Missing feature theory in ASR make sure you miss the right type of features
2023年人工智能现代科技知识考试题与答案
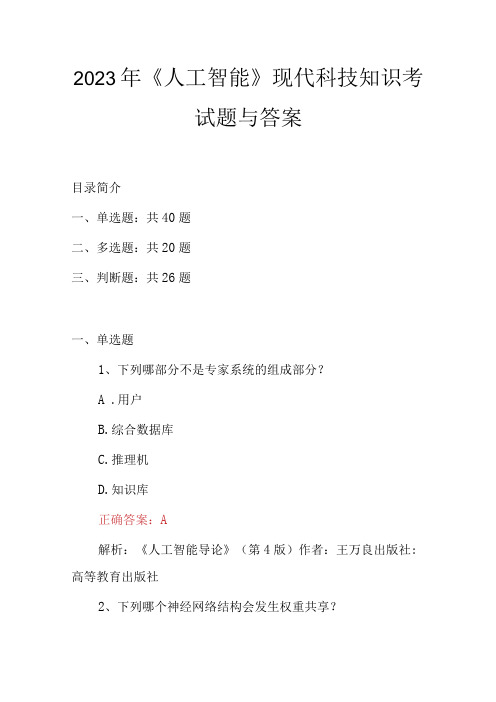
2023年《人工智能》现代科技知识考试题与答案目录简介一、单选题:共40题二、多选题:共20题三、判断题:共26题一、单选题1、下列哪部分不是专家系统的组成部分?A .用户B.综合数据库C.推理机D.知识库正确答案:A解析:《人工智能导论》(第4版)作者:王万良出版社: 高等教育出版社2、下列哪个神经网络结构会发生权重共享?A.卷积神经网络B.循环神经网络C.全连接神经网络D. A 和B正确答案:D解析:《深度学习、优化与识别》作者:焦李成出版社: 清华大学出版社3、下列哪个不属于常用的文本分类的特征选择算法?A.卡方检验值B.互信息C .信息增益D.主成分分析正确答案:D解析:《自然语言处理》作者:刘挺出版社:高等教育出版社4、下列哪个不是人工智能的技术应用领域?A.搜索技术B.数据挖掘C.智能控制D .编译原理解析:《走进人工智能》作者:周旺出版社:高等教育出版社5、Q(s,a)是指在给定状态s的情况下,采取行动a之后,后续的各个状态所能得到的回报()。
A.总和B.最大值C.最小值D.期望值正确答案:D解析:《深度学习、优化与识别》作者:焦李成出版社: 清华大学出版社6、数据科学家可能会同时使用多个算法(模型)进行预测,并且最后把这些算法的结果集成起来进行最后的预测(集成学习),以下对集成学习说法正确的是()。
A.单个模型之间有高相关性B.单个模型之间有低相关性C,在集成学习中使用“平均权重”而不是“投票”会比较好D.单个模型都是用的一个算法解析:《机器学习方法》作者:李航出版社:清华大学出版社7、以下哪种技术对于减少数据集的维度会更好?A.删除缺少值太多的列B.删除数据差异较大的列C.删除不同数据趋势的列D.都不是正确答案:A解析:《机器学习》作者:周志华出版社:清华大学出版社8、在强化学习过程中,学习率越大,表示采用新的尝试得到的结果比例越(),保持旧的结果的比例越()。
A .大,小B.大,大C.小,大D.小,小正确答案:A解析:《深度学习、优化与识别》作者:焦李成出版社: 清华大学出版社9、以下哪种方法不属于特征选择的标准方法?A.嵌入B.过滤C ,包装D.抽样正确答案:D解析:《深度学习、优化与识别》作者:焦李成出版社: 清华大学出版社10、要想让机器具有智能,必须让机器具有知识。
一种神经机器翻译中稀有词模糊语义表示方法
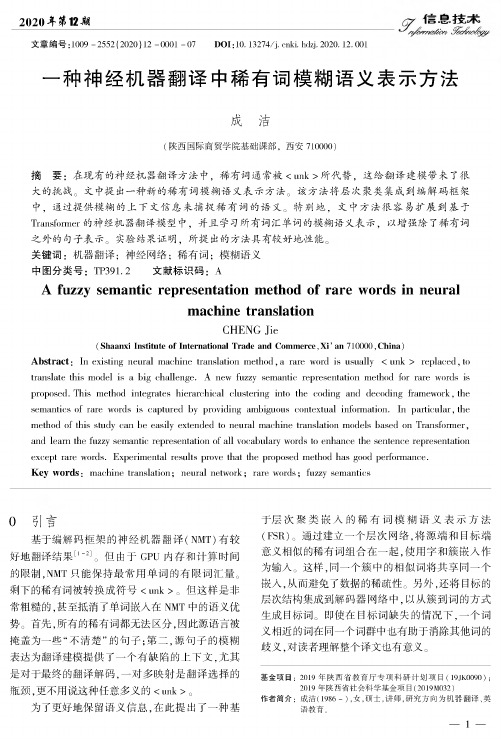
2020耳第12剧文章编号:1009-2552(2020)12-0001-07DOI:10.13274/ki.hdzj.2020.12.001一种神经机器翻译中稀有词模糊语义表示方法成洁(陕西国际商贸学院基础课部,西安710000)摘要:在现有的神经机器翻译方法中,稀有词通常被<unk>所代替,这给翻译建模带来了很大的挑战。
文中提出一种新的稀有词模糊语义表示方法。
该方法将层次聚类集成到编解码框架中,通过提供模糊的上下文信息来捕捉稀有词的语义。
特别地,文中方法很容易扩展到基于Transformer的神经机器翻译模型中,并且学习所有词汇单词的模糊语义表示,以增强除了稀有词之外的句子表示。
实验结果证明,所提出的方法具有较好地性能。
关键词:机器翻译;神经网络;稀有词;模糊语义中图分类号:TP391.2文献标识码:AA fuzzy semantic representation method of rare words in neuralmachine translationCHENG Jie(Shaanxi Institute of International Trade and Commerce,Xi'an710000,China) Abstract:In existing neural machine translation method,a rare word is usually<unk>replaced,to translate this model is a big challenge.A new fuzzy semantic representation method for rare words is proposed.This method integrates hierarchical clustering into the coding and decoding framework,the semantics of rare words is captured by providing ambiguous contextual information.In particular,the method of this study can be easily extended to neural machine translation models based on Transformer, and learn the fuzzy semantic representation of all vocabulary words to enhance the sentence representation except rare words.Experimental results prove that the proposed method has good performance.Key words:machine translation;neural network;rare words;fuzzy semantics0引言基于编解码框架的神经机器翻译(NMT)有较好地翻译结果[l-2]o但由于GPU内存和计算时间的限制,NMT只能保持最常用单词的有限词汇量o 剩下的稀有词被转换成符号<unk>。
自动驾驶中的图像语义分割与特征提取方法研究
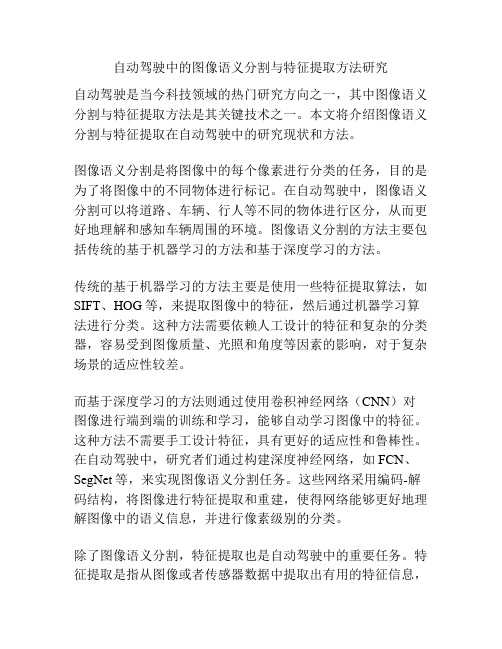
自动驾驶中的图像语义分割与特征提取方法研究自动驾驶是当今科技领域的热门研究方向之一,其中图像语义分割与特征提取方法是其关键技术之一。
本文将介绍图像语义分割与特征提取在自动驾驶中的研究现状和方法。
图像语义分割是将图像中的每个像素进行分类的任务,目的是为了将图像中的不同物体进行标记。
在自动驾驶中,图像语义分割可以将道路、车辆、行人等不同的物体进行区分,从而更好地理解和感知车辆周围的环境。
图像语义分割的方法主要包括传统的基于机器学习的方法和基于深度学习的方法。
传统的基于机器学习的方法主要是使用一些特征提取算法,如SIFT、HOG等,来提取图像中的特征,然后通过机器学习算法进行分类。
这种方法需要依赖人工设计的特征和复杂的分类器,容易受到图像质量、光照和角度等因素的影响,对于复杂场景的适应性较差。
而基于深度学习的方法则通过使用卷积神经网络(CNN)对图像进行端到端的训练和学习,能够自动学习图像中的特征。
这种方法不需要手工设计特征,具有更好的适应性和鲁棒性。
在自动驾驶中,研究者们通过构建深度神经网络,如FCN、SegNet等,来实现图像语义分割任务。
这些网络采用编码-解码结构,将图像进行特征提取和重建,使得网络能够更好地理解图像中的语义信息,并进行像素级别的分类。
除了图像语义分割,特征提取也是自动驾驶中的重要任务。
特征提取是指从图像或者传感器数据中提取出有用的特征信息,用于自动驾驶系统的决策和控制。
常用的特征有纹理特征、颜色特征等,通过提取这些特征,可以对图像进行分析和理解。
传统的特征提取方法多采用手工设计的特征提取器,如HOG、SIFT等。
这些特征提取方法需要人工设计特征提取器,难以适应复杂场景。
而基于深度学习的特征提取方法则能够通过数据驱动的方式自动地学习图像中的特征。
研究者们通过设计卷积神经网络,如VGG、ResNet等,来提取图像中的特征向量。
这些网络通过多层网络的堆叠和卷积操作,能够自动地提取出图像中的抽象特征,使得特征具有更好的可区分性和表达能力。
人工智能导论王万良思考题答案
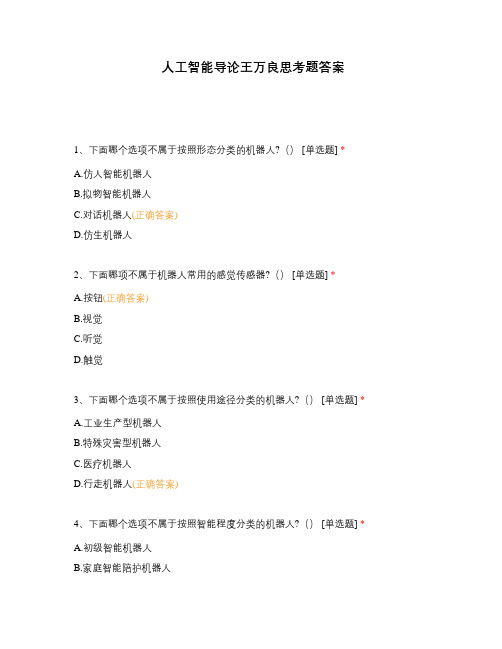
人工智能导论王万良思考题答案1、下面哪个选项不属于按照形态分类的机器人?() [单选题] *A.仿人智能机器人B.拟物智能机器人C.对话机器人(正确答案)D.仿生机器人2、下面哪项不属于机器人常用的感觉传感器?() [单选题] *A.按钮(正确答案)B.视觉C.听觉D.触觉3、下面哪个选项不属于按照使用途径分类的机器人?() [单选题] *A.工业生产型机器人B.特殊灾害型机器人C.医疗机器人D.行走机器人(正确答案)4、下面哪个选项不属于按照智能程度分类的机器人?() [单选题] *A.初级智能机器人B.家庭智能陪护机器人C.高级智能机器D.农业机器人(正确答案)5、机器人一般按哪两种方式工作?() *A.将程序事先写好在存储器中(正确答案)B.示教-再现方式(正确答案)C.手动控制D.自我学习6、下面哪些选项属于机器人常用的传感器? *A.碰撞传感器(正确答案)B.激光雷达传感器(正确答案)C.视觉传感器(正确答案)D.超声传感器(正确答案)7、麦克风传感器可用于检测语音? [判断题] *对(正确答案)错8、热释电传感器可用于检测温度? [判断题] *对错(正确答案)9、碰撞传感器用于检测障碍物时使用? [判断题] *对(正确答案)错10、激光雷达传感器可用于获取障碍物的精确位置? [判断题] *对(正确答案)错11、自然语言理解,又称(),是人工智能的一个重要分支,属于计算机科学的一部分 [单选题] *A.人机对话(正确答案)B.人机交互C.语言合成D.语言生成12、下面哪个选项不属于自然语言理解的常用任务?() [单选题] *A.中文文本分词B.文本表示C.命名实体识别D.文本情感识别(正确答案)13、自然语言处理领域具有两个鲜明特征:一是(),二是真实可用性 [单选题] *A.小规模性B.大规模性(正确答案)C.乱序性D.有序性14、要想提取出“有用”的信息,仅提取关键词、统计词频等是远远不够的,必须对用户数据(尤其是发言、评论等)进行()。
隐语义模型常用的训练方法

隐语义模型常用的训练方法隐语义模型(Latent Semantic Model)是一种常用的文本表示方法,它可以将文本表示为一个低维的向量空间中的点,从而方便进行文本分类、聚类等任务。
在实际应用中,如何训练一个高效的隐语义模型是非常重要的。
本文将介绍隐语义模型常用的训练方法。
一、基于矩阵分解的训练方法1.1 SVD分解SVD(Singular Value Decomposition)分解是一种基于矩阵分解的方法,它可以将一个矩阵分解为三个矩阵相乘的形式,即A=UΣV^T。
其中U和V都是正交矩阵,Σ是对角线上元素为奇异值的对角矩阵。
在隐语义模型中,我们可以将用户-物品评分矩阵R分解为两个低维矩阵P和Q相乘的形式,即R≈PQ^T。
其中P表示用户向量矩阵,Q表示物品向量矩阵。
具体地,在SVD分解中,我们首先需要将评分矩阵R进行预处理。
一般来说,我们需要减去每个用户或每个物品评分的平均值,并对剩余部分进行归一化处理。
然后,我们可以使用SVD分解将处理后的评分矩阵R分解为P、Q和Σ三个矩阵。
