遗传算法适应度函数源代码produceobjectivevalue
遗传算法代码python
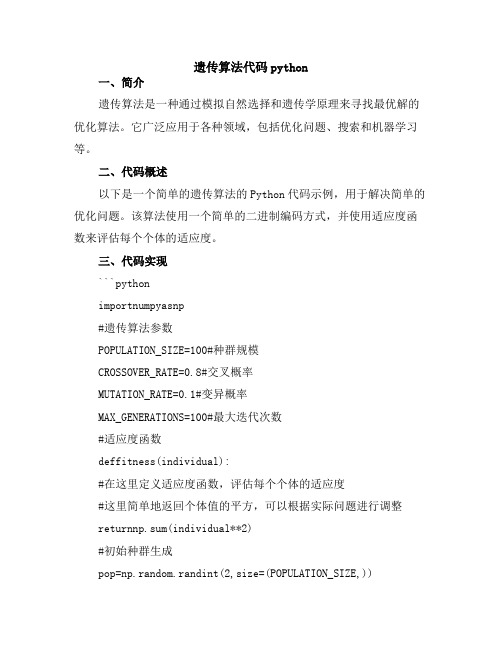
遗传算法代码python一、简介遗传算法是一种通过模拟自然选择和遗传学原理来寻找最优解的优化算法。
它广泛应用于各种领域,包括优化问题、搜索和机器学习等。
二、代码概述以下是一个简单的遗传算法的Python代码示例,用于解决简单的优化问题。
该算法使用一个简单的二进制编码方式,并使用适应度函数来评估每个个体的适应度。
三、代码实现```pythonimportnumpyasnp#遗传算法参数POPULATION_SIZE=100#种群规模CROSSOVER_RATE=0.8#交叉概率MUTATION_RATE=0.1#变异概率MAX_GENERATIONS=100#最大迭代次数#适应度函数deffitness(individual):#在这里定义适应度函数,评估每个个体的适应度#这里简单地返回个体值的平方,可以根据实际问题进行调整returnnp.sum(individual**2)#初始种群生成pop=np.random.randint(2,size=(POPULATION_SIZE,))#迭代过程forgenerationinrange(MAX_GENERATIONS):#评估种群中每个个体的适应度fitness_values=np.apply_along_axis(fitness,1,pop)#选择种群selected_idx=np.random.choice(np.arange(POPULATION_SIZE), size=POPULATION_SIZE,replace=True,p=fitness_values/fitness_va lues.sum())selected_pop=pop[selected_idx]#交叉操作ifCROSSOVER_RATE>np.random.rand():cross_points=np.random.rand(POPULATION_SIZE,2)<0.5#随机选择交叉点cross_pop=np.array([np.hstack((individual[cross_points[i, 0]:cross_points[i,1]]+individual[cross_points[i,1]:],other))f ori,otherinenumerate(selected_pop)]).T#合并个体并随机交叉得到新的个体cross_pop=cross_pop[cross_points]#将交叉后的个体重新排列成原始种群大小selected_pop=np.vstack((selected_pop,cross_pop))#将新个体加入种群中#变异操作ifMUTATION_RATE>np.random.rand():mutated_pop=selected_pop+np.random.randn(POPULATION_SIZE, 1)*np.sqrt(np.log(POPULATION_SIZE))*(selected_pop!=pop).astyp e(np.float)#根据变异概率对个体进行变异操作,得到新的个体种群mutated_pop=mutated_pop[mutated_pop!=0]#将二进制种群中值为0的个体去掉,因为这些个体是随机的二进制串,不是解的一部分,不应该参与变异操作selected_pop=mutated_pop[:POPULATION_SIZE]#将新种群中除最后一个以外的部分加入原始种群中(即新的种群被排除了适应度最差的个体)#选择当前最好的个体(用于更新最优解)best_idx=np.argmax(fitness_values)best_solution=selected_pop[best_idx]print(f"Generation{generation}:Bestsolution:{best_solutio n}")```四、使用示例假设要解决一个简单的优化问题:求一个一维函数的最小值。
遗传算法C语言源代码(一元函数和二元函数)

.专业整理 .C语言遗传算法代码以下为遗传算法的源代码,计算一元代函数的代码和二元函数的代码以+++++++++++++++++++++++++++++++++++++为分割线分割开来,请自行选择适合的代码,使用时请略看完代码的注释,在需要更改的地方更改为自己需要的代码。
+++++++++++++++++++++++++++++++一元函数代码++++++++++++++++++++++++++++#include <stdio.h>#include<stdlib.h>#include<time.h>#include<math.h>#define POPSIZE 1000#define maximization 1#define minimization 2#define cmax 100#define cmin 0#define length1 20#define chromlength length1// 染色体长度//注意,你是求最大值还是求最小值.专业整理 .//变量的上下限的修改开始float min_x1=-2;//变量的下界float max_x1=-1;//变量的上界//变量的上下限的修改结束int popsize;// 种群大小int maxgeneration;// 最大世代数double pc;// 交叉率double pm;// 变异率struct individual{char chrom[chromlength+1];double value;double fitness;// 适应度};int generation;// 世代数int best_index;int worst_index;struct individual bestindividual;// 最佳个体.专业整理 . struct individual worstindividual; //最差个体struct individual currentbest;struct individual population[POPSIZE];//函数声明void generateinitialpopulation();void generatenextpopulation();void evaluatepopulation();long decodechromosome(char *,int,int);void calculateobjectvalue();void calculatefitnessvalue();void findbestandworstindividual();void performevolution();void selectoperator();void crossoveroperator();void mutationoperator();void input();void outputtextreport();void generateinitialpopulation( )// 种群初始化{int i,j;.专业整理 .for (i=0;i<popsize; i++){for( j=0;j<chromlength;j++){population[i].chrom[j]=(rand()%20<10)?'0':'1';}population[i].chrom[chromlength]='\0';}}void generatenextpopulation()// 生成下一代{selectoperator();crossoveroperator();mutationoperator();}void evaluatepopulation()// 评价个体,求最佳个体{calculateobjectvalue();calculatefitnessvalue();findbestandworstindividual();}long decodechromosome(char *string ,int point,int length) //给染色体解码{int i;long decimal=0;char*pointer;for(i=0,pointer=string+point;i<length;i++,pointer++)if(*pointer-'0'){decimal +=(long)pow(2,i);}return (decimal);}void calculateobjectvalue()// 计算函数值{int i;long temp1,temp2;double x1;for (i=0; i<popsize; i++){temp1=decodechromosome(population[i].chrom,0,length1);x1=(max_x1-min_x1)*temp1/(1024*1024-1)+min_x1;//目标函数修改开始population[i].value=(pow(x1,5)-3*x1-1)*(pow(x1,5)-3*x1-1);//目标函数修改结束}}void calculatefitnessvalue()//计算适应度{int i;double temp;for(i=0;i<popsize;i++){if(functionmode==maximization){if((population[i].value+cmin)>0.0){temp=cmin+population[i].value;}else{temp=0.0;}}else if (functionmode==minimization){if(population[i].value<cmax){temp=cmax-population[i].value;}else{ temp=0.0;}}population[i].fitness=temp;}}void findbestandworstindividual( ) //求最佳个体和最差个体{int i;double sum=0.0;bestindividual=population[0];worstindividual=population[0];.专业整理 .for (i=1;i<popsize; i++){if (population[i].fitness>bestindividual.fitness){bestindividual=population[i];best_index=i;}else if (population[i].fitness<worstindividual.fitness) {worstindividual=population[i];worst_index=i;}sum+=population[i].fitness;}if (generation==0){currentbest=bestindividual;}else{if(bestindividual.fitness>=currentbest.fitness){currentbest=bestindividual;}}}void performevolution() //演示评价结果{if (bestindividual.fitness>currentbest.fitness){ currentbest=population[best_index];}else{population[worst_index]=currentbest;}}void selectoperator() //比例选择算法{int i,index;double p,sum=0.0;double cfitness[POPSIZE];struct individual newpopulation[POPSIZE];for(i=0;i<popsize;i++){sum+=population[i].fitness;}for(i=0;i<popsize; i++){cfitness[i]=population[i].fitness/sum;}for(i=1;i<popsize; i++){cfitness[i]=cfitness[i-1]+cfitness[i];}for (i=0;i<popsize;i++){p=rand()%1000/1000.0;index=0;while (p>cfitness[index]){index++;}newpopulation[i]=population[index];}for(i=0;i<popsize; i++){population[i]=newpopulation[i];}}void crossoveroperator() //交叉算法{int i,j;int index[POPSIZE];int point,temp;double p;char ch;for (i=0;i<popsize;i++){index[i]=i;}for (i=0;i<popsize;i++){point=rand()%(popsize-i);temp=index[i];index[i]=index[point+i];index[point+i]=temp;}for (i=0;i<popsize-1;i+=2){p=rand()%1000/1000.0;if (p<pc){point=rand()%(chromlength-1)+1;for ( j=point; j<chromlength;j++){ch=population[index[i]].chrom[j];population[index[i]].chrom[j]=population[index[i+1]].chrom[j];population[index[i+1]].chrom[j]=ch;}}}}void mutationoperator() //变异操作{int i,j;double p;for (i=0;i<popsize;i++){for( j=0;j<chromlength;j++){p=rand()%1000/1000.0;if (p<pm){population[i].chrom[j]=(population[i].chrom[j]=='0')?'1':'0';}}}}void input() //数据输入{ //printf(" 初始化全局变量 :\n");//printf("种群大小 (50-500) : ");//scanf("%d", &popsize);popsize=500;if((popsize%2) != 0){//printf( "种群大小已设置为偶数\n");popsize++;};//printf("最大世代数 (100-300) : ");//scanf("%d", &maxgeneration); maxgeneration=200;//printf("交叉率 (0.2-0.99) : ");//scanf("%f", &pc);pc=0.95;//printf("变异率 (0.001-0.1) :");//scanf("%f", &pm);pm=0.03;}void outputtextreport()//数据输出{int i;double sum;double average;sum=0.0;for(i=0;i<popsize;i++){sum+=population[i].value;}average=sum/popsize;printf("当前世代=%d\n当前世代平均函数值=%f\n当前世代最优函数值=%f\n",generation,average,population[best_index].value);}void main()// 主函数{ int i;long temp1,temp2;double x1,x2;generation=0;input();generateinitialpopulation();evaluatepopulation();while(generation<maxgeneration){generation++;generatenextpopulation();evaluatepopulation();performevolution();outputtextreport();}printf("\n");printf("统计结果 :");printf("\n");//printf("最大函数值等于:%f\n",currentbest.fitness);printf(" 其染色体编码为:");for (i=0;i<chromlength;i++){printf("%c",currentbest.chrom[i]);}printf("\n");temp1=decodechromosome(currentbest.chrom,0,length1);x1=(max_x1-min_x1)*temp1/(1024*1024-1)+min_x1;printf("x1=%lf\n",x1);.专业整理 .//这是需要修改的地方printf(" 最优值等于:%f\n",(pow(x1,5)-3*x1-1)*(pow(x1,5)-3*x1-1));}+++++++++++++++++++++++++二元函数代码+++++++++++++++++++++++++++++++++++++++++#include <stdio.h>#include<stdlib.h>#include<time.h>#include<math.h>#define POPSIZE 500#define maximization 1#define minimization 2#define cmax 100#define cmin 0#define length1 20#define length2 20#define chromlength length1+length2// 染色体长度//-----------求最大还是最小值int functionmode=maximization;//-----------//-----------变量上下界float min_x1=0;float max_x1=3;float min_x2=1;float max_x2=5;//-----------int popsize;// 种群大小int maxgeneration;// 最大世代数double pc;// 交叉率double pm;// 变异率struct individual{char chrom[chromlength+1];double value;double fitness;// 适应度};int generation;// 世代数int best_index;int worst_index;struct individual bestindividual;// 最佳个体struct individual worstindividual; //最差个体struct individual currentbest;struct individual population[POPSIZE];//函数声明void generateinitialpopulation();void generatenextpopulation();void evaluatepopulation();long decodechromosome(char *,int,int);void calculateobjectvalue();void calculatefitnessvalue();void findbestandworstindividual();void performevolution();void selectoperator();void crossoveroperator();void mutationoperator();void input();void outputtextreport();void generateinitialpopulation( )// 种群初始化{int i,j;for (i=0;i<popsize; i++){for( j=0;j<chromlength;j++){population[i].chrom[j]=(rand()%40<20)?'0':'1';}population[i].chrom[chromlength]='\0';}}void generatenextpopulation()// 生成下一代{selectoperator();crossoveroperator();mutationoperator();}void evaluatepopulation()// 评价个体,求最佳个体{calculateobjectvalue();calculatefitnessvalue();findbestandworstindividual();}long decodechromosome(char *string ,int point,int length) //给染色体解码{int i;long decimal=0;char*pointer;for(i=0,pointer=string+point;i<length;i++,pointer++)if(*pointer-'0'){decimal +=(long)pow(2,i);}return (decimal);}void calculateobjectvalue()// 计算函数值{int i;long temp1,temp2;double x1,x2;for (i=0; i<popsize; i++){temp1=decodechromosome(population[i].chrom,0,length1);temp2=decodechromosome(population[i].chrom,length1,length2);x1=(max_x1-min_x1)*temp1/(1024*1024-1)+min_x1;x2=(max_x2-min_x2)*temp2/(1024*1024-1)+min_x2;//-----------函数population[i].value=x1*x1+sin(x1*x2)-x2*x2;//-----------}}void calculatefitnessvalue()//计算适应度{int i;double temp;for(i=0;i<popsize;i++){if(functionmode==maximization){if((population[i].value+cmin)>0.0){temp=cmin+population[i].value;}else{temp=0.0;}}else if (functionmode==minimization){if(population[i].value<cmax){temp=cmax-population[i].value;}else{ temp=0.0;}}population[i].fitness=temp;}}void findbestandworstindividual( ) //求最佳个体和最差个体{int i;double sum=0.0;bestindividual=population[0];worstindividual=population[0];for (i=1;i<popsize; i++){if (population[i].fitness>bestindividual.fitness){bestindividual=population[i];best_index=i;}else if (population[i].fitness<worstindividual.fitness){worstindividual=population[i];worst_index=i;}sum+=population[i].fitness;}if (generation==0){currentbest=bestindividual;}else{if(bestindividual.fitness>=currentbest.fitness){currentbest=bestindividual;}}}void performevolution() //演示评价结果{if (bestindividual.fitness>currentbest.fitness){currentbest=population[best_index];}else{population[worst_index]=currentbest;}}void selectoperator() //比例选择算法{int i,index;double p,sum=0.0;double cfitness[POPSIZE];struct individual newpopulation[POPSIZE];for(i=0;i<popsize;i++){sum+=population[i].fitness;}for(i=0;i<popsize; i++){cfitness[i]=population[i].fitness/sum;}for(i=1;i<popsize; i++){cfitness[i]=cfitness[i-1]+cfitness[i];}for (i=0;i<popsize;i++){p=rand()%1000/1000.0;index=0;while (p>cfitness[index]){index++;}newpopulation[i]=population[index];}for(i=0;i<popsize; i++){population[i]=newpopulation[i];}}void crossoveroperator() //交叉算法{int i,j;int index[POPSIZE];int point,temp;double p;char ch;for (i=0;i<popsize;i++){index[i]=i;}for (i=0;i<popsize;i++){point=rand()%(popsize-i);temp=index[i];index[i]=index[point+i];index[point+i]=temp;}for (i=0;i<popsize-1;i+=2){p=rand()%1000/1000.0;if (p<pc){point=rand()%(chromlength-1)+1;for ( j=point; j<chromlength;j++){ch=population[index[i]].chrom[j];population[index[i]].chrom[j]=population[index[i+1]].chrom[j];population[index[i+1]].chrom[j]=ch;}}}}void mutationoperator() //变异操作{int i,j;double p;for (i=0;i<popsize;i++){for( j=0;j<chromlength;j++){p=rand()%1000/1000.0;if (p<pm){population[i].chrom[j]=(population[i].chrom[j]=='0')?'1':'0';}}}}void input() //数据输入{ //printf(" 初始化全局变量 :\n");//printf("种群大小 (50-500) : ");//scanf("%d", &popsize);popsize=200;if((popsize%2) != 0){//printf( "种群大小已设置为偶数\n");popsize++;};//printf("最大世代数 (100-300) : ");//scanf("%d", &maxgeneration);maxgeneration=200;//printf("交叉率 (0.2-0.99): ");//scanf("%f", &pc);pc=0.9;//printf("变异率 (0.001-0.1):");//scanf("%f", &pm);pm=0.003;}void outputtextreport()//数据输出{int i;double sum;double average;sum=0.0;for(i=0;i<popsize;i++){sum+=population[i].value;}average=sum/popsize;printf("当前世代=%d\n当前世代平均函数值=%f\n当前世代最优函数值=%f\n",generation,average,population[best_index].value);}void main()// 主函数{ int i;long temp1,temp2;double x1,x2;generation=0;input();generateinitialpopulation();evaluatepopulation();while(generation<maxgeneration){generation++;generatenextpopulation();evaluatepopulation();performevolution();outputtextreport();}printf("\n");printf("统计结果 :");printf("\n");//printf("最大函数值等于:%f\n",currentbest.fitness);printf(" 其染色体编码为:");for (i=0;i<chromlength;i++){printf("%c",currentbest.chrom[i]);}printf("\n");temp1=decodechromosome(currentbest.chrom,0,length1);temp2=decodechromosome(currentbest.chrom,length1,length2);x1=(max_x1-min_x1)*temp1/(1024*1024-1)+min_x1;x2=(max_x2-min_x2)*temp2/(1024*1024-1)+min_x2;printf("x=%lf,y=%lf\n",x1,x2);//-----------修改函数printf(" 最大值 =%f\n",x1*x1+sin(x1*x2)-x2*x2);//-----------}。
遗传算法代码
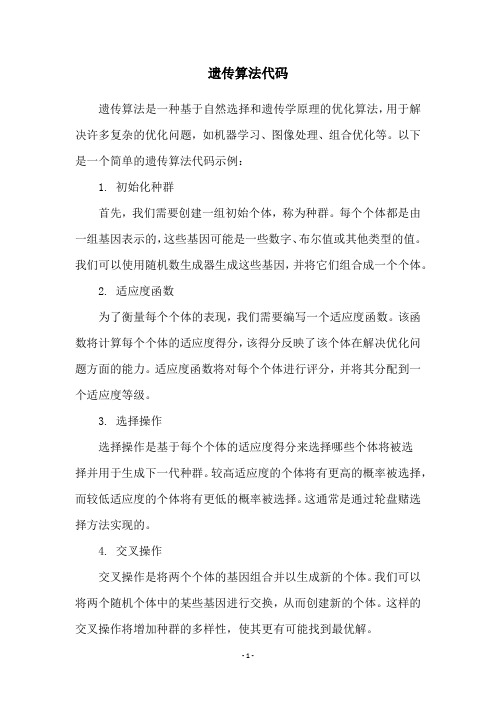
遗传算法代码遗传算法是一种基于自然选择和遗传学原理的优化算法,用于解决许多复杂的优化问题,如机器学习、图像处理、组合优化等。
以下是一个简单的遗传算法代码示例:1. 初始化种群首先,我们需要创建一组初始个体,称为种群。
每个个体都是由一组基因表示的,这些基因可能是一些数字、布尔值或其他类型的值。
我们可以使用随机数生成器生成这些基因,并将它们组合成一个个体。
2. 适应度函数为了衡量每个个体的表现,我们需要编写一个适应度函数。
该函数将计算每个个体的适应度得分,该得分反映了该个体在解决优化问题方面的能力。
适应度函数将对每个个体进行评分,并将其分配到一个适应度等级。
3. 选择操作选择操作是基于每个个体的适应度得分来选择哪些个体将被选择并用于生成下一代种群。
较高适应度的个体将有更高的概率被选择,而较低适应度的个体将有更低的概率被选择。
这通常是通过轮盘赌选择方法实现的。
4. 交叉操作交叉操作是将两个个体的基因组合并以生成新的个体。
我们可以将两个随机个体中的某些基因进行交换,从而创建新的个体。
这样的交叉操作将增加种群的多样性,使其更有可能找到最优解。
5. 变异操作变异操作是用于引入种群中的随机性的操作。
在变异操作中,我们将随机选择一个个体,并随机更改其中的一个或多个基因。
这将引入新的、未经探索的基因组合,从而增加种群的多样性。
6. 迭代随着种群不断进化,每个个体的适应度得分也将不断提高。
我们将重复执行选择、交叉和变异操作,以生成新的个体,并淘汰旧的个体。
这个不断迭代的过程将继续,直到达到预设的迭代次数或找到最优解为止。
这是一个简单的遗传算法代码示例,它演示了如何使用遗传算法来解决优化问题。
在实际应用中,我们可以进一步对算法进行优化,以获得更好的结果。
详解用python实现简单的遗传算法
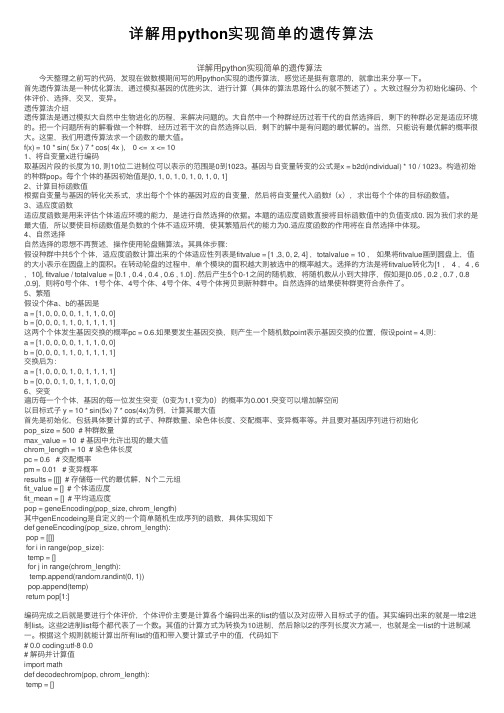
详解⽤python实现简单的遗传算法详解⽤python实现简单的遗传算法今天整理之前写的代码,发现在做数模期间写的⽤python实现的遗传算法,感觉还是挺有意思的,就拿出来分享⼀下。
⾸先遗传算法是⼀种优化算法,通过模拟基因的优胜劣汰,进⾏计算(具体的算法思路什么的就不赘述了)。
⼤致过程分为初始化编码、个体评价、选择,交叉,变异。
遗传算法介绍遗传算法是通过模拟⼤⾃然中⽣物进化的历程,来解决问题的。
⼤⾃然中⼀个种群经历过若⼲代的⾃然选择后,剩下的种群必定是适应环境的。
把⼀个问题所有的解看做⼀个种群,经历过若⼲次的⾃然选择以后,剩下的解中是有问题的最优解的。
当然,只能说有最优解的概率很⼤。
这⾥,我们⽤遗传算法求⼀个函数的最⼤值。
f(x) = 10 * sin( 5x ) 7 * cos( 4x ), 0 <= x <= 101、将⾃变量x进⾏编码取基因⽚段的长度为10, 则10位⼆进制位可以表⽰的范围是0到1023。
基因与⾃变量转变的公式是x = b2d(individual) * 10 / 1023。
构造初始的种群pop。
每个个体的基因初始值是[0, 1, 0, 1, 0, 1, 0, 1, 0, 1]2、计算⽬标函数值根据⾃变量与基因的转化关系式,求出每个个体的基因对应的⾃变量,然后将⾃变量代⼊函数f(x),求出每个个体的⽬标函数值。
3、适应度函数适应度函数是⽤来评估个体适应环境的能⼒,是进⾏⾃然选择的依据。
本题的适应度函数直接将⽬标函数值中的负值变成0. 因为我们求的是最⼤值,所以要使⽬标函数值是负数的个体不适应环境,使其繁殖后代的能⼒为0.适应度函数的作⽤将在⾃然选择中体现。
4、⾃然选择⾃然选择的思想不再赘述,操作使⽤轮盘赌算法。
其具体步骤:假设种群中共5个个体,适应度函数计算出来的个体适应性列表是fitvalue = [1 ,3, 0, 2, 4] ,totalvalue = 10 ,如果将fitvalue画到圆盘上,值的⼤⼩表⽰在圆盘上的⾯积。
遗传算法matlab代码
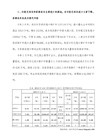
fplot(f,[xmin,xmax]);%隐函数画图
grid on;hold on;
plot(x,fit,'k*');%作图,画初始种群的适应度图像
title('(a)染色体的初始位置');%标题
xlabel('x');ylabel('f(x)');%标记轴
close all;
clc;%清屏
tic;%计时器开始计时
n=20;ger=100;pc=0.65;pm=0.05;%初始化参数
%以上为经验值,可以更改。
% 生成初始种群
v=init_population(n,22); %得到初始种群,22串长,生成20*22的0-1矩阵
[N,L]=size(v); %得到初始规模行,列
v=fliplr(v); %实现左右翻转颠倒
[s,c]=size(v); %c代表串长。求行,列
aux=0:1:c-1; %21维向量
aux=ones(s,1)*aux;%权值向量矩阵
x1=sum((v.*2.^aux)');%权值 %注意转置 %sum是求列和
x=xymin+(xymax-xymin)*x1./(2^c-1); %最大值4194303;
disp(sprintf('Number of generations:%d',ger));
disp(sprintf('Population size:%d',N));
disp(sprintf('Crossover probability:%.3f',pc));
遗传算法案例及源代码
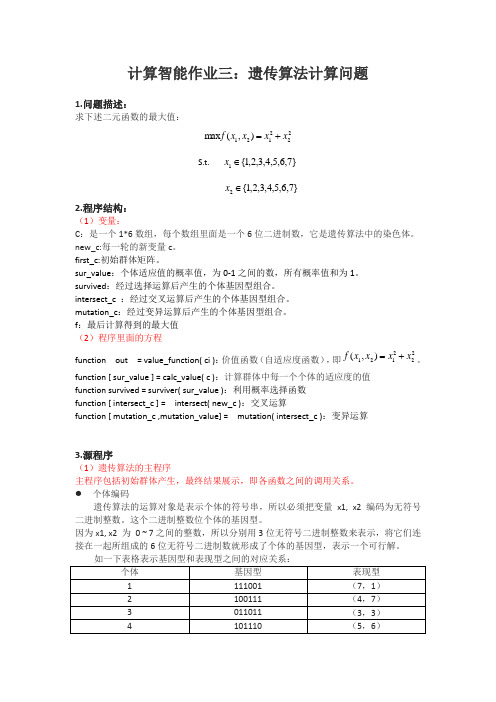
计算智能作业三:遗传算法计算问题1.问题描述:求下述二元函数的最大值:222121),(m ax x x x x f +=S.t. }7,6,5,4,3,2,1{1∈x }7,6,5,4,3,2,1{2∈x2.程序结构:(1)变量:C :是一个1*6数组,每个数组里面是一个6位二进制数,它是遗传算法中的染色体。
new_c:每一轮的新变量c 。
first_c:初始群体矩阵。
sur_value :个体适应值的概率值,为0-1之间的数,所有概率值和为1。
survived :经过选择运算后产生的个体基因型组合。
intersect_c :经过交叉运算后产生的个体基因型组合。
mutation_c :经过变异运算后产生的个体基因型组合。
f :最后计算得到的最大值 (2)程序里面的方程function out = value_function( ci ):价值函数(自适应度函数),即222121),(x x x x f +=。
function [ sur_value ] = calc_value( c ):计算群体中每一个个体的适应度的值function survived = surviver( sur_value ):利用概率选择函数 function [ intersect_c ] = intersect( new_c ):交叉运算function [ mutation_c ,mutation_value] = mutation( intersect_c ):变异运算3.源程序(1)遗传算法的主程序主程序包括初始群体产生,最终结果展示,即各函数之间的调用关系。
个体编码遗传算法的运算对象是表示个体的符号串,所以必须把变量 x1, x2 编码为无符号二进制整数。
这个二进制整数位个体的基因型。
因为x1, x2 为 0 ~ 7之间的整数,所以分别用3位无符号二进制整数来表示,将它们连接在一起所组成的6位无符号二进制数就形成了个体的基因型,表示一个可行解。
(完整版)遗传算法c语言代码
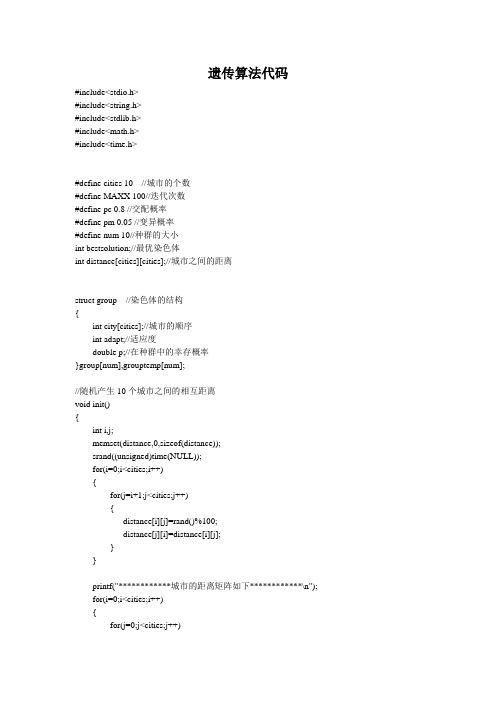
srand((unsigned)time(NULL));
for(i=0;i<num;i++)
{
bianyip[i]=(rand()%100);
bianyip[i]/=100;
}
//确定可以变异的染色体
t=0;
for(i=0;i<num;i++)
{
if(bianyip[i]<pm)
printf("\n******************是否想再一次计算(y or n)***********************\n");
fflush(stdin);
scanf("%c",&choice);
}while(choice=='y');
return 0;
}
{
flag=0;
break;
}
}
if(flag)
{
group[i].city[j]=t;
j++;
}
}
}
printf("************初始种群如下****************\n");
for(i=0;i<num;i++)
{
for(j=0;j<cities;j++)
printf("%4d",group[i].city[j]);
{
group[i].p=1-(double)group[i].adapt/(double)biggestsum;
biggestp+=group[i].p;
(完整版)遗传算法简介及代码详解
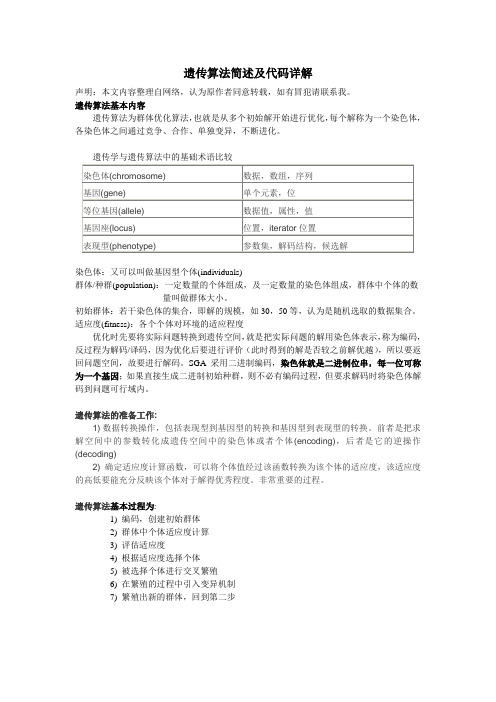
遗传算法简述及代码详解声明:本文内容整理自网络,认为原作者同意转载,如有冒犯请联系我。
遗传算法基本内容遗传算法为群体优化算法,也就是从多个初始解开始进行优化,每个解称为一个染色体,各染色体之间通过竞争、合作、单独变异,不断进化。
遗传学与遗传算法中的基础术语比较染色体:又可以叫做基因型个体(individuals)群体/种群(population):一定数量的个体组成,及一定数量的染色体组成,群体中个体的数量叫做群体大小。
初始群体:若干染色体的集合,即解的规模,如30,50等,认为是随机选取的数据集合。
适应度(fitness):各个个体对环境的适应程度优化时先要将实际问题转换到遗传空间,就是把实际问题的解用染色体表示,称为编码,反过程为解码/译码,因为优化后要进行评价(此时得到的解是否较之前解优越),所以要返回问题空间,故要进行解码。
SGA采用二进制编码,染色体就是二进制位串,每一位可称为一个基因;如果直接生成二进制初始种群,则不必有编码过程,但要求解码时将染色体解码到问题可行域内。
遗传算法的准备工作:1) 数据转换操作,包括表现型到基因型的转换和基因型到表现型的转换。
前者是把求解空间中的参数转化成遗传空间中的染色体或者个体(encoding),后者是它的逆操作(decoding)2) 确定适应度计算函数,可以将个体值经过该函数转换为该个体的适应度,该适应度的高低要能充分反映该个体对于解得优秀程度。
非常重要的过程。
遗传算法基本过程为:1) 编码,创建初始群体2) 群体中个体适应度计算3) 评估适应度4) 根据适应度选择个体5) 被选择个体进行交叉繁殖6) 在繁殖的过程中引入变异机制7) 繁殖出新的群体,回到第二步实例一:(建议先看实例二)求 []30,0∈x 范围内的()210-=x y 的最小值1) 编码算法选择为"将x 转化为2进制的串",串的长度为5位(串的长度根据解的精度设 定,串长度越长解得精度越高)。
python 遗传算法适应度函数
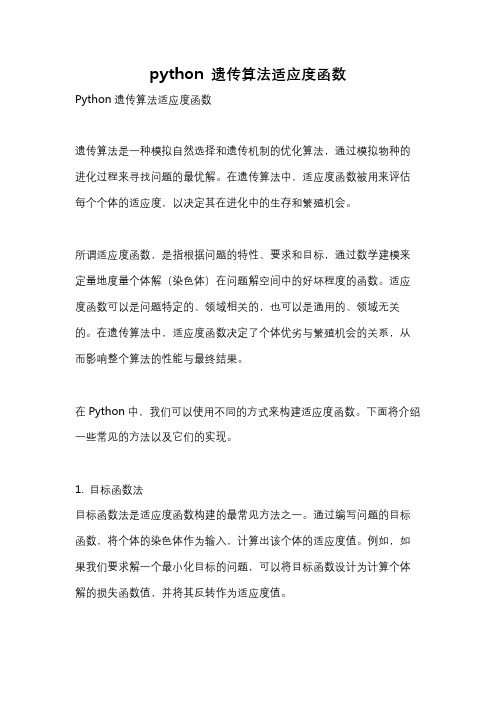
python 遗传算法适应度函数Python遗传算法适应度函数遗传算法是一种模拟自然选择和遗传机制的优化算法,通过模拟物种的进化过程来寻找问题的最优解。
在遗传算法中,适应度函数被用来评估每个个体的适应度,以决定其在进化中的生存和繁殖机会。
所谓适应度函数,是指根据问题的特性、要求和目标,通过数学建模来定量地度量个体解(染色体)在问题解空间中的好坏程度的函数。
适应度函数可以是问题特定的、领域相关的,也可以是通用的、领域无关的。
在遗传算法中,适应度函数决定了个体优劣与繁殖机会的关系,从而影响整个算法的性能与最终结果。
在Python中,我们可以使用不同的方式来构建适应度函数。
下面将介绍一些常见的方法以及它们的实现。
1. 目标函数法目标函数法是适应度函数构建的最常见方法之一。
通过编写问题的目标函数,将个体的染色体作为输入,计算出该个体的适应度值。
例如,如果我们要求解一个最小化目标的问题,可以将目标函数设计为计算个体解的损失函数值,并将其反转作为适应度值。
例如,假设我们要解决一个最小化函数的问题,其中目标函数为f(x) = x^2。
我们可以使用Python代码来实现适应度函数:pythondef fitness_function(chromosome):x = decode(chromosome) # 解码染色体,得到个体参数值fitness_value = x2 # 计算适应度值return fitness_value在这个例子中,我们假设染色体表示一个实数变量x,通过解码染色体,我们可以得到x的数值,然后计算函数f(x)的值作为适应度值。
