智能视频监控系统
智能视频监控系统公司简介

智能视频监控系统公司简介在当今数字化、信息化的时代,安全保障成为了各行各业以及人们日常生活中至关重要的需求。
智能视频监控系统作为一种高效、可靠的安全防范手段,正发挥着越来越重要的作用。
而在这个领域中,我们的公司凭借着卓越的技术、专业的团队和优质的服务,成为了行业内的佼佼者。
我们是一家专注于智能视频监控系统研发、生产、销售和服务的高科技企业。
公司成立于_____年,总部位于_____,在全国多个城市设有分支机构和售后服务中心。
多年来,我们一直致力于为客户提供最先进、最可靠的智能视频监控解决方案,以满足不同行业和场景的安全需求。
公司拥有一支高素质、经验丰富的研发团队,成员均来自于国内知名高校和科研机构,具备深厚的专业知识和丰富的实践经验。
他们紧跟科技发展的前沿趋势,不断探索和创新,将最新的技术应用于智能视频监控系统的研发中。
目前,公司已经拥有多项自主知识产权和核心技术,包括视频图像分析算法、智能识别技术、大数据处理技术等,这些技术的应用使得我们的智能视频监控系统在性能、准确性和稳定性方面都处于行业领先水平。
在产品方面,我们的智能视频监控系统涵盖了前端设备、传输设备、存储设备和后端管理平台等多个环节,形成了一套完整的解决方案。
前端设备包括高清摄像机、智能球机、半球摄像机等,具备高清晰度、宽动态范围、低照度等优良性能,能够在各种复杂环境下清晰地捕捉图像。
传输设备采用先进的网络技术,确保视频数据的稳定、快速传输。
存储设备则提供了大容量、高可靠性的存储空间,保证视频数据的安全存储。
后端管理平台是整个系统的核心,具备视频监控、录像回放、报警管理、智能分析等多种功能,用户可以通过简单直观的操作界面,轻松实现对监控系统的管理和控制。
我们的智能视频监控系统广泛应用于多个领域,如公共安全、交通管理、金融行业、教育医疗、商业零售等。
在公共安全领域,我们的系统为公安机关提供了有力的支持,帮助他们及时发现和处理各类违法犯罪行为,保障社会的安定和谐。
视频监控系统概述
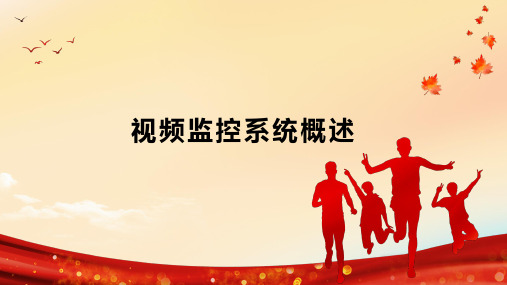
内容摘要
智慧视频监控系统是一种基于数字视频技术和人工智能技术的先进监控系统。 它利用高清晰度的摄像头进行图像采集,通过高效的视频编码技术将图像传输到 监控中心,再利用人工智能技术对图像进行分析和处理,从而实现对目标物体的 实时监控和智能识别。
内容摘要
相比于传统的视频监控系统,智慧视频监控系统具有以下优势: 1、智能识别:智慧视频监控系统能够自动识别目标物体,如人脸、车辆、行 为等,实现了智能化的监控和管理。
谢谢观看
一、智能视频监控系统设计理念
一、智能视频监控系统设计理念
智能视频监控系统的设计理念是将视频信息与人工智能(AI)技术相结合, 实现视频数据的智能分析、处理和存储。通过这种方式,智能视频监控系统能够 自动化地识别异常情况、检测目标、跟踪动态事件,从而大大提高监控的效率和 准确性。
二、智能视频监控系统架构
六、未来发展趋势
六、未来发展趋势
随着、物联网等技术的不断发展,视频监控系统的未来发展趋势将更加智能 化和网络化。智能化方面,视频监控系统将引入更多的技术,如目标检测、人脸 识别等,进一步提高监控的准确性和效率。网络化方面,随着5G等网络技术的普 及和应用,视频监控系统的传输速度和稳定性将得到进一步提升,同时将促进更 多智能设备的互联互通,实现更加智能化的管理和服务。
内容摘要
2、实时监控:通过高清摄像头和高性能的视频编码技术,智慧视频监控系统 能够实时传输图像,保证了监控的及时性和准确性。
内容摘要
3、高效存储:智慧视频监控系统采用了高效的数据存储技术,能够将大量的 视频数据进行存储和管理,方便后期的查询和分析。
内容摘要
4、远程控制:通过互联网技术,智慧视频监控系统可以实现远程控制和管理, 方便用户对监控设备进行操作和管理。
各类型视频监控系统的架构简介
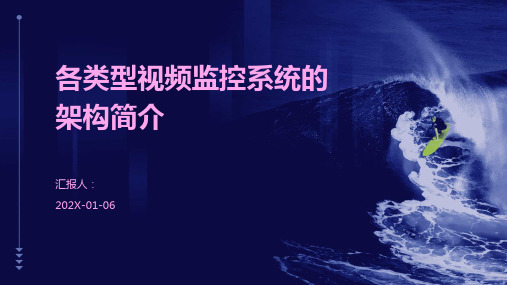
汇报人: 202X-01-06
目 录
• 模拟视频监控系统 • 网络视频监控系统 • 智能视频监控系统 • 高清视频监控系统 • 云视频监控系统
01
模拟视频监控系统
模拟摄像机
模拟摄像机是早期视频监控系统中的主要设备, 通过将摄像机拍摄的模拟信号传输到监控中心。
模拟摄像机通常采用CCD或CMOS传感器,能够 提供较好的图像质量。
02
智能应用软件通常采用可视化界面设计,方便用户进行直观的
操作和监控。
智能应用软件还支持多种操作系统和平台,如Windows、
03
Android、iOS等,方便用户在不同设备上使用。
04
高清视频监控系统
高清摄像机
1
高清摄像机是高清视频监控系统的核心组成部分 ,负责采集高清视频信号。
2
高清摄像机通常采用百万像素或更高像素的传感 器,能够提供高清晰度、高分辨率的图像。
IP摄像机支持远程控制,可以通过网络进行远程调节摄像机的角度、焦距等参数。
网络传输设备
网络传输设备包括交换机、路 由器等,用于将IP摄像机采集 的视频信号传输到NVR/DVR
等存储设备。
网络传输设备支持多种网络 协议,能够保证视频信号的
稳定传输。
网络传输设备还支持远程管理 和维护,方便对整个监控系统
智能分析摄像机还可以支持多种接口 协议,如ONVIF、RTSP等,方便与其 他安防设备进行集成和联动。
智能分析摄像机通常采用高性能的图 像传感器和处理器,能够实时对视频 图像进行智能分析,如人脸识别、行 为识别、异常检测等。
智能分析服务器
智能分析服务器是智能视频监控 系统的核心组件之一,负责接收 和处理摄像机传输的视频数据。
智能化智能视频监控系统的技术要求

智能化智能视频监控系统的技术要求智能视频监控系统是目前安防领域的一项重要技术,它将传统的视频监控系统与人工智能和大数据分析相结合,能够实现对各种场景下的视频进行智能化分析和处理。
在不同的应用场景下,智能视频监控系统的技术要求也不尽相同。
下面将介绍一些智能视频监控系统的通用技术要求。
首先,智能视频监控系统需要具备高清晰度和高画质的视频采集能力。
随着高清晰度摄像头的普及和应用,智能视频监控系统需要能够接受高清晰度视频流,并保证视频质量的稳定和清晰。
其次,智能视频监控系统需要具备智能化的视频分析能力。
这包括人脸识别、行为识别、目标跟踪等功能。
通过使用人工智能算法和大数据分析技术,智能视频监控系统能够自动识别出视频中的人脸、行为和目标,并进行分析和判定。
这对于预防犯罪、保护公共安全有着重要的作用。
此外,智能视频监控系统需要具备实时性和可扩展性。
实时性是指系统需要能够实时对视频流进行处理和分析,及时预警和发现异常情况。
可扩展性是指系统需要能够灵活地扩展和接入新的设备和功能。
智能视频监控系统还需要具备数据存储和管理的能力。
视频监控系统产生的数据量庞大,因此系统需要能够对数据进行有效的存储和管理,包括数据的备份、归档和检索等功能。
此外,智能视频监控系统还需要具备远程监控和管理的能力。
通过网络连接,用户可以随时随地通过远程客户端对视频监控系统进行监控和管理,包括查看视频、设置参数、接收报警信息等。
最后,智能视频监控系统需要具备抗干扰和安全可靠的能力。
系统需要能够抵抗各种噪声和干扰,并保证视频数据的安全和可靠。
这包括数据加密、权限管理、防火墙等安全机制的应用。
总之,智能视频监控系统的技术要求包括高清晰度的视频采集能力、智能化的视频分析能力、实时性和可扩展性、数据存储和管理能力、远程监控和管理能力以及抗干扰和安全可靠的能力。
这些技术要求能够使智能视频监控系统在各种应用场景下发挥出更好的性能和效果。
除了上述提到的技术要求之外,智能视频监控系统还有一些辅助功能和特殊要求,以满足不同场景下的需求。
智能视频监控有什么用
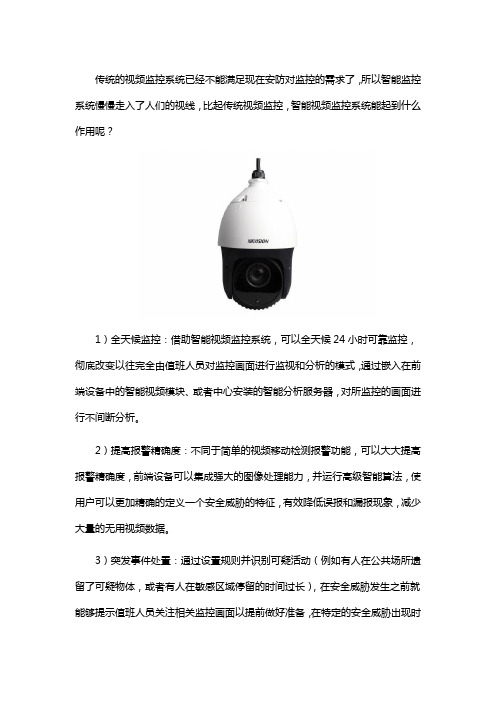
传统的视频监控系统已经不能满足现在安防对监控的需求了,所以智能监控系统慢慢走入了人们的视线,比起传统视频监控,智能视频监控系统能起到什么作用呢?