其中,P和Q都是低维矩阵,Σ是对角线上元素为奇异值的对角矩阵。
通过调整P和Q的维度,我们可以控制模型的复杂度。
在训练过程中,我们需要使用梯度下降等方法来最小化预测评分与实际评分之间的误差。
具体地,在每次迭代中,我们可以随机选择一个用户-物品对(ui),计算预测评分pui,并根据实际评分rui更新P 和Q中相应向量的值。
具体地,更新公式如下:pu=pu+η(euiq-uλpu)qi=qi+η(euip-uλqi)其中η是学习率,λ是正则化参数,eui=rui-pui表示预测评分与实际评分之间的误差。
1.2 NMF分解NMF(Nonnegative Matrix Factorization)分解是另一种基于矩阵分解的方法,在隐语义模型中也有广泛应用。
与SVD不同的是,在NMF中要求所有矩阵元素都为非负数。
具体地,在NMF中,我们需要将评分矩阵R进行预处理,并将其分解为P和Q两个非负矩阵相乘的形式,即R≈PQ。
人工智能岗位招聘笔试题及解答(某世界500强集团)

招聘人工智能岗位笔试题及解答(某世界500强集团)(答案在后面)一、单项选择题(本大题有10小题,每小题2分,共20分)1、以下哪项不属于人工智能的基本技术?A、机器学习B、自然语言处理C、计算机视觉D、基因编辑2、以下哪种算法不适合用于分类问题?A、决策树B、支持向量机C、K-最近邻(KNN)D、神经网络3、以下哪个技术不属于人工智能领域常用的机器学习算法?A. 决策树B. 深度学习C. 搜索算法D. 贝叶斯网络4、在以下机器学习模型中,哪个模型适用于处理非线性关系?A. 线性回归B. 支持向量机(SVM)C. K-最近邻(KNN)D. 线性判别分析(LDA)5、以下哪个算法不属于深度学习中的神经网络算法?A. 卷积神经网络(CNN)B. 支持向量机(SVM)C. 隐马尔可夫模型(HMM)D. 递归神经网络(RNN)6、在以下机器学习任务中,哪个任务通常需要较高的计算资源?A. 监督学习B. 无监督学习C. 强化学习D. 半监督学习7、题干:以下哪项技术不是人工智能领域的关键技术之一?A、机器学习B、自然语言处理C、量子计算D、神经网络8、题干:在以下哪种情况下,人工智能系统最有可能出现“过拟合”现象?A、数据集较大,特征较多B、数据集较小,特征较少C、数据集适中,特征适中D、数据集较大,特征适中9、以下哪种技术不属于深度学习中的卷积神经网络(CNN)常用的激活函数?A. ReLUB. SigmoidC. SoftmaxD. Tanh二、多项选择题(本大题有10小题,每小题4分,共40分)1、以下哪些技术属于人工智能领域的基础技术?()A、机器学习B、自然语言处理C、计算机视觉D、神经网络E、数据挖掘2、以下关于人工智能伦理的表述中,哪些是正确的?()A、人工智能系统应确保用户隐私和数据安全B、人工智能系统应避免歧视和偏见C、人工智能系统应具备自我意识和情感D、人工智能系统应遵循法律法规和道德规范E、人工智能系统应完全替代人类工作3、以下哪些技术属于人工智能领域?()A. 机器学习B. 深度学习C. 自然语言处理D. 机器视觉E. 云计算4、以下关于人工智能伦理问题的描述,哪些是正确的?()A. 人工智能系统应确保用户数据隐私B. 人工智能系统应避免歧视C. 人工智能系统应具备自我意识D. 人工智能系统应保证决策透明度E. 人工智能系统应具备情感识别能力5、以下哪些是人工智能领域中常见的机器学习算法?()A. 神经网络B. 决策树C. 随机森林D. 暴力破解6、以下哪些是人工智能领域中常用的深度学习框架?()A. TensorFlowB. PyTorchC. KerasD. Caffe7、题目:下列哪些技术属于监督学习的例子?A. 决策树B. K-均值聚类C. 支持向量机D. 主成分分析(PCA)E. 随机森林8、题目:在构建机器学习模型时,以下哪些方法可以用来处理数据中的缺失值?A. 删除含有缺失值的数据行B. 使用统计方法如平均数、中位数填充缺失值C. 使用插值法在时间序列数据中填补空缺D. 通过机器学习算法预测缺失值E. 不做任何处理直接建模9、以下哪些技术属于人工智能领域的关键技术?()A、机器学习B、自然语言处理C、神经网络D、遗传算法E、数据库技术三、判断题(本大题有10小题,每小题2分,共20分)1、人工智能中的深度学习模型只能用于图像识别任务。
《计算机视觉》题集
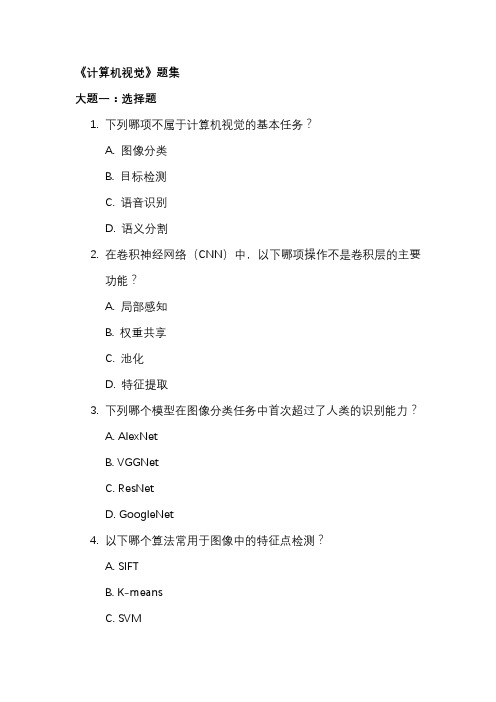
《计算机视觉》题集大题一:选择题1.下列哪项不属于计算机视觉的基本任务?A. 图像分类B. 目标检测C. 语音识别D. 语义分割2.在卷积神经网络(CNN)中,以下哪项操作不是卷积层的主要功能?A. 局部感知B. 权重共享C. 池化D. 特征提取3.下列哪个模型在图像分类任务中首次超过了人类的识别能力?A. AlexNetB. VGGNetC. ResNetD. GoogleNet4.以下哪个算法常用于图像中的特征点检测?A. SIFTB. K-meansC. SVMD. AdaBoost5.在目标检测任务中,IoU (Intersection over Union)主要用于衡量什么?A. 检测框与真实框的重叠程度B. 模型的检测速度C. 模型的准确率D. 模型的召回率6.下列哪项技术可以用于提高模型的泛化能力,减少过拟合?A. 数据增强B. 增加模型复杂度C. 减少训练数据量D. 使用更大的学习率7.在深度学习中,批归一化 (Batch Normalization)的主要作用是什么?A. 加速模型训练B. 提高模型精度C. 减少模型参数D. 防止梯度消失8.下列哪个激活函数常用于解决梯度消失问题?A. SigmoidB. TanhC. ReLUD. Softmax9.在进行图像语义分割时,常用的评估指标是?A. 准确率B. 召回率C. mIoU(mean Intersection over Union)D. F1分数10.下列哪个不是深度学习框架?A. TensorFlowB. PyTorchC. OpenCVD. Keras大题二:填空题1.计算机视觉中的“三大任务”包括图像分类、目标检测和______。
2.在深度学习模型中,为了防止梯度爆炸,常采用的技术是______。
3.在卷积神经网络中,池化层的主要作用是进行______。
4.YOLO算法是一种流行的______算法。
5.在进行图像增强时,常用的技术包括旋转、缩放、______和翻转等。
slam算法工程师招聘笔试题与参考答案(某世界500强集团)2024年
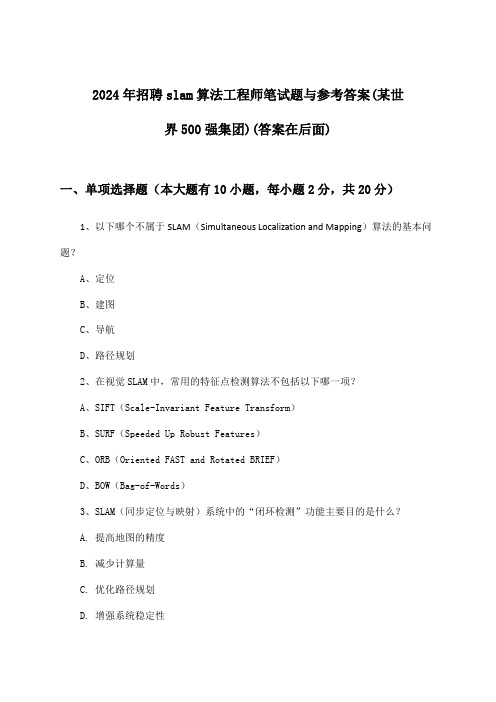
2024年招聘slam算法工程师笔试题与参考答案(某世界500强集团)(答案在后面)一、单项选择题(本大题有10小题,每小题2分,共20分)1、以下哪个不属于SLAM(Simultaneous Localization and Mapping)算法的基本问题?A、定位B、建图C、导航D、路径规划2、在视觉SLAM中,常用的特征点检测算法不包括以下哪一项?A、SIFT(Scale-Invariant Feature Transform)B、SURF(Speeded Up Robust Features)C、ORB(Oriented FAST and Rotated BRIEF)D、BOW(Bag-of-Words)3、SLAM(同步定位与映射)系统中的“闭环检测”功能主要目的是什么?A. 提高地图的精度B. 减少计算量C. 优化路径规划D. 增强系统稳定性4、在视觉SLAM中,以下哪种方法通常用于提取特征点?A. SIFT(尺度不变特征变换)B. SURF(加速稳健特征)C. ORB(Oriented FAST and Rotated BRIEF)D. 以上都是5、SLAM(Simultaneous Localization and Mapping)算法的核心目标是什么?A. 实现无人驾驶车辆在未知环境中的自主导航B. 构建三维空间地图并实时更新C. 实现机器人路径规划D. 以上都是6、以下哪种传感器不适合用于SLAM系统?A. 激光雷达B. 摄像头C. 声呐D. 超声波传感器7、以下关于SLAM(同步定位与映射)系统的描述中,哪个是错误的?A. SLAM系统通常需要在未知环境中进行定位与建图。
B. SLAM系统通常需要使用传感器来获取环境信息。
C. SLAM系统可以实时生成地图并更新位置信息。
D. SLAM系统不需要进行初始化定位。
8、以下关于视觉SLAM(视觉同步定位与映射)系统的描述中,哪个是正确的?A. 视觉SLAM系统只依赖于视觉传感器进行定位与建图。
强化学习算法中的稀疏表示学习方法详解(五)
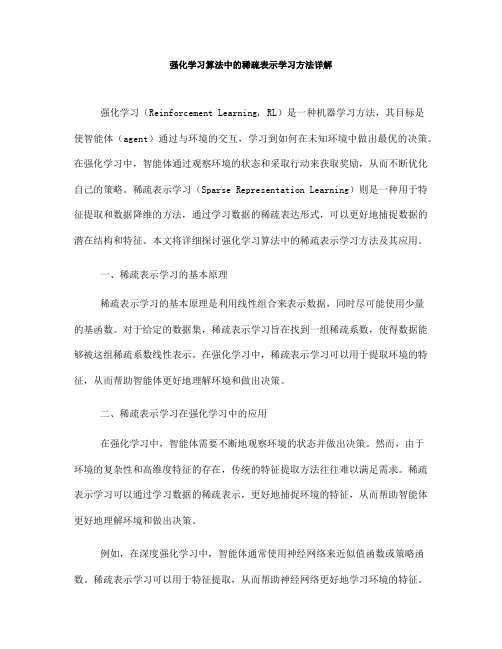
强化学习算法中的稀疏表示学习方法详解强化学习(Reinforcement Learning, RL)是一种机器学习方法,其目标是使智能体(agent)通过与环境的交互,学习到如何在未知环境中做出最优的决策。
在强化学习中,智能体通过观察环境的状态和采取行动来获取奖励,从而不断优化自己的策略。
稀疏表示学习(Sparse Representation Learning)则是一种用于特征提取和数据降维的方法,通过学习数据的稀疏表达形式,可以更好地捕捉数据的潜在结构和特征。
本文将详细探讨强化学习算法中的稀疏表示学习方法及其应用。
一、稀疏表示学习的基本原理稀疏表示学习的基本原理是利用线性组合来表示数据,同时尽可能使用少量的基函数。
对于给定的数据集,稀疏表示学习旨在找到一组稀疏系数,使得数据能够被这组稀疏系数线性表示。
在强化学习中,稀疏表示学习可以用于提取环境的特征,从而帮助智能体更好地理解环境和做出决策。
二、稀疏表示学习在强化学习中的应用在强化学习中,智能体需要不断地观察环境的状态并做出决策。
然而,由于环境的复杂性和高维度特征的存在,传统的特征提取方法往往难以满足需求。
稀疏表示学习可以通过学习数据的稀疏表示,更好地捕捉环境的特征,从而帮助智能体更好地理解环境和做出决策。
例如,在深度强化学习中,智能体通常使用神经网络来近似值函数或策略函数。
稀疏表示学习可以用于特征提取,从而帮助神经网络更好地学习环境的特征。
通过学习数据的稀疏表示,可以更好地捕捉环境的潜在结构和特征,从而提高智能体的决策能力。
三、稀疏表示学习方法在强化学习中,常用的稀疏表示学习方法包括字典学习、压缩感知和稀疏自编码器等。
这些方法都可以用于学习数据的稀疏表示,从而帮助智能体更好地理解环境和做出决策。
1. 字典学习字典学习是一种常用的稀疏表示学习方法,其目标是学习一组基函数(字典),使得数据能够被这组基函数线性表示。
在强化学习中,可以使用字典学习来提取环境的特征,从而帮助智能体更好地理解环境和做出决策。
基于神经网络的自然语言语义表征方法
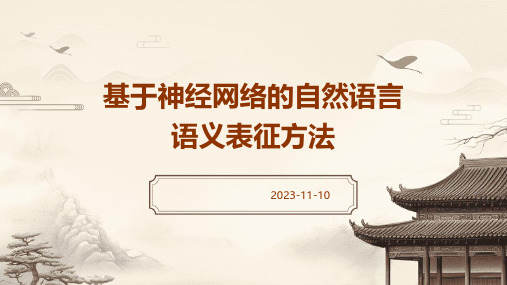
基于神经网络的自然语言语义表征方法2023-11-10目录CATALOGUE•引言•自然语言语义表征的相关研究•基于神经网络的语义表示模型•基于神经网络的语义匹配算法•基于神经网络的语义生成算法•基于神经网络的自然语言语义表征方法的应用场景与展望01 CATALOGUE引言研究背景与意义背景随着互联网和大数据技术的发展,自然语言处理(NLP)成为人工智能领域的重要研究方向。