2. 约束函数法在某些问题中,除了优化目标外,还存在一些额外的约束条件。
适应度函数的计算需要考虑这些约束条件,以保证生成的个体解是可行解。
在这种情况下,我们可以编写约束函数来判断个体解是否满足约束条件,并根据满足程度来计算适应度值。
例如,假设我们要解决一个带约束条件的问题,其中目标函数为f(x) = x^2,约束条件为x >= 0。
遗传算法 c语言代码
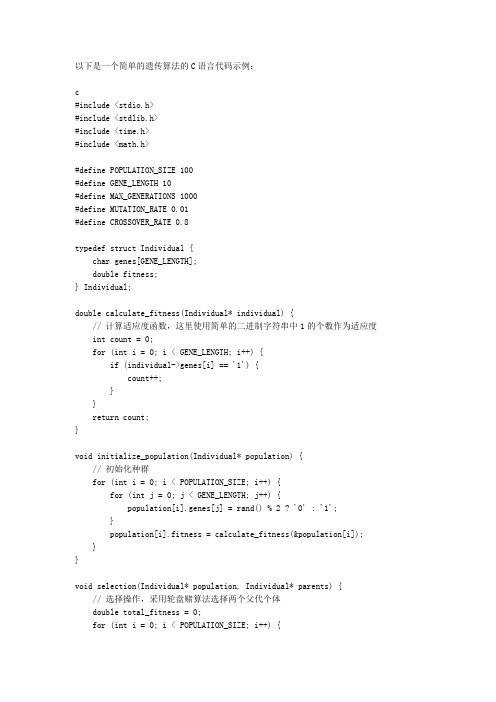
以下是一个简单的遗传算法的C语言代码示例:c#include <stdio.h>#include <stdlib.h>#include <time.h>#include <math.h>#define POPULATION_SIZE 100#define GENE_LENGTH 10#define MAX_GENERATIONS 1000#define MUTATION_RATE 0.01#define CROSSOVER_RATE 0.8typedef struct Individual {char genes[GENE_LENGTH];double fitness;} Individual;double calculate_fitness(Individual* individual) {// 计算适应度函数,这里使用简单的二进制字符串中1的个数作为适应度 int count = 0;for (int i = 0; i < GENE_LENGTH; i++) {if (individual->genes[i] == '1') {count++;}}return count;}void initialize_population(Individual* population) {// 初始化种群for (int i = 0; i < POPULATION_SIZE; i++) {for (int j = 0; j < GENE_LENGTH; j++) {population[i].genes[j] = rand() % 2 ? '0' : '1';}population[i].fitness = calculate_fitness(&population[i]); }}void selection(Individual* population, Individual* parents) {// 选择操作,采用轮盘赌算法选择两个父代个体double total_fitness = 0;for (int i = 0; i < POPULATION_SIZE; i++) {total_fitness += population[i].fitness;}double rand1 = rand() / (double)RAND_MAX * total_fitness;double rand2 = rand() / (double)RAND_MAX * total_fitness;double cumulative_fitness = 0;int parent1_index = -1, parent2_index = -1;for (int i = 0; i < POPULATION_SIZE; i++) {cumulative_fitness += population[i].fitness;if (rand1 < cumulative_fitness && parent1_index == -1) {parent1_index = i;}if (rand2 < cumulative_fitness && parent2_index == -1) {parent2_index = i;}}parents[0] = population[parent1_index];parents[1] = population[parent2_index];}void crossover(Individual* parents, Individual* offspring) {// 交叉操作,采用单点交叉算法生成两个子代个体int crossover_point = rand() % GENE_LENGTH;for (int i = 0; i < crossover_point; i++) {offspring[0].genes[i] = parents[0].genes[i];offspring[1].genes[i] = parents[1].genes[i];}for (int i = crossover_point; i < GENE_LENGTH; i++) {offspring[0].genes[i] = parents[1].genes[i];offspring[1].genes[i] = parents[0].genes[i];}offspring[0].fitness = calculate_fitness(&offspring[0]);offspring[1].fitness = calculate_fitness(&offspring[1]);}void mutation(Individual* individual) {// 变异操作,以一定概率翻转基因位上的值for (int i = 0; i < GENE_LENGTH; i++) {if (rand() / (double)RAND_MAX < MUTATION_RATE) {individual->genes[i] = individual->genes[i] == '0' ? '1' : '0'; }}individual->fitness = calculate_fitness(individual);}void replace(Individual* population, Individual* offspring) {// 替换操作,将两个子代个体中适应度更高的一个替换掉种群中适应度最低的一个个体int worst_index = -1;double worst_fitness = INFINITY;for (int i = 0; i < POPULATION_SIZE; i++) {if (population[i].fitness < worst_fitness) {worst_index = i;worst_fitness = population[i].fitness;}}if (offspring[0].fitness > worst_fitness || offspring[1].fitness > worst_fitness) {if (offspring[0].fitness > offspring[1].fitness) {population[worst_index] = offspring[0];} else {population[worst_index] = offspring[1];}}}。
遗传算法java代码
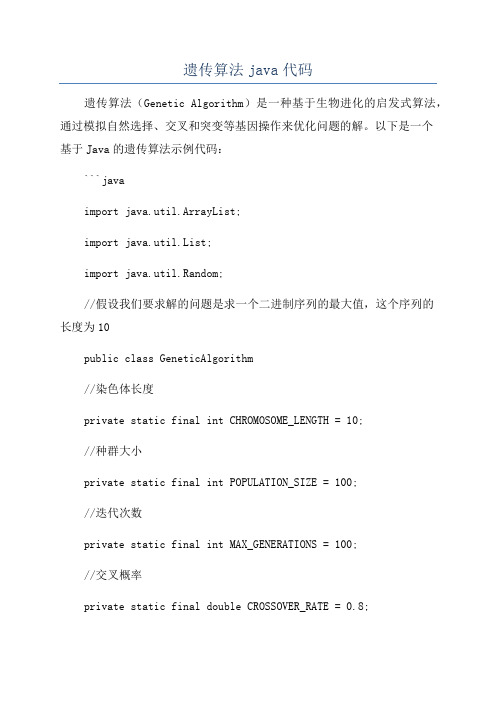
遗传算法java代码遗传算法(Genetic Algorithm)是一种基于生物进化的启发式算法,通过模拟自然选择、交叉和突变等基因操作来优化问题的解。
以下是一个基于Java的遗传算法示例代码:```javaimport java.util.ArrayList;import java.util.List;import java.util.Random;//假设我们要求解的问题是求一个二进制序列的最大值,这个序列的长度为10public class GeneticAlgorithm//染色体长度private static final int CHROMOSOME_LENGTH = 10;//种群大小private static final int POPULATION_SIZE = 100;//迭代次数private static final int MAX_GENERATIONS = 100;//交叉概率private static final double CROSSOVER_RATE = 0.8;//变异概率private static final double MUTATION_RATE = 0.1; //随机数生成器private static final Random RANDOM = new Random(; //个体类private static class Individual//染色体private int[] chromosome;//适应度private double fitness;public Individuachromosome = new int[CHROMOSOME_LENGTH];fitness = 0.0;}//初始化染色体public void initializeChromosomfor (int i = 0; i < CHROMOSOME_LENGTH; i++) chromosome[i] = RANDOM.nextInt(2);}}//计算个体的适应度public void calculateFitnesint decimalValue = 0;for (int i = CHROMOSOME_LENGTH - 1; i >= 0; i--) decimalValue += chromosome[i] * Math.pow(2, CHROMOSOME_LENGTH - i - 1);}fitness = decimalValue;}//获取个体的适应度public double getFitnesreturn fitness;}//获取染色体public int[] getChromosomreturn chromosome;}//设置染色体public void setChromosome(int[] chromosome)this.chromosome = chromosome;}}//生成初始种群private static List<Individual> createInitialPopulatioList<Individual> population = new ArrayList<>(;for (int i = 0; i < POPULATION_SIZE; i++)Individual individual = new Individual(;individual.initializeChromosome(;population.add(individual);}return population;}//选择父母个体private static Individual selectParent(List<Individual> population)double sumFitness = 0.0;for (Individual individual : population)sumFitness += individual.getFitness(;}double randomFitness = RANDOM.nextDouble( * sumFitness;double cumulativeFitness = 0.0;for (Individual individual : population)cumulativeFitness += individual.getFitness(;if (cumulativeFitness > randomFitness)return individual;}}return population.get(0);}//交叉操作private static Individual crossover(Individual parent1, Individual parent2)Individual offspring = new Individual(;int[] parent1Chromosome = parent1.getChromosome(;int[] parent2Chromosome = parent2.getChromosome(;int crossoverPoint = RANDOM.nextInt(CHROMOSOME_LENGTH - 1) + 1;int[] offspringChromosome = new int[CHROMOSOME_LENGTH];System.arraycopy(parent1Chromosome, 0, offspringChromosome, 0, crossoverPoint);System.arraycopy(parent2Chromosome, crossoverPoint, offspringChromosome, crossoverPoint, CHROMOSOME_LENGTH - crossoverPoint);offspring.setChromosome(offspringChromosome);return offspring;}//变异操作private static void mutate(Individual individual)int[] chromosome = individual.getChromosome(;for (int i = 0; i < CHROMOSOME_LENGTH; i++)if (RANDOM.nextDouble( < MUTATION_RATE)chromosome[i] = chromosome[i] == 0 ? 1 : 0;}}individual.setChromosome(chromosome);}//遗传算法主函数public static void main(String[] args)List<Individual> population = createInitialPopulation(;for (int generation = 0; generation < MAX_GENERATIONS; generation++)for (Individual individual : population)individual.calculateFitness(;}Individual bestIndividual = population.get(0);for (Individual individual : population)if (individual.getFitness( > bestIndividual.getFitness() bestIndividual = individual;}}System.out.println("Generation: " + generation + " Best Individual: " + bestIndividual.getFitness();List<Individual> newPopulation = new ArrayList<>(;while (newPopulation.size( < POPULATION_SIZE)Individual parent1 = selectParent(population);Individual parent2 = selectParent(population);Individual offspring = crossover(parent1, parent2);mutate(offspring);newPopulation.add(offspring);}population = newPopulation;}}```以上示例代码实现了一个简单的二进制序列的最大化遗传算法。
python遗传算法代码
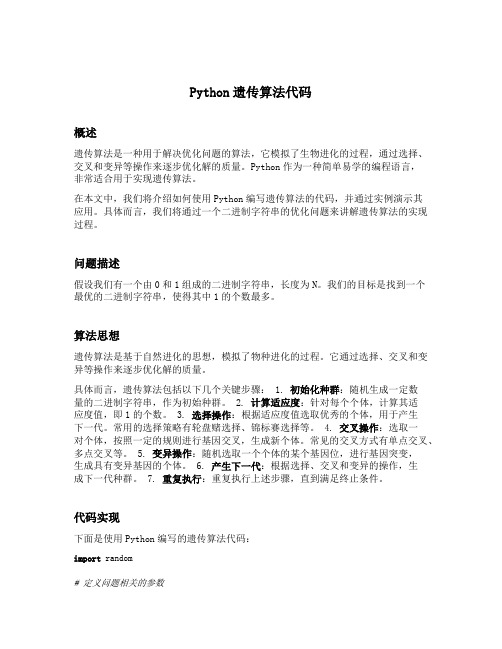
Python遗传算法代码概述遗传算法是一种用于解决优化问题的算法,它模拟了生物进化的过程,通过选择、交叉和变异等操作来逐步优化解的质量。
Python作为一种简单易学的编程语言,非常适合用于实现遗传算法。
在本文中,我们将介绍如何使用Python编写遗传算法的代码,并通过实例演示其应用。
具体而言,我们将通过一个二进制字符串的优化问题来讲解遗传算法的实现过程。
问题描述假设我们有一个由0和1组成的二进制字符串,长度为N。
我们的目标是找到一个最优的二进制字符串,使得其中1的个数最多。
算法思想遗传算法是基于自然进化的思想,模拟了物种进化的过程。
它通过选择、交叉和变异等操作来逐步优化解的质量。
具体而言,遗传算法包括以下几个关键步骤: 1. 初始化种群:随机生成一定数量的二进制字符串,作为初始种群。
2. 计算适应度:针对每个个体,计算其适应度值,即1的个数。
3. 选择操作:根据适应度值选取优秀的个体,用于产生下一代。
常用的选择策略有轮盘赌选择、锦标赛选择等。
4. 交叉操作:选取一对个体,按照一定的规则进行基因交叉,生成新个体。
常见的交叉方式有单点交叉、多点交叉等。
5. 变异操作:随机选取一个个体的某个基因位,进行基因突变,生成具有变异基因的个体。
6. 产生下一代:根据选择、交叉和变异的操作,生成下一代种群。
7. 重复执行:重复执行上述步骤,直到满足终止条件。
代码实现下面是使用Python编写的遗传算法代码:import random# 定义问题相关的参数N = 20 # 二进制串的长度POP_SIZE = 50 # 种群大小GENERATIONS = 100 # 迭代代数SELECT_RATE = 0.2 # 选择概率CROSS_RATE = 0.8 # 交叉概率MUTATE_RATE = 0.01 # 变异概率# 生成初始种群def generate_population(pop_size):return [random.choices([0, 1], k=N) for _ in range(pop_size)]# 计算个体的适应度def fitness(individual):return sum(individual)# 选择操作def select(population, select_rate):fitness_values = [fitness(individual) for individual in population]total_fitness = sum(fitness_values)probabilities = [fitness_value / total_fitness for fitness_value in fitnes s_values]selected_population = random.choices(population, probabilities, k=int(pop_ size * select_rate))return selected_population# 交叉操作def crossover(parent_a, parent_b):cross_point = random.randint(0, N-1)child_a = parent_a[:cross_point] + parent_b[cross_point:]child_b = parent_b[:cross_point] + parent_a[cross_point:]return child_a, child_b# 变异操作def mutate(individual, mutate_rate):mutated_individual = individual.copy()for i in range(N):if random.random() < mutate_rate:mutated_individual[i] = 1 - mutated_individual[i]return mutated_individual# 产生下一代种群def generate_next_population(population, select_rate, cross_rate, mutate_rate): selected_population = select(population, select_rate)next_population = selected_population.copy()while len(next_population) < len(population):parent_a = random.choice(selected_population)parent_b = random.choice(selected_population)if random.random() < cross_rate:child_a, child_b = crossover(parent_a, parent_b)else:child_a, child_b = parent_a, parent_bchild_a = mutate(child_a, mutate_rate)child_b = mutate(child_b, mutate_rate)next_population.append(child_a)next_population.append(child_b)return next_population# 主函数def main():population = generate_population(POP_SIZE)for generation in range(GENERATIONS):population = generate_next_population(population, SELECT_RATE, CROSS_R ATE, MUTATE_RATE)best_individual = max(population, key=fitness)print(f"Generation: {generation}, Best Individual: {best_individual}, Fitness: {fitness(best_individual)}")if __name__ == "__main__":main()实例演示假设我们将二进制串的长度设为20,种群大小为50,迭代代数为100,选择概率为0.2,交叉概率为0.8,变异概率为0.01。