1)全天候监控:借助智能视频监控系统,可以全天候24小时可靠监控,彻底改变以往完全由值班人员对监控画面进行监视和分析的模式,通过嵌入在前端设备中的智能视频模块、或者中心安装的智能分析服务器,对所监控的画面进行不间断分析。
2)提高报警精确度:不同于简单的视频移动检测报警功能,可以大大提高报警精确度,前端设备可以集成强大的图像处理能力,并运行高级智能算法,使用户可以更加精确的定义一个安全威胁的特征,有效降低误报和漏报现象,减少大量的无用视频数据。
3)突发事件处置:通过设置规则并识别可疑活动(例如有人在公共场所遗留了可疑物体,或者有人在敏感区域停留的时间过长),在安全威胁发生之前就能够提示值班人员关注相关监控画面以提前做好准备,在特定的安全威胁出现时
采取相应的预案,有效防止在混乱中由于人为因素而造成的延误。
4)提高安全部门的保护级别:协助政府或其他机构的安全部门提高室外区域或者公共环境的安全防护。
此类应用主要包括:高级视频移动侦测、物体追踪、人物面部识别、车辆识别、和非法滞留等。
5)视频资源的其他应用:除了安全相关类应用之外,智能视频还可以应用到一些非安全相关类的应用当中。
此类应用主要包括:人数统计、人群控制、注意力控制和交通流量控制等,可以利用智能视频监控系统进行远程视频会议的参加人数统计等功能。
以上就是视频监控能起到的作用了。
如果大家对这类监控系统还有什么疑问的话,成都慧翼科技建议大家找专业的商家多咨询,然后决定是否购买监控。
智能监控系统方案
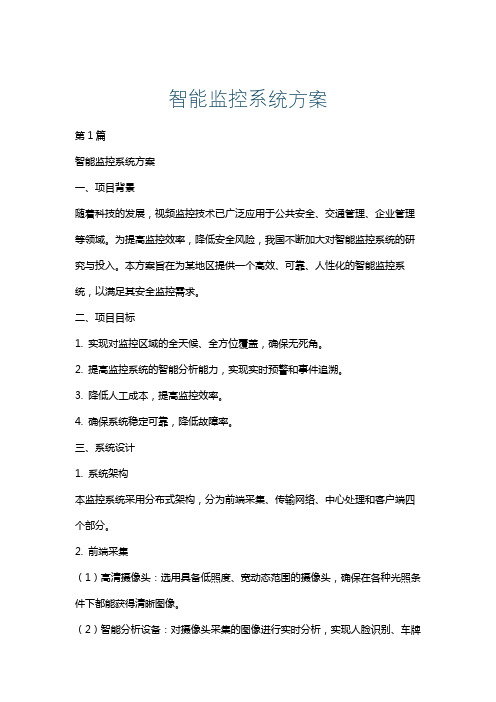
智能监控系统方案第1篇智能监控系统方案一、项目背景随着科技的发展,视频监控技术已广泛应用于公共安全、交通管理、企业管理等领域。
为提高监控效率,降低安全风险,我国不断加大对智能监控系统的研究与投入。
本方案旨在为某地区提供一个高效、可靠、人性化的智能监控系统,以满足其安全监控需求。
二、项目目标1. 实现对监控区域的全天候、全方位覆盖,确保无死角。
2. 提高监控系统的智能分析能力,实现实时预警和事件追溯。
3. 降低人工成本,提高监控效率。
4. 确保系统稳定可靠,降低故障率。
三、系统设计1. 系统架构本监控系统采用分布式架构,分为前端采集、传输网络、中心处理和客户端四个部分。
2. 前端采集(1)高清摄像头:选用具备低照度、宽动态范围的摄像头,确保在各种光照条件下都能获得清晰图像。
(2)智能分析设备:对摄像头采集的图像进行实时分析,实现人脸识别、车牌识别、行为分析等功能。
3. 传输网络采用光纤、无线等传输方式,确保数据传输的高速、稳定和安全。
4. 中心处理(1)视频存储:采用分布式存储技术,确保视频数据的长期保存和快速检索。
(2)视频分析:利用大数据和人工智能技术,对视频数据进行深度挖掘和分析,实现事件预警和追溯。
(3)中心管理平台:实现对前端设备、传输网络和客户端的统一管理,提高系统运行效率。
5. 客户端客户端分为PC端和移动端,满足用户在不同场景下的监控需求。
四、功能模块1. 实时监控实时显示监控区域内的图像,支持多画面分割、轮巡等功能。
2. 录像回放支持视频数据的快速检索和回放,满足事件追溯的需求。
3. 智能分析(1)人脸识别:实现对人脸的自动抓拍、比对和识别,提高安全防范能力。
(2)车牌识别:自动识别车辆牌照,实现车辆信息的快速查询。
(3)行为分析:对监控区域内的人员行为进行实时分析,发现异常行为立即报警。
4. 预警报警系统可针对各类异常事件进行预警,并通过短信、电话等方式及时通知相关人员。
5. 远程访问支持远程客户端访问,便于用户随时随地了解监控区域的情况。
智能视频监控系统具备的功能
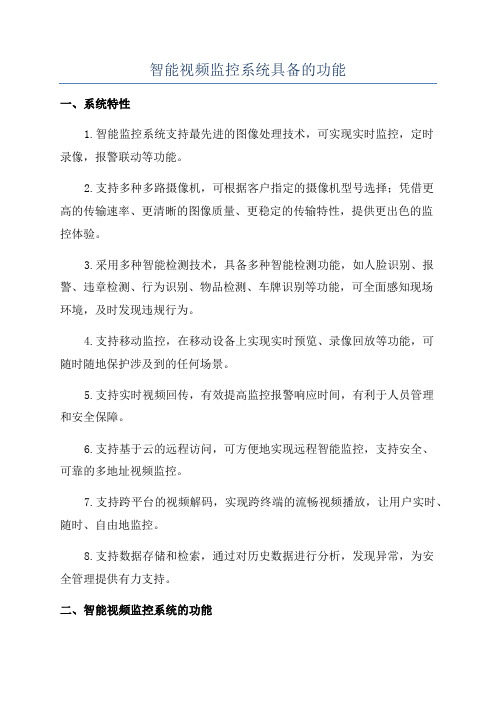
智能视频监控系统具备的功能
一、系统特性
1.智能监控系统支持最先进的图像处理技术,可实现实时监控,定时
录像,报警联动等功能。
2.支持多种多路摄像机,可根据客户指定的摄像机型号选择;凭借更
高的传输速率、更清晰的图像质量、更稳定的传输特性,提供更出色的监
控体验。
3.采用多种智能检测技术,具备多种智能检测功能,如人脸识别、报警、违章检测、行为识别、物品检测、车牌识别等功能,可全面感知现场
环境,及时发现违规行为。
4.支持移动监控,在移动设备上实现实时预览、录像回放等功能,可
随时随地保护涉及到的任何场景。
5.支持实时视频回传,有效提高监控报警响应时间,有利于人员管理
和安全保障。
6.支持基于云的远程访问,可方便地实现远程智能监控,支持安全、
可靠的多地址视频监控。
7.支持跨平台的视频解码,实现跨终端的流畅视频播放,让用户实时、随时、自由地监控。
8.支持数据存储和检索,通过对历史数据进行分析,发现异常,为安
全管理提供有力支持。
二、智能视频监控系统的功能
1.实时视频监控:支持实时监控。
智能视频监控子系统技术方案
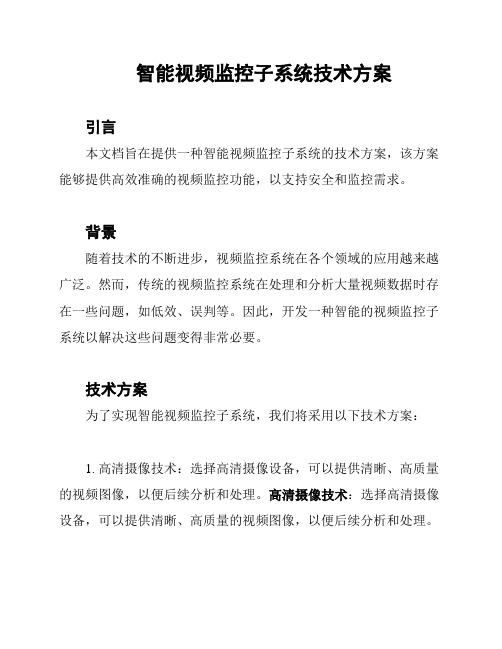
智能视频监控子系统技术方案引言本文档旨在提供一种智能视频监控子系统的技术方案,该方案能够提供高效准确的视频监控功能,以支持安全和监控需求。
背景随着技术的不断进步,视频监控系统在各个领域的应用越来越广泛。
然而,传统的视频监控系统在处理和分析大量视频数据时存在一些问题,如低效、误判等。
因此,开发一种智能的视频监控子系统以解决这些问题变得非常必要。
技术方案为了实现智能视频监控子系统,我们将采用以下技术方案:1. 高清摄像技术:选择高清摄像设备,可以提供清晰、高质量的视频图像,以便后续分析和处理。
高清摄像技术:选择高清摄像设备,可以提供清晰、高质量的视频图像,以便后续分析和处理。
2. 视频数据采集:通过网络将摄像设备采集到的视频数据传输到中央服务器,以便进行后续处理和存储。
视频数据采集:通过网络将摄像设备采集到的视频数据传输到中央服务器,以便进行后续处理和存储。
3. 视频数据存储:将采集到的视频数据存储在中央服务器的硬盘中,并进行备份,确保数据的安全性和可靠性。
视频数据存储:将采集到的视频数据存储在中央服务器的硬盘中,并进行备份,确保数据的安全性和可靠性。
4. 视频分析与智能识别:利用图像处理和机器研究算法对视频数据进行分析和智能识别。
例如,识别人脸、车辆、行为等。
这样可以自动检测异常情况,并快速采取相应的措施。
视频分析与智能识别:利用图像处理和机器学习算法对视频数据进行分析和智能识别。
例如,识别人脸、车辆、行为等。
这样可以自动检测异常情况,并快速采取相应的措施。
5. 报警与联动:当系统检测到异常情况时,自动触发报警,并与其他系统进行联动,如告警通知、视频云存储等。
报警与联动:当系统检测到异常情况时,自动触发报警,并与其他系统进行联动,如告警通知、视频云存储等。
6. 远程监控与管理:通过互联网或移动应用程序,实现远程监控和管理功能。
用户可以随时随地访问视频监控系统,查看实时视频、回放录像等。
远程监控与管理:通过互联网或移动应用程序,实现远程监控和管理功能。
智能视频监控系统的设计与设计的开题报告

智能视频监控系统的设计与设计的开题报告一、选题背景随着社会的发展和技术水平的提升,越来越多的场所需要视频监控系统来保证安全。
传统的监控系统存在着诸如人力成本高、监控盲区、管理不便等问题,而智能视频监控系统能够通过智能分析和控制,实现监控目标的自动识别和追踪,以及异常行为的预警和预防,大大提高安全性和管理效率。
二、选题意义智能视频监控系统是基于图像处理、模式识别、机器学习等领域的技术,是智能安防领域的重要应用。
它能够应用于各类公共场所、工业园区、物流中心等的安全管理,解决传统视频监控系统存在的诸多问题。
同时,该系统还能够与其他系统(如门禁系统)进行集成,构建更加完善的安防体系。
三、研究内容本课题拟设计一种基于深度学习技术的智能视频监控系统,主要包括以下内容:1.视频采集和传输:通过摄像头采集监控画面,并通过网络传输到服务器端。
2.图像处理和特征提取:对视频画面进行图像处理,提取关键特征(如行人、车辆、面部等)。
3.目标跟踪和识别:通过深度学习模型对视频帧进行分析,实现目标跟踪和识别。
4.异常行为检测:在目标跟踪和识别的基础上,通过行为分析实现异常行为的检测和预警。
5.数据可视化和管理:将监控数据以可视化的形式展示,并提供管理界面方便进行数据管理和应急响应。
四、研究方案1. 系统架构设计:系统整体架构采用C/S模式,前端采用Java开发,后端使用Python,数据库使用MySQL。
2. 模型设计:采用深度学习技术进行目标跟踪和识别,具体采用yolov3等深度学习模型。
3. 视频处理:使用OpenCV等开源视觉库进行图像处理、特征提取等相关操作。
4. 异常行为检测:基于目标跟踪和识别的结果,使用行为识别算法,检测并分类异常行为,提供预警功能。
5. 数据可视化和管理:采用Web技术进行页面开发,实现数据可视化和管理功能。
五、预期成果本课题预期完成的主要成果为:1.基于深度学习技术的智能视频监控系统设计与实现。
智能小区系统的智能视频监控方案
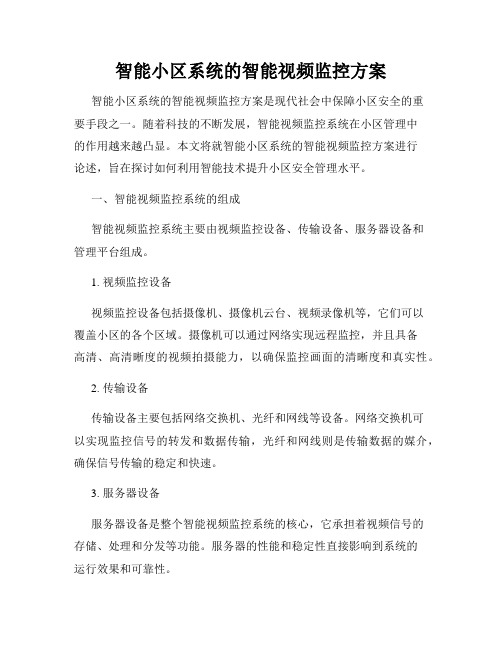
智能小区系统的智能视频监控方案智能小区系统的智能视频监控方案是现代社会中保障小区安全的重要手段之一。
随着科技的不断发展,智能视频监控系统在小区管理中的作用越来越凸显。
本文将就智能小区系统的智能视频监控方案进行论述,旨在探讨如何利用智能技术提升小区安全管理水平。
一、智能视频监控系统的组成智能视频监控系统主要由视频监控设备、传输设备、服务器设备和管理平台组成。
1. 视频监控设备视频监控设备包括摄像机、摄像机云台、视频录像机等,它们可以覆盖小区的各个区域。
摄像机可以通过网络实现远程监控,并且具备高清、高清晰度的视频拍摄能力,以确保监控画面的清晰度和真实性。
2. 传输设备传输设备主要包括网络交换机、光纤和网线等设备。
网络交换机可以实现监控信号的转发和数据传输,光纤和网线则是传输数据的媒介,确保信号传输的稳定和快速。
3. 服务器设备服务器设备是整个智能视频监控系统的核心,它承担着视频信号的存储、处理和分发等功能。
服务器的性能和稳定性直接影响到系统的运行效果和可靠性。
4. 管理平台管理平台是智能视频监控系统的控制中心,它可以实时监控视频画面,对监控范围内的异常情况进行报警和处理,同时也可以对历史视频进行回放和查询等操作。
二、智能视频监控系统的功能和特点智能视频监控系统相比传统的监控系统具有以下几个功能和特点:1. 视频智能分析智能视频监控系统可以通过视频智能分析技术,实现对监控画面的自动识别和分析。
比如可以对异常行为进行识别,如人员聚集、闯入、打斗等,及时报警并采取相应措施。
2. 远程监控智能视频监控系统可以实现远程监控,管理员可以通过手机、电脑等设备实时查看监控画面,随时掌握小区的安全状况。
同时,也可以远程操作云台,进行全方位的监控。
3. 高清画质智能视频监控系统利用高清技术,可以拍摄高质量的监控画面,确保画面的清晰度和真实性。
高清画质可以更好地辨别人脸、车牌等重要信息,提供有效的证据。
4. 多功能管理智能视频监控系统可以与其他小区管理系统进行接口对接,实现多功能管理。
智能监控系统的关键技术与发展趋势
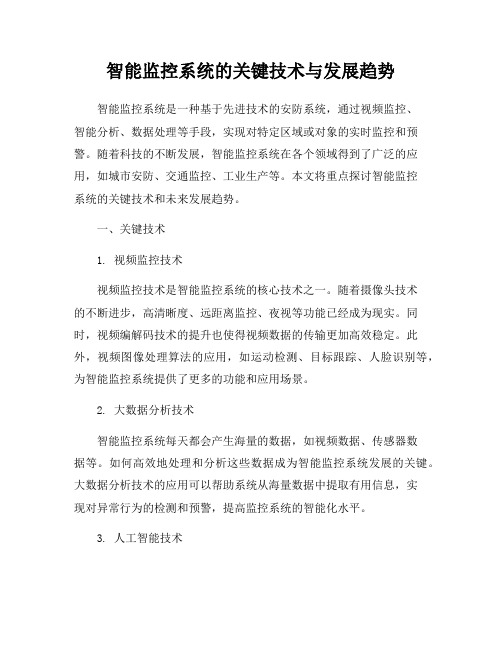
智能监控系统的关键技术与发展趋势智能监控系统是一种基于先进技术的安防系统,通过视频监控、智能分析、数据处理等手段,实现对特定区域或对象的实时监控和预警。
随着科技的不断发展,智能监控系统在各个领域得到了广泛的应用,如城市安防、交通监控、工业生产等。
本文将重点探讨智能监控系统的关键技术和未来发展趋势。
一、关键技术1. 视频监控技术视频监控技术是智能监控系统的核心技术之一。
随着摄像头技术的不断进步,高清晰度、远距离监控、夜视等功能已经成为现实。
同时,视频编解码技术的提升也使得视频数据的传输更加高效稳定。
此外,视频图像处理算法的应用,如运动检测、目标跟踪、人脸识别等,为智能监控系统提供了更多的功能和应用场景。
2. 大数据分析技术智能监控系统每天都会产生海量的数据,如视频数据、传感器数据等。
如何高效地处理和分析这些数据成为智能监控系统发展的关键。
大数据分析技术的应用可以帮助系统从海量数据中提取有用信息,实现对异常行为的检测和预警,提高监控系统的智能化水平。
3. 人工智能技术人工智能技术在智能监控系统中的应用越来越广泛。
深度学习、神经网络等技术的发展,使得监控系统能够更好地理解和分析视频数据,实现更精准的目标识别和行为分析。
同时,人工智能技术还可以实现监控系统的自主学习和优化,提高系统的智能化水平和自适应能力。
4. 云计算和物联网技术云计算和物联网技术的发展为智能监控系统的远程管理和数据存储提供了更多可能。
通过云平台,监控系统可以实现远程监控、数据备份和共享,提高系统的可靠性和稳定性。
同时,物联网技术的应用可以实现监控设备之间的互联互通,实现信息的实时传输和共享,为智能监控系统的发展提供更多可能性。
二、发展趋势1. 智能化和自动化未来智能监控系统的发展趋势是智能化和自动化。
随着人工智能技术的不断发展,监控系统将更加智能化,能够实现更精准的目标识别、行为分析和预警。
同时,系统将更加自动化,实现对异常事件的自动处理和应急响应,减轻人工干预的压力,提高系统的效率和可靠性。
智能视频监控解决方案
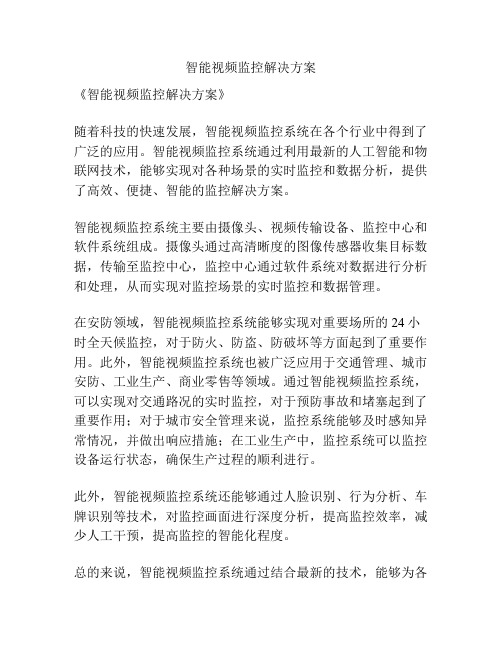
智能视频监控解决方案
《智能视频监控解决方案》
随着科技的快速发展,智能视频监控系统在各个行业中得到了广泛的应用。
智能视频监控系统通过利用最新的人工智能和物联网技术,能够实现对各种场景的实时监控和数据分析,提供了高效、便捷、智能的监控解决方案。
智能视频监控系统主要由摄像头、视频传输设备、监控中心和软件系统组成。
摄像头通过高清晰度的图像传感器收集目标数据,传输至监控中心,监控中心通过软件系统对数据进行分析和处理,从而实现对监控场景的实时监控和数据管理。
在安防领域,智能视频监控系统能够实现对重要场所的24小时全天候监控,对于防火、防盗、防破坏等方面起到了重要作用。
此外,智能视频监控系统也被广泛应用于交通管理、城市安防、工业生产、商业零售等领域。
