美赛特等奖原版论文集--37075
7315A-国际一等奖

V2b , 惯性矩 MOI, 恢复系数 COR, 球棒的质量,
击球的位置, 不能仅仅利用一个力矩的理论或者自 己的印象在头脑中简单的得出结论。 我们将在第 III 部分展开讨论。
图 1 球-棒碰撞前后的速度变化示意图 第二个方面是能量问题。原始的撞击前的能量 在撞击过程中和撞击后变成了很多种形式的能量:例 如球最终的动能,刚体模式中损耗的能量(棒平移和 自转过程中损耗的能量),球棒的振动能量,球与棒 撞击挤压过程中损耗的能量,以及由弹簧效应重新产 生的一些能量(只有金属棒中才考虑)。如上所述, 最终能量转化成了很多种形式,不仅仅包括球最终的 能量,所以我们不能简单的决定最佳击球点的位置, 理论的基础,实验中的数据以及模 型的调整都是必不会可少的,这些 问题将在第五部分予以讨论。
Team # 7315
Page 3 of 19
3.3.3 结果与分析……………………………..…………………….……………………………..……………...17 3.3.4优缺点分析…………………………….………………………….……………………..…………...….18 4.结论.............................................................................................. ..................................................................... 19 5. 未来的工作................................................................................................................................................ 19 5.1 其他分析问题的方法?.................................................................................................................19 5.2 其他方法的局限性
美国大学生数学建模竞赛优秀论文
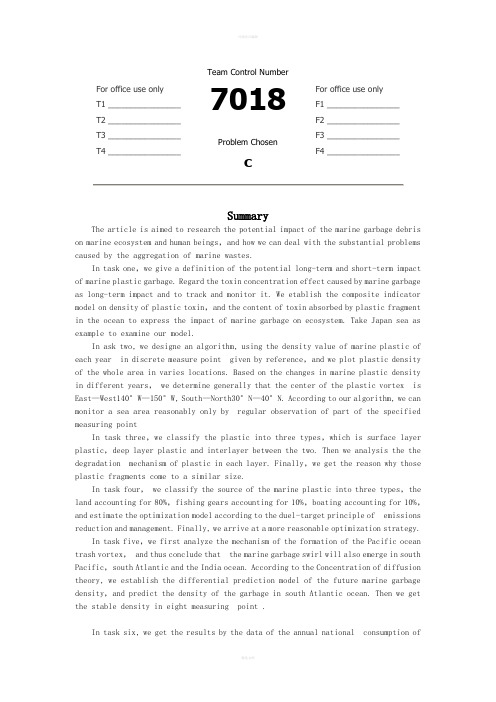
For office use onlyT1________________ T2________________ T3________________ T4________________Team Control Number7018Problem ChosencFor office use onlyF1________________F2________________F3________________F4________________ SummaryThe article is aimed to research the potential impact of the marine garbage debris on marine ecosystem and human beings,and how we can deal with the substantial problems caused by the aggregation of marine wastes.In task one,we give a definition of the potential long-term and short-term impact of marine plastic garbage. Regard the toxin concentration effect caused by marine garbage as long-term impact and to track and monitor it. We etablish the composite indicator model on density of plastic toxin,and the content of toxin absorbed by plastic fragment in the ocean to express the impact of marine garbage on ecosystem. Take Japan sea as example to examine our model.In ask two, we designe an algorithm, using the density value of marine plastic of each year in discrete measure point given by reference,and we plot plastic density of the whole area in varies locations. Based on the changes in marine plastic density in different years, we determine generally that the center of the plastic vortex is East—West140°W—150°W, South—North30°N—40°N. According to our algorithm, we can monitor a sea area reasonably only by regular observation of part of the specified measuring pointIn task three,we classify the plastic into three types,which is surface layer plastic,deep layer plastic and interlayer between the two. Then we analysis the the degradation mechanism of plastic in each layer. Finally,we get the reason why those plastic fragments come to a similar size.In task four, we classify the source of the marine plastic into three types,the land accounting for 80%,fishing gears accounting for 10%,boating accounting for 10%,and estimate the optimization model according to the duel-target principle of emissions reduction and management. Finally, we arrive at a more reasonable optimization strategy.In task five,we first analyze the mechanism of the formation of the Pacific ocean trash vortex, and thus conclude that the marine garbage swirl will also emerge in south Pacific,south Atlantic and the India ocean. According to the Concentration of diffusion theory, we establish the differential prediction model of the future marine garbage density,and predict the density of the garbage in south Atlantic ocean. Then we get the stable density in eight measuring point .In task six, we get the results by the data of the annual national consumption ofpolypropylene plastic packaging and the data fitting method, and predict the environmental benefit generated by the prohibition of polypropylene take-away food packaging in the next decade. By means of this model and our prediction,each nation will reduce releasing 1.31 million tons of plastic garbage in next decade.Finally, we submit a report to expediction leader,summarize our work and make some feasible suggestions to the policy- makers.Task 1:Definition:●Potential short-term effects of the plastic: the hazardeffects will be shown in the short term.●Potential long-term effects of the plastic: thepotential effects, of which hazards are great, willappear after a long time.The short- and long-term effects of the plastic on the ocean environment:In our definition, the short-term and long-term effects of the plastic on the ocean environment are as follows.Short-term effects:1)The plastic is eaten by marine animals or birds.2) Animals are wrapped by plastics, such as fishing nets, which hurt or even kill them.3)Deaden the way of the passing vessels.Long-term effects:1)Enrichment of toxins through the food chain: the waste plastic in the ocean has no natural degradation in theshort-term, which will first be broken down into tinyfragments through the role of light, waves,micro-organisms, while the molecular structure has notchanged. These "plastic sands", easy to be eaten byplankton, fish and other, are Seemingly very similar tomarine life’s food,causing the enrichment and delivery of toxins.2)Accelerate the greenhouse effect: after a long-term accumulation and pollution of plastics, the waterbecame turbid, which will seriously affect the marineplants (such as phytoplankton and algae) inphotosynthesis. A large number of plankton’s deathswould also lower the ability of the ocean to absorbcarbon dioxide, intensifying the greenhouse effect tosome extent.To monitor the impact of plastic rubbish on the marine ecosystem:According to the relevant literature, we know that plastic resin pellets accumulate toxic chemicals , such as PCBs、DDE , and nonylphenols , and may serve as a transport medium and soure of toxins to marine organisms that ingest them[]2. As it is difficult for the plastic garbage in the ocean to complete degradation in the short term, the plastic resin pellets in the water will increase over time and thus absorb more toxins, resulting in the enrichment of toxins and causing serious impact on the marine ecosystem.Therefore, we track the monitoring of the concentration of PCBs, DDE, and nonylphenols containing in the plastic resin pellets in the sea water, as an indicator to compare the extent of pollution in different regions of the sea, thus reflecting the impact of plastic rubbish on ecosystem.To establish pollution index evaluation model: For purposes of comparison, we unify the concentration indexes of PCBs, DDE, and nonylphenols in a comprehensive index.Preparations:1)Data Standardization2)Determination of the index weightBecause Japan has done researches on the contents of PCBs,DDE, and nonylphenols in the plastic resin pellets, we illustrate the survey conducted in Japanese waters by the University of Tokyo between 1997 and 1998.To standardize the concentration indexes of PCBs, DDE,and nonylphenols. We assume Kasai Sesside Park, KeihinCanal, Kugenuma Beach, Shioda Beach in the survey arethe first, second, third, fourth region; PCBs, DDE, andnonylphenols are the first, second, third indicators.Then to establish the standardized model:j j jij ij V V V V V min max min --= (1,2,3,4;1,2,3i j ==)wherej V max is the maximum of the measurement of j indicator in the four regions.j V min is the minimum of the measurement of j indicatorstandardized value of j indicator in i region.According to the literature [2], Japanese observationaldata is shown in Table 1.Table 1. PCBs, DDE, and, nonylphenols Contents in Marine PolypropyleneTable 1 Using the established standardized model to standardize, we have Table 2.In Table 2,the three indicators of Shioda Beach area are all 0, because the contents of PCBs, DDE, and nonylphenols in Polypropylene Plastic Resin Pellets in this area are the least, while 0 only relatively represents the smallest. Similarly, 1 indicates that in some area the value of a indicator is the largest.To determine the index weight of PCBs, DDE, and nonylphenolsWe use Analytic Hierarchy Process (AHP) to determine the weight of the three indicators in the general pollution indicator. AHP is an effective method which transforms semi-qualitative and semi-quantitative problems into quantitative calculation. It uses ideas of analysis and synthesis in decision-making, ideally suited for multi-index comprehensive evaluation.Hierarchy are shown in figure 1.Fig.1 Hierarchy of index factorsThen we determine the weight of each concentrationindicator in the generall pollution indicator, and the process are described as follows:To analyze the role of each concentration indicator, we haveestablished a matrix P to study the relative proportion.⎥⎥⎥⎦⎤⎢⎢⎢⎣⎡=111323123211312P P P P P P P Where mn P represents the relative importance of theconcentration indicators m B and n B . Usually we use 1,2,…,9 and their reciprocals to represent different importance. The greater the number is, the more important it is. Similarly, the relative importance of m B and n B is mn P /1(3,2,1,=n m ).Suppose the maximum eigenvalue of P is m ax λ, then theconsistency index is1max --=n nCI λThe average consistency index is RI , then the consistencyratio isRICI CR = For the matrix P of 3≥n , if 1.0<CR the consistency isthougt to be better, of which eigenvector can be used as the weight vector.We get the comparison matrix accoding to the harmful levelsof PCBs, DDE, and nonylphenols and the requirments ofEPA on the maximum concentration of the three toxins inseawater as follows:⎥⎥⎥⎦⎤⎢⎢⎢⎣⎡=165416131431P We get the maximum eigenvalue of P by MATLAB calculation0012.3max =λand the corresponding eigenvector of it is()2393.02975.09243.0,,=W1.0042.012.1047.0<===RI CI CR Therefore,we determine the degree of inconsistency formatrix P within the permissible range. With the eigenvectors of p as weights vector, we get thefinal weight vector by normalization ()1638.02036.06326.0',,=W . Defining the overall target of pollution for the No i oceanis i Q , among other things the standardized value of threeindicators for the No i ocean is ()321,,i i i i V V V V = and the weightvector is 'W ,Then we form the model for the overall target of marine pollution assessment, (3,2,1=i )By the model above, we obtained the Value of the totalpollution index for four regions in Japanese ocean in Table 3T B W Q '=In Table3, the value of the total pollution index is the hightest that means the concentration of toxins in Polypropylene Plastic Resin Pellets is the hightest, whereas the value of the total pollution index in Shioda Beach is the lowest(we point up 0 is only a relative value that’s not in the name of free of plastics pollution)Getting through the assessment method above, we can monitor the concentration of PCBs, DDE and nonylphenols in the plastic debris for the sake of reflecting the influence to ocean ecosystem.The highter the the concentration of toxins,the bigger influence of the marine organism which lead to the inrichment of food chain is more and more dramatic.Above all, the variation of toxins’ concentration simultaneously reflects the distribution and time-varying of marine litter. We can predict the future development of marine litter by regularly monitoring the content of these substances, to provide data for the sea expedition of the detection of marine litter and reference for government departments to make the policies for ocean governance.Task 2:In the North Pacific, the clockwise flow formed a never-ending maelstrom which rotates the plastic garbage. Over the years, the subtropical eddy current in North Pacific gathered together the garbage from the coast or the fleet, entrapped them in the whirlpool, and brought them to the center under the action of the centripetal force, forming an area of 3.43 million square kilometers (more than one-third of Europe) .As time goes by, the garbage in the whirlpool has the trend of increasing year by year in terms of breadth, density, and distribution. In order to clearly describe the variability of the increases over time and space, according to “Count Densities of Plastic Debris from Ocean Surface Samples North Pacific Gyre 1999—2008”, we analyze the data, exclude them with a great dispersion, and retain them with concentrated distribution, while the longitude values of the garbage locations in sampled regions of years serve as the x-coordinate value of a three-dimensional coordinates, latitude values as the y-coordinate value, the Plastic Count per cubic Meter of water of the position as the z-coordinate value. Further, we establish an irregular grid in the yx plane according to obtained data, and draw a grid line through all the data points. Using the inverse distance squared method with a factor, which can not only estimate the Plastic Count per cubic Meter of water of any position, but also calculate the trends of the Plastic Counts per cubic Meter of water between two original data points, we can obtain the unknown grid points approximately. When the data of all the irregular grid points are known (or approximately known, or obtained from the original data), we can draw the three-dimensional image with the Matlab software, which can fully reflect the variability of the increases in the garbage density over time and space.Preparations:First, to determine the coordinates of each year’s sampled garbage.The distribution range of garbage is about the East - West 120W-170W, South - North 18N-41N shown in the “Count Densities of Plastic Debris from Ocean Surface Samples North Pacific Gyre 1999--2008”, we divide a square in the picture into 100 grids in Figure (1) as follows:According to the position of the grid where the measuring point’s center is, we can identify the latitude and longitude for each point, which respectively serve as the x- and y- coordinate value of the three-dimensional coordinates.To determine the Plastic Count per cubic Meter of water. As the “Plastic Count per cubic Meter of water” provided by “Count Densities of P lastic Debris from Ocean Surface Samples North Pacific Gyre 1999--2008”are 5 density interval, to identify the exact values of the garbage density of one year’s different measuring points, we assume that the density is a random variable which obeys uniform distribution in each interval.Uniform distribution can be described as below:()⎪⎩⎪⎨⎧-=01a b x f ()others b a x ,∈We use the uniform function in Matlab to generatecontinuous uniformly distributed random numbers in each interval, which approximately serve as the exact values of the garbage density andz-coordinate values of the three-dimensional coordinates of the year’s measuring points.Assumptions(1)The data we get is accurate and reasonable.(2)Plastic Count per cubic Meter of waterIn the oceanarea isa continuous change.(3)Density of the plastic in the gyre is a variable by region.Density of the plastic in the gyre and its surrounding area is interdependent , However, this dependence decreases with increasing distance . For our discussion issue, Each data point influences the point of each unknown around and the point of each unknown around is influenced by a given data point. The nearer a given data point from the unknown point, the larger the role.Establishing the modelFor the method described by the previous,we serve the distributions of garbage density in the “Count Pensities of Plastic Debris from Ocean Surface Samples North Pacific Gyre 1999--2008”as coordinates ()z y,, As Table 1:x,Through analysis and comparison, We excluded a number of data which has very large dispersion and retained the data that is under the more concentrated the distribution which, can be seen on Table 2.In this way, this is conducive for us to get more accurate density distribution map.Then we have a segmentation that is according to the arrangement of the composition of X direction and Y direction from small to large by using x co-ordinate value and y co-ordinate value of known data points n, in order to form a non-equidistant Segmentation which has n nodes. For the Segmentation we get above,we only know the density of the plastic known n nodes, therefore, we must find other density of the plastic garbage of n nodes.We only do the sampling survey of garbage density of the north pacificvortex,so only understand logically each known data point has a certain extent effect on the unknown node and the close-known points of density of the plastic garbage has high-impact than distant known point.In this respect,we use the weighted average format, that means using the adverse which with distance squared to express more important effects in close known points. There're two known points Q1 and Q2 in a line ,that is to say we have already known the plastic litter density in Q1 and Q2, then speculate the plastic litter density's affects between Q1、Q2 and the point G which in the connection of Q1 and Q2. It can be shown by a weighted average algorithm22212221111121GQ GQ GQ Z GQ Z Z Q Q G +*+*=in this formula GQ expresses the distance between the pointG and Q.We know that only use a weighted average close to the unknown point can not reflect the trend of the known points, we assume that any two given point of plastic garbage between the changes in the density of plastic impact the plastic garbage density of the unknown point and reflecting the density of plastic garbage changes in linear trend. So in the weighted average formula what is in order to presume an unknown point of plastic garbage density, we introduce the trend items. And because the greater impact at close range point, and thus the density of plastic wastes trends close points stronger. For the one-dimensional case, the calculation formula G Z in the previous example modify in the following format:2212122212212122211111112121Q Q GQ GQ GQ Q Q GQ Z GQ Z GQ Z Z Q Q Q Q G ++++*+*+*=Among them, 21Q Q known as the separation distance of the known point, 21Q Q Z is the density of plastic garbage which is the plastic waste density of 1Q and 2Q for the linear trend of point G . For the two-dimensional area, point G is not on the line 21Q Q , so we make a vertical from the point G and cross the line connect the point 1Q and 2Q , and get point P , the impact of point P to 1Q and 2Q just like one-dimensional, and the one-dimensional closer of G to P , the distant of G to P become farther, the smaller of the impact, so the weighting factor should also reflect the GP in inversely proportional to a certain way, then we adopt following format:221212222122121222211111112121Q Q GQ GP GQ GQ Q Q GQ GP Z GQ Z GQ Z Z P Q Q Q Q G ++++++*+*+*=Taken together, we speculated following roles:(1) Each known point data are influence the density of plastic garbage of each unknown point in the inversely proportional to the square of the distance;(2) the change of density of plastic garbage between any two known points data, for each unknown point are affected, and the influence to each particular point of their plastic garbage diffuse the straight line along the two known particular point; (3) the change of the density of plastic garbage between any two known data points impact a specific unknown points of the density of plastic litter depends on the three distances: a. the vertical distance to a straight line which is a specific point link to a known point;b. the distance between the latest known point to a specific unknown point;c. the separation distance between two known data points.If we mark 1Q ,2Q ,…,N Q as the location of known data points,G as an unknown node, ijG P is the intersection of the connection of i Q ,j Q and the vertical line from G to i Q ,j Q()G Q Q Z j i ,,is the density trend of i Q ,j Q in the of plasticgarbage points and prescribe ()G Q Q Z j i ,,is the testing point i Q ’ s density of plastic garbage ,so there are calculation formula:()()∑∑∑∑==-==++++*=Ni N ij ji i ijGji i ijG N i Nj j i G Q Q GQ GPQ Q GQ GP G Q Q Z Z 11222222111,,Here we plug each year’s observational data in schedule 1 into our model, and draw the three-dimensional images of the spatial distribution of the marine garbage ’s density with Matlab in Figure (2) as follows:199920002002200520062007-2008(1)It’s observed and analyzed that, from 1999 to 2008, the density of plastic garbage is increasing year by year and significantly in the region of East – West 140W-150W, south - north 30N-40N. Therefore, we can make sure that this region is probably the center of the marine litter whirlpool. Gathering process should be such that the dispersed garbage floating in the ocean move with the ocean currents and gradually close to the whirlpool region. At the beginning, the area close to the vortex will have obviously increasable about plastic litter density, because of this centripetal they keeping move to the center of the vortex ,then with the time accumulates ,the garbage density in the center of the vortex become much bigger and bigger , at last it becomes the Pacific rubbish island we have seen today.It can be seen that through our algorithm, as long as the reference to be able to detect the density in an area which has a number of discrete measuring points,Through tracking these density changes ,we Will be able to value out all the waters of the density measurement through our models to determine,This will reduce the workload of the marine expedition team monitoring marine pollution significantly, and also saving costs .Task 3:The degradation mechanism of marine plasticsWe know that light, mechanical force, heat, oxygen, water, microbes, chemicals, etc. can result in the degradation of plastics . In mechanism ,Factors result in the degradation can be summarized as optical ,biological,and chemical。
2010 美赛 MCM 优秀论文

3 Center of Minimum Distance Model.................................. 5
2012年美国高中生数学建模竞赛特等奖论文

题目:How Much Gas Should I Buy This Week?题目来源:2012年第十五届美国高中生数学建模竞赛(HiMCM)B题获奖等级:特等奖,并授予INFORMS奖论文作者:深圳中学2014届毕业生李依琛、王喆沛、林桂兴、李卓尔指导老师:深圳中学张文涛AbstractGasoline is the bleed that surges incessantly within the muscular ground of city; gasoline is the feast that lures the appetite of drivers. “To fill or not fill?” That is the question flustering thousands of car owners. This paper will guide you to predict the gasoline prices of the coming week with the currently available data with respect to swift changes of oil prices. Do you hold any interest in what pattern of filling up the gas tank can lead to a lower cost in total?By applying the Time series analysis method, this paper infers the price in the imminent week. Furthermore, we innovatively utilize the average prices of the continuous two weeks to predict the next two week’s average price; similarly, employ the four-week-long average prices to forecast the average price of four weeks later. By adopting the data obtained from 2011and the comparison in different aspects, we can obtain the gas price prediction model :G t+1=0.0398+1.6002g t+−0.7842g t−1+0.1207g t−2+ 0.4147g t−0.5107g t−1+0.1703g t−2+ε .This predicted result of 2012 according to this model is fairly ideal. Based on the prediction model,We also establish the model for how to fill gasoline. With these models, we had calculated the lowest cost of filling up in 2012 when traveling 100 miles a week is 637.24 dollars with the help of MATLAB, while the lowest cost when traveling 200 miles a week is 1283.5 dollars. These two values are very close to the ideal value of cost on the basis of the historical figure, which are 635.24 dollars and 1253.5 dollars respectively. Also, we have come up with the scheme of gas fulfillment respectively. By analyzing the schemes of gas filling, we can discover that when you predict the future gasoline price going up, the best strategy is to fill the tank as soon as possible, in order to lower the gas fare. On the contrary, when the predicted price tends to decrease, it is wiser and more economic for people to postpone the filling, which encourages people to purchase a half tank of gasoline only if the tank is almost empty.For other different pattern for every week’s “mileage driven”, we calculate the changing point of strategies-changed is 133.33 miles.Eventually, we will apply the models -to the analysis of the New York City. The result of prediction is good enough to match the actual data approximately. However, the total gas cost of New York is a little higher than that of the average cost nationally, which might be related to the higher consumer price index in the city. Due to the limit of time, we are not able to investigate further the particular factors.Keywords: gasoline price Time series analysis forecast lowest cost MATLABAbstract ---------------------------------------------------------------------------------------1 Restatement --------------------------------------------------------------------------------------21. Assumption----------------------------------------------------------------------------------42. Definitions of Variables and Models-----------------------------------------------------4 2.1 Models for the prediction of gasoline price in the subsequent week------------4 2.2 The Model of oil price next two weeks and four weeks--------------------------5 2.3 Model for refuel decision-------------------------------------------------------------52.3.1 Decision Model for consumer who drives 100 miles per week-------------62.3.2 Decision Model for consumer who drives 200 miles per week-------------73. Train and Test Model by 2011 dataset---------------------------------------------------8 3.1 Determine the all the parameters in Equation ② from the 2011 dataset-------8 3.2 Test the Forecast Model of gasoline price by the dataset of gasoline price in2012-------------------------------------------------------------------------------------10 3.3 Calculating ε --------------------------------------------------------------------------12 3.4 Test Decision Models of buying gasoline by dataset of 2012-------------------143.4.1 100 miles per week---------------------------------------------------------------143.4.2 200 miles per week---------------------------------------------------------------143.4.3 Second Test for the Decision of buying gasoline-----------------------------154. The upper bound will change the Decision of buying gasoline---------------------155. An analysis of New York City-----------------------------------------------------------16 5.1 The main factor that will affect the gasoline price in New York City----------16 5.2 Test Models with New York data----------------------------------------------------185.3 The analysis of result------------------------------------------------------------------196. Summery& Advantage and disadvantage-----------------------------------------------197. Report----------------------------------------------------------------------------------------208. Appendix------------------------------------------------------------------------------------21 Appendix 1(main MATLAB programs) ------------------------------------------------21 Appendix 2(outcome and graph) --------------------------------------------------------34The world market is fluctuating swiftly now. As the most important limited energy, oil is much accounted of cars owners and dealer. We are required to make a gas-buying plan which relates to the price of gasoline, the volume of tank, the distance that consumer drives per week, the data from EIA and the influence of other events in order to help drivers to save money.We should use the data of 2011 to build up two models that discuss two situations: 100miles/week or 200miles/week and use the data of 2012 to test the models to prove the model is applicable. In the model, consumer only has three choices to purchase gas each week, including no gas, half a tank and full tank. At the end, we should not only build two models but also write a simple but educational report that can attract consumer to follow this model.1.Assumptiona)Assume the consumer always buy gasoline according to the rule of minimumcost.b)Ignore the difference of the gasoline weight.c)Ignore the oil wear on the way to gas stations.d)Assume the tank is empty at the beginning of the following models.e)Apply the past data of crude oil price to predict the future price ofgasoline.(The crude oil price can affect the gasoline price and we ignore thehysteresis effect on prices of crude oil towards prices of gasoline.)2.Definitions of Variables and Modelst stands for the sequence number of week in any time.(t stands for the current week. (t-1) stands for the last week. (t+1) stands for the next week.c t: Price of crude oil of the current week.g t: Price of gasoline of the t th week.P t: The volume of oil of the t th week.G t+1: Predicted price of gasoline of the (t+1)th week.α,β: The coefficient of the g t and c t in the model.d: The variable of decision of buying gasoline.(d=1/2 stands for buying a half tank gasoline)2.1 Model for the prediction of gasoline price in the subsequent weekWhether to buy half a tank oil or full tank oil depends on the short-term forecast about the gasoline prices. Time series analysis is a frequently-used method to expect the gasoline price trend. It can be expressed as:G t+1=α1g t+α2g t−1+α3g t−2+α4g t−3+…αn+1g t−n+ε ----Equation ①ε is a parameter that reflects the influence towards the trend of gasoline price in relation to several aspects such as weather data, economic data, world events and so on.Due to the prices of crude oil can influence the future prices of gasoline; we will adopt the past prices of crude oil into the model for gasoline price forecast.G t+1=(α1g t+α2g t−1+α3g t−2+α4g t−3+⋯αn+1g t−n)+(β1g t+β2g t−1+β3g t−2+β4g t−3+⋯βn+1g t−n)+ε----Equation ②We will use the 2011 data set to calculate the all coefficients and the best delay periods n.2.2 The Model of oil price next two weeks and four weeksWe mainly depend on the prediction of change of gasoline price in order to make decision that the consumer should buy half a tank or full tank gas. When consumer drives 100miles/week, he can drive whether 400miles most if he buys full tank gas or 200miles most if he buys half a tank gas. When consumer drives 200miles/week, full tank gas can be used two weeks most or half a tank can be used one week most. Thus, we should consider the gasoline price trend in four weeks in future.Equation ②can also be rewritten asG t+1=(α1g t+β1g t)+(α2g t−1+β2g t−1)+(α3g t−2+β3g t−2)+⋯+(αn+1g t−n+βn+1g t−n)+ε ----Equation ③If we define y t=α1g t+β1g t,y t−1=α2g t−1+β2g t−1, y t−2=α3g t−2+β3g t−2……, and so on.Equation ③can change toG t+1=y t+y t−1+y t−2+⋯+y t−n+ε ----Equation ④We use y(t−1,t)denote the average price from week (t-1) to week (t), which is.y(t−1,t)=y t−1+y t2Accordingly, the average price from week (t-3) to week (t) isy(t−3,t)=y t−3+y t−2+y t−1+y t.4Apply Time series analysis, we can get the average price from week (t+1) to week (t+2) by Equation ④,G(t+1,t+2)=y(t−1,t)+y(t−3,t−2)+y(t−5,t−4), ----Equation ⑤As well, the average price from week (t+1) to week (t+4) isG(t+1,t+4)=y(t−3,t)+y(t−7,t−4)+y(t−11,t−8). ----Equation ⑥2.3 Model for refuel decisionBy comparing the present gasoline price with the future price, we can decide whether to fill half or full tank.The process for decision can be shown through the following flow chart.Chart 1For the consumer, the best decision is to get gasoline with the lowest prices. Because a tank of gasoline can run 2 or 4 week, so we should choose a time point that the price is lowest by comparison of the gas prices at present, 2 weeks and 4 weeks later separately. The refuel decision also depends on how many free spaces in the tank because we can only choose half or full tank each time. If the free spaces are less than 1/2, we can refuel nothing even if we think the price is the lowest at that time.2.3.1 Decision Model for consumer who drives 100 miles per week.We assume the oil tank is empty at the beginning time(t=0). There are four cases for a consumer to choose a best refuel time when the tank is empty.i.g t>G t+4and g t>G t+2, which means the present gasoline price is higherthan that either two weeks or four weeks later. It is economic to fill halftank under such condition. ii. g t <Gt +4 and g t <G t +2, which means the present gasoline price is lower than that either two weeks or four weeks later. It is economic to fill fulltank under such condition. iii. Gt +4>g t >G t +2, which means the present gasoline price is higher than that two weeks later but lower than that four weeks later. It is economic to fillhalf tank under such condition. iv. Gt +4<g t <G t +2, which means the present gasoline price is higher than that four weeks later but lower than that two weeks later. It is economic to fillfull tank under such condition.If other time, we should consider both the gasoline price and the oil volume in the tank to pick up a best refuel time. In summary, the decision model for running 100 miles a week ist 2t 4t 2t 4t 2t 4t 2t 4t 11111411111ˆˆ(1)1((1)&max(,))24442011111ˆˆˆˆ1/2((1)&G G G (&))(0(1G G )&)4424411ˆˆˆ(1)0&(G 4G G (G &)t i t i t t t t i t i t t t t t t i t t d t or d t g d d t g or d t g d t g or ++++----+++-++<--<<--<>⎧⎪=<--<<<--<<<⎨⎪⎩--=><∑∑∑∑∑t 2G ˆ)t g +<----Equation ⑦d i is the decision variable, d i =1 means we fill full tank, d i =1/2 means we fill half tank. 11(1)4t i tdt ---∑represents the residual gasoline volume in the tank. The method of prices comparison was analyzed in the beginning part of 2.3.1.2.3.2 Decision Model for consumer who drives 200 miles per week.Because even full tank can run only two weeks, the consumer must refuel during every two weeks. There are two cases to decide whether to buy half or full tank when the tank is empty. This situation is much simpler than that of 100 miles a week. The process for decision can also be shown through the following flow chart.Chart 2The two cases for deciding buy half or full tank are: i. g t >Gt +1, which means the present gasoline price is higher than the next week. We will buy half tank because we can buy the cheaper gasoline inthe next week. ii. g t <Gt +1, which means the present gasoline price is lower than the next week. To buy full tank is economic under such situation.But we should consider both gasoline prices and free tank volume to decide our refueling plan. The Model is111t 11t 111(1)1220111ˆ1/20(1)((1)0&)22411ˆ(1&G )0G 2t i t t i t i t t t t t i t t d t d d t or d t g d t g ----++<--<⎧⎪=<--<--=>⎨⎪⎩--=<∑∑∑∑ ----Equation ⑧3. Train and Test Model by the 2011 datasetChart 33.1 Determine all the parameters in Equation ② from the 2011 dataset.Using the weekly gas data from the website and the weekly crude price data from , we can determine the best delay periods n and calculate all the parameters in Equation ②. For there are two crude oil price dataset (Weekly Cushing OK WTI Spot Price FOB and Weekly Europe Brent SpotPrice FOB), we use the average value as the crude oil price without loss of generality. We tried n =3, 4 and 5 respectively with 2011 dataset and received comparison graph of predicted value and actual value, including corresponding coefficient.(A ) n =3(the hysteretic period is 3)Graph 1 The fitted price and real price of gasoline in 2011(n=3)We find that the nearby effect coefficient of the price of crude oil and gasoline. This result is same as our anticipation.