遗传算法matlab实现源程序

附页:一.遗传算法源程序:clc;clear;population;%评价目标函数值for uim=1:popsizevector=population(uim,:);obj(uim)=hanshu(hromlength,vector,phen); end%obj%min(obj)clear uim;objmin=min(obj);for sequ=1:popsizeif obj(sequ)==objminopti=population(sequ,:);endendclear sequ;fmax=22000;%==for gen=1:maxgen%选择操作%将求最小值的函数转化为适应度函数for indivi=1:popsizeobj1(indivi)=1/obj(indivi);endclear indivi;%适应度函数累加总合total=0;for indivi=1:popsizetotal=total+obj1(indivi);endclear indivi;%每条染色体被选中的几率for indivi=1:popsizefitness1(indivi)=obj1(indivi)/total;endclear indivi;%各条染色体被选中的范围for indivi=1:popsizefitness(indivi)=0;for j=1:indivifitness(indivi)=fitness(indivi)+fitness1(j);endendclear j;fitness;%选择适应度高的个体for ranseti=1:popsizeran=rand;while (ran>1||ran<0)ran=rand;endran;if ran<=fitness(1)newpopulation(ranseti,:)=population(1,:);elsefor fet=2:popsizeif (ran>fitness(fet-1))&&(ran<=fitness(fet))newpopulation(ranseti,:)=population(fet,:);endendendendclear ran;newpopulation;%交叉for int=1:2:popsize-1popmoth=newpopulation(int,:);popfath=newpopulation(int+1,:);popcross(int,:)=popmoth;popcross(int+1,:)=popfath;randnum=rand;if(randnum< P>cpoint1=round(rand*hromlength);cpoint2=round(rand*hromlength);while (cpoint2==cpoint1)cpoint2=round(rand*hromlength);endif cpoint1>cpoint2tem=cpoint1;cpoint1=cpoint2;cpoint2=tem;endcpoint1;cpoint2;for term=cpoint1+1:cpoint2for ss=1:hromlengthif popcross(int,ss)==popfath(term)tem1=popcross(int,ss);popcross(int,ss)=popcross(int,term);popcross(int,term)=tem1;endendclear tem1;endfor term=cpoint1+1:cpoint2for ss=1:hromlengthif popcross(int+1,ss)==popmoth(term)tem1=popcross(int+1,ss);popcross(int+1,ss)=popcross(int+1,term);popcross(int+1,term)=tem1;endendclear tem1;endendclear term;endclear randnum;popcross;%变异操作newpop=popcross;for int=1:popsizerandnum=rand;if randnumcpoint12=round(rand*hromlength);cpoint22=round(rand*hromlength);if (cpoint12==0)cpoint12=1;endif (cpoint22==0)cpoint22=1;endwhile (cpoint22==cpoint12)cpoint22=round(rand*hromlength);if cpoint22==0;cpoint22=1;endendtemp=newpop(int,cpoint12);newpop(int,cpoint12)=newpop(int,cpoint22);newpop(int,cpoint22)=temp;endendnewpop;clear cpoint12;clear cpoint22;clear randnum;clear int;for ium=1:popsizevector1=newpop(ium,:);obj1(ium)=hanshu(hromlength,vector1,phen);endclear ium;obj1max=max(obj1);for ar=1:popsizeif obj1(ar)==obj1maxnewpop(ar,:)=opti;endend%遗传操作结束二.粒子群算法源程序:%------初始格式化-------------------------------------------------- clear all;clc;format long;%------给定初始化条件---------------------------------------------- c1=1.4962;%学习因子1c2=1.4962;%学习因子2w=0.7298;%惯性权重MaxDT=100;%最大迭代次数D=2;%搜索空间维数(未知数个数)N=40;%初始化群体个体数目eps=10^(-6);%设置精度(在已知最小值时候用)%------初始化种群的个体(可以在这里限定位置和速度的范围)------------ for i=1:Nfor j=1:Dx(i,j)=randn;%随机初始化位置v(i,j)=randn;%随机初始化速度endend%------先计算各个粒子的适应度,并初始化Pi和Pg---------------------- for i=1:Np(i)=fitness(x(i,:),D);y(i,:)=x(i,:);endpg=x(1,:);%Pg为全局最优for i=2:Nif fitness(x(i,:),D)<FITNESS(pg,D)pg=x(i,:);endend%------进入主要循环,按照公式依次迭代,直到满足精度要求------------ for t=1:MaxDTtfor i=1:Nv(i,:)=w*v(i,:)+c1*rand*(y(i,:)-x(i,:))+c2*rand*(pg-x(i,:)); x(i,:)=x(i,:)+v(i,:);if fitness(x(i,:),D)<p(i)p(i)=fitness(x(i,:),D);y(i,:)=x(i,:);endif p(i)<FITNESS(pg,D)pg=y(i,:);endendPbest(t)=fitness(pg,D);end%------进入主要循环,按照公式依次迭代,直到满足精度要求------------ for t=1:MaxDTfor i=1:Nv(i,:)=w*v(i,:)+c1*rand*(y(i,:)-x(i,:))+c2*rand*(pg-x(i,:));x(i,:)=x(i,:)+v(i,:);if fitness(x(i,:),D)<p(i)p(i)=fitness(x(i,:),D);y(i,:)=x(i,:);endif p(i)<FITNESS(pg,D)pg=y(i,:);endendPbest(t)=fitness(pg,D);end%------最后给出计算结果disp('*************************************************************') disp('函数的全局最优位置为:')Solution=pg'disp('最后得到的优化极值为:')Result=fitness(pg,D)disp('*************************************************************') [X,Y]=meshgrid(-500:2:500);Z=X.*sin(sqrt(X))+Y.*(sin(sqrt(Y)));hold oncontour(X,Y,Z)plot(x(:,1),x(:,2),'*');hold off标准文档实用文案。
遗传算法源代码

这是一个非常简单的遗传算法源代码,是由Denis Cormier (North Carolina State University)开发的,Sita S.Raghavan (University of North Carolina at Charlotte)修正。
代码保证尽可能少,实际上也不必查错。
对一特定的应用修正此代码,用户只需改变常数的定义并且定义“评价函数”即可。
注意代码的设计是求最大值,其中的目标函数只能取正值;且函数值和个体的适应值之间没有区别。
该系统使用比率选择、精华模型、单点杂交和均匀变异。
如果用Gaussian 变异替换均匀变异,可能得到更好的效果。
代码没有任何图形,甚至也没有屏幕输出,主要是保证在平台之间的高可移植性。
读者可以从,目录coe/evol中的文件prog.c中获得。
要求输入的文件应该命名为‘gadata.txt’;系统产生的输出文件为‘galog.txt’。
输入的文件由几行组成:数目对应于变量数。
且每一行提供次序——对应于变量的上下界。
如第一行为第一个变量提供上下界,第二行为第二个变量提供上下界,等等。
/******************************************************************** ******//* This is a simple genetic algorithm implementation where the *//* evaluation function takes positive values only and the *//* fitness of an individual is the same as the value of the *//* objective function *//******************************************************************** ******/#include <stdio.h>#include <stdlib.h>#include <math.h>/* Change any of these parameters to match your needs */#define POPSIZE 50 /* population size */#define MAXGENS 1000 /* max. number of generations */#define NVARS 3 /* no. of problem variables */#define PXOVER 0.8 /* probability of crossover */#define PMUTATION 0.15 /* probability of mutation */#define TRUE 1#define FALSE 0int generation; /* current generation no. */int cur_best; /* best individual */FILE *galog; /* an output file */struct genotype /* genotype (GT), a member of the population */double gene[NVARS]; /* a string of variables */double fitness; /* GT's fitness */double upper[NVARS]; /* GT's variables upper bound */double lower[NVARS]; /* GT's variables lower bound */double rfitness; /* relative fitness */double cfitness; /* cumulative fitness */};struct genotype population[POPSIZE+1]; /* population */struct genotype newpopulation[POPSIZE+1]; /* new population; */ /* replaces the *//* old generation *//* Declaration of procedures used by this genetic algorithm */void initialize(void);double randval(double, double);void evaluate(void);void keep_the_best(void);void elitist(void);void select(void);void crossover(void);void Xover(int,int);void swap(double *, double *);void mutate(void);void report(void);/***************************************************************/ /* Initialization function: Initializes the values of genes */ /* within the variables bounds. It also initializes (to zero) */ /* all fitness values for each member of the population. It */ /* reads upper and lower bounds of each variable from the */ /* input file `gadata.txt'. It randomly generates values */ /* between these bounds for each gene of each genotype in the */ /* population. The format of the input file `gadata.txt' is */ /* var1_lower_bound var1_upper bound */ /* var2_lower_bound var2_upper bound ... */ /***************************************************************/void initialize(void){FILE *infile;int i, j;double lbound, ubound;if ((infile = fopen("gadata.txt","r"))==NULL){fprintf(galog,"\nCannot open input file!\n");exit(1);}/* initialize variables within the bounds */for (i = 0; i < NVARS; i++){fscanf(infile, "%lf",&lbound);fscanf(infile, "%lf",&ubound);for (j = 0; j < POPSIZE; j++){population[j].fitness = 0;population[j].rfitness = 0;population[j].cfitness = 0;population[j].lower[i] = lbound;population[j].upper[i]= ubound;population[j].gene[i] = randval(population[j].lower[i], population[j].upper[i]);}}fclose(infile);}/***********************************************************//* Random value generator: Generates a value within bounds *//***********************************************************/double randval(double low, double high){double val;val = ((double)(rand()%1000)/1000.0)*(high - low) + low;return(val);}/*************************************************************//* Evaluation function: This takes a user defined function. *//* Each time this is changed, the code has to be recompiled. *//* The current function is: x[1]^2-x[1]*x[2]+x[3] *//*************************************************************/void evaluate(void){int mem;int i;double x[NVARS+1];for (mem = 0; mem < POPSIZE; mem++){for (i = 0; i < NVARS; i++)x[i+1] = population[mem].gene[i];population[mem].fitness = (x[1]*x[1]) - (x[1]*x[2]) + x[3];}}/***************************************************************//* Keep_the_best function: This function keeps track of the *//* best member of the population. Note that the last entry in *//* the array Population holds a copy of the best individual *//***************************************************************/void keep_the_best(){int mem;int i;cur_best = 0; /* stores the index of the best individual */for (mem = 0; mem < POPSIZE; mem++){if (population[mem].fitness > population[POPSIZE].fitness){cur_best = mem;population[POPSIZE].fitness = population[mem].fitness;}}/* once the best member in the population is found, copy the genes */ for (i = 0; i < NVARS; i++)population[POPSIZE].gene[i] = population[cur_best].gene[i];}/****************************************************************//* Elitist function: The best member of the previous generation */ /* is stored as the last in the array. If the best member of */ /* the current generation is worse then the best member of the */ /* previous generation, the latter one would replace the worst */ /* member of the current