Greedy Deep Diction Learning
deeplearning知识总结

机器学习——深度学习(Deep Learning)分类:Machine Learning2012-08-04 09:4988415人阅读评论(55)收藏举报algorithmclassificationfeaturesfunctionhierarchyDeep Learning是机器学习中一个非常接近AI的领域,其动机在于建立、模拟人脑进行分析学习的神经网络,最近研究了机器学习中一些深度学习的相关知识,本文给出一些很有用的资料和心得。
Key Words:有监督学习与无监督学习,分类、回归,密度估计、聚类,深度学习,Sparse DBN,1. 有监督学习和无监督学习给定一组数据(input,target)为Z=(X,Y)。
有监督学习:最常见的是regression & classification。
regression:Y是实数vector。
回归问题,就是拟合(X,Y)的一条曲线,使得下式cost functionL最小。
classification:Y是一个finite number,可以看做类标号。
分类问题需要首先给定有label 的数据训练分类器,故属于有监督学习过程。
分类问题中,cost function L(X,Y)是X属于类Y的概率的负对数。
,其中f i(X)=P(Y=i | X);无监督学习:无监督学习的目的是学习一个function f,使它可以描述给定数据的位置分布P(Z)。
包括两种:density estimation & clustering.density estimation就是密度估计,估计该数据在任意位置的分布密度clustering就是聚类,将Z聚集几类(如K-Means),或者给出一个样本属于每一类的概率。
由于不需要事先根据训练数据去train聚类器,故属于无监督学习。
PCA和很多deep learning算法都属于无监督学习。
2. 深度学习Deep Learning介绍Depth 概念:depth: the length of the longest path from an input to an output.Deep Architecture 的三个特点:深度不足会出现问题;人脑具有一个深度结构(每深入一层进行一次abstraction,由lower-layer的features描述而成的feature构成,就是上篇中提到的feature hierarchy问题,而且该hierarchy是一个稀疏矩阵);认知过程逐层进行,逐步抽象3篇文章介绍Deep Belief Networks,作为DBN的breakthrough3.Deep Learning Algorithm 的核心思想:把learning hierarchy 看做一个network,则①无监督学习用于每一层网络的pre-train;②每次用无监督学习只训练一层,将其训练结果作为其higher一层的输入;③用监督学习去调整所有层这里不负责任地理解下,举个例子在Autoencoder中,无监督学习学的是feature,有监督学习用在fine-tuning. 比如每一个neural network 学出的hidden layer就是feature,作为下一次神经网络无监督学习的input……这样一次次就学出了一个deep的网络,每一层都是上一次学习的hidden layer。
deep learning的方法

Deep Learning的方法主要包括以下步骤:
1. 特征学习:Deep Learning通过逐层训练的方式,从底层开始,先学习最简单的特征,然后使用这些特征作为更高层的输入,训练更复杂的特征。
这种方式可以让网络自动地学习到数据本身的内在结构,从而提取到更有代表性的特征。
2. 模型选择:选择合适的神经网络模型,例如多层感知器(MLP)、卷积神经网络(CNN)、循环神经网络(RNN)等,根据实际问题的需求进行选择。
3. 训练模型:使用大量的数据对模型进行训练,通过反向传播算法对模型进行优化,不断调整模型参数,以使得模型能够更好地拟合数据。
4. 模型评估:在训练完成后,使用测试集对模型进行评估,计算模型的准确率、精确率、召回率等指标,以评估模型的性能。
5. 模型优化:根据评估结果,对模型进行进一步的优化和调整,例如增加网络深度、改进模型结构、使用更先进的优化算法等,以提高模型的性能。
总的来说,Deep Learning的方法是通过逐层特征学习和大量数据训练来提高模型的性能,能够有效地处理复杂的非线性问题,并在语音识别、图像处理、自然语言处理等领域取得了很好的效果。
机器学习与人工智能领域中常用的英语词汇
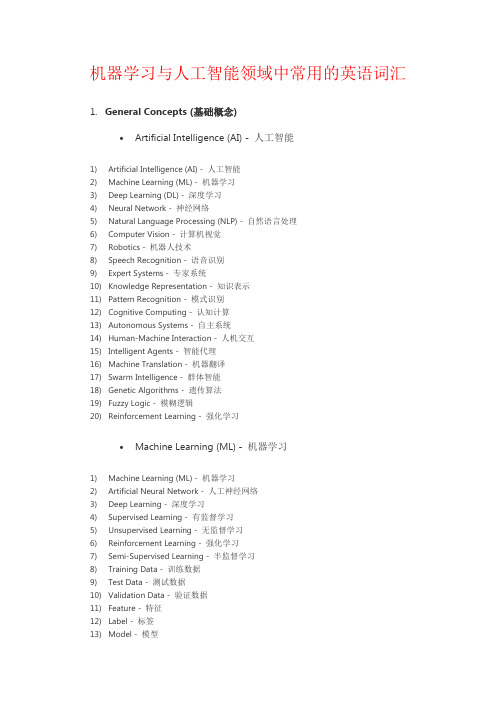
机器学习与人工智能领域中常用的英语词汇1.General Concepts (基础概念)•Artificial Intelligence (AI) - 人工智能1)Artificial Intelligence (AI) - 人工智能2)Machine Learning (ML) - 机器学习3)Deep Learning (DL) - 深度学习4)Neural Network - 神经网络5)Natural Language Processing (NLP) - 自然语言处理6)Computer Vision - 计算机视觉7)Robotics - 机器人技术8)Speech Recognition - 语音识别9)Expert Systems - 专家系统10)Knowledge Representation - 知识表示11)Pattern Recognition - 模式识别12)Cognitive Computing - 认知计算13)Autonomous Systems - 自主系统14)Human-Machine Interaction - 人机交互15)Intelligent Agents - 智能代理16)Machine Translation - 机器翻译17)Swarm Intelligence - 群体智能18)Genetic Algorithms - 遗传算法19)Fuzzy Logic - 模糊逻辑20)Reinforcement Learning - 强化学习•Machine Learning (ML) - 机器学习1)Machine Learning (ML) - 机器学习2)Artificial Neural Network - 人工神经网络3)Deep Learning - 深度学习4)Supervised Learning - 有监督学习5)Unsupervised Learning - 无监督学习6)Reinforcement Learning - 强化学习7)Semi-Supervised Learning - 半监督学习8)Training Data - 训练数据9)Test Data - 测试数据10)Validation Data - 验证数据11)Feature - 特征12)Label - 标签13)Model - 模型14)Algorithm - 算法15)Regression - 回归16)Classification - 分类17)Clustering - 聚类18)Dimensionality Reduction - 降维19)Overfitting - 过拟合20)Underfitting - 欠拟合•Deep Learning (DL) - 深度学习1)Deep Learning - 深度学习2)Neural Network - 神经网络3)Artificial Neural Network (ANN) - 人工神经网络4)Convolutional Neural Network (CNN) - 卷积神经网络5)Recurrent Neural Network (RNN) - 循环神经网络6)Long Short-Term Memory (LSTM) - 长短期记忆网络7)Gated Recurrent Unit (GRU) - 门控循环单元8)Autoencoder - 自编码器9)Generative Adversarial Network (GAN) - 生成对抗网络10)Transfer Learning - 迁移学习11)Pre-trained Model - 预训练模型12)Fine-tuning - 微调13)Feature Extraction - 特征提取14)Activation Function - 激活函数15)Loss Function - 损失函数16)Gradient Descent - 梯度下降17)Backpropagation - 反向传播18)Epoch - 训练周期19)Batch Size - 批量大小20)Dropout - 丢弃法•Neural Network - 神经网络1)Neural Network - 神经网络2)Artificial Neural Network (ANN) - 人工神经网络3)Deep Neural Network (DNN) - 深度神经网络4)Convolutional Neural Network (CNN) - 卷积神经网络5)Recurrent Neural Network (RNN) - 循环神经网络6)Long Short-Term Memory (LSTM) - 长短期记忆网络7)Gated Recurrent Unit (GRU) - 门控循环单元8)Feedforward Neural Network - 前馈神经网络9)Multi-layer Perceptron (MLP) - 多层感知器10)Radial Basis Function Network (RBFN) - 径向基函数网络11)Hopfield Network - 霍普菲尔德网络12)Boltzmann Machine - 玻尔兹曼机13)Autoencoder - 自编码器14)Spiking Neural Network (SNN) - 脉冲神经网络15)Self-organizing Map (SOM) - 自组织映射16)Restricted Boltzmann Machine (RBM) - 受限玻尔兹曼机17)Hebbian Learning - 海比安学习18)Competitive Learning - 竞争学习19)Neuroevolutionary - 神经进化20)Neuron - 神经元•Algorithm - 算法1)Algorithm - 算法2)Supervised Learning Algorithm - 有监督学习算法3)Unsupervised Learning Algorithm - 无监督学习算法4)Reinforcement Learning Algorithm - 强化学习算法5)Classification Algorithm - 分类算法6)Regression Algorithm - 回归算法7)Clustering Algorithm - 聚类算法8)Dimensionality Reduction Algorithm - 降维算法9)Decision Tree Algorithm - 决策树算法10)Random Forest Algorithm - 随机森林算法11)Support Vector Machine (SVM) Algorithm - 支持向量机算法12)K-Nearest Neighbors (KNN) Algorithm - K近邻算法13)Naive Bayes Algorithm - 朴素贝叶斯算法14)Gradient Descent Algorithm - 梯度下降算法15)Genetic Algorithm - 遗传算法16)Neural Network Algorithm - 神经网络算法17)Deep Learning Algorithm - 深度学习算法18)Ensemble Learning Algorithm - 集成学习算法19)Reinforcement Learning Algorithm - 强化学习算法20)Metaheuristic Algorithm - 元启发式算法•Model - 模型1)Model - 模型2)Machine Learning Model - 机器学习模型3)Artificial Intelligence Model - 人工智能模型4)Predictive Model - 预测模型5)Classification Model - 分类模型6)Regression Model - 回归模型7)Generative Model - 生成模型8)Discriminative Model - 判别模型9)Probabilistic Model - 概率模型10)Statistical Model - 统计模型11)Neural Network Model - 神经网络模型12)Deep Learning Model - 深度学习模型13)Ensemble Model - 集成模型14)Reinforcement Learning Model - 强化学习模型15)Support Vector Machine (SVM) Model - 支持向量机模型16)Decision Tree Model - 决策树模型17)Random Forest Model - 随机森林模型18)Naive Bayes Model - 朴素贝叶斯模型19)Autoencoder Model - 自编码器模型20)Convolutional Neural Network (CNN) Model - 卷积神经网络模型•Dataset - 数据集1)Dataset - 数据集2)Training Dataset - 训练数据集3)Test Dataset - 测试数据集4)Validation Dataset - 验证数据集5)Balanced Dataset - 平衡数据集6)Imbalanced Dataset - 不平衡数据集7)Synthetic Dataset - 合成数据集8)Benchmark Dataset - 基准数据集9)Open Dataset - 开放数据集10)Labeled Dataset - 标记数据集11)Unlabeled Dataset - 未标记数据集12)Semi-Supervised Dataset - 半监督数据集13)Multiclass Dataset - 多分类数据集14)Feature Set - 特征集15)Data Augmentation - 数据增强16)Data Preprocessing - 数据预处理17)Missing Data - 缺失数据18)Outlier Detection - 异常值检测19)Data Imputation - 数据插补20)Metadata - 元数据•Training - 训练1)Training - 训练2)Training Data - 训练数据3)Training Phase - 训练阶段4)Training Set - 训练集5)Training Examples - 训练样本6)Training Instance - 训练实例7)Training Algorithm - 训练算法8)Training Model - 训练模型9)Training Process - 训练过程10)Training Loss - 训练损失11)Training Epoch - 训练周期12)Training Batch - 训练批次13)Online Training - 在线训练14)Offline Training - 离线训练15)Continuous Training - 连续训练16)Transfer Learning - 迁移学习17)Fine-Tuning - 微调18)Curriculum Learning - 课程学习19)Self-Supervised Learning - 自监督学习20)Active Learning - 主动学习•Testing - 测试1)Testing - 测试2)Test Data - 测试数据3)Test Set - 测试集4)Test Examples - 测试样本5)Test Instance - 测试实例6)Test Phase - 测试阶段7)Test Accuracy - 测试准确率8)Test Loss - 测试损失9)Test Error - 测试错误10)Test Metrics - 测试指标11)Test Suite - 测试套件12)Test Case - 测试用例13)Test Coverage - 测试覆盖率14)Cross-Validation - 交叉验证15)Holdout Validation - 留出验证16)K-Fold Cross-Validation - K折交叉验证17)Stratified Cross-Validation - 分层交叉验证18)Test Driven Development (TDD) - 测试驱动开发19)A/B Testing - A/B 测试20)Model Evaluation - 模型评估•Validation - 验证1)Validation - 验证2)Validation Data - 验证数据3)Validation Set - 验证集4)Validation Examples - 验证样本5)Validation Instance - 验证实例6)Validation Phase - 验证阶段7)Validation Accuracy - 验证准确率8)Validation Loss - 验证损失9)Validation Error - 验证错误10)Validation Metrics - 验证指标11)Cross-Validation - 交叉验证12)Holdout Validation - 留出验证13)K-Fold Cross-Validation - K折交叉验证14)Stratified Cross-Validation - 分层交叉验证15)Leave-One-Out Cross-Validation - 留一法交叉验证16)Validation Curve - 验证曲线17)Hyperparameter Validation - 超参数验证18)Model Validation - 模型验证19)Early Stopping - 提前停止20)Validation Strategy - 验证策略•Supervised Learning - 有监督学习1)Supervised Learning - 有监督学习2)Label - 标签3)Feature - 特征4)Target - 目标5)Training Labels - 训练标签6)Training Features - 训练特征7)Training Targets - 训练目标8)Training Examples - 训练样本9)Training Instance - 训练实例10)Regression - 回归11)Classification - 分类12)Predictor - 预测器13)Regression Model - 回归模型14)Classifier - 分类器15)Decision Tree - 决策树16)Support Vector Machine (SVM) - 支持向量机17)Neural Network - 神经网络18)Feature Engineering - 特征工程19)Model Evaluation - 模型评估20)Overfitting - 过拟合21)Underfitting - 欠拟合22)Bias-Variance Tradeoff - 偏差-方差权衡•Unsupervised Learning - 无监督学习1)Unsupervised Learning - 无监督学习2)Clustering - 聚类3)Dimensionality Reduction - 降维4)Anomaly Detection - 异常检测5)Association Rule Learning - 关联规则学习6)Feature Extraction - 特征提取7)Feature Selection - 特征选择8)K-Means - K均值9)Hierarchical Clustering - 层次聚类10)Density-Based Clustering - 基于密度的聚类11)Principal Component Analysis (PCA) - 主成分分析12)Independent Component Analysis (ICA) - 独立成分分析13)T-distributed Stochastic Neighbor Embedding (t-SNE) - t分布随机邻居嵌入14)Gaussian Mixture Model (GMM) - 高斯混合模型15)Self-Organizing Maps (SOM) - 自组织映射16)Autoencoder - 自动编码器17)Latent Variable - 潜变量18)Data Preprocessing - 数据预处理19)Outlier Detection - 异常值检测20)Clustering Algorithm - 聚类算法•Reinforcement Learning - 强化学习1)Reinforcement Learning - 强化学习2)Agent - 代理3)Environment - 环境4)State - 状态5)Action - 动作6)Reward - 奖励7)Policy - 策略8)Value Function - 值函数9)Q-Learning - Q学习10)Deep Q-Network (DQN) - 深度Q网络11)Policy Gradient - 策略梯度12)Actor-Critic - 演员-评论家13)Exploration - 探索14)Exploitation - 开发15)Temporal Difference (TD) - 时间差分16)Markov Decision Process (MDP) - 马尔可夫决策过程17)State-Action-Reward-State-Action (SARSA) - 状态-动作-奖励-状态-动作18)Policy Iteration - 策略迭代19)Value Iteration - 值迭代20)Monte Carlo Methods - 蒙特卡洛方法•Semi-Supervised Learning - 半监督学习1)Semi-Supervised Learning - 半监督学习2)Labeled Data - 有标签数据3)Unlabeled Data - 无标签数据4)Label Propagation - 标签传播5)Self-Training - 自训练6)Co-Training - 协同训练7)Transudative Learning - 传导学习8)Inductive Learning - 归纳学习9)Manifold Regularization - 流形正则化10)Graph-based Methods - 基于图的方法11)Cluster Assumption - 聚类假设12)Low-Density Separation - 低密度分离13)Semi-Supervised Support Vector Machines (S3VM) - 半监督支持向量机14)Expectation-Maximization (EM) - 期望最大化15)Co-EM - 协同期望最大化16)Entropy-Regularized EM - 熵正则化EM17)Mean Teacher - 平均教师18)Virtual Adversarial