MIT 2010 人工智能 期末试卷
机器学习考试卷 midterm2010f

Σ0 =
10 04
, Σ1 =
40 01
6
3 Logistic Regression [18 pts]
We consider here a discriminative approach for solving the classification problem illustrated in Figure 1.
10-701/15-781 Machine Learning - Midterm Exam, Fall 2010
Aarti Singh Carnegie Mellon University
1. Personal info:
• Name: • Andrew account: • E-mail address:
6. You have 90 minutes.
7. Good luck!
Question
1 2 3 4 5 6
Topic
Short questions Bayes Optimal Classification Logistic Regression Regression SVM Boosting Total
8. The depth of a learned decision tree can be larger than the number of training examples used to create the tree.
For the following problems, circle the correct answers: 1. Consider the following data set:
5. Work efficiently. Some questions are easier, some more difficult. Be sure to give yourself time to answer all of the easy ones, and avoid getting bogged down in the more difficult ones before you have answered the easier ones.
人工智能期末考试卷(1)评分标准及标准答案

(此文档为Word格式,下载后可以任意编辑修改!)试卷装订封面人工智能期末考试卷(1)一、填空题(每空1分,共10分)1智能具有五个特征,分别为① 学习能力、自适应能力、②记忆与思维能力、表达能力和感知能力。
2. 机器的③ 感知能力是让机器自动获取知识的基本条件,而知识的自动获取一直是智能系统研究中最困难的问题之一。
3•从研究的角度不同,对人工智能的研究可分两大阵营:④ 联接和⑤符号。
其中⑤符号的理论基础为数理逻辑。
4. ⑥问题规约方法是一种将复杂问题变换为比较简单的子问题,子问题再转换为更简单的子问题,最终将问题转换为对本原问题的知识表示方法。
5. 鲁宾逊提出了⑦归结原理使机器定理证明成为可能。
6. 当某个算符被认为是问题求解的决定步骤时,此算符为⑧关键算符。
7. 宽度优先搜索与深度优先搜索方法的一个致命的缺点是当问题比较复杂是可能会发生⑨组合爆炸。
8. 语义网络⑩方法是1968年由J.R.Quilian 在研究人类联想记忆时提出的心理学模型。
1972年,Simon首先将⑩用于自然语言理解系统。
二、简答题(共30分)1. 什么是A*算法的可纳性?(4分)答:在搜索图存在从初始状态节点到目标状态节点解答路径的情况下,若一个搜索法总能找到最短(代价最小)的解答路径,则称算法具有可采纳性。
2. 在一般图搜索算法中,当对某一个节点n进行扩展时,n的后继节点可分为三类,请举例说明对这三类节点的不同的处理方法。
(8分)答:把SNS中的子节点分为三类:(1)全新节点,(2)已出现于OPEN表的节点,(3 )已出现于CLOSE表的节点;/后二类子节点实际上意味着具有新老两个父节点;(3分)*加第1类子节点于OPEN表,并建立从子节点到父节点n的指;(1分)*比较第2类子节点经由新、老父节点到达初始状态节点s的路径代价,若经由新父节点的代价较小,则移动子节点指向新父节点(2分)•对于第3类子节点作与第2类同样的处理,并把这些子节点从CLOSE 表中移出,重新加入OPEN表;(2分)3. 请简述不确定性推理的含义。
《人工智能》试卷A及答案
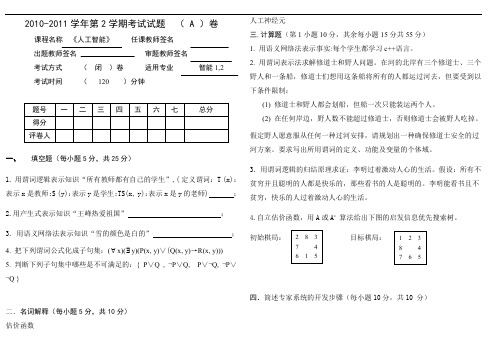