在NLP中,语义表征是理解语言的关键,而神经网络在过去的十年中取得了重大进展,为语义表征提供了新的解决方案。
意义语义表征是实现自然语言处理的关键,它能够将自然语言文本转化为计算机可理解的语义表示,从而支持机器对人类语言的自动理解、生成和对话等任务。
基于神经网络的自然语言语义表征方法在许多应用领域都具有广泛的应用前景,如智能客服、智能家居、自动驾驶等。
本文主要研究基于神经网络的自然语言语义表征方法,包括词向量表示、句向量表示和语义匹配等关键技术。
具体研究内容包括:1)如何利用神经网络学习词向量表示;2)如何构建句向量表示;3)如何利用句向量进行语义匹配。
研究内容本文采用基于深度学习的神经网络模型进行研究。
首先,利用词向量表示方法将文本中的单词转化为计算机可处理的向量;然后,通过构建句向量表示模型将文本中的句子转化为句向量;最后,利用语义匹配模型判断两个句子的语义相似度。
主要采用的技术包括词嵌入、循环神经网络(RNN)、长短时记忆网络(LSTM)、Transformer和注意力机制等。
研究方法研究内容与方法02CATALOGUE自然语言语义表征的相关研究该方法主要基于词汇的语义关系来构建语义网络,进而对自然语言进行语义表征。
基于词汇学的方法基于句法的方法基于语料库的方法该方法主要通过分析句子的语法结构来理解句子的语义,进而实现自然语言的语义表征。
该方法主要通过大量的语料库来学习语言的语义,进而实现自然语言的语义表征。
03传统的自然语言语义表征方法02011基于神经网络的自然语言语义表征方法23该方法通过训练神经网络来学习词汇的分布式表示,从而实现对自然语言语义的表征。
深度学习题集
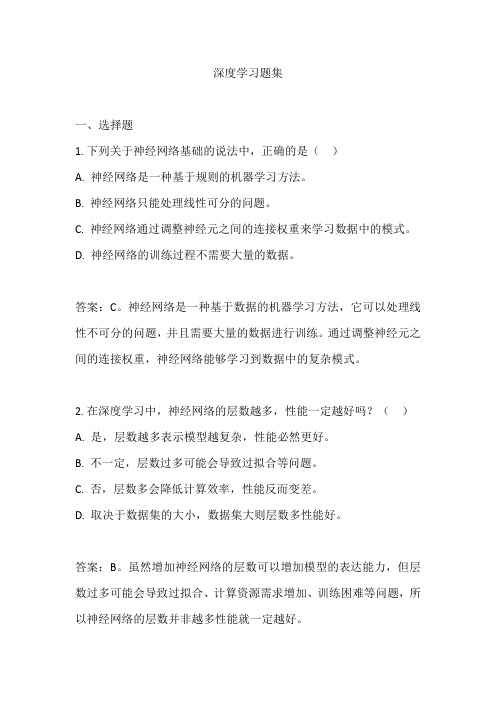
深度学习题集一、选择题1. 下列关于神经网络基础的说法中,正确的是()A. 神经网络是一种基于规则的机器学习方法。
B. 神经网络只能处理线性可分的问题。
C. 神经网络通过调整神经元之间的连接权重来学习数据中的模式。
D. 神经网络的训练过程不需要大量的数据。
答案:C。
神经网络是一种基于数据的机器学习方法,它可以处理线性不可分的问题,并且需要大量的数据进行训练。
通过调整神经元之间的连接权重,神经网络能够学习到数据中的复杂模式。
2. 在深度学习中,神经网络的层数越多,性能一定越好吗?()A. 是,层数越多表示模型越复杂,性能必然更好。
B. 不一定,层数过多可能会导致过拟合等问题。
C. 否,层数多会降低计算效率,性能反而变差。
D. 取决于数据集的大小,数据集大则层数多性能好。
答案:B。
虽然增加神经网络的层数可以增加模型的表达能力,但层数过多可能会导致过拟合、计算资源需求增加、训练困难等问题,所以神经网络的层数并非越多性能就一定越好。
3. 激活函数在神经网络中的主要作用是什么?()A. 增加神经网络的复杂度。
B. 提高神经网络的计算速度。
C. 引入非线性,使神经网络能够学习复杂的函数。
D. 减少神经网络的参数数量。
答案:C。
激活函数的主要作用是引入非线性,使得神经网络能够学习和表示复杂的函数关系。
如果没有激活函数,神经网络将只能学习线性函数,无法处理复杂的现实问题。
4. 下列哪个激活函数在输入为负数时输出为零?()A. Sigmoid 函数。
B. Tanh 函数。
C. ReLU 函数。
D. Softmax 函数。
答案:C。
ReLU(Rectified Linear Unit)函数在输入为负数时输出为零,在输入为正数时输出等于输入。
Sigmoid 函数和Tanh 函数在输入为负数时输出不为零,Softmax 函数主要用于多分类问题,不是在输入为负数时输出为零的函数。
5. 对于深度神经网络,以下哪种说法是正确的?()A. 深度神经网络的训练时间与网络层数成正比。
人工智能导论试卷
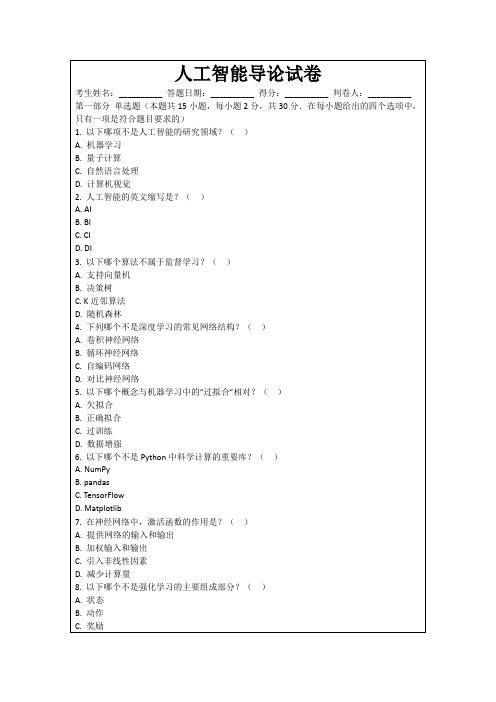
7.在卷积神经网络中,池化层的目的是减少参数数量和计算量。()
8.人工智能的发展完全依赖于硬件性能的提升。()
9.在自然语言处理中,词袋模型考虑了词语的顺序关系。()
10.人工智能在医疗诊断中的应用已经达到或超过了专业医生的水平。()
(以下为答题纸)
第四部分主观题(本题共2小题,每题10分,共20分)
人工智能导论试卷
考生姓名:__________答题日期:__________得分:__________判卷人:__________
第一部分单选题(本题共15小题,每小题2分,共30分.在每小题给出的四个选项中,只有一项是符合题目要求的)
1.以下哪项不是人工智能的研究领域?()
A.机器学习
B.量子计算
A.状态
B.动作
C.奖励
D.损失函数
9.在人工智能中,下列哪个领域涉及让机器理解人类语言?()
A.机器视觉
B.自然语言处理
C.语音识别
D.机器人学
10.以下哪个算法通常用于降维?()
A.主成分分析
B.支持向量机
C. K均值聚类
D.决策树
11.在机器学习中,下列哪个方法用于处理缺失值?()
A.均值填充
C.自然语言处理
D.计算机视觉
2.人工智能的英文缩写是?()
A. AI
B. BI
C. CI
D. DI
3.以下哪个算法不属于监督学习?()
A.支持向量机
B.决策树
C. K近邻算法
D.随机森林
4.下列哪个不是深度学习的常见网络结构?()
A.卷积神经网络
B.循环神经网络
C.自编码网络
人工智能中的语义推理与知识推理

人工智能中的语义推理与知识推理人工智能(Artificial Intelligence,简称AI)是现代科学技术领域的重要研究方向,旨在开发能够模拟人类智能的机器系统。
在实现这一目标的过程中,语义推理和知识推理作为两个关键技术,扮演着重要角色。
本文将从定义、原理、应用等方面阐述人工智能中的语义推理与知识推理,并探讨它们的相互关系以及未来发展的趋势。
一、语义推理语义推理(Semantic Reasoning)是指根据事物之间的内在关联,推导出新的语义信息的过程。
语义推理主要基于语义学和逻辑学的原理,以及计算机中的专门算法实现。
它能够从已有的语义信息中发现潜在的语义关系,进而进行推理和推断。
语义推理的主要原理之一是基于语义网络(Semantic Network)的表达方式。
语义网络是一种用于表示知识和语义关系的图结构。
其中节点代表事物或概念,边代表事物或概念之间的语义关系。
通过对语义网络的分析和推理,可以发现隐藏在知识背后的潜在语义关系。
另一个重要的语义推理原理是基于逻辑表达式的形式化推理。
利用逻辑推理规则,可以将自然语言表达的语义转化为形式化的逻辑表达式,进而进行推理过程。
逻辑推理的一个经典方法是基于命题逻辑的推理,它能够根据已知事实和逻辑规则推导出新的逻辑结论。
语义推理在人工智能中具有广泛的应用。
例如,在自然语言处理中,语义推理可用于理解和解释自然语言中的隐含意义和歧义。
在推荐系统中,语义推理可以在用户历史和商品信息的基础上推测用户的偏好和行为。
此外,语义推理还被应用于智能搜索、信息抽取、智能问答等领域。
二、知识推理知识推理(Knowledge Reasoning)是根据已有的知识,从中抽取新的知识或进行推理的过程。
它主要基于人类的知识表示和推理方式,以及计算机中的专门算法实现。
知识推理的目标是通过对已知知识的利用,从中推导出新的知识和规则。
知识推理的主要原理之一是基于规则的推理。
规则是一种基于条件和结果的描述,它表示特定条件下的行为或结果。
语义模糊性1
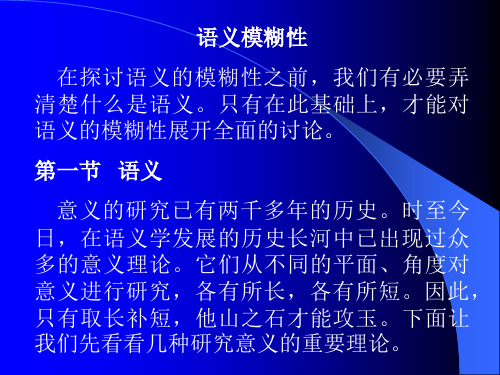
Wittgenstein认为,每个词都有用法,而词的用法是由规则规定的,词本身并没有什么意义。为此,他提出了“语言游戏”(language game)的概念。语言游戏表明语言并不仅是说话的工具,要使用语言就好比玩一次“游戏”(如打牌、下棋等),大家都要按事先约好的规则办事。但是,什么叫用法?什么是关系到语义的用法? Wittgenstein并没有作出回答。
又如,William Shakespeare 与 Swan of Avon 指的都是同一对象,但是它们的意义却不一样,正因为如此,我们才会说“Swan of Avon was Shakespeare”。弗雷格指出,所谓相同不是指事物与事物之间的关系,而是指事物的名称与名称之间的关系,或者说符号与符号之间的关系。A—A 与 A—B的认识价值不同。A—A 是先验真理,而 A一B包含经验知识,它告诉人们:被称为A的事物与被称为B的事物是同一事物。
首先从事言语行为研究的是牛津大学的奥斯汀(J.Austin),他在 How to Do Things With Words 中提出了三个概念:(1)言内行为(locutionary act),(2)言外行为(illocutionary act),(3)言后行为(perlocutionary act)。言内行为指的是“说话”这一行为本身,它大体与传统意义上的“意指”相同,即指发出语音、音节、说出单词、词组和句子等。这一行为本身并不能构成言语交际,但在实施这个行为之中,我们通常实施了一个言外行为,有时还同时实施了一个言后行为。
feature_importances_函数 -回复
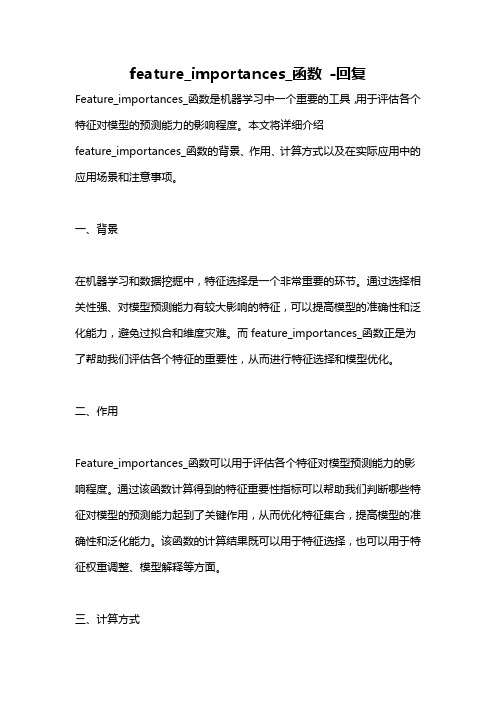
feature_importances_函数-回复Feature_importances_函数是机器学习中一个重要的工具,用于评估各个特征对模型的预测能力的影响程度。
本文将详细介绍feature_importances_函数的背景、作用、计算方式以及在实际应用中的应用场景和注意事项。
一、背景在机器学习和数据挖掘中,特征选择是一个非常重要的环节。
通过选择相关性强、对模型预测能力有较大影响的特征,可以提高模型的准确性和泛化能力,避免过拟合和维度灾难。
而feature_importances_函数正是为了帮助我们评估各个特征的重要性,从而进行特征选择和模型优化。
二、作用Feature_importances_函数可以用于评估各个特征对模型预测能力的影响程度。
通过该函数计算得到的特征重要性指标可以帮助我们判断哪些特征对模型的预测能力起到了关键作用,从而优化特征集合,提高模型的准确性和泛化能力。
该函数的计算结果既可以用于特征选择,也可以用于特征权重调整、模型解释等方面。
三、计算方式Feature_importances_函数的计算方式因模型而异。
不同的机器学习算法有不同的方式来计算特征重要性,下面以随机森林算法为例来说明计算方式。
随机森林是一种常用的集成学习算法,其通过多个决策树的预测结果的平均值或投票结果来进行最终的预测。
在随机森林中,特征重要性一般是通过测量每个特征的重要性分数来计算的。
特征重要性分数可以通过计算每个特征被使用的次数来衡量。
在决策树的构建过程中,特征被用来进行节点的分裂。
而在随机森林中,会构建多个决策树,每个决策树会随机选择特征子集进行分裂。
因此,特征被选择作为节点分裂特征的次数越多,其重要性分数就越高。
特征重要性分数还可以通过计算每个特征对预测准确率的贡献来衡量。
特征对模型的预测能力的贡献可以通过计算预测准确率的提升或下降来评估。
如果在只使用某个特征进行预测时,模型的预测准确率明显下降,则说明该特征对模型的预测能力有较大的影响,其重要性分数就越高。
