Learning and Vision Mobile Robotics Group Research Report
人工智能英文怎么说

人工智能英文怎么说Artificial Intelligence, commonly referred to as AI, is a rapidly growing field that encompasses the development and implementation of intelligent machines and computer systems. It has become a transformative technology, revolutionizing various industries and sectors worldwide. In this article, we will explore the different terminologies and expressions used to refer to artificial intelligence in the English language.1. Artificial Intelligence (AI)Artificial Intelligence is the most widely used term to describe the field. It refers to the simulation of human intelligence in machines that are programmed to perform tasks with human-like abilities. AI encompasses a wide range of technologies, including machine learning, natural language processing, computer vision, and robotics.2. Machine IntelligenceMachine Intelligence is another commonly used term for artificial intelligence. It emphasizes the ability of machines to exhibit intelligent behavior and make decisions based on data and algorithms. Machine Intelligence includes both rule-based systems and systems that learn from experience.3. Cognitive ComputingCognitive Computing refers to the development of computer systems that can mimic human thought processes, such as problem-solving, decision-making, and language understanding. It involves using AI techniques toenable machines to understand and interact with humans in a more natural and intuitive way.4. Deep LearningDeep Learning is a subset of machine learning that focuses on training artificial neural networks with multiple layers to recognize patterns and make predictions. Deep Learning algorithms have been highly successful in various tasks, including image and speech recognition, natural language processing, and autonomous driving.5. Neural NetworksNeural Networks are computational models inspired by the structure and function of the human brain. These networks consist of interconnected nodes, called neurons, that can process and transmit information. Neural networks have been instrumental in advancing AI capabilities, allowing machines to learn and make decisions based on vast amounts of data.6. Expert SystemsExpert Systems utilize AI techniques to replicate human expertise in specific domains. These systems are designed to mimic the knowledge and decision-making processes of human experts, providing intelligent solutions and recommendations in areas such as medicine, finance, and engineering.7. Robotic IntelligenceRobotic Intelligence refers to the integration of AI technologies into robotic systems, enabling them to perceive and interact with their environment. Robotic Intelligence combines computer vision, machinelearning, and sensor technology to create robots capable of performing complex tasks autonomously.8. Natural Language Processing (NLP)Natural Language Processing is a branch of AI that focuses on the interaction between computers and human language. It involves the development of algorithms and models that enable machines to understand, interpret, and generate human language in both written and spoken forms. NLP is used in applications such as virtual assistants, machine translation, and sentiment analysis.9. Computer VisionComputer Vision is the field of AI that focuses on enabling machines to interpret and understand visual information from images or videos. It involves algorithms and techniques for image recognition, object detection, facial recognition, and scene understanding. Computer Vision has found applications in surveillance, autonomous vehicles, and medical imaging, among others.10. Smart SystemsSmart Systems refer to AI-enabled systems that can perceive, learn, and adapt to their environment. These systems utilize sensors, data analytics, and AI algorithms to provide intelligent services and functionality. Smart Systems are used in various contexts, including smart homes, smart cities, and Internet of Things (IoT) applications.In conclusion, artificial intelligence, or AI, encompasses a wide range of terminologies and expressions in the English language. From deep learningand neural networks to cognitive computing and robotic intelligence, these terms reflect the diverse aspects and applications of AI technology in today's world. As AI continues to advance, new expressions and terminologies are likely to emerge, shaping the future of intelligent machines and systems.。
如何成为人工智能工程师英语作文
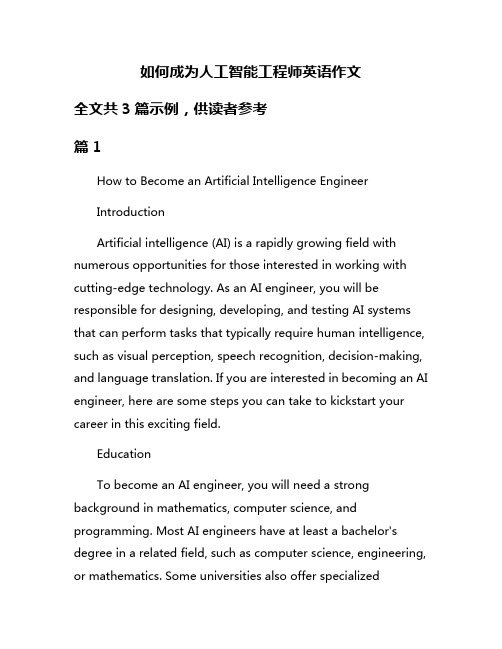
如何成为人工智能工程师英语作文全文共3篇示例,供读者参考篇1How to Become an Artificial Intelligence EngineerIntroductionArtificial intelligence (AI) is a rapidly growing field with numerous opportunities for those interested in working with cutting-edge technology. As an AI engineer, you will be responsible for designing, developing, and testing AI systems that can perform tasks that typically require human intelligence, such as visual perception, speech recognition, decision-making, and language translation. If you are interested in becoming an AI engineer, here are some steps you can take to kickstart your career in this exciting field.EducationTo become an AI engineer, you will need a strong background in mathematics, computer science, and programming. Most AI engineers have at least a bachelor's degree in a related field, such as computer science, engineering, or mathematics. Some universities also offer specializedprograms in artificial intelligence or machine learning, which can provide you with the specific knowledge and skills you need to succeed in this field.In addition to formal education, it is important to continue learning and staying up-to-date with the latest developments in AI technology. There are many online courses and tutorials available that can help you expand your knowledge and skillset in areas such as machine learning, deep learning, natural language processing, and computer vision.ExperienceHands-on experience is also crucial for becoming an AI engineer. Look for opportunities to work on AI projects, either independently or as part of a team. You can also participate in hackathons, competitions, and open-source projects to showcase your skills and build a portfolio of work that demonstrates your expertise in artificial intelligence.NetworkingNetworking is another important aspect of becoming an AI engineer. Attend conferences, meetups, and workshops in the field of artificial intelligence to connect with other professionals and learn from their experiences. Building a strong network canhelp you stay informed about job opportunities, industry trends, and new technologies in AI.SpecializeArtificial intelligence is a broad field with many specialized areas, such as machine learning, deep learning, natural language processing, computer vision, and robotics. Consider focusing on a specific area of AI that interests you and developing expertise in that area. Specialization can help you stand out as a candidate and attract job opportunities in your chosen field.Stay CuriousFinally, it is important to stay curious and keep exploring new ideas and technologies in the field of artificial intelligence. The field of AI is constantly evolving, with new breakthroughs and innovations happening all the time. By staying curious and open-minded, you can continue to grow and develop as an AI engineer and contribute to the advancement of this exciting technology.ConclusionBecoming an AI engineer requires a combination of education, experience, networking, specialization, and curiosity. By following these steps and putting in the effort to build yourskills and knowledge, you can pursue a rewarding career in artificial intelligence and make a meaningful impact in this rapidly growing field.篇2How to Become an Artificial Intelligence EngineerWith the rapid advancement of technology, artificial intelligence has become one of the most sought-after fields in the job market. As an artificial intelligence engineer, you will work on developing algorithms, machine learning models, and other technologies that enable computers to perform tasks that typically require human intelligence. In this article, we will discuss the steps you need to take to become a successful artificial intelligence engineer.1. Obtain a solid foundation in computer science: To become an artificial intelligence engineer, you need to have a strong background in computer science. This includes knowledge of programming languages such as Python, Java, C++, and others, as well as a good understanding of data structures, algorithms, and computer architecture.2. Learn machine learning and deep learning: Machine learning and deep learning are essential skills for artificialintelligence engineers. You should study machine learning algorithms, such as linear regression, logistic regression, support vector machines, and neural networks, as well as deep learning frameworks like TensorFlow and PyTorch.3. Gain experience with data: Data is the fuel that powers artificial intelligence systems. As an artificial intelligence engineer, you will need to work with large datasets to train your machine learning models. This requires knowledge of data processing techniques, data visualization, and statistical analysis.4. Specialize in a specific area of artificial intelligence: Artificial intelligence is a broad field that encompasses many different subfields. Some of the most popular areas of specialization include natural language processing, computer vision, robotics, and reinforcement learning. Choose a specialization that interests you and focus on developing your skills in that area.5. Earn a degree in computer science or a related field: Whilea degree is not always necessary to become an artificial intelligence engineer, having a degree can make it easier to land a job in the field. Consider pursuing a bachelor's or master's degree in computer science, mathematics, statistics, or a related field.6. Build a portfolio of projects: To demonstrate your skills as an artificial intelligence engineer, you should work on a variety of projects that showcase your abilities. This could include building a recommendation system, developing a chatbot, or creating a computer vision application. Make sure to document your projects and share them on platforms like GitHub.7. Stay up-to-date with the latest developments in artificial intelligence: The field of artificial intelligence is constantly evolving, with new technologies and breakthroughs emerging all the time. To stay competitive as an artificial intelligence engineer, you should stay informed about the latest research papers, conferences, and trends in the field.Becoming an artificial intelligence engineer requires dedication, hard work, and a passion for technology. By following these steps and continually honing your skills, you can build a successful career in this exciting and fast-growing field. Good luck on your journey to becoming an artificial intelligence engineer!