2010-2011学年第2学期考试试题 ( A )卷课程名称 《人工智能》 任课教师签名出题教师签名 审题教师签名考试方式 ( 闭 )卷 适用专业 智能1,2考试时间 ( 120 )分钟一、填空题(每小题5分,共25分)1. 用谓词逻辑表示知识“所有教师都有自己的学生”,( 定义谓词:T (x):表示x 是教师;S (y):表示y 是学生;TS(x, y):表示x 是y 的老师) ; 2.用产生式表示知识“王峰热爱祖国” ; 3. 用语义网络法表示知识“雪的颜色是白的” ; 4. 把下列谓词公式化成子句集:(∀x)(∃y)(P(x, y)∨(Q(x, y)→R(x, y))) 5. 判断下列子句集中哪些是不可满足的:{ P ∨Q , ¬P ∨Q, P ∨¬Q, ¬P ∨¬Q }二.名词解释(每小题5分,共10分) 估价函数人工神经元三.计算题(第1小题10分,其余每小题15分共55分) 1. 用语义网络法表示事实:每个学生都学习c++语言。
2. 用谓词表示法求解修道士和野人问题。
在河的北岸有三个修道士、三个野人和一条船,修道士们想用这条船将所有的人都运过河去,但要受到以下条件限制: (1) 修道士和野人都会划船,但船一次只能装运两个人。
(2) 在任何岸边,野人数不能超过修道士,否则修道士会被野人吃掉。
假定野人愿意服从任何一种过河安排,请规划出一种确保修道士安全的过河方案。
要求写出所用谓词的定义、功能及变量的个体域。
3.用谓词逻辑的归结原理求证:李明过着激动人心的生活。
假设:所有不贫穷并且聪明的人都是快乐的,那些看书的人是聪明的。
李明能看书且不贫穷,快乐的人过着激动人心的生活。
4.自立估价函数,用A 或A * 算法给出下图的启发信息优先搜索树。
初始棋局:目标棋局:四.简述专家系统的开发步骤(每小题10分,共10 分)答案一、填空题(每空1分,共10分)1. (∀x)(∃y)(T (x)→ TS(x, y) ∧S (y))2. (love, Wang Feng, country) 或(热爱,王峰,祖国)3.4. S={P(x, f(x))∨¬Q(x, f(x))∨R(x, f(x))}5. 不可满足,其归结过程为:二.1.估价函数用来估计节点重要性的函数。
人工智能期末考试试题
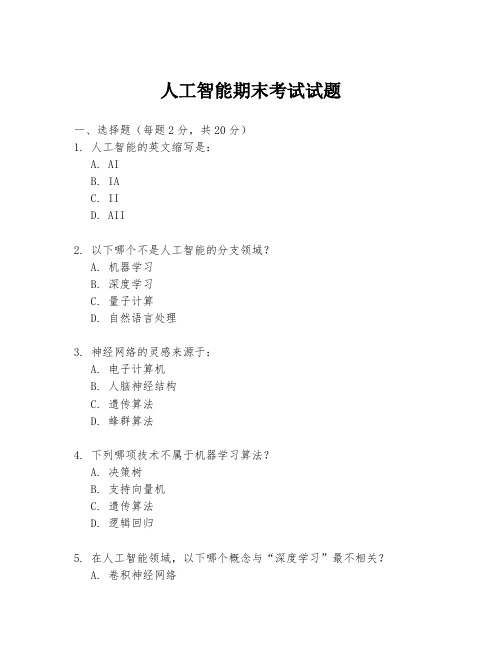
人工智能期末考试试题一、选择题(每题2分,共20分)1. 人工智能的英文缩写是:A. AIB. IAC. IID. AII2. 以下哪个不是人工智能的分支领域?A. 机器学习B. 深度学习C. 量子计算D. 自然语言处理3. 神经网络的灵感来源于:A. 电子计算机B. 人脑神经结构C. 遗传算法D. 蜂群算法4. 下列哪项技术不属于机器学习算法?A. 决策树B. 支持向量机C. 遗传算法D. 逻辑回归5. 在人工智能领域,以下哪个概念与“深度学习”最不相关?A. 卷积神经网络B. 循环神经网络C. 专家系统D. 长短期记忆网络二、简答题(每题10分,共30分)1. 请简述人工智能与机器学习之间的关系。
2. 解释什么是监督学习和无监督学习,并给出一个实际应用的例子。
3. 描述深度学习在图像识别领域的应用。
三、论述题(每题25分,共50分)1. 论述人工智能在医疗领域的应用及其潜在的伦理问题。
2. 讨论人工智能对就业市场的影响,包括正面和负面的影响。
四、案例分析题(共30分)阅读以下案例:某公司开发了一款智能客服机器人,能够处理客户咨询和解决问题。
请分析该机器人可能面临的技术挑战,并提出解决方案。
五、编程题(共20分)编写一个简单的Python程序,实现一个基于决策树的分类器,对以下数据集进行分类:数据集:```特征1, 特征2, 类别1, 2, 正2, 1, 负3, 3, 正1, 1, 负```要求:- 使用sklearn库中的决策树分类器。
- 训练模型并预测新数据点 [2, 2] 的类别。
六、开放性问题(共10分)你认为人工智能在未来10年内将如何改变我们的日常生活?请给出你的观点和理由。
请注意:所有答案需根据题目要求,结合人工智能的相关知识进行回答。
人工智能期末试题及答案完++++整版(最新)

一单项选择题(每小题2分,共10分)1.首次提出“人工智能”是在(D )年A.1946B.1960C.1916D.19562. 人工智能应用研究的两个最重要最广泛领域为:BA.专家系统、自动规划B. 专家系统、机器学习C. 机器学习、智能控制D. 机器学习、自然语言理解3. 下列不是知识表示法的是 A 。
A:计算机表示法 B:“与/或”图表示法C:状态空间表示法 D:产生式规则表示法4. 下列关于不确定性知识描述错误的是 C 。
A:不确定性知识是不可以精确表示的B:专家知识通常属于不确定性知识C:不确定性知识是经过处理过的知识D:不确定性知识的事实与结论的关系不是简单的“是”或“不是”。
5. 下图是一个迷宫,S0是入口,S g是出口,把入口作为初始节点,出口作为目标节点,通道作为分支,画出从入口S0出发,寻找出口Sg的状态树。
根据深度优先搜索方法搜索的路径是 C 。
A:s0-s4-s5-s6-s9-sg B:s0-s4-s1-s2-s3-s6-s9-sgC:s0-s4-s1-s2-s3-s5-s6-s8-s9-sg D:s0-s4-s7-s5-s6-s9-sg二填空题(每空2分,共20分)1.目前人工智能的主要学派有三家:符号主义、进化主义和连接主义。
