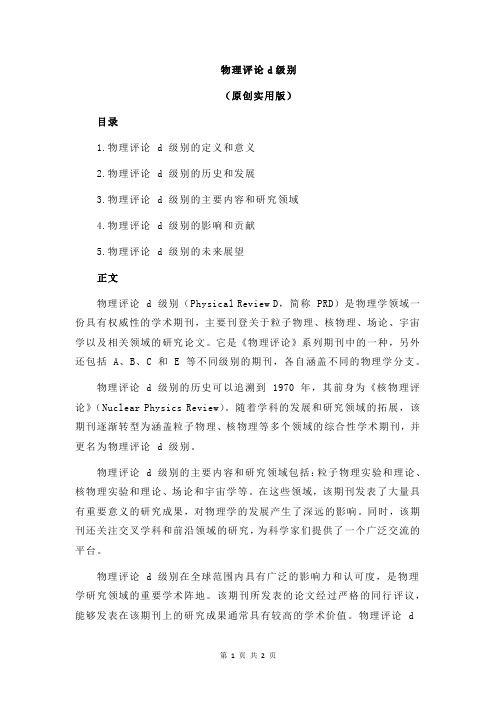
物理评论d级别(原创实用版)目录1.物理评论 d 级别的定义和意义2.物理评论 d 级别的历史和发展3.物理评论 d 级别的主要内容和研究领域4.物理评论 d 级别的影响和贡献5.物理评论 d 级别的未来展望正文物理评论 d 级别(Physical Review D,简称 PRD)是物理学领域一份具有权威性的学术期刊,主要刊登关于粒子物理、核物理、场论、宇宙学以及相关领域的研究论文。
它是《物理评论》系列期刊中的一种,另外还包括 A、B、C 和 E 等不同级别的期刊,各自涵盖不同的物理学分支。
物理评论 d 级别的历史可以追溯到 1970 年,其前身为《核物理评论》(Nuclear Physics Review)。
随着学科的发展和研究领域的拓展,该期刊逐渐转型为涵盖粒子物理、核物理等多个领域的综合性学术期刊,并更名为物理评论 d 级别。
物理评论 d 级别的主要内容和研究领域包括:粒子物理实验和理论、核物理实验和理论、场论和宇宙学等。
在这些领域,该期刊发表了大量具有重要意义的研究成果,对物理学的发展产生了深远的影响。
同时,该期刊还关注交叉学科和前沿领域的研究,为科学家们提供了一个广泛交流的平台。
物理评论 d 级别在全球范围内具有广泛的影响力和认可度,是物理学研究领域的重要学术阵地。
该期刊所发表的论文经过严格的同行评议,能够发表在该期刊上的研究成果通常具有较高的学术价值。
物理评论 d级别为物理学领域的学者提供了宝贵的学术资源,对推动学科的发展和创新做出了巨大贡献。
面对未来的挑战和机遇,物理评论 d 级别将继续秉承科学、严谨、创新的办刊理念,致力于为物理学研究提供更好的学术交流平台。
物质颗粒大小在水中的溶解快慢实验步骤 -回复

物质颗粒大小在水中的溶解快慢实验步骤-回复问题:物质颗粒大小在水中的溶解快慢实验步骤引言:物质的溶解是化学实验中一个重要的研究内容。
不同物质的颗粒大小对其在水中的溶解速度会有显著的影响。
本文将介绍一种简单实用的实验步骤,以探究物质颗粒大小对其在水中溶解速度的影响。
一、准备材料和设备1. 目标物质:选择两种具有不同颗粒大小的物质进行比较实验,如砂糖和食盐。
2. 水:实验中所需的水应保持纯净,可使用蒸馏水或去离子水。
3. 量筒:用于准确测量水的体积。
4. 温度计:用于记录实验时水的温度。
5. 手提搅拌器:用于加速物质的溶解过程。
6. 计时器:用于测量物质在水中的溶解时间。
7. 磁力搅拌器(可选):用于提供均匀的搅拌。
二、实验步骤1. 准备两个温度相等的容器,将等量的水(例如100毫升)分别倒入两个容器中。
2. 将目标物质分别称取等量(例如5克)加入两个容器中,并记下溶质的初始质量。
3. 用手提搅拌器分别在两个容器中搅拌,直到目标物质都完全溶解。
4. 记录下两个容器中的物质溶解所需的时间,并进行比较。
5. 可选操作:使用磁力搅拌器代替手提搅拌器进行实验,以获得均匀的搅拌速度。
三、数据处理和分析1. 比较两种物质在水中的溶解时间,进而比较它们的溶解速度。
2. 分析溶质的颗粒大小对其溶解的影响。
根据实验结果,讨论颗粒大小与溶解速度之间的关系,并提出合理的解释。
3. 讨论实验误差可能的来源,如温度、搅拌速度等。
并提出改进实验的建议,以减小误差。
结论:1. 通过本实验可以发现,物质的颗粒大小对其在水中的溶解速度有明显的影响。
2. 颗粒较小的物质通常溶解速度更快,这是因为颗粒大小较小的物质具有更大的比表面积,能够更快与溶剂接触。
3. 实验结果可以应用于生活和工业实践中,帮助我们更好地理解和掌握物质的溶解特性,以及合理利用这些特性。
延伸思考:1. 实验过程中,还可以考虑加入温度变量,以研究温度对物质溶解速度的影响。
dynabeads_m450tosylactivated

dynabeads_m450tosylactivatedProduct ContentsDynabeads ? M-450 Tosylactivated contains 4 × 108 beads/mL in distilled water.Product DescriptionDynabeads ? M-450 Tosylactivatedcoupled with antibodies or other ligands provide a versatile tool for isolation of both cells and non-cell targets(e.g. proteins and other biomolecules). Their size makes them particularly suitable for stimulation and expansion of e.g. T cells. Cells can be directlyisolated from any sample such as whole blood, bone marrow, mononuclear cell suspensions (MNC) or tissue digests Dynabeads ? M-450 Tosylactivated react covalently with proteins (e.g. antibodies) or other ligands containing primary amino or sulphydryl groups. Dynabeads ? M-450 Tosylactivated bind proteins physically and chemically with an increasing number of covalent bonds with higher temperature and pH.Once coupled with the specific ligand, the beads are mixed with the sample in a tube. The beads bind to the target during a short incubation, and then the bead-bound target is separated by a magnet. Positive isolation – Discard thesupernatant and use the bead-bound target for downstream applications.Depletion – Discard the bead-bound target and use the remaining, untouched sample for downstream applications Note: For protein purification and immunoassays we recommend using Dynabeads ? M-280 Tosylactivated or Dynabeads ? MyOne ? Tosylactivated.Dynabeads ? M-450 TosylactivatedFor research use only. Not for human or animal therapeutic or diagnostic use.Catalog no. 14013 Store at 2?C to 8?CRev. Date: June 2012 (Rev. 003)Required MaterialsMagnet (DynaMag portfolio). See /doc/59918ddadd3383c4ba4cd26d.html /magnets for recommendations.Mixer allowing tilting and rotation of tubes (e.g. HulaMixer Sample Mixer). Buffer 1: 0.1 M sodium phosphate buffer, pH 7.4–8.0 or 0.1 M sodium borate buffer, pH 7.6–9.5.Buffer 2: Ca 2+ and Mg 2+ free phosphate buffered saline (PBS) supplemented with 0.1% bovine serum albumin (BSA) and 2 mM EDTA, pH 7.4.Note: BSA can be replaced by human serum albumin (HSA) or fetal calfserum (FCS). EDTA can be replaced by sodium citrate.Buffer 3: 0.2 M Tris w/0.1% BSA, pH 8.5.Specific ligands.Optional: BSA for blocking and sodium azide as a preservative.Caution: Sodium azide may react with lead and copper plumbing to form highly explosive metal azides.General GuidelinesVisit /doc/59918ddadd3383c4ba4cd26d.html /samplepreparation for recommended sample preparation procedures.Use a mixer that provides tilting and rotation of the tubes to ensure that the beads do not settle in the tube.BuffersSugars and stabilizers may interfere with binding and should be removed from the ligand preparation.Coupling buffers should not contain any reactive groups (amines, thiols and hydroxyls) e.g. tris, glycine, or proteins.The pH and the ionic strength of the coupling buffer can be varied, but is generally carried out in 0.1 M phosphate buffer at pH 7.4–8.0. Tosyl groups are more reactive at higher pH, therefore 0.1 M borate buffer pH 7.6–9.5 can be used depending on the ligand stability. Buffers with higher ionic strength improve the coupling efficiency.CouplingThe physical adsorption of the ligand to the bead surface is rapid, while the formation of covalent bonds will need more time. Maximal chemical binding isachieved after 16–24 hours at 37?C. Coupling at 18?C to 25?C (room temperature) will require incubation time >48 hours to obtain the same degree of chemical binding. If a lower coupling temperature is required due to ligand stability, use Dynabeads ? M-450 Epoxy.BlockingAdding a blocking protein such as 0.01–0.5% with BSA to the coupling solution may increase functionality of the coupled antibodies in cell isolation protocols. Blocking protein is generally added to coupling solution after 0–30 min. Both the incubation time before blocking and blocking protein concentration should be optimized.Cell IsolationThe efficiency of antibody-antigen binding can be increased for some applications by using a spacer molecule e.g. a secondary antibody coupled to the beads prior to coupling of the primary antibody.The optimal bead concentration during coupling is 4–8 × 108 beads per mL. The choice of primary antibody is the most important factor for successful cell isolation. Note that some antibodies may show reduced antigen-binding efficiency when coated onto beads, even though the antibody shows good results in other immunological assays.To avoid non-specific binding of cells (e.g. monocytes, B cells), add aggregated IgG to the cell sample to block Fc-receptors prior to adding the primary antibodies.When incubating beads and cells, the incubation temperature must be 2°C to 8°C to reduce phagocytic activity and other metabolic processes.Never use less than 25 µL (1 × 107) beads/mL cell sample and at least 4 beads per target cell.If the concentration of cells is increased or the target cell concentration exceeds 2.5 × 106 