通过智能视频监控系统,可以实现对交通路况的实时监控,对于预防事故和堵塞起到了重要作用;对于城市安全管理来说,监控系统能够及时感知异常情况,并做出响应措施;在工业生产中,监控系统可以监控设备运行状态,确保生产过程的顺利进行。
此外,智能视频监控系统还能够通过人脸识别、行为分析、车牌识别等技术,对监控画面进行深度分析,提高监控效率,减少人工干预,提高监控的智能化程度。
总的来说,智能视频监控系统通过结合最新的技术,能够为各
个行业提供高效、便捷、智能的监控解决方案,对于提高工作效率、保障安全稳定起到了至关重要的作用。
随着科技的不断进步和发展,相信智能视频监控系统在未来会有更加广阔的应用前景。
智能智慧校园视频监控系统设计
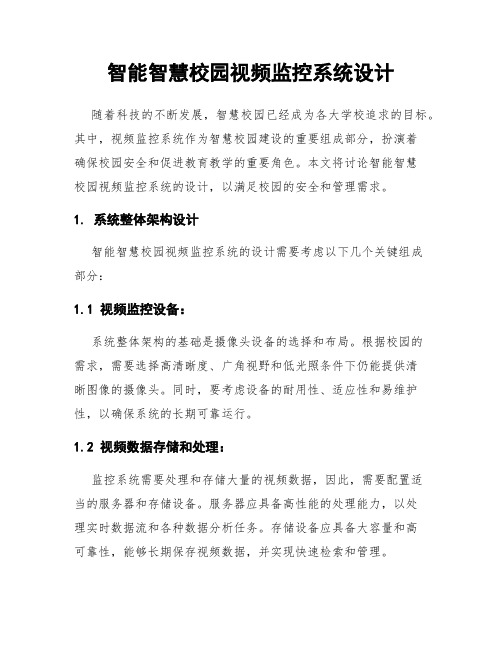
智能智慧校园视频监控系统设计随着科技的不断发展,智慧校园已经成为各大学校追求的目标。
其中,视频监控系统作为智慧校园建设的重要组成部分,扮演着确保校园安全和促进教育教学的重要角色。
本文将讨论智能智慧校园视频监控系统的设计,以满足校园的安全和管理需求。
1. 系统整体架构设计智能智慧校园视频监控系统的设计需要考虑以下几个关键组成部分:1.1 视频监控设备:系统整体架构的基础是摄像头设备的选择和布局。
根据校园的需求,需要选择高清晰度、广角视野和低光照条件下仍能提供清晰图像的摄像头。
同时,要考虑设备的耐用性、适应性和易维护性,以确保系统的长期可靠运行。
1.2 视频数据存储和处理:监控系统需要处理和存储大量的视频数据,因此,需要配置适当的服务器和存储设备。
服务器应具备高性能的处理能力,以处理实时数据流和各种数据分析任务。
存储设备应具备大容量和高可靠性,能够长期保存视频数据,并实现快速检索和管理。
1.3 网络架构和通信设备:为了实现校园各个角落的全方位监控,视频监控系统需要构建高速稳定的网络架构。
除了校园内部的网络设备,还需要考虑与外部安全机构的通信设备,以实现迅速响应和联动。
1.4 软件平台和应用开发:智慧校园视频监控系统应该具备易用的软件平台和友好的用户界面,方便操作人员进行实时监控和管理。
同时,应该考虑开发一些智能分析应用,如异常行为检测、人流量统计和区域入侵检测等,以提高系统的智能化和自动化程度。
2. 安全性和隐私保护设计在设计智能智慧校园视频监控系统时,安全性和隐私保护是重要的考虑因素。
2.1 访问控制:为了保护系统免受未经授权访问和恶意攻击,应该采用严格的访问控制机制。
系统应实施用户身份验证和权限管理,确保只有经过授权的人员可以访问监控数据和系统设置。
2.2 数据加密:监控系统中的数据传输和存储都应该采用加密机制,以防止数据被窃取、篡改或恶意破坏。
采用安全的加密算法,加密传输通道和存储设备,保障数据的机密性和完整性。
智能视频监控技术及其主要应用
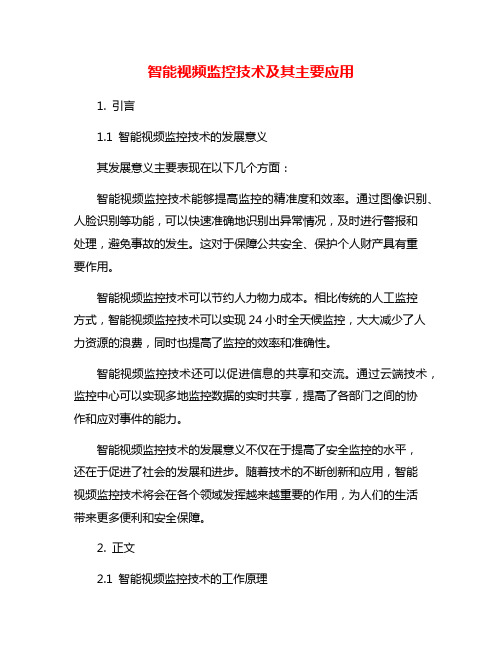
智能视频监控技术及其主要应用1. 引言1.1 智能视频监控技术的发展意义其发展意义主要表现在以下几个方面:智能视频监控技术能够提高监控的精准度和效率。
通过图像识别、人脸识别等功能,可以快速准确地识别出异常情况,及时进行警报和处理,避免事故的发生。
这对于保障公共安全、保护个人财产具有重要作用。
智能视频监控技术可以节约人力物力成本。
相比传统的人工监控方式,智能视频监控技术可以实现24小时全天候监控,大大减少了人力资源的浪费,同时也提高了监控的效率和准确性。
智能视频监控技术还可以促进信息的共享和交流。
通过云端技术,监控中心可以实现多地监控数据的实时共享,提高了各部门之间的协作和应对事件的能力。
智能视频监控技术的发展意义不仅在于提高了安全监控的水平,还在于促进了社会的发展和进步。
随着技术的不断创新和应用,智能视频监控技术将会在各个领域发挥越来越重要的作用,为人们的生活带来更多便利和安全保障。
2. 正文2.1 智能视频监控技术的工作原理智能视频监控技术的工作原理主要是通过摄像头捕捉视频图像,然后利用图像处理和分析算法对视频进行实时监控和识别。
摄像头将实时拍摄到的视频信号传输到计算机或监控设备中,然后经过数字化处理,将视频信号转换为数字图像。
接着,图像处理算法对图像进行分析和检测,提取出关键信息,比如目标物体的位置、移动轨迹、行为特征等。
然后,利用机器学习和人工智能技术,将提取出的信息与预先建立的数据库进行比对和识别,从而实现对目标物体的跟踪和监控。
智能视频监控技术的工作原理可以简单总结为:采集视频图像、处理图像信息、分析识别目标、输出监控结果。
通过不断优化和改进算法,智能视频监控技术能够实现更加精准和高效的监控任务,应用范围也越来越广泛。
随着物联网、大数据、云计算等技术的不断发展,智能视频监控技术将会在各个领域发挥更加重要的作用,提升安全防范水平、提高生产效率、改善城市管理等方面发挥积极作用。
2.2 智能视频监控技术的主要技术特点1. 视频分析能力:智能视频监控技术具有强大的视频分析能力,可以自动识别出视频中的人、车辆、物体等,并根据预先设定的规则进行报警或提醒。
智能视频监控系统项目计划书

智能视频监控系统项目计划书一、项目背景随着社会的发展和科技的进步,安全防范的需求日益增长。
智能视频监控系统作为一种高效、可靠的安全防范手段,正逐渐得到广泛的应用。
无论是在公共场所、商业区域还是工业场所,智能视频监控系统都能够提供实时的监控和有效的预警,保障人员和财产的安全。
二、项目目标本项目的目标是开发一套先进的智能视频监控系统,具备以下功能:1、实时视频监控:能够对监控区域进行 24 小时不间断的视频采集和传输。
2、智能分析:能够对视频内容进行实时分析,包括人员识别、行为分析、异常检测等。
3、报警功能:当检测到异常情况时,能够及时发出报警信号,并将相关信息推送至指定人员。
4、数据存储与检索:能够对监控视频进行长期存储,并支持快速检索和回放。
三、项目团队项目团队由以下人员组成:1、项目经理:负责项目的整体规划、协调和推进。
2、硬件工程师:负责监控设备的选型、安装和调试。
3、软件工程师:负责监控系统软件的开发和维护。
4、算法工程师:负责智能分析算法的研究和实现。
5、测试工程师:负责对系统进行测试和质量保障。
四、项目进度安排本项目预计耗时____个月,具体进度安排如下:1、第 1 2 个月:需求调研和分析与潜在用户进行沟通,了解其对智能视频监控系统的需求和期望。
对市场上现有的智能视频监控系统进行调研,分析其优缺点。
2、第 3 4 个月:系统设计确定系统的整体架构和技术方案。
完成硬件和软件的设计文档。
3、第 5 8 个月:开发与测试硬件工程师进行监控设备的采购和安装调试。
软件工程师和算法工程师进行系统软件和智能分析算法的开发。
测试工程师对系统进行集成测试和性能测试。
4、第 9 10 个月:试点应用选择部分区域进行系统的试点应用,收集用户反馈。
5、第 11 12 个月:优化与推广根据试点应用的反馈,对系统进行优化和完善。
进行系统的推广和市场宣传。
五、项目预算本项目的预算主要包括以下几个方面:1、硬件设备采购费用:包括摄像头、服务器、存储设备等,预计费用为____万元。
智能视频监控系统技术方案

智能视频监控系统技术方案1. 背景介绍随着科技的不断进步,智能视频监控系统在各个领域得到了广泛应用。
该系统利用先进的技术,通过将视频图像与算法相结合,能够自动识别、分析和处理视频信息,从而实现对监控区域的实时监测和智能化管理。
2. 技术方案概述本技术方案旨在设计一套高效、智能的视频监控系统,具备以下主要特点:2.1 视频采集与传输采用高清摄像头对监控区域进行视频采集,并通过网络传输视频图像和数据。
为了保证图像质量和实时性,可以采用高速、稳定的网络传输协议。
2.2 视频存储与管理系统将采集到的视频实时存储,建立视频数据库,并进行合理的管理和分级存储。
通过对视频数据的备份和索引,能够快速检索和回放视频信息。
2.3 视频分析与处理利用人工智能算法,对视频进行智能分析和处理。
系统可以实现目标检测、行为识别、异常报警等功能。
通过对视频内容进行深度研究和模式识别,提高系统的智能化程度。
2.4 远程监控与控制用户可以通过手机应用或网页等方式,实现对监控系统的远程监控和控制。
可以实时查看监控画面、检索历史视频、调整监控参数等。
2.5 数据安全与隐私保护为了保障系统数据的安全性和用户隐私,系统应具备完善的安全机制和数据加密保护措施。
同时,严格管理系统的访问权限,防止非法操作和数据泄露。
3. 实施计划为了有效地实施智能视频监控系统,我们提出以下实施计划:3.1 需求分析和功能设计首先,进行详细的需求调研和分析,明确系统的功能和性能要求。
根据需求分析结果,设计系统的功能模块和交互界面。
3.2 硬件设备配置根据系统需求,选取适当的硬件设备,包括高清摄像头、服务器、存储设备等。
确保硬件设备的兼容性和可靠性。
3.3 软件开发和测试基于需求分析和功能设计,进行软件开发和测试工作。
利用先进的编程语言和开发工具,实现系统的各项功能,并进行充分测试和优化。
3.4 系统部署和调试完成软件开发后,进行系统部署和调试工作。
将系统部署到实际的监控场景中,并进行综合测试和性能评估。
智能视频监控市场分析

智能视频监控市场分析在当今数字化和信息化的时代,智能视频监控已成为保障公共安全、提升企业运营效率以及改善生活质量的重要手段。
随着技术的不断进步和应用场景的日益丰富,智能视频监控市场正呈现出蓬勃发展的态势。
智能视频监控系统的核心在于能够实时获取、分析和处理视频数据,从而为用户提供有价值的信息和预警。
与传统的视频监控相比,智能视频监控具有更高的自动化程度和智能化水平,能够有效减少人工干预,提高监控效率和准确性。
从市场需求方面来看,公共安全领域一直是智能视频监控的主要应用场景之一。
政府部门为了维护社会治安、打击犯罪,对于智能视频监控系统的需求持续增长。
例如,城市中的交通要道、公共场所、重要设施等都需要安装先进的视频监控设备,以实现对异常情况的及时发现和处理。
此外,随着城市化进程的加速,城市规模不断扩大,人口流动日益频繁,这也进一步加大了公共安全管理的难度,从而推动了智能视频监控市场的发展。
在企业领域,智能视频监控同样具有广泛的应用前景。
制造业企业可以通过监控生产线,及时发现生产过程中的问题,提高生产效率和产品质量;物流企业可以监控仓库和运输车辆,保障货物的安全和运输的及时性;金融机构可以监控营业场所,防范金融犯罪等。
这些应用场景都对智能视频监控系统提出了不同的需求,促使市场不断推出更加多样化和定制化的产品和解决方案。
在家庭领域,随着人们对生活安全和便利性的重视程度不断提高,智能视频监控也逐渐走进千家万户。
家庭用户可以通过手机等移动设备随时随地查看家中的情况,实现对老人、儿童和宠物的远程监护,同时还能对家庭安全进行实时预警。
从技术发展的角度来看,智能视频监控系统正朝着高清化、智能化和融合化的方向发展。
高清化意味着视频图像的清晰度越来越高,能够提供更多的细节信息,有助于提高监控的准确性和可靠性。
智能化则体现在系统能够自动分析视频数据,识别出异常行为、目标对象等,大大减轻了人工监控的负担。
融合化是指智能视频监控系统与其他技术,如物联网、大数据、云计算等的深度融合,实现更广泛的数据采集和更强大的数据分析能力。
智能监控助力视频监控系统在政府机构中的应用案例

智能监控助力视频监控系统在政府机构中的应用案例近年来,随着科技的不断发展,智能监控系统逐渐被广泛应用于各个领域。
作为一种高效、安全、可靠的监控工具,它在政府机构中的应用案例尤为引人注目。
本文将通过几个实际案例,介绍智能监控是如何助力政府机构提高工作效率、保障安全的。
案例一:交通管理智能监控系统在政府交通管理部门中的应用,可以帮助监测交通违法行为、改善交通拥堵、提高交通效率。
例如,某市政府引入了智能监控系统来管理交通信号灯。
通过监控设备的智能化分析,系统能够根据实时路况情况,智能调整信号灯的时间,以最优化交通流量和信号配时。
同时,该系统还能检测交通违法行为,如闯红灯、逆行等,并自动将违法行为录像存档,便于后续交通执法。
案例二:安全保卫在政府机构中,安全问题一直是一个重要的关注点。
智能监控系统的应用可以有效提升政府机构的安全保卫能力。
例如,在某市政府办公楼安装了智能监控设备,可以实时监测楼内各个区域的安全情况。
同时,监控系统还具备人脸识别功能,对于未经授权的人员进入办公楼或敏感区域时,系统会自动发出警报,并通过短信或邮件通知相关人员,及时采取必要的安全措施。
案例三:环境监测政府机构对于环境问题的管理和监测也十分重要。
智能监控系统在环境监测领域的应用案例也有不少。
例如,某市政府委托一家科技公司开发了一套智能环境监测系统,用于监测空气质量和噪音污染。
该系统通过多个传感器实时采集环境数据,并进行数据分析和处理,生成环境指标报告。
这些报告可以帮助政府机构及时了解环境变化,采取相应的环境保护措施。
案例四:警务管理智能监控系统在政府警务管理中发挥着重要作用。
某市警察局引入了智能监控系统,用于犯罪预防和犯罪侦查。
通过监控摄像头的分布和智能化监测分析,系统可以实时监测各个街区和公共场所的安全情况,识别可疑人员和可疑行为。
当系统检测到异常情况时,会自动发出警报,并将相关信息推送给警方,并提供录像等证据以供后续侦查和处理。
智能视频监控系统公司简介

智能视频监控系统公司简介嘿,您知道吗?在这个充满变化和挑战的时代,有一家公司正在默默地守护着我们的安全,那就是公司名称智能视频监控系统公司。
这家公司可不是一般的牛!它成立于具体年份,从成立之初就怀揣着一个伟大的梦想,那就是用最先进的技术,为大家打造一个安全无虞的环境。
走进公司名称的研发中心,您会看到一群充满激情和创造力的技术大神们。
他们整天埋头苦干,对着电脑屏幕上那些密密麻麻的代码,就像是在和神秘的数字世界进行一场激烈的较量。
为了让监控系统更加智能、更加精准,他们可谓是绞尽了脑汁。
我曾经亲眼看到过一次他们的头脑风暴会议,那场面,真的是热火朝天!大家你一言我一语,各种新奇的想法不断涌现。
有个小伙子激动地站起来,手舞足蹈地阐述着自己对新算法的设想,眼睛里都闪着兴奋的光芒。
公司名称的智能视频监控系统那可真是一绝!它不仅能够清晰地捕捉到每一个画面,而且还具备强大的分析能力。
比如说,它能在人群中迅速识别出可疑人员,还能及时发现异常的行为和动作。