(B)n=4(the hysteretic period is 4)Graph 2 The fitted price and real price of gasoline in 2011(n=4)(C) n=5(the hysteretic period is 5)Graph 3 The fitted price and real price of gasoline in 2011(n=5)Via comparing the three figures above, we can easily found that the predictive validity of n=3(the hysteretic period is 3) is slightly better than that of n=4(the hysteretic period is 4) and n=5(the hysteretic period is 5) so we choose the model of n=3 to be the prediction model of gasoline price.G t+1=0.0398+1.6002g t+−0.7842g t−1+0.1207g t−2+ 0.4147g t−0.5107g t−1+0.1703g t−2+ε----Equation ⑨3.2 Test the Forecast Model of gasoline price by the dataset of gasoline price in 2012Next, we apply models in terms of different hysteretic periods(n=3,4,5 respectively), which are shown in Equation ②,to forecast the gasoline price which can be acquired currently in 2012 and get the graph of the forecast price and real price of gasoline:Graph 4 The real price and forecast price in 2012(n=3)Graph 5 The real price and forecast price in 2012(n=4)Graph 6 The real price and forecast price in 2012(n=5)Conserving the error of observation, predictive validity is best when n is 3, but the differences are not obvious when n=4 and n=5. However, a serious problem should be drawn to concerns: consumers determines how to fill the tank by using the trend of oil price. If the trend prediction is wrong (like predicting oil price will rise when it actually falls), consumers will lose. We use MATLAB software to calculate the amount of error time when we use the model of Equation ⑨to predict the price of gasoline in 2012. The graph below shows the result.It’s not difficult to find the prediction effect is the best when n is 3. Therefore, we determined to use Equation ⑨as the prediction model of oil price in 2012.G t+1=0.0398+1.6002g t+−0.7842g t−1+0.1207g t−2+ 0.4147g t−0.5107g t−1+0.1703g t−2+ε3.3 Calculating εSince political occurences, economic events and climatic changes can affect gasoline price, it is undeniable that a ε exists between predicted prices and real prices. We can use Equation ②to predict gasoline prices in 2011 and then compare them with real data. Through the difference between predicted data and real data, we can estimate the value of ε .The estimating process can be shown through the following flow chartChart 4We divide the international events into three types: extra serious event, major event and ordinary event according to the criteria of influence on gas prices. Then we evaluate the value: extra serious event is 3a, major event is 2a, and ordinary event is a. With inference to the comparison of the forecast price and real price in 2011, we find that large deviation of data exists at three time points: May 16,2011, Aug 08,2011 andOct 10,2011. After searching, we find that some important international events happened nearly at the three time points. We believe that these events which occurred by chance affect the international prices of gasoline so the predicted prices deviate from the actual prices. The table of events and the calculation of the value of a areTherefore, by generalizing several sets of particular data and events, we can estimate the value of a:a=26.84 ----Equation ⑩The calculating process is shown as the following graph.Since now we have obtained the approximate value of a, we can evaluate the future prices according to currently known gasoline prices and crude oil prices. To improve our model, we can look for factors resulting in some major turning point in the graph of gasoline prices. On the ground that the most influential factors on prices in 2012 are respectively graded, the difference between fact and prediction can be calculated.3.4 Test Decision Models of buying gasoline by the dataset of 2012First, we use Equation ⑨to calculate the gasoline price of next week and use Equation ⑤and Equation ⑥to calculate the gasoline price trend of next two to four weeks. On the basis above, we calculate the total cost, and thus receive schemes of buying gasoline of 100miles per week according to Equation ⑦and Equation ⑧. Using the same method, we can easily obtain the pattern when driving 200 miles per week. The result is presented below.We collect the important events which will affect the gasoline price in 2012 as well. Therefore, we calculate and adjust the predicted price of gasoline by Equation ⑩. We calculate the scheme of buying gasoline again. The result is below:3.4.1 100 miles per weekT2012 = 637.2400 (If the consumer drives 100 miles per week, the total cost inTable 53.4.2 200 miles per weekT2012 = 1283.5 (If the consumer drives 200 miles per week, the total cost in 2012 is 1283.5 USD). The scheme calculated by software is below:Table 6According to the result of calculating the buying-gasoline scheme from the model, we can know: when the gasoline price goes up, we should fill up the tank first and fill up again immediately after using half of gasoline. It is economical to always keep the tank full and also to fill the tank in advance in order to spend least on gasoline fee. However, when gasoline price goes down, we have to use up gasoline first and then fill up the tank. In another words, we need to delay the time of filling the tank in order to pay for the lowest price. In retrospect to our model, it is very easy to discover that the situation is consistent with life experience. However, there is a difference. The result is based on the calculation from the model, while experience is just a kind of intuition.3.4.3 Second Test for the Decision of buying gasolineSince the data in 2012 is historical data now, we use artificial calculation to get the optimal value of buying gasoline. The minimum fee of driving 100 miles per week is 635.7440 USD. The result of calculating the model is 637.44 USD. The minimum fee of driving 200 miles per week is 1253.5 USD. The result of calculating the model is 1283.5 USD. The values we calculate is close to the result of the model we build. It means our model prediction effect is good. (we mention the decision people made every week and the gas price in the future is unknown. We can only predict. It’s normal to have deviation. The buying-gasoline fee which is based on predicted calculation must be higher than the minimum buying-gasoline fee which is calculated when all the gas price data are known.)We use MATLAB again to calculate the total buying-gasoline fee when n=4 and n=5. When n=4,the total fee of driving 100 miles per week is 639.4560 USD and the total fee of driving 200 miles per week is 1285 USD. When n=5, the total fee of driving 100 miles per week is 639.5840 USD and the total fee of driving 200 miles per week is 1285.9 USD. The total fee are all higher the fee when n=3. It means it is best for us to take the average prediction model of 3 phases.4. The upper bound will change the Decision of buying gasoline.Assume the consumer has a mileage driven of x1miles per week. Then, we can use 200to indicate the period of consumption, for half of a tank can supply 200-mile x1driving. Here are two situations:<1.5①200x1>1.5②200x1In situation①, the consumer is more likely to apply the decision of 200-mile consumer’s; otherwise, it is wiser to adopt the decision of 100-mile consumer’s. Therefore, x1is a critical value that changes the decision if200=1.5x1x1=133.3.Thus, the mileage driven of 133.3 miles per week changes the buying decision.Then, we consider the full-tank buyers likewise. The 100-mile consumer buys half a tank once in four weeks; the 200-mile consumer buys half a tank once in two weeks. The midpoint of buying period is 3 weeks.Assume the consumer has a mileage driven of x2miles per week. Then, we can to illustrate the buying period, since a full tank contains 400 gallons. There use 400x2are still two situations:<3③400x2>3④400x2In situation③, the consumer needs the decision of 200-mile consumer’s to prevent the gasoline from running out; in the latter situation, it is wiser to tend to the decision of 100-mile consumer’s. Therefore, x2is a critical value that changes the decision if400=3x2x2=133.3We can find that x2=x1=133.3.To wrap up, there exists an upper bound on “mileage driven”, that 133.3 miles per week is the value to switch the decision for buying weekly gasoline. The following picture simplifies the process.Chart 45. An analysis of New Y ork City5.1 The main factors that will affect the gasoline price in New York CityBased on the models above, we decide to estimate the price of gasoline according to the data collected and real circumstances in several cities. Specifically, we choose New York City as a representative one.New York City stands in the North East in the United States, with the largest population throughout the country as 8.2 million. The total area of New York City is around 1300 km2, with the land area as 785.6 km2(303.3 mi2). One of the largest trading centers in the world, New York City has a high level of resident’s consumption. As a result, the level of the price of gasoline in New York City is higher than the average regular oil price of the United States. The price level of gasoline and its fluctuation are the main factors of buying decision.Another reasonable factor we expect is the distribution of gas stations. According to the latest report, there are approximately 1670 gas stations in the city area (However, after the impact of hurricane Sandy, about 90 gas stations have been temporarily out of use because of the devastation of Sandy, and there is still around 1580 stations remaining). From the information above, we can calculate the density of gas stations thatD(gasoline station)= t e amount of gas stationstotal land area =1670 stations303.3 mi2=5.506 stations per mi2This is a respectively high value compared with several other cities the United States. It also indicates that the average distance between gas stations is relatively small. The fact that we can neglect the distance for the cars to get to the station highlights the role of the fluctuation of the price of gasoline in New York City.Also, there are approximately 1.8 million residents of New York City hold the driving license. Because the exact amount of cars in New York City is hard to determine, we choose to analyze the distribution of possible consumers. Thus, we can directly estimate the density of consumers in New York City in a similar way as that of gas stations:D(gasoline consumers)= t e amount of consumerstotal land area = 1.8 million consumers303.3 mi2=5817consumers per mi2Chart 5In addition, we expect that the fluctuation of the price of crude oil plays a critical role of the buying decision. The media in New York City is well developed, so it is convenient for citizens to look for the data of the instant price of crude oil, then to estimate the price of gasoline for the coming week if the result of our model conforms to the assumption. We will include all of these considerations in our modification of the model, which we will discuss in the next few steps.For the analysis of New York City, we apply two different models to estimate the price and help consumers make the decision.5.2 Test Models with New York dataAmong the cities in US, we pick up New York as an typical example. The gas price data is downloaded from the website () and is used in the model described in Section 2 and 3.The gas price curves between the observed data and prediction data are compared in next Figure.Figure 6The gas price between the observed data and predicted data of New York is very similar to Figure 3 in US case.Since there is little difference between the National case and New York case, the purchase strategy is same. Following the same procedure, we can compare the gas cost between the historical result and predicted result.For the case of 100 miles per week, the total cost of observed data from Feb to Oct of 2012 in New York is 636.26USD, while the total cost of predicted data in the same period is 638.78USD, which is very close. It proves that our prediction model is good. For the case of 200 miles per week, the total cost of observed data from Feb to Oct of 2012 in New York is 1271.2USD, while the total cost of predicted data in the same period is 1277.6USD, which is very close. It proves that our prediction model is good also.5.3 The analysis of resultBy comparing, though density of gas stations and density of consumers of New York is a little higher than other places but it can’t lower the total buying-gas fee. Inanother words, density of gas stations and density of consumers are not the actual factors of affecting buying-gas fee.On the other hand, we find the gas fee in New York is a bit higher than the average fee in US. We can only analyze preliminary it is because of the higher goods price in New York. We need to add price factor into prediction model. We can’t improve deeper because of the limited time. The average CPI table of New York City and USA is below:Datas Statistics website(/xg_shells/ro2xg01.htm)6. Summery& Advantage and disadvantageTo reach the solution, we make graphs of crude oil and gasoline respectively and find the similarity between them. Since the conditions are limited that consumers can only drive 100miles per week or 200miles per week, we separate the problem into two parts according to the limitation. we use Time series analysis Method to predict the gasoline price of a future period by the data of several periods in the past. Then we take the influence of international events, economic events and weather changes and so on into consideration by adding a parameter. We give each factor a weight consequently and find the rules of the solution of 100miles per week and 200miles per week. Then we discuss the upper bound and clarify the definition of upper bound to solve the problem.According to comparison from many different aspects, we confirm that the model expressed byEquation ⑨is the best. On the basis of historical data and the decision model of buying gasoline(Equation ⑦and Equation ⑧), we calculate that the actual least cost of buying gasoline is 635.7440 USD if the consumer drives 100 miles per week (the result of our model is 637.24 USD) and the actual least cost of buying gasoline is 1253.5 USD(the result of our model is 1283.5 USD) if the consumer drives 100 miles per week. The result we predicted is similar to the actual result so the predictive validity of our model is finer.Disadvantages:1.The events which we predicted are difficult to quantize accurately. The turningpoint is difficult for us to predict accurately as well.2.We only choose two kinds of train of thought to develop models so we cannotevaluate other methods that we did not discuss in this paper. Other models which are built up by other train of thought are possible to be the optimal solution.。
The sweet spot-2010MCM paper.
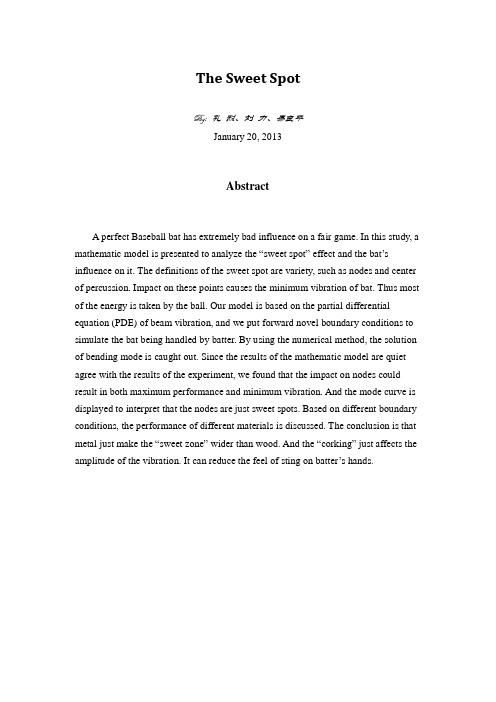
List of Figures
Fig. 1 Mode Shapes for the Prismatic Wood Beam ............................................... 11 Fig. 2 Mode Shapes for the Prismatic Wood Beam ............................................... 11 Fig. 3 Mode Shapes for the Prismatic Aluminum Beam ....................................... 12 Fig. 4 Mode Shape Comparison between the Prismatic Hollow Aluminum Beam and Prismatic Wood Beam .......................................................................... 13 Fig. 5 Cork in a Bat .................................................................................................. 14 Fig. 6 Superposition of Wood Mode Shapes .......................................................... 14
Contents
Abstract............................................................................................................... 1 1 Introduction ................................................................................................... 3 1.1 Notation and Definitions ..................................................................... 3 1.2 Brief Explanations of the Sweet Spot ................................................. 4 1.3 Energy Model ....................................................................................... 5 1.4 General Assumptions ........................................................................... 5 2 Vibration Model ............................................................................................ 6 2.1 Outline of Vibration Model ............................................................... 6 2.2 The Partial Differential Equation...................................................... 6 3 Method Descriptions .................................................................................... 9 3.1 Simplification ....................................................................................... 9 3.2 Bending Mode Analysis .................................................................... 10 4 Results and Interpretations ......................................................................... 10 4.1 Why isn’t the sweet spot at the end of the bat? ............................... 10 4.2 How does the material work?............................................................ 12 4.3 Corking?............................................................................................. of Model ....................................................... 15 References ........................................................................................................ 15
05年美国大学生数学建模竞赛A题特等奖论文翻译
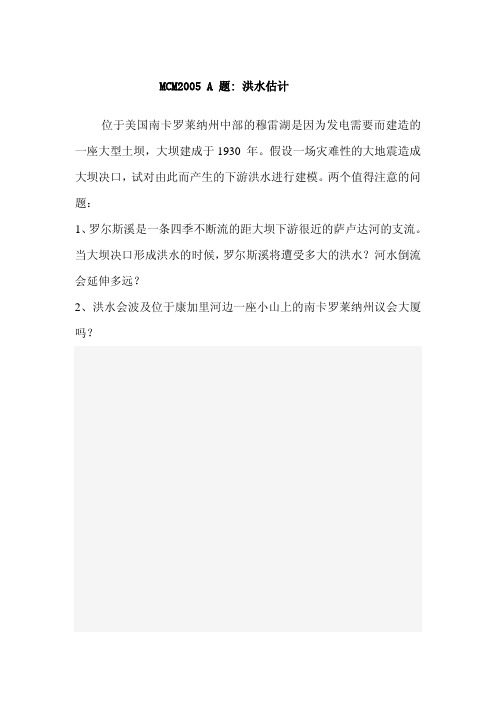
在每一时刻流出水的体积等于裂口的面积乘以水的速率乘以时间:
h h Vwater leaeing = wbreach (
−
lake
s)
dam
water
leaving ttime
step
其中:V 是体积, w 是宽度, h 是高度, s 是速度, t 是时间。
我们假设该湖是一个大的直边贮槽,所以当水的高度确定时,其面积不改变。 这意味着,湖的高度等于体积除以面积
在南卡罗莱那州的中央,一个湖被一个 75 年的土坝抑制。如果大坝被地震破坏 将会发生什么事?这个担心是基于1886年发生在查尔斯顿的一场地震,科学家们 相信它里氏7.3级[联邦能源管理委员会2002]。断层线的位置几乎直接在穆雷湖 底(SCIway 2000;1997,1998年南CarolinaGeological调查)和在这个地区小地震 的频率迫使当局考虑这样一个灾难的后果。
)
−
1 2
ห้องสมุดไป่ตู้
λ
2
(u
n j +1
−
2u j
+
n
+
u
n j
)
这里的上层指数表示时间和较低的空间, λ 是时间与空间步长的大小的比值。
(我们的模型转换以距离和时间做模型的单位,因此每个步长为 1)。第二个条 件的作用是受潮尖峰,因为它看起来不同并补偿于在每个点的任一边上的点。
我们发现该模型对粗糙度参数 n 高度敏感(注意,这是只在通道中的有效粗 糙度)。当 n 很大(即使在大河流的标准值 0.03)。对水流 和洪水堆积的倾向有 较高的抗拒,这将导致过量的陡水深资料,而且往往使模型崩溃。幸运的是,我
我们的任务是预测沿着Saluda河从湖穆雷大坝到哥伦比亚的水位变化,如果 发生了1886年相同规模的地震破坏了大坝。特别是支流罗尔斯溪会回流多远和哥 伦比亚南卡罗来纳州的州议会大厦附近的水位会多高。
2014MCM-B优秀论文
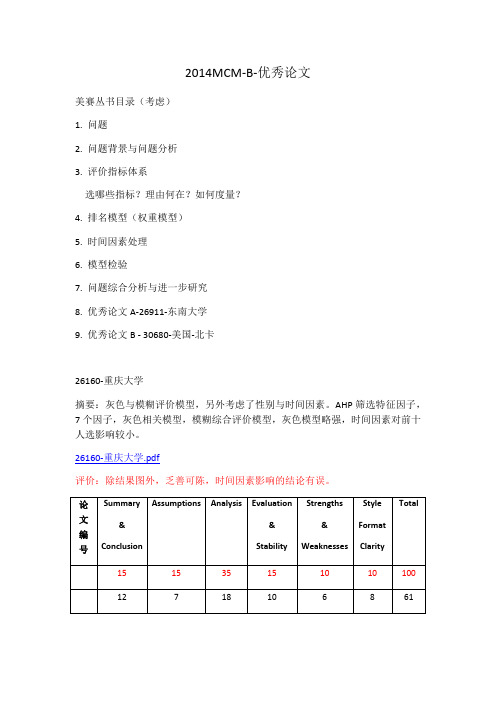
2014MCM-B-优秀论文美赛丛书目录(考虑)1. 问题2. 问题背景与问题分析3. 评价指标体系选哪些指标?理由何在?如何度量?4. 排名模型(权重模型)5. 时间因素处理6. 模型检验7. 问题综合分析与进一步研究8. 优秀论文A-26911-东南大学9. 优秀论文B - 30680-美国-北卡26160-重庆大学摘要:灰色与模糊评价模型,另外考虑了性别与时间因素。
AHP筛选特征因子,7个因子,灰色相关模型,模糊综合评价模型,灰色模型略强,时间因素对前十人选影响较小。
26160-重庆大学.pdf评价:除结果图外,乏善可陈,时间因素影响的结论有误。
26636-外经贸大学摘要:灰色相关模型,依据专家意见选择了四个评价指标:NCAA冠军,Pct,胜场数,教练报酬。
模糊相容矩阵确定各个评价指标的权值,结果与ESPN作比较。
最后讨论了时间因素,发现规律:“从前”的教练的胜率要远远高于“现在”的教练,但其他三个指标所受到的影响很小。
引入滑动平均方法,将时间因素纳入胜率计算模型中,这是本文的一个亮点。
Shannon熵用于评价稳定性。
讨论了参数敏感性。
便利与普适是我们模型的最大优点,但存在指标选择的主观性。