如何解决稀疏编码中的标签丢失问题
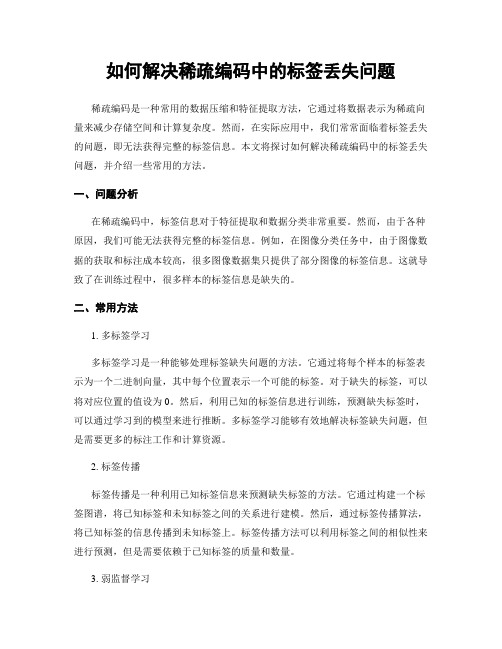
如何解决稀疏编码中的标签丢失问题稀疏编码是一种常用的数据压缩和特征提取方法,它通过将数据表示为稀疏向量来减少存储空间和计算复杂度。
然而,在实际应用中,我们常常面临着标签丢失的问题,即无法获得完整的标签信息。
本文将探讨如何解决稀疏编码中的标签丢失问题,并介绍一些常用的方法。
一、问题分析在稀疏编码中,标签信息对于特征提取和数据分类非常重要。
然而,由于各种原因,我们可能无法获得完整的标签信息。
例如,在图像分类任务中,由于图像数据的获取和标注成本较高,很多图像数据集只提供了部分图像的标签信息。
这就导致了在训练过程中,很多样本的标签信息是缺失的。
二、常用方法1. 多标签学习多标签学习是一种能够处理标签缺失问题的方法。
它通过将每个样本的标签表示为一个二进制向量,其中每个位置表示一个可能的标签。
对于缺失的标签,可以将对应位置的值设为0。
然后,利用已知的标签信息进行训练,预测缺失标签时,可以通过学习到的模型来进行推断。
多标签学习能够有效地解决标签缺失问题,但是需要更多的标注工作和计算资源。
2. 标签传播标签传播是一种利用已知标签信息来预测缺失标签的方法。
它通过构建一个标签图谱,将已知标签和未知标签之间的关系进行建模。
然后,通过标签传播算法,将已知标签的信息传播到未知标签上。
标签传播方法可以利用标签之间的相似性来进行预测,但是需要依赖于已知标签的质量和数量。
3. 弱监督学习弱监督学习是一种能够处理标签缺失问题的方法。
它通过利用弱标签或者部分标签来进行训练和预测。
弱监督学习可以通过利用数据的其他信息来进行标签的推断,例如文本数据的关键词、图像数据的上下文信息等。
弱监督学习方法可以在标签缺失的情况下,仍然能够进行有效的特征提取和数据分类。
三、实践案例以图像分类任务为例,假设我们只有一部分图像的标签信息。
我们可以利用多标签学习方法,将每个图像的标签表示为一个二进制向量。
然后,通过已知标签的图像进行训练,学习到一个分类模型。
技术服务机器学习算法考核试卷
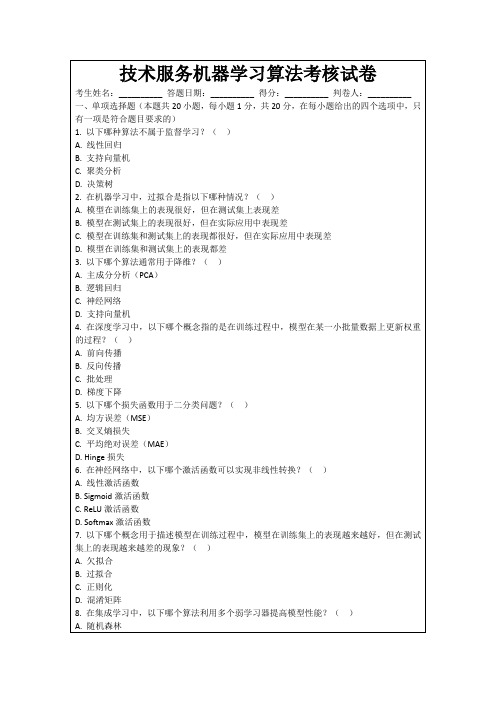
4.以下哪些是深度学习中的常见激活函数?()
A. Sigmoid
B. ReLU
C. Softmax
D. MSE
5.以下哪些损失函数常用于分类问题?()
A.交叉熵损失
B. Hinge损失
C.均方误差(MSE)
D.平均绝对误差(MAE)
6.以下哪些方法可以用于特征选择?()
A.过滤式
B.包裹式
1. ABD
2. ABD
3. ACD
4. ABC
5. AB
6. ABC
7. ABCD
8. ABC
9. ABC
10. ABC
11. ABC
12. ABCD
13. ABC
14. ABC
15. ABCD
16. ABC
17. ABCD
18. ABC
19. ABC
20. ABC
三、填空题
1.过拟合
2. ReLU
A.矩阵分解
B.决策树
C.支持向量机
D. K近邻算法
18.在强化学习中,以下哪个概念指的是智能体在某一状态采取某一动作后,获得的奖励与状态转移的概率?()
A.策略
B.值函数
C.动作值函数
D.状态值函数
19.以下哪个算法通常用于图像识别?()
A.卷积神经网络(CNN)
B.循环神经网络(RNN)
C.支持向量机
A.线性激活函数
B. Sigmoid激活函数
C. ReLU激活函数
D. Softmax激活函数
7.以下哪个概念用于描述模型在训练过程中,模型在训练集上的表现越来越好,但在测试集上的表现越来越差的现象?()
A.欠拟合
基于缺失特征的文本无关说话人识别鲁棒性研究的开题报告

基于缺失特征的文本无关说话人识别鲁棒性研究的开题报告一、研究背景及意义说话人识别是语音识别中的一个热门研究方向。
在语音识别中,识别器往往依赖于不同特征进行模型训练,如梅尔频率倒谱系数(MFCC)、线性预测编码(LPC)等。
然而,由于政治、语言、口音等方面的原因,有些说话人可能会出现在开发集合测试集示例之外。
因此,在现实场景中,存在着缺失某些特征的情况,这将导致已有模型的鲁棒性和可靠性受到影响。
近年来,深度学习技术的发展,尤其是使用深度神经网络(DNN)在语音识别中取得了巨大成功,使得语音识别性能大幅度提高。
同时,在说话人识别领域,利用深度学习进行建模的方法也得到了广泛应用。
对于缺失特征的鲁棒性问题,可以采用深度神经网络的分类能力进行缓解。
二、研究内容及目标本文研究内容为基于缺失特征的文本无关说话人识别鲁棒性研究。
研究采用深度神经网络作为分类器,通过实验方法验证了该方法的有效性。
具体研究流程如下:1. 收集并处理语音数据集;2. 对语音数据集进行特征提取;3. 采用不同的随机缺失方式生成缺失特征的语音,以此验证模型在不同缺失率下的鲁棒性表现;4. 设计深度神经网络模型,并采用缺失特征的语音数据集进行训练;5. 在测试集上对模型进行性能评估。
本文的目标是探究在实际场景中缺失特征对文本无关说话人识别的影响,研究利用深度学习方法提升模型鲁棒性的技术途径,为实际应用提供理论依据。
三、研究方法本文将针对文本无关说话人识别问题,设计基于深度神经网络的分类模型。
具体的研究方法包括:1. 数据集采集与处理:本文将采用公开数据集作为实验数据,如TIMIT等。
数据集处理包括音频清洗和预处理,以及特征提取。
常用的特征提取方法包括MFCC、LPC、MFBE等。
2. 随机生成缺失数据:本文将采用随机方式生成不同缺失率的缺失特征的语音数据。
3. 深度神经网络模型构建与训练:本文将基于TensorFlow等深度学习平台构建深度神经网络模型进行训练,其中包括卷积神经网络(CNN)、循环神经网络(RNN)等。
feature_importances_函数 -回复

feature_importances_函数-回复feature_importances_函数是机器学习中常用的一个函数,用于评估特征的重要性。
在机器学习算法中,特征选择是一个重要的步骤,它有助于提高模型的准确性和解释性。
本文将详细介绍feature_importances_函数,包括其原理、应用场景以及如何使用该函数评估特征的重要性。
首先,我们来了解一下feature_importances_函数的原理。
在机器学习中,该函数通常用于集成学习算法,比如随机森林和梯度提升树。
这些算法通过组合多个弱学习器,来构建一个强大的集成模型。
而feature_importances_函数所做的就是评估每个特征在集成模型中的重要性。
feature_importances_函数的输出是一个数组,该数组的长度等于特征的数量。
数组中的每个元素表示对应特征的重要性得分,得分越高表示该特征对模型的影响越大。
在一些实现中,特征重要性得分被归一化到[0,1]之间,这样可以更方便地比较不同特征的重要性。
接下来,我们来看一些应用场景,可以通过feature_importances_函数来选择最重要的特征。
首先,特征选择可以帮助我们降低模型的复杂性,从而避免过拟合的问题。
通过删除那些对模型没有贡献或贡献很小的特征,我们可以简化模型并提高其泛化能力。
其次,在解释模型的预测结果时,特征选择也非常有用。
通过分析特征的重要性,我们可以了解到哪些因素对模型的预测结果有重要影响。
这对于领域专家和业务决策者来说,具有很高的实用性。
最后,我们来看一下如何使用feature_importances_函数来评估特征的重要性。
首先,我们需要选择一个合适的集成学习算法,比如随机森林。
然后,我们可以通过训练一个具有一组特征的模型,并调用feature_importances_函数来获得特征的重要性得分。
根据得分的大小,我们可以选择保留最重要的特征或删除对模型性能没有贡献的特征。
- 1、下载文档前请自行甄别文档内容的完整性,平台不提供额外的编辑、内容补充、找答案等附加服务。
- 2、"仅部分预览"的文档,不可在线预览部分如存在完整性等问题,可反馈申请退款(可完整预览的文档不适用该条件!)。
- 3、如文档侵犯您的权益,请联系客服反馈,我们会尽快为您处理(人工客服工作时间:9:00-18:30)。
MISSING FEATURE THEORY IN ASR:MAKE SURE YOU MISS THERIGHT TYPE OF FEATURESJohan de Veth,Febe de Wet,Bert Cranen Louis BovesA RT,Department of Language and Speech,University of Nijmegen,P.O.Box9103,6500HD Nijmegen,THE NETHERLANDSemail:J.deVeth,F.deWet,B.Cranen,L.Boves@let.kun.nlABSTRACTIn this paper we investigate acoustic backing-off as an opera-tionalization of Missing Feature Theory to increase recognition robustness in adverse acoustic conditions.Acoustic backing-off effectively removes the detrimental influence of outlier values from the local decisions in the Viterbi algorithm.It does so with-out prior knowledge about the specific feature vector elements which are unreliable;thus,the technique avoids the need for ex-plicit outlier detection.From the theory underlying Missing Fea-ture Theory it appears that acoustic feature representations which smear local spectro-temporal distortions over all feature vector elements are inherently unsuitable.Our experiments in the con-text of connected digit recognition over the telephone are pre-sented that confirm this prediction.Our results show that fea-ture representations which minimize distortion smearing are most suited to be used in combination with Missing Feature Theory. Using additive band limited noise as a distortion,we found that acoustic backing-off can