美赛一等奖论文-中文翻译版
中英文对照论文-2005美赛B题The Booth Tolls for Thee_收费站模型
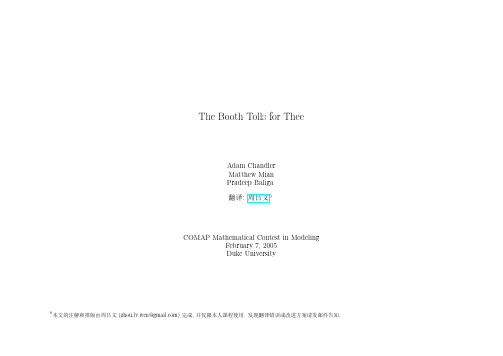
In fact, the models mostly agree that given L lanes, a number of booths around B = ⌊1.65L + 0.9⌋, where ⌊x⌋ is the greatest integer less than x, will minimize the total human cost associated with the plaza.
The Booth Tolls for Thee
Adam Chandler Matthew Mian Pradeep Baliga 翻译: 周吕文0
COMAP Mathematical Contest in Modeling February 7, 2005 Duke University
美赛数学建模A题翻译版论文
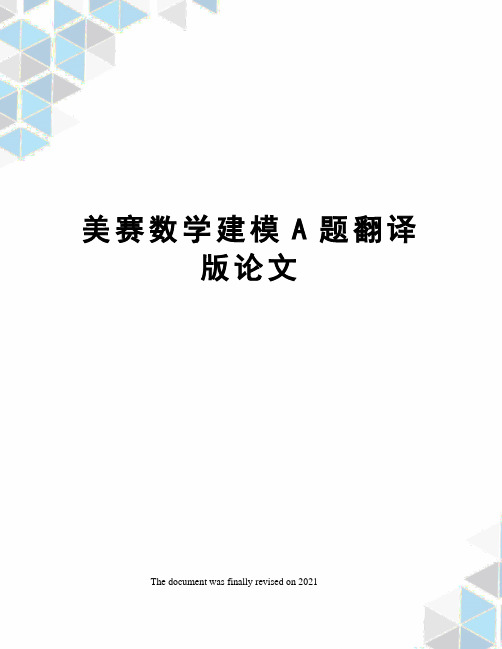
美赛数学建模A题翻译版论文The document was finally revised on 2021数学建模竞赛(MCM / ICM)汇总表基于细胞的高速公路交通模型自动机和蒙特卡罗方法总结基于元胞自动机和蒙特卡罗方法,我们建立一个模型来讨论“靠右行”规则的影响。
首先,我们打破汽车的运动过程和建立相应的子模型car-generation的流入模型,对于匀速行驶车辆,我们建立一个跟随模型,和超车模型。
然后我们设计规则来模拟车辆的运动模型。
我们进一步讨论我们的模型规则适应靠右的情况和,不受限制的情况, 和交通情况由智能控制系统的情况。
我们也设计一个道路的危险指数评价公式。
我们模拟双车道高速公路上交通(每个方向两个车道,一共四条车道),高速公路双向三车道(总共6车道)。
通过计算机和分析数据。
我们记录的平均速度,超车取代率、道路密度和危险指数和通过与不受规则限制的比较评估靠右行的性能。
我们利用不同的速度限制分析模型的敏感性和看到不同的限速的影响。
左手交通也进行了讨论。
根据我们的分析,我们提出一个新规则结合两个现有的规则(靠右的规则和无限制的规则)的智能系统来实现更好的的性能。
1介绍术语假设2模型设计的元胞自动机流入模型跟随模型超车模型超车概率超车条件危险指数两套规则CA模型靠右行无限制行驶规则3补充分析模型加速和减速概率分布的设计设计来避免碰撞4模型实现与计算机5数据分析和模型验证平均速度快车的平均速度密度超车几率危险指数6在不同速度限制下敏感性评价模型7驾驶在左边8交通智能系统智能系统的新规则模型的适应度智能系统结果9结论10优点和缺点优势弱点引用附录。
1 Introduction今天,大约65%的世界人口生活在右手交通的国家和35%在左手交通的国家交通流量。
[worldstandards。
欧盟,2013] 右手交通的国家,比如美国和中国,法规要求驾驶在靠路的右边行走。
多车道高速公路在这些国家经常使用一个规则,要求司机在最右边开车除非他们超过另一辆车,在这种情况下,他们移动到左边的车道、通过,返回到原来的车道。
2012年美国大学生数学建模竞赛B题特等奖文章翻译
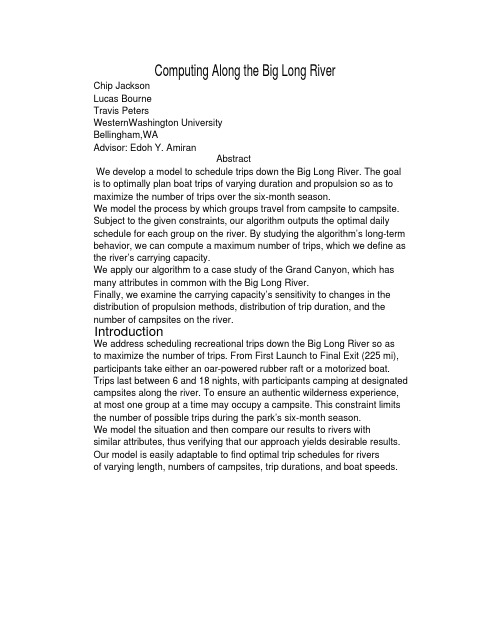
We develop a model to schedule trips down the Big Long River. The goalComputing Along the Big Long RiverChip JacksonLucas BourneTravis PetersWesternWashington UniversityBellingham,WAAdvisor: Edoh Y. AmiranAbstractis to optimally plan boat trips of varying duration and propulsion so as tomaximize the number of trips over the six-month season.We model the process by which groups travel from campsite to campsite.Subject to the given constraints, our algorithm outputs the optimal dailyschedule for each group on the river. By studying the algorithm’s long-termbehavior, we can compute a maximum number of trips, which we define asthe river’s carrying capacity.We apply our algorithm to a case study of the Grand Canyon, which hasmany attributes in common with the Big Long River.Finally, we examine the carrying capacity’s sensitivity to changes in thedistribution of propulsion methods, distribution of trip duration, and thenumber of campsites on the river.IntroductionWe address scheduling recreational trips down the Big Long River so asto maximize the number of trips. From First Launch to Final Exit (225 mi),participants take either an oar-powered rubber raft or a motorized boat.Trips last between 6 and 18 nights, with participants camping at designatedcampsites along the river. To ensure an authentic wilderness experience,at most one group at a time may occupy a campsite. This constraint limitsthe number of possible trips during the park’s six-month season.We model the situation and then compare our results to rivers withsimilar attributes, thus verifying that our approach yields desirable results.Our model is easily adaptable to find optimal trip schedules for riversof varying length, numbers of campsites, trip durations, and boat speeds.No two groups can occupy the same campsite at the same time.Campsites are distributed uniformly along the river.Trips are scheduled during a six-month period of the year.Group trips range from 6 to 18 nights.Motorized boats travel 8 mph on average.Oar-powered rubber rafts travel 4 mph on average.There are only two types of boats: oar-powered rubber rafts and motorizedTrips begin at First Launch and end at Final Exit, 225 miles downstream.*simulates river-trip scheduling as a function of a distribution of trip*can be applied to real-world rivers with similar attributes (i.e., the Grand*is flexible enough to simulate a wide range of feasible inputs; andWhat is the carrying capacity of the riverÿhe maximum number ofHow many new groups can start a river trip on any given day?How should trips of varying length and propulsion be scheduled toDefining the Problemmaximize the number of trips possible over a six-month season?groups that can be sent down the river during its six-month season?Model OverviewWe design a model thatCanyon);lengths (either 6, 12, or 18 days), a varying distribution of propulsionspeeds, and a varying number of campsites.The model predicts the number of trips over a six-month season. It alsoanswers questions about the carrying capacity of the river, advantageousdistributions of propulsion speeds and trip lengths, how many groups canstart a river trip each day, and how to schedule trips.ConstraintsThe problem specifies the following constraints:boats.AssumptionsWe can prescribe the ratio of oar-powered river rafts to motorized boats that go onto the river each day.There can be problems if too many oar-powered boats are launched with short trip lengths.The duration of a trip is either 12 days or 18 days for oar-powered rafts, and either 6 days or 12 days for motorized boats.This simplification still allows our model to produce meaningful results while letting us compare the effect of varying trip lengths.There can only be one group per campsite per night.This agrees with the desires of the river manager.Each day, a group can only move downstream or remain in its current campsiteÿt cannot move back upstream.This restricts the flow of groups to a single direction, greatly simplifying how we can move groups from campsite to campsite.Groups can travel only between 8 a.m. and 6 p.m., a maximum of 9hours of travel per day (one hour is subtracted for breaks/lunch/etc.).This implies that per day, oar-powered rafts can travel at most 36 miles, and motorized boats at most 72 miles. This assumption allows us to determine which groups can reasonably reach a given campsite.Groups never travel farther than the distance that they can feasibly travelin a single day: 36 miles per day for oar-powered rafts and 72 miles per day for motorized boats.We ignore variables that could influence maximum daily travel distance, such as weather and river conditions.There is no way of accurately including these in the model.Campsites are distributed uniformly so that the distance between campsites is the length of the river divided by the number of campsites.We can thus represent the river as an array of equally-spaced campsites.A group must reach the end of the river on the final day of its trip:A group will not leave the river early even if able to.A group will not have a finish date past the desired trip length.This assumption fits what we believe is an important standard for theriver manager and for the quality of the trips.MethodsWe define some terms and phrases:Open campsite: Acampsite is open if there is no groupcurrently occupying it: Campsite cn is open if no group gi is assigned to cn.Moving to an open campsite: For a group gi, its campsite cn, moving to some other open campsite cm ÿ= cn is equivalent to assigning gi to the new campsite. Since a group can move only downstream, or remain at their current campsite, we must have m ÿ n.Waitlist: The waitlist for a given day is composed of the groups that are not yet on the river but will start their trip on the day when their ranking onthe waitlist and their ability to reach a campsite c includes them in theset Gc of groups that can reach campsite c, and the groups are deemed “the highest priority.” Waitlisted groups are initialized with a current campsite value of c0 (the zeroth campsite), and are assumed to have priority P = 1 until they are moved from the waitlist onto the river.Off