26636-外经贸.pdf评价:指标体系以及评价模型一般,有点投机,时间因素讨论、模型结果检验以及敏感性检验是亮点,结果对比表达清晰明了,可信度高。
缺假设与“conclusion”,是硬伤。
26911-东南大学三阶段全面评价模型,指标体系(胜率,稳定性,获得冠军数量,个人报酬,点击率,个人荣誉,职业联赛排名),谷歌趋势统计方法,线性拟合方法,加权和模型,AHP+最大熵模型,灰色相关分析,综合排名26911-东南大学.pdf评价:非常全面,思路很清晰,表达很简洁,值得效仿。
具体说:指标意义讨论充分;指标取值实用、合理;时间因素考虑到位;权重确定有技术含量;结果表达清晰;文章节奏把握好。
如果按更高标准衡量,第二种权重体系中GRA的作用不大显著。
美国数学建模比赛一等奖
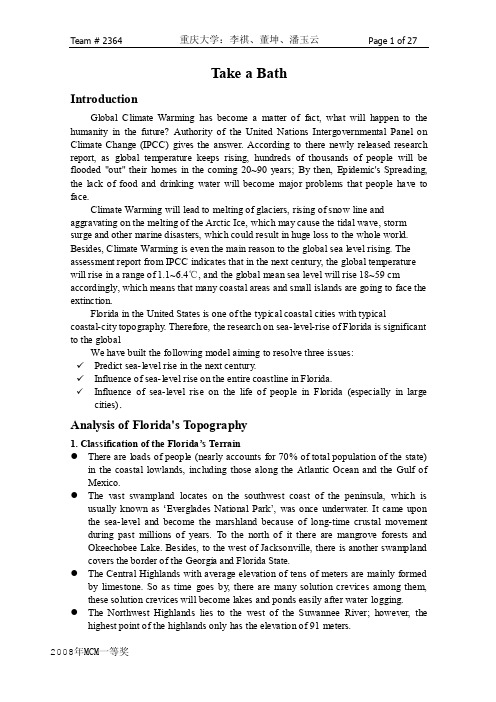
Figure 1.Panoramic satellite map of Florida The picture was taken at the altitude of 879.7km, and we saved a copy of it as standard picture. Next, we chose 17 key points on the picture and connected each point by straight lines to form a close polygonal, which can roughly represent the border of the whole State (Red points and Green lines), shown in Figure 2:
Analysis of Florida's Topography
1. Classification of the Florida’s Terrain There are loads of people (nearly accounts for 70% of total population of the state) in the coastal lowlands, including those along the Atlantic Ocean and the Gulf of Mexico. The vast swampland locates on the southwest coast of the peninsula, which is usually known as „Everglades National Park‟, was once underwater. It came upon the sea- level and become the marshland because of long-time crustal movement during past millions of years. To the north of it there are mangrove forests and Okeechobee Lake. Besides, to the west of Jacksonville, there is another swampland covers the border of the Georgia and Florida State. The Central Highlands with average elevation of tens of meters are mainly formed by limestone. So as time goes by, there are many solution crevices among them, these solution crevices will become lakes and ponds easily after water logging. The Northwest Highlands lies to the west of the Suwannee River; however, the highest point of the highlands only has the elevation of 91 meters. 2008年MCM一等奖
2015美赛H奖论文
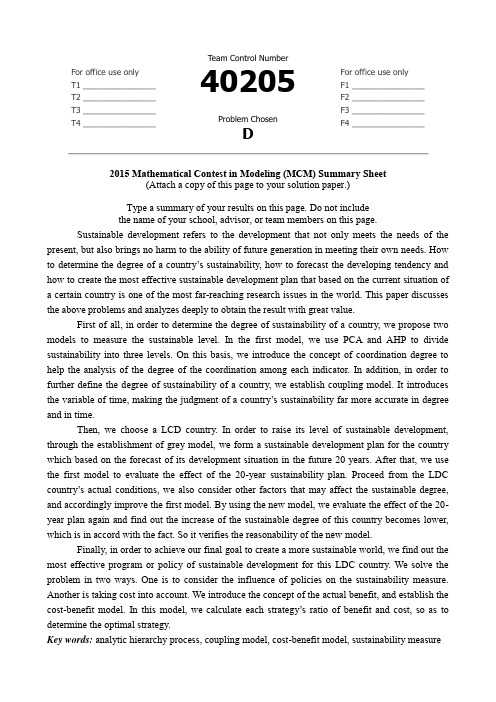
D
2015 Mathematical Contest in Modeling (MCM) Summary Sheet (Attach a copy of this page to your solution paper.) Type a summary of your results on this page. Do not include the name of your school, advisor, or team members on this page. Sustainable development refers to the development that not only meets the needs of the present, but also brings no harm to the ability of future generation in meeting their own needs. How to determine the degree of a country’s sustainability, how to forecast the developing tendency and how to create the most effective sustainable development plan that based on the current situation of a certain country is one of the most far-reaching research issues in the world. This paper discusses the above problems and analyzes deeply to obtain the result with great value. First of all, in order to determine the degree of sustainability of a country, we propose two models to measure the sustainable level. In the first model, we use PCA and AHP to divide sustainability into three levels. On this basis, we introduce the concept of coordination degree to help the analysis of the degree of the coordination among each indicator. In addition, in order to further define the degree of sustainability of a country, we establish coupling model. It introduces the variable of time, making the judgment of a country’s sustainability far more accurate in degree and in time. Then, we choose a LCD country. In order to raise its level of sustainable development, through the establishment of grey model, we form a sustainable development plan for the country which based on the forecast of its development situation in the future 20 years. After that, we use the first model to evaluate the effect of the 20-year sustainability plan. Proceed from the LDC country’s actual conditions, we also consider other factors that may affect the sustainable degree, and accordingly improve the first model. By using the new model, we evaluate the effect of the 20year plan again and find out the increase of the sustainable degree of this country becomes lower, which is in accord with the fact. So it verifies the reasonability of the new model. Finally, in order to achieve our final goal to create a more sustainable world, we find out the most effective program or policy of sustainable development for this LDC country. We solve the problem in two ways. One is to consider the influence of policies on the sustainability measure. Another is taking cost into account. We introduce the concept of the actual benefit, and establish the cost-benefit model. In this model, we calculate each strategy’s ratio of benefit and cost, so as to determine the optimal strategy. Key words: analytic hierarchy process, coupling model, cost-benefit model, sustainability measure
美赛优秀论文2010MCM的B特等奖翻译

摘要:该模型最大的挑战是如何建立连环杀手犯罪行为的模型。
因为找出受害者之间的联系是非常困难的;因此,我们预测罪犯的下一个目标地点,而不是具体目标是谁。
这种预测一个罪犯的犯罪的空间格局叫做犯罪空间情报分析。
研究表明:最暴力的连环杀手的犯罪范围一般在一个径向带中央点附近:例如家庭,办公室,以及其他一些犯罪行为高发区(例如城镇妓女集中区)。
这些‘锚点’为我们的模型提供了基础。
我们假设整个分析域是一个潜在的犯罪现场。
罪犯的活动不受到任何条件约束;并且该区域足够大包括所有的打击点。
我们考虑的是一个可度量的空间,为预测算法创建了空间可能性。
此外;我们假设罪犯为一个暴力的系列犯罪者,因为研究表明窃贼和纵火犯不可能遵循某一空间模式。
一个锚点与多个锚点有着实质性的不同,首先讨论单个锚点的案例,建立坐标系并把罪犯最后犯罪地点与犯罪序列表示出来,并估计以前案件发生地地点,评估模型的可靠性,并且我们得到未来可能发生犯罪行为的锚点。
对于多个锚点的案例,我们通过聚类与排序的方法:将所给数据划分为几组。
在每组中找一个最重要的锚点,每一个分区都给定一个权值。
我们进行单点测试,利用以前的锚点预测最近的一个锚点,并且与其实际位置相比较。
我们从文献中摘录七个数据集,并且用其中四个改善我们的模型,检测其序列变化,地理集中位置和总锚点的数目。
然后通过其他三点来评估我们的模型。
结果显示多个锚点的模型的结果比较优。
引言:通过研究文献中以得出的连环案件罪犯地理空间往往是围绕罪犯日常活动的几个锚点附近的区域。
我们建立的预测模型就是在其规律下建立的,并且预测出一个表面的可能值和度量值。
第一个方案是通过重心法寻找出单个可能的锚点。
第二个方案是假设2到4个锚点,并且利用聚类算法的排序与分组的方法。
两种方案都是利用统计方法来缩小预测未来犯罪的地点区域背景:1981年peter sutcliffe的逮捕是法医生物学家stuart kind 通过利用数理原理成功预测出约克郡开膛手的住处的一个标志目前,信息密集型模型是通过热图技术建立确定特殊犯罪类型的热点或者是找出犯罪活动与某一地区之间的联系比率。
2017年美赛论文C题M奖中文国际一等奖
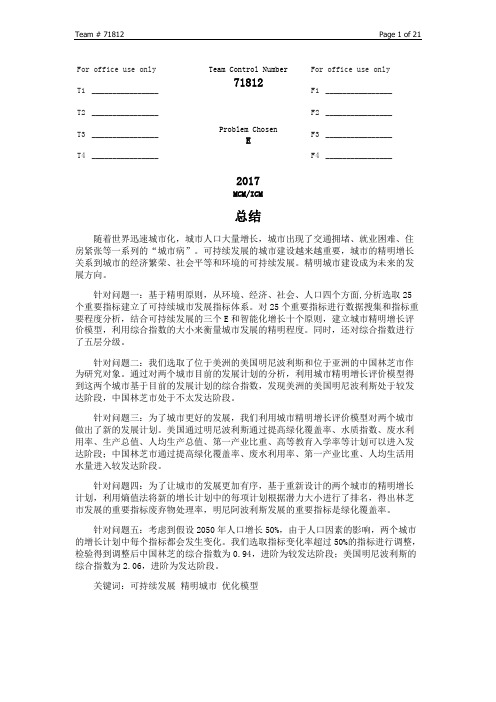
For office use only T1________________ T2________________ T3________________ T4________________ Team Control Number71812Problem ChosenEFor office use onlyF1________________F2________________F3________________F4________________ 2017MCM/ICM总结随着世界迅速城市化,城市人口大量增长,城市出现了交通拥堵、就业困难、住房紧张等一系列的“城市病”。
可持续发展的城市建设越来越重要,城市的精明增长关系到城市的经济繁荣、社会平等和环境的可持续发展。
精明城市建设成为未来的发展方向。
针对问题一:基于精明原则,从环境、经济、社会、人口四个方面,分析选取25个重要指标建立了可持续城市发展指标体系。
对25个重要指标进行数据搜集和指标重要程度分析,结合可持续发展的三个E和智能化增长十个原则,建立城市精明增长评价模型,利用综合指数的大小来衡量城市发展的精明程度。
同时,还对综合指数进行了五层分级。
针对问题二:我们选取了位于美洲的美国明尼波利斯和位于亚洲的中国林芝市作为研究对象。
通过对两个城市目前的发展计划的分析,利用城市精明增长评价模型得到这两个城市基于目前的发展计划的综合指数,发现美洲的美国明尼波利斯处于较发达阶段,中国林芝市处于不太发达阶段。
针对问题三:为了城市更好的发展,我们利用城市精明增长评价模型对两个城市做出了新的发展计划。
美国通过明尼波利斯通过提高绿化覆盖率、水质指数、废水利用率、生产总值、人均生产总值、第一产业比重、高等教育入学率等计划可以进入发达阶段;中国林芝市通过提高绿化覆盖率、废水利用率、第一产业比重、人均生活用水量进入较发达阶段。
针对问题四:为了让城市的发展更加有序,基于重新设计的两个城市的精明增长计划,利用熵值法将新的增长计划中的每项计划根据潜力大小进行了排名,得出林芝市发展的重要指标废弃物处理率,明尼阿波利斯发展的重要指标是绿化覆盖率。
美赛国际特等奖团队获奖者徐乾:不疾不徐,自有乾坤
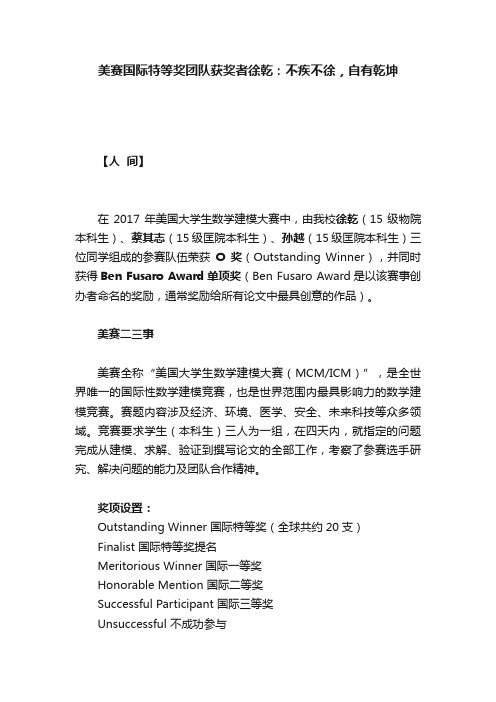
美赛国际特等奖团队获奖者徐乾:不疾不徐,自有乾坤【人间】在2017年美国大学生数学建模大赛中,由我校徐乾(15级物院本科生)、蔡其志(15级匡院本科生)、孙越(15级匡院本科生)三位同学组成的参赛队伍荣获O奖(Outstanding Winner),并同时获得Ben Fusaro Award单项奖(Ben Fusaro Award是以该赛事创办者命名的奖励,通常奖励给所有论文中最具创意的作品)。
美赛二三事美赛全称“美国大学生数学建模大赛(MCM/ICM)”,是全世界唯一的国际性数学建模竞赛,也是世界范围内最具影响力的数学建模竞赛。
赛题内容涉及经济、环境、医学、安全、未来科技等众多领域。
竞赛要求学生(本科生)三人为一组,在四天内,就指定的问题完成从建模、求解、验证到撰写论文的全部工作,考察了参赛选手研究、解决问题的能力及团队合作精神。
奖项设置:Outstanding Winner 国际特等奖(全球共约20支)Finalist 国际特等奖提名Meritorious Winner 国际一等奖Honorable Mention 国际二等奖Successful Participant 国际三等奖Unsuccessful 不成功参与2017年,全球共有8843支参赛队伍参加。
其中获得O奖的有13支队伍,而Ben Fusaro Award创意奖,全球仅一支队伍,可见其分量之重。
由于蔡其志和孙越两位同学正在交换,我们此次只采访了徐乾同学。
徐乾南京大学物理学院2015级本科生大一学分绩4.66,大二学分绩4.78,均位列年级第一曾获国家奖学金、拔尖计划一等奖学金、郑钢奖学金、人民奖学金特长奖等2017南青朋辈之星标兵南京大学2015-2016学年优秀学生标兵南京大学第20届基础学科论坛一等奖“一个世界有你,一个世界没有你,让这两者的不同最大化,这就是你一生的意义。
”在接受采访的过程中,徐乾同学引用了李开复的这句话。
美国大学生数学建模大赛优秀论文一等奖摘要
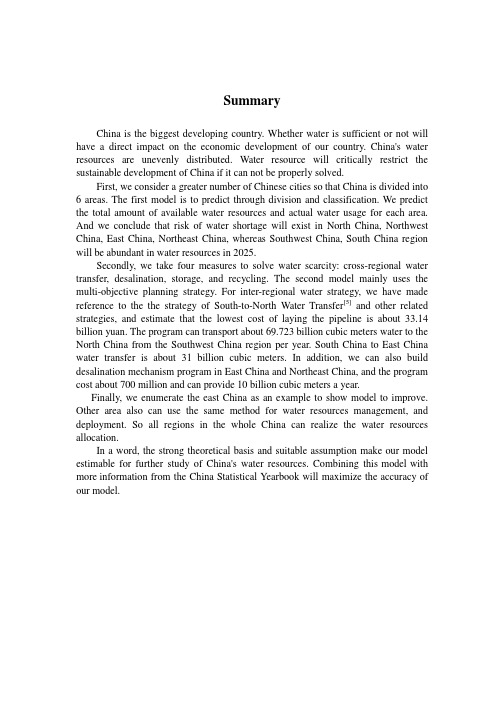
SummaryChina is the biggest developing country. Whether water is sufficient or not will have a direct impact on the economic development of our country. China's water resources are unevenly distributed. Water resource will critically restrict the sustainable development of China if it can not be properly solved.First, we consider a greater number of Chinese cities so that China is divided into 6 areas. The first model is to predict through division and classification. We predict the total amount of available water resources and actual water usage for each area. And we conclude that risk of water shortage will exist in North China, Northwest China, East China, Northeast China, whereas Southwest China, South China region will be abundant in water resources in 2025.Secondly, we take four measures to solve water scarcity: cross-regional water transfer, desalination, storage, and recycling. The second model mainly uses the multi-objective planning strategy. For inter-regional water strategy, we have made reference to the the strategy of South-to-North Water Transfer[5]and other related strategies, and estimate that the lowest cost of laying the pipeline is about 33.14 billion yuan. The program can transport about 69.723 billion cubic meters water to the North China from the Southwest China region per year. South China to East China water transfer is about 31 billion cubic meters. In addition, we can also build desalination mechanism program in East China and Northeast China, and the program cost about 700 million and can provide 10 billion cubic meters a year.Finally, we enumerate the east China as an example to show model to improve. Other area also can use the same method for water resources management, and deployment. So all regions in the whole China can realize the water resources allocation.In a word, the strong theoretical basis and suitable assumption make our model estimable for further study of China's water resources. Combining this model with more information from the China Statistical Yearbook will maximize the accuracy of our model.。
2005 B M Giving Queueing the Booth

Giving Queueing the BoothMCM Team851February7th,20051Team8512 Contents1Introduction31.1Assumptions (3)2Model62.1r<κ,λ,µ (7)2.2κ<r<λ<µ (7)2.3κ<λ<r<µ (10)2.3.1Section1 (10)2.3.2Section2 (11)2.3.3Section3 (12)2.4κ<λ<µ<r (13)2.4.1Section1 (14)2.4.2Section2 (14)2.4.3Section3 (14)2.4.4Section4 (15)2.4.5Section5 (15)2.4.6Section6 (16)2.5mn <p (17)2.5.1λ<r<µ (17)2.5.2λ<µ<r (18)3Strengths and Weaknesses of our Model193.1Strengths (19)3.2Weaknesses (19)3.3Possible Improvements (20)4Results214.1Estimation of Parameters (21)4.2Results from Program (22)4.3When m=n (26)5Conclusions285.1n=m (28)Team8513 1IntroductionDelays at tolling systems are ubiquitous in major road networks throughout the western world.In fact traffic has created problems for urban centers of civilization throughout the ages.“Vehicular traffic,except for chariots and official vehicles,was prohibited from entering Rome during the hours of daylight”for a period in thefirst century[3,Page3].“At least one(Roman) emperor was forced to issue a proclamation threatening the death penalty to those whose chariots and carts blocked the way”of traffic[1,Section1.2] Most toll booth systems involve a major highway with a certain number of lanes,which suddenly increases to a larger number of lanes.Each of these lanes then passes through a toll booth where a tolling system charges the motorist the required toll.Traditionally this has involved the exchange of actual currency,which delayed traffic,although modern electronic payment systems are eliminating this feature(see[15]).The roadway then squeezes back to the initial number of lanes at the end of the toll area.Increasing the number of booths so that the ratio of booths to initial lanes is much larger than1will result in delays at the actual booth being virtually eliminated, however as the largeflow of traffic is squeezed back down to the initial number of lanes results in major congestion,especially at“rush hour”.If the number of toll booths equals the initial number of lanes,then there is no squeezing of traffic,however large tailback occur at the toll booths themselves.The challenge(faced by traffic authorities throughout the western world)is to find an intermediate value that minimizes congestion.Our aim was that the delay time for a rush hour trip through our system in comparison with a trip through with no traffic be minimized1.1AssumptionsWe made a number of assumptions in order to simplify our system and allow us to construct our model.•We assume all vehicles entering our system are identical.We ignore differences between cars,vans,trucks,lorries,etc.•We assume that all vehicles move at the same speed.We assume traf-fic is slowed in the toll plaza relative to the previous section of road, perhaps by imposing a lower speed limit speed limit.Of course in this scenario we now have to assume no-one breaks the speed limitTeam8514•We assume that the vehicles are evenly distributed throughout the lanes,i.e.there is no preference for any lane.•We allow vehicles to be in one of two states,either moving at afixed speed or stopped.•If the number of vehicles arriving at a particular section(namely plaza, toll booths or squeeze point)of our system is greater than the maximum capacity of our system,then that capacity of vehicles pass through, and the remainder form a“virtual queue”based on a“first-come,first-served”basis.(It should be noted that in this report plaza will refer to the wide section before the toll booth and the whole area to be modelled referred to as the system).If vehicles arrive at a section anda queue has already formed,those vehicles join the back of the queue.This is essentially,in the nomenclature of queueing theory,a blocked customers delayed system with parallel servers,see[10,Chap.3]and [7,Pg.38]respectively•All toll booths are identical.In reality almost all modern toll roads em-ploy an electronic system that allows motorists to pass through without stopping,e.g.[15].we are also ignoring the presence of specialist lanes,nes devoted exclusively to heavy goods vehicles.•Our toll plazas are of large enough length that congestion at the squeeze point does not back up as far as the toll booths,which would delay passage of vehicles through the booths and similarly congestion at the toll booths does not affect traffic entering the plaza.•We divide the lanes into discrete intervals of constant length.This length is the length of the vehicle plus the length between successive vehicles,which we assume to be constant.We then model vehicles to be points moving between successive intervals.Since we have assumed constant vehicle speed this means that there is a characteristic time,θ, associated with the passage of vehicles from one interval to the next.•We assume all vehicles arriving at an empty toll booth pass through in the same time,τ.We ignore all possible technical malfunctions, customer delay,etc.For ease of modelling we assumeτis an integer multiple ofθ,τ=pθ,p>1Team8515•We assume that if a vehicle arrives at the toll booths and there is a free booth the vehicle will pass through that booth.•We ignore all external factors other than the incoming traffic for the toll plaza.For example during the transition from domestic currencies to the single European currency,most European toll booths experienced delays,see,e.g.[14].We ignore these such factors.•We assumed the trafficflow would have the general shape shown in Figure1.1.This is a justified assumption for a radial route in anFigure1:The general trend for trafficflowurban centre,see[1,Chap.6].The accepted time span for the peak in Figure is of the order2hours and we will assume it is exactly2hours when considering explicit examples in the results section.•In order to measure congestion we decided to measure the average delay time of the vehicles who arrive in the system during the period of congestion.In other words we decided to measure the extra time spent waiting during congestion compared to the time it would take to pass through the system if there was absolutely no other traffic present, and average this over all vehicles entering the system while there is congestion.We defined an optimal system to be one which minimizes this average delay time.Team8516 2ModelWe began by considering a function h(t)that gives the instantaneous rate of traffic incoming on our system.While this continuous approach may seem to contradict our assumptions that give a discrete nature to our approach,this issue will be addressed shortly.We then multiplied our function by1+γwhereγis a random real number between−0.01and0.01.This gives us a function f(t)which contains an inherent randomness which we believe is an integral part of trafficflow. We now considerκ,the maximum rate at which cars can leave our system. By the definition ofθthis will beκ=n θwhere n is the number of lanes after the squeeze point(and before the toll plaza).Analogously we will have the maximum rate at which vehicles can pass through the toll booth to beλ=m τwhere m is the number of toll booths.Since we are assuming the same speed and lane-interval length in all parts of our system we will have a maximumrate in the plaza ofµ=m θ.If we denote by r the peak of our function h(t)then the behavior of our system can be characterized by the relative values of r,κ,λandµ.We note that for any traffic approaching our system the max rate that will ever approach the squeeze point isλsince the toll booths will delay any traffic flowing at a greater rate.This means that in order for congestion to occur at the squeeze point in our system we requireκ<λEquivalentlyn θ<mτ⇔mn>τθTeam8517mn>p(1) Condition(1)will be crucial to the analysis of our model.It is a necessary but not sufficient condition for congestion to occur at the squeeze point. 