Training - 虚拟对抗训练19)Tri-training - 三重训练20)Mix Match - 混合匹配•Feature - 特征1)Feature - 特征2)Feature Engineering - 特征工程3)Feature Extraction - 特征提取4)Feature Selection - 特征选择5)Input Features - 输入特征6)Output Features - 输出特征7)Feature Vector - 特征向量8)Feature Space - 特征空间9)Feature Representation - 特征表示10)Feature Transformation - 特征转换11)Feature Importance - 特征重要性12)Feature Scaling - 特征缩放13)Feature Normalization - 特征归一化14)Feature Encoding - 特征编码15)Feature Fusion - 特征融合16)Feature Dimensionality Reduction - 特征维度减少17)Continuous Feature - 连续特征18)Categorical Feature - 分类特征19)Nominal Feature - 名义特征20)Ordinal Feature - 有序特征•Label - 标签1)Label - 标签2)Labeling - 标注3)Ground Truth - 地面真值4)Class Label - 类别标签5)Target Variable - 目标变量6)Labeling Scheme - 标注方案7)Multi-class Labeling - 多类别标注8)Binary Labeling - 二分类标注9)Label Noise - 标签噪声10)Labeling Error - 标注错误11)Label Propagation - 标签传播12)Unlabeled Data - 无标签数据13)Labeled Data - 有标签数据14)Semi-supervised Learning - 半监督学习15)Active Learning - 主动学习16)Weakly Supervised Learning - 弱监督学习17)Noisy Label Learning - 噪声标签学习18)Self-training - 自训练19)Crowdsourcing Labeling - 众包标注20)Label Smoothing - 标签平滑化•Prediction - 预测1)Prediction - 预测2)Forecasting - 预测3)Regression - 回归4)Classification - 分类5)Time Series Prediction - 时间序列预测6)Forecast Accuracy - 预测准确性7)Predictive Modeling - 预测建模8)Predictive Analytics - 预测分析9)Forecasting Method - 预测方法10)Predictive Performance - 预测性能11)Predictive Power - 预测能力12)Prediction Error - 预测误差13)Prediction Interval - 预测区间14)Prediction Model - 预测模型15)Predictive Uncertainty - 预测不确定性16)Forecast Horizon - 预测时间跨度17)Predictive Maintenance - 预测性维护18)Predictive Policing - 预测式警务19)Predictive Healthcare - 预测性医疗20)Predictive Maintenance - 预测性维护•Classification - 分类1)Classification - 分类2)Classifier - 分类器3)Class - 类别4)Classify - 对数据进行分类5)Class Label - 类别标签6)Binary Classification - 二元分类7)Multiclass Classification - 多类分类8)Class Probability - 类别概率9)Decision Boundary - 决策边界10)Decision Tree - 决策树11)Support Vector Machine (SVM) - 支持向量机12)K-Nearest Neighbors (KNN) - K最近邻算法13)Naive Bayes - 朴素贝叶斯14)Logistic Regression - 逻辑回归15)Random Forest - 随机森林16)Neural Network - 神经网络17)SoftMax Function - SoftMax函数18)One-vs-All (One-vs-Rest) - 一对多(一对剩余)19)Ensemble Learning - 集成学习20)Confusion Matrix - 混淆矩阵•Regression - 回归1)Regression Analysis - 回归分析2)Linear Regression - 线性回归3)Multiple Regression - 多元回归4)Polynomial Regression - 多项式回归5)Logistic Regression - 逻辑回归6)Ridge Regression - 岭回归7)Lasso Regression - Lasso回归8)Elastic Net Regression - 弹性网络回归9)Regression Coefficients - 回归系数10)Residuals - 残差11)Ordinary Least Squares (OLS) - 普通最小二乘法12)Ridge Regression Coefficient - 岭回归系数13)Lasso Regression Coefficient - Lasso回归系数14)Elastic Net Regression Coefficient - 弹性网络回归系数15)Regression Line - 回归线16)Prediction Error - 预测误差17)Regression Model - 回归模型18)Nonlinear Regression - 非线性回归19)Generalized Linear Models (GLM) - 广义线性模型20)Coefficient of Determination (R-squared) - 决定系数21)F-test - F检验22)Homoscedasticity - 同方差性23)Heteroscedasticity - 异方差性24)Autocorrelation - 自相关25)Multicollinearity - 多重共线性26)Outliers - 异常值27)Cross-validation - 交叉验证28)Feature Selection - 特征选择29)Feature Engineering - 特征工程30)Regularization - 正则化2.Neural Networks and Deep Learning (神经网络与深度学习)•Convolutional Neural Network (CNN) - 卷积神经网络1)Convolutional Neural Network (CNN) - 卷积神经网络2)Convolution Layer - 卷积层3)Feature Map - 特征图4)Convolution Operation - 卷积操作5)Stride - 步幅6)Padding - 填充7)Pooling Layer - 池化层8)Max Pooling - 最大池化9)Average Pooling - 平均池化10)Fully Connected Layer - 全连接层11)Activation Function - 激活函数12)Rectified Linear Unit (ReLU) - 线性修正单元13)Dropout - 随机失活14)Batch Normalization - 批量归一化15)Transfer Learning - 迁移学习16)Fine-Tuning - 微调17)Image Classification - 图像分类18)Object Detection - 物体检测19)Semantic Segmentation - 语义分割20)Instance Segmentation - 实例分割21)Generative Adversarial Network (GAN) - 生成对抗网络22)Image Generation - 图像生成23)Style Transfer - 风格迁移24)Convolutional Autoencoder - 卷积自编码器25)Recurrent Neural Network (RNN) - 循环神经网络•Recurrent Neural Network (RNN) - 循环神经网络1)Recurrent Neural Network (RNN) - 循环神经网络2)Long Short-Term Memory (LSTM) - 长短期记忆网络3)Gated Recurrent Unit (GRU) - 门控循环单元4)Sequence Modeling - 序列建模5)Time Series Prediction - 时间序列预测6)Natural Language Processing (NLP) - 自然语言处理7)Text Generation - 文本生成8)Sentiment Analysis - 情感分析9)Named Entity Recognition (NER) - 命名实体识别10)Part-of-Speech Tagging (POS Tagging) - 词性标注11)Sequence-to-Sequence (Seq2Seq) - 序列到序列12)Attention Mechanism - 注意力机制13)Encoder-Decoder Architecture - 编码器-解码器架构14)Bidirectional RNN - 双向循环神经网络15)Teacher Forcing - 强制教师法16)Backpropagation Through Time (BPTT) - 通过时间的反向传播17)Vanishing Gradient Problem - 梯度消失问题18)Exploding Gradient Problem - 梯度爆炸问题19)Language Modeling - 语言建模20)Speech Recognition - 语音识别•Long Short-Term Memory (LSTM) - 长短期记忆网络1)Long Short-Term Memory (LSTM) - 长短期记忆网络2)Cell State - 细胞状态3)Hidden State - 隐藏状态4)Forget Gate - 遗忘门5)Input Gate - 输入门6)Output Gate - 输出门7)Peephole Connections - 窥视孔连接8)Gated Recurrent Unit (GRU) - 门控循环单元9)Vanishing Gradient Problem - 梯度消失问题10)Exploding Gradient Problem - 梯度爆炸问题11)Sequence Modeling - 序列建模12)Time Series Prediction - 时间序列预测13)Natural Language Processing (NLP) - 自然语言处理14)Text Generation - 文本生成15)Sentiment Analysis - 情感分析16)Named Entity Recognition (NER) - 命名实体识别17)Part-of-Speech Tagging (POS Tagging) - 词性标注18)Attention Mechanism - 注意力机制19)Encoder-Decoder Architecture - 编码器-解码器架构20)Bidirectional LSTM - 双向长短期记忆网络•Attention Mechanism - 注意力机制1)Attention Mechanism - 注意力机制2)Self-Attention - 自注意力3)Multi-Head Attention - 多头注意力4)Transformer - 变换器5)Query - 查询6)Key - 键7)Value - 值8)Query-Value Attention - 查询-值注意力9)Dot-Product Attention - 点积注意力10)Scaled Dot-Product Attention - 缩放点积注意力11)Additive Attention - 加性注意力12)Context Vector - 上下文向量13)Attention Score - 注意力分数14)SoftMax Function - SoftMax函数15)Attention Weight - 注意力权重16)Global Attention - 全局注意力17)Local Attention - 局部注意力18)Positional Encoding - 位置编码19)Encoder-Decoder Attention - 编码器-解码器注意力20)Cross-Modal Attention - 跨模态注意力•Generative Adversarial Network (GAN) - 生成对抗网络1)Generative Adversarial Network (GAN) - 生成对抗网络2)Generator - 生成器3)Discriminator - 判别器4)Adversarial Training - 对抗训练5)Minimax Game - 极小极大博弈6)Nash Equilibrium - 纳什均衡7)Mode Collapse - 模式崩溃8)Training Stability - 训练稳定性9)Loss Function - 损失函数10)Discriminative Loss - 判别损失11)Generative Loss - 生成损失12)Wasserstein GAN (WGAN) - Wasserstein GAN(WGAN)13)Deep Convolutional GAN (DCGAN) - 深度卷积生成对抗网络(DCGAN)14)Conditional GAN (c GAN) - 条件生成对抗网络(c GAN)15)Style GAN - 风格生成对抗网络16)Cycle GAN - 循环生成对抗网络17)Progressive Growing GAN (PGGAN) - 渐进式增长生成对抗网络(PGGAN)18)Self-Attention GAN (SAGAN) - 自注意力生成对抗网络(SAGAN)19)Big GAN - 大规模生成对抗网络20)Adversarial Examples - 对抗样本•Encoder-Decoder - 编码器-解码器1)Encoder-Decoder Architecture - 编码器-解码器架构2)Encoder - 编码器3)Decoder - 解码器4)Sequence-to-Sequence Model (Seq2Seq) - 序列到序列模型5)State Vector - 状态向量6)Context Vector - 上下文向量7)Hidden State - 隐藏状态8)Attention Mechanism - 注意力机制9)Teacher Forcing - 强制教师法10)Beam Search - 束搜索11)Recurrent Neural Network (RNN) - 循环神经网络12)Long Short-Term Memory (LSTM) - 长短期记忆网络13)Gated Recurrent Unit (GRU) - 门控循环单元14)Bidirectional Encoder - 双向编码器15)Greedy Decoding - 贪婪解码16)Masking - 遮盖17)Dropout - 随机失活18)Embedding Layer - 嵌入层19)Cross-Entropy Loss - 交叉熵损失20)Tokenization - 令牌化•Transfer Learning - 迁移学习1)Transfer Learning - 迁移学习2)Source Domain - 源领域3)Target Domain - 目标领域4)Fine-Tuning - 微调5)Domain Adaptation - 领域自适应6)Pre-Trained Model - 预训练模型7)Feature Extraction - 特征提取8)Knowledge Transfer - 知识迁移9)Unsupervised Domain Adaptation - 无监督领域自适应10)Semi-Supervised Domain Adaptation - 半监督领域自适应11)Multi-Task Learning - 多任务学习12)Data Augmentation - 数据增强13)Task Transfer - 任务迁移14)Model Agnostic Meta-Learning (MAML) - 与模型无关的元学习(MAML)15)One-Shot Learning - 单样本学习16)Zero-Shot Learning - 零样本学习17)Few-Shot Learning - 少样本学习18)Knowledge Distillation - 知识蒸馏19)Representation Learning - 表征学习20)Adversarial Transfer Learning - 对抗迁移学习•Pre-trained Models - 预训练模型1)Pre-trained Model - 预训练模型2)Transfer Learning - 迁移学习3)Fine-Tuning - 微调4)Knowledge Transfer - 知识迁移5)Domain Adaptation - 领域自适应6)Feature Extraction - 特征提取7)Representation Learning - 表征学习8)Language Model - 语言模型9)Bidirectional Encoder Representations from Transformers (BERT) - 双向编码器结构转换器10)Generative Pre-trained Transformer (GPT) - 生成式预训练转换器11)Transformer-based Models - 基于转换器的模型12)Masked Language Model (MLM) - 掩蔽语言模型13)Cloze Task - 填空任务14)Tokenization - 令牌化15)Word Embeddings - 词嵌入16)Sentence Embeddings - 句子嵌入17)Contextual Embeddings - 上下文嵌入18)Self-Supervised Learning - 自监督学习19)Large-Scale Pre-trained Models - 大规模预训练模型•Loss Function - 损失函数1)Loss Function - 损失函数2)Mean Squared Error (MSE) - 均方误差3)Mean Absolute Error (MAE) - 平均绝对误差4)Cross-Entropy Loss - 交叉熵损失5)Binary Cross-Entropy Loss - 二元交叉熵损失6)Categorical Cross-Entropy Loss - 分类交叉熵损失7)Hinge Loss - 合页损失8)Huber Loss - Huber损失9)Wasserstein Distance - Wasserstein距离10)Triplet Loss - 三元组损失11)Contrastive Loss - 对比损失12)Dice Loss - Dice损失13)Focal Loss - 焦点损失14)GAN Loss - GAN损失15)Adversarial Loss - 对抗损失16)L1 Loss - L1损失17)L2 Loss - L2损失18)Huber Loss - Huber损失19)Quantile Loss - 分位数损失•Activation Function - 激活函数1)Activation Function - 激活函数2)Sigmoid Function - Sigmoid函数3)Hyperbolic Tangent Function (Tanh) - 双曲正切函数4)Rectified Linear Unit (Re LU) - 矩形线性单元5)Parametric Re LU (P Re LU) - 参数化Re LU6)Exponential Linear Unit (ELU) - 指数线性单元7)Swish Function - Swish函数8)Softplus Function - Soft plus函数9)Softmax Function - SoftMax函数10)Hard Tanh Function - 硬双曲正切函数11)Softsign Function - Softsign函数12)GELU (Gaussian Error Linear Unit) - GELU(高斯误差线性单元)13)Mish Function - Mish函数14)CELU (Continuous Exponential Linear Unit) - CELU(连续指数线性单元)15)Bent Identity Function - 弯曲恒等函数16)Gaussian Error Linear Units (GELUs) - 高斯误差线性单元17)Adaptive Piecewise Linear (APL) - 自适应分段线性函数18)Radial Basis Function (RBF) - 径向基函数•Backpropagation - 反向传播1)Backpropagation - 反向传播2)Gradient Descent - 梯度下降3)Partial Derivative - 偏导数4)Chain Rule - 链式法则5)Forward Pass - 前向传播6)Backward Pass - 反向传播7)Computational Graph - 计算图8)Neural Network - 神经网络9)Loss Function - 损失函数10)Gradient Calculation - 梯度计算11)Weight Update - 权重更新12)Activation Function - 激活函数13)Optimizer - 优化器14)Learning Rate - 学习率15)Mini-Batch Gradient Descent - 小批量梯度下降16)Stochastic Gradient Descent (SGD) - 随机梯度下降17)Batch Gradient Descent - 批量梯度下降18)Momentum - 动量19)Adam Optimizer - Adam优化器20)Learning Rate Decay - 学习率衰减•Gradient Descent - 梯度下降1)Gradient Descent - 梯度下降2)Stochastic