the River: We consider the first space off of the river to be the “final campsite” cfinal, and it is always an open campsite (so that any number of groups can be assigned to it. This is consistent with the understanding that any number of groups can move off of the river in a single day.The Farthest Empty CampsiteOurscheduling algorithm uses an array as the data structure to represent the river, with each element of the array being a campsite. The algorithm begins each day by finding the open campsite c that is farthest down the river, then generates a set Gc of all groups that could potentially reach c that night. Thus,Gc = {gi | li +mi . c},where li is the groupÿs current location and mi is the maximum distance that the group can travel in one day.. The requirement that mi + li . c specifies that group gi must be able to reach campsite c in one day.. Gc can consist of groups on the river and groups on the waitlist.. If Gc = ., then we move to the next farthest empty campsite.located upstream, closer to the start of the river. The algorithm always runs from the end of the river up towards the start of the river.. IfGc ÿ= ., then the algorithm attempts tomovethe groupwith the highest priority to campsite c.The scheduling algorithm continues in this fashion until the farthestempty campsite is the zeroth campsite c0. At this point, every group that was able to move on the river that day has been moved to a campsite, and we start the algorithm again to simulate the next day.PriorityOnce a set Gc has been formed for a specific campsite c, the algorithm must decide which group to move to that campsite. The priority Pi is a measure of how far ahead or behind schedule group gi is:. Pi > 1: group gi is behind schedule;. Pi < 1: group gi is ahead of schedule;. Pi = 1: group gi is precisely on schedule.We attempt to move the group with the highest priority into c.Some examples of situations that arise, and how priority is used to resolve them, are outlined in Figures 1 and 2.Priorities and Other ConsiderationsOur algorithm always tries to move the group that is the most behind schedule, to try to ensure that each group is camped on the river for aFigure 1. The scheduling algorithm has found that the farthest open campsite is Campsite 6 and Groups A, B, and C can feasibly reach it. Group B has the highest priority, so we move Group B to Campsite 6.Figure 2. As the scheduling algorithm progresses past Campsite 6, it finds that the next farthest open campsite is Campsite 5. The algorithm has calculated that Groups A and C can feasibly reach it; since PA > PC, Group A is moved to Campsite 5.number of nights equal to its predetermined trip length. However, in someinstances it may not be ideal to move the group with highest priority tothe farthest feasible open campsite. Such is the case if the group with thehighest priority is ahead of schedule (P <1).We provide the following rules for handling group priorities:?If gi is behind schedule, i.e. Pi > 1, then move gi to c, its farthest reachableopen campsite.?If gi is ahead of schedule, i.e. Pi < 1, then calculate diai, the number ofnights that the group has already been on the river times the averagedistance per day that the group should travel to be on schedule. If theresult is greater than or equal (in miles) to the location of campsite c, thenmove gi to c. Doing so amounts to moving gi only in such a way that itis no longer ahead of schedule.?Regardless of Pi, if the chosen c = cfinal, then do not move gi unless ti =di. This feature ensures that giÿ trip will not end before its designatedend date.Theonecasewhere a groupÿ priority is disregardedisshownin Figure 3.Scheduling SimulationWe now demonstrate how our model could be used to schedule rivertrips.In the following example, we assume 50 campsites along the 225-mileriver, and we introduce 4 groups to the river each day. We project the tripFigure 3. The farthest open campsite is the campsite off the river. The algorithm finds that GroupD could move there, but GroupD has tD > dD.that is, GroupD is supposed to be on the river for12 nights but so far has spent only 11.so Group D remains on the river, at some campsite between 171 and 224 inclusive.schedules of the four specific groups that we introduce to the river on day25. We choose a midseason day to demonstrate our modelÿs stability overtime. The characteristics of the four groups are:. g1: motorized, t1 = 6;. g2: oar-powered, t2 = 18;. g3: motorized, t3 = 12;. g4: oar-powered, t4 = 12.Figure 5 shows each groupÿs campsite number and priority value foreach night spent on the river. For instance, the column labeled g2 givescampsite numbers for each of the nights of g2ÿs trip. We find that each giis off the river after spending exactly ti nights camping, and that P ÿ 1as di ÿ ti, showing that as time passes our algorithm attempts to get (andkeep) groups on schedule. Figures 6 and 7 display our results graphically.These findings are consistent with the intention of our method; we see inthis small-scale simulation that our algorithm produces desirable results.Case StudyThe Grand CanyonThe Grand Canyon is an ideal case study for our model, since it sharesmany characteristics with the Big Long River. The Canyonÿs primary riverrafting stretch is 226 miles, it has 235 campsites, and it is open approximatelysix months of the year. It allows tourists to travel by motorized boat or byoar-powered river raft for a maximum of 12 or 18 days, respectively [Jalbertet al. 2006].Using the parameters of the Grand Canyon, we test our model by runninga number of simulations. We alter the number of groups placed on thewater each day, attempting to find the carrying capacity for the river.theFigure 7. Priority values of groups over the course of each trip. Values converge to P = 1 due to the algorithm’s attempt to keep groups on schedule.maximumnumber of possible trips over a six-month season. The main constraintis that each trip must last the group’s planned trip duration. Duringits summer season, the Grand Canyon typically places six new groups onthe water each day [Jalbert et al. 2006], so we use this value for our first simulation.In each simulation, we use an equal number of motorized boatsand oar-powered rafts, along with an equal distribution of trip lengths.Our model predicts the number of groups that make it off the river(completed trips), how many trips arrive past their desired end date (latetrips), and the number of groups that did not make it off the waitlist (totalleft on waitlist). These values change as we vary the number of new groupsplaced on the water each day (groups/day).Table 1 indicates that a maximum of 18 groups can be sent down theriver each day. Over the course of the six-month season, this amounts to nearly 3,000 trips. Increasing groups/day above 18 is likely to cause latetrips (some groups are still on the river when our simulation ends) and long waitlists. In Simulation 1, we send 1,080 groups down river (6 groups/day?80 days) but only 996 groups make it off; the other groups began near the end of the six-month period and did not reach the end of their trip beforethe end of the season. These groups have negligible impact on our results and we ignore them.Sensitivity Analysis of Carrying CapacityManagers of the Big Long River are faced with a similar task to that of the managers of the Grand Canyon. Therefore, by finding an optimal solutionfor the Grand Canyon, we may also have found an optimal solution forthe Big Long River. However, this optimal solution is based on two key assumptions:?Each day, we put approximately the same number of