2. 问题的状态空间包含三种说明的集合,初始状态集合S 、操作符集合F以及目标状态集合G 。
3、启发式搜索中,利用一些线索来帮助足迹选择搜索方向,这些线索称为启发式(Heuristic)信息。
4、计算智能是人工智能研究的新内容,涉及神经计算、模糊计算和进化计算等。
5、不确定性推理主要有两种不确定性,即关于结论的不确定性和关于证据的不确定性。
三名称解释(每词4分,共20分)人工智能专家系统遗传算法机器学习数据挖掘答:(1)人工智能人工智能(Artificial Intelligence) ,英文缩写为AI。
它是研究、开发用于模拟、延伸和扩展人的智能的理论、方法、技术及应用系统的一门新的技术科学。
人工智能机器学习技术章节习题及答案期末考试试卷题库及答案 (1)

第6章 参考答案1,熵是表示随机变量不确定地度量,是对所有可能发生地事件产生地信息量地期望。
对于二分类来说,即一个随机事件有两种可能性:发生与不发生,设发生地概率为p,则不发生地概率为1-p,于是其熵地计算公式为:22H *log (1)*log (1)p p p p =----假如一个随机事件有三种可能地状态:状态1,状态2,状态3,其概率分别为P 1,P 2,P 3,则有P 1+P 2+P 3=1,于是它地熵地计算公式为:121222323H *log *log *log p p p p p p =---熵越大,不确定性就越大,熵越小,不确定性就越小(即确定性越大)。
极端地情况下,假如一个随机事件必然要发生,即其概率为1,那么它就没有不确定性了,此时熵最小为0.条件熵是给定某种条件地情况下重新计算地熵,假设给定地条件X 有状态1,状态2两种可能性,则其概率分别为P x1,P x2,则条件熵地计算公式为 1122H **x x x x x p H p H =+其中H x1,H x2分别为X 在状态1与状态2下计算地熵。
很明显,当给出一定地条件时,随机事件地不确定性减小,即条件熵要小于熵。
熵与条件熵地差就是信息增益。
信息增益表示地是当给出一定地条件时,事件地不确定性减少地程度。
2,采用ID3算法,先计算总地熵,再计算各个条件熵,然后计算出信息增益,选择信息增益最大地那个属性进行划分,然后再对各个子树进行同样地步骤划分。
参考决策树如图所示。
第6章第2题 决策树3,贝叶斯概率公式为: 有房子贷款有工作 不贷款信贷情况年龄不贷款不贷款贷款 是 是 否否好 一般 老年 中、青年()(A |)(|)()()(|)或者 (|)()(|)i i i i i iP B P B P B A P A P B P A B P B A P B P A B ==∑ 先验概率是指根据以前经验与分析得到地概率;后验概率是在事情已经发生地情况下,要求这件事情发生地原因是由某个因素引起地可能性地大小,即后验概率是已经知道结果,来推断该结果是由何种原因引起。
大学人工智能期末考试题库
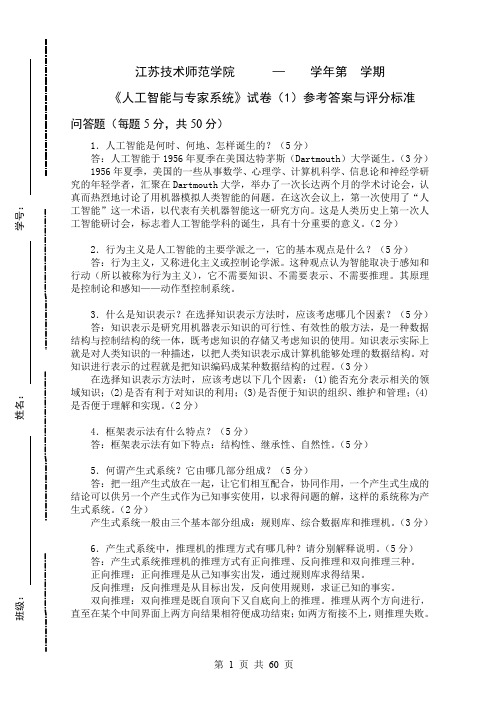
第 2 页 共 60 页
然后将已知条件和问题用谓词公式表示出来,并将问题公式的否定与谓词 ANSWER 做析取,得到子句集:(3 分)
(1)~Brother(x,y)∨~Father(z,x)∨Father(z,y) (2) Brother(John,Peter) (3) Father(David,John) (4)~Father(u,Peter)∨ANSWER(u) 应用归结原理进行归结: (3 分) (5)~Brother(John,y)∨Father(David,y)
第 3 页 共 60 页
2.求如下图所示的交通图中最小费用路线,设出发地是 A 城,目的地是 E 城,边 上的数字代表交通费。(1)画出本问题的代价树;(2)对代价树进行广度优先搜索 和深度优先搜索,得到的路线分别是什么? (8 分)
解:代价树如下:(4 分)
广度优先搜索得到的路线:A→C→D→E (2 分) 深度优先搜索得到的路线:A→C→D→E (2 分)
属性 不可询问 不可询问 可询问
可询问
第 4 页 共 60 页
F8
有很多公园
F9
是南方国家
F10
有法国餐馆
F11
有意大利餐馆
F12
有本地的烹调传统
F13
有民俗学传统
F14
有茂盛的草木
F15
是古老的城市
F16
是历史名城
F17
是值得旅游的城市
可询问 可询问 可询问
不可询问 可询问
(2)画出 CITY 库的与/或树 解:(1)不可询问、可询问、可询问、可询问、可询问、可询问、不可询问、
9.证据传递的不确定性指什么?(5 分) 答:在推理过程中常常有这种情况:一条规则的结论又是另一条规则的前提。 这样,不确定的初始证据就会沿着这条推理链向下传递,其不确定性在传递的过程 中会伴随着规则的不确定性不断地放大或缩小。 (5 分)