population */ /****************************************************************/void elitist(){int i;double best, worst; /* best and worst fitness values */ int best_mem, worst_mem; /* indexes of the best and worst member */best = population[0].fitness;worst = population[0].fitness;for (i = 0; i < POPSIZE - 1; ++i){if(population[i].fitness > population[i+1].fitness){if (population[i].fitness >= best){best = population[i].fitness;best_mem = i;}if (population[i+1].fitness <= worst){worst = population[i+1].fitness;worst_mem = i + 1;}}else{if (population[i].fitness <= worst){worst = population[i].fitness;worst_mem = i;}if (population[i+1].fitness >= best){best = population[i+1].fitness;best_mem = i + 1;}}}/* if best individual from the new population is better than *//* the best individual from the previous population, then *//* copy the best from the new population; else replace the *//* worst individual from the current population with the *//* best one from the previous generation */if (best >= population[POPSIZE].fitness){for (i = 0; i < NVARS; i++)population[POPSIZE].gene[i] = population[best_mem].gene[i]; population[POPSIZE].fitness = population[best_mem].fitness;}else{for (i = 0; i < NVARS; i++)population[worst_mem].gene[i] = population[POPSIZE].gene[i]; population[worst_mem].fitness = population[POPSIZE].fitness;}}/**************************************************************//* Selection function: Standard proportional selection for *//* maximization problems incorporating elitist model - makes *//* sure that the best member survives *//**************************************************************/void select(void){int mem, i, j, k;double sum = 0;double p;/* find total fitness of the population */for (mem = 0; mem < POPSIZE; mem++){sum += population[mem].fitness;}/* calculate relative fitness */for (mem = 0; mem < POPSIZE; mem++){population[mem].rfitness = population[mem].fitness/sum;}population[0].cfitness = population[0].rfitness;/* calculate cumulative fitness */for (mem = 1; mem < POPSIZE; mem++){population[mem].cfitness = population[mem-1].cfitness + population[mem].rfitness;}/* finally select survivors using cumulative fitness. */for (i = 0; i < POPSIZE; i++){p = rand()%1000/1000.0;if (p < population[0].cfitness)newpopulation[i] = population[0];else{for (j = 0; j < POPSIZE;j++)if (p >= population[j].cfitness &&p<population[j+1].cfitness)newpopulation[i] = population[j+1];}}/* once a new population is created, copy it back */for (i = 0; i < POPSIZE; i++)population[i] = newpopulation[i];}/***************************************************************//* Crossover selection: selects two parents that take part in *//* the crossover. Implements a single point crossover *//***************************************************************/void crossover(void){int i, mem, one;int first = 0; /* count of the number of members chosen */double x;for (mem = 0; mem < POPSIZE; ++mem){x = rand()%1000/1000.0;if (x < PXOVER){++first;if (first % 2 == 0)Xover(one, mem);elseone = mem;}}}/**************************************************************/ /* Crossover: performs crossover of the two selected parents. */ /**************************************************************/void Xover(int one, int two){int i;int point; /* crossover point *//* select crossover point */if(NVARS > 1){if(NVARS == 2)point = 1;elsepoint = (rand() % (NVARS - 1)) + 1;for (i = 0; i < point; i++)swap(&population[one].gene[i], &population[two].gene[i]);}}/*************************************************************/ /* Swap: A swap procedure that helps in swapping 2 variables */ /*************************************************************/void swap(double *x, double *y){double temp;temp = *x;*x = *y;*y = temp;}/**************************************************************//* Mutation: Random uniform mutation. A variable selected for *//* mutation is replaced by a random value between lower and *//* upper bounds of this variable *//**************************************************************/void mutate(void){int i, j;double lbound, hbound;double x;for (i = 0; i < POPSIZE; i++)for (j = 0; j < NVARS; j++){x = rand()%1000/1000.0;if (x < PMUTATION){/* find the bounds on the variable to be mutated */ lbound = population[i].lower[j];hbound = population[i].upper[j];population[i].gene[j] = randval(lbound, hbound);}}}/***************************************************************//* Report function: Reports progress of the simulation. Data *//* dumped into the output file are separated by commas *//***************************************************************/void report(void){int i;double best_val; /* best population fitness */double avg; /* avg population fitness */double stddev; /* std. deviation of population fitness */ double sum_square; /* sum of square for std. calc */double square_sum; /* square of sum for std. calc */double sum; /* total population fitness */sum = 0.0;sum_square = 0.0;for (i = 0; i < POPSIZE; i++){sum += population[i].fitness;sum_square += population[i].fitness * population[i].fitness; }avg = sum/(double)POPSIZE;square_sum = avg * avg * POPSIZE;stddev = sqrt((sum_square - square_sum)/(POPSIZE - 1));best_val = population[POPSIZE].fitness;fprintf(galog, "\n%5d, %6.3f, %6.3f, %6.3f \n\n", generation, best_val, avg, stddev);}/**************************************************************//* Main function: Each generation involves selecting the best *//* members, performing crossover & mutation and then *//* evaluating the resulting population, until the terminating *//* condition is satisfied *//**************************************************************/void main(void){int i;if ((galog = fopen("galog.txt","w"))==NULL){exit(1);}generation = 0;fprintf(galog, "\n generation best average standard \n");fprintf(galog, " number value fitness deviation \n");initialize();evaluate();keep_the_best();while(generation<MAXGENS){generation++;select();crossover();mutate();report();evaluate();elitist();}fprintf(galog,"\n\n Simulation completed\n");fprintf(galog,"\n Best member: \n");for (i = 0; i < NVARS; i++){fprintf (galog,"\n var(%d) = %3.3f",i,population[POPSIZE].gene[i]); }fprintf(galog,"\n\n Best fitness = %3.3f",population[POPSIZE].fitness); fclose(galog);printf("Success\n");}/***************************************************************//******************************************************************** ******//* This is a simple genetic algorithm implementation where the *//* evaluation function takes positive values only and the *//* fitness of an individual is the same as the value of the *//* objective function *//******************************************************************** ******/#include <stdio.h>#include <stdlib.h>#include <math.h>/* Change any of these parameters to match your needs */#define POPSIZE 50 /* population size */#define MAXGENS 1000 /* max. number of generations */#define NVARS 3 /* no. of problem variables */#define PXOVER 0.8 /* probability of crossover */#define PMUTATION 0.15 /* probability of mutation */#define TRUE 1#define FALSE 0int generation; /* current generation no. */int cur_best; /* best individual */FILE *galog; /* an output file */struct genotype /* genotype (GT), a member of the population */ {double gene[NVARS]; /* a string of variables */double fitness; /* GT's fitness */double upper[NVARS]; /* GT's variables upper bound */double lower[NVARS]; /* GT's variables lower bound */double rfitness; /* relative fitness */double cfitness; /* cumulative fitness */};struct genotype population[POPSIZE+1]; /* population */struct genotype newpopulation[POPSIZE+1]; /* new population; */ /* replaces the *//* old generation *//* Declaration of procedures used by this genetic algorithm */void initialize(void);double randval(double, double);void evaluate(void);void keep_the_best(void);void elitist(void);void select(void);void crossover(void);void Xover(int,int);void swap(double *, double *);void mutate(void);void report(void);/***************************************************************/ /* Initialization function: Initializes the values of genes */ /* within the variables bounds. It also initializes (to zero) */ /* all fitness values for each member of the population. It */ /* reads upper and lower bounds of each variable from the */ /* input file `gadata.txt'. It randomly generates values */ /* between these bounds for each gene of each genotype in the */ /* population. The format of the input file `gadata.txt' is */ /* var1_lower_bound var1_upper bound */ /* var2_lower_bound var2_upper bound ... */ /***************************************************************/void initialize(void){FILE *infile;int i, j;double lbound, ubound;if ((infile = fopen("gadata.txt","r"))==NULL){fprintf(galog,"\nCannot open input file!