Conditional Random Field for tracking user behavior based on his eye’s
crf 条件随机场模型 语义分割

crf 条件随机场模型语义分割全文共四篇示例,供读者参考第一篇示例:条件随机场(Conditional Random Field, CRF)是一种统计学习模型,广泛应用于图像处理、自然语言处理等领域。
在语义分割任务中,CRF模型被用来提高像素级别的分类性能,从而实现对图像中不同物体的精确分割。
语义分割是计算机视觉领域的一个重要任务,其目的是将图像中的每个像素分配到一个特定的类别中,例如背景、道路、汽车等。
传统的像素级别分类方法往往有较高的误差率,无法很好地处理物体边界和细节。
引入CRF模型可以提高语义分割的精确度和鲁棒性。
CRF模型是一种有向图模型,用于描述随机变量之间的依赖关系。
在语义分割任务中,CRF模型将每个像素看作一个节点,并通过定义条件概率分布来学习像素之间的权重关系。
CRF模型考虑了局部和全局信息,并通过最大化条件概率来进行推理,从而实现对图像的语义分割。
CRF模型在语义分割中的应用主要包括两个方面:特征提取和后处理。
在特征提取方面,CRF模型可以学习像素间的空间相关性、颜色一致性等特征,有效提升图像分类性能。
在后处理方面,CRF模型可以对分割结果进行平滑处理,减少噪声和边缘混叠现象。
在实际应用中,CRF模型通常与深度学习模型结合使用,构建端到端的语义分割网络。
深度学习模型用于提取图像的高层语义特征,CRF 模型则用于优化像素级别的分类结果,相互补充、互相改进,实现更加精确的语义分割。
除了在图像处理领域,CRF模型还被广泛应用于自然语言处理、机器翻译等领域。
在自然语言处理中,CRF模型可以用于标注命名实体、识别句法结构等任务,提高文本处理的准确度和效率。
第二篇示例:条件随机场模型(CRF,Conditional Random Fields)是一种用于序列标注和结构化预测问题的概率图模型,经常用于自然语言处理和计算机视觉领域。
在语义分割任务中,CRF模型有着重要的应用价值,可以有效地提高图像分割的准确性和稳定性。
条件随机场
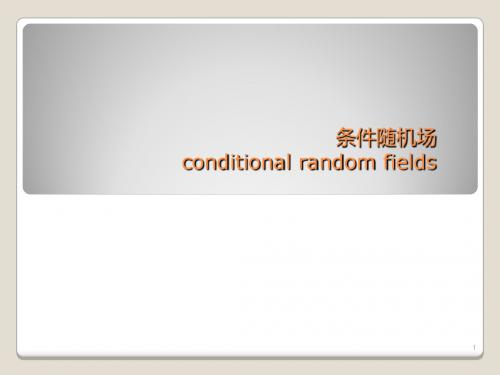
4
一个举例: (1,0), (1,0), (2,0), (2, 1) 产生式模型: p(x, y): P(1, 0) = 1/2, P(1, 1) = 0, P(2, 0) = 1/4, P(2, 1) = 1/4. 判别式模型: P(y|x): P(0|1) = 1, P(1|1) = 0, P(0|2) = 1/2, P(1|2) = 1/2
( )
C2
(x4 , x2 , x3 X )
3
X4
无向图:联合分布的因式分解 无向图:
12
若一个分布可用一个特定的图因式分解,则称该 分布满足无向因式分解性质F F 定理:对任意图和任意分布:F => G F 定理:(Hammersley-Clifford):对严格正的分 布和任意图: G => F
26
条件随机场
27
我们不妨拿种地来打个比方。其中有两个概念:位置(site),相空 间 (phase space)。“位置”好比是一亩亩农田;“相空间”好 比是种的各种庄稼。我们可以给不同的地种上不同的庄稼,这就好比 给随机场的每个“位置”,赋予相空间里不同的值。所以,俗气点说 ,随机场就是在哪块地里种什么庄稼的事情。 简单地讲,随机场可以看成是一组随机变量的集合(这组随机变量对 应同一个样本空间)。当给每一个位置中按照某种分布随机赋予相空 间的一个值之后,其全体就叫做随机场。 当然,这些随机变量之间可能有依赖关系,一般来说,也只有当这些 变量之间有依赖关系的时候,我们将其单独拿出来看成一个随机场才 有实际意义。
5
o和s分别代表观察序列和标记序列 产生式模型 构建o和s的联合分布p(s,o) 判别式模型 构建o和s的条件分布p(s|o) 产生式模型中,观察序列作为模型的一部分; 判别式模型中,观察序列只作为条件,因此可 以针对观察序列设计灵活的特征。
CRRT学习心得
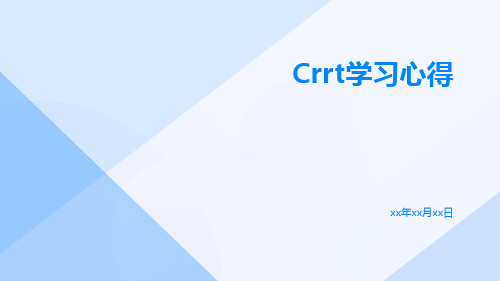
VS
详细描述
Crrt,全称条件随机场(Conditional Random Field),是一种用于预测序列 数据的模型,特别适合于标注问题。它利 用条件概率对观察序列和标签序列进行建 模,通过最大化一个关于观察序列和标签 序列的联合词
掌握Crrt算法的处理流程,包括输入、前向传播、后向 传播、推断等步骤。
1. 编程语言
如Python,Java等编程语言是实现Crrt算 法的常用语言。
03
Crrt与其他算法比较
Crrt与KNN比较
• 总结词:相同点、不同点 • 相同点 • 都可以用于分类问题 • 都需要对输入数据进行相似性度量 • 都对输入数据的特征进行提取 • 不同点 • KNN是一种懒散学习算法,模型训练只发生在预测阶段,导致训练时间较长 • Crrt是一种结构化学习算法,通过构建一个判别模型进行训练和预测
Crrt与决策树比较
• 总结词:相同点、不同点 • 相同点 • 都可以用于分类和回归问题 • 都具有白盒特性,模型可解释性强 • 都对输入数据的特征进行提取 • 不同点 • 决策树是一种基于树的算法,通过递归地将数据集划分成若干个子集来构建模型,过度拟合问题较严重 • Crrt是一种基于集成学习的算法,通过多个弱学习器来共同决策,能够减轻过拟合问题
Crrt与神经网络比较
• 总结词:相同点、不同点 • 相同点 • 都对输入数据的特征进行提取 • 都具有黑盒特性,模型较为复杂 • 都支持批量和在线学习 • 不同点 • 神经网络是一种基于神经元的算法,可以模拟人脑神经元的连接方式,具有很强的表达能力,但容易过拟
合,需要更多的数据和计算资源 • Crrt是一种基于集成学习的算法,通过多个弱学习器来共同决策,能够减轻过拟合问题,同时需要的参数较
图像分割与目标检测中的不确定性建模研究
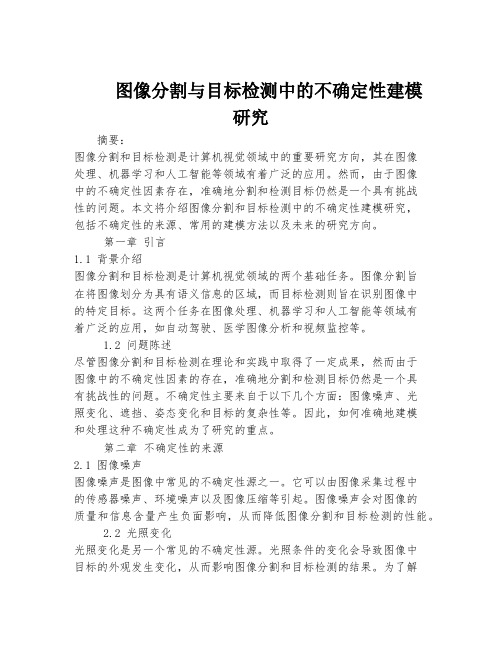
图像分割与目标检测中的不确定性建模研究摘要:图像分割和目标检测是计算机视觉领域中的重要研究方向,其在图像处理、机器学习和人工智能等领域有着广泛的应用。
然而,由于图像中的不确定性因素存在,准确地分割和检测目标仍然是一个具有挑战性的问题。
本文将介绍图像分割和目标检测中的不确定性建模研究,包括不确定性的来源、常用的建模方法以及未来的研究方向。
第一章引言1.1 背景介绍图像分割和目标检测是计算机视觉领域的两个基础任务。
图像分割旨在将图像划分为具有语义信息的区域,而目标检测则旨在识别图像中的特定目标。
这两个任务在图像处理、机器学习和人工智能等领域有着广泛的应用,如自动驾驶、医学图像分析和视频监控等。
1.2 问题陈述尽管图像分割和目标检测在理论和实践中取得了一定成果,然而由于图像中的不确定性因素的存在,准确地分割和检测目标仍然是一个具有挑战性的问题。
不确定性主要来自于以下几个方面:图像噪声、光照变化、遮挡、姿态变化和目标的复杂性等。
因此,如何准确地建模和处理这种不确定性成为了研究的重点。
第二章不确定性的来源2.1 图像噪声图像噪声是图像中常见的不确定性源之一。
它可以由图像采集过程中的传感器噪声、环境噪声以及图像压缩等引起。