人工智能期末试题 (2)
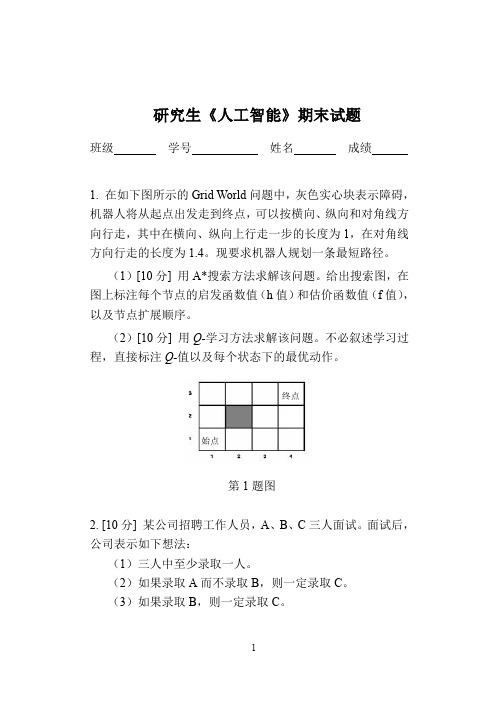
研究生《人工智能》期末试题班级学号姓名成绩1. 在如下图所示的Grid World问题中,灰色实心块表示障碍,机器人将从起点出发走到终点,可以按横向、纵向和对角线方向行走,其中在横向、纵向上行走一步的长度为1,在对角线方向行走的长度为1.4。
现要求机器人规划一条最短路径。
(1)[10分] 用A*搜索方法求解该问题。
给出搜索图,在图上标注每个节点的启发函数值(h值)和估价函数值(f值),以及节点扩展顺序。
(2)[10分] 用Q-学习方法求解该问题。
不必叙述学习过程,直接标注Q-值以及每个状态下的最优动作。
终点始点第1题图2. [10分] 某公司招聘工作人员,A、B、C三人面试。
面试后,公司表示如下想法:(1)三人中至少录取一人。
(2)如果录取A而不录取B,则一定录取C。
(3)如果录取B,则一定录取C。
求证:用Resolution Refutation推理方法证明公司一定录取C。
3. [10分] 请综合利用Single-layer Perceptron和Decision Tree 表示如下布尔函数:()CA¬∧。
(注:XOR表示异或运BXOR算)。
(提示:可将该函数看作一个复合函数)4. [10分] 给定数据集合及其决策结果如下:样本决策结果属性A1属性A2属性A31 No 3 3 52 Yes 36 13 503 Yes 15 14 304 No 14 22 65 No 23 7 246 Yes 4 18 8其中,根据A1, A2和A3的属性值是否大于10来进行决策。
基于该数据集,利用Maximum Information Gain准则生成一棵Decision Tree。
给出该Decision Tree及其生成过程。
(提示:6.1≈)3log25. 在如下图所示的Bayesian Belief Network中,I, H, L, P, E均为布尔型变量。
I表示是否聪明(Intelligent),H表示是否诚实(Honest),L表示是否有许多竞选资金(LotsOfCompainFunds),P表示是否受欢迎(Popular),E 表示是否竞选成功(Elected)。
- 1、下载文档前请自行甄别文档内容的完整性,平台不提供额外的编辑、内容补充、找答案等附加服务。
- 2、"仅部分预览"的文档,不可在线预览部分如存在完整性等问题,可反馈申请退款(可完整预览的文档不适用该条件!)。
- 3、如文档侵犯您的权益,请联系客服反馈,我们会尽快为您处理(人工客服工作时间:9:00-18:30)。
Final for6.034Spring2010Name:2020202020G ood luck!6.034Spring2010Final page1of11Question#120points Indicate whether the following statement is true and briefly justify your answer.(i)(2points)If we add more training data,the number of support vectorswill always increase.(ii)(2points)For a given training set,support vectors are invariant to the kernel choice.(iii)(2points)There is a distribution overfive variables that can be represented by anyfive node Bayesian network topology.(iv)(2points)The largest factor created by the variable elimination algorithm is no bigger than the largest initial factor.(v)(2points)The variable elimination algorithm can require time exponential in the size of the Bayesian network.