groups onto theriver; and?the river has about one campsite per mile.We can make these assumptions for the Grand Canyon because they are true for the Grand Canyon, but we do not know if they are true for the Big Long River.To deal with these unknowns,wecreate Table 3. Its values are generatedby fixing the number Y of campsites on the river and the ratio R of oarpowered rafts to motorized boats launched each day, and then increasingthe number of trips added to the river each day until the river reaches peak carrying capacity.The peak carrying capacities in Table 3 can be visualized as points ina three-dimensional space, and we can find a best-fit surface that passes (nearly) through the data points. This best-fit surface allows us to estimatethe peak carrying capacity M of the river for interpolated values. Essentially, it givesM as a function of Y and R and shows how sensitiveM is tochanges in Y and/or R. Figure 7 is a contour diagram of this surface.The ridge along the vertical line R = 1 : 1 predicts that for any givenvalue of Y between 100 and 300, the river will have an optimal value ofM when R = 1 : 1. Unfortunately, the formula for this best-fit surface is rather complex, and it doesn’t do an accurate job of extrapolating beyond the data of Table 3; so it is not a particularly useful tool for the peak carrying capacity for other values ofR. The best method to predict the peak carrying capacity is just to use our scheduling algorithm.Sensitivity Analysis of Carrying Capacity re R and DWe have treatedM as a function ofR and Y , but it is still unknown to us how M is affected by the mix of trip durations of groups on the river (D).For example, if we scheduled trips of either 6 or 12 days, how would this affect M? The river managers want to know what mix of trips of varying duration and speed will utilize the river in the best way possible.We use our scheduling algorithm to attempt to answer this question.We fix the number of campsites at 200 and determine the peak carrying capacity for values of R andD. The results of this simulation are displayed in Table 4.Table 4 is intended to address the question of what mix of trip durations and speeds will yield a maximum carrying capacity. For example: If the river managers are currently scheduling trips of length?6, 12, or 18: Capacity could be increased either by increasing R to be closer to 1:1 or by decreasing D to be closer to ? or 12.?12 or 18: Decrease D to be closer to ? or 12.?6 or 12: Increase R to be closer to 4:1.ConclusionThe river managers have asked how many more trips can be added tothe Big Long Riverÿ season. Without knowing the specifics ofhowthe river is currently being managed, we cannot give an exact answer. However, by applying our modelto a study of the GrandCanyon,wefound results which could be extrapolated to the context of the Big Long River. Specifically, the managers of the Big Long River could add approximately (3,000 - X) groups to the rafting season, where X is the current number of trips and 3,000 is the capacity predicted by our scheduling algorithm. Additionally, we modeled how certain variables are related to each other; M, D, R, and Y . River managers could refer to our figures and tables to see how they could change their current values of D, R, and Y to achieve a greater carrying capacity for the Big Long River.We also addressed scheduling campsite placement for groups moving down the Big Long River through an algorithm which uses priority values to move groups downstream in an orderly manner.Limitations and Error AnalysisCarrying Capacity OverestimationOur model has several limitations. It assumes that the capacity of theriver is constrained only by the number of campsites, the trip durations,and the transportation methods. We maximize the river’s carrying capacity, even if this means that nearly every campsite is occupied each night.This may not be ideal, potentially leading to congestion or environmental degradation of the river. Because of this, our model may overestimate the maximum number of trips possible over long periods of time. Environmental ConcernsOur case study of the Grand Canyon is evidence that our model omits variables. We are confident that the Grand Canyon could provide enough campsites for 3,000 trips over a six-month period, as predicted by our algorithm. However, since the actual figure is around 1,000 trips [Jalbert et al.2006], the error is likely due to factors outside of campsite capacity, perhaps environmental concerns.Neglect of River SpeedAnother variable that our model ignores is the speed of the river. Riverspeed increases with the depth and slope of the river channel, makingour assumption of constant maximum daily travel distance impossible [Wikipedia 2012]. When a river experiences high flow, river speeds can double, and entire campsites can end up under water [National Park Service 2008]. Again, the results of our model don’t reflect these issues. ReferencesC.U. Boulder Dept. of Applied Mathematics. n.d. Fitting a surface to scatteredx-y-z data points. /computing/Mathematica/Fit/ .Jalbert, Linda, Lenore Grover-Bullington, and Lori Crystal, et al. 2006. Colorado River management plan. 2006./grca/parkmgmt/upload/CRMPIF_s.pdf .National Park Service. 2008. Grand Canyon National Park. High flowriver permit information. /grca/naturescience/high_flow2008-permit.htm .Sullivan, Steve. 2011. Grand Canyon River Statistics Calendar Year 2010./grca/planyourvisit/upload/Calendar_Year_2010_River_Statistics.pdf .Wikipedia. 2012. River. /wiki/River .Memo to Managers of the Big Long RiverIn response to your questions regarding trip scheduling and river capacity,we are writing to inform you of our findings.Our primary accomplishment is the development of a scheduling algorithm.If implemented at Big Long River, it could advise park rangerson how to optimally schedule trips of varying length and propulsion. Theoptimal schedule will maximize the number of trips possible over the sixmonth season.Our algorithm is flexible, taking a variety of different inputs. Theseinclude the number and availability of campsites, and parameters associatedwith each tour group. Given the necessary inputs, we can output adaily schedule. In essence, our algorithm does this by using the state of theriver from the previous day. Schedules consist of campsite assignments foreach group on the river, as well those waiting to begin their trip. Given knowledge of future waitlists, our algorithm can output schedules monthsin advance, allowing managementto schedule the precise campsite locationof any group on any future date.Sparing you the mathematical details, allow us to say simply that ouralgorithm uses a priority system. It prioritizes groups who are behindschedule by allowing them to move to further campsites, and holds backgroups who are ahead of schedule. In this way, it ensures that all trips willbe completed in precisely the length of time the passenger had planned for.But scheduling is only part of what our algorithm can do. It can alsocompute a maximum number of possible trips over the six-month season.We call this the carrying capacity of the river. If we find we are below ourcarrying capacity, our algorithm can tell us how many more groups wecould be adding to the water each day. Conversely, if we are experiencingriver congestion, we can determine how many fewer groups we should beadding each day to get things running smoothly again.An interesting finding of our algorithm is how the ratio of oar-poweredriver rafts to motorized boats affects the number of trips we can send downstream. When dealing with an even distribution of trip durations (from 6 to18 days), we recommend a 1:1 ratio to maximize the river’s carrying capacity.If the distribution is skewed towards shorter trip durations, then ourmodel predicts that increasing towards a 4:1 ratio will cause the carryingcapacity to increase. If the distribution is skewed the opposite way, towards longer trip durations, then the carrying capacity of the river will always beless than in the previous two cases—so this is not recommended.Our algorithm has been thoroughly tested, and we believe that it isa powerful tool for determining the river’s carrying capacity, optimizing daily schedules, and ensuring that people will be able to complete their trip as planned while enjoying a true wilderness experience.Sincerely yours,Team 13955。