图像噪声会对图像的质量和信息含量产生负面影响,从而降低图像分割和目标检测的性能。
2.2 光照变化光照变化是另一个常见的不确定性源。
光照条件的变化会导致图像中目标的外观发生变化,从而影响图像分割和目标检测的结果。
为了解决这个问题,研究者们提出了各种光照不变模型和算法。
2.3 遮挡遮挡是图像分割和目标检测中常见的挑战之一。
当目标被其他物体部分或完全遮挡时,算法很难准确地分割和检测出目标。
因此,如何有效地处理遮挡是图像分割和目标检测中的一个重要问题。
2.4 姿态变化目标的姿态变化也会带来不确定性。
例如,当目标发生旋转、倾斜或变形时,传统的算法往往难以准确地分割和检测目标。
因此,姿态不变模型和算法的研究成为了研究的热点。
条件随机场在自然语言处理中的应用(八)
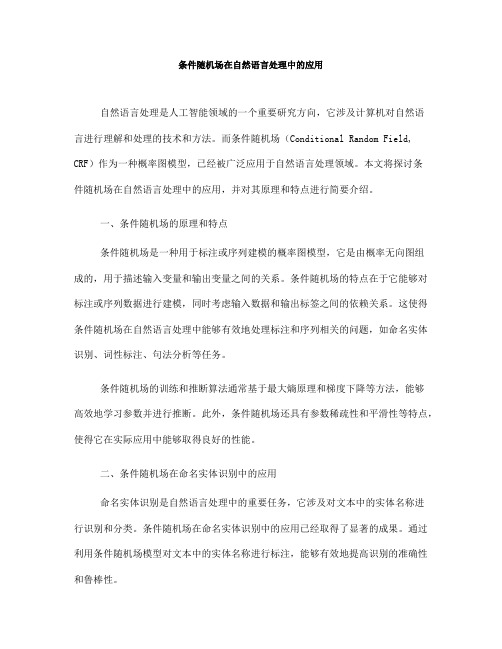
条件随机场在自然语言处理中的应用自然语言处理是人工智能领域的一个重要研究方向,它涉及计算机对自然语言进行理解和处理的技术和方法。
而条件随机场(Conditional Random Field, CRF)作为一种概率图模型,已经被广泛应用于自然语言处理领域。
本文将探讨条件随机场在自然语言处理中的应用,并对其原理和特点进行简要介绍。
一、条件随机场的原理和特点条件随机场是一种用于标注或序列建模的概率图模型,它是由概率无向图组成的,用于描述输入变量和输出变量之间的关系。
条件随机场的特点在于它能够对标注或序列数据进行建模,同时考虑输入数据和输出标签之间的依赖关系。
这使得条件随机场在自然语言处理中能够有效地处理标注和序列相关的问题,如命名实体识别、词性标注、句法分析等任务。
条件随机场的训练和推断算法通常基于最大熵原理和梯度下降等方法,能够高效地学习参数并进行推断。
此外,条件随机场还具有参数稀疏性和平滑性等特点,使得它在实际应用中能够取得良好的性能。
二、条件随机场在命名实体识别中的应用命名实体识别是自然语言处理中的重要任务,它涉及对文本中的实体名称进行识别和分类。
条件随机场在命名实体识别中的应用已经取得了显著的成果。
通过利用条件随机场模型对文本中的实体名称进行标注,能够有效地提高识别的准确性和鲁棒性。
条件随机场在命名实体识别中的优势在于它能够考虑上下文信息和标注序列之间的依赖关系,提高了模型的泛化能力。
此外,条件随机场还能够利用大量的特征信息进行建模,从而提高了模型的判别能力和鲁棒性。
因此,条件随机场在命名实体识别中得到了广泛的应用,并取得了令人满意的效果。
三、条件随机场在词性标注中的应用词性标注是自然语言处理中的另一个重要任务,它涉及对文本中的词语进行词性标注。
条件随机场在词性标注中也发挥了重要作用。
通过利用条件随机场模型对文本中的词性进行标注,能够有效地提高标注的准确性和一致性。
条件随机场在词性标注中的应用优势在于它能够充分利用上下文信息和词性标注序列之间的依赖关系,提高了模型的泛化能力。
条件随机场模型在医疗保险欺诈检测中的应用

医疗保险欺诈一直是一个严重的社会问题,它不仅导致了医疗资源的浪费,也损害了医保系统的公平性和可持续性。
为了有效地检测医疗保险欺诈行为,人们利用各种机器学习和数据挖掘技术,其中条件随机场模型便是一种被广泛应用的方法。
条件随机场(Conditional Random Field, CRF)是一种概率图模型,它在给定输入随机变量的条件下,对输出随机变量建模。
在医疗保险欺诈检测中,CRF模型可以被用来分析和建模医疗保险数据,识别异常行为和欺诈模式。
首先,CRF模型可以用来建立医疗保险数据的关联性。
医疗保险数据通常包括患者的个人信息、就诊记录、疾病诊断、药物处方等内容,这些数据之间存在着复杂的关联关系。
CRF模型可以通过学习这些数据之间的关联性,识别出异常的数据模式和行为,从而发现潜在的欺诈行为。
其次,CRF模型可以用来识别医疗保险欺诈的特征。
医疗保险欺诈行为通常表现为异常的就诊模式、频繁的药物处方、虚假的疾病诊断等特征。
CRF模型可以通过学习这些特征的分布规律,构建欺诈行为的模式,从而识别出潜在的欺诈案例。
此外,CRF模型还可以用来建立医疗保险数据的时空关系。
医疗保险数据通常包括患者的就诊时间、地点等信息,这些信息之间存在着时空的关联关系。
CRF模型可以通过学习这些时空关系,发现异常的就诊轨迹和活动模式,从而识别出潜在的欺诈行为。
在实际应用中,人们可以利用CRF模型对医疗保险数据进行建模和分析,发现潜在的欺诈案例。
通过结合其他机器学习和数据挖掘技术,如聚类分析、异常检测等方法,人们可以构建一个完整的医疗保险欺诈检测系统,提高欺诈检测的准确性和效率。
总的来说,条件随机场模型在医疗保险欺诈检测中具有重要的应用前景。
它可以帮助人们分析和建模医疗保险数据,识别异常行为和欺诈模式,从而有效地检测和预防医疗保险欺诈行为。
随着机器学习和数据挖掘技术的不断发展,相信CRF 模型在医疗保险欺诈检测领域会发挥越来越重要的作用。
时间序列预测中的马尔可夫过程

时间序列预测中的马尔可夫过程时间序列预测是一种重要的数据分析方法,它可以帮助我们理解和预测未来的趋势和模式。
马尔可夫过程是时间序列预测中常用的一种模型,它基于马尔可夫性质,通过分析过去的数据来预测未来的状态。
马尔可夫过程是一种具有马尔可夫性质的随机过程,即未来的状态只与当前的状态有关,与过去的状态无关。
这种性质使得马尔可夫过程在时间序列预测中具有很大的应用潜力。
在马尔可夫过程中,每个状态都有一个转移概率,表示从当前状态转移到下一个状态的概率。
通过分析这些转移概率,我们可以推断出未来的状态。
马尔可夫过程在实际应用中有着广泛的应用。
例如,在股票市场中,我们可以将股票的价格看作是一个马尔可夫过程,通过分析过去的价格走势,我们可以预测未来的价格走势。
在天气预测中,我们可以将天气的状态看作是一个马尔可夫过程,通过分析过去的天气情况,我们可以预测未来的天气情况。
在自然语言处理中,我们可以将文本的生成看作是一个马尔可夫过程,通过分析过去的文本数据,我们可以生成新的文本。
然而,马尔可夫过程也存在一些限制和挑战。
首先,马尔可夫过程假设未来的状态只与当前的状态有关,与过去的状态无关。
这在某些情况下可能不成立,例如,在股票市场中,未来的价格可能受到多个因素的影响,而不仅仅是当前的价格。
其次,马尔可夫过程假设转移概率是固定的,不随时间变化。
然而,在实际应用中,转移概率可能会随时间变化,例如,在天气预测中,转移概率可能会受到季节和气候变化的影响。
为了克服这些限制和挑战,研究人员提出了许多改进和扩展的马尔可夫过程模型。
例如,隐马尔可夫模型(Hidden Markov Model,HMM)是一种扩展的马尔可夫过程模型,它引入了隐藏状态和观测状态的概念。
通过分析观测状态和隐藏状态之间的关系,HMM可以更准确地预测未来的状态。
另外,条件随机场(Conditional Random Field,CRF)是一种基于马尔可夫过程的图模型,它可以对序列数据进行建模和预测。
条件随机场在电力系统中的应用(八)

条件随机场在电力系统中的应用一、电力系统的重要性电力系统是现代社会的重要基础设施之一,它为工业生产、农业生产、居民生活提供了必不可少的电力能源。
随着社会的发展和科学技术的进步,对电力系统的要求也越来越高。
因此,如何提高电力系统的效率和安全性成为了亟待解决的问题。