这就像是给我们的生活安装了一双超级敏锐的眼睛,任何风吹草动都逃不过它的“法眼”。
而且哦,他们的服务也是超级贴心。
不管是安装调试,还是后期的维护升级,都有专业的团队随时待命。
记得有一次,一家商场的监控系统在深夜突然出现了故障。
接到电话后,公司名称的技术人员二话不说,立刻就赶了过去。
在寒冷的冬夜里,他们忙前忙后,终于在最短的时间内解决了问题,让商场的安保工作得以顺利进行。
在产品方面,公司名称不断推陈出新。
从最初的简单监控,到如今的智能化分析,每一次的进步都凝聚着团队的智慧和汗水。
他们的监控设备不仅适用于商场、学校、医院这些公共场所,就连一些小企业和家庭也能轻松用上。
在市场竞争如此激烈的今天,公司名称凭借着过硬的技术和优质的服务,赢得了众多客户的信赖和好评。
和其他同类公司相比,公司名称的优势可太明显啦!他们不仅注重技术的创新,还特别关注用户的体验。
总是能想用户之所想,急用户之所急。
智能监控系统的工作原理
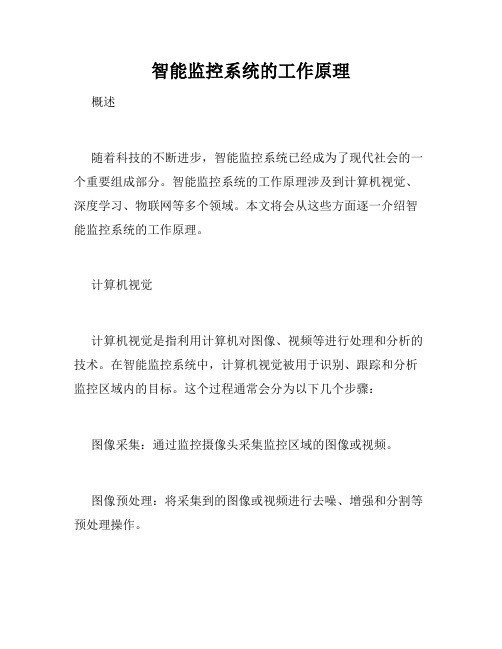
智能监控系统的工作原理概述随着科技的不断进步,智能监控系统已经成为了现代社会的一个重要组成部分。
智能监控系统的工作原理涉及到计算机视觉、深度学习、物联网等多个领域。
本文将会从这些方面逐一介绍智能监控系统的工作原理。
计算机视觉计算机视觉是指利用计算机对图像、视频等进行处理和分析的技术。
在智能监控系统中,计算机视觉被用于识别、跟踪和分析监控区域内的目标。
这个过程通常会分为以下几个步骤:图像采集:通过监控摄像头采集监控区域的图像或视频。
图像预处理:将采集到的图像或视频进行去噪、增强和分割等预处理操作。
目标检测:利用目标检测算法对预处理后的图像或视频进行分析,找到其中的目标物体。
目标跟踪:根据目标检测的结果,对目标物体进行跟踪,实现实时监控。
这些步骤可以帮助智能监控系统快速准确地识别、跟踪和分析监控区域内的目标物体,从而实现自动报警、数据分析等功能。
深度学习深度学习是一种利用多层神经网络对数据进行建模和分析的技术。
在智能监控系统中,深度学习被用于增强目标识别和跟踪的准确性,同时减少误报率。
深度学习通过训练神经网络来实现对监控图像或视频的分类、识别和跟踪,从而提高智能监控系统的性能。
深度学习的训练过程大致可以分为以下几个步骤:数据采集:采集监控区域内的图像和视频数据。
数据标注:对采集到的数据进行标记,例如将其中的目标物体进行框选和命名。
模型设计:设计一个适合于目标识别和跟踪的神经网络模型。
模型训练:利用标记好的数据对神经网络模型进行训练。
模型优化:根据训练结果对神经网络模型进行优化,提高准确率和效率。
这个过程可以帮助智能监控系统从大量的数据中学习到模式和规律,从而实现对监控图像和视频的准确识别和跟踪。
物联网物联网是指利用互联网和传感器等技术将各种设备进行互联的一个概念。
在智能监控系统中,物联网被用于实现监控设备之间的互联和数据传输。
通过利用传感器对监控区域内的环境进行感知,并将感知到的数据通过互联网传输到云服务器上,实现对监控区域内的数据进行实时监控和分析。
- 1、下载文档前请自行甄别文档内容的完整性,平台不提供额外的编辑、内容补充、找答案等附加服务。
- 2、"仅部分预览"的文档,不可在线预览部分如存在完整性等问题,可反馈申请退款(可完整预览的文档不适用该条件!)。
- 3、如文档侵犯您的权益,请联系客服反馈,我们会尽快为您处理(人工客服工作时间:9:00-18:30)。
This article appeared in a journal published by Elsevier.The attached copy is furnished to the author for internal non-commercial research and education use,including for instruction at the authors institutionand sharing with colleagues.Other uses,including reproduction and distribution,or selling or licensing copies,or posting to personal,institutional or third partywebsites are prohibited.In most cases authors are permitted to post their version of thearticle(e.g.in Word or Tex form)to their personal website orinstitutional repository.Authors requiring further informationregarding Elsevier’s archiving and manuscript policies areencouraged to visit:/copyrightVs-star:A visual interpretation system for visual surveillanceKaiqi Huang *,Tieniu TanNational Laboratory of Pattern Recognition,Institute of Automation,Chinese Academy of Sciences,Chinaa r t i c l e i n f o Article history:Received 17September 2009Available online 1June 2010Communicated by A.ShokoufandehKeywords:Visual interpretation SurveillanceEnhanced security Systema b s t r a c tIn recent year,intelligent visual surveillance has become more and more important for enhanced secu-rity.In this paper,we will introduce some recent work in image and video understanding.First,we will give an introduction of the related video surveillance system in recent years,in particular,we will describe algorithms and systems developed in our group for the automatic interpretation of human and vehicle motion in surveillance videos,where automatic interpretation involves object motion detec-tion,object classification and recognition,object tracking and the analysis of object behaviors in order to detect abnormal behaviors.We also give some examples of the real applications of Vs-star system.Ó2010Published by Elsevier B.V.1.IntroductionSecurity has become a major world-wild concern since the event of September 11,2001in USA and the bomb attacks in Lon-don on the July 2005.Video surveillance is a critical component of any effective security system.Most current video surveillance systems are monitored by relatively small teams of human opera-tors.Even though there may be a very large number of cameras.Typically a human watches a set of screens which cycle from one camera to another every few seconds.In addition to problems of fatigue and boredom,the human attention span is limited both spatially and temporally.Studies have shown that the average human can attentively track the movements of up to four indepen-dent targets simultaneously and can detect changes to the targets but not any change to nearby parts of the background (Sears and Pylyshyn,2000).When targets and distractors are too close it be-comes difficult to separate out the targets and maintain tracking.Also,if the targets move too fast,the common people are unable to track them accurately (Cavanaugh and Alvarez,2005).To over-come these limitations the computer vision and pattern recogni-tion field is developing automated systems for the real-time monitoring of humans and vehicles.These systems can interpret the events in the camera and generate an alarm if a suspicious per-son or an abnormal activity is detected.In this paper,we present some of our recent work in image and video understanding using the CASIA visual surveillance system for enhanced security.In particular,we will describe algorithms and systems for the automatic interpretation of human and vehicle motion in surveillance videos,where automatic interpretationinvolves object detection,object classification and recognition,ob-ject tracking and the analysis of object behaviors.2.Related workIn recent years,many automated vision based surveillance sys-tems have been proposed,motivated by commercial,military and industrial applications.Here we give a brief review of these sys-tems.Interested readers can be referred to Aggarwal and Cai (1999)and Collins et al.(2000)for detailed surveys.The VIEWS system (Corrall,1991)is one of the earliest video surveillance systems for describing scene activity.It is designed to operate in controlled environments where a great deal of infor-mation can be specified in advance.This information includes cam-era models,ground plane representation,salient regions of the environment,3-D object models and behavior models.The VIEWS system requires significant computational power to achieve real-time performance.An ambitious system,VSAM (Video Surveillance and Monitor-ing)(Collins et al.,2000)provided the capability to detect,track,localize and visualize objects within a known environment sur-veyed by a distributed network of cameras.Moving objects are classified as human,human group or vehicle.The motions of indi-vidual humans are classified as running or walking.Among the earlier automated monitoring systems,Pfinder (Wren,1997)is perhaps the most well known.The full body of per-son is tacked in a scene that contains only one unoccluded person in an upright posture.It uses a unimodal background model to locate the moving person.For military applications,Boult et al.