篇3How to Become an Artificial Intelligence EngineerWith the rapid development of technology, artificial intelligence has become one of the hottest fields in recent years. As a result, the demand for skilled artificial intelligence engineers is on the rise. If you are interested in pursuing a career in this field, here is a guide on how to become an artificial intelligence engineer.1. Get a solid education:To become an artificial intelligence engineer, you will need to have a strong educational background in computer science, mathematics, and other related fields. A bachelor's degree in computer science is typically required, with courses in algorithms, data structures, machine learning, and artificial intelligence.2. Gain practical experience:In addition to formal education, practical experience is essential to become a successful artificial intelligence engineer. You can participate in internships, research projects, oropen-source contributions to gain hands-on experience in the field.3. Specialize in artificial intelligence:Once you have a solid educational background and practical experience, it is important to specialize in artificial intelligence.You can pursue a master's degree or a Ph.D. in artificial intelligence or a related field to deepen your knowledge and expertise in the field.4. Develop technical skills:To excel as an artificial intelligence engineer, you will need to have strong technical skills in programming languages such as Python, Java, or C++. You will also need to have a solid understanding of machine learning algorithms, neural networks, and data analytics.5. Stay updated on industry trends:The field of artificial intelligence is constantly evolving, with new technologies and techniques being developed all the time. To stay ahead of the curve, it is important to stay updated on industry trends and advancements in the field.6. Build a strong network:Networking is essential in any field, including artificial intelligence. By attending conferences, meetups, and workshops, you can connect with other professionals in the field, learn from their experiences, and stay updated on job opportunities.7. Pursue certifications:To demonstrate your expertise in artificial intelligence, you can pursue certifications such as the Google TensorFlow Developer Certificate or the IBM Data Science Professional Certificate. These certifications can help boost your resume and showcase your skills to potential employers.In conclusion, becoming an artificial intelligence engineer requires a combination of education, practical experience, technical skills, and networking. By following the steps outlined above, you can position yourself for a successful career in this exciting field.。
斯坦福大学人工智能所有课程介绍
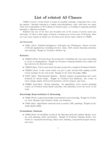
List of related AI Classes CS229covered a broad swath of topics in machine learning,compressed into a sin-gle quarter.Machine learning is a hugely inter-disciplinary topic,and there are many other sub-communities of AI working on related topics,or working on applying machine learning to different problems.Stanford has one of the best and broadest sets of AI courses of pretty much any university.It offers a wide range of classes,covering most of the scope of AI issues.Here are some some classes in which you can learn more about topics related to CS229:AI Overview•CS221(Aut):Artificial Intelligence:Principles and Techniques.Broad overview of AI and applications,including robotics,vision,NLP,search,Bayesian networks, and learning.Taught by Professor Andrew Ng.Robotics•CS223A(Win):Robotics from the perspective of building the robot and controlling it;focus on manipulation.Taught by Professor Oussama Khatib(who builds the big robots in the Robotics Lab).•CS225A(Spr):A lab course from the same perspective,taught by Professor Khatib.•CS225B(Aut):A lab course where you get to play around with making mobile robots navigate in the real world.Taught by Dr.Kurt Konolige(SRI).•CS277(Spr):Experimental Haptics.Teaches haptics programming and touch feedback in virtual reality.Taught by Professor Ken Salisbury,who works on robot design,haptic devices/teleoperation,robotic surgery,and more.•CS326A(Latombe):Motion planning.An algorithmic robot motion planning course,by Professor Jean-Claude Latombe,who(literally)wrote the book on the topic.Knowledge Representation&Reasoning•CS222(Win):Logical knowledge representation and reasoning.Taught by Profes-sor Yoav Shoham and Professor Johan van Benthem.•CS227(Spr):Algorithmic methods such as search,CSP,planning.Taught by Dr.Yorke-Smith(SRI).Probabilistic Methods•CS228(Win):Probabilistic models in AI.Bayesian networks,hidden Markov mod-els,and planning under uncertainty.Taught by Professor Daphne Koller,who works on computational biology,Bayes nets,learning,computational game theory, and more.1Perception&Understanding•CS223B(Win):Introduction to computer vision.Algorithms for processing and interpreting image or camera information.Taught by Professor Sebastian Thrun, who led the DARPA Grand Challenge/DARPA Urban Challenge teams,or Pro-fessor Jana Kosecka,who works on vision and robotics.•CS224S(Win):Speech recognition and synthesis.Algorithms for large vocabu-lary continuous speech recognition,text-to-speech,conversational dialogue agents.Taught by Professor Dan Jurafsky,who co-authored one of the two most-used textbooks on NLP.•CS224N(Spr):Natural language processing,including parsing,part of speech tagging,information extraction from text,and more.Taught by Professor Chris Manning,who co-authored the other of the two most-used textbooks on NLP.•CS224U(Win):Natural language understanding,including computational seman-tics and pragmatics,with application to question answering,summarization,and inference.Taught by Professors Dan Jurafsky and Chris Manning.Multi-agent systems•CS224M(Win):Multi-agent systems,including game theoretic foundations,de-signing systems that induce agents to coordinate,and multi-agent learning.Taught by Professor Yoav Shoham,who works on economic models of multi-agent interac-tions.•CS227B(Spr):General game playing.Reasoning and learning methods for playing any of a broad class of games.Taught by Professor Michael Genesereth,who works on computational logic,enterprise management and e-commerce.Convex Optimization•EE364A(Win):Convex Optimization.Convexity,duality,convex programs,inte-rior point methods,algorithms.Taught by Professor Stephen Boyd,who works on optimization and its application to engineering problems.AI Project courses•CS294B/CS294W(Win):STAIR(STanford AI Robot)project.Project course with no lectures.By drawing from machine learning and all other areas of AI, we’ll work on the challenge problem of building a general-purpose robot that can carry out home and office chores,such as tidying up a room,fetching items,and preparing meals.Taught by Professor Andrew Ng.2。
关于人工智能的发展情况英语作文

关于人工智能的发展情况英语作文英文回答:Artificial intelligence (AI) has evolved significantly over the past few decades, making tremendous strides in various domains. Here are some key developments in AI's growth:1. Machine Learning and Deep Learning:Machine learning and deep learning algorithms have revolutionized AI. These techniques allow computers to learn patterns and make predictions from data without explicit programming. Machine learning models have achieved state-of-the-art performance in image recognition, natural language processing, and speech recognition.2. Natural Language Processing (NLP):NLP has made significant advancements, enablingcomputers to understand and generate human language. AI models can now translate languages, summarize text, answer questions, and engage in conversations. This has led to breakthroughs in customer service, information retrieval, and language-based applications.3. Computer Vision:Computer vision algorithms have made it possible for computers to "see" and interpret visual information. AI models can now identify objects, faces, and scenes with high accuracy. This has applications in security, autonomous vehicles, and medical imaging.4. Robotics:AI has played a crucial role in the advancement of robotics. AI-powered robots can now navigate complex environments, manipulate objects, and interact with humans. They are being used in manufacturing, healthcare, and space exploration.5. Generative AI:Generative AI models, such as GANs (Generative Adversarial Networks), have demonstrated the ability to generate realistic images, text, and music. These modelsare pushing the boundaries of art and entertainment andhave potential applications in content creation and design.6. Edge AI:Edge AI involves deploying AI models on devices like smartphones and embedded systems. This allows for real-time data processing and decision-making at the edge, reducing latency and improving efficiency.7. Ethical and Societal Considerations:As AI advances, it raises ethical and societal concerns regarding privacy, bias, accountability, and job displacement. It is crucial to develop ethical AI practices and policies to ensure responsible and beneficial use of AI.中文回答:人工智能发展情况。
计算机视觉与人工智能

计算机视觉与人工智能引言:计算机视觉(Computer Vision)和人工智能(Artificial Intelligence)是两个快速发展的领域,它们不仅各自有着广泛的应用,而且两者之间也有着密不可分的联系。
本文将探讨计算机视觉和人工智能的定义、历史发展、应用领域以及未来发展趋势。
一、计算机视觉的定义与发展1. 计算机视觉的定义计算机视觉是指使计算机能够模拟和实现人类视觉系统的一种技术。
通过计算机视觉技术,计算机可以感知、理解和解释图像或视频数据,并进行相应的处理和分析。
2. 计算机视觉的发展历程计算机视觉作为一门学科源于会议世纪六七十年代,当时主要通过数字图像处理来实现对图像数据的分析和处理。
然而,由于当时计算机计算能力的限制以及算法的不完善,计算机视觉的发展进展缓慢。
随着计算机性能的提升以及图像采集技术的改进,计算机视觉逐渐迎来了快速发展的时期。
到了21世纪,计算机视觉在图像分析、目标检测、人脸识别等领域取得了显著的成果。
二、人工智能的定义与发展1. 人工智能的定义人工智能是指使计算机具备像人类智能一样的学习、推理、自然语言处理、问题解决和决策能力的一种技术。
通过人工智能技术,计算机可以模拟和实现人类思维和行为。
2. 人工智能的发展历程人工智能的概念最早可以追溯到20世纪50年代,当时的研究主要集中在逻辑推理和问题解决方面。
然而,由于计算机的局限性以及算法的不完善,人工智能在当时的发展进展有限。
20世纪90年代以后,随着计算机计算能力的提升、机器学习算法的发展以及大数据的普及,人工智能开始进入快速发展的阶段。
现在,人工智能已经应用于诸如语音识别、自然语言处理、机器人领域等广泛领域,并取得了很好的成果。
三、的联系与区别1. 的联系计算机视觉和人工智能都是人类对智能的模拟与实现,它们有着密不可分的联系。
计算机视觉通过感知、理解和解释图像数据,将复杂的视觉信息转化为具有意义的数据。
而人工智能则通过学习、推理和决策等技术,使计算机具备智能行为和决策能力。
机器人技术的发展英语作文
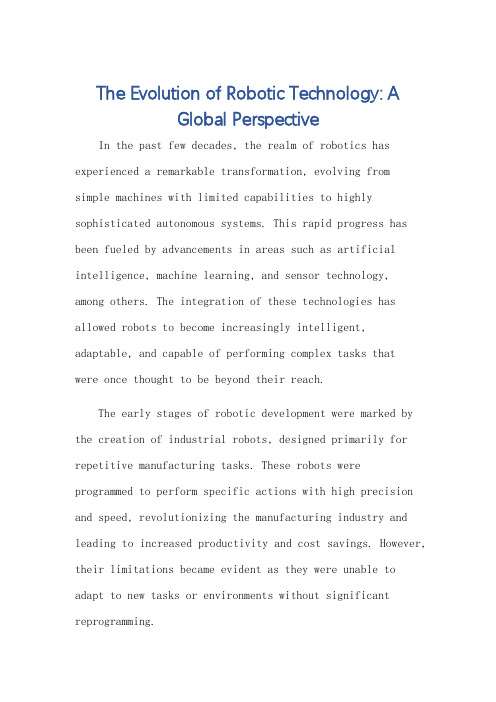
The Evolution of Robotic Technology: AGlobal PerspectiveIn the past few decades, the realm of robotics has experienced a remarkable transformation, evolving from simple machines with limited capabilities to highly sophisticated autonomous systems. This rapid progress has been fueled by advancements in areas such as artificial intelligence, machine learning, and sensor technology, among others. The integration of these technologies has allowed robots to become increasingly intelligent, adaptable, and capable of performing complex tasks thatwere once thought to be beyond their reach.The early stages of robotic development were marked by the creation of industrial robots, designed primarily for repetitive manufacturing tasks. These robots were programmed to perform specific actions with high precision and speed, revolutionizing the manufacturing industry and leading to increased productivity and cost savings. However, their limitations became evident as they were unable to adapt to new tasks or environments without significant reprogramming.With the advent of artificial intelligence and machine learning, robots have become capable of learning and adapting to new situations. This has enabled them to perform a wide range of tasks, from assisting in surgical procedures to navigating complex environments like space stations. Autonomous robots, such as self-driving cars and drones, are now a reality, thanks to advancements in sensor technology and computer vision.The future of robotics looks even more promising. With the continuous development of new technologies, robots are expected to become even more intelligent and autonomous. They may even reach a point where they can collaborate and work alongside humans seamlessly, enhancing ourcapabilities and taking on tasks that are too dangerous or complex for us to handle.However, the rapid growth of robotics also presents challenges. One of the main concerns is the potential displacement of human workers by robots. As robots become more capable, there is a risk that they may replace humans in many jobs, leading to job losses and social upheaval. It is, therefore, crucial that we invest in retraining andreskilling programs to help workers adapt to the changing job market.Another challenge is the ethical implications of robotic technology. As robots become more autonomous, we need to consider the ethical questions they raise, such as who should be responsible for their actions and how we should program them to make ethical decisions.Despite these challenges, the evolution of robotic technology holds immense potential for positive transformation. It has the potential to revolutionize various industries, improve productivity, and enhance the quality of life for millions of people. By addressing the challenges head-on and investing in research and development, we can ensure that the future of robotics is one that benefits society at large.**机器人技术的演进:全球视角**在过去的几十年里,机器人领域经历了显著的变化,从功能有限的简单机器发展到高度复杂且高度自主的系统。