二、条件随机场的概念条件随机场(Conditional Random Field,CRF)是一种概率图模型,它主要用于对序列标注、分割和结构化预测等问题进行建模和求解。
条件随机场具有很强的建模能力,能够很好地处理输入变量之间的关联性,因此在电力系统中具有广泛的应用前景。
三、条件随机场在电力设备故障诊断中的应用电力系统中的设备故障是一个常见且严重的问题,一旦出现故障可能会导致供电中断,给生产和生活带来严重影响。
利用条件随机场对电力设备的运行状态进行建模,可以实现对设备运行状态的实时监测和故障诊断。
通过分析设备的运行数据,可以对设备的状态进行预测,并及时采取措施进行维修和保养,从而提高电力系统的可靠性和稳定性。
四、条件随机场在电力负荷预测中的应用电力负荷预测是电力系统运行和规划的重要组成部分,准确的负荷预测能够有效地指导电力调度和供需平衡。
条件随机场可以很好地处理负荷数据的时空关联性,提高负荷预测的准确性和稳定性。
通过对历史负荷数据的分析和建模,可以实现对未来负荷的准确预测,为电力系统的规划和运行提供重要参考。
五、条件随机场在电力设备状态评估中的应用电力设备的状态评估是保证电力系统安全稳定运行的重要手段,传统的基于规则的状态评估方法存在着局限性和不足。
条件随机场可以很好地捕捉设备运行状态之间的复杂关系,通过对设备状态数据的建模和分析,可以实现对设备状态的准确评估,并及时发现潜在的问题和隐患,为设备的维护和管理提供科学依据。
六、条件随机场在电力故障风险评估中的应用电力系统的故障风险评估是预防故障和提高系统可靠性的重要手段,传统的基于统计的风险评估方法存在着样本数据不足和模型假设不准确等问题。
- 1、下载文档前请自行甄别文档内容的完整性,平台不提供额外的编辑、内容补充、找答案等附加服务。
- 2、"仅部分预览"的文档,不可在线预览部分如存在完整性等问题,可反馈申请退款(可完整预览的文档不适用该条件!)。
- 3、如文档侵犯您的权益,请联系客服反馈,我们会尽快为您处理(人工客服工作时间:9:00-18:30)。
Conditional Random Field for tracking user behavior based on his eye’smovements1Trinh Minh Tri Do Thierry ArtièresLIP6, Université Paris 6 LIP6, Université Paris 68 rue du capitaine Scott 8 rue du capitaine Scott75015, Paris, France 75015, Paris, Francedo@poleia.lip6.fr Thierry.artieres@lip6.frAbstractConditional Random Fields offer some advantages over traditionalmodels for sequence labeling. These conditional models havemainly been introduced up to now in the information retrievalcontext for information extraction or POS-tagging tasks. This paperinvestigates the use of these models for signal processing andsegmentation. In this context, the input we consider is a signal thatis represented as a sequence of real-valued feature vectors and thetraining is performed using only partially labeled data. We proposea few models for dealing with such signals and provideexperimental results on the data from the eye movement challenge.1IntroductionHidden Markov models (HMM) have long been the most popular technique for sequence segmentation, e.g. identifying the sequence of phones that best matches a speech signal. Today HMM is still the core technique in most of speech engines or handwriting recognition systems. However, HMM suffer two major drawbacks. First, they rely on strong independence assumptions on the data being processed. Second, they are generative models that are most often learned in a non discriminant way. This comes from their generative nature, since HMM define a joint distribution P(X,Y) over the pair of the input sequence (observations) X and the output sequence (labels) Y. Recently, conditional models including Maximum Entropy Markov models [1] and Condition Random Fields [2] have been proposed for sequence labeling. These models aim at estimating the conditional distribution P(Y/X) and exhibit, at least in theory, strong advantages over HMMs. Being conditional models, they do not assume independence assumptions on the input data, and they are learned in a discriminant way. However, they rely on the manual and careful design of relevant features.1This work was supported in part by the IST Program of the European Community, under the PASCAL Network of Excellence, IST-2002-506778.Conditional