(vi)(2points)Observing an additional node(i.e.making the node an evidence node)in a Bayesian network can only increase the number of variable pairs which are conditionally independent.6.034Spring2010Final page2of11Each of the five plots (A to E)below shows the result of training a soft-margin SVM on a two-class data set (labels are 0and 1)with di fferent kernel functions and regularization parameter C .4682345A(vii)(8points)For each plot,find the best matching set of learning parameters(i.e.kernel function and C ).Each set of parameters can only be used once.PlotKernel C Linear 1Linear 1000Polynomial (degree 2)1000Polynomial (degree 5)1000Gaussian/RBF 10006.034Spring 2010Final page 3of 11Question#220points Here,you plan to use a naive Bayesian classifier to build a personal computer troubleshooting system for novices to decide whether the hardware is bad or ok. However,for simplicity,this decision must be based only on three noisy boolean (true/false)observations:•N:Computer makes loud(n)oises•F:Computer(f)reezes frequently•A:(A)pplications run slowlyCause:H∈{bad,ok}Evidence:N,F,A∈{true,false}You goal is to build a classifier that models P(H|N,F,A).(a)(4points)Under the naive Bayes assumption,write an expression for P(H|N,F,A)in terms of only probabilities of the forms P(H),P(N|H),P(F|H),andP(A|H)(b)(6points)The company has records for several recent user support cases:H N F Aok false false trueok false true falseok false true falseok true false falseok false false falsebad true false falsebad true true falsebad false true trueGiven this data,fill the tables below with the parameter estimates for theparameters of the naive Bayesian classifie Laplace correction(aka“add-one”smoothing)for feature estimates P(N|H),P(F|H),and P(A|H)but not for class prior estimates P(H).H P(H) bad3/8 ok/N H P(N|H)true bad/false bad/true ok/false ok/F H P(F|H)true bad/false bad/true ok/false ok/A H P(A|H)true bad/false bad/true ok/false ok/6.034Spring2010Final page4of11Suppose you are given an observation of a new case:N=false,F=true, A=true.(c)(4points)Calculate the joint probability P(H,N=false,F=true,A=true).Please leave the probabilities in fractional form.H P(H,N=false,F=true,A=true)okbad(d)(2points)State the prediction of your naive Bayes classifier,i.e.bad or ok.