(2001)present a system for monitoring uncooperative and camouflaged targets.They focus on low level analysis such as object detection and tracking.0167-8655/$-see front matter Ó2010Published by Elsevier B.V.doi:10.1016/j.patrec.2010.05.029*Corresponding author.Tel.:+861062653768;fax:+861062551993.E-mail address:kqhuang@ (K.Huang).The W4system(Haritaoglu et al.,2000)uses dynamic appear-ance models to track people.Single person and group are distin-guished using projection histograms.The heads of the people in a group are tracked individually.Fujiyoshi et al.(2001))developed a system to detect and track multiple people and vehicles in a cluttered scene and monitor activities over a large area and extended periods of time.Their sys-tem could also classify objects as a person,group of peoples,vehi-cles,and so on,using shape and color information.Recently,Shah et al.(2007)propose an automatic surveillance system named Knight.The Knight system cannot only detect,track and classify objects in a realistic scene surveyed by a camera net-work,but also canflag significant events and present a summary in terms of key frames and a textual description of observed activ-ities.In contrast with VSAM,Knight is being developed for com-mercial application.It is deserved to be mentioned that some work is not introduced by system while they play important role in the automated surveil-lance system.Stauffer and Grimson(2000)used an adaptive mul-timodal background subtraction method named Adaptive Mixture Gaussian Model(GMM)for object detection that can deal with slow changes in illumination,repeated motion from back-ground clutter,and long-term scene changes.Their background subtraction method performed well in realistic scenes and was adopted by many systems.They also proposed the detection of unusual activities by statistically learning the common patterns of activities over time.They tracked detected objects using a multi-ple hypothesis tracker.Ricquebourg and Bouthemy(2000)pro-posed people tracking algorithm by exploiting spatiotemporal slices.Their detection scheme involves the combination of inten-sity,temporal differences between three successive images,and a comparison between the current image and a background refer-ence image,which is reconstructed and updated online.There are many other systems with specific features adapted to different requirements.These systems include the RoboGuard sys-tem(Birk and Kenn,2001),which focuses on visual interpretation for surveillance,bile platform,the IBM Face Cataloger system (PeopleVision:IBM research,xxxx),which records the person tracked by a tracker and identified the person using a frontal view of face obtained by a pan-tile-zoom(PTZ)camera.In this paper,we will also introduce one automatic visual sur-veillance system for visual interpretation of human and vehicle motion named Vs-star system,which is for the purpose of research and some components have been used for real applications.3.Vs-star:Casia visual surveillance system and algorithmsWe have developed a visual interpretation system for visual surveillance named visual surveillance star(Vs-star).The purpose of Vs-star is to describe the motions of humans and vehicles in a surveillance scene and give a warning if these motions indicate a breach of security.Fig.1shows the framework of -pared with other systems for commercial applications,our frame-work focuses on core topics for research,also some real applications of techniques will be introduced at the end of this pa-per.Vs-star integrates low level object detection and tracking,high level activity analysis and semantic interpretation.In the next sec-tion,we will introduce the components of Vs-star shown in Fig.1.3.1.Moving object detectionMoving object detection is a critical early step in video surveil-lance.The moving objects provide a focus of attention for later pro-cesses such as tracking and object classification.One convenient and effective way for moving object detection is background subtraction.This method constructs a reference background mod-el,and then,takes difference between the current image and back-ground model to detect moving object.Therefore,an accurate and robust background model is prerequisite.Much work has been done on thisfield(Wren,1997;Stauffer and Grimson,2000;Huang,2006;Karmann and Von Brandt, 1990;Koller et al.,1994;Elgammal et al.,2002;Radke et al., 2005).Gaussian Mixture Model(GMM)(Stauffer and Grimson, 2000)is one of the widely used method for moving object detec-tion.It models the time series of color observations at a given pixel as a mixture of Gaussians,and a subset of the Gaussians is used to describe the scene background.The parameters for each Gaussian are updated as new observations are obtained,so it can adapt to gradual background changes.In(Elgammal et al.,2002),the authors use the Kernel Density Estimation(KDE)method to repre-sent the color distribution for each pixel.Some other methods are proposed based on these two methods(Wang et al.,2006;Sheikh and Shah,2005).Wang et al.