cells/mL, the bead volume must be increased accordingly. Cell concentration can be up to 1 × 108 cells/mL.Remove density gradient media (e.g. Ficoll): Wash cells prior to adding antibodies or beads.Serum may contain soluble factors (e.g. antibodies or cell surface antigens), which can interfere with the cell isolation protocol. Washing the cells once may reduce this interference.Storage of Coupled BeadsCoupled beads may typically be stored in Buffer 2 at 2°C to 8°C for months or even years. Stability of coupled beads depends on ligand stability and must be determined separately.A final concentration of 0.02% sodium azide can be added as a preservative to the storage buffer. The preservative must be removed by washing prior to cell isolation.Release of CellsSome cells exhibit high turnover of expressed antigens and will be released from the beads during 6–24 hours of incubation under standard culture conditions (37°C).ProtocolsWash the Dynabeads ?See Table 1 for volume recommendation.1. Resuspend the beads in the vial (i.e. vortex for >30 sec, or tilt and rotate for 5 min).2. Transfer the desired volume of beads to a tube.3. Add the same volume of Buffer 1, or at least 1 mL, and resuspend.4. Place the tube in a magnet for 1 min and discard the supernatant.5. Remove the tube from the magnet and resuspend the washed beads in the same volume of Buffer 1 as the initial volume of beads (step 2).Prepare SampleCells can be directly isolated from any samples such as whole blood, bonemarrow, MNC suspensions or tissue digests. See “General guidelines” for sample preparation.MNC should be re-suspended at 1 × 107 cells/mL in Buffer 2.MNC: ~2 × 109 cellsWhole blood/buffy coat: ~200 mLFor support visit /doc/59918ddadd3383c4ba4cd26d.html /support or emailtechsupport@/doc/59918ddadd3383c4ba4cd26d.html/doc/59918ddadd3383c4ba4cd26d.html2012 Life Technologies Corporation. All rights reserved. The trademarks mentioned herein are the property of Life Technologies Corporation and/or its affiliate(s) or their respective owners.Life TeChnoLogies CoRPoRATion And/oR iTs AffiLiATe(s) disCLAim ALL wARRAnTies wiTh ResPeCT To This doCumenT, exPRessed oR imPLied, inCLuding buT noT LimiTed To Those of meRChAnTAbiLiTy oR fiTness foR A PARTiCuLAR PuRPose oR non-infRingemenT. To The exTenT ALLowed by LAw, in no evenT shALL Life TeChnoLogies And/oR iTs AffiLiATe(s) be LiAbLe, wheTheR in ConTRACT, ToRT, wARRAnTy, oR undeR Any sTATuTe oR on Any oTheR bAsis foR sPeCiAL, inCidenTAL, indiReCT, PuniTive, muLTiPLe oR ConsequenTiAL dAmAges in ConneCTion wiTh oR ARising fRom This doCumenT, inCLuding buT noT LimiTed To The use TheReof .SPEC-06055on labels is the symbol for catalog number.REF Table 1: Coupling of ligand to Dynabeads ? M-450 Epoxy.Description of MaterialsDynabeads ? M-450 Epoxy are uniform, superparamagnetic polystyrene beads (4.5 µm diameter) covered with surface epoxy groups suitable for physical and chemical binding of antibodies and other bio-molecules.