(e)(2points)Calculate the posterior probability P(H=ok|N=false,F=true,A=true).(Please leave it in fractional form.)(f)(2points)Suppose you use hard-margin SVMs instead.Give a kernel func-tion that can separate the training data.If no such kernel exists,briefly explain why.6.034Spring2010Final page5of11Question#320points Assume there are two types of conditions:(S)inus congestion and(F)lu.Sinus congestion is caused by(A)llergy or theflu.There are three observed symptoms for these conditions:(H)eadache,(R)unny nose,and fe(V)er.Runny nose and headaches are directly caused by sinus con-gestion(only),while fever comes from having theflu(only).For example, allergies only cause runny noses indirectly.Assume each variable is binary (i.e.true/false).(a)(5points)Consider the four Bayesian networks shown.Indicate which onemodels the domain(as described above)best.Best network:i/ii/iii/iv(b)(5points)Assume we wanted to remove the Sinus congestion(S)-ing the least number of edges,draw a Bayesian network over the remaining variables which can encode the original model’s marginal distribution over the remaining variables.6.034Spring2010Final page6of11The following samples were drawn from the correct Bayesian network using prior sampling:Sample#A S R H F V1true true true false false false2true true false true true false3true false false false false false4true false false true true false5true true false true false false(c)(2points)Give the sample estimate of P(F=true)or state why it cannotbe computed.(d)(2points)Give the sample estimate of P(F=true|H=true)or state whyit cannot be computed.(e)(2points)Give the sample estimate of P(F=true|V=true)or state whyit cannot be computed.(f)(4points)For rejection sampling in general(not necessarily on these sam-ples),which query will require more samples to compute a certain degree of accuracy,P(F|H)or P(F|H,A)?Briefly justify.6.034Spring2010Final page7of11Question#420points Consider the following pairs of Bayesian networks.If the two networks have identical conditional independence properties,indicate same and write one of their shared