Random Fields (CRF) has been shown to overcome traditional Markovian models in a series of information retrieval tasks such as information extraction, named entity recognition… Yet, CRF have to be extended to more general signal classification tasks. Indeed, the information retrieval context is very specific, considering for instance the nature of the input and output data. Designing relevant features for such data is maybe easier than for many other data. Also, algorithms proposed for training CRF require a fully labeled training database. This labeling may be available in information retrieval tasks since there is a kind of equivalence between nodes and labels but it is generally not available in other signal processing tasks. Hence, the previous usages of CRF do not fit well with many sequence classification and segmentation tasks concerning signals such as speech, handwriting etc. Input data is rougher; it is a sequence of real-valued feature vectors without precise semantic interpretation. Defining relevant features is then difficult. Also, training databases are not fully labeled. In speech and handwriting recognition for instance, data are labeled, at best, at the unit (phoneme or letter) level while it is often desirable to use a number of states for each unit, or even a number of modalities for a same unit (e.g. allograph in handwriting recognition).This paper investigates the use of CRF models for such more general signal classification and segmentation tasks. We first introduce CRF and an extension called segmental CRF. Then we describe how to use CRF for dealing with multimodal classes and signal data and discuss corresponding inference and training algorithms. At last, we report experimental results concerning the eye movement challenge.2 Conditional Random Fields fo r sequen tial dataSequence labeling consists in identifying the sequence of labels T y y Y ,...,1= thatbest matches a sequence of observations T x x X ...1=: )/(max arg *X Y P Y Y =. CRF are a particular instance of random fields. Figure 1 illustrates the difference between traditional HMM models and CRF. HMM (Fig. 1-a) are directed models where independence assumptions between two variables are expressed by the absence of edges. CRF are undirected graphical models, Figure 1-b shows a CRF with a chain structure. One must notice that CRF being conditional models, node X is observed so that X has not to be modeled. Hence CRF do not require any assumption about the distribution of X . From the random field theory, on can show [2] that the likelihood ()X Y P / may be parameterized as:())(,/),(.')',(.),(.X Z e e e W X Y P WY X F W Y Y X F W Y X F W ==∑ (1) Where ()X Z W =∑')',(.Y Y X F W e is a normalization factor, ),(Y X F is a feature vector and W is a weight vector. Features F(X,Y) are computed on maximal cliques of the graph. In the case of a chain structure (Fig. 1-b), these cliques are edges and vertices (i.e. a vertice y t or an edge (y t-1 , y t )).In some cases there is a need to relax the Markovian hypothesis by allowing the process not to be Markovian within a state. [3] proposed