Gradient Descent (SGD) - 随机梯度下降3)Mini-Batch Gradient Descent - 小批量梯度下降4)Batch Gradient Descent - 批量梯度下降5)Learning Rate - 学习率6)Momentum - 动量7)Adaptive Moment Estimation (Adam) - 自适应矩估计8)RMSprop - 均方根传播9)Learning Rate Schedule - 学习率调度10)Convergence - 收敛11)Divergence - 发散12)Adagrad - 自适应学习速率方法13)Adadelta - 自适应增量学习率方法14)Adamax - 自适应矩估计的扩展版本15)Nadam - Nesterov Accelerated Adaptive Moment Estimation16)Learning Rate Decay - 学习率衰减17)Step Size - 步长18)Conjugate Gradient Descent - 共轭梯度下降19)Line Search - 线搜索20)Newton's Method - 牛顿法•Learning Rate - 学习率1)Learning Rate - 学习率2)Adaptive Learning Rate - 自适应学习率3)Learning Rate Decay - 学习率衰减4)Initial Learning Rate - 初始学习率5)Step Size - 步长6)Momentum - 动量7)Exponential Decay - 指数衰减8)Annealing - 退火9)Cyclical Learning Rate - 循环学习率10)Learning Rate Schedule - 学习率调度11)Warm-up - 预热12)Learning Rate Policy - 学习率策略13)Learning Rate Annealing - 学习率退火14)Cosine Annealing - 余弦退火15)Gradient Clipping - 梯度裁剪16)Adapting Learning Rate - 适应学习率17)Learning Rate Multiplier - 学习率倍增器18)Learning Rate Reduction - 学习率降低19)Learning Rate Update - 学习率更新20)Scheduled Learning Rate - 定期学习率•Batch Size - 批量大小1)Batch Size - 批量大小2)Mini-Batch - 小批量3)Batch Gradient Descent - 批量梯度下降4)Stochastic Gradient Descent (SGD) - 随机梯度下降5)Mini-Batch Gradient Descent - 小批量梯度下降6)Online Learning - 在线学习7)Full-Batch - 全批量8)Data Batch - 数据批次9)Training Batch - 训练批次10)Batch Normalization - 批量归一化11)Batch-wise Optimization - 批量优化12)Batch Processing - 批量处理13)Batch Sampling - 批量采样14)Adaptive Batch Size - 自适应批量大小15)Batch Splitting - 批量分割16)Dynamic Batch Size - 动态批量大小17)Fixed Batch Size - 固定批量大小18)Batch-wise Inference - 批量推理19)Batch-wise Training - 批量训练20)Batch Shuffling - 批量洗牌•Epoch - 训练周期1)Training Epoch - 训练周期2)Epoch Size - 周期大小3)Early Stopping - 提前停止4)Validation Set - 验证集5)Training Set - 训练集6)Test Set - 测试集7)Overfitting - 过拟合8)Underfitting - 欠拟合9)Model Evaluation - 模型评估10)Model Selection - 模型选择11)Hyperparameter Tuning - 超参数调优12)Cross-Validation - 交叉验证13)K-fold Cross-Validation - K折交叉验证14)Stratified Cross-Validation - 分层交叉验证15)Leave-One-Out Cross-Validation (LOOCV) - 留一法交叉验证16)Grid Search - 网格搜索17)Random Search - 随机搜索18)Model Complexity - 模型复杂度19)Learning Curve - 学习曲线20)Convergence - 收敛3.Machine Learning Techniques and Algorithms (机器学习技术与算法)•Decision Tree - 决策树1)Decision Tree - 决策树2)Node - 节点3)Root Node - 根节点4)Leaf Node - 叶节点5)Internal Node - 内部节点6)Splitting Criterion - 分裂准则7)Gini Impurity - 基尼不纯度8)Entropy - 熵9)Information Gain - 信息增益10)Gain Ratio - 增益率11)Pruning - 剪枝12)Recursive Partitioning - 递归分割13)CART (Classification and Regression Trees) - 分类回归树14)ID3 (Iterative Dichotomiser 3) - 迭代二叉树315)C4.5 (successor of ID3) - C4.5(ID3的后继者)16)C5.0 (successor of C4.5) - C5.0(C4.5的后继者)17)Split Point - 分裂点18)Decision Boundary - 决策边界19)Pruned Tree - 剪枝后的树20)Decision Tree Ensemble - 决策树集成•Random Forest - 随机森林1)Random Forest - 随机森林2)Ensemble Learning - 集成学习3)Bootstrap Sampling - 自助采样4)Bagging (Bootstrap Aggregating) - 装袋法5)Out-of-Bag (OOB) Error - 袋外误差6)Feature Subset - 特征子集7)Decision Tree - 决策树8)Base Estimator - 基础估计器9)Tree Depth - 树深度10)Randomization - 随机化11)Majority Voting - 多数投票12)Feature Importance - 特征重要性13)OOB Score - 袋外得分14)Forest Size - 森林大小15)Max Features - 最大特征数16)Min Samples Split - 最小分裂样本数17)Min Samples Leaf - 最小叶节点样本数18)Gini Impurity - 基尼不纯度19)Entropy - 熵20)Variable Importance - 变量重要性•Support Vector Machine (SVM) - 支持向量机1)Support Vector Machine (SVM) - 支持向量机2)Hyperplane - 超平面3)Kernel Trick - 核技巧4)Kernel Function - 核函数5)Margin - 间隔6)Support Vectors - 支持向量7)Decision Boundary - 决策边界8)Maximum Margin Classifier - 最大间隔分类器9)Soft Margin Classifier - 软间隔分类器10) C Parameter - C参数11)Radial Basis Function (RBF) Kernel - 径向基函数核12)Polynomial Kernel - 多项式核13)Linear Kernel - 线性核14)Quadratic Kernel - 二次核15)Gaussian Kernel - 高斯核16)Regularization - 正则化17)Dual Problem - 对偶问题18)Primal Problem - 原始问题19)Kernelized SVM - 核化支持向量机20)Multiclass SVM - 多类支持向量机•K-Nearest Neighbors (KNN) - K-最近邻1)K-Nearest Neighbors (KNN) - K-最近邻2)Nearest Neighbor - 最近邻3)Distance Metric - 距离度量4)Euclidean Distance - 欧氏距离5)Manhattan Distance - 曼哈顿距离6)Minkowski Distance - 闵可夫斯基距离7)Cosine Similarity - 余弦相似度8)K Value - K值9)Majority Voting - 多数投票10)Weighted KNN - 加权KNN11)Radius Neighbors - 半径邻居12)Ball Tree - 球树13)KD Tree - KD树14)Locality-Sensitive Hashing (LSH) - 局部敏感哈希15)Curse of Dimensionality - 维度灾难16)Class Label - 类标签17)Training Set - 训练集18)Test Set - 测试集19)Validation Set - 验证集20)Cross-Validation - 交叉验证•Naive Bayes - 朴素贝叶斯1)Naive Bayes - 朴素贝叶斯2)Bayes' Theorem - 贝叶斯定理3)Prior Probability - 先验概率4)Posterior Probability - 后验概率5)Likelihood - 似然6)Class Conditional Probability - 类条件概率7)Feature Independence Assumption - 特征独立假设8)Multinomial Naive Bayes - 多项式朴素贝叶斯9)Gaussian Naive Bayes - 高斯朴素贝叶斯10)Bernoulli Naive Bayes - 伯努利朴素贝叶斯11)Laplace Smoothing - 拉普拉斯平滑12)Add-One Smoothing - 加一平滑13)Maximum A Posteriori (MAP) - 最大后验概率14)Maximum Likelihood Estimation (MLE) - 最大似然估计15)Classification - 分类16)Feature Vectors - 特征向量17)Training Set - 训练集18)Test Set - 测试集19)Class Label - 类标签20)Confusion Matrix - 混淆矩阵•Clustering - 聚类1)Clustering - 聚类2)Centroid - 质心3)Cluster Analysis - 聚类分析4)Partitioning Clustering - 划分式聚类5)Hierarchical Clustering - 层次聚类6)Density-Based Clustering - 基于密度的聚类7)K-Means Clustering - K均值聚类8)K-Medoids Clustering - K中心点聚类9)DBSCAN (Density-Based Spatial Clustering of Applications with Noise) - 基于密度的空间聚类算法10)Agglomerative Clustering - 聚合式聚类11)Dendrogram - 系统树图12)Silhouette Score - 轮廓系数13)Elbow Method - 肘部法则14)Clustering Validation - 聚类验证15)Intra-cluster Distance - 类内距离16)Inter-cluster Distance - 类间距离17)Cluster Cohesion - 类内连贯性18)Cluster Separation - 类间分离度19)Cluster Assignment - 聚类分配20)Cluster Label - 聚类标签•K-Means - K-均值1)K-Means - K-均值2)Centroid - 质心3)Cluster - 聚类4)Cluster Center - 聚类中心5)Cluster Assignment - 聚类分配6)Cluster Analysis - 聚类分析7)K Value - K值8)Elbow Method - 肘部法则9)Inertia - 惯性10)Silhouette Score - 轮廓系数11)Convergence - 收敛12)Initialization - 初始化13)Euclidean Distance - 欧氏距离14)Manhattan Distance - 曼哈顿距离15)Distance Metric - 距离度量16)Cluster Radius - 聚类半径17)Within-Cluster Variation - 类内变异18)Cluster Quality - 聚类质量19)Clustering Algorithm - 聚类算法20)Clustering Validation - 聚类验证•Dimensionality Reduction - 降维1)Dimensionality Reduction - 降维2)Feature Extraction - 特征提取3)Feature Selection - 特征选择4)Principal Component Analysis (PCA) - 主成分分析5)Singular Value Decomposition (SVD) - 奇异值分解6)Linear Discriminant Analysis (LDA) - 线性判别分析7)t-Distributed Stochastic Neighbor Embedding (t-SNE) - t-分布随机邻域嵌入8)Autoencoder - 自编码器9)Manifold Learning - 流形学习10)Locally Linear Embedding (LLE) - 局部线性嵌入11)Isomap - 等度量映射12)Uniform Manifold Approximation and Projection (UMAP) - 均匀流形逼近与投影13)Kernel PCA - 核主成分分析14)Non-negative Matrix Factorization (NMF) - 非负矩阵分解15)Independent Component Analysis (ICA) - 独立成分分析16)Variational Autoencoder (VAE) - 变分自编码器17)Sparse Coding - 稀疏编码18)Random Projection - 随机投影19)Neighborhood Preserving Embedding (NPE) - 保持邻域结构的嵌入20)Curvilinear Component Analysis (CCA) - 曲线成分分析•Principal Component Analysis (PCA) - 主成分分析1)Principal Component Analysis (PCA) - 主成分分析2)Eigenvector - 特征向量3)Eigenvalue - 特征值4)Covariance Matrix - 协方差矩阵。
GreedyLayer-WiseTrainingofDeepNetworks
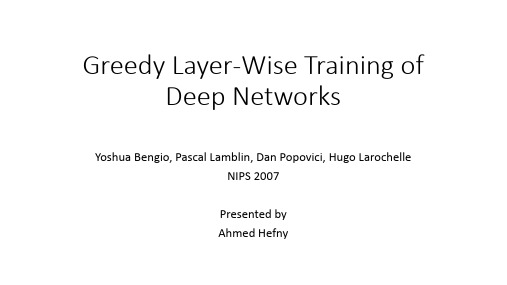
Greedy Layer-Wise Training of Deep NetworksYoshua Bengio, Pascal Lamblin, Dan Popovici, Hugo LarochelleNIPS 2007Presented byAhmed HefnyStory so far …•Deep neural nets are more expressive: Can learn wider classes offunctions with less hidden units (parameters) and training examples.•Unfortunately they are not easy to train with randomly initializedgradient-based methods.Story so far …•Hinton et. al. (2006) proposed greedy unsupervised layer-wisetraining:•Greedy layer-wise: Train layers sequentially starting from bottom(input) layer.•Unsupervised: Each layer learns a higher-level representation ofthe layer below. The training criterion does not depend on thelabels.•Each layer is trained as a Restricted Boltzman Machine. (RBM is thebuilding block of Deep Belief Networks).•The trained model can be fine tuned using a supervised method.RBM 0RBM 1RBM 2This paper•Extends the concept to: •Continuous variables •Uncooperative input distributions•Simultaneous Layer Training•Explores variations to better understand the training method:•What if we use greedy supervised layer-wise training ?•What if we replace RBMs with auto-encoders ?RBM 0RBM 1 RBM 2Outline•Review•Restricted Boltzman Machines•Deep Belief Networks•Greedy layer-wise Training •Supervised Fine-tuning •Extensions•Continuous Inputs•Uncooperative Input Distributions •Simultaneous Training •Analysis ExperimentsOutline•Review•Restricted Boltzman Machines•Deep Belief Networks•Greedy layer-wise Training •Supervised Fine-tuning •Extensions•Continuous Inputs•Uncooperative Input Distributions •Simultaneous Training •Analysis ExperimentsRestricted Boltzman Machinevℎ Undirected bipartite graphical model with connections betweenvisible nodes and hidden nodes.Corresponds to joint probability distribution P v,ℎ=1Zexp(−energy(v,ℎ))=1Z exp(v ′Wℎ+b ′v +c ′ℎ)Restricted Boltzman Machinevℎ Undirected bipartite graphical model with connections betweenvisible nodes and hidden nodes.Corresponds to joint probability distribution P v,ℎ=1Z exp(ℎ′Wv +b ′v +c ′ℎ)Q ℎv = P(ℎj |v)j Q ℎj =1v =sigm(c j + W jk v k k ) P v ℎ= P(v k |ℎ)kP v k =1ℎ=sigm(b k + W jk ℎj j )Factorized ConditionalsRestricted Boltzman Machine (Training) •Given input vectors V0, adjust θ=(W,b,c) to increase log P V0log P v0=log P(v0,ℎ)ℎ=log exp−energy v0,ℎ−log exp−energy v,ℎv,ℎℎðlog P v0ðθ=−Qℎv0ℎðenergy v0,ℎðθ+P(v,ℎ)v,ℎðenergy v,ℎðθðlog P v0ðθk =−Qℎv0ℎðenergy v0,ℎðθk+P v Q(ℎk|v)ℎkvðenergy v,ℎðθkRestricted Boltzman Machine (Training) •Given input vectors V0, adjust θ=(W,b,c) to increase log P V0log P v0=log P(v0,ℎ)ℎ=log exp−energy v0,ℎ−log exp−energy v,ℎv,ℎℎðlog P v0ðθ=−Qℎv0ℎðenergy v0,ℎðθ+P(v,ℎ)v,ℎðenergy v,ℎðθðlog P v0ðθk =−Qℎv0ℎðenergy v0,ℎðθk+P v Q(ℎk|v)ℎkvðenergy v,ℎðθkRestricted Boltzman Machine (Training) •Given input vectors V0, adjust θ=(W,b,c) to increase log P V0log P v0=log P(v0,ℎ)ℎ=log exp−energy v0,ℎ−log exp−energy v,ℎv,ℎℎðlog P v0ðθ=−Qℎv0ℎðenergy v0,ℎðθ+P(v,ℎ)v,ℎðenergy v,ℎðθðlog P v0ðθk=−Qℎv0ℎðenergy v0,ℎðθk+P v Q(ℎk|v)ℎkvðenergy v,ℎðθk Sample ℎ0 given v0Sample v1 and ℎ1 using Gibbs samplingRestricted Boltzman Machine (Training) •Now we can perform stochastic gradient descent on data