independence(or none if they assert none.)Otherwise, indicate different and write an independence property that one has but the other.For example,in the following case you would answer as shown:different:right has A⊥B(a)(3points)(b)(3points)(c)(3points)(d)(3points)6.034Spring2010Final page8of11Consider the following Bayesian network.(e)(8points)For each the following conditional independence(CI)property,indicate whether it is true,false,or unknown.CI property AssertionN⊥T True/False/UnknownP⊥E|N True/False/UnknownP⊥N|{A,C}True/False/UnknownE⊥A|{C,N}True/False/Unknown6.034Spring2010Final page9of11Question#520pointsThe next parts involve computing various quan-tities in the network below.These questions are designed so that they can be answered with min-imum computation.If youfind yourself doing co-pious amount of computation for each part,step back and consider whether there is a simpler way to deduce theanswer.A P(A) true0.1 false0.9B A P(B|A)true true0.5false true0.5true false0.8false false0.2C A P(C|A)true true0.4false true0.6true false0.7false false0.3D B P(D|B)true true0.9false true0.1true false0.2false false0.8(a)(2points)P(A=true,B=false,C=true,D=false)(b)(2points)P(A=true,B=true)(c)(2points)P(B=true)(d)(2points)P(A=true|B=true)(e)(2points)P(D=true|A=true)(f)(2points)P(D=true|A=true,C=true)6.034Spring2010Final page10of11Consider computing the following distributions in the above network using various methods:(i)P(A|B=true,C=true,D=true)(ii)P(C|D=true)(iii)P(D|A=true)(iv)P(D)(g)(2points)Which query is least expensive using inference by enumeration?Briefly justify.(h)(2points)Which query is most improved by using likelihood weightinginstead of rejection sampling(in terms of number of samples required)?Briefly justify.(i)(2points)When computing P(D)with variable elimination,what factor(s)is/are eliminated byfirst eliminating variable A from the active list?(j)(2points)When computing P(D)with variable elimination,show the new factor(in tabular form)that is created byfirst eliminating variable A from the active list.6.034Spring2010Final page11of11。