\n");exit(1);}/* initialize variables within the bounds */for (i = 0; i < NVARS; i++){fscanf(infile, "%lf",&lbound);fscanf(infile, "%lf",&ubound);for (j = 0; j < POPSIZE; j++){population[j].fitness = 0;population[j].rfitness = 0;population[j].cfitness = 0;population[j].lower[i] = lbound;population[j].upper[i]= ubound;population[j].gene[i] = randval(population[j].lower[i], population[j].upper[i]);}}fclose(infile);}/***********************************************************//* Random value generator: Generates a value within bounds *//***********************************************************/double randval(double low, double high){double val;val = ((double)(rand()%1000)/1000.0)*(high - low) + low;return(val);}/*************************************************************//* Evaluation function: This takes a user defined function. *//* Each time this is changed, the code has to be recompiled. *//* The current function is: x[1]^2-x[1]*x[2]+x[3] *//*************************************************************/void evaluate(void){int mem;int i;double x[NVARS+1];for (mem = 0; mem < POPSIZE; mem++){for (i = 0; i < NVARS; i++)x[i+1] = population[mem].gene[i];population[mem].fitness = (x[1]*x[1]) - (x[1]*x[2]) + x[3];}}/***************************************************************//* Keep_the_best function: This function keeps track of the *//* best member of the population. Note that the last entry in *//* the array Population holds a copy of the best individual *//***************************************************************/void keep_the_best(){int mem;int i;cur_best = 0; /* stores the index of the best individual */for (mem = 0; mem < POPSIZE; mem++){if (population[mem].fitness > population[POPSIZE].fitness){cur_best = mem;population[POPSIZE].fitness = population[mem].fitness;}}/* once the best member in the population is found, copy the genes */ for (i = 0; i < NVARS; i++)population[POPSIZE].gene[i] = population[cur_best].gene[i];}/****************************************************************/ /* Elitist function: The best member of the previous generation */ /* is stored as the last in the array. If the best member of */ /* the current generation is worse then the best member of the */ /* previous generation, the latter one would replace the worst */ /* member of the current population */ /****************************************************************/void elitist(){int i;double best, worst; /* best and worst fitness values */ int best_mem, worst_mem; /* indexes of the best and worst member */best = population[0].fitness;worst = population[0].fitness;for (i = 0; i < POPSIZE - 1; ++i){if(population[i].fitness > population[i+1].fitness){if (population[i].fitness >= best){best = population[i].fitness;best_mem = i;}if (population[i+1].fitness <= worst){worst = population[i+1].fitness;worst_mem = i + 1;}}else{if (population[i].fitness <= worst){worst = population[i].fitness;worst_mem = i;}if (population[i+1].fitness >= best){best = population[i+1].fitness;best_mem = i + 1;}}}/* if best individual from the new population is better than *//* the best individual from the previous population, then *//* copy the best from the new population; else replace the *//* worst individual from the current population with the *//* best one from the previous generation */if (best >= population[POPSIZE].fitness){for (i = 0; i < NVARS; i++)population[POPSIZE].gene[i] = population[best_mem].gene[i]; population[POPSIZE].fitness = population[best_mem].fitness;}else{for (i = 0; i < NVARS; i++)population[worst_mem].gene[i] = population[POPSIZE].gene[i]; population[worst_mem].fitness = population[POPSIZE].fitness;}}/**************************************************************//* Selection function: Standard proportional selection for *//* maximization problems incorporating elitist model - makes *//* sure that the best member survives *//**************************************************************/void select(void){int mem, i, j, k;double sum = 0;double p;/* find total fitness of the population */for (mem = 0; mem < POPSIZE; mem++){sum += population[mem].fitness;}/* calculate relative fitness */for (mem = 0; mem < POPSIZE; mem++){population[mem].rfitness = population[mem].fitness/sum;}population[0].cfitness = population[0].rfitness;/* calculate cumulative fitness */for (mem = 1; mem < POPSIZE; mem++){population[mem].cfitness = population[mem-1].cfitness + population[mem].rfitness;}/* finally select survivors using cumulative fitness. */for (i = 0; i < POPSIZE; i++){p = rand()%1000/1000.0;if (p < population[0].cfitness)newpopulation[i] = population[0];else{for (j = 0; j < POPSIZE;j++)if (p >= population[j].cfitness &&p<population[j+1].cfitness)newpopulation[i] = population[j+1];}}/* once a new population is created, copy it back */for (i = 0; i < POPSIZE; i++)population[i] = newpopulation[i];}/***************************************************************//* Crossover selection: selects two parents that take part in *//* the crossover. Implements a single point crossover *//***************************************************************/void crossover(void){int i, mem, one;int first = 0; /* count of the number of members chosen */double x;for (mem = 0; mem < POPSIZE; ++mem){x = rand()%1000/1000.0;if (x < PXOVER){++first;if (first % 2 == 0)Xover(one, mem);elseone = mem;}}}/**************************************************************/ /* Crossover: performs crossover of the two selected parents. */ /**************************************************************/void Xover(int one, int two){int i;int point; /* crossover point *//* select crossover point */if(NVARS > 1){if(NVARS == 2)point = 1;elsepoint = (rand() % (NVARS - 1)) + 1;for (i = 0; i < point; i++)swap(&population[one].gene[i], &population[two].gene[i]);}}/*************************************************************/ /* Swap: A swap procedure that helps in swapping 2 variables */ /*************************************************************/void swap(double *x, double *y){double temp;temp = *x;*x = *y;*y = temp;}/**************************************************************//* Mutation: Random uniform mutation. A variable selected for *//* mutation is replaced by a random value between lower and *//* upper bounds of this variable *//**************************************************************/void mutate(void){int i, j;double lbound, hbound;double x;for (i = 0; i < POPSIZE; i++)for (j = 0; j < NVARS; j++){x = rand()%1000/1000.0;if (x < PMUTATION){/* find the bounds on the variable to be mutated */ lbound = population[i].lower[j];hbound = population[i].upper[j];population[i].gene[j] = randval(lbound, hbound);}}}/***************************************************************//* Report function: Reports progress of the simulation. Data *//* dumped into the output file are separated by commas *//***************************************************************/void report(void){int i;double best_val; /* best population fitness */double avg; /* avg population fitness */double stddev; /* std. deviation of population fitness */ double sum_square; /* sum of square for std. calc */double square_sum; /* square of sum for std. calc */double sum; /* total population fitness */sum = 0.0;sum_square = 0.0;for (i = 0; i < POPSIZE; i++){sum += population[i].fitness;sum_square += population[i].fitness * population[i].fitness; }avg = sum/(double)POPSIZE;square_sum = avg * avg * POPSIZE;stddev = sqrt((sum_square - square_sum)/(POPSIZE - 1));best_val = population[POPSIZE].fitness;fprintf(galog, "\n%5d, %6.3f, %6.3f, %6.3f \n\n", generation, best_val, avg, stddev);}/**************************************************************//* Main function: Each generation involves selecting the best *//* members, performing crossover & mutation and then *//* evaluating the resulting population, until the terminating *//* condition is satisfied *//**************************************************************/void main(void){int i;if ((galog = fopen("galog.txt","w"))==NULL){exit(1);}generation = 0;fprintf(galog, "\n generation best average standard \n");fprintf(galog, " number value fitness deviation \n");initialize();evaluate();keep_the_best();while(generation<MAXGENS){generation++;select();范文范例参考指导crossover();mutate();report();evaluate();elitist();}fprintf(galog,"\n\n Simulation completed\n");fprintf(galog,"\n Best member: \n");for (i = 0; i < NVARS; i++){fprintf (galog,"\n var(%d) = %3.3f",i,population[POPSIZE].gene[i]); }fprintf(galog,"\n\n Best fitness = %3.3f",population[POPSIZE].fitness); fclose(galog);printf("Success\n");}/***************************************************************/word格式整理。
自适应遗传算法代码

/*华南理工大学电子与信息学院苏勇2004年4月*/
/**********************************************************************/
void freeall(),nomemory(char *),report();
void writepop(),writechrom(unsigned *);
void preselect();
void statistics(struct individual *);
void title(),repchar (FILE *,char *,int);
initpop();
statistics(oldpop);
initreport();
}
void initdata() /*遗传算法参数输入*/
{
char answer[2];
popsize=30;
if((popsize%2) != 0)
{
fprintf(outfp, "种群大小已设置为偶数\n");
int generation; /*最佳个体生成代*/
};
struct individual *oldpop; /*当前代种群*/
struct individual *newpop; /*新一代种群*/
struct bestever bestfit; /*最佳个体*/
double sumfitness; /*种群中个体适应度累计*/
nomemory("newpop chromosomes");
遗传算法代码
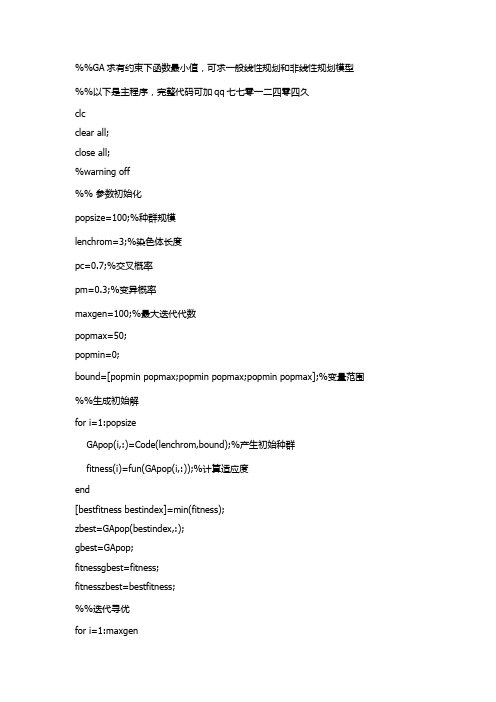