2.1r<κ,λ,µIn this case theflow of traffic into our system is less than the maximum capacity of each individual section of the system.Consequently there is no congestion in our system and so corresponds to light traffic.We neednot concern ourselves with such behavior since varying mn has no effect oncongestion.2.2κ<r<λ<µIt is clear thatτ=pθ>θand soλ=mτ≤mθ=µin general.Suppose congestion occurs at the squeeze point but not at the toll booth.This means condition(1)must hold.If this congestion is to occur we also requireκ<r≤λ.This behavior is shown in Figure2.We nowΜΛΚFigure2:The relationship between r,κ,λandµproceed as follows.We define∆ρ(t)=f(t) for f(t)<λλfor f(t)≥λTeam8518 The second condition may seem redundant since r<λ,but it should be noted that r is the peak of our function h and not of our function f.It is entirely possible that our“noise”termγwould cause f(t)to exceedλand thus∆ρto exceedλ.However∆ρrefers to a discrete arrival rate at the squeeze point,and we cannot have this exceedingλso we apply the above careful conditional definition.Based on this we calculate the number of vehicles arriving at the squeeze point at time t as∆c(t)=θ∆ρ(t)We are now ready to apply our model.We set up the following difference equations for the function c(t)which refers to the number of vehicles trying to go through the squeeze point.It includes those who will get through at time t.It can be thought of as the number of people in the(virtual)queue. In order to simplify matters we take t=0when h(t)=κ,which is valid since we have assumed h(t)is monotonic in the region before the peak and so by the discussion earlier in this section there will be no congestion for t<0. Our initial condition isc(0)=∆c(0)This simply says that the number of vehicles trying to pass through the squeeze point at time0is those that arrive at time0,since there is no congestion before this,and consequently no(virtual)queue.This now givesc(θ)=c(0)−n+∆c(θ)since we have c(0)−n remaining in the(virtual)queue from time0and∆c(θ) arrive at timeθ.Repeating this givesc(iθ)=c((i−1)θ)−n+∆c(iθ)where i=1,2,...Since our function f peaks at some value less thanλand then falls belowκthis means c(t)will eventually begin to fall and at some point become≤0.At this point we define c(t)to be zero and our model is finished since there is now no more congestionc(Nθ)≤0,c(Nθ):=0This means that our model gives the following difference equation with spec-ified initial and terminating conditionsc(0)=∆c(0)c(iθ)=c((i−1)θ)−n+∆c(iθ)(2)c(Nθ)≤0,c(Nθ):=0Team8519ΚThis can be solved recursively.The above approach highlights the discrete nature of our approach alluded to earlier.In effect we are incrementing time in discrete intervals ofθand allowing each vehicle to either move forward one space increment or to remain in situ.During each time interval of sizeθ,c((i−1)θ)−n vehicles are delayed by amountθ,which gives usT delay=Ni=0(c(iθ)−n)θfor the total delay time.The total number of vehicles arriving during con-gestion is,clearly,given byV total=Ni=0∆c(iθ)Hence our“optimization parameter”ist delay=T delayV total=Ni=0(c(iθ)−n)Ni=0∆c(iθ)(3)where the overbar represents mean.It would now seem to simply be a computing problem to vary the ratio m to minimize the mean delay time as given by(13).However this is not quite the case.Suppose we are faced with the challenge of designing an optimal toll plaza for an already existing highway.This means n,τ,θ, f and r are determined by factors outside our control(pre-existing values,Team85110 efficiency of booths,speed of cars in plaza,incoming traffic and incoming traffic respectively).This means that m is our only free parameter,which influencesλ.The crucial point is thatλonly affects whether or not this particular version of our model is valid.To generalize,what our assumptions say is that if the rate of influx of traffic is too low to result in congestion at the toll booths,then the congestion at the squeeze point is independent of m, the number of toll booths.While this is clearly not a perfect model of reality, it is not as far removed from the truth as one might think.Intuitively,there should be a correlation between these two quantities.However the overall number of vehicles“squeezing in”and the number of lanes into which they squeeze are constants so the dependence may not be that strong.If this is the case then our model is a good approximation,especially when we consider that in later sections,the model is much better.2.3κ<λ<r<µTake the case where,congestion occurs at both the squeeze point and the toll booths.Again condition(1)must hold as well asκ<λ<r<µ.We now define temporarily∆ρ(t)=f(t) for f(t)<λλfor f(t)≥λwhich is exactly the same as the previous section,and∆β(t)=f(t) forλ<f(t)<µµfor f(t)≥µrefereing to a discrete arrival at the toll booths this time.To model this in a clear and concise way,the model was broken into three separate sections.2.3.1Section1This starts at the point where f(t)first intersects the lineκand is set to be t=0.Before this point,f(t)is always belowκso no congestion can occur.Above this point however,congestion occurs at the squeeze point. The conditions in this section are the same as the beginning of the model inTeam85111ΛΚ123ΛΚ1232.2,so it is reasonable to take the difference equation for congestion at the squeeze point to bec(0)=∆c(0)(4)c(iθ)=c((i−1)θ)−n+∆c(iθ)with the terminating conditions removed for the moment.Section1ends, however as f(t)increases to the point where it intersectsλ.This is labelled t=t o.2.3.2Section2The second section intuitively begins at the point where congestion begins to occur at the toll booths.A difference equation is constructed here much in the same way as for the squeeze point in2.2.The number of vehicles at time t arriving at the booths is given in time intervals ofτthough∆b(t)=τ∆β(t)Team85112 We now define b(t)to be the number of vehicles trying to go through the toll booths or the number in the(virtual)queue.There will be no congestion for f(t)<λso we can assume the initial condition:b(t0)=∆b(t0)The general difference equation then becomes:b(t0+iτ)=b(t0+(i−1)τ)−m+∆b(t0+iτ)again with i=1,2,....The function f peaks at a value r<µand then decreases belowλ,meaning that at some value of t,b(t)≤0.After this point,there will be no more congestion at the toll booths,and so is the terminal condition for b(t)and is defined as0to avoid negative numbers:b(t0+Mτ)≤0,b(t0+Mτ):=0The difference equation with initial and terminal conditions for the toll booth isb(t0)=∆b(t0)b(t o+iτ)=b(t0+(i−1)τ)−m+∆b(t o+iτ)(5)b(t o+Mτ)≤0,b(t o+Mτ):=0The effect of the congestion at the toll booths on the squeeze point is that the rate of influx of vehicles is constantly atλ.This gives a general difference equation for the section ofc(t0+iθ)=c(t0+(i−1)θ)−n+λθ2.3.3Section3The third and last section begins where section2ends,at t=t0+Mτ.Here, there is no more queuing at the toll booths,but queuing continues at the squeeze point.(As can be seen from the graph above,there is a sharp drop at the start of section2until f[t]is obtained again.Though this seems counter-intuitive, it was noticed,by chance,while sitting over coffee during a break that some-one who joined a long queue at the end of a busy period,still had no oneTeam 85113behind when he reached the counter!)The general difference equation for the squeeze point reverts back to that of 2.2,since f [t ]is now below λagain.The terminating condition can also be taken from the model in 2.2givingc (Nθ)≤0,c (Nθ):=0(6)We can now redefine c (t )properly over all three sections ∆ρ(t )= f (t ) for t <t o ,t o +Mτ<t λfor t o <t <t o +MτThe total delay time is then given by:T delay =[Ni =0(c (iθ)−n )]θ+[M i =0(b (t o +iτ)−m )]τand with the total number of cars delayed given byV total =Ni =0 f (iθ)we get our “optimization parameter”to bet delay =T delay V total =[ N i =0(c (iθ)−n )]θ+[ M i =0(c (t o +iτ)−m )]τ N i =0∆c (iθ)(7)2.4κ<λ<µ<rThis is perhaps the most complicated part of our model,with more traffic than the plaza can handle.The intuitive thinking behind this is that,in the event of unusually large traffic,for example a sports event or concert,the maximum rate for the plaza itself is too low for the rate of traffic.In effect,a queue forms before the plaza itself.The model for this is simply an extra tier onto the model for 2.3.Much the same as 2.3is an extra tier on top of 2.2.Here,the graph of f [x ]is split first into three sections as in part B.Team 85114ΚΛΜ1234562.4.1Section 1This is the same as section 1in 2.3(also the same as the beginning of 2.2)and as such,the start point is set to t =0and end point set to t =t o ,the rest of the difference equation also follows from 2.3c (0)=∆c (0)c (iθ)=c ((i −1)θ)−n +∆c (iθ)(8)2.4.2Section 2In order to simplify the model,section 2was taken to be the same as in part B,i.e.from the end point of section 1to the terminating point of the queuing at the toll booth.As a result of this,the behavior of the queuing at the squeeze point is exactly the same as in part B:c (t 0+iθ)=c (t 0+(i −1)θ)−n +λθThe queuing at the booth and plaza are entirely contained in section 2but will be broken down into further sections later.2.4.3Section 3This is again the last section in terms of chronological order,but labelled in this way,is exactly the same as 2.3.3,and as such the difference equation,by the same arguments isTeam85115c(iθ)=c((i−1)θ)−n+∆c(iθ)(9)c(Nθ)≤0,c(Nθ):=02.4.4Section4Sections4to6are now analogous to2.3withµinstead ofλandλinstead ofκ.There is also,however a time shift,with t=0going to t=t o.Hence the equations for the queuing at the toll booth becomeb(t0)=∆b(t0)(10)b(t o+iτ)=b(t0+(i−1)τ)−m+∆b(t o+iτ) These apply until f(t)>µ,when the congestion at the plaza kicks in. This time is labelled t=t1and is the starting point for section5.2.4.5Section5The queuing at the plaza is described by similar difference equations to the queuing at the squeeze point in that it depends on the characteristic time θ.However,because there are m lanes instead of n,m vehicles get through to the plaza in each time intervalθ.There is also a time scale based on the congestion at the plaza beginning at t=t1.Thefinished equation reads∆α(t)= f(t)∆a(t)=θ∆α(t)(11)c(t1)=∆a(t1)a(t1+iθ)=a(t1+(i−1)θ)−m+∆a(t1+iθ)a(t1+Qθ)≤0,a(t1+Qθ):=0The traffic now arriving at the booth is at a constant rate ofµ.This has the same effect as on the squeeze point in both2.3.2and2.4.2.The congestion is modelled in the following way until there is no more congestion at the plazaTeam85116b(t o+iτ)=b(t0+(i−1)τ)−m+µτThere is one major difference though between the model for2.3and the whole of2.4.2here.Because the queuing at the plaza is measured in time intervals ofθand the queuing at the booth in intervals ofτ=pθ,the congestion at the plaza may not end on an integer number ofτfor the booth.Intuitively,ifθandτare out of sync,there will be a slight delay in the effect onτ.As a result,the next integer value of t1+iτafter the termination of a(t)is taken as the end of2.4.2for the boothb(t1+Dθ)s.t.D= Qθτ2.4.6Section6Here,we have no more congestion at the plaza,and we aren’t considering the queuing at the squeeze point(already covered as section2),so the only concern is the toll booth itself.Hence this section starts at t1+Dτ.It then follows the basic difference equation for the booth,with the appropriate time scaling,terminating in the usual way.b(t o+Mτ)≤0,b(t o+Mτ):=0Now the three different functions for the arriving traffic at each section need to be defined properly∆ρ(t)=f(t) for t<t o,t o+Mτ<t λfor t o<t<t o+Mτ∆β(t)=f(t) for t<t1,t1+Dτ<tµfor t1<t<t1+Dτ∆α(t)= f(t)(12)Team85117 The summations of these functions over their respective time spanT delay=[Ni=0(c(iθ)−n)]θ+[Mi=0(b(t o+iτ)−m)]τ+[Qi=0(a(t1+iθ)−m)]θTotal number of cars delayedV total=Ni=0f(iθ)“optimization parameter”t delay=T delayV total=T delay=[Ni=0(c(iθ)−n)]θ+[Mi=0(b(t o+iτ)−m)]τ+[Qi=0(a(t1+iθ)−m)]θNi=0∆c(iθ)(13)2.5mn<pHere are the last remaining variations of the model,where the condition 1doesn’t hold,i.e.λ<κ.In this scenario,maximum rate of vehicles that leave the booth will never be enough to cause congestion at the squeeze point.The case where m=n is a special case of this scenario.2.5.1λ<r<µIn this situation,traffic congestion occurs at the toll booths,but nowhere else.It is very similar to the model for2.2but with a number of differences. Instead of c(t)we use b(t)because the congestion is at the toll booths and not the squeeze point.θalso changes toτevery where andκandλgo toλandµrespectively.This gives the difference equationsb(0)=∆b(0)b(iτ)=b((i−1)τ)−m+∆b(iτ)b(Nτ)≤0,b(Nτ):=0(14)Team85118ΜΛTotal delay time for systemT delay=[Ni=0(b(iτ)−m)]τThe total number of vehicles arriving during congestionV total=Ni=0∆b(iτ)Our“optimization parameter”ist delay=T delayV total=[Ni=0(b(iτ)−m)]τNi=0∆b(iτ)(15)where the overbar represents mean.2.5.2λ<µ<rIn this section,the peak rate of traffic influx is greater than plaza can deal with as well and so congestion builds up there as well as at the booths.This is analogous to2.3with a few minor adjustments again.c and b go to b and a respectivelyθandτswap andκandλgo toλandµrespectively.Thefinal average comes out ast delay=T delayV total=[Ni=0(b(iτ)−m)]τ+[Mi=0(a(t o+iθ)−m)]θNi=0∆b(iτ)(16)Team85119ΜΛ3Strengths and Weaknesses of our Model 3.1Strengths•The model we have chosen is easily programmed and the program we wrote(in Mathematica5.0)is included as an Appendix.•None of the inputs for our model are specific to certain types of toll booths.Parameters such as h(t),τ,etc can be easily measured by a team of statistical consultants.•If our data is as good an approximation of reality as we think it is,then the behavior of our model should be stable for any realistic variations.•Our model contains a random nature ensured by the presence ofγ, which makes it more realistic of real-life.•Our model will in theory give an optimal ratio of m to n3.2Weaknesses•While we have a random element to our data,repeated studies and standard probability or queueing theory have established the need to consider a quasi-random Poisson arrival model for this situation(see, for example,[6,Section3.5])•Almost all of the assumptions we have made for the nature of the movement of vehicles through our system are highly unrealistic.ForTeam85120 example,vehicles will not be homogenous,will not move in discrete lengths over discrete time intervals,will not arrive in continuous time.•Even if our discrete model is valid,there is absolutely no justificationfor assuming p=τθis an integer if we are considering a general tollplaza.•If1person experiences a1hour delay and99people experience no wait, then,by our definition of optimal,this is better than100people waiting for half a minute.This is not an ideal scenario,and while this example is highly extreme,it does indicate that we may not be optimizing what we think we are.3.3Possible Improvements•Clearly from above,a poisson arrival would be the obvious generaliza-tion of our model•Another added feature that would bring our model closer to reality would be to correlate the likelihood of a vehicle going through a booth to the distance from the lane the vehicle is on to the nearest free toll-booth•While there any many more possibilities,these seem to be the two key aspects of reality that we are ignoring.Team 851214ResultsIn order to obtain real data to test our model,we chose the Westlink M50toll plaza in Dublin,Ireland.Some of our team members had some limited personal experience of this particulartoll plaza.4.1Estimation of ParametersAs already mentioned in the assumptions,we restrict our attention to the two busiest hours,and approximate this as the parabola shown.TimeTrafficFlowOf course the change of flow will never be as uniform as this,so adding randomness to the model gives the following distribution for our rate of flow.On the Westlink M50,2lanes fan out into 5for the toll plaza,so in ournotation,m =5and n =2.From before,we require p <m nin order for congestion to occur at the constriction after the toll booths.Therefore,since mn=2.5,we must take p =1or 2so as to be able to apply our model.We have τ=p Θ,i.e.p relates how long one spends passing through the constriction to the amount of time to go through the toll booth,and so p =2is the more realistic value to take (intuitively,the time spent driving through the booth will be longer,since,for example,the car must stop to pay the toll).The length a particular vehicle could range anywhere,from 3.6meters for a Toyota Yaris for instance (see [12]),up to 25meters for large trucks(seeTeam 85122TimeTrafficFlow[13]).Θ=lv ,where l is the average vehicle length and v the average speedof a vehicle at the constriction,so taking Θ=4seconds or 1900hours is ing statistics relating to the number of vehicles which use the M50,we approximated the maximum traffic flow during the busy two hour period to be,on average,4000vehicles per hour.4.2Results from ProgramThe Mathematica programs in the appendix calculate the average waiting time t delay (in minutes)for a vehicle travelling through the toll system.Wefixed n =2,Θ=1900,and p =2,and then varied m and r ,the peak traffic flow,to get values for the waiting time.m took values from 2to 14,while r =2000,3000,4000,5000,6000.We obtained the data shown in the following tables:Team85123r m t delay20002 2.97507312.15334 1.58025 1.563336 1.587277 1.585218 1.558299 1.5582910 1.5582911 1.5582912 1.5582913 1.5582914 1.55829r m t delay3000274.78013 3.02861418.1931513.90786 2.26458718.2488818.2488918.24881018.24881118.24881218.24881318.24881418.2488Team85124r m t delay40002220.114342.35994 2.96445562.3328632.2738713.54568 3.01919937.74191037.74191137.74191237.74191337.74191437.7419r m t delay50002430.1953116.371429.7735110.105686.7116752.9446829.3646913.602110 3.70615110.02424541258.31831358.31831458.3183Team85125r m t delay60002706.5073217.076474.61985196.886111.3557113.582874.6693948.4941028.1131113.938712 4.46381130.2686461479.3435We now hadfive sets of fourteen data points(m,t delay).We had reckoned r to be4000on average,and so we applied the following weightings to the values for r,based on how likely that level of trafficflow would occur:r weighting20000.0130000.240000.5850000.260000.01We felt that the distributions offlows would be normal with mean4000, and that those weights chosen would approximate this:In each of thefive sets of data points corresponding to different values of r,the t delay was multiplied by the the respective weight.Finally,we summed the t delay components of corresponding points in allfive data sets to give one set of fourteen points.When plotted(see below),we get a graph of m versus the mean weighted delay time:Clearly,for our model of the Westlink M50with n=2roads,m=4and m=8minimize the delay time.The time difference is so small,and will fluctuate with the randomness in the model,so either number of roads will minimize delays.。