2014年美赛数学建模A题翻译版论文

2014年美赛数学建模A题翻译版论文D流入模型,或vehicle-generation模型,模拟了随机到达高速公路的入口处的车辆。
对于每一个车道,前六个细胞在元胞自动机中设置为vehicle-generation区域。
我们假设每辆车的到达服从二项概率分布。
让ts表示采样时间间隔和N表示在ts时间内车辆的总数。
然后N可以近似服从泊松概率分布。
让Pt(N)表示N的可能性,于是我们有ts表示在一秒,我们可以分配N的期望的值的范围从0到3.6。
N作为在每一秒中到达的总车辆,N的期望能有效地反映交通状况。
λ越小,交通越轻松。
因此我们能够模拟不同流量条件下,交通的轻或重,通过分配相应的值λ。
λ的值设定后,我们得到了进入高速公路的车辆模拟每一秒的随机号码。
每个车道然后随机分配进入。
我们的车辆模型支持两种不同的速度范围, 假设所有车辆的初始速度设置为20 m / s。
这种做法带来了简化而不削弱结果。
这是因为由于交通密度控制和加速度的分布概率的引入,所有车辆的速度往往是一个值。
当交通密度低,车辆可以自由加速到最大速度,而不用担心冲突,因此收敛速度在允许的最高速度而不用担心撞车。
当交通密度高,所有的通道将充满车辆,交通流的速度是由车道上速度最慢的车决定,因此收敛速度是在较低的速度限制。
经过初步分析,收敛速度模型稍后将合理的实现。
利用泊松概率分布使流入模型接近现实和实用。
由于收敛趋势,一样的初速度在不改变的情况下就能得到简化。
2.3 Vehicle-Following Model美国联邦公路管理局的部门定义司机的反应时间PIEV时间。
PIEV时间由四部分组成:•感知过程:司机在驾驶环境中感知的变化。
•理解过程:司机分析关于变化的信息。
•评估过程:司机决定根据他的驾驶行为分析。
•意志过程:司机执行驾驶行为我们应用PIEV在匀速行驶模型和超车模型。
在每次循环中,我们首先获得每辆车的速度和位置,计算差距,然后确定驾驶行为(无论继续或改变车道超车后)。
美赛金奖论文
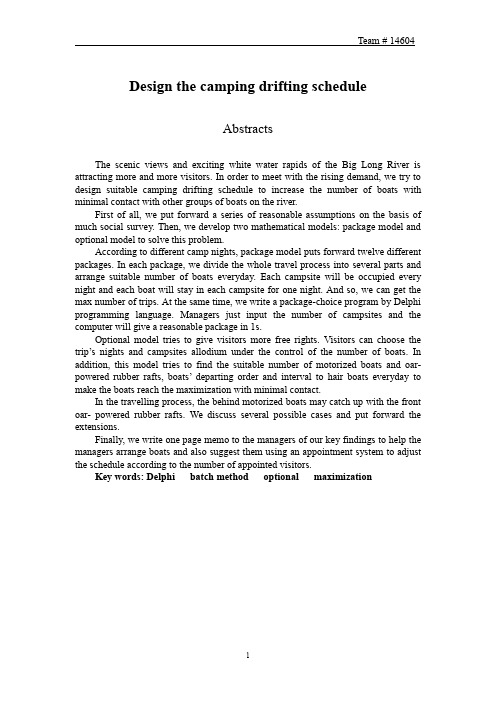
1
Team # 14604
Catalogue
Abstracts ........................................................................................................................................... 1 Contents ............................................................................................................................................ 3 1. Introduction ................................................................................................................................... 3 1.1 Restatement of the Problem ................................................................................................ 3 1.2 Survey of the Previous Research......................................................................................... 3 2. Assumptions .................................................................................................................................. 4 3. Parameters ..................................................................................................................................... 4 4. Model A ----------Package model .................................................................................................. 6 4.1 Motivation ........................................................................................................................... 6 4.2 Development ....................................................................................................................... 6 4.2.1 Module 1: Introduce of model A .............................................................................. 6 4.2.2 Module 2: Solution of model A .............................................................................. 10 4.3 Conclusion ........................................................................................................................ 11 5. Model B----------Optional model ................................................................................................ 12 5.1 Motivation ......................................................................................................................... 12 5.2 Development ..................................................................................................................... 12 5.2.1 Module B: Choose oar- powered rubber rafts or motorized boats either ............... 12 5.2.2 Module 2: Choose mix of oar- powered rubber rafts and motorized boats ............ 14 5.3 Initial arrangement ............................................................................................................ 17 5.4. Deepened model B ........................................................................................................... 18 5.4.1 Choose the campsites allodium .............................................................................. 18 5.4.2 Choose the oar- powered rubber rafts or motorized boats allodium ...................... 19 5.5 An example of reasonable arrangement ............................................................................ 19 5.6 The strengths and weakness .............................................................................................. 20 6. Extensions ................................................................................................................................... 21 7. Memo .......................................................................................................................................... 25 8. References ................................................................................................................................... 26 9. Appendices .................................................................................................................................. 27 9.1 Appendix I .................................................................................................. 27 9.2 Appendix II ....................................................................................................................... 29
2005年美国大学生数学建模竞赛优秀论文翻译
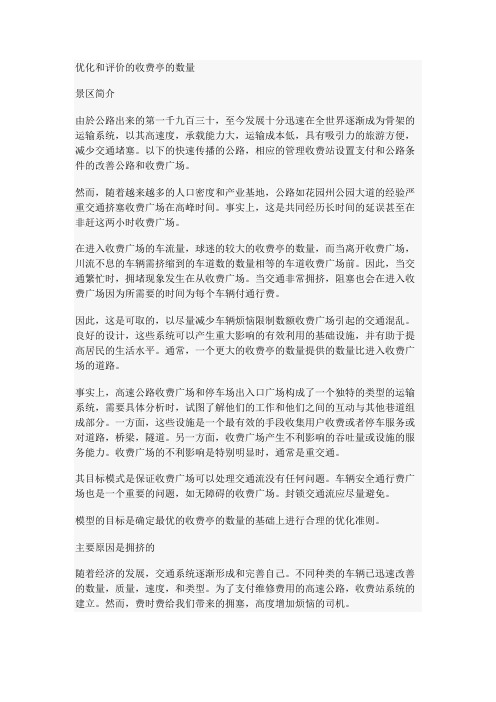
优化和评价的收费亭的数量景区简介由於公路出来的第一千九百三十,至今发展十分迅速在全世界逐渐成为骨架的运输系统,以其高速度,承载能力大,运输成本低,具有吸引力的旅游方便,减少交通堵塞。
以下的快速传播的公路,相应的管理收费站设置支付和公路条件的改善公路和收费广场。
然而,随着越来越多的人口密度和产业基地,公路如花园州公园大道的经验严重交通挤塞收费广场在高峰时间。
事实上,这是共同经历长时间的延误甚至在非赶这两小时收费广场。
在进入收费广场的车流量,球迷的较大的收费亭的数量,而当离开收费广场,川流不息的车辆需挤缩到的车道数的数量相等的车道收费广场前。
因此,当交通繁忙时,拥堵现象发生在从收费广场。
当交通非常拥挤,阻塞也会在进入收费广场因为所需要的时间为每个车辆付通行费。
因此,这是可取的,以尽量减少车辆烦恼限制数额收费广场引起的交通混乱。
良好的设计,这些系统可以产生重大影响的有效利用的基础设施,并有助于提高居民的生活水平。
通常,一个更大的收费亭的数量提供的数量比进入收费广场的道路。
事实上,高速公路收费广场和停车场出入口广场构成了一个独特的类型的运输系统,需要具体分析时,试图了解他们的工作和他们之间的互动与其他巷道组成部分。
一方面,这些设施是一个最有效的手段收集用户收费或者停车服务或对道路,桥梁,隧道。
另一方面,收费广场产生不利影响的吞吐量或设施的服务能力。
收费广场的不利影响是特别明显时,通常是重交通。
其目标模式是保证收费广场可以处理交通流没有任何问题。
车辆安全通行费广场也是一个重要的问题,如无障碍的收费广场。
封锁交通流应尽量避免。
模型的目标是确定最优的收费亭的数量的基础上进行合理的优化准则。
主要原因是拥挤的随着经济的发展,交通系统逐渐形成和完善自己。
不同种类的车辆已迅速改善的数量,质量,速度,和类型。
为了支付维修费用的高速公路,收费站系统的建立。
然而,费时费给我们带来的拥塞,高度增加烦恼的司机。
一般来说,在收费亭的数量大于数量的车道。
05年美国大学生数学建模竞赛A题特等奖论文翻译
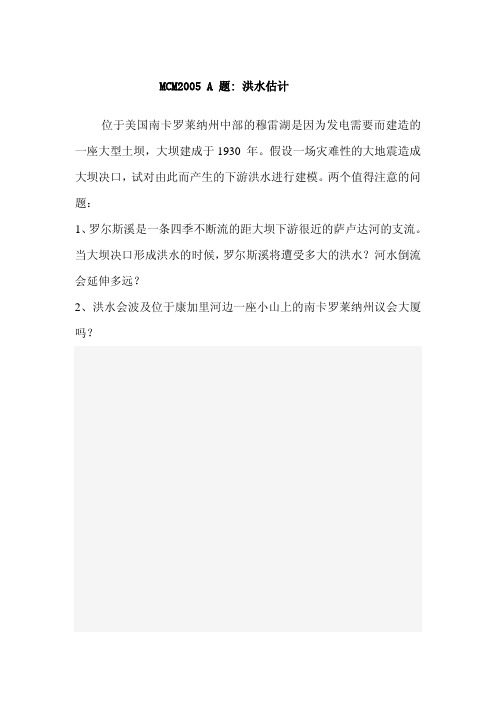
在每一时刻流出水的体积等于裂口的面积乘以水的速率乘以时间:
h h Vwater leaeing = wbreach (
−
lake
s)
dam
water
leaving ttime
step
其中:V 是体积, w 是宽度, h 是高度, s 是速度, t 是时间。
我们假设该湖是一个大的直边贮槽,所以当水的高度确定时,其面积不改变。 这意味着,湖的高度等于体积除以面积
在南卡罗莱那州的中央,一个湖被一个 75 年的土坝抑制。如果大坝被地震破坏 将会发生什么事?这个担心是基于1886年发生在查尔斯顿的一场地震,科学家们 相信它里氏7.3级[联邦能源管理委员会2002]。断层线的位置几乎直接在穆雷湖 底(SCIway 2000;1997,1998年南CarolinaGeological调查)和在这个地区小地震 的频率迫使当局考虑这样一个灾难的后果。
)
−
1 2
ห้องสมุดไป่ตู้
λ
2
(u
n j +1
−
2u j
+
n
+
u
n j
)
这里的上层指数表示时间和较低的空间, λ 是时间与空间步长的大小的比值。
(我们的模型转换以距离和时间做模型的单位,因此每个步长为 1)。第二个条 件的作用是受潮尖峰,因为它看起来不同并补偿于在每个点的任一边上的点。
我们发现该模型对粗糙度参数 n 高度敏感(注意,这是只在通道中的有效粗 糙度)。当 n 很大(即使在大河流的标准值 0.03)。对水流 和洪水堆积的倾向有 较高的抗拒,这将导致过量的陡水深资料,而且往往使模型崩溃。幸运的是,我
我们的任务是预测沿着Saluda河从湖穆雷大坝到哥伦比亚的水位变化,如果 发生了1886年相同规模的地震破坏了大坝。特别是支流罗尔斯溪会回流多远和哥 伦比亚南卡罗来纳州的州议会大厦附近的水位会多高。
美赛B题O奖论文中英对照版
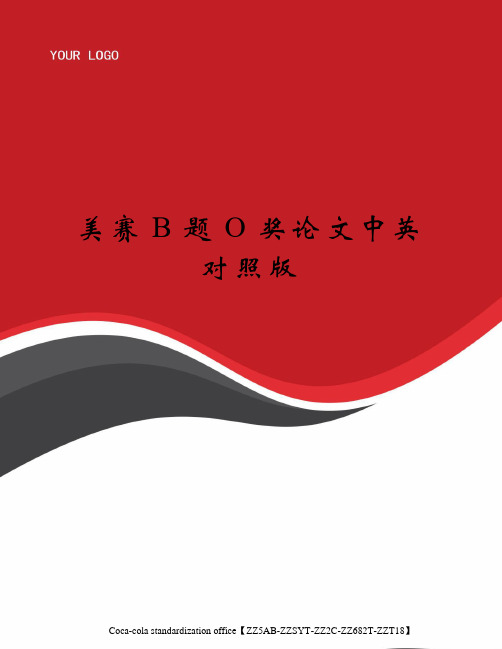