%%GA求有约束下函数最小值,可求一般线性规划和非线性规划模型%%以下是主程序,完整代码可加qq七七零一二四零四久clcclear all;close all;%warning off%% 参数初始化popsize=100;%种群规模lenchrom=3;%染色体长度pc=0.7;%交叉概率pm=0.3;%变异概率maxgen=100;%最大迭代代数popmax=50;popmin=0;bound=[popmin popmax;popmin popmax;popmin popmax];%变量范围%%生成初始解for i=1:popsizeGApop(i,:)=Code(lenchrom,bound);%产生初始种群fitness(i)=fun(GApop(i,:));%计算适应度end[bestfitness bestindex]=min(fitness);zbest=GApop(bestindex,:);gbest=GApop;fitnessgbest=fitness;fitnesszbest=bestfitness;%%迭代寻优for i=1:maxgenGApop=Select(GApop,fitness,popsize);GApop=Cross(pc,lenchrom,GApop,popsize,bound);%交叉操作GApop=Mutation(pm,lenchrom,GApop,popsize,[i,maxgen],bound);%变异操作 pop=GApop;for j=1:popsizeif 1.0*pop(j,1)-1.0*pop(j,2)+1.0*pop(j,3)<=20if 3*pop(j,1)+2*pop(j,2)+4*pop(j,3)<=42if 3*pop(j,1)+2*pop(j,2)<=30fitness(j)=fun(pop(j,:));%适应度值endendendif fitness(j)<fitnessgbest%个体最优更新gbest(j,:)=pop(j,:);fitnessgbest=fitness(j);endif fitness(j)<fitnesszbest%种群最优更新zbest=pop(j,:);fitnesszbest=fitness(j);endendyy(i)=fitnesszbest;end%% 结果disp '***************best particle number******************'zbest%%plot(yy,'linewidth',2);title(['适应度曲线' '终止代数=' num2str(maxgen)]); xlabel('进化代数');ylabel('适应度');grid on%%输出结果子程序如下。
遗传算法Matlab源代码
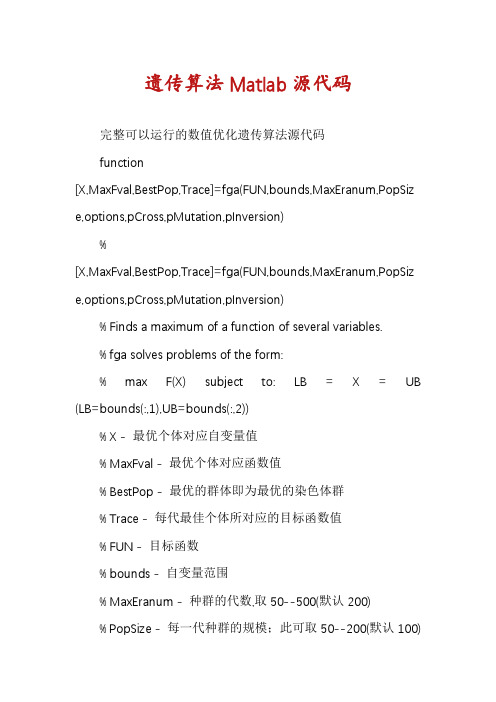
遗传算法Matlab源代码完整可以运行的数值优化遗传算法源代码function[X,MaxFval,BestPop,Trace]=fga(FUN,bounds,MaxEranum,PopSiz e,options,pCross,pMutation,pInversion)%[X,MaxFval,BestPop,Trace]=fga(FUN,bounds,MaxEranum,PopSiz e,options,pCross,pMutation,pInversion)% Finds a maximum of a function of several variables.% fga solves problems of the form:% max F(X) subject to: LB = X = UB (LB=bounds(:,1),UB=bounds(:,2))% X - 最优个体对应自变量值% MaxFval - 最优个体对应函数值% BestPop - 最优的群体即为最优的染色体群% Trace - 每代最佳个体所对应的目标函数值% FUN - 目标函数% bounds - 自变量范围% MaxEranum - 种群的代数,取50--500(默认200)% PopSize - 每一代种群的规模;此可取50--200(默认100)% pCross - 交叉概率,一般取0.5--0.85之间较好(默认0.8)% pMutation - 初始变异概率,一般取0.05-0.2之间较好(默认0.1)% pInversion - 倒位概率,一般取0.05-0.3之间较好(默认0.2) % options - 1*2矩阵,options(1)=0二进制编码(默认0),option(1)~=0十进制编码,option(2)设定求解精度(默认1e-4)T1=clock;%检验初始参数if nargin2, error('FMAXGA requires at least three input arguments'); endif nargin==2, MaxEranum=150;PopSize=100;options=[1 1e-4];pCross=0.85;pMutation=0.1;pInversion=0.25;endif nargin==3, PopSize=100;options=[1 1e-4];pCross=0.85;pMutation=0.1;pInversion=0.25;endif nargin==4, options=[1 1e-4];pCross=0.85;pMutation=0.1;pInversion=0.25;endif nargin==5, pCross=0.85;pMutation=0.1;pInversion=0.25;endif nargin==6, pMutation=0.1;pInversion=0.25;endif nargin==7, pInversion=0.25;endif (options(1)==0|options(1)==1)find((bounds(:,1)-bounds(:,2))0)error('数据输入错误,请重新输入:');end% 定义全局变量global m n NewPop children1 children2 VarNum% 初始化种群和变量precision = options(2);bits = ceil(log2((bounds(:,2)-bounds(:,1))' ./ precision));%由设定精度划分区间VarNum = size(bounds,1);[Pop] = InitPop(PopSize,bounds,bits,options);%初始化种群[m,n] = size(Pop);fit = zeros(1,m);NewPop = zeros(m,n);children1 = zeros(1,n);children2 = zeros(1,n);pm0 = pMutation;BestPop = zeros(MaxEranum,n);%分配初始解空间BestPop,TraceTrace = zeros(1,MaxEranum);完整可以运行的数值优化遗传算法源代码Lb = ones(PopSize,1)*bounds(:,1)';Ub = ones(PopSize,1)*bounds(:,2)';%二进制编码采用多点交叉和均匀交叉,并逐步增大均匀交叉概率%浮点编码采用离散交叉(前期)、算术交叉(中期)、AEA重组(后期)OptsCrossOver = [ones(1,MaxEranum)*options(1);...round(unidrnd(2*(MaxEranum-[1:MaxEranum]))/MaxEranum)]';%浮点编码时采用两种自适应变异和一种随机变异(自适应变异发生概率为随机变异发生的2倍)OptsMutation = [ones(1,MaxEranum)*options(1);unidrnd(5,1,MaxEranum)]';if options(1)==3D=zeros(n);CityPosition=bounds;D = sqrt((CityPosition(:, ones(1,n)) - CityPosition(:, ones(1,n))').^2 +...(CityPosition(:,2*ones(1,n)) - CityPosition(:,2*ones(1,n))').^2 );end%========================================================================== % 进化主程序%%===================================== ===================================== eranum = 1;H=waitbar(0,'Please wait...');while(eranum=MaxEranum)for j=1:mif options(1)==1%eval(['[fit(j)]=' FUN '(Pop(j,:));']);%但执行字符串速度比直接计算函数值慢fit(j)=feval(FUN,Pop(j,:));%计算适应度elseif options(1)==0%eval(['[fit(j)]=' FUN '(b2f(Pop(j,:),bounds,bits));']);fit(j)=feval(FUN,(b2f(Pop(j,:),bounds,bits)));elsefit(j)=-feval(FUN,Pop(j,:),D);endend[Maxfit,fitIn]=max(fit);%得到每一代最大适应值Meanfit(eranum)=mean(fit);BestPop(eranum,:)=Pop(fitIn,:);Trace(eranum)=Maxfit;if options(1)==1Pop=(Pop-Lb)./(Ub-Lb);%将定义域映射到[0,1]:[Lb,Ub]--[0,1] ,Pop--(Pop-Lb)./(Ub-Lb)endswitch round(unifrnd(0,eranum/MaxEranum))%进化前期尽量使用实行锦标赛选择,后期逐步增大非线性排名选择case {0} [selectpop]=TournamentSelect(Pop,fit,bits);%锦标赛选择case {1}[selectpop]=NonlinearRankSelect(Pop,fit,bits);%非线性排名选择end完整可以运行的数值优化遗传算法源代码[CrossOverPop]=CrossOver(selectpop,pCross,OptsCrossOver(er anum,:));%交叉[MutationPop]=Mutation(CrossOverPop,fit,pMutation,VarNum,O ptsMutation(eranum,:)); %变异[InversionPop]=Inversion(MutationPop,pInversion);%倒位%更新种群if options(1)==1Pop=Lb+InversionPop.*(Ub-Lb);%还原PopelsePop=InversionPop;endpMutation=pm0+(eranum^3)*(pCross/2-pm0)/(eranum^4); %逐步增大变异率至1/2交叉率percent=num2str(round(100*eranum/MaxEranum));waitbar(eranum/MaxEranum,H,['Evolution complete ',percent,'%']);eranum=eranum+1;endclose(H);% 格式化输出进化结果和解的变化情况t=1:MaxEranum;plot(t,Trace,t,Meanfit);legend('解的变化','种群的变化');title('函数优化的遗传算法');xlabel('进化世代数');ylabel('每一代最优适应度');[MaxFval,MaxFvalIn]=max(Trace);if options(1)==1|options(1)==3X=BestPop(MaxFvalIn,:);elseif options(1)==0X=b2f(BestPop(MaxFvalIn,:),bounds,bits);endhold on;plot(MaxFvalIn,MaxFval,'*');text(MaxFvalIn+5,MaxFval,['FMAX=' num2str(MaxFval)]);str1=sprintf(' Best generation:\n %d\n\n Best X:\n %s\n\n MaxFval\n %f\n',...MaxFvalIn,num2str(X),MaxFval);disp(str1);% -计时T2=clock;elapsed_time=T2-T1;if elapsed_time(6)0elapsed_time(6)=elapsed_time(6)+60;elapsed_time(5)=elapsed_time(5)-1;endif elapsed_time(5)0elapsed_time(5)=elapsed_time(5)+60;elapsed_time(4)=elapsed_t ime(4)-1;end完整可以运行的数值优化遗传算法源代码str2=sprintf('elapsed_time\n %d (h) %d (m) %.4f (s)',elapsed_time(4),elapsed_time(5),elapsed_time(6));disp(str2);%===================================== ===================================== % 遗传操作子程序%%===================================== ===================================== % -- 初始化种群--% 采用浮点编码和二进制Gray编码(为了克服二进制编码的Hamming悬崖缺点)function [initpop]=InitPop(popsize,bounds,bits,options)numVars=size(bounds,1);%变量数目rang=(bounds(:,2)-bounds(:,1))';%变量范围if options(1)==1initpop=zeros(popsize,numVars);initpop=(ones(popsize,1)*rang).*(rand(popsize,numVars))+(ones (popsize,1)*bounds(:,1)');elseif options(1)==0precision=options(2);%由求解精度确定二进制编码长度len=sum(bits);initpop=zeros(popsize,len);%The whole zero encoding individualfor i=2:popsize-1pop=round(rand(1,len));pop=mod(([0 pop]+[pop 0]),2);%i=1时,b(1)=a(1);i1时,b(i)=mod(a(i-1)+a(i),2)%其中原二进制串:a(1)a(2)...a(n),Gray串:b(1)b(2)...b(n)initpop(i,:)=pop(1:end-1);endinitpop(popsize,:)=ones(1,len);%The whole one encoding individualelsefor i=1:popsizeinitpop(i,:)=randperm(numVars);%为Tsp问题初始化种群endend% -- 二进制串解码--function [fval] = b2f(bval,bounds,bits)% fval - 表征各变量的十进制数% bval - 表征各变量的二进制编码串% bounds - 各变量的取值范围% bits - 各变量的二进制编码长度scale=(bounds(:,2)-bounds(:,1))'./(2.^bits-1); %The range of the variablesnumV=size(bounds,1);cs=[0 cumsum(bits)];for i=1:numVa=bval((cs(i)+1):cs(i+1));fval(i)=sum(2.^(size(a,2)-1:-1:0).*a)*scale(i)+bounds(i,1);end% -- 选择操作--完整可以运行的数值优化遗传算法源代码% 采用基于轮盘赌法的非线性排名选择% 各个体成员按适应值从大到小分配选择概率:% P(i)=(q/1-(1-q)^n)*(1-q)^i, 其中P(0)P(1)...P(n), sum(P(i))=1function [NewPop]=NonlinearRankSelect(OldPop,fit,bits) global m n NewPopfit=fit';selectprob=fit/sum(fit);%计算各个体相对适应度(0,1)q=max(selectprob);%选择最优的概率x=zeros(m,2);x(:,1)=[m:-1:1]';[y x(:,2)]=sort(selectprob);r=q/(1-(1-q)^m);%标准分布基值newfit(x(:,2))=r*(1-q).^(x(:,1)-1);%生成选择概率newfit=[0 cumsum(newfit)];%计算各选择概率之和rNums=rand(m,1);newIn=1;while(newIn=m)NewPop(newIn,:)=OldPop(length(find(rNums(newIn)newfit)),:);newIn=newIn+1;end% -- 锦标赛选择(含精英选择) --function [NewPop]=TournamentSelect(OldPop,fit,bits)global m n NewPopnum=floor(m./2.^(1:10));num(find(num==0))=[];L=length(num);a=sum(num);b=m-a;PopIn=1;while(PopIn=L)r=unidrnd(m,num(PopIn),2^PopIn);[LocalMaxfit,In]=max(fit(r),[],2);SelectIn=r((In-1)*num(PopIn)+[1:num(PopIn)]');NewPop(sum(num(1:PopIn))-num(PopIn)+1:sum(num(1:PopIn)),:)=OldPop(SelectIn,:);PopIn=PopIn+1;r=[];In=[];LocalMaxfit=[];endif b1NewPop((sum(num)+1):(sum(num)+b-1),:)=OldPop(unidrnd(m,1,b-1),:);end[GlobalMaxfit,I]=max(fit);%保留每一代中最佳个体NewPop(end,:)=OldPop(I,:);% -- 交叉操作--function [NewPop]=CrossOver(OldPop,pCross,opts)global m n NewPopr=rand(1,m);完整可以运行的数值优化遗传算法源代码y1=find(rpCross);y2=find(r=pCross);len=length(y1);if len==1|(len2mod(len,2)==1)%如果用来进行交叉的染色体的条数为奇数,将其调整为偶数y2(length(y2)+1)=y1(len);y1(len)=[];endi=0;if length(y1)=2if opts(1)==1%浮点编码交叉while(i=length(y1)-2)NewPop(y1(i+1),:)=OldPop(y1(i+1),:);NewPop(y1(i+2),:)=OldPop(y1(i+2),:);if opts(2)==0n1%discret crossoverPoints=sort(unidrnd(n,1,2));NewPop(y1(i+1),Points(1):Points(2))=OldPop(y1(i+2),Points(1):Po ints(2));NewPop(y1(i+2),Points(1):Points(2))=OldPop(y1(i+1),Points(1):Po ints(2));elseif opts(2)==1%arithmetical crossoverPoints=round(unifrnd(0,pCross,1,n));CrossPoints=find(Points==1);r=rand(1,length(CrossPoints));NewPop(y1(i+1),CrossPoints)=r.*OldPop(y1(i+1),CrossPoints)+(1 -r).*OldPop(y1(i+2),CrossPoints);NewPop(y1(i+2),CrossPoints)=r.*OldPop(y1(i+2),CrossPoints)+(1 -r).