achieve a word error rate reduction of when within vectorfiltered mel-frequency log-energy coef-ficients are used.1.INTRODUCTIONIn automatic speech recognition(ASR),adverse acoustic condi-tions are likely to cause contamination of one or more compo-nents of the incoming feature vectors.When a feature obtains un-usual values(compared to training conditions)and if no measures are taken to handle these disturbed features differently from the undisturbed features,it may be expected that recognition perfor-mance will drop.Recently,it was suggested that Missing Feature Theory(MFT)can be used to improve robustness of ASR under adverse acoustic conditions[1],[2],[3].By using only the reli-able parts of the acoustic information and disregarding unreliable acoustic features,recognition performance can almost be main-tained at the level for undisturbed conditions.In standard HMM recognizers,feature distributions are often modeled by means of Gaussian probability density functions. However,it is rather unlikely that the tails of a Gaussian distrib-ution are reliable estimators of the less frequently occurring fea-ture values.As a consequence,it might not be such a good idea to define the contribution to the local distance function used dur-ing the dynamic programming as a quadratic function over the entire feature value range.In[4],[5]it was proposed to model feature value observations by means of two distributions:the one obtained from the training data and a uniform distribution which represents all feature values not seen during training.Local dis-tance computation interpolates between these two distributions; the weight assigned to either distribution can be varied so as to increase or decrease the contribution of the unseen values.This strategy was called acoustic backing-off and it was shown that it can be considered as an implementation of MFT which(1)is suited to be used in a conventional ASR system,(2)in principle allows one to use any feature representation as long as at least part of the acoustic feature vector is undisturbed,(3)contrary to the approach suggested in[2]does not require prior information about the corrupted features and(4)does not rely on an explicit detection mechanism for identifying disturbed feature vector el-ements as opposed to the approaches suggested in[6],[7]. However,the application of MFT is not as straightforward as it might seem,since there appears to be an interaction between MFT,as applied during recognition,and the signal pre-processing steps associated with typical ASR systems[5].Normalizing and orthogonalizing transforms are widely used in state-of-the-art ASR systems,e.g.gain normalization,channel normalization, Discrete Cosine Transform(DCT),Linear Discriminant Analy-sis(LDA).The main reason for using normalization transforms is that they yield statistically more stable feature values.As a result,speech can be represented more reliably and more effi-ciently,as reflected by improved recognition capability and faster training and recognition procedures,respectively.Orthogonal-ization is generally applied to remove correlations between raw spectral features so that a full-covariance matrix can be replaced by a more efficient diagonal variance matrix,i.e.its elements can be estimated reliably with less data.For clean speech data, these transforms generally improve recognition performance sig-nificantly.In this paper we will discuss why,under acoustically adverse conditions,simultaneous application of MFT on the one hand,and normalization and orthogonalization transforms on the other hand,may become undesirable.An intuitive understand-ing of the reasons behind this incompatibility may be obtained by considering the following reasoning.The basic pre-supposition in MFT is that a feature vector can be decomposed into a part which is virtually unaffected and another part which contains distorted features.As long as the loss of in-formation about the speech signal represented by the disturbed features is relatively small,MFT predicts that recognition per-formance can be maintained at a level which is comparable to the undisturbed case,simply by discarding the disturbed features. However,a complication arises when the raw incoming features arefirst transformed by means of an algorithm which uses all fea-ture vector elements to calculate a transformed vector.In this case,the misleading information due to the disturbances which are present in a restricted number of raw features,will be smeared out over the entire normalized(orthogonalized)vector.If this happens,there is little hope that MFT can effectively help in re-covering from the disturbances.In our opinion one of the challenges in building robust ASR al-gorithms isfinding a proper combination of MFT and acoustic feature representations.The experiments in this paper