* Adjust the Buffer volumes to fit to the tube you are using.Limited Use Label LicenseThe purchase of this product conveys to the purchaser the limited, nontransferable right to use the purchased amount of the product only to perform internal research for the sole benefit of the purchaser. No right to resell this product or any of its components is conveyed expressly, by implication, or by estoppel. This product is for internal research purposes only and is not for use in commercial applications of any kind, including, without limitation, quality control and commercial services such as reporting the results of purchaser’s activities for a fee or other form of consideration. For information on obtaining additional rights, please contact outlicensing@/doc/59918ddadd3383c4ba4cd26d.html or Out Licensing, Life Technologies, 5791 Van Allen Way, Carlsbad, California 92008.Manufactured by Life Technologies AS, Norway. Life Technologies AS complies with the Quality System Standards ISO 9001:2008 and ISO 13485:2003.Limited Product WarrantyLife Technologies Corporation and/or its affiliate(s) warrant their products as set forth in the Life Technologies' GeneralTerms and Conditions of Sale found on Life Technologies' website at/doc/59918ddadd3383c4ba4cd26d.html /termsandconditions . If you have any questions, please contact Life Technologies at /doc/59918ddadd3383c4ba4cd26d.html /support .Couple Ligand to the Dynabeads ?This is a general procedure for antibody coupling to 1 mL (4 × 108) Dynabeads ? M-450 Tosylactivated. It is not recommended to couple lower volumes than 200 µL beads. When coupling larger volumes, scale up all volumes accordingly, as shown in Table 1.Use approximately 200 µg antibody (Ab)/1 mL (4 × 108) beads. Calculate the Ab volume from the Ab concentration(µg/mL). 1. Transfer 1 mL of washed and resuspended beads to a tube.2. Place the tube in a magnet for 1 min and discard the supernatant. Remove the tube from the magnet.3. Resuspend the beads in Buffer 1 (1 mL minus antibody volume) and add 200 µg antibodies during mixing to reach a total coupling volume of 1 mL.4. O ptional : Incubate for 15 min and then add BSA to 0.01–0.1% w/v.5. Incubate for 16–24 hours at room temperature with gentle tilting and rotation.6. Place the tube in a magnet for 1 min and discard the supernatant.7. Add 1 mL Buffer 2, mix and incubate for 5 min at 2°C to 8°C with gentle tilting and rotation. '8. Apply to magnet for 1 min, remove the supernatant, remove from the magnet and repeat steps 7+8 once.9. O ptional: Incubate for 24 hours at room temperature or 4 hours at 37°C in 1 mL Buffer 3 to de-activate remaining free tosyl groups. Apply to magnet for 1 min and remove the supernata10. Add 1 mL Buffer 2, mix and incubate for 5 min at 2°C to 8°C with gentle tilting and rotation.11. Remove the beads from the magnet and resuspend the coated beads in 1 mL Buffer 2 (to obtain 4 × 108 beads/mL). Isolate CellsUse "Wash the Dynabeads ?" procedure, replacing Buffer 1 with Buffer 2 to remove any soluble ligand prior to cell isolation. This protocol is based on 1 × 107 MNC or 1 mL whole blood, but is directly scalable from 1 × 107 to 4 × 108 cells or 1–40 mL whole blood. When working with volumes lower than 1 × 107 cells or 1 mL blood, use the same volumes as for 1 × 107 cells or 1 mL blood. When working with larger volumes, scale up all volumes accordingly, as shown in Table 2.1. Add 25 µL pre-coupled, washed and resuspended beads to 1 mL prepared sample (1 × 107 MNC).2. Incubate for 20 min (positive isolation) or 30 min (depletion) at 2°C to 8°C