美赛B题O奖论文中英对照版Coca-cola standardization office【ZZ5AB-ZZSYT-ZZ2C-ZZ682T-ZZT18】2017MCM/ICMSummary Sheet(Your team's summary should be included as the first page of your electronic submission.)Type a summary of your results on this page. Do not include the name of your school, advisor, or teammembers on this page.A New Type of Toll Plaza Based on Bionics-HoneycombSummaryIn this paper, we analyze the performance of commonly used toll plaza basedon our proposed mathematical model. A new improved toll plaza is proposed toreduce the cost, decrease the probability of collision at the merging point andincrease the throughput.The distribution of our proposed tollbooths resembles the honeycomb. At thecenter of each regular hexagonal honeycomb, there are two tollbooths, whichserve two separated vehicle streams. The vehicles in these two separated streamsare merged in advance before they continue their journey on the highway. Due tothe specific pattern of the new toll plaza, the total area can be reducedsignificantly. Meanwhile, the average wasted time caused by queuing can bediminished, which means that the throughput will be raised. Additionally, bysplitting the merging procedure into two stages, the possibility of accidentscan also be decreased.The main contributions of this paper are as follows:(1) The new designed cellular architecture can greatly reduce theconstruction area compared with traditional linear distributed toll gates.(2) We analyze the throughput of toll plazas by means of the queuingtheory. To verify our theory, we simulate the behavior of the large number ofvehicles passing the toll plaza with the help of PTV- VISSIM. Simulation resultsshow that the ideal cellular distributed toll booths have better resultscompared with traditional toll stations, especially when the traffic flow isheavy, the average travel time reduced by about 55% and the average delay timeof each lane is reduced by about 70%.(3) W e analyze the influence of the proportions of different types oftollbooths to our design. According to relevant documents, the impact of exact-change tollbooths is similar to manual tollbooths, so we only consider two kindsof tollbooths: human-staffed tollbooths and E-ZPass tollbooths. PTV-VISSIMsimulation results show that full ETC tollbooth is 8 times faster than full MTCtollbooth.(4) We simulate the performance of the cellular toll plaza under differenttraffic throughput. Simulation results show that the average transit timeremains at about 11 seconds under different throughputs from 0 to 2000 (Unit:veh / h ). We can infer that this model is not sensitive to traffic flowvariations and has strong robustness which is suitable for practicalFor office use only F1 F2 F3 F4Team Control Number 56731 Problem Chosen B For office use onlyT1 T2 T3 T4construction.(5)To further reduce the possibility of accident, we improve the cellulartollbooth concept model: make the transition zone more smooth and arrangedifferent kinds of tollbooths more equitable.(6)For self-driving vehicles, in the center of the toll plaza, we reservespecial E-ZPass tollbooths, which match the characteristics of autonomousvehicles: safer and faster.Electronic toll collection and autonomous vehicles are the trends of modern transportation, our new designed model can improve the performance of toll plazain the aspects of cost, throughput and accident prevention.一种基于仿生蜂窝的新型收费广场概要在本文中,我们基于我们提出的数学模型分析了常用收费广场的表现。
2011年美国数学建模大赛一等奖获奖论文

Team #10076
Page 2 of 21
Contents
1 Introduction 2 Restatement of Problem 3 Basic Assumptions 4 Analysis of the Problem 4.1 Definitions of Zero-Potential Surface and “vertical 4.2 Turn Of Snowboarding . . . . . . . . . . . . . . . 4.3 Snowboarding on the Deck . . . . . . . . . . . . . 4.4 Snowboarding on the Quarter Arc . . . . . . . . . 5 “Vertical air” Model 6 Results and Sensitivity Analysis 6.1 Results . . . . . . . . . . . . . . . . . . . . . . . . . . . . . . . . . . . . . 6.2 Sensitivity Analysis . . . . . . . . . . . . . . . . . . . . . . . . . . . . . . 7 Model Applications And Analysis 7.1 Applications . . . . . . . . . . . . . . . . . . . . . . . . . . . . . . . . . . 7.2 Analysis . . . . . . . . . . . . . . . . . . . . . . . . . . . . . . . . . . . . 8 Maximum Twist Model 8.1 Analysis of Problem . . . . . . . . . . . . . . . . . . . . . . . . . . . . . 8.2 Modeling . . . . . . . . . . . . . . . . . . . . . . . . . . . . . . . . . . . . 8.3 Results and Sensitivity Analysis . . . . . . . . . . . . . . . . . . . . . . . 9 Tradeoffs to Develop a ”Practical” Course 9.1 Halfpipe Framework . . . . . . . . . . . . . . . . . . . . . . . . . . . . . 9.2 Snow in the Halfpipe . . . . . . . . . . . . . . . . . . . . . . . . . . . . . 3 3 4 6 6 7 10 10 14 16 16 16 17 17 17 18 18 18 19 20 20 20
美赛优秀论文2010MCM的B特等奖翻译

摘要:该模型最大的挑战是如何建立连环杀手犯罪行为的模型。
因为找出受害者之间的联系是非常困难的;因此,我们预测罪犯的下一个目标地点,而不是具体目标是谁。
这种预测一个罪犯的犯罪的空间格局叫做犯罪空间情报分析。
研究表明:最暴力的连环杀手的犯罪范围一般在一个径向带中央点附近:例如家庭,办公室,以及其他一些犯罪行为高发区(例如城镇妓女集中区)。
这些‘锚点’为我们的模型提供了基础。
我们假设整个分析域是一个潜在的犯罪现场。
罪犯的活动不受到任何条件约束;并且该区域足够大包括所有的打击点。
我们考虑的是一个可度量的空间,为预测算法创建了空间可能性。
此外;我们假设罪犯为一个暴力的系列犯罪者,因为研究表明窃贼和纵火犯不可能遵循某一空间模式。
一个锚点与多个锚点有着实质性的不同,首先讨论单个锚点的案例,建立坐标系并把罪犯最后犯罪地点与犯罪序列表示出来,并估计以前案件发生地地点,评估模型的可靠性,并且我们得到未来可能发生犯罪行为的锚点。
对于多个锚点的案例,我们通过聚类与排序的方法:将所给数据划分为几组。
在每组中找一个最重要的锚点,每一个分区都给定一个权值。
我们进行单点测试,利用以前的锚点预测最近的一个锚点,并且与其实际位置相比较。
我们从文献中摘录七个数据集,并且用其中四个改善我们的模型,检测其序列变化,地理集中位置和总锚点的数目。
然后通过其他三点来评估我们的模型。
结果显示多个锚点的模型的结果比较优。
引言:通过研究文献中以得出的连环案件罪犯地理空间往往是围绕罪犯日常活动的几个锚点附近的区域。
我们建立的预测模型就是在其规律下建立的,并且预测出一个表面的可能值和度量值。
第一个方案是通过重心法寻找出单个可能的锚点。
第二个方案是假设2到4个锚点,并且利用聚类算法的排序与分组的方法。
两种方案都是利用统计方法来缩小预测未来犯罪的地点区域背景:1981年peter sutcliffe的逮捕是法医生物学家stuart kind 通过利用数理原理成功预测出约克郡开膛手的住处的一个标志目前,信息密集型模型是通过热图技术建立确定特殊犯罪类型的热点或者是找出犯罪活动与某一地区之间的联系比率。
2017年美赛论文C题M奖中文国际一等奖
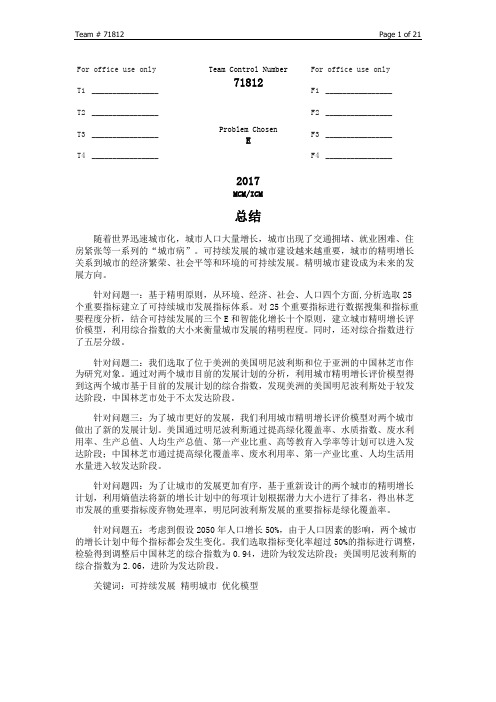
For office use only T1________________ T2________________ T3________________ T4________________ Team Control Number71812Problem ChosenEFor office use onlyF1________________F2________________F3________________F4________________ 2017MCM/ICM总结随着世界迅速城市化,城市人口大量增长,城市出现了交通拥堵、就业困难、住房紧张等一系列的“城市病”。
可持续发展的城市建设越来越重要,城市的精明增长关系到城市的经济繁荣、社会平等和环境的可持续发展。
精明城市建设成为未来的发展方向。
针对问题一:基于精明原则,从环境、经济、社会、人口四个方面,分析选取25个重要指标建立了可持续城市发展指标体系。
对25个重要指标进行数据搜集和指标重要程度分析,结合可持续发展的三个E和智能化增长十个原则,建立城市精明增长评价模型,利用综合指数的大小来衡量城市发展的精明程度。
同时,还对综合指数进行了五层分级。
针对问题二:我们选取了位于美洲的美国明尼波利斯和位于亚洲的中国林芝市作为研究对象。
通过对两个城市目前的发展计划的分析,利用城市精明增长评价模型得到这两个城市基于目前的发展计划的综合指数,发现美洲的美国明尼波利斯处于较发达阶段,中国林芝市处于不太发达阶段。
针对问题三:为了城市更好的发展,我们利用城市精明增长评价模型对两个城市做出了新的发展计划。
美国通过明尼波利斯通过提高绿化覆盖率、水质指数、废水利用率、生产总值、人均生产总值、第一产业比重、高等教育入学率等计划可以进入发达阶段;中国林芝市通过提高绿化覆盖率、废水利用率、第一产业比重、人均生活用水量进入较发达阶段。
针对问题四:为了让城市的发展更加有序,基于重新设计的两个城市的精明增长计划,利用熵值法将新的增长计划中的每项计划根据潜力大小进行了排名,得出林芝市发展的重要指标废弃物处理率,明尼阿波利斯发展的重要指标是绿化覆盖率。
美国中学生数学建模竞赛获奖论文
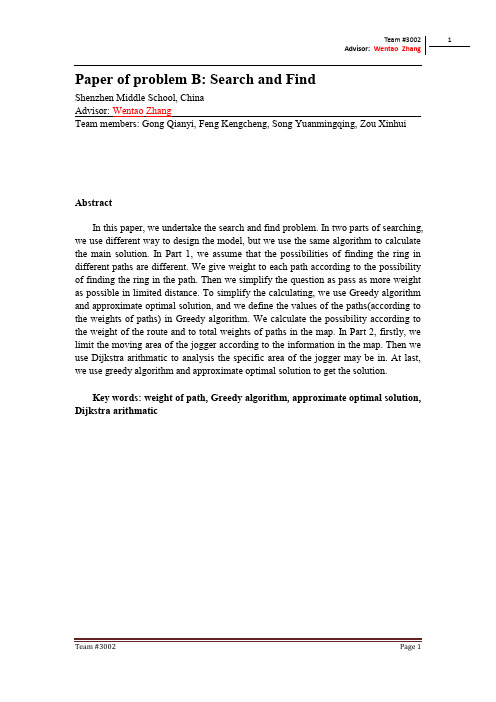
Abstract
In this paper, we undertake the search and find problem. In two parts of searching, we use different way to design the model, but we use the same algorithm to calculate the main solution. In Part 1, we assume that the possibilities of finding the ring in different paths are different. We give weight to each path according to the possibility of finding the ring in the path. Then we simplify the question as pass as more weight as possible in limited distance. To simplify the calculating, we use Greedy algorithm and approximate optimal solution, and we define the values of the paths(according to the weights of paths) in Greedy algorithm. We calculate the possibility according to the weight of the route and to total weights of paths in the map. In Part 2, firstly, we limit the moving area of the jogger according to the information in the map. Then we use Dijkstra arithmatic to analysis the specific area of the jogger may be in. At last, we use greedy algorithm and approximate optimal solution to get the solution.