人工智能介绍英文版
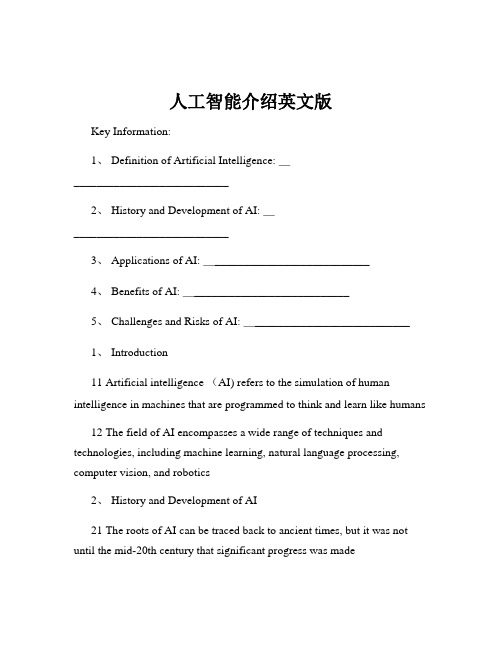
人工智能介绍英文版Key Information:1、 Definition of Artificial Intelligence: ____________________________2、 History and Development of AI: ____________________________3、 Applications of AI: ____________________________4、 Benefits of AI: ____________________________5、 Challenges and Risks of AI: ____________________________1、 Introduction11 Artificial intelligence (AI) refers to the simulation of human intelligence in machines that are programmed to think and learn like humans12 The field of AI encompasses a wide range of techniques and technologies, including machine learning, natural language processing, computer vision, and robotics2、 History and Development of AI21 The roots of AI can be traced back to ancient times, but it was not until the mid-20th century that significant progress was made22 In the 1950s, the concept of AI was formally introduced, and early research focused on developing algorithms for problemsolving and decisionmaking23 Over the years, advancements in computing power, data availability, and algorithmic improvements have led to significant breakthroughs in AI3、 Applications of AI31 Healthcare311 AI is used in medical diagnosis, drug discovery, and patient monitoring312 Machine learning algorithms can analyze large amounts of medical data to identify patterns and predict diseases32 Finance321 In the financial sector, AI is employed for fraud detection, risk assessment, and investment decisionmaking322 Automated trading systems use AI to make rapid and informed trading decisions33 Transportation331 Selfdriving cars and intelligent transportation systems rely on AI for navigation and traffic management332 AI can optimize routes and improve the efficiency of public transportation4、 Benefits of AI41 Increased Efficiency and Productivity411 AIpowered systems can perform tasks faster and more accurately than humans, leading to improved operational efficiency412 Automation of repetitive tasks frees up human resources for more complex and creative work42 Improved DecisionMaking421 By analyzing large amounts of data, AI can provide valuable insights and predictions to support decisionmaking processes422 Businesses and organizations can make more informed and strategic decisions based on AIdriven analytics43 Enhanced Customer Experience431 AIpowered chatbots and virtual assistants offer 24/7 customer service, providing quick and accurate responses432 Personalized recommendations based on AI algorithms enhance the customer experience and increase customer satisfaction5、 Challenges and Risks of AI51 Ethical and Moral Concerns511 Issues such as bias in algorithms, data privacy, and the potential for autonomous weapons raise ethical questions512 Ensuring that AI is developed and used in an ethical and responsible manner is crucial52 Job Displacement521 The automation of certain jobs by AI may lead to unemployment and the need for workforce reskilling and upskilling522 However, new job opportunities are also emerging in the field of AI and related technologies53 Security Risks531 AI systems can be vulnerable to cyberattacks and malicious use532 Safeguarding AI infrastructure and data is essential to prevent security breaches6、 Conclusion61 AI has the potential to bring significant benefits and transform various aspects of our lives62 However, it is essential to address the challenges and risks associated with its development and use to ensure a positive impact on society63 Continued research and ethical considerations will be crucial in shaping the future of AI。
机器人学、机器视觉与控制 英文版
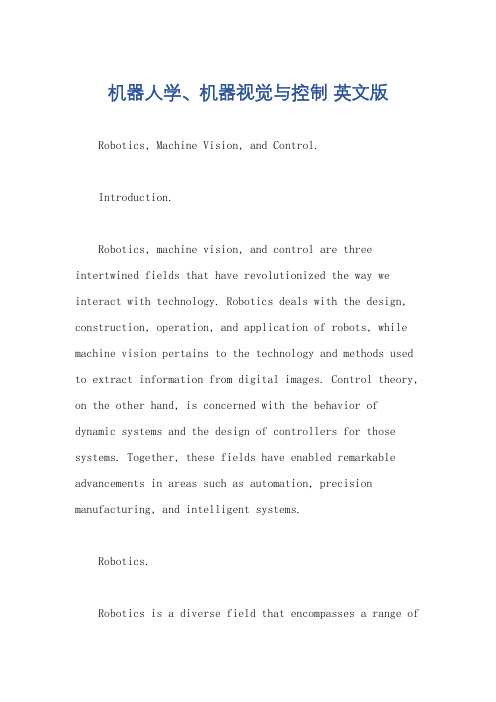
机器人学、机器视觉与控制英文版Robotics, Machine Vision, and Control.Introduction.Robotics, machine vision, and control are three intertwined fields that have revolutionized the way we interact with technology. Robotics deals with the design, construction, operation, and application of robots, while machine vision pertains to the technology and methods used to extract information from digital images. Control theory, on the other hand, is concerned with the behavior of dynamic systems and the design of controllers for those systems. Together, these fields have enabled remarkable advancements in areas such as automation, precision manufacturing, and intelligent systems.Robotics.Robotics is a diverse field that encompasses a range oftechnologies and applications. Robots can be classified based on their purpose, mobility, or structure. Industrial robots are designed for repetitive tasks in manufacturing, while service robots are used in sectors like healthcare, domestic assistance, and security. Mobile robots, such as autonomous vehicles and drones, are capable of navigating their environment and performing complex tasks.The heart of any robot is its control system, which is responsible for decision-making, motion planning, and execution. Modern robots often employ sensors to perceive their environment and advanced algorithms to process this information. The field of robotics is constantly evolving, with new technologies such as artificial intelligence, deep learning, and human-robot interaction promising even more capabilities in the future.Machine Vision.Machine vision is a crucial component of many robotic and automated systems. It involves the use of cameras, sensors, and algorithms to capture, process, and understanddigital images. Machine vision systems can identify objects, read text, detect patterns, and measure dimensions withhigh precision.In industrial settings, machine vision is used fortasks like quality control, part recognition, and robot guidance. In healthcare, it's employed for diagnostic imaging, surgical assistance, and patient monitoring. Machine vision technology is also finding its way into consumer products, such as smartphones and self-driving cars, where it enables advanced features like face recognition, augmented reality, and autonomous navigation.Control Theory.Control theory is the study of how to design systemsthat can adapt their behavior to achieve desired outcomes.It's at the core of robotics and machine vision, as it governs how systems respond to changes in their environment. Control systems can be analog or digital, and they range from simple switches and sensors to complex algorithms running on powerful computers.In robotics, control theory is used to govern the movement of robots, ensuring they can accurately andreliably perform tasks. Machine vision systems also rely on control theory to process and interpret images in real-time. Advanced control strategies, such as adaptive control,fuzzy logic, and reinforcement learning, are enablingrobots and automated systems to adapt to changingconditions and learn from experience.Conclusion.Robotics, machine vision, and control theory are converging to create a new era of intelligent, autonomous systems. As these fields continue to evolve, we can expectto see even more remarkable advancements in areas like precision manufacturing, healthcare, transportation, and beyond. The potential impact of these technologies onsociety is immense, and it's exciting to imagine what the future holds.。
人工智能的知识点了解人工智能应用和发展的前沿领域
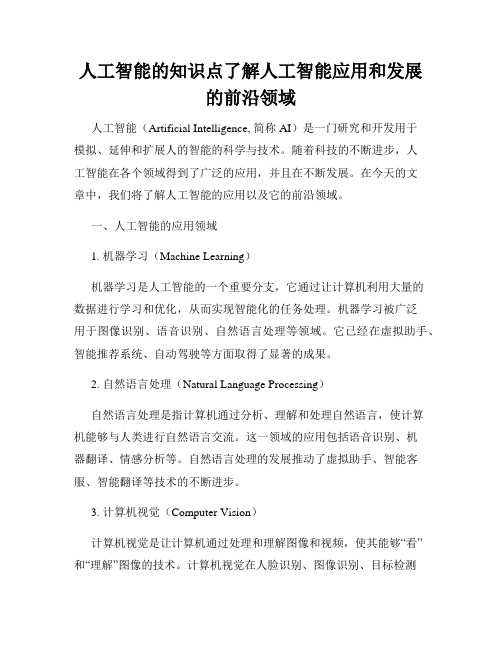
人工智能的知识点了解人工智能应用和发展的前沿领域人工智能(Artificial Intelligence, 简称AI)是一门研究和开发用于模拟、延伸和扩展人的智能的科学与技术。
随着科技的不断进步,人工智能在各个领域得到了广泛的应用,并且在不断发展。
在今天的文章中,我们将了解人工智能的应用以及它的前沿领域。
一、人工智能的应用领域1. 机器学习(Machine Learning)机器学习是人工智能的一个重要分支,它通过让计算机利用大量的数据进行学习和优化,从而实现智能化的任务处理。
机器学习被广泛用于图像识别、语音识别、自然语言处理等领域。
它已经在虚拟助手、智能推荐系统、自动驾驶等方面取得了显著的成果。
2. 自然语言处理(Natural Language Processing)自然语言处理是指计算机通过分析、理解和处理自然语言,使计算机能够与人类进行自然语言交流。
这一领域的应用包括语音识别、机器翻译、情感分析等。
自然语言处理的发展推动了虚拟助手、智能客服、智能翻译等技术的不断进步。
3. 计算机视觉(Computer Vision)计算机视觉是让计算机通过处理和理解图像和视频,使其能够“看”和“理解”图像的技术。
计算机视觉在人脸识别、图像识别、目标检测等领域有广泛的应用。
它在安防监控、医学影像分析、自动驾驶等方面发挥着重要作用。
4. 机器人技术(Robotics)机器人技术是将人工智能与机械工程相结合,开发能够模拟人类智能并执行任务的机器人系统。
机器人技术已经在制造业、医疗保健、农业等领域得到广泛应用。
随着人工智能的不断发展,机器人将扮演更加重要的角色,实现更多复杂的任务。
二、人工智能的前沿领域1. 强化学习(Reinforcement Learning)强化学习是一种让计算机通过与环境不断交互学习最优策略的方法。
通过奖励与惩罚体系,强化学习使计算机在没有明确指示的情况下自主学习和优化。
强化学习在游戏领域、自动驾驶和机器人导航等领域具有很高的潜力。
移动机器人相关本科教材
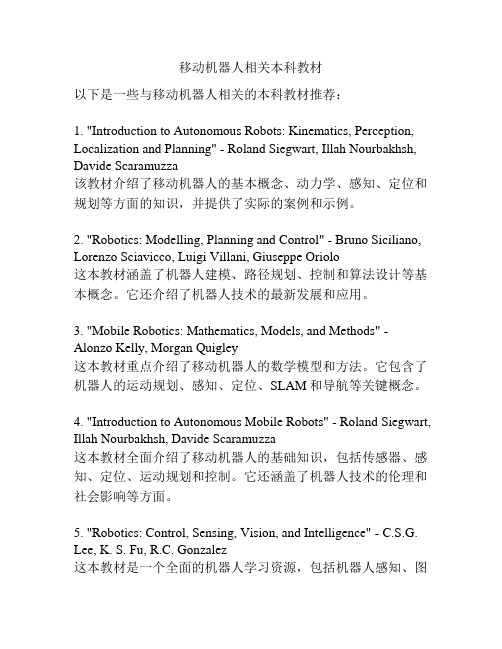
移动机器人相关本科教材以下是一些与移动机器人相关的本科教材推荐:1. "Introduction to Autonomous Robots: Kinematics, Perception, Localization and Planning" - Roland Siegwart, Illah Nourbakhsh, Davide Scaramuzza该教材介绍了移动机器人的基本概念、动力学、感知、定位和规划等方面的知识,并提供了实际的案例和示例。
2. "Robotics: Modelling, Planning and Control" - Bruno Siciliano, Lorenzo Sciavicco, Luigi Villani, Giuseppe Oriolo这本教材涵盖了机器人建模、路径规划、控制和算法设计等基本概念。
它还介绍了机器人技术的最新发展和应用。
3. "Mobile Robotics: Mathematics, Models, and Methods" - Alonzo Kelly, Morgan Quigley这本教材重点介绍了移动机器人的数学模型和方法。
它包含了机器人的运动规划、感知、定位、SLAM和导航等关键概念。
4. "Introduction to Autonomous Mobile Robots" - Roland Siegwart, Illah Nourbakhsh, Davide Scaramuzza这本教材全面介绍了移动机器人的基础知识,包括传感器、感知、定位、运动规划和控制。
它还涵盖了机器人技术的伦理和社会影响等方面。
5. "Robotics: Control, Sensing, Vision, and Intelligence" - C.S.G. Lee, K. S. Fu, R.C. Gonzalez这本教材是一个全面的机器人学习资源,包括机器人感知、图像处理、控制、运动规划和人工智能等方面的内容。
美国人工智能专业大学排名TOP20
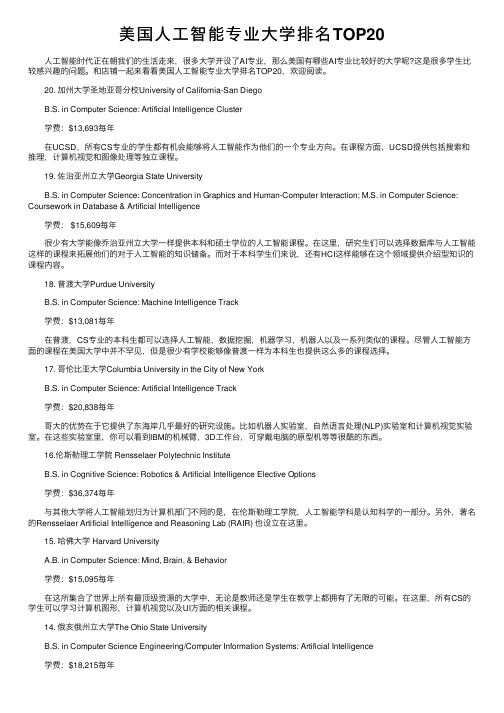
美国⼈⼯智能专业⼤学排名TOP20 ⼈⼯智能时代正在朝我们的⽣活⾛来,很多⼤学开设了AI专业,那么美国有哪些AI专业⽐较好的⼤学呢?这是很多学⽣⽐较感兴趣的问题。
和店铺⼀起来看看美国⼈⼯智能专业⼤学排名TOP20,欢迎阅读。
20. 加州⼤学圣地亚哥分校University of California-San Diego B.S. in Computer Science: Artificial Intelligence Cluster 学费:$13,693每年 在UCSD,所有CS专业的学⽣都有机会能够将⼈⼯智能作为他们的⼀个专业⽅向。
在课程⽅⾯,UCSD提供包括搜索和推理,计算机视觉和图像处理等独⽴课程。
19. 佐治亚州⽴⼤学Georgia State University B.S. in Computer Science: Concentration in Graphics and Human-Computer Interaction; M.S. in Computer Science: Coursework in Database & Artificial Intelligence 学费: $15,609每年 很少有⼤学能像乔治亚州⽴⼤学⼀样提供本科和硕⼠学位的⼈⼯智能课程。
在这⾥,研究⽣们可以选择数据库与⼈⼯智能这样的课程来拓展他们的对于⼈⼯智能的知识储备。
⽽对于本科学⽣们来说,还有HCI这样能够在这个领域提供介绍型知识的课程内容。
18. 普渡⼤学Purdue University B.S. in Computer Science: Machine Intelligence Track 学费:$13,081每年 在普渡,CS专业的本科⽣都可以选择⼈⼯智能,数据挖掘,机器学习,机器⼈以及⼀系列类似的课程。
尽管⼈⼯智能⽅⾯的课程在美国⼤学中并不罕见,但是很少有学校能够像普渡⼀样为本科⽣也提供这么多的课程选择。
Mobile Robotics
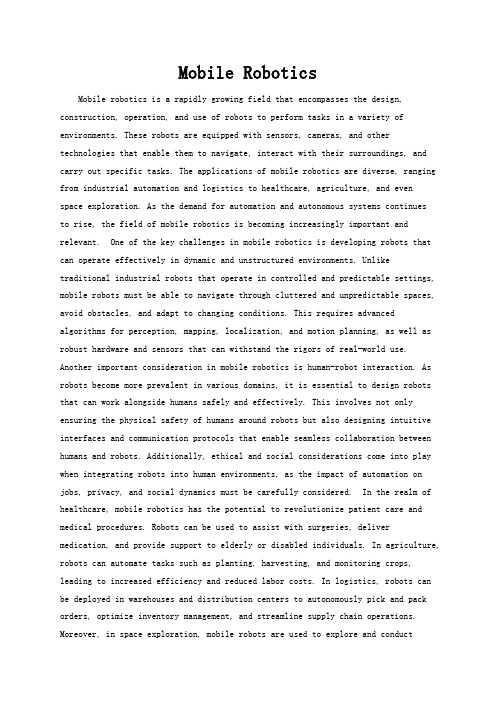