with gentle tilting and rotation.3. O ptional: Add 1 mL Buffer 2 to limit trapping of unbound cells.4. Place the tube in a magnet for 2 min.5. F or depletion: Transfer the supernatant containing the unbound cells to a fresh tube for further experiments. orTable 2: Volumes for cell isolation.increase/ optimize the amount of beads. ** Adjust the Buffer 2 volumes to fit to the tube you are using.For positive isolation: Discard the supernatant and gently wash the bead-bound cells 4 times by:a. Add 1 mL Buffer 2.b. Place the tube in the magnet for 1 min and discard the supernatant. Remove the tube from the magnet.c. Repeat steps 6a-6b three times.6. Resuspend the cells in buffer/medium for downstream application.。
物理评论d级别
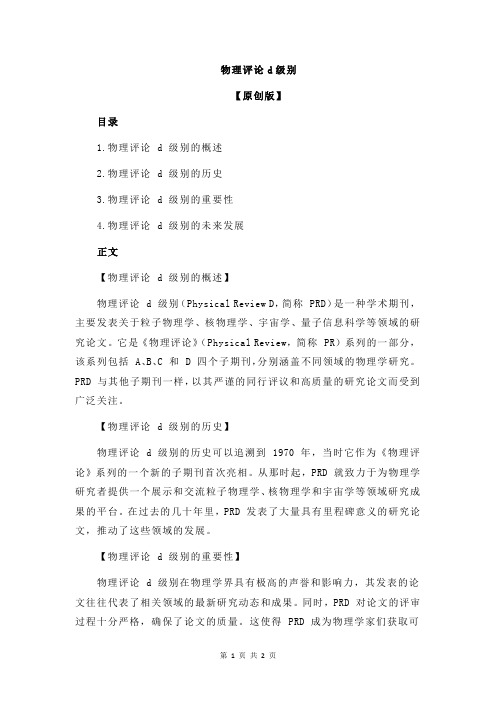
物理评论d级别【原创版】目录1.物理评论 d 级别的概述2.物理评论 d 级别的历史3.物理评论 d 级别的重要性4.物理评论 d 级别的未来发展正文【物理评论 d 级别的概述】物理评论 d 级别(Physical Review D,简称 PRD)是一种学术期刊,主要发表关于粒子物理学、核物理学、宇宙学、量子信息科学等领域的研究论文。
它是《物理评论》(Physical Review,简称 PR)系列的一部分,该系列包括 A、B、C 和 D 四个子期刊,分别涵盖不同领域的物理学研究。
PRD 与其他子期刊一样,以其严谨的同行评议和高质量的研究论文而受到广泛关注。
【物理评论 d 级别的历史】物理评论 d 级别的历史可以追溯到 1970 年,当时它作为《物理评论》系列的一个新的子期刊首次亮相。
从那时起,PRD 就致力于为物理学研究者提供一个展示和交流粒子物理学、核物理学和宇宙学等领域研究成果的平台。
在过去的几十年里,PRD 发表了大量具有里程碑意义的研究论文,推动了这些领域的发展。
【物理评论 d 级别的重要性】物理评论 d 级别在物理学界具有极高的声誉和影响力,其发表的论文往往代表了相关领域的最新研究动态和成果。
同时,PRD 对论文的评审过程十分严格,确保了论文的质量。
这使得 PRD 成为物理学家们获取可靠研究信息的重要来源。
此外,PRD 也为我国物理学家提供了一个向世界展示研究成果的窗口,促进了国内外学术交流与合作。
【物理评论 d 级别的未来发展】面对未来,物理评论 d 级别将继续秉持严谨的学术态度,关注粒子物理学、核物理学、宇宙学等领域的最新研究进展。
随着科学技术的不断发展,PRD 有望在量子信息科学等新兴领域发挥更大的作用。
张立群教授获得美国化学学会Sparks-Thomas奖

张立群教授获得美国化学学会Sparks-Thomas奖
赵秀英
【期刊名称】《橡胶工业》
【年(卷),期】2011(58)12
【摘要】2011年10月11—14日,美国化学学会橡胶专业委员会(ACS Rubber Division)在俄亥俄州克利夫兰市召开了第180届学术与技术会议,会上宣布张立群教授获得Sparks—Thomas奖。
这一结果登载在该会议的Show Daily上。
【总页数】1页(P720-720)
【关键词】美国化学学会;专业委员会;Daily;技术会议;俄亥俄州;Show;橡胶
【作者】赵秀英
【作者单位】北京化工大学先进弹性体材料研究中心
【正文语种】中文
【中图分类】TQ33
【相关文献】
1.“从事科学研究是很酷的事”对话国际电化学学会田岛奖获得者美国加州大学圣克鲁兹分校陈少伟教授 [J], 陈健
2.NaOH/尿素水溶剂体系创建者中国第一位“美国化学会安塞姆·佩恩奖”获得者武汉大学教授、博士生导师中国科学院院士——张俐娜 [J], ;
3.北京化工大学张立群教授获得美国化学学会Sparks-Thomas奖 [J],
4.北京化工大学张立群教授获得日本化学工学会亚洲研究奖 [J], 赵秀英
因版权原因,仅展示原文概要,查看原文内容请购买。
- 1、下载文档前请自行甄别文档内容的完整性,平台不提供额外的编辑、内容补充、找答案等附加服务。
- 2、"仅部分预览"的文档,不可在线预览部分如存在完整性等问题,可反馈申请退款(可完整预览的文档不适用该条件!)。
- 3、如文档侵犯您的权益,请联系客服反馈,我们会尽快为您处理(人工客服工作时间:9:00-18:30)。
3.1 Find Buddy Algorithms
Buddies are the users that have similar rating activity, as far as a common set of items is concerned. We employ two algorithms of calculating buddies, respectively based
2 Department of Computer Science, University of Crete P.O. Box 2208, GR-71409, Heraklion, Greece
1 Introduction
Recommendation systems have been a popular topic of research ever since the ubiquity of the web made it clear that people of hugely varying backgrounds would be able to access and query the same underlying data. Content-based [1] and collaborative filtering [1], [2] algorithms are usually used to form recommendations, while additional hybrid techniques have been proposed as well. We introduce an alternative way of building user profiles based on both explicit and implicit ratings, as well as, profile-based recommendation algorithms. We advocate the use of such algorithms in order to automate the discovery of dynamic, virtual communities. As an application of the proposed techniques, we implemented MRS, a web-based information system that provides recommendations for cinema movies. The interested reader is strongly encouraged to visit the web site (http://diat11.csd.uoc.gr) to obtain a more detailed view.