(2006)propose a dynamic conditional randomfield model for object and moving cast shadow segmenta-tion.Sheikh and Shah(2005)directly modeled the dependencies between the domain(location)and range(color)by using a non-parametric density estimation method over a joint domain-range representation of image pixels.Due to the self learning capacity to variations in lighting,peri-odic dynamic scene,GMM as the background model is considered in many surveillance systems and also in our framework.However, it still has some shortcomings.The number of Gaussians should be decided beforehand.Another limitation is that it does not model the spatial dependencies of neighboring background pixel colors explicitly.Therefore,some false positive pixels will be produced in dynamic scenes where dynamic texture does not repeat exactly. In Vs-star system,we propose an on-line auto-regressive model to capture and predict the behavior of dynamic scenes as in Fig.2 (Zhou et al.,2008).The proposed method has three novel contributions:(1)A probabilistic framework for moving object segmentationin dynamic scene is proposed.Under this framework,we unify background modeling,moving object detection and shadow removing by constructing probability density func-tions(PDFs)of background,shadow and foreground based on an extended GMM.The method in(Rittscher et al., 2000)also considers background,foreground and shadow based on Hidden Markov Model(HMM).However,parame-ters of this model are learned off-line and this method fails to distinguish shadow from dark vehicles,which results in poor parameter estimation.(2)Extended GMM which model spatial dependencies of neigh-boring pixel colors explicitly is considered in our system.It is inspired by the work of Sheikh and Shah(2005).Moreover, the extended GMM can determine the number of Gaussian components dynamically by Gaussian merging and deleting rules.(3)We also consider feedback of tracking results to accuratePDF of foreground.The authors(Sheikh and Shah,2005)also construct PDF of foreground,but tracking information is neglected.The images as Fig.3show the results with Extended GMM on the scene with wind,which caused the clutter background.On this scene,shadow is also distinctive.Ground truth is given manually.It is evident that swaying tree deteriorates the performance of tradi-tional GMM as shown in the forth and last rows of Fig.3.While with our method,the contour of shadow has been removed and that majority of hollows arefilled by our method.The second and the third row show indoor scene,where the nominally moving2266K.Huang,T.Tan/Pattern Recognition Letters31(2010)2265–2285camera was simulated by moving the original pictures left and right(motion is about4pixels).The shadow here is insignificant. It shows that a slight movement will also cause substantial degra-dation in performance of GMM,especially on the neighbor of edge. Fig.4shows the per-frame moving object detection rates according to precision and recall for the video of Fig.3.Precision and recall are defined as:Precision¼#of true positive detected total#of true positive detected;Recall¼#of true positive detectedtotal#of true positives:The results in Fig.4are shown for three different learning rates oftraditional GMM.The shadow removing method is Cucchiara et al.(2001).Thesefigures demonstrate that our method can deal withdynamic scene and cast shadow effectively considering spatialinformation and PDF of shadow.3.2.Object trackingObject tracking,as the following step of moving object detec-tion,has always been a hot topic in thefield of surveillance.Theaim of object tracking is to locate the moving objects in the wholevideo sequences so that the complete trajectory of an object can be obtained.There are mainly two major steps of object tracking:tar-get representation and data association(Gilbert and Bowden, 2006).Although substantial work has been done on object tracking, there are still many problems deserved further investigation. Firstly,occlusion due to self-occlusion or occlusion between differ-ent objects brings uncertainties into the data association.Secondly, tracking objects can be difficult due to loss of information caused by projection of the3D world onto a2D image plane.Thirdly,as the need of wide area visual surveillance,the use of multiple cam-eras also brings challenges into object tracking.Next,we will give a brief introduction to the tracking methods in our group.According to the number of cameras in surveillance scenarios, we divide object tracking problem into two subset problems:in-tra-camera tracking(single camera tracking)and inter-camera tracking(multi-camera tracking).3.2.1.Intra-camera trackingIn intra-camera tracking,the inputs to the tracking module are foreground regions corresponding to the moving objects.Back-ground subtraction algorithm(Cucchiara et al.,2001)is applied to extract the foreground regions.According to the representation of moving objects,tracking methods can be divided into four major categories:region-based tracking,active-contour-based tracking, feature-based tracking,and model-based tracking.It should bedetection in dynamic scene.The top row are original images,the second row are background images,the third row are results results obtained by GMM and the shadow removing method in(Aggarwal and Cai,1999),thefifth row shows results obtained last row are the results of our method.pointed out that this classification is not absolute and the algo-rithms from different categories can be integrated together.Here, feature is low level information such as color and so on,region and active contour are middle level and model can be high level for tracking,active contour can be looked as one feature and we can also get features from region,so we introduced the tracking algorithms from feature-based and model-based methods.3.2.1.1.Feature-based tracking.Feature-based tracking algorithms perform recognition and tracking of objects by extracting ele-ments,clustering them into higher level features and then match-ing the features between images.In our system,we employ‘‘color”as the feature for tracking which is a global feature.To deal with occlusion,we introduce a Bayesian network which involves human 2D ellipse models and an extra hidden process for occlusion rela-tion.In contrast to previous work,the appearance of the target is modeled as a statistical color spatial model other than an appear-ance template.This color spatial model captures the multi-modal patterns of color of the target.The human models are initialized when people arefirstly detected as targets and then updated at each new frame before occlusion.The observation likelihood is then proposed based on the Bhattacharyya distance to people tracking through occlusions.The tracking process is performed using a Condensation algorithm.Fig.5illustrates key frames where two people are tracked through occlusions.More results can be found in Min et al.