Mobile RoboticsMobile robotics is a rapidly growing field that encompasses the design, construction, operation, and use of robots to perform tasks in a variety of environments. These robots are equipped with sensors, cameras, and other technologies that enable them to navigate, interact with their surroundings, and carry out specific tasks. The applications of mobile robotics are diverse, ranging from industrial automation and logistics to healthcare, agriculture, and even space exploration. As the demand for automation and autonomous systems continuesto rise, the field of mobile robotics is becoming increasingly important and relevant. One of the key challenges in mobile robotics is developing robots that can operate effectively in dynamic and unstructured environments. Unliketraditional industrial robots that operate in controlled and predictable settings, mobile robots must be able to navigate through cluttered and unpredictable spaces, avoid obstacles, and adapt to changing conditions. This requires advanced algorithms for perception, mapping, localization, and motion planning, as well as robust hardware and sensors that can withstand the rigors of real-world use. Another important consideration in mobile robotics is human-robot interaction. As robots become more prevalent in various domains, it is essential to design robots that can work alongside humans safely and effectively. This involves not only ensuring the physical safety of humans around robots but also designing intuitive interfaces and communication protocols that enable seamless collaboration between humans and robots. Additionally, ethical and social considerations come into play when integrating robots into human environments, as the impact of automation on jobs, privacy, and social dynamics must be carefully considered. In the realm of healthcare, mobile robotics has the potential to revolutionize patient care and medical procedures. Robots can be used to assist with surgeries, deliver medication, and provide support to elderly or disabled individuals. In agriculture, robots can automate tasks such as planting, harvesting, and monitoring crops, leading to increased efficiency and reduced labor costs. In logistics, robots can be deployed in warehouses and distribution centers to autonomously pick and pack orders, optimize inventory management, and streamline supply chain operations. Moreover, in space exploration, mobile robots are used to explore and conductexperiments in environments that are too hazardous or remote for humans to access directly. The field of mobile robotics is also driving innovation in artificial intelligence and machine learning. As robots interact with their environments and learn from their experiences, they generate vast amounts of data that can be leveraged to improve their performance and decision-making capabilities. This data can be used to train machine learning models that enable robots to recognize patterns, make predictions, and adapt to new situations. Furthermore, advancements in computer vision and sensor technologies are enabling robots to perceive and understand the world around them with increasing accuracy and precision. Despite the many opportunities and advancements in mobile robotics, there are also challenges and limitations that need to be addressed. One of the main challenges is the need for robust and reliable systems that can operate in diverse and often harsh environments. Robots must be able to withstand temperature extremes, moisture, dust, and physical impacts while maintaining their functionality and safety. Additionally, the power and energy requirements of mobile robots pose a significant constraint, as they must operate for extended periods without recharging or refueling. This necessitates the development of efficient power sources, energy management systems, and autonomous recharging capabilities. Another challenge in mobile robotics is the need for standardization and interoperability. As robots are deployed in various applications and industries, there is a growing need for common standards and protocols that enable different robots and systems to work together seamlessly. This includes standardizing communication interfaces, data formats, and control systems to facilitate interoperability and integration. Furthermore, there is a need for open platforms and frameworks that enable developers to build and deploy robotic applications more easily and efficiently. In conclusion, mobile robotics is a dynamic and multidisciplinary field that holds great promise for addressing a wide range of societal and industrial challenges. From healthcare and agriculture to logistics and space exploration, robots are increasingly being used to perform tasks that are difficult, dangerous, or impractical for humans. As the field continues to advance, it is essential to address the technical, ethical, and societal implications of deploying robots in various domains. By developing robust andreliable systems, addressing human-robot interaction challenges, and driving innovation in artificial intelligence and machine learning, mobile robotics has the potential to transform industries and improve the quality of life for people around the world.。
未来机器人的利与弊英语作文
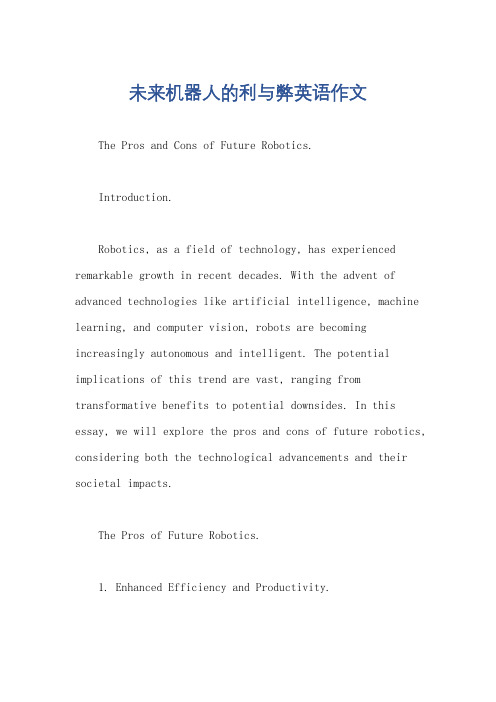
未来机器人的利与弊英语作文The Pros and Cons of Future Robotics.Introduction.Robotics, as a field of technology, has experienced remarkable growth in recent decades. With the advent of advanced technologies like artificial intelligence, machine learning, and computer vision, robots are becoming increasingly autonomous and intelligent. The potential implications of this trend are vast, ranging from transformative benefits to potential downsides. In this essay, we will explore the pros and cons of future robotics, considering both the technological advancements and their societal impacts.The Pros of Future Robotics.1. Enhanced Efficiency and Productivity.Robots are capable of performing tasks with greater precision and speed than humans. In manufacturing, for example, robots can operate machines without fatigue, reducing errors and downtime. This increased efficiency can lead to faster production cycles, cost savings, and a competitive edge for businesses.2. Safety Enhancements.Robotics has the potential to significantly improve safety in hazardous environments. Whether it's exploring deep mines, responding to disasters, or performing surgical procedures, robots can reduce the risk of harm to human personnel. By taking on these dangerous tasks, robotsenable safer working conditions for humans.3. Healthcare Innovations.In healthcare, robots are already making a significant impact. Surgical robots can perform complex operations with greater precision than human hands, while rehabilitation robots help patients recover from strokes or other injuries.Future robots may even be able to diagnose diseases based on advanced imaging and analysis techniques.4. Aiding in Human Development.Robotics can serve as powerful educational tools, enabling students to learn through hands-on experience. Robots can be programmed to simulate real-world scenarios, allowing students to experiment and learn in a safe and controlled environment. This can be particularly beneficial in fields like engineering, where practical experience is crucial.The Cons of Future Robotics.1. Job Displacement.As robots become more capable, they are likely to displace workers in many industries. Automation has already begun to affect job markets, and this trend is expected to accelerate in the future. This could lead to significant economic and social upheaval, as workers struggle to findnew employment opportunities.2. Privacy and Security Concerns.Robots equipped with advanced sensors and AI capabilities could pose a threat to privacy. These robots could potentially collect sensitive information about individuals, raising concerns about data misuse and privacy breaches. Additionally, the increasing interconnectedness of robots could create new vulnerabilities for cyber attacks.3. Ethical Dilemmas.The development of autonomous robots capable of making decisions raises ethical concerns. Who should be responsible for the actions of a robot? What happens if a robot causes harm? These are complex questions that require careful consideration and debate.4. Social Isolation.As robots become more prevalent in our daily lives, there is a concern that they could contribute to social isolation. If individuals become reliant on robots for companionship and social interaction, they may withdraw from human contact, leading to loneliness and social dysfunction.Conclusion.The future of robotics promises both remarkable benefits and potential challenges. The key lies in how we approach these technologies, ensuring that their development is guided by ethical principles and a concern for societal well-being. By investing in education, preparing workers for the changing job market, and establishing robust frameworks for data privacy and robot ethics, we can harness the power of robotics to create a better, safer, and more equitable world.。
人工智能题材的细分板块
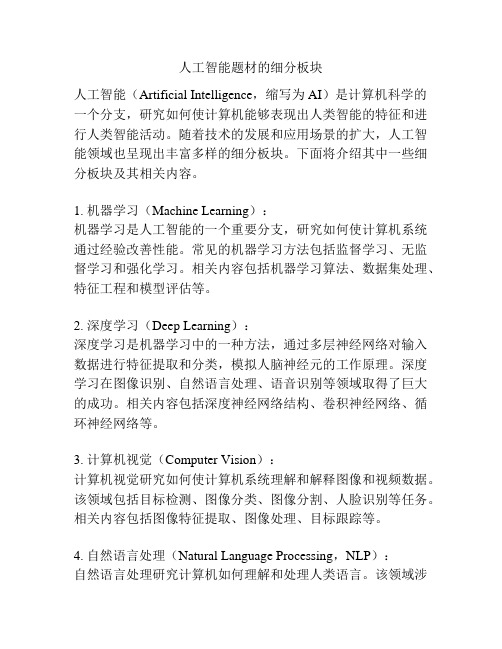
人工智能题材的细分板块人工智能(Artificial Intelligence,缩写为AI)是计算机科学的一个分支,研究如何使计算机能够表现出人类智能的特征和进行人类智能活动。
随着技术的发展和应用场景的扩大,人工智能领域也呈现出丰富多样的细分板块。
下面将介绍其中一些细分板块及其相关内容。
1. 机器学习(Machine Learning):机器学习是人工智能的一个重要分支,研究如何使计算机系统通过经验改善性能。
常见的机器学习方法包括监督学习、无监督学习和强化学习。
相关内容包括机器学习算法、数据集处理、特征工程和模型评估等。
2. 深度学习(Deep Learning):深度学习是机器学习中的一种方法,通过多层神经网络对输入数据进行特征提取和分类,模拟人脑神经元的工作原理。
深度学习在图像识别、自然语言处理、语音识别等领域取得了巨大的成功。
相关内容包括深度神经网络结构、卷积神经网络、循环神经网络等。
3. 计算机视觉(Computer Vision):计算机视觉研究如何使计算机系统理解和解释图像和视频数据。
该领域包括目标检测、图像分类、图像分割、人脸识别等任务。
相关内容包括图像特征提取、图像处理、目标跟踪等。
4. 自然语言处理(Natural Language Processing,NLP):自然语言处理研究计算机如何理解和处理人类语言。
该领域涉及机器翻译、文本分析、问答系统、情感分析等任务。
相关内容包括词向量表示、语义分析、语言生成等技术。
5. 机器人学(Robotics):机器人学研究如何设计和开发具有感知、决策和执行能力的机器人系统。
机器人学涵盖硬件、软件和控制等方面,与人工智能的结合可以使机器人更加智能化和自主化。
相关内容包括机器人感知技术、运动规划、机器人学习等。
6. 增强学习(Reinforcement Learning):增强学习是一种通过试错和奖励机制来训练智能系统的方法。
在这种学习方式下,智能系统通过与环境交互,通过优化奖励函数来实现目标任务。
人工智能技术英语作文
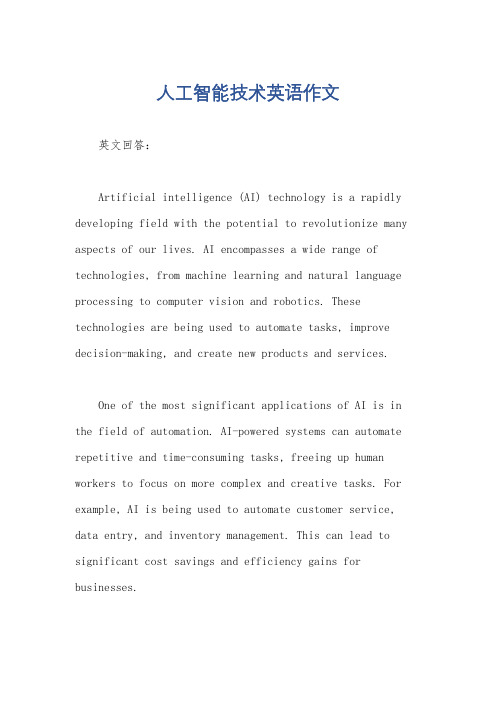
人工智能技术英语作文英文回答:Artificial intelligence (AI) technology is a rapidly developing field with the potential to revolutionize many aspects of our lives. AI encompasses a wide range of technologies, from machine learning and natural language processing to computer vision and robotics. These technologies are being used to automate tasks, improve decision-making, and create new products and services.One of the most significant applications of AI is in the field of automation. AI-powered systems can automate repetitive and time-consuming tasks, freeing up human workers to focus on more complex and creative tasks. For example, AI is being used to automate customer service, data entry, and inventory management. This can lead to significant cost savings and efficiency gains for businesses.AI is also being used to improve decision-making in a variety of industries. AI-powered systems can analyze large amounts of data and identify patterns and trends that would be difficult or impossible for humans to detect. This can help businesses make better decisions about product development, marketing, and investment. For example, AI is being used to predict customer demand, identify fraud, and assess risk.In addition to automation and decision-making, AI is also being used to create new products and services. For example, AI is being used to develop self-driving cars, medical diagnosis tools, and personalized learning systems. These products and services have the potential to improve our lives in many ways, from making it easier to get around to improving our health and well-being.Of course, there are also concerns about the potential negative impacts of AI. Some people worry that AI could lead to job losses, inequality, and even war. It is important to be aware of these concerns and to take steps to mitigate them. However, it is also important to rememberthat AI has the potential to be a powerful force for goodin the world. By using AI responsibly, we can ensure thatits benefits are shared by all.中文回答:人工智能技术。
机器人的重要性英语作文