for this semi-Markov CRF (SCRF). The main idea of these models is to use segmental features, computed on a segment of observations associated to a same label (i.e. node). Consider a segmentation of an input sequence T x x x X ,...,,21=, this segmentation may be described as a sequence of segments J s s s S ,...,,21=, with T J ≤and ()j j j j y l e s ,,=where j e (j l ) stands for the entering (leaving) time in state (i.e. label)j y . Segmental features are computed over segments of observations j j l e x x ,..., corresponding to a particular label j y . SCRF aims at computing )/(X S P defined as in Eq. (1). To enable efficient dynamic programming, one assumes that the features can be expressed in terms of functions of X , s j and y j-1, these are segmental features:),,,,(),,(),(1111j j Sj j j j S j j l e y y X F s y X F S X F =−=−==(2) Inference in CRF and SCRF is performed with dynamic programming like algorithm. Depending on the underlying structure (chain, tree, or anything else) one can use Viterbi, Belief Propagation [4] or Loopy Belief Propagation [5]. Training in CRF consists in maximizing the log-likelihood L(W) based on a fully labeleddatabase of K samples, (){}K k k k Y X BA 1,==, where k X is a sequence of observationsand Y k is the corresponding sequence of labels.∑∑==−==Nk k W k k K k k k X Z Y X F W W X Y P W L 11))(log ),(.((),/(log )( (3)This convex criterion may be optimized using gradient ascent methods. Note that computing )(X Z W includes a summation over an exponential number of label sequences that may be computed efficiently using dynamic programming. Training SCRF is very similar to CRF training and also relies on a fully labeled database.(a) (b)Figure 1: Dynamic representation of HMM (a) and CRF (b) as graphical models, wheregrey nodes represent observed variables.3 Semi-Markov CRF for signal segmentationWe investigate the use of segmental CRF for signal segmentation. When dealing with real signals, one has to consider the continuous nature of input data, the multimodality of the classes and one has to develop algorithms for learning models without a fully labeled dataset. Hence, in the following we will consider that, during training, the label Y k corresponding to input sequence X k consists in the sequence of classes in X k , whatever the length of the segments associated to these classes.To take into account multimodality (e.g. a letter may be written with different styles) we investigate the use of a few states in a Segmental CRF model for each class, each one corresponds to a modality of the class. We will note K the number of states sharing the same label. Since there are several states corresponding to the same label, there are a number of segmentations S that correspond to a particular label sequence Y. Following [6] we introduce hidden variables for multimodality and segmentation information and build upon their work to develop inference and training algorithms with incomplete data. Hence, when conditioned on an input X the likelihood of a label sequence Y is defined as:()()∑∑∑∑∑∈∈=='')(),,(.)