(2004),Lou et al.(2002).In general,as these algorithms operate on2-D image planes, feature-based tracking algorithms can work in real-time and track multiple objects which are required in heavy thruway scenes,etc. However,there are several serious deficiencies in feature-based tracking algorithms:The recognition rate of objects based on2-D image features is low,because of the nonlinear distortion during perspective pro-jection and the image variations with the viewpoint’s movement.These algorithms are generally unable to recover3-D pose of objects.The stability of dealing effectively with occlusion,overlapping and interference of unrelated structures is generally poor.Model-based tracking in next part provides an effective solution to above problems.3.2.1.2.Model-based tracking.Model-based tracking algorithms track objects by matching projected3D object models,which are produced with prior knowledge.The models are usually constructed off-line with manual measurement,such as Computer Aided Design(CAD)tools or computer vision techniques.According to difference of human(non-rigid object)and vehicle(rigid object),we model and track these two types of objects separately (Huazhong et al.,2004;Huazhong et al.,2004).For human,we construct human body models by articulated truncated cones and a sphere as a trade-off between accuracy and complexity as Fig.6.For simplicity and efficiency,the human body model is rep-resented by a12-dimensional state vector composed of the global position of the human body and relative positions of different body parts.Fig.7shows tracking results in complex real-world outdoor scenes.For vehicle model,3D wire-frame vehicle models are mainly adapted(Tan and Baker,2000;Tan et al.,1998)and an improved extended Kalmanfilter is used for tracking(Jianguang et al.,2005),which outperforms the traditional extended Kalman filter when the vehicle carries out a complicated maneuver.The 3D model-based tracking algorithms can be applied even when objects greatly change their orientations during the motion.3.2.2.Inter-camera trackingWith the increasing number of the cameras(overlap or non-overlap)in surveillance,multi-camera tracking becomes more and more important.Main issues of multi-camera tracking include camera installation,calibration of multiple cameras,correspon-dence between multiple cameras,automated camera switching and data fusion,etc.We focus on correspondence between multi-ple overlapped and non-overlapped cameras.Firstly,multiple cam-eras are used for improving the tracking of people when people are within the common ground plane of the multiple views,such as in Fig.9.Secondly,people are tracked continuously from one camera to another.Cameras are widely separated in this case.Experimen-tal setup is shown in Fig.12.3.2.2.1.Principal axis-based correspondence between multiple cam-eras for people tracking.For overlapped cameras,the principle axis of each person is proposed to match people across multiple views (Weiming et al.,2006).Estimation of principle axes does not rely on accurate motion detection since the influence of the imperfec-tion of motion detection is counteracted by the symmetrical distri-butions of the error along the principle axis,which makes principle axes an appealing attribute in real applications.The principle axis of an isolated person is determined by minimizing the median of squared perpendicular distance between the foreground pixels and a vertical axis in Fig.8.The detection of principle axes of peo-ple in a group and under occlusion can be carried out similarly.The correspondence likelihood reflecting the similarity of pairs of prin-cipal axes of people is constructed according to the relationshipdetection rates for our method and GMM with shadow removing method in(Cucchiara et al.(2001)).The learning rates for GMMbetween‘‘ground-points”of people,which is detected in each cam-era view,and the intersections of principal axes,which is detected in different camera views and transformed to the same view as illustrated in Fig.9.The experimental results on several real video sequences from outdoor environments in NLPR database(CASIA gait database,xxxx)and PETS database(PETs database,xxxx)have demonstrated the effectiveness,efficiency,and robustness as in Figs.10and11respectively.3.2.2.2.Continuously tracking objects across multiple widely separated cameras.Another classic multi-camera tracking is handover(Cai et al.,2007;Gilbert and Bowden,2006).That is to say,we need give a single ID to an object when object travels from one camera to another.and Okada,2006)to compensate for illumination changes and camera distortions.The experiments consist of three cameras with non-overlapping fields of view.The cameras are widely separated,including two outdoor settings and one indoor setting.The layout is shown in Fig.12a.Some of the experimental results are shown in Fig.13. More details can be found in(Cai et al.,2007).3.3.Object classification and recognition3.3.1.Real-time object classificationMoving object classification is a popular topic in intelligent vi-sual surveillance.With object type information known,more spe-cific and accurate methods can be developed to recognize highlevel actions of video objects.Especially in traffic scene videos, classification of moving objects into predefined categories allows the operator to program the monitoring system by specifying events of interest,such as alarming when a pedestrian is coming into a forbidden area or alarming when a vehicle is running in a re-verse direction.Much work has been done in object classification.In(Brown, 2004;Quming and Aggarwal,2001),foreground objects are de-tected using motion information and some image features,like area,compactness,speed and bounding box aspect ratio are ex-tracted for training