*OldPop(y1(i+1),CrossPoints); else %AEA recombination Points=round(unifrnd(0,pCross,1,n));CrossPoints=find(Points==1);v=unidrnd(4,1,2);NewPop(y1(i+1),CrossPoints)=(floor(10^v(1)*OldPop(y1(i+1),Cro ssPoints))+...10^v(1)*OldPop(y1(i+2),CrossPoints)-floor(10^v(1)*OldPop(y1(i+2),CrossPoints)))/10^v(1);NewPop(y1(i+2),CrossPoints)=(floor(10^v(2)*OldPop(y1(i+2),Cro ssPoints))+...10^v(2)*OldPop(y1(i+1),CrossPoints)-floor(10^v(2)*OldPop(y1(i+1),CrossPoints)))/10^v(2);endi=i+2;endelseif opts(1)==0%二进制编码交叉while(i=length(y1)-2)if opts(2)==0[NewPop(y1(i+1),:),NewPop(y1(i+2),:)]=EqualCrossOver(OldPop( y1(i+1),:),OldPop(y1(i+2),:)); else[NewPop(y1(i+1),:),NewPop(y1(i+2),:)]=MultiPointCross(OldPop( y1(i+1),:),OldPop(y1(i+2),:)); endi=i+2;endelse %Tsp问题次序杂交for i=0:2:length(y1)-2xPoints=sort(unidrnd(n,1,2));NewPop([y1(i+1)y1(i+2)],xPoints(1):xPoints(2))=OldPop([y1(i+2)y1(i+1)],xPoints(1):xPoints(2));完整可以运行的数值优化遗传算法源代码%NewPop(y1(i+2),xPoints(1):xPoints(2))=OldPop(y1(i+1),xPo ints(1):xPoints(2));temp=[OldPop(y1(i+1),xPoints(2)+1:n)OldPop(y1(i+1),1:xPoints(2))];for del1i=xPoints(1):xPoints(2)temp(find(temp==OldPop(y1(i+2),del1i)))=[];endNewPop(y1(i+1),(xPoints(2)+1):n)=temp(1:(n-xPoints(2)));NewPop(y1(i+1),1:(xPoints(1)-1))=temp((n-xPoints(2)+1):end);temp=[OldPop(y1(i+2),xPoints(2)+1:n)OldPop(y1(i+2),1:xPoints(2))];for del2i=xPoints(1):xPoints(2)temp(find(temp==OldPop(y1(i+1),del2i)))=[];endNewPop(y1(i+2),(xPoints(2)+1):n)=temp(1:(n-xPoints(2)));NewPop(y1(i+2),1:(xPoints(1)-1))=temp((n-xPoints(2)+1):end);endendendNewPop(y2,:)=OldPop(y2,:);% -二进制串均匀交叉算子function[children1,children2]=EqualCrossOver(parent1,parent2) global n children1 children2hidecode=round(rand(1,n));%随机生成掩码crossposition=find(hidecode==1);holdposition=find(hidecode==0);children1(crossposition)=parent1(crossposition);%掩码为1,父1为子1提供基因children1(holdposition)=parent2(holdposition);%掩码为0,父2为子1提供基因children2(crossposition)=parent2(crossposition);%掩码为1,父2为子2提供基因children2(holdposition)=parent1(holdposition);%掩码为0,父1为子2提供基因% -二进制串多点交叉算子function[Children1,Children2]=MultiPointCross(Parent1,Parent2)%交叉点数由变量数决定global n Children1 Children2 VarNumChildren1=Parent1;Children2=Parent2;Points=sort(unidrnd(n,1,2*VarNum));for i=1:VarNumChildren1(Points(2*i-1):Points(2*i))=Parent2(Points(2*i-1):Points(2*i));Children2(Points(2*i-1):Points(2*i))=Parent1(Points(2*i-1):Points(2*i));end% -- 变异操作--function[NewPop]=Mutation(OldPop,fit,pMutation,VarNum,opts) global m n NewPopNewPop=OldPop;r=rand(1,m);MutIn=find(r=pMutation);L=length(MutIn);完整可以运行的数值优化遗传算法源代码i=1;if opts(1)==1%浮点变异maxfit=max(fit);upfit=maxfit+0.05*abs(maxfit);if opts(2)==1|opts(2)==3while(i=L)%自适应变异(自增或自减)Point=unidrnd(n);T=(1-fit(MutIn(i))/upfit)^2;q=abs(1-rand^T);%if q1%按严格数学推理来说,这段程序是不能缺少的% q=1%endp=OldPop(MutIn(i),Point)*(1-q);if unidrnd(2)==1NewPop(MutIn(i),Point)=p+q;elseNewPop(MutIn(i),Point)=p;endi=i+1;endelseif opts(2)==2|opts(2)==4%AEA变异(任意变量的某一位变异)while(i=L)Point=unidrnd(n);T=(1-abs(upfit-fit(MutIn(i)))/upfit)^2;v=1+unidrnd(1+ceil(10*T));%v=1+unidrnd(5+ceil(10*eranum/MaxEranum));q=mod(floor(OldPop(MutIn(i),Point)*10^v),10);NewPop(MutIn(i),Point)=OldPop(MutIn(i),Point)-(q-unidrnd(9))/10^v;i=i+1;endelsewhile(i=L)Point=unidrnd(n);if round(rand)NewPop(MutIn(i),Point)=OldPop(MutIn(i),Point)*(1-rand);elseNewPop(MutIn(i),Point)=OldPop(MutIn(i),Point)+(1-OldPop(MutIn(i),Point))*rand; endi=i+1;endendelseif opts(1)==0%二进制串变异if L=1while i=Lk=unidrnd(n,1,VarNum); %设置变异点数(=变量数)for j=1:length(k)if NewPop(MutIn(i),k(j))==1NewPop(MutIn(i),k(j))=0;else完整可以运行的数值优化遗传算法源代码NewPop(MutIn(i),k(j))=1;endendi=i+1;endendelse%Tsp变异if opts(2)==1|opts(2)==2|opts(2)==3|opts(2)==4numMut=ceil(pMutation*m);r=unidrnd(m,numMut,2);[LocalMinfit,In]=min(fit(r),[],2);SelectIn=r((In-1)*numMut+[1:numMut]');while(i=numMut)mPoints=sort(unidrnd(n,1,2));if mPoints(1)~=mPoints(2)NewPop(SelectIn(i),1:mPoints(1)-1)=OldPop(SelectIn(i),1:mPoints(1)-1);NewPop(SelectIn(i),mPoints(1):mPoints(2)-1)=OldPop(SelectIn(i),mPoints(1)+1:mPoints(2));NewPop(SelectIn(i),mPoints(2))=OldPop(SelectIn(i),mPoints(1));NewPop(SelectIn(i),mPoints(2)+1:n)=OldPop(SelectIn(i),mPoints( 2)+1:n);elseNewPop(SelectIn(i),:)=OldPop(SelectIn(i),:);endi=i+1;endr=rand(1,m);MutIn=find(r=pMutation);L=length(MutIn);while i=LmPoints=sort(unidrnd(n,1,2));rIn=randperm(mPoints(2)-mPoints(1)+1);NewPop(MutIn(i),mPoints(1):mPoints(2))=OldPop(MutIn(i),mPoin ts(1)+rIn-1);i=i+1;endendend% -- 倒位操作--function [NewPop]=Inversion(OldPop,pInversion)global m n NewPopNewPop=OldPop;r=rand(1,m);PopIn=find(r=pInversion);len=length(PopIn);if len=1while(i=len)d=sort(unidrnd(n,1,2));完整可以运行的数值优化遗传算法源代码NewPop(PopIn(i),d(1):d(2))=OldPop(PopIn(i),d(2):-1:d(1)); i=i+1;。
遗传算法经典MATLAB代码
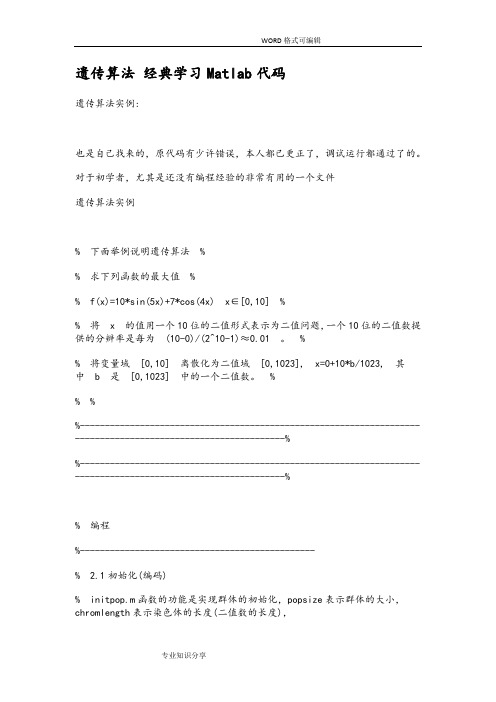
遗传算法经典学习Matlab代码遗传算法实例:也是自己找来的,原代码有少许错误,本人都已更正了,调试运行都通过了的。
对于初学者,尤其是还没有编程经验的非常有用的一个文件遗传算法实例% 下面举例说明遗传算法%% 求下列函数的最大值%% f(x)=10*sin(5x)+7*cos(4x) x∈[0,10]%% 将x 的值用一个10位的二值形式表示为二值问题,一个10位的二值数提供的分辨率是每为(10-0)/(2^10-1)≈0.01。
%% 将变量域[0,10] 离散化为二值域[0,1023], x=0+10*b/1023, 其中 b 是[0,1023] 中的一个二值数。
%% %%--------------------------------------------------------------------------------------------------------------%%--------------------------------------------------------------------------------------------------------------%% 编程%-----------------------------------------------% 2.1初始化(编码)% initpop.m函数的功能是实现群体的初始化,popsize表示群体的大小,chromlength表示染色体的长度(二值数的长度),% 长度大小取决于变量的二进制编码的长度(在本例中取10位)。
%遗传算法子程序%Name: initpop.m%初始化function pop=initpop(popsize,chromlength)pop=round(rand(popsize,chromlength)); % rand随机产生每个单元为{0,1} 行数为popsize,列数为chromlength的矩阵,% roud对矩阵的每个单元进行圆整。
遗传算法解释及代码(一看就懂)

遗传算法解释及代码(一看就懂)遗传算法( GA , Genetic Algorithm ) ,也称进化算法。
遗传算法是受达尔文的进化论的启发,借鉴生物进化过程而提出的一种启发式搜索算法。
因此在介绍遗传算法前有必要简单的介绍生物进化知识。
一.进化论知识作为遗传算法生物背景的介绍,下面内容了解即可:种群(Population):生物的进化以群体的形式进行,这样的一个群体称为种群。
个体:组成种群的单个生物。
基因 ( Gene ) :一个遗传因子。
染色体 ( Chromosome ):包含一组的基因。
生存竞争,适者生存:对环境适应度高的、牛B的个体参与繁殖的机会比较多,后代就会越来越多。
适应度低的个体参与繁殖的机会比较少,后代就会越来越少。
遗传与变异:新个体会遗传父母双方各一部分的基因,同时有一定的概率发生基因变异。
简单说来就是:繁殖过程,会发生基因交叉( Crossover ) ,基因突变( Mutation ) ,适应度( Fitness )低的个体会被逐步淘汰,而适应度高的个体会越来越多。
那么经过N代的自然选择后,保存下来的个体都是适应度很高的,其中很可能包含史上产生的适应度最高的那个个体。
二.遗传算法思想借鉴生物进化论,遗传算法将要解决的问题模拟成一个生物进化的过程,通过复制、交叉、突变等操作产生下一代的解,并逐步淘汰掉适应度函数值低的解,增加适应度函数值高的解。
这样进化N代后就很有可能会进化出适应度函数值很高的个体。
举个例子,使用遗传算法解决“0-1背包问题”的思路:0-1背包的解可以编码为一串0-1字符串(0:不取,1:取);首先,随机产生M个0-1字符串,然后评价这些0-1字符串作为0-1背包问题的解的优劣;然后,随机选择一些字符串通过交叉、突变等操作产生下一代的M个字符串,而且较优的解被选中的概率要比较高。
这样经过G代的进化后就可能会产生出0-1背包问题的一个“近似最优解”。
编码:需要将问题的解编码成字符串的形式才能使用遗传算法。
- 1、下载文档前请自行甄别文档内容的完整性,平台不提供额外的编辑、内容补充、找答案等附加服务。
- 2、"仅部分预览"的文档,不可在线预览部分如存在完整性等问题,可反馈申请退款(可完整预览的文档不适用该条件!)。
- 3、如文档侵犯您的权益,请联系客服反馈,我们会尽快为您处理(人工客服工作时间:9:00-18:30)。
frt1(frt11+2)=dij(chromochushi(suijidata(1)一1)+1,1)/50;
frt2(frt22+2)=dij(chromochushi(suijidata(2)一l)+1,1)/50;
end
end
for i=l:rusernumber(2) %size(frt2,2)
for j=1:i
frt2leij(i)= frt2leij(i)+frt2(j);
end
end
for i=l:rusernumber(3) %size(frt3,2)
frt3(frt33+2)=dij(chromochushi(11)+1,1)/50;
%%计算到达各个客户的时间
%%将第1条线路,服务时间加入到到达客户的时间里面去
if rusernumber(l)>1
for i=3:suijidata(1)-1
frtl(i-1)=frtl(i-1)+ti(chromochushi(i-1));
fori=suijidata(2)+1:10; %%计算第1条路径各路段距离和时间
fr(3)=fr(3)+dij(chromochushi(i)+l,chromochushi(i+l)+1);
frt3(i-suijidata(2)+1)=dij(chromochushi(i)+1,chromochushi(i+l)+l)/50;
end
fr(l)=dij(1,chromochushi(2)+1); %%线路1,从车场到第1个用户的距离
fr(2)=dij(1,chromochushi(suijidata(1)+l)+l); %线路2,从车场到第1个用户的距离
fr(3)=dij(1,chromochushi(suijidata(2)+l)+1); %线路3,从车场到第1个用户的距离
%%%%%%3.计算种群适应度函数值子程序
function[fall]=produceobjectivevalue(chromosomesize,zhongqunscale,chromosome,zeroposition)
for ijk=1:zhongqunscale
%%%获得单个染色体
for i=1:chromosomesize
frt33=frt33+l;
end
end
%%%计算每条线路的最后一个客户到场站的距离和路段时间
fr(1)=fr(l)+dij(chromochushi(suijidata(1)-l)+l,1);
fr(2)=fr(2)+dij(chromochushi(suijidata(2)-l)+l,l);
for j=1:i
frt2leij(i)= frt3leij(i)+frt3(j);
end
end
for i=2:suijidata(1)-1
if frtlleij(i-1)<ei(chromochushi(i))
fr(1)=fr(l)+50*(ei(chromoehushi(i))-frtlleij(i-1));
frt2(i-suijidata(1)+1)=dij(chromochushi(i)+1,chromochushi(i+l)+l)/50;
frt22=frt22+l;
end
end
%%%计算第3条线路的路段距离与路段时间
frt33=0;
if rusernumber(3)>1
frt22=0;
if rusernumber(2)>l
fori=suijidata(1)+1:suijidata(2)-2; %%计算第1条路径各路段距离和时间
fr(2)=fr(2)+dij(chromochushi(i)+l,chromochushi(i+l)+1);
%%%定义3条路径的客户数量
for1=1:3
rusernumber(i)=0;
end
%%%求解
rusernumber(l)=suijidata(1)-2;
rusernumber(2)=suijidata(2)-suijidata(l)-1:
rusernumber(3)=size(chromochushi,2)-suijidata(2)-l;
end
end
%%将第2条线路,服务时间加入到到达客户的时间里面去
if rusernumber(2)>l
k=2;
for i=suijidata(1)+2:suijidata(2)-1
frt2(k)=frt2(k)+ti(chromochushi(i-1));
k=k+1;
fr(2)=fr(l)+50*(ei(chromoehushi(i))-frt2leij(i-suijidata(1)));
end
if frt2leij(i-suijidata(1))>li(chromochushi(i))
fr(2)=fr(2)+50*(frt2leij(i-suijidata(1))-li(chromochushi(i)));
for i=1:rusernumber(1)+l
frt1(i)=0;
end
for i=1:rusernumber(2)+l
frt2(i)=0;
end
for i=1:rusernumbe%%对3条线路进行计算
%%%计算3条路径,车场到达第一个客户的距离和路段时间
end
end
%%计算3条线路中到达各个客户的时间
for i=l:rusernumber(l) %size(frtl,2)
frt1leij(i)=0;
end
for i=l:rusernumber(2) %size(frt2,2)
frt2leij(i)=0;
end
end
end
%%将第3条线路,服务时间加入到到达客户的时间里面去
if rusernumber(3)>1
k=2;
for i=suijidata(2)+2:chromosomesize-1
frt3(k)=frt3(k)+ti(chromochushi(i-1));
k=k+l;
%%%3条线路,从车场到第1个用户的路段时间
frtl(1)=fr(1)/50;
frtl(2)=fr(2)/50;
frtl(3)=fr(3)/50;
%%计算每条路径的第一个客户到最后一个客户的距离和路段时间
frt11=0;
if rusernumber(l)>1
fori=2:suijidata(1)-2; %%计算第1条路径各路段距离和时间
fr(l)=fr(1)+dij(chromochushi(i)+l,chromochushi(i+l)+1);
frtl(i)=dij(chromochushi(i)+1,chromochushi(i+l)+l)/50;
frt11=frt11+l;
end
end
%%%计算第2条线路的路段距离与路段时间
end
if frtlleij(i-1)>li(chromochushi(i))
fr(1)=fr(l)+50*(frtlleij(i-1)-li(chromochushi(i)));
end
end
for i=suijidata(1)+1:suijidata(2)-1
if frt2leij(i-suijidata(1))<ei(chromochushi(i))
for i=l:rusernumber(3) %size(frt3,2)
frt3leij(i)=0;
end
for i=l:rusernumber(l) %size(frtl,2)
for j=1:i
frt1leij(i)= frt1leij(i)+frt1(j);
chromochushi(i)=chromosome(ijk,i);
end
for i=1:2
suijidata(i)=zeroposition(ijk,i);
end
%%定义一个3列的数组表示3条线路的目标函数值
for i=1:3
fr(i)=0;
end
%%%计算每条路径的客户数量