(//S M Y S S M M S X F W Y S S ee X S P X Y P (4) Where S(Y) stands for the set of segmentations S (defined as in §2) corresponding to the sequence of labels Y , M denotes a sequence of hidden variables, with {}K mi ,...,1∈. The use of hidden variables (S,M) makes inference expensive:⎥⎦⎤⎢⎣⎡==∑∈M Y S S M S X F W Y Y e X Y P Y ),(),,(.max arg )/(max arg *(5) Where Y , S and M have the same length, say T . This expression cannot be computed with a dynamic programming routine since the maximum and sum operators cannot be exchanged. However, if one uses a Viterbi approximation where summation is replaced with the maximum operator, and one assumes that ),,(.M S X F W e may be factorized in a product of T independent terms then the double maximization may be computed efficiently. Hence we use:()⎥⎦⎤⎢⎣⎡≈∈W X M S P Y M Y S S Y ,/,Max max arg ),(*(6)Training aims at maximizing the log-likelihood L(W). Using Eq. (4), the derivative of the likelihood of the k th training example is computed as:∑∑∑∑−=∈'')()',',().,/','(),,().,,/,()(S M k v k Y S S M k v k k vk M S X F W X M S P M S X F W X Y M S P w W L k δδ (7)This criterion is expressed in terms of expected values of the features under the current weight vector that are ),,(),,/,(M S X F E k v W k X k Y M S P and ),,(),/,(M S X F E k v W k X M S P . These terms may be calculated using a forward-backward like algorithm since the CRF is assumed to have a chain structure. Based on the chain structure of the models we used two types of features: local features (computed on vertices) ),,,(1t t t m q y X F and transition features computed on edges,),,,,,,(1112−−−t t t t t t m m q q y y X F .4 Eye movement challenge dataHere is a quick description of the challenge and of the data for the competition 1 of the challenge, see [7] for more details. The eye movement challenge concerns implicit feedback for information retrieval. The experimental setup is as follows. A subject was first shown a question, and then a list of ten sentences (called titles), one of which contained the correct answer (C). Five of the sentences were known to be irrelevant (I), and four relevant for the question (R). The subject was instructed to identify the correct answer and then press ’enter’ (which ended the eye movement measurement) and then type in the associated number in the next screen. There are 50 such assignments, shown to 11 subjects. The assignments were in Finnish, the mother tongue of the subjects. The objective of the challenge is, for a given assignment, to predict the correct classification labels (I, R, C) of the ten sentences (actually only those that have been viewed by the user) based on the eye movementsalone. The database is divided in a training set of 336 assignments and a test set (the validation set according to challenge terminology) of 149 assignments. The data of an assignment is in the form of a time series of 22-dimensional eye movement features derived from the ones generally used in eye movement research (see [7]), such as fixation duration, pupils diameter etc. It must be noticed that there is a 23th feature that consists in the number of the title being