intend to show that every possible effort should be taken to minimize the dispersion of disturbances.Although this holds true both for the within vector dimension and for the time(across vector)dimen-sion,this paper mainly focusses on the effects of within-vector smearing.In the rest of the paper,we will assume that the incoming speech is represented as a set of mel frequency log energy coefficents (MFLECs).To distinguish these input vectors from the feature vectors that result from pre-processing,i.e.those which are actu-ally used for recognition,we will call these melfilter bank outputs raw input features.When talking about feature values we mean the vector elements that result after pre-processing.The HMMs used during experimentation were based on four dif-ferent feature representations,i.e.:1.within-vector averaged mel-frequency log-energy coeffi-cients(WV A-MFLECs)2.mel-frequency cepstral coefficients(MFCCs)3.within vectorfiltered mel-frequency log-energy coeffi-cients(WVF-MFLECs)[8],and4.sub-band mel-frequency cepstral coefficients(SB-MFCCs)[9].Details about these feature representations will be given in sec-tion3.For the moment it suffices to note that thefirst two of these representations(WV A-MFLECs and MFCCs)are calcu-lated from the entire vector of raw input features.As a conse-quence,any distortion in the raw input features is dispersed over all feature values that are used during recognition.The last two representations(WVF-MFLECs and SB-MFCCs)are designed so that distortions which are present in part of the raw input fea-ture vector do not necessarily spread over the entire feature vector that results after pre-processing.In other words,given the type of distortion applied,these representations guarantee that part of the feature vector remains unaffected.The rest of this paper is organized as follows.First,in sections 2to5,we describe the experimental set-up that we used in more detail.In section6we compare the recognition performance for the four different types of features.We evaluated system perfor-mance with clean and disturbed data for each of the four acoustic representation techniques,with and without applying MFT in the form of acoustic backing-off.Finally,our conclusions are pre-sented in section7.2.SPEECH MATERIALThe speech material for our experiments was taken from the Dutch POLYPHONE corpus[10].Speech was recorded over the public switched telephone network in the Netherlands.Among other things,the speakers were asked to read several connected digit strings.The number of digits in each string varied between 3and16.For training we reserved a set of1997strings(16582 digits).Care was taken so as to balance the training material with respect to(1)an equal number of male and female speakers, (2)an equal number of speakers from each of the12provinces in the Netherlands and(3)an equal number of tokens per digit. For cross-validation during training(cf.[11])we used504digit string utterances(4300digits).All the models were evaluated with an independent set of1008test utterances(8300digits).The cross-validation test set and the independent test set were bal-anced with regards to the number of males and females,the cover-age of different regions in the country as well as to an equal num-ber of tokens per digit.None of the utterances used for training or testing had a high background noise level.3.ACOUSTIC FEATURESWe used four different types of acoustic features for our exper-iments:within-vector averaged mel-frequency log-energy co-efficients(WV A-MFLECs),mel-frequency cepstral coefficients (MFCCs),within-vectorfiltered mel-frequency log-energy coef-ficients(WVF-MFLECs)and sub-band mel-frequency cepstral coefficients(SB-MFCCs).In each case wefirst computed acoustic feature vectors consisting of16mel-frequency log-energy coefficients(MFLECs)using the following set-up.Speech signals were recorded from a primary rate ISDN telephone connection and stored in A-law format.Af-ter conversion to the linear domain,a25ms Hamming window shifted with10ms steps and a pre-emphasis factor of0.98were applied.Based on a Fast Fourier Transform,16filter band energy values were calculated,with thefilter bands triangularly shaped and uniformly distributed on a mel-frequency scale(covering0-2143.6mel;this corresponds to the linear range of0-4000Hz).In addition to the16MFLECs,we also computed the log-energy for each frame.These signal processing steps were performed using HTK2.1[12].For the WV A-MFLECs,we computed the average within-vector log-energy value for each frame.This within-vector average (WV A)was subtracted from each of the original16MFLEC val-ues yielding16WV A-MFLEC values.We subtracted the aver-age value(computed over the whole utterance)for all16WV A-MFLEC values as an implementation of a channel normalization (CN)technique.Finally,we