2010MCM&ICM特等奖论文全集

TheUMAP JournalPublisherCOMAP ,Inc.Vol.31,No.2Executive PublisherSolomon A.GarfunkelILAP EditorChris ArneyDept.of Math’l Sciencesitary AcademyWest Point,NY 10996david.arney@On Jargon EditorYves NievergeltDept.of MathematicsEastern Washington Univ.Cheney,WA 99004ynievergelt@Reviews EditorJames M.CargalMathematics Dept.Troy University—Montgomery Campus231Montgomery St.Montgomery,AL 36104jmcargal@Chief Operating Of ficerLaurie W.Arag´onProduction ManagerGeorge WardCopy EditorJulia CollinsDistributionJohn Tomicek Editor Paul J.Campbell Beloit College 700College St.Beloit,WI 53511–5595campbell@ Associate Editors Don Adolphson Chris Arney Aaron Archer Ron Barnes Arthur Benjamin Robert Bosch James M.Cargal Murray K.Clayton Lisette De Pillis James P .Fink Solomon A.Garfunkel William B.Gearhart William C.Giauque Richard Haberman Jon Jacobsen Walter Meyer Yves Nievergelt Michael O’Leary Catherine A.Roberts John S.Robertson Philip D.Straf fin J.T.Sutcliffe Brigham Young Univ.Army Research Of fice AT&T Shannon b.U.of Houston—Downtn Harvey Mudd College Oberlin College Troy U.—Montgomery U.of Wisc.—Madison Harvey Mudd College Gettysburg College COMAP ,Inc.Calif.State U.,Fullerton Brigham Young Univ.Southern Methodist U.Harvey Mudd College Adelphi University Eastern Washington U.Towson University College of the Holy Cross Georgia Military College Beloit College St.Mark’s School,DallasSubscription Rates for 2010 Calendar Year: Volume 31Institutional Web Memberships do not provide print materials. Web memberships allow members to search our online catalog, download COMAP print materials, and reproduce them for classroom use.(Domestic) #3030 $467 (Outside U.S.) #3030 $467Institutional Memberships receive print copies of The UMAP Journal quarterly, our annual CD collection UMAP Modules, Tools for Teaching, and our organizational newsletter Consortium.(Domestic) #3040 $312 (Outside U.S.) #3041 $351Institutional Plus Memberships receive print copies of the quarterly issues of The UMAP Journal, our annual CD collection UMAP Modules, Tools for Teaching, our organizational newsletter Consortium, and online membership that allows members to search our online catalog, download COMAP print materials, and reproduce them for classroom use.(Domestic) #3070 $615 (Outside U.S.) #3071 $659For individual membership options visit for more information.To order, send a check or money order to COMAP, or call toll-free1-800-77-COMAP (1-800-772-6627).The UMAP Journal is published quarterly by the Consortium for Mathematics and Its Applications (COMAP), Inc., Suite 3B, 175 Middlesex Tpke.,Bedford, MA, 01730, in coop-eration with the American Mathematical Association of Two-Y ear Colleges (AMATY C), the Mathematical Association of America (MAA), the National Council of Teachers of Mathematics (NCTM), the American Statistical Association (ASA), the Society for Industrial and Applied Mathematics (SIAM), and The Institute for Operations Re-search and the Management Sciences (INFORMS). The Journal acquaints readers with a wide variety of professional applications of the mathematical sciences and provides a forum for the discussion of new directions in mathematical education (ISSN 0197-3622).Periodical rate postage paid at Boston, MA and at additional mailing offices.Send address changes to: info@COMAP, Inc., Suite 3B, 175 Middlesex Tpke., Bedford, MA, 01730© Copyright 2010 by COMAP, Inc. All rights reserved.Mathematical Contest in Modeling (MCM)®, High School Mathematical Contest in Modeling (HiMCM)®, and Interdisciplinary Contest in Modeling(ICM)®are registered trade marks of COMAP, Inc.Vol.31,No.22010Table of ContentsEditorialThe Modeling Contests in2010Paul J.Campbell (93)MCM Modeling ForumResults of the2010Mathematical Contest in ModelingFrank R.Giordano (95)The Sweet Spot:A Wave Model of Baseball BatsYang Mou,Peter Diao,and Rajib Quabili (105)Judges’Commentary:The Outstanding Sweet Spot Papers Michael Tortorella (123)Centroids,Clusters,and Crime:Anchoring the Geographic Profiles of Serial CriminalsAnil S.Damle,Colin G.West,and Eric J.Benzel (129)Judges’Commentary:The Outstanding Geographic Profiling Papers Marie Vanisko (149)Judges’Commentary:The Fusaro Award for theGeographic Profiling ProblemMarie Vanisko and Peter Anspach (153)ICM Modeling ForumResults of the2010Interdisciplinary Contest in ModelingChris Arney (157)Shedding Light on Marine PollutionBrittany Harris,Chase Peaslee,and Kyle Perkins (165)Author’s Commentary:The Marine Pollution ProblemMiriam C.Goldstein (175)Judges’Commentary:The Outstanding Marine Pollution Papers Rodney Sturdivant (179)Editorial93EditorialThe2010Modeling ContestsPaul J.CampbellMathematics and Computer ScienceBeloit CollegeBeloit,WI53511–5595campbell@BackgroundBased on Ben Fusaro’s suggestion for an“applied Putnam”contest,in 1985COMAP introduced the Mathematical Contest in Modeling(MCM)R . Since then,this Journal has devoted an issue each year to the Outstanding contest papers.Even after substantial editing,that issue has sometimes run to more than three times the size of an ordinary issue.From2005through 2009,some papers appeared in electronic form only.The2,254MCM teams in2010was almost double the number in2008.Also,since the introduction in1999of the Interdisciplinary Contest in Modeling(ICM)R (which has separate funding and sponsorship from the MCM),the Journal has devoted a second of its four annual issues to Out-standing papers from that contest.A New Designation for PapersIt has become increasingly difficult to identify just a handful of Out-standing papers for each problem.After14Outstanding MCM teams in 2007,there have been9in each year since,despite more teams competing.The judges have been overwhelmed by increasing numbers of Merito-rious papers from which to select the truly Outstanding.As a result,this year there is a new designation of Finalist teams,between Outstanding and Meritorious.It recognizes the less than1%of papers that reached thefinal (seventh)round of judging but were not selected as Outstanding.Each Finalist paper displayed some modeling that distinguished it from the rest of the Meritorious papers.We think that the Finalist papers deserve special The UMAP Journal31(2)(2010)93–94.c Copyright2010by COMAP,Inc.All rights reserved. Permission to make digital or hard copies of part or all of this work for personal or classroom use is granted without fee provided that copies are not made or distributed for profit or commercial advantage and that copies bear this notice.Abstracting with credit is permitted,but copyrights for components of this work owned by others than COMAP must be honored.To copy otherwise, to republish,to post on servers,or to redistribute to lists requires prior permission from COMAP.94The UMAP Journal31.2(2010)recognition,and the mathematical professional societies are investigating ways to recognize the Finalist papers.Just One Contest Issue Each YearTaking up two of the four Journal issues each year,and sometimes two-thirds of the pages,the amount of material from the two contests has come to overbalance the other content of the Journal.The Executive Publisher,Sol Garfunkel,and I have decided to return more of the Journal to its original purpose,as set out30years ago,to: acquaint readers with a wide variety of professional applications of the mathematical sciences,and provide a forum for discussions of new directions in mathematical education.[Finney and Garfunkel1980,2–3] Henceforth,we plan to devote just a single issue of the Journal each year to the two contests combined.That issue—this issue—will appear during the summer and contain•reports on both contests,including the problem statements and names of the Outstanding teams and their members;•authors’,judges’,and practitioners’commentaries(as available)on the problems and the Outstanding papers;and•just one Outstanding paper from each problem.Available separately from COMAP on a CD-ROM very soon after the contests(as in2009and again this year)will be:•full original versions of all of the Outstanding papers,and•full results for all teams.Your RoleThe ever-increasing engagement of students in the contests has been astonishing;the steps above help us to cope with this success.There will now be more room in the Journal for material on mathemati-cal modeling,applications of mathematics,and ideas and perspectives on mathematics education at the collegiate level—articles,UMAP Modules, Minimodules,ILAP Modules,guest editorials.We look forward to your contribution.ReferenceFinney,Ross L.,and Solomon Garfunkel.1980.UMAP and The UMAP Jour-nal.The UMAP Journal0:1–4.Results of the2010MCM95 Modeling ForumResults of the2010Mathematical Contest in Modeling Frank R.Giordano,MCM DirectorNaval Postgraduate School1University CircleMonterey,CA93943–5000frgiorda@IntroductionA total of2,254teams of undergraduates from hundreds of institutions and departments in14countries,spent a weekend in February working on applied mathematics problems in the26th Mathematical Contest in Modeling(MCM)R .The2010MCM began at8:00P.M.EST on Thursday,February18,and ended at8:00P.M.EST on Monday,February22.During that time,teams of up to three undergraduates researched,modeled,and submitted a solution to one of two open-ended modeling problems.Students registered,obtained contest materials,downloaded the problem and data,and entered completion data through COMAP’s MCM Website.After a weekend of hard work,solution papers were sent to COMAP on Monday.Two of the top papers appear in this issue of The UMAP Journal,together with commentaries.In addition to this special issue of The UMAP Journal,COMAP has made available a special supplementary2010MCM-ICM CD-ROM containing the press releases for the two contests,the results,the problems,and original ver-sions of the Outstanding rmation about ordering the CD-ROM is at /product/cdrom/index.html or from(800) 772–6627.Results and winning papers from thefirst25contests were published in special issues of Mathematical Modeling(1985–1987)and The UMAP Journal (1985–2009).The1994volume of Tools for Teaching,commemorating the tenth anniversary of the contest,contains the20problems used in thefirst10years of the contest and a winning paper for each year.That volume and the special The UMAP Journal31(2)(2010)95–104.c Copyright2010by COMAP,Inc.All rights reserved. Permission to make digital or hard copies of part or all of this work for personal or classroom use is granted without fee provided that copies are not made or distributed for profit or commercial advantage and that copies bear this notice.Abstracting with credit is permitted,but copyrights for components of this work owned by others than COMAP must be honored.To copy otherwise, to republish,to post on servers,or to redistribute to lists requires prior permission from COMAP.96The UMAP Journal31.2(2010)MCM issues of the Journal for the last few years are available from COMAP.The 1994volume is also available on COMAP’s special Modeling Resource CD-ROM. Also available is The MCM at21CD-ROM,which contains the20problems from the second10years of the contest,a winning paper from each year,and advice from advisors of Outstanding teams.These CD-ROMs can be ordered from COMAP at /product/cdrom/index.html.This year,the two MCM problems represented significant challenges:•Problem A,“The Sweet Spot,”asked teams to explain why the spot on a baseball bat where maximum power is transferred to the ball is not at the end of the bat and to determine whether“corking”a bat(hollowing it out and replacing the hardwood with cork)enhances the“sweet spot”effect.•Problem B,“Criminology,”asked teams to develop geographical profiling to aid police infinding serial criminals.In addition to the MCM,COMAP also sponsors the Interdisciplinary Con-test in Modeling(ICM)R and the High School Mathematical Contest in Mod-eling(HiMCM)R :•The ICM runs concurrently with MCM and for the next several years will offer a modeling problem involving an environmental topic.Results of this year’s ICM are on the COMAP Website at / undergraduate/contests.The contest report,an Outstanding paper,and commentaries appear in this issue.•The HiMCM offers high school students a modeling opportunity similar to the MCM.Further details about the HiMCM are at ap.com/highschool/contests.2010MCM Statistics•2,254teams participated•15high school teams(<1%)•358U.S.teams(21%)•1,890foreign teams(79%),from Australia,Canada,China,Finland,Ger-many,Indonesia,Ireland,Jamaica,Malaysia,Pakistan,Singapore,South Africa,United Kingdom•9Outstanding Winners(<0.5%)•12Finalists(0.5%)•431Meritorious Winners(19%)•542Honorable Mentions(24%)•1,245Successful Participants(55%)Results of the2010MCM97Problem A:The Sweet SpotExplain the“sweet spot”on a baseball bat.Every hitter knows that there is a spot on the fat part of a baseball bat where maximum power is transferred to the ball when hit.Why isn’t this spot at the end of the bat?A simple explanation based on torque might seem to identify the end of the bat as the sweet spot,but this is known to be empirically incorrect.Develop a model that helps explain this empiricalfinding.Some players believe that“corking”a bat(hollowing out a cylinder in the head of the bat andfilling it with cork or rubber,then replacing a wood cap) enhances the“sweet spot”effect.Augment your model to confirm or deny this effect.Does this explain why Major League Baseball prohibits“corking”?Does the material out of which the bat is constructed matter?That is,does this model predict different behavior for wood(usually ash)or metal(usually aluminum)bats?Is this why Major League Baseball prohibits metal bats? Problem B:CriminologyIn1981,Peter Sutcliffe was convicted of13murders and subjecting a number of other people to vicious attacks.One of the methods used to narrow the search for Mr.Sutcliffe was tofind a“center of mass”of the locations of the attacks. In the end,the suspect happened to live in the same town predicted by this technique.Since that time,a number of more sophisticated techniques have been developed to determine the“geographical profile”of a suspected serial criminal based on the locations of the crimes.Your team has been asked by a local police agency to develop a method to aid in their investigations of serial criminals.The approach that you develop should make use of at least two different schemes to generate a geographical profile.You should develop a technique to combine the results of the different schemes and generate a useful prediction for law enforcement officers.The prediction should provide some kind of estimate or guidance about possible locations of the next crime based on the time and locations of the past crime scenes.If you make use of any other evidence in your estimate,you must provide specific details about how you incorporate the extra information.Your method should also provide some kind of estimate about how reliable the estimate will be in a given situation,including appropriate warnings.In addition to the required one-page summary,your report should include an additional two-page executive summary.The executive summary should provide a broad overview of the potential issues.It should provide an overview of your approach and describe situations when it is an appropriate tool and situations in which it is not an appropriate tool.The executive summary will be read by a chief of police and should include technical details appropriate to the intended audience.98The UMAP Journal31.2(2010)The ResultsThe solution papers were coded at COMAP headquarters so that names and affiliations of the authors would be unknown to the judges.Each paper was then read preliminarily by two“triage”judges at either Appalachian State University(Sweet Spot Problem)or at the National Security Agency(Crimi-nology Problem).At the triage stage,the summary and overall organization are the basis for judging a paper.If the judges’scores diverged for a paper,the judges conferred;if they still did not agree,a third judge evaluated the paper.AdditionalRegional Judging sites were created at the itary Academy and at the Naval Postgraduate School to support the growing number of contest submissions.Final judging took place at the Naval Postgraduate School,Monterey,CA. The judges classified the papers as follows:Honorable SuccessfulOutstanding Finalist Meritorious Mention Participation Total Sweet Spot Problem45180217533939 Criminology Problem57251325712130091243154212452239 We list here the9teams that the judges designated as Outstanding;the list of all participating schools,advisors,and results is at the COMAP Website.Outstanding TeamsInstitution and Advisor Team MembersSweet Spot Problem“An Optimal Model of‘Sweet Spot’Effect”Huazhong University of Science andTechnology Wuhan,Hubei,China Liang Gao Zhe Xiong Qipei Mei Fei Han“The Sweet Spot:A Wave Model of Baseball Bats”Princeton University Princeton,NJ Robert Calderbank Yang Mou Peter Diao Rajib QuabiliResults of the2010MCM99“Brody Power Model:An Analysis of Baseball’s‘Sweet Spot’”itary Academy West Point,NY Elizabeth Schott David CovellBen Garlick Chandler Williams“An Identification of‘Sweet Spot’”Zhejiang University Hangzhou,China Xinxin XuCong ZhaoYuguang YangZuogong Yue Criminology Papers“Predicting a Serial Criminal’s Next Crime Location Using Geographic Profiling”Bucknell University Lewisburg,PA Nathan C.Ryan Bryan Ward Ryan Ward Dan Cavallaro“Following the Trail of Data”Rensselaer Polytechnic Institute Troy,NYPeter R.Kramer Yonatan Naamad Joseph H.Gibney Emily P.Meissen“From Kills to Kilometers:Using Centrographic Techniques and Rational Choice Theory forGeographical Profiling of Serial Killers”Tufts University Medford,MA Scott MacLachlan Daniel Brady Liam Clegg Victor Minden“Centroids,Clusters,and Crime:Anchoring the Geographic Profile of Serial Criminals”University of Colorado—Boulder