viewed (between 1 and 10).5Experi mentsWe applied segmental CRF as those described in §3 to eye movement data. The aim is to label the ten titles with their correct labels (I, R, C). This may be done though segmenting an input sequence with a CRF whose states correspond to labels I, R and C. We investigated a number of models for this. All models have been trained with a regularized likelihood criterion in order to avoid over fitting [6]. These models work on vectors of segmental features computed over segments of observations. A simple way to define segmental feature vector would be the average feature vector over the observations of the segment. However, the average operator is not necessarily relevant. We used ideas in [7] to choose the most adequate aggregation operator (sum, mean or max) for each of the 22 features.The first model is a simple one. It is a SCRF model with three nodes, one for class R, one for class C and one for class I. It works on segmental features where segments correspond to sequences where the user visits one particular title. There is no transition features, corresponding to the change from one title to another one. This model is called 3NL for 3 Nodes CRF with Local features only (no transition features) and 3NLT if transitions features are added. It must be noticed that since a title may be visited more than on time in an assignment it is desirable that the labeling algorithm be consistent, i.e. finds a unique label for every title. This is ensured, whatever the model used, by adding constraints in the decoding algorithm. One can design more complicated models by distinguishing between the different visits of a same title. For example, one can imagine that a user who visits a title a second or a third time will not behave as he did the first time. Maybe he may take more time or quickly scan all the words in the title… Hence, we investigated the use of SCRF models with two or three states per class (I, R, C). In the two states models, a first state is dedicated to the first visit to a title of class R, C or I. The second state is dedicated to all other posterior visits to this title. When using 3 states per class, we distinguish among the first visit, the last visit and intermediate visits to a title. These models are named 6NL and 9NL depending on their number of states per class (2 or 3) if they make use of local features, and 6NLT and 9NLT if they make use of local and transition features.Finally, we investigate the use of multimodal models. Going back to the first model 3NL, we consider the use of a few states per class, this time corresponding to different ways of visiting a title (there is no chronological constraints). Models are named 3N2ML for 3 states, 2 Modes per class, and Local features.Table 1 reports experimental results for various SCRF-based models and for 4 additional systems. The first one is a benchmark HMM system. It works on the same input representation (feature vectors) and has the same number of states as there are nodes in the 6NL model. The three other systems are combination systems combine three classifiers