数学建模 美赛特等奖论文(中文版)分析溃坝:针对南卡罗来纳州大坝坍塌建立模型

分析溃坝:针对南卡罗来纳州大坝坍塌建立模型 摘要萨鲁达大坝建立在卡罗莱纳州的墨累湖与萨鲁达河之间,如果发生地震大坝就会坍塌。
本文通过建立模型来分析以下四种大坝决口时水的流量以及洪水泛滥时水的流量:● 大坝的绝大部分被瞬间侵蚀看成是大坝瞬间彻底坍塌;● 大坝的绝大部分被缓慢侵蚀看成是大坝延期彻底坍塌;● 管涌就是先形成一个小孔,最终形成一个裂口;● 溢出就是大坝被侵蚀后,形成一个梯形的裂口。
本文建立了两个模型来描述下游洪水的泛滥情况。
两个模型都采用离散网格的方法,将一个地区看成是一个网格,每个网格都包含洪水的深度和体积。
复力模型运用了网格的速度、重力以及邻近网格的压力来模拟水流。
下坡模型假定水流速度与邻近网格间水位高度的成正比例。
下坡模型是高效率的、直观的、灵活的,可以适用于已知海拔的任何地区。
它的两个参数稳定并限制了水流,但该模型的预测很少依赖于它们的静态值。
对于萨鲁达溃坝,洪水总面积为25.106km ;它还没有到达国会大厦。
罗威克里克的洪水向上游延伸了km 4.4,覆盖面积达24.26.1km -变量及假设表1说明了用来描述和模拟模型的变量,表2列出了模拟程序中的参数。
表 1模型中的变量.变量 定义溃坝时的水流量速率1TF Q 瞬间彻底坍塌2TF Q 延期彻底坍塌PIPE Q 管涌OT Q 溢出peak Q 最大流速溃坝时水流出到停止所用时间1TF t 瞬间彻底坍塌2TF t 延期彻底坍塌PIPE t 管涌OT t 溢出V ∆ 溃坝后从墨累湖里流出的水的总体积Lm Vol 墨累湖的原来体积LM Area 墨累湖的原来面积breach d 从裂口到坝顶距离breach t 从裂口开始到溃坝形成的时间 近似圆锥的墨累湖的侧面一般假设● 正常水位是在溃坝前的湖水位置。
● 河道中的水流不随季节变化而变动。
● 墨累湖里的水的容积可以看作为一个正圆锥(图1 )。
表2 模拟程序中的参数 参数 所取值 意义BREACH_TYPE 变量 瞬间彻底坍塌,延期彻底坍,管涌,溢出模型中的一种 T ∆ 0.10 时间不长的长度(s)MIN_DEPTH 0001.0 网格空时的水的深度(m) FINAT T 100000 大坝彻底决口所用时间 b T 3600 溃坝达最大值的时间(s) peak Q 25000 溃坝的最大流速(m 3/s) breach d 30 蓄水池的最初深度(m) LM Volume 910714.2⨯ 墨累湖的总体积(m 3) LM Area 610202⨯ 墨累湖的总面积(m 2)k 504.0 扩散因素 (控制两网格间交换的水的数量) MAX_LOSS_FRAC 25.0 单位网格中水的最大流失量图 1. 水库近似一个正圆锥.大坝假设● 萨鲁达大坝在以下四种方式之一坍塌:-瞬间彻底坍塌,-延期彻底坍塌,-管涌,-溢出。
美国大学生数学建模大赛优秀论文一等奖摘要
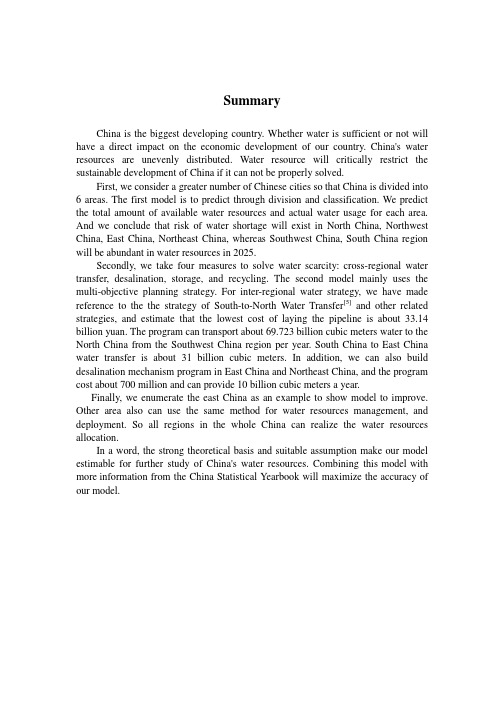
SummaryChina is the biggest developing country. Whether water is sufficient or not will have a direct impact on the economic development of our country. China's water resources are unevenly distributed. Water resource will critically restrict the sustainable development of China if it can not be properly solved.First, we consider a greater number of Chinese cities so that China is divided into 6 areas. The first model is to predict through division and classification. We predict the total amount of available water resources and actual water usage for each area. And we conclude that risk of water shortage will exist in North China, Northwest China, East China, Northeast China, whereas Southwest China, South China region will be abundant in water resources in 2025.Secondly, we take four measures to solve water scarcity: cross-regional water transfer, desalination, storage, and recycling. The second model mainly uses the multi-objective planning strategy. For inter-regional water strategy, we have made reference to the the strategy of South-to-North Water Transfer[5]and other related strategies, and estimate that the lowest cost of laying the pipeline is about 33.14 billion yuan. The program can transport about 69.723 billion cubic meters water to the North China from the Southwest China region per year. South China to East China water transfer is about 31 billion cubic meters. In addition, we can also build desalination mechanism program in East China and Northeast China, and the program cost about 700 million and can provide 10 billion cubic meters a year.Finally, we enumerate the east China as an example to show model to improve. Other area also can use the same method for water resources management, and deployment. So all regions in the whole China can realize the water resources allocation.In a word, the strong theoretical basis and suitable assumption make our model estimable for further study of China's water resources. Combining this model with more information from the China Statistical Yearbook will maximize the accuracy of our model.。
美赛论文翻译
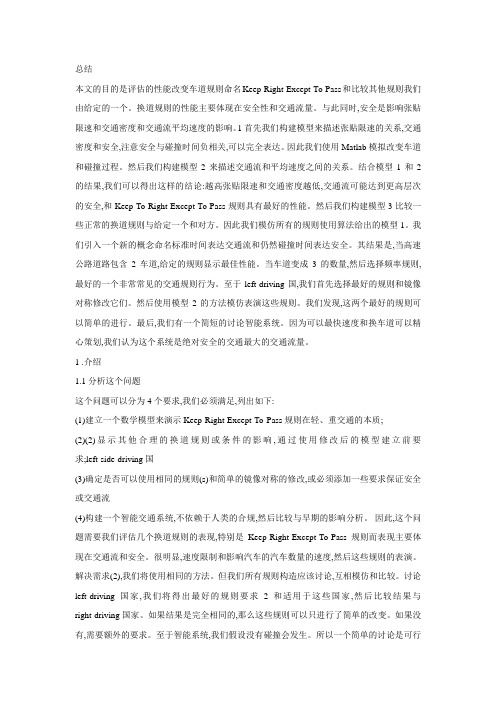
总结本文的目的是评估的性能改变车道规则命名Keep-Right-Except-To-Pass和比较其他规则我们由给定的一个。
换道规则的性能主要体现在安全性和交通流量。
与此同时,安全是影响张贴限速和交通密度和交通流平均速度的影响。
1首先我们构建模型来描述张贴限速的关系,交通密度和安全,注意安全与碰撞时间负相关,可以完全表达。
因此我们使用Matlab模拟改变车道和碰撞过程。
然后我们构建模型2来描述交通流和平均速度之间的关系。
结合模型1和2的结果,我们可以得出这样的结论:越高张贴限速和交通密度越低,交通流可能达到更高层次的安全,和Keep-To-Right-Except-To-Pass规则具有最好的性能。
然后我们构建模型3比较一些正常的换道规则与给定一个和对方。
因此我们模仿所有的规则使用算法给出的模型1。
我们引入一个新的概念命名标准时间表达交通流和仍然碰撞时间表达安全。
其结果是,当高速公路道路包含2车道,给定的规则显示最佳性能。
当车道变成3的数量,然后选择频率规则,最好的一个非常常见的交通规则行为。
至于left-driving国,我们首先选择最好的规则和镜像对称修改它们。
然后使用模型2的方法模仿表演这些规则。
我们发现,这两个最好的规则可以简单的进行。
最后,我们有一个简短的讨论智能系统。
因为可以最快速度和换车道可以精心策划,我们认为这个系统是绝对安全的交通最大的交通流量。
1 .介绍1.1分析这个问题这个问题可以分为4个要求,我们必须满足,列出如下:(1)建立一个数学模型来演示Keep-Right-Except-To-Pass规则在轻、重交通的本质;(2)(2)显示其他合理的换道规则或条件的影响,通过使用修改后的模型建立前要求;left-side-driving国(3)确定是否可以使用相同的规则(s)和简单的镜像对称的修改,或必须添加一些要求保证安全或交通流(4)构建一个智能交通系统,不依赖于人类的合规,然后比较与早期的影响分析。
[DOC]-数学建模美赛论文标准格式参考--中英文对照
![[DOC]-数学建模美赛论文标准格式参考--中英文对照](https://img.taocdn.com/s3/m/259f8b6fa0116c175e0e4822.png)