log-likelihood•Stop based on some criterion(e.g. reconstruction error −log P(v1=x|v0=x)Deep Belief Network•A DBN is a model of the formP x,g1,g2,…,g l=P(x|g1)P g1g2…P g l−2g l−1P(g l−1,g l)x=g0 denotes input variablesg denotes hidden layers of causal variablesDeep Belief Network•A DBN is a model of the formP x,g1,g2,…,g l=P(x|g1)P g1g2…P g L−2g L−1P(g L−1,g L)x=g0 denotes input variablesg denotes hidden layers of causal variablesDeep Belief Network•A DBN is a model of the formP x,g 1,g 2,…,g l =P(x|g 1) P g 1g 2…P g l−2g l−1P(g l−1,g l )x =g 0 denotes input variablesg denotes hidden layers of causal variablesP(g l−1,g l ) is an RBM P g i g i+1= P(g j i |g i+1)j P g j i g i+1=sigm(b j i + W kj i g k i+1)n i+1k RBM = InfinitelyDeep network withtied weightsGreedy layer-wise training •P(g1|g0) is intractable •Approximate with Q(g1|g0)•Treat bottom two layers as an RBM•Fit parameters using contrastive divergenceGreedy layer-wise training •P(g1|g0) is intractable •Approximate with Q(g1|g0)•Treat bottom two layers as an RBM•Fit parameters using contrastive divergence •That gives an approximate P g1•We need to match it with P(g1)Greedy layer-wise training •Approximate P g l g l−1≈Q(g l|g l−1)•Treat layers l−1,l as an RBM•Fit parameters using contrastive divergence•Sample g0l−1 recursively using Q g i g i−1 starting from g0Outline•Review•Restricted Boltzman Machines•Deep Belief Networks•Greedy layer-wise Training •Supervised Fine-tuning •Extensions•Continuous Inputs•Uncooperative Input Distributions •Simultaneous Training •Analysis ExperimentsSupervised Fine Tuning (In this paper)•Use greedy layer-wise training to initialize weights of all layers except output layer.•For fine-tuning, use stochastic gradient descent of a cost function on the outputs where the conditional expected values of hidden nodes are approximated using mean-field.E g i g i−1=μi−1=μi=sigm(b i+W iμi−1)Supervised Fine Tuning (In this paper)•Use greedy layer-wise training to initialize weights of all layers except output layer.•Use backpropagationOutline•Review•Restricted Boltzman Machines•Deep Belief Networks•Greedy layer-wise Training •Supervised Fine-tuning •Extensions•Continuous Inputs•Uncooperative Input Distributions •Simultaneous Training •Analysis ExperimentsContinuous Inputs•Recall RBMs:•Qℎj v∝Qℎj,v∝expℎj w′v+b jℎj∝exp(w′v+b j)ℎj=exp(a vℎj)•If we restrict ℎj∈I={0,1} then normalization gives us binomial with p given by sigmoid.•Instead, if I=[0,∞] we get exponential density•If I is closed interval then we get truncated exponentialContinuous Inputs (Case for truncated exponential [0,1])•SamplingFor truncated exponential, inverse CDF can be usedh j=F−1U=log(1−U×(1−exp a v)a(v)where U is sampled uniformly from [0,1] •Conditional ExpectationEℎj v=11−exp (−a v)−1a(v)Continuous Inputs•To handle Gaussian inputs, we need to augment the energy function with a term quadratic in ℎ.•For a diagonal covariance matrixPℎj v=a vℎj+d jℎj2GivingEℎj z=a(x)/2d2Continuous Hidden Nodes ?Continuous Hidden Nodes ?•Truncated ExponentialEℎj v=11−exp (−a v)−1a(v)•GaussianEℎj v=a(v)/2d2Uncooperative Input Distributions•Settingx~p xy=f x+noise•No particular relation between p and f, (e.g. Gaussian and sinus)Uncooperative Input Distributions•Settingx~p xy=f x+noise•No particular relation between p and f, (e.g. Gaussian and sinus) •Problem: Unsupvervised pre-training may not help predictionOutline•Review•Restricted Boltzman Machines•Deep Belief Networks•Greedy layer-wise Training •Supervised Fine-tuning •Extensions•Analysis ExperimentsUncooperative Input Distributions •Proposal: Mix unsupervised and supervised training for each layerTemp. Ouptut LayerStochastic Gradient of input log likelihoodby Contrastive DivergenceStochastic Gradient of prediction errorCombined UpdateSimultaneous Layer Training•Greedy Layer-wise Training•For each layer•Repeat Until Criterion Met•Sample layer input (by recursively applying trained layers to data)•Update parameters using contrastive divergenceSimultaneous Layer Training•Simultaneous Training•Repeat Until Criterion Met•Sample input to all layers•Update parameters of all layers using contrastive divergence•Simpler: One criterion for the entire network •Takes more timeOutline•Review•Restricted Boltzman Machines•Deep Belief Networks•Greedy layer-wise Training •Supervised Fine-tuning •Extensions•Continuous Inputs•Uncooperative Input Distributions •Simultaneous Training •Analysis ExperimentsExperiments•Does greedy unsupervised pre-training help ? •What if we replace RBM with auto-encoders ? •What if we do greedy supervised pre-training ?•Does continuous variable modeling help ? •Does partially supervised pre-training help ?Experiment 1•Does greedy unsupervised pre-training help ? •What if we replace RBM with auto-encoders ? •What if we do greedy supervised pre-training ?•Does continuous variable modeling help ? •Does partially supervised pre-training help ?Experiment 1Experiment 1Experiment 1(MSE and Training Errors)Partially Supervised < Unsupervised Pre-training < No Pre-trainingGaussian < BinomialExperiment 2•Does greedy unsupervised pre-training help ? •What if we replace RBM with auto-encoders ? •What if we do greedy supervised pre-training ?•Does continuous variable modeling help ? •Does partially supervised pre-training help ?Experiment 2•Auto Encoders•Learn a compact representation to reconstruct Xp x=sigm c+Wsigm b+W′x •Trained to minimize reconstruction cross-entropyR=−x i log p x i+i (1−x i)log p1−x iX XExperiment 2(500~1000) layer width 20 nodes in last two layersExperiment 2•Auto-encoder pre-training outperforms supervised pre-training but is still outperformed by RBM.•Without pre-training, deep nets do not generalize well, but they can still fit the data if the output layers are wide enough.Conclusions•Unsupervised pre-training is important for deep networks. •Partial supervision further enhances results, especially when input distribution and the function to be estimated are not closely related. •Explicitly modeling conditional inputs is better than using binomial models.Thanks。
人工智能中的深度学习理论与方法

人工智能中的深度学习理论与方法
一、深度学习的定义
深度学习(Deep Learning)是一种人工智能,它可以从数据中自动学习复杂的模式。
它模拟生物大脑的神经网络,使机器能够从历史数据中自动学习知识和模式,而不需要人为指定规则。
特别是,深度学习可以针对大规模复杂数据集进行自动特征提取,其中包括图像、语音和文本。
深度学习已被广泛应用于语音识别、自然语言处理、图像分类和机器翻译等任务上,并取得了显著的成功。
1. 深度神经网络(Deep Neural Networks):深度神经网络通过串联多个原子神经网络,从而形成多层、多维的神经网络。
它通过学习多层次的特征表示,使用多层结构来表示复杂的数据模式。
最常见的深度神经网络模型有多层感知器(MLP)、卷积神经网络(CNN)、循环神经网络(RNN)和递归神经网络(Recurrent Neural Networks)。
2. 卷积神经网络(Convolutional Neural Networks):卷积神经网络(CNN)是一种特殊的深度神经网络,它利用卷积核的内积运算来提取图像的特征,从而更好的把握图像的空间特征,并为更高层次的操作和理解提供更强大的计算能力。
掌握机器学习中的集成学习和深度强化学习算法
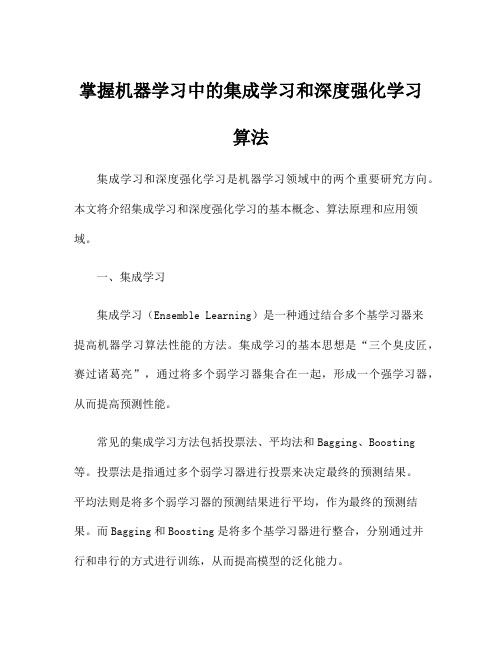
掌握机器学习中的集成学习和深度强化学习算法集成学习和深度强化学习是机器学习领域中的两个重要研究方向。
本文将介绍集成学习和深度强化学习的基本概念、算法原理和应用领域。
一、集成学习集成学习(Ensemble Learning)是一种通过结合多个基学习器来提高机器学习算法性能的方法。
集成学习的基本思想是“三个臭皮匠,赛过诸葛亮”,通过将多个弱学习器集合在一起,形成一个强学习器,从而提高预测性能。
常见的集成学习方法包括投票法、平均法和Bagging、Boosting 等。
投票法是指通过多个弱学习器进行投票来决定最终的预测结果。
平均法则是将多个弱学习器的预测结果进行平均,作为最终的预测结果。
而Bagging和Boosting是将多个基学习器进行整合,分别通过并行和串行的方式进行训练,从而提高模型的泛化能力。
集成学习的应用非常广泛,其中最著名的应用之一是随机森林(Random Forest)。
随机森林是一种基于决策树的集成学习算法,通过多个决策树的投票或平均来进行分类或回归任务。
随机森林具有较强的鲁棒性和泛化能力,在各种实际应用中取得了良好的效果。
二、深度强化学习深度强化学习(Deep Reinforcement Learning)是结合深度学习和强化学习的一种方法。
强化学习是一种通过智能体在环境中执行动作并得到奖励信号,以达到最大化累积奖励的学习方法。
深度学习则是一种模仿人脑神经网络的学习方法,利用多层神经网络对输入特征进行高层抽象和表示学习。
深度强化学习的核心是使用深度神经网络来近似值函数或者策略函数。
一种经典的深度强化学习算法是深度Q网络(Deep Q-Network,DQN)。
DQN通过深度神经网络来逼近动作值函数(Q函数),从而实现智能体在环境中选取最优动作。
DQN具有较强的逼近能力和泛化能力,在很多领域,特别是游戏领域取得了非常好的效果。
深度强化学习在很多领域都有着广泛的应用。
例如,在机器人领域,深度强化学习可以用于实现机器人的自主导航和控制;在自然语言处理和机器翻译领域,深度强化学习可以用于语言模型的训练和优化;在金融领域,深度强化学习可以通过学习交易模式来进行股票交易。
基于深度学习的特征提取方法(八)
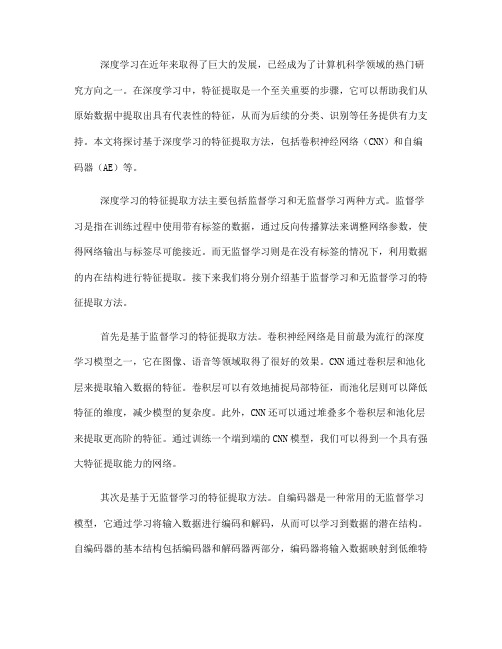
深度学习在近年来取得了巨大的发展,已经成为了计算机科学领域的热门研究方向之一。
在深度学习中,特征提取是一个至关重要的步骤,它可以帮助我们从原始数据中提取出具有代表性的特征,从而为后续的分类、识别等任务提供有力支持。
本文将探讨基于深度学习的特征提取方法,包括卷积神经网络(CNN)和自编码器(AE)等。
深度学习的特征提取方法主要包括监督学习和无监督学习两种方式。
监督学习是指在训练过程中使用带有标签的数据,通过反向传播算法来调整网络参数,使得网络输出与标签尽可能接近。
而无监督学习则是在没有标签的情况下,利用数据的内在结构进行特征提取。
接下来我们将分别介绍基于监督学习和无监督学习的特征提取方法。
首先是基于监督学习的特征提取方法。
卷积神经网络是目前最为流行的深度学习模型之一,它在图像、语音等领域取得了很好的效果。
CNN通过卷积层和池化层来提取输入数据的特征。
卷积层可以有效地捕捉局部特征,而池化层则可以降低特征的维度,减少模型的复杂度。
此外,CNN还可以通过堆叠多个卷积层和池化层来提取更高阶的特征。
通过训练一个端到端的CNN模型,我们可以得到一个具有强大特征提取能力的网络。
其次是基于无监督学习的特征提取方法。
自编码器是一种常用的无监督学习模型,它通过学习将输入数据进行编码和解码,从而可以学习到数据的潜在结构。
自编码器的基本结构包括编码器和解码器两部分,编码器将输入数据映射到低维特征空间,而解码器则将低维特征空间的表示映射回原始输入空间。
通过训练自编码器模型,我们可以得到一个具有良好特征提取能力的编码器网络。
除了上述的方法之外,还有一些其他的特征提取方法,如基于稀疏编码的特征提取、基于降维技术的特征提取等。
这些方法在不同的应用场景下都有着广泛的应用。
总的来说,基于深度学习的特征提取方法在实际应用中取得了很好的效果。
通过这些方法,我们可以从原始数据中提取出具有代表性的特征,为后续的任务提供有力支持。
未来,随着深度学习技术的不断发展,相信会有更多更好的特征提取方法被提出,从而推动深度学习在各个领域的发展。
深度学习常用名词解析

深度学习常用名词解析深度学习:英文DL(Deep Learning),指多层的人工神经网络和训练它的方法。
一层大量的神经网络会把大量的矩阵数字作为输入,通过非线性激活方法获取权重,再产生另一个数据集和作为输出。
Epoch:在模型训练的时候含义是训练集中的所有样本训练完一次。
这里的一指的是:当一个完整的数据集通过了神经网络一次并且返回了一次。
如果Epoch过少会欠拟合,反之Epoch过多会过拟合.1个epoch=iteration数×batchsize数举个例子:训练集有1000个样本,batchsize=10。
训练完整个样本集需要:100次iteration,1次epoch。
为什么要使用多个epoch?当一个 epoch 对于计算机而言太庞大的时候,就需要把它分成多个小块。
在神经网络中传递完整的数据集一次是不够的,而且我们需要将完整的数据集在同样的神经网络中传递多次。
但是请记住,我们使用的是有限的数据集,并且我们使用一个迭代过程即梯度下降,优化学习过程和图示。
因此仅仅更新权重一次或者说使用一个 epoch 是不够的。
随着 epoch 数量增加,神经网络中的权重的更新次数也增加,曲线从欠拟合变得过拟合。
Iteration:翻译为迭代。
迭代是重复反馈的动作,神经网络中我们希望通过迭代,进行多次的训练以达到所需的目标或结果。
1个iteration=1个正向通过+1个反向通过=使用batchsize个样本训练一次。
每次迭代的结果将作为下一次迭代的初始值。
Batchsize:转化为批量大小。
简单来说,批量大小将决定我们每次训练的样本数量(参数变化受机器性能限制,电脑配置差的话不能设置太大)。
记住公式:1个iteration=使用batchsize个样本训练一次。
在深度学习中,一般采用SGD训练(随机梯度下降),即每次训练在训练集中取batchsize个样本训练;(1)经验总结:Batch_Size的正确选择是为了在内存效率和内存容量之间寻找最佳平衡相对于正常数据集,如果Batch_Size过小,训练数据就会非常难收敛,从而导underfitting。
Python中的深度学习和长短时记忆神经网络

Python中的深度学习和长短时记忆神经网络深度学习是指一种模拟人类神经网络的学习方式,它可以处理大规模数据和复杂模型,以便实现高精度的预测和分类。
深度学习的应用广泛,其中最为流行的就是图像识别、语音识别和自然语言处理等领域。
而在深度学习算法中,长短时记忆神经网络(LSTM)是一个重要的结构,广泛应用于序列的建模和预测。
LSTM是一种特殊的循环神经网络,它能够捕捉到序列数据中的长期依赖性,以及背后的规律和结构。
因此,LSTM已经被广泛地应用于自然语言处理、机器翻译、语音识别、图像处理等领域。
深度学习算法的基本构成如下:输入层、隐藏层和输出层。
其中,每一层都包含若干个神经元,每个神经元接收并计算所有输入信号,将结果传递给下一层的神经元。
该过程可以迭代多次,以便不断调整网络参数,改进模型的性能。
在深度学习中,隐藏层的数量越多,可以发现的规律也就越复杂。
长短时记忆神经网络(LSTM)构建在深度学习的基础之上,它可以处理包含大量时序特征的多维数据。
LSTM网络主要包括四个关键的模块:输入门、输出门、遗忘门和记忆细胞。
输入门负责控制信息的流入,输出门负责控制信息的流出,遗忘门用于控制记忆单元中数据的保留和遗忘过程,记忆细胞则用于存储和更新重要的信息。
以自然语言处理为例,我们可以用LSTM建立一个模型,以便预测下一个可能的单词。
该模型的输入为一句话中的前N个单词,每个单词会被转换为一个固定长度的向量,这些向量将被送入双向LSTM网络中进行处理。
在这个过程中,LSTM会学习到语句的语法、意思和上下文,提高模型的准确性。
在深度学习中,LSTM与其他深度学习的应用相比,拥有更好的表现和可扩展性。
这是因为LSTM具有适应动态时间序列数据集的能力,以及强大的建模和推理能力。
此外,LSTM还具有更强的容错能力,可快速从错误中恢复,保持模型的稳定性。
总而言之,深度学习和长短时记忆神经网络是目前最流行并且最有效的机器学习方法之一。