computed the16corresponding time derivatives(delta-coefficients).Combining these with the16sta-tic WV A-MFLECs,log-energy and delta log-energy yielded34-dimensional feature vectors.In the case of MFCCs,(c1,...,c12)were computed from the raw MFLECs using the DCT.Cepstrum mean subtraction(CMS)was then applied to the twelve MFCCs as a CN technique.We used the off-line version of this CN technique,i.e.the cepstrum mean was computed using the whole utterance.Finally,we computed the time derivatives and added these to the12channel normal-ized MFCCs.Together with log-energy and delta log-energy we obtained26-dimensional acoustic feature vectors.SB-MFCCs were computed by computing(,...,)inde-pendently for thefirst8MFLEC values(covering0-1218Hz) and(,...,)for the second8MFLECs(covering1015-4000Hz).Next,we proceeded exactly as with the MFCCs,i.e. subtracting the mean computed over the whole utterance for CN and computing the deltas.Together with log-energy and delta log-energy we arrived in this manner at26-dimensional feature vectors.The WVF-MFLECs were computed by applying thefilterwithin each frame for coefficients2-15.Coefficients1and 16were just copied.After thisfilter and copy operation,the mean value computed over the whole utterance was subtracted as a form of CN.Next the deltas were computed.The static and delta WVF-MFLECs were combined together with log-energy and delta log-energy to arrive at34-dimensional feature vectors.4.DISTORTIONSIdeally,what we are striving tofind is an acoustic representation technique which is immune against broad band,non-stationary noise and not just band limited,stationary noise.However,we decided to start the investigation with a simplified problem in or-der to gain insight into the way the acoustic representations are affected by different kinds of noise.We added band limited,stationary noise to the speech signals at a level of5dBA,i.e.both the speech and noise energy levels were weighted according to the A-scale[13].The band limited noise signals were obtained byfiltering Gaussian white noise signals using afifth order ellipticalfilter.The cut-off frequencies of the band-passfilter were chosen such that approximately one quar-ter of the resulting raw input features would be contaminated by noise.Furthermore,the value of the high cut-off frequency en-sured that the noise distortions were limited to thefirst set of sub-bands in the case of the SB-MFCC feature representation.5.HIDDEN MARKOV MODELING The ten Dutch digit words were described with18context inde-pendent phone models.In addition we used three different mod-els for silence,background noises and out-of-vocabulary speech. For our most simple description,each phone unit was represented as a left-to-right hidden Markov model(HMM)consisting of three states,with the emission pdf of each state in the form of a single Gaussian pdf and only self-loops and transitions to the next state.For these models the total number of different states was63(54for the phones plus9for the noise models).We used HTK2.1for training and testing HMMs[12].We followed the cross-validation scheme described in[11]to determine the opti-mal number of Baum-Welch iterations.The more complex mod-els were obtained through subsequent mixture splitting.We split up to four times,resulting in different recognition systems with2, 4,8and16Gaussians per state(containing respectively126,252, 504and1008Gaussians in total).We used diagonal covariance matrices for all HMMs and each model set was trained only once, using undisturbed features.The recognition syntax used during cross-validation and testing was such that connected digit strings, varying in length from3to16digits,could be recognised.6.RESULTS AND DISCUSSIONIn order to determine a proper reference system for each feature representation,we computed the word error rate(WER)for the best HMMs according to the cross-validation development test set at1,2,4,8and16Gaussians per state.The WER was defined asclean dBA3.4(0.4)66.7(1.0) acoustic backing-offclean dBA3.2(0.4)73.8(1.0) acoustic backing-offclean dBA2.4(0.3)50.1(1.1) acoustic backing-offclean dBA3.3(0.4)49.6(1.1) acoustic backing-offis combined with a feature representation which only partially smears distortions:WER=for WVF-MFLECs and WER =for SB-MFCCs.This result shows that one can bene-fit most from an implementation of MFT when spectrally local distortions are kept local in the feature vector components of the representation used for modeling and recognition.Finally,it can be observed that the WER reduction in the case of WVF-MFLECs is,whereas in the case of SB-MFCCs it is.Thus,acoustic backing-off appears to be more effec-tive in the case of WVF-MFLECs.Most probably,thisfinding can be explained by the fact that the fraction of disturbed fea-ture components within each feature vector is