votes.A first comment about the results is that all SCRF models outperform the HMM system. Also, using more complex models is not systematically better useful. We investigated two ways for this, firstly by taking into account the number of the visit (increasing the number of states), secondly by taking into account multimodality(increasing the number of modes). Using a few states per class in order to take into account the number of a visit of a title allows reaching up to 73% (9NL) while allowing multimodality leads to poorer results. Also, we did not succeed in using efficiently transition features, this is still under investigation. At last, voting systems did not improve much over singles classifiers although HMM and SCRF systems tend to be complimentary. There is certainly some room for improvements here. Note however that we observed more stability in the results of voting systems when training and testing on various parts of the database.Table 1 – Comparison of various systems on the eye movement challenge task.Technique System’s name#states- #modes Features Accuracy (%)1 L 71SCRF 3NL 3-2 L 71.5- 3N2ML 3-- 3NLT 3 - 1 L + T 68.91 L 71.8- 6NL 6-1 L 73.2- 9NL 9-2 L 70.8-- 9N2ML 9- 9NLT 9 - 1 L + T 69.4HMM HMM 6 states L 66.2Combination of 3NL, 6NL, 9NL L 72.1Combination of HMM, 6NL, 9NL L 72.3Combination of HMM, 3NL, 6NL L 71.96ConclusionWe presented systems based on conditional random fields for signal classification and segmentation. In order to process signals such as eye movement, speech or handwriting, we investigated the use of segmental conditional random fields and introduced the use of hidden variables in order to handle partially labeled data and multimodal classes. Experimental results on the eye movement challenge data show that our CRF models outperform HMM, but all results are rather close showing the difficulty of the task.Re fer e nce s[1] McCallum, A., Freitag, D., and Pereira, F. (2000) Maximum entropy Markov models for information extraction and segmentation. In Proc. ICML.[2] Lafferty, J., McCallum, A., and Pereira, F. (2001) Conditional random fields: Probabilistic models for segmenting and labeling sequence data. International Conf. on Machine Learning, 282–289. Morgan Kaufmann, San Francisco, CA.[3] Sarawagi, S., and Cohen, W. (2004)Semi-Markov Conditional Random Fields for Information Extraction. Advances in Neural Information Processing Systems.[4] Weiss, Y. (2001)Correctness of belief propagation in Gaussian graphical models of arbitrary topology. Neural Computation, 13:2173-2200.[5] Murphy, K., Weiss, Y., and Jordan, M. (1999) Loopy belief propagation for approximate inference: an empirical study. In Proc. of the Conf. on Uncertainty in AI.[6] Quattoni A., Collins M. and Darrel T. (2004). Conditional Random Fields for Object Recognition. In Advances in Neural Information Processing Systems 17.[7] Salojärvi, J., Puolamäki, K., Simola, J., Kovanen, L., Kojo, I., Kaski, S. (2005) Inferring Relevance from Eye Movements: Feature Extraction. Helsinki University of Technology, Publications in Computer and Information Science, Report A82.。