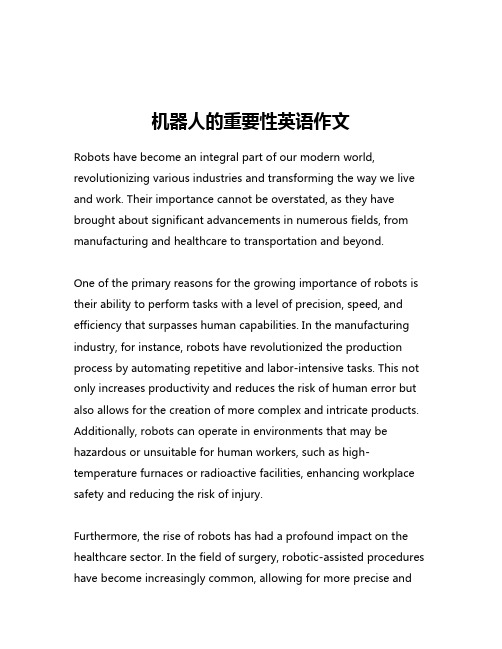
机器人的重要性英语作文Robots have become an integral part of our modern world, revolutionizing various industries and transforming the way we live and work. Their importance cannot be overstated, as they have brought about significant advancements in numerous fields, from manufacturing and healthcare to transportation and beyond.One of the primary reasons for the growing importance of robots is their ability to perform tasks with a level of precision, speed, and efficiency that surpasses human capabilities. In the manufacturing industry, for instance, robots have revolutionized the production process by automating repetitive and labor-intensive tasks. This not only increases productivity and reduces the risk of human error but also allows for the creation of more complex and intricate products. Additionally, robots can operate in environments that may be hazardous or unsuitable for human workers, such as high-temperature furnaces or radioactive facilities, enhancing workplace safety and reducing the risk of injury.Furthermore, the rise of robots has had a profound impact on the healthcare sector. In the field of surgery, robotic-assisted procedures have become increasingly common, allowing for more precise andminimally invasive interventions. This has led to faster recovery times, reduced scarring, and improved patient outcomes. Robots are also being used in rehabilitation and physical therapy, where they can provide personalized and consistent exercises tailored to the needs of individual patients. This has been particularly beneficial for individuals with disabilities or mobility challenges, as it has enabled them to regain greater independence and improve their quality of life.Another area where robots have demonstrated their importance is in the field of transportation. Autonomous vehicles, powered by advanced sensors and artificial intelligence, have the potential to revolutionize the way we move around. These self-driving cars can navigate through traffic, avoid collisions, and transport passengers and goods more efficiently, potentially reducing congestion, emissions, and the risk of human error. Additionally, robots are being used in the logistics and supply chain industries, where they can optimize the movement and storage of goods, leading to faster and more reliable deliveries.Beyond these practical applications, the importance of robots also lies in their potential to contribute to scientific and technological advancements. Robots can be used to explore and study environments that are inaccessible or hazardous for humans, such as the deep ocean or the surface of other planets. This has led togroundbreaking discoveries and a better understanding of our world and the universe. Furthermore, the development of robots has spurred the advancement of fields like artificial intelligence, machine learning, and computer vision, which have applications far beyond the realm of robotics itself.However, the importance of robots extends beyond their practical applications. They have also had a significant impact on the way we work and live. The automation of certain tasks has led to concerns about job displacement, and it is essential to address these concerns through policies and initiatives that support the retraining and reemployment of displaced workers. Additionally, as robots become more sophisticated and autonomous, there are ethical and philosophical questions that must be grappled with, such as the implications of AI decision-making and the potential for robots to replace human interactions.Despite these challenges, the importance of robots cannot be overstated. They have transformed industries, improved lives, and opened up new frontiers of scientific exploration. As technology continues to advance, the role of robots in our society is likely to become even more prominent, and it is crucial that we embrace and harness their potential in a responsible and ethical manner. By doing so, we can unlock the full benefits of this transformative technology and create a better future for all.。
基于深度学习的人手视觉追踪机器人
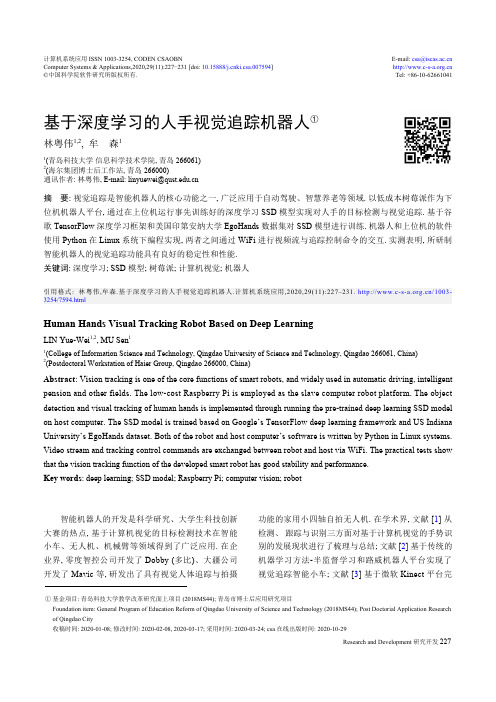
基于深度学习的人手视觉追踪机器人①林粤伟1,2, 牟 森11(青岛科技大学 信息科学技术学院, 青岛 266061)2(海尔集团博士后工作站, 青岛 266000)通讯作者: 林粤伟, E-mail: ******************.cn摘 要: 视觉追踪是智能机器人的核心功能之一, 广泛应用于自动驾驶、智慧养老等领域. 以低成本树莓派作为下位机机器人平台, 通过在上位机运行事先训练好的深度学习SSD 模型实现对人手的目标检测与视觉追踪. 基于谷歌TensorFlow 深度学习框架和美国印第安纳大学EgoHands 数据集对SSD 模型进行训练. 机器人和上位机的软件使用Python 在Linux 系统下编程实现, 两者之间通过WiFi 进行视频流与追踪控制命令的交互. 实测表明, 所研制智能机器人的视觉追踪功能具有良好的稳定性和性能.关键词: 深度学习; SSD 模型; 树莓派; 计算机视觉; 机器人引用格式: 林粤伟,牟森.基于深度学习的人手视觉追踪机器人.计算机系统应用,2020,29(11):227–231. /1003-3254/7594.htmlHuman Hands Visual Tracking Robot Based on Deep LearningLIN Yue-Wei 1,2, MU Sen 11(College of Information Science and Technology, Qingdao University of Science and Technology, Qingdao 266061, China)2(Postdoctoral Workstation of Haier Group, Qingdao 266000, China)Abstract : Vision tracking is one of the core functions of smart robots, and widely used in automatic driving, intelligent pension and other fields. The low-cost Raspberry Pi is employed as the slave computer robot platform. The object detection and visual tracking of human hands is implemented through running the pre-trained deep learning SSD model on host computer. The SSD model is trained based on Google’s TensorFlow deep learning framework and US Indiana University’s EgoHands dataset. Both of the robot and host computer’s software is written by Python in Linux systems.Video stream and tracking control commands are exchanged between robot and host via WiFi. The practical tests show that the vision tracking function of the developed smart robot has good stability and performance.Key words : deep learning; SSD model; Raspberry Pi; computer vision; robot智能机器人的开发是科学研究、大学生科技创新大赛的热点, 基于计算机视觉的目标检测技术在智能小车、无人机、机械臂等领域得到了广泛应用. 在企业界, 零度智控公司开发了Dobby (多比)、大疆公司开发了Mavic 等, 研发出了具有视觉人体追踪与拍摄功能的家用小四轴自拍无人机. 在学术界, 文献[1] 从检测、 跟踪与识别三方面对基于计算机视觉的手势识别的发展现状进行了梳理与总结; 文献[2]基于传统的机器学习方法-半监督学习和路威机器人平台实现了视觉追踪智能小车; 文献[3]基于微软Kinect 平台完计算机系统应用 ISSN 1003-3254, CODEN CSAOBNE-mail: ************.cn Computer Systems & Applications,2020,29(11):227−231 [doi: 10.15888/ki.csa.007594] ©中国科学院软件研究所版权所有.Tel: +86-10-62661041① 基金项目: 青岛科技大学教学改革研究面上项目(2018MS44); 青岛市博士后应用研究项目Foundation item: General Program of Education Reform of Qingdao University of Science and Technology (2018MS44); Post Doctorial Application Research of Qingdao City收稿时间: 2020-01-08; 修改时间: 2020-02-08, 2020-03-17; 采用时间: 2020-03-24; csa 在线出版时间: 2020-10-29成了视觉追踪移动机器人控制系统的设计; 文献[4]对服务机器人视觉追踪过程中的运动目标检测与跟踪算法进行研究并在ROS (Robot Operating System, 机器人操作系统)机器人平台进行实现.上述视觉追踪功能的实现大多采用传统的目标检测方法, 基于图像特征和机器学习, 且所采用平台成本相对较高. 近年随着大数据与人工智能技术的兴起, 利用深度学习直接将分类标记好的图像数据集输入深度卷积神经网络大大提升了图像分类、目标检测的精确度. 国内外基于Faster R-CNN (Faster Region-Convolutional Neural Network, 更快的区域卷积神经网络)、YOLO (You Only Look Once, 一种single-stage 目标检测算法)、SSD (Single Shot multibox Detector, 单步多框检测器)等模型的深度学习算法得到广泛应用,如文献[5]将改进的深度学习算法应用于中国手语识别. 本文基于深度学习[6]技术, 在低成本树莓派[7]平台上设计实现了视觉追踪智能机器人(小车), 小车能够通过摄像头识别人手并自动追踪跟随人手. 与现有研究的主要不同之处在于使用了更为经济的低成本树莓派作为机器人平台, 并且在目标检测的算法上使用了基于TensorFlow [8]深度学习框架的SSD 模型, 而不是基于传统的图像特征和机器学习算法.1 关键技术1.1 系统架构如图1, 整个系统分为机器人小车(下位机)和主控电脑(上位机)两部分. 上位机基于深度学习卷积神经网络做出预测, 下位机负责机器人的行进以及视频数据采集与传输, 两者之间通过WiFi 通信. 其中, 小车主控板为开源的树莓派3代B 开发板, CPU (ARM 芯片)主频1.2 GHz, 运行有树莓派定制的嵌入式Linux 操作系统, 配以板载WiFi 模块、CSI 接口摄像头、底盘构成下位机部分. 上位机操作运行事先训练好的SSD 模型[9]. 小车摄像头采集图像数据, 将其通过WiFi 传输给上位机, 并作为SSD 模型的输入. SSD 模型如果从输入的图像中检测到人手, 会得到人手在图像中的位置, 据此决定小车的运动方向和距离(需要保持人手在图像中央), 进而向小车发送控制命令, 指示运动方向和距离. 小车收到上位机发来的远程控制命令后,做出前进、转向等跟踪人手的动作. 智能小车和主控电脑两端皆运行用Python [10]编写的脚本程序.1.2 深度学习SSD 模型SSD 模型全名为Single Shot multibox Detector [9],是一种基于深度学习的one stage (一次)目标检测模型. SSD 模型由一个基础网络(base network)的输出级后串行连接几种不同的辅助网络构成, 如图2所示. 不同于之前two stage 的Region CNN [11], SSD 模型是一个one stage 模型, 即只需在一个网络中即可完成目标检测, 效率更高.摄像头控制图传传输图像发送命令SSD 模型树莓派图1 智能机器人系统架构S S D300300351210241024512256256256Image 3838Conv4_3Conv6_21919Conv6(FC6)1919Conv7(FC7)1919Conv9_255Conv10_2Conv11_2331Conv: 3×3×1024Conv: 1×1×1024Conv: 1×1×256Conv: 3×3×512−s2Conv: 1×1×128Conv: 3×3×256−s2Conv: 1×1×128Conv: 3×3×256−s1Conv: 1×1×128Conv: 3×3×256−s1VGG-16Through Conv5_3 layerClassifier: Conv:3×3×(4×(Classes+4))Classifier: Conv:3×3×(6×(Classes+4))Conv: Conv:3×3×(4×(Classes+4))D e t e c t i o n s : 8732 p e r c l a s sN o n -m a x i m u m s u p p r e s s i o n74.3 mAP 59 FPS图2 SSD 模型SSD 模型采用多尺度特征预测的方法得到多个不同尺寸的特征图[9]. 假设模型检测时采用m 层特征图,则得到第k 个特征图的默认框比例公式如式(1):S k =S min +S max −S minm −1(k −1),k ∈{1,2,···,m }(1)其中, S k 表示特征图上的默认框大小相对于输入原图计算机系统应用2020 年 第 29 卷 第 11 期的比例(scale). 一般取S min =0.2, S max =0.9. m 为特征图个数.SSD 模型的损失函数定义为位置损失与置信度损失的加权和[9], 如式(2)所示:L (x ,c ,l ,g )=1N(L conf (x ,c )+αL loc (x ,l ,g ))(2)其中, N 表示与真实物体框相匹配的默认框数量; c 是预测框的置信度; l 为预测框的位置信息; g 是真实框的位置信息; α是一个权重参数, 将它设为1; L loc (x,l,g )位置损失是预测框与真实框的Smooth L1损失函数;L conf (x,c )是置信度损失, 这里采用交叉熵损失函数.1.3 TensorFlow 平台使用谷歌TensorFlow 深度学习框架对SSD 模型进行训练. TensorFlow 能够将复杂的数据结构传输至人工智能神经网络中进行学习和预测, 近年广泛应用于图像分类、机器翻译等领域. TensorFlow 有着强大的Python API 函数, 而本文实现的智能小车和主控电脑端运行的程序皆为Python 脚本, 可以方便的调用Python API 函数.2 设计与实现系统主程序软件流程如图3所示. 上位机运行自行编写的Python 脚本作为主程序, 接收下位机发来的图像, 并将其输入到事先训练好的深度学习SSD 模型中, 以检测人手目标. 若检测到人手, 则产生、发送控制命令至下位机. 下位机运行两个自行编写的Python 脚本, 其中一个脚本基于开源的mjpg-streamer 软件采集、传输图像至上位机, 另一个接收来自上位机的控制命令并通过GPIO 端口控制车轮运动.机器人上电Linux 系统启动采集图像图传进程控制进程打开摄像头把采集到的图像以流的方式通过 IP 网络传输到上位机机器人掉电启动进程连接 WiFi AP启动 http 服务器是否有上位机控制命令?控制车轮Y N智能机器人/下位机 (服务端)电脑/上位机 (客户端)连接 WiFi AP 进程启动接收图像将图像输入SSD 模型进行识别是否检测到人手?根据人手在图像中的位置生成并发送命令进程退出YNhttp/WiFi 通信图3 主程序软件流程2.1 深度学习SSD 模型训练上位机电脑和CPU 型号为联想Thinkpad E540酷睿i5 (第4代), 操作系统为Ubuntu 16.04 LTS 64位,TensorFlow 版本为v1.4.0, 采用TensorFlow Object Detection API 的SSD MobileNet V1模型. 训练数据直接使用了美国印第安纳大学计算机视觉实验室公开的EgoHands 数据集, 该数据集是一个向外界开放下载的1.2 GB 的已经标注好的数据集, 用谷歌眼镜采集第一视角下的人手图像数据, 例如玩牌、下棋等场景下人手的姿态. 首先对数据集进行数据整理, 将其转换为TensorFlow 专有的TF Record 数据集格式文件, 然后修改TensorFlow 目标检测训练配置文件ssd_2020 年 第 29 卷 第 11 期计算机系统应用mobilenet_v1_coco.config. 训练全程在电脑上由通用CPU运行, 共运行26小时. 结束训练后将protobuf格式的二进制文件(真正的SSD模型)保存下来以便下文介绍的上位机Python主程序调用.2.2 上位机设计考虑到小车回传视频的帧数比较高, 且深度学习神经网络的计算也是一件耗时的任务, 在上位机主程序(Python脚本)中建立了两个队列, 一个输入队列用来存储下位机传来的原始图像, 一个输出队列用来存储经神经网络运算处理之后带有标注结果的图像. 上位机通过开源软件OpenCV的cv2.videoCapture类用文件的方式读取视频信息. 运行SSD目标检测模型进行人手识别时, 会得到目标的标注矩形框中心, 当中心落到整幅图像的左侧并超出一定距离时, 产生turnleft 左转指令; 当中心落到整幅图像右侧且超出一定距离的时, 产生turnright右转指令; 当中心落到图像的上半部分并超过一定距离时, 产生forward前进指令. 距离值默认设定为60个像素, 该参数可修改. 预测小车行进方向功能的伪代码如算法1所示.算法1. 上位机预测行进方向伪代码Require: 距离阈值(默认为60像素) while 全部程序就绪 do if 没有识别到目标: Continue; else if 识别到目标: if 识别到目标面积过大, 目标离摄像头太近: Send(“stop”); else: if 目标中心 x<640/2–距离阈值: Send(“turnleft”); if 目标中心 x>640/2+距离阈值: Send(“turnright”); else: Send(“forward”); end while2.3 下位机设计下位机基于低成本树莓派平台实现, 使用开源软件Bottle部署了一个多线程的HTTP服务器, 该服务器接收上位机发出的HTTP POST请求, 提取其中的控制命令进行运动控制. 使用开源软件mjpg-streamer控制网络摄像头采集图像, 并将图像数据以视频流的方式通过IP网络传输到上位机客户端.3 测试结果与评估搭建局域网环境(也支持广域网), 使上位机和下位机接入同一无线路由器. 当摄像头采集到的画面右侧出现人手时, 如图4所示的实时图像中, 标注方框标记出了检测到的人手的位置, 同时控制台输出turnright (右转)控制命令, 此时小车向右侧做出移动. 当屏幕中没有人手时, 画面上面没有用彩色画出的区域, 上位机的终端也不打印输出任何控制命令.图4 人手目标检测功能测试结果功能方面, 针对人手在小车视野的不同位置情况进行所研制小车人手视觉追踪的功能测试. 比如, 当人手在小车前方且完整出现时, 上位机应发出forward (前进)命令, 进而小车收到该命令后向前行进. 当小车视野里没有或只有部分人手时, 应当无命令输出, 小车原地不动. 功能测试用例如表1所示, 测试结果均为预期的正常结果. 性能方面, 所采用基于深度学习SSD 模型的人手目标检测算法的准确性与实时性较好, 算法的mAP (平均精准度) 为74%, 检测速率40 fps左右,可以较好的满足系统要求.表1 人手视觉功能测试结果测试用例输出命令小车动作手在小车正前方60 cm处forward向前行进手在小车左前方60 cm处turnleft向左行进手在小车右前方60 cm处turnright向右行进手在小车正前方130 cm处forward向前行进手在小车左前方130 cm处turnleft向左行进手在小车右前方130 cm处turnright向右行进视野里只有半只手无输出原地不动手在小车视野下方无输出原地不动小车(机器人平台)外观如图5所示. 另外, 由于动态视频文件无法在论文中展示, 这里展示的是录制好计算机系统应用2020 年 第 29 卷 第 11 期的测试视频中2个帧的截图, 如图6所示, 从小车的位置变化可以看出其可以追踪人手.图5 机器人外观图6 追踪人手4 结论本文利用深度学习SSD 目标检测模型对目标进行识别, 将识别的结果用于修正智能小车机器人的行进路线, 满足了智能机器人的视觉追踪功能需求. 其特色主要在于采用了低成本树莓派, 以及深度学习而非传统的神经网络识别算法, 省去了设置特征的步骤. 系统暂时只能用来识别人手, 小车能够跟随人手移动, 功能稳定性与性能良好. 若要识别追踪其他物体, 可以使用其他自己制作或第三方数据集对SSD 模型进行训练, 以把网络的识别对象训练成拟追踪的目标类型. 未来也可应用5G 通信模块, 进行更为稳定低时延的视频传输与控制.参考文献Rautaray SS, Agrawal A. Vision based hand gesturerecognition for human computer interaction: A survey.Artificial Intelligence Review, 2015, 43(1): 1–54. [doi: 10.1007/s10462-012-9356-9]1张子洋, 孙作雷, 曾连荪. 视觉追踪机器人系统构建研究.电子技术应用, 2016, 42(10): 123–126, 130.2王道全. 基于视觉的智能追踪机器人的设计研究[硕士学位论文]. 青岛: 青岛科技大学, 2016.3周燕秋. 服务机器人视觉追踪技术研究[硕士学位论文].上海: 上海师范大学, 2018.4周舟, 韩芳, 王直杰. 改进SSD 算法在中国手语识别上的应用. 计算机工程与应用: 1–7. /kcms/detail/11.2127.TP.20191207.1137.006.html . [2020-03-19].5Goodfellow I, Bengio Y, Courville A. Deep Learning 深度学习. 赵申剑, 黎彧君, 符天凡, 等译. 北京: 人民邮电出版社,2017.6许艳, 孟令军, 王志国. 基于树莓派的元器件检测系统设计. 电子技术应用, 2019, 45(11): 63–67, 71.7郑泽宇, 梁博文, 顾思宇. TensorFlow: 实战Google 深度学习框架. 2版. 北京: 电子工业出版社, 2018.8Liu W, Anguelov D, Erhan D, et al . SSD: Single shotMultiBox detector. Proceedings of the 14th European Conference on Computer Vision. Amsterdam. 2016. 21–37.9Chun W. Python 核心编程. 孙波翔, 李斌, 李晗, 译. 3版. 北京: 人民邮电出版社, 2016.10Girshick R, Donahue J, Darrell T, et al . Rich featurehierarchies for accurate object detection and semantic segmentation. Proceedings of the 2014 IEEE Conference on Computer Vision and Pattern Recognition. Columbus, OH,USA. 2014. 580–587.112020 年 第 29 卷 第 11 期计算机系统应用。