smallest in the case the WVF-MFLECs.For WVF-MFLECs the number of disturbed static WVF-MFLECs is6,which is also the number of disturbed delta WVF-MFLECs.In addition,the log-energy and delta log-energy are disturbed.Thus,the distortions are present in14of the34feature vector components(corresponding to).For the SB-MFCCs,the distortions are present in thefirst6sub-band cepstral coefficients,the corresponding deltas,in log-energy and in delta log-energy.In that case,14of26feature vector com-ponents are affected by the distortion(corresponding to). This may prove to be an inherent advantage of WVF-MFLECs over SB-MFCCs.This hypothesis is presently under investiga-tion for large vocabulary continuous speech recognition.Of course,besides the relative amount of distorted feature vector components,the relative amount of information in the unaffected feature vector components must also be taken into consideration here.For instance,if it appears that the sub-band cepstra derived from the high frequency part of the MFLECs contain less infor-mation than the sub-band cepstra corresponding to the lower fre-quency half,the type of distortion applied in the experiments for this paper has biased the comparison.Nevertheless,it is tempting to speculate that the higher effectiveness of our implementation of MFT in the case of WVF-MFLECs compared to SB-MFCCs may be primarily attributed to the inherently smaller amount of smear-ing.Experiments are under way to investigate this issue further.7.CONCLUSIONSWe investigated the effectiveness of acoustic backing-off as an implementation of MFT for four different acoustic feature repre-sentations when the speech utterances were distorted by band lim-ited additive noise(SNR=5dBA).We used two representations that smear spectrally local distortions over all feature vector com-ponents and two representations that limit smearing to a sub-set of the feature vector components used for modeling and recogni-tion.For the two representations with full smearing we found that the effectiveness of acoustic backing-off as an implementation of MFT is limited.In both cases we found a WER at a level of. For the two representations that only partially smear spectrally lo-cal distortions over all feature vector components,we found that recognition robustness is already significantly improved by using a conventional local distance computation.For both methods a WER at a level of is found.Additionally,the WER is sub-stantially improved when acoustic backing-off is applied.In the case of WVF-MFLECs,acoustic backing-off is capable of reduc-ing the WER by to an absolute level of.We interpret our results as support in favour of the idea that limiting smearing of spectrally local distortions is a key factor in successful appli-cation of MFT.ACKNOWLEDGEMENTPart of this research was carried out within the framework of the Priority Programme Language and Speech Technology(TST). The TST-Programme is sponsored by NWO(Dutch Organisation for Scientific Research).8.REFERENCES1.M.Cooke,A.Morris&P.Green,‘Recognising occludedspeech’,in Proc.ESCA Workshop on the Auditory Basis of Speech Perception,Keele Univ.,UK,pp.297-300,1996. 2.R.Lippmann&B.Carlson,‘Using missing feature theory toactively select features for robust speech recognition with in-terruptions,filtering,and noise’,in Proc.Eurospeech-97,pp.37-40,1997.3. A.Morris,M.Cooke&P.Green,‘Some solutions to themissing feature problem in data classification,with applica-tions to noise robust ASR’,in Proc.ICASSP-98,pp.737-740,1998.4.J.de Veth,B.Cranen&L.Boves,‘Acoustic backing-off inthe local distance computation for robust automatic speech recognition’,in Proc.ICSLP-98,pp.1427-1430,1998.5.J.de Veth,B.Cranen&L.Boves,‘Acoustic backing-off asan implementation of missing feature theory’,submitted for publication.6.S.Dupont,H.Bourlard&C.Ris,‘Robust speech recogni-tion based on multi-stream features’,in Proc.ESCA-NATO Workshop on Robust Speech Recognition for Unknown Communication Channels,pp.95-98,1997.7.S.Tibrewala&H.Hermansky,‘Sub-band based recognitionof noisy speech’,in Proc.ICASSP-97,pp.1255-1258,1997.8. C.Nadeu,J.Hernando&M.Gorricho,‘On the decorrela-tion offilter-bank energies in speech recognition’,in Proc.Eurospeech-95,pp.1381-1384,1995.9.S.Okawa, E.Bocchieri&A.Potamianos,‘Multi-bandspeech recognition in noisy environments’,in Proc.ICASSP-98,pp.641-644,1998.10. E.A.den Os,T.I.Boogaart,L.Boves&E.Klabbers,‘TheDutch Polyphone corpus’,in Proc.Eurospeech-95,pp.825-828,1995.11.J.de Veth&L.Boves,‘Channel normalization techniquesfor automatic speech recognition over the telephone’,Speech Communication,vol.25,pp.149-164,1998.12.S.Young,J.Jansen,J.Odell,D.Ollason&P.Woodland,‘The HTK book(for HTK Version2.1)’,Cambridge Univer-sity,UK,1995.13.J.R.Hassall&K.Zaveri,‘Acoustic noise measurements’,Br¨u el&Kjær,Denmark,1979.。