深度学习中的正则化方法
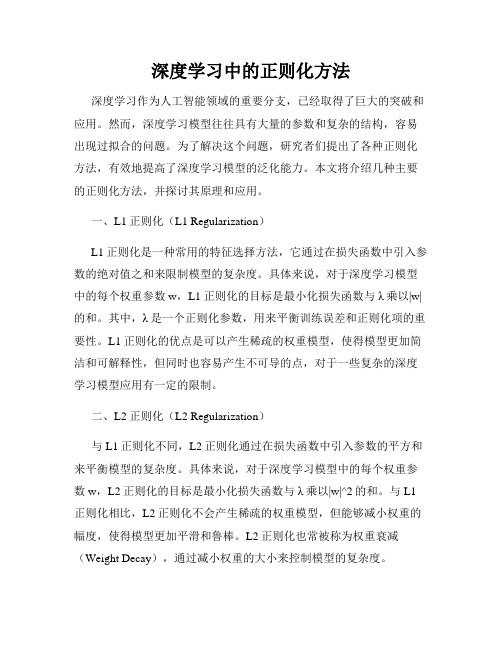
深度学习中的正则化方法深度学习作为人工智能领域的重要分支,已经取得了巨大的突破和应用。
然而,深度学习模型往往具有大量的参数和复杂的结构,容易出现过拟合的问题。
为了解决这个问题,研究者们提出了各种正则化方法,有效地提高了深度学习模型的泛化能力。
本文将介绍几种主要的正则化方法,并探讨其原理和应用。
一、L1正则化(L1 Regularization)L1正则化是一种常用的特征选择方法,它通过在损失函数中引入参数的绝对值之和来限制模型的复杂度。
具体来说,对于深度学习模型中的每个权重参数w,L1正则化的目标是最小化损失函数与λ乘以|w|的和。
其中,λ是一个正则化参数,用来平衡训练误差和正则化项的重要性。
L1正则化的优点是可以产生稀疏的权重模型,使得模型更加简洁和可解释性,但同时也容易产生不可导的点,对于一些复杂的深度学习模型应用有一定的限制。
二、L2正则化(L2 Regularization)与L1正则化不同,L2正则化通过在损失函数中引入参数的平方和来平衡模型的复杂度。
具体来说,对于深度学习模型中的每个权重参数w,L2正则化的目标是最小化损失函数与λ乘以|w|^2的和。
与L1正则化相比,L2正则化不会产生稀疏的权重模型,但能够减小权重的幅度,使得模型更加平滑和鲁棒。
L2正则化也常被称为权重衰减(Weight Decay),通过减小权重的大小来控制模型的复杂度。
三、Dropout正则化Dropout正则化是一种广泛应用于深度学习模型的正则化方法,通过在训练过程中随机将部分神经元的输出置为0来减小模型的复杂度。
具体来说,每个神经元的输出被设置为0的概率为p,而被保留的概率为1-p。
这样做的好处是能够迫使网络学习到多个不同的子网络,从而提高模型的泛化能力。
在测试模型时,通常会将所有神经元的输出乘以p来保持一致性。
四、Batch NormalizationBatch Normalization是一种通过对每一层的输入进行归一化处理来加速训练和提高模型的泛化能力的方法。
基于深度学习的特征提取方法(五)
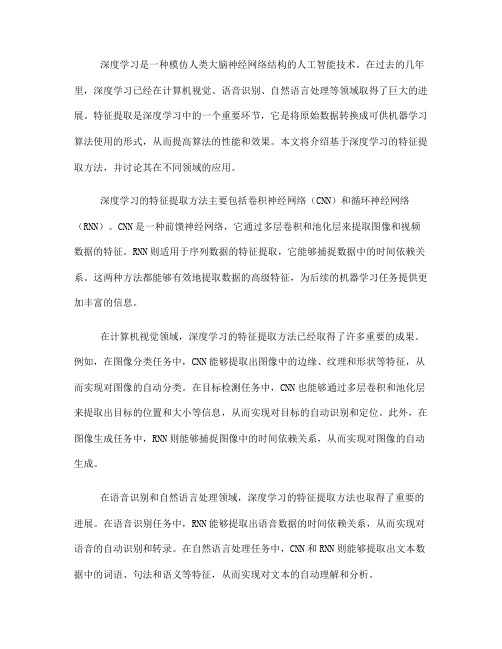
深度学习是一种模仿人类大脑神经网络结构的人工智能技术。
在过去的几年里,深度学习已经在计算机视觉、语音识别、自然语言处理等领域取得了巨大的进展。
特征提取是深度学习中的一个重要环节,它是将原始数据转换成可供机器学习算法使用的形式,从而提高算法的性能和效果。
本文将介绍基于深度学习的特征提取方法,并讨论其在不同领域的应用。
深度学习的特征提取方法主要包括卷积神经网络(CNN)和循环神经网络(RNN)。
CNN是一种前馈神经网络,它通过多层卷积和池化层来提取图像和视频数据的特征。
RNN则适用于序列数据的特征提取,它能够捕捉数据中的时间依赖关系。
这两种方法都能够有效地提取数据的高级特征,为后续的机器学习任务提供更加丰富的信息。
在计算机视觉领域,深度学习的特征提取方法已经取得了许多重要的成果。
例如,在图像分类任务中,CNN能够提取出图像中的边缘、纹理和形状等特征,从而实现对图像的自动分类。
在目标检测任务中,CNN也能够通过多层卷积和池化层来提取出目标的位置和大小等信息,从而实现对目标的自动识别和定位。
此外,在图像生成任务中,RNN则能够捕捉图像中的时间依赖关系,从而实现对图像的自动生成。
在语音识别和自然语言处理领域,深度学习的特征提取方法也取得了重要的进展。
在语音识别任务中,RNN能够提取出语音数据的时间依赖关系,从而实现对语音的自动识别和转录。
在自然语言处理任务中,CNN和RNN则能够提取出文本数据中的词语、句法和语义等特征,从而实现对文本的自动理解和分析。
除了传统的深度学习方法,还有一些新的特征提取方法也值得关注。
例如,生成对抗网络(GAN)能够通过两个神经网络的对抗训练来提取数据的高级特征,从而实现对数据的自动生成和增强。
另外,自动编码器(Autoencoder)也能够通过无监督学习来提取数据的高级特征,从而实现对数据的自动降维和重构。
总之,基于深度学习的特征提取方法在计算机视觉、语音识别、自然语言处理等领域都取得了重要的进展。
自然语言处理中的decoding的常见方法的原理及优缺点

自然语言处理中的decoding的常见方法的原理及优缺点自然语言处理(NaturalLanguageProcessing,NLP)是计算机科学与语言学的交叉学科,它研究计算机如何处理和理解自然语言。
在NLP中,decoding是一个非常重要的环节,它的作用是根据上下文信息,将一段文本转换成另一种形式,比如翻译、摘要等。
本文将介绍在NLP中decoding的常见方法及其原理及优缺点。
1. 贪心算法(Greedy Algorithm)贪心算法是一种简单的解码方法,它的核心思想是每一步都选择最优的解,然后不断地累加到最终结果。
在NLP中,贪心算法常用于序列标注和机器翻译等任务中。
例如,在机器翻译中,贪心算法会根据当前输入的单词,选择概率最高的译文作为输出结果。
由于贪心算法只考虑当前步骤的最优解,因此其效率较高,但是其无法保证全局最优解,容易陷入局部最优解。
2. 束搜索(Beam Search)束搜索是一种基于贪心算法的优化方法。
它在解码过程中,保留多个备选解,称之为“束”。
每次选择最可能的k个备选解作为下一步的备选解,并在这些备选解中选择最优的解作为当前的输出结果。
束搜索的优点是可以保证在一定程度上找到全局最优解,但是k值的大小会影响搜索效率和解码质量。
3. 生成式模型(Generation Model)生成式模型是利用概率模型生成目标序列的方法。
在NLP中,生成式模型常用于语言生成和机器翻译等任务中。
例如,在机器翻译中,生成式模型会利用译文单词的概率模型,生成最优的输出结果。
由于生成式模型考虑了全局的概率信息,因此其能够得到更加准确的结果。
但是,生成式模型的缺点是计算复杂度较高,且难以处理长文本的概率。
4. 判别式模型(Discriminative Model)判别式模型是基于给定特征条件下,预测目标序列的方法。
在NLP中,判别式模型常用于序列标注和情感分析等任务中。
例如,在情感分析中,判别式模型会根据文本的特征,预测文本的情感极性。
Greedy layer-wise training of deep networks
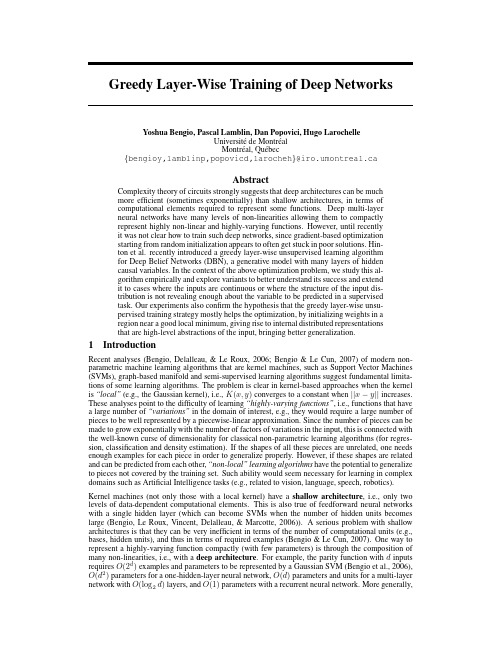
Yoshua Bengio,Pascal Lamblin,Dan Popovici,Hugo LarochelleUniversit´e de Montr´e alMontr´e al,Qu´e bec{bengioy,lamblinp,popovicd,larocheh}@iro.umontreal.caAbstractComplexity theory of circuits strongly suggests that deep architectures can be muchmore efficient(sometimes exponentially)than shallow architectures,in terms ofcomputational elements required to represent some functions.Deep multi-layerneural networks have many levels of non-linearities allowing them to compactlyrepresent highly non-linear and highly-varying functions.However,until recentlyit was not clear how to train such deep networks,since gradient-based optimizationstarting from random initialization appears to often get stuck in poor solutions.Hin-ton et al.recently introduced a greedy layer-wise unsupervised learning algorithmfor Deep Belief Networks(DBN),a generative model with many layers of hiddencausal variables.In the context of the above optimization problem,we study this al-gorithm empirically and explore variants to better understand its success and extendit to cases where the inputs are continuous or where the structure of the input dis-tribution is not revealing enough about the variable to be predicted in a supervisedtask.Our experiments also confirm the hypothesis that the greedy layer-wise unsu-pervised training strategy mostly helps the optimization,by initializing weights in aregion near a good local minimum,giving rise to internal distributed representationsthat are high-level abstractions of the input,bringing better generalization.1IntroductionRecent analyses(Bengio,Delalleau,&Le Roux,2006;Bengio&Le Cun,2007)of modern non-parametric machine learning algorithms that are kernel machines,such as Support Vector Machines (SVMs),graph-based manifold and semi-supervised learning algorithms suggest fundamental limita-tions of some learning algorithms.The problem is clear in kernel-based approaches when the kernel is“local”(e.g.,the Gaussian kernel),i.e.,K(x,y)converges to a constant when||x−y||increases. These analyses point to the difficulty of learning“highly-varying functions”,i.e.,functions that have a large number of“variations”in the domain of interest,e.g.,they would require a large number of pieces to be well represented by a piecewise-linear approximation.Since the number of pieces can be made to grow exponentially with the number of factors of variations in the input,this is connected with the well-known curse of dimensionality for classical non-parametric learning algorithms(for regres-sion,classification and density estimation).If the shapes of all these pieces are unrelated,one needs enough examples for each piece in order to generalize properly.However,if these shapes are related and can be predicted from each other,“non-local”learning algorithms have the potential to generalize to pieces not covered by the training set.Such ability would seem necessary for learning in complex domains such as Artificial Intelligence tasks(e.g.,related to vision,language,speech,robotics). Kernel machines(not only those with a local kernel)have a shallow architecture,i.e.,only two levels of data-dependent computational elements.This is also true of feedforward neural networks with a single hidden layer(which can become SVMs when the number of hidden units becomes large(Bengio,Le Roux,Vincent,Delalleau,&Marcotte,2006)).A serious problem with shallow architectures is that they can be very inefficient in terms of the number of computational units(e.g., bases,hidden units),and thus in terms of required examples(Bengio&Le Cun,2007).One way to represent a highly-varying function compactly(with few parameters)is through the composition of many non-linearities,i.e.,with a deep architecture.For example,the parity function with d inputs requires O(2d)examples and parameters to be represented by a Gaussian SVM(Bengio et al.,2006), O(d2)parameters for a one-hidden-layer neural network,O(d)parameters and units for a multi-layer network with O(logd)layers,and O(1)parameters with a recurrent neural network.More generally,2boolean functions(such as the function that computes the multiplication of two numbers from their d-bit representation)expressible by O(log d)layers of combinatorial logic with O(d)elements in each layer may require O(2d)elements when expressed with only2layers(Utgoff&Stracuzzi,2002; Bengio&Le Cun,2007).When the representation of a concept requires an exponential number of elements,e.g.,with a shallow circuit,the number of training examples required to learn the concept may also be impractical.Formal analyses of the computational complexity of shallow circuits can be found in(Hastad,1987)or(Allender,1996).They point in the same direction:shallow circuits are much less expressive than deep ones.However,until recently,it was believed too difficult to train deep multi-layer neural networks.Empiri-cally,deep networks were generally found to be not better,and often worse,than neural networks with one or two hidden layers(Tesauro,1992).As this is a negative result,it has not been much reported in the machine learning literature.A reasonable explanation is that gradient-based optimization starting from random initialization may get stuck near poor solutions.An approach that has been explored with some success in the past is based on constructively adding layers.This was previously done using a supervised criterion at each stage(Fahlman&Lebiere,1990;Lengell´e&Denoeux,1996).Hinton, Osindero,and Teh(2006)recently introduced a greedy layer-wise unsupervised learning algorithm for Deep Belief Networks(DBN),a generative model with many layers of hidden causal variables.The training strategy for such networks may hold great promise as a principle to help address the problem of training deep networks.Upper layers of a DBN are supposed to represent more“abstract”concepts that explain the input observation x,whereas lower layers extract“low-level features”from x.They learn simpler conceptsfirst,and build on them to learn more abstract concepts.This strategy,studied in detail here,has not yet been much exploited in machine learning.We hypothesize that three aspects of this strategy are particularly important:first,pre-training one layer at a time in a greedy way;sec-ond,using unsupervised learning at each layer in order to preserve information from the input;and finally,fine-tuning the whole network with respect to the ultimate criterion of interest.Wefirst extend DBNs and their component layers,Restricted Boltzmann Machines(RBM),so that they can more naturally handle continuous values in input.Second,we perform experiments to better understand the advantage brought by the greedy layer-wise unsupervised learning.The basic question to answer is whether or not this approach helps to solve a difficult optimization problem.In DBNs, RBMs are used as building blocks,but applying this same strategy using auto-encoders yielded similar results.Finally,we discuss a problem that occurs with the layer-wise greedy unsupervised procedure when the input distribution is not revealing enough of the conditional distribution of the target variable given the input variable.We evaluate a simple and successful solution to this problem.2Deep Belief NetsLet x be the input,and g i the hidden variables at layer i,with joint distributionP(x,g1,g2,...,g )=P(x|g1)P(g1|g2)···P(g −2|g −1)P(g −1,g ),where all the conditional layers P(g i|g i+1)are factorized conditional distributions for which compu-tation of probability and sampling are easy.In Hinton et al.