and classification.However,most of these fea-tures are based on2D space,which are often degraded with projective distortion especially in far-field traffic scene videos.these collected videos.As we only focus on moving objects in traffic scene surveillance,motion information is employed here to detect targets of interest in videos.With regions of interest detected,every detected foreground region isfixed to a square according to its mass center,which is then normalized to the same size20Â20.These foreground targets are then labeled manually.In this way,we col-lect the whole data set including58,958vehicles,56,942pedestri-ans and3297other individuals as outliers.Samples of the database are illustrated in Fig.42.Experiments are conducted with different number of grids and the accuracy is shown in Fig.15.As we can see, the accuracy increases with smaller size of sub-regions,which dem-onstrates the advantage of the sub-region strategy.Gaussian assumption is adopted as prior information.Experiments are con-ducted to illustrate its effectiveness for classification.ExperimentalFig.9.Geometrical relationship between principle axes.results with Gaussian assumption and that with simply clustering are shown in Tables1and2.It is evident that the approach using Gaussian assumption is much better,which demonstrate the effec-tiveness of Gaussian Assumption.3.3.2.Gait based human recognitionObject classification just gives the coarse result of the object type such as pedestrian and vehicle,while some time,object recog-nition is urgently needed especial for human identification.Gait is an attractive biometric feature for human identification at a dis-tance,and recently has gained much interest from computer vision researchers.Gait is a particular way or manner of moving on foot (Shakhnarovich et al.,2001).Compared with those traditional bio-metric features,such as face,iris,palm print andfinger print,gait has many unique advantages such as non-contact,non-invasive and perceivable at a distance.Many gait recognition algorithms have been developed in re-cent years(Human ID Challenge Problem at USF,xxxx;Sudeep et al.,2005).Some of them are model-based approaches(Wang et al.,2004;Wang et al.,2003),some are appearance-based ones (Yu et al.,2004;Yu et al.,2007;Wang et al.,2003).Model-based approaches aim to explicitly model the human body or motion, and they usually perform model matching in each frame of a walk-ing sequence in order that the parameters such as trajectories,limb lengths and angular speeds are measured on the model.The major-ity of current approaches are appearance-based.There are many gait databases for this research such as(Shutler et al.,2002;Auto-matic Gait Recognition for Human ID at a Distance at Soton,xxxx; Center for Biometrics and Chinese Academy of Science Security Re-search,xxxx),while no enough variations have been considered in these databases.We have created a large gait database,the CASIA Gait Database (CASIA gait database,xxxx)including three datasets:Dataset A (small set,shown in Fig.16),Dataset B(multi-view set shown in Fig.17)and Dataset C(infrared set shown in Fig.18).Based on these databases,we make deep research into gait recognition (Wang et al.,2004;Wang et al.,2003;Yu et al.,2004;Yu et al., 2007;Wang et al.,2003;Yu et al.,2009),gait recognition based on Procrustes shape analysis is model-free(Wang et al.,2004), which achieve good results in CASIA Database(Dataset A).Consid-ering the body appearance and the dynamics of human walking motion information of gait(Wang et al.,2003),a silhouette analy-sis based gait recognition algorithm using Principal Component Analysis(PCA)is proposed(Yu et al.,2004).The algorithm implic-itly captures the structural and transitional characteristics of gait, especially the shape cues of body biometrics.Although it is very simple in essence,the experimental results are surprisingly prom-ising in CASIA Database(Dataset A).Due to the difficulties of auto-matic parameter recovery from video,few methods except (Cunado et al.,2003;D’ıaz de Le’on and Sucar,2000)used Prior information,we propose a gait recognition method with combina-tion of model-based and appearance-based approaches(Wang et al.,2003).It not only analyzes spatiotemporal motion pattern of gait dynamics but also derives a compact statistical appearance description of gait as a continuum.3.4.Activity recognition and abnormity analysisIn visual surveillance for security,motion detection,tracking and suspicious object classification and recognition can make sense in some degree,while understanding human physical behav-ior for surveillance is more significant,which is also driven by a wide spectrum of other applications in various areas such asinteractive virtual reality systems,advanced and perceptual human–computer interfaces(HCI),content-based video storage and retrieval sports,and so forth(Haritaoglu et al.,1998).Here, according to the view of surveillance,we focus on shape based near-field activity analysis and trajectory based far-field activity analysis.3.4.1.Trajectory based activity analysisTrajectory that records the object’s position from entering to exiting a scene is one of the most useful information for behavior of moving objects.Much work on trajectory analysis for visual sur-veillance focused on learning several statistical motion routes most commonly taken by motion objects in a scene.Johnson and Hogg (1996)learn probability density functions of object trajectories generated from image sequences.The movement of an object is de-scribed in terms of a sequence offlow vectors,where each vector consists of four elements representing the position and velocity of the object in the image plane.The patterns of object trajectories are formed with two competitive learning networks which are con-nected by leaky neurons.Stauffer and Grimson(2000)learn motionFig.12.(a)The layout of the camera system and(b)three views from three widely separated cameras. correspondence of a group of people through occlusion:From the top to bottom,the frame numbers are878,。