人工智能机器人实验室前景广阔丨Engineering
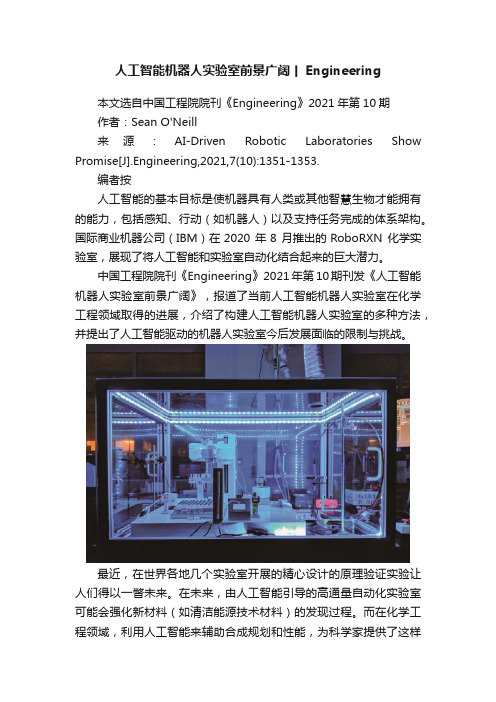
人工智能机器人实验室前景广阔丨Engineering本文选自中国工程院院刊《Engineering》2021年第10期作者:Sean O'Neill来源:AI-Driven Robotic Laboratories Show Promise[J].Engineering,2021,7(10):1351-1353.编者按人工智能的基本目标是使机器具有人类或其他智慧生物才能拥有的能力,包括感知、行动(如机器人)以及支持任务完成的体系架构。
国际商业机器公司(IBM)在2020 年8 月推出的RoboRXN 化学实验室,展现了将人工智能和实验室自动化结合起来的巨大潜力。
中国工程院院刊《Engineering》2021年第10期刊发《人工智能机器人实验室前景广阔》,报道了当前人工智能机器人实验室在化学工程领域取得的进展,介绍了构建人工智能机器人实验室的多种方法,并提出了人工智能驱动的机器人实验室今后发展面临的限制与挑战。
最近,在世界各地几个实验室开展的精心设计的原理验证实验让人们得以一瞥未来。
在未来,由人工智能引导的高通量自动化实验室可能会强化新材料(如清洁能源技术材料)的发现过程。
而在化学工程领域,利用人工智能来辅助合成规划和性能,为科学家提供了这样一种前景:他们只需要一个想法和一个互联网连接,就可在最先进的远程实验室里生成新分子。
2020 年8 月,国际商业机器公司(IBM)宣布推出RoboRXN 化学实验室,让人们看到了将人工智能和实验室自动化结合起来的潜力。
该系统既可提供化学配方来生产目标有机分子,还可以通过市售的硬件,如IBM的演示器将这些分子自动合成。
该演示器由位于瑞士Füllinsdorf 的Chemspeed Technologies 公司制造,是一种Flex自动合成工作站(图1)。
图1 IBM的RoboRXN化学实验室系统合成分子的实时照片。
在图的左下方,可以看到自动合成工作站6 个反应室中的几个。
机器人种类及介绍作文英文
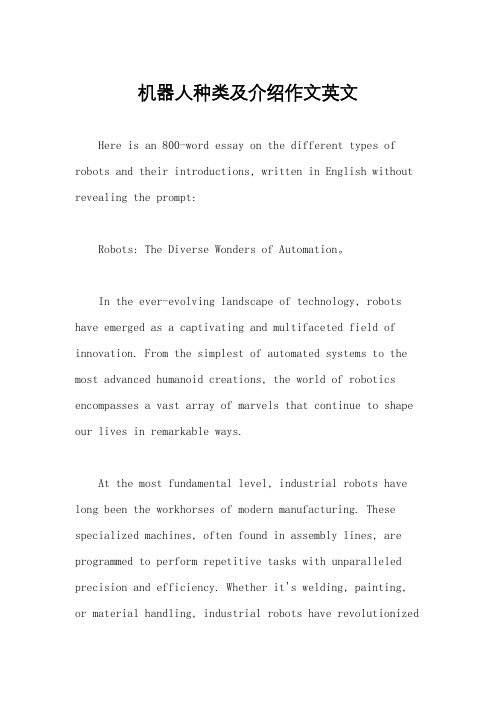
机器人种类及介绍作文英文Here is an 800-word essay on the different types of robots and their introductions, written in English without revealing the prompt:Robots: The Diverse Wonders of Automation。
In the ever-evolving landscape of technology, robots have emerged as a captivating and multifaceted field of innovation. From the simplest of automated systems to the most advanced humanoid creations, the world of robotics encompasses a vast array of marvels that continue to shape our lives in remarkable ways.At the most fundamental level, industrial robots have long been the workhorses of modern manufacturing. These specialized machines, often found in assembly lines, are programmed to perform repetitive tasks with unparalleled precision and efficiency. Whether it's welding, painting, or material handling, industrial robots have revolutionizedthe way we produce goods, driving down costs and improving product quality.Stepping beyond the factory floor, service robots have emerged as invaluable assistants in a wide range of applications. Domestic robots, such as autonomous vacuum cleaners and lawn mowers, have made household chores a breeze, freeing up time for more enjoyable pursuits. In the healthcare sector, robotic surgical systems have transformed medical procedures, allowing for minimally invasive interventions with enhanced precision and reduced patient recovery times.Venturing into the realm of exploration, unmannedaerial vehicles (UAVs), commonly known as drones, have become indispensable tools for surveying, mapping, and even emergency response. These aerial robots can access remote or hazardous areas, gathering valuable data and providing critical support in times of crisis. Similarly, underwater robots, or autonomous underwater vehicles (AUVs), have revolutionized marine research, exploration, and environmental monitoring, allowing scientists to study thedepths of our oceans with unprecedented detail.One of the most captivating developments in robotics is the rise of humanoid robots. These advanced machines, designed to mimic the form and functionality of the human body, have the potential to interact with us inincreasingly natural and intuitive ways. From social robots that can engage in conversation and provide companionship to assistive robots that can aid individuals with disabilities, these humanoid creations are blurring the lines between man and machine.Alongside these physical manifestations of robotics, the field of artificial intelligence (AI) has given rise to a new breed of virtual robots, or chatbots. These software-based agents can engage in natural language processing, allowing them to understand and respond to human queries with remarkable accuracy. From customer service chatbots to virtual personal assistants, these AI-powered robots are transforming the way we interact with technology, providing instant access to information and streamlining a wide range of tasks.As the capabilities of robots continue to expand, their applications have also diversified. In the realm of education, robotic platforms are being used to enhance learning experiences, allowing students to engage with interactive simulations and explore complex concepts in engaging and immersive ways. In the field of space exploration, robotic probes and rovers have ventured to the farthest reaches of our solar system, gathering invaluable data and paving the way for future human missions.The future of robotics holds even greater promise, as researchers and engineers push the boundaries of what is possible. Advancements in areas such as machine learning, computer vision, and materials science are enabling the development of increasingly autonomous, adaptable, and intelligent robots. From self-driving cars that promise to revolutionize transportation to robotic exoskeletons that can augment human physical capabilities, the potential applications of these technologies are truly boundless.As we navigate this exciting era of robotics, it isimportant to consider the ethical and societal implications of these advancements. Questions surrounding job displacement, privacy, and the responsible development of autonomous systems must be carefully addressed to ensurethat the benefits of robotics are equitably distributed and that the risks are mitigated.Nonetheless, the world of robotics remains a testamentto human ingenuity and the relentless pursuit of innovation. From the factory floor to the depths of the ocean, from the skies above to the frontiers of space, robots continue to push the boundaries of what is possible, transforming the way we live, work, and explore our world. As we embracethis technological revolution, we can't help but be captivated by the diverse wonders of automation and the endless possibilities that lie ahead.。
机器人的未来英语作文
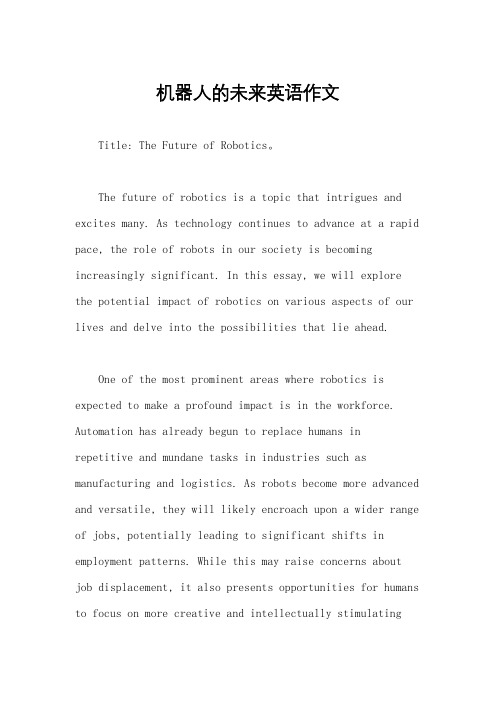
机器人的未来英语作文Title: The Future of Robotics。
The future of robotics is a topic that intrigues and excites many. As technology continues to advance at a rapid pace, the role of robots in our society is becoming increasingly significant. In this essay, we will explore the potential impact of robotics on various aspects of our lives and delve into the possibilities that lie ahead.One of the most prominent areas where robotics is expected to make a profound impact is in the workforce. Automation has already begun to replace humans inrepetitive and mundane tasks in industries such as manufacturing and logistics. As robots become more advanced and versatile, they will likely encroach upon a wider range of jobs, potentially leading to significant shifts in employment patterns. While this may raise concerns about job displacement, it also presents opportunities for humans to focus on more creative and intellectually stimulatingendeavors.In addition to transforming the workforce, robotics holds great promise in fields such as healthcare and eldercare. Robots equipped with artificial intelligence and advanced sensors can assist doctors and nurses in diagnosis, treatment, and patient care. They can also provide companionship and support to the elderly, helping them maintain their independence and quality of life. Furthermore, robotic prosthetics and exoskeletons offerhope to individuals with disabilities, enabling them to regain mobility and functionality.Moreover, robotics has the potential to revolutionize transportation and logistics. Autonomous vehicles,including cars, trucks, and drones, have the ability to enhance safety, reduce traffic congestion, and optimize delivery routes. By leveraging technologies such as machine learning and computer vision, these vehicles can navigate complex environments with precision and efficiency. As a result, our transportation systems are poised to become more sustainable and accessible in the future.Another area where robotics is making strides is in space exploration. Robots are increasingly being deployed to explore distant planets, moons, and asteroids, gathering data and conducting experiments in environments that are hostile to humans. These robotic explorers serve as pioneers, laying the groundwork for future human missions to space. Furthermore, advancements in robotics are driving the development of space mining technologies, which could unlock valuable resources beyond Earth.Furthermore, robotics has the potential torevolutionize education and entertainment. Robots can serve as interactive tutors, providing personalized learning experiences tailored to individual students' needs. They can also act as entertainers, engaging audiences in immersive and interactive performances. From theme parks to classrooms, robots are poised to play a significant role in shaping the future of entertainment and education.However, along with the numerous opportunities that robotics presents, there are also challenges and ethicalconsiderations that must be addressed. Questions surrounding job displacement, privacy concerns, and the ethical use of artificial intelligence require careful consideration and thoughtful regulation. Additionally, ensuring that robotics technologies are accessible and inclusive to all segments of society is essential to prevent exacerbating existing inequalities.In conclusion, the future of robotics holds immense potential to transform various aspects of our lives, from the way we work and travel to how we learn and explore the cosmos. By harnessing the power of technology responsibly and ethically, we can shape a future where robots serve as valuable allies, augmenting human capabilities and enhancing our quality of life. As we stand on the brink of a new era of innovation and discovery, the possibilities are limitless, and the journey ahead promises to be both thrilling and transformative.。
- 1、下载文档前请自行甄别文档内容的完整性,平台不提供额外的编辑、内容补充、找答案等附加服务。
- 2、"仅部分预览"的文档,不可在线预览部分如存在完整性等问题,可反馈申请退款(可完整预览的文档不适用该条件!)。
- 3、如文档侵犯您的权益,请联系客服反馈,我们会尽快为您处理(人工客服工作时间:9:00-18:30)。