(2006)one considers the hidden layer g i a binary random vector with n i elements g ij:P(g i|g i+1)=n ij=1P(g i j|g i+1)with P(g i j=1|g i+1)=sigm(b i j+n i+1 k=1W i kj g i+1k)(1)where sigm(t)=1/(1+e−t),the b ij are biases for unit j of layer i,and W i is the weight matrix forlayer i.If we denote g0=x,the generative model for thefirst layer P(x|g1)also follows(1).2.1Restricted Boltzmann machinesThe top-level prior P(g −1,g )is a Restricted Boltzmann Machine(RBM)between layer −1 and layer .To lighten notation,consider a generic RBM with input layer activations v(for visi-ble units)and hidden layer activations h(for hidden units).It has the following joint distribution: P(v,h)=1The layer-to-layer conditionals associated with the RBM factorize like in(1)and give rise to P(v k=1|h)=sigm(b k+ j W jk h j)and Q(h j=1|v)=sigm(c j+ k W jk v k).2.2Gibbs Markov chain and log-likelihood gradient in an RBMTo obtain an estimator of the gradient on the log-likelihood of an RBM,we consider a Gibbs Markov chain on the(visible units,hidden units)pair of variables.Gibbs sampling from an RBM proceeds by sampling h given v,then v given h,etc.Denote v t for the t-th v sample from that chain,starting at t=0with v0,the“input observation”for the RBM.Therefore,(v k,h k)for k→∞is a sample from the joint P(v,h).The log-likelihood of a value v0under the model of the RBM islog P(v0)=log h P(v0,h)=log h e−energy(v0,h)−log v,h e−energy(v,h)and its gradient with respect toθ=(W,b,c)is∂log P(v0)∂θ+ v k,h k P(v k,h k)∂energy(v k,h k)∂θ+E hk ∂energy(v k,h k)(fitting p )will yield improvement on the training criterion for the previous layer (likelihood with respect to p −1).The greedy layer-wise training algorithm for DBNs is quite simple,as illustrated by the pseudo-code in Algorithm TrainUnsupervisedDBN of the Appendix.2.4Supervised fine-tuningAs a last training stage,it is possible to fine-tune the parameters of all the layers together.For exam-ple Hinton et al.(2006)propose to use the wake-sleep algorithm (Hinton,Dayan,Frey,&Neal,1995)to continue unsupervised training.Hinton et al.(2006)also propose to optionally use a mean-field ap-proximation of the posteriors P (g i |g 0),by replacing the samples g i −1j at level i −1by their bit-wisemean-field expected value µi −1j ,with µi =sigm(b i +W i µi −1).According to these propagation rules,the whole network now deterministically computes internal representations as functions of the network input g 0=x .After unsupervised pre-training of the layers of a DBN following Algorithm TrainUnsupervisedDBN (see Appendix)the whole network can be further optimized by gradient descent with respect to any deterministically computable training criterion that depends on these rep-resentations.For example,this can be used (Hinton &Salakhutdinov,2006)to fine-tune a very deep auto-encoder,minimizing a reconstruction error.It is also possible to use this as initialization of all except the last layer of a traditional multi-layer neural network,using gradient descent to fine-tune the whole network with respect to a supervised training criterion.Algorithm DBNSupervisedFineTuning in the appendix contains pseudo-code for supervised fine-tuning,as part of the global supervised learning algorithm TrainSupervisedDBN .Note that better results were obtained when using a 20-fold larger learning rate with the supervised criterion (here,squared error or cross-entropy)updates than in the contrastive divergence updates.3Extension to continuous-valued inputsWith the binary units introduced for RBMs and DBNs in Hinton et al.(2006)one can “cheat”and handle continuous-valued inputs by scaling them to the (0,1)interval and considering each input con-tinuous value as the probability for a binary random variable to take the value 1.This has worked well for pixel gray levels,but it may be inappropriate for other kinds of input variables.Previous work on continuous-valued input in RBMs include (Chen &Murray,2003),in which noise is added to sigmoidal units,and the RBM forms a special form of Diffusion Network (Movellan,Mineiro,&Williams,2002).We concentrate here on simple extensions of the RBM framework in which only the energy function and the allowed range of values are changed.Linear energy:exponential or truncated exponentialConsider a unit with value y of an RBM,connected to units z of the other layer.p (y |z )can be obtained from the terms in the exponential that contain y ,which can be grouped in ya (z )for linear energy functions as in (2),where a (z )=b +w z with b the bias of unit y ,and w the vector of weights connecting unit y to units z .If we allow y to take any value in interval I ,the conditional densityof y becomes p (y |z )=exp (ya (z ))1y ∈Ia (z ).The conditional expectation of u given z is interesting becauseit has a sigmoidal-like saturating and monotone non-linearity:E [y |z ]=1a (z ).A sampling from the truncated exponential is easily obtained from a uniform sample U ,using the inverse cumulative F −1of the conditional density y |z :F −1(U )=log(1−U ×(1−exp (a (z ))))c l a s s i f i c a t i o n e r r o r o n t r a i n i n g s e tFigure 1:Training classification error vs training iteration,on the Cotton price task,for deep net-work without pre-training,for DBN with unsuper-vised pre-training,and DBN with partially super-vised pre-training.Illustrates optimization diffi-culty of deep networks and advantage of partially supervised training.AbaloneCotton train.valid.test.train.valid.test.2.Logistic regression···44.0%42.6%45.0%4.DBN,binomial inputs,partially supervised 4.39 4.45 4.2843.3%41.1%43.7%6.DBN,Gaussian inputs,partially supervised4.234.434.1827.5%28.4%31.4%Table 1:Mean squared prediction error on Abalone task and classification error on Cotton task,showing improvement with Gaussian units.this case the variance is unconditional,whereas the mean depends on the inputs of the unit:for a unit y with inputs z and inverse variance d 2,E [y |z ]=a (z )Training each layer as an auto-encoderWe want to verify that the layer-wise greedy unsupervised pre-training principle can be applied when using an auto-encoder instead of the RBM as a layer building block.Let x be the input vector with x i∈(0,1).For a layer with weights matrix W,hidden biases column vector b and input biases column vector c,the reconstruction probability for bit i is p i(x),with the vector of proba-bilities p(x)=sigm(c+W sigm(b+W x)).The training criterion for the layer is the average of negative log-likelihoods for predicting x from p(x).For example,if x is interpreted either as a sequence of bits or a sequence of bit probabilities,we minimize the reconstruction cross-entropy: R=− i x i log p i(x)+(1−x i)log(1−p i(x)).We report several experimental results using this training criterion for each layer,in comparison to the contrastive divergence algorithm for an RBM. Pseudo-code for a deep network obtained by training each layer as an auto-encoder is given in Ap-pendix(Algorithm TrainGreedyAutoEncodingDeepNet).One question that arises with auto-encoders in comparison with RBMs is whether the auto-encoders will fail to learn a useful representation when the number of units is not strictly decreasing from one layer to the next(since the networks could theoretically just learn to be the identity and perfectly min-imize the reconstruction error).However,our experiments suggest that networks with non-decreasing layer sizes generalize well.This might be due to weight decay and stochastic gradient descent,prevent-ing large weights:optimization falls in a local minimum which corresponds to a good transformation of the input(that provides a good initialization for supervised training of the whole net). Greedy layer-wise supervised trainingA reasonable question to ask is whether the fact that each layer is trained in an unsupervised way is critical or not.An alternative algorithm is supervised,greedy and layer-wise:train each new hidden layer as the hidden layer of a one-hidden layer supervised neural network NN(taking as input the output of the last of previously trained layers),and then throw away the output layer of NN and use the parameters of the hidden layer of NN as pre-training initialization of the new top layer of the deep net, to map the output of the previous layers to a hopefully better representation.Pseudo-code for a deep network obtained by training each layer as the hidden layer of a supervised one-hidden-layer neural network is given in Appendix(Algorithm TrainGreedySupervisedDeepNet). Experiment2.We compared the performance on the MNIST digit classification task obtained withfive algorithms: (a)DBN,(b)deep network whose layers are initialized as auto-encoders,(c)above described su-pervised greedy layer-wise algorithm to pre-train each layer,(d)deep network with no pre-training (random initialization),(e)shallow network(1hidden layer)with no pre-training.Thefinalfine-tuning is done by adding a logistic regression layer on top of the network and train-ing the whole network by stochastic gradient descent on the cross-entropy with respect to the target classification.The networks have the following architecture:784inputs,10outputs,3hidden layers with variable number of hidden units,selected by validation set performance(typically selected layer sizes are between500and1000).The shallow network has a single hidden layer.An L2weight decay hyper-parameter is also optimized.The DBN was slower to train and less experiments were performed,so that longer training and more appropriately chosen sizes of layers and learning rates could yield better results(Hinton2006,unpublished,reports1.15%error on the MNIST test set).Experiment2Experiment3train.valid.test train.valid.test DBN,unsupervised pre-training0% 1.2% 1.2%0% 1.5% 1.5%Deep net,auto-associator pre-training0% 1.4% 1.4%0% 1.4% 1.6%Deep net,supervised pre-training0% 1.7% 2.0%0% 1.8% 1.9%Deep net,no pre-training.004% 2.1% 2.4%.59% 2.1% 2.2%Shallow net,no pre-training.004% 1.8% 1.9% 3.6% 4.7% 5.0%pre-training)or a shallow network,and that,without pre-training,deep networks tend to perform worse than shallow networks.The results also suggest that unsupervised greedy layer-wise pre-training can perform significantly better than purely supervised