[DOC]-数学建模美赛论文标准格式参考--中英文对照数学建模美赛论文标准格式参考--中英文对照Your Paper's Title Starts Here: Please Centeruse Helvetica (Arial) 14论文的题目从这里开始:用Helvetica (Arial)14号FULL First Author1, a, FULL Second Author2,b and Last Author3,c 第一第二第三作者的全名1Full address of first author, including country第一作者的地址全名,包括国家2Full address of second author, including country第二作者的地址全名,包括国家3List all distinct addresses in the same way第三作者同上aemail, bemail, cemail第一第二第三作者的邮箱地址Keywords: List the keywords covered in your paper. These keywords will also be used by the publisher to produce a keyword index.关键字: 列出你论文中的关键词。
这些关键词将会被出版者用作制作一个关键词索引。
For the rest of the paper, please use Times Roman (Times New Roman) 12论文的其他部分请用Times Roman (Times New Roman) 12号字Abstract. This template explains and demonstrates how to prepareyour camera-ready paper for Trans Tech Publications. The best is to read these instructions and follow the outline of this text.Please make the page settings of your word processor to A4 format(21 x 29,7 cm or 8 x 11 inches); with the margins: bottom 1.5 cm (0.59 in) and top 2.5 cm (0.98 in), right/left margins must be 2 cm (0.78 in).摘要:这个模板解释和示范供稿技术刊物有限公司时,如何准备你的供相机使用文件。
美赛论文中文版(2008)、特等奖
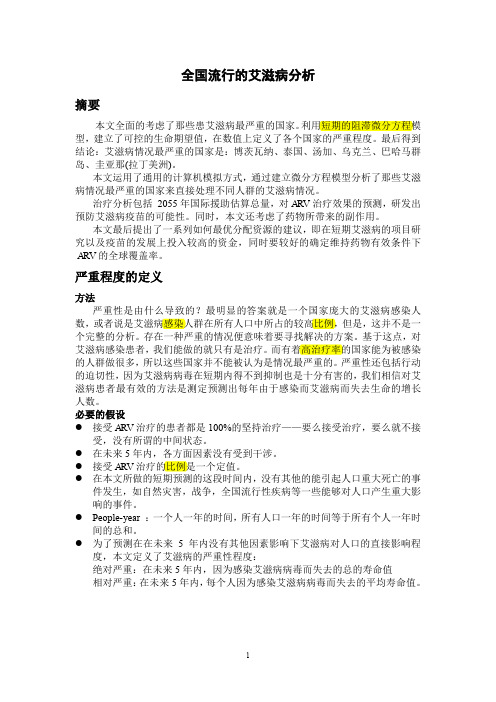
全国流行的艾滋病分析摘要本文全面的考虑了那些患艾滋病最严重的国家。
利用短期的阻滞微分方程模型,建立了可控的生命期望值,在数值上定义了各个国家的严重程度。
最后得到结论:艾滋病情况最严重的国家是:博茨瓦纳、泰国、汤加、乌克兰、巴哈马群岛、圭亚那(拉丁美洲)。
本文运用了通用的计算机模拟方式,通过建立微分方程模型分析了那些艾滋病情况最严重的国家来直接处理不同人群的艾滋病情况。
治疗分析包括2055年国际援助估算总量,对ARV治疗效果的预测,研发出预防艾滋病疫苗的可能性。
同时,本文还考虑了药物所带来的副作用。
本文最后提出了一系列如何最优分配资源的建议,即在短期艾滋病的项目研究以及疫苗的发展上投入较高的资金,同时要较好的确定维持药物有效条件下ARV的全球覆盖率。
严重程度的定义方法严重性是由什么导致的?最明显的答案就是一个国家庞大的艾滋病感染人数,或者说是艾滋病感染人群在所有人口中所占的较高比例,但是,这并不是一个完整的分析。
存在一种严重的情况便意味着要寻找解决的方案。
基于这点,对艾滋病感染患者,我们能做的就只有是治疗。
而有着高治疗率的国家能为被感染的人群做很多,所以这些国家并不能被认为是情况最严重的。
严重性还包括行动的迫切性,因为艾滋病病毒在短期内得不到抑制也是十分有害的,我们相信对艾滋病患者最有效的方法是测定预测出每年由于感染而艾滋病而失去生命的增长人数。
必要的假设●接受ARV治疗的患者都是100%的坚持治疗——要么接受治疗,要么就不接受,没有所谓的中间状态。
●在未来5年内,各方面因素没有受到干涉。
●接受ARV治疗的比例是一个定值。
●在本文所做的短期预测的这段时间内,没有其他的能引起人口重大死亡的事件发生,如自然灾害,战争,全国流行性疾病等一些能够对人口产生重大影响的事件。
●People-year :一个人一年的时间,所有人口一年的时间等于所有个人一年时间的总和。
●为了预测在在未来5年内没有其他因素影响下艾滋病对人口的直接影响程度,本文定义了艾滋病的严重性程度:绝对严重:在未来5年内,因为感染艾滋病病毒而失去的总的寿命值相对严重:在未来5年内,每个人因为感染艾滋病病毒而失去的平均寿命值。
美赛论文模板(中文版)
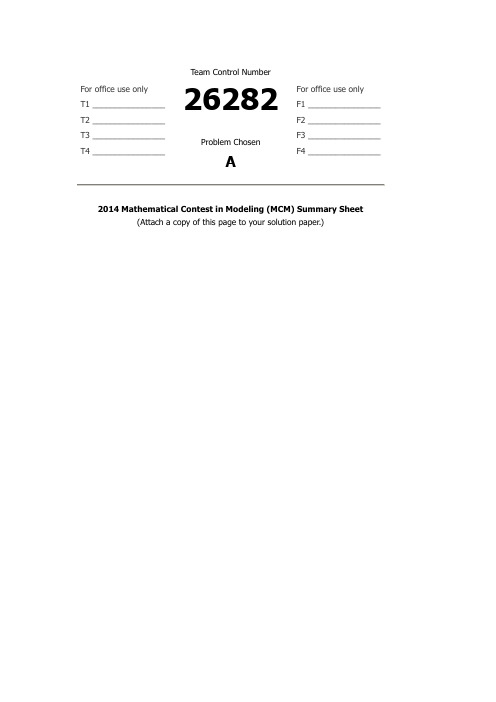
For office use onlyT1________________ T2________________ T3________________ T4________________Team Control Number 26282Problem ChosenAFor office use onlyF1________________F2________________F3________________F4________________2014 Mathematical Contest in Modeling (MCM) Summary Sheet (Attach a copy of this page to your solution paper.)1.Introduction近年来,世界上的交通拥堵问题越来越严重,严重的交通拥堵问题引发了人们的对现行交通规则的思考。
在汽车驾驶规则是右侧的国家多车道高速公路经常遵循除非超车否则靠右行驶的交通规则,那么这个交通规则是否能够对交通拥堵起着什么作用呢?在汽车驾驶规则是右侧的国家多车道高速公路经常遵循以下原则:司机必须在最右侧驾驶,除非他们正在超车,超车时必须先移到左侧车道在超车后再返回。
根据这个规则,在美国单向的3车道高速公路上,最左侧的车道是超车道,这条车道的目的就是超车。
现在我们提出了4个问题:1、什么是低负荷和高负荷,如何界定他们?2、这条规则在提升车流量的方面是否有效?3、这条规则在安全问题上所起的作用?4、这条规则对速度的限制?1.1 Survey of Previous Research1.2 Restatement of the problem本题需要我们建立一个数学模型对这个规则进行评价。
我们需要解决的问题如下:●什么是低负荷和高负荷,如何界定他们?●这条规则在提升车流量的方面是否有效?●这条规则在安全问题上所起的作用?●这条规则对速度的限制?●对于靠左行的规则,该模型能否可以使用??(待定)●如果交通运输完全在智能系统的控制下,会怎样影响建立的模型?针对以上问题,我们的解题思路和方法如下所示:◆我们根据交通密度对低负荷和高负荷进行界定,交通密度是指:在某时刻,每单位道路长度内一条道路的车辆数。
- 1、下载文档前请自行甄别文档内容的完整性,平台不提供额外的编辑、内容补充、找答案等附加服务。
- 2、"仅部分预览"的文档,不可在线预览部分如存在完整性等问题,可反馈申请退款(可完整预览的文档不适用该条件!)。
- 3、如文档侵犯您的权益,请联系客服反馈,我们会尽快为您处理(人工客服工作时间:9:00-18:30)。
目录问题回顾 (3)问题分析: (4)模型假设: (6)符号定义 (7)4.1---------- (8)4.2 有热水输入的温度变化模型 (17)4.2.1模型假设与定义 (17)4.2.2 模型的建立The establishment of the model (18)4.2.3 模型求解 (19)4.3 有人存在的温度变化模型Temperature model of human presence (21)4.3.1 模型影响因素的讨论Discussion influencing factors of the model (21)4.3.2模型的建立 (25)4.3.3 Solving model (29)5.1 优化目标的确定 (29)5.2 约束条件的确定 (31)5.3模型的求解 (32)5.4 泡泡剂的影响 (35)5.5 灵敏度的分析 (35)8 non-technical explanation of the bathtub (37)Summary人们经常在充满热水的浴缸里得到清洁和放松。
本文针对只有一个简单的热水龙头的浴缸,建立一个多目标优化模型,通过调整水龙头流量大小和流入水的温度来使整个泡澡过程浴缸内水温维持基本恒定且不会浪费太多水。
首先分析浴缸中水温度变化的具体情况。
根据能量转移的特点将浴缸中的热量损失分为两类情况:沿浴缸四壁和底面向空气中丧失的热量根据傅里叶导热定律求出;沿水面丧失的热量根据水由液态变为气态的焓变求出。
因涉及的参数过多,将系数进行回归分析的得到一个一元二次函数。
结合两类热量建立了温度关于时间的微分方程。
加入阻滞因子考虑环境温湿度升高对水温的影响,最后得到水温度随时间的变化规律(见图**)。
优化模型考虑保持水龙头匀速流入热水的情况。
将过程分为浴缸未加满和浴缸加满而水从排水口溢出的两种情况,根据能量守恒定律优化上述微分方程,建立一个有热源的情况下水的温度随时间变化的分段模型,(见图**)接下来考虑人在浴缸中对水温的影响。
我们从各个方面进行分析:人的体温恒定在37℃左右,能量仅因人的生理代谢而丧失,这一部分数量过小可以不考虑;而人在水中人的体积和运动都将引起浴缸中水散热面积和总质量的变化,从而改变了热量的损失情况。
因人的运动是连续且随机的,利用MATLAB生成随机数表示人进入水中的体积变化量,将运动过程离散化。
为体现其振荡的特点,我们利用三角函数拟合后离散的数据,以频率和振幅的变化来反映实际现象。
将得到的函数与上述模型相结合,作图分析其变化规律(见图**)。