Algorithm 4. Recommendation algorithm based on user preference to categories Worst case: (max-number of categories for an item)*(max-number of not rated items)
Algorithm 3. Recommendation algorithm based on user explicit ratings to items Worst case: (max-number of buddies) * (max-number of not rated items)
3.3 Recommendation Algorithm Based on User Preference to Categories
2 Two Alternative User Models
Explicit ratings are considered when a specific user rates a specific item with a specific rating. We consider that the total of a user’s explicit ratings comprises his profile. Explicit ratings to an item are also considered implicit ratings to the categories that this item belongs to. Implicit ratings are used to build an alternative user profile that expresses user preference to specific category of items.
given against the negative ones 4. Calculate the average of positive percentages of all these categories 5. If the average is bigger than a threshold then recommend it to the user 6. Continue with the second step until there are not any remaining items
1. Find users that have rated at least once 2. Compare the profile of the subject user with each one of these users
a. Find the items that both have rated b. Use PCC to calculate the relevance of the two profiles based on explicit ratings 3. If the PCC is greater than a threshold then consider the user as “buddy” else not 4. Continue with the second step until there are not remaining users
a. Take the occasional user’s profile based on genre preference b. Take the subject user’s profile based on genre preference c. Calculate the PCC between the two profiles 3. If the PCC is greater than a threshold then consider the user as “buddy” else not 4. Continue with the second step until there are not remaining users
Recommenmic Virtual Communities 3
1. Find the items that user has not rated yet 2. For each one of them find the categories in which the item belongs to 3. For each one of these categories calculate the percentage of the positive ratings that the user has
Algorithm 1. Find Buddies according to explicit ratings
1. Find users that have rated at least once 2. Find the relevance between each user and the subject user
User preference to categories can also be used to form recommendations. We propose the following algorithm in order to form recommendations exploiting the user model built by implicit ratings to categories.
Algorithm 2. Find Buddies According to user category preferences
3.2 Recommendation Algorithm Based on User’s Explicit Ratings
The following algorithm recommends items that users with similar profile like. In order to do so, it uses the “Find Buddies according to explicit ratings” algorithm to calculate buddies and puts into practice the pure collaborative filtering method [2].
Recommendation Based Discovery of Dynamic Virtual Communities 2
on the alternative user models. Both make use of the Pearson Correlation Coefficient [3] (PCC) measure in order to calculate the relevance of two user profiles and their complexity is linear on the number of users that have rated at least once.