greedy layer-wise pre-training.A possible expla-nation is that the greedy supervised procedure is too greedy:in the learned hidden units representation it may discard some of the information about the target,information that cannot be captured easily by a one-hidden-layer neural network but could be captured by composing more hidden layers. Experiment3However,there is something troubling in the Experiment2results(Table2):all the networks,even those without greedy layer-wise pre-training,perform almost perfectly on the training set,which would appear to contradict the hypothesis that the main effect of the layer-wise greedy strategy is to help the optimization(with poor optimization one would expect poor training error).A possible explanation coherent with our initial hypothesis and with the above results is captured by the following hypothesis.Without pre-training,the lower layers are initialized poorly,but still allowing the top two layers to learn the training set almost perfectly,because the output layer and the last hidden layer form a standard shallow but fat neural network.Consider the top two layers of the deep network with pre-training:it presumably takes as input a better representation,one that allows for better generalization.Instead,the network without pre-training sees a“random”transformation of the input, one that preserves enough information about the input tofit the training set,but that does not help to generalize.To test that hypothesis,we performed a second series of experiments in which we constrain the top hidden layer to be small(20hidden units).The Experiment3results(Table2)clearly confirm our hypothesis.With no pre-training,training error degrades significantly when there are only20 hidden units in the top hidden layer.In addition,the results obtained without pre-training were found to have extremely large variance indicating high sensitivity to initial conditions.Overall,the results in the tables and in Figure1are consistent with the hypothesis that the greedy layer-wise procedure essentially helps to better optimize the deep networks,probably by initializing the hidden layers so that they represent more meaningful representations of the input,which also yields to better generalization. Continuous training of all layers of a DBNWith the layer-wise training algorithm for DBNs(TrainUnsupervisedDBN in Appendix),one element that we would like to dispense with is having to decide the number of training iterations for each layer.It would be good if we did not have to explicitly add layers one at a time,i.e.,if we could train all layers simultaneously,but keeping the“greedy”idea that each layer is pre-trained to model its input,ignoring the effect of higher layers.To achieve this it is sufficient to insert a line in TrainUnsupervisedDBN,so that RBMupdate is called on all the layers and the stochastic hidden values are propagated all the way up.Experiments with this variant demonstrated that it works at least as well as the original algorithm.The advantage is that we can now have a single stopping criterion(for the whole network).Computation time is slightly greater,since we do more computations initially(on the upper layers),which might be wasted(before the lower layers converge to a decent representation),but time is saved on optimizing hyper-parameters.This variant may be more appealing for on-line training on very large data-sets,where one would never cycle back on the training data. 5Dealing with uncooperative input distributionsIn classification problems such as MNIST where classes are well separated,the structure of the input distribution p(x)naturally contains much information about the target variable y.Imagine a super-vised learning task in which the input distribution is mostly unrelated with y.In regression problems, which we are interested in studying here,this problem could be much more prevalent.For example imagine a task in which x∼p(x)and the target y=f(x)+noise(e.g.,p is Gaussian and f=sinus) with no particular relation between p and f.In such settings we cannot expect the unsupervised greedy layer-wise pre-training procedure to help in training deep supervised networks.To deal with such uncooperative input distributions,we propose to train each layer with a mixed training criterion that combines the unsupervised objective(modeling or reconstructing the input)and a supervised ob-jective(helping to predict the target).A simple algorithm thus adds the updates on the hidden layer weights from the unsupervised algorithm(Contrastive Divergence or reconstruction error gradient) with the updates from the gradient on a supervised prediction error,using a temporary output layer,as with the greedy layer-wise supervised training algorithm.In our experiments it appeared sufficient to perform that partial supervision with thefirst layer only,since once the predictive information about the target is“forced”into the representation of thefirst layer,it tends to stay in the upper layers.The results in Figure1and Table1clearly show the advantage of this partially supervised greedy trainingalgorithm,in the case of thefinancial dataset.Pseudo-code for partially supervising thefirst(or later layer)is given in Algorithm TrainPartiallySupervisedLayer(in the Appendix).6ConclusionThis paper is motivated by the need to develop good training algorithms for deep architectures,since these can be much more representationally efficient than shallow ones such as SVMs and one-hidden-layer neural nets.We study Deep Belief Networks applied to supervised learning tasks,and the prin-ciples that could explain the good performance they have yielded.The three principal contributions of this paper are the following.First we extended RBMs and DBNs in new ways to naturally handle continuous-valued inputs,showing examples where much better predictive models can thus be ob-tained.Second,we performed experiments which support the hypothesis that the greedy unsupervised layer-wise training strategy helps to optimize deep networks,but suggest that better generalization is also obtained because this strategy initializes upper layers with better representations of relevant high-level abstractions.These experiments suggest a general principle that can be applied beyond DBNs, and we obtained similar results when each layer is initialized as an auto-associator instead of as an RBM.Finally,although we found that it is important to have an unsupervised component to train each layer(a fully supervised greedy layer-wise strategy performed worse),we studied supervised tasks in which the structure of the input distribution is not revealing enough of the conditional density of y given x.In that case the DBN unsupervised greedy layer-wise strategy appears inadequate and we proposed a simplefix based on partial supervision,that can yield significant improvements. ReferencesAllender,E.(1996).Circuit complexity before the dawn of the new millennium.In16th Annual Conference on Foundations of Software Technology and Theoretical Computer Science,pp.1–18.Lecture Notes in Computer Science1180.Bengio,Y.,Delalleau,O.,&Le Roux,N.(2006).The curse of highly variable functions for local kernel machines.In Weiss,Y.,Sch¨o lkopf,B.,&Platt,J.(Eds.),Advances in Neural Information Processing Systems18,pp.107–114.MIT Press,Cambridge,MA.Bengio,Y.,&Le Cun,Y.(2007).Scaling learning algorithms towards AI.In Bottou,L.,Chapelle,O.,DeCoste,D.,&Weston,J.(Eds.),Large Scale Kernel Machines.MIT Press.Bengio,Y.,Le Roux,N.,Vincent,P.,Delalleau,O.,&Marcotte,P.(2006).Convex neural networks.In Weiss,Y.,Sch¨o lkopf,B.,&Platt,J.(Eds.),Advances in Neural Information Processing Systems18,pp.123–130.MIT Press,Cambridge,MA.Chen,H.,&Murray,A.(2003).A continuous restricted boltzmann machine with an implementable training algorithm.IEE Proceedings of Vision,Image and Signal Processing,150(3),153–158. Fahlman,S.,&Lebiere,C.(1990).The cascade-correlation learning architecture.In Touretzky,D.(Ed.), Advances in Neural Information Processing Systems2,pp.524–532Denver,CO.Morgan Kaufmann, San Mateo.Hastad,J.T.(1987).Computational Limitations for Small Depth Circuits.MIT Press,Cambridge,MA. Hinton,G.E.,Osindero,S.,&Teh,Y.(2006).A fast learning algorithm for deep belief nets.Neural Computa-tion,18,1527–1554.Hinton,G.(2002).Training products of experts by minimizing contrastive divergence.Neural Computation, 14(8),1771–1800.Hinton,G.,Dayan,P.,Frey,B.,&Neal,R.(1995).The wake-sleep algorithm for unsupervised neural networks.Science,268,1558–1161.Hinton,G.,&Salakhutdinov,R.(2006).Reducing the dimensionality of data with neural networks.Science, 313(5786),504–507.Lengell´e,R.,&Denoeux,T.(1996).Training MLPs layer by layer using an objective function for internal representations.Neural Networks,9,83–97.Movellan,J.,Mineiro,P.,&Williams,R.(2002).A monte-carlo EM approach for partially observable diffusion processes:theory and applications to neural networks.Neural Computation,14,1501–1544. Tesauro,G.(1992).Practical issues in temporal difference learning.Machine Learning,8,257–277. Utgoff,P.,&Stracuzzi,D.(2002).Many-layered learning.Neural Computation,14,2497–2539. Welling,M.,Rosen-Zvi,M.,&Hinton,G.E.(2005).Exponential family harmoniums with an application to information retrieval.In Advances in Neural Information Processing Systems,V ol.17Cambridge,MA.MIT Press.。
深度学习及其在图像识别和语音识别中的应用
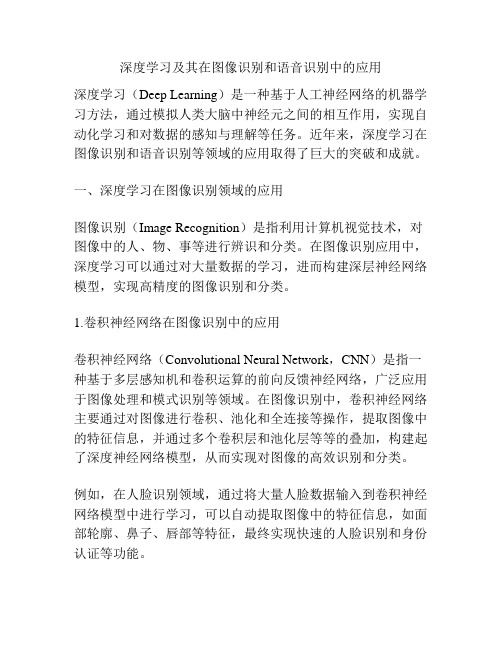
深度学习及其在图像识别和语音识别中的应用深度学习(Deep Learning)是一种基于人工神经网络的机器学习方法,通过模拟人类大脑中神经元之间的相互作用,实现自动化学习和对数据的感知与理解等任务。
近年来,深度学习在图像识别和语音识别等领域的应用取得了巨大的突破和成就。
一、深度学习在图像识别领域的应用图像识别(Image Recognition)是指利用计算机视觉技术,对图像中的人、物、事等进行辨识和分类。
在图像识别应用中,深度学习可以通过对大量数据的学习,进而构建深层神经网络模型,实现高精度的图像识别和分类。
1.卷积神经网络在图像识别中的应用卷积神经网络(Convolutional Neural Network,CNN)是指一种基于多层感知机和卷积运算的前向反馈神经网络,广泛应用于图像处理和模式识别等领域。
在图像识别中,卷积神经网络主要通过对图像进行卷积、池化和全连接等操作,提取图像中的特征信息,并通过多个卷积层和池化层等等的叠加,构建起了深度神经网络模型,从而实现对图像的高效识别和分类。
例如,在人脸识别领域,通过将大量人脸数据输入到卷积神经网络模型中进行学习,可以自动提取图像中的特征信息,如面部轮廓、鼻子、唇部等特征,最终实现快速的人脸识别和身份认证等功能。
2.循环神经网络在图像描述中的应用循环神经网络(Recurrent Neural Network,RNN)是一种能够对不定长序列数据进行建模和学习的神经网络模型。
在图像识别领域中,循环神经网络主要应用于图片描述的生成,通过对输入的图片进行特征提取和语义分析,并结合语言模型来生成准确、自然的图片描述。
例如,在一张照片中,就可以包含许多细节和内容,而人类在面对这样的图片时通常能够快速准确地描述应用到图像描述生成,通过对大量带有图片标签的数据进行学习,循环神经网络可以根据图片特征和上下文信息,自动生成准确、生动的图片描述。
二、深度学习在语音识别领域的应用语音识别是指识别并转写语音信号中所包含的语音内容,是一种基于人工智能技术和模式识别技术的应用。