Boulder,COAnne M.Dougherty Anil S.Damle Colin G.West Eric J.Benzel“Tracking Serial Criminals with a Road Metric”University of Washington Seattle,WAJames Allen Morrow Ian Zemke Mark Bun Jerry Li100The UMAP Journal31.2(2010)Awards and ContributionsEach participating MCM advisor and team member received a certificate signed by the Contest Director and the appropriate Head Judge.INFORMS,the Institute for Operations Research and the Management Sciences,recognized the teams from Princeton University(Sweet Spot Prob-lem)and Tufts University(Criminology Problem)as INFORMS Outstand-ing teams and provided the following recognition:•a letter of congratulations from the current president of INFORMS to each team member and to the faculty advisor;•a check in the amount of$300to each team member;•a bronze plaque for display at the team’s institution,commemorating team members’achievement;•individual certificates for team members and faculty advisor as a per-sonal commemoration of this achievement;and•a one-year student membership in INFORMS for each team member, which includes their choice of a professional journal plus the OR/MS Today periodical and the INFORMS newsletter.The Society for Industrial and Applied Mathematics(SIAM)designated one Outstanding team from each problem as a SIAM Winner.The teams were from Huazhong University of Science and Technology(Sweet Spot Problem)and Rensselaer PolytechnicInstitute(CriminologyProblem).Each of the team members was awarded a$300cash prize,and the teams received partial expenses to present their results in a special Minisymposium at the SIAM Annual Meeting in Pittsburgh,PA in July.Their schools were given a framed hand-lettered certificate in gold leaf.The Mathematical Association of America(MAA)designated one Out-standing North American team from each problem as an MAA Winner. The teams were from the itary Academy(Sweet Spot Problem)and the University of Colorado—Boulder(Criminology Problem).With partial travel support from the MAA,the teams presented their solution at a spe-cial session of the MAA Mathfest in Pittsburgh,PA in August.Each team member was presented a certificate by an official of the MAA Committee on Undergraduate Student Activities and Chapters.Ben Fusaro AwardOne Meritorious or Outstanding paper was selected for each problem for the Ben Fusaro Award,named for the Founding Director of the MCM and awarded for the seventh time this year.It recognizes an especially creative approach;details concerning the award,its judging,and Ben Fusaro are inResults of the2010MCM101 Vol.25(3)(2004):195–196.The Ben Fusaro Award winners were Prince-ton University(Sweet Spot Problem)and Duke University(Criminology Problem).A commentary on the latter appears in this issue.JudgingDirectorFrank R.Giordano,Naval Postgraduate School,Monterey,CA Associate DirectorWilliam P.Fox,Dept.of Defense Analysis,Naval Postgraduate School, Monterey,CASweet Spot ProblemHead JudgeMarvin S.Keener,Executive Vice-President,Oklahoma State University, Stillwater,OKAssociate JudgesWilliam C.Bauldry,Chair,Dept.of Mathematical Sciences,Appalachian State University,Boone,NC(Head Triage Judge)Patrick J.Driscoll,Dept.of Systems Engineering,itary Academy, West Point,NY(INFORMS Judge)J.Douglas Faires,Youngstown State University,Youngstown,OHBen Fusaro,Dept.of Mathematics,Florida State University,Tallahassee,FL (SIAM Judge)Michael Jaye,Dept.of Mathematical Sciences,Naval Postgraduate School, Monterey,CAJohn L.Scharf,Mathematics Dept.,Carroll College,Helena,MT (MAA Judge)Michael Tortorella,Dept.of Industrial and Systems Engineering, Rutgers University,Piscataway,NJ(Problem Author)Richard Douglas West,Francis Marion University,Florence,SC Criminology ProblemHead JudgeMaynard Thompson,Mathematics Dept.,University of Indiana, Bloomington,INAssociate JudgesPeter Anspach,National Security Agency,Ft.Meade,MD(Head Triage Judge)Kelly Black,Mathematics Dept.,Union College,Schenectady,NY102The UMAP Journal31.2(2010)Jim Case(SIAM Judge)William P.Fox,Dept.of Defense Analysis,Naval Postgraduate School, Monterey,CAFrank R.Giordano,Naval Postgraduate School,Monterey,CAVeena Mendiratta,Lucent Technologies,Naperville,ILDavid H.Olwell,Naval Postgraduate School,Monterey,CAMichael O’Leary,Towson State University,Towson,MD(Problem Author) Kathleen M.Shannon,Dept.of Mathematics and Computer Science, Salisbury University,Salisbury,MD(MAA Judge)Dan Solow,Case Western Reserve University,Cleveland,OH (INFORMS Judge)Marie Vanisko,Dept.of Mathematics,Carroll College,Helena,MT (Ben Fusaro Award Judge)Regional Judging Session at itary AcademyHead JudgesPatrick J.Driscoll,Dept.of Systems Engineering,United States Military Academy(USMA),West Point,NYAssociate JudgesTim Elkins,Dept.of Systems Engineering,USMADarrall Henderson,Sphere Consulting,LLCSteve Horton,Dept.of Mathematical Sciences,USMATom Meyer,Dept.of Mathematical Sciences,USMAScott Nestler,Dept.of Mathematical Sciences,USMARegional Judging Session at Naval Postgraduate SchoolHead JudgesWilliam P.Fox,Dept.of Defense Analysis,Naval Postgraduate School, Monterey,CAFrank R. Giordano,Naval Postgraduate School,Monterey,CA Associate JudgesMatt Boensel,Robert Burks,Peter Gustaitis,Michael Jaye,and Greg Mislick —all from the Naval Postgraduate School,Monterey,CATriage Session for Sweet Spot ProblemHead Triage JudgeWilliam C.Bauldry,Chair,Dept.of Mathematical Sciences,Appalachian State University,Boone,NCAssociate JudgesJeffry Hirst,Greg Rhoads,and Kevin Shirley—all from Dept.of Mathematical Sciences,Appalachian State University, Boone,NCResults of the2010MCM103Triage Session for Criminology ProblemHead Triage JudgePeter Anspach,National Security Agency(NSA),Ft.Meade,MD Associate JudgesJim CaseOther judges from inside and outside NSA,who wish not to be named.Sources of the ProblemsThe Sweet Spot Problem was contributed by Michael Tortorella(Rutgers University),and the Criminology Problem by Michael O’Leary(Towson University)and Kelly Black(Clarkson University). AcknowledgmentsMajor funding for the MCM is provided by the National Security Agency (NSA)and by COMAP.Additional support is provided by the Institute for Operations Research and the Management Sciences(INFORMS),the Soci-ety for Industrial and Applied Mathematics(SIAM),and the Mathematical Association of America(MAA).We are indebted to these organizations for providing judges and prizes.We also thank for their involvement and support the MCM judges and MCM Board members for their valuable and unflagging efforts,as well as •Two Sigma Investments.(This group of experienced,analytical,and technicalfinancial professionals based in New York builds and operates sophisticated quantitative trading strategies for domestic and interna-tional markets.Thefirm is successfully managing several billion dol-lars using highly-automated trading technologies.For more information about Two Sigma,please visit .)•Jane Street Capital,LLC.(This proprietary tradingfirm operates around the clock and around the globe.“We bring a deep understanding of markets,a scientific approach,and innovative technology to bear on the problem of trading profitably in the world’s highly competitivefinancial markets,focusing primarily on equities and equity derivatives.Founded in2000,Jane Street employes over200people in offices in new York,Lon-don,and Tokyo.Our entrepreneurial culture is driven by our talented team of traders and programmers.”For more information about Jane Street Capital,please visit .)104The UMAP Journal31.2(2010)CautionsTo the reader of research journals:Usually a published paper has been presented to an audience,shown to colleagues,rewritten,checked by referees,revised,and edited by a jour-nal editor.Each paper here is the result of undergraduates working on a problem over a weekend.Editing(and usually substantial cutting)has taken place;minor errors have been corrected,wording altered for clarity or economy,and style adjusted to that of The UMAP Journal.The student authors have proofed the results.Please peruse their efforts in that context.To the potential MCM Advisor:It might be overpowering to encounter such output from a weekend of work by a small team of undergraduates,but these solution papers are highly atypical.A team that prepares and participates will have an enrich-ing learning experience,independent of what any other team does.COMAP’s MathematicalContest in Modeling and InterdisciplinaryCon-test in Modeling are the only international modeling contests in which students work in teams.Centering its educational philosophy on mathe-matical modeling,COMAP uses mathematical tools to explore real-world problems.It serves the educational community as well as the world of work by preparing students to become better-informed and better-prepared citi-zens.Editor’s NoteThe complete roster of participating teams and results has become too long to reproduce in the printed copy of the Journal.It can now be found at the COMAP Website,in separatefiles for each problem:/undergraduate/contests/mcm/contests/ 2010/results/2010_MCM_Problem_A.pdf/undergraduate/contests/mcm/contests/ 2010/results/2010_MCM_Problem_B.pdf。
美国大学生数学建模竞赛二等奖论文

美国⼤学⽣数学建模竞赛⼆等奖论⽂The P roblem of R epeater C oordination SummaryThis paper mainly focuses on exploring an optimization scheme to serve all the users in a certain area with the least repeaters.The model is optimized better through changing the power of a repeater and distributing PL tones,frequency pairs /doc/d7df31738e9951e79b8927b4.html ing symmetry principle of Graph Theory and maximum coverage principle,we get the most reasonable scheme.This scheme can help us solve the problem that where we should put the repeaters in general cases.It can be suitable for the problem of irrigation,the location of lights in a square and so on.We construct two mathematical models(a basic model and an improve model)to get the scheme based on the relationship between variables.In the basic model,we set a function model to solve the problem under a condition that assumed.There are two variables:‘p’(standing for the power of the signals that a repeater transmits)and‘µ’(standing for the density of users of the area)in the function model.Assume‘p’fixed in the basic one.And in this situation,we change the function model to a geometric one to solve this problem.Based on the basic model,considering the two variables in the improve model is more reasonable to most situations.Then the conclusion can be drawn through calculation and MATLAB programming.We analysis and discuss what we can do if we build repeaters in mountainous areas further.Finally,we discuss strengths and weaknesses of our models and make necessary recommendations.Key words:repeater maximum coverage density PL tones MATLABContents1.Introduction (3)2.The Description of the Problem (3)2.1What problems we are confronting (3)2.2What we do to solve these problems (3)3.Models (4)3.1Basic model (4)3.1.1Terms,Definitions,and Symbols (4)3.1.2Assumptions (4)3.1.3The Foundation of Model (4)3.1.4Solution and Result (5)3.1.5Analysis of the Result (8)3.1.6Strength and Weakness (8)3.1.7Some Improvement (9)3.2Improve Model (9)3.2.1Extra Symbols (10)Assumptions (10)3.2.2AdditionalAdditionalAssumptions3.2.3The Foundation of Model (10)3.2.4Solution and Result (10)3.2.5Analysis of the Result (13)3.2.6Strength and Weakness (14)4.Conclusions (14)4.1Conclusions of the problem (14)4.2Methods used in our models (14)4.3Application of our models (14)5.Future Work (14)6.References (17)7.Appendix (17)Ⅰ.IntroductionIn order to indicate the origin of the repeater coordination problem,the following background is worth mentioning.With the development of technology and society,communications technology has become much more important,more and more people are involved in this.In order to ensure the quality of the signals of communication,we need to build repeaters which pick up weak signals,amplify them,and retransmit them on a different frequency.But the price of a repeater is very high.And the unnecessary repeaters will cause not only the waste of money and resources,but also the difficulty of maintenance.So there comes a problem that how to reduce the number of unnecessary repeaters in a region.We try to explore an optimized model in this paper.Ⅱ.The Description of the Problem2.1What problems we are confrontingThe signals transmit in the way of line-of-sight as a result of reducing the loss of the energy. As a result of the obstacles they meet and the natural attenuation itself,the signals will become unavailable.So a repeater which just picks up weak signals,amplifies them,and retransmits them on a different frequency is needed.However,repeaters can interfere with one another unless they are far enough apart or transmit on sufficiently separated frequencies.In addition to geographical separation,the“continuous tone-coded squelch system”(CTCSS),sometimes nicknamed“private line”(PL),technology can be used to mitigate interference.This system associates to each repeater a separate PL tone that is transmitted by all users who wish to communicate through that repeater. The PL tone is like a kind of password.Then determine a user according to the so called password and the specific frequency,in other words a user corresponds a PL tone(password)and a specific frequency.Defects in line-of-sight propagation caused by mountainous areas can also influence the radius.2.2What we do to solve these problemsConsidering the problem we are confronting,the spectrum available is145to148MHz,the transmitter frequency in a repeater is either600kHz above or600kHz below the receiver frequency.That is only5users can communicate with others without interferences when there’s noPL.The situation will be much better once we have PL.However the number of users that a repeater can serve is limited.In addition,in a flat area ,the obstacles such as mountains ,buildings don’t need to be taken into account.Taking the natural attenuation itself is reasonable.Now the most important is the radius that the signals transmit.Reducing the radius is a good way once there are more users.With MATLAB and the method of the coverage in Graph Theory,we solve this problem as follows in this paper.Ⅲ.Models3.1Basic model3.1.1Terms,Definitions,and Symbols3.1.2Assumptions●A user corresponds a PLz tone (password)and a specific frequency.●The users in the area are fixed and they are uniform distribution.●The area that a repeater covers is a regular hexagon.The repeater is in the center of the regular hexagon.●In a flat area ,the obstacles such as mountains ,buildings don’t need to be taken into account.We just take the natural attenuation itself into account.●The power of a repeater is fixed.3.1.3The Foundation of ModelAs the number of PLz tones (password)and frequencies is fixed,and a user corresponds a PLz tone (password)and a specific frequency,we can draw the conclusion that a repeater can serve the limited number of users.Thus it is clear that the number of repeaters we need relates to the density symboldescriptionLfsdfminrpµloss of transmission the distance of transmission operating frequency the number of repeaters that we need the power of the signals that a repeater transmits the density of users of the areaof users of the area.The radius of the area that a repeater covers is also related to the ratio of d and the radius of the circular area.And d is related to the power of a repeater.So we get the model of function()min ,r f p µ=If we ignore the density of users,we can get a Geometric model as follows:In a plane which is extended by regular hexagons whose side length are determined,we move a circle until it covers the least regular hexagons.3.1.4Solution and ResultCalculating the relationship between the radius of the circle and the side length of the regular hexagon.[]()()32.4420lg ()20lg Lfs dB d km f MHz =++In the above formula the unit of ’’is .Lfs dB The unit of ’’is .d Km The unit of ‘‘is .f MHz We can conclude that the loss of transmission of radio is decided by operating frequency and the distance of transmission.When or is as times as its former data,will increase f d 2[]Lfs .6dB Then we will solve the problem by using the formula mentioned above.We have already known the operating frequency is to .According to the 145MHz 148MHz actual situation and some authority material ,we assume a system whose transmit power is and receiver sensitivity is .Thus we can conclude that ()1010dBm mW +106.85dBm ?=.Substituting and to the above formula,we can get the Lfs 106.85dBm ?145MHz 148MHz average distance of transmission .()6.4d km =4mile We can learn the radius of the circle is 40mile .So we can conclude the relationship between the circle and the side length of regular hexagon isR=10d.1)The solution of the modelIn order to cover a certain plane with the least regular hexagons,we connect each regular hexagon as the honeycomb.We use A(standing for a figure)covers B(standing for another figure), only when As don’t overlap each other,the number of As we use is the smallest.Figure1According to the Principle of maximum flow of Graph Theory,the better of the symmetry ofthe honeycomb,the bigger area that it covers(Fig1).When the geometric centers of the circle andthe honeycomb which can extend are at one point,extend the honeycomb.Then we can get Fig2,Fig4:Figure2Fig3demos the evenly distribution of users.Figure4Now prove the circle covers the least regular hexagons.Look at Fig5.If we move the circle slightly as the picture,you can see three more regular hexagons are needed.Figure 52)ResultsThe average distance of transmission of the signals that a repeater transmit is 4miles.1000users can be satisfied with 37repeaters founded.3.1.5Analysis of the Result1)The largest number of users that a repeater can serveA user corresponds a PL and a specific frequency.There are 5wave bands and 54different PL tones available.If we call a code include a PL and a specific frequency,there are 54*5=270codes.However each code in two adjacent regular hexagons shouldn’t be the same in case of interfering with each other.In order to have more code available ,we can distribute every3adjacent regular hexagons 90codes each.And that’s the most optimized,because once any of the three regular hexagons have more codes,it will interfere another one in other regular hexagon.2)Identify the rationality of the basic modelNow we considering the influence of the density of users,according to 1),90*37=3330>1000,so here the number of users have no influence on our model.Our model is rationality.3.1.6Strength and Weakness●Strength:In this paper,we use the model of honeycomb-hexagon structure can maximize the use of resources,avoiding some unnecessary interference effectively.It is much more intuitive once we change the function model to the geometric model.