Learning and Vision Mobile Robotics Group Research Report2002-2003J.Andrade,F.Moreno,R.Alqu´e zar,J.Aranda,J.Climent,A.Grau,E.Mu˜n oz,F.Serratosa,E.Staffetti,J.Verg´e s,T.Vidal,and A.SanfeliuInstitut de Rob`o tica i Inform`a tica Industrial,UPC-CSICLlorens Artigas4-6,08028Barcelona,Spainlrobots@iri.upc.esAbstractThis article presents the current trends on wheeled mobile robotics being pursued at IRI-UPC-CSIC. It includes an overview of recent results produced in our group in a wide range of areas,includ-ing robot localization,color invariance,segmenta-tion,tracking,visual servoing,and object and face recognition.Keywords:robot localization,color invariance, segmentation,tracking,recognition.1INTRODUCTIONThe Learning and Vision Mobile Robotics Group, and interdisciplinary team of researchers,is the product of a joint effort between the Institut de Rob`o tica i Inform`a tica Industrial and the Departa-ment d’Enginyeria de Sistemas,Autom`a tica i In-form`a tica Industrial at the Universitat Polit`e cnica de Catalunya,and the Departament d’Enginyeria Inform`a tica i Matem`a tiques at the Universitat Rovira i Virgili.Headed by Prof. A.Sanfeliu,as of today,it em-braces5professors,2posdoctoral associates,and 3PhD students.The group,consolidated in1996, has given rise to3PhD thesis and7final year projects.Within the last6years,the group has published7peer reviewed journal articles,7book chapters,6conference proceeding editorials,and presented articles in over40international confer-ences(35indexed in SCI),and15national confer-ences.Furthermore,within the past two years,the mobile robotics platforms developed in our group have been portrayed numerous times on live and printed media[5,7,10,19,20,21].Financial support comes mainly from a continued set of projects from the Interministerial Council of Science and Technology(CICYT)TAP96-0629-C04-03,TAP98-0473,and DPI2001-2223.2CURRENT RESEARCHAREASDuring the last year,our efforts have been tai-lored at giving our mobile platforms the ability to navigate autonomusly in unknown structured set-tings.In this sense,we have contributed new in-sight in the classical simultaneous localization and map building problem,from a control systems the-ory point of view[1,2,6].Furthermore,we have developed new feature validation techniques that improve the robustness of typical map building al-gorithms[3,4].Moreover,very good results have been achieved in the tracking of subjects under varying illumi-nation conditions and in cluttered scenes.On the one hand,we have mastered now the use of histogram based techniques to color segmentation and illumination normalization[8,22,23].On the other hand,we have tested different statistical estimation paradigms to track subject candidates using not only color information but shape as well [11,12].Most of our video demonstrations from last year show results in this topic.With respect to3d object recognition and sub-ject identification,we have formalized and vali-dated a compact representation for a set of at-tributed graphs.We call this new formulation a function described graph,and it borrows the ca-pability of probablisitic modelling from random graphs.FDG’s are the result of a long time effort in the search for a compact representation of mul-tiple view model descriptors[13,14,15,16,17,18]. In the following sections we summarize the key contributions from three selected publications[1, 11,14].Each of them tackles very different prob-lems typically encountered in mobile robotics ap-plications.3SIMULTANEOUSLOCALIZATION AND MAPBUILDINGTo univocally identify landmarks from sensor data,we study several landmark representations,XXIV Jornadas de AutomáticaLeón, September 10-12, 2003, ISBN 84-931846-7-5and the mathematical foundation necessary to ex-tract the features that build them from images and laser range data.The features extracted from just one sensor may not suffice in the invariant characterization of landmarks and objects,push-ing for the combination of information from mul-tiple sources.Once landmarks are accurately extracted and identified,the second part of the problem is to use these observations for the localization of the robot,as well as the refinement of the landmark location estimates.We consider robot motion and sensor observations as stochastic processes,and treat the problem from an estimation theoretic point of view,dealing with noise by using prob-abilistic methods.The main drawback we encounter is that current estimation techniques have been devised for static environments,and that they lack robustness in more realistic situations.To aid in those situa-tions in which landmark observations might not be consistent in time,we propose a new set of temporal landmark quality functions,and show how by incorporating these functions in the data association tests,the overall estimation-theoretic approach to map building and localization is im-proved.The basic idea consists on using the his-tory of data association mismatches for the com-putation of the likelihood of future data associa-tion,together with the spatial compatibility tests already available.Special attention is paid in that the removal of spurious landmarks from the map does not vio-late the basic convergence properties of the local-ization and map building algorithms already de-scribed in the literature;namely,asymptotic con-vergence and full correlation.We contribute also an in depth analysis of the fully correlated model to localization and map building from a control systems theory point of view.Con-sidering the fact that the Kalmanfilter is nothing else but an optimal observer,we analyze the im-plications of having a state vector that is being revised by fully correlated noise measurements. We end up revealing theoretically and with exper-iments the strong limitations of using a fully cor-related noise driven estimation theoretic approach to map building and localization in relation to the total number of landmarks used.Partial observability hinders full reconstructibility of the state space,making thefinal map estimate dependant on the initial observations,and does not guarantee convergence to a positive definite covariance matrix.Partial controllability on the other hand,makes thefilter beleive after a num-ber of iterations,that it has accurate estimates of the landmark states,with their corresponding Kalman gains converging to zero.That is,after a few steps,innovations are useless.We show how to palliate the effects of full correlation and partial controllability.Any map building and localization algorithm for mobile robotics that is to work in real time must be able to relate observations and model matches in an expeditious way.Some of the landmark compatibility tests are computationally expensive, and their application has to be carefully designed. We touch upon the time complexity issues of the various landmark compatibility tests used,and also on the desirable properties of our chosen map data structure.Furthermore,we propose a series of tasks that must be handled when dealing with landmark data association.From model compati-bility tests,to search space reduction and hypoth-esis formation,to the actual association of obser-vations and models.Figure1a shows some of the model compatibil-ity heuristics devised for the validation of straight lines extracted from laser range data into walls. Frame b shows data as extracted from a laser rangefinder,and2σcovariance ellipses around hy-pothesized landmark estimates.The third frame shows a virtual reality model of the map con-structed during a run of the algorithm.4FUSION OF COLOR AND SHAPE FOR OBJECTTRACKING UNDERVARYING ILLUMINATION Color represents a visual feature commonly used for object detection and tracking systems,spe-cially in thefield of human-computer interaction. For such cases in which the environment is rela-tively simple,with controlled lighting conditions and an uncluttered background,color can be con-sidered a robust cue.The problem appears when we are dealing with scenes with varying illumina-tion conditions and confusing background.Thus,an important challenge for any color track-ing system to work in real unconstrained environ-ments,is the ability to accommodate variations in the amount of source light reflected from the tracked surface.The choice of different color spaces like HSL,nor-malized color rgb,or the color space(B−G,G−R,R+G+B),can give some robustness against varying illumination,highlights,interreflections or changes in surface orientation for an analysis of different color spaces.But none of these transfor-a)b)c)Figure 1:Concurrent mobile robot localization and map building.a)Hypothesis search range for walls extracted from a laser range scan.b)The blue dots indicate sensor raw data coming from a laser range finder.The green lines represent walls inferred from consecutive readings.The red lines indicate the estimated robot trajectory.c)Graph-ical representation of the map built.mations is general enough to cope with arbitrary changes in illumination.Instead of searching for color constancy,other ap-proaches try to adapt the color distribution over time.One such technique is to use Gaussian mixtures models to estimate densities of color,and under the assumption that lighting conditions change smoothly over time,the models are recur-sively adapted.Another option is to parameter-ize the color distribution as a random vector and to use second order Markov model to predict the evolution of the corresponding color histogram.These techniques perform much better than the mere change of color space representation,but have the drawback that they do not check for the goodness of the adaptation,which can still lead to failure.The fusion of several visual modules using differ-ent criteria offers more reliability than methods that only use one feature.As an example,sys-tems that track in real-time an individual might model the head of a person by an ellipse and use intensity gradients and color histograms to up-date the head position over time.In [12],color histograms are fused with stereovision informa-tion in order to dynamically adapt the size of the tracked head.These real time applications how-ever are constrained only to the tracking of ellip-tical shapes.A new methodology that addresses the problems present in the approaches described above,results in a robust tracking system able to cope with clut-tered scenes and varying illumination conditions.The fusion is done using the CONDENSATION algo-rithm that formulates multiple hypothesis about the estimation of the object’s color distribution and validates them taking into account the con-tour information of the object [9].Four sets of sequence results are summarized in Figure 2to illustrate the robustness of our sys-tem under different conditions.In the first ex-periment we show how our system is able to ac-commodate color by applying it over a synthetic sequence of circles moving around and changing randomly its color.In the upper left frame of the figure the path of the color distributions for the tracked circle is shown.The second experiment is to track a colored rectangle.It has to be pointed out that in the previous experiment we used the RGB color space,but in the present and subse-quent experiments the color space used was the (B −G,G −R,R +G +B )in order to provide robustness to specular higlights.The last two ex-periments,correspond to outdoor scenes,where although the change in illumination conditions is limited,they are useful to show that our methodFigure2:Results of the4experiments. works with non-uniform shapes(third experiment of a beatle tracking),and in cluttered scenarios (fourth experiment of a snail tracking).5FUNCTION-DESCRIBEDGRAPHS FOR MODELLINGOBJECTS REPRESENTED BYSETS