deeplinik方法论
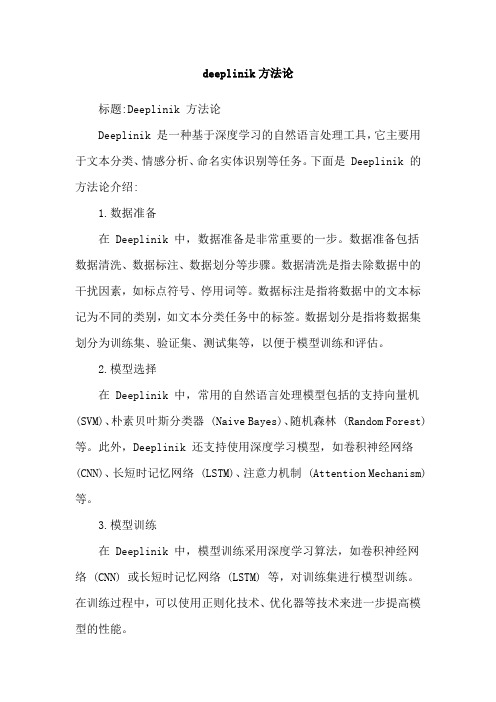
deeplinik方法论标题:Deeplinik 方法论Deeplinik 是一种基于深度学习的自然语言处理工具,它主要用于文本分类、情感分析、命名实体识别等任务。
下面是 Deeplinik 的方法论介绍:1.数据准备在 Deeplinik 中,数据准备是非常重要的一步。
数据准备包括数据清洗、数据标注、数据划分等步骤。
数据清洗是指去除数据中的干扰因素,如标点符号、停用词等。
数据标注是指将数据中的文本标记为不同的类别,如文本分类任务中的标签。
数据划分是指将数据集划分为训练集、验证集、测试集等,以便于模型训练和评估。
2.模型选择在 Deeplinik 中,常用的自然语言处理模型包括的支持向量机(SVM)、朴素贝叶斯分类器 (Naive Bayes)、随机森林 (Random Forest) 等。
此外,Deeplinik 还支持使用深度学习模型,如卷积神经网络(CNN)、长短时记忆网络 (LSTM)、注意力机制 (Attention Mechanism) 等。
3.模型训练在 Deeplinik 中,模型训练采用深度学习算法,如卷积神经网络 (CNN) 或长短时记忆网络 (LSTM) 等,对训练集进行模型训练。
在训练过程中,可以使用正则化技术、优化器等技术来进一步提高模型的性能。
4.模型评估在 Deeplinik 中,模型评估通常使用交叉验证、测试集等方法。
在交叉验证中,可以使用训练集来训练模型,使用测试集来评估模型的性能。
在测试集评估中,可以使用测试集来评估模型的泛化能力。
5.模型优化在 Deeplinik 中,模型优化是指通过调整模型参数、增加模型复杂度等方式来进一步提高模型性能。
在模型优化过程中,可以使用可视化技术、比较技术等方法来寻找优化方向。
6.模型应用在 Deeplinik 中,模型应用是指将训练好的模型应用于实际任务中,如文本分类、情感分析、命名实体识别等。
在模型应用过程中,需要对模型进行微调,以适应实际任务的需求。
深度学习(Deep-Learning)学习笔记整理]
![深度学习(Deep-Learning)学习笔记整理]](https://img.taocdn.com/s3/m/361f2e317375a417866f8f43.png)
十一、参考文献和Deep Learning学习资源一、概述Artificial Intelligence,也就是人工智能,就像长生不老和星际漫游一样,是人类最美好的梦想之一。
虽然计算机技术已经取得了长足的进步,但是到目前为止,还没有一台电脑能产生“自我”的意识。
是的,在人类和大量现成数据的帮助下,电脑可以表现的十分强大,但是离开了这两者,它甚至都不能分辨一个喵星人和一个汪星人。
图灵(图灵,大家都知道吧。
计算机和人工智能的鼻祖,分别对应于其著名的“图灵机”和“图灵测试”)在1950 年的论文里,提出图灵试验的设想,即,隔墙对话,你将不知道与你谈话的,是人还是电脑。
这无疑给计算机,尤其是人工智能,预设了一个很高的期望值。
但是半个世纪过去了,人工智能的进展,远远没有达到图灵试验的标准。
这不仅让多年翘首以待的人们,心灰意冷,认为人工智能是忽悠,相关领域是“伪科学”。
但是自2006 年以来,机器学习领域,取得了突破性的进展。
图灵试验,至少不是那么可望而不可及了。
至于技术手段,不仅仅依赖于云计算对大数据的并行处理能力,而且依赖于算法。
这个算法就是,Deep Learning。
借助于Deep Learning 算法,人类终于找到了如何处理“抽象概念”这个亘古难题的方法。
2012年6月,《纽约时报》披露了Google Brain项目,吸引了公众的广泛关注。
这个项目是由著名的斯坦福大学的机器学习教授Andrew Ng和在大规模计算机系统方面的世界顶尖专家JeffDean共同主导,用16000个CPU Core的并行计算平台训练一种称为“深度神经网络”(DNN,Deep Neural Networks)的机器学习模型(内部共有10亿个节点。
这一网络自然是不能跟人类的神经网络相提并论的。
要知道,人脑中可是有150多亿个神经元,互相连接的节点也就。
强化学习算法中的探索与利用技巧(七)

强化学习算法中的探索与利用技巧强化学习是一种通过试错来学习最佳行为的机器学习方法,其核心思想是在与环境的交互中,通过获得的奖励和惩罚来调整自身的行为策略。
在强化学习算法中,探索与利用是一个重要的问题,即如何在探索未知情况的同时最大化利用已有知识。
本文将从多个角度探讨强化学习算法中的探索与利用技巧。
首先,我们来谈谈强化学习中的“探索”策略。
在面对未知情况时,探索是至关重要的,因为只有通过不断尝试新的行为,智能体才能积累更多的经验和知识。
常见的探索策略包括ε-greedy策略、Softmax策略和Upper Confidence Bound (UCB)策略等。
ε-greedy策略是一种简单有效的探索策略,它以ε的概率选择随机动作进行探索,以1-ε的概率选择当前最优的动作进行利用。
这种策略在平衡探索和利用之间取得了不错的效果,但是对ε的选择非常敏感,过大会导致过多的探索,而过小则会导致过度利用已有知识。
Softmax策略则是一种基于概率分布的探索策略,它通过对动作的估值进行Softmax变换,以概率选择动作。
这种策略在探索和利用之间有较好的平衡,但也需要对温度参数进行合理的调整。
UCB策略则是一种基于置信上界的探索策略,它通过对每个动作的置信上界进行计算,选择置信上界最大的动作进行探索。
这种策略在探索未知情况时表现较好,但对动作价值的估计要求比较高。
其次,我们谈谈强化学习中的“利用”策略。
一旦智能体积累了一定的经验和知识,如何最大化利用这些知识就成为了一个重要问题。
在强化学习算法中,经验的积累通常通过值函数的估计来实现,例如Q-learning算法和SARSA算法。
这些算法通过不断更新值函数来估计每个状态和动作的价值,从而指导智能体的行为。
在利用已有知识时,通常采用贪心策略,即选择当前最优的动作进行利用。
然而,贪心策略会导致智能体陷入局部最优解,无法进行更深层次的探索。
因此,在利用策略的选择上,需要考虑一定的随机性,以保证智能体在利用已有知识的同时继续进行探索。
deep learning 心得
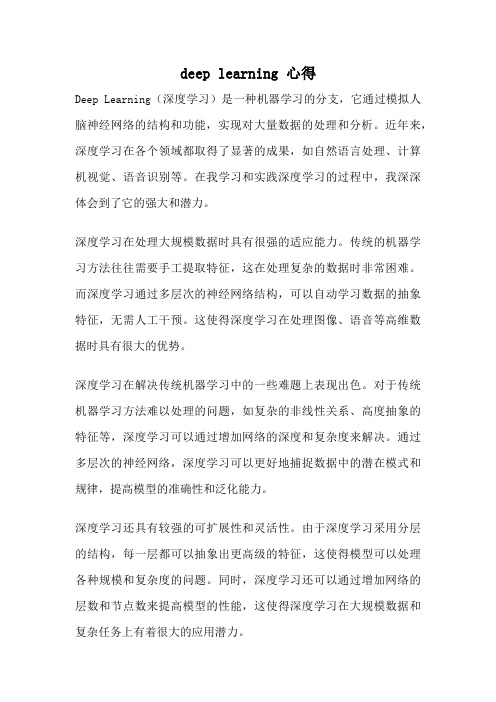
deep learning 心得Deep Learning(深度学习)是一种机器学习的分支,它通过模拟人脑神经网络的结构和功能,实现对大量数据的处理和分析。
近年来,深度学习在各个领域都取得了显著的成果,如自然语言处理、计算机视觉、语音识别等。
在我学习和实践深度学习的过程中,我深深体会到了它的强大和潜力。
深度学习在处理大规模数据时具有很强的适应能力。
传统的机器学习方法往往需要手工提取特征,这在处理复杂的数据时非常困难。
而深度学习通过多层次的神经网络结构,可以自动学习数据的抽象特征,无需人工干预。
这使得深度学习在处理图像、语音等高维数据时具有很大的优势。
深度学习在解决传统机器学习中的一些难题上表现出色。
对于传统机器学习方法难以处理的问题,如复杂的非线性关系、高度抽象的特征等,深度学习可以通过增加网络的深度和复杂度来解决。
通过多层次的神经网络,深度学习可以更好地捕捉数据中的潜在模式和规律,提高模型的准确性和泛化能力。
深度学习还具有较强的可扩展性和灵活性。
由于深度学习采用分层的结构,每一层都可以抽象出更高级的特征,这使得模型可以处理各种规模和复杂度的问题。
同时,深度学习还可以通过增加网络的层数和节点数来提高模型的性能,这使得深度学习在大规模数据和复杂任务上有着很大的应用潜力。
然而,深度学习也存在一些挑战和限制。
首先,深度学习的学习过程需要大量标记样本,这对于某些领域来说是一项耗时且昂贵的工作。
其次,深度学习的模型结构和参数设置较为复杂,需要较高的计算资源和算法优化技术。
此外,深度学习的解释性较差,很难解释模型的决策过程和推理规则。
尽管如此,深度学习仍然是目前最为热门和前沿的技术之一,具有广阔的应用前景。
在自然语言处理领域,深度学习已经取得了很大的突破,如机器翻译、情感分析等。
在计算机视觉领域,深度学习在图像分类、目标检测等任务上也取得了很高的准确性。
此外,深度学习还在医学影像分析、金融风控等领域展现出巨大的潜力。
greedy_decoder pytorch 原理
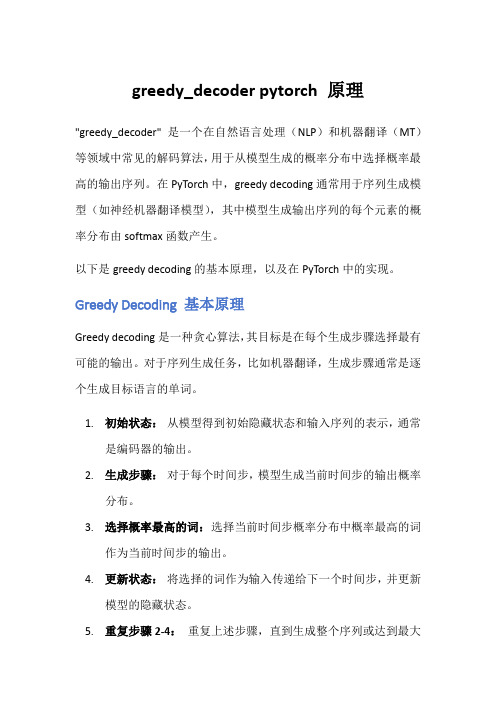
greedy_decoder pytorch 原理"greedy_decoder" 是一个在自然语言处理(NLP)和机器翻译(MT)等领域中常见的解码算法,用于从模型生成的概率分布中选择概率最高的输出序列。
在PyTorch中,greedy decoding通常用于序列生成模型(如神经机器翻译模型),其中模型生成输出序列的每个元素的概率分布由softmax函数产生。
以下是greedy decoding的基本原理,以及在PyTorch中的实现。
Greedy Decoding 基本原理Greedy decoding是一种贪心算法,其目标是在每个生成步骤选择最有可能的输出。
对于序列生成任务,比如机器翻译,生成步骤通常是逐个生成目标语言的单词。
1.初始状态:从模型得到初始隐藏状态和输入序列的表示,通常是编码器的输出。
2.生成步骤:对于每个时间步,模型生成当前时间步的输出概率分布。
3.选择概率最高的词:选择当前时间步概率分布中概率最高的词作为当前时间步的输出。
4.更新状态:将选择的词作为输入传递给下一个时间步,并更新模型的隐藏状态。
5.重复步骤2-4:重复上述步骤,直到生成整个序列或达到最大生成长度。
PyTorch 中的Greedy Decoding 实现在PyTorch中,可以通过以下方式实现greedy decoding:import torchdef greedy_decode(model, src_input, max_len, start_symbol): """Greedy decoding for sequence generation.Args:model: 模型,应该包含一个生成方法。
src_input: 输入序列。
max_len: 最大生成长度。
start_symbol: 序列生成的起始符号。
Returns:输出的生成序列。
"""with torch.no_grad():# 编码器部分,得到初始状态和输入序列的表示encoder_outputs, encoder_hidden =model.encoder(src_input)# 初始化解码器输入为起始符号decoder_input =torch.tensor([[start_symbol]], dtype=torch.l ong, device=device)# 初始化解码器的隐藏状态为编码器的最终隐藏状态decoder_hidden =encoder_hidden# 存储生成的序列decoded_seq =[start_symbol]# Greedy decoding 循环for_ in range(max_len):# 解码器生成当前时间步的输出decoder_output, decoder_hidden =model.decoder(dec oder_input, decoder_hidden, encoder_outputs)# 选择概率最高的词作为当前时间步的输出_, topi =decoder_output.topk(1)decoder_input =topi.squeeze().detach()# 将选择的词添加到生成序列中decoded_seq.append(decoder_input.item())# 如果当前时间步输出为终止符号,停止生成if decoder_input.item() ==EOS_token:breakreturn decoded_seq这是一个简单的greedy decoding实现,其中模型应该具有encoder 和decoder两个组件。
- 1、下载文档前请自行甄别文档内容的完整性,平台不提供额外的编辑、内容补充、找答案等附加服务。
- 2、"仅部分预览"的文档,不可在线预览部分如存在完整性等问题,可反馈申请退款(可完整预览的文档不适用该条件!)。
- 3、如文档侵犯您的权益,请联系客服反馈,我们会尽快为您处理(人工客服工作时间:9:00-18:30)。
II. LITERATURE REVIEW We will briefly review prior studies on dictionary learning, stacked autoencoders and deep Boltzmann machines. A. Dictionary Learning Early studies in dictionary learning wanted to learn a basis for
Greedy Deep Dictionary Learning
Snigdha Tariyal, Angshul Majumdar, Member IEEE, Richa Singh, Senior Member IEEE, and Mayank Vatsa, Senior Member, IEEE
Abstract—In this work we propose a new deep learning tool – deep dictionary learning. Multi-level dictionaries are learnt in a greedy fashion – one layer at a time. This requires solving a simple (shallow) dictionary learning problem; the solution to this is well known. We apply the proposed technique on some benchmark deep learning datasets. We compare our results with other deep learning tools like stacked autoencoder and deep belief network; and state-of-the-art supervised dictionary learning tools like discriminative K-SVD and label consistent K-SVD. Our method yields better results than all. Index Terms—Deep Learning, Dictionary Learning, Feature Extraction
I
I. INTRODUCTION
N recent years there has been a lot of interest in dictionary learning. However the concept of dictionary learning has been around for much longer. Its application in vision [1] and information retrieval [2] dates back to the late 90’s. In those days, the term ‘dictionary learning’ had not been coined; researchers were using the term ‘matrix factorization’. The goal was to learn an empirical basis from the data. It basically required decomposing the data matrix to a basis / dictionary matrix and a feature matrix – hence the name ‘matrix factorization’. The current popularity of dictionary learning owes to K-SVD [3, 4]. K-SVD is an algorithm to decompose a matrix (training data) into a dense basis and sparse coefficients. However the concept of such a dense-sparse decomposition predates K-SVD [5]. Since the advent of K-SVD in 2006, there have been a plethora of work on this topic. Dictionary learning can be used both for unsupervised problems (mainly inverse problems in image processing) as well as for problems arising in supervised feature extraction. Dictionary learning has been used in virtually all inverse problems arising in image processing starting from simple image [6, 7] and video [8] denoising, image inpainting [9], to more complex problems like color image restoration [10], inverse half toning [11] and even medical image reconstruction [12, 13]. Solving inverse problems is not the goal of this work; we are more interested in dictionary learning from the perspective of machine learning. We briefly discussed [6-13] for the sake of completeness. Mathematical transforms like DCT, wavelet, curvelet, Gabor etc. have been widely used in image classification problems
[14-16]. These techniques used these transforms as a sparsifying step followed by statistical feature extraction methods like PCA or LDA before feeding the features to a classifier. Just as dictionary learning is replacing such fixed transforms (wavelet, DCT, curvelet etc.) in signal processing problems, it is also replacing them in feature extraction scenarios. Dictionary learning gives researchers the opportunity to design dictionaries to yield not only sparse representation (like curvelet, wavelet, DCT etc.) but also discriminative information. Initial techniques proposed naïve approaches which learnt specific dictionaries for each class [17-19]. Later approaches incorporated discriminative penalties into the dictionary learning framework. One such technique is to include softmax discriminative cost function [20-22]; other discriminative penalties include Fisher discrimination criterion [23], linear predictive classification error [24, 25] and hinge loss function [26, 27]. In [28, 29] discrimination is introduced by forcing the learned features to map to corresponding class labels. All prior studies on dictionary learning (DL) are ‘shallow’ learning models just like a restricted boltzman machine (RBM) [30] and autoencoder (AE) [31]. DL, RBM and AE – all fall under the broader topic of representation learning. In DL, the cost function is Euclidean distance between the data and the representation given the learned basis; for RBM it is Boltzman energy; in AE, the cost is the Euclidean reconstruction error between the data and the decoded representation / features. Almost at the same time, when dictionary learning started gaining popularity, researchers in machine learning observed that better (more abstract and compact) representation can be achieved by going deeper. Deep Belief Network (DBN) is formed by stacking one RBM after the other [32, 33]. Similarly stacked autoencoder (SAE) were created by one AE inside the other [34, 35]. Following the success of DBN and SAE, we propose to learn multi-level deep dictionaries. This is the first work on deep dictionary learning. The rest of the paper will be organized into ห้องสมุดไป่ตู้everal sections….