利用以上温度变化的优化模型,结合用水量建立多目标优化模型。
将热水浴与缸中水温差、浴缸水温偏离最适温度最值进行正向化和归一化再加权求和定义为舒适度。
在流量维持稳定的情况下,要求舒适度越大而用水量越小。
因该优化模型中的约束条件中含有微分方程,难以求解,则对其进行离散仿真,采用模拟退火算法求解全局最优解。
最后讨论了加入泡泡剂后对模型的影响,求得矩形浴缸尺寸为长*宽*高=1.5m*0.6m*0.5m时,最优的热水温度、热水输入速率为T1= 63.4℃,f=0.33L/s。
然后对浴缸形状体积和人的形状体积等影响因素进行灵敏性分析,发现结果受浴缸体积的影响最大。
ﻩ问题回顾这是一个关于能量变化的连续型问题。
家庭中普通的浴缸无法像SPA的高端浴缸那样控制水温也没有热水喷射系统,泡澡的时间过长则会导致水变凉。
因此只能打开水龙头让热水源源不断的流进来,但是当水满了之后就打开排水口使得水从排水口流出,按照此种办法使得浴缸中的水保持相对恒定的温度。
Thisis acontinuous problem about energy changes. Households in the ord inary bathtub cannotbe like the SPA's high-endbathtub that control water temperature,there is no hot water injectionsystem, ba th toolong will cause the water cools.Thereforeonly open the faucettoletwater flow inasteady stream, butwhen thetub reaches its capacity,excesswater escapes t hrough an overflow drain, in accordance with such an approach makes thewater inthebathtubtomaintain a relatively consta nttemperature.我们知道水的温度是随着时间的变化而变化的,但是当加入热水后,浴缸中的水温将会发生变化,他不可以看作是一个恒温物体,因此我们需要建立一个浴缸中的水温关于位置和时间的变化而变化的模型,确定一个最佳的策略,决定水龙头入水量的大小和入水量的时间等因素,使得整个浴缸从头到尾的水都能够保持温度,还要不能浪费太多的水。
Weknowthat the water temperaturevariesover time,butwhen added to hot water, bath water temperature willchange,it cannot be seenas aconstant object, so we shoulddevelopa model of the temperature of thebath tub water inspaceand time to determine the best strategy the p erson in thebathtub can adopt to keep the temperature eventhroughout the bathtub and as closeaspossibleto the initial temperaturewithout wasting too muchwater.为了详细考虑现实生活中可能发生的情况,我们还要考虑人对温度变化过程的影响。
除了上述涉及到的因素外,浴缸的大小和形状、人的大小和形状、以及人在浴缸中的动作等。
例如可以考虑因人的运动使得水蒸发速率加快,在第一次加水时加入了泡泡剂来帮助清洁的等因素对模型的影响。
For a detailed consideration of real-life situations thatmayoccur, we have to consider the human impact on the process temperaturechanges. In addi tion to factors relatedtothe above,the size and shape of the tub,human size andshape,as well ashumanaction in t he tub.For example, consider theperson's movement sot hat the water evaporationrateaccelerated in the first addition of water is added to helpclean the bubble agentandother factors on the modelIn addition to the required one-page summaryforyourMCMsubmission,your report must include aone-page non-technical explanation for users of the bathtubthat describesyourstrategy while explainingwhyit is so difficulttoget an evenly maintained temperature throughout thebath water.问题分析:这个问题是一个连续型优化问题,我们应该从最基础的问题分析起,逐步完善和优化,得到最优解。
最开始我们找到一些关于数据,包括一般情况下室温为25℃,洗澡的最适合的温度为39℃,浴缸取的是市面上发展较好的玻璃钢材料的中空保温的浴缸。
Thisproblem is a continuous optimization problem,we startfrom themost basic problemanalysis, and gradually improve andoptimize toget the optimal solution.Tostart,wecollected somedata, including general roomtemperature 25℃,the mostsuitabletemperature bath for 39 ℃, andthe bathtub is FRPmaterial.首先我们考虑的是影响浴缸中水温度变化的因素。
将浴缸中的热量损失分为两类情况,沿浴缸四壁和底面的热量丧失和沿水面因水的蒸发丧失,两类情况设计的计算方法不同。
对于缸壁和底面丧失的热量,在水温达到恒定后,热量丧失主要是因浴缸上的热量向空气中散发,因此可以确定这一部分散发的热量。
而沿水面蒸发的部分我们不能仅仅考虑热对流引起的热量损失,还应该考虑水由液态变为气态,发生了物态变化所吸收的热量,因此我们需要考虑焓变的因素。
其中涉及到的变量过多,对于一些类似于相对湿度和大气压等值可以假定为定值,而对于一些随时间变化的值,因最终考虑的是温度与时间的关系,我们将各参数整合在一起,以一个关于时间的函数来表示。
最后将得到的二者丧失的能量相加,获得丧失的总能量,结合水的质量和比热容,将能量的损失转换成温度的变化。
限定浴缸尺寸大小,做出温度随时间的变化规律。
同时我们也必须考虑空气的温度随时间的变化,而不是恒定在25℃,对模型优化加入阻滞因子,考虑环境温湿度升高对水温的影响。
First, we considered the factors affecting the waterinthe bathtub temperature. There aretwoforms of bathtub water heat loss,heat conduction alongthe wallsand bottom of bathtub and water evaporationalong surface of thewater. Fortheheat loss of heat conduction alongthewalls andbottomof bathtub, itis mainly due to the heat bath on thecirculated air, sowe candetermine this part of the heat. For the heatloss of waterevaporation along surfaceof the water,we notonly considered the heat losscausedby thermal convection,butalso theheatlosscaused by water phase change, which is fromaliquid toagaseousstate,so we consider theenthalpychange. Toobtain the relationshipbetweentemperatureand time,we sum the energy loss ofthe two together to ge tthetotal energy loss, combined with the massof waterandthe specific heatcapacity, the energy lossisconverted into a changein temperature,and draw temperature variation with timefigure.Atthesame time,we have toconsider the air temperature changes overtime,ratherthan constant at 25℃, and the environmental effects ofelevatedtemperature andhu midity onthe watertemperature, retardation factorwas added to the model,因洗澡时可以一边洗一边加入热水,此时我们要考虑在有热源引入的情况下水温的变化。