OF ATTRIBUTEDGRAPHSA function-described graph(FDG)is a model that contains probabilistic and structural descriptions of a set of attributed graphs(AGs)to maintain,to the most,the local features of the AGs that belong to the set and other AGs that are“near”them,as well as to allow the rejection of the AGs that do not belong to it or are“far”of them.Let us con-sider,as an example,the3D-object modelling and recognition problem.The basic idea is that only a single FDG is synthesised from the graphs that represent several views of a3D-object.Therefore, in the recognition process,only one comparison is needed between each model represented by an FDG and the unclassified object(view of a3D-object)represented by a graph.Random graphs are on the other hand,one of the earliest approaches used to represent a set of AGs. In this approach,AGs are extended to include probabilistic information.Wong et al.first de-fined the General Random Graphs(GRGs)for modelling classes of patterns described by AGs through a joint probability space of random vari-ables ranging over pattern primitives(vertices)and relations(arcs).Due to the computational intractability of GRGs,caused by the difficulty in estimating and handling the high-order joint prob-ability distribution,First-Order Random Graphs (FORGs)were proposed for real applications. Strong simplifications were made in FORGs to al-low the use of random graphs in practical cases; more precisely,the following assumptions were made:a)the random vertices are mutually inde-pendent;b)given values for the random vertices, the random arcs are independent;c)the arcs are independent of the vertices except for the vertices that they connect.FDGs can be seen as a type of simplification of the GRGs,different from FORGs,in which some structural constraints are recorded.A drawback of FORGs is that the strong assumptions about the statistical independence of nodes and arcs may lead to an excessive generalisation of the sample graphs when synthesising a FORG.To alleviate this weakness,a qualitative information of the joint probabilities of two nodes is incorporated into FDGs,thus improving the representational power of FORGs with a negligible increase of com-putational cost.5.1APPLICATION OF FDGS FORMODELLING ANDRECOGNITION OF OBJECTS FDGs are applied here to3D-object representa-tion and recognition.The attribute of the vertices is the average hue of the region(cyclic range from 0to49)and the attribute of the edges is the differ-ence between the colours of the two neighbouring regions.Wefirst present an experimental validation of FDGs using artificial3D-objects in which the ad-jacency graphs have been extracted manually and afterwards we present a real application on an image database in which the graphs have been extracted automatically.The advantages of the experimental validation are that the results do not depend on the segmentation process and that we can use a supervised synthesis,since we know which vertices of the AGs represent the same pla-nar face of the object.Thus,we can discern be-tween the effects of computing a distance measure using different values of the costs on the2nd-order relations.In the real application,we show the capacity of FDGs to keep the structural and se-mantic knowledge of an object despite the noise introduced by the segmentation process and an automatic synthesis.Figure3:10views of5artificial objects designed with a CAD program.5.1.1SUPER VISED SYNTHESIS ONARTIFICIAL OBJECTSWe designedfive objects using a CAD program (see Figure3).After that,we tookfive sets of views from these objects and from these views we extracted a total of101adjacency graphs.To ob-tain the AGs of the test set and of the reference set,we modified the attribute values of the ver-tices and arcs of the adjacency graphs by adding zero-mean Gausian noise with different variances. Moreover,some vertices and arcs where inserted and deleted randomly in some cases.The FDGs were synthesised using the AGs that belonged to the same3D-object and using the synthesis given a common labelling from a set of AGs described in.Table1shows the ratio of correctness for different levels of noise and applying several costs on the an-tagonisms and occurrences.We see that the bests results appear always when we use moderate2nd-order costs.Furthermore,when noise increases, the recognition ratio decreases drastically when we use high costs but there is only a slight decrease when we use moderate costs.Moreover,in Table 2we compare the FDGs(with2nd-order costs)to other two methods.The FDG classifier always ob-tains better results than the3-Nearest Neighbours and the Random Graph classifiers.The differ-ence of the ratio between FDGs and the other two methods increases when the noise also increases. FORGs obtain better results than the3-N.N.only when the noise is high.5.1.2UNSUPER VISED SYNTHESISON REAL LIFE OBJECTSImages were extracted from the database COIL-100from Columbia University.It is composed of 100isolated objects and for each object there are 72views(one view each5degrees).Adjacency graphs are obtained by color segmentation.Fig-ure4shows20objects at angle100and their seg-mented images with the adjacency graphs.The test set was composed by36views per object (taken at the angles0,10,20and so on),whereas the reference set was composed by the36remain-ing views(taken at the angles5,15,25and so on).FDGs were synthesised automatically using the AGs in the reference set that represent the same object.The method of incremental synthe-sis,in which the FDGs are updated while new AGs are sequentially presented,was applied.We made6different experiments in which the number of FDGs that represents each3D-object varied.If the3D-object was represented by only one FDG, the36AGs from the reference set that represent the3D-object were used to synthesise the FDG.If it was represented by2FDGs,the18first and con-secutive AGs from the reference set were used to synthesise one of the FDGs and the other18AGs were used to synthesise the other FDG.A similar method was used for the other experiments with 3,4,6and9FDGs per3D-object.Similarly to the previous experimental results,the correctness is higher when2nd-order relations are used with a moderate cost.The best result ap-pears when each object is represented by4FDGs, that is,each FDG represents90degrees of the3D-object.When objects are represented by9FDGs, each FDG represents40degrees of the3D-object and4AGs per FDG,there is poor probabilistic knowledge and therefore the costs on the vertices and arcs are coarse.Moreover,when objects are represented by only1or2FDGs,there are too much spurious regions(produced in the segmenta-tion process)to keep the structural and semantic knowledge of the object.References[1]J.Andrade-Cetto.Environment Learning forIndoor Mobile Robots.PhD thesis,UPC, Barcelona,Apr.2003.[2]J.Andrade-Cetto and A.Sanfeliu.Concur-rent map building and localization on in-door dynamic environments.Int.J.Pattern Recogn.,16(3):361–374,May2002.[3]J.Andrade-Cetto and A.Sanfeliu.Concur-rent map building and localization with land-mark validation.In Proc.16th IAPR Int.Conf.Pattern Recog.,volume2,pages693–696,Quebec,Aug.2002.IEEE Comp.Soc.[4]J.Andrade-Cetto and A.Sanfeliu.Concur-rent map building and localization with tem-poral landmark validation.Accepted for pre-sentation in2003IEEE International Confer-ence on Robotics and Automation,2003.Table1:FDGs ratio of correctness.Num.Vertices Ins&Del00000121Standard Deviation024812008Cost Antag Cost OccuModerate Moderate10098979592898583High Null10092898784615457Null High10091898885625959High High10095908680605356Moderate Null10092919187807575Null Moderate10095929186817776Null Null10090898886706768Table2:FDGs(moderate2nd order costs),FORGs and3-NN ratio of correctness.Num Vertices Ins.or Del.00000121Standard Deviation024812008FDGs(Moderate costs)10098979592898583Random Graphs(FORGs)100908988867067683-N.N.(Edit Op.Distance)10098826252905858[5]Canal Blau TV.The IRI Robots in CanalBlau,May2002.[6]A.Checa,J.Andrade,and A.Sanfeliu.Con-strucci´o de mapes per robots m´o bils equipats amb sensors l`a ser de profunditat.Technical Report IRI-DT-03-01,IRI,UPC,Mar.2003.[7]El Peri´o dico de Catalunya.El RobotDom´e stico Llama a la Puerta,Apr.2002.[8]A.Grau,J.Climent, F.Serratosa,andA.Sanfeliu.Texprint:A new algorithmto discriminate textures structurally.In T.Caelli,A.Amin,R.P.W.Duin,M.Kamel, and D.de Ridder,editors,Proc.IAPR Int.Workshop Syntactical Structural Pattern Recog.,volume2396of Lect.Notes Comp.Sci.,pages368–377,Windsor,Aug.2002.Springer-Verlag.[9]M.Isard and A.Blake.Condensation-con-ditional density propagation for visual put.Vision,29(1):5–28,Aug.1998.[10]La Polit`e cnica,Num 4.UPC.CriaturesCibern`e tiques,Nov.2001.[11]F.Moreno,J.Andrade-Cetto,and A.San-feliu.Fusion of color and shape for ob-ject tracking under varying illumination.InF.J.Perales,A.J.C.Campilho,N.P.dela Blanca,and A.Sanfeliu,editors,Proc.1st Iberian Conf.Pattern Recog.Image Anal., volume2652of Lect.Notes Comp.Sci.,pages 580–588,Puerto de Andratx,Jun.2003.Springer-Verlag.[12]F.Moreno, A.Tarrida,J.Andrade-Cetto,and A.Sanfeliu.3d real-time head tracking fusing color histograms and stereovision.In Proc.16th IAPR Int.Conf.Pattern Recog., volume1,pages368–371,Quebec,Aug.2002.IEEE Comp.Soc.[13]A.Sanfeliu,R.Alqu´e zar,J.Andrade,J.Cli-ment,F.Serratosa,and J.Verg´e s.Graph-based representations and techniques for im-age processing and image analysis.Pattern Recogn.,35:639–650,Mar.2002.[14]F.Serratosa,R.Alqu´e zar,and A.Sanfeliu.Function-described graphs for modelling ob-jects represented by sets of attributed graphs.Pattern Recognition,volume36,pages781–798,2003.[15]F.Serratosa,R.Alqu´e zar,and A.Sanfe-liu.Estimating the joint probability dis-tribution of random vertices and arcs by means of second-order random graphs.In T.Caelli,A.Amin,R.P.W.Duin,M.Kamel, and D.de Ridder,editors,Proc.IAPR Int.Workshop Syntactical Structural Pattern Recog.,volume2396of Lect.Notes Comp.Sci.,pages252–260,Windsor,Aug.2002.Springer-Verlag.[16]F.Serratosa,R.Alqu´e zar,and A.Sanfeliu.Synthesis of function-described graphs and clustering of attributed graphs.Int.J.Pat-tern Recogn.,16(6):621–655,2002.[17]F.Serratosa,A.Sanfeliu,and R.Alqu´e zar.Modelling and recognizing3d-objects de-Figure4:The20selected objects and the segmented images with the AGs.scribed by multiple views using function-described graphs.In Proc.16th IAPR Int.Conf.Pattern Recog.,volume2,pages140–143,Quebec,Aug.2002.IEEE Comp.Soc.[18]E.Staffetti, A.Grau, F.Serratosa,andA.Sanfeliu.Oriented matroids for shape rep-resentation and indexing.In F.J.Perales,A.J.C.Campilho,N.P.de la Blanca,andA.Sanfeliu,editors,Proc.1st Iberian Conf.Pattern Recog.Image Anal.,volume2652of Lect.Notes Comp.Sci.,pages1012–1019, Puerto de Andratx,Jun.2003.Springer-Verlag.[19]Televisi´o de Catalunya.Robot Casol`a,May2003.[20]Televisi´o n Espa˜n rme Semanal,Jan.2002.[21]UPC Libraries.More Than Just Machines,Jan.2003.[22]J.Verg´e s-Llah´ıand A.Sanfeliu.Colour con-stancy algorithm based on the minimization of the distance between colour histograms.In F.J.Perales,A.J.C.Campilho,N.P.de la Blanca,and A.Sanfeliu,editors,Proc.1st Iberian Conf.Pattern Recog.Image Anal., volume2652of Lect.Notes Comp.Sci.,pages 1066–1073,Puerto de Andratx,Jun.2003.Springer-Verlag.[23]J.Verg´e s-Llah´ı,A.Tarrida,and A.Sanfeliu.New approaches to colour histogram adap-tation in face tracking tasks.In Proc.16th IAPR Int.Conf.Pattern Recog.,pages381–384,Quebec,Aug.2002.IEEE Comp.Soc.。