●Weakness:Since each hexagon get too close to another one.Once there are somebuildingsor terrain fluctuations between two repeaters,it can lead to the phenomenon that certain areas will have no signals.In addition,users are distributed evenly is not reasonable.The users are moving,for example some people may get a party.3.1.7Some ImprovementAs we all know,the absolute evenly distribution is not exist.So it is necessary to say something about the normal distribution model.The maximum accommodate number of a repeater is 5*54=270.As for the first model,it is impossible that 270users are communicating in a same repeater.Look at Fig 6.If there are N people in the area 1,the maximum number of the area 2to area 7is 3*(270-N).As 37*90=3330is much larger than 1000,our solution is still reasonable to this model.Figure 63.2Improve Model3.2.1Extra SymbolsSigns and definitions indicated above are still valid.Here are some extra signs and definitions.symboldescription Ra the radius of the circular flat area the side length of a regular hexagon3.2.2Additional AdditionalAssumptionsAssumptions ●The radius that of a repeater covers is adjustable here.●In some limited situations,curved shape is equal to straight line.●Assumptions concerning the anterior process are the same as the Basic Model3.2.3The Foundation of ModelThe same as the Basic Model except that:We only consider one variable(p)in the function model of the basic model ;In this model,we consider two varibles(p and µ)of the function model.3.2.4Solution and Result1)SolutionIf there are 10,000users,the number of regular hexagons that we need is at least ,thus according to the the Principle of maximum flow of Graph Theory,the 10000111.1190=result that we draw needed to be extended further.When the side length of the figure is equal to 7Figure 7regular hexagons,there are 127regular hexagons (Fig 7).Assuming the side length of a regular hexagon is ,then the area of a regular hexagon is a .The area of regular hexagons is equal to a circlewhose radiusis 22a =1000090R.Then according to the formula below:.221000090a R π=We can get.9.5858R a =Mapping with MATLAB as below (Fig 8):Figure 82)Improve the model appropriatelyEnlarge two part of the figure above,we can get two figures below (Fig 9and Fig 10):Figure 9AREAFigure 10Look at the figure above,approximatingAREA a rectangle,then obtaining its area to getthe number of users..The length of the rectangle is approximately equal to the side length of the regular hexagon ,athe width of the rectangle is ,thus the area of AREA is ,then R ?*R awe can get the number of users in AREA is(),2**10000 2.06R a R π=????????9.5858R a =As 2.06<<10,000,2.06can be ignored ,so there is no need to set up a repeater in.There are 6suchareas(92,98,104,110,116,122)that can be ignored.At last,the number of repeaters we should set up is,1276121?=2)Get the side length of the regular hexagon of the improved modelThus we can getmile=km 40 4.1729.5858a == 1.6* 6.675a =3)Calculate the power of a repeaterAccording to the formula[]()()32.4420lg ()20lg Lfs dB d km f MHz =++We get32.4420lg 6.67520lg14592.156Los =++=32.4420lg 6.67520lg14892.334Los =++=So we get106.85-92.156=14.694106.85-92.334=14.516As the result in the basic model,we can get the conclusion the power of a repeater is from 14.694mW to 14.516mW.3.2.5Analysis of the ResultAs 10,000users are much more than 1000users,the distribution of the users is more close toevenly distribution.Thus the model is more reasonable than the basic one.More repeaters are built,the utilization of the outside regular hexagon are higher than the former one.3.2.6Strength and Weakness●Strength:The model is more reasonable than the basic one.●Weakness:Repeaters don’t cover all the area,some places may not receive signals.And thefoundation of this model is based on the evenly distribution of the users in the area,if the situation couldn’t be satisfied,the interference of signals will come out.Ⅳ.Conclusions4.1Conclusions of the problem●Generally speaking,the radius of the area that a repeater covers is4miles in our basic model.●Using the model of honeycomb-hexagon structure can maximize the use of resources,avoiding some unnecessary interference effectively.●The minimum number of repeaters necessary to accommodate1,000simultaneous users is37.The minimum number of repeaters necessary to accommodate10,000simultaneoususers is121.●A repeater's coverage radius relates to external environment such as the density of users andobstacles,and it is also determined by the power of the repeater.4.2Methods used in our models●Analysis the problem with MATLAB●the method of the coverage in Graph Theory4.3Application of our models●Choose the ideal address where we set repeater of the mobile phones.●How to irrigate reasonably in agriculture.●How to distribute the lights and the speakers in squares more reasonably.Ⅴ.Future WorkHow we will do if the area is mountainous?5.1The best position of a repeater is the top of the mountain.As the signals are line-of-sight transmission and reception.We must find a place where the signals can transmit from the repeater to users directly.So the top of the mountain is a good place.5.2In mountainous areas,we must increase the number of repeaters.There are three reasons for this problem.One reason is that there will be more obstacles in the mountainous areas. The signals will be attenuated much more quickly than they transmit in flat area.Another reason is that the signals are line-of-sight transmission and reception,we need more repeaters to satisfy this condition.Then look at Fig11and Fig12,and you will know the third reason.It can be clearly seen that hypotenuse is larger than right-angleFig11edge(R>r).Thus the radius will become smaller.In this case more repeaters are needed.Fig125.3In mountainous areas,people may mainly settle in the flat area,so the distribution of users isn’t uniform.5.4There are different altitudes in the mountainous areas.So in order to increase the rate of resources utilization,we can set up the repeaters in different altitudes.5.5However,if there are more repeaters,and some of them are on mountains,more money will be/doc/d7df31738e9951e79b8927b4.html munication companies will need a lot of money to build them,repair them when they don’t work well and so on.As a result,the communication costs will be high.What’s worse,there are places where there are many mountains but few persons. Communication companies reluctant to build repeaters there.But unexpected things often happen in these places.When people are in trouble,they couldn’t communicate well with the outside.So in my opinion,the government should take some measures to solve this problem.5.6Another new method is described as follows(Fig13):since the repeater on high mountains can beFig13Seen easily by people,so the tower which used to transmit and receive signals can be shorter.That is to say,the tower on flat areas can be a little taller..Ⅵ.References[1]YU Fei,YANG Lv-xi,"Effective cooperative scheme based on relay selection",SoutheastUniversity,Nanjing,210096,China[2]YANG Ming,ZHAO Xiao-bo,DI Wei-guo,NAN Bing-xin,"Call Admission Control Policy based on Microcellular",College of Electical and Electronic Engineering,Shijiazhuang Railway Institute,Shijiazhuang Heibei050043,China[3]TIAN Zhisheng,"Analysis of Mechanism of CTCSS Modulation",Shenzhen HYT Co,Shenzhen,518057,China[4]SHANGGUAN Shi-qing,XIN Hao-ran,"Mathematical Modeling in Bass Station Site Selectionwith Lingo Software",China University of Mining And Technology SRES,Xuzhou;Shandong Finance Institute,Jinan Shandon,250014[5]Leif J.Harcke,Kenneth S.Dueker,and David B.Leeson,"Frequency Coordination in the AmateurRadio Emergency ServiceⅦ.AppendixWe use MATLAB to get these pictures,the code is as follows:1-clc;clear all;2-r=1;3-rc=0.7;4-figure;5-axis square6-hold on;7-A=pi/3*[0:6];8-aa=linspace(0,pi*2,80);9-plot(r*exp(i*A),'k','linewidth',2);10-g1=fill(real(r*exp(i*A)),imag(r*exp(i*A)),'k');11-set(g1,'FaceColor',[1,0.5,0])12-g2=fill(real(rc*exp(i*aa)),imag(rc*exp(i*aa)),'k');13-set(g2,'FaceColor',[1,0.5,0],'edgecolor',[1,0.5,0],'EraseMode','x0r')14-text(0,0,'1','fontsize',10);15-Z=0;16-At=pi/6;17-RA=-pi/2;18-N=1;At=-pi/2-pi/3*[0:6];19-for k=1:2;20-Z=Z+sqrt(3)*r*exp(i*pi/6);21-for pp=1:6;22-for p=1:k;23-N=N+1;24-zp=Z+r*exp(i*A);25-zr=Z+rc*exp(i*aa);26-g1=fill(real(zp),imag(zp),'k');27-set(g1,'FaceColor',[1,0.5,0],'edgecolor',[1,0,0]);28-g2=fill(real(zr),imag(zr),'k');29-set(g2,'FaceColor',[1,0.5,0],'edgecolor',[1,0.5,0],'EraseMode',xor';30-text(real(Z),imag(Z),num2str(N),'fontsize',10);31-Z=Z+sqrt(3)*r*exp(i*At(pp));32-end33-end34-end35-ezplot('x^2+y^2=25',[-5,5]);%This is the circular flat area of radius40miles radius 36-xlim([-6,6]*r) 37-ylim([-6.1,6.1]*r)38-axis off;Then change number19”for k=1:2;”to“for k=1:3;”,then we get another picture:Change the original programme number19“for k=1:2;”to“for k=1:4;”,then we get another picture:。
历年美赛数学建模优秀论文大全
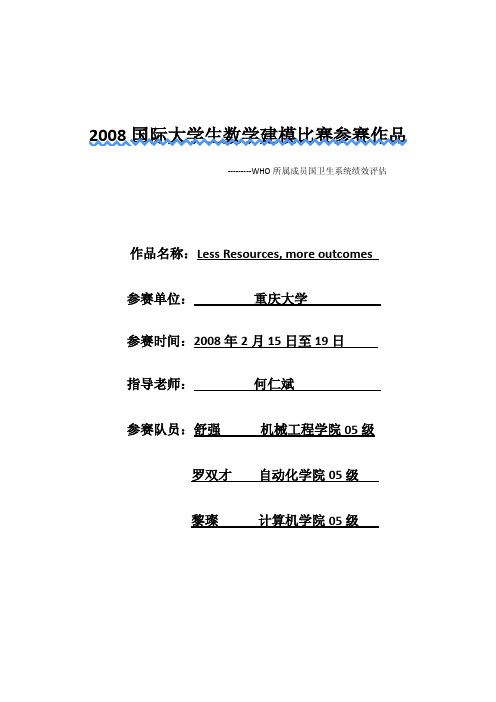
2008国际大学生数学建模比赛参赛作品---------WHO所属成员国卫生系统绩效评估作品名称:Less Resources, more outcomes参赛单位:重庆大学参赛时间:2008年2月15日至19日指导老师:何仁斌参赛队员:舒强机械工程学院05级罗双才自动化学院05级黎璨计算机学院05级ContentLess Resources, More Outcomes (4)1. Summary (4)2. Introduction (5)3. Key Terminology (5)4. Choosing output metrics for measuring health care system (5)4.1 Goals of Health Care System (6)4.2 Characteristics of a good health care system (6)4.3 Output metrics for measuring health care system (6)5. Determining the weight of the metrics and data processing (8)5.1 Weights from statistical data (8)5.2 Data processing (9)6. Input and Output of Health Care System (9)6.1 Aspects of Input (10)6.2 Aspects of Output (11)7. Evaluation System I : Absolute Effectiveness of HCS (11)7.1Background (11)7.2Assumptions (11)7.3Two approaches for evaluation (11)1. Approach A : Weighted Average Evaluation Based Model (11)2. Approach B: Fuzzy Comprehensive Evaluation Based Model [19][20] (12)7.4 Applying the Evaluation of Absolute Effectiveness Method (14)8. Evaluation system II: Relative Effectiveness of HCS (16)8.1 Only output doesn’t work (16)8.2 Assumptions (16)8.3 Constructing the Model (16)8.4 Applying the Evaluation of Relative Effectiveness Method (17)9. EAE VS ERE: which is better? (17)9.1 USA VS Norway (18)9.2 USA VS Pakistan (18)10. Less Resources, more outcomes (19)10.1Multiple Logistic Regression Model (19)10.1.1 Output as function of Input (19)10.1.2Assumptions (19)10.1.3Constructing the model (19)10.1.4. Estimation of parameters (20)10.1.5How the six metrics influence the outcomes? (20)10.2 Taking USA into consideration (22)10.2.1Assumptions (22)10.2.2 Allocation Coefficient (22)10.3 Scenario 1: Less expenditure to achieve the same goal (24)10.3.1 Objective function: (24)10.3.2 Constraints (25)10.3.3 Optimization model 1 (25)10.3.4 Solutions of the model (25)10.4. Scenario2: More outcomes with the same expenditure (26)10.4.1Objective function (26)10.4.2Constraints (26)10.4.3 Optimization model 2 (26)10.4.4Solutions to the model (27)15. Strengths and Weaknesses (27)Strengths (27)Weaknesses (27)16. References (28)Less Resources, More Outcomes1. SummaryIn this paper, we regard the health care system (HCS) as a system with input and output, representing total expenditure on health and its goal attainment respectively. Our goal is to minimize the total expenditure on health to archive the same or maximize the attainment under given expenditure.First, five output metrics and six input metrics are specified. Output metrics are overall level of health, distribution of health in the population,etc. Input metrics are physician density per 1000 population, private prepaid plans as % private expenditure on health, etc.Second, to evaluate the effectiveness of HCS, two evaluation systems are employed in this paper:●Evaluation of Absolute Effectiveness(EAE)This evaluation system only deals with the output of HCS,and we define Absolute Total Score (ATS) to quantify the effectiveness. During the evaluation process, weighted average sum of the five output metrics is defined as ATS, and the fuzzy theory is also employed to help assess HCS.●Evaluation of Relative Effectiveness(ERE)This evaluation system deals with the output as well as its input, and also we define Relative Total Score (RTS) to quantify the effectiveness. The measurement to ATS is units of output produced by unit of input.Applying the two kinds of evaluation system to evaluate HCS of 34 countries (USA included), we can find some countries which rank in a higher position in EAE get a relatively lower rank in ERE, such as Norway and USA, indicating that their HCS should have been able to archive more under their current resources .Therefore, taking USA into consideration, we try to explore how the input influences the output and archive the goal: less input, more output. Then three models are constructed to our goal:●Multiple Logistic RegressionWe model the output as function of input by the logistic equation. In more detains, we model ATS (output) as the function of total expenditure on health system. By curve fitting, we estimate the parameters in logistic equation, and statistical test presents us a satisfactory result.●Linear Optimization Model on minimizing the total expenditure on healthWe try to minimize the total expenditure and at the same time archive the same, that is to get a ATS of 0.8116. We employ software to solve the model, and by the analysis of the results. We cut it to 2023.2 billion dollars, compared to the original data 2109.8 billion dollars.●Linear Optimization Model on maximizing the attainment. We try to maximize the attainment (absolute total score) under the same total expenditure in2007.And we optimize the ATS to 0.8823, compared to the original data 0.8116.Finally, we discuss strengths and weaknesses of our models and make necessary recommendations to the policy-makers。
2012年美国大学生数学建模竞赛B题特等奖文章翻译要点

2012年美赛B题题目翻译:到Big Long River(225英里)游玩的游客可以享受那里的风景和振奋人心的急流。
远足者没法到达这条河,唯一去的办法是漂流过去。
这需要几天的露营。
河流旅行始于First Launch,在Final Exit结束,共225英里的顺流。
旅客可以选择依靠船桨来前进的橡皮筏,它的速度是4英里每小时,或者选择8英里每小时的摩托船。
旅行从开始到结束包括大约6到18个晚上的河中的露营。
负责管理这条河的政府部门希望让每次旅行都能尽情享受野外经历,同时能尽量少的与河中其他的船只相遇。
当前,每年经过Big Long河的游客有X组,这些漂流都在一个为期6个月时期内进行,一年中的其他月份非常冷,不会有漂流。
在Big Long上有Y处露营地点,平均分布于河廊。
随着漂流人数的增加,管理者被要求应该允许让更多的船只漂流。
他们要决定如何来安排最优的方案:包括旅行时间(以在河上的夜晚数计算)、选择哪种船(摩托还是桨船),从而能够最好地利用河中的露营地。
换句话说,Big Long River在漂流季节还能增加多少漂流旅行数?管理者希望你能给他们最好的建议,告诉他们如何决定河流的容纳量,记住任两组旅行队都不能同时占据河中的露营地。
此外,在你的摘要表一页,准备一页给管理者的备忘录,用来描述你的关键发现。
沿着大朗河露营摘要我们开发了一个模型来安排沿大河的行程。
我们的目标是为了优化乘船旅行的时间,从而使6个月的旅游旺季出游人数最大化。
我们模拟团体从营地到营地旅行的过程。
根据给定的约束条件,我们的算法输出了每组沿河旅行最佳的日程安排。
通过研究算法的长期反应,我们可以计算出旅行的最大数量,我们定义为河流的承载能力。
我们的算法适应于科罗多拉大峡谷的个案分析,该问题的性质与大长河问题有许多共同之处。
最后,我们考察当改变推进方法,旅程时间分布,河上的露营地数量时承载能力的变化的敏感性。
我们解决了使沿大朗河出游人数最大化的休闲旅行计划。
- 1、下载文档前请自行甄别文档内容的完整性,平台不提供额外的编辑、内容补充、找答案等附加服务。
- 2、"仅部分预览"的文档,不可在线预览部分如存在完整性等问题,可反馈申请退款(可完整预览的文档不适用该条件!)。
- 3、如文档侵犯您的权益,请联系客服反馈,我们会尽快为您处理(人工客服工作时间:9:00-18:30)。
Team Control Number
37075
Problem Chosen
C
For office use only F1 ________________ F2 ________________ F3 ________________ F4 ________________
2015 Mathematical Contest in Modeling (MCM) Summary Sheet
Organizational Churn: A Roll of the Dice?
Network science is essential in many interdisciplinary studies due to its potential to deal with complex systems. Since the organization of ICM forms a network structure, network science can be utilized to analyze dynamic processes within the company, e.g. the diffusion effects of organizational churn.
Ultimately, we incorporate methods from team science and approaches from multilayer networks in our context to combine Human Capital network with other network layers and discuss how to improve our estimation on organizational churn.
3
3.1 Constructing Human Capital Network . . .
In this paper, we construct a Human Capital network according to the hierarchical structure of ICM and create a simple yet effective model to capture the dynamic processes, which includes organizational churn, promotion and recruitment. For organizational churn, we propose and implement our probabilistic churn model inspired by Bayesian learning principles, which estimates and updates the likelihood of individual churn using the Beta-Binomial distribution. Then we develop three promotion measures based on working experience, inclination to churn, and closeness centrality. Moreover, we propose several means of controlling the recruitment rate from the HR manager's perspective, and further define some key concepts for evaluation, such as dissatisfaction and productivity.
Through extensive simulations, we show that our model is flexible enough to encompass most features of the current situation and yield convincing productivity and cost results. We further extend our model to scenarios with higher churn rates, and discover an interesting fact that higher churn rates lead to lower productivity-cost ratios. In an extreme case with no recruitment, we discover differentiated HR health degeneration among different offices over two years through introduces extra computational costs.
Organizational Churn: A Roll of the Dice?
Contents
1 Introduction
2
2 Fundamental Assumptions
2
3 Preliminaries
In summary, our model is